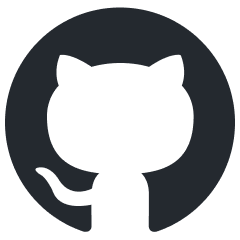
AI
The definitive, open-source Swift framework for interfacing with generative AI.
Stars: 106
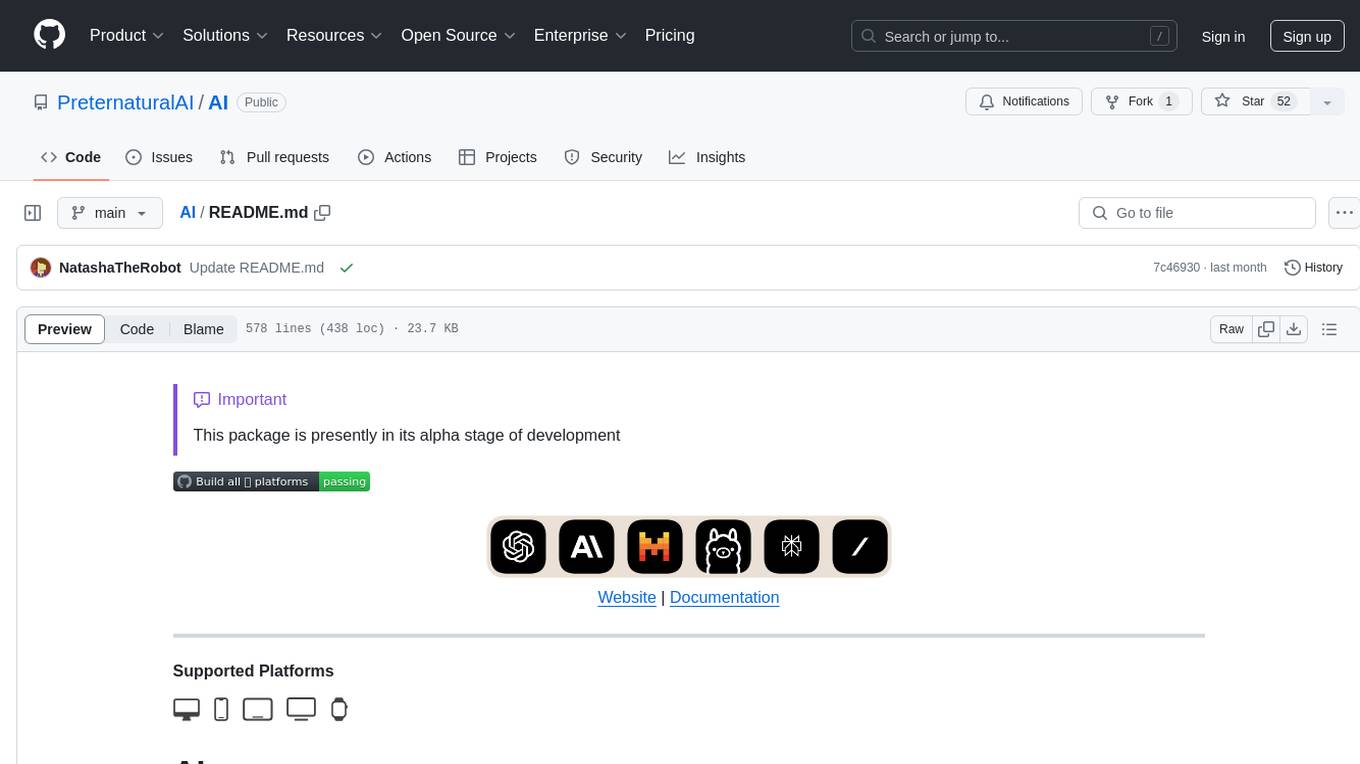
AI is an open-source Swift framework for interfacing with generative AI. It provides functionalities for text completions, image-to-text vision, function calling, DALLE-3 image generation, audio transcription and generation, and text embeddings. The framework supports multiple AI models from providers like OpenAI, Anthropic, Mistral, Groq, and ElevenLabs. Users can easily integrate AI capabilities into their Swift projects using AI framework.
README:
[!IMPORTANT] This package is presently in its alpha stage of development
The definitive, open-source Swift framework for interfacing with generative AI.
- Import the framework
- Initialize an AI Client
- LLM Clients Abstraction
- Supported Models
- Completions
- DALLE-3 Image Generation
- Audio
- Text Embeddings
Roadmap
Acknowledgements
License
- Open your Swift project in Xcode.
- Go to
File
->Add Package Dependency
. - In the search bar, enter this URL.
- Choose the version you'd like to install.
- Click
Add Package
.
+ import AI
Initialize an instance of an AI API provider of your choice. Here are some examples:
import AI
// OpenAI / GPT
import OpenAI
let client: OpenAI.Client = OpenAI.Client(apiKey: "YOUR_API_KEY")
// Anthropic / Claude
import Anthropic
let client: Anthropic.Client = Anthropic.Client(apiKey: "YOUR_API_KEY")
// Mistral
import Mistral
let client: Mistral.Client = Mistral.Client(apiKey: "YOUR_API_KEY")
// Groq
import Groq
let client: Groq.Client = Groq.Client(apiKey: "YOUR_API_KEY")
// ElevenLabs
import ElevenLabs
let client: ElevenLabs.Client = ElevenLabs.Client(apiKey: "YOUR_API_KEY")
You can now use client
as an interface to the supported providers.
If you need to abstract out the LLM Client (for example, if you want to allow your user to choose between clients), simply initialize an instance of LLMRequestHandling
with an LLM API provider of your choice. Here are some examples:
import AI
import OpenAI
import Anthropic
import Mistral
import Groq
// OpenAI / GPT
let client: any LLMRequestHandling = OpenAI.Client(apiKey: "YOUR_API_KEY")
// Anthropic / Claude
let client: any LLMRequestHandling = Anthropic.Client(apiKey: "YOUR_API_KEY")
// Mistral
let client: any LLMRequestHandling = Mistral.Client(apiKey: "YOUR_API_KEY")
// Groq
let client: any LLMRequestHandling = Groq.Client(apiKey: "YOUR_API_KEY")
You can now use client
as an interface to an LLM as provided by the underlying provider.
Each AI Client supports multiple models. For example:
// OpenAI GPT Models
let gpt_4o_Model: OpenAI.Model = .gpt_4o
let gpt_4_Model: OpenAI.Model = .gpt_4
let gpt_3_5_Model: OpenAI.Model = .gpt_3_5
let otherGPTModels: OpenAI.Model = .chat(.gpt_OTHER_MODEL_OPTIONS)
// Open AI Text Embedding Models
let smallTextEmbeddingsModel: OpenAI.Model = .embedding(.text_embedding_3_small)
let largeTextEmbeddingsModel: OpenAI.Model = .embedding(.text_embedding_3_large)
let adaTextEmbeddingsModel: OpenAI.Model = .embedding(.text_embedding_ada_002)
// Anthropic Models
let caludeHaikuModel: Anthropic.Model = .haiku
let claudeSonnetModel: Anthropic.Model = .sonnet
let claudeOpusModel: Anthropic.Model = .opus
// Mistral Models
let mistralTiny: Mistral.Model = .mistral_tiny
let mistralSmall: Mistral.Model = Mistral.Model.mistral_small
let mistralMedium: Mistral.Model = Mistral.Model.mistral_medium
// Groq Models
let gemma_7b: Groq.Model = .gemma_7b
let llama3_8b: Groq.Model = .llama3_8b
let llama3_70b: Groq.Model = .llama3_70b
let mixtral_8x7b: Groq.Model = .mixtral_8x7b
// ElevenLabs Models
let multilingualV2: ElevenLabs.Model = .MultilingualV2
let turboV2: ElevenLabs.Model = .TurboV2 // English
let multilingualV1: ElevenLabs.Model = .MultilingualV1
let englishV1: ElevenLabs.Model = .EnglishV1
Modern Large Language Models (LLMs) operate by receiving a series of inputs, often in the form of messages or prompts, and completing the inputs with the next probable output based on calculations performed by their complex neural network architectures that leverage the vast amounts of data on which it was trained.
You can use the LLMRequestHandling.complete(_:model:)
function to generate a chat completion for a specific model of your choice. For example:
import AI
import OpenAI
let client: any LLMRequestHandling = OpenAI.Client(apiKey: "YOUR_KEY")
// the system prompt is optional
let systemPrompt: PromptLiteral = "You are an extremely intelligent assistant."
let userPrompt: PromptLiteral = "What is the meaning of life?"
let messages: [AbstractLLM.ChatMessage] = [
.system(systemPrompt),
.user(userPrompt)
]
// Each of these is Optional
let parameters = AbstractLLM.ChatCompletionParameters(
// .max or maximum amount of tokens is default
tokenLimit: .fixed(200),
// controls the randomness of the result
temperatureOrTopP: .temperature(1.2),
// stop sequences that indicate to the model when to stop generating further text
stops: ["END OF CHAPTER"],
// check the function calling section below
functions: nil)
let model: OpenAI.Model = .gpt_4o
do {
let result: String = try await client.complete(
messages,
parameters: parameters,
model: model,
as: .string)
return result
} catch {
print(error)
}
Language models (LLMs) are rapidly evolving and expanding into multimodal capabilities. This shift signifies a major transformation in the field, as LLMs are no longer limited to understanding and generating text. With Vision, LLMs can take an image as an input, and provide information about the content of the image.
import AI
import OpenAI
let client: any LLMRequestHandling = OpenAI.Client(apiKey: "YOUR_KEY")
let systemPrompt: PromptLiteral = "You are a VisionExpertGPT. You will receive an image. Your job is to list all the items in the image and write a one-sentence poem about each item. Make sure your poems are creative, capturing the essence of each item in an evocative and imaginative way."
let userPrompt: PromptLiteral = "List the items in this image and write a short one-sentence poem about each item. Only reply with the items and poems. NOTHING MORE."
// Image or NSImage is supported
let imageLiteral = try PromptLiteral(image: imageInput)
let model = OpenAI.Model.gpt_4o
let messages: [AbstractLLM.ChatMessage] = [
.system(systemPrompt),
.user {
.concatenate(separator: nil) {
userPrompt
imageLiteral
}
}]
let result: String = try await client.complete(
messages,
model: model,
as: .string
)
return result
Adding function calling in your completion requests allows your app to receive a structured JSON response from an LLM, ensuring a consistent data format.
To demonstrate how powerful Function Calling can be, we will use the example of using a screenshot organizing app. The PhotoKit API already has a functionality to identify only photos that are screenshots. So just getting the user’s screenshots and putting them into an app is something that is simple enough to accomplish.
But now, with the power of LLMs, we can easily organize the screenshots by categories, provide a summary for each one, and add search functionality across all screenshots by having clear detailed text descriptions. In the future, we can add additional information, such as extracting any text or links included in the screenshot to make it easily actionable, and even extract specific elements from the screenshot.
To make a function call, we must first image an function in our app that would save the screenshot. What parameters does it need? These function parameters is what the LLM Function Calling tool will return for us so that we can call our function:
// Note that since LLMs are trained mainly on web APIs, we have to image web API function names for better results
func addScreenshotAnalysisToDB(
with title: String,
summary: String,
description: String,
category: String
) {
// this function does not exist in our app, but we pretend that it does for the purpose of using function calling to get a JSON response of the function parameters.
}
import OpenAI
import CorePersistence
let client = OpenAI.Client(apiKey: "YOUR_API_KEY")
let systemPrompt: PromptLiteral = """
You are an AI trained to analyze mobile screenshots and provide detailed information about them. Your task is to examine a given screenshot and generate the following details:
* Title: Create a concise title (3-5 words) that accurately represents the content of the screenshot.
* Summary: Write a brief, one-sentence summary providing an overview of what is depicted in the screenshot.
* Description: Compose a comprehensive description that elaborates on the contents of the screenshot. Include key details and keywords to facilitate easy searching.
* Category: Assign a single-word tag or category that best describes the screenshot. Examples include 'music', 'art', 'movie', 'fashion', etc. Avoid using 'app' as a category since all items are app-related.
Make sure your responses are clear, specific, and relevant to the content of the screenshot.
"""
let userPrompt: PromptLiteral = "Please analyze the attached screenshot and provide the following details: (1) a concise title (3-5 words) that describes the screenshot, (2) a brief one-sentence summary of the screenshot content, (3) a detailed description including key details and keywords for easy searching, and (4) a single-word category that best describes the screenshot (e.g., music, art, movie, fashion)."
let screenshotImageLiteral = try PromptLiteral(image: screenshot)
let messages: [AbstractLLM.ChatMessage] = [
.system(systemPrompt),
.user {
.concatenate(separator: nil) {
userPrompt
screenshotImageLiteral
}
}]
struct AddScreenshotFunctionParameters: Codable, Hashable, Sendable {
let title: String
let summary: String
let description: String
let category: String
}
do {
let screenshotFunctionParameterSchema: JSONSchema = try JSONSchema(
type: AddScreenshotFunctionParameters.self,
description: "Detailed information about a mobile screenshot for organizational purposes.",
propertyDescriptions: [
"title": "A concise title (3-5 words) that accurately represents the content of the screenshot.",
"summary": "A brief, one-sentence summary providing an overview of what is depicted in the screenshot.",
"description": "A comprehensive description that elaborates on the contents of the screenshot. Include key details and keywords to facilitate easy searching.",
"category": "A single-word tag or category that best describes the screenshot. Examples include: 'music', 'art', 'movie', 'fashion', etc. Avoid using 'app' as a category since all items are app-related."
],
required: true
)
let screenshotAnalysisProperties: [String : JSONSchema] = ["screenshot_analysis_parameters" : screenshotFunctionParameterSchema]
let addScreenshotAnalysisFunction = AbstractLLM.ChatFunctionDefinition(
name: "add_screenshot_analysis_to_db",
context: "Adds analysis of a mobile screenshot to the database",
parameters: JSONSchema(
type: .object,
description: "Screenshot Analysis",
properties: screenshotAnalysisProperties
)
)
let functionCall: AbstractLLM.ChatFunctionCall = try await client.complete(
messages,
functions: [addScreenshotAnalysisFunction],
as: .functionCall
)
struct ScreenshotAnalysisResult: Codable, Hashable, Sendable {
let screenshotAnalysisParameters: AddScreenshotFunctionParameters
}
let result = try functionCall.decode(ScreenshotAnalysisResult.self)
print(result.screenshotAnalysisParameters)
} catch {
print(error)
}
With OpenAI's DALLE-3, text-to-image generation is as easy as just providing a prompt. This gives us, as Apple Developers, the opportunity to include very personalized images for all kinds of use-cases instead of using any generic stock images.
For instance, consider we are building a personal journal app. With the DALLE-3 Image Generation API by OpenAI, we can generate a unique, beautiful image for each journal entry.
import AI
import OpenAI
let client: any LLMRequestHandling = OpenAI.Client(apiKey: "YOUR_KEY")
// user's journal entry for today.
// Note that the imagePrompt should be less than 4000 characters.
let imagePrompt = "Today was an unforgettable day in Japan, filled with awe and wonder at every turn. We began our journey in the bustling streets of Tokyo, where the neon lights and towering skyscrapers left us mesmerized. The serene beauty of the Meiji Shrine provided a stark contrast, offering a peaceful retreat amidst the city's chaos. We indulged in delicious sushi at a local restaurant, the flavors so fresh and vibrant. Later, we took a train to Kyoto, where the sight of the historic temples and the tranquil Arashiyama Bamboo Grove left us breathless. The day ended with a soothing dip in an onsen, the hot springs melting away all our fatigue. Japan's blend of modernity and tradition, coupled with its unparalleled hospitality, made this trip a truly magical experience."
let images = try await openAIClient.createImage(
prompt: imagePrompt,
// either standard or hd (costs more)
quality: OpenAI.Image.Quality.standard,
// 1024x1024, 1792x1024, or 1024x1792 supported
size: OpenAI.Image.Size.w1024h1024,
// either vivid or natural
style: OpenAI.Image.Style.vivid
if let imageURL = images.first?.url {
return URL(string: imageURL)
}
Adding audio generation and transcription to mobile apps is becoming increasingly important as users grow more comfortable speaking directly to apps for responses or having their audio input transcribed efficiently. Preternatural enables seamless integration with these cutting-edge, continually improving AI technologies.
Whisper, created and open-sourced by OpenAI, is an Automatic Speech Recognition (ASR) system trained on 680,000 hours of mostly English audio content collected from the web. This makes Whisper particularly impressive at transcribing audio with background noise and varying accents compared to its predecessors. Another notable feature is its ability to transcribe audio with correct sentence punctuation.
import OpenAI
let client = OpenAI.Client(apiKey: "YOUR_API_KEY")
// supported formats include flac, mp3, mp4, mpeg, mpga, m4a, ogg, wav, or webm
let audioFile = URL(string: "YOUR_AUDIO_FILE_URL_PATH")
// Optional - great for including correct spelling of audio-specific keywords
// For example, here we provide the correct spelling for company-spefic words in an earnings call
let prompt = "ZyntriQix, Digique Plus, CynapseFive, VortiQore V8, EchoNix Array, OrbitalLink Seven, DigiFractal Matrix, PULSE, RAPT, B.R.I.C.K., Q.U.A.R.T.Z., F.L.I.N.T."
// Optional - Supplying the input language in ISO-639-1 format will improve accuracy and latency.
// While Whisper supports 98 languages, note that languages other than English have a high error rate, so test thoroughly
let language: LargeLanguageModels.ISO639LanguageCode = .en
// The sampling temperature, between 0 and 1.
// Higher values like 0.8 will make the output more random, while lower values like 0.2 will make it more focused and deterministic.
// If set to 0, the model will use log probability to automatically increase the temperature until certain thresholds are hit.
let temperature = 0
// Optional - Setting Timestamp Granularities provides the time stamps for roughly every sentence in the transcription.
// note that the timestampGranularties is an array of granularities, so you can inlcude both .segment and .word granularities, or simple one of them
let timestampGranularities: [OpenAI.AudioTranscription.TimestampGranularity] = [.segment, .word]
do {
let transcription = try await openAIClient.createTranscription(
audioFile: audioFile,
prompt: prompt,
language: language,
temperature: temperature,
timestampGranularities: timestampGranularities
)
let fullTranscription = transcription.text
let segements = transcription.segments
let words = transcription.words
} catch {
print(error)
}
Preternatural offers a simple Text-to-Speech (TTS) integration with OpenAI:
import OpenAI
let client = OpenAI.Client(apiKey: "YOUR_API_KEY")
// OpenAI offers two Text-to-Speech (TTS) Models at this time.
// The tts-1 is the latest text to speech model, optimized for speed and is ideal to use for real-time text to speech use cases.
let tts_1: OpenAI.Model.Speech = .tts_1
// The tts-1-hd is the latest text to speech model, optimized for quality.
let tts_1_hd: OpenAI.Model.Speech = .tts_1_hd
// text for audio generation
let textInput = "In a quiet, unassuming village nestled deep in a lush, verdant valley, young Elara leads a simple life, dreaming of adventure beyond the horizon. Her village is filled with ancient folklore and tales of mystical relics, but none capture her imagination like the legend of the Enchanted Amulet—a powerful artifact said to grant its bearer the ability to control time."
// OpenAI currently offers 6 voice options
// Listen to voice samples are on their website: https://platform.openai.com/docs/guides/text-to-speech
let alloy: OpenAI.Speech.Voice = .alloy
let echo: OpenAI.Speech.Voice = .echo
let fable: OpenAI.Speech.Voice = .fable
let onyx: OpenAI.Speech.Voice = .onyx
let nova: OpenAI.Speech.Voice = .nova
let shimmer: OpenAI.Speech.Voice = .shimmer
// The OpenAI API offers the ability to adjust the speed of the audio.
// Speed between 0.25 and 4.0 could be selected, with 1.0 as the default.
let speed = 1.0
let speech: OpenAI.Speech = try await openAIClient.createSpeech(
model: tts_1,
text: textInput,
voice: alloy,
speed: speed)
let audioData = speech.data
ElevenLabs is a voice AI research & deployment company providing the ability to generate speech in hundreds of new and existing voices in 29 languages. They also allow voice cloning - provide only 1 minute of audio and you could generate a new voice!
import ElevenLabs
let client = ElevenLabs.Client(apiKey: "YOUR_API_KEY")
// ElevenLabs offers Multilingual and English-specific models
// More details on their website here: https://elevenlabs.io/docs/speech-synthesis/models
let model: ElevenLabs.Model = .MultilingualV2
// Select the voice you would like for the audio on the ElevenLabs website
// Note that you first have to add voices from the Voice Lab, then check your Voices for the ID
let voiceID = "4v7HtLWqY9rpQ7Cg2GT4"
let textInput = "In a quiet, unassuming village nestled deep in a lush, verdant valley, young Elara leads a simple life, dreaming of adventure beyond the horizon. Her village is filled with ancient folklore and tales of mystical relics, but none capture her imagination like the legend of the Enchanted Amulet—a powerful artifact said to grant its bearer the ability to control time."
// Optional - if you set any or all settings to nil, default values will be used
let voiceSettings: ElevenLabs.VoiceSettings = .init(
// Increasing stability will make the voice more consistent between re-generations, but it can also make it sounds a bit monotone. On longer text fragments it is recommended to lower this value.
// this is a double between 0 (more variable) and 1 (more stable)
stability: 0.5,
// Increasing the Similarity Boost setting enhances the overall voice clarity and targets speaker similarity.
// this is a double between 0 (Low) and 1 (High)
similarityBoost: 0.75,
// High values are recommended if the style of the speech should be exaggerated compared to the selected voice. Higher values can lead to more instability in the generated speech. Setting this to 0 will greatly increase generation speed and is the default setting.
// this is a double between 0 (Low) and 1 (High)
styleExaggeration: 0.0,
// Boost the similarity of the synthesized speech and the voice at the cost of some generation speed.
speakerBoost: true)
do {
let speech = try await client.speech(
for: textInput,
voiceID: voiceID,
voiceSettings: voiceSettings,
model: model
)
return speech
} catch {
print(error)
}
Text embedding models are translators for machines. They convert text, such as sentences or paragraphs, into sets of numbers, which the machine can easily use in complex calculations. The biggest use-case for Text Embeddings is improving Search in your application.
Just simply provide any text and the model will return an embedding (an array of doubles) of that text back.
import AI
import OpenAI
let client: any LLMRequestHandling = OpenAI.Client(apiKey: "YOUR_KEY")
// supported models (Only OpenAI Embeddings Models are supported)
let smallTextEmbeddingsModel: OpenAI.Model = .embedding(.text_embedding_3_small)
let largeTextEmbeddingsModel: OpenAI.Model = .embedding(.text_embedding_3_large)
let adaTextEmbeddingsModel: OpenAI.Model = .embedding(.text_embedding_ada_002)
let textInput = "Hello, Text Embeddings!"
let textEmbeddingsModel: OpenAI.Model = .embedding(.text_embedding_3_small)
let embeddings = try await LLMManager.client.textEmbeddings(
for: [textInput],
model: textEmbeddingsModel)
return embeddings.data.first?.embedding.description
- [x] OpenAI
- [x] Anthropic
- [x] Mistral
- [x] Ollama
- [ ] Perplexity
- [ ] Groq
This package is licensed under the MIT License.
For Tasks:
Click tags to check more tools for each tasksFor Jobs:
Alternative AI tools for AI
Similar Open Source Tools
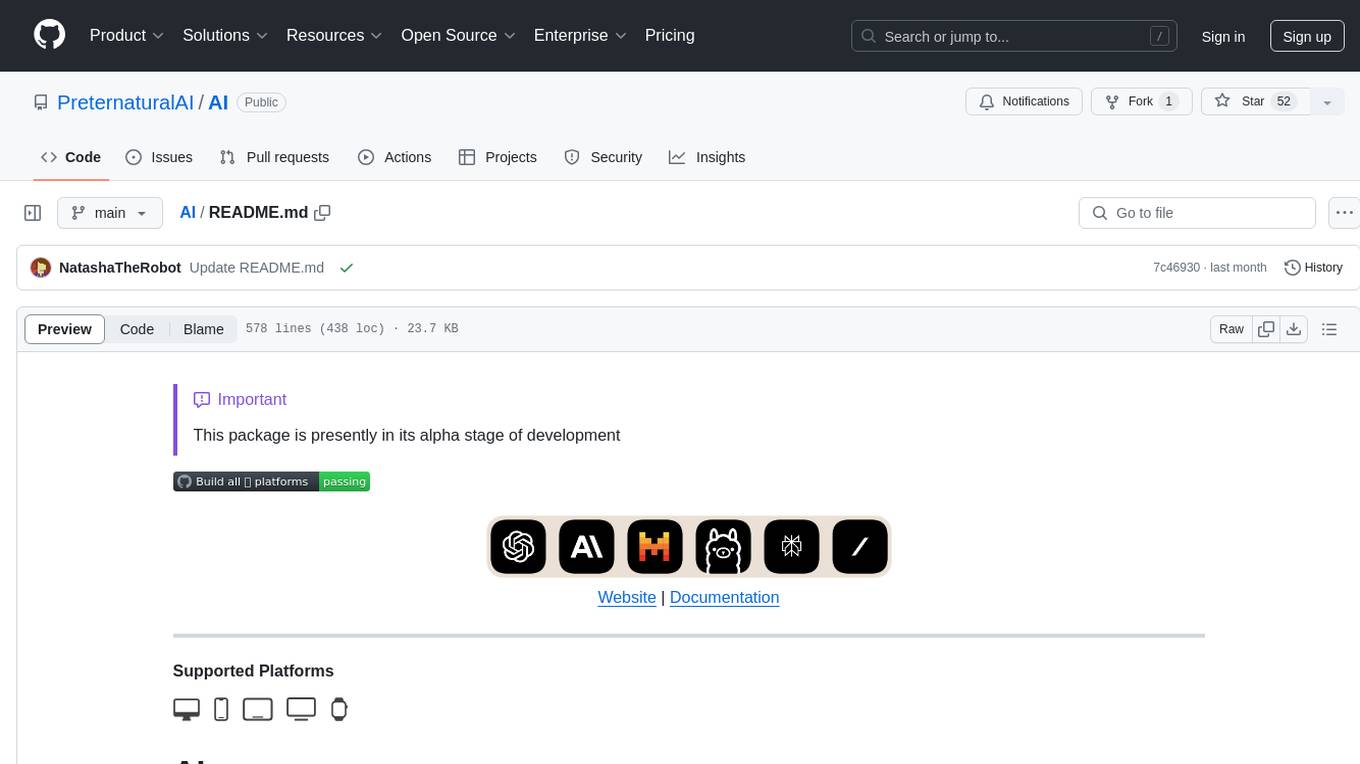
AI
AI is an open-source Swift framework for interfacing with generative AI. It provides functionalities for text completions, image-to-text vision, function calling, DALLE-3 image generation, audio transcription and generation, and text embeddings. The framework supports multiple AI models from providers like OpenAI, Anthropic, Mistral, Groq, and ElevenLabs. Users can easily integrate AI capabilities into their Swift projects using AI framework.
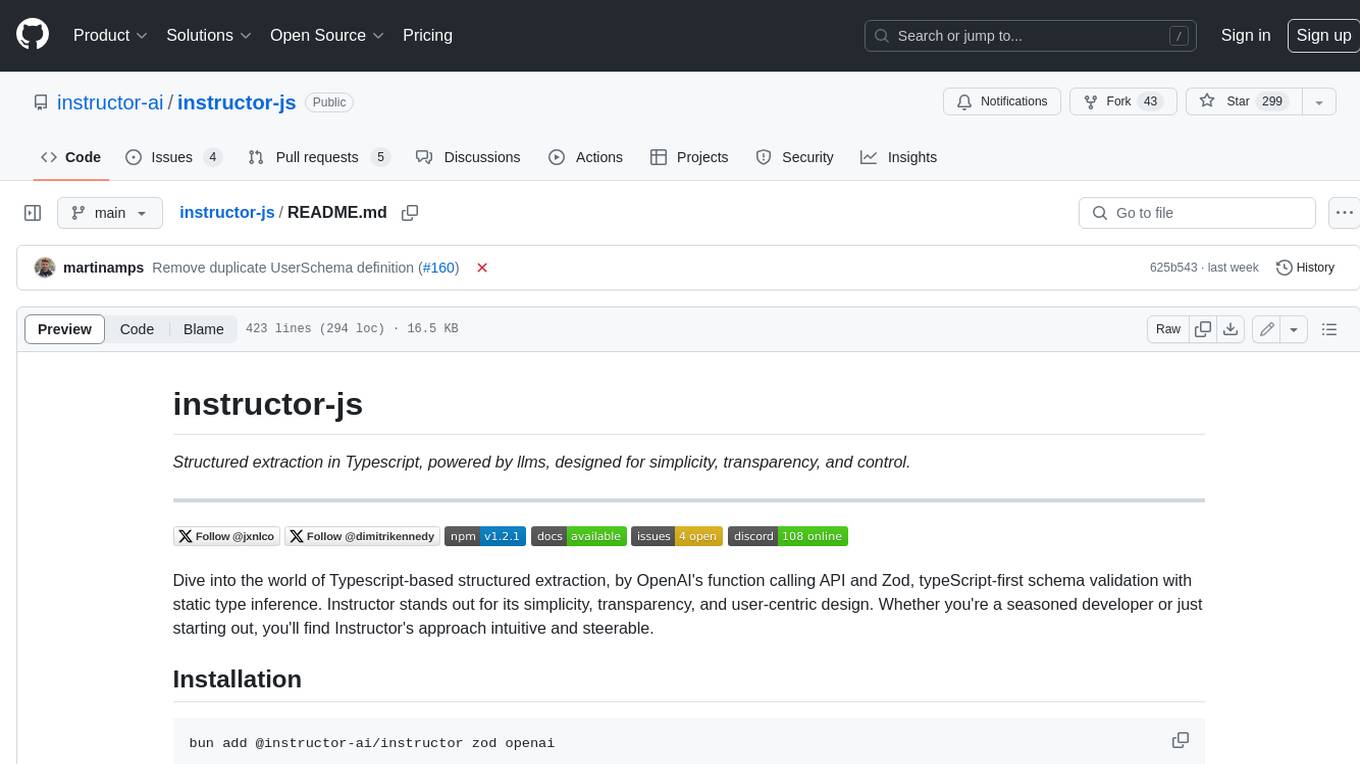
instructor-js
Instructor is a Typescript library for structured extraction in Typescript, powered by llms, designed for simplicity, transparency, and control. It stands out for its simplicity, transparency, and user-centric design. Whether you're a seasoned developer or just starting out, you'll find Instructor's approach intuitive and steerable.
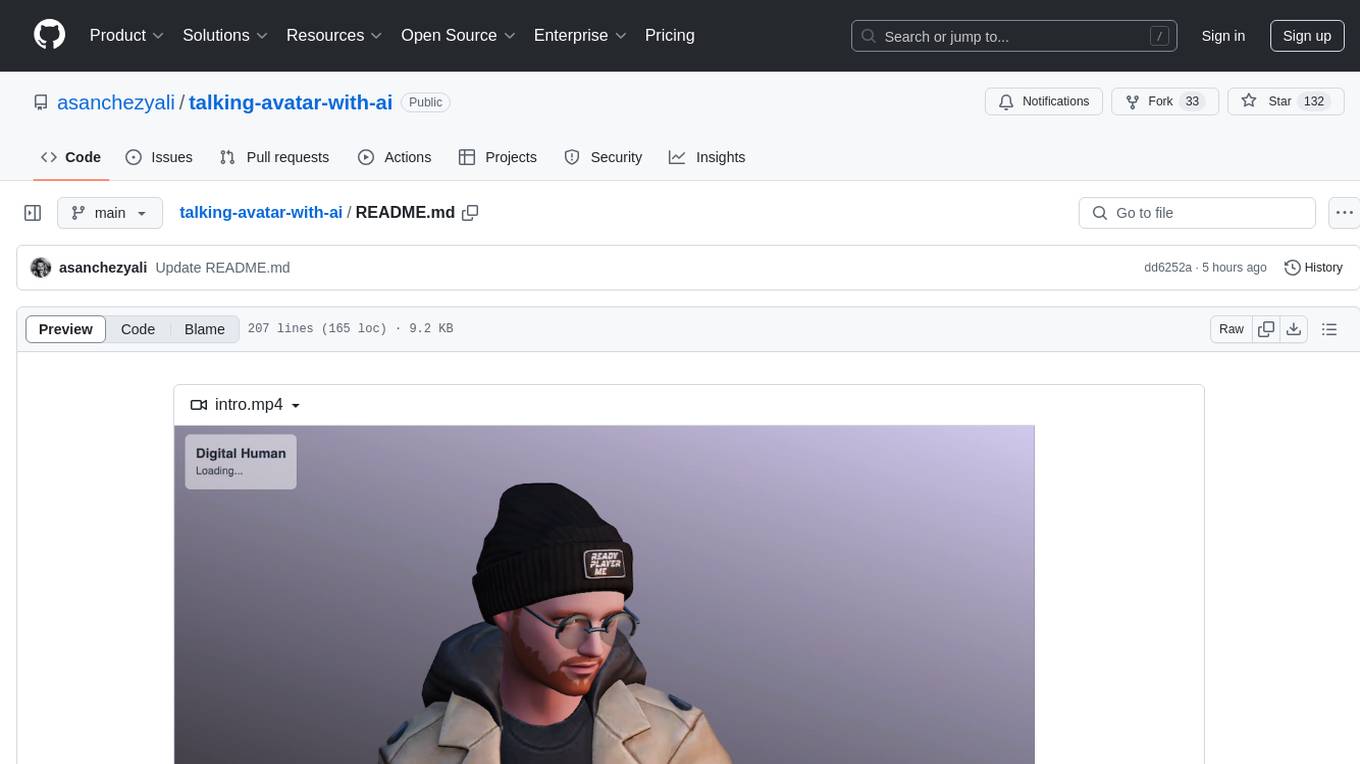
talking-avatar-with-ai
The 'talking-avatar-with-ai' project is a digital human system that utilizes OpenAI's GPT-3 for generating responses, Whisper for audio transcription, Eleven Labs for voice generation, and Rhubarb Lip Sync for lip synchronization. The system allows users to interact with a digital avatar that responds with text, facial expressions, and animations, creating a realistic conversational experience. The project includes setup for environment variables, chat prompt templates, chat model configuration, and structured output parsing to enhance the interaction with the digital human.
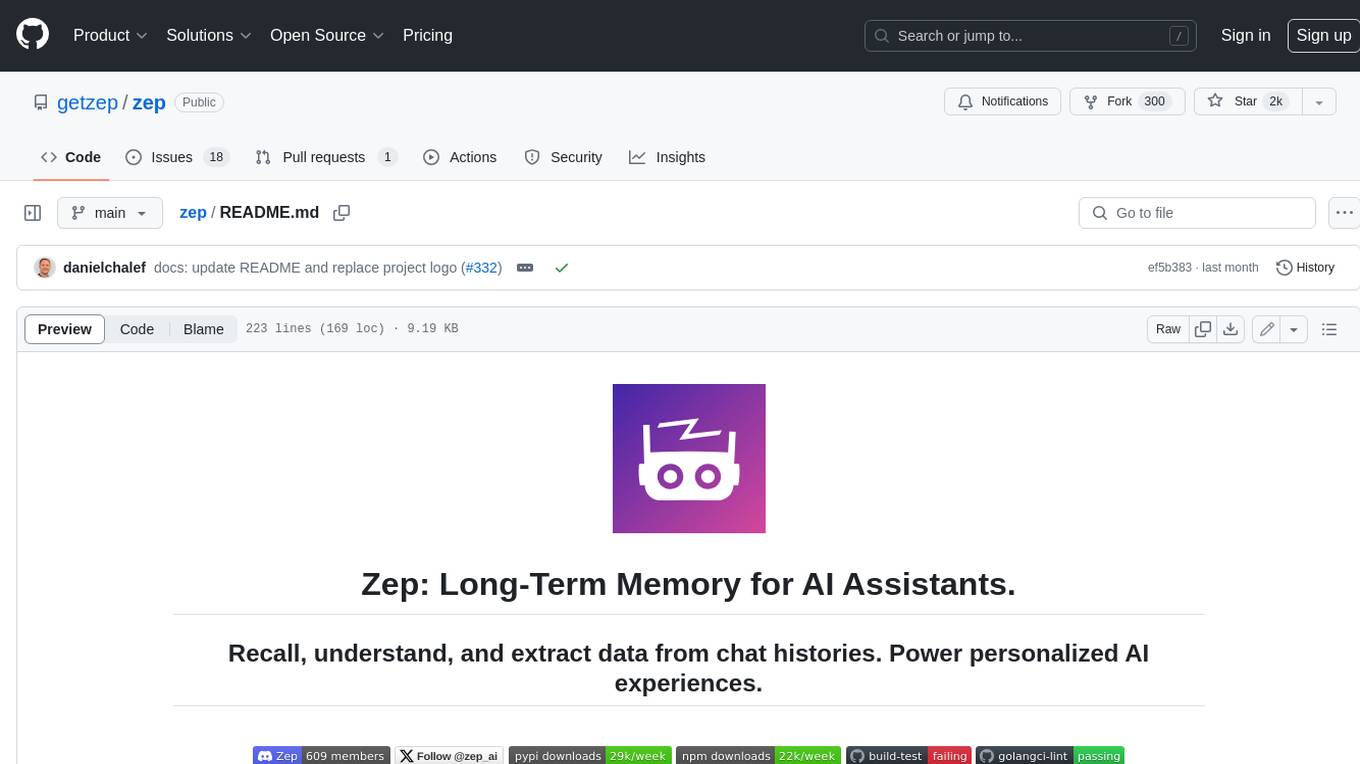
zep
Zep is a long-term memory service for AI Assistant apps. With Zep, you can provide AI assistants with the ability to recall past conversations, no matter how distant, while also reducing hallucinations, latency, and cost. Zep persists and recalls chat histories, and automatically generates summaries and other artifacts from these chat histories. It also embeds messages and summaries, enabling you to search Zep for relevant context from past conversations. Zep does all of this asyncronously, ensuring these operations don't impact your user's chat experience. Data is persisted to database, allowing you to scale out when growth demands. Zep also provides a simple, easy to use abstraction for document vector search called Document Collections. This is designed to complement Zep's core memory features, but is not designed to be a general purpose vector database. Zep allows you to be more intentional about constructing your prompt: 1. automatically adding a few recent messages, with the number customized for your app; 2. a summary of recent conversations prior to the messages above; 3. and/or contextually relevant summaries or messages surfaced from the entire chat session. 4. and/or relevant Business data from Zep Document Collections.
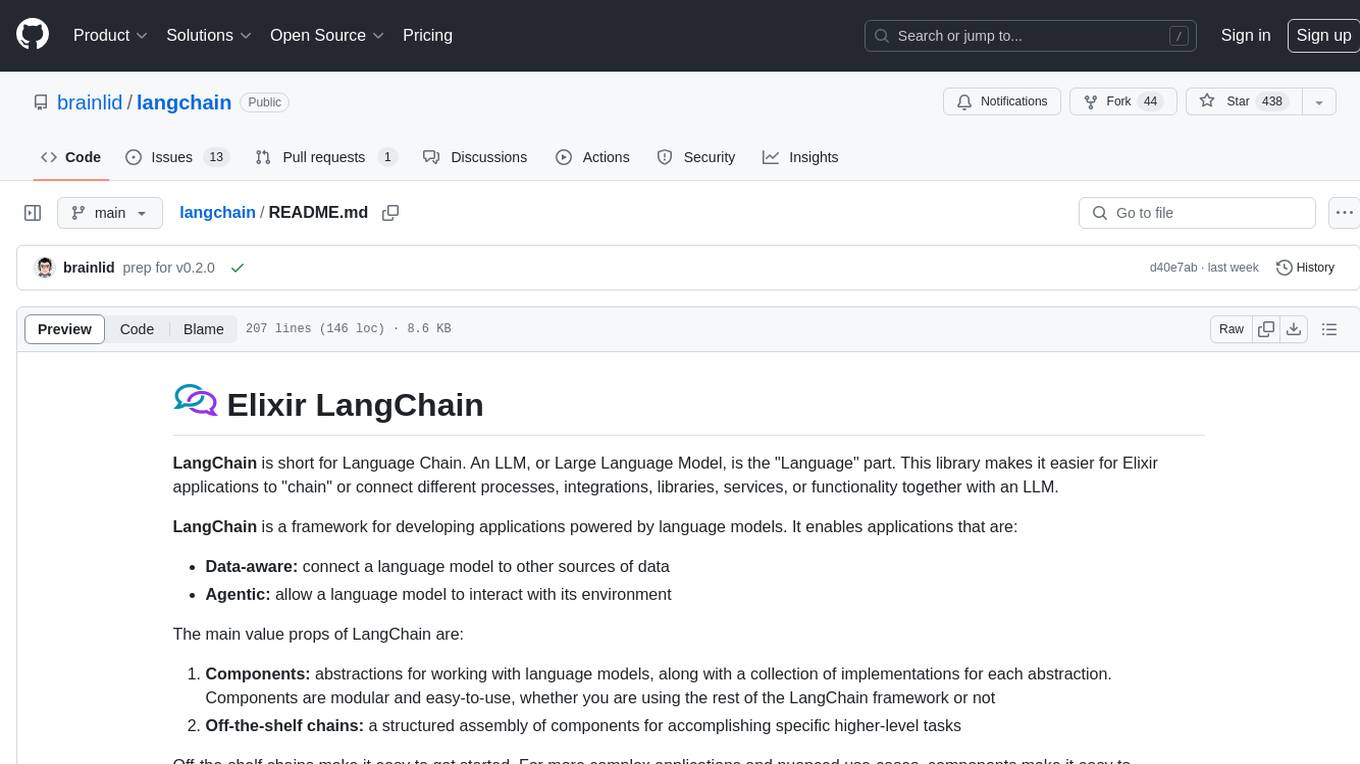
langchain
LangChain is a framework for developing Elixir applications powered by language models. It enables applications to connect language models to other data sources and interact with the environment. The library provides components for working with language models and off-the-shelf chains for specific tasks. It aims to assist in building applications that combine large language models with other sources of computation or knowledge. LangChain is written in Elixir and is not aimed for parity with the JavaScript and Python versions due to differences in programming paradigms and design choices. The library is designed to make it easy to integrate language models into applications and expose features, data, and functionality to the models.
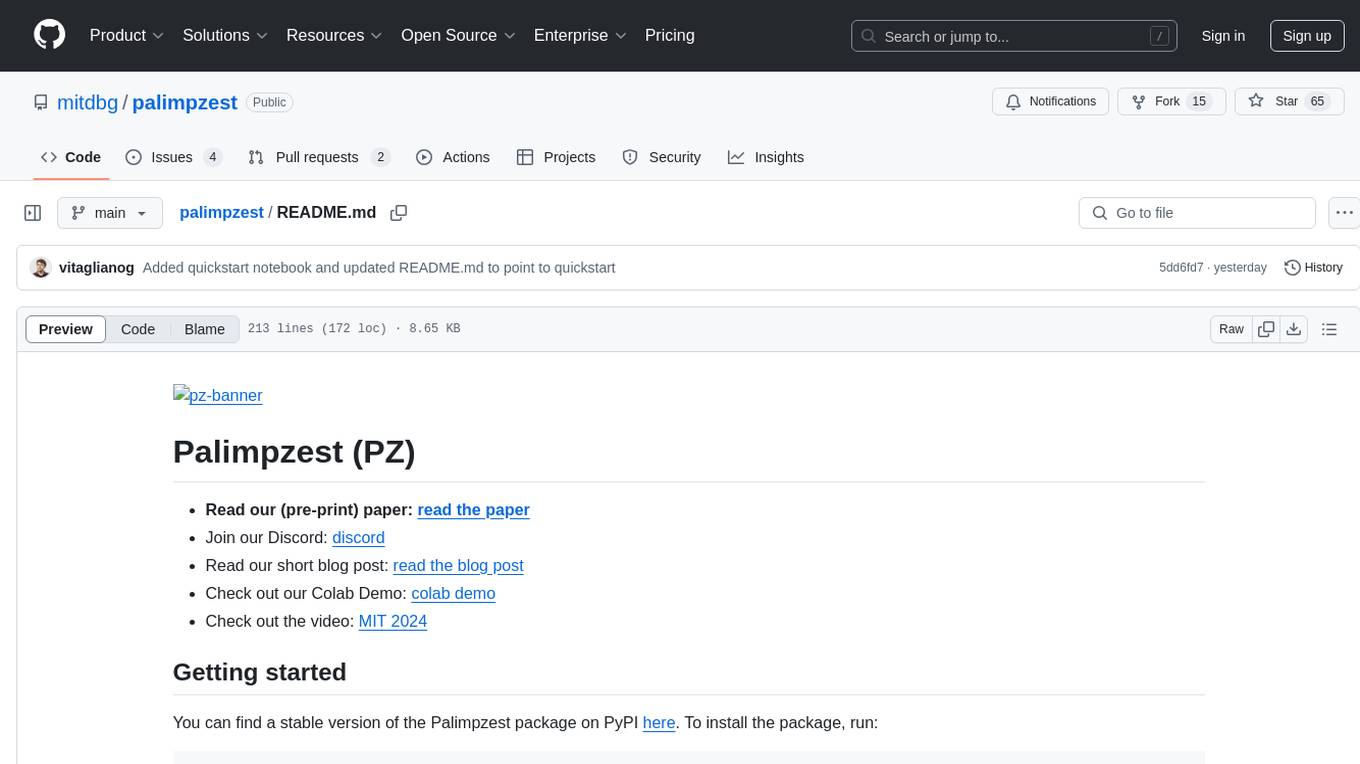
palimpzest
Palimpzest (PZ) is a tool for managing and optimizing workloads, particularly for data processing tasks. It provides a CLI tool and Python demos for users to register datasets, run workloads, and access results. Users can easily initialize their system, register datasets, and manage configurations using the CLI commands provided. Palimpzest also supports caching intermediate results and configuring for parallel execution with remote services like OpenAI and together.ai. The tool aims to streamline the workflow of working with datasets and optimizing performance for data extraction tasks.
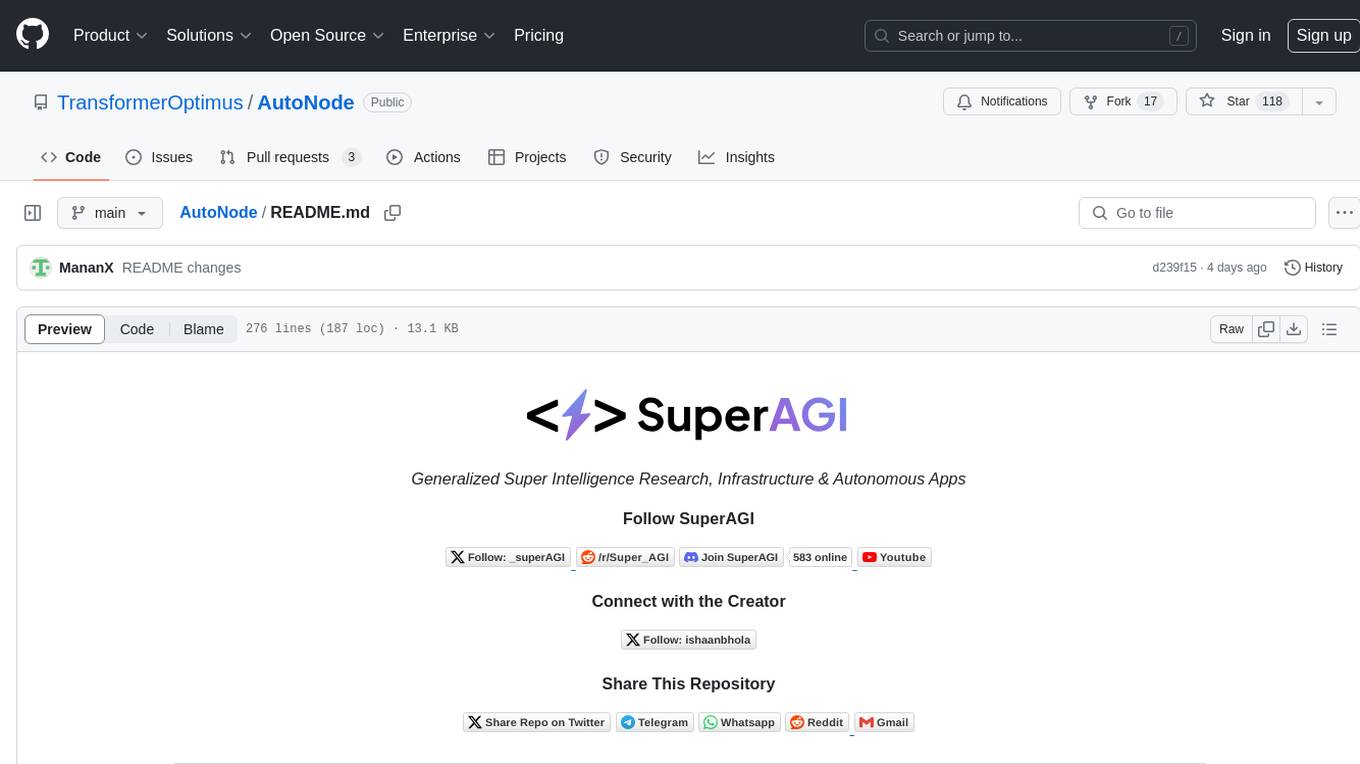
AutoNode
AutoNode is a self-operating computer system designed to automate web interactions and data extraction processes. It leverages advanced technologies like OCR (Optical Character Recognition), YOLO (You Only Look Once) models for object detection, and a custom site-graph to navigate and interact with web pages programmatically. Users can define objectives, create site-graphs, and utilize AutoNode via API to automate tasks on websites. The tool also supports training custom YOLO models for object detection and OCR for text recognition on web pages. AutoNode can be used for tasks such as extracting product details, automating web interactions, and more.
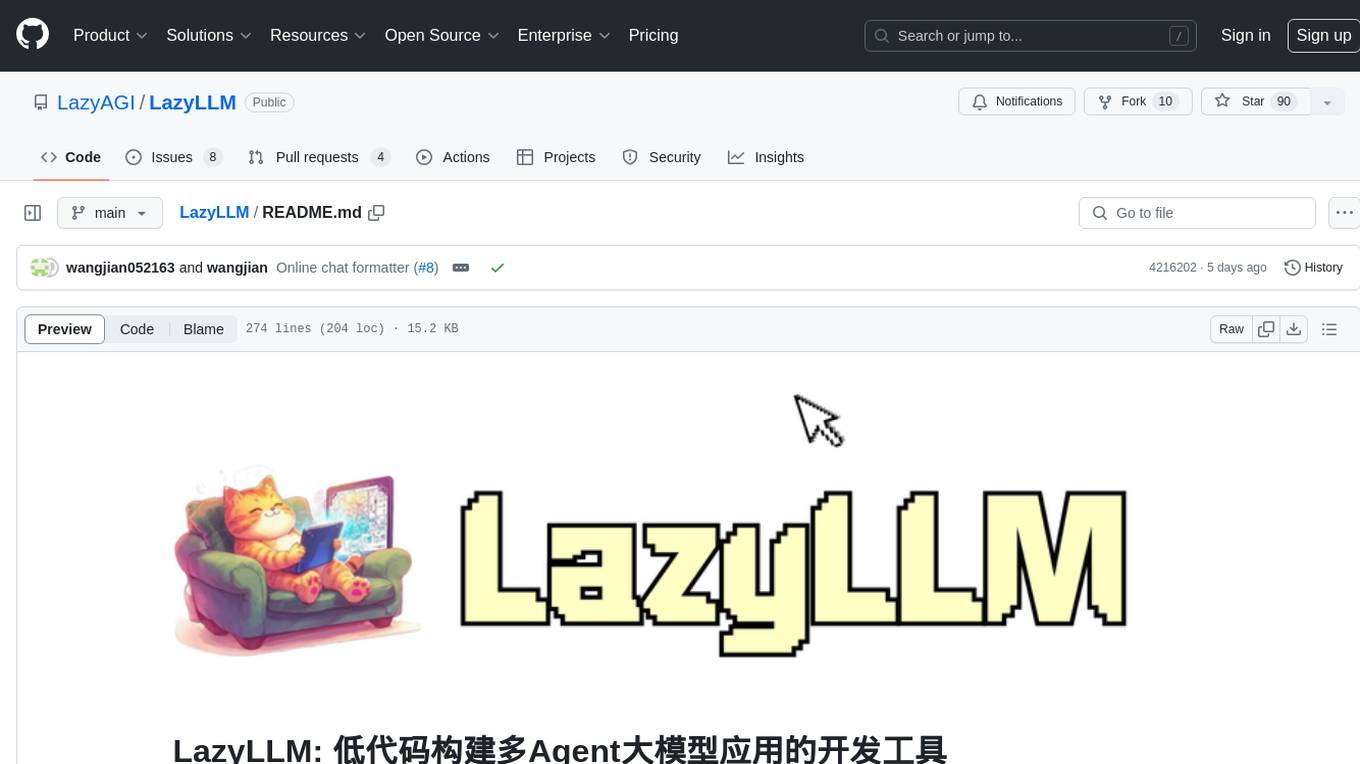
LazyLLM
LazyLLM is a low-code development tool for building complex AI applications with multiple agents. It assists developers in building AI applications at a low cost and continuously optimizing their performance. The tool provides a convenient workflow for application development and offers standard processes and tools for various stages of application development. Users can quickly prototype applications with LazyLLM, analyze bad cases with scenario task data, and iteratively optimize key components to enhance the overall application performance. LazyLLM aims to simplify the AI application development process and provide flexibility for both beginners and experts to create high-quality applications.
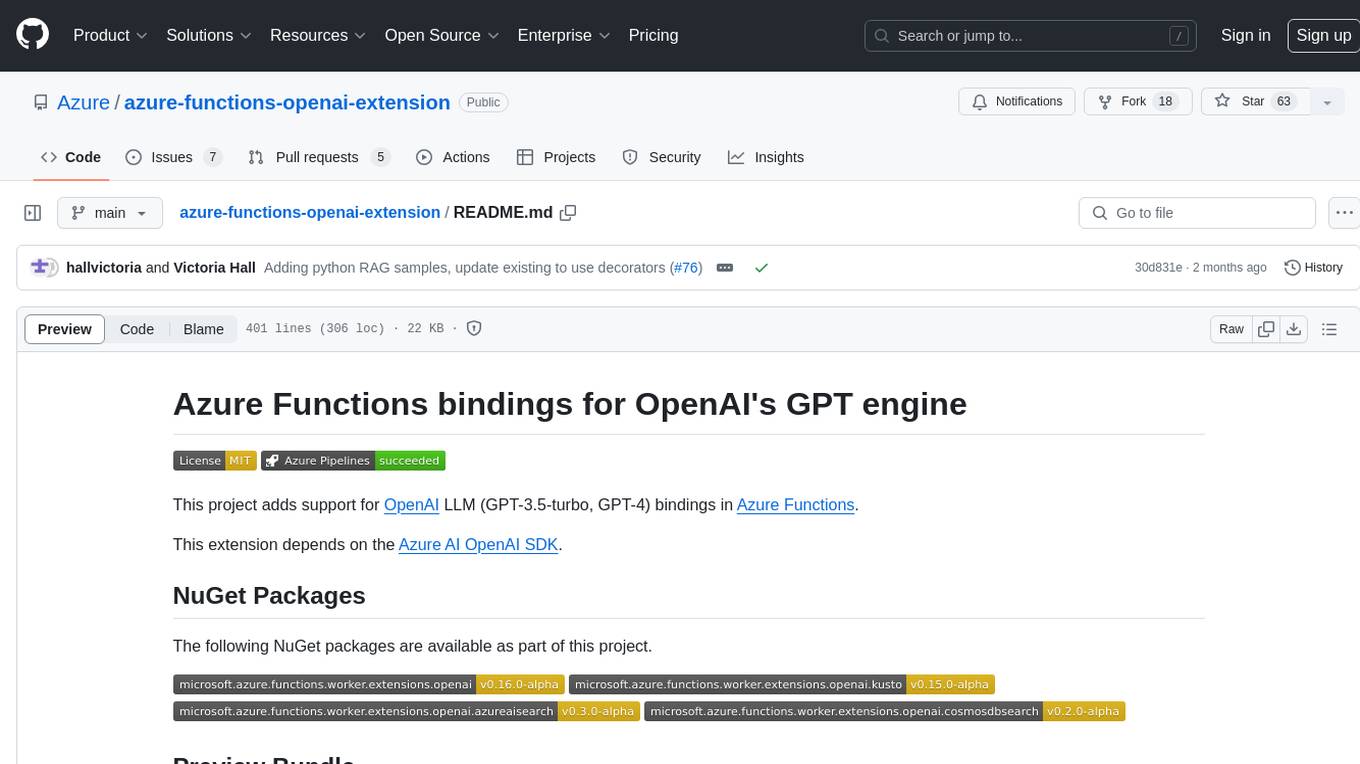
azure-functions-openai-extension
Azure Functions OpenAI Extension is a project that adds support for OpenAI LLM (GPT-3.5-turbo, GPT-4) bindings in Azure Functions. It provides NuGet packages for various functionalities like text completions, chat completions, assistants, embeddings generators, and semantic search. The project requires .NET 6 SDK or greater, Azure Functions Core Tools v4.x, and specific settings in Azure Function or local settings for development. It offers features like text completions, chat completion, assistants with custom skills, embeddings generators for text relatedness, and semantic search using vector databases. The project also includes examples in C# and Python for different functionalities.
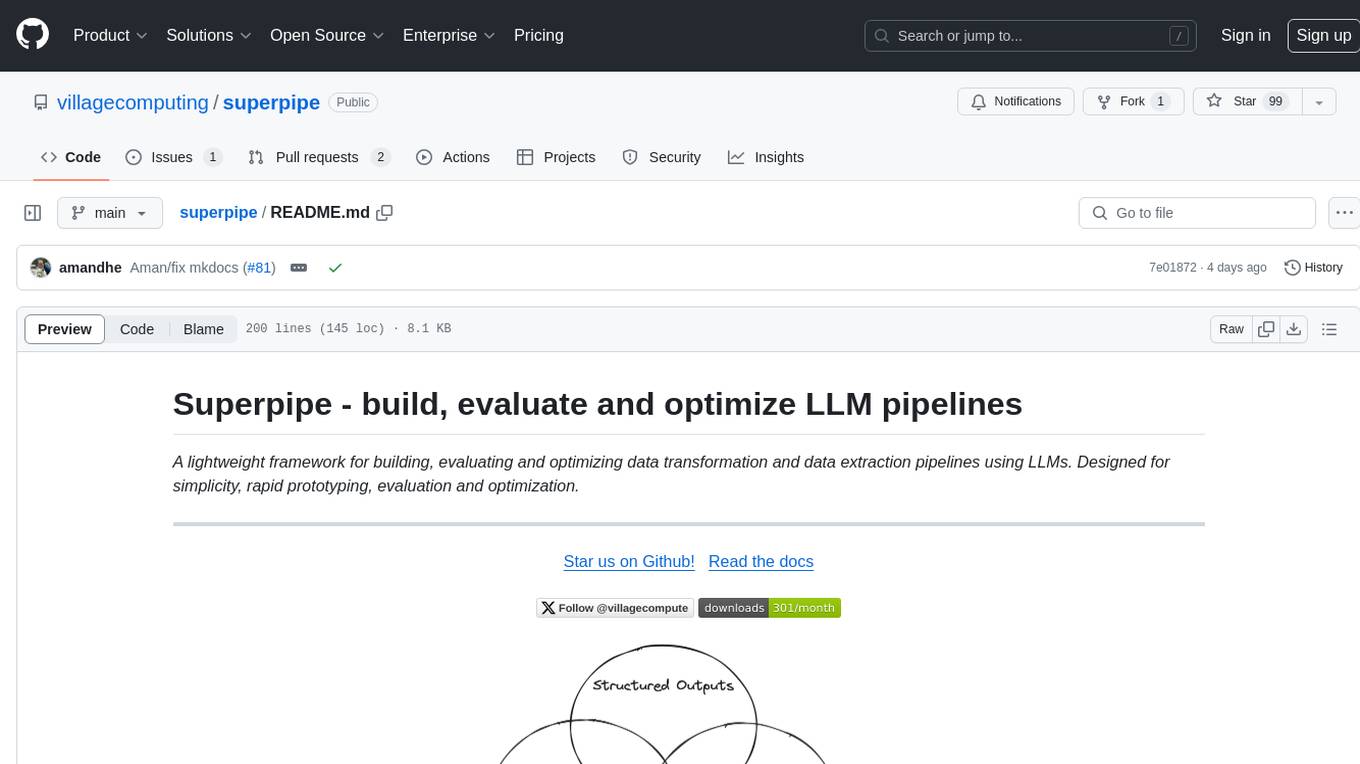
superpipe
Superpipe is a lightweight framework designed for building, evaluating, and optimizing data transformation and data extraction pipelines using LLMs. It allows users to easily combine their favorite LLM libraries with Superpipe's building blocks to create pipelines tailored to their unique data and use cases. The tool facilitates rapid prototyping, evaluation, and optimization of end-to-end pipelines for tasks such as classification and evaluation of job departments based on work history. Superpipe also provides functionalities for evaluating pipeline performance, optimizing parameters for cost, accuracy, and speed, and conducting grid searches to experiment with different models and prompts.
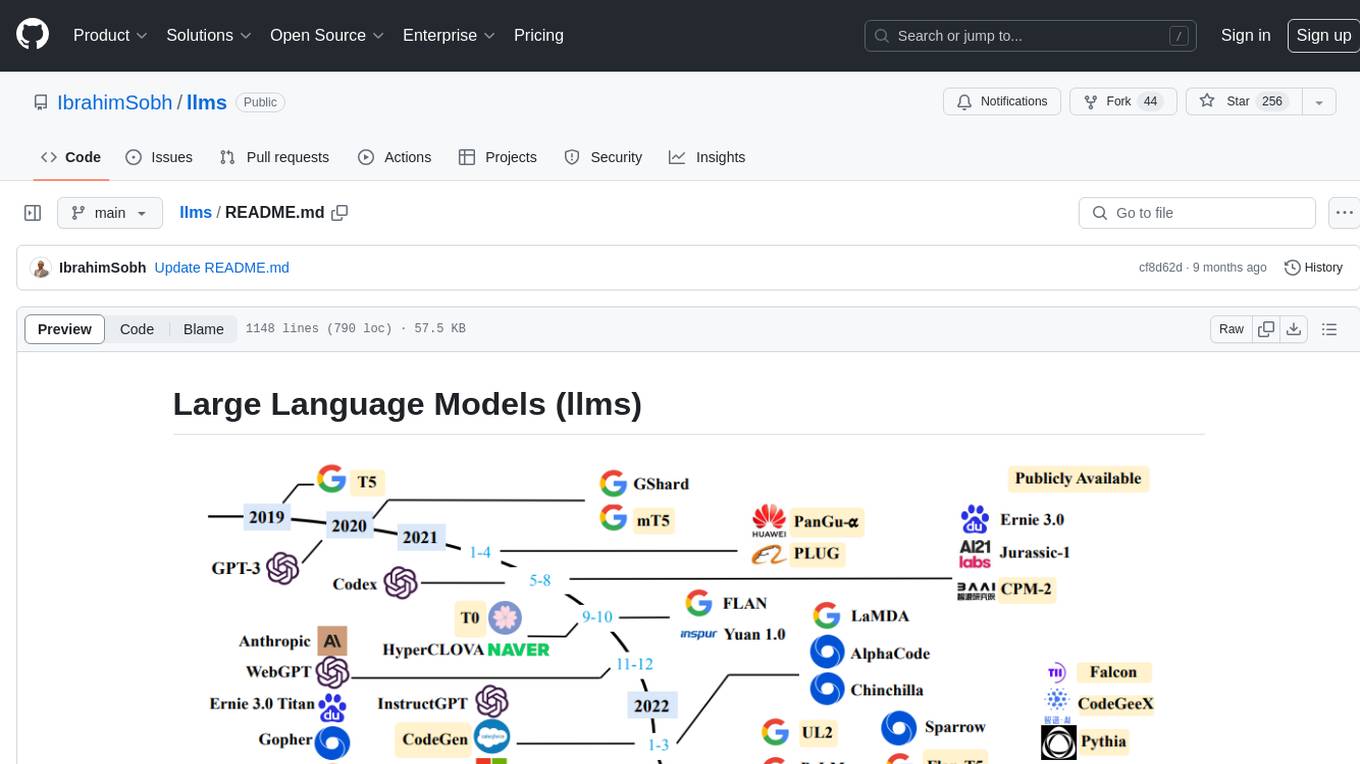
llms
The 'llms' repository is a comprehensive guide on Large Language Models (LLMs), covering topics such as language modeling, applications of LLMs, statistical language modeling, neural language models, conditional language models, evaluation methods, transformer-based language models, practical LLMs like GPT and BERT, prompt engineering, fine-tuning LLMs, retrieval augmented generation, AI agents, and LLMs for computer vision. The repository provides detailed explanations, examples, and tools for working with LLMs.
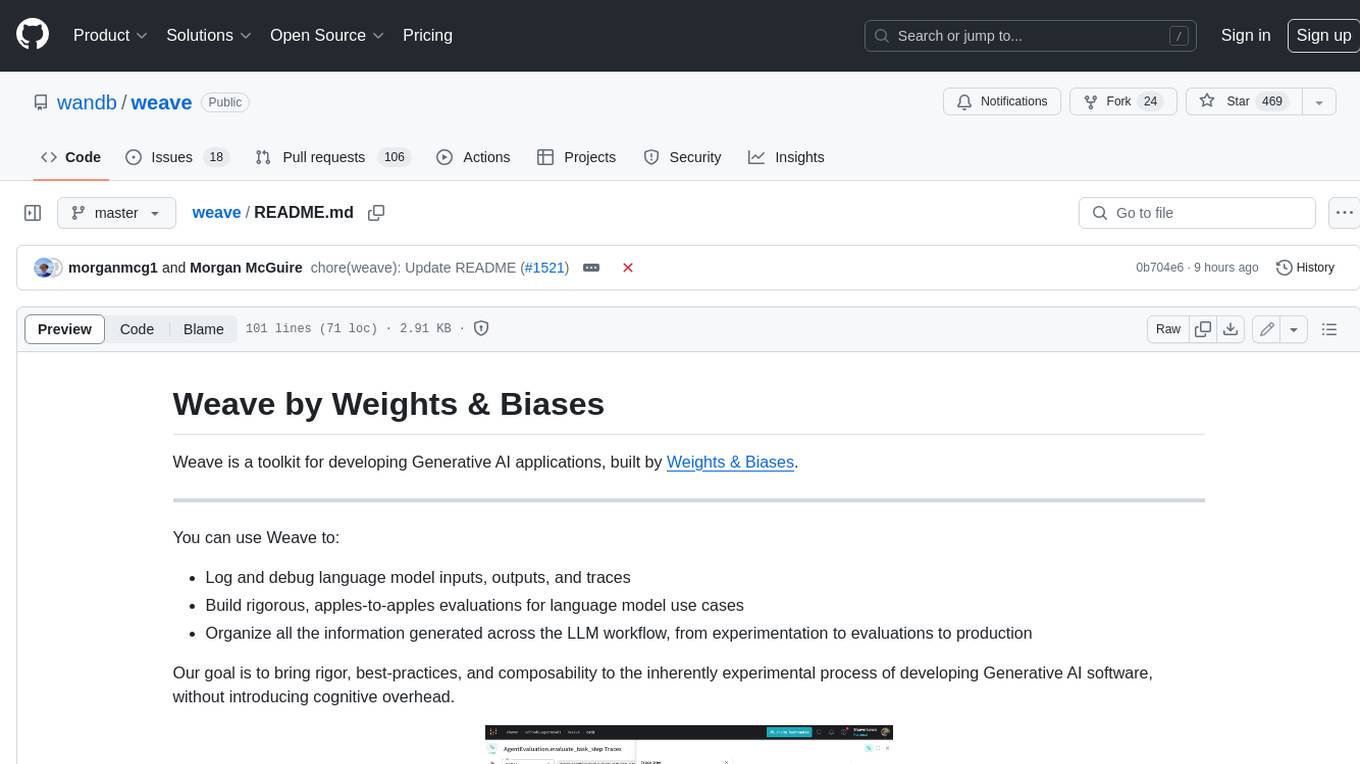
weave
Weave is a toolkit for developing Generative AI applications, built by Weights & Biases. With Weave, you can log and debug language model inputs, outputs, and traces; build rigorous, apples-to-apples evaluations for language model use cases; and organize all the information generated across the LLM workflow, from experimentation to evaluations to production. Weave aims to bring rigor, best-practices, and composability to the inherently experimental process of developing Generative AI software, without introducing cognitive overhead.
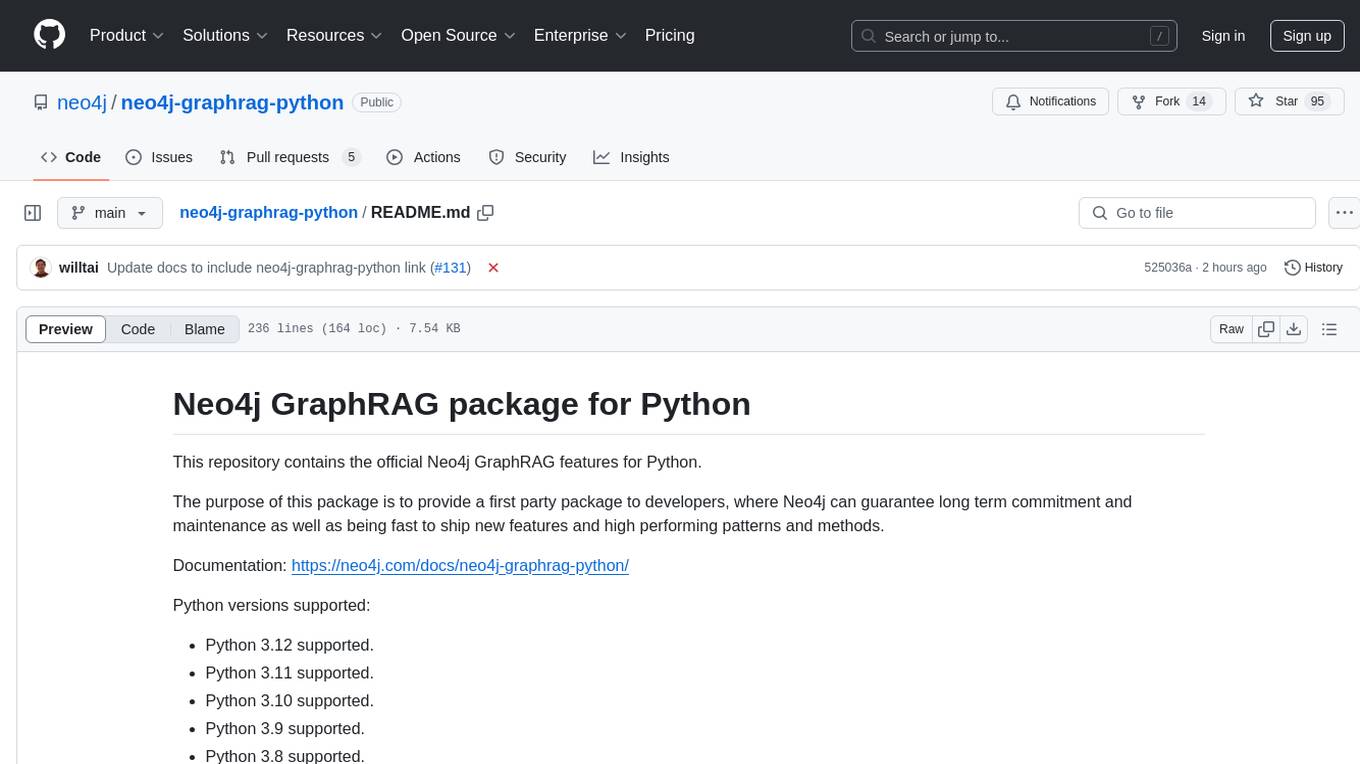
neo4j-graphrag-python
The Neo4j GraphRAG package for Python is an official repository that provides features for creating and managing vector indexes in Neo4j databases. It aims to offer developers a reliable package with long-term commitment, maintenance, and fast feature updates. The package supports various Python versions and includes functionalities for creating vector indexes, populating them, and performing similarity searches. It also provides guidelines for installation, examples, and development processes such as installing dependencies, making changes, and running tests.
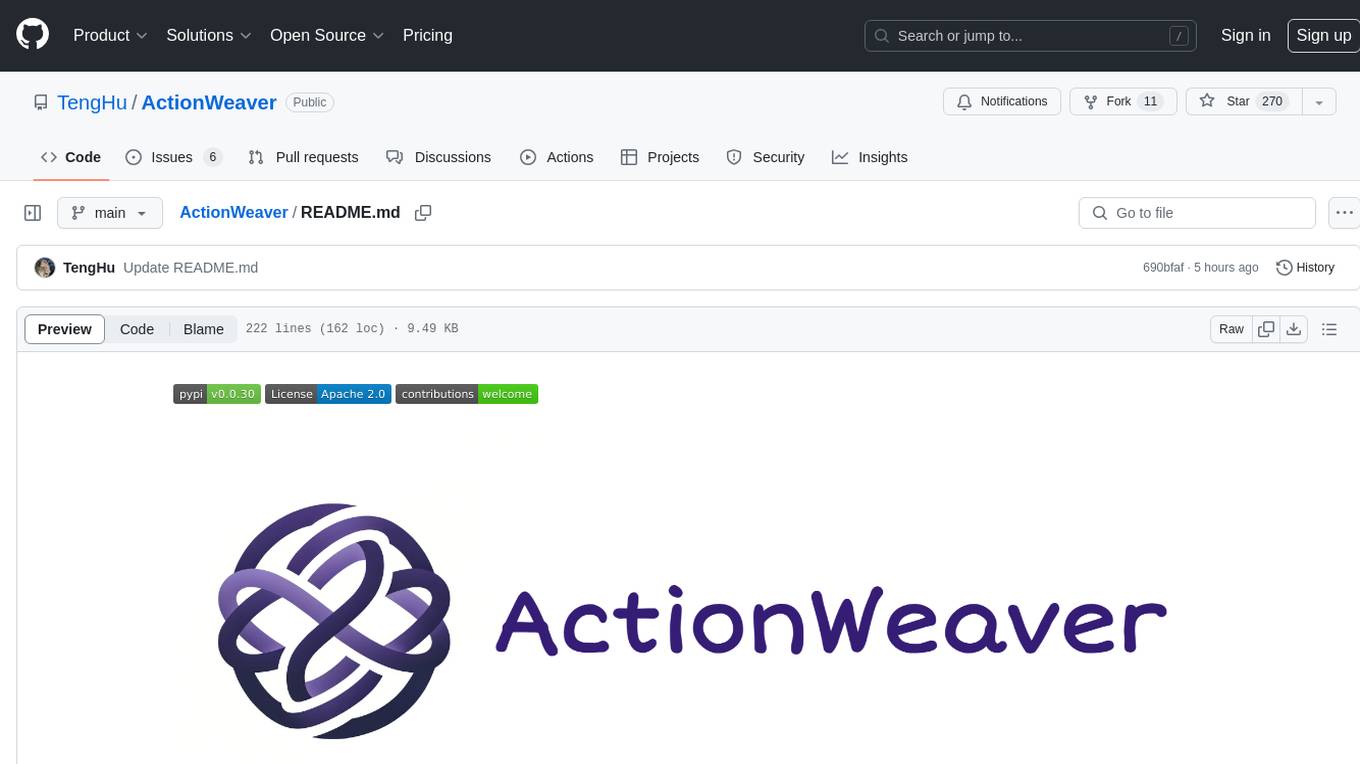
ActionWeaver
ActionWeaver is an AI application framework designed for simplicity, relying on OpenAI and Pydantic. It supports both OpenAI API and Azure OpenAI service. The framework allows for function calling as a core feature, extensibility to integrate any Python code, function orchestration for building complex call hierarchies, and telemetry and observability integration. Users can easily install ActionWeaver using pip and leverage its capabilities to create, invoke, and orchestrate actions with the language model. The framework also provides structured extraction using Pydantic models and allows for exception handling customization. Contributions to the project are welcome, and users are encouraged to cite ActionWeaver if found useful.
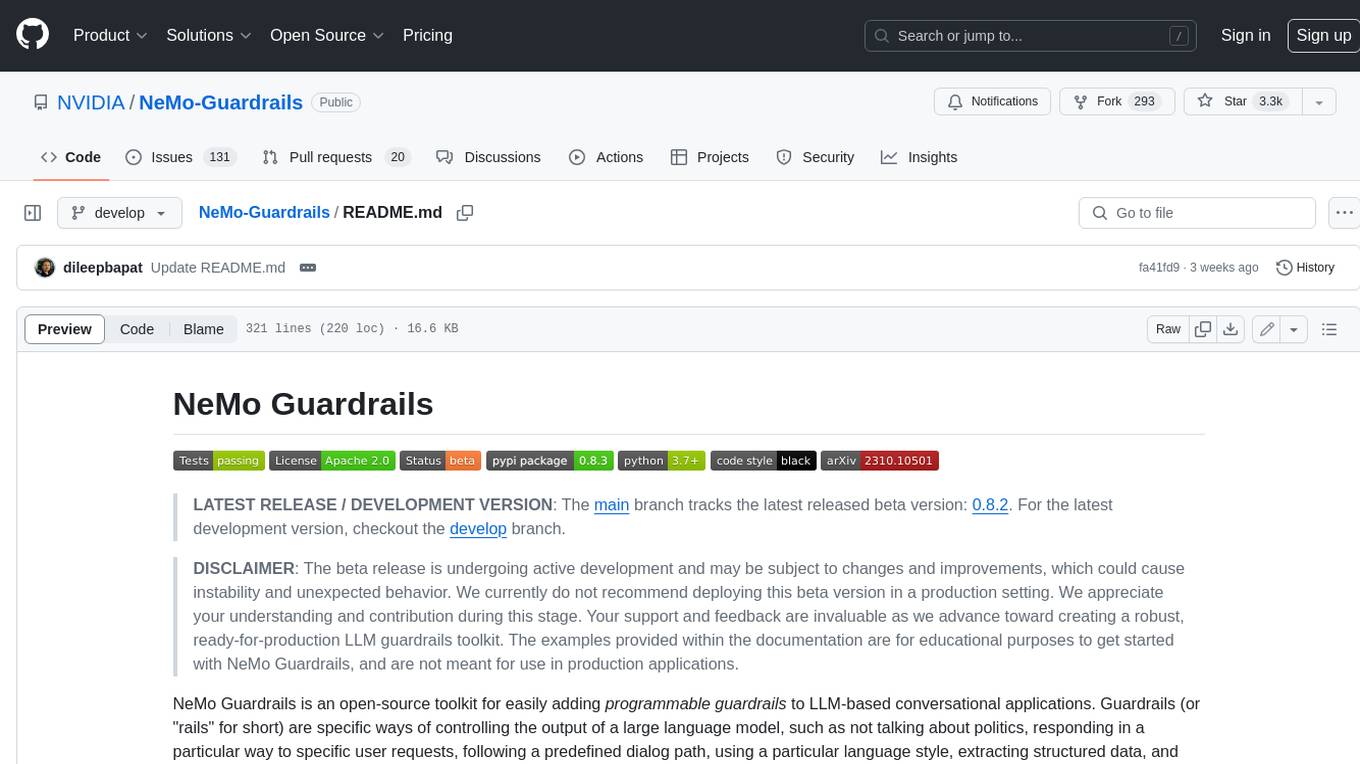
NeMo-Guardrails
NeMo Guardrails is an open-source toolkit for easily adding _programmable guardrails_ to LLM-based conversational applications. Guardrails (or "rails" for short) are specific ways of controlling the output of a large language model, such as not talking about politics, responding in a particular way to specific user requests, following a predefined dialog path, using a particular language style, extracting structured data, and more.
For similar tasks
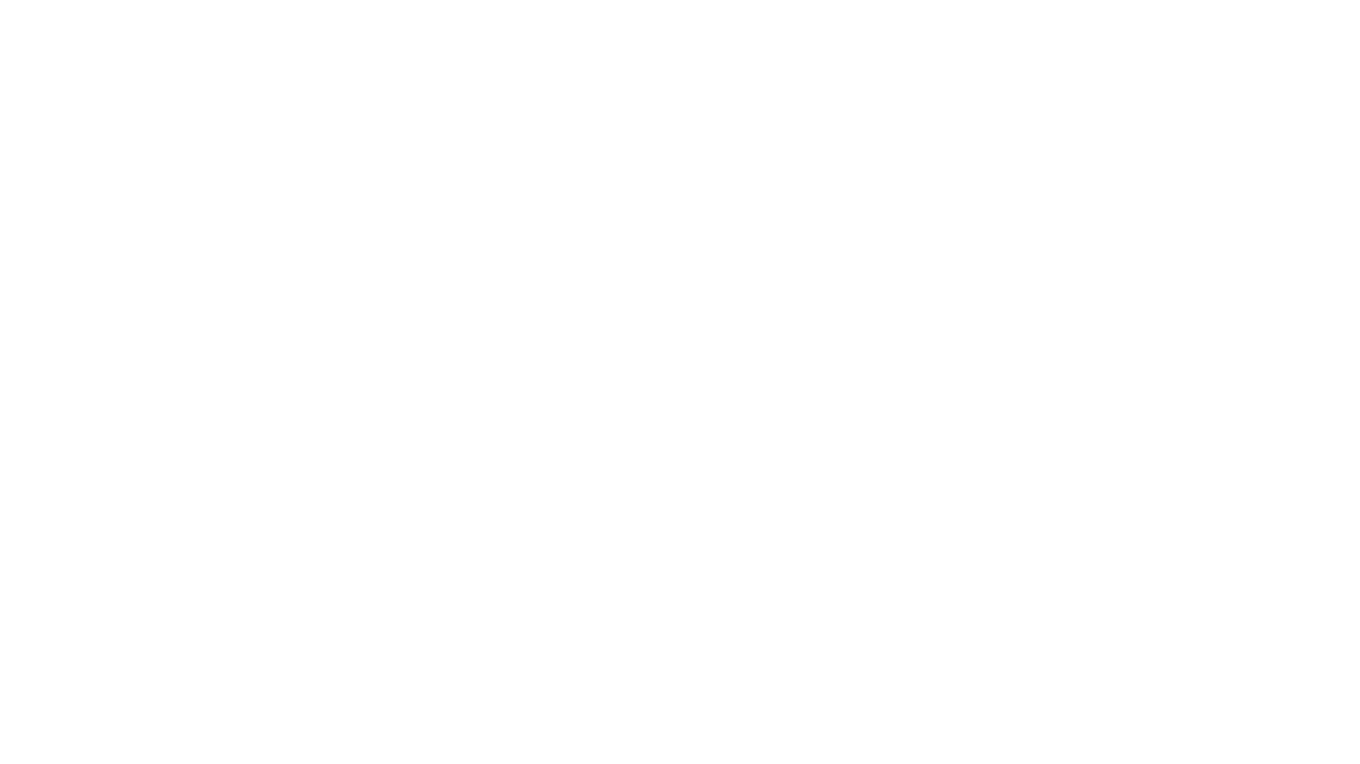
HPT
Hyper-Pretrained Transformers (HPT) is a novel multimodal LLM framework from HyperGAI, trained for vision-language models capable of understanding both textual and visual inputs. The repository contains the open-source implementation of inference code to reproduce the evaluation results of HPT Air on different benchmarks. HPT has achieved competitive results with state-of-the-art models on various multimodal LLM benchmarks. It offers models like HPT 1.5 Air and HPT 1.0 Air, providing efficient solutions for vision-and-language tasks.
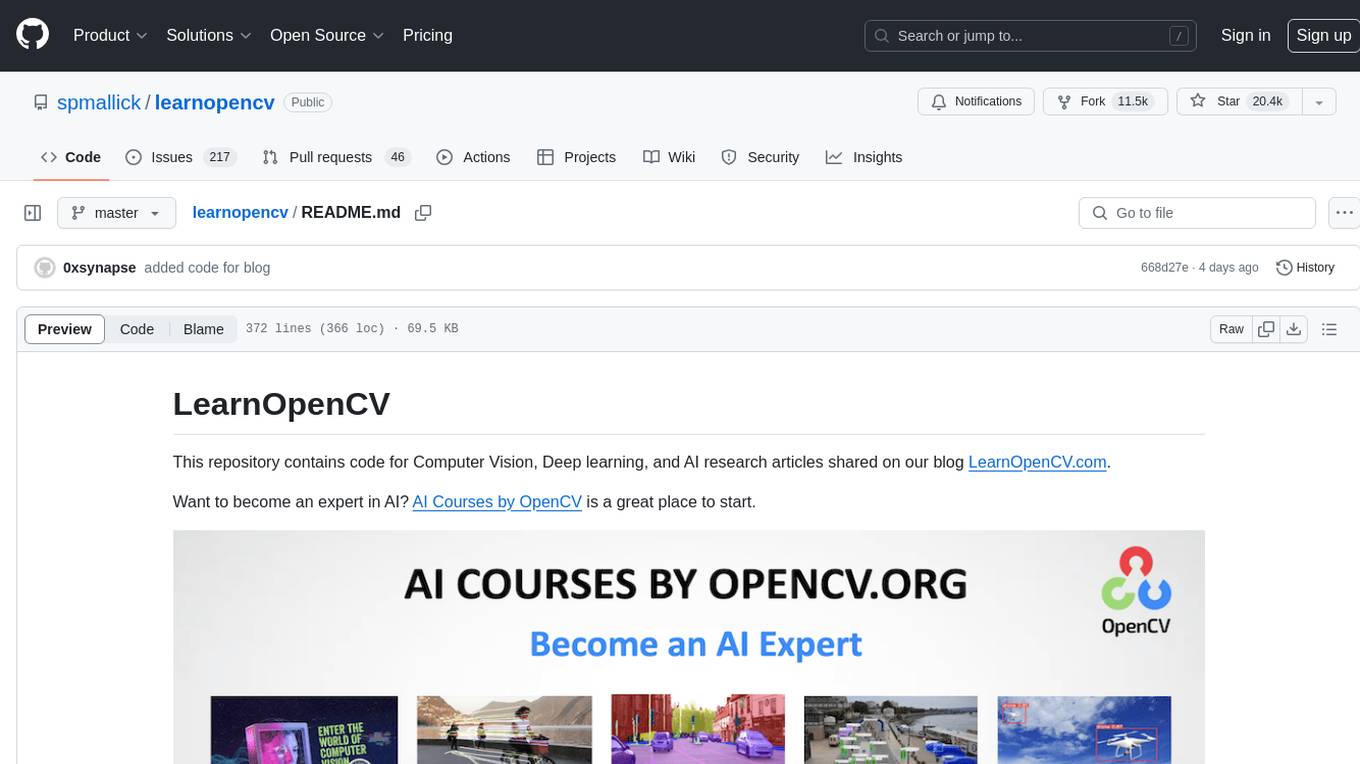
learnopencv
LearnOpenCV is a repository containing code for Computer Vision, Deep learning, and AI research articles shared on the blog LearnOpenCV.com. It serves as a resource for individuals looking to enhance their expertise in AI through various courses offered by OpenCV. The repository includes a wide range of topics such as image inpainting, instance segmentation, robotics, deep learning models, and more, providing practical implementations and code examples for readers to explore and learn from.
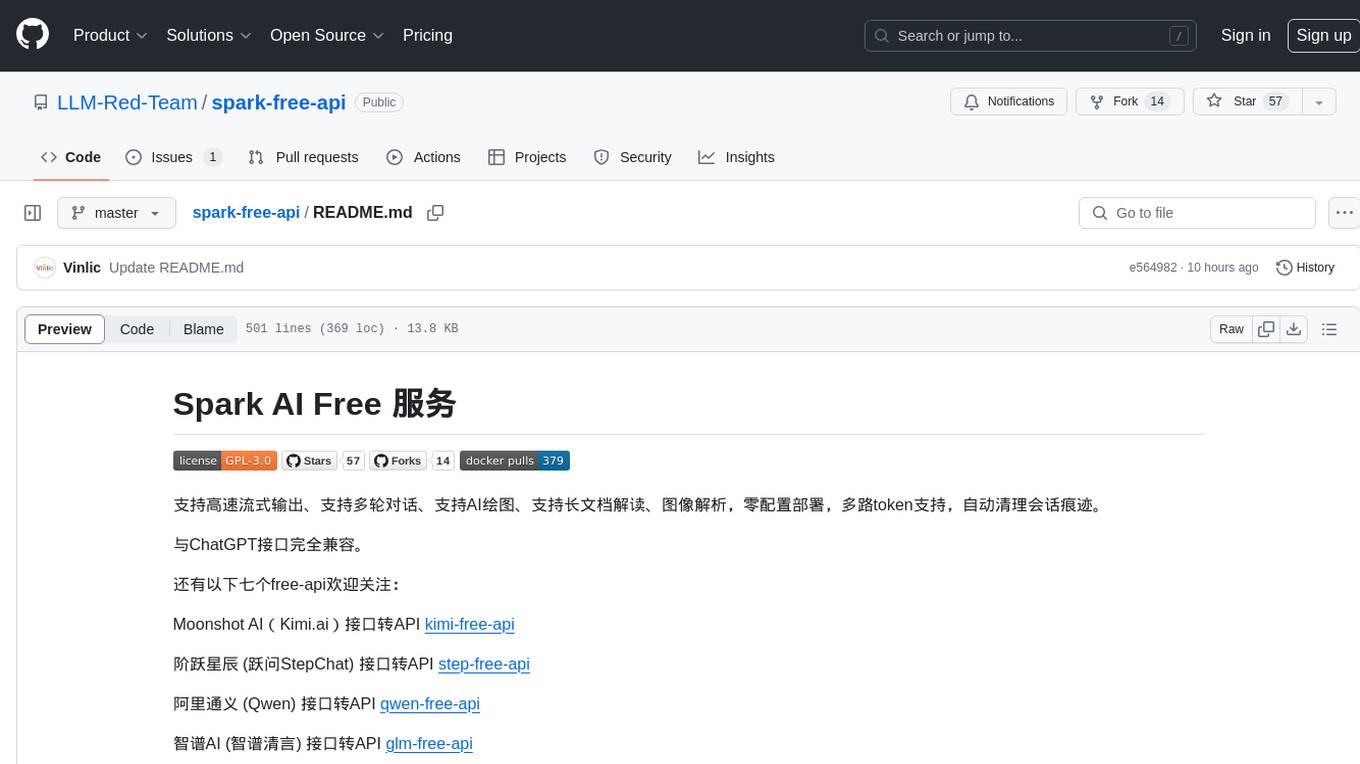
spark-free-api
Spark AI Free 服务 provides high-speed streaming output, multi-turn dialogue support, AI drawing support, long document interpretation, and image parsing. It offers zero-configuration deployment, multi-token support, and automatic session trace cleaning. It is fully compatible with the ChatGPT interface. The repository includes multiple free-api projects for various AI services. Users can access the API for tasks such as chat completions, AI drawing, document interpretation, image analysis, and ssoSessionId live checking. The project also provides guidelines for deployment using Docker, Docker-compose, Render, Vercel, and native deployment methods. It recommends using custom clients for faster and simpler access to the free-api series projects.
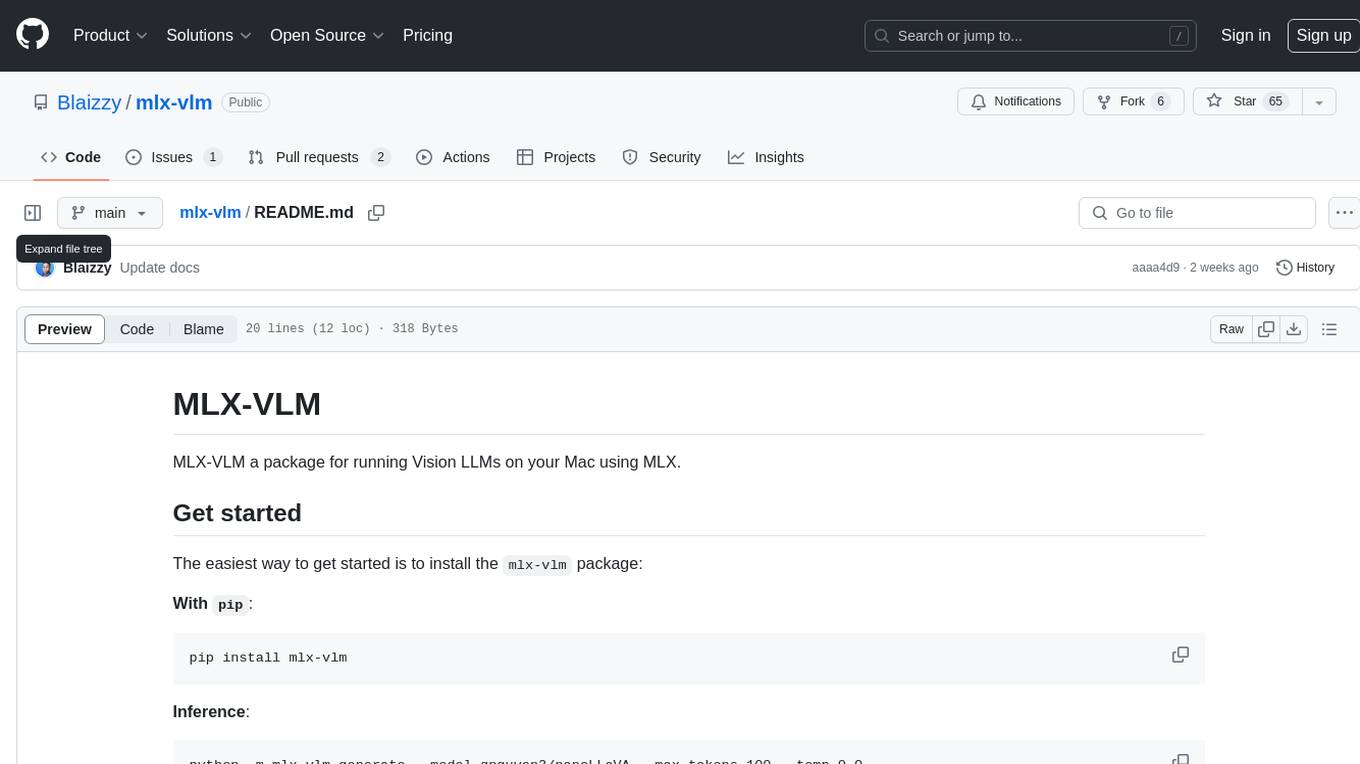
mlx-vlm
MLX-VLM is a package designed for running Vision LLMs on Mac systems using MLX. It provides a convenient way to install and utilize the package for processing large language models related to vision tasks. The tool simplifies the process of running LLMs on Mac computers, offering a seamless experience for users interested in leveraging MLX for vision-related projects.
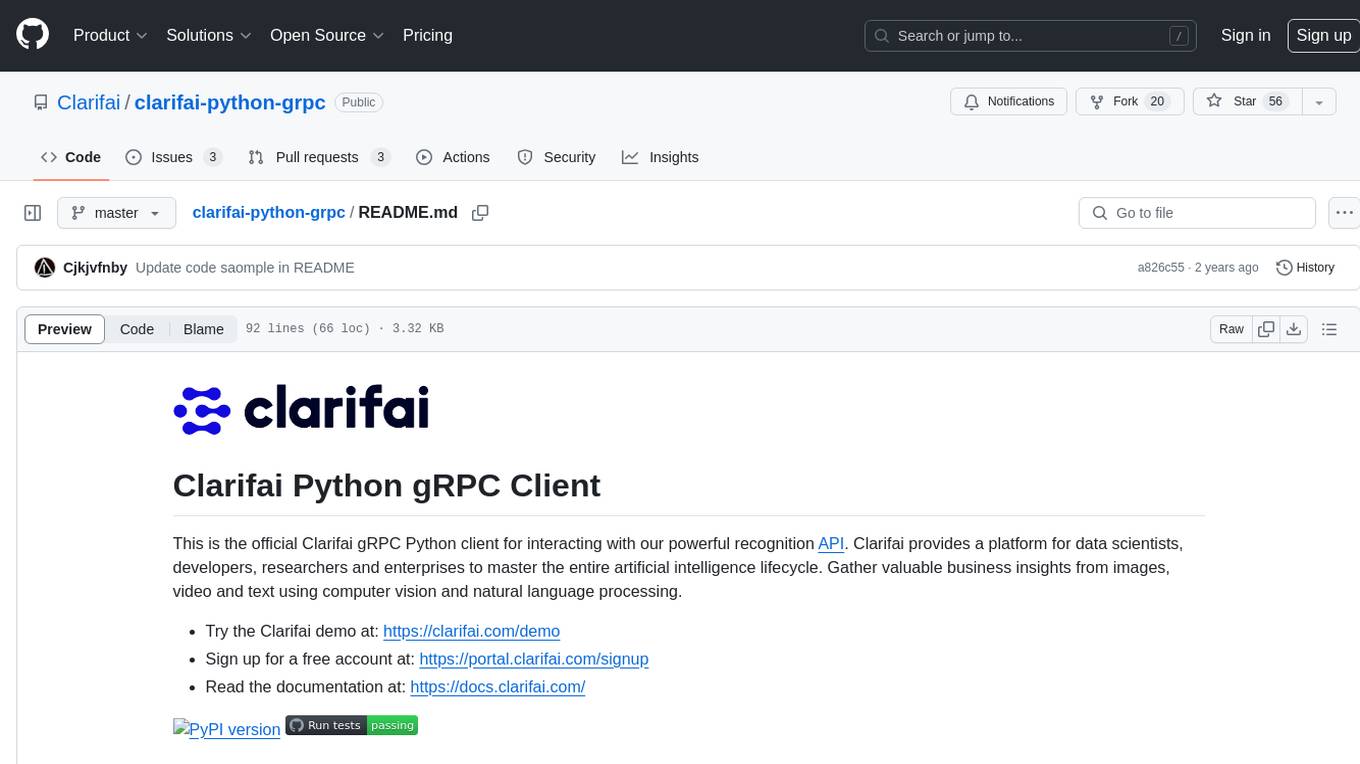
clarifai-python-grpc
This is the official Clarifai gRPC Python client for interacting with their recognition API. Clarifai offers a platform for data scientists, developers, researchers, and enterprises to utilize artificial intelligence for image, video, and text analysis through computer vision and natural language processing. The client allows users to authenticate, predict concepts in images, and access various functionalities provided by the Clarifai API. It follows a versioning scheme that aligns with the backend API updates and includes specific instructions for installation and troubleshooting. Users can explore the Clarifai demo, sign up for an account, and refer to the documentation for detailed information.
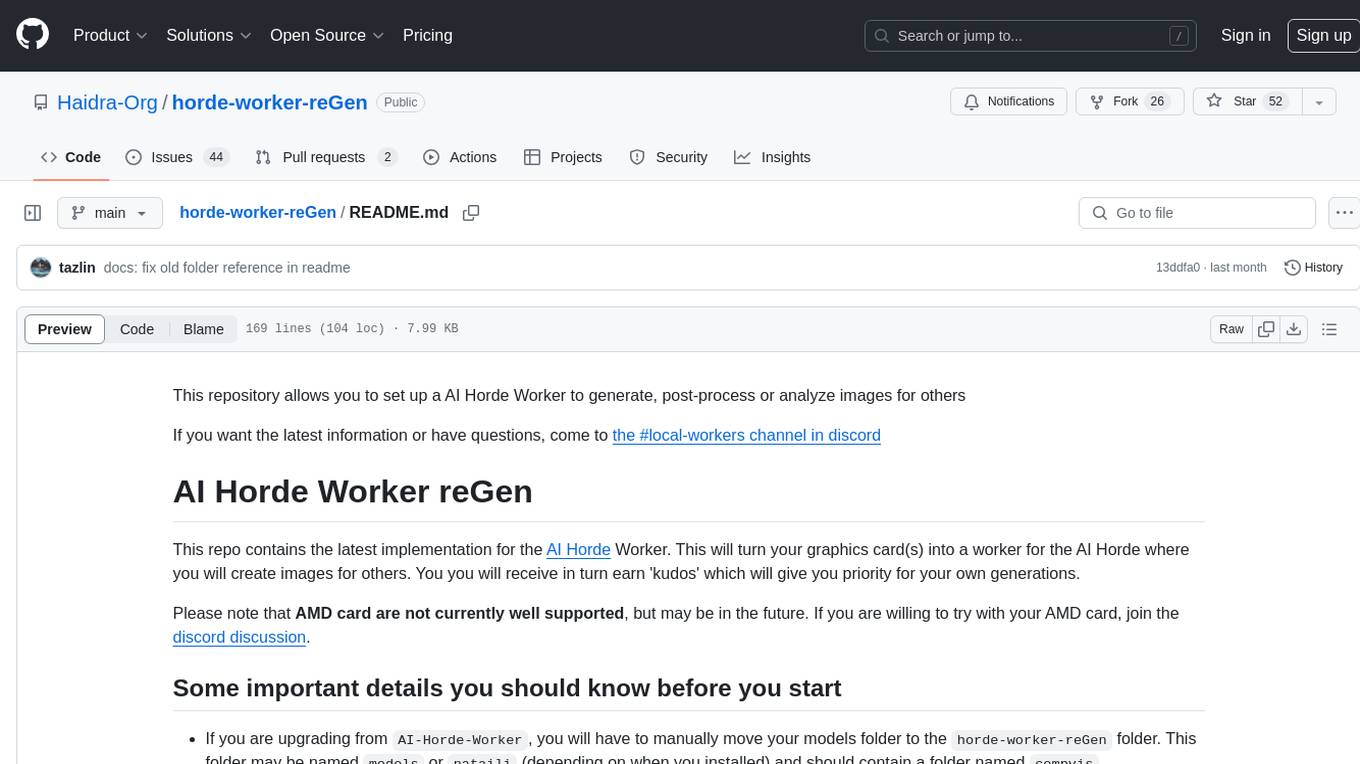
horde-worker-reGen
This repository provides the latest implementation for the AI Horde Worker, allowing users to utilize their graphics card(s) to generate, post-process, or analyze images for others. It offers a platform where users can create images and earn 'kudos' in return, granting priority for their own image generations. The repository includes important details for setup, recommendations for system configurations, instructions for installation on Windows and Linux, basic usage guidelines, and information on updating the AI Horde Worker. Users can also run the worker with multiple GPUs and receive notifications for updates through Discord. Additionally, the repository contains models that are licensed under the CreativeML OpenRAIL License.
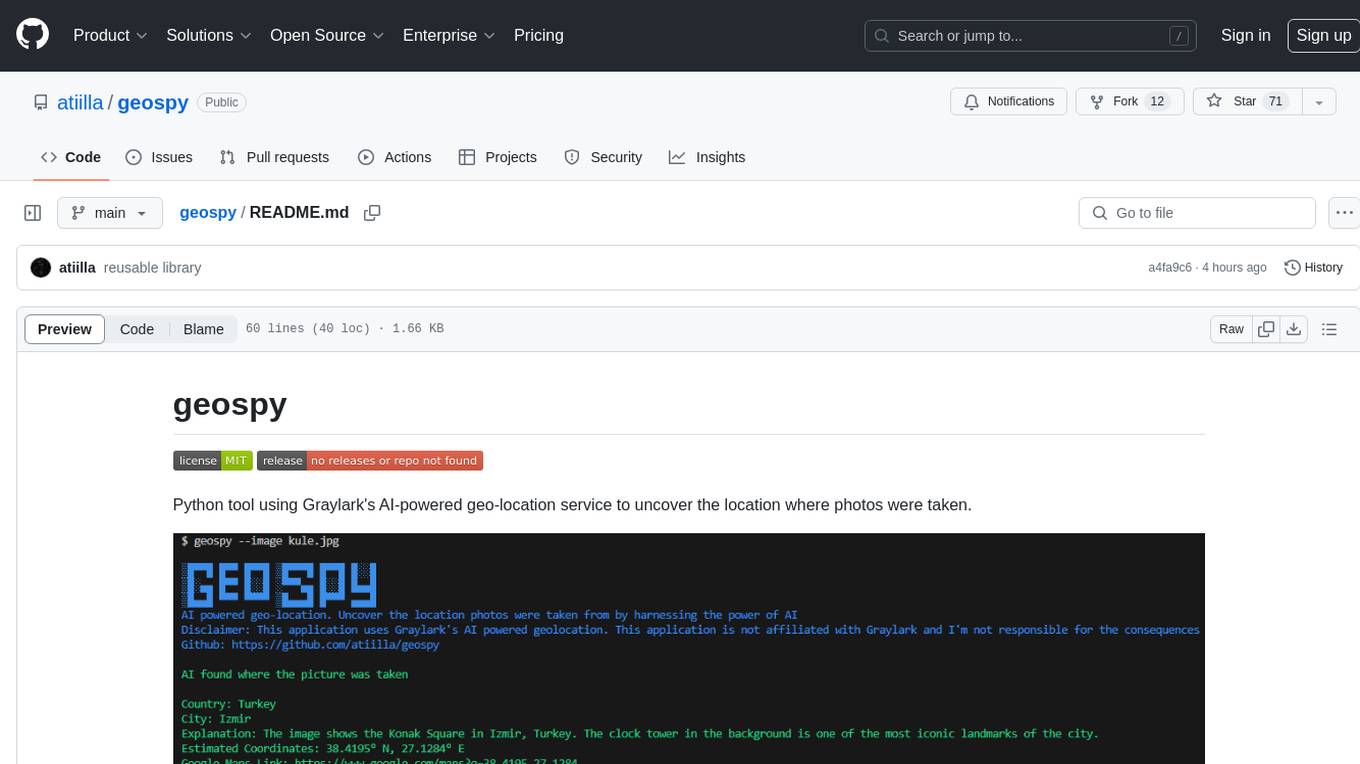
geospy
Geospy is a Python tool that utilizes Graylark's AI-powered geolocation service to determine the location where photos were taken. It allows users to analyze images and retrieve information such as country, city, explanation, coordinates, and Google Maps links. The tool provides a seamless way to integrate geolocation services into various projects and applications.
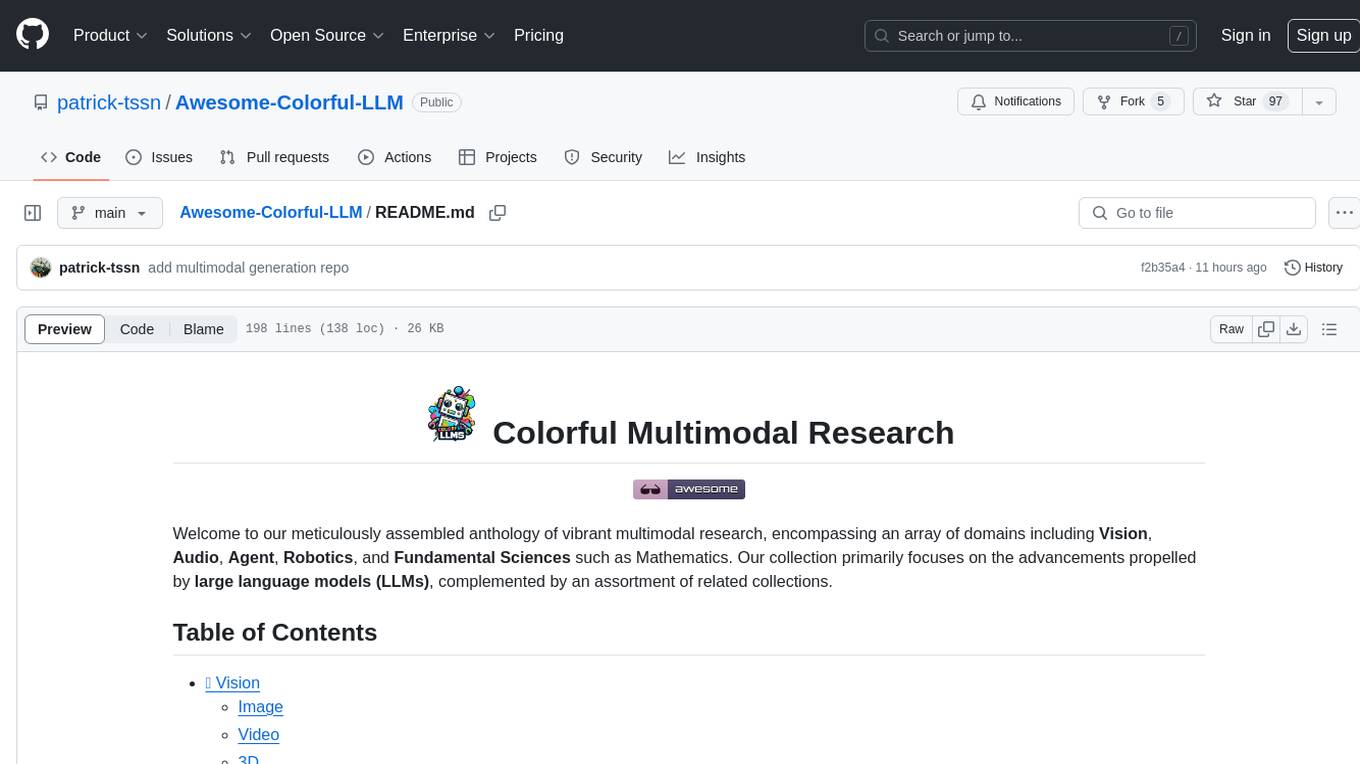
Awesome-Colorful-LLM
Awesome-Colorful-LLM is a meticulously assembled anthology of vibrant multimodal research focusing on advancements propelled by large language models (LLMs) in domains such as Vision, Audio, Agent, Robotics, and Fundamental Sciences like Mathematics. The repository contains curated collections of works, datasets, benchmarks, projects, and tools related to LLMs and multimodal learning. It serves as a comprehensive resource for researchers and practitioners interested in exploring the intersection of language models and various modalities for tasks like image understanding, video pretraining, 3D modeling, document understanding, audio analysis, agent learning, robotic applications, and mathematical research.
For similar jobs
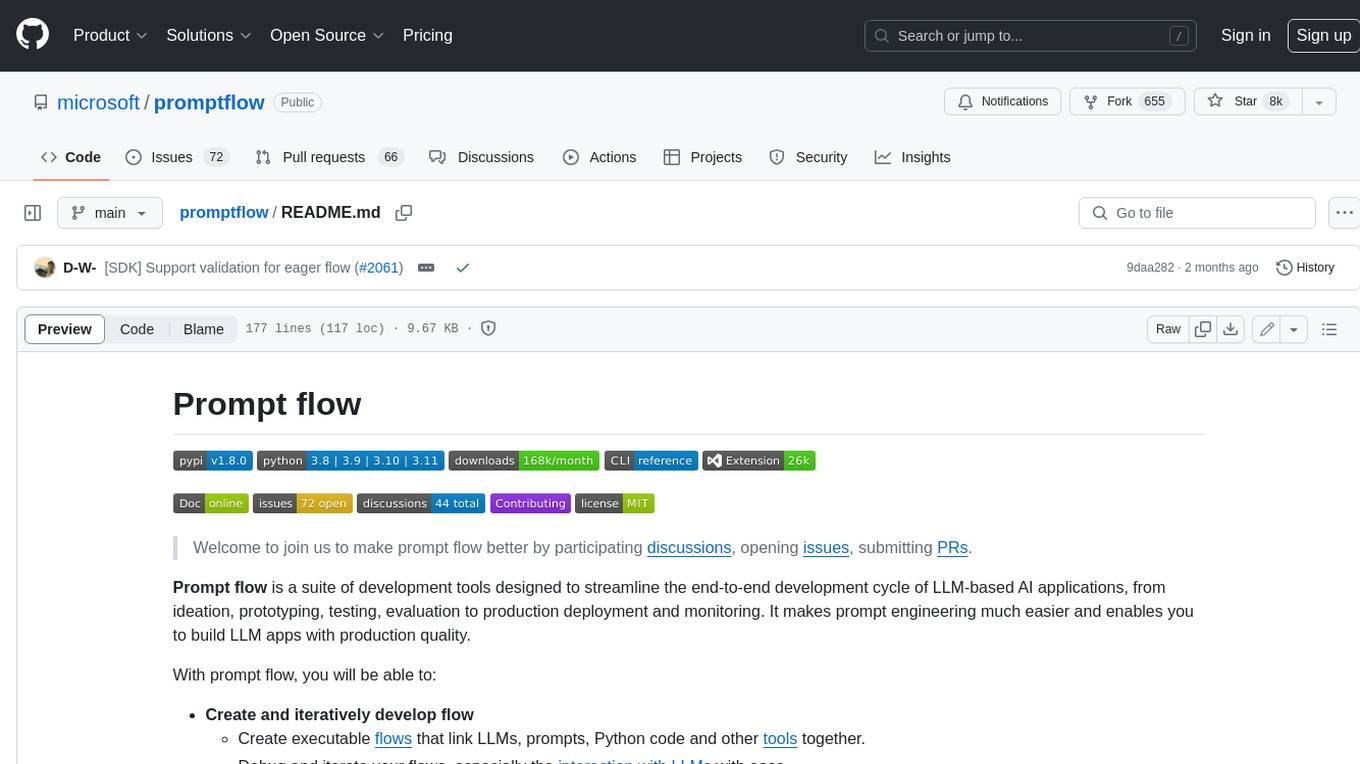
promptflow
**Prompt flow** is a suite of development tools designed to streamline the end-to-end development cycle of LLM-based AI applications, from ideation, prototyping, testing, evaluation to production deployment and monitoring. It makes prompt engineering much easier and enables you to build LLM apps with production quality.
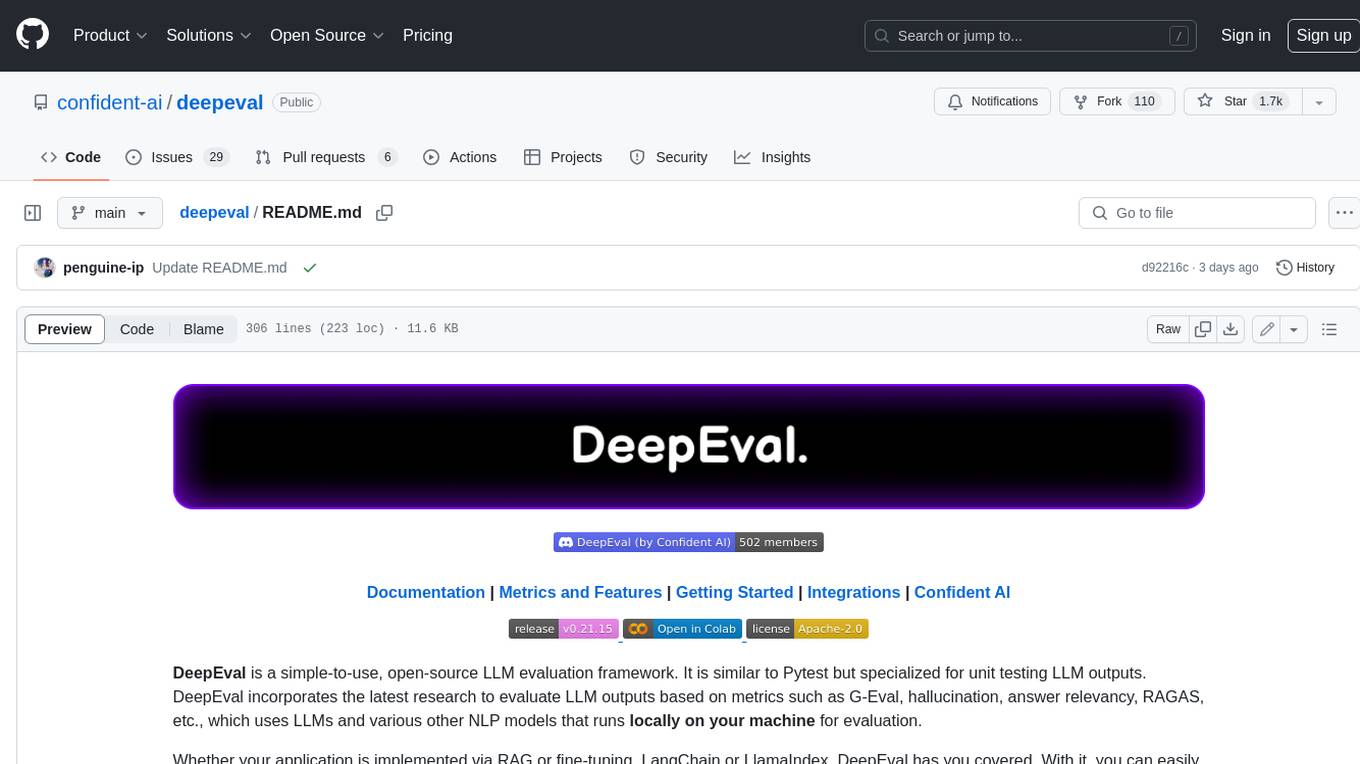
deepeval
DeepEval is a simple-to-use, open-source LLM evaluation framework specialized for unit testing LLM outputs. It incorporates various metrics such as G-Eval, hallucination, answer relevancy, RAGAS, etc., and runs locally on your machine for evaluation. It provides a wide range of ready-to-use evaluation metrics, allows for creating custom metrics, integrates with any CI/CD environment, and enables benchmarking LLMs on popular benchmarks. DeepEval is designed for evaluating RAG and fine-tuning applications, helping users optimize hyperparameters, prevent prompt drifting, and transition from OpenAI to hosting their own Llama2 with confidence.
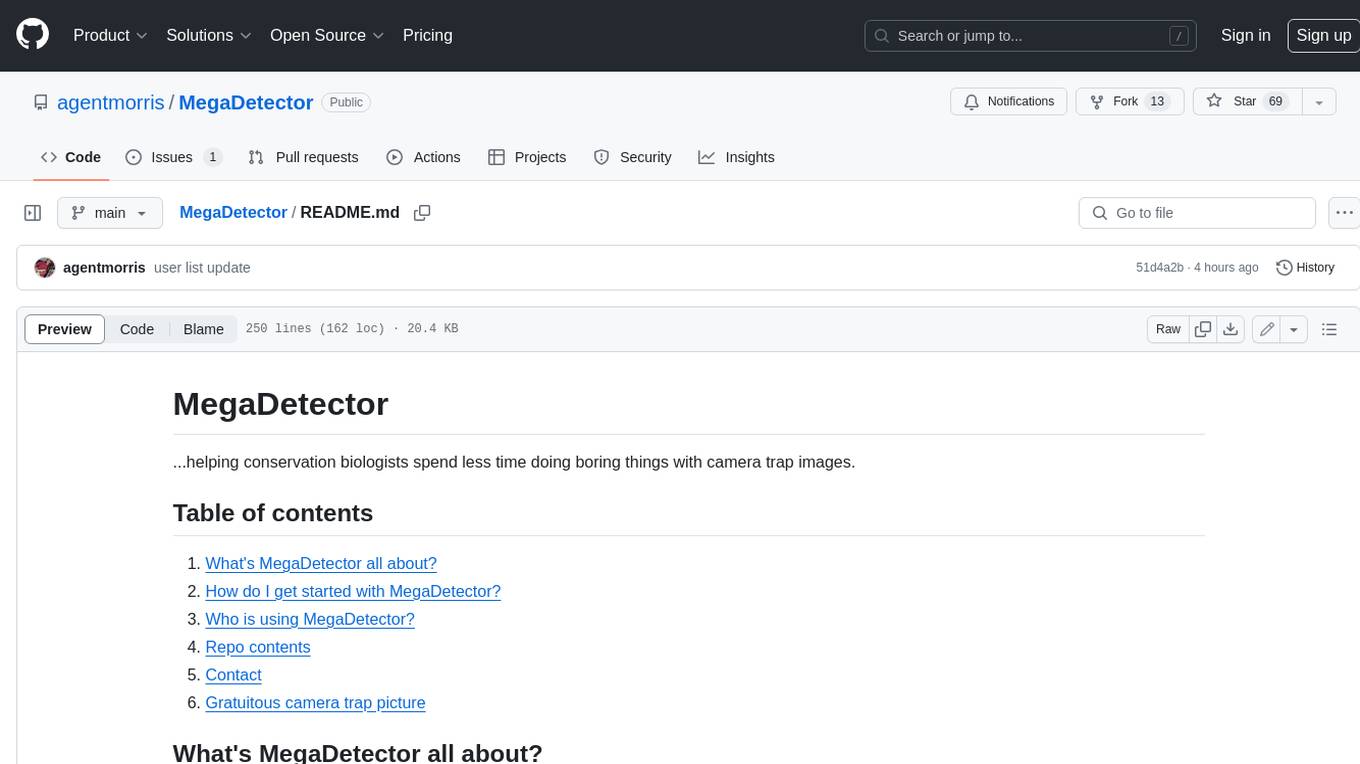
MegaDetector
MegaDetector is an AI model that identifies animals, people, and vehicles in camera trap images (which also makes it useful for eliminating blank images). This model is trained on several million images from a variety of ecosystems. MegaDetector is just one of many tools that aims to make conservation biologists more efficient with AI. If you want to learn about other ways to use AI to accelerate camera trap workflows, check out our of the field, affectionately titled "Everything I know about machine learning and camera traps".
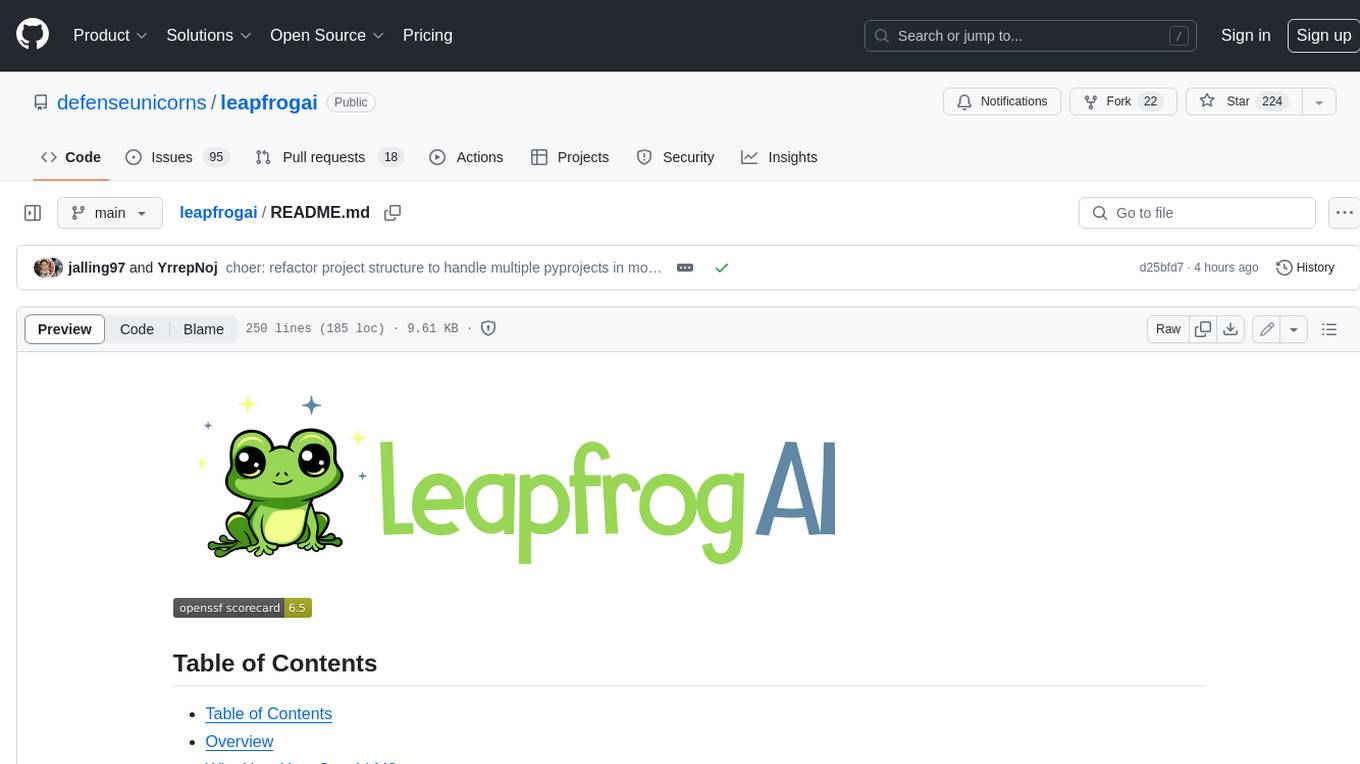
leapfrogai
LeapfrogAI is a self-hosted AI platform designed to be deployed in air-gapped resource-constrained environments. It brings sophisticated AI solutions to these environments by hosting all the necessary components of an AI stack, including vector databases, model backends, API, and UI. LeapfrogAI's API closely matches that of OpenAI, allowing tools built for OpenAI/ChatGPT to function seamlessly with a LeapfrogAI backend. It provides several backends for various use cases, including llama-cpp-python, whisper, text-embeddings, and vllm. LeapfrogAI leverages Chainguard's apko to harden base python images, ensuring the latest supported Python versions are used by the other components of the stack. The LeapfrogAI SDK provides a standard set of protobuffs and python utilities for implementing backends and gRPC. LeapfrogAI offers UI options for common use-cases like chat, summarization, and transcription. It can be deployed and run locally via UDS and Kubernetes, built out using Zarf packages. LeapfrogAI is supported by a community of users and contributors, including Defense Unicorns, Beast Code, Chainguard, Exovera, Hypergiant, Pulze, SOSi, United States Navy, United States Air Force, and United States Space Force.
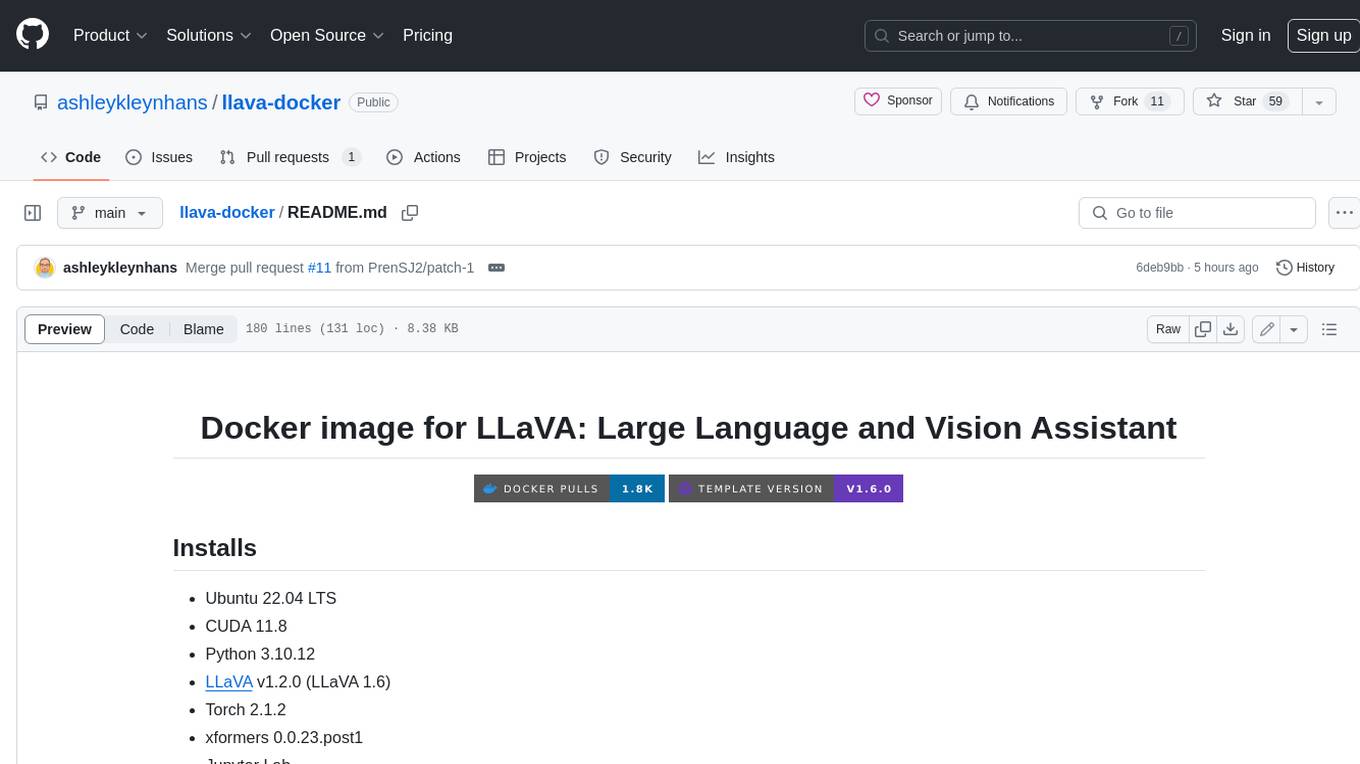
llava-docker
This Docker image for LLaVA (Large Language and Vision Assistant) provides a convenient way to run LLaVA locally or on RunPod. LLaVA is a powerful AI tool that combines natural language processing and computer vision capabilities. With this Docker image, you can easily access LLaVA's functionalities for various tasks, including image captioning, visual question answering, text summarization, and more. The image comes pre-installed with LLaVA v1.2.0, Torch 2.1.2, xformers 0.0.23.post1, and other necessary dependencies. You can customize the model used by setting the MODEL environment variable. The image also includes a Jupyter Lab environment for interactive development and exploration. Overall, this Docker image offers a comprehensive and user-friendly platform for leveraging LLaVA's capabilities.
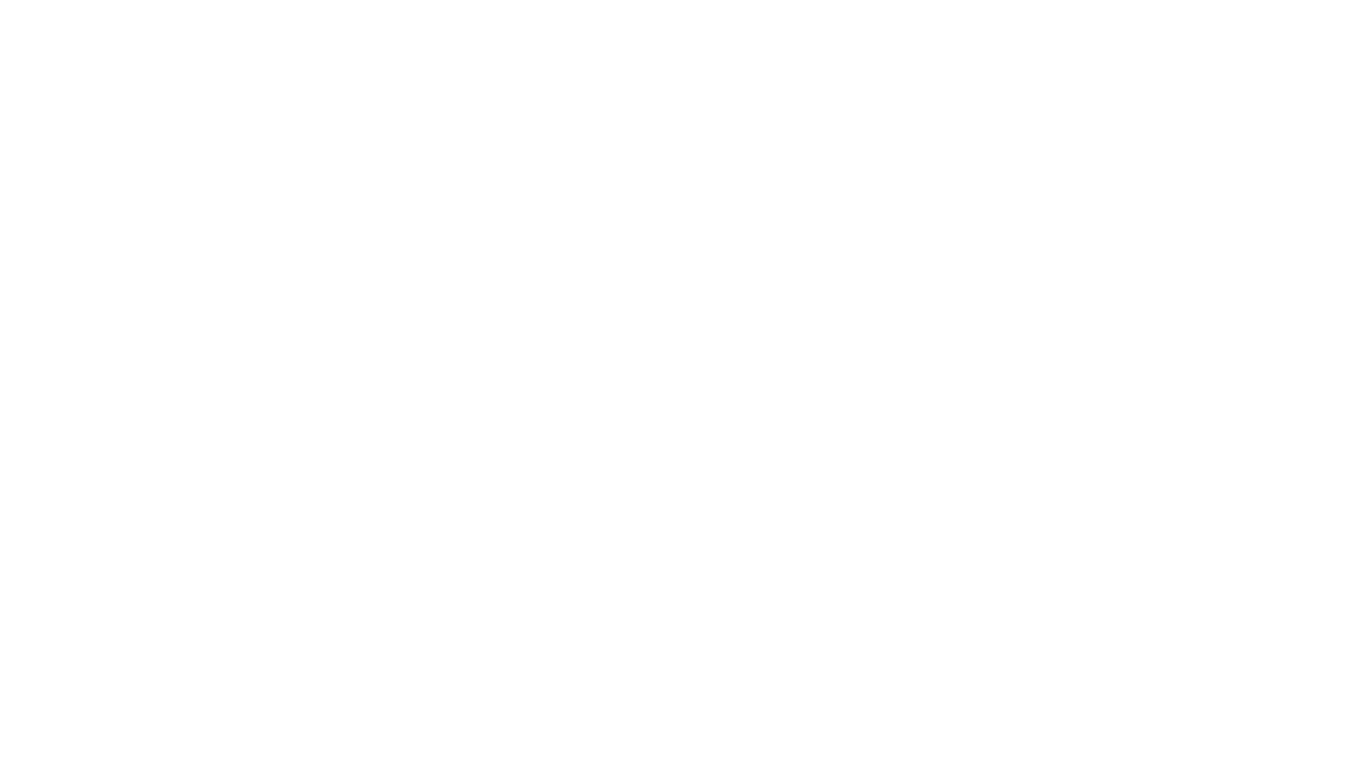
carrot
The 'carrot' repository on GitHub provides a list of free and user-friendly ChatGPT mirror sites for easy access. The repository includes sponsored sites offering various GPT models and services. Users can find and share sites, report errors, and access stable and recommended sites for ChatGPT usage. The repository also includes a detailed list of ChatGPT sites, their features, and accessibility options, making it a valuable resource for ChatGPT users seeking free and unlimited GPT services.
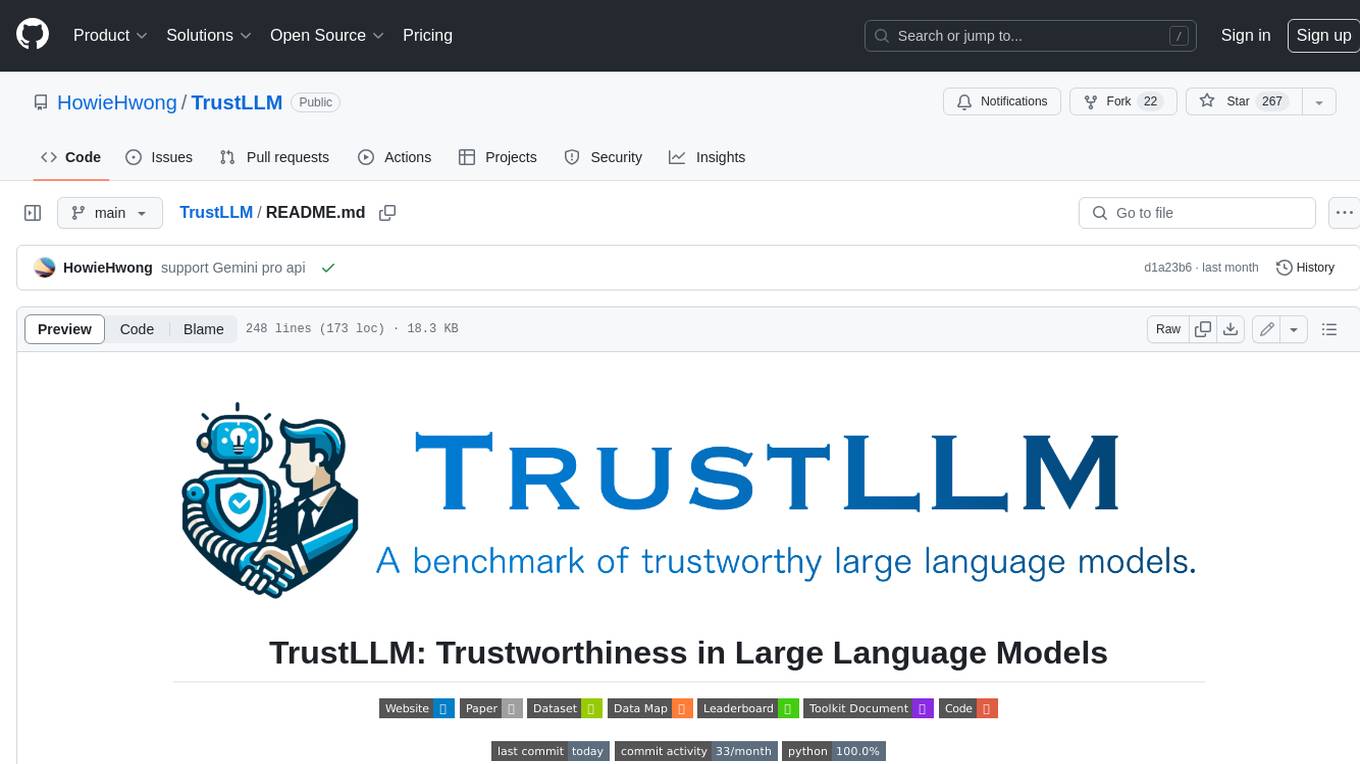
TrustLLM
TrustLLM is a comprehensive study of trustworthiness in LLMs, including principles for different dimensions of trustworthiness, established benchmark, evaluation, and analysis of trustworthiness for mainstream LLMs, and discussion of open challenges and future directions. Specifically, we first propose a set of principles for trustworthy LLMs that span eight different dimensions. Based on these principles, we further establish a benchmark across six dimensions including truthfulness, safety, fairness, robustness, privacy, and machine ethics. We then present a study evaluating 16 mainstream LLMs in TrustLLM, consisting of over 30 datasets. The document explains how to use the trustllm python package to help you assess the performance of your LLM in trustworthiness more quickly. For more details about TrustLLM, please refer to project website.
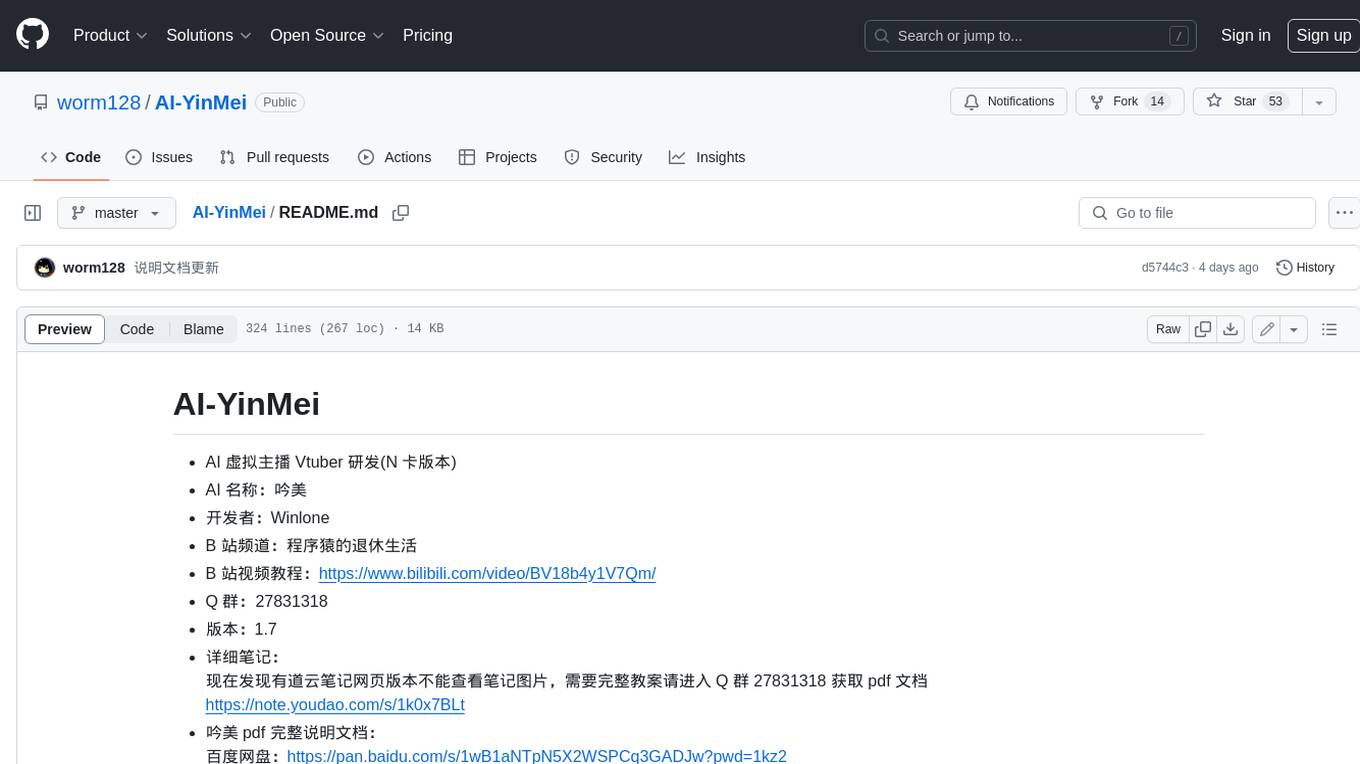
AI-YinMei
AI-YinMei is an AI virtual anchor Vtuber development tool (N card version). It supports fastgpt knowledge base chat dialogue, a complete set of solutions for LLM large language models: [fastgpt] + [one-api] + [Xinference], supports docking bilibili live broadcast barrage reply and entering live broadcast welcome speech, supports Microsoft edge-tts speech synthesis, supports Bert-VITS2 speech synthesis, supports GPT-SoVITS speech synthesis, supports expression control Vtuber Studio, supports painting stable-diffusion-webui output OBS live broadcast room, supports painting picture pornography public-NSFW-y-distinguish, supports search and image search service duckduckgo (requires magic Internet access), supports image search service Baidu image search (no magic Internet access), supports AI reply chat box [html plug-in], supports AI singing Auto-Convert-Music, supports playlist [html plug-in], supports dancing function, supports expression video playback, supports head touching action, supports gift smashing action, supports singing automatic start dancing function, chat and singing automatic cycle swing action, supports multi scene switching, background music switching, day and night automatic switching scene, supports open singing and painting, let AI automatically judge the content.