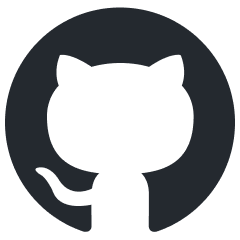
BentoDiffusion
BentoDiffusion: A collection of diffusion models served with BentoML
Stars: 325
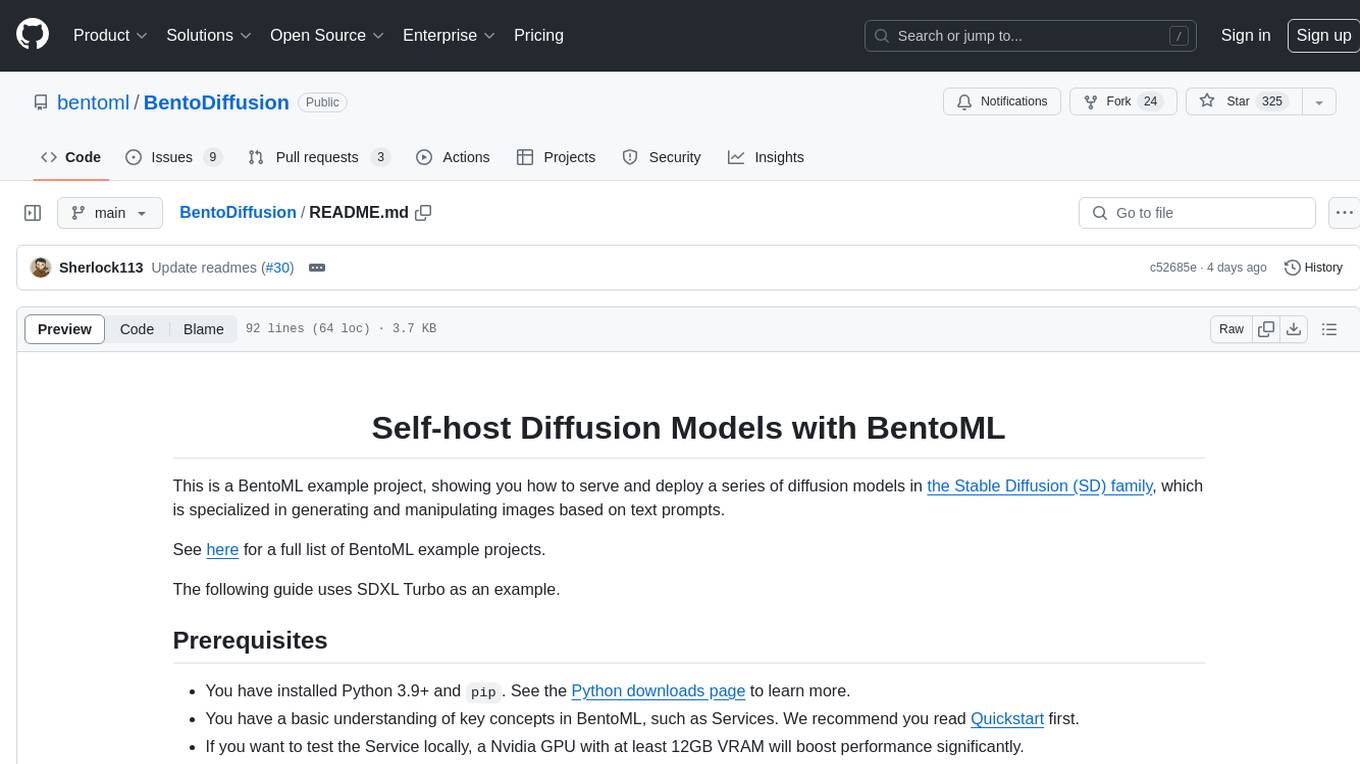
BentoDiffusion is a BentoML example project that demonstrates how to serve and deploy diffusion models in the Stable Diffusion (SD) family. These models are specialized in generating and manipulating images based on text prompts. The project provides a guide on using SDXL Turbo as an example, along with instructions on prerequisites, installing dependencies, running the BentoML service, and deploying to BentoCloud. Users can interact with the deployed service using Swagger UI or other methods. Additionally, the project offers the option to choose from various diffusion models available in the repository for deployment.
README:
This is a BentoML example project, showing you how to serve and deploy a series of diffusion models in the Stable Diffusion (SD) family, which is specialized in generating and manipulating images based on text prompts.
See here for a full list of BentoML example projects.
The following guide uses SDXL Turbo as an example.
- You have installed Python 3.9+ and
pip
. See the Python downloads page to learn more. - You have a basic understanding of key concepts in BentoML, such as Services. We recommend you read Quickstart first.
- If you want to test the Service locally, a Nvidia GPU with at least 12GB VRAM will boost performance significantly.
- (Optional) We recommend you create a virtual environment for dependency isolation for this project. See the Conda documentation or the Python documentation for details.
git clone https://github.com/bentoml/BentoDiffusion.git
cd BentoDiffusion/sdxl-turbo
pip install -r requirements.txt
We have defined a BentoML Service in service.py
. Run bentoml serve
in your project directory to start the Service.
$ bentoml serve .
2024-01-18T18:31:49+0800 [INFO] [cli] Starting production HTTP BentoServer from "service:SDXLTurboService" listening on http://localhost:3000 (Press CTRL+C to quit)
Loading pipeline components...: 100%
The server is now active at http://localhost:3000. You can interact with it using the Swagger UI or in other different ways.
CURL
curl -X 'POST' \
'http://localhost:3000/txt2img' \
-H 'accept: image/*' \
-H 'Content-Type: application/json' \
-d '{
"prompt": "A cinematic shot of a baby racoon wearing an intricate italian priest robe.",
"num_inference_steps": 1,
"guidance_scale": 0
}'
Python client
import bentoml
with bentoml.SyncHTTPClient("http://localhost:3000") as client:
result = client.txt2img(
prompt="A cinematic shot of a baby racoon wearing an intricate italian priest robe.",
num_inference_steps=1,
guidance_scale=0.0
)
For detailed explanations of the Service code, see Stable Diffusion XL Turbo.
After the Service is ready, you can deploy the application to BentoCloud for better management and scalability. Sign up if you haven't got a BentoCloud account.
Make sure you have logged in to BentoCloud, then run the following command to deploy it.
bentoml deploy .
Once the application is up and running on BentoCloud, you can access it via the exposed URL.
Note: For custom deployment in your own infrastructure, use BentoML to generate an OCI-compliant image.
To deploy a different diffusion model, go to the corresponding subdirectories of this repository.
For Tasks:
Click tags to check more tools for each tasksFor Jobs:
Alternative AI tools for BentoDiffusion
Similar Open Source Tools
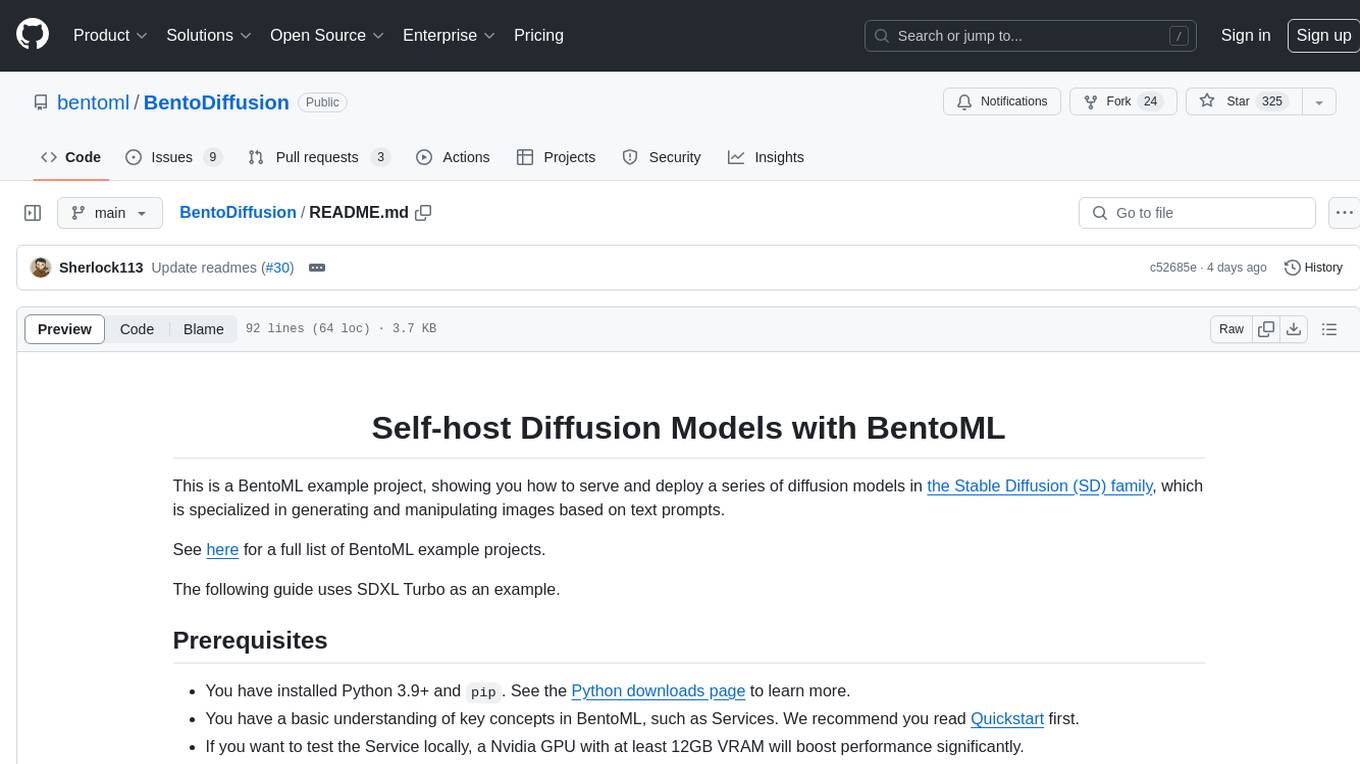
BentoDiffusion
BentoDiffusion is a BentoML example project that demonstrates how to serve and deploy diffusion models in the Stable Diffusion (SD) family. These models are specialized in generating and manipulating images based on text prompts. The project provides a guide on using SDXL Turbo as an example, along with instructions on prerequisites, installing dependencies, running the BentoML service, and deploying to BentoCloud. Users can interact with the deployed service using Swagger UI or other methods. Additionally, the project offers the option to choose from various diffusion models available in the repository for deployment.
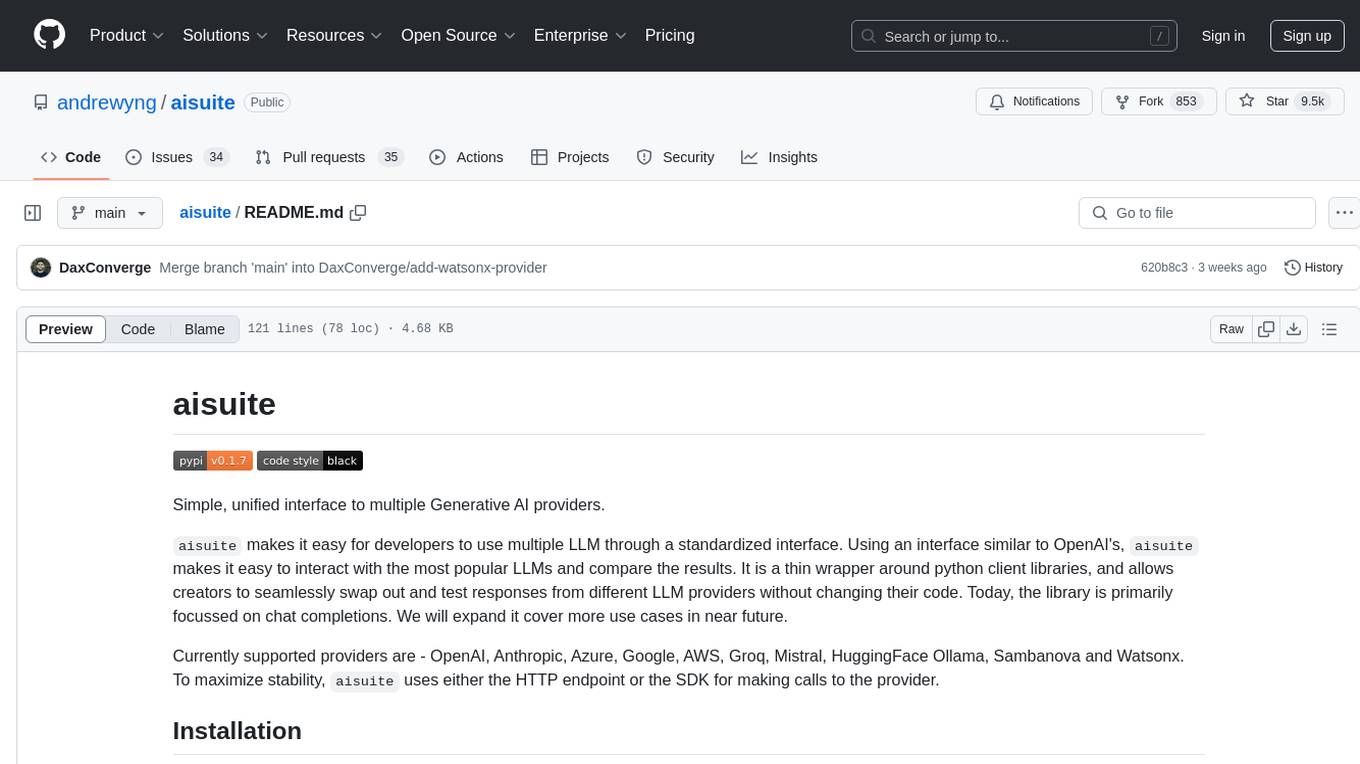
aisuite
Aisuite is a simple, unified interface to multiple Generative AI providers. It allows developers to easily interact with various Language Model (LLM) providers like OpenAI, Anthropic, Azure, Google, AWS, and more through a standardized interface. The library focuses on chat completions and provides a thin wrapper around python client libraries, enabling creators to test responses from different LLM providers without changing their code. Aisuite maximizes stability by using HTTP endpoints or SDKs for making calls to the providers. Users can install the base package or specific provider packages, set up API keys, and utilize the library to generate chat completion responses from different models.
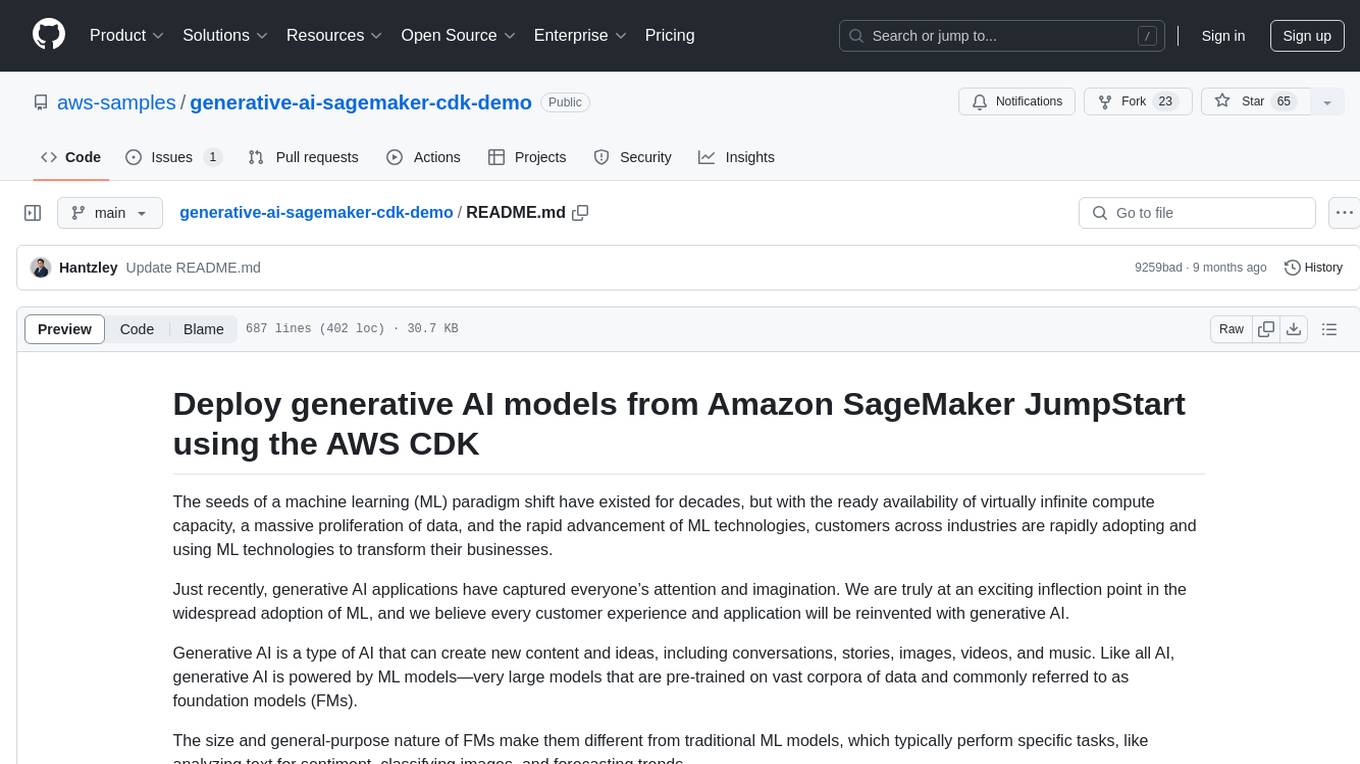
generative-ai-sagemaker-cdk-demo
This repository showcases how to deploy generative AI models from Amazon SageMaker JumpStart using the AWS CDK. Generative AI is a type of AI that can create new content and ideas, such as conversations, stories, images, videos, and music. The repository provides a detailed guide on deploying image and text generative AI models, utilizing pre-trained models from SageMaker JumpStart. The web application is built on Streamlit and hosted on Amazon ECS with Fargate. It interacts with the SageMaker model endpoints through Lambda functions and Amazon API Gateway. The repository also includes instructions on setting up the AWS CDK application, deploying the stacks, using the models, and viewing the deployed resources on the AWS Management Console.
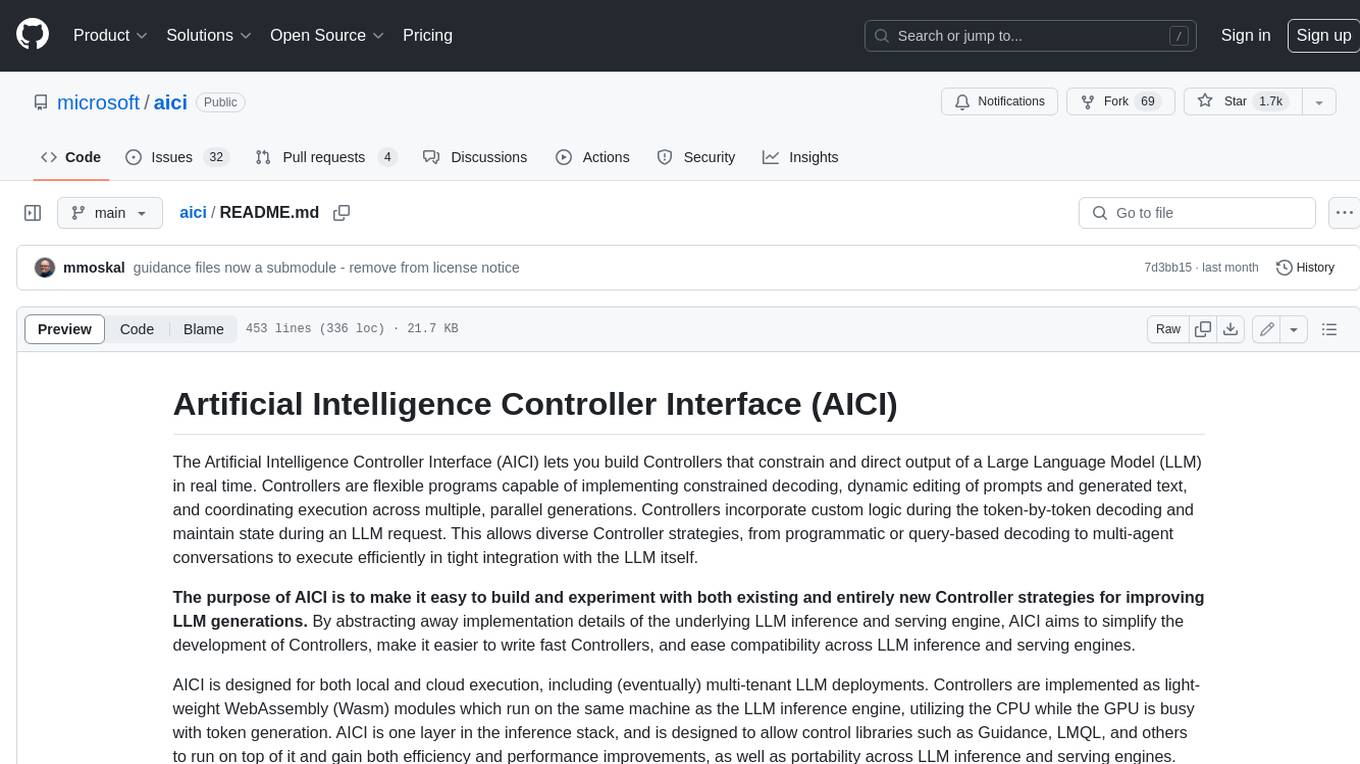
aici
The Artificial Intelligence Controller Interface (AICI) lets you build Controllers that constrain and direct output of a Large Language Model (LLM) in real time. Controllers are flexible programs capable of implementing constrained decoding, dynamic editing of prompts and generated text, and coordinating execution across multiple, parallel generations. Controllers incorporate custom logic during the token-by-token decoding and maintain state during an LLM request. This allows diverse Controller strategies, from programmatic or query-based decoding to multi-agent conversations to execute efficiently in tight integration with the LLM itself.
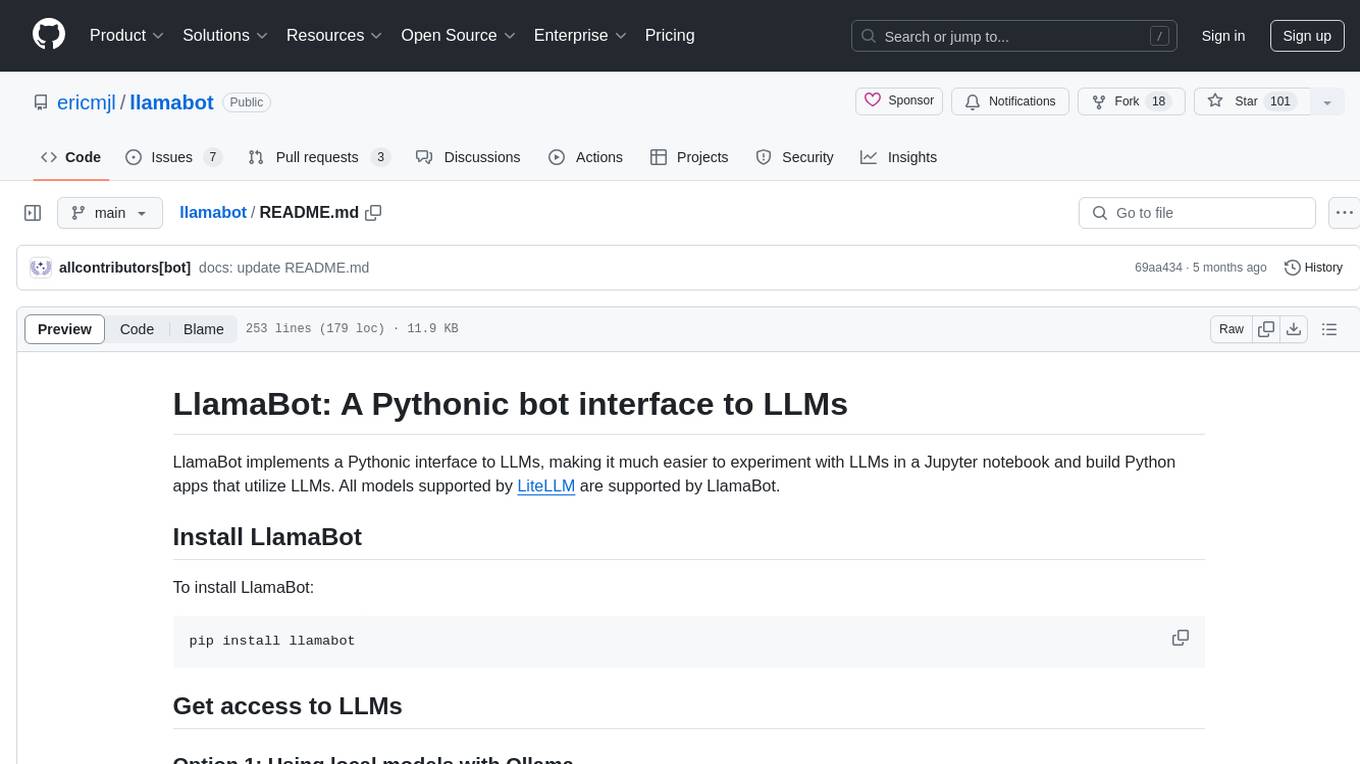
llamabot
LlamaBot is a Pythonic bot interface to Large Language Models (LLMs), providing an easy way to experiment with LLMs in Jupyter notebooks and build Python apps utilizing LLMs. It supports all models available in LiteLLM. Users can access LLMs either through local models with Ollama or by using API providers like OpenAI and Mistral. LlamaBot offers different bot interfaces like SimpleBot, ChatBot, QueryBot, and ImageBot for various tasks such as rephrasing text, maintaining chat history, querying documents, and generating images. The tool also includes CLI demos showcasing its capabilities and supports contributions for new features and bug reports from the community.
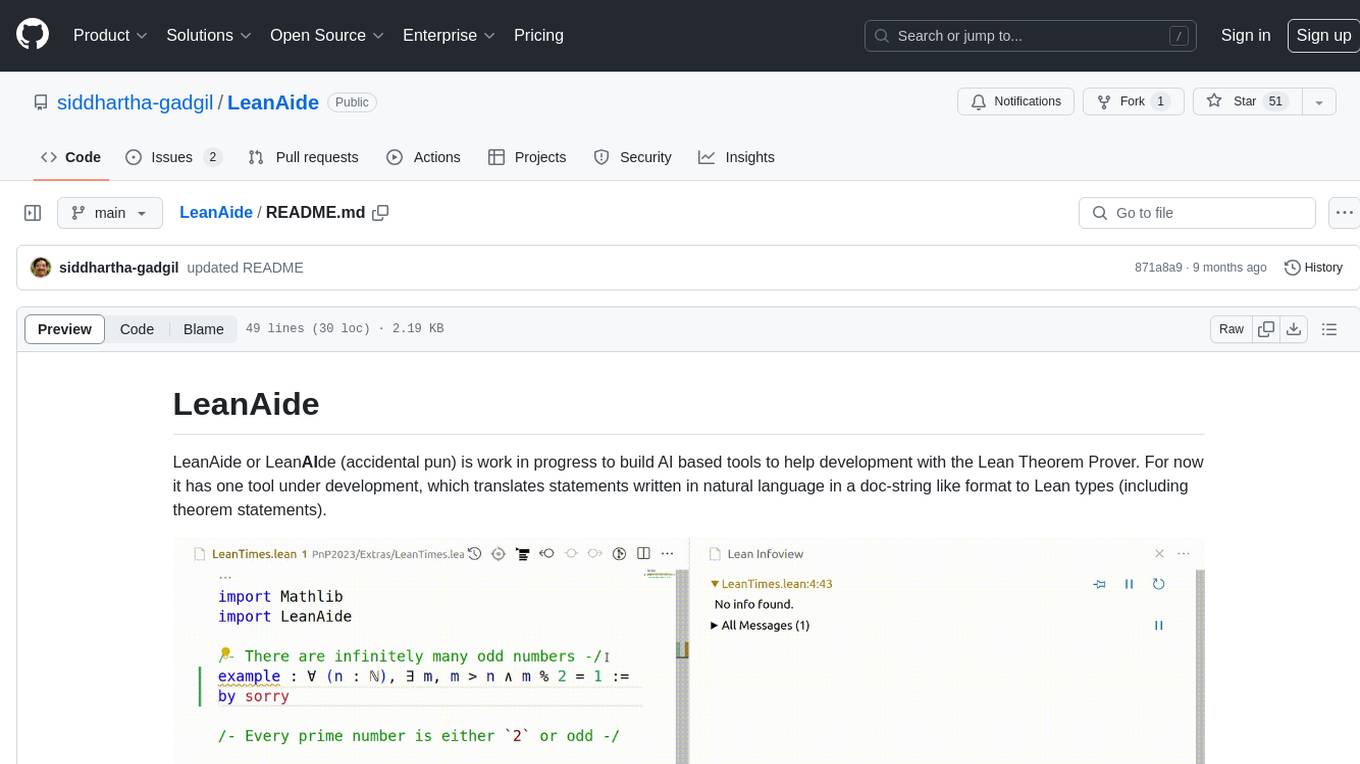
LeanAide
LeanAide is a work in progress AI tool designed to assist with development using the Lean Theorem Prover. It currently offers a tool that translates natural language statements to Lean types, including theorem statements. The tool is based on GPT 3.5-turbo/GPT 4 and requires an OpenAI key for usage. Users can include LeanAide as a dependency in their projects to access the translation functionality.
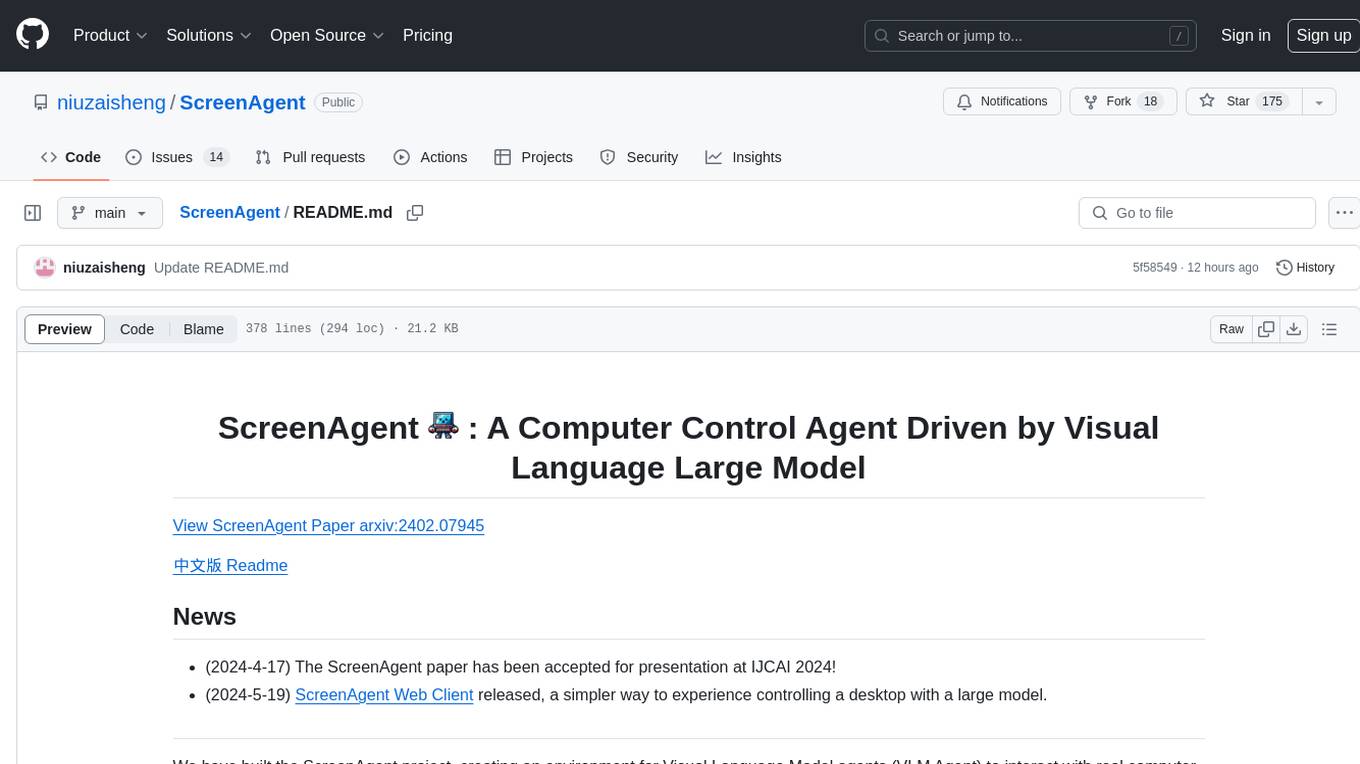
ScreenAgent
ScreenAgent is a project focused on creating an environment for Visual Language Model agents (VLM Agent) to interact with real computer screens. The project includes designing an automatic control process for agents to interact with the environment and complete multi-step tasks. It also involves building the ScreenAgent dataset, which collects screenshots and action sequences for various daily computer tasks. The project provides a controller client code, configuration files, and model training code to enable users to control a desktop with a large model.
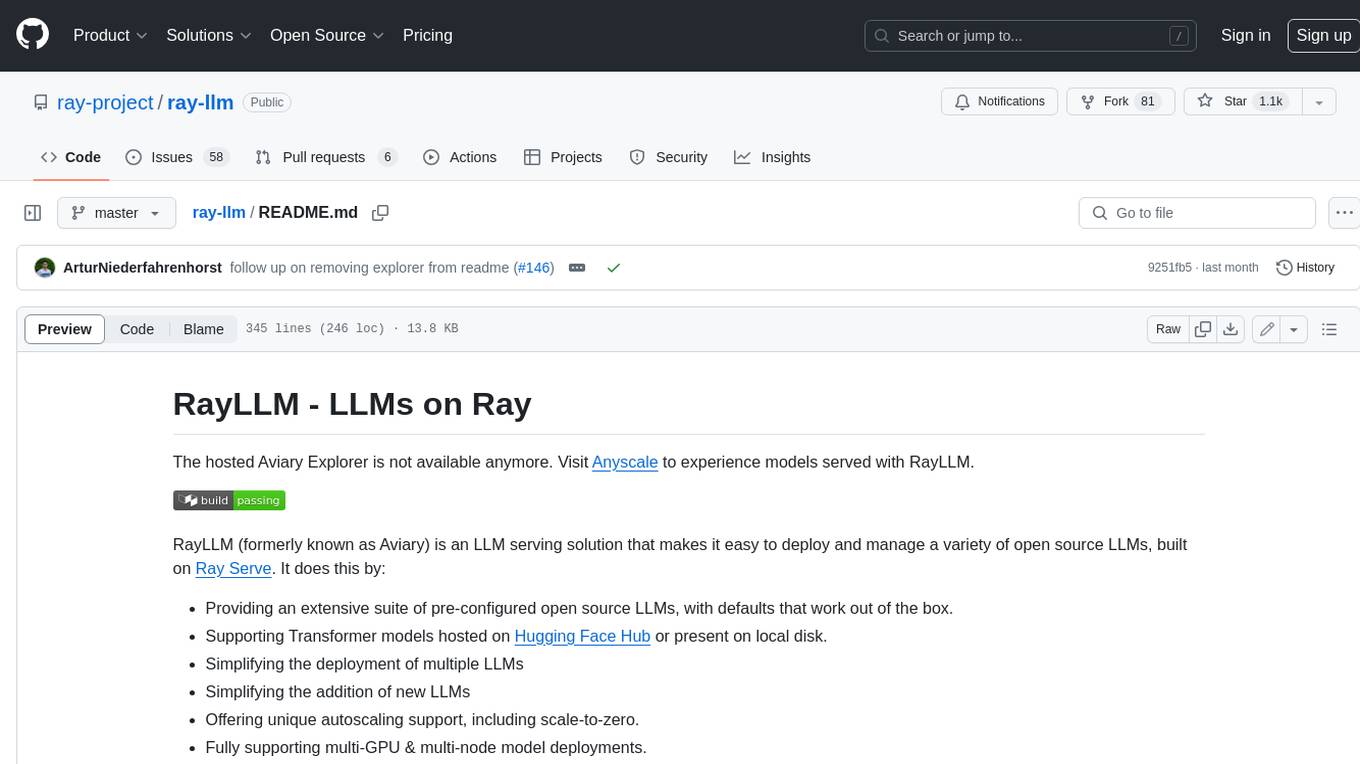
ray-llm
RayLLM (formerly known as Aviary) is an LLM serving solution that makes it easy to deploy and manage a variety of open source LLMs, built on Ray Serve. It provides an extensive suite of pre-configured open source LLMs, with defaults that work out of the box. RayLLM supports Transformer models hosted on Hugging Face Hub or present on local disk. It simplifies the deployment of multiple LLMs, the addition of new LLMs, and offers unique autoscaling support, including scale-to-zero. RayLLM fully supports multi-GPU & multi-node model deployments and offers high performance features like continuous batching, quantization and streaming. It provides a REST API that is similar to OpenAI's to make it easy to migrate and cross test them. RayLLM supports multiple LLM backends out of the box, including vLLM and TensorRT-LLM.
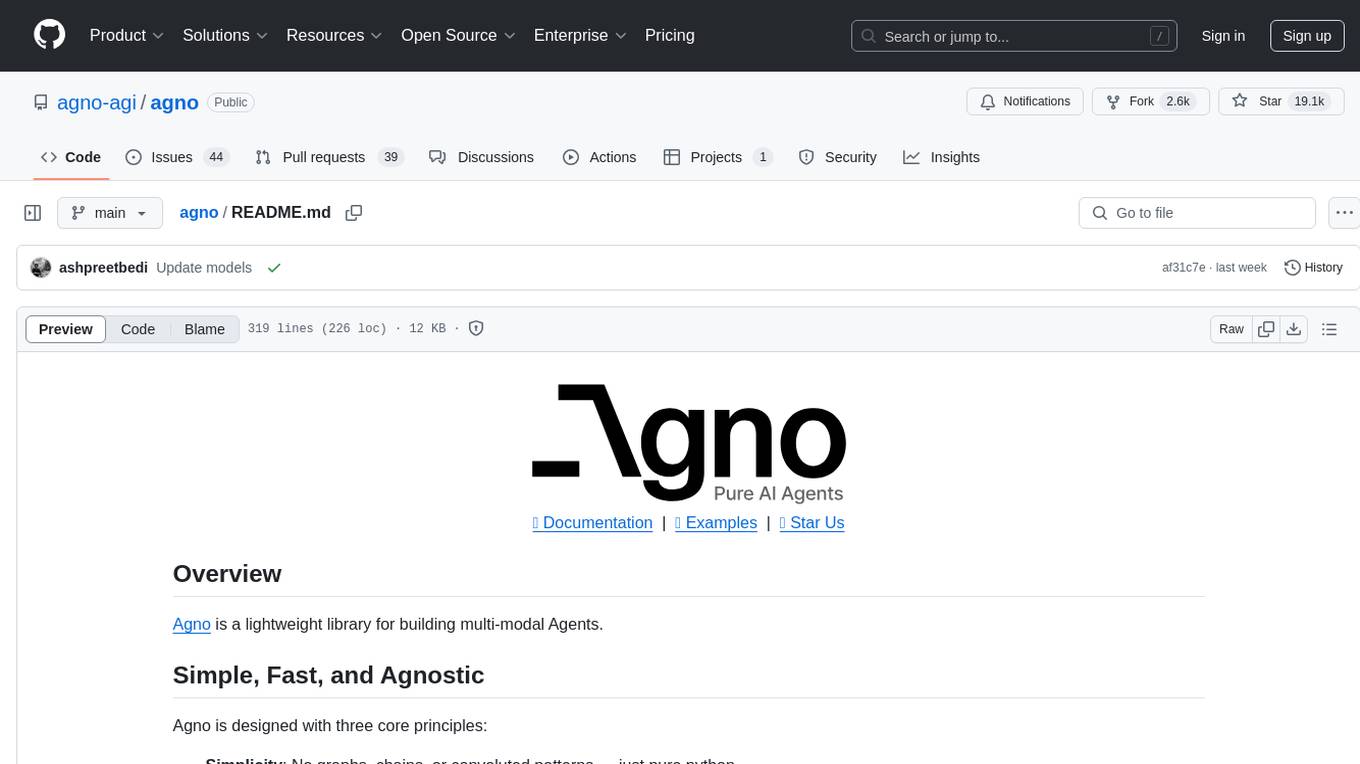
agno
Agno is a lightweight library for building multi-modal Agents. It is designed with core principles of simplicity, uncompromising performance, and agnosticism, allowing users to create blazing fast agents with minimal memory footprint. Agno supports any model, any provider, and any modality, making it a versatile container for AGI. Users can build agents with lightning-fast agent creation, model agnostic capabilities, native support for text, image, audio, and video inputs and outputs, memory management, knowledge stores, structured outputs, and real-time monitoring. The library enables users to create autonomous programs that use language models to solve problems, improve responses, and achieve tasks with varying levels of agency and autonomy.
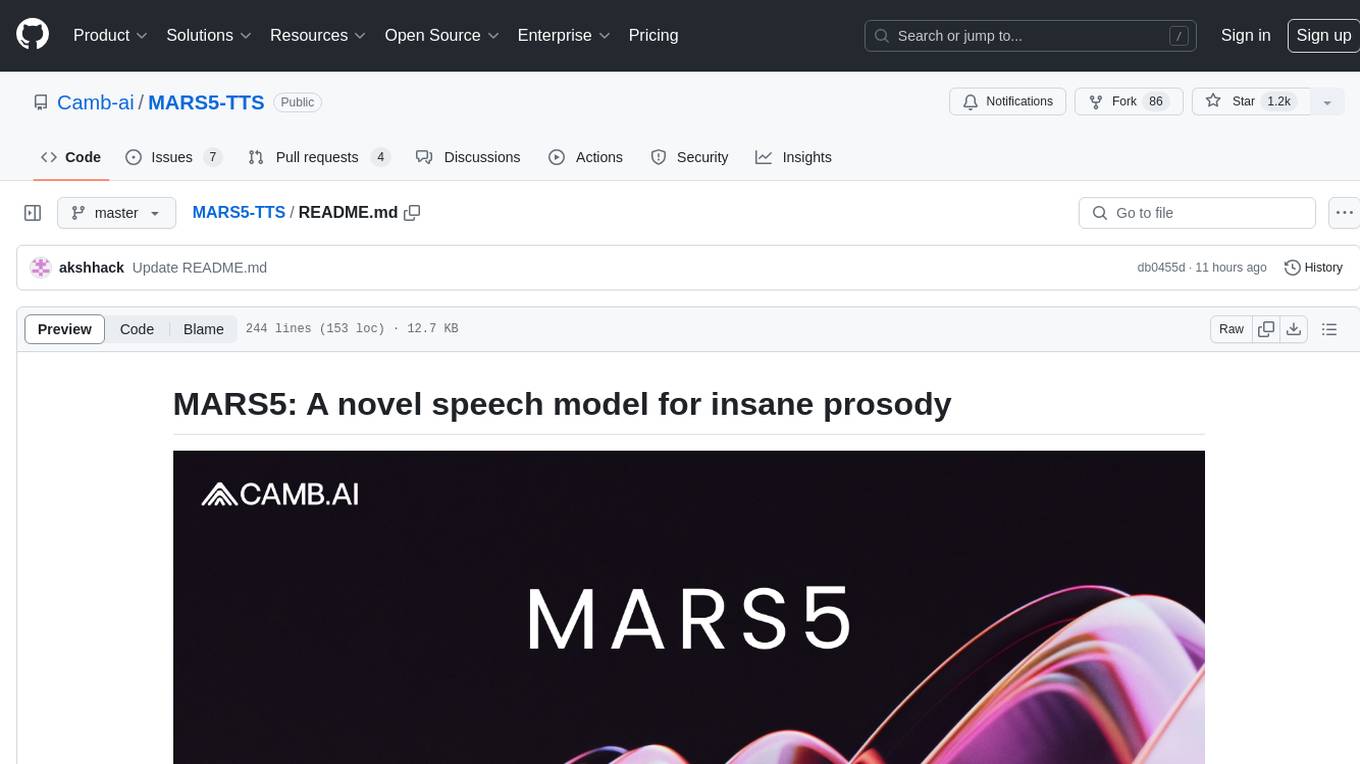
MARS5-TTS
MARS5 is a novel English speech model (TTS) developed by CAMB.AI, featuring a two-stage AR-NAR pipeline with a unique NAR component. The model can generate speech for various scenarios like sports commentary and anime with just 5 seconds of audio and a text snippet. It allows steering prosody using punctuation and capitalization in the transcript. Speaker identity is specified using an audio reference file, enabling 'deep clone' for improved quality. The model can be used via torch.hub or HuggingFace, supporting both shallow and deep cloning for inference. Checkpoints are provided for AR and NAR models, with hardware requirements of 750M+450M params on GPU. Contributions to improve model stability, performance, and reference audio selection are welcome.
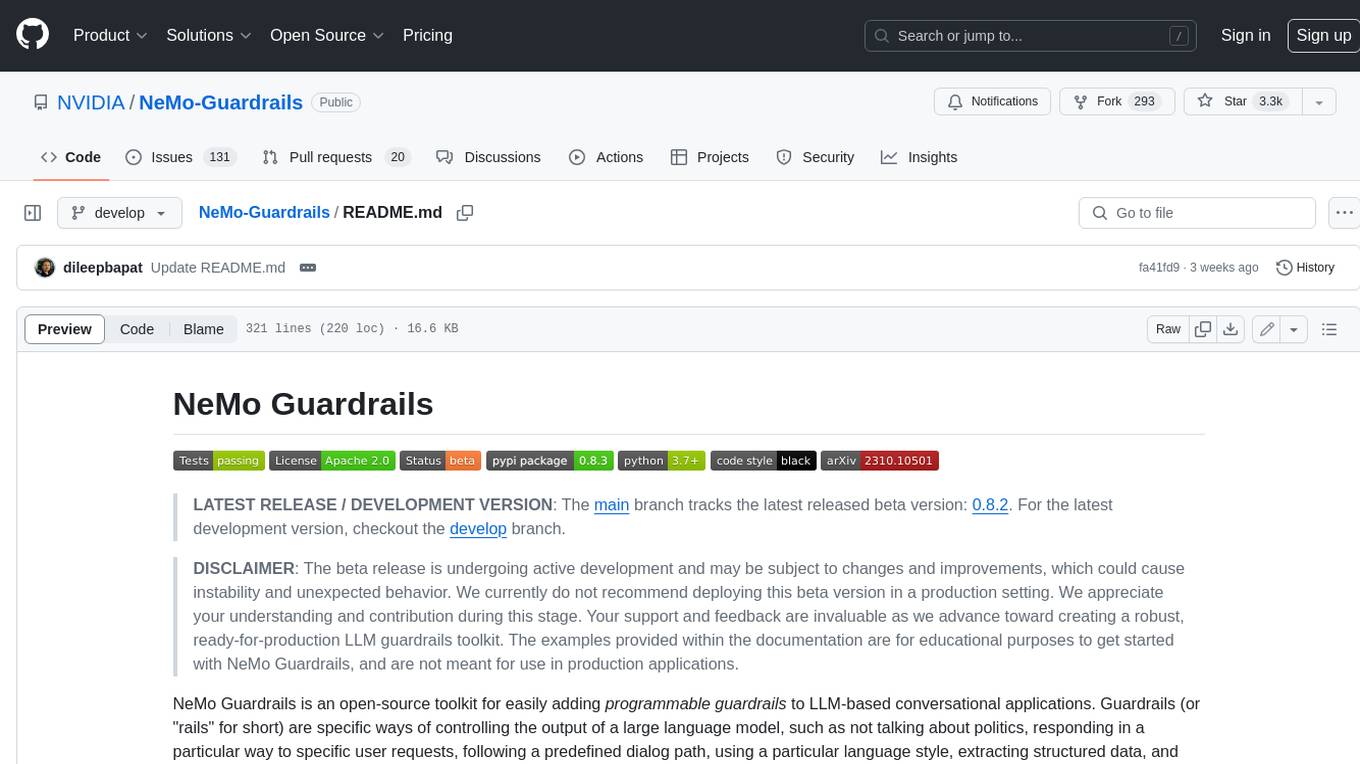
NeMo-Guardrails
NeMo Guardrails is an open-source toolkit for easily adding _programmable guardrails_ to LLM-based conversational applications. Guardrails (or "rails" for short) are specific ways of controlling the output of a large language model, such as not talking about politics, responding in a particular way to specific user requests, following a predefined dialog path, using a particular language style, extracting structured data, and more.
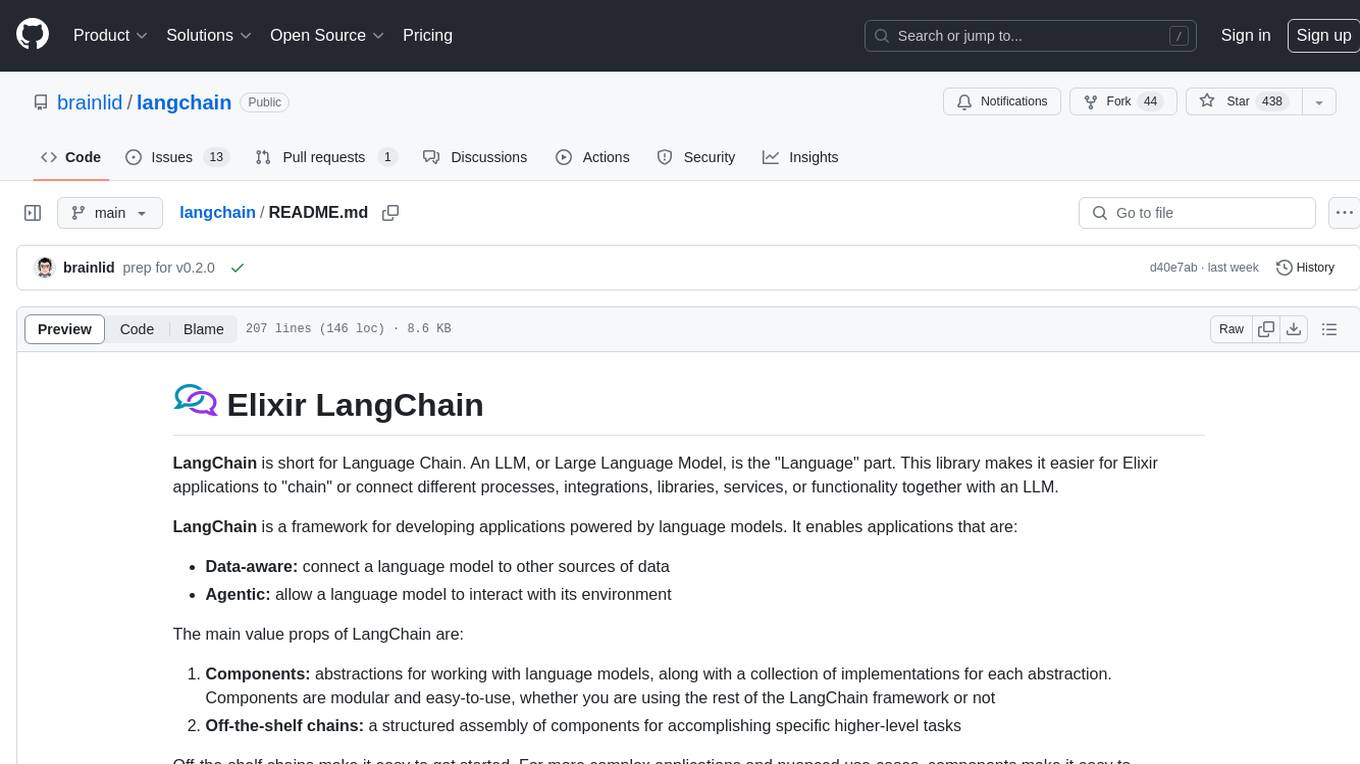
langchain
LangChain is a framework for developing Elixir applications powered by language models. It enables applications to connect language models to other data sources and interact with the environment. The library provides components for working with language models and off-the-shelf chains for specific tasks. It aims to assist in building applications that combine large language models with other sources of computation or knowledge. LangChain is written in Elixir and is not aimed for parity with the JavaScript and Python versions due to differences in programming paradigms and design choices. The library is designed to make it easy to integrate language models into applications and expose features, data, and functionality to the models.
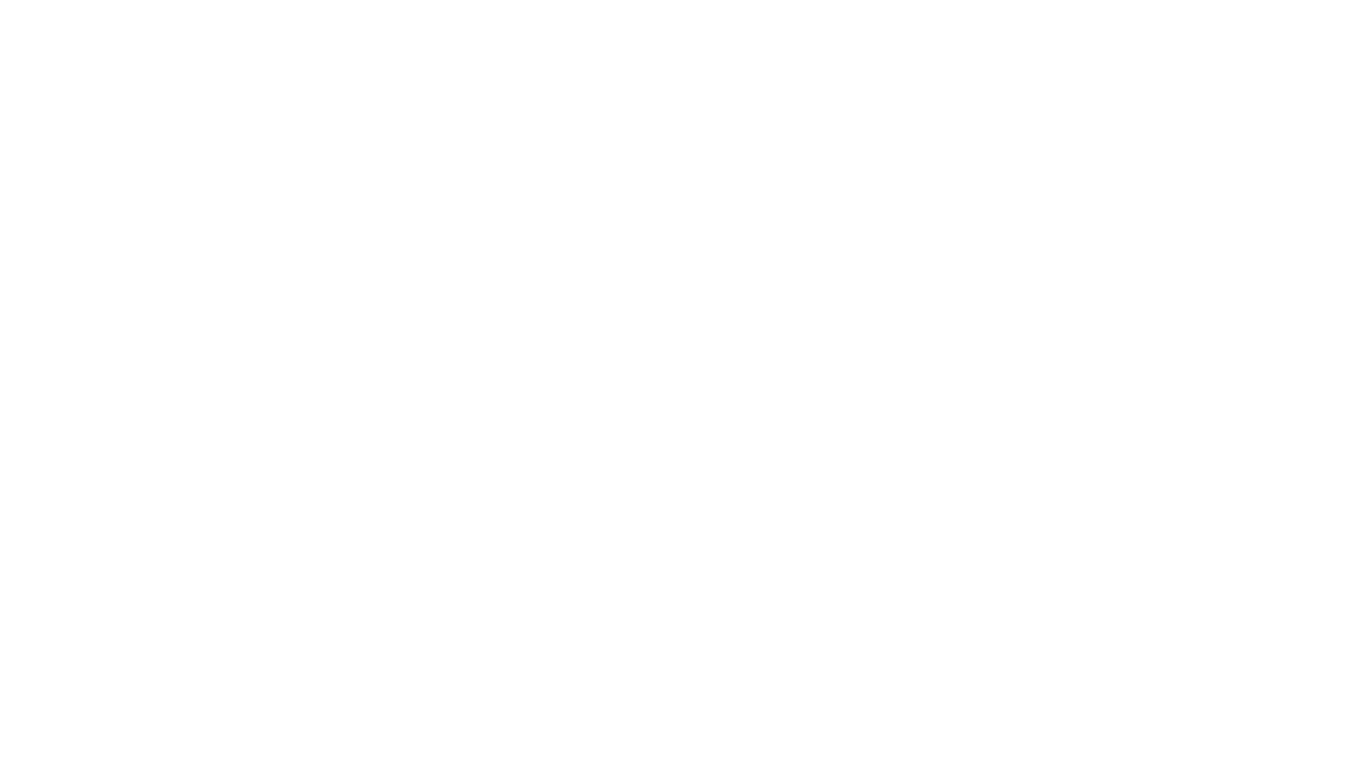
rag-experiment-accelerator
The RAG Experiment Accelerator is a versatile tool that helps you conduct experiments and evaluations using Azure AI Search and RAG pattern. It offers a rich set of features, including experiment setup, integration with Azure AI Search, Azure Machine Learning, MLFlow, and Azure OpenAI, multiple document chunking strategies, query generation, multiple search types, sub-querying, re-ranking, metrics and evaluation, report generation, and multi-lingual support. The tool is designed to make it easier and faster to run experiments and evaluations of search queries and quality of response from OpenAI, and is useful for researchers, data scientists, and developers who want to test the performance of different search and OpenAI related hyperparameters, compare the effectiveness of various search strategies, fine-tune and optimize parameters, find the best combination of hyperparameters, and generate detailed reports and visualizations from experiment results.
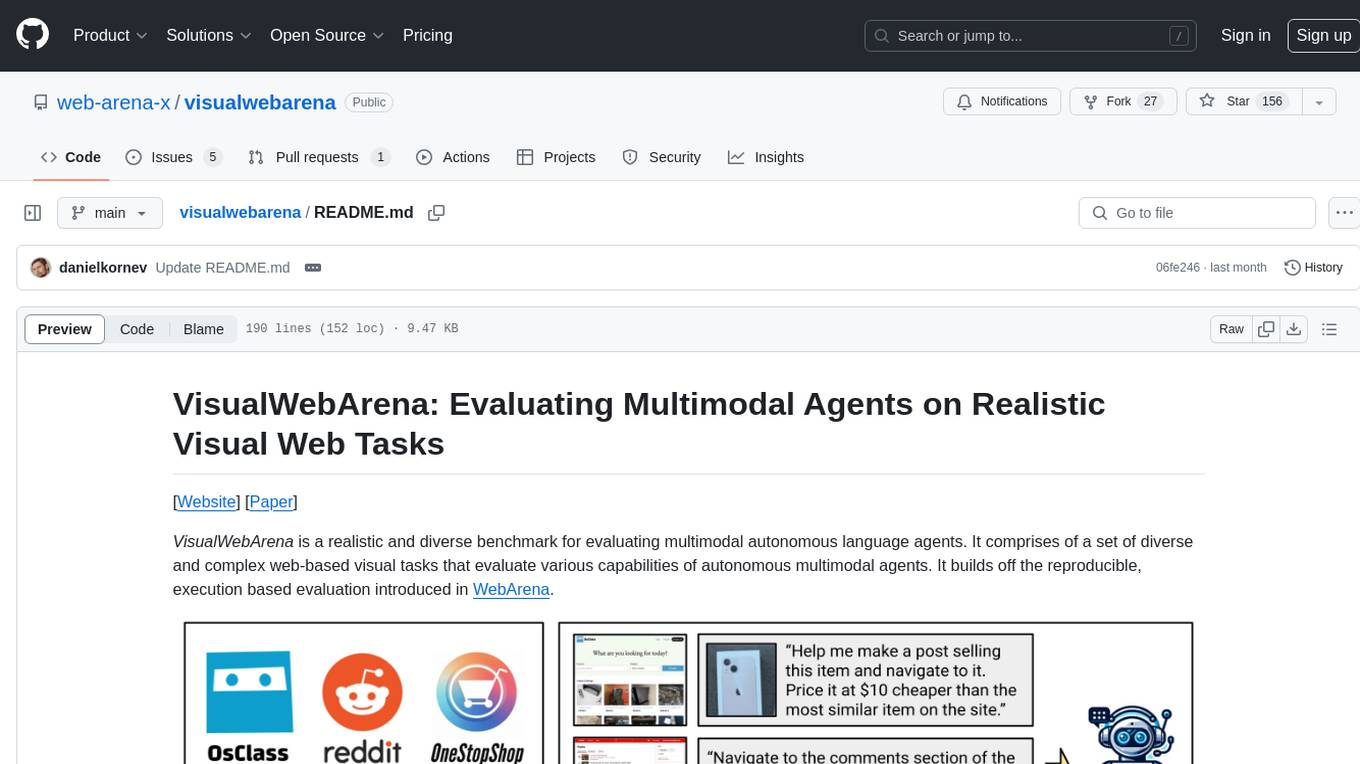
visualwebarena
VisualWebArena is a benchmark for evaluating multimodal autonomous language agents through diverse and complex web-based visual tasks. It builds on the reproducible evaluation introduced in WebArena. The repository provides scripts for end-to-end training, demos to run multimodal agents on webpages, and tools for setting up environments for evaluation. It includes trajectories of the GPT-4V + SoM agent on VWA tasks, along with human evaluations on 233 tasks. The environment supports OpenAI models and Gemini models for evaluation.
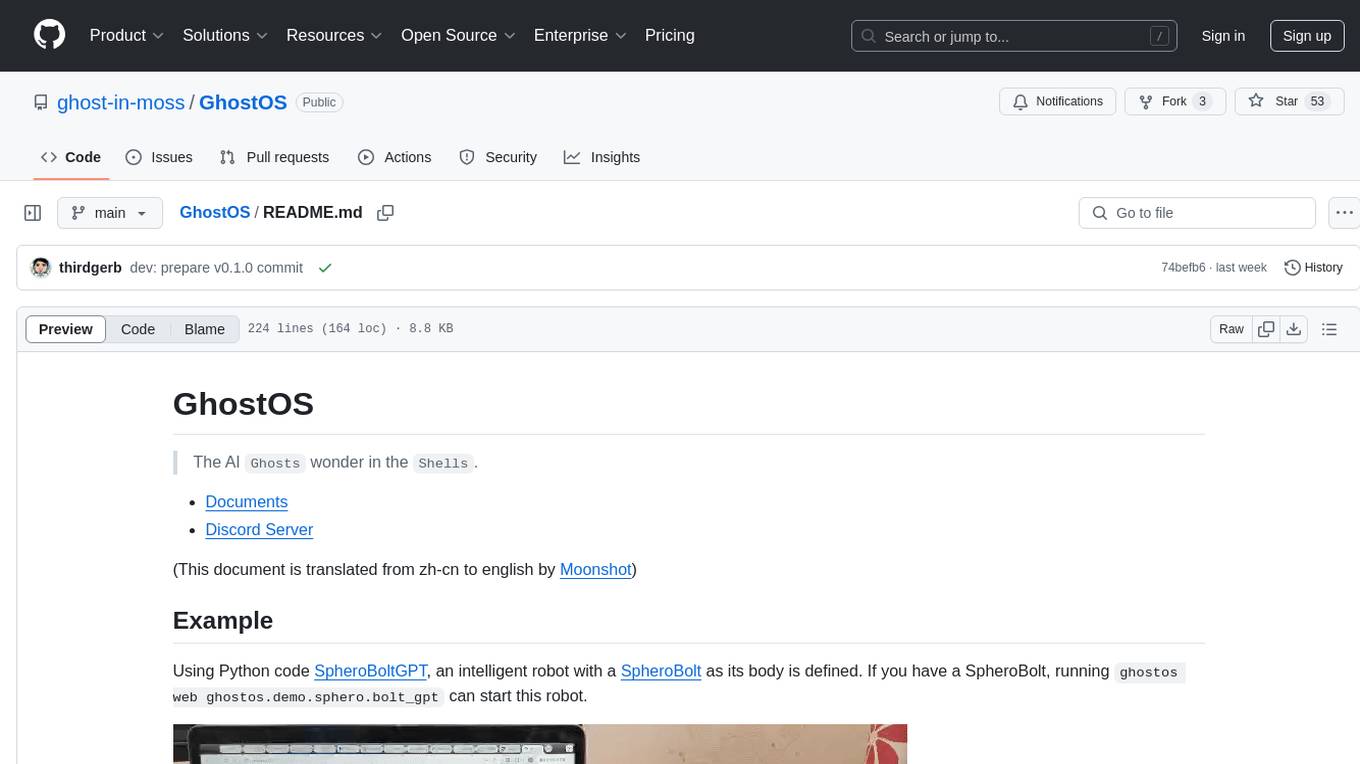
GhostOS
GhostOS is an AI Agent framework designed to replace JSON Schema with a Turing-complete code interaction interface (Moss Protocol). It aims to create intelligent entities capable of continuous learning and growth through code generation and project management. The framework supports various capabilities such as turning Python files into web agents, real-time voice conversation, body movements control, and emotion expression. GhostOS is still in early experimental development and focuses on out-of-the-box capabilities for AI agents.
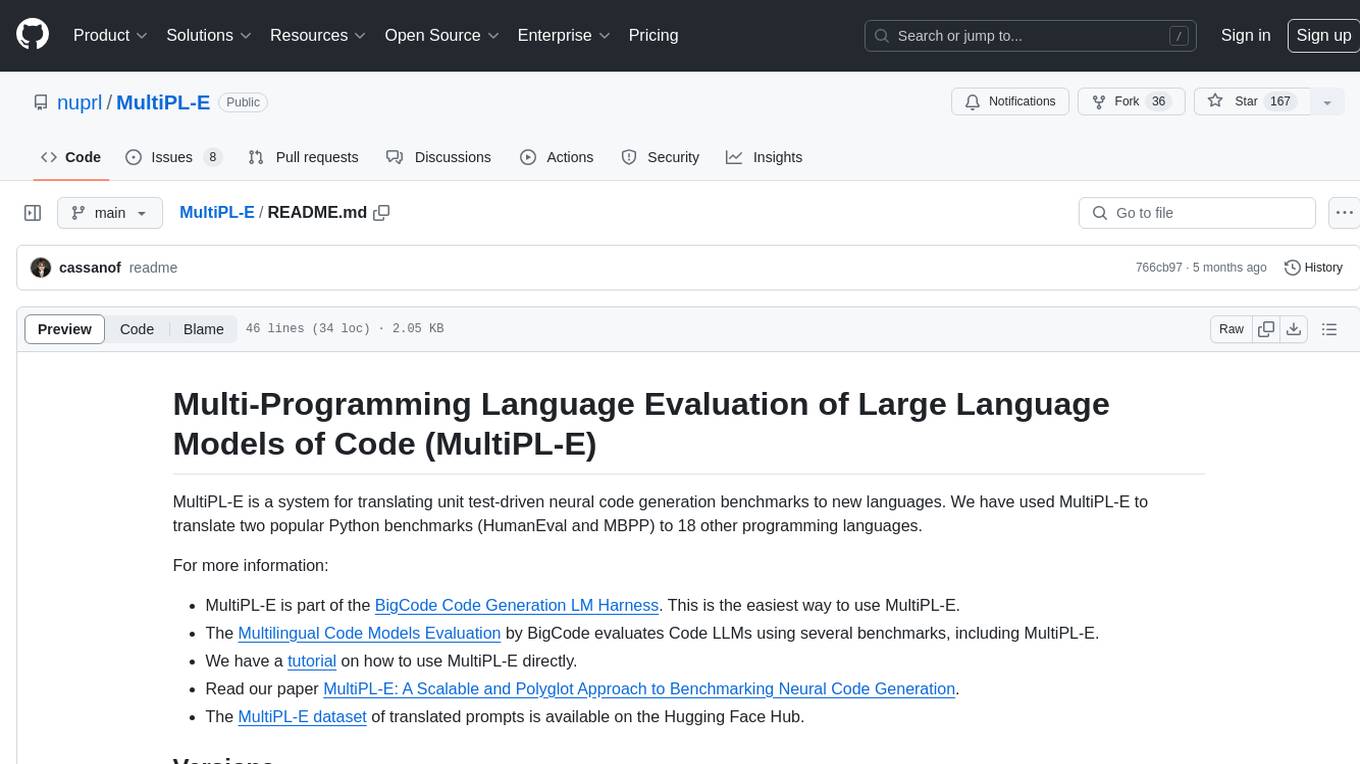
MultiPL-E
MultiPL-E is a system for translating unit test-driven neural code generation benchmarks to new languages. It is part of the BigCode Code Generation LM Harness and allows for evaluating Code LLMs using various benchmarks. The tool supports multiple versions with improvements and new language additions, providing a scalable and polyglot approach to benchmarking neural code generation. Users can access a tutorial for direct usage and explore the dataset of translated prompts on the Hugging Face Hub.
For similar tasks
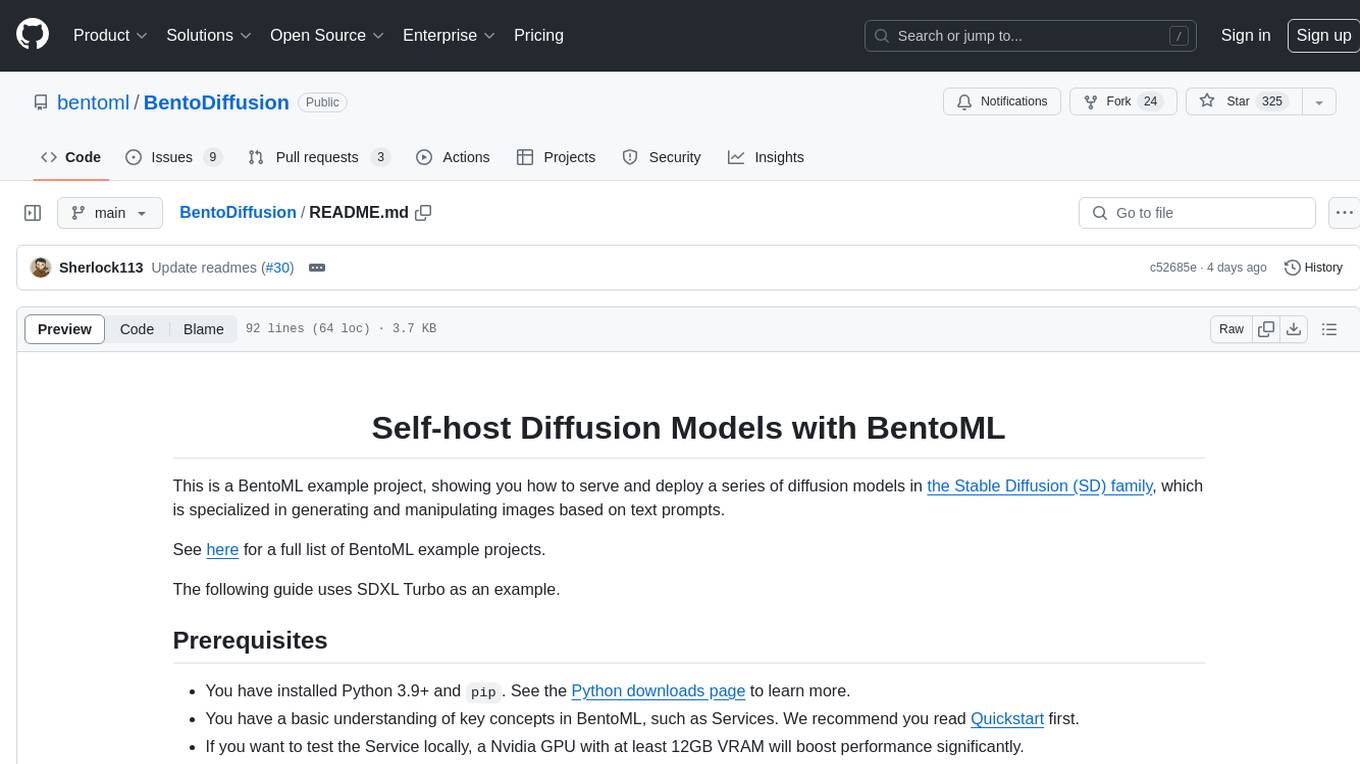
BentoDiffusion
BentoDiffusion is a BentoML example project that demonstrates how to serve and deploy diffusion models in the Stable Diffusion (SD) family. These models are specialized in generating and manipulating images based on text prompts. The project provides a guide on using SDXL Turbo as an example, along with instructions on prerequisites, installing dependencies, running the BentoML service, and deploying to BentoCloud. Users can interact with the deployed service using Swagger UI or other methods. Additionally, the project offers the option to choose from various diffusion models available in the repository for deployment.
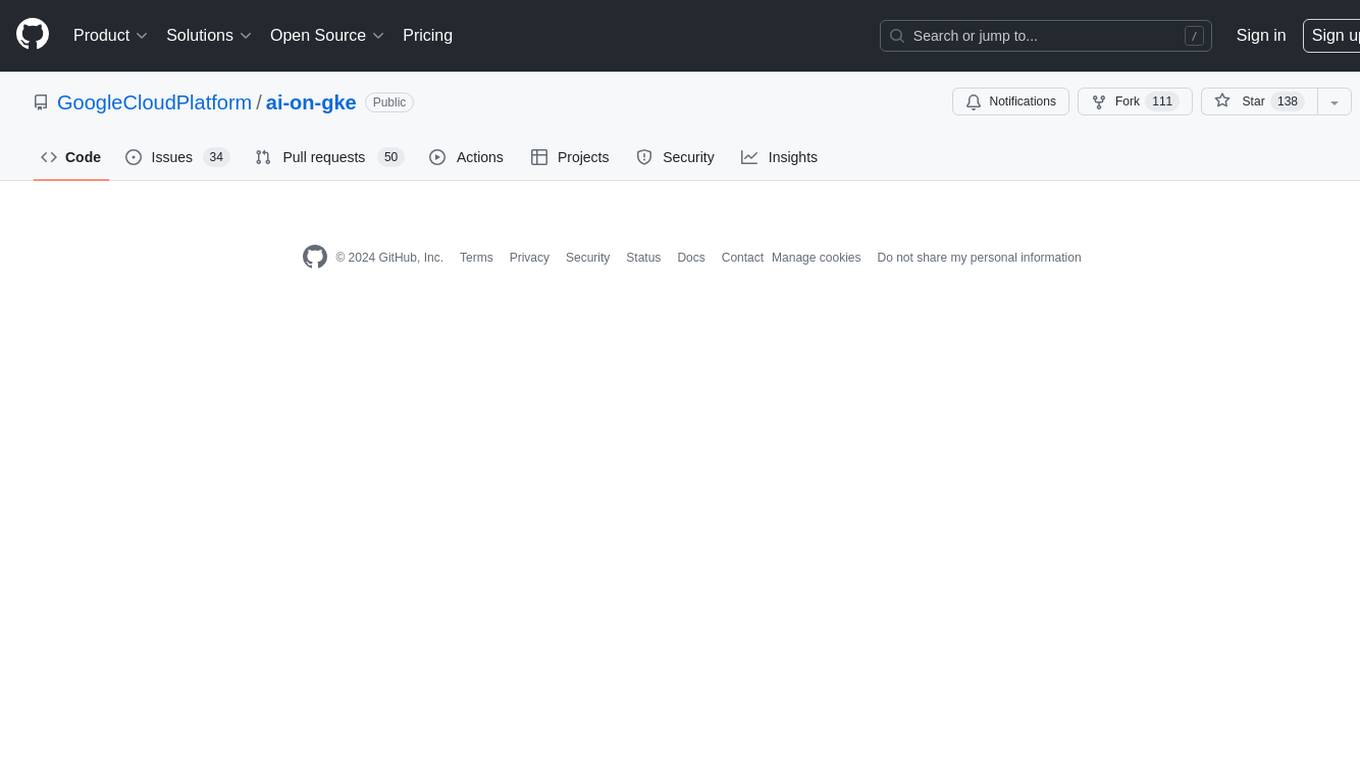
ai-on-gke
This repository contains assets related to AI/ML workloads on Google Kubernetes Engine (GKE). Run optimized AI/ML workloads with Google Kubernetes Engine (GKE) platform orchestration capabilities. A robust AI/ML platform considers the following layers: Infrastructure orchestration that support GPUs and TPUs for training and serving workloads at scale Flexible integration with distributed computing and data processing frameworks Support for multiple teams on the same infrastructure to maximize utilization of resources
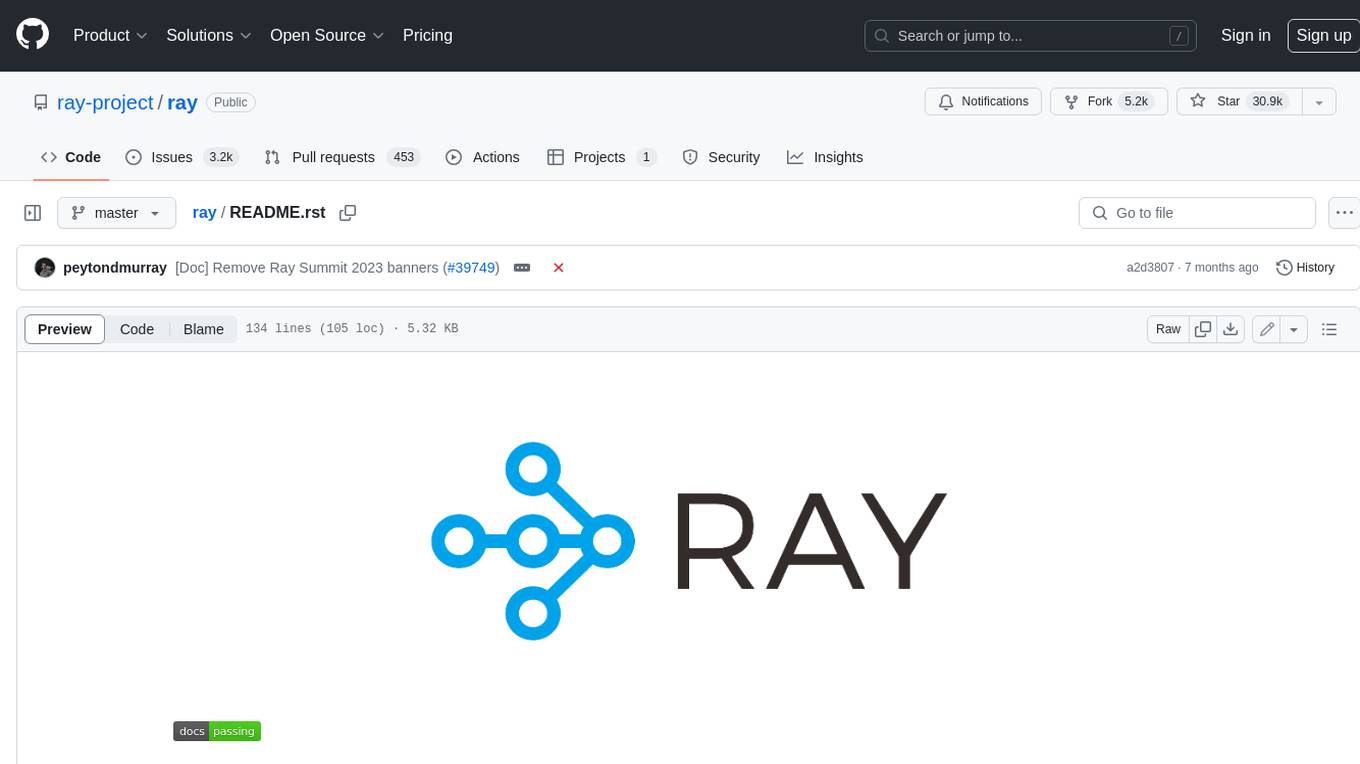
ray
Ray is a unified framework for scaling AI and Python applications. It consists of a core distributed runtime and a set of AI libraries for simplifying ML compute, including Data, Train, Tune, RLlib, and Serve. Ray runs on any machine, cluster, cloud provider, and Kubernetes, and features a growing ecosystem of community integrations. With Ray, you can seamlessly scale the same code from a laptop to a cluster, making it easy to meet the compute-intensive demands of modern ML workloads.
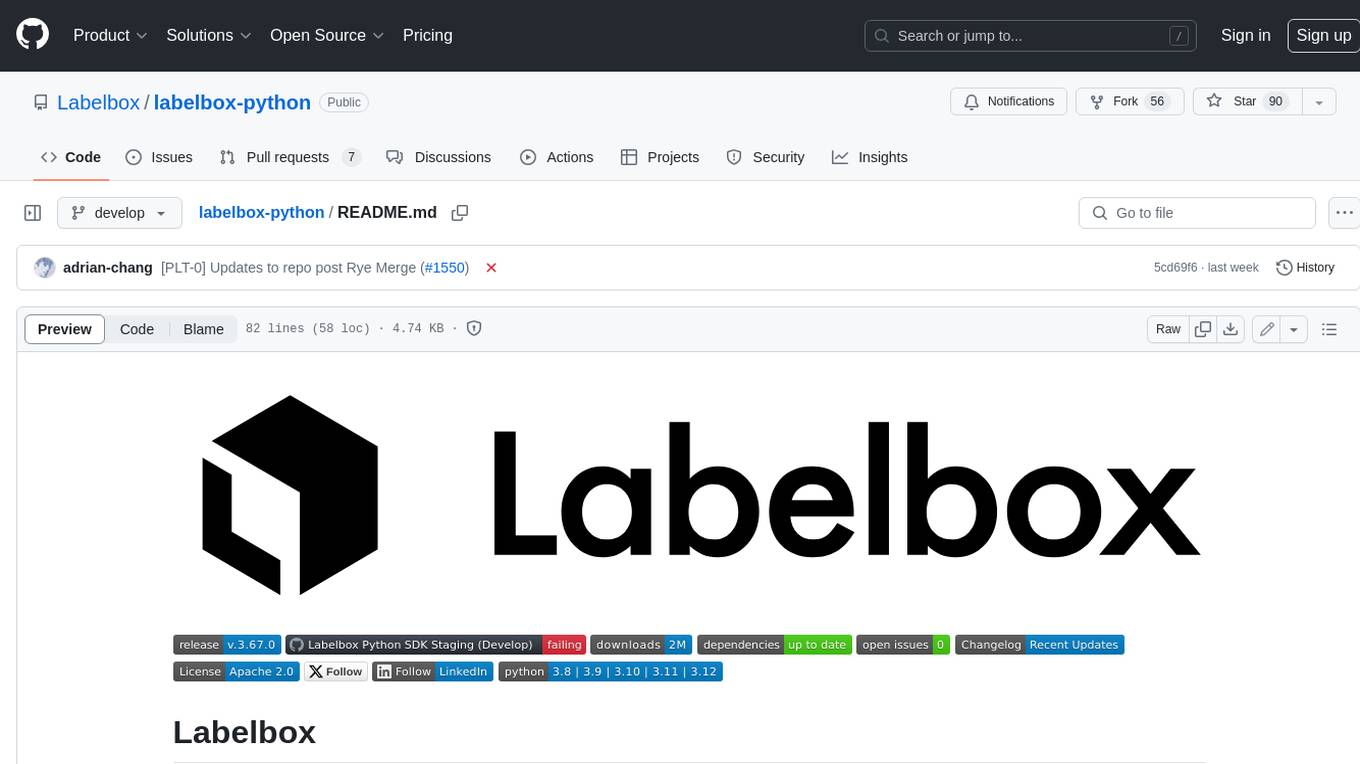
labelbox-python
Labelbox is a data-centric AI platform for enterprises to develop, optimize, and use AI to solve problems and power new products and services. Enterprises use Labelbox to curate data, generate high-quality human feedback data for computer vision and LLMs, evaluate model performance, and automate tasks by combining AI and human-centric workflows. The academic & research community uses Labelbox for cutting-edge AI research.
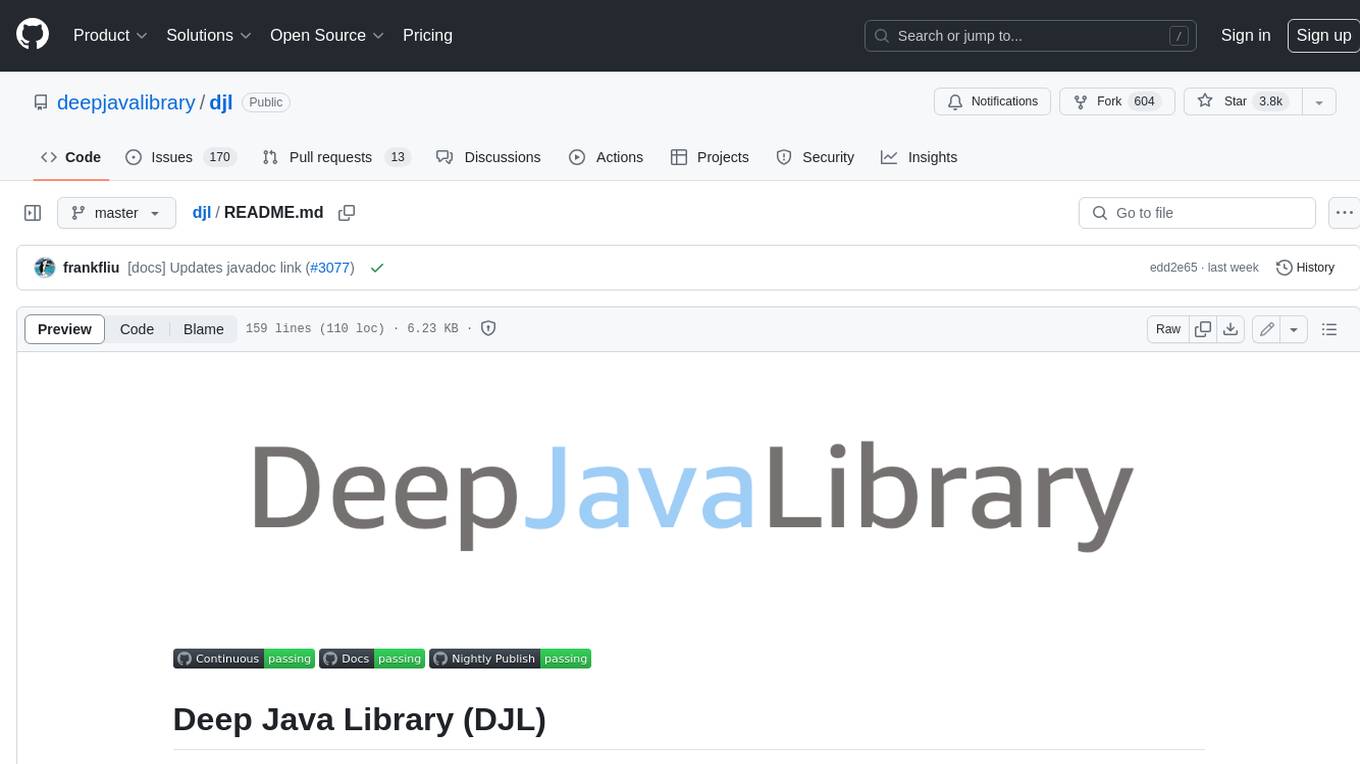
djl
Deep Java Library (DJL) is an open-source, high-level, engine-agnostic Java framework for deep learning. It is designed to be easy to get started with and simple to use for Java developers. DJL provides a native Java development experience and allows users to integrate machine learning and deep learning models with their Java applications. The framework is deep learning engine agnostic, enabling users to switch engines at any point for optimal performance. DJL's ergonomic API interface guides users with best practices to accomplish deep learning tasks, such as running inference and training neural networks.
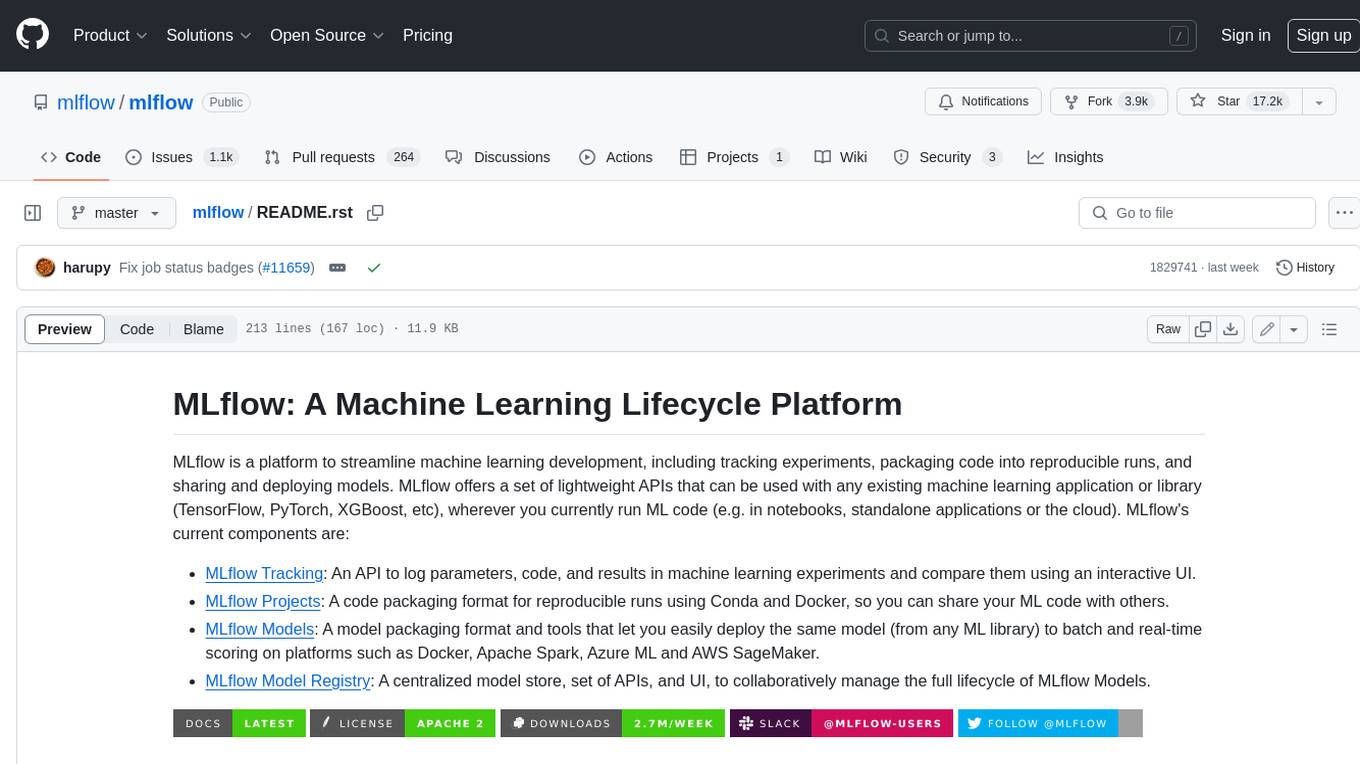
mlflow
MLflow is a platform to streamline machine learning development, including tracking experiments, packaging code into reproducible runs, and sharing and deploying models. MLflow offers a set of lightweight APIs that can be used with any existing machine learning application or library (TensorFlow, PyTorch, XGBoost, etc), wherever you currently run ML code (e.g. in notebooks, standalone applications or the cloud). MLflow's current components are:
* `MLflow Tracking
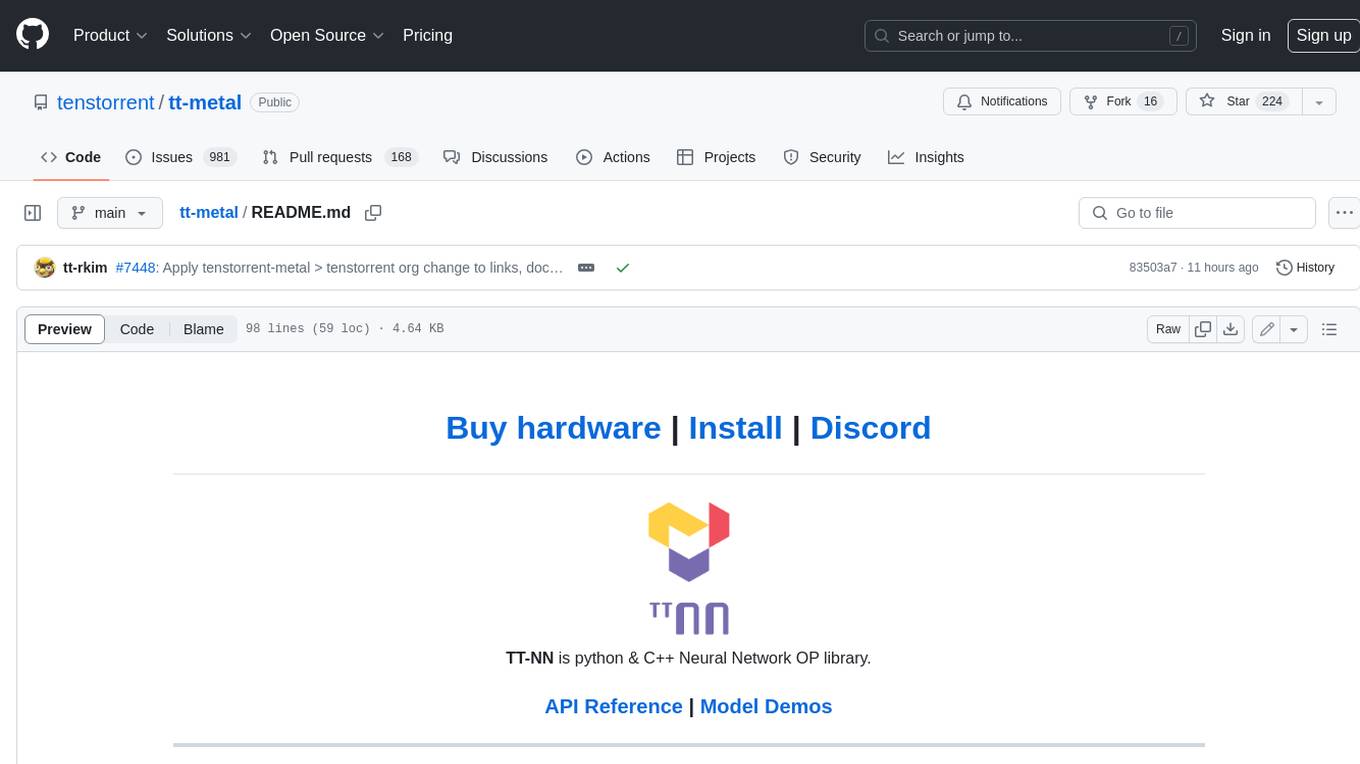
tt-metal
TT-NN is a python & C++ Neural Network OP library. It provides a low-level programming model, TT-Metalium, enabling kernel development for Tenstorrent hardware.
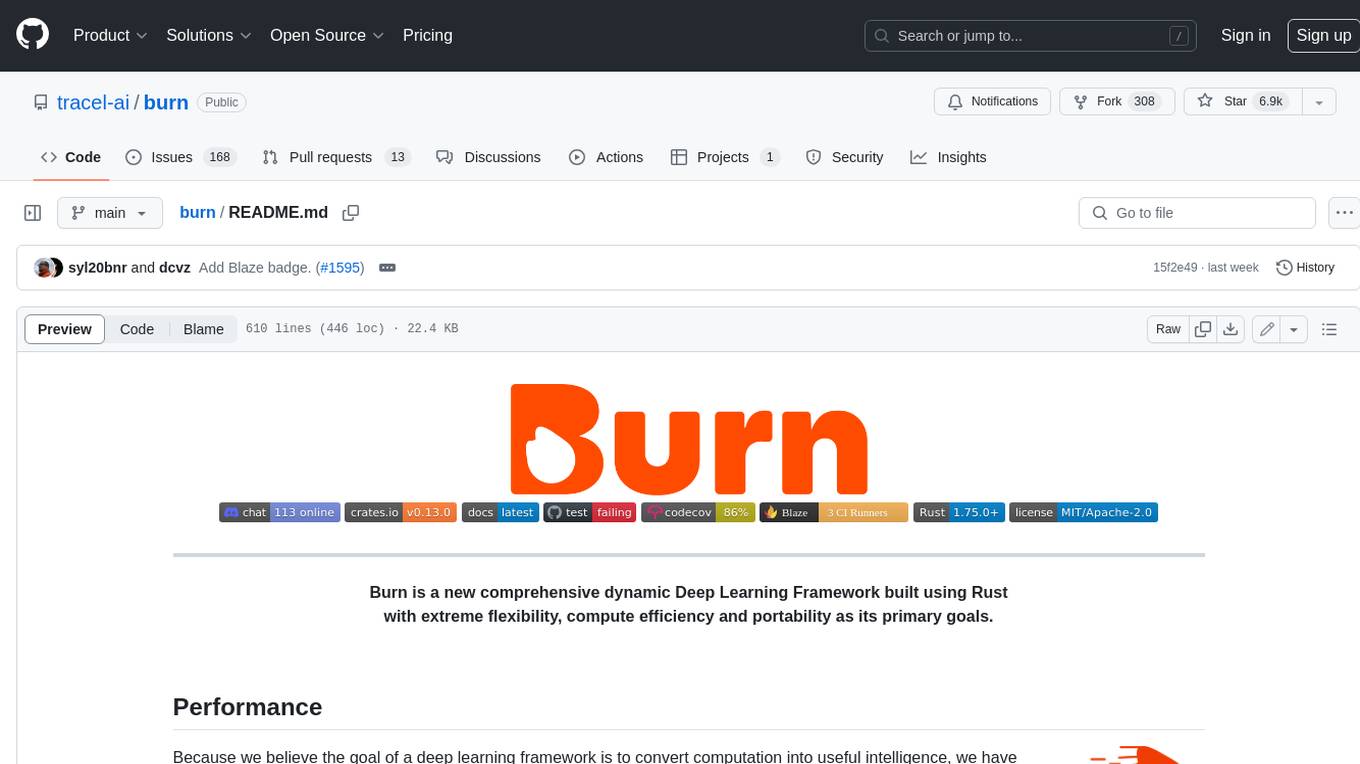
burn
Burn is a new comprehensive dynamic Deep Learning Framework built using Rust with extreme flexibility, compute efficiency and portability as its primary goals.
For similar jobs
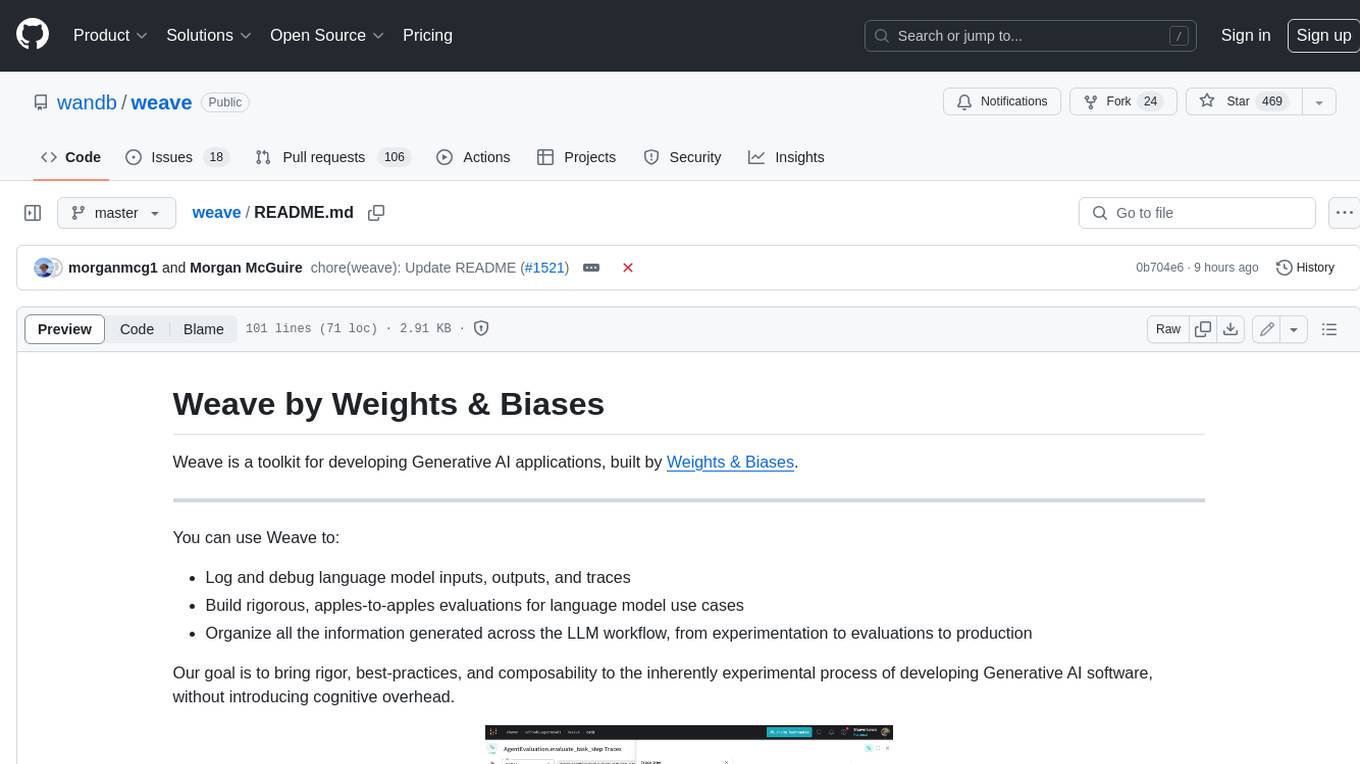
weave
Weave is a toolkit for developing Generative AI applications, built by Weights & Biases. With Weave, you can log and debug language model inputs, outputs, and traces; build rigorous, apples-to-apples evaluations for language model use cases; and organize all the information generated across the LLM workflow, from experimentation to evaluations to production. Weave aims to bring rigor, best-practices, and composability to the inherently experimental process of developing Generative AI software, without introducing cognitive overhead.
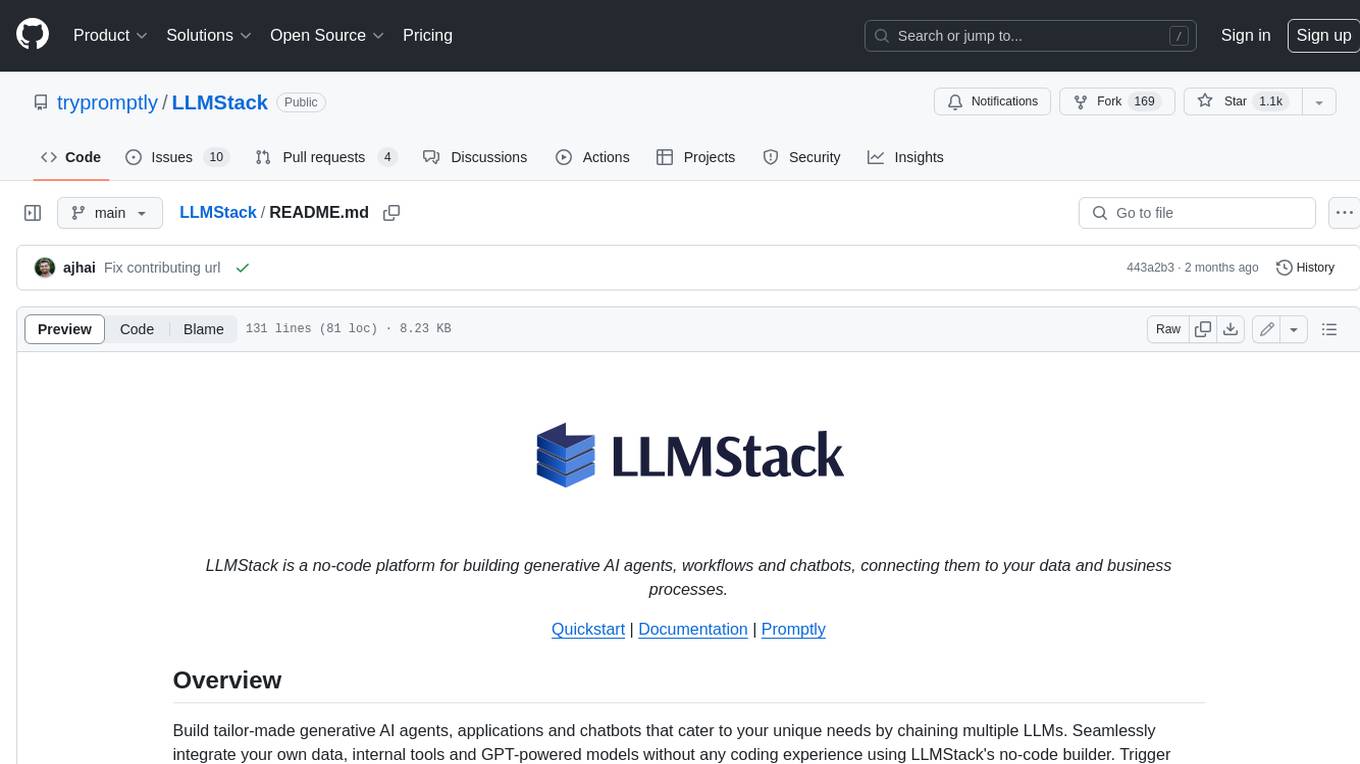
LLMStack
LLMStack is a no-code platform for building generative AI agents, workflows, and chatbots. It allows users to connect their own data, internal tools, and GPT-powered models without any coding experience. LLMStack can be deployed to the cloud or on-premise and can be accessed via HTTP API or triggered from Slack or Discord.
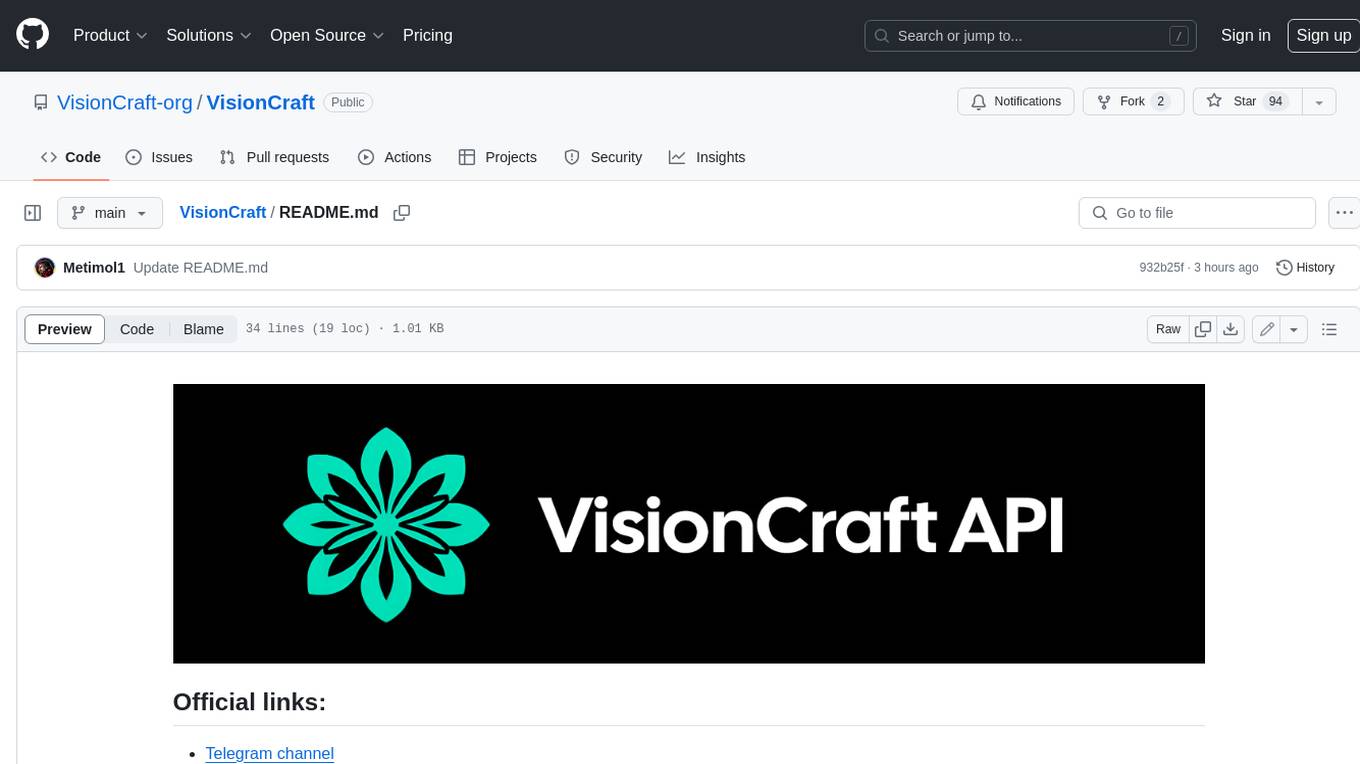
VisionCraft
The VisionCraft API is a free API for using over 100 different AI models. From images to sound.
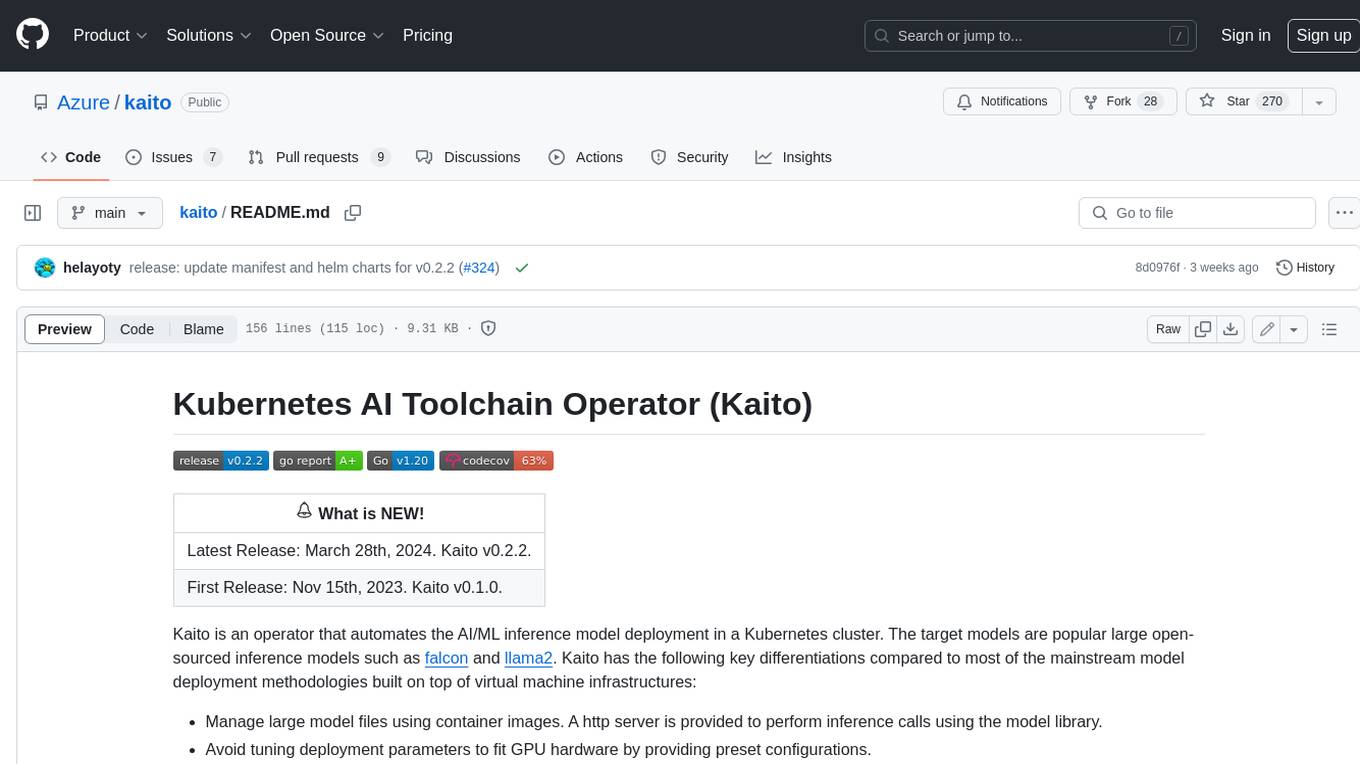
kaito
Kaito is an operator that automates the AI/ML inference model deployment in a Kubernetes cluster. It manages large model files using container images, avoids tuning deployment parameters to fit GPU hardware by providing preset configurations, auto-provisions GPU nodes based on model requirements, and hosts large model images in the public Microsoft Container Registry (MCR) if the license allows. Using Kaito, the workflow of onboarding large AI inference models in Kubernetes is largely simplified.
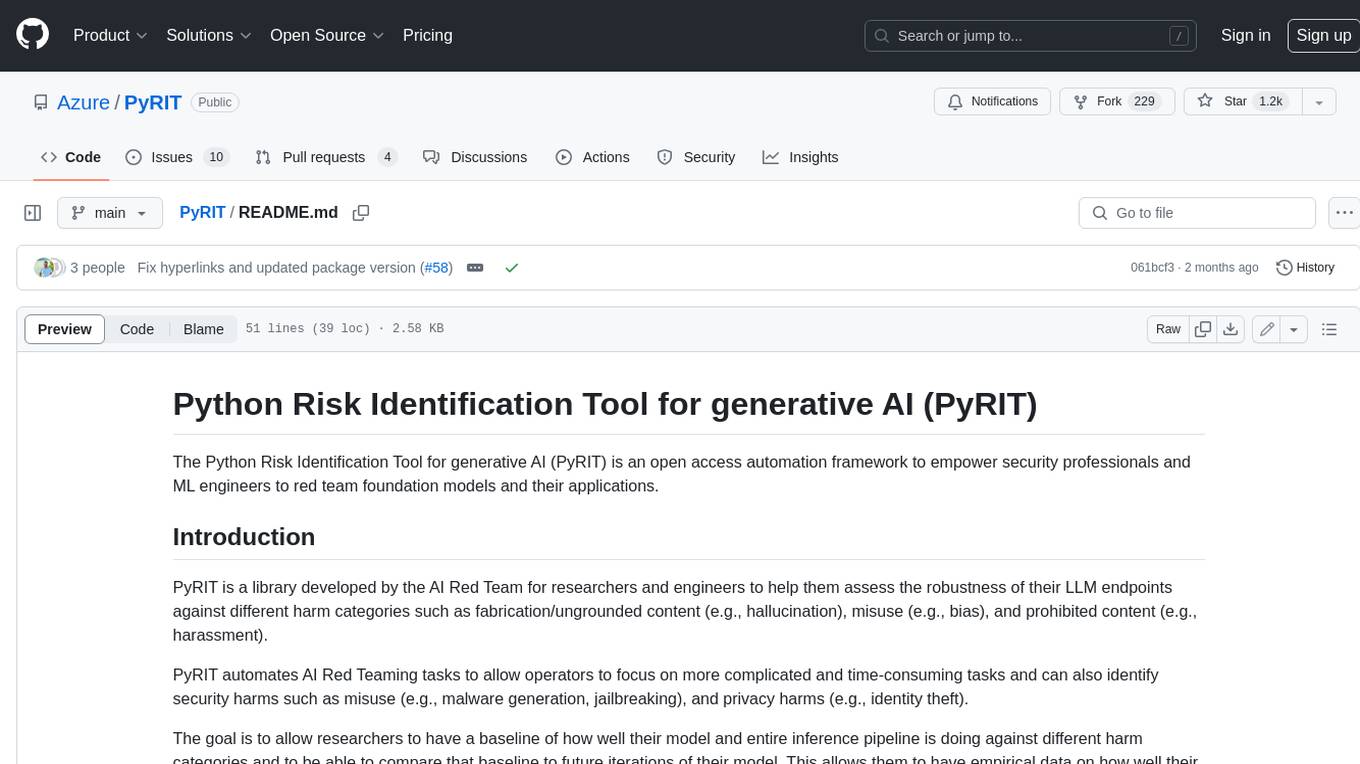
PyRIT
PyRIT is an open access automation framework designed to empower security professionals and ML engineers to red team foundation models and their applications. It automates AI Red Teaming tasks to allow operators to focus on more complicated and time-consuming tasks and can also identify security harms such as misuse (e.g., malware generation, jailbreaking), and privacy harms (e.g., identity theft). The goal is to allow researchers to have a baseline of how well their model and entire inference pipeline is doing against different harm categories and to be able to compare that baseline to future iterations of their model. This allows them to have empirical data on how well their model is doing today, and detect any degradation of performance based on future improvements.
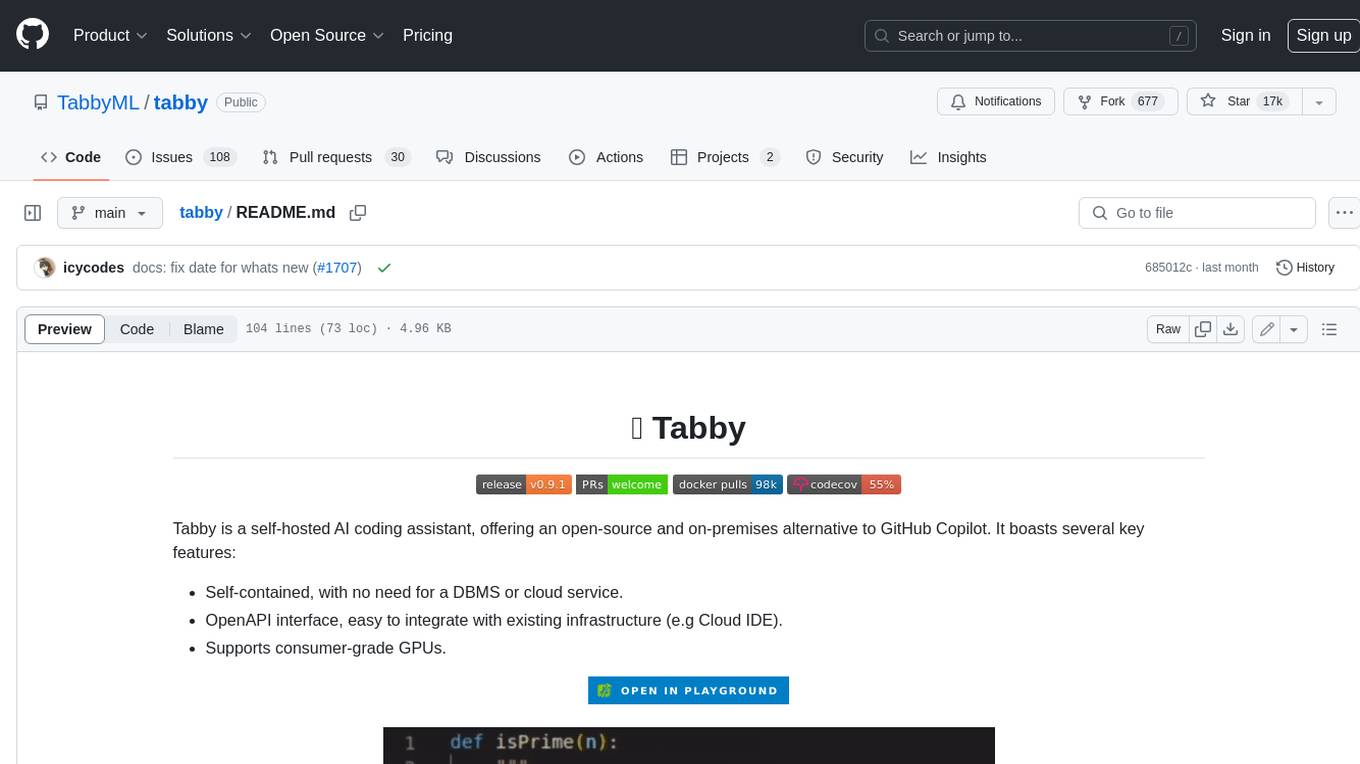
tabby
Tabby is a self-hosted AI coding assistant, offering an open-source and on-premises alternative to GitHub Copilot. It boasts several key features: * Self-contained, with no need for a DBMS or cloud service. * OpenAPI interface, easy to integrate with existing infrastructure (e.g Cloud IDE). * Supports consumer-grade GPUs.
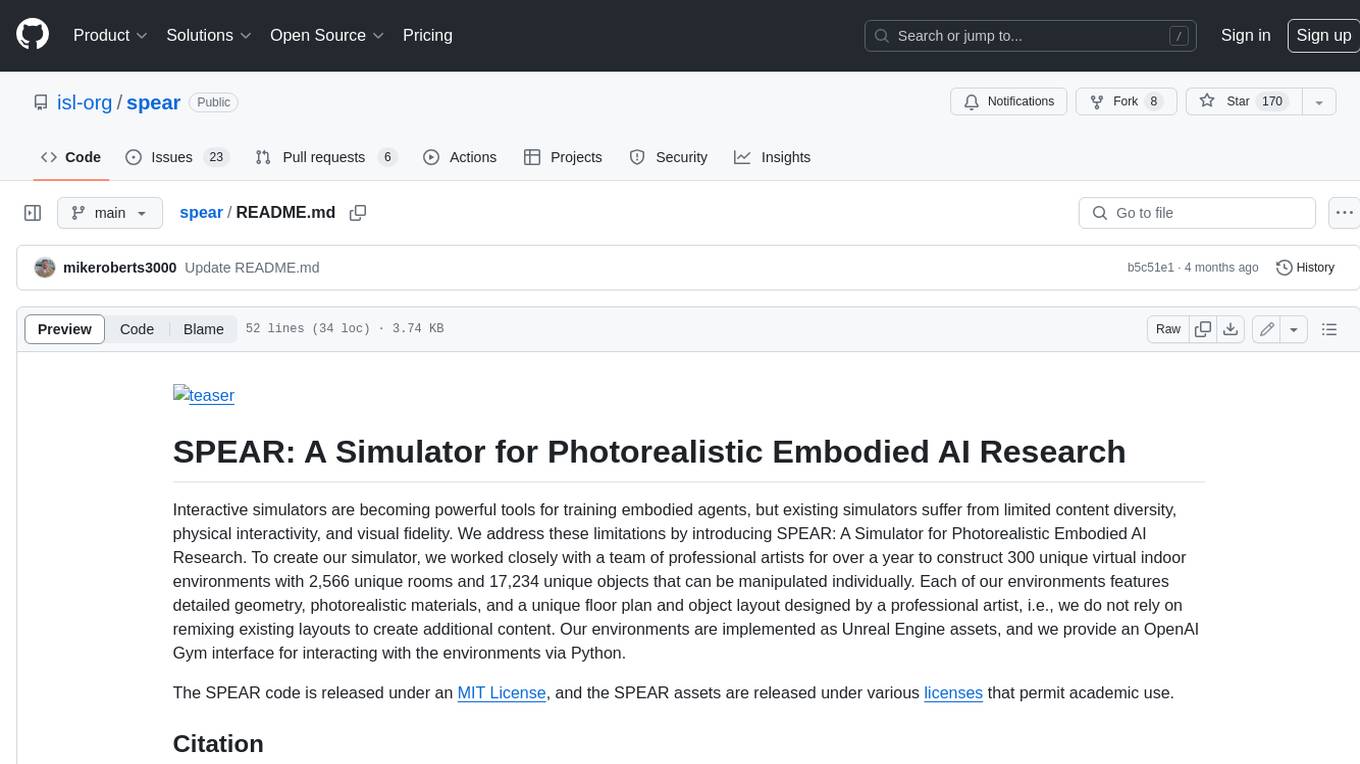
spear
SPEAR (Simulator for Photorealistic Embodied AI Research) is a powerful tool for training embodied agents. It features 300 unique virtual indoor environments with 2,566 unique rooms and 17,234 unique objects that can be manipulated individually. Each environment is designed by a professional artist and features detailed geometry, photorealistic materials, and a unique floor plan and object layout. SPEAR is implemented as Unreal Engine assets and provides an OpenAI Gym interface for interacting with the environments via Python.
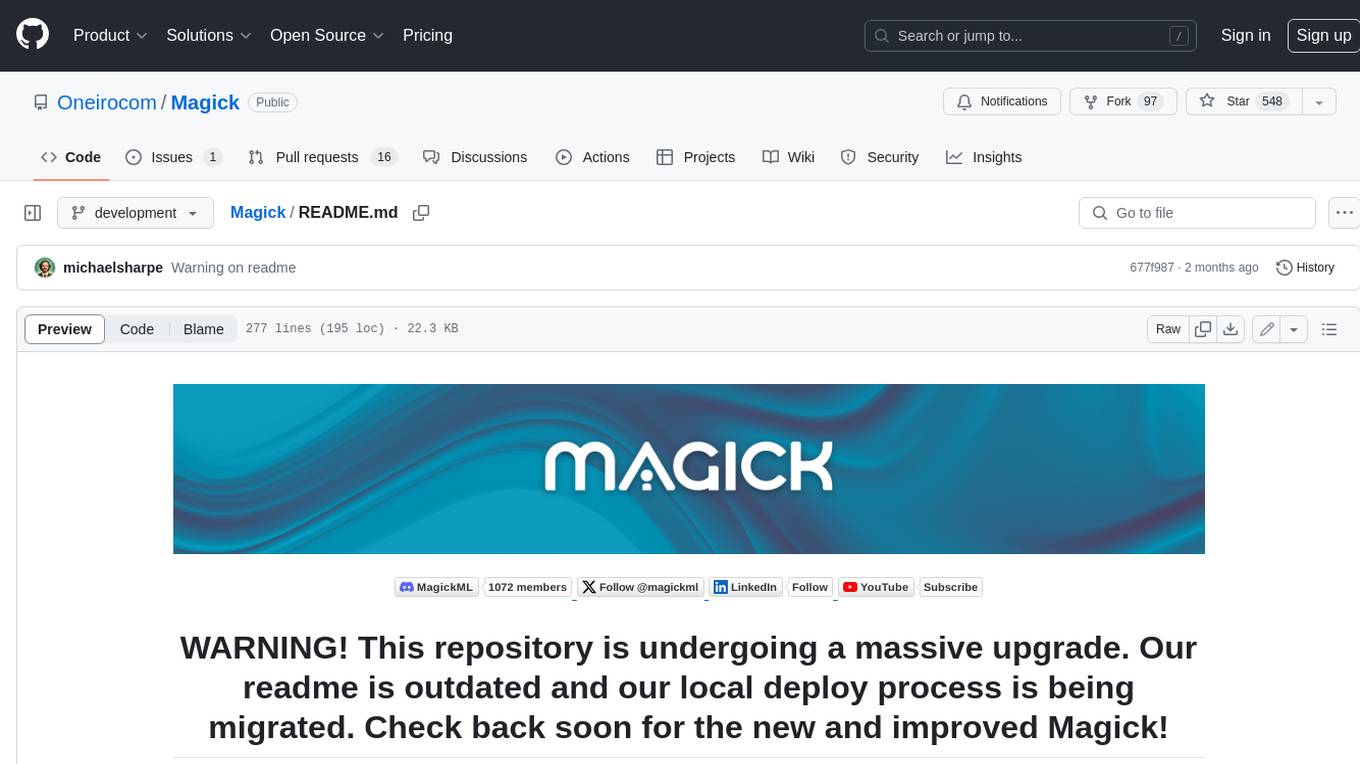
Magick
Magick is a groundbreaking visual AIDE (Artificial Intelligence Development Environment) for no-code data pipelines and multimodal agents. Magick can connect to other services and comes with nodes and templates well-suited for intelligent agents, chatbots, complex reasoning systems and realistic characters.