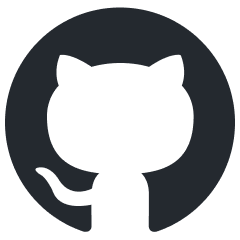
langchain
Elixir implementation of a LangChain style framework that lets Elixir projects integrate with and leverage LLMs.
Stars: 830
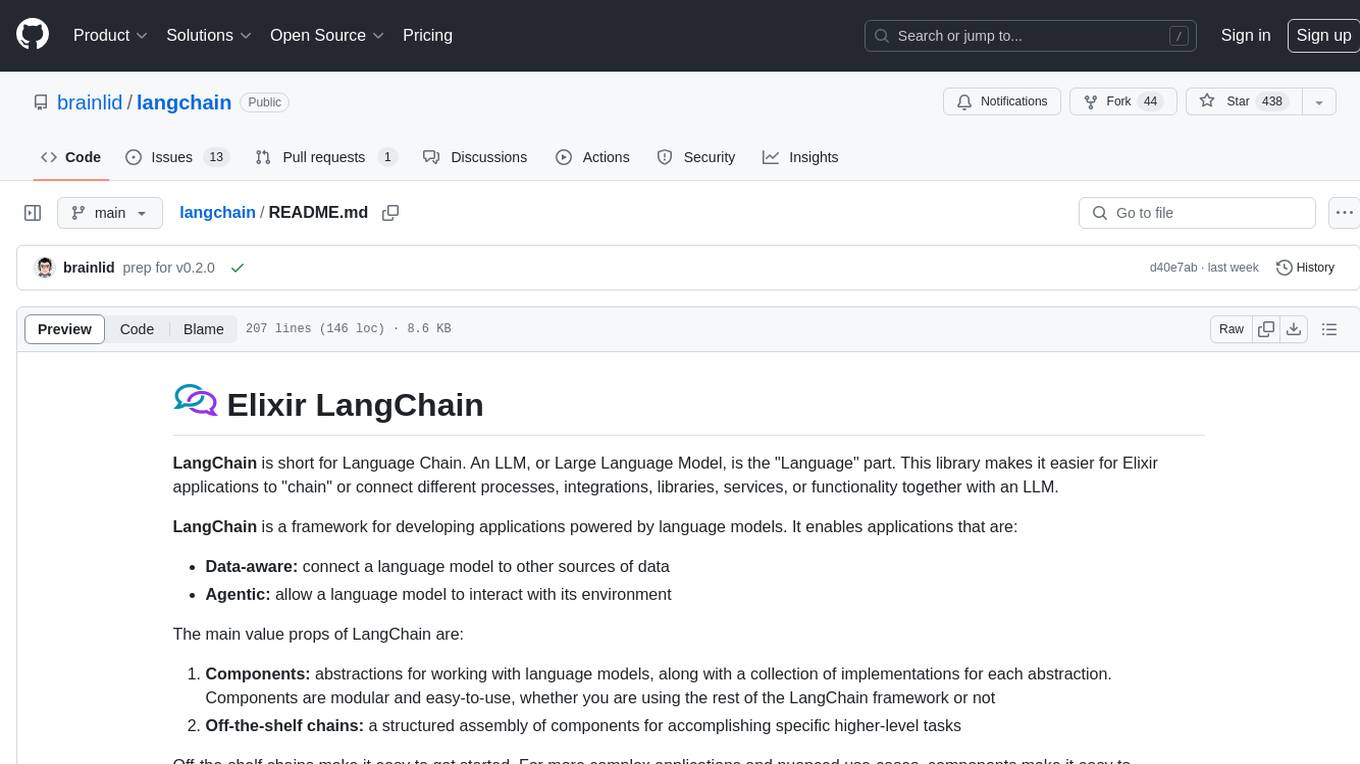
LangChain is a framework for developing Elixir applications powered by language models. It enables applications to connect language models to other data sources and interact with the environment. The library provides components for working with language models and off-the-shelf chains for specific tasks. It aims to assist in building applications that combine large language models with other sources of computation or knowledge. LangChain is written in Elixir and is not aimed for parity with the JavaScript and Python versions due to differences in programming paradigms and design choices. The library is designed to make it easy to integrate language models into applications and expose features, data, and functionality to the models.
README:
Elixir LangChain enables Elixir applications to integrate AI services and self-hosted models into an application.
Currently supported AI services:
- OpenAI ChatGPT
- OpenAI DALL-e 2 - image generation
- Anthropic Claude
- Google Gemini
- Google Vertex AI (Google's enterprise offering)
- Ollama
- Mistral
- Bumblebee self-hosted models - including Llama, Mistral and Zephyr
- LMStudio via their OpenAI compatibility API
- Perplexity
LangChain is short for Language Chain. An LLM, or Large Language Model, is the "Language" part. This library makes it easier for Elixir applications to "chain" or connect different processes, integrations, libraries, services, or functionality together with an LLM.
LangChain is a framework for developing applications powered by language models. It enables applications that are:
- Data-aware: connect a language model to other sources of data
- Agentic: allow a language model to interact with its environment
The main value props of LangChain are:
- Components: abstractions for working with language models, along with a collection of implementations for each abstraction. Components are modular and easy-to-use, whether you are using the rest of the LangChain framework or not
- Off-the-shelf chains: a structured assembly of components for accomplishing specific higher-level tasks
Off-the-shelf chains make it easy to get started. For more complex applications and nuanced use-cases, components make it easy to customize existing chains or build new ones.
Large Language Models (LLMs) are emerging as a transformative technology, enabling developers to build applications that they previously could not. But using these LLMs in isolation is often not enough to create a truly powerful app - the real power comes when you can combine them with other sources of computation or knowledge.
This library is aimed at assisting in the development of those types of applications.
The online documentation can be found here.
Check out the demo project that you can download and review.
This library is written in Elixir and intended to be used with Elixir applications. The original libraries are LangChain JS/TS and LangChain Python.
The JavaScript and Python projects aim to integrate with each other as seamlessly as possible. The intended integration is so strong that that all objects (prompts, LLMs, chains, etc) are designed in a way where they can be serialized and shared between the two languages.
This Elixir version does not aim for parity with the JavaScript and Python libraries. Why not?
- JavaScript and Python are both Object Oriented languages. Elixir is Functional. We're not going to force a design that doesn't apply.
- The JS and Python versions started before conversational LLMs were standard. They put a lot of effort into preserving history (like a conversation) when the LLM didn't support it. We're not doing that here.
This library was heavily inspired by, and based on, the way the JavaScript library actually worked and interacted with an LLM.
The package can be installed by adding langchain
to your list of dependencies
in mix.exs
:
def deps do
[
{:langchain, "0.2.0"}
]
end
The Release Candidate includes many additional features and some breaking changes.
def deps do
[
{:langchain, "0.3.0-rc.0"}
]
end
Currently, the library is written to use the Req
library for making API calls.
You can configure an organization ID, and API key for OpenAI's API, but this library also works with other compatible APIs as well as other services and even local models running on Bumblebee.
config/runtime.exs
:
config :langchain, openai_key: System.fetch_env!("OPENAI_API_KEY")
config :langchain, openai_org_id: System.fetch_env!("OPENAI_ORG_ID")
# OR
config :langchain, openai_key: "YOUR SECRET KEY"
config :langchain, openai_org_id: "YOUR_OPENAI_ORG_ID"
config :langchain, :anthropic_key, System.fetch_env!("ANTHROPIC_API_KEY")
It's possible to use a function or a tuple to resolve the secret:
config :langchain, openai_key: {MyApp.Secrets, :openai_api_key, []}
config :langchain, openai_org_id: {MyApp.Secrets, :openai_org_id, []}
# OR
config :langchain, openai_key: fn -> System.fetch_env!("OPENAI_API_KEY") end
config :langchain, openai_org_id: fn -> System.fetch_env!("OPENAI_ORG_ID") end
The API keys should be treated as secrets and not checked into your repository.
For fly.io, adding the secrets looks like this:
fly secrets set OPENAI_API_KEY=MyOpenAIApiKey
fly secrets set ANTHROPIC_API_KEY=MyAnthropicApiKey
A list of models to use:
- Anthropic Claude models
- Anthropic models on AWS Bedrock
- OpenAI models
- OpenAI models on Azure
- Gemini AI models
ChatGPT and Claude both offer prefix-based prompt caching, which can offer cost and performance benefits for longer prompts. Gemini offers context caching, which is similar.
- ChatGPT's prompt caching is automatic for prompts longer than 1024 tokens, caching the longest common prefix.
- Claude's prompt caching is not automatic. It's prefixing processes tools, system, and then messages, in that order, up to and including the block designated with {"cache_control": {"type": "ephemeral"}} . See LangChain.ChatModels.ChatAnthropicTest and for an example.
- Gemini's context caching requires a separate call which is not supported by Langchain.
The central module in this library is LangChain.Chains.LLMChain
. Most other pieces are either inputs to this, or structures used by it. For understanding how to use the library, start there.
A really powerful feature of LangChain is making it easy to integrate an LLM into your application and expose features, data, and functionality from your application to the LLM.
A LangChain.Function
bridges the gap between the LLM and our application code. We choose what to expose and using context
, we can ensure any actions are limited to what the user has permission to do and access.
For an interactive example, refer to the project Livebook notebook "LangChain: Executing Custom Elixir Functions".
The following is an example of a function that receives parameter arguments.
alias LangChain.Function
alias LangChain.Message
alias LangChain.Chains.LLMChain
alias LangChain.ChatModels.ChatOpenAI
alias LangChain.Utils.ChainResult
# map of data we want to be passed as `context` to the function when
# executed.
custom_context = %{
"user_id" => 123,
"hairbrush" => "drawer",
"dog" => "backyard",
"sandwich" => "kitchen"
}
# a custom Elixir function made available to the LLM
custom_fn =
Function.new!(%{
name: "custom",
description: "Returns the location of the requested element or item.",
parameters_schema: %{
type: "object",
properties: %{
thing: %{
type: "string",
description: "The thing whose location is being requested."
}
},
required: ["thing"]
},
function: fn %{"thing" => thing} = _arguments, context ->
# our context is a pretend item/location location map
{:ok, context[thing]}
end
})
# create and run the chain
{:ok, updated_chain} =
LLMChain.new!(%{
llm: ChatOpenAI.new!(),
custom_context: custom_context,
verbose: true
})
|> LLMChain.add_tools(custom_fn)
|> LLMChain.add_message(Message.new_user!("Where is the hairbrush located?"))
|> LLMChain.run(mode: :while_needs_response)
# print the LLM's answer
IO.puts(ChainResult.to_string!(updated_chain))
# => "The hairbrush is located in the drawer."
There are several services or self-hosted applications that provide an OpenAI compatible API for ChatGPT-like behavior. To use a service like that, the endpoint
of the ChatOpenAI
struct can be pointed to an API compatible endpoint
for chats.
For example, if a locally running service provided that feature, the following code could connect to the service:
{:ok, updated_chain} =
LLMChain.new!(%{
llm: ChatOpenAI.new!(%{endpoint: "http://localhost:1234/v1/chat/completions"}),
})
|> LLMChain.add_message(Message.new_user!("Hello!"))
|> LLMChain.run()
Bumblebee hosted chat models are supported. There is built-in support for Llama 2, Mistral, and Zephyr models.
Currently, function calling is only supported for llama 3.1 Json Tool calling for Llama 2, Mistral, and Zephyr is NOT supported. There is an example notebook in the notebook folder.
ChatBumblebee.new!(%{
serving: @serving_name,
template_format: @template_format,
receive_timeout: @receive_timeout,
stream: true
})
The serving
is the module name of the Nx.Serving
that is hosting the model.
See the LangChain.ChatModels.ChatBumblebee
documentation for more details.
To run all the tests including the ones that perform live calls against the OpenAI API, use the following command:
mix test --include live_call
mix test --include live_open_ai
mix test --include live_ollama_ai
mix test --include live_anthropic
mix test --include live_mistral_ai
mix test test/tools/calculator_test.exs --include live_call
NOTE: This will use the configured API credentials which creates billable events.
Otherwise, running the following will only run local tests making no external API calls:
mix test
Executing a specific test, whether it is a live_call
or not, will execute it creating a potentially billable event.
When doing local development on the LangChain
library itself, rename the .envrc_template
to .envrc
and populate it with your private API values. This is only used when running live test when explicitly requested.
Use a tool like Direnv or Dotenv to load the API values into the ENV when using the library locally.
For Tasks:
Click tags to check more tools for each tasksFor Jobs:
Alternative AI tools for langchain
Similar Open Source Tools
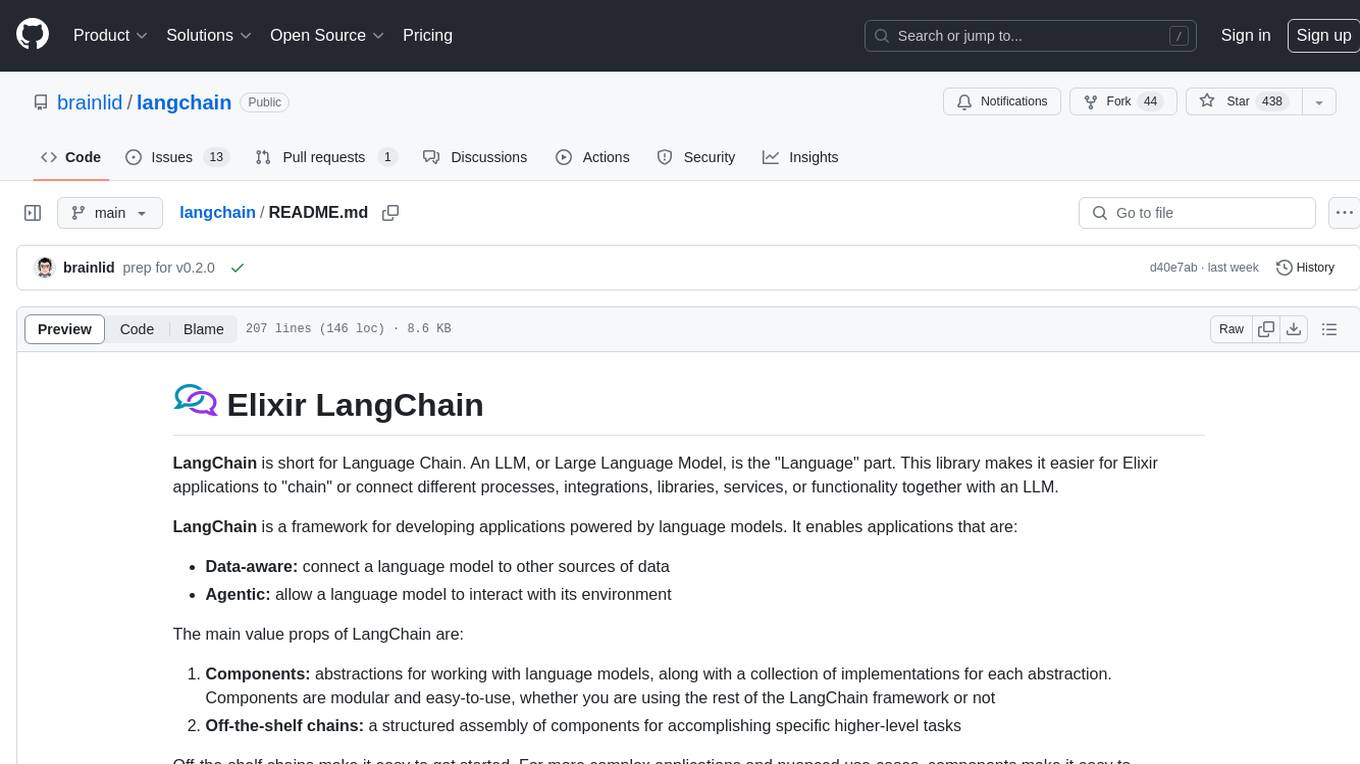
langchain
LangChain is a framework for developing Elixir applications powered by language models. It enables applications to connect language models to other data sources and interact with the environment. The library provides components for working with language models and off-the-shelf chains for specific tasks. It aims to assist in building applications that combine large language models with other sources of computation or knowledge. LangChain is written in Elixir and is not aimed for parity with the JavaScript and Python versions due to differences in programming paradigms and design choices. The library is designed to make it easy to integrate language models into applications and expose features, data, and functionality to the models.
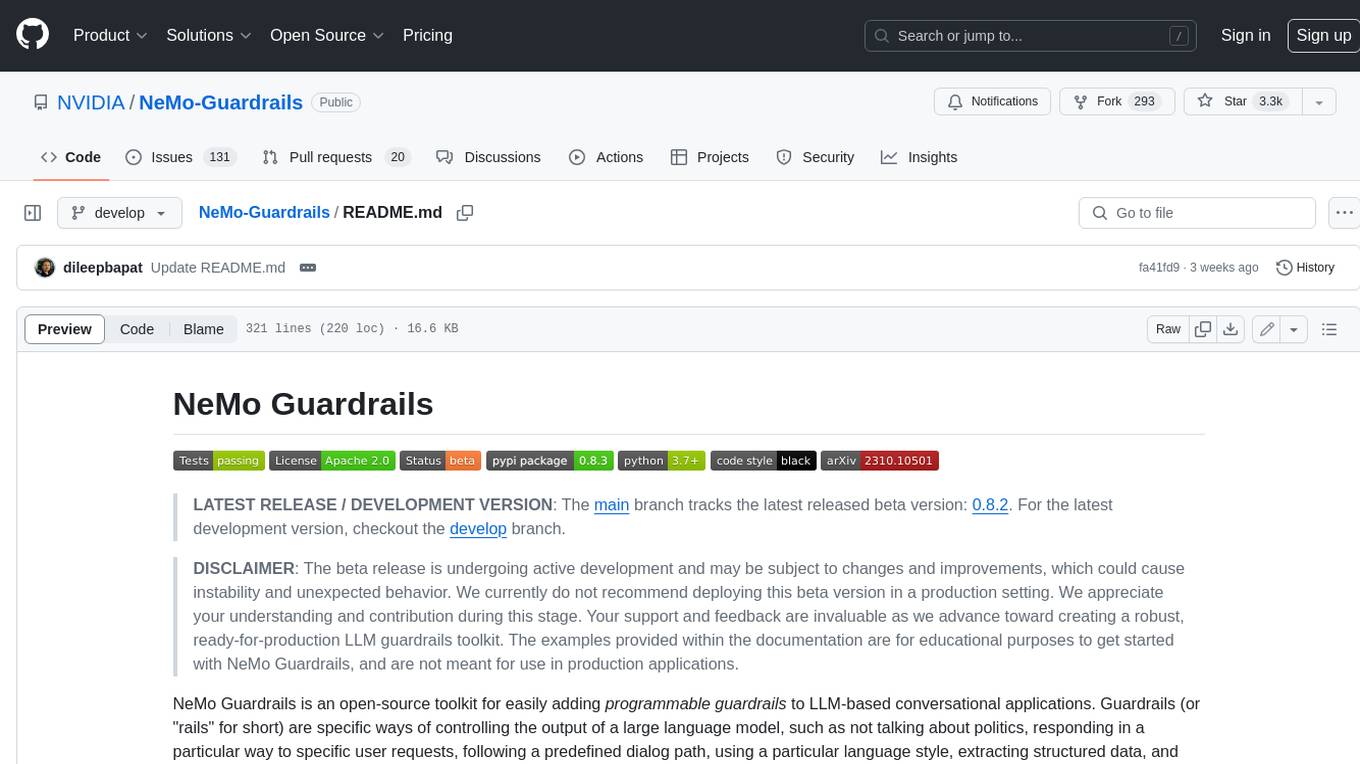
NeMo-Guardrails
NeMo Guardrails is an open-source toolkit for easily adding _programmable guardrails_ to LLM-based conversational applications. Guardrails (or "rails" for short) are specific ways of controlling the output of a large language model, such as not talking about politics, responding in a particular way to specific user requests, following a predefined dialog path, using a particular language style, extracting structured data, and more.
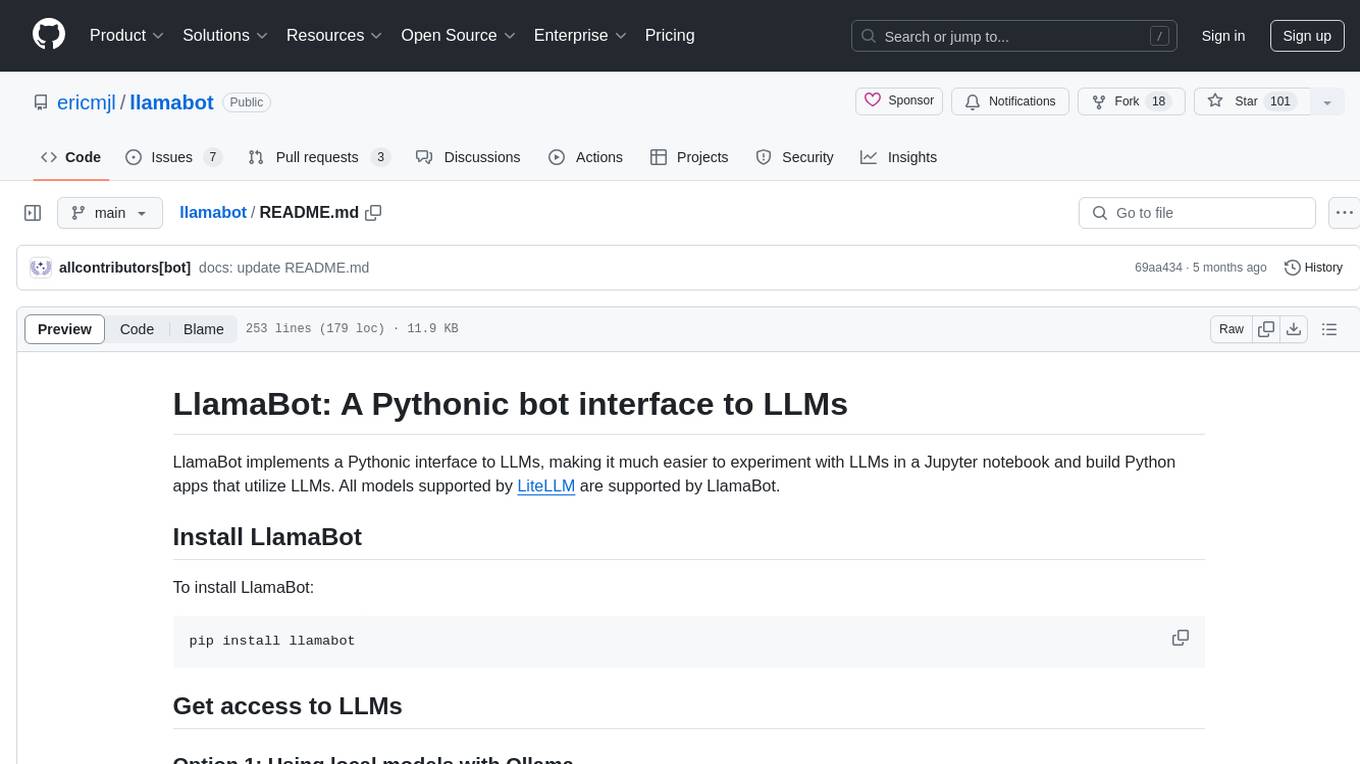
llamabot
LlamaBot is a Pythonic bot interface to Large Language Models (LLMs), providing an easy way to experiment with LLMs in Jupyter notebooks and build Python apps utilizing LLMs. It supports all models available in LiteLLM. Users can access LLMs either through local models with Ollama or by using API providers like OpenAI and Mistral. LlamaBot offers different bot interfaces like SimpleBot, ChatBot, QueryBot, and ImageBot for various tasks such as rephrasing text, maintaining chat history, querying documents, and generating images. The tool also includes CLI demos showcasing its capabilities and supports contributions for new features and bug reports from the community.
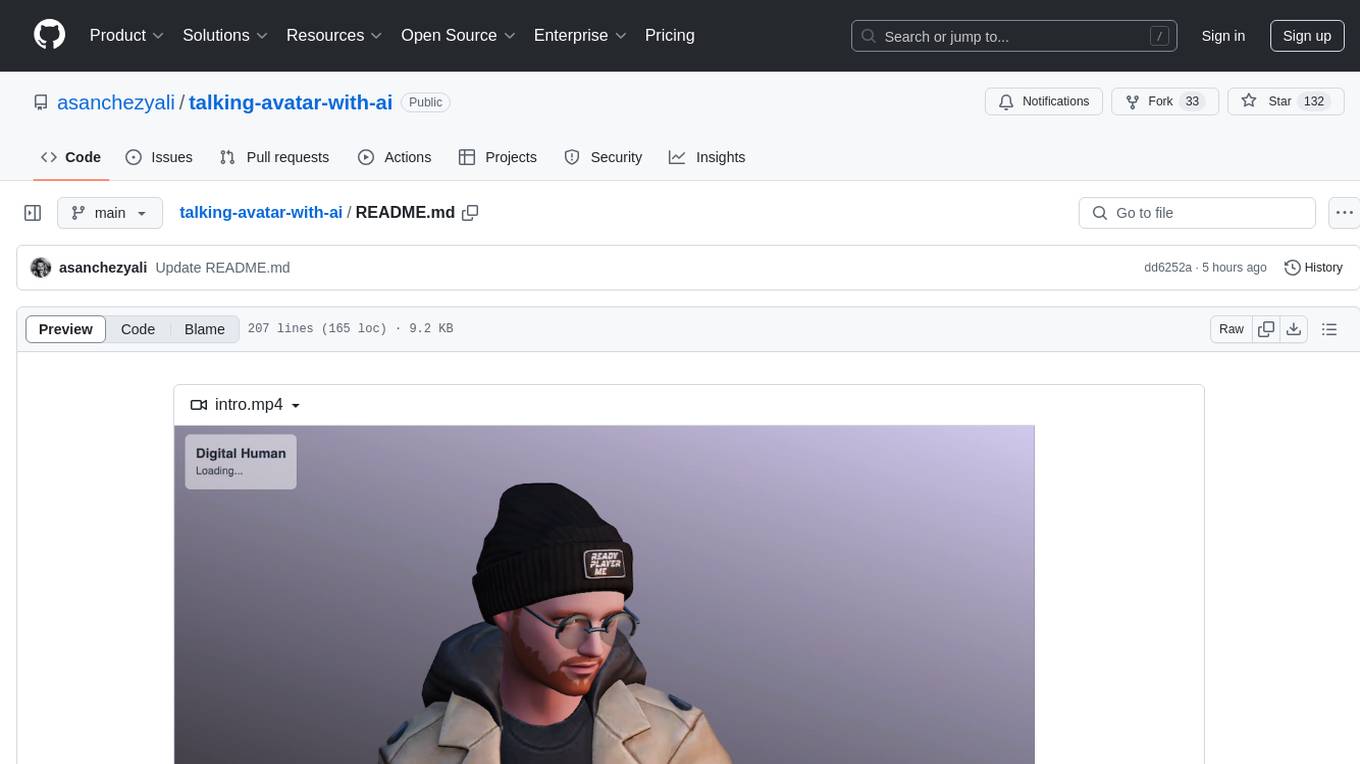
talking-avatar-with-ai
The 'talking-avatar-with-ai' project is a digital human system that utilizes OpenAI's GPT-3 for generating responses, Whisper for audio transcription, Eleven Labs for voice generation, and Rhubarb Lip Sync for lip synchronization. The system allows users to interact with a digital avatar that responds with text, facial expressions, and animations, creating a realistic conversational experience. The project includes setup for environment variables, chat prompt templates, chat model configuration, and structured output parsing to enhance the interaction with the digital human.
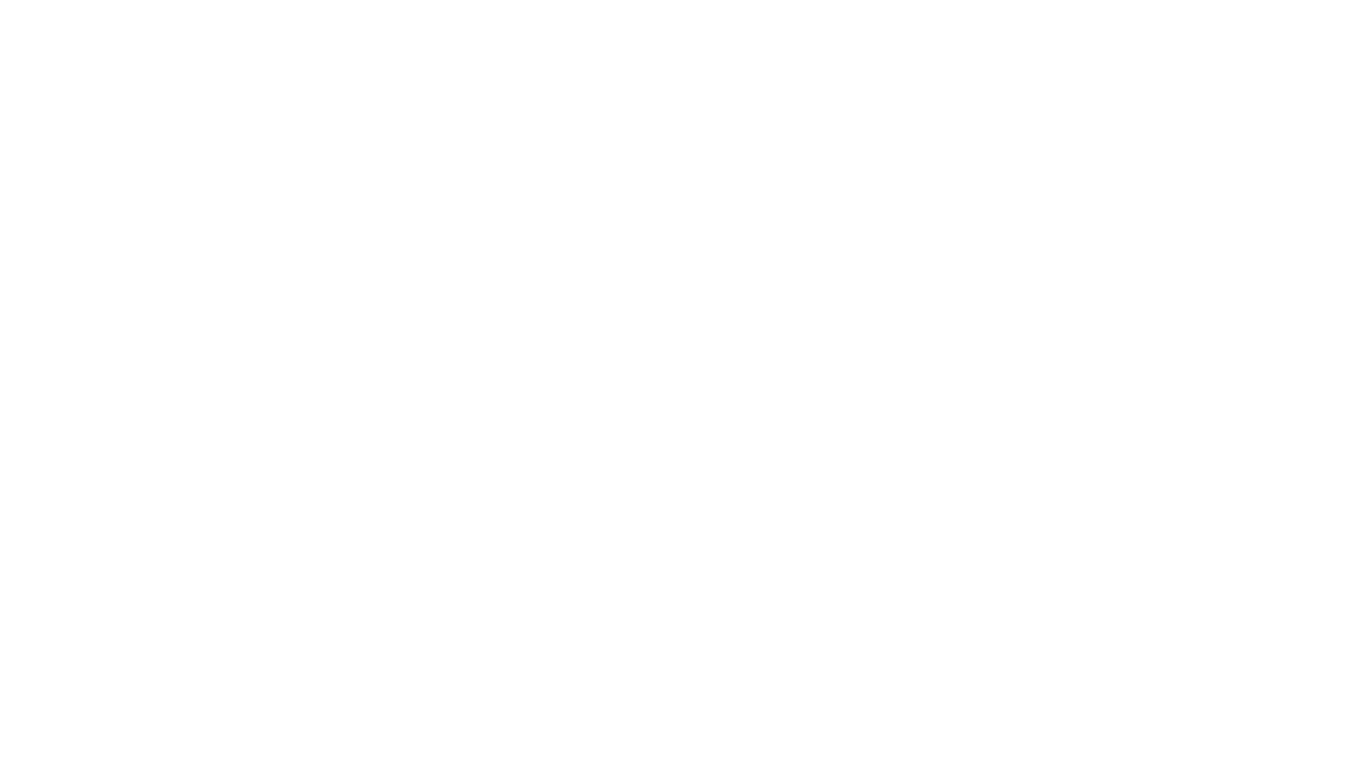
rag-experiment-accelerator
The RAG Experiment Accelerator is a versatile tool that helps you conduct experiments and evaluations using Azure AI Search and RAG pattern. It offers a rich set of features, including experiment setup, integration with Azure AI Search, Azure Machine Learning, MLFlow, and Azure OpenAI, multiple document chunking strategies, query generation, multiple search types, sub-querying, re-ranking, metrics and evaluation, report generation, and multi-lingual support. The tool is designed to make it easier and faster to run experiments and evaluations of search queries and quality of response from OpenAI, and is useful for researchers, data scientists, and developers who want to test the performance of different search and OpenAI related hyperparameters, compare the effectiveness of various search strategies, fine-tune and optimize parameters, find the best combination of hyperparameters, and generate detailed reports and visualizations from experiment results.
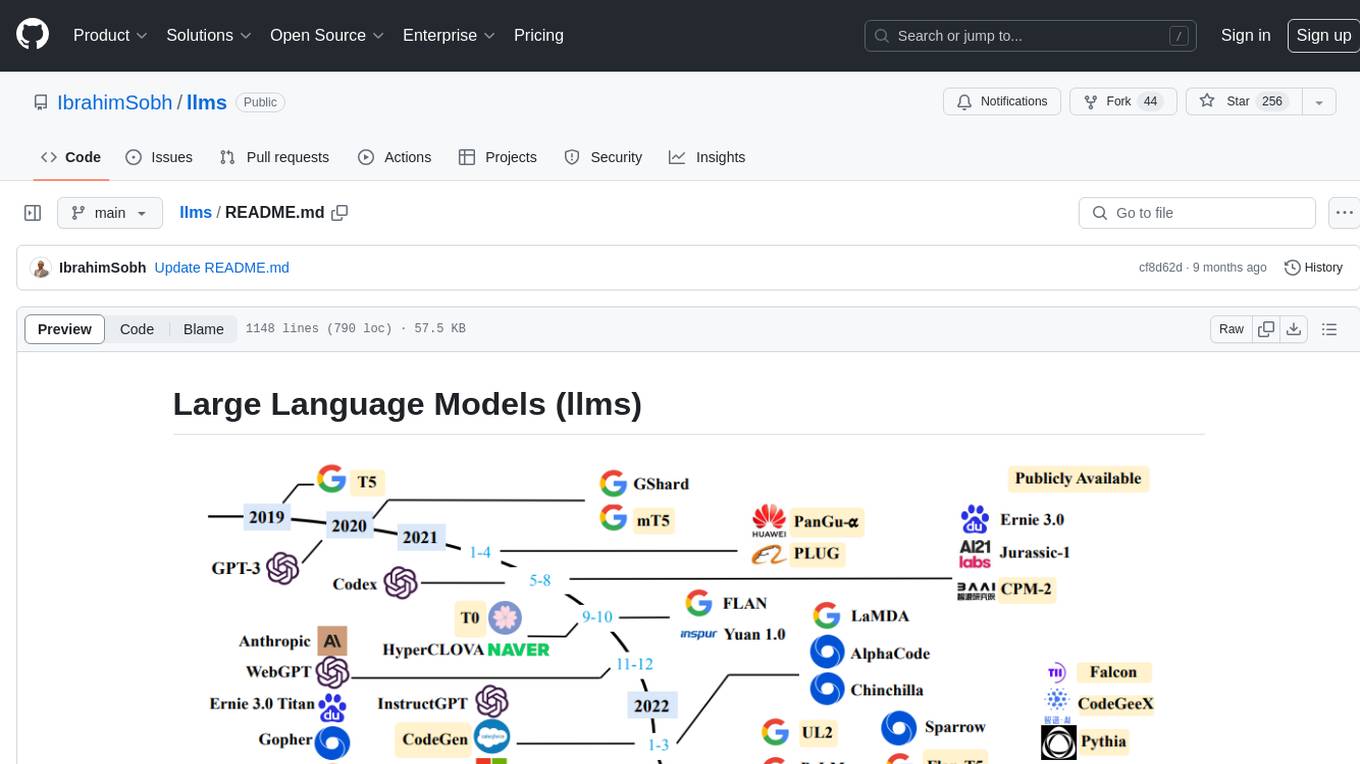
llms
The 'llms' repository is a comprehensive guide on Large Language Models (LLMs), covering topics such as language modeling, applications of LLMs, statistical language modeling, neural language models, conditional language models, evaluation methods, transformer-based language models, practical LLMs like GPT and BERT, prompt engineering, fine-tuning LLMs, retrieval augmented generation, AI agents, and LLMs for computer vision. The repository provides detailed explanations, examples, and tools for working with LLMs.
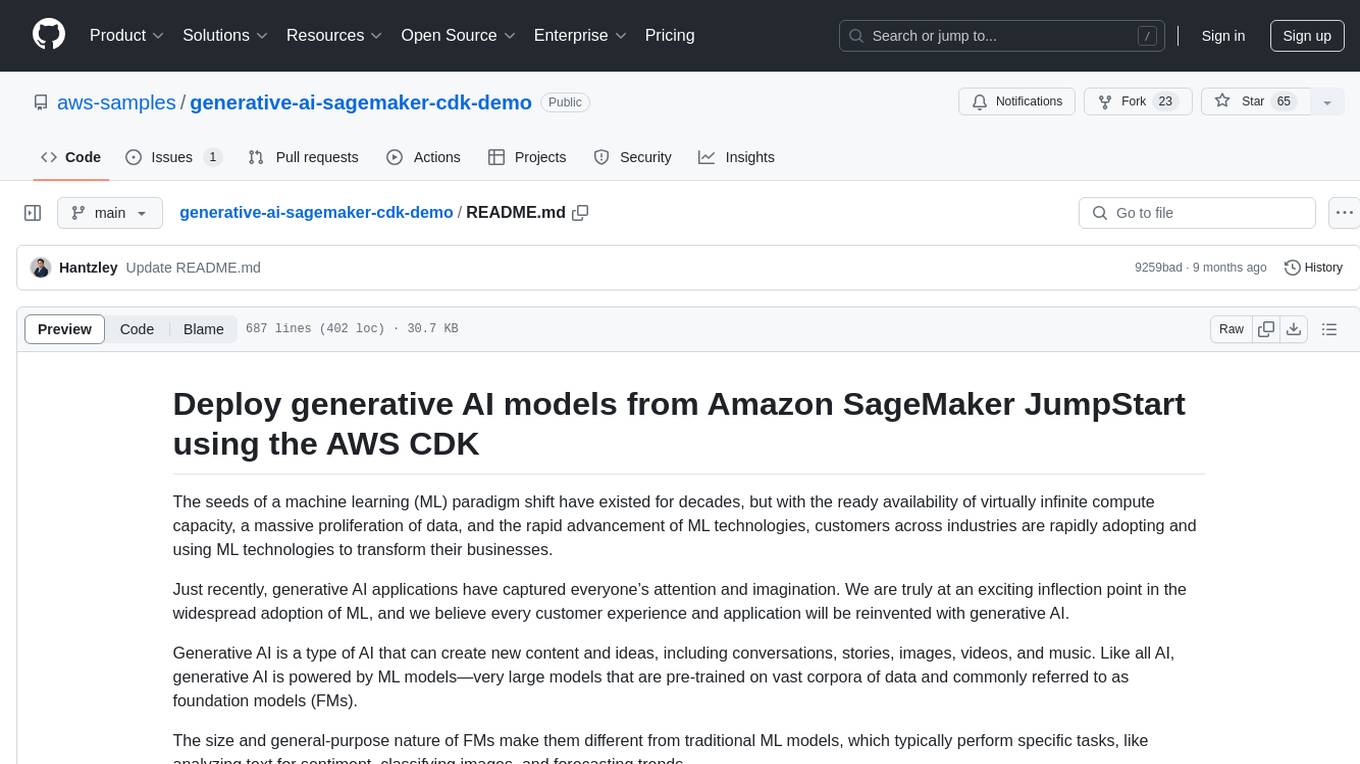
generative-ai-sagemaker-cdk-demo
This repository showcases how to deploy generative AI models from Amazon SageMaker JumpStart using the AWS CDK. Generative AI is a type of AI that can create new content and ideas, such as conversations, stories, images, videos, and music. The repository provides a detailed guide on deploying image and text generative AI models, utilizing pre-trained models from SageMaker JumpStart. The web application is built on Streamlit and hosted on Amazon ECS with Fargate. It interacts with the SageMaker model endpoints through Lambda functions and Amazon API Gateway. The repository also includes instructions on setting up the AWS CDK application, deploying the stacks, using the models, and viewing the deployed resources on the AWS Management Console.
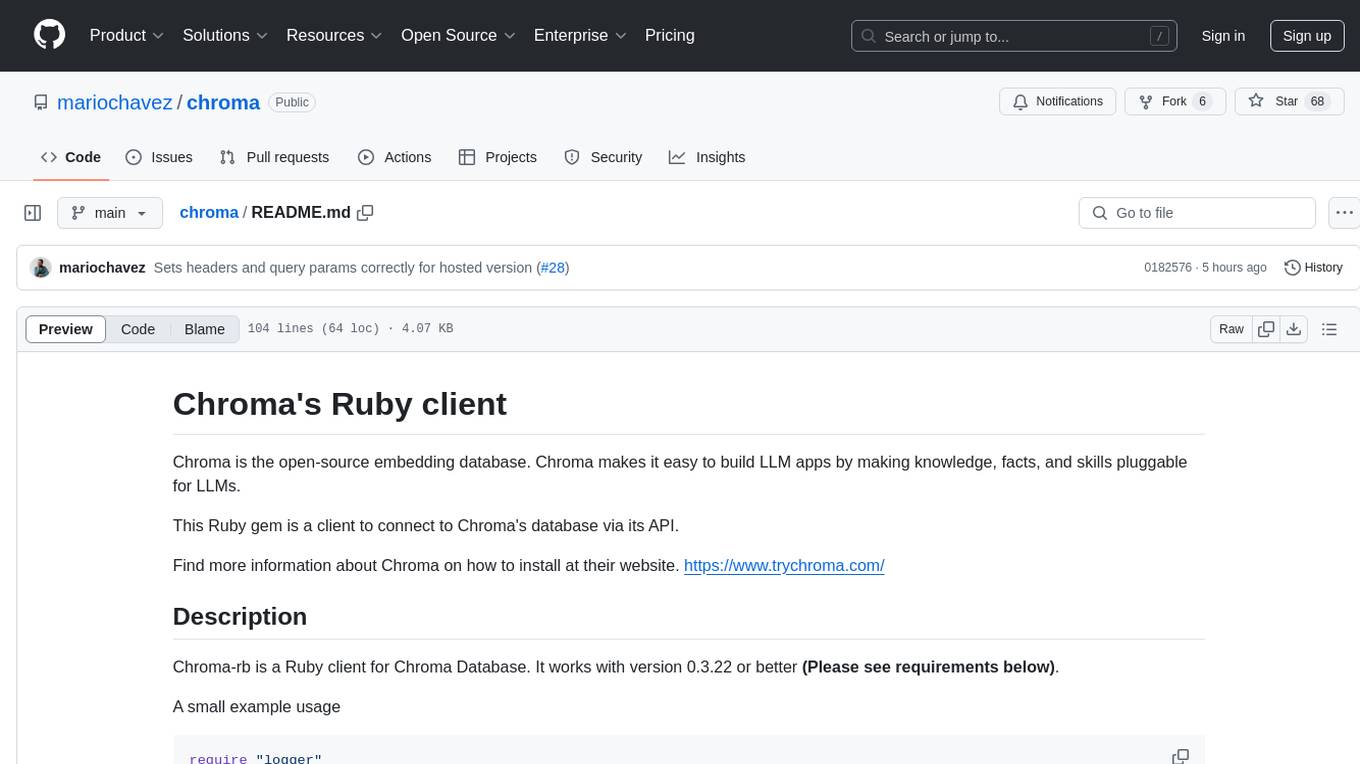
chroma
Chroma is an open-source embedding database that simplifies building LLM apps by enabling the integration of knowledge, facts, and skills for LLMs. The Ruby client for Chroma Database, chroma-rb, facilitates connecting to Chroma's database via its API. Users can configure the host, check server version, create collections, and add embeddings. The gem supports Chroma Database version 0.3.22 or newer, requiring Ruby 3.1.4 or later. It can be used with the hosted Chroma service at trychroma.com by setting configuration options like api_key, tenant, and database. Additionally, the gem provides integration with Jupyter Notebook for creating embeddings using Ollama and Nomic embed text with a Ruby HTTP client.
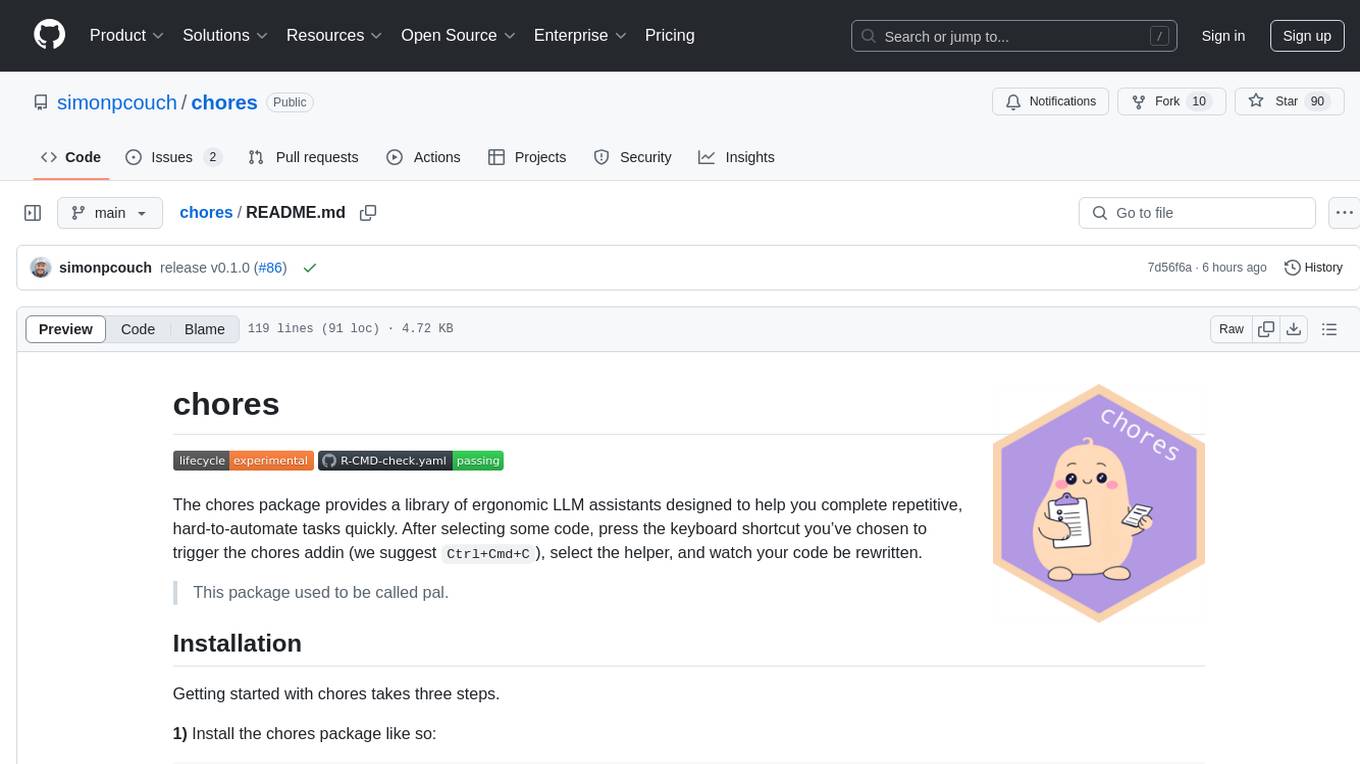
chores
The chores package provides a library of ergonomic LLM assistants designed to help users complete repetitive, hard-to-automate tasks quickly. Users can select code, trigger the chores addin, choose a helper, and watch their code be rewritten. The package offers chore helpers for tasks like converting to cli, testthat, and documenting functions with roxygen. Users can also create their own chore helpers by providing instructions in a markdown file. The cost of using helpers depends on the length of the prompt and the model chosen.
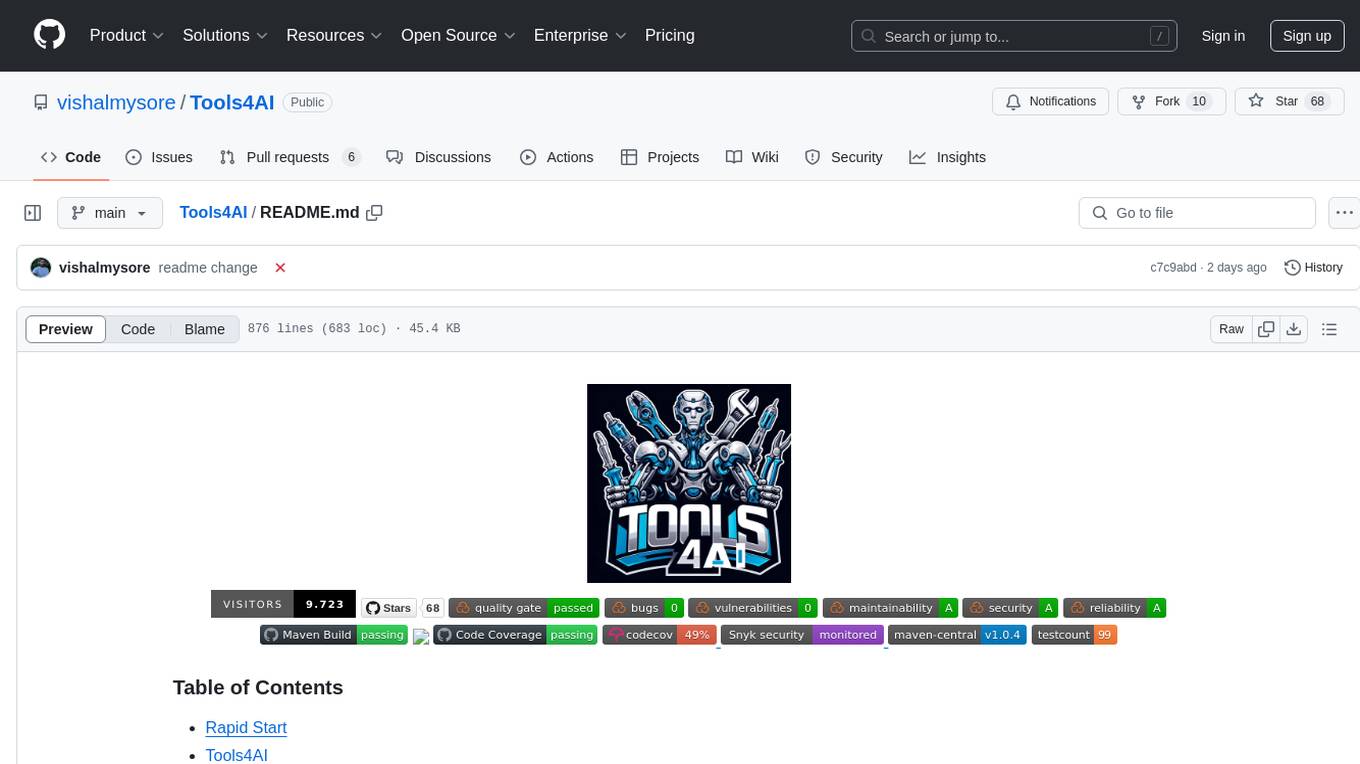
Tools4AI
Tools4AI is a Java-based Agentic Framework for building AI agents to integrate with enterprise Java applications. It enables the conversion of natural language prompts into actionable behaviors, streamlining user interactions with complex systems. By leveraging AI capabilities, it enhances productivity and innovation across diverse applications. The framework allows for seamless integration of AI with various systems, such as customer service applications, to interpret user requests, trigger actions, and streamline workflows. Prompt prediction anticipates user actions based on input prompts, enhancing user experience by proactively suggesting relevant actions or services based on context.
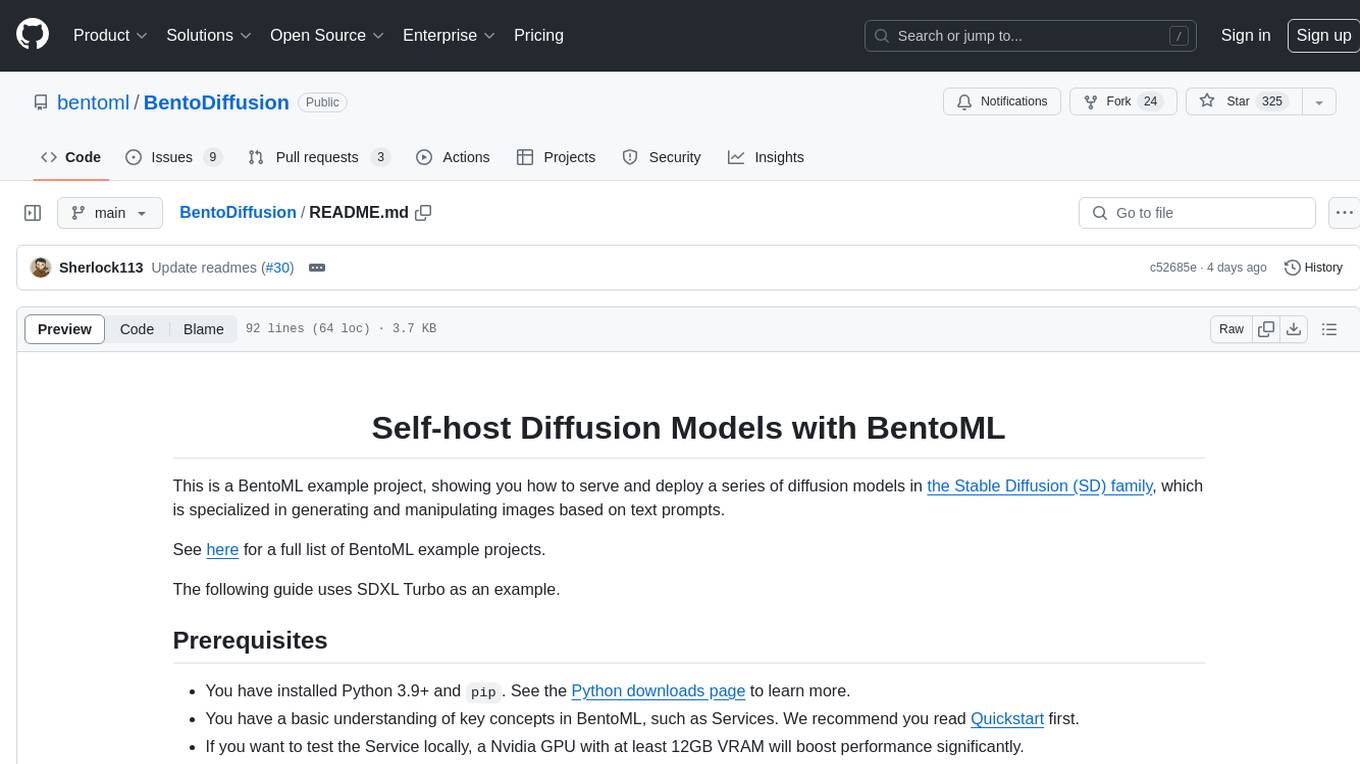
BentoDiffusion
BentoDiffusion is a BentoML example project that demonstrates how to serve and deploy diffusion models in the Stable Diffusion (SD) family. These models are specialized in generating and manipulating images based on text prompts. The project provides a guide on using SDXL Turbo as an example, along with instructions on prerequisites, installing dependencies, running the BentoML service, and deploying to BentoCloud. Users can interact with the deployed service using Swagger UI or other methods. Additionally, the project offers the option to choose from various diffusion models available in the repository for deployment.
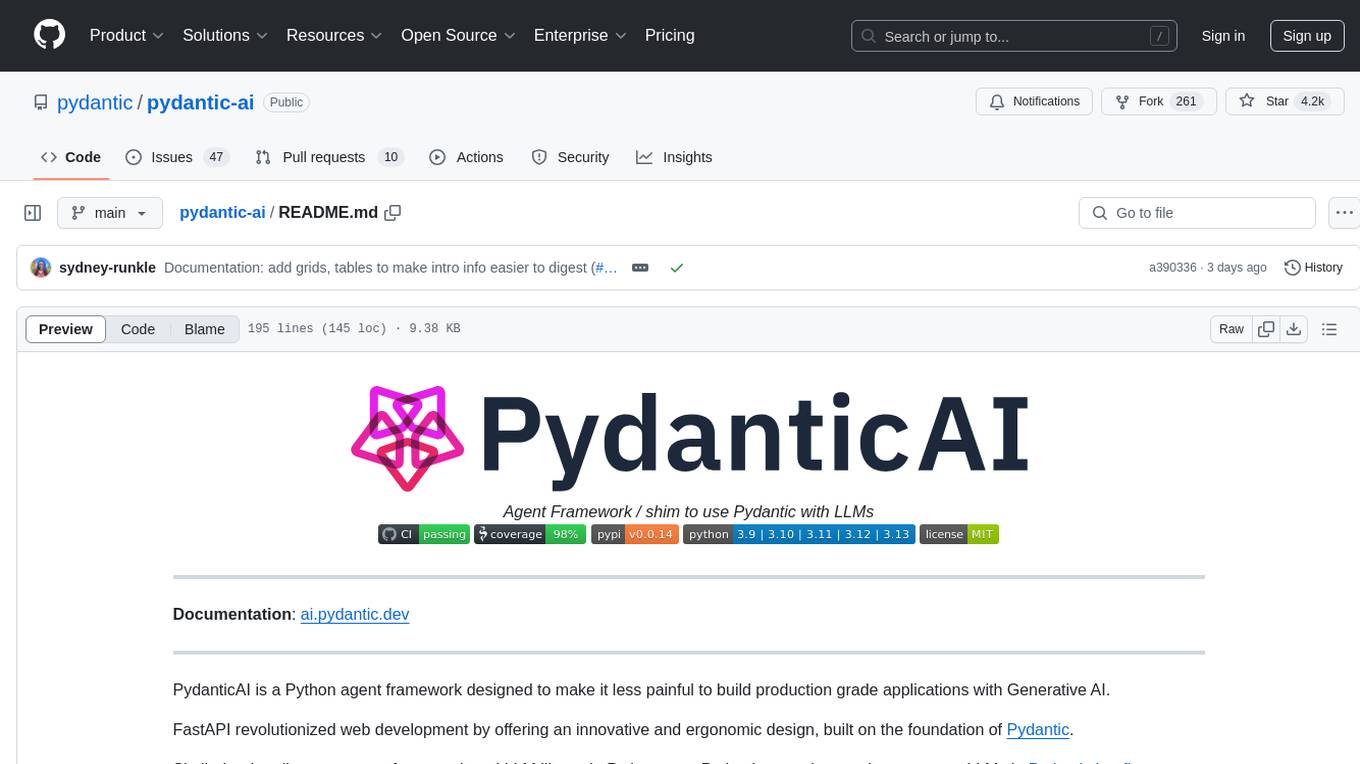
pydantic-ai
PydanticAI is a Python agent framework designed to make it less painful to build production grade applications with Generative AI. It is built by the Pydantic Team and supports various AI models like OpenAI, Anthropic, Gemini, Ollama, Groq, and Mistral. PydanticAI seamlessly integrates with Pydantic Logfire for real-time debugging, performance monitoring, and behavior tracking of LLM-powered applications. It is type-safe, Python-centric, and offers structured responses, dependency injection system, and streamed responses. PydanticAI is in early beta, offering a Python-centric design to apply standard Python best practices in AI-driven projects.
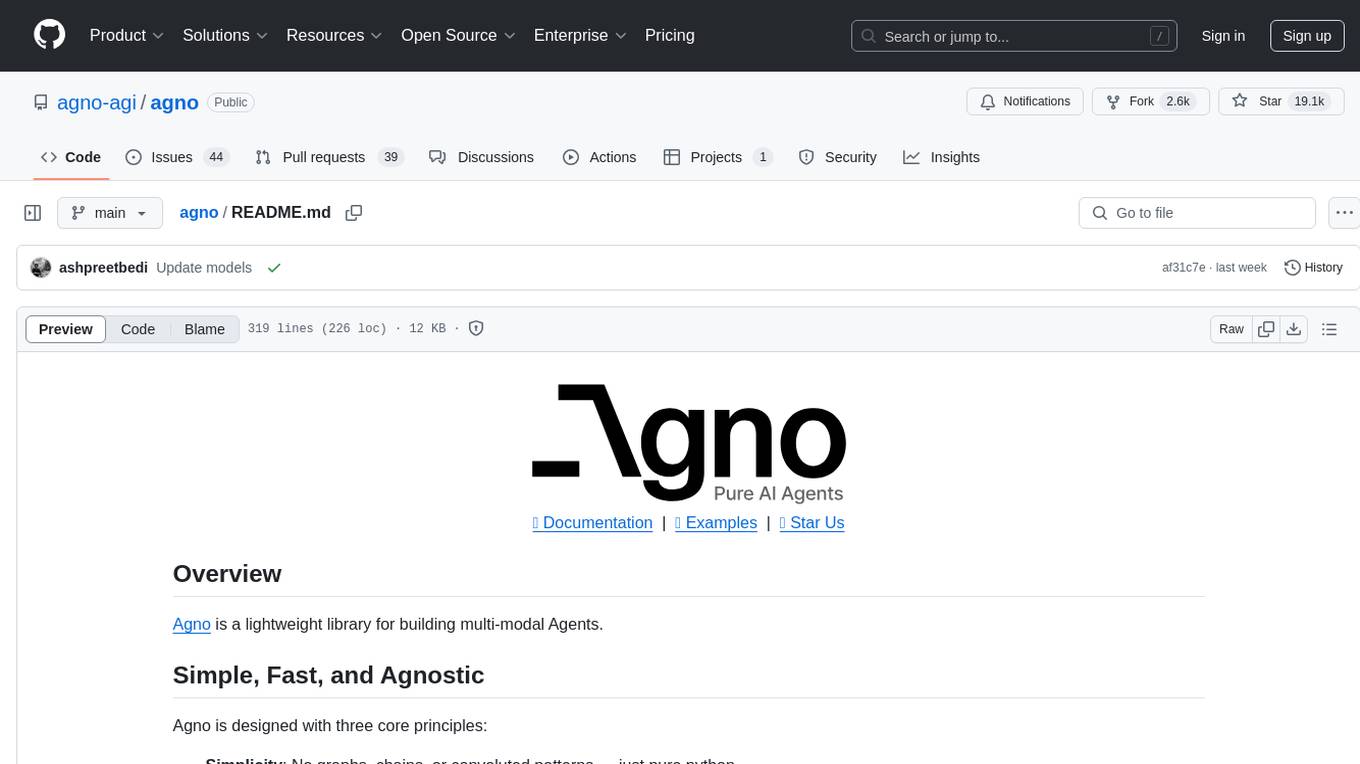
agno
Agno is a lightweight library for building multi-modal Agents. It is designed with core principles of simplicity, uncompromising performance, and agnosticism, allowing users to create blazing fast agents with minimal memory footprint. Agno supports any model, any provider, and any modality, making it a versatile container for AGI. Users can build agents with lightning-fast agent creation, model agnostic capabilities, native support for text, image, audio, and video inputs and outputs, memory management, knowledge stores, structured outputs, and real-time monitoring. The library enables users to create autonomous programs that use language models to solve problems, improve responses, and achieve tasks with varying levels of agency and autonomy.
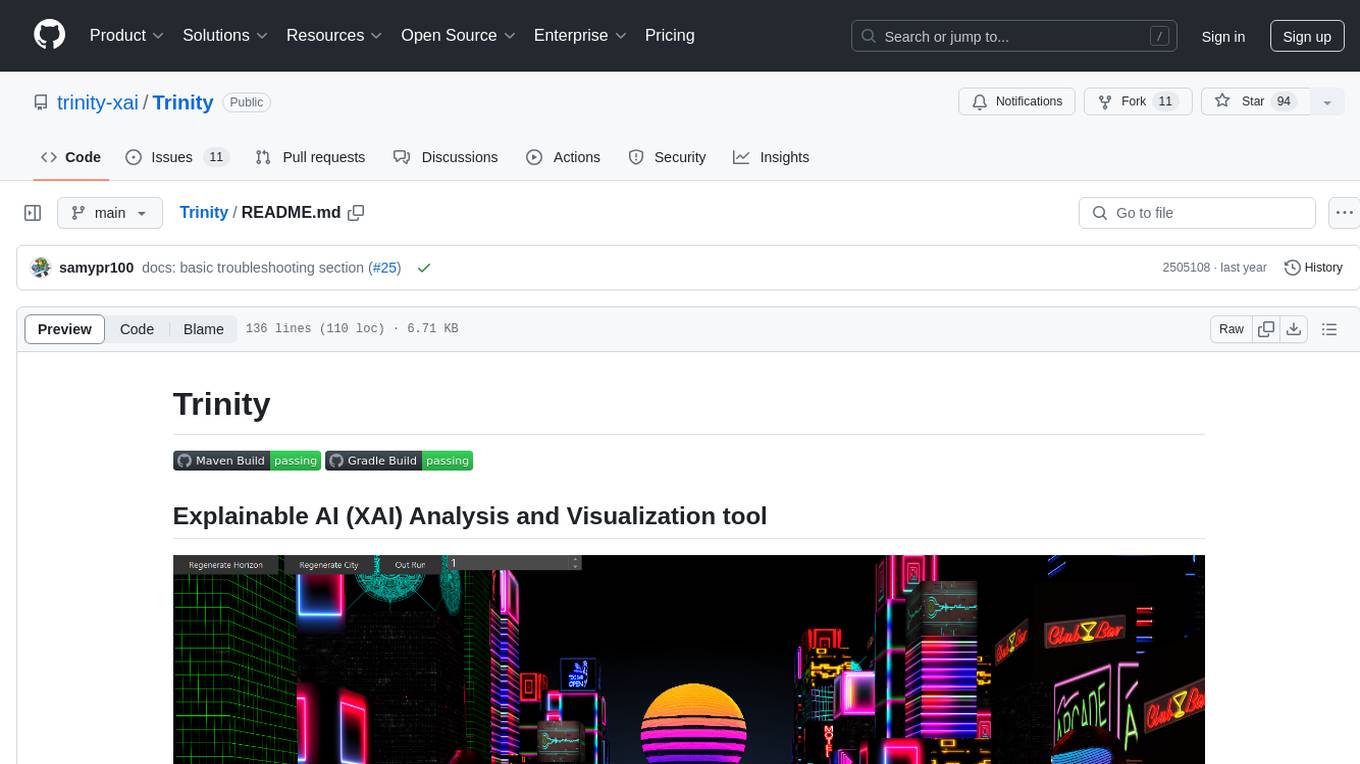
Trinity
Trinity is an Explainable AI (XAI) Analysis and Visualization tool designed for Deep Learning systems or other models performing complex classification or decoding. It provides performance analysis through interactive 3D projections that are hyper-dimensional aware, allowing users to explore hyperspace, hypersurface, projections, and manifolds. Trinity primarily works with JSON data formats and supports the visualization of FeatureVector objects. Users can analyze and visualize data points, correlate inputs with classification results, and create custom color maps for better data interpretation. Trinity has been successfully applied to various use cases including Deep Learning Object detection models, COVID gene/tissue classification, Brain Computer Interface decoders, and Large Language Model (ChatGPT) Embeddings Analysis.
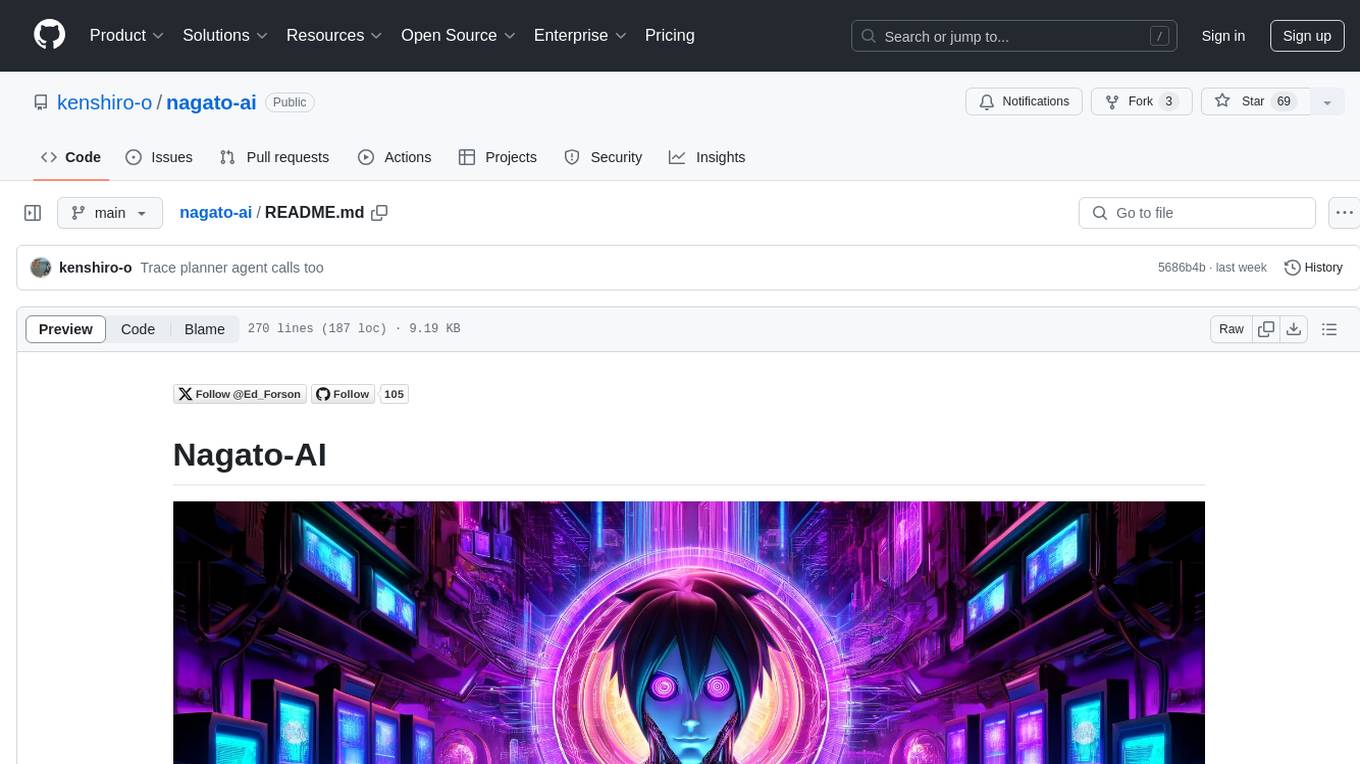
nagato-ai
Nagato-AI is an intuitive AI Agent library that supports multiple LLMs including OpenAI's GPT, Anthropic's Claude, Google's Gemini, and Groq LLMs. Users can create agents from these models and combine them to build an effective AI Agent system. The library is named after the powerful ninja Nagato from the anime Naruto, who can control multiple bodies with different abilities. Nagato-AI acts as a linchpin to summon and coordinate AI Agents for specific missions. It provides flexibility in programming and supports tools like Coordinator, Researcher, Critic agents, and HumanConfirmInputTool.
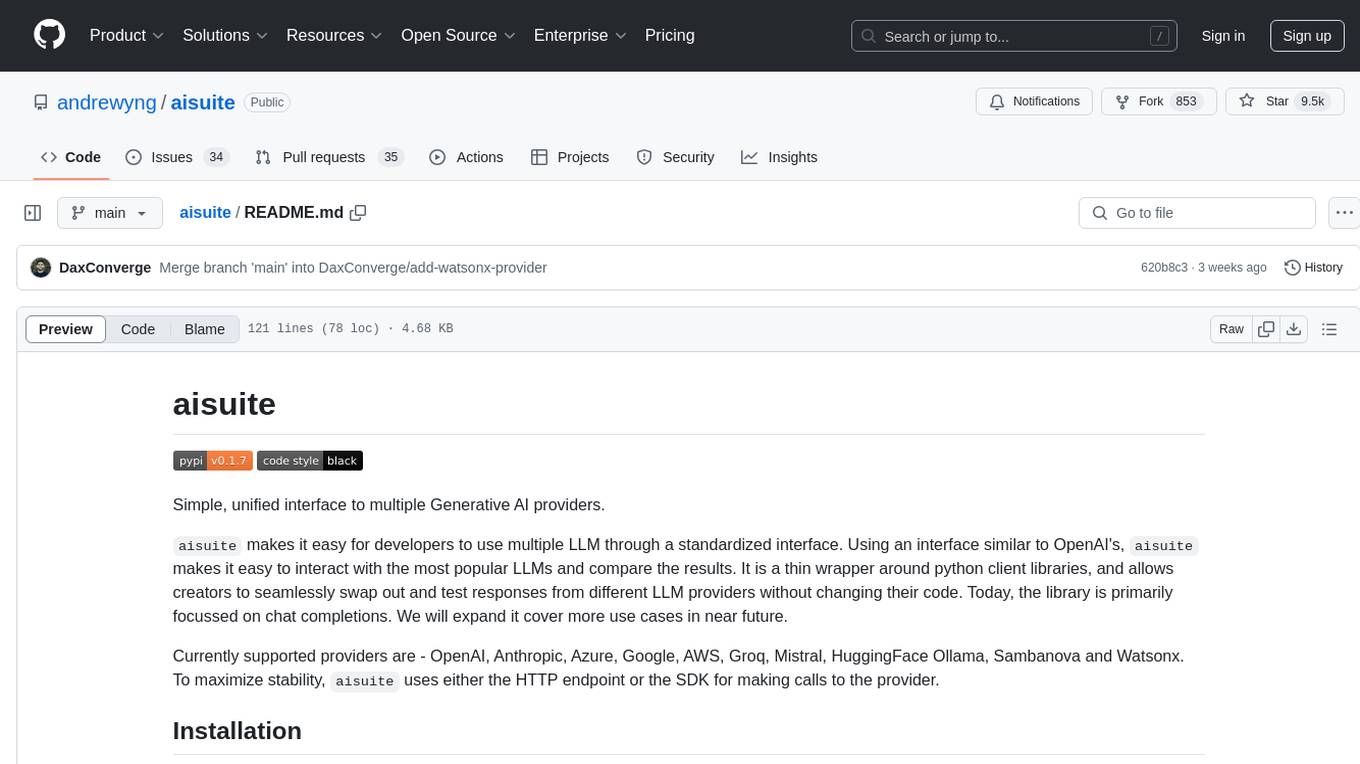
aisuite
Aisuite is a simple, unified interface to multiple Generative AI providers. It allows developers to easily interact with various Language Model (LLM) providers like OpenAI, Anthropic, Azure, Google, AWS, and more through a standardized interface. The library focuses on chat completions and provides a thin wrapper around python client libraries, enabling creators to test responses from different LLM providers without changing their code. Aisuite maximizes stability by using HTTP endpoints or SDKs for making calls to the providers. Users can install the base package or specific provider packages, set up API keys, and utilize the library to generate chat completion responses from different models.
For similar tasks
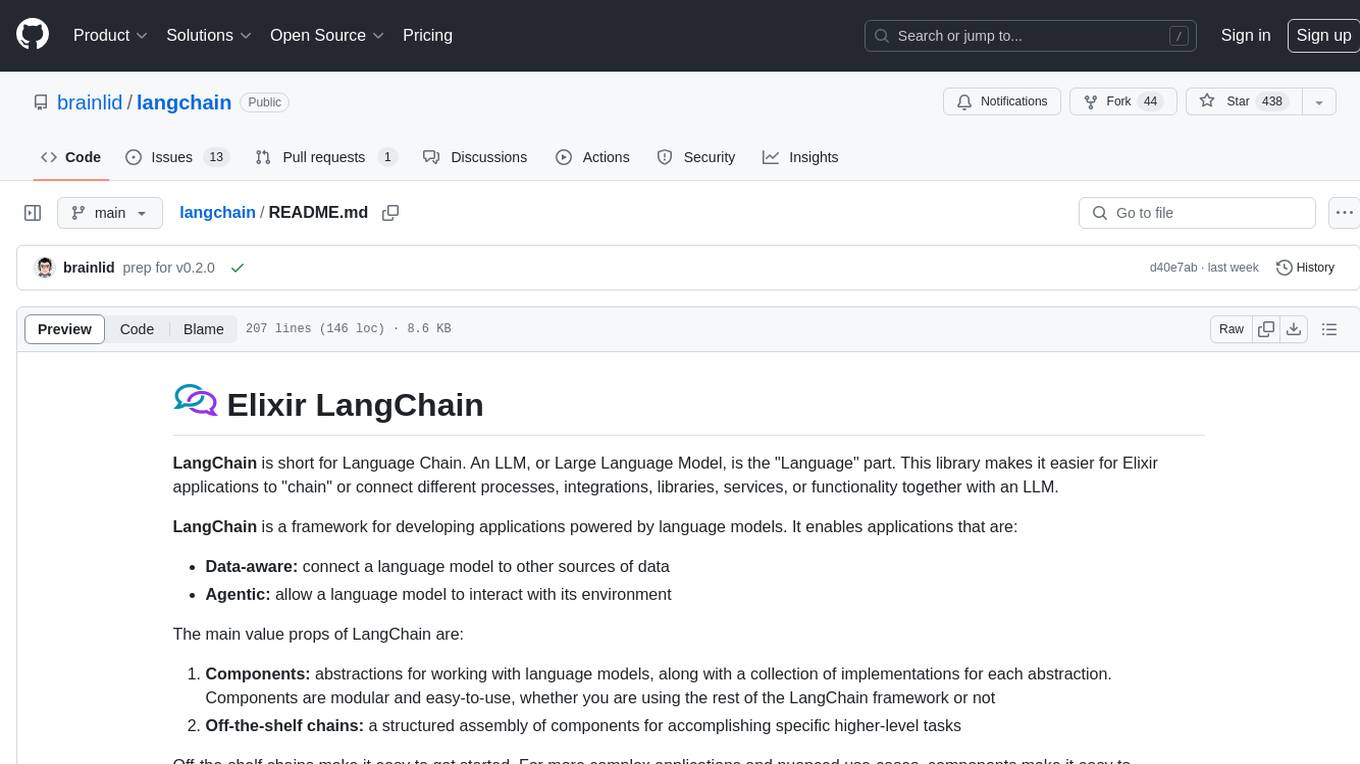
langchain
LangChain is a framework for developing Elixir applications powered by language models. It enables applications to connect language models to other data sources and interact with the environment. The library provides components for working with language models and off-the-shelf chains for specific tasks. It aims to assist in building applications that combine large language models with other sources of computation or knowledge. LangChain is written in Elixir and is not aimed for parity with the JavaScript and Python versions due to differences in programming paradigms and design choices. The library is designed to make it easy to integrate language models into applications and expose features, data, and functionality to the models.
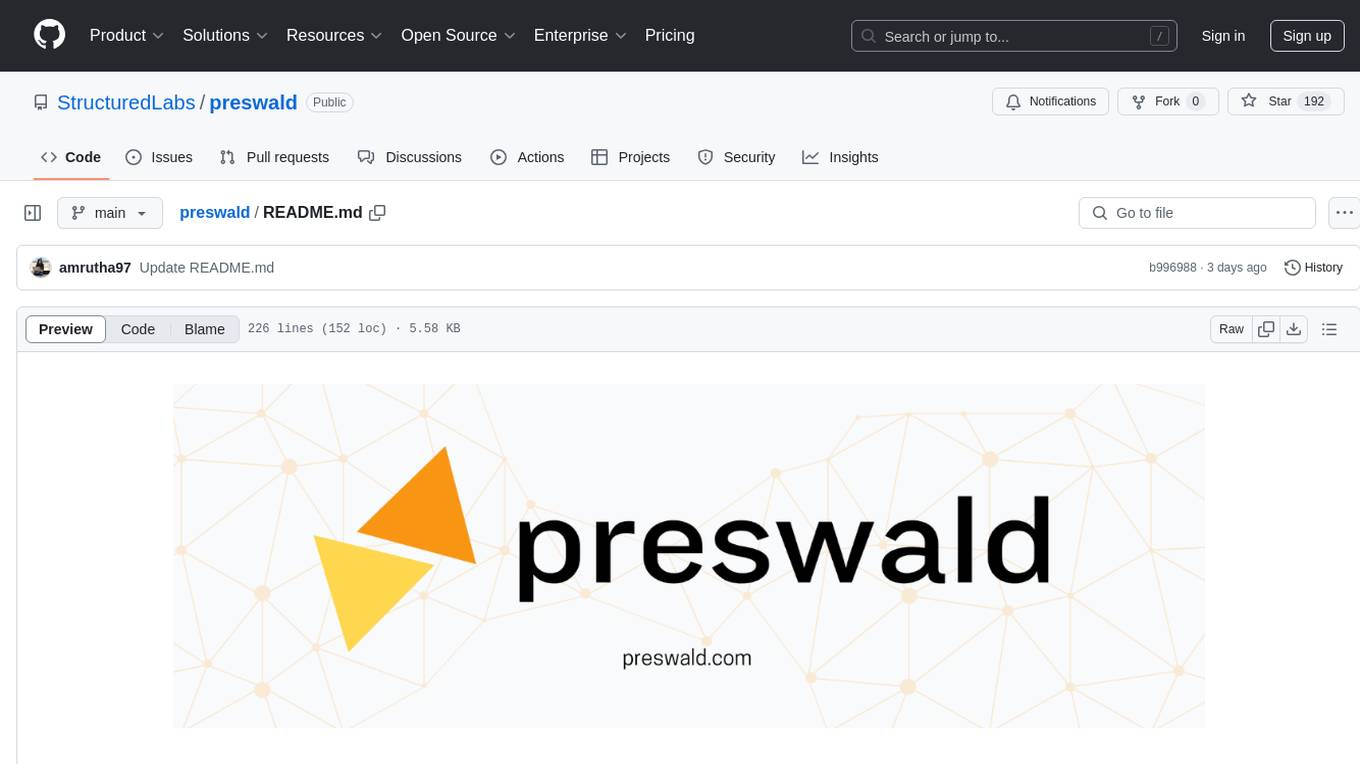
preswald
Preswald is a full-stack platform for building, deploying, and managing interactive data applications in Python. It simplifies the process by combining ingestion, storage, transformation, and visualization into one lightweight SDK. With Preswald, users can connect to various data sources, customize app themes, and easily deploy apps locally. The platform focuses on code-first simplicity, end-to-end coverage, and efficiency by design, making it suitable for prototyping internal tools or deploying production-grade apps with reduced complexity and cost.
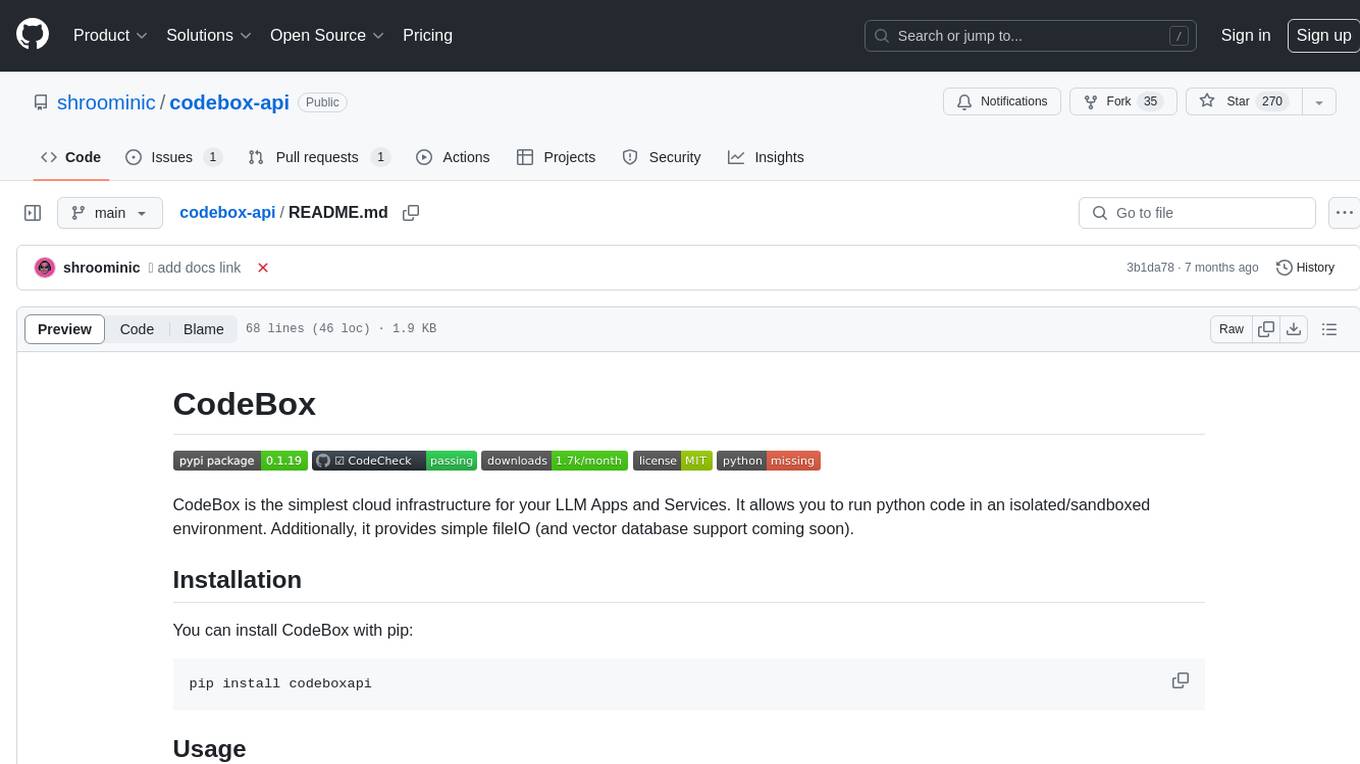
codebox-api
CodeBox is a cloud infrastructure tool designed for running Python code in an isolated environment. It also offers simple file input/output capabilities and will soon support vector database operations. Users can install CodeBox using pip and utilize it by setting up an API key. The tool allows users to execute Python code snippets and interact with the isolated environment. CodeBox is currently in early development stages and requires manual handling for certain operations like refunds and cancellations. The tool is open for contributions through issue reporting and pull requests. It is licensed under MIT and can be contacted via email at [email protected].
For similar jobs
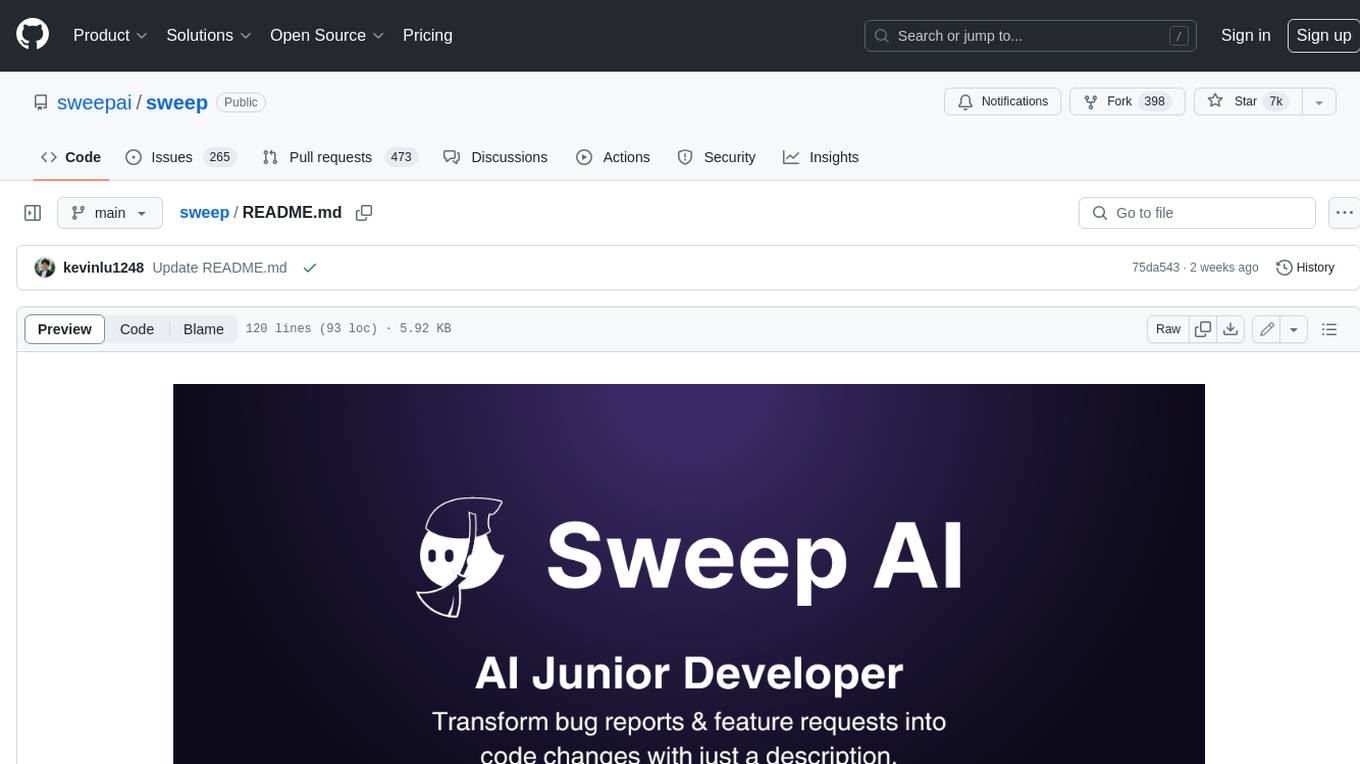
sweep
Sweep is an AI junior developer that turns bugs and feature requests into code changes. It automatically handles developer experience improvements like adding type hints and improving test coverage.
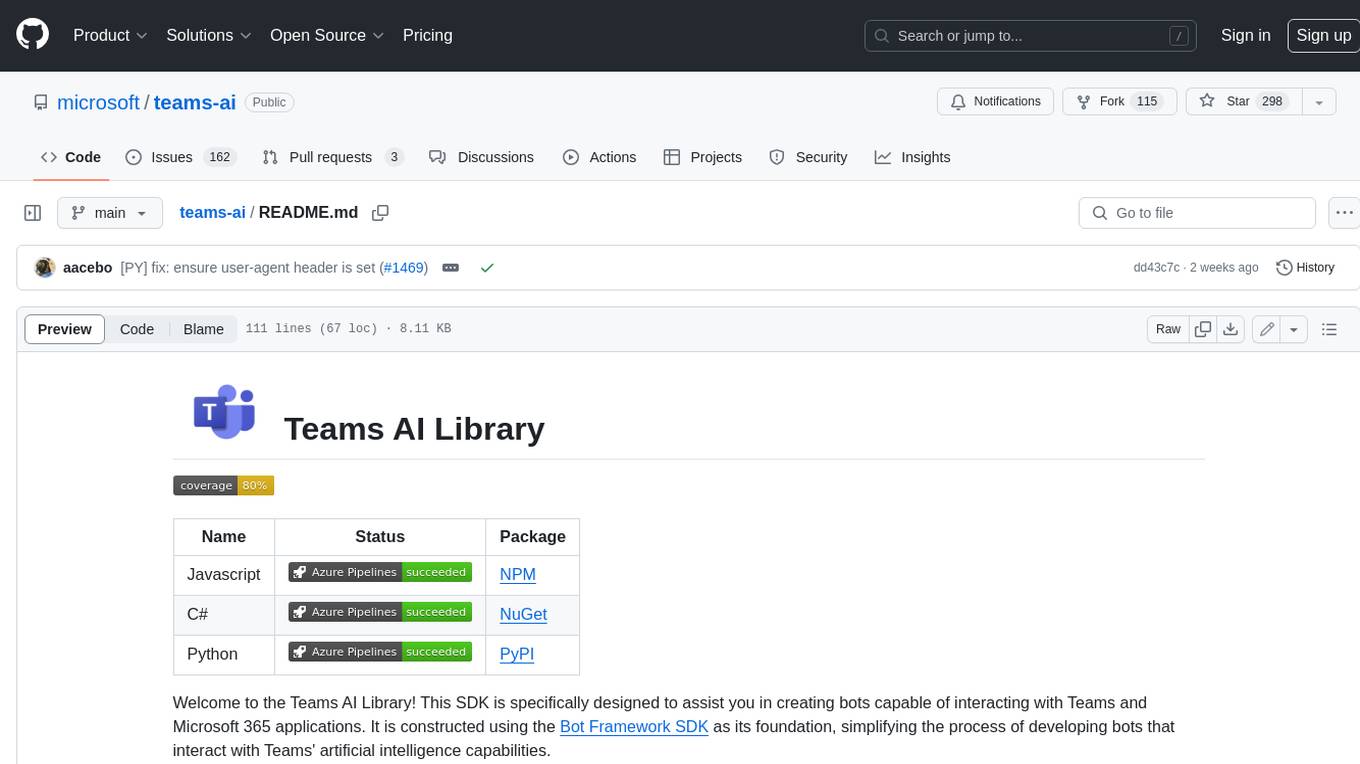
teams-ai
The Teams AI Library is a software development kit (SDK) that helps developers create bots that can interact with Teams and Microsoft 365 applications. It is built on top of the Bot Framework SDK and simplifies the process of developing bots that interact with Teams' artificial intelligence capabilities. The SDK is available for JavaScript/TypeScript, .NET, and Python.
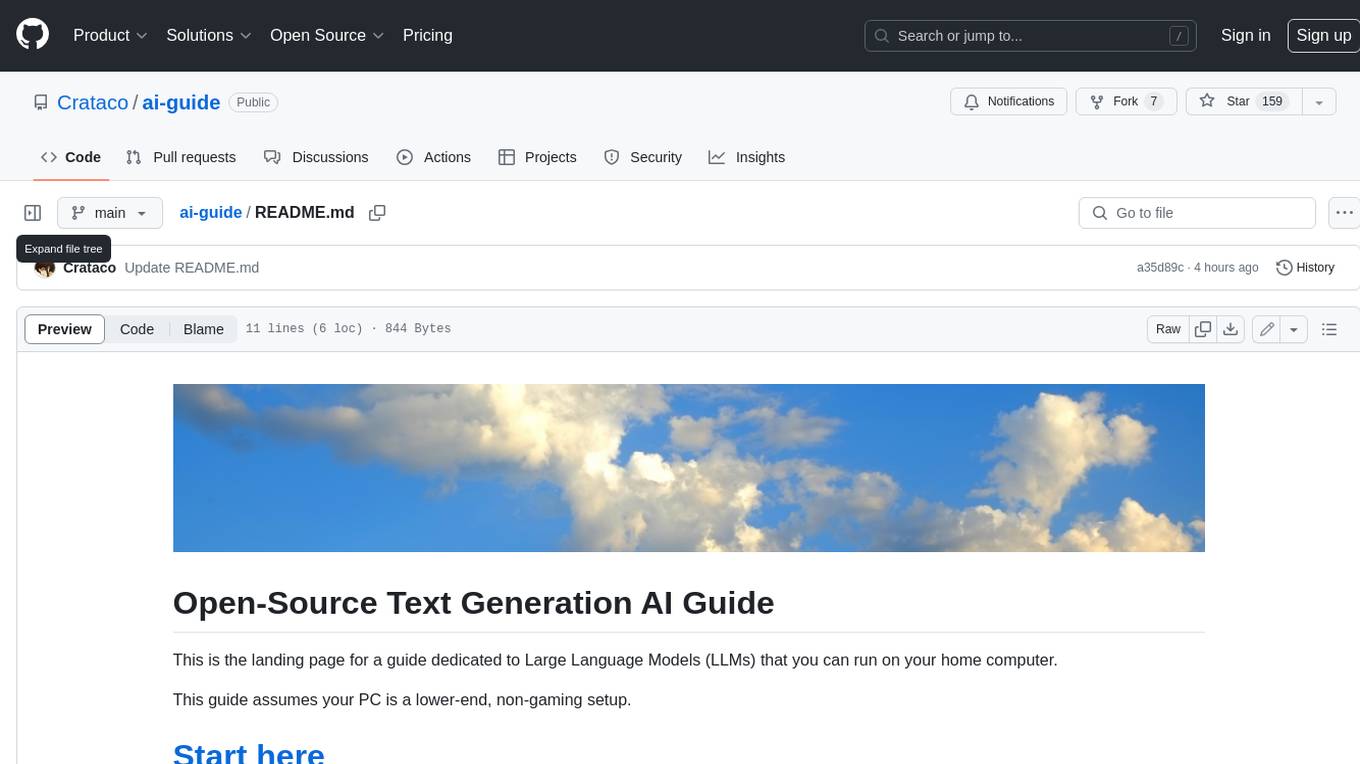
ai-guide
This guide is dedicated to Large Language Models (LLMs) that you can run on your home computer. It assumes your PC is a lower-end, non-gaming setup.
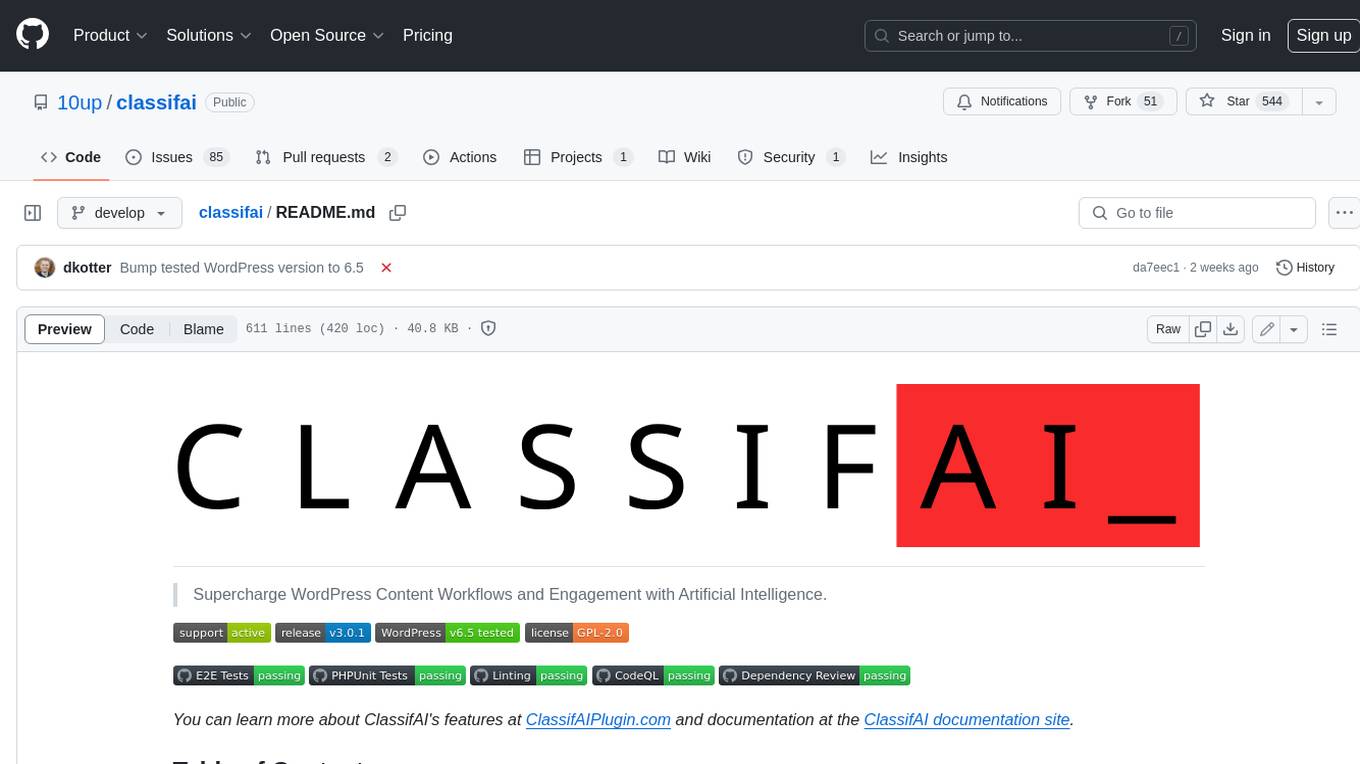
classifai
Supercharge WordPress Content Workflows and Engagement with Artificial Intelligence. Tap into leading cloud-based services like OpenAI, Microsoft Azure AI, Google Gemini and IBM Watson to augment your WordPress-powered websites. Publish content faster while improving SEO performance and increasing audience engagement. ClassifAI integrates Artificial Intelligence and Machine Learning technologies to lighten your workload and eliminate tedious tasks, giving you more time to create original content that matters.
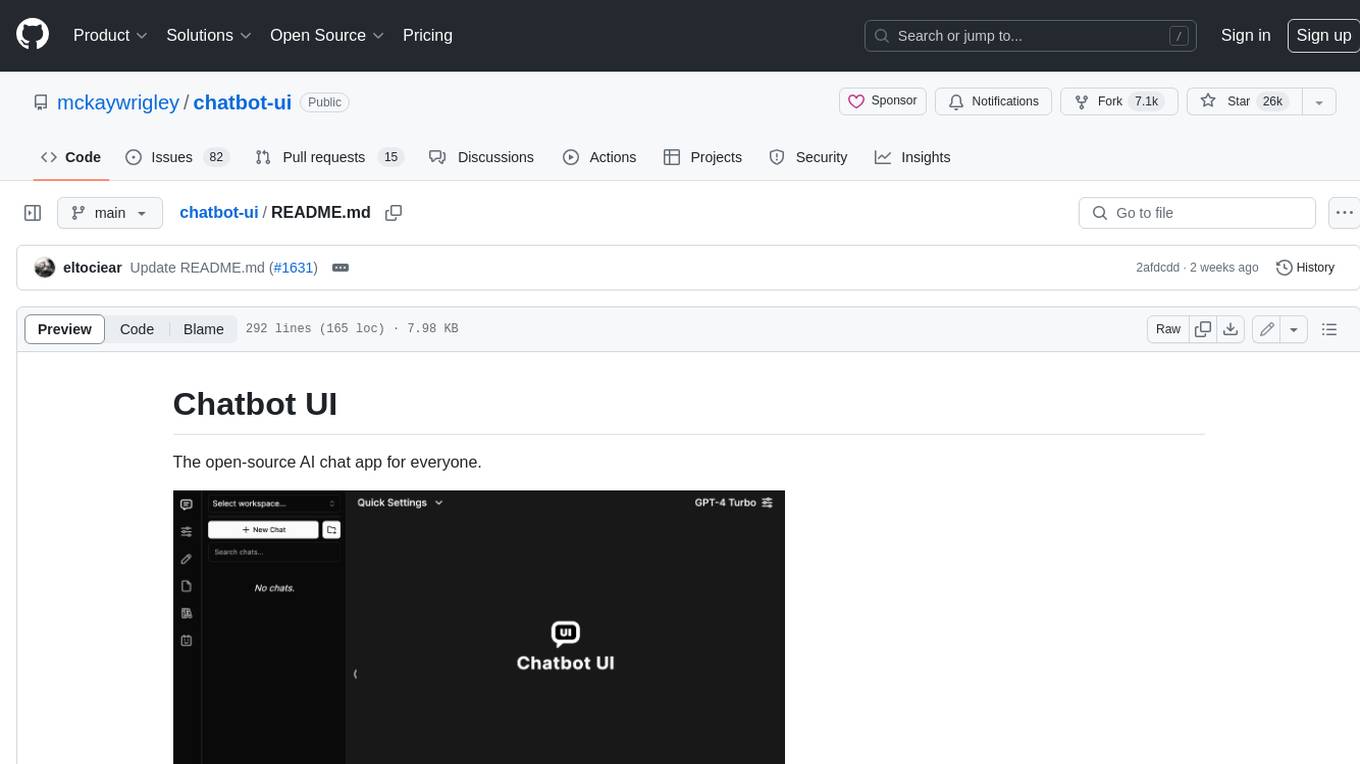
chatbot-ui
Chatbot UI is an open-source AI chat app that allows users to create and deploy their own AI chatbots. It is easy to use and can be customized to fit any need. Chatbot UI is perfect for businesses, developers, and anyone who wants to create a chatbot.
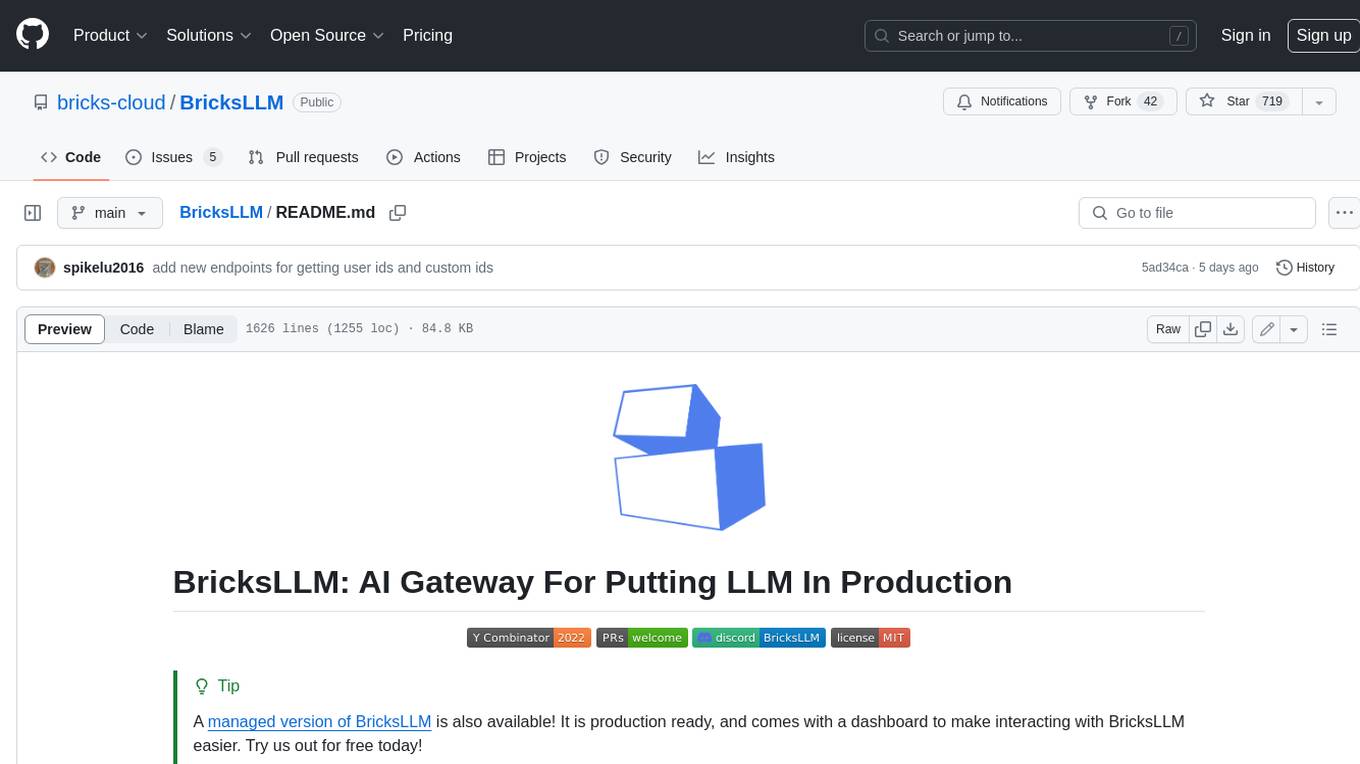
BricksLLM
BricksLLM is a cloud native AI gateway written in Go. Currently, it provides native support for OpenAI, Anthropic, Azure OpenAI and vLLM. BricksLLM aims to provide enterprise level infrastructure that can power any LLM production use cases. Here are some use cases for BricksLLM: * Set LLM usage limits for users on different pricing tiers * Track LLM usage on a per user and per organization basis * Block or redact requests containing PIIs * Improve LLM reliability with failovers, retries and caching * Distribute API keys with rate limits and cost limits for internal development/production use cases * Distribute API keys with rate limits and cost limits for students
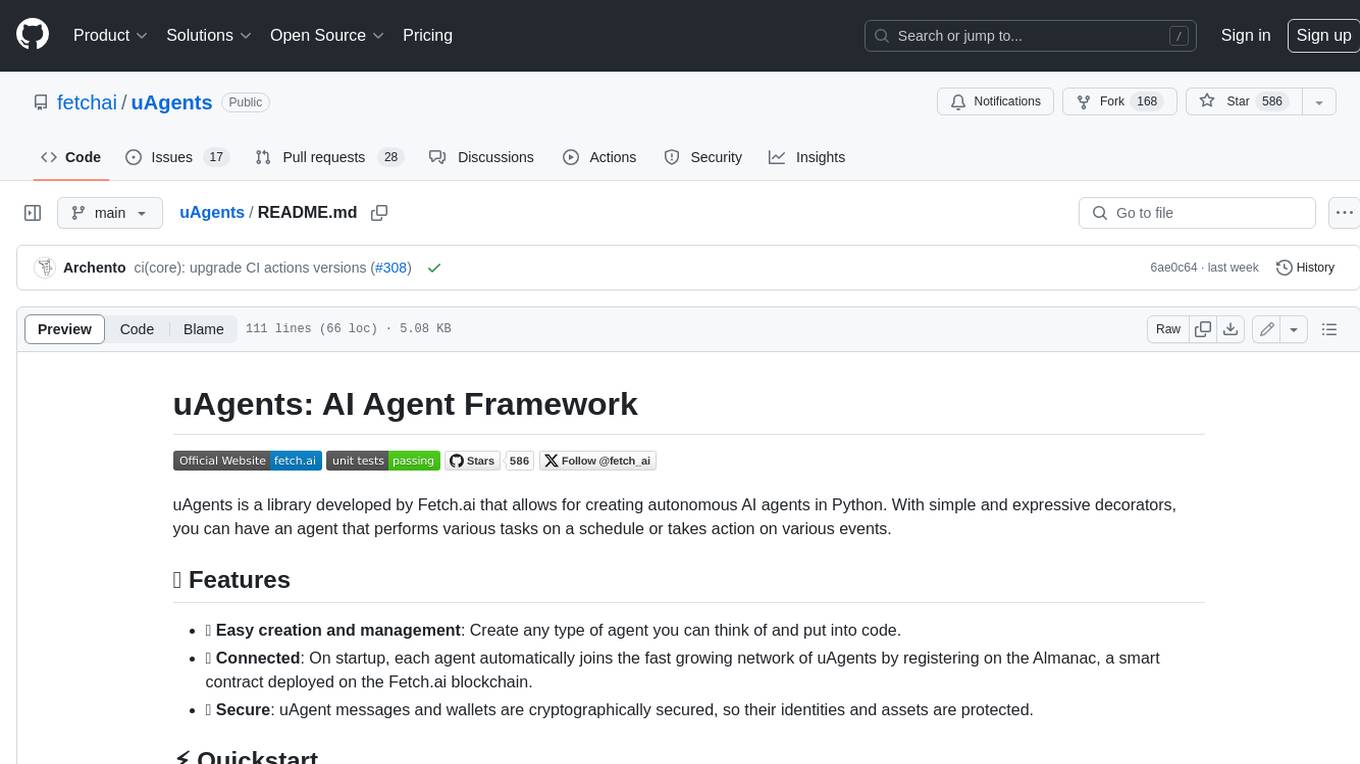
uAgents
uAgents is a Python library developed by Fetch.ai that allows for the creation of autonomous AI agents. These agents can perform various tasks on a schedule or take action on various events. uAgents are easy to create and manage, and they are connected to a fast-growing network of other uAgents. They are also secure, with cryptographically secured messages and wallets.
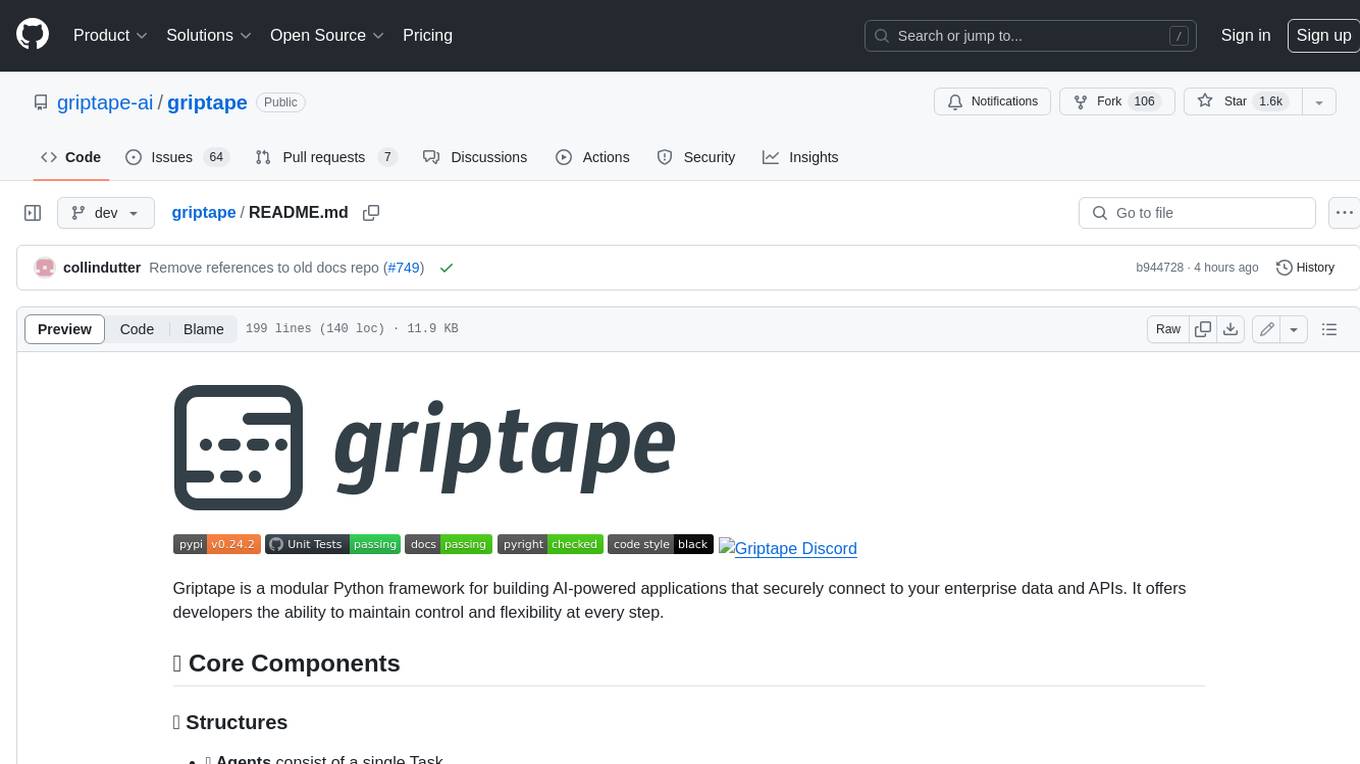
griptape
Griptape is a modular Python framework for building AI-powered applications that securely connect to your enterprise data and APIs. It offers developers the ability to maintain control and flexibility at every step. Griptape's core components include Structures (Agents, Pipelines, and Workflows), Tasks, Tools, Memory (Conversation Memory, Task Memory, and Meta Memory), Drivers (Prompt and Embedding Drivers, Vector Store Drivers, Image Generation Drivers, Image Query Drivers, SQL Drivers, Web Scraper Drivers, and Conversation Memory Drivers), Engines (Query Engines, Extraction Engines, Summary Engines, Image Generation Engines, and Image Query Engines), and additional components (Rulesets, Loaders, Artifacts, Chunkers, and Tokenizers). Griptape enables developers to create AI-powered applications with ease and efficiency.