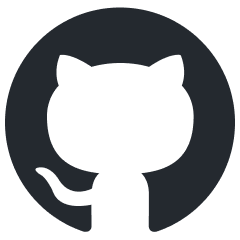
aici
AICI: Prompts as (Wasm) Programs
Stars: 1849
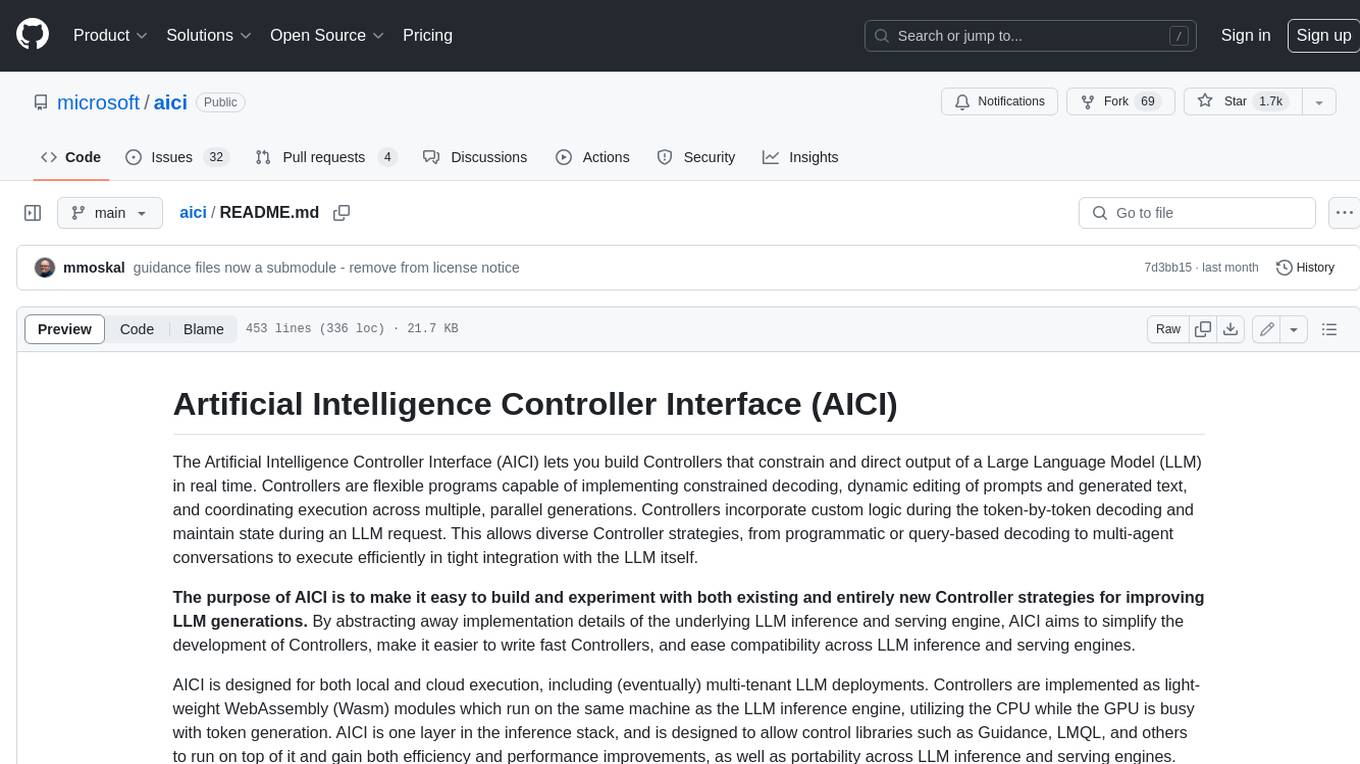
The Artificial Intelligence Controller Interface (AICI) lets you build Controllers that constrain and direct output of a Large Language Model (LLM) in real time. Controllers are flexible programs capable of implementing constrained decoding, dynamic editing of prompts and generated text, and coordinating execution across multiple, parallel generations. Controllers incorporate custom logic during the token-by-token decoding and maintain state during an LLM request. This allows diverse Controller strategies, from programmatic or query-based decoding to multi-agent conversations to execute efficiently in tight integration with the LLM itself.
README:
The Artificial Intelligence Controller Interface (AICI) lets you build Controllers that constrain and direct output of a Large Language Model (LLM) in real time. Controllers are flexible programs capable of implementing constrained decoding, dynamic editing of prompts and generated text, and coordinating execution across multiple, parallel generations. Controllers incorporate custom logic during the token-by-token decoding and maintain state during an LLM request. This allows diverse Controller strategies, from programmatic or query-based decoding to multi-agent conversations to execute efficiently in tight integration with the LLM itself.
The purpose of AICI is to make it easy to build and experiment with both existing and entirely new Controller strategies for improving LLM generations. By abstracting away implementation details of the underlying LLM inference and serving engine, AICI aims to simplify the development of Controllers, make it easier to write fast Controllers, and ease compatibility across LLM inference and serving engines.
AICI is designed for both local and cloud execution, including (eventually) multi-tenant LLM deployments. Controllers are implemented as light-weight WebAssembly (Wasm) modules which run on the same machine as the LLM inference engine, utilizing the CPU while the GPU is busy with token generation. AICI is one layer in the inference stack, and is designed to allow control libraries such as Guidance, LMQL, and others to run on top of it and gain both efficiency and performance improvements, as well as portability across LLM inference and serving engines.
AICI currently integrates with llama.cpp, HuggingFace Transformers, and rLLM (custom tch-based LLM inference engine), with vLLM in the works.
AICI is:
- Flexible: Controllers can be written in any language that can compile to Wasm (Rust, C, C++, ...), or be interpreted inside Wasm (Python, JavaScript, ...)
- Secure: Controllers are sandboxed and cannot access the filesystem, network, or any other resources
- Fast: Wasm modules are compiled to native code and run in parallel with the LLM inference engine, inducing only a minimal overhead to the generation process
AICI is a prototype, designed and built at Microsoft Research.
- Artificial Intelligence Controller Interface (AICI)
- QuickStart: Example Walkthrough
- Comprehensive Guide: Exploring Further
- Architecture
- Security
- Performance
- Flexibility
- Acknowledgements
- Contributing
- Trademarks
In this quickstart, we'll guide you through the following steps:
- Set up rLLM Server and AICI Runtime.
- Build and deploy a Controller.
- Use AICI to control LLM output, so you can customize a LLM to follow specific rules when generating text.
To compile AICI components, you need to set up your development environment for Rust. For this quickstart you also need Python 3.11 or later to create a controller.
[!NOTE] Windows users: please use WSL2 or the included devcontainer. Adding native Windows support is tracked here.
MacOS users: please make sure you have XCode command line tools installed by running
xcode-select -p
and, if not installed, runxcode-select --install
.CUDA: the CUDA build relies on specific libtorch installation. It's highly recommended you use the included devcontainer.
If you're using devcontainer, you can skip to the next section.
Using the system package manager, install the necessary tools for building code in the repository, including git
, cmake
and ccache
.
For instance in WSL / Ubuntu using apt
:
sudo apt-get install --assume-yes --no-install-recommends \
build-essential cmake ccache pkg-config libssl-dev libclang-dev clang llvm-dev git-lfs
or using Homebrew on macOS:
brew install git cmake ccache
Then install Rust, Rustup and Cargo, following the instructions provided here and here:
curl --proto '=https' --tlsv1.2 -sSf https://sh.rustup.rs | sh
After installation, verify that the rustup --version
command is accessible by running it from the terminal. If the command isn't recognized, try opening a new terminal session.
Next install wasm32-wasi Rust component:
rustup target add wasm32-wasi
If you already had Rust installed, or are getting complaints from Cargo about outdated versions, run:
rustup update
Last, to work with Python controllers and scripts (like this tutorial), run this command to install the required packages:
pip install pytest pytest-forked ujson posix_ipc numpy requests
The rLLM server has two backends, one based on libtorch
and CUDA
(rllm-cuda
), and the other based on llama.cpp
(rllm-llamacpp
).
The rllm-cuda
backend only works with NVidia GPUs with compute capability 8.0 or later
(A100 and later; RTX 30x0 and later) and requires a fiddly setup of libtorch
-- it's strongly recommended to use the included devcontainer.
While this guide focuses on the rllm-llamacpp
backend,
the build steps are the same for rllm-cuda
, modulo the folder name.
After dev env setup above, clone the AICI repository and proceed with the next steps outlined below.
Use the following command to build and run aicirt
and rllm-llamacpp
:
cd rllm/rllm-llamacpp
./server.sh phi2
You can pass other model names as argument (run ./server.sh
without arguments to see available models).
You can also use a HuggingFace URL to .gguf
file or a local path to a .gguf
file.
(For rllm-cuda
use HuggingFace model id or path to folder).
./server.sh orca
You can find more details about rllm-llamacpp
here.
The rLLM server provides a HTTP interface, utilized for configuration tasks and processing requests. You can also use this interface to promptly verify its status. For instance, if you open http://127.0.0.1:4242/v1/models, you should see:
{
"object": "list",
"data": [
{
"object": "model",
"id": "TheBloke/phi-2-GGUF",
"created": 946810800,
"owned_by": "owner"
}
]
}
confirming that the selected model is loaded.
AICI allows hosting custom logic, called Controllers, that initiate, terminate, and interact with LLMs token generation. Controllers take input arguments, process them, and return a result with logs, LLM tokens, and variables.
The repository includes some examples, in particular:
- jsctrl: a controller that accepts JavaScript code as input for execution. This code can interact with the model to generate text and tokens.
- pyctrl: a controller that accepts Python code as input for execution. This code can also interact with the model to generate text and tokens.
In this example we'll utilize pyctrl to manage token generation using a simple Python script. If you want, you can build and upload pyctrl, however by default the server will automatically download the latest release of pyctrl from GitHub.
In general, controllers require building and deployment, while scripts (Python or JavaScript) are sent with each request.
The following illustrates the relationship between the rLLM server, the AICI runtime, and the controller:
erDiagram
Host ||--|{ CPU : ""
Host ||--|{ GPU : ""
CPU ||--|| "rLLM Server" : execute
CPU ||--|{ "AICI Runtime" : execute
"AICI Runtime" ||--|| "Controller" : instantiate
GPU ||--|{ "LLM token generation" : execute
Suppose we aim for a model to generate a list, adhering to a specific format and containing only five items.
Typically, achieving this involves prompt engineering, crafting the prompt precisely with clear instructions, such as:
What are the five most popular types of vehicles?
Return the result as a numbered list.
Do not add explanations, only the list.
The prompt would also vary depending on the model in use, given that each model tends to add explanations and understands instructions in different ways.
With AICI, we shift control back to code, and we can simplify the prompt to:
What are the most popular types of vehicles?
using code to:
- Limit the list to 5 items
- Prevent the model from adding some initial explanation
- Format to a numbered list
- Stop the model from adding some text after the list.
Let's create a list-of-five.py
python file with the following content:
import pyaici.server as aici
# Force the model to generate a well formatted list of 5 items, e.g.
# 1. name 1
# 2. name 2
# 3. name 3
# 4. name 4
# 5. name 5
async def main():
# This is the prompt we want to run.
# Note how the prompt doesn't mention a number of vehicles or how to format the result.
prompt = "What are the most popular types of vehicles?\n"
# Tell the model to generate the prompt string, ie. let's start with the prompt "to complete"
await aici.FixedTokens(prompt)
# Store the current position in the token generation process
marker = aici.Label()
for i in range(1,6):
# Tell the model to generate the list number
await aici.FixedTokens(f"{i}.")
# Wait for the model to generate a vehicle name and end with a new line
await aici.gen_text(stop_at = "\n")
await aici.FixedTokens("\n")
# Store the tokens generated in a result variable
aici.set_var("result", marker.text_since())
aici.start(main())
Running the script is not too different from sending a prompt. In this case, we're sending control logic and instructions all together.
To see the final result, execute the following command:
./aici.sh run list-of-five.py
Result:
Running with tagged AICI Controller: gh:microsoft/aici/pyctrl
[0]: FIXED 'What are the most popular types of vehicles?\n'
[0]: FIXED '1.'
[0]: GEN ' Cars\n'
[0]: FIXED '2.'
[0]: GEN ' Motorcycles\n'
[0]: FIXED '3.'
[0]: GEN ' Bicycles\n'
[0]: FIXED '4.'
[0]: GEN ' Trucks\n'
[0]: FIXED '5.'
[0]: GEN ' Boats\n'
[0]: FIXED '\n'
[DONE]
[Response] What are the most popular types of vehicles?
1. Cars
2. Motorcycles
3. Bicycles
4. Trucks
5. Boats
response saved to tmp/response.json
Usage: {'sampled_tokens': 16, 'ff_tokens': 37, 'cost': 69}
Timing: {'http_response': 0.05193686485290527, 'data0': 0.05199289321899414, 'first_token': 0.0658726692199707, 'last_token': 0.1784682273864746}
Tokens/sec: {'prompt': 861.0913072488067, 'sampling': 89.65181217019571}
Storage: {'result': '1. Cars\n2. Motorcycles\n3. Bicycles\n4. Trucks\n5. Boats\n\n'}
This repository contains a number of components, and which ones you need depends on your use case.
You can use an existing controller module.
We provide PyCtrl and JsCtrl
that let you script controllers using server-side Python and JavaScript, respectively.
The pyaici package contains aici
command line tool that lets you
upload and run scripts with any controller
(we also provide REST API definition for the curious).
π§βπ»Python code samples for scripting PyCtrl and a JavaScript Hello World for JSCtrl
We anticipate libraries will be built on top of controllers. We provide an example in promptlib - a client-side Python library that generates interacts with DeclCtrl via the pyaici package.
π§βπ» Example notebook that uses PromptLib to interact with DeclCtrl.
The controllers can be run in a cloud or local AICI-enabled LLM inference engine. You can run the provided reference engine (rLLM) locally with either libtorch+CUDA or llama.cpp backend.
To develop a new controller, use a Rust starter project that shows usage of aici_abi library, which simplifies implementing the low-level AICI interface.
π§βπ»Sample code for a minimal new controller to get you started
To add AICI support to a new LLM inference engine, you will need to implement LLM-side of the protocol that talks to AICI runtime.
Finally, you may want to modify any of the provided components - PRs are most welcome!
AICI abstracts LLM inference engine from the controller and vice-versa, as in the picture below. The rounded nodes are aspirational. Additional layers can be built on top - we provide promptlib, but we strongly believe that Guidance, LMQL, SGLang, Outlines, jsonformer, LMFE, etc. can also run on top of AICI (either with custom controllers or utilizing PyCtrl or JsCtrl).
graph TD
PyCtrl -- AICI --> aicirt[AICI-runtime]
JsCtrl -- AICI --> aicirt
guidance([GuidanceCtrl]) -- AICI --> aicirt
lmql([LMQL Ctrl]) -- AICI --> aicirt
aicirt -- POSIX SHM --> rLLM
aicirt -- POSIX SHM --> llama[llama.cpp]
aicirt -- POSIX SHM --> pyaici
pyaici -- Python --> vLLM(vLLM)
pyaici -- Python --> hf[HF Transformers]
The pyaici package makes it easier to integrate AICI with Python-based LLM inference engines. Take a look at integration with HuggingFace Transformers, though note that it doesn't support forking (generation of multiple sequences in parallel). The vLLM REST server is currently out of date. Please use the rLLM-cuda or rLLM-llama.cpp for now.
-
aicirt
runs in a separate process, and can run under a different user than the LLM engine - Wasm modules are sandboxed by Wasmtime
- Wasm only have access to
aici_host_*
functions, implemented in hostimpl.rs -
aicirt
also exposes a partial WASI interface; however almost all the functions are no-op, except forfd_write
which shims file descriptors 1 and 2 (stdout and stderr) to print debug messages - each Wasm module runs in a separate process, helping with Spectre/Meltdown mitigation and allowing limits on CPU usage
In particular, Wasm modules cannot access the filesystem, network, or any other resources. They also cannot spin threads or access any timers (this is relevant for Spectre/Meltdown attacks).
Most of computation in AICI Controllers occurs on the CPU, in parallel with the logit generation on the GPU. The generation occurs in steps, where logits are generated in parallel for a new token for each sequence in a batch (typically between 1 and 50). This involves reading the whole model and KV caches for sequences in the batch from the GPU memory. For optimal batch throughput, the model and KV caches should utilize a major fraction of the GPU memory, and reading the whole memory takes about 40ms on A100 GPU (80GB).
Thus, each step of generation takes on the order of 20-50ms. With careful engineering, this is more than enough to compute the set of allowed tokens in Rust compiled to Wasm. These can be combined either natively in Rust, or via Python or JavaScript interpreters we provide.
For example, computing allowed token set in the 32000-strong vocabulary of Llama model takes:
- about 2.0ms for Yacc grammar of the C programming language
- about 0.3ms for a regular expression
- about 0.2ms for a substring constraint, from 4kB string
The above numbers are for a single sequence, however each sequence is processed in separate process, and thus if there is more cores than sequences (which is typical), they do not change. They also include overhead of calling into Python interpreter implemented in Wasm, and then back into Rust-generated Wasm code for the constraint itself. They are all well within the 20-50ms budget, so do not affect the generation time at all.
There is also some overhead in the critical path of sampling. It comes down to about 0.3ms per generation step when executing 10 sequences in parallel (this is irrespective of the constraint used). The overhead goes up to around 0.7ms for 40 sequences (though it has not been fully optimized yet).
WebAssembly is designed to have minimal overhead, compared to native code. In our experience, highly optimized Rust code is less than 2x slower when run in Wasmtime than native. This is 10-100x better than JavaScript or Python.
All measurements done on AMD EPYC 7V13 with nVidia A100 GPU with 80GB of VRAM.
The low-level interface that AICI runtime provides allows for:
- interaction with the LLM inference engine before, during, and after every generated token
- constraining decoding to a set of tokens
- backtracking KV-cache to a previous state
- fast-forwarding several tokens at a time (if they are known)
- forking generation into multiple branches
- communication between forks via shared variables
- utility functions for converting between tokens and byte strings
It can be utilized from any language that compiles to Wasm.
This repository provides a Rust library that makes it easy to implement controllers in Rust, and provides efficient implementations of specific constraints (regular expressions, yacc grammars, substrings). We also provide Python and JavaScript interpreters that allow to glue these constraints together. All of these can be easily extended.
- Flash Attention kernels are copied from flash-attention repo; see BSD LICENSE
- Paged Attention kernels are copied from vLLM repo; see Apache LICENSE
- OpenAI API definitions are copied and modified from candle-vllm; see MIT LICENSE
- cache_engine.rs, config.rs, and scheduler.rs are loosely based on vLLM
- llama.rs, phi.rs and logits.rs are based on candle-transformers
- specific Python library files are copied from RustPython (as we only use a subset of them)
- the example ANSI C grammar is based on https://www.lysator.liu.se/c/ANSI-C-grammar-y.html by Jeff Lee (from 1985)
If you find the AI Controller Interface and its ideas for defining a new layer in the LLM inference stack useful, please cite the package using the following reference:
- Michal Moskal, Madan Musuvathi, Emre KΔ±cΔ±man. AI Controller Interface, (2024), GitHub repository. https://github.com/microsoft/aici
Bibtex:
@misc{Moskal2024,
author = {Moskal, Michal and Musuvathi, Madan and {K\i c\i man}, Emre},
title = {{AI Controller Interface}},
year = {2024},
publisher = {{GitHub}},
journal = {{GitHub} repository},
howpublished = {\url{https://github.com/microsoft/aici/}}
}
This project welcomes contributions and suggestions. Most contributions require you to agree to a Contributor License Agreement (CLA) declaring that you have the right to, and actually do, grant us the rights to use your contribution. For details, visit https://cla.opensource.microsoft.com.
When you submit a pull request, a CLA bot will automatically determine whether you need to provide a CLA and decorate the PR appropriately (e.g., status check, comment). Simply follow the instructions provided by the bot. You will only need to do this once across all repos using our CLA.
This project has adopted the Microsoft Open Source Code of Conduct. For more information see the Code of Conduct FAQ or contact [email protected] with any additional questions or comments.
This project may contain trademarks or logos for projects, products, or services. Authorized use of Microsoft trademarks or logos is subject to and must follow Microsoft's Trademark & Brand Guidelines. Use of Microsoft trademarks or logos in modified versions of this project must not cause confusion or imply Microsoft sponsorship. Any use of third-party trademarks or logos are subject to those third-party's policies.
For Tasks:
Click tags to check more tools for each tasksFor Jobs:
Alternative AI tools for aici
Similar Open Source Tools
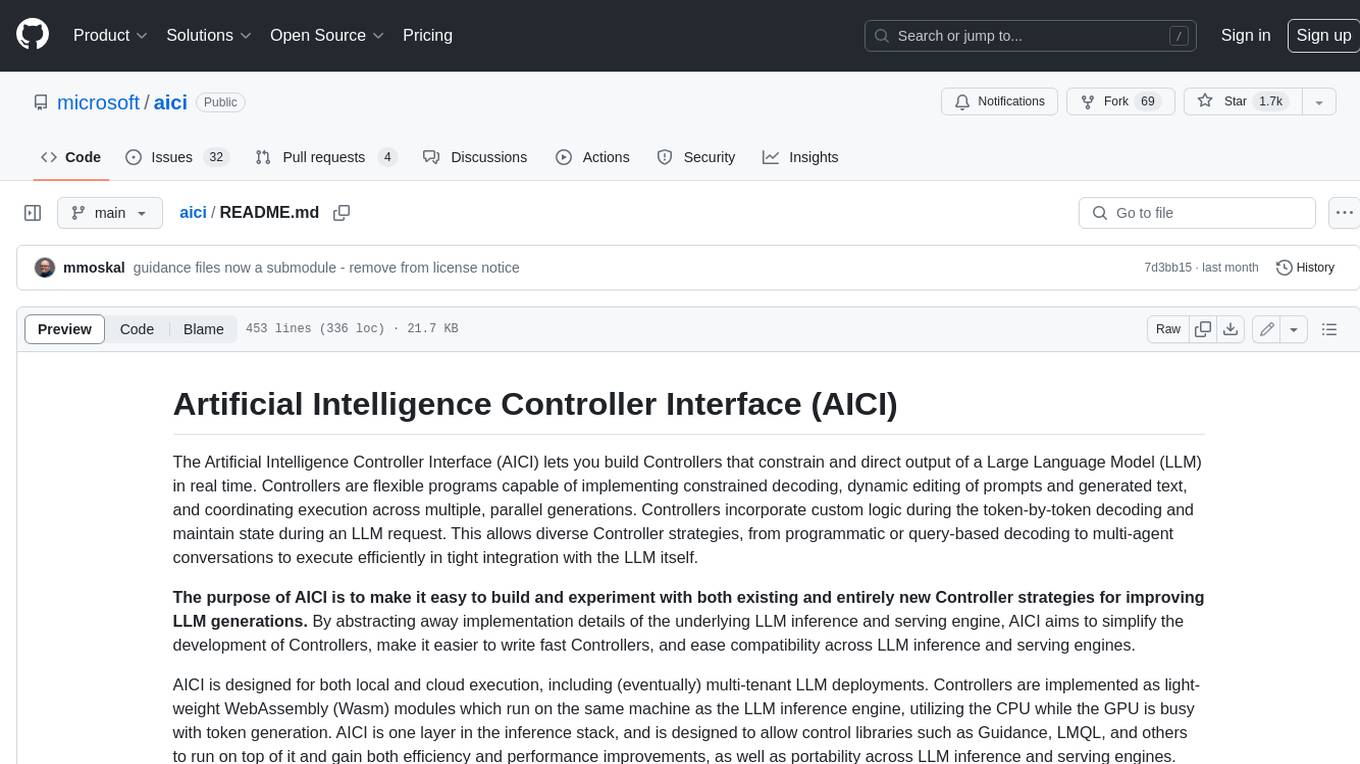
aici
The Artificial Intelligence Controller Interface (AICI) lets you build Controllers that constrain and direct output of a Large Language Model (LLM) in real time. Controllers are flexible programs capable of implementing constrained decoding, dynamic editing of prompts and generated text, and coordinating execution across multiple, parallel generations. Controllers incorporate custom logic during the token-by-token decoding and maintain state during an LLM request. This allows diverse Controller strategies, from programmatic or query-based decoding to multi-agent conversations to execute efficiently in tight integration with the LLM itself.
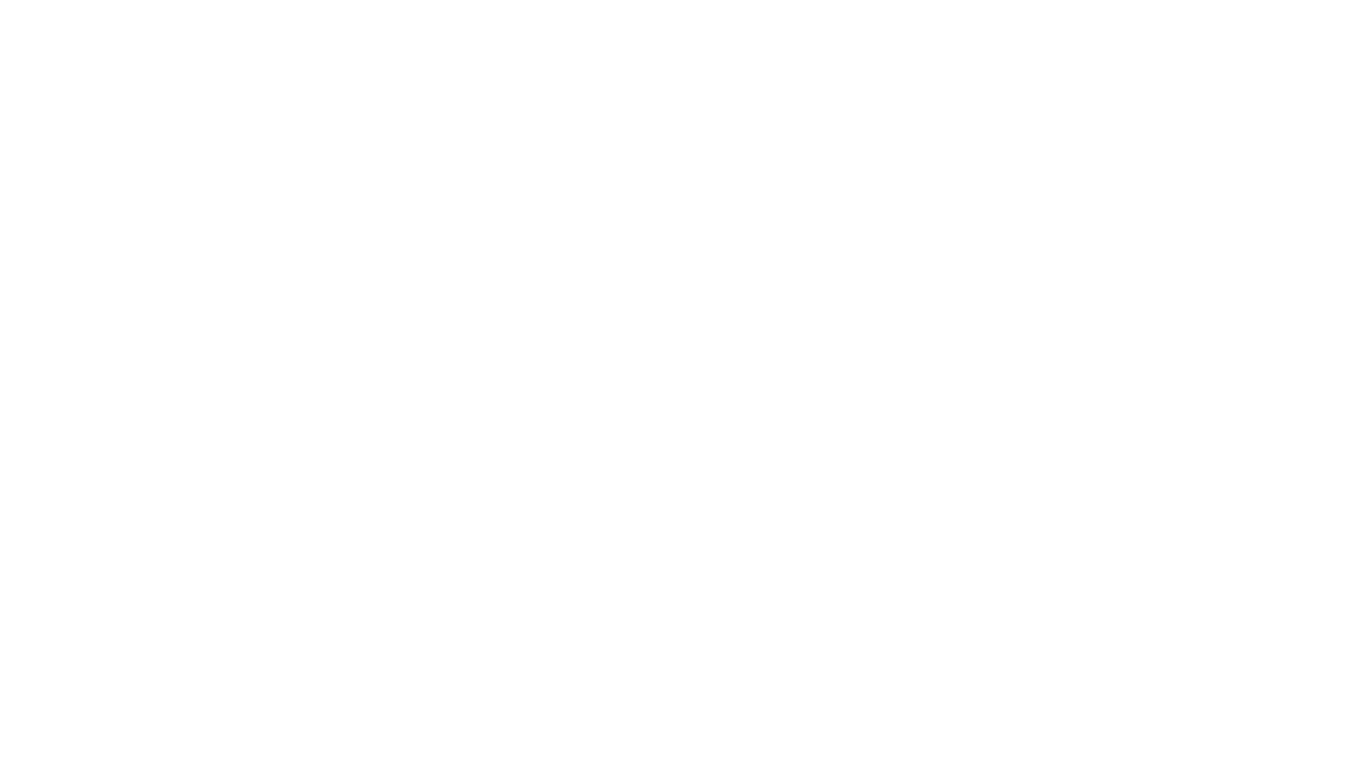
project_alice
Alice is an agentic workflow framework that integrates task execution and intelligent chat capabilities. It provides a flexible environment for creating, managing, and deploying AI agents for various purposes, leveraging a microservices architecture with MongoDB for data persistence. The framework consists of components like APIs, agents, tasks, and chats that interact to produce outputs through files, messages, task results, and URL references. Users can create, test, and deploy agentic solutions in a human-language framework, making it easy to engage with by both users and agents. The tool offers an open-source option, user management, flexible model deployment, and programmatic access to tasks and chats.
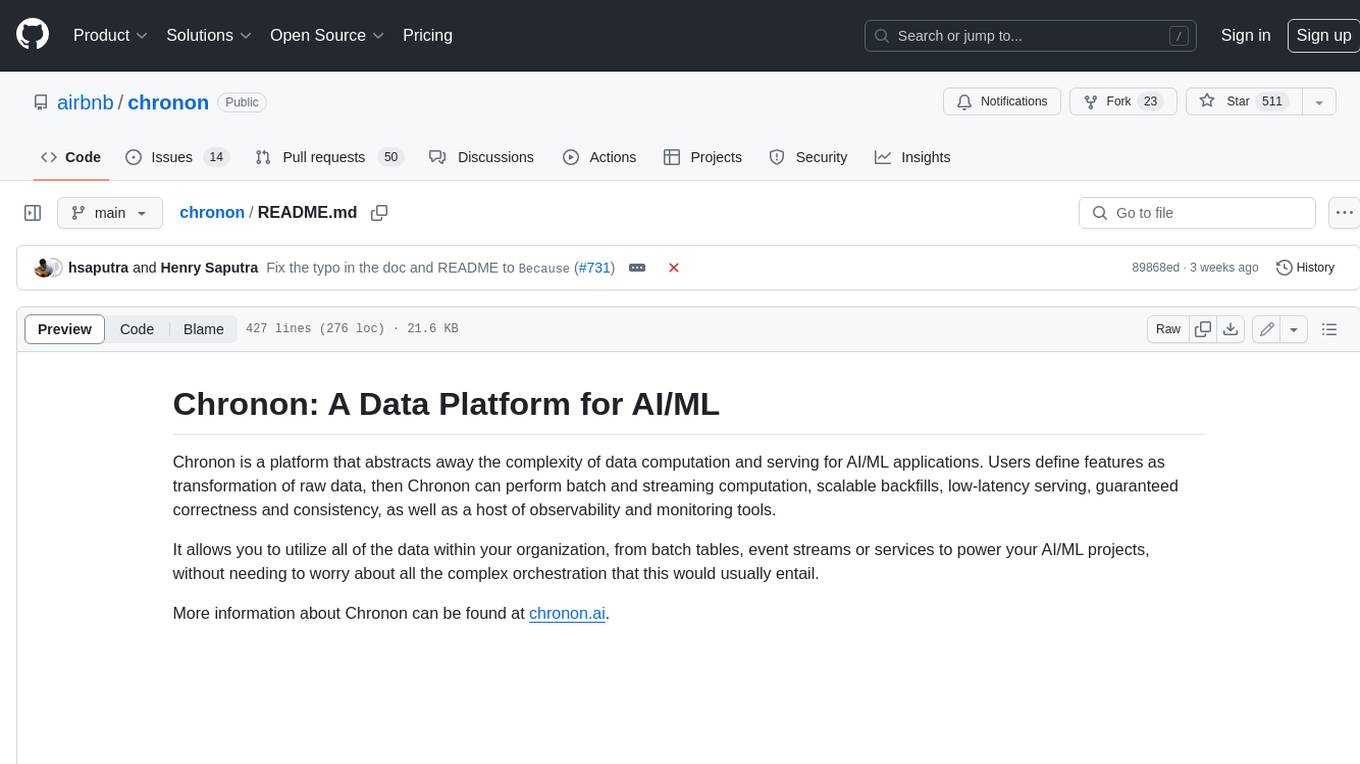
chronon
Chronon is a platform that simplifies and improves ML workflows by providing a central place to define features, ensuring point-in-time correctness for backfills, simplifying orchestration for batch and streaming pipelines, offering easy endpoints for feature fetching, and guaranteeing and measuring consistency. It offers benefits over other approaches by enabling the use of a broad set of data for training, handling large aggregations and other computationally intensive transformations, and abstracting away the infrastructure complexity of data plumbing.
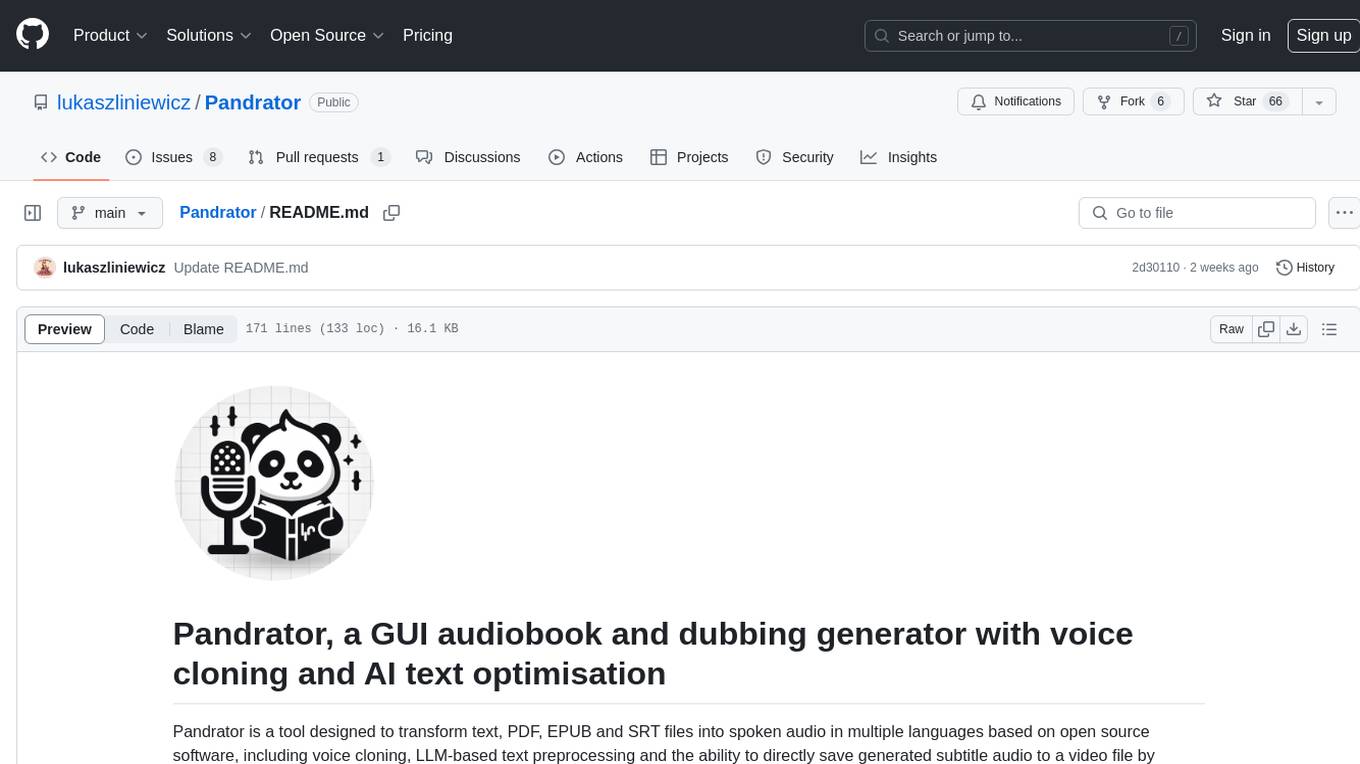
Pandrator
Pandrator is a GUI tool for generating audiobooks and dubbing using voice cloning and AI. It transforms text, PDF, EPUB, and SRT files into spoken audio in multiple languages. It leverages XTTS, Silero, and VoiceCraft models for text-to-speech conversion and voice cloning, with additional features like LLM-based text preprocessing and NISQA for audio quality evaluation. The tool aims to be user-friendly with a one-click installer and a graphical interface.
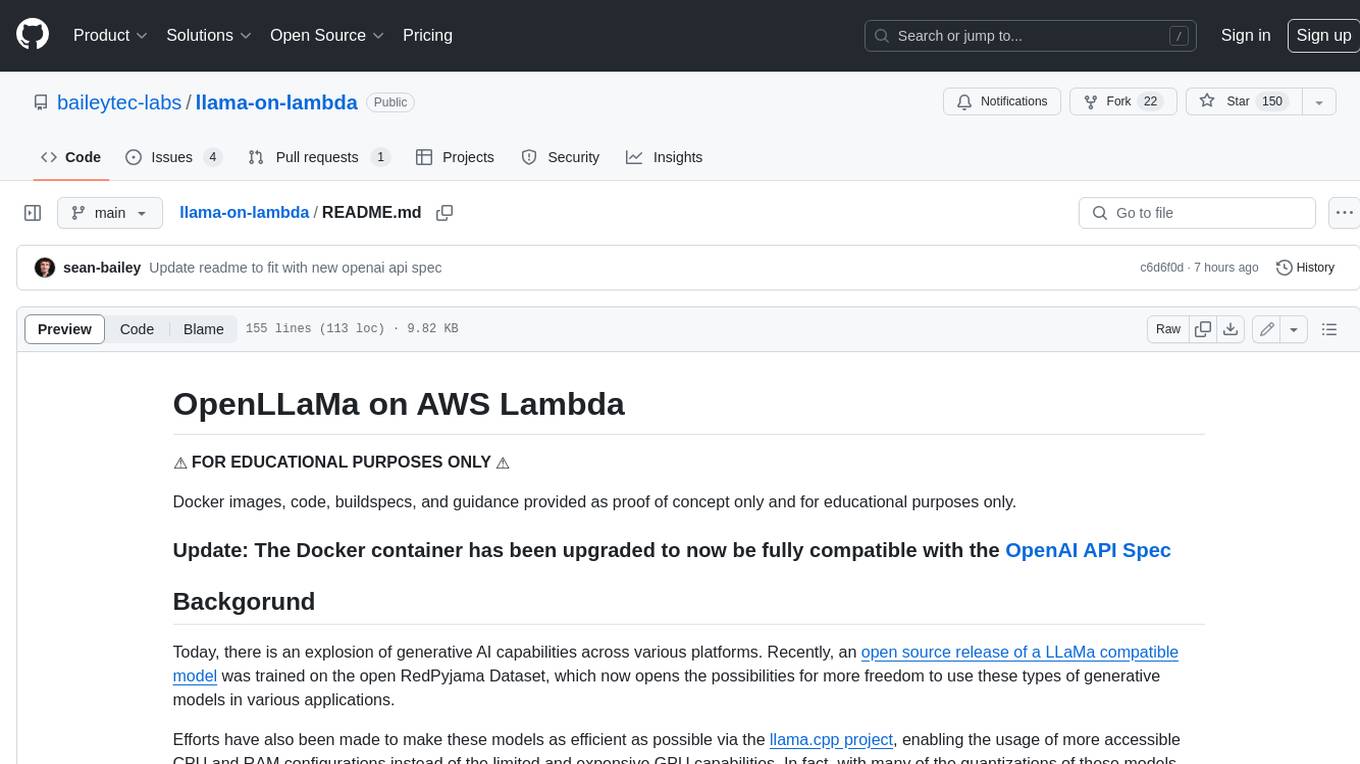
llama-on-lambda
This project provides a proof of concept for deploying a scalable, serverless LLM Generative AI inference engine on AWS Lambda. It leverages the llama.cpp project to enable the usage of more accessible CPU and RAM configurations instead of limited and expensive GPU capabilities. By deploying a container with the llama.cpp converted models onto AWS Lambda, this project offers the advantages of scale, minimizing cost, and maximizing compute availability. The project includes AWS CDK code to create and deploy a Lambda function leveraging your model of choice, with a FastAPI frontend accessible from a Lambda URL. It is important to note that you will need ggml quantized versions of your model and model sizes under 6GB, as your inference RAM requirements cannot exceed 9GB or your Lambda function will fail.
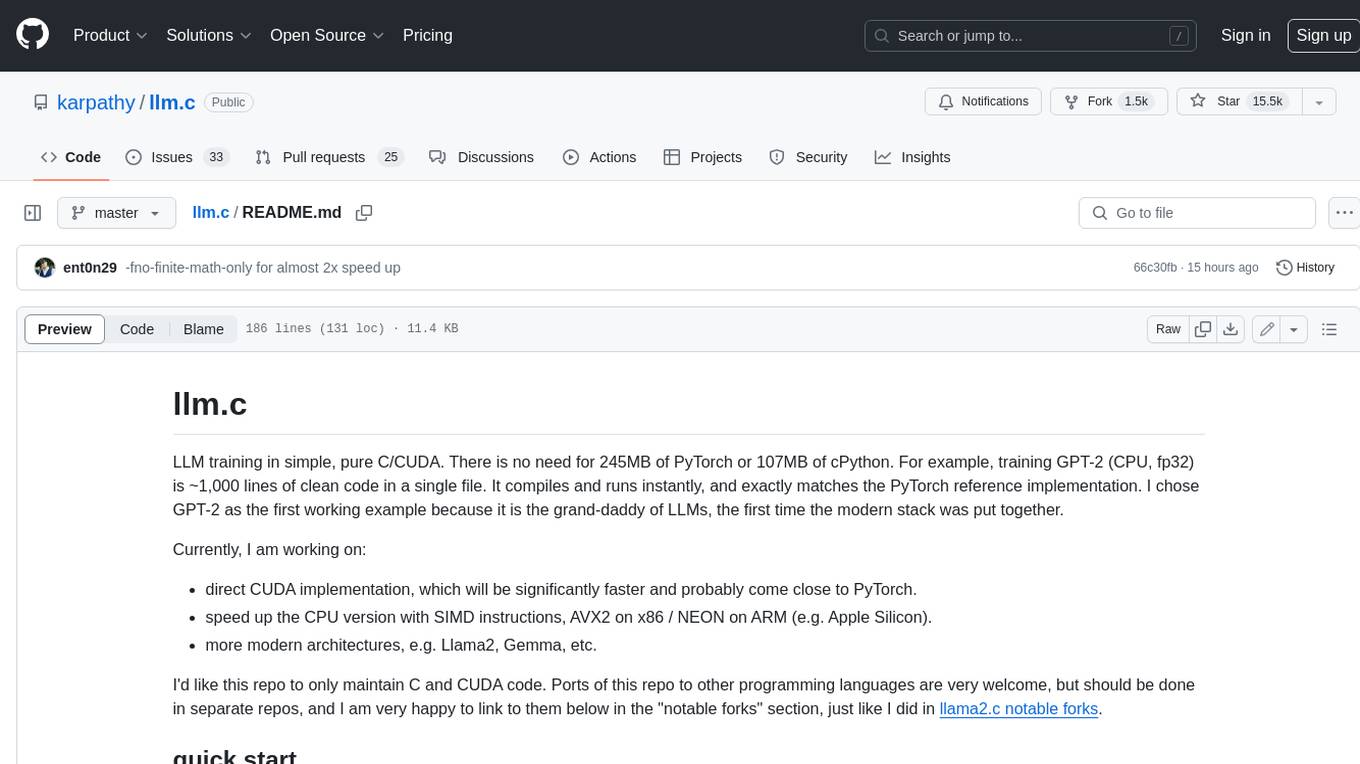
llm.c
LLM training in simple, pure C/CUDA. There is no need for 245MB of PyTorch or 107MB of cPython. For example, training GPT-2 (CPU, fp32) is ~1,000 lines of clean code in a single file. It compiles and runs instantly, and exactly matches the PyTorch reference implementation. I chose GPT-2 as the first working example because it is the grand-daddy of LLMs, the first time the modern stack was put together.
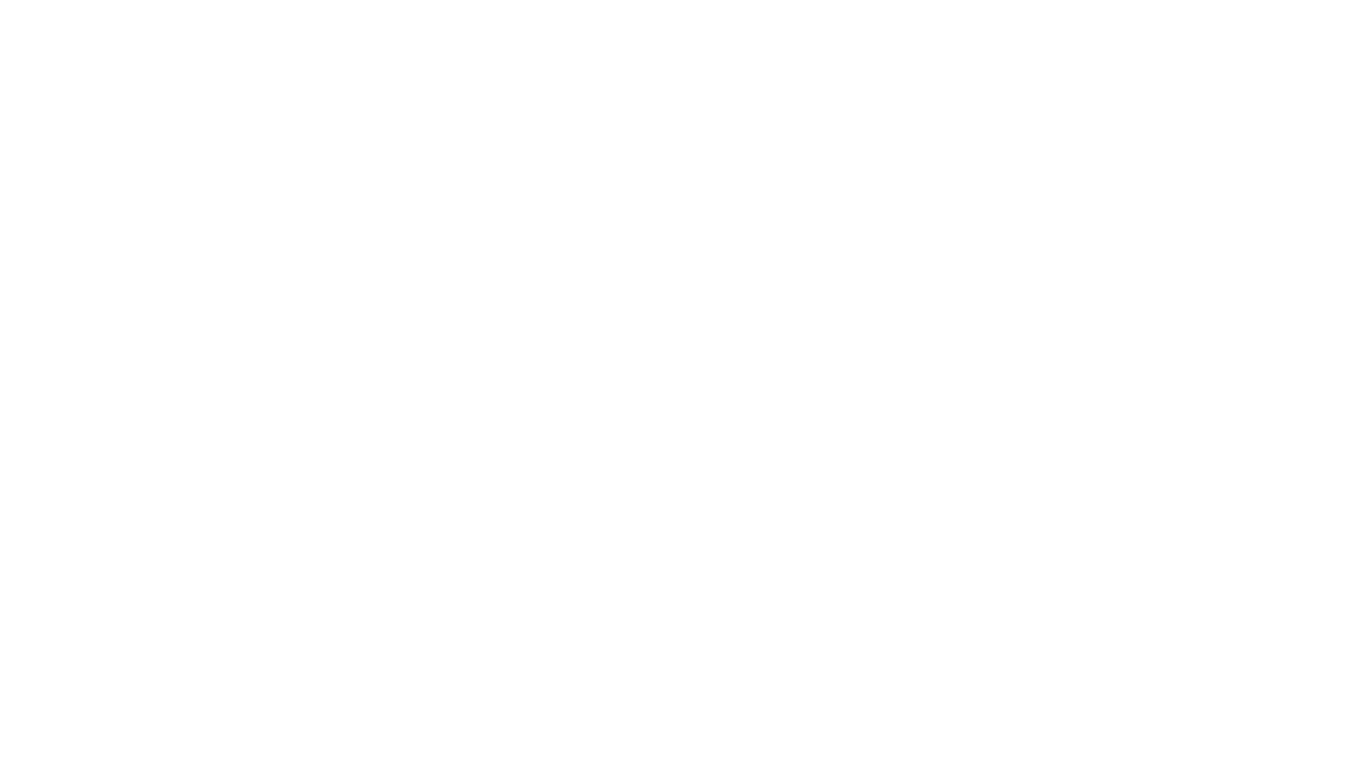
airflow
Apache Airflow (or simply Airflow) is a platform to programmatically author, schedule, and monitor workflows. When workflows are defined as code, they become more maintainable, versionable, testable, and collaborative. Use Airflow to author workflows as directed acyclic graphs (DAGs) of tasks. The Airflow scheduler executes your tasks on an array of workers while following the specified dependencies. Rich command line utilities make performing complex surgeries on DAGs a snap. The rich user interface makes it easy to visualize pipelines running in production, monitor progress, and troubleshoot issues when needed.
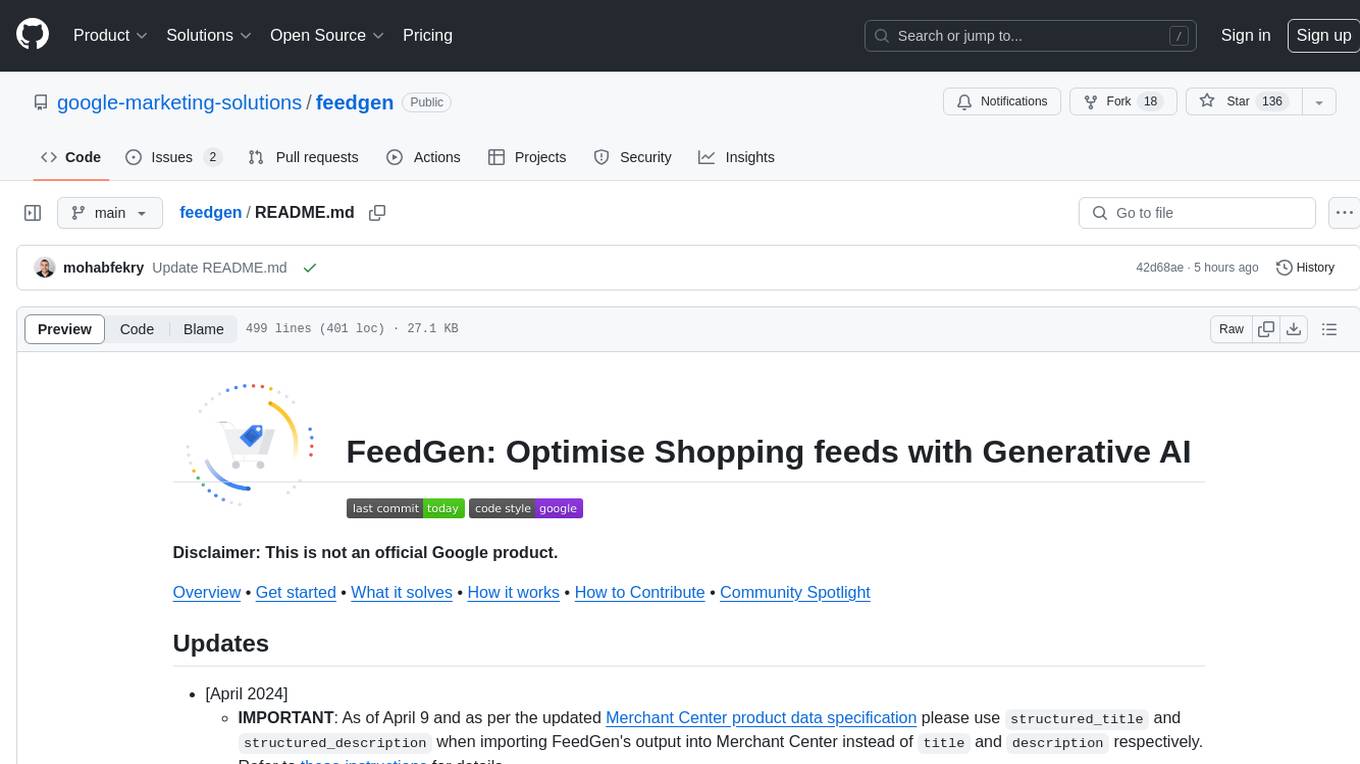
feedgen
FeedGen is an open-source tool that uses Google Cloud's state-of-the-art Large Language Models (LLMs) to improve product titles, generate more comprehensive descriptions, and fill missing attributes in product feeds. It helps merchants and advertisers surface and fix quality issues in their feeds using Generative AI in a simple and configurable way. The tool relies on GCP's Vertex AI API to provide both zero-shot and few-shot inference capabilities on GCP's foundational LLMs. With few-shot prompting, users can customize the model's responses towards their own data, achieving higher quality and more consistent output. FeedGen is an Apps Script based application that runs as an HTML sidebar in Google Sheets, allowing users to optimize their feeds with ease.
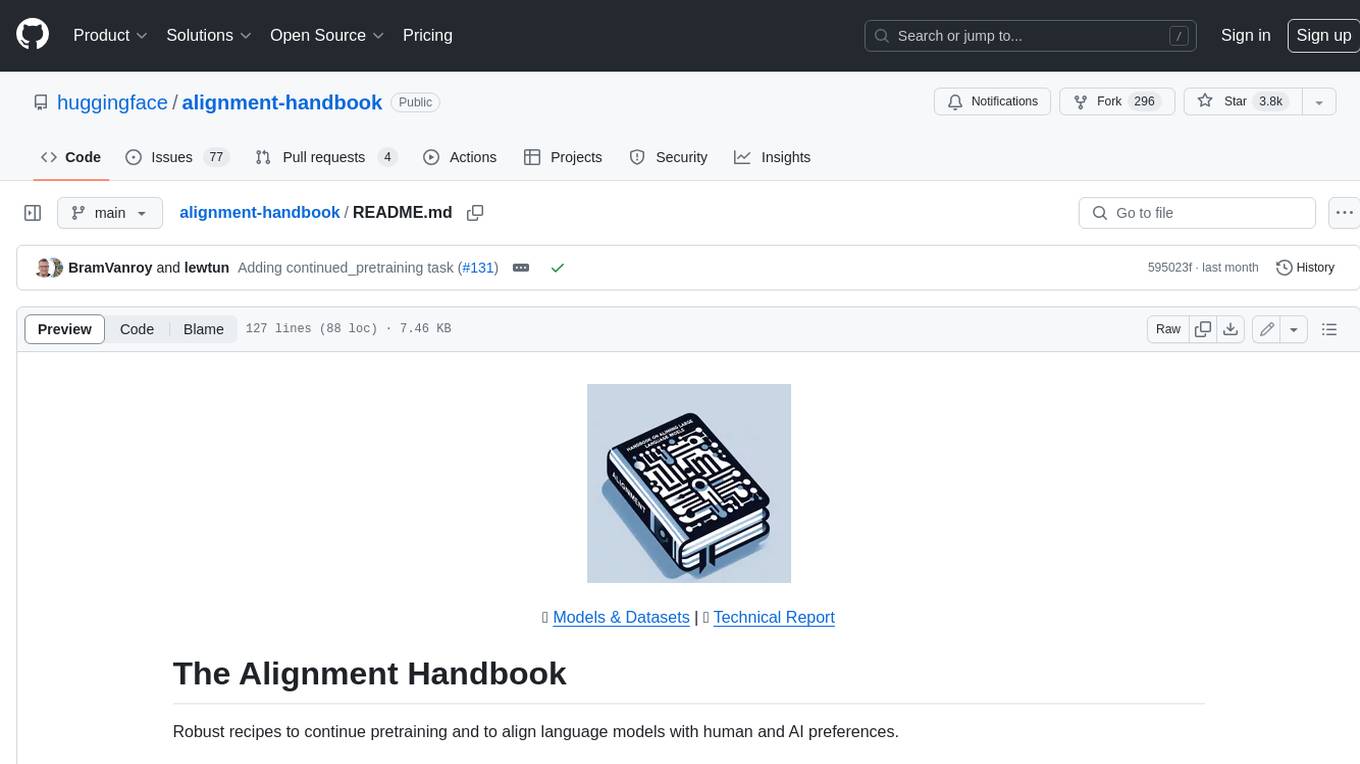
alignment-handbook
The Alignment Handbook provides robust training recipes for continuing pretraining and aligning language models with human and AI preferences. It includes techniques such as continued pretraining, supervised fine-tuning, reward modeling, rejection sampling, and direct preference optimization (DPO). The handbook aims to fill the gap in public resources on training these models, collecting data, and measuring metrics for optimal downstream performance.
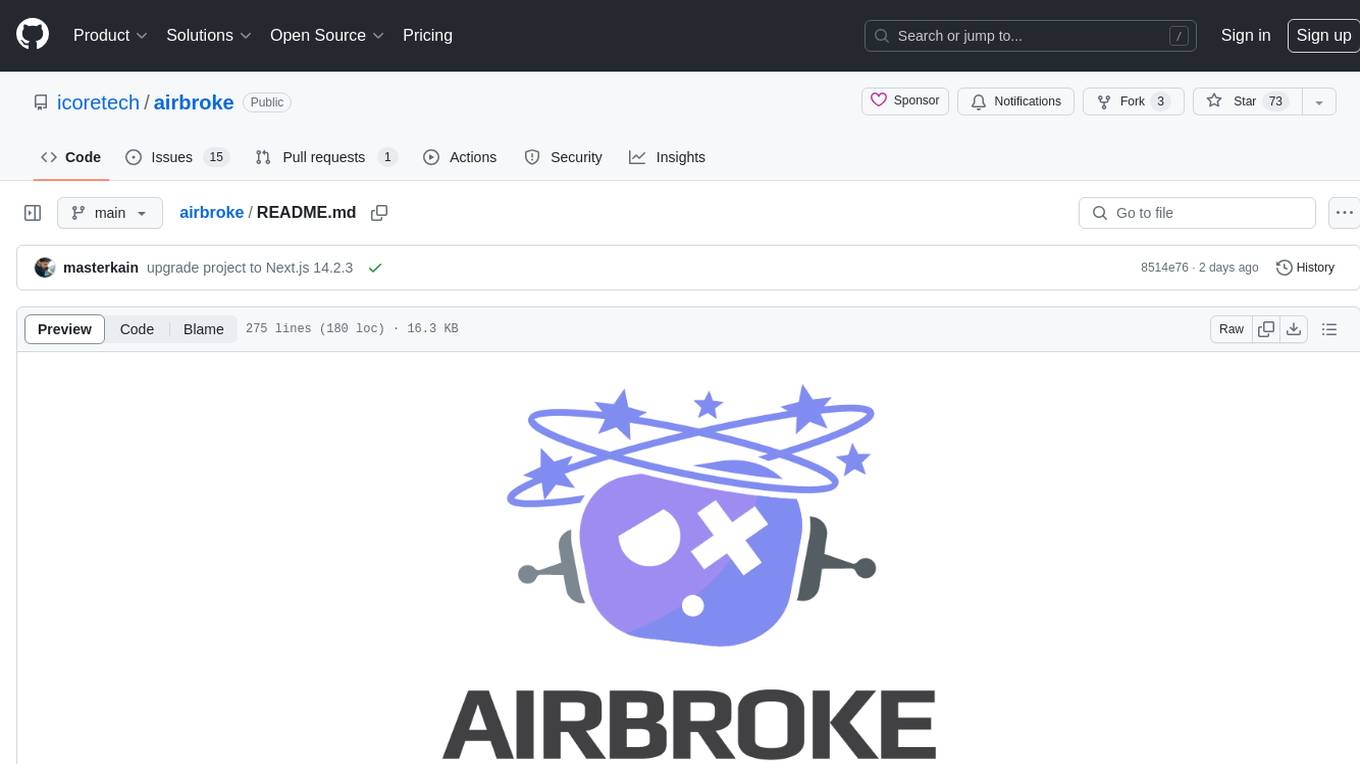
airbroke
Airbroke is an open-source error catcher tool designed for modern web applications. It provides a PostgreSQL-based backend with an Airbrake-compatible HTTP collector endpoint and a React-based frontend for error management. The tool focuses on simplicity, maintaining a small database footprint even under heavy data ingestion. Users can ask AI about issues, replay HTTP exceptions, and save/manage bookmarks for important occurrences. Airbroke supports multiple OAuth providers for secure user authentication and offers occurrence charts for better insights into error occurrences. The tool can be deployed in various ways, including building from source, using Docker images, deploying on Vercel, Render.com, Kubernetes with Helm, or Docker Compose. It requires Node.js, PostgreSQL, and specific system resources for deployment.
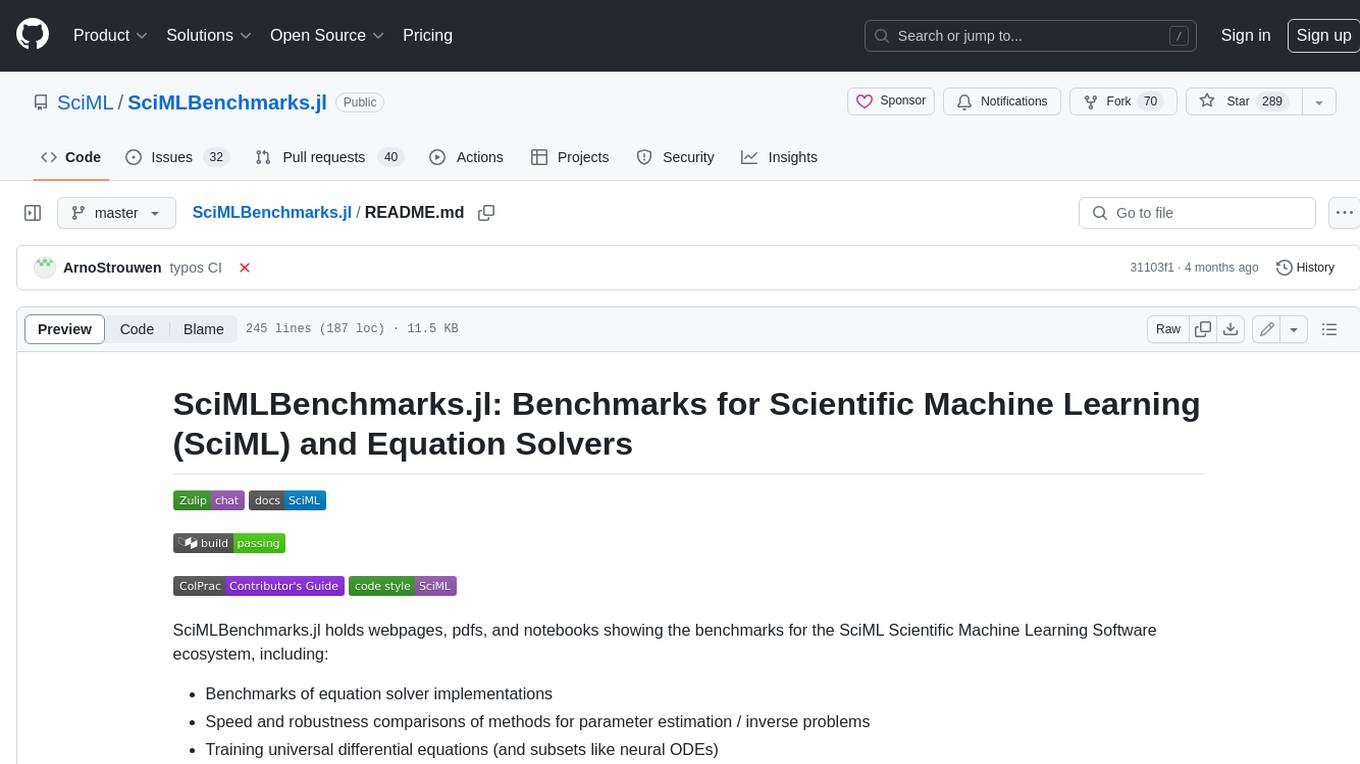
SciMLBenchmarks.jl
SciMLBenchmarks.jl holds webpages, pdfs, and notebooks showing the benchmarks for the SciML Scientific Machine Learning Software ecosystem, including: * Benchmarks of equation solver implementations * Speed and robustness comparisons of methods for parameter estimation / inverse problems * Training universal differential equations (and subsets like neural ODEs) * Training of physics-informed neural networks (PINNs) * Surrogate comparisons, including radial basis functions, neural operators (DeepONets, Fourier Neural Operators), and more The SciML Bench suite is made to be a comprehensive open source benchmark from the ground up, covering the methods of computational science and scientific computing all the way to AI for science.
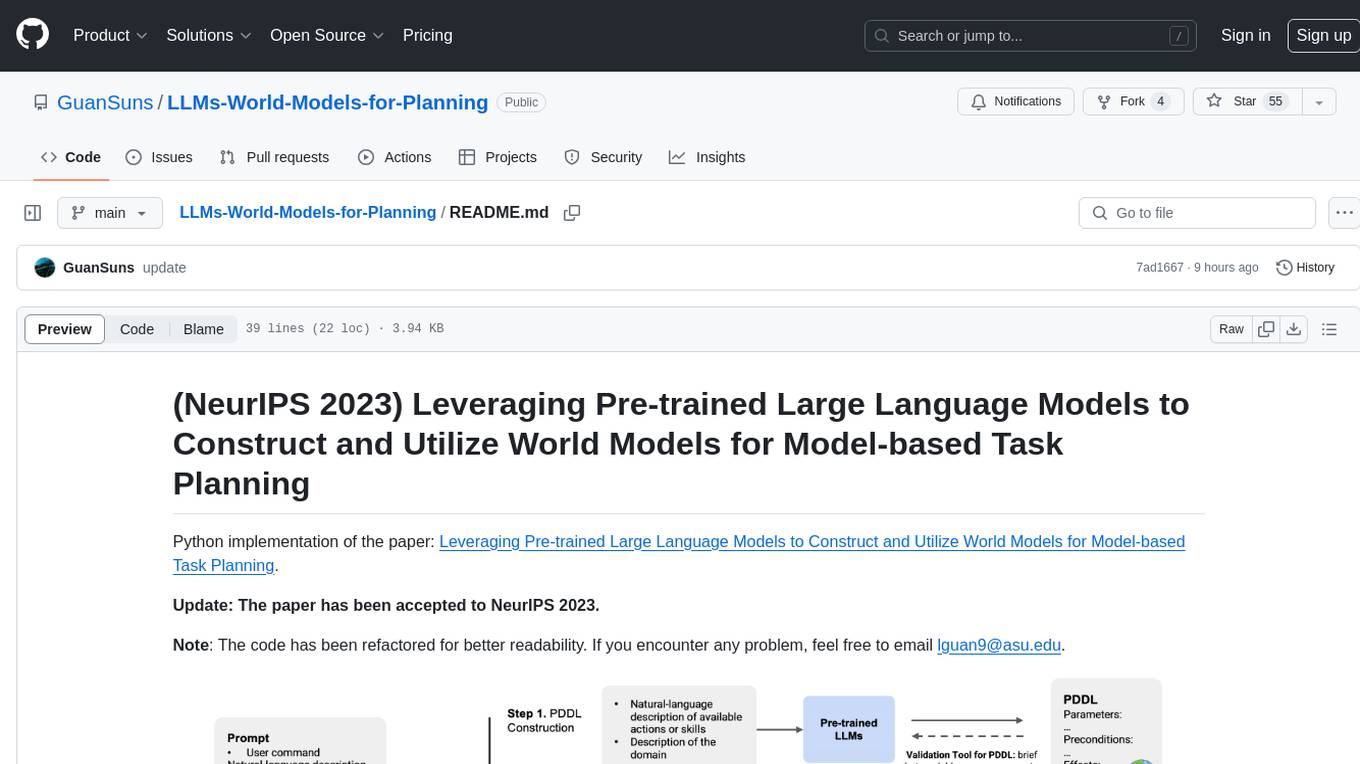
LLMs-World-Models-for-Planning
This repository provides a Python implementation of a method that leverages pre-trained large language models to construct and utilize world models for model-based task planning. It includes scripts to generate domain models using natural language descriptions, correct domain models based on feedback, and support plan generation for tasks in different domains. The code has been refactored for better readability and includes tools for validating PDDL syntax and handling corrective feedback.
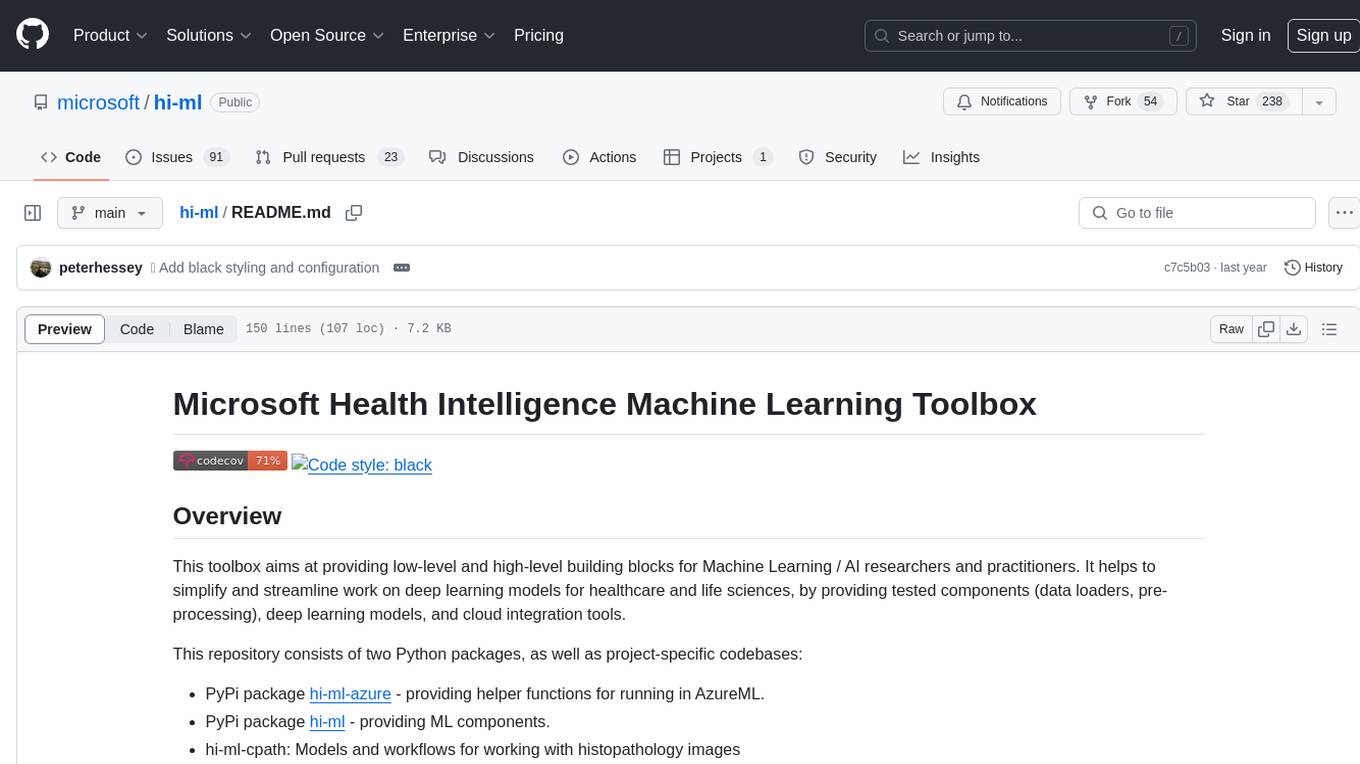
hi-ml
The Microsoft Health Intelligence Machine Learning Toolbox is a repository that provides low-level and high-level building blocks for Machine Learning / AI researchers and practitioners. It simplifies and streamlines work on deep learning models for healthcare and life sciences by offering tested components such as data loaders, pre-processing tools, deep learning models, and cloud integration utilities. The repository includes two Python packages, 'hi-ml-azure' for helper functions in AzureML, 'hi-ml' for ML components, and 'hi-ml-cpath' for models and workflows related to histopathology images.
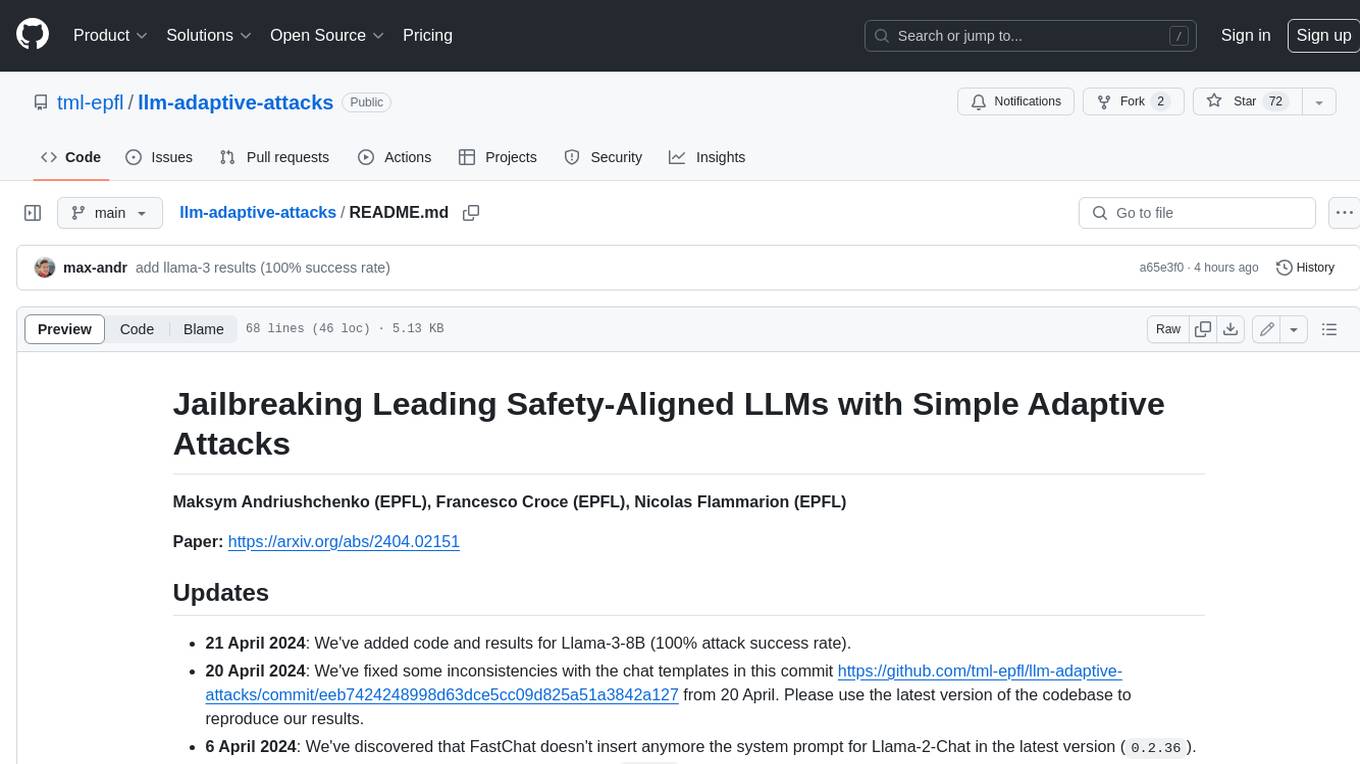
llm-adaptive-attacks
This repository contains code and results for jailbreaking leading safety-aligned LLMs with simple adaptive attacks. We show that even the most recent safety-aligned LLMs are not robust to simple adaptive jailbreaking attacks. We demonstrate how to successfully leverage access to logprobs for jailbreaking: we initially design an adversarial prompt template (sometimes adapted to the target LLM), and then we apply random search on a suffix to maximize the target logprob (e.g., of the token ``Sure''), potentially with multiple restarts. In this way, we achieve nearly 100% attack success rate---according to GPT-4 as a judge---on GPT-3.5/4, Llama-2-Chat-7B/13B/70B, Gemma-7B, and R2D2 from HarmBench that was adversarially trained against the GCG attack. We also show how to jailbreak all Claude models---that do not expose logprobs---via either a transfer or prefilling attack with 100% success rate. In addition, we show how to use random search on a restricted set of tokens for finding trojan strings in poisoned models---a task that shares many similarities with jailbreaking---which is the algorithm that brought us the first place in the SaTML'24 Trojan Detection Competition. The common theme behind these attacks is that adaptivity is crucial: different models are vulnerable to different prompting templates (e.g., R2D2 is very sensitive to in-context learning prompts), some models have unique vulnerabilities based on their APIs (e.g., prefilling for Claude), and in some settings it is crucial to restrict the token search space based on prior knowledge (e.g., for trojan detection).
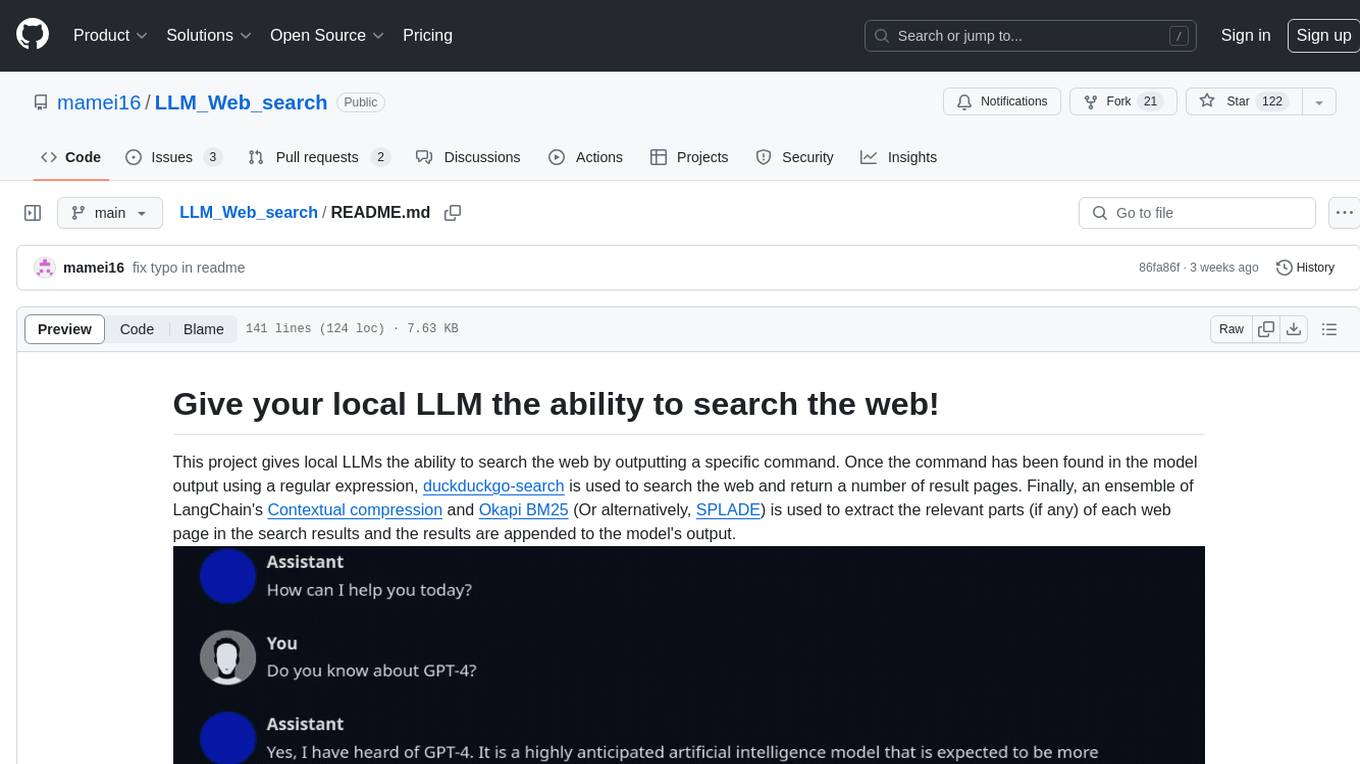
LLM_Web_search
LLM_Web_search project gives local LLMs the ability to search the web by outputting a specific command. It uses regular expressions to extract search queries from model output and then utilizes duckduckgo-search to search the web. LangChain's Contextual compression and Okapi BM25 or SPLADE are used to extract relevant parts of web pages in search results. The extracted results are appended to the model's output.
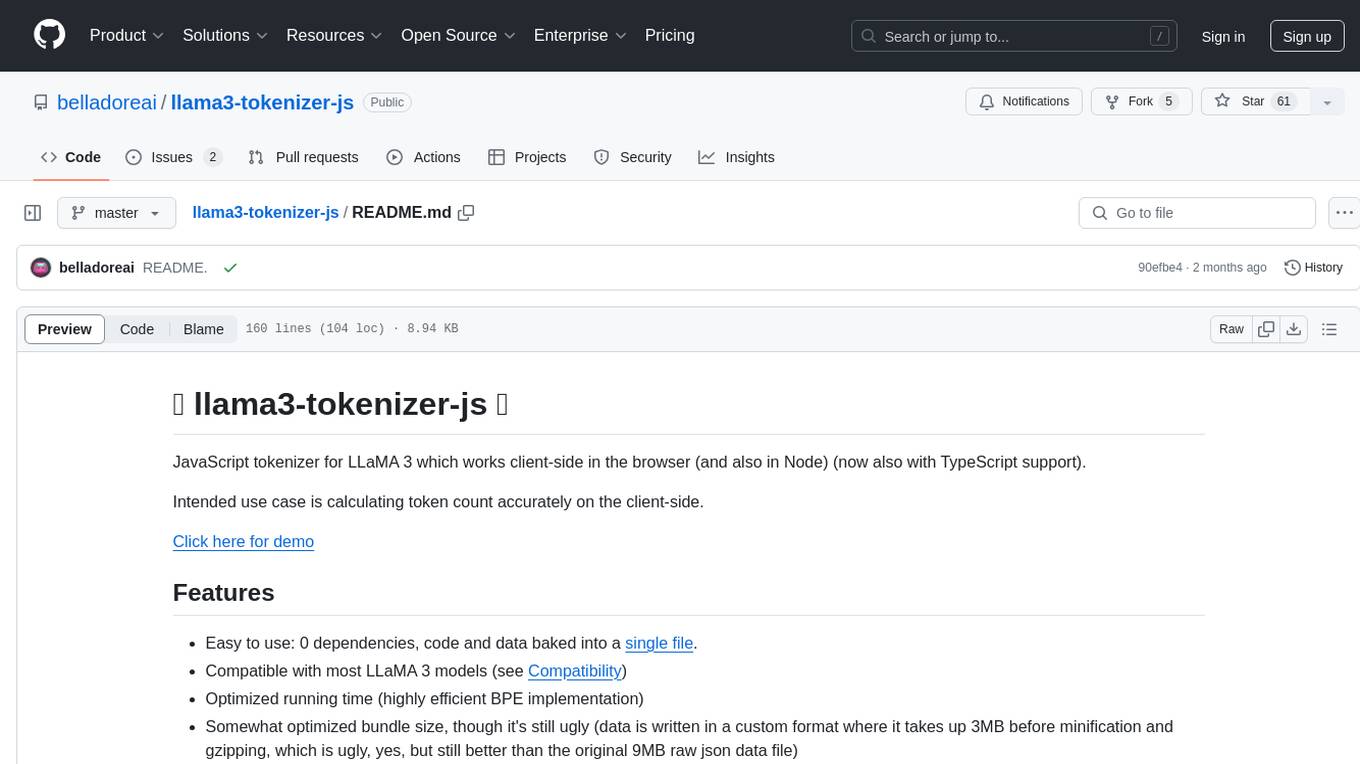
llama3-tokenizer-js
JavaScript tokenizer for LLaMA 3 designed for client-side use in the browser and Node, with TypeScript support. It accurately calculates token count, has 0 dependencies, optimized running time, and somewhat optimized bundle size. Compatible with most LLaMA 3 models. Can encode and decode text, but training is not supported. Pollutes global namespace with `llama3Tokenizer` in the browser. Mostly compatible with LLaMA 3 models released by Facebook in April 2024. Can be adapted for incompatible models by passing custom vocab and merge data. Handles special tokens and fine tunes. Developed by belladore.ai with contributions from xenova, blaze2004, imoneoi, and ConProgramming.
For similar tasks
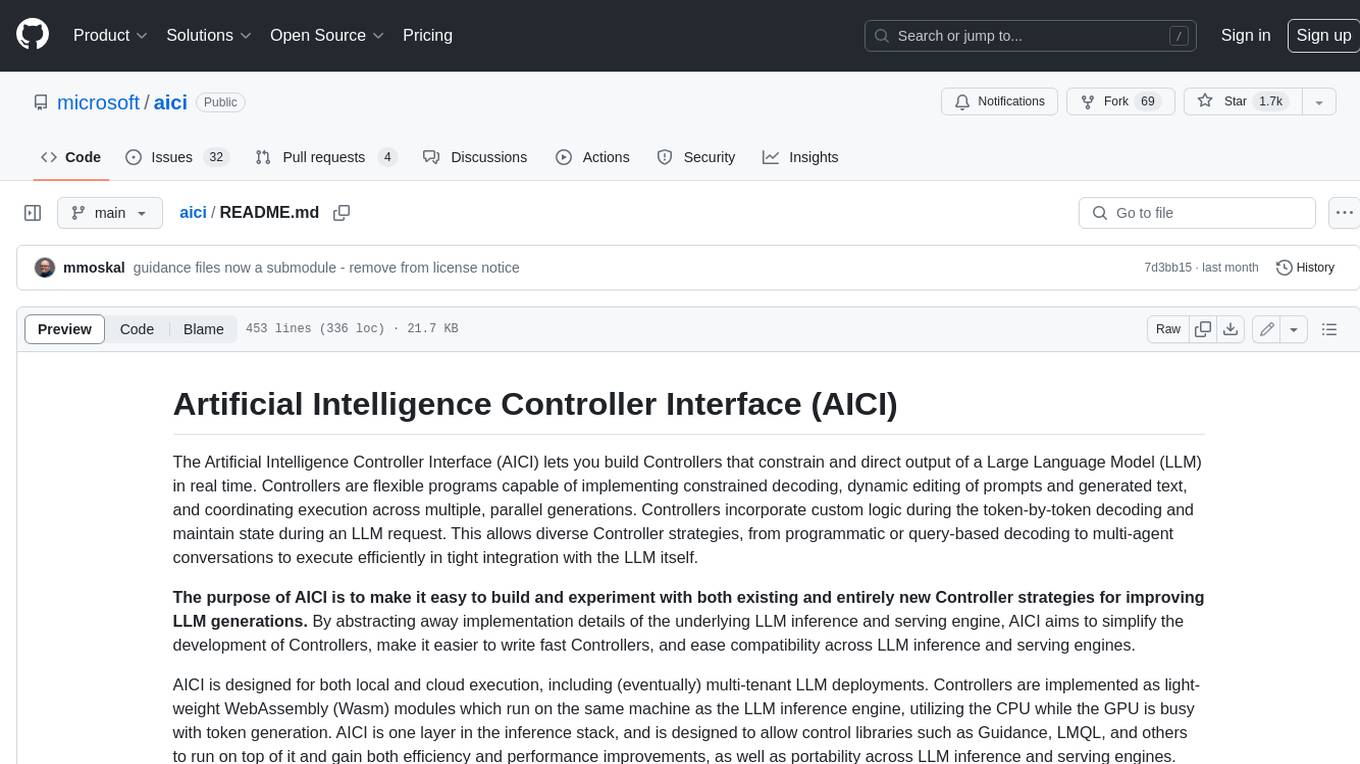
aici
The Artificial Intelligence Controller Interface (AICI) lets you build Controllers that constrain and direct output of a Large Language Model (LLM) in real time. Controllers are flexible programs capable of implementing constrained decoding, dynamic editing of prompts and generated text, and coordinating execution across multiple, parallel generations. Controllers incorporate custom logic during the token-by-token decoding and maintain state during an LLM request. This allows diverse Controller strategies, from programmatic or query-based decoding to multi-agent conversations to execute efficiently in tight integration with the LLM itself.
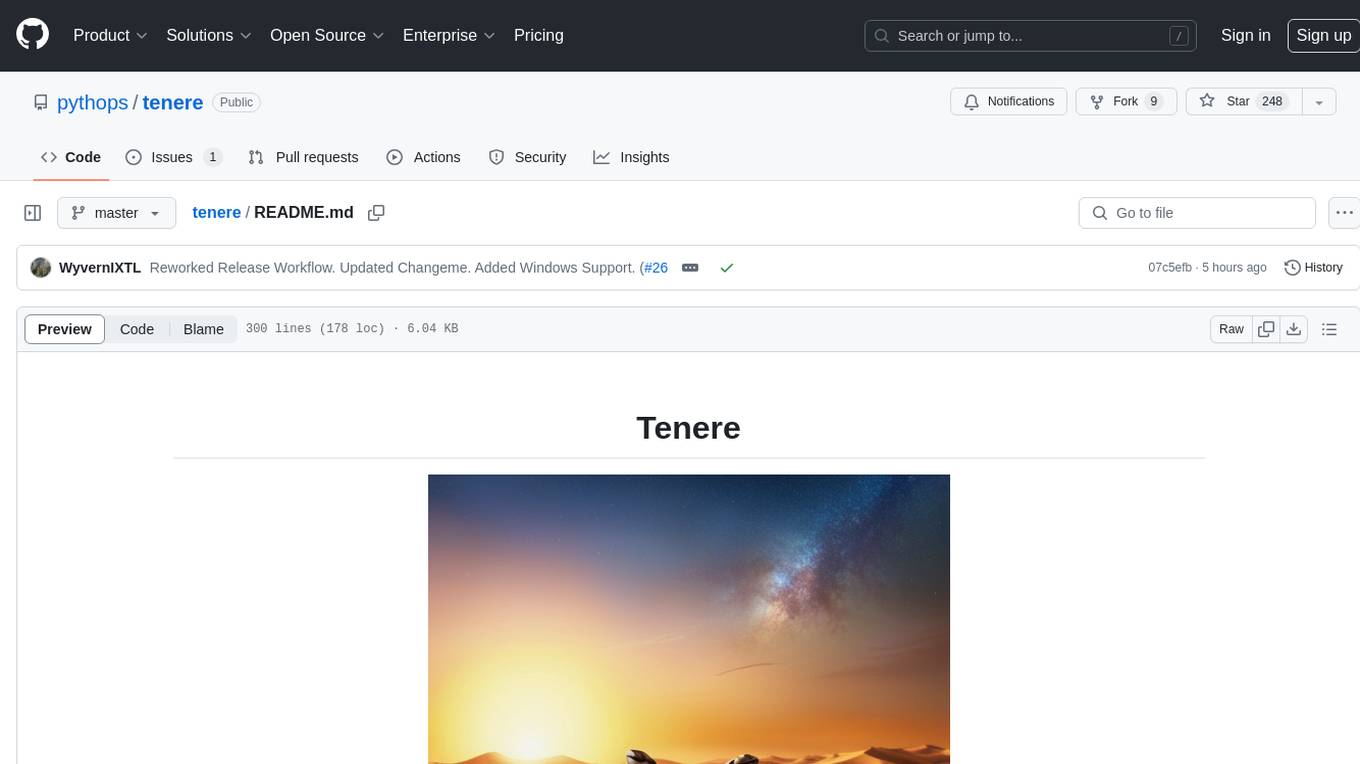
tenere
Tenere is a TUI interface for Language Model Libraries (LLMs) written in Rust. It provides syntax highlighting, chat history, saving chats to files, Vim keybindings, copying text from/to clipboard, and supports multiple backends. Users can configure Tenere using a TOML configuration file, set key bindings, and use different LLMs such as ChatGPT, llama.cpp, and ollama. Tenere offers default key bindings for global and prompt modes, with features like starting a new chat, saving chats, scrolling, showing chat history, and quitting the app. Users can interact with the prompt in different modes like Normal, Visual, and Insert, with various key bindings for navigation, editing, and text manipulation.
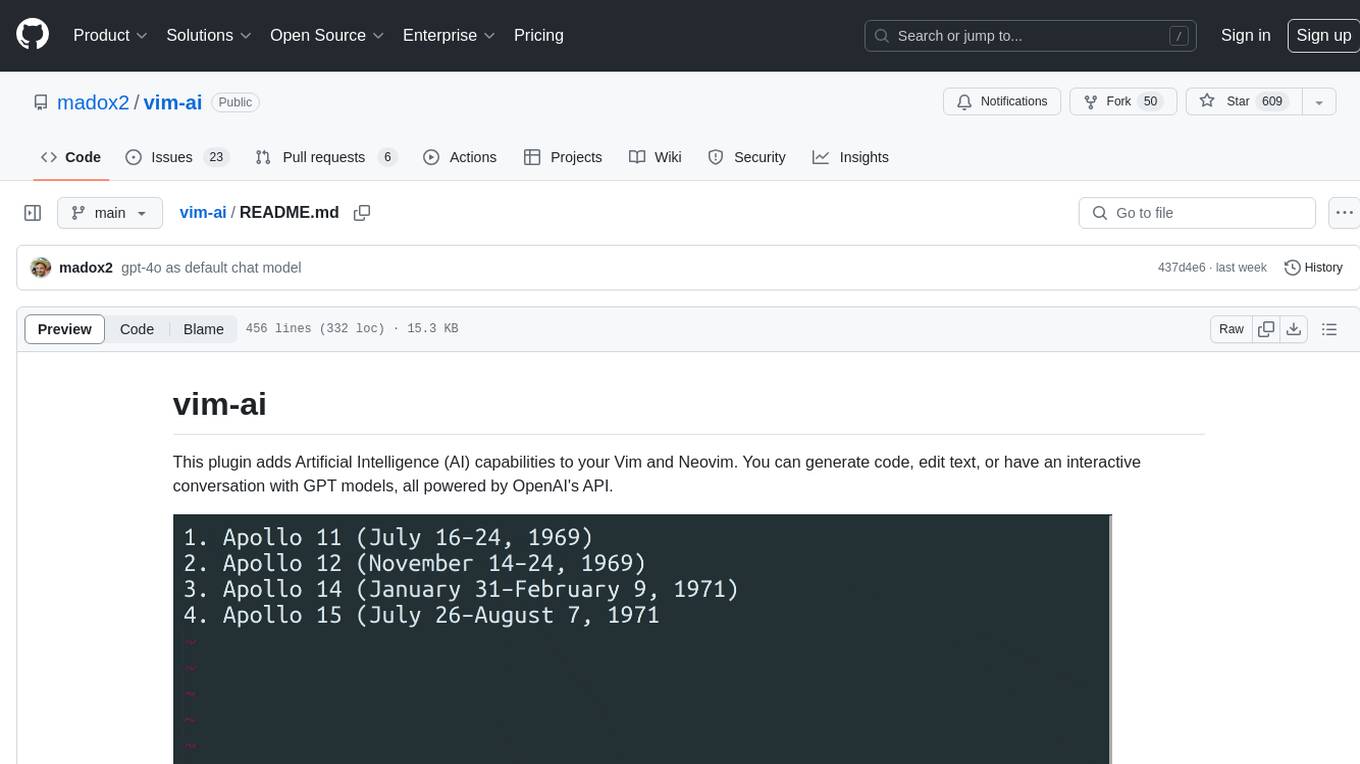
vim-ai
vim-ai is a plugin that adds Artificial Intelligence (AI) capabilities to Vim and Neovim. It allows users to generate code, edit text, and have interactive conversations with GPT models powered by OpenAI's API. The plugin uses OpenAI's API to generate responses, requiring users to set up an account and obtain an API key. It supports various commands for text generation, editing, and chat interactions, providing a seamless integration of AI features into the Vim text editor environment.
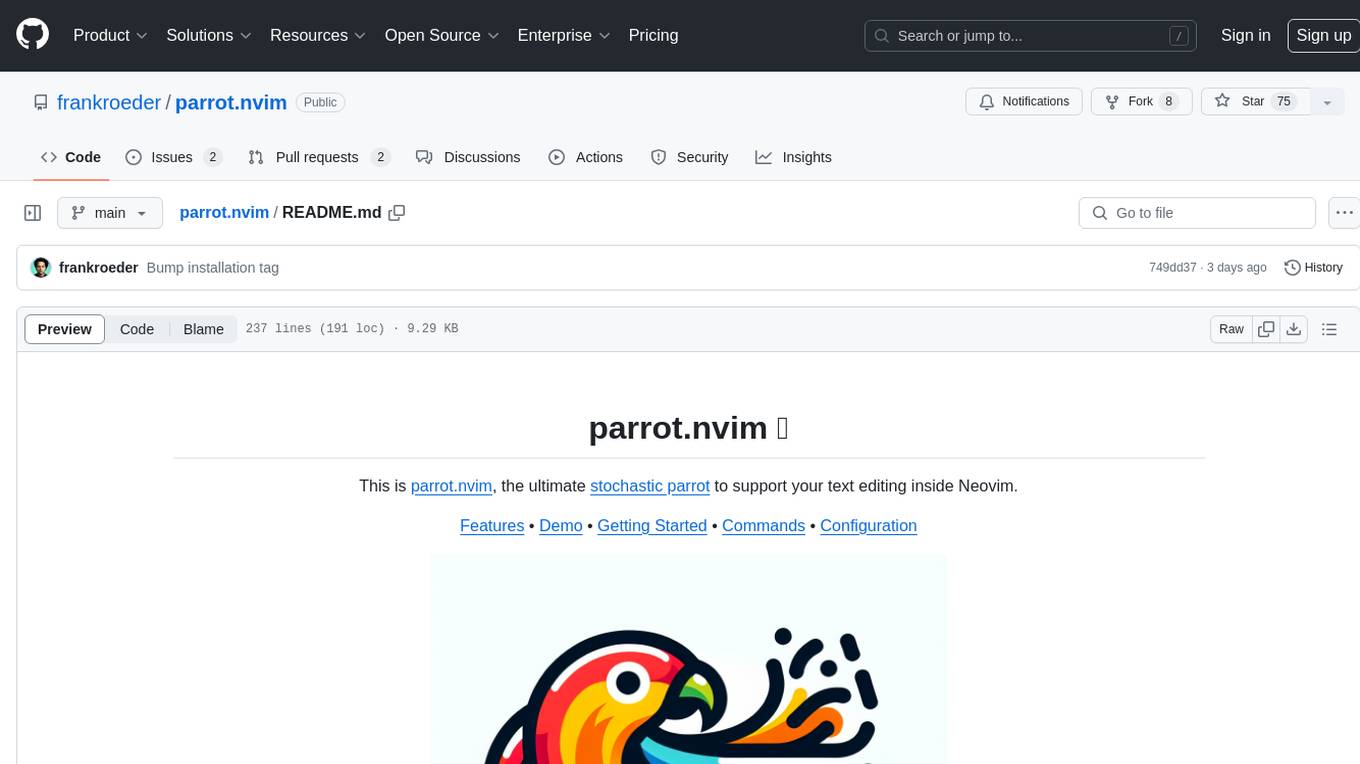
parrot.nvim
Parrot.nvim is a Neovim plugin that prioritizes a seamless out-of-the-box experience for text generation. It simplifies functionality and focuses solely on text generation, excluding integration of DALLE and Whisper. It supports persistent conversations as markdown files, custom hooks for inline text editing, multiple providers like Anthropic API, perplexity.ai API, OpenAI API, Mistral API, and local/offline serving via ollama. It allows custom agent definitions, flexible API credential support, and repository-specific instructions with a `.parrot.md` file. It does not have autocompletion or hidden requests in the background to analyze files.
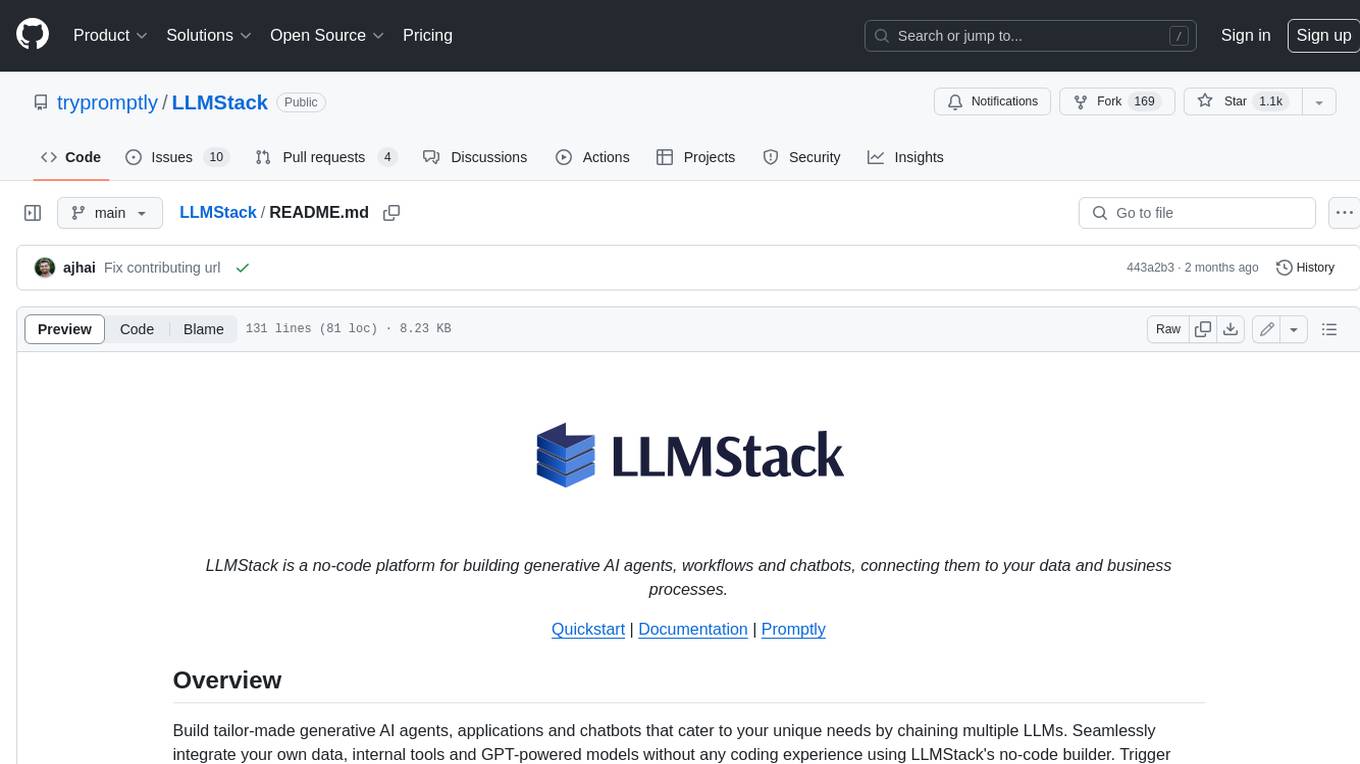
LLMStack
LLMStack is a no-code platform for building generative AI agents, workflows, and chatbots. It allows users to connect their own data, internal tools, and GPT-powered models without any coding experience. LLMStack can be deployed to the cloud or on-premise and can be accessed via HTTP API or triggered from Slack or Discord.
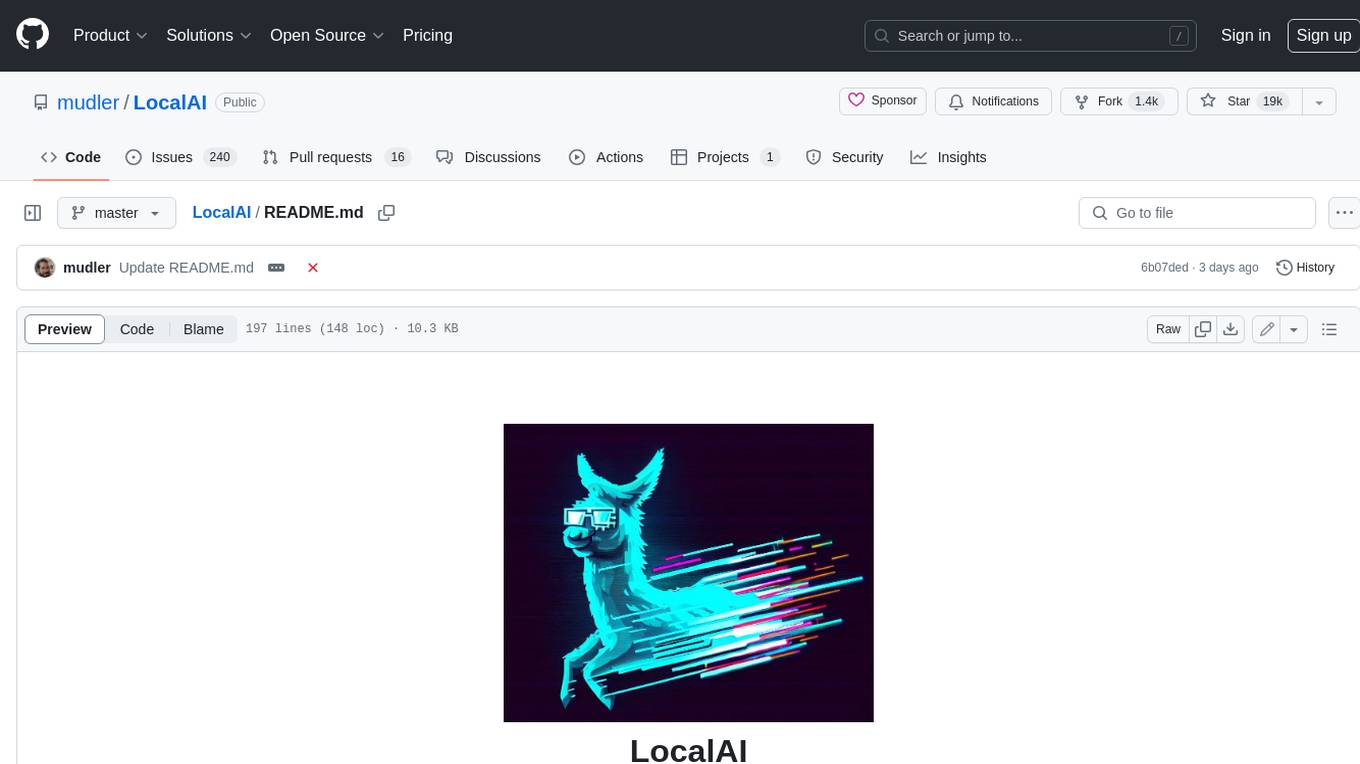
LocalAI
LocalAI is a free and open-source OpenAI alternative that acts as a drop-in replacement REST API compatible with OpenAI (Elevenlabs, Anthropic, etc.) API specifications for local AI inferencing. It allows users to run LLMs, generate images, audio, and more locally or on-premises with consumer-grade hardware, supporting multiple model families and not requiring a GPU. LocalAI offers features such as text generation with GPTs, text-to-audio, audio-to-text transcription, image generation with stable diffusion, OpenAI functions, embeddings generation for vector databases, constrained grammars, downloading models directly from Huggingface, and a Vision API. It provides a detailed step-by-step introduction in its Getting Started guide and supports community integrations such as custom containers, WebUIs, model galleries, and various bots for Discord, Slack, and Telegram. LocalAI also offers resources like an LLM fine-tuning guide, instructions for local building and Kubernetes installation, projects integrating LocalAI, and a how-tos section curated by the community. It encourages users to cite the repository when utilizing it in downstream projects and acknowledges the contributions of various software from the community.
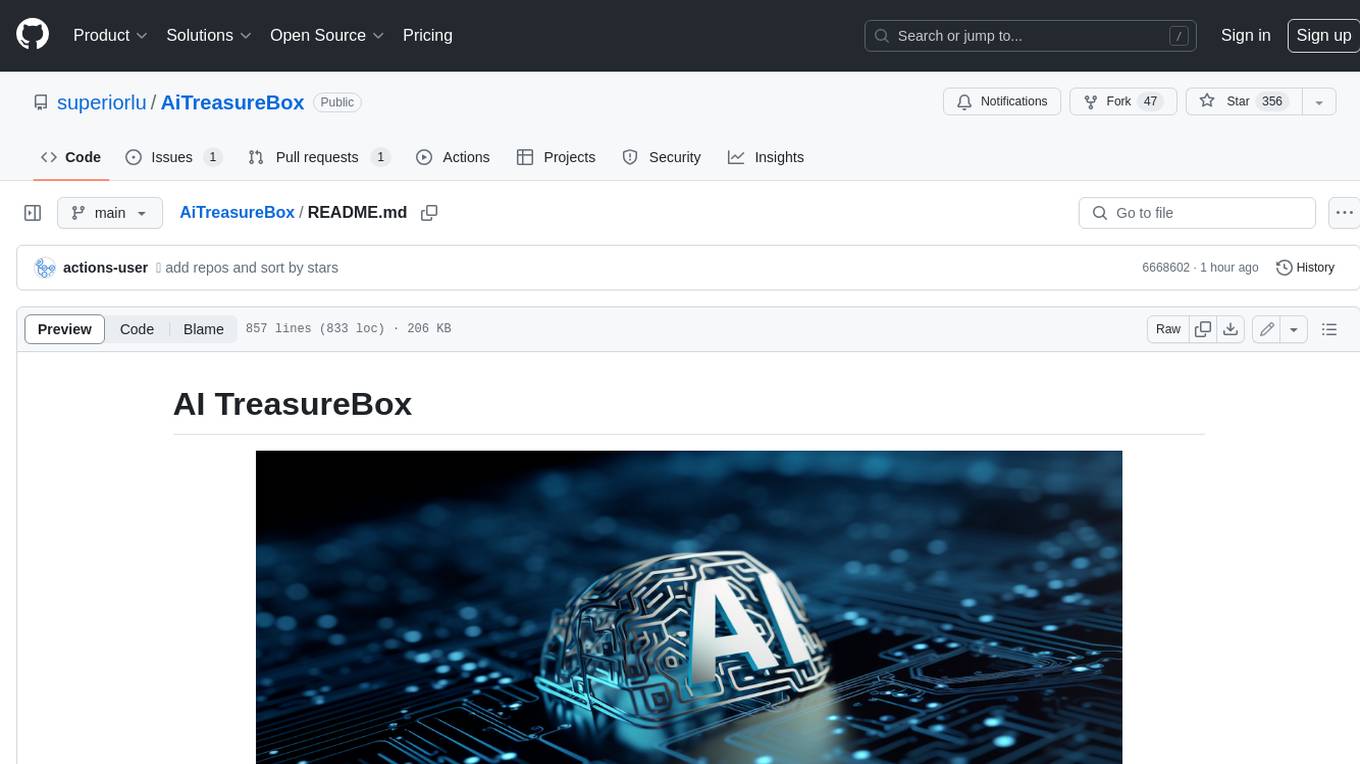
AiTreasureBox
AiTreasureBox is a versatile AI tool that provides a collection of pre-trained models and algorithms for various machine learning tasks. It simplifies the process of implementing AI solutions by offering ready-to-use components that can be easily integrated into projects. With AiTreasureBox, users can quickly prototype and deploy AI applications without the need for extensive knowledge in machine learning or deep learning. The tool covers a wide range of tasks such as image classification, text generation, sentiment analysis, object detection, and more. It is designed to be user-friendly and accessible to both beginners and experienced developers, making AI development more efficient and accessible to a wider audience.
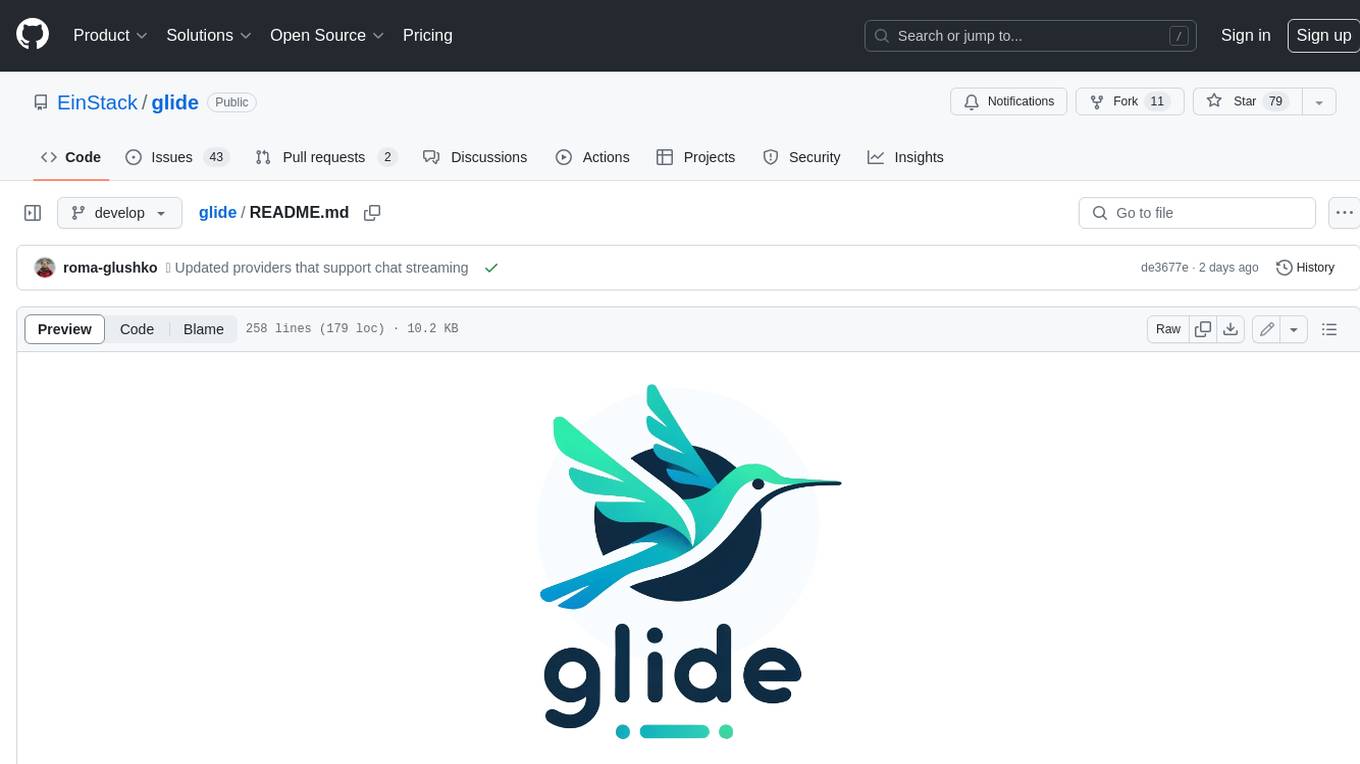
glide
Glide is a cloud-native LLM gateway that provides a unified REST API for accessing various large language models (LLMs) from different providers. It handles LLMOps tasks such as model failover, caching, key management, and more, making it easy to integrate LLMs into applications. Glide supports popular LLM providers like OpenAI, Anthropic, Azure OpenAI, AWS Bedrock (Titan), Cohere, Google Gemini, OctoML, and Ollama. It offers high availability, performance, and observability, and provides SDKs for Python and NodeJS to simplify integration.
For similar jobs
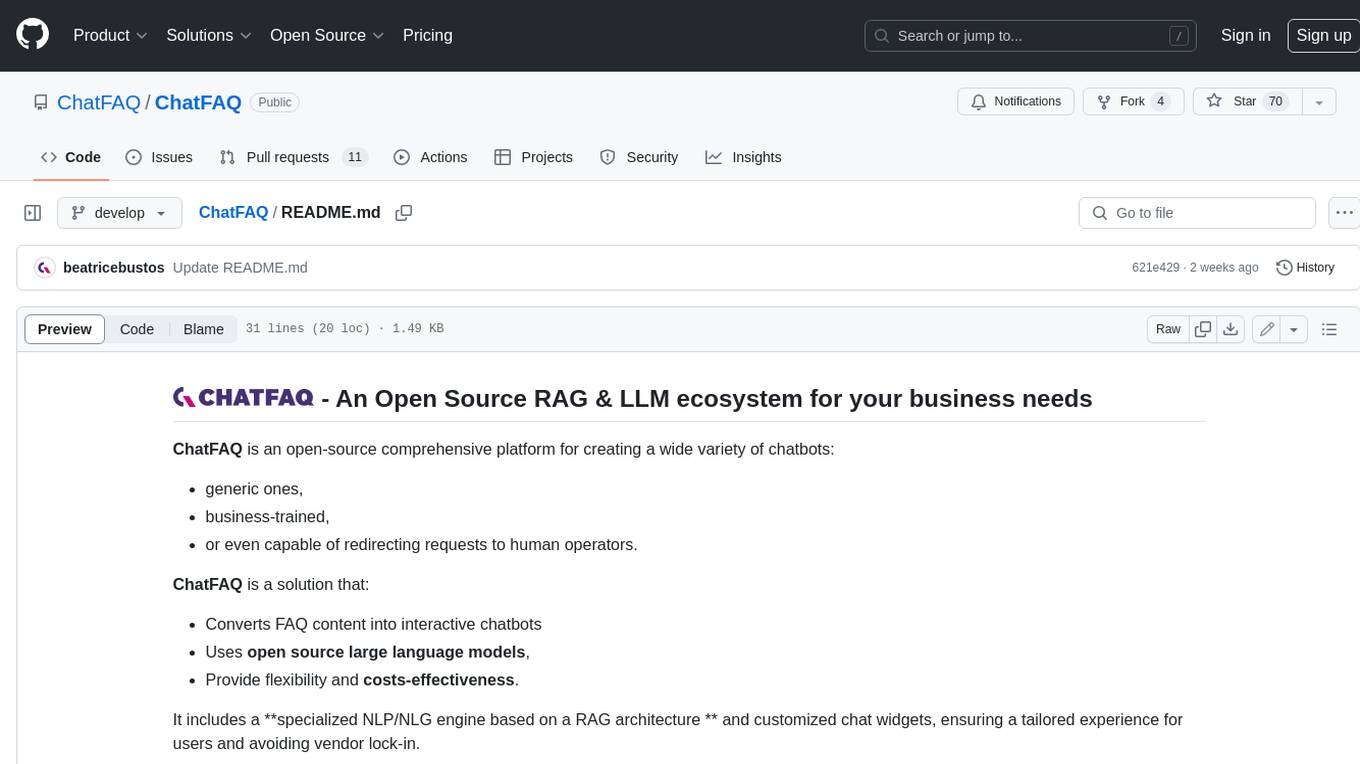
ChatFAQ
ChatFAQ is an open-source comprehensive platform for creating a wide variety of chatbots: generic ones, business-trained, or even capable of redirecting requests to human operators. It includes a specialized NLP/NLG engine based on a RAG architecture and customized chat widgets, ensuring a tailored experience for users and avoiding vendor lock-in.
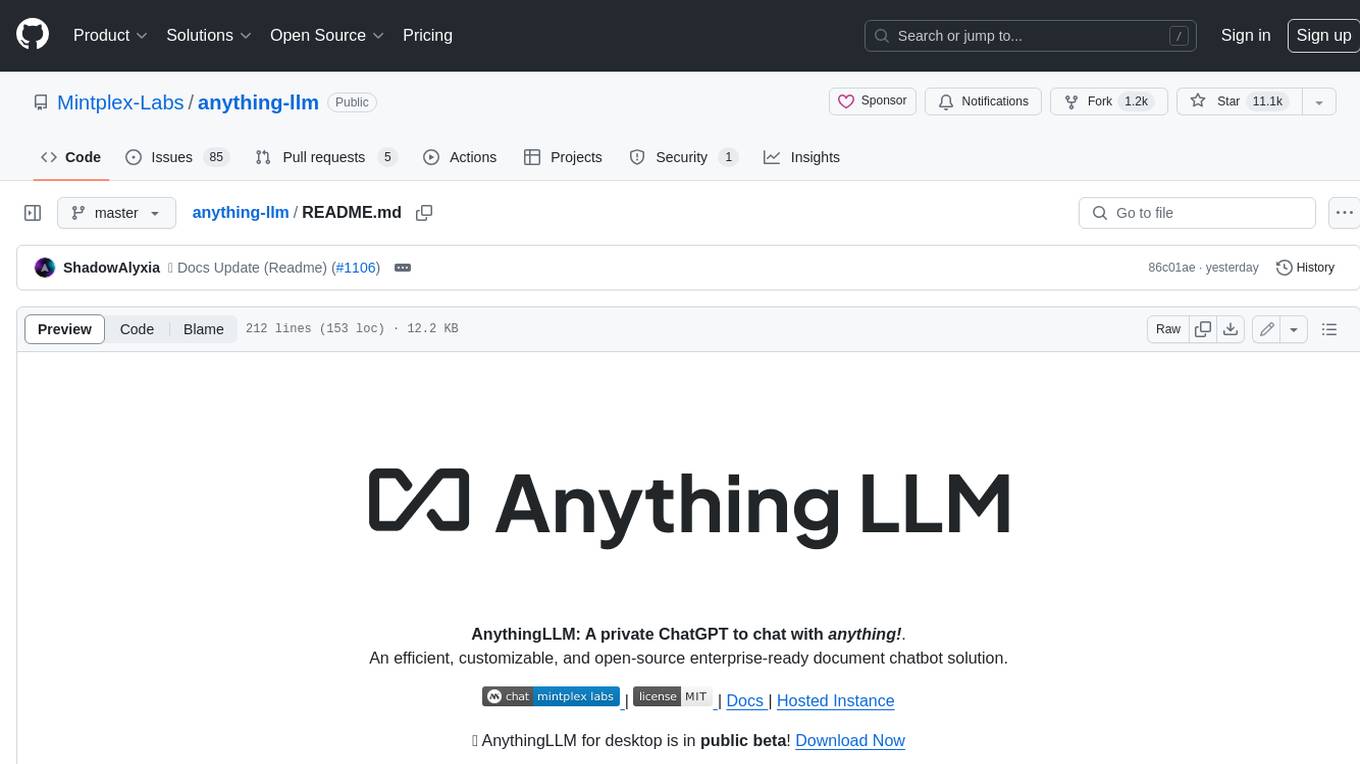
anything-llm
AnythingLLM is a full-stack application that enables you to turn any document, resource, or piece of content into context that any LLM can use as references during chatting. This application allows you to pick and choose which LLM or Vector Database you want to use as well as supporting multi-user management and permissions.
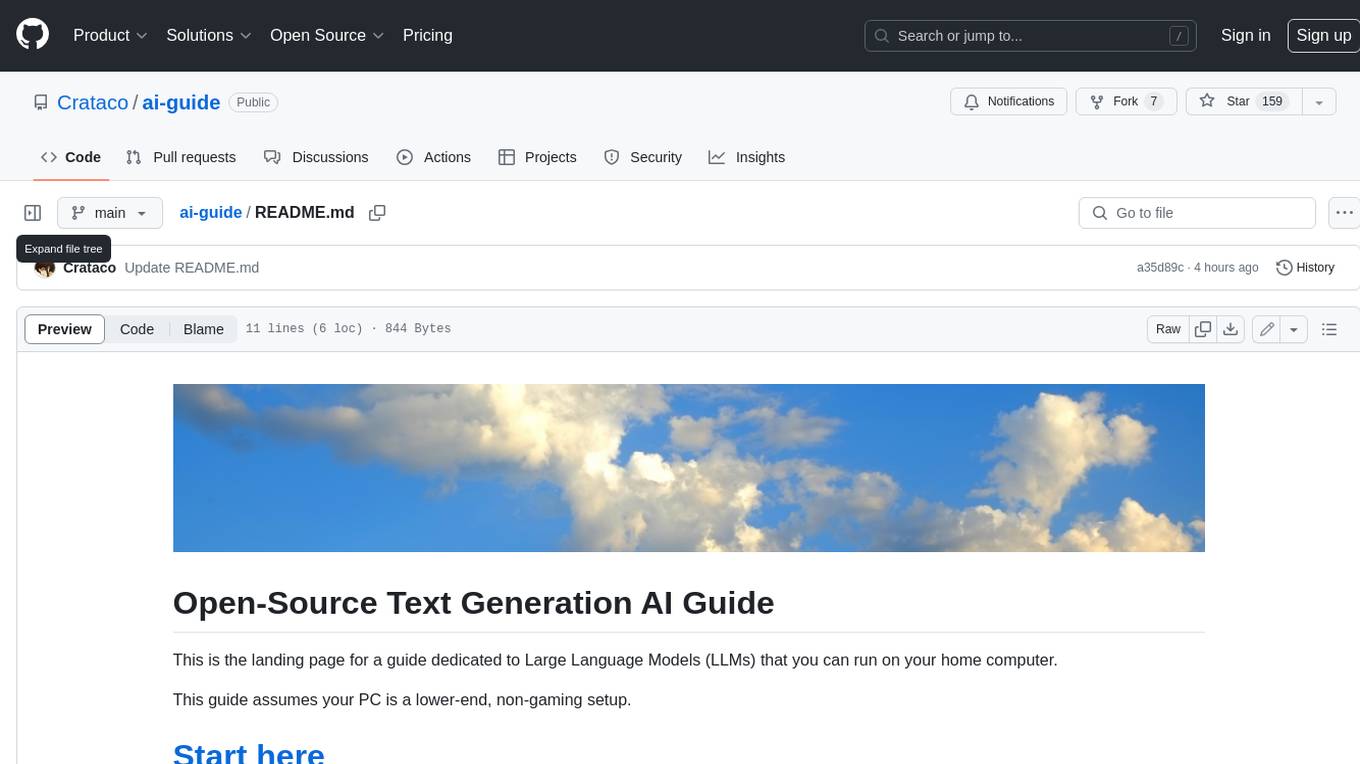
ai-guide
This guide is dedicated to Large Language Models (LLMs) that you can run on your home computer. It assumes your PC is a lower-end, non-gaming setup.
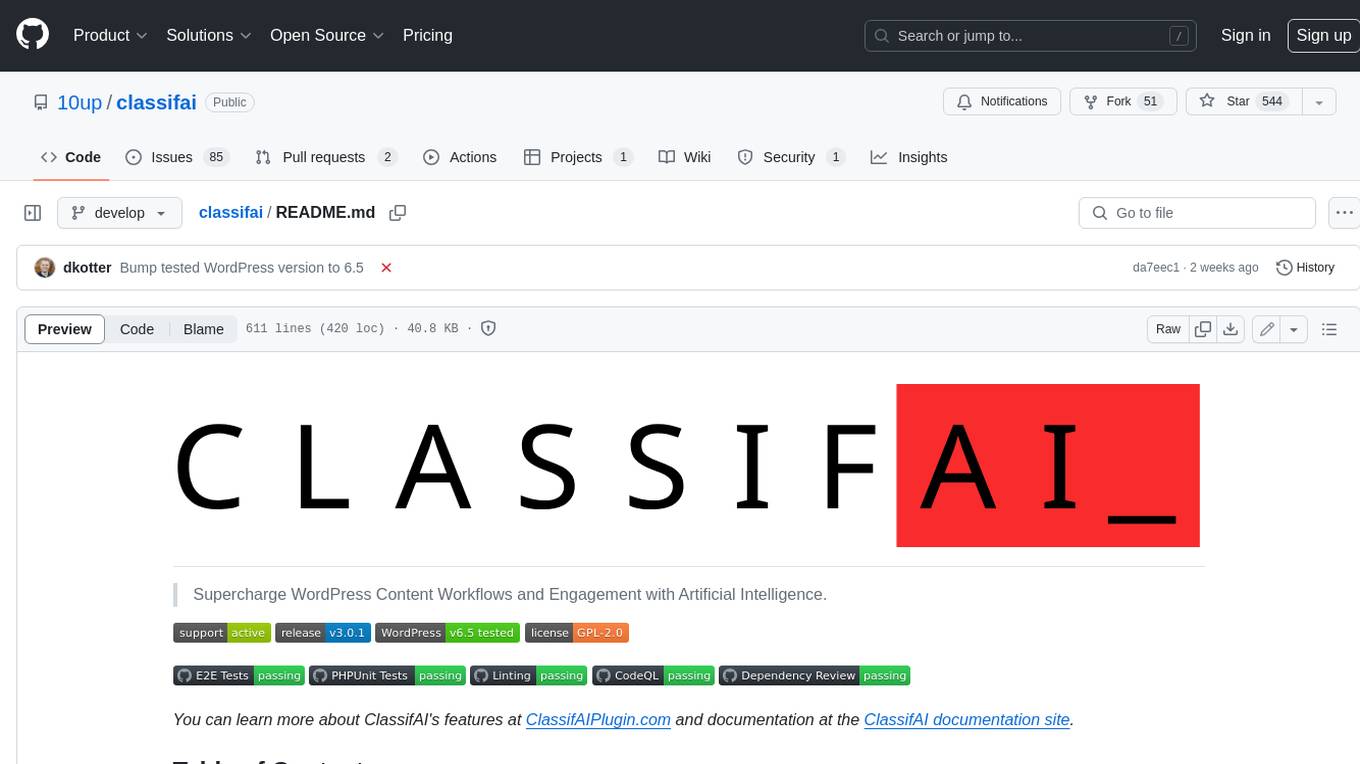
classifai
Supercharge WordPress Content Workflows and Engagement with Artificial Intelligence. Tap into leading cloud-based services like OpenAI, Microsoft Azure AI, Google Gemini and IBM Watson to augment your WordPress-powered websites. Publish content faster while improving SEO performance and increasing audience engagement. ClassifAI integrates Artificial Intelligence and Machine Learning technologies to lighten your workload and eliminate tedious tasks, giving you more time to create original content that matters.
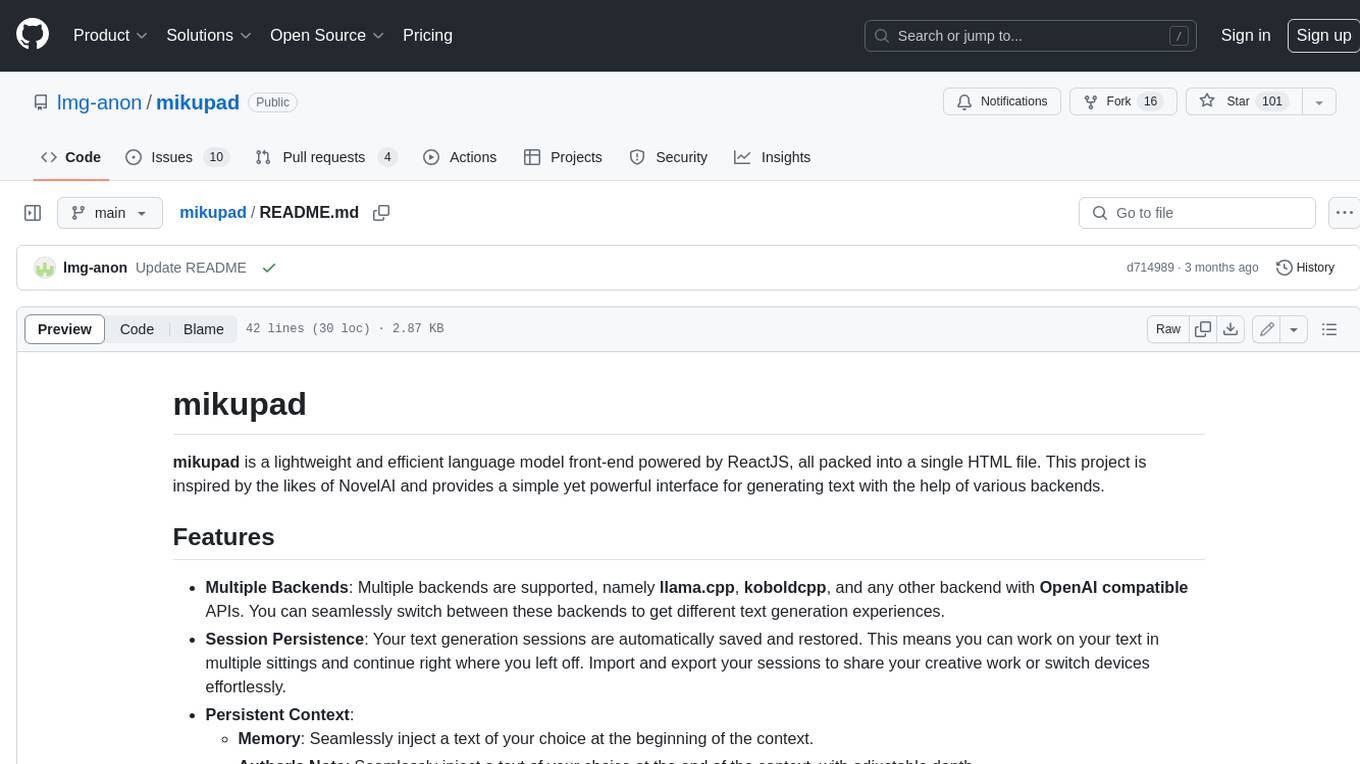
mikupad
mikupad is a lightweight and efficient language model front-end powered by ReactJS, all packed into a single HTML file. Inspired by the likes of NovelAI, it provides a simple yet powerful interface for generating text with the help of various backends.
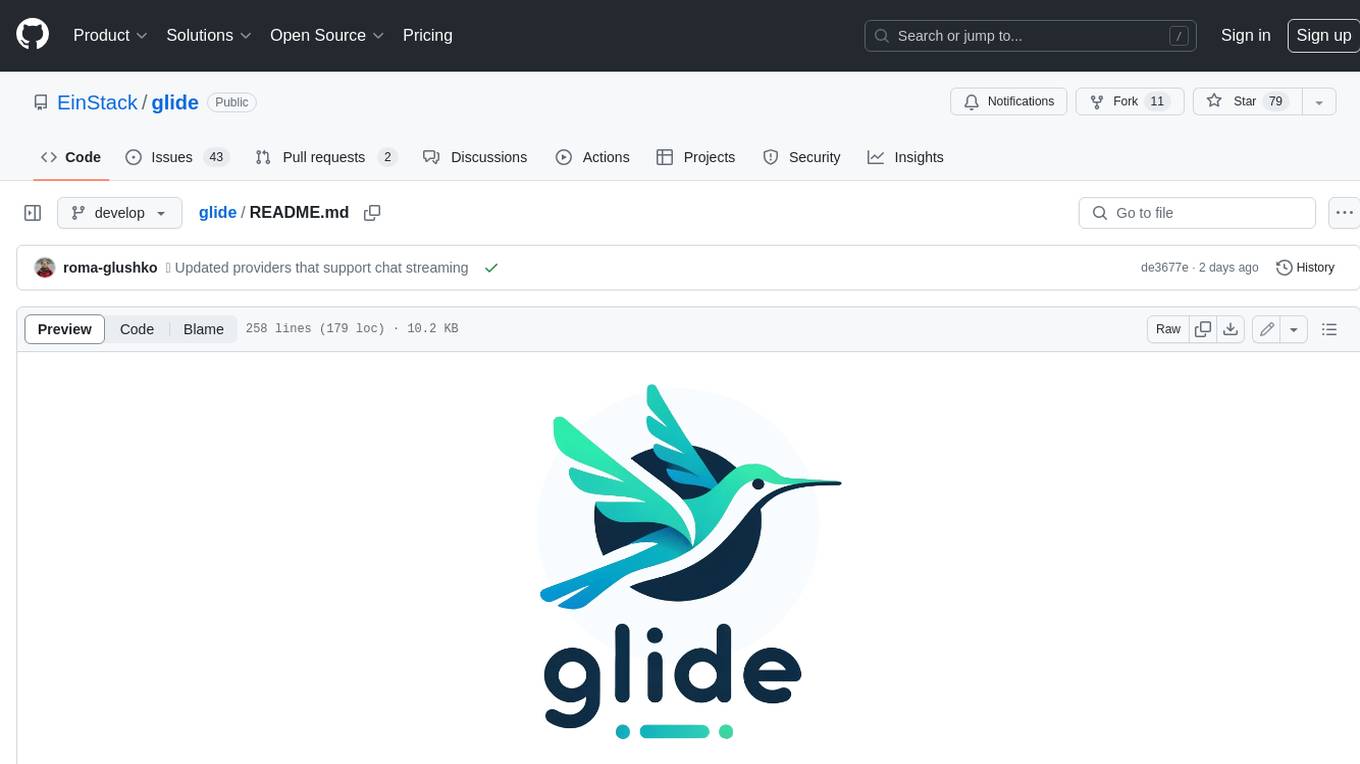
glide
Glide is a cloud-native LLM gateway that provides a unified REST API for accessing various large language models (LLMs) from different providers. It handles LLMOps tasks such as model failover, caching, key management, and more, making it easy to integrate LLMs into applications. Glide supports popular LLM providers like OpenAI, Anthropic, Azure OpenAI, AWS Bedrock (Titan), Cohere, Google Gemini, OctoML, and Ollama. It offers high availability, performance, and observability, and provides SDKs for Python and NodeJS to simplify integration.
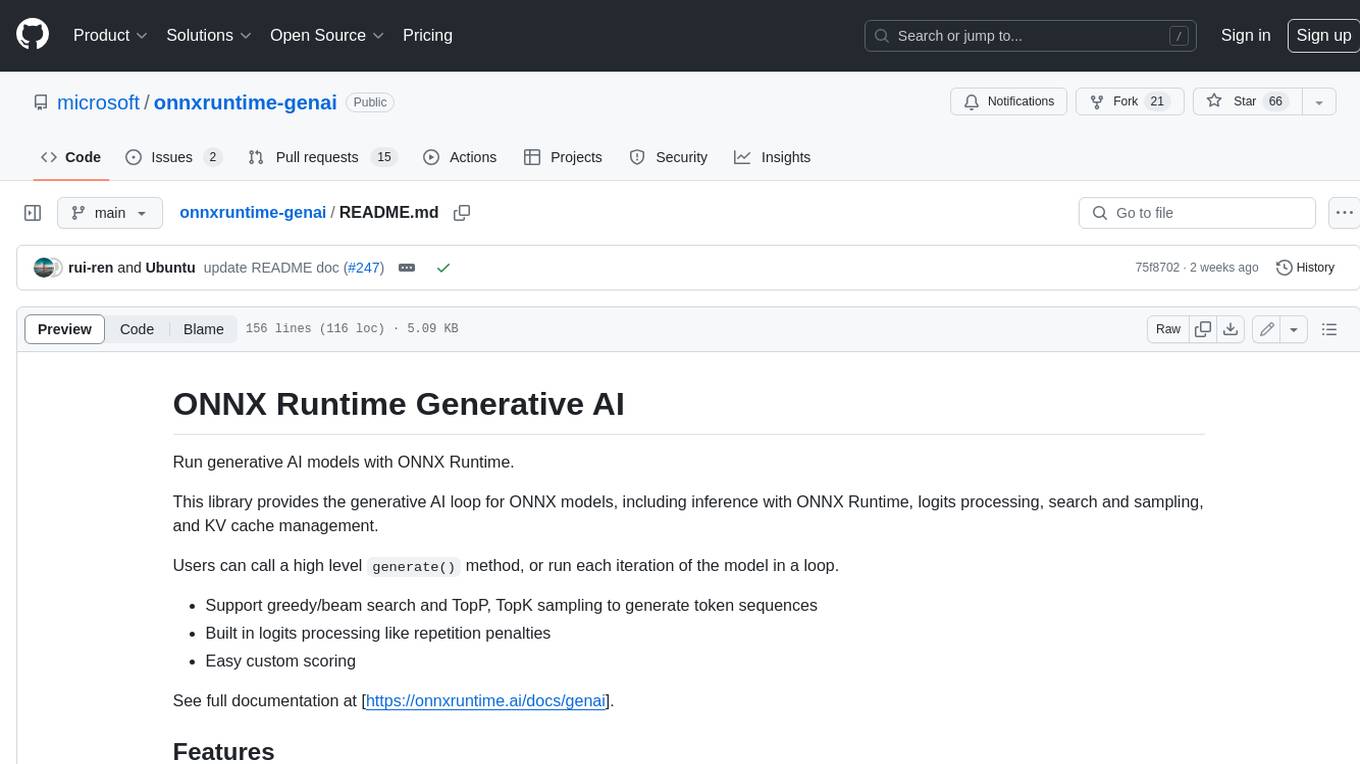
onnxruntime-genai
ONNX Runtime Generative AI is a library that provides the generative AI loop for ONNX models, including inference with ONNX Runtime, logits processing, search and sampling, and KV cache management. Users can call a high level `generate()` method, or run each iteration of the model in a loop. It supports greedy/beam search and TopP, TopK sampling to generate token sequences, has built in logits processing like repetition penalties, and allows for easy custom scoring.
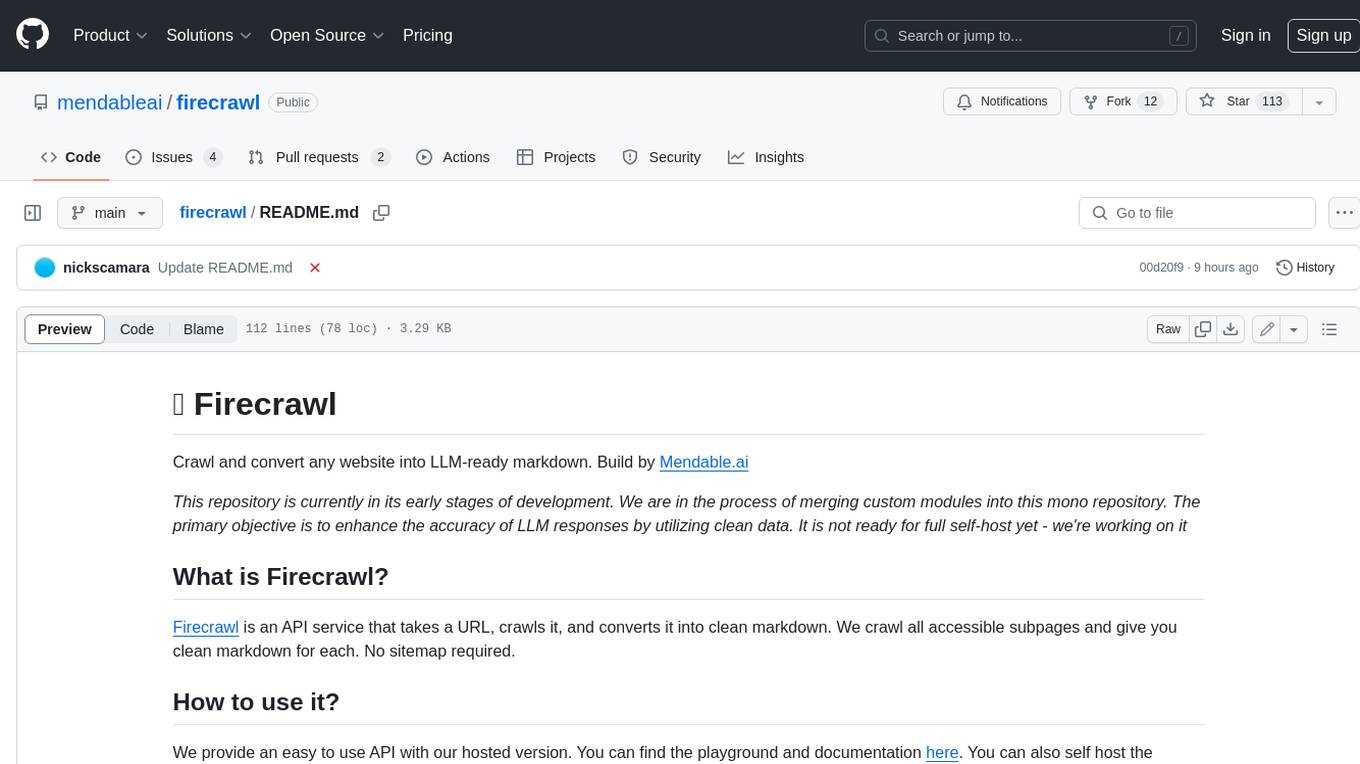
firecrawl
Firecrawl is an API service that takes a URL, crawls it, and converts it into clean markdown. It crawls all accessible subpages and provides clean markdown for each, without requiring a sitemap. The API is easy to use and can be self-hosted. It also integrates with Langchain and Llama Index. The Python SDK makes it easy to crawl and scrape websites in Python code.