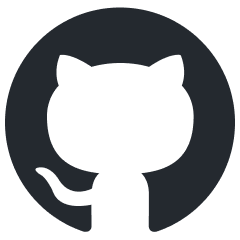
generative-ai-sagemaker-cdk-demo
Deploy Generative AI models from Amazon SageMaker JumpStart using AWS CDK
Stars: 65
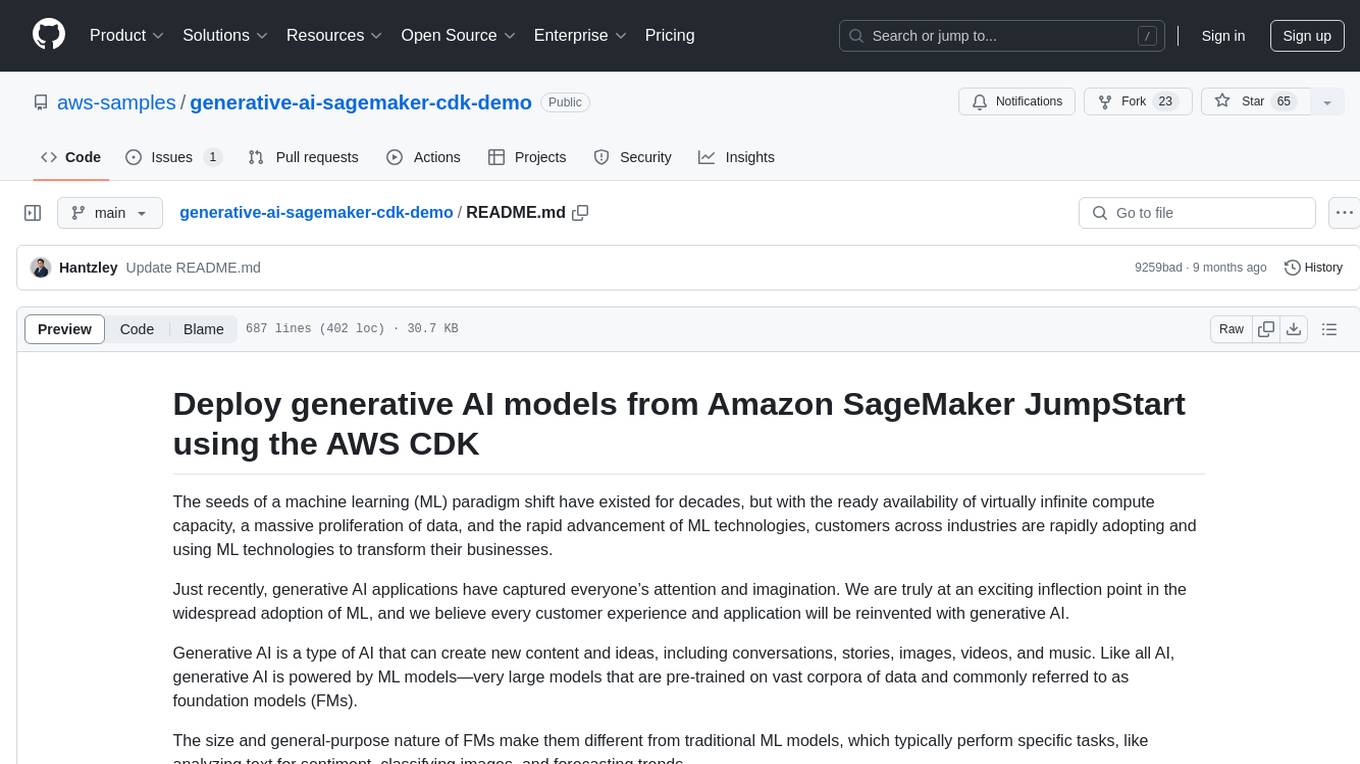
This repository showcases how to deploy generative AI models from Amazon SageMaker JumpStart using the AWS CDK. Generative AI is a type of AI that can create new content and ideas, such as conversations, stories, images, videos, and music. The repository provides a detailed guide on deploying image and text generative AI models, utilizing pre-trained models from SageMaker JumpStart. The web application is built on Streamlit and hosted on Amazon ECS with Fargate. It interacts with the SageMaker model endpoints through Lambda functions and Amazon API Gateway. The repository also includes instructions on setting up the AWS CDK application, deploying the stacks, using the models, and viewing the deployed resources on the AWS Management Console.
README:
The seeds of a machine learning (ML) paradigm shift have existed for decades, but with the ready availability of virtually infinite compute capacity, a massive proliferation of data, and the rapid advancement of ML technologies, customers across industries are rapidly adopting and using ML technologies to transform their businesses.
Just recently, generative AI applications have captured everyone’s attention and imagination. We are truly at an exciting inflection point in the widespread adoption of ML, and we believe every customer experience and application will be reinvented with generative AI.
Generative AI is a type of AI that can create new content and ideas, including conversations, stories, images, videos, and music. Like all AI, generative AI is powered by ML models—very large models that are pre-trained on vast corpora of data and commonly referred to as foundation models (FMs).
The size and general-purpose nature of FMs make them different from traditional ML models, which typically perform specific tasks, like analyzing text for sentiment, classifying images, and forecasting trends.
With tradition ML models, in order to achieve each specific task, you need to gather labeled data, train a model, and deploy that model. With foundation models, instead of gathering labeled data for each model and training multiple models, you can use the same pre-trained FM to adapt various tasks. You can also customize FMs to perform domain-specific functions that are differentiating to your businesses, using only a small fraction of the data and compute required to train a model from scratch.
Generative AI has the potential to disrupt many industries by revolutionizing the way content is created and consumed. Original content production, code generation, customer service enhancement, and document summarization are typical use cases of generative AI.
Amazon SageMaker JumpStart provides pre-trained, open-source models for a wide range of problem types to help you get started with ML. You can incrementally train and tune these models before deployment. JumpStart also provides solution templates that set up infrastructure for common use cases, and executable example notebooks for ML with Amazon SageMaker.
With over 600 pre-trained models available and growing every day, JumpStart enables developers to quickly and easily incorporate cutting-edge ML techniques into their production workflows. You can access the pre-trained models, solution templates, and examples through the JumpStart landing page in Amazon SageMaker Studio. You can also access JumpStart models using the SageMaker Python SDK. For information about how to use JumpStart models programmatically, see Use SageMaker JumpStart Algorithms with Pretrained Models.
In April 2023, AWS unveiled Amazon Bedrock, which provides a way to build generative AI-powered apps via pre-trained models from startups including AI21 Labs, Anthropic, and Stability AI. Amazon Bedrock also offers access to Titan foundation models, a family of models trained in-house by AWS. With the serverless experience of Amazon Bedrock, you can easily find the right model for your needs, get started quickly, privately customize FMs with your own data, and easily integrate and deploy them into your applications using the AWS tools and capabilities you’re familiar with (including integrations with SageMaker ML features like Amazon SageMaker Experiments to test different models and Amazon SageMaker Pipelines to manage your FMs at scale) without having to manage any infrastructure.
In this post, we show how to deploy image and text generative AI models from JumpStart using the AWS Cloud Development Kit (AWS CDK). The AWS CDK is an open-source software development framework to define your cloud application resources using familiar programming languages like Python.
We use the Stable Diffusion model for image generation and the FLAN-T5-XL model for natural language understanding (NLU) and text generation from Hugging Face in JumpStart.
The web application is built on Streamlit, an open-source Python library that makes it easy to create and share beautiful, custom web apps for ML and data science. We host the web application using Amazon Elastic Container Service (Amazon ECS) with AWS Fargate and it is accessed via an Application Load Balancer. Fargate is a technology that you can use with Amazon ECS to run containers without having to manage servers or clusters or virtual machines. The generative AI model endpoints are launched from JumpStart images in Amazon Elastic Container Registry (Amazon ECR). Model data is stored on Amazon Simple Storage Service (Amazon S3) in the JumpStart account. The web application interacts with the models via Amazon API Gateway and AWS Lambda functions as shown in the following diagram.
API Gateway provides the web application and other clients a standard RESTful interface, while shielding the Lambda functions that interface with the model. This simplifies the client application code that consumes the models. The API Gateway endpoints are publicly accessible in this example, allowing for the possibility to extend this architecture to implement different API access controls and integrate with other applications.
In this post, we walk you through the following steps:
- Install the AWS Command Line Interface (AWS CLI) and AWS CDK v2 on your local machine.
- Clone and set up the AWS CDK application.
- Deploy the AWS CDK application.
- Use the image generation AI model.
- Use the text generation AI model.
- View the deployed resources on the AWS Management Console.
We provide an overview of the code in this project in the appendix at the end of this post.
You must have the following prerequisites:
- An AWS account
- The AWS CLI v2
- Python 3.6 or later
- node.js 14.x or later
- The AWS CDK v2
- Docker v20.10 or later
You can deploy the infrastructure in this tutorial from your local computer or you can use AWS Cloud9 as your deployment workstation. AWS Cloud9 comes pre-loaded with AWS CLI, AWS CDK and Docker. If you opt for AWS Cloud9, create the environment from the AWS console.
The estimated cost to complete this post is $50, assuming you leave the resources running for 8 hours. Make sure you delete the resources you create in this post to avoid ongoing charges.
If you don’t already have the AWS CLI on your local machine, refer to Installing or updating the latest version of the AWS CLI and Configuring the AWS CLI.
Install the AWS CDK Toolkit globally using the following node package manager command:
npm install -g aws-cdk-lib@latest
Run the following command to verify the correct installation and print the version number of the AWS CDK:
cdk --version
Make sure you have Docker installed on your local machine. Issue the following command to verify the version:
docker --version
On your local machine, clone the AWS CDK application with the following command:
git clone https://github.com/aws-samples/generative-ai-sagemaker-cdk-demo.git
Navigate to the project folder:
cd generative-ai-sagemaker-cdk-demo
Before we deploy the application, let's review the directory structure:
.
├── LICENSE
├── README.md
├── app.py
├── cdk.json
├── code
│ ├── lambda_txt2img
│ │ └── txt2img.py
│ └── lambda_txt2nlu
│ └── txt2nlu.py
├── construct
│ └── sagemaker_endpoint_construct.py
├── images
│ ├── architecture.png
│ ├── ...
├── requirements-dev.txt
├── requirements.txt
├── source.bat
├── stack
│ ├── __init__.py
│ ├── generative_ai_demo_web_stack.py
│ ├── generative_ai_txt2img_sagemaker_stack.py
│ ├── generative_ai_txt2nlu_sagemaker_stack.py
│ └── generative_ai_vpc_network_stack.py
├── tests
│ ├── __init__.py
│ └── ...
└── web-app
├── Dockerfile
├── Home.py
├── configs.py
├── img
│ └── sagemaker.png
├── pages
│ ├── 2_Image_Generation.py
│ └── 3_Text_Generation.py
└── requirements.txt
The stack
folder contains the code for each stack in the AWS CDK application. The code
folder contains the code for the Amazon Lambda functions. The repository also contains the web application located under the folder web-app
.
The cdk.json
file tells the AWS CDK Toolkit how to run your application.
This application was tested in the us-east-1
Region but it should work in any Region that has the required services and inference instance type ml.g4dn.4xlarge
specified in app.py.
This project is set up like a standard Python project. Create a Python virtual environment using the following code:
python3 -m venv .venv
Use the following command to activate the virtual environment:
source .venv/bin/activate
If you’re on a Windows platform, activate the virtual environment as follows:
.venv\Scripts\activate.bat
After the virtual environment is activated, upgrade pip to the latest version:
python3 -m pip install --upgrade pip
Install the required dependencies:
pip install -r requirements.txt
Before you deploy any AWS CDK application, you need to bootstrap a space in your account and the Region you’re deploying into. To bootstrap in your default Region, issue the following command:
cdk bootstrap
If you want to deploy into a specific account and Region, issue the following command:
cdk bootstrap aws://ACCOUNT-NUMBER/REGION
For more information about this setup, visit Getting started with the AWS CDK.
The AWS CDK application contains multiple stacks as shown in the following diagram.
You can list stacks in your CDK application with the following command:
cdk list
You should get the following output:
GenerativeAiTxt2imgSagemakerStack
GenerativeAiTxt2nluSagemakerStack
GenerativeAiVpcNetworkStack
GenerativeAiDemoWebStack
Other useful AWS CDK commands:
-
cdk ls
- Lists all stacks in the app -
cdk synth
- Emits the synthesized AWS CloudFormation template -
cdk deploy
- Deploys this stack to your default AWS account and Region -
cdk diff
- Compares the deployed stack with current state -
cdk docs
- Opens the AWS CDK documentation
The next section shows you how to deploy the AWS CDK application.
The AWS CDK application will be deployed to the default Region based on your workstation configuration. If you want to force the deployment in a specific Region, set your AWS_DEFAULT_REGION
environment variable accordingly.
At this point, you can deploy the AWS CDK application. First you launch the VPC network stack:
cdk deploy GenerativeAiVpcNetworkStack
If you are prompted, enter y
to proceed with the deployment. You should see a list of AWS resources that are being provisioned in the stack. This step takes around 3 minutes to complete.
Then you launch the web application stack:
cdk deploy GenerativeAiDemoWebStack
After analyzing the stack, the AWS CDK will display the resource list in the stack. Enter y to proceed with the deployment. This step takes around 5 minutes.
Note down the WebApplicationServiceURL
from the output as you will use it later. You can also retrieve it later in the CloudFormation console, under the GenerativeAiDemoWebStack
stack outputs.
Now, launch the image generation AI model endpoint stack:
cdk deploy GenerativeAiTxt2imgSagemakerStack
This step takes around 8 minutes. The image generation model endpoint is deployed, we can now use it.
The first example demonstrates how to utilize Stable Diffusion, a powerful generative modeling technique that enables the creation of high-quality images from text prompts.
- Access the web application using the
WebApplicationServiceURL
from the output of theGenerativeAiDemoWebStack
in your browser.
-
In the navigation pane, choose Image Generation.
-
The SageMaker Endpoint Name and API GW Url fields will be pre-populated, but you can change the prompt for the image description if you’d like.
-
Choose Generate image.
The application will make a call to the SageMaker endpoint. It takes a few seconds. A picture with the charasteristics in your image description will be displayed.
The second example centers around using the FLAN-T5-XL model, which is a foundation or large language model (LLM), to achieve in-context learning for text generation while also addressing a broad range of natural language understanding (NLU) and natural language generation (NLG) tasks.
Some environments might limit the number of endpoints you can launch at a time. If this is the case, you can launch one SageMaker endpoint at a time. To stop a SageMaker endpoint in the AWS CDK app, you have to destroy the deployed endpoint stack and before launching the other endpoint stack. To turn down the image generation AI model endpoint, issue the following command:
cdk destroy GenerativeAiTxt2imgSagemakerStack
Then launch the text generation AI model endpoint stack:
cdk deploy GenerativeAiTxt2nluSagemakerStack
Enter y
at the prompts.
After the text generation model endpoint stack is launched, complete the following steps:
- Go back to the web application and choose Text Generation in the navigation pane.
- The Input Context field is pre-populated with a conversation between a customer and an agent regarding an issue with the customers phone, but you can enter your own context if you’d like.
Below the context, you will find some prepopulated queries in the dropdown menu options.
- Choose a query and choose Generate Response.
You can also enter your own query in the Input Query field and choose Generate Response.
On the AWS CloudFormation console, choose Stacks in the navigation pane to view the stacks deployed.
On the Amazon ECS console, you can see the clusters on the Clusters page.
On the AWS Lambda console, you can see the functions on the Functions page.
On the API Gateway console, you can see the API Gateway endpoints on the APIs page.
On the SageMaker console, you can see the deployed model endpoints on the Endpoints page.
When the stacks are launched, some parameters are generated. These are stored in the AWS Systems Manager Parameter Store. To view them, choose Parameter Store in the navigation pane on the AWS Systems Manager console.
To avoid unnecessary cost, clean up all the infrastructure created with the following command on your workstation:
cdk destroy --all
Enter y
at the prompt. This step takes around 10 minutes. Check if all resources are deleted on the console. Also delete the assets S3 buckets created by the AWS CDK on the Amazon S3 console as well as the assets repositories on Amazon ECR.
As demonstrated in this post, you can use the AWS CDK to deploy generative AI models in JumpStart. We showed an image generation example and a text generation example using a user interface powered by Streamlit, Lambda, and API Gateway.
You can now build your generative AI projects using pre-trained AI models in JumpStart. You can also extend this project to fine-tune the foundation models for your use case and control access to API Gateway endpoints.
We invite you to test the solution and contribute to the project on GitHub.
This sample code is made available under a modified MIT license. See the LICENSE file for more information. Also, review the respective licenses for the stable diffusion and flan-t5-xl models on Hugging Face.
Hantzley Tauckoor is an APJ Partner Solutions Architecture Leader based in Singapore. He has 20 years’ experience in the ICT industry spanning multiple functional areas, including solutions architecture, business development, sales strategy, consulting, and leadership. He leads a team of Senior Solutions Architects that enable partners to develop joint solutions, build technical capabilities, and steer them through the implementation phase as customers migrate and modernize their applications to AWS. Outside work, he enjoys spending time with his family, watching movies, and hiking.
Kwonyul Choi is a CTO at BABITALK, a Korean beauty care platform startup, based in Seoul. Prior to this role, Kownyul worked as Software Development Engineer at AWS with a focus on AWS CDK and Amazon SageMaker.
Arunprasath Shankar is a Senior AI/ML Specialist Solutions Architect with AWS, helping global customers scale their AI solutions effectively and efficiently in the cloud. In his spare time, Arun enjoys watching sci-fi movies and listening to classical music.
Satish Upreti is a Migration Lead PSA and Security SME in the partner organization in APJ. Satish has 20 years of experience spanning on-premises private cloud and public cloud technologies. Since joining AWS in August 2020 as a migration specialist, he provides extensive technical advice and support to AWS partners to plan and implement complex migrations.
In this section, we provide an overview of the code in this project.
AWS CDK Application
The main AWS CDK application is contained in the app.py
file in the root directory. The project consists of multiple stacks, so we have to import the stacks:
#!/usr/bin/env python3
import aws_cdk as cdk
from stack.generative_ai_vpc_network_stack import GenerativeAiVpcNetworkStack
from stack.generative_ai_demo_web_stack import GenerativeAiDemoWebStack
from stack.generative_ai_txt2nlu_sagemaker_stack import GenerativeAiTxt2nluSagemakerStack
from stack.generative_ai_txt2img_sagemaker_stack import GenerativeAiTxt2imgSagemakerStack
We define our generative AI models and get the related URIs from SageMaker:
from script.sagemaker_uri import *
import boto3
region_name = boto3.Session().region_name
env={"region": region_name}
#Text to Image model parameters
TXT2IMG_MODEL_ID = "model-txt2img-stabilityai-stable-diffusion-v2-1-base"
TXT2IMG_INFERENCE_INSTANCE_TYPE = "ml.g4dn.4xlarge"
TXT2IMG_MODEL_TASK_TYPE = "txt2img"
TXT2IMG_MODEL_INFO = get_sagemaker_uris(model_id=TXT2IMG_MODEL_ID,
model_task_type=TXT2IMG_MODEL_TASK_TYPE,
instance_type=TXT2IMG_INFERENCE_INSTANCE_TYPE,
region_name=region_name)
#Text to NLU image model parameters
TXT2NLU_MODEL_ID = "huggingface-text2text-flan-t5-xl"
TXT2NLU_INFERENCE_INSTANCE_TYPE = "ml.g4dn.4xlarge"
TXT2NLU_MODEL_TASK_TYPE = "text2text"
TXT2NLU_MODEL_INFO = get_sagemaker_uris(model_id=TXT2NLU_MODEL_ID,
model_task_type=TXT2NLU_MODEL_TASK_TYPE,
instance_type=TXT2NLU_INFERENCE_INSTANCE_TYPE,
region_name=region_name)
The function get_sagemaker_uris
retrieves all the model information from Amazon JumpStart. See script/sagemaker_uri.py.
Then, we instantiate the stacks:
app = cdk.App()
network_stack = GenerativeAiVpcNetworkStack(app, "GenerativeAiVpcNetworkStack", env=env)
GenerativeAiDemoWebStack(app, "GenerativeAiDemoWebStack", vpc=network_stack.vpc, env=env)
GenerativeAiTxt2nluSagemakerStack(app, "GenerativeAiTxt2nluSagemakerStack", env=env, model_info=TXT2NLU_MODEL_INFO)
GenerativeAiTxt2imgSagemakerStack(app, "GenerativeAiTxt2imgSagemakerStack", env=env, model_info=TXT2IMG_MODEL_INFO)
app.synth()
The first stack to launch is the VPC stack, GenerativeAiVpcNetworkStack
. The web application stack, GenerativeAiDemoWebStack
, is dependent on the VPC stack. The dependency is done through parameter passing vpc=network_stack.vpc
.
See app.py for the full code.
VPC network stack
In the GenerativeAiVpcNetworkStack
stack we create a VPC with a public subnet and a private subnet spanning across two Availability Zones (AZs):
self.output_vpc = ec2.Vpc(self, "VPC",
nat_gateways=1,
ip_addresses=ec2.IpAddresses.cidr("10.0.0.0/16"),
max_azs=2,
subnet_configuration=[
ec2.SubnetConfiguration(name="public",subnet_type=ec2.SubnetType.PUBLIC,cidr_mask=24),
ec2.SubnetConfiguration(name="private",subnet_type=ec2.SubnetType.PRIVATE_WITH_EGRESS,cidr_mask=24)
]
)
See /stack/generative_ai_vpc_network_stack.py for the full code.
Demo web application stack
In the GenerativeAiDemoWebStack
stack we launch Lambda functions and respective Amazon API Gateway endpoints through which the web application interacts with the SageMaker model endpoints. See the following code snippet:
# Defines an AWS Lambda function for Image Generation service
lambda_txt2img = _lambda.Function(
self, "lambda_txt2img",
runtime=_lambda.Runtime.PYTHON_3_9,
code=_lambda.Code.from_asset("code/lambda_txt2img"),
handler="txt2img.lambda_handler",
role=role,
timeout=Duration.seconds(180),
memory_size=512,
vpc_subnets=ec2.SubnetSelection(
subnet_type=ec2.SubnetType.PRIVATE_WITH_EGRESS
),
vpc=vpc
)
# Defines an Amazon API Gateway endpoint for Image Generation service
txt2img_apigw_endpoint = apigw.LambdaRestApi(
self, "txt2img_apigw_endpoint",
handler=lambda_txt2img
)
The web application is containerized and hosted on Amazon ECS with Fargate. See the following code snippet:
# Create Fargate service
fargate_service = ecs_patterns.ApplicationLoadBalancedFargateService(
self, "WebApplication",
cluster=cluster, # Required
cpu=2048, # Default is 256 (512 is 0.5 vCPU, 2048 is 2 vCPU)
desired_count=1, # Default is 1
task_image_options=ecs_patterns.ApplicationLoadBalancedTaskImageOptions(
image=image,
container_port=8501,
),
#load_balancer_name="gen-ai-demo",
memory_limit_mib=4096, # Default is 512
public_load_balancer=True) # Default is True
See /stack/generative_ai_demo_web_stack.py for the full code.
Image generation SageMaker model endpoint stack
The GenerativeAiTxt2imgSagemakerStack
stack creates the image generation model endpoint from SageMaker JumpStart and stores the endpoint name in AWS Systems Manager Parameter Store. This parameter will be used by the web application. See the following code:
endpoint = SageMakerEndpointConstruct(self, "TXT2IMG",
project_prefix = "GenerativeAiDemo",
role_arn= role.role_arn,
model_name = "StableDiffusionText2Img",
model_bucket_name = model_info["model_bucket_name"],
model_bucket_key = model_info["model_bucket_key"],
model_docker_image = model_info["model_docker_image"],
variant_name = "AllTraffic",
variant_weight = 1,
instance_count = 1,
instance_type = model_info["instance_type"],
environment = {
"MMS_MAX_RESPONSE_SIZE": "20000000",
"SAGEMAKER_CONTAINER_LOG_LEVEL": "20",
"SAGEMAKER_PROGRAM": "inference.py",
"SAGEMAKER_REGION": model_info["region_name"],
"SAGEMAKER_SUBMIT_DIRECTORY": "/opt/ml/model/code",
},
deploy_enable = True
)
ssm.StringParameter(self, "txt2img_sm_endpoint", parameter_name="txt2img_sm_endpoint", string_value=endpoint.endpoint_name)
See /stack/generative_ai_txt2img_sagemaker_stack.py for the full code.
NLU and text generation SageMaker model endpoint stack
The GenerativeAiTxt2nluSagemakerStack
stack creates the NLU and text generation model endpoint from JumpStart and stores the endpoint name in Systems Manager Parameter Store. This parameter will also be used by the web application. See the following code:
endpoint = SageMakerEndpointConstruct(self, "TXT2NLU",
project_prefix = "GenerativeAiDemo",
role_arn= role.role_arn,
model_name = "HuggingfaceText2TextFlan",
model_bucket_name = model_info["model_bucket_name"],
model_bucket_key = model_info["model_bucket_key"],
model_docker_image = model_info["model_docker_image"],
variant_name = "AllTraffic",
variant_weight = 1,
instance_count = 1,
instance_type = model_info["instance_type"],
environment = {
"MODEL_CACHE_ROOT": "/opt/ml/model",
"SAGEMAKER_ENV": "1",
"SAGEMAKER_MODEL_SERVER_TIMEOUT": "3600",
"SAGEMAKER_MODEL_SERVER_WORKERS": "1",
"SAGEMAKER_PROGRAM": "inference.py",
"SAGEMAKER_SUBMIT_DIRECTORY": "/opt/ml/model/code/",
"TS_DEFAULT_WORKERS_PER_MODEL": "1"
},
deploy_enable = True
)
ssm.StringParameter(self, "txt2nlu_sm_endpoint", parameter_name="txt2nlu_sm_endpoint", string_value=endpoint.endpoint_name)
See /stack/generative_ai_txt2nlu_sagemaker_stack.py for the full code.
The Web application
The web application is located in the /web-app directory. It is a Streamlit application that is containerized as per the Dockerfile:
FROM --platform=linux/x86_64 python:3.9
EXPOSE 8501
WORKDIR /app
COPY requirements.txt ./requirements.txt
RUN pip3 install -r requirements.txt
COPY . .
CMD streamlit run Home.py \
--server.headless true \
--browser.serverAddress="0.0.0.0" \
--server.enableCORS false \
--browser.gatherUsageStats false
To learn more about Streamlit, see Streamlit documentation.
For Tasks:
Click tags to check more tools for each tasksFor Jobs:
Alternative AI tools for generative-ai-sagemaker-cdk-demo
Similar Open Source Tools
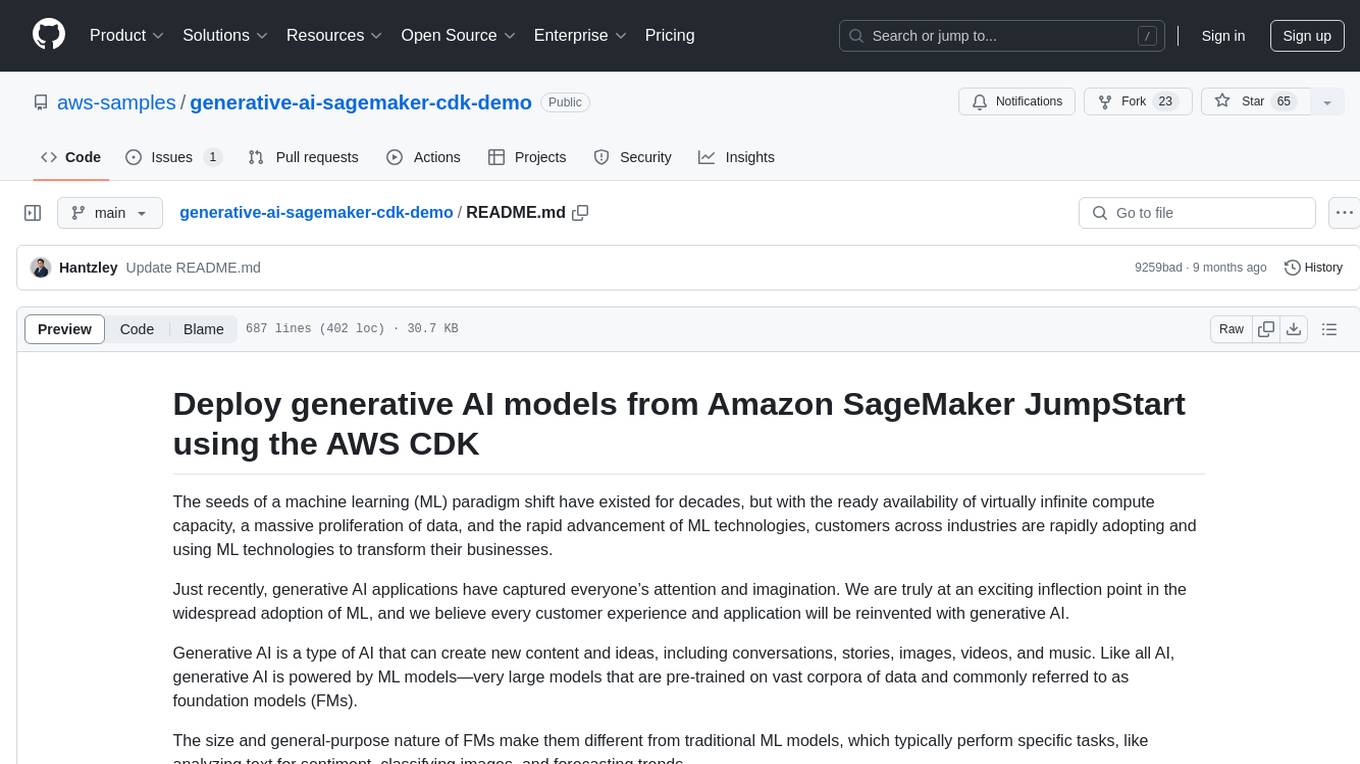
generative-ai-sagemaker-cdk-demo
This repository showcases how to deploy generative AI models from Amazon SageMaker JumpStart using the AWS CDK. Generative AI is a type of AI that can create new content and ideas, such as conversations, stories, images, videos, and music. The repository provides a detailed guide on deploying image and text generative AI models, utilizing pre-trained models from SageMaker JumpStart. The web application is built on Streamlit and hosted on Amazon ECS with Fargate. It interacts with the SageMaker model endpoints through Lambda functions and Amazon API Gateway. The repository also includes instructions on setting up the AWS CDK application, deploying the stacks, using the models, and viewing the deployed resources on the AWS Management Console.
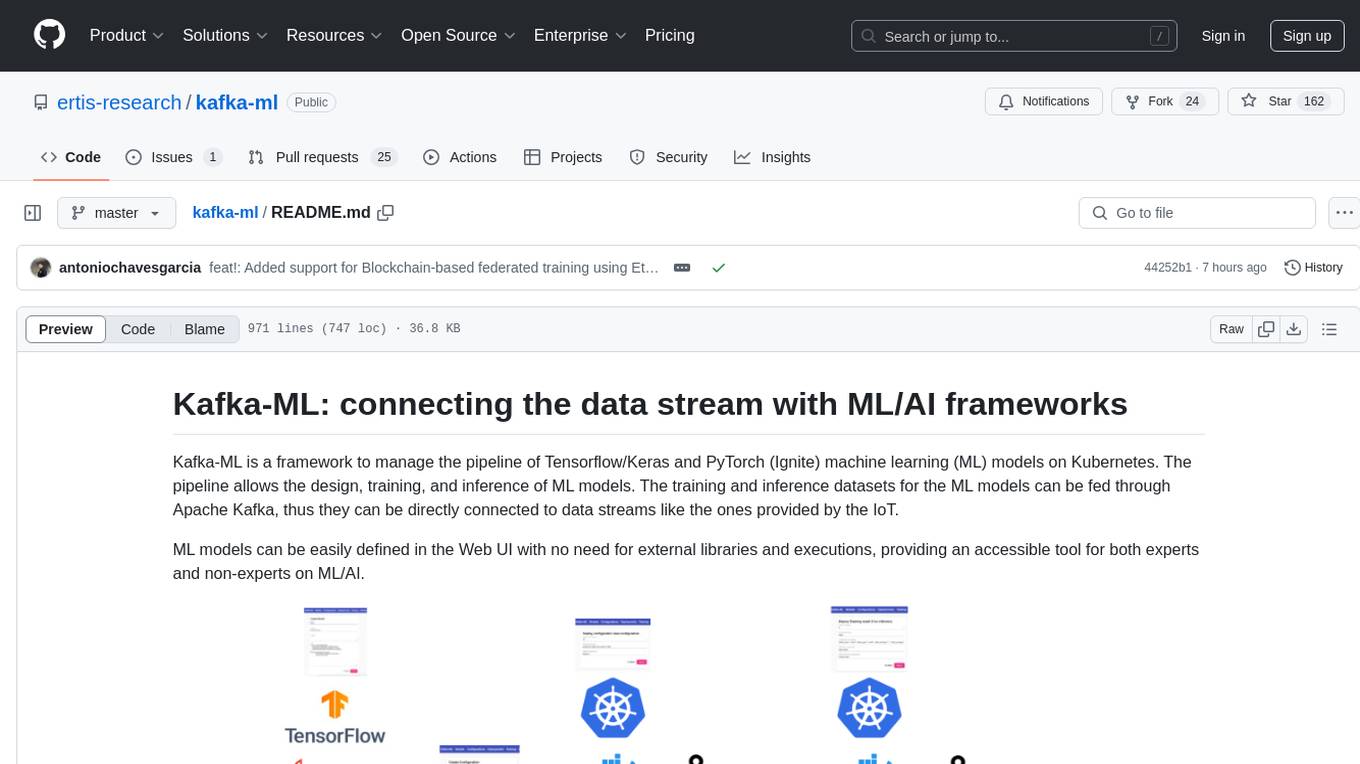
kafka-ml
Kafka-ML is a framework designed to manage the pipeline of Tensorflow/Keras and PyTorch machine learning models on Kubernetes. It enables the design, training, and inference of ML models with datasets fed through Apache Kafka, connecting them directly to data streams like those from IoT devices. The Web UI allows easy definition of ML models without external libraries, catering to both experts and non-experts in ML/AI.
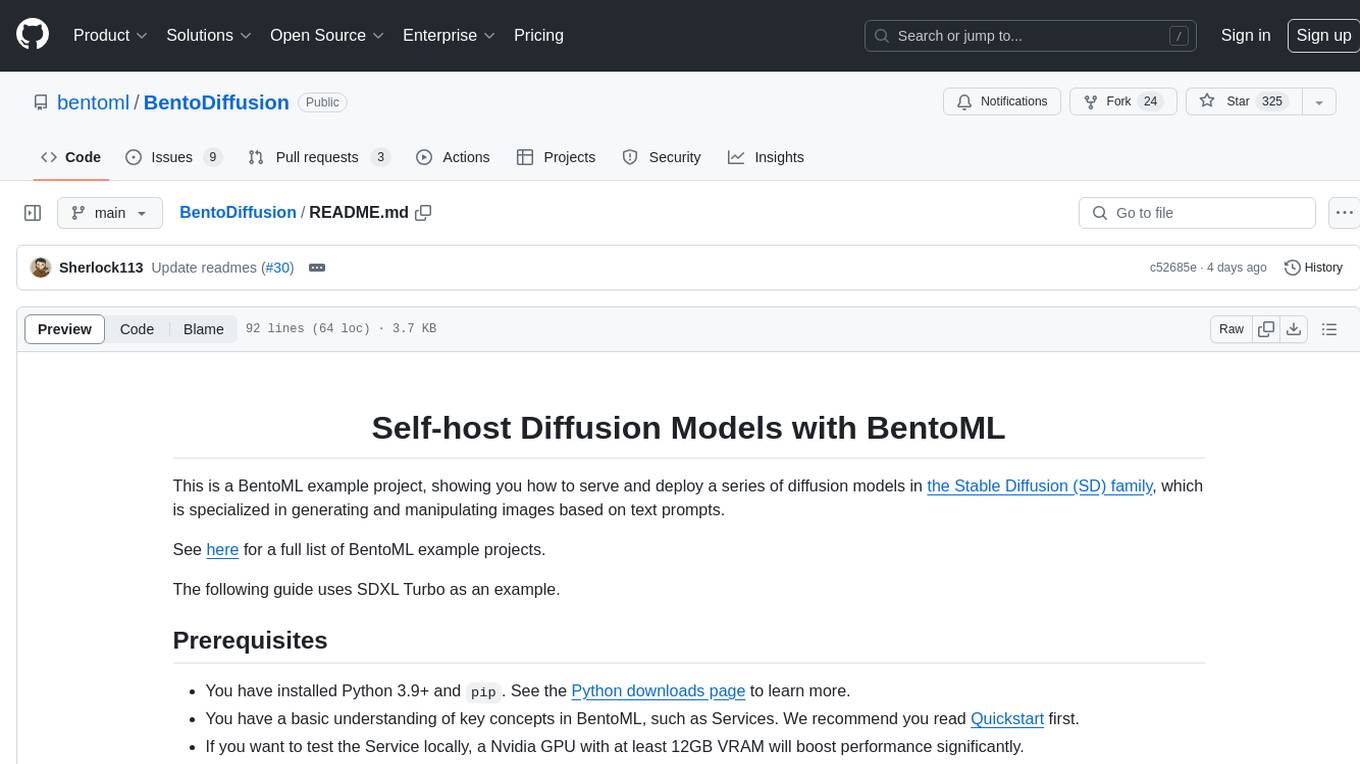
BentoDiffusion
BentoDiffusion is a BentoML example project that demonstrates how to serve and deploy diffusion models in the Stable Diffusion (SD) family. These models are specialized in generating and manipulating images based on text prompts. The project provides a guide on using SDXL Turbo as an example, along with instructions on prerequisites, installing dependencies, running the BentoML service, and deploying to BentoCloud. Users can interact with the deployed service using Swagger UI or other methods. Additionally, the project offers the option to choose from various diffusion models available in the repository for deployment.
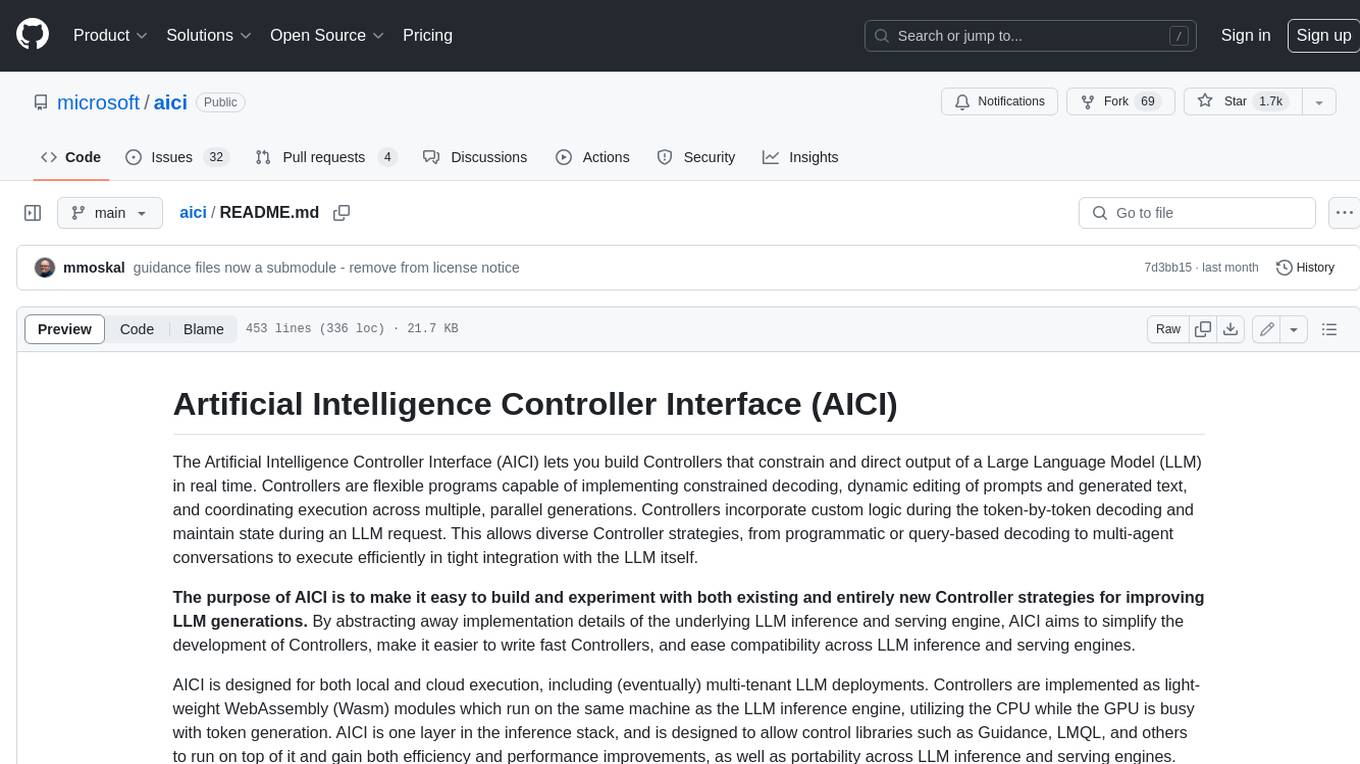
aici
The Artificial Intelligence Controller Interface (AICI) lets you build Controllers that constrain and direct output of a Large Language Model (LLM) in real time. Controllers are flexible programs capable of implementing constrained decoding, dynamic editing of prompts and generated text, and coordinating execution across multiple, parallel generations. Controllers incorporate custom logic during the token-by-token decoding and maintain state during an LLM request. This allows diverse Controller strategies, from programmatic or query-based decoding to multi-agent conversations to execute efficiently in tight integration with the LLM itself.
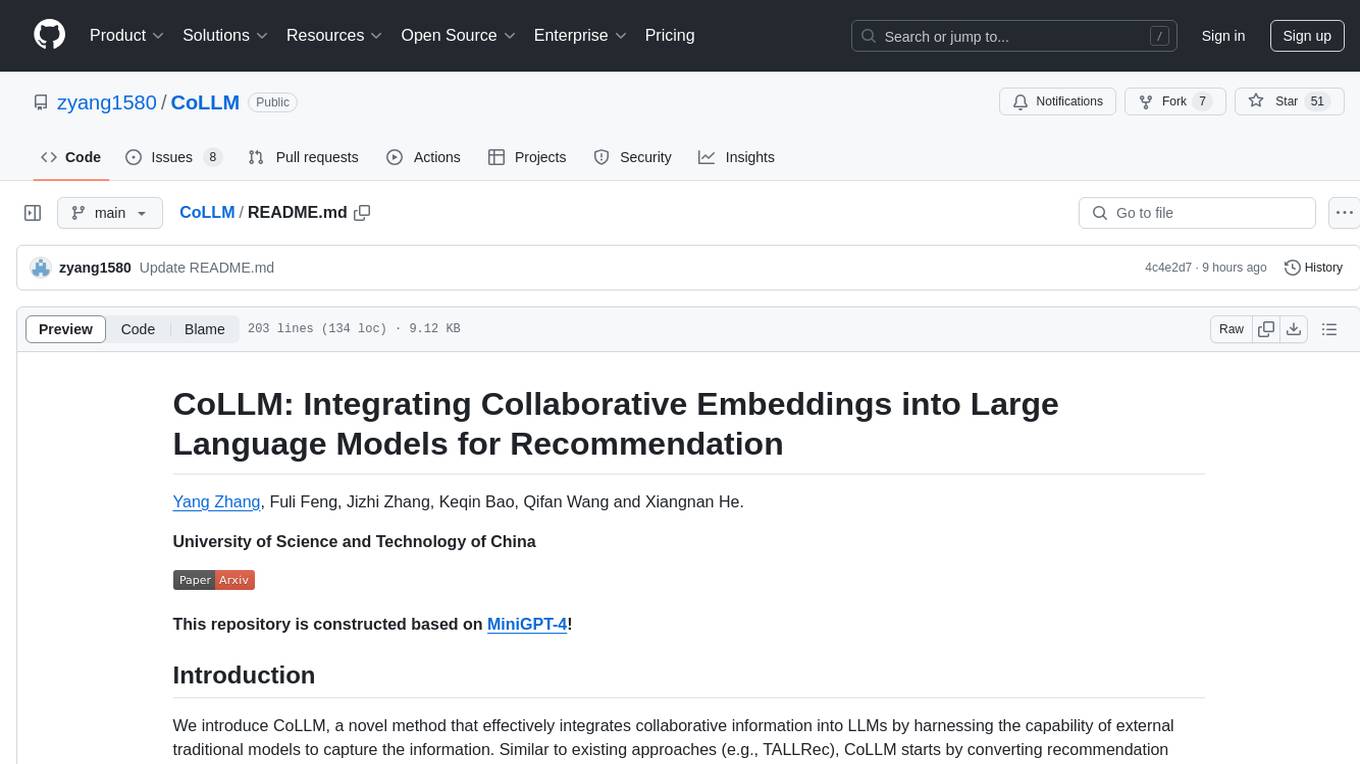
CoLLM
CoLLM is a novel method that integrates collaborative information into Large Language Models (LLMs) for recommendation. It converts recommendation data into language prompts, encodes them with both textual and collaborative information, and uses a two-step tuning method to train the model. The method incorporates user/item ID fields in prompts and employs a conventional collaborative model to generate user/item representations. CoLLM is built upon MiniGPT-4 and utilizes pretrained Vicuna weights for training.
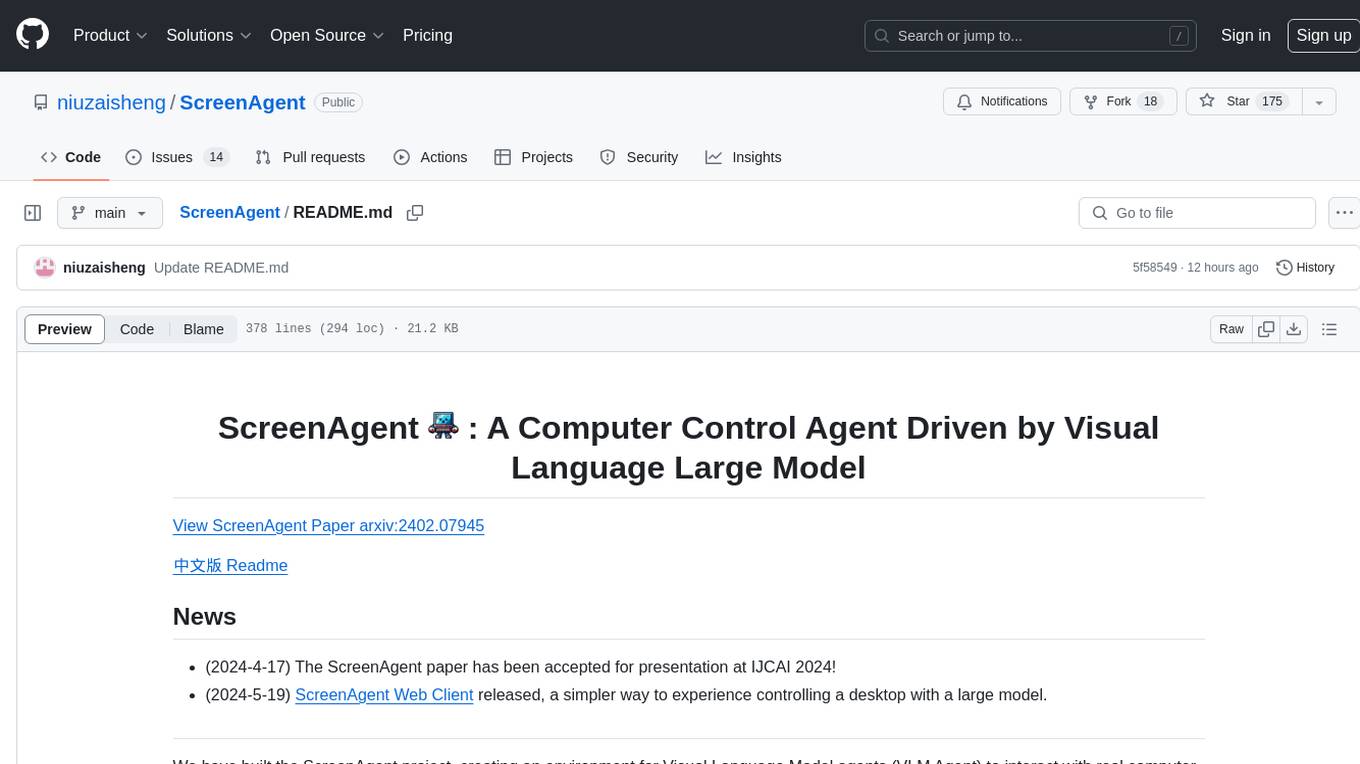
ScreenAgent
ScreenAgent is a project focused on creating an environment for Visual Language Model agents (VLM Agent) to interact with real computer screens. The project includes designing an automatic control process for agents to interact with the environment and complete multi-step tasks. It also involves building the ScreenAgent dataset, which collects screenshots and action sequences for various daily computer tasks. The project provides a controller client code, configuration files, and model training code to enable users to control a desktop with a large model.
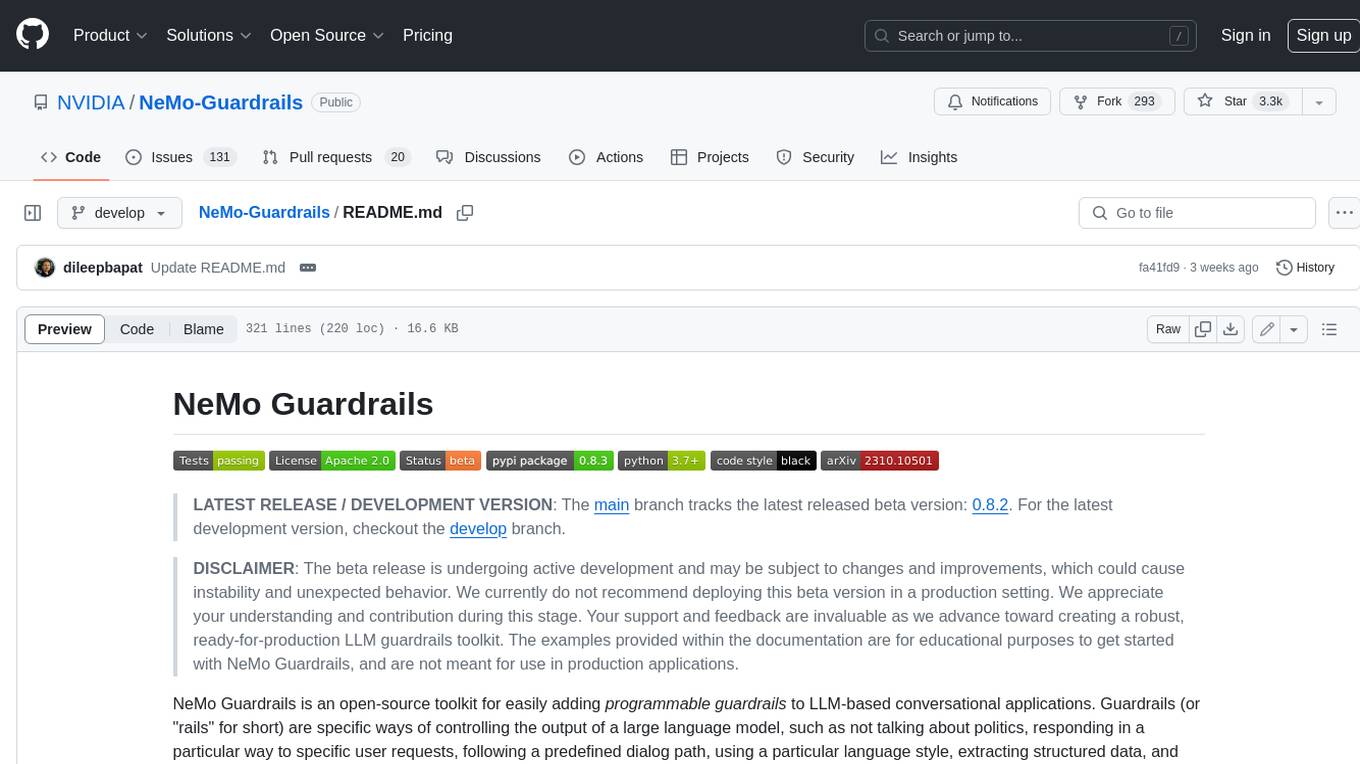
NeMo-Guardrails
NeMo Guardrails is an open-source toolkit for easily adding _programmable guardrails_ to LLM-based conversational applications. Guardrails (or "rails" for short) are specific ways of controlling the output of a large language model, such as not talking about politics, responding in a particular way to specific user requests, following a predefined dialog path, using a particular language style, extracting structured data, and more.
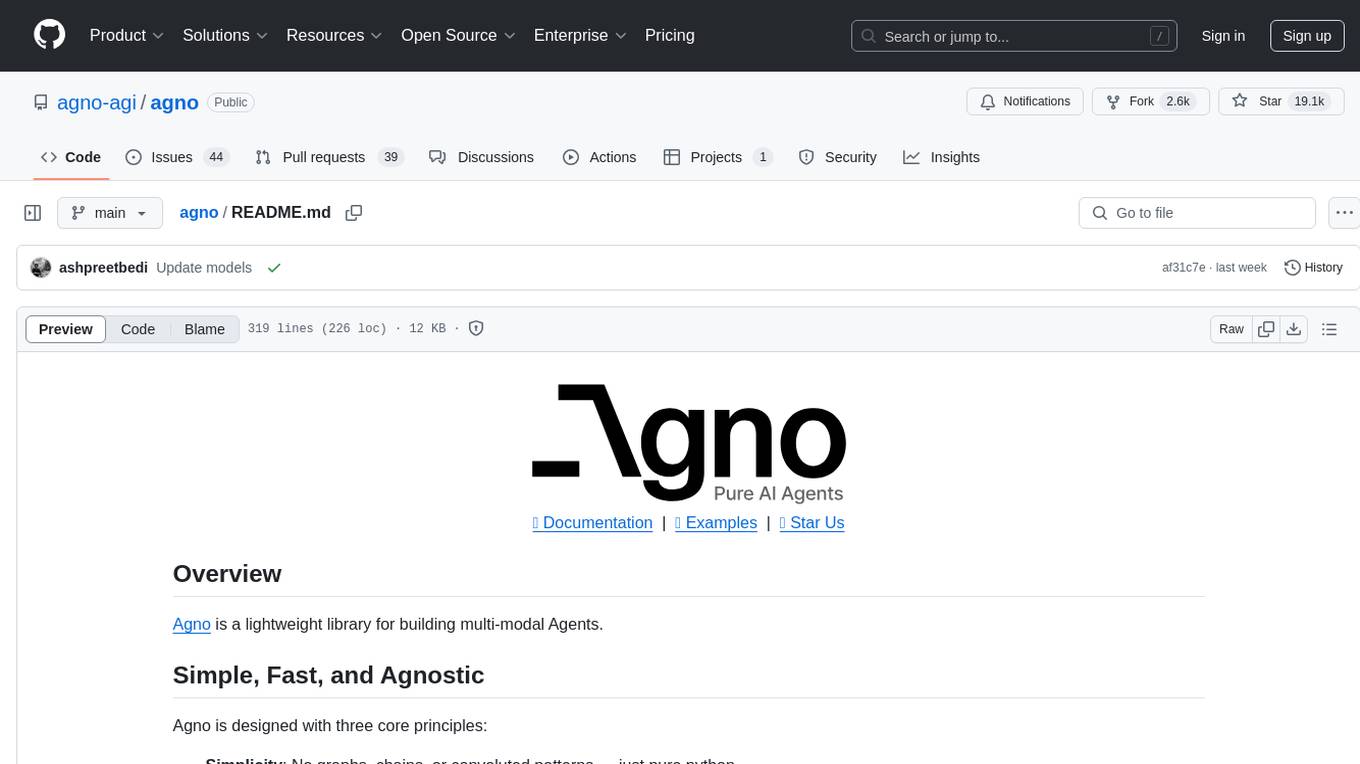
agno
Agno is a lightweight library for building multi-modal Agents. It is designed with core principles of simplicity, uncompromising performance, and agnosticism, allowing users to create blazing fast agents with minimal memory footprint. Agno supports any model, any provider, and any modality, making it a versatile container for AGI. Users can build agents with lightning-fast agent creation, model agnostic capabilities, native support for text, image, audio, and video inputs and outputs, memory management, knowledge stores, structured outputs, and real-time monitoring. The library enables users to create autonomous programs that use language models to solve problems, improve responses, and achieve tasks with varying levels of agency and autonomy.
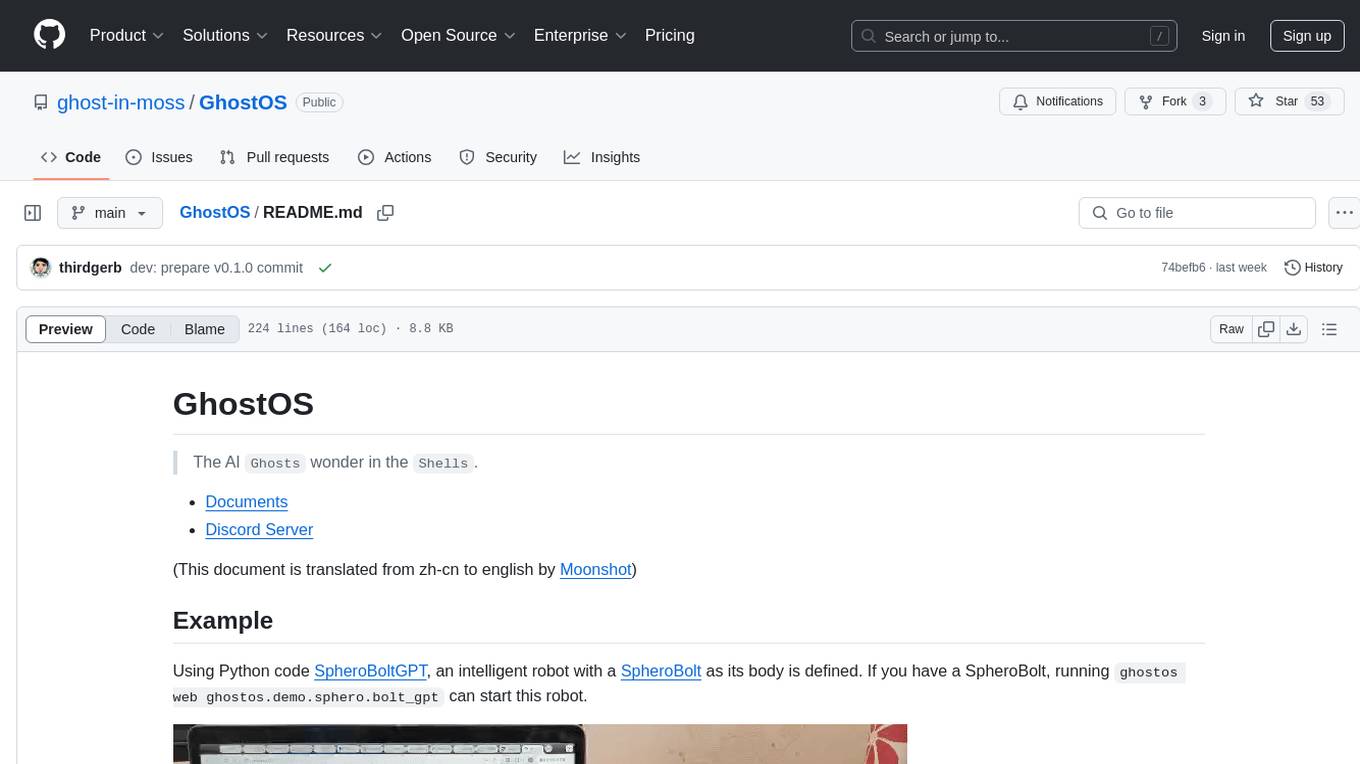
GhostOS
GhostOS is an AI Agent framework designed to replace JSON Schema with a Turing-complete code interaction interface (Moss Protocol). It aims to create intelligent entities capable of continuous learning and growth through code generation and project management. The framework supports various capabilities such as turning Python files into web agents, real-time voice conversation, body movements control, and emotion expression. GhostOS is still in early experimental development and focuses on out-of-the-box capabilities for AI agents.
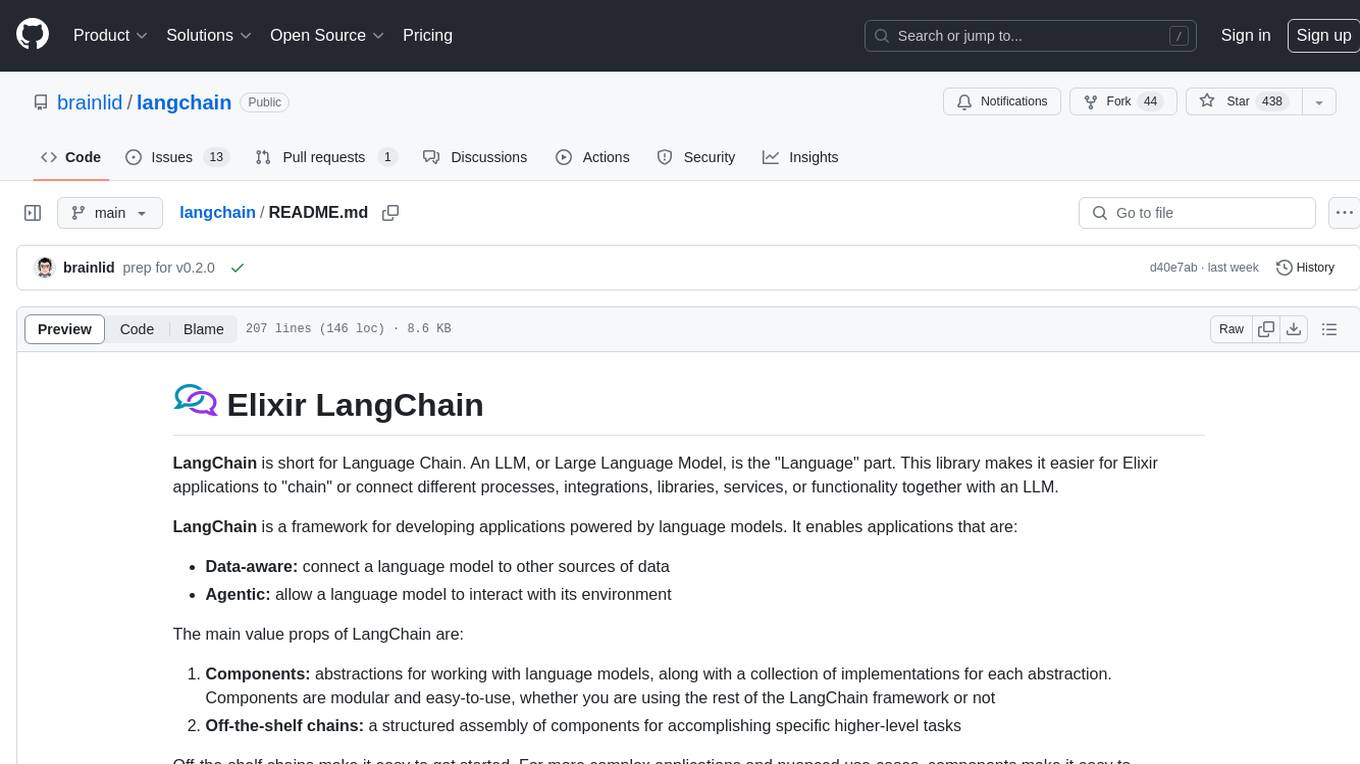
langchain
LangChain is a framework for developing Elixir applications powered by language models. It enables applications to connect language models to other data sources and interact with the environment. The library provides components for working with language models and off-the-shelf chains for specific tasks. It aims to assist in building applications that combine large language models with other sources of computation or knowledge. LangChain is written in Elixir and is not aimed for parity with the JavaScript and Python versions due to differences in programming paradigms and design choices. The library is designed to make it easy to integrate language models into applications and expose features, data, and functionality to the models.
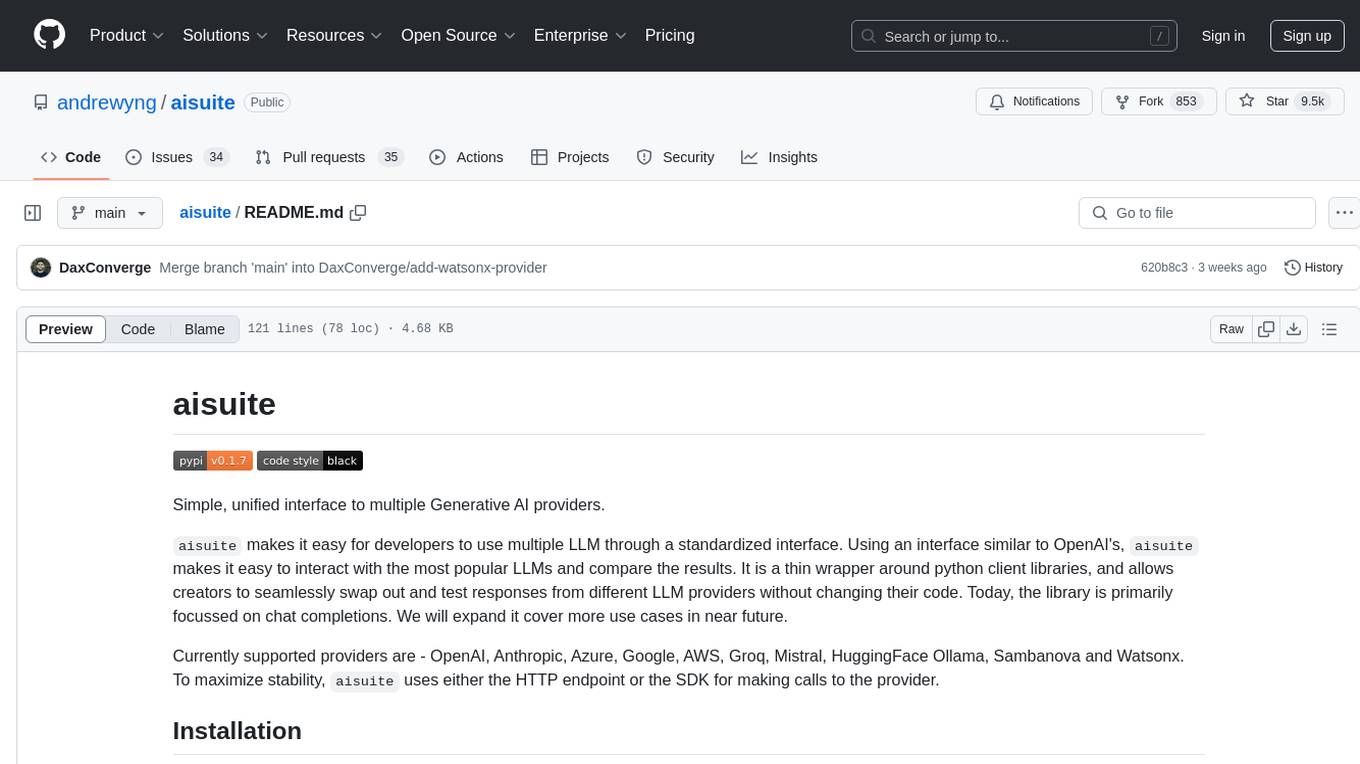
aisuite
Aisuite is a simple, unified interface to multiple Generative AI providers. It allows developers to easily interact with various Language Model (LLM) providers like OpenAI, Anthropic, Azure, Google, AWS, and more through a standardized interface. The library focuses on chat completions and provides a thin wrapper around python client libraries, enabling creators to test responses from different LLM providers without changing their code. Aisuite maximizes stability by using HTTP endpoints or SDKs for making calls to the providers. Users can install the base package or specific provider packages, set up API keys, and utilize the library to generate chat completion responses from different models.
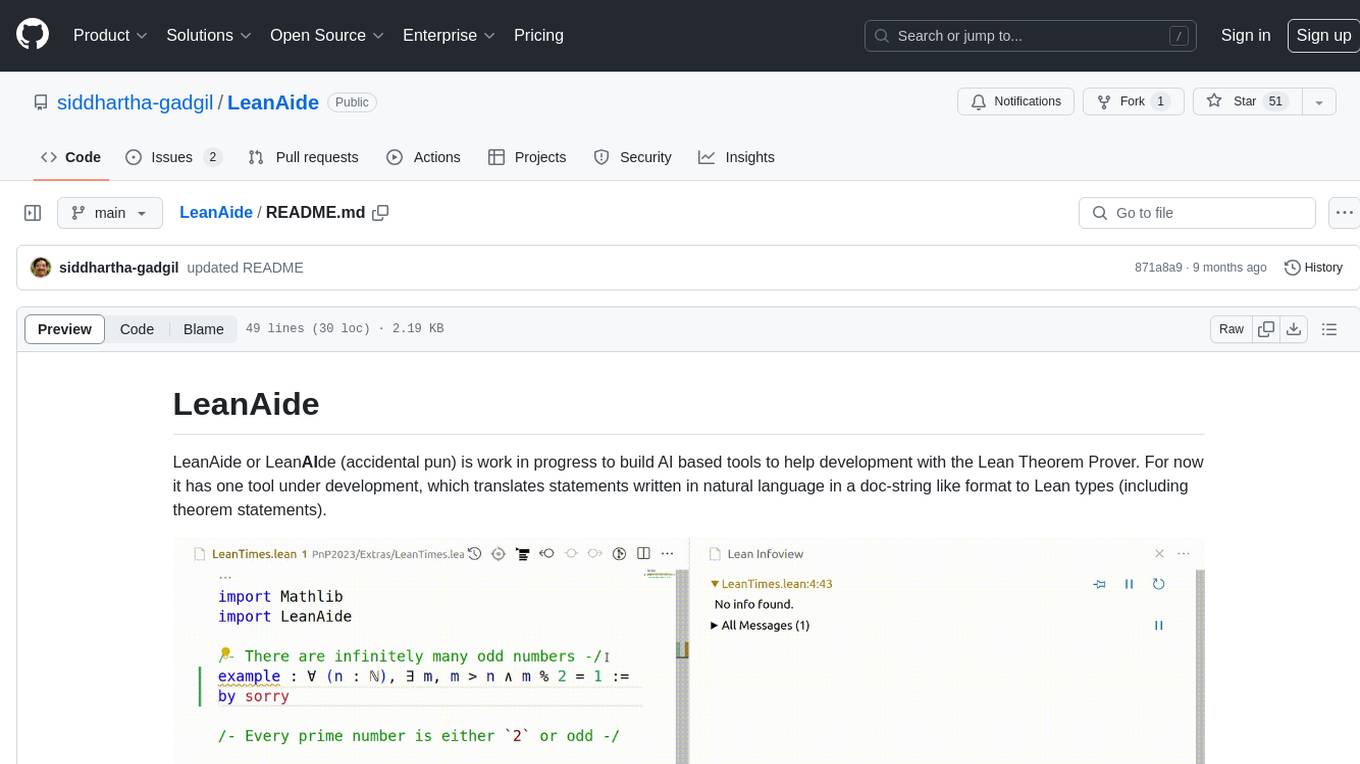
LeanAide
LeanAide is a work in progress AI tool designed to assist with development using the Lean Theorem Prover. It currently offers a tool that translates natural language statements to Lean types, including theorem statements. The tool is based on GPT 3.5-turbo/GPT 4 and requires an OpenAI key for usage. Users can include LeanAide as a dependency in their projects to access the translation functionality.
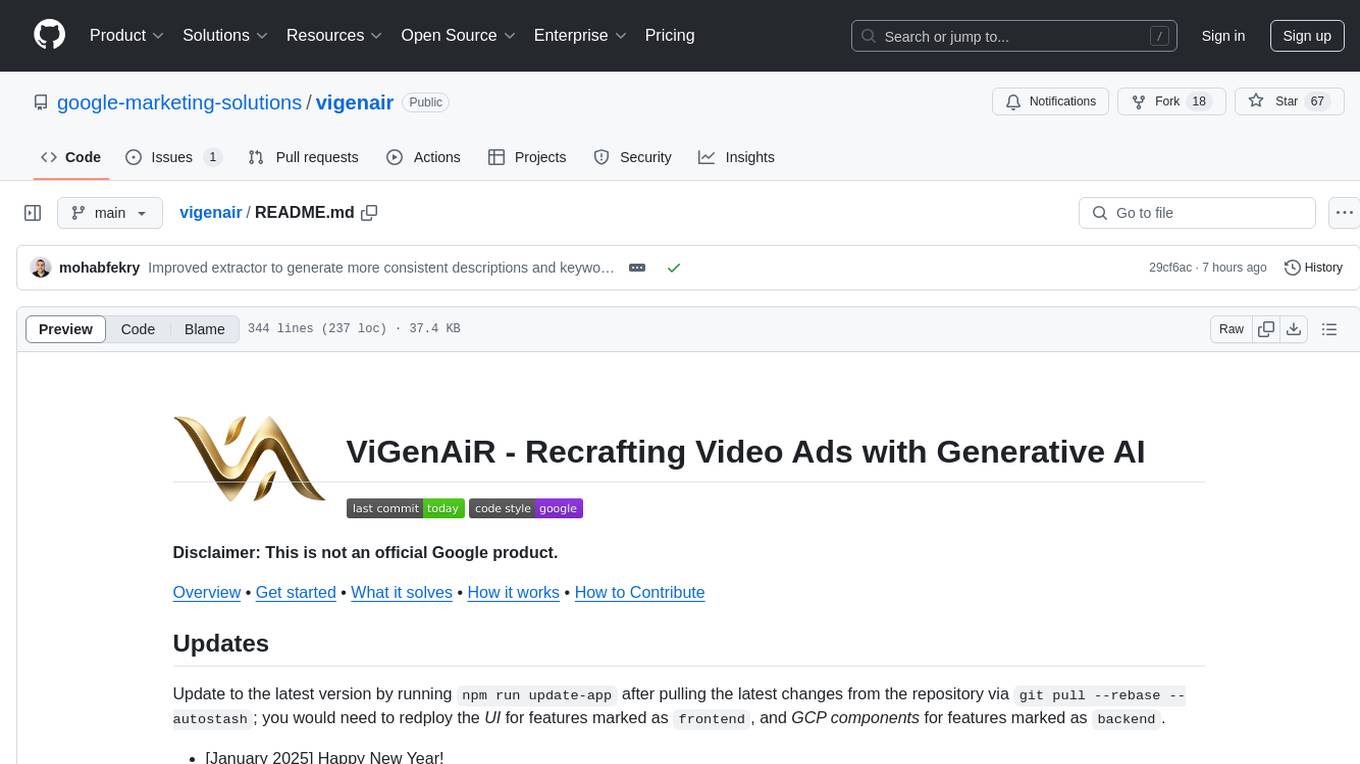
vigenair
ViGenAiR is a tool that harnesses the power of Generative AI models on Google Cloud Platform to automatically transform long-form Video Ads into shorter variants, targeting different audiences. It generates video, image, and text assets for Demand Gen and YouTube video campaigns. Users can steer the model towards generating desired videos, conduct A/B testing, and benefit from various creative features. The tool offers benefits like diverse inventory, compelling video ads, creative excellence, user control, and performance insights. ViGenAiR works by analyzing video content, splitting it into coherent segments, and generating variants following Google's best practices for effective ads.
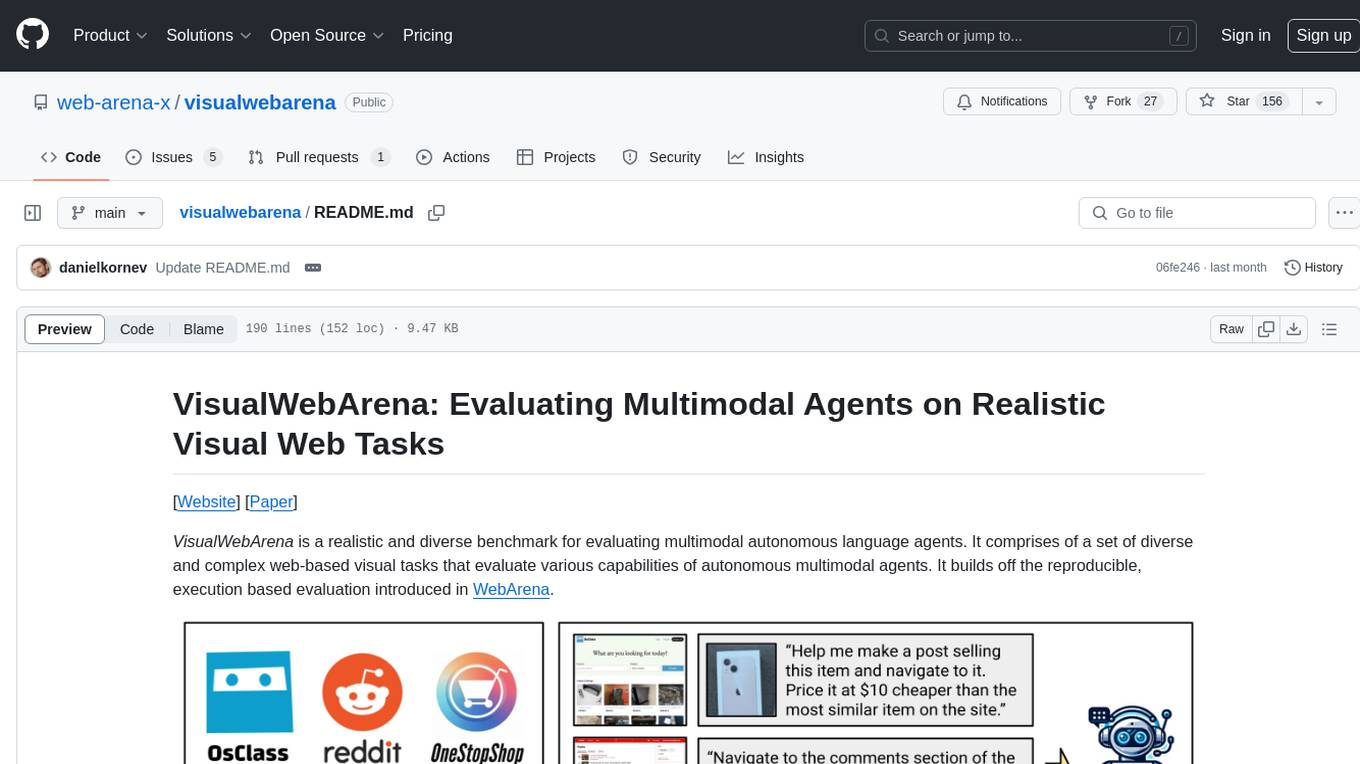
visualwebarena
VisualWebArena is a benchmark for evaluating multimodal autonomous language agents through diverse and complex web-based visual tasks. It builds on the reproducible evaluation introduced in WebArena. The repository provides scripts for end-to-end training, demos to run multimodal agents on webpages, and tools for setting up environments for evaluation. It includes trajectories of the GPT-4V + SoM agent on VWA tasks, along with human evaluations on 233 tasks. The environment supports OpenAI models and Gemini models for evaluation.
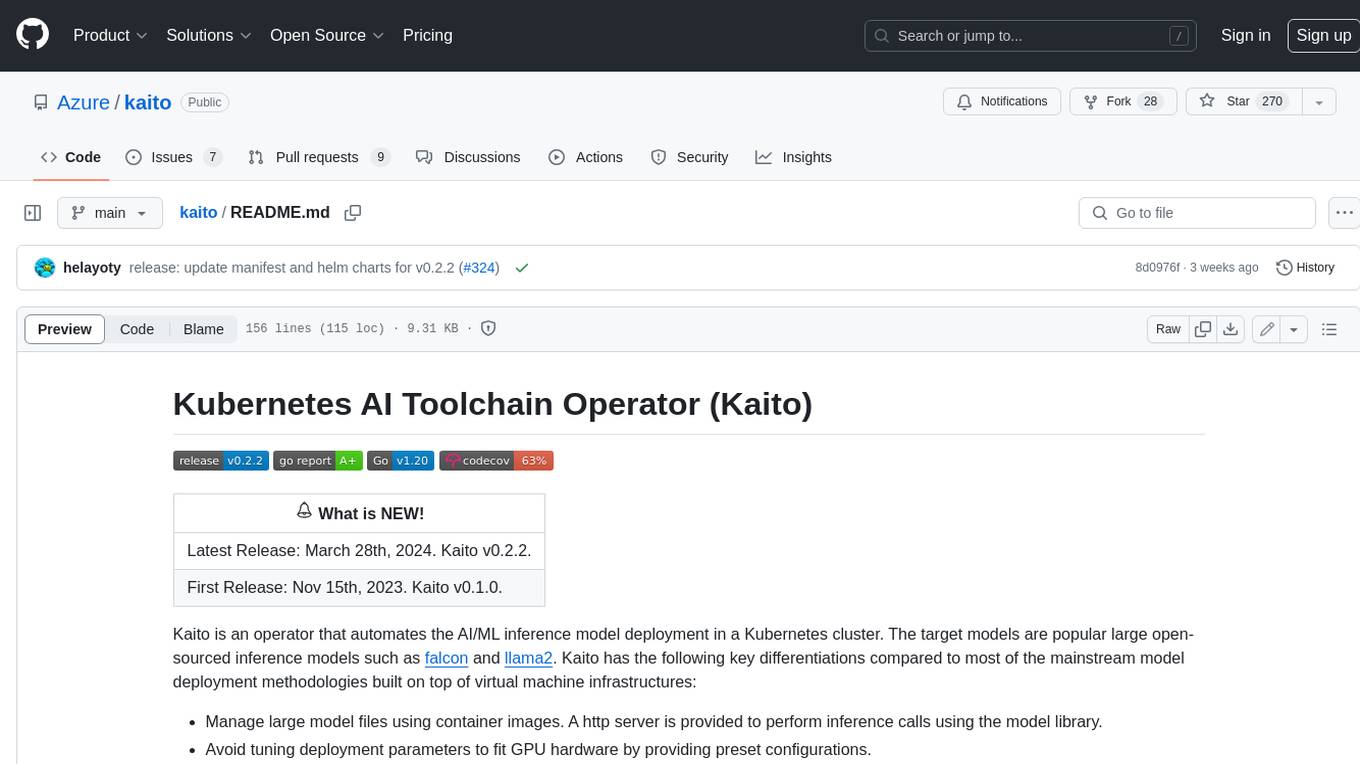
kaito
Kaito is an operator that automates the AI/ML inference model deployment in a Kubernetes cluster. It manages large model files using container images, avoids tuning deployment parameters to fit GPU hardware by providing preset configurations, auto-provisions GPU nodes based on model requirements, and hosts large model images in the public Microsoft Container Registry (MCR) if the license allows. Using Kaito, the workflow of onboarding large AI inference models in Kubernetes is largely simplified.
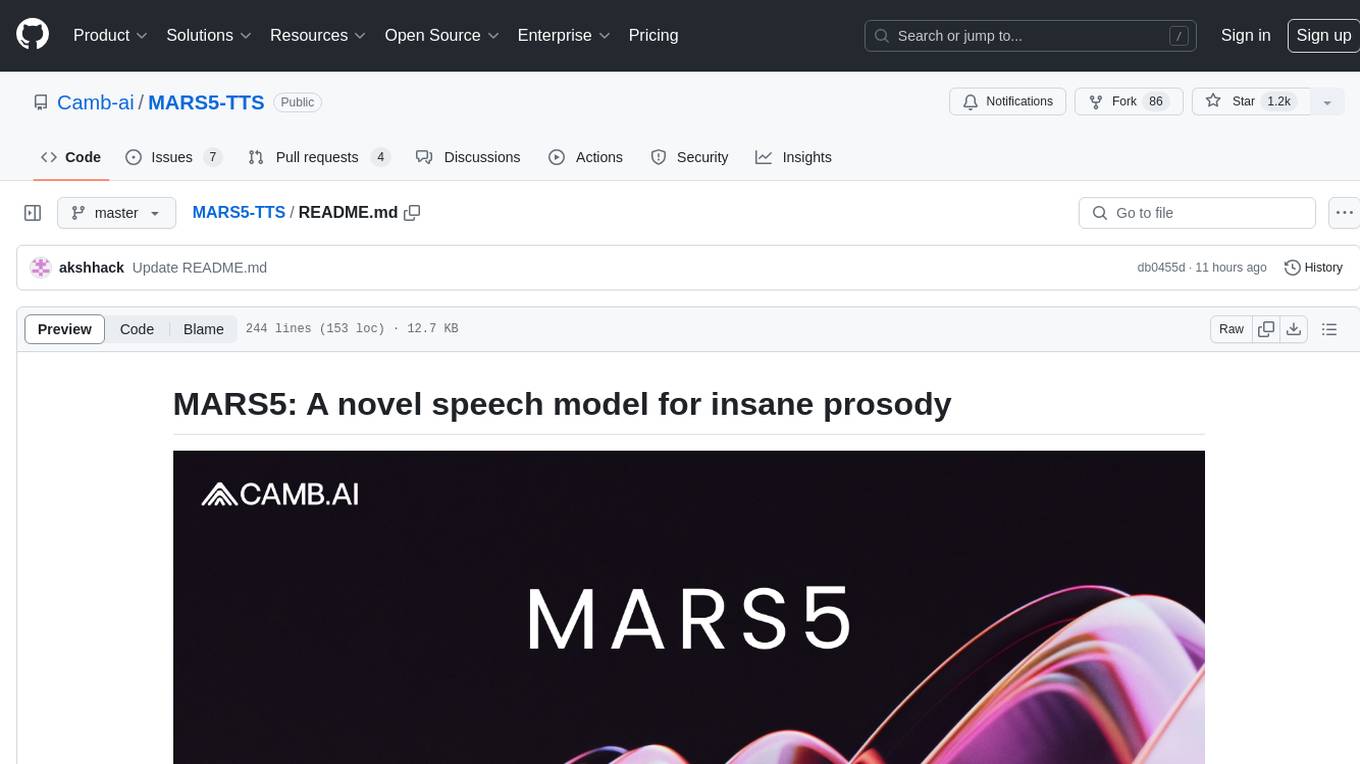
MARS5-TTS
MARS5 is a novel English speech model (TTS) developed by CAMB.AI, featuring a two-stage AR-NAR pipeline with a unique NAR component. The model can generate speech for various scenarios like sports commentary and anime with just 5 seconds of audio and a text snippet. It allows steering prosody using punctuation and capitalization in the transcript. Speaker identity is specified using an audio reference file, enabling 'deep clone' for improved quality. The model can be used via torch.hub or HuggingFace, supporting both shallow and deep cloning for inference. Checkpoints are provided for AR and NAR models, with hardware requirements of 750M+450M params on GPU. Contributions to improve model stability, performance, and reference audio selection are welcome.
For similar tasks
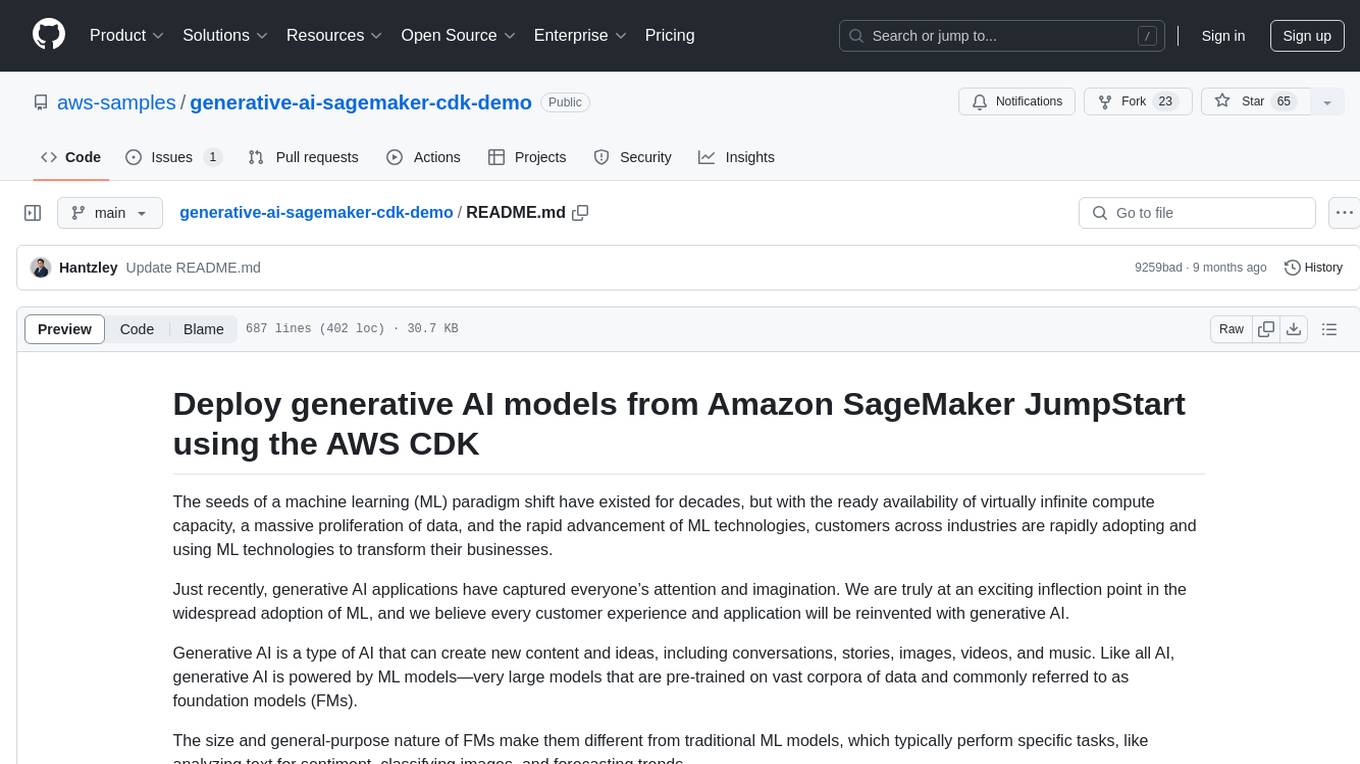
generative-ai-sagemaker-cdk-demo
This repository showcases how to deploy generative AI models from Amazon SageMaker JumpStart using the AWS CDK. Generative AI is a type of AI that can create new content and ideas, such as conversations, stories, images, videos, and music. The repository provides a detailed guide on deploying image and text generative AI models, utilizing pre-trained models from SageMaker JumpStart. The web application is built on Streamlit and hosted on Amazon ECS with Fargate. It interacts with the SageMaker model endpoints through Lambda functions and Amazon API Gateway. The repository also includes instructions on setting up the AWS CDK application, deploying the stacks, using the models, and viewing the deployed resources on the AWS Management Console.
For similar jobs
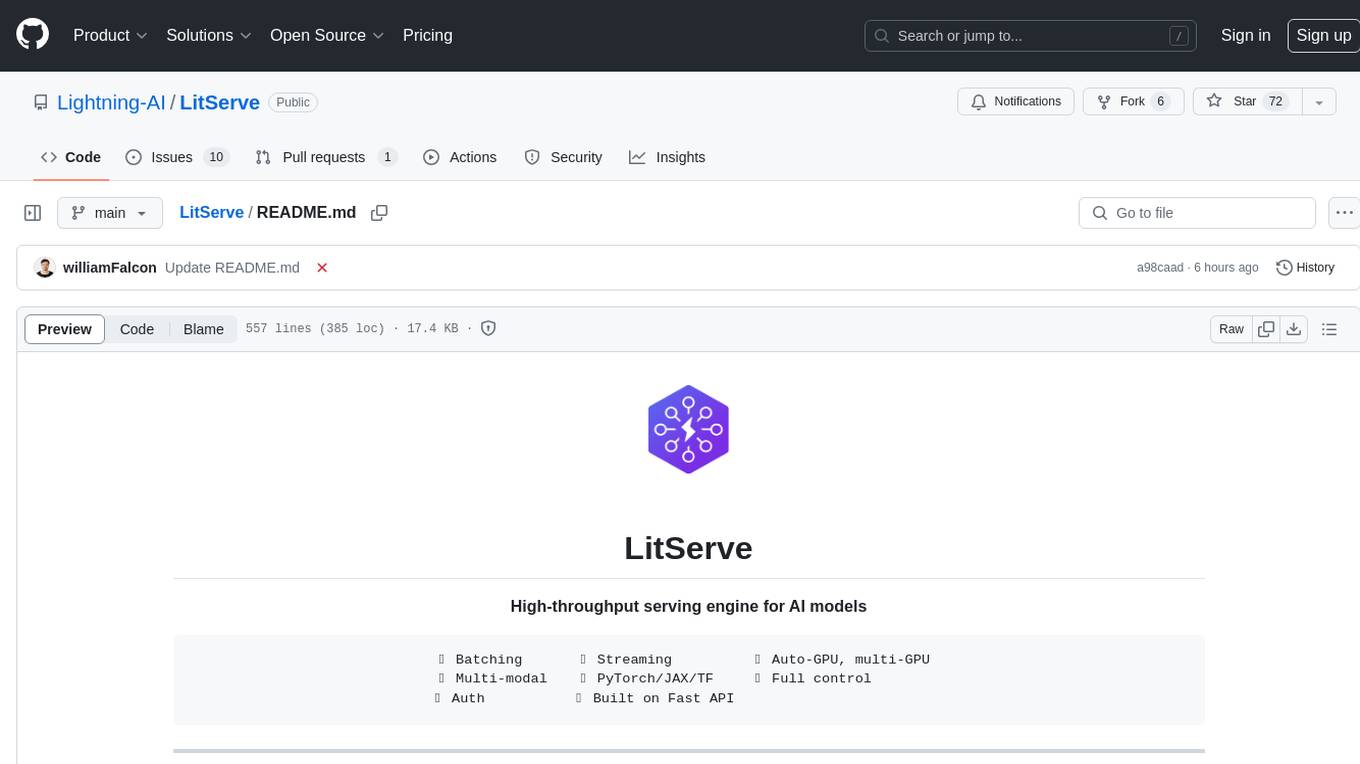
LitServe
LitServe is a high-throughput serving engine designed for deploying AI models at scale. It generates an API endpoint for models, handles batching, streaming, and autoscaling across CPU/GPUs. LitServe is built for enterprise scale with a focus on minimal, hackable code-base without bloat. It supports various model types like LLMs, vision, time-series, and works with frameworks like PyTorch, JAX, Tensorflow, and more. The tool allows users to focus on model performance rather than serving boilerplate, providing full control and flexibility.
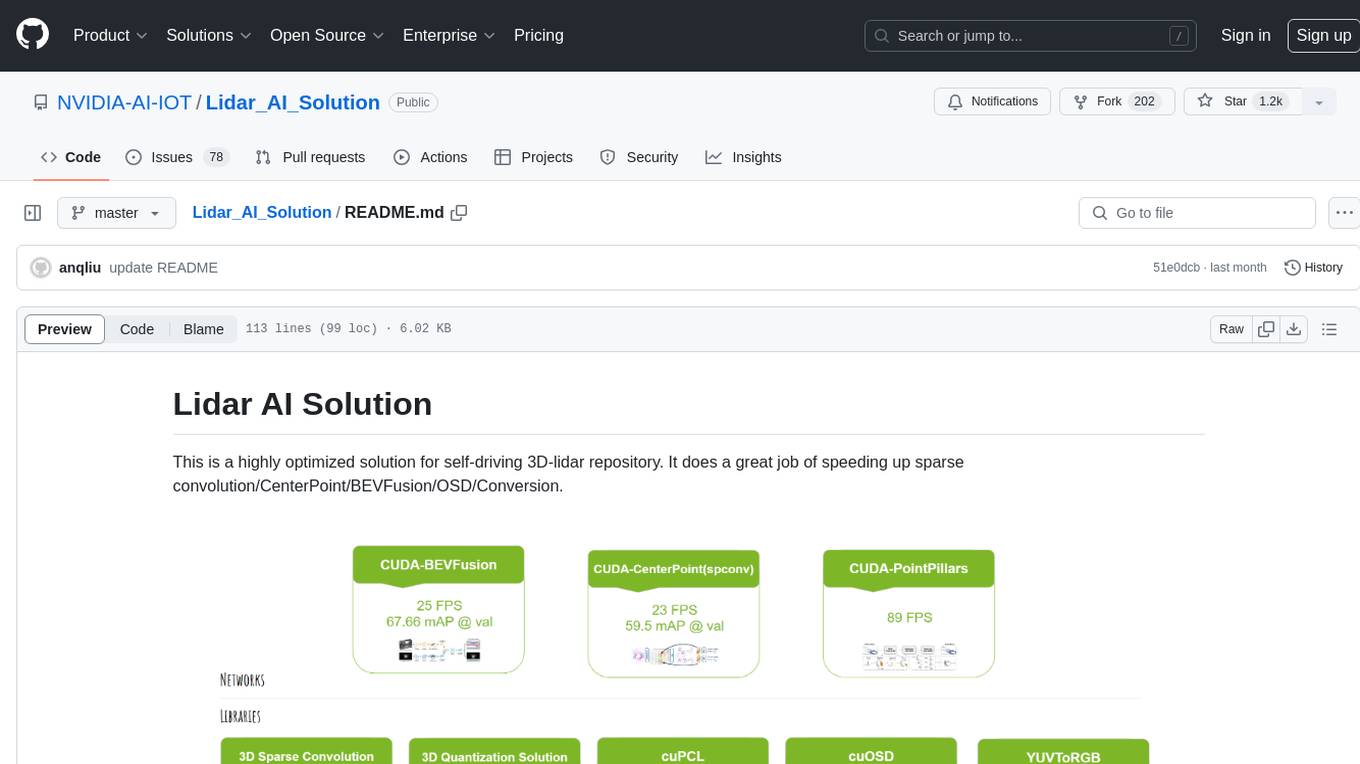
Lidar_AI_Solution
Lidar AI Solution is a highly optimized repository for self-driving 3D lidar, providing solutions for sparse convolution, BEVFusion, CenterPoint, OSD, and Conversion. It includes CUDA and TensorRT implementations for various tasks such as 3D sparse convolution, BEVFusion, CenterPoint, PointPillars, V2XFusion, cuOSD, cuPCL, and YUV to RGB conversion. The repository offers easy-to-use solutions, high accuracy, low memory usage, and quantization options for different tasks related to self-driving technology.
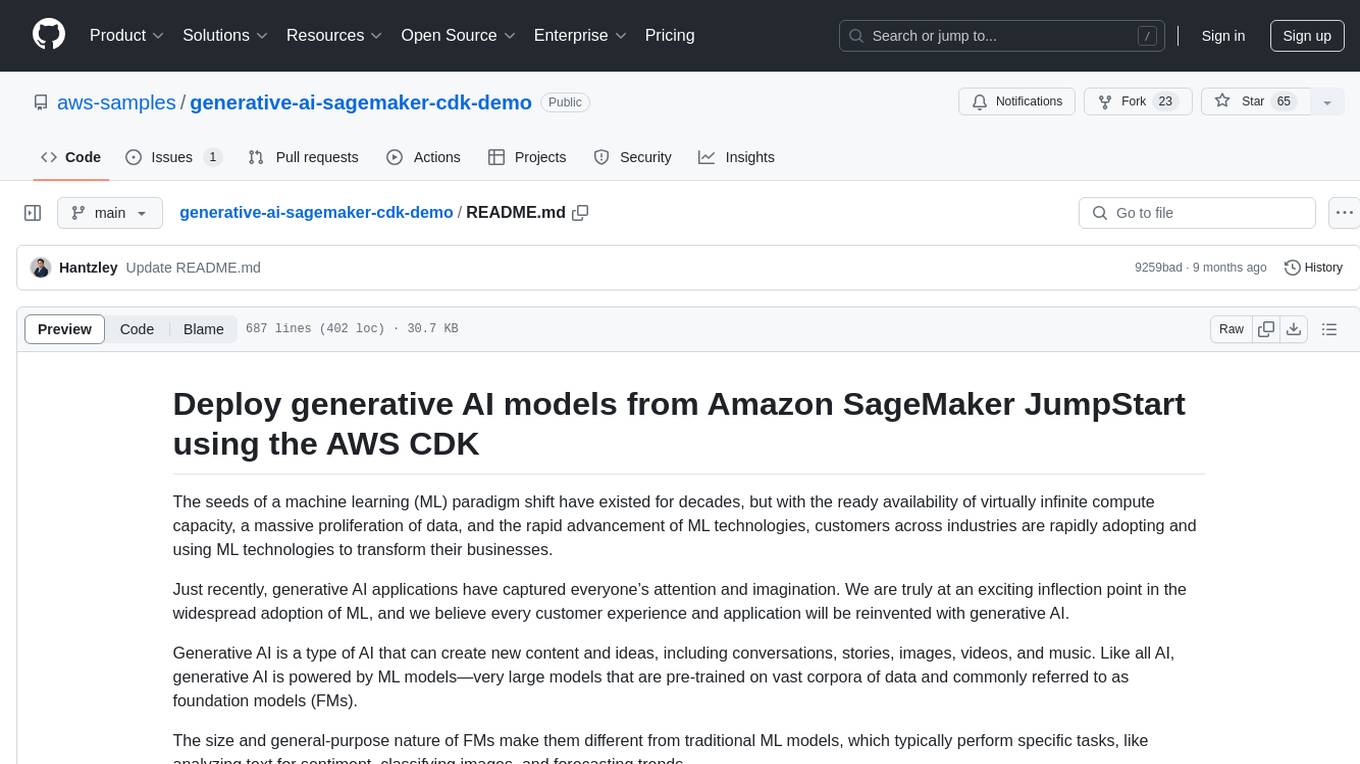
generative-ai-sagemaker-cdk-demo
This repository showcases how to deploy generative AI models from Amazon SageMaker JumpStart using the AWS CDK. Generative AI is a type of AI that can create new content and ideas, such as conversations, stories, images, videos, and music. The repository provides a detailed guide on deploying image and text generative AI models, utilizing pre-trained models from SageMaker JumpStart. The web application is built on Streamlit and hosted on Amazon ECS with Fargate. It interacts with the SageMaker model endpoints through Lambda functions and Amazon API Gateway. The repository also includes instructions on setting up the AWS CDK application, deploying the stacks, using the models, and viewing the deployed resources on the AWS Management Console.
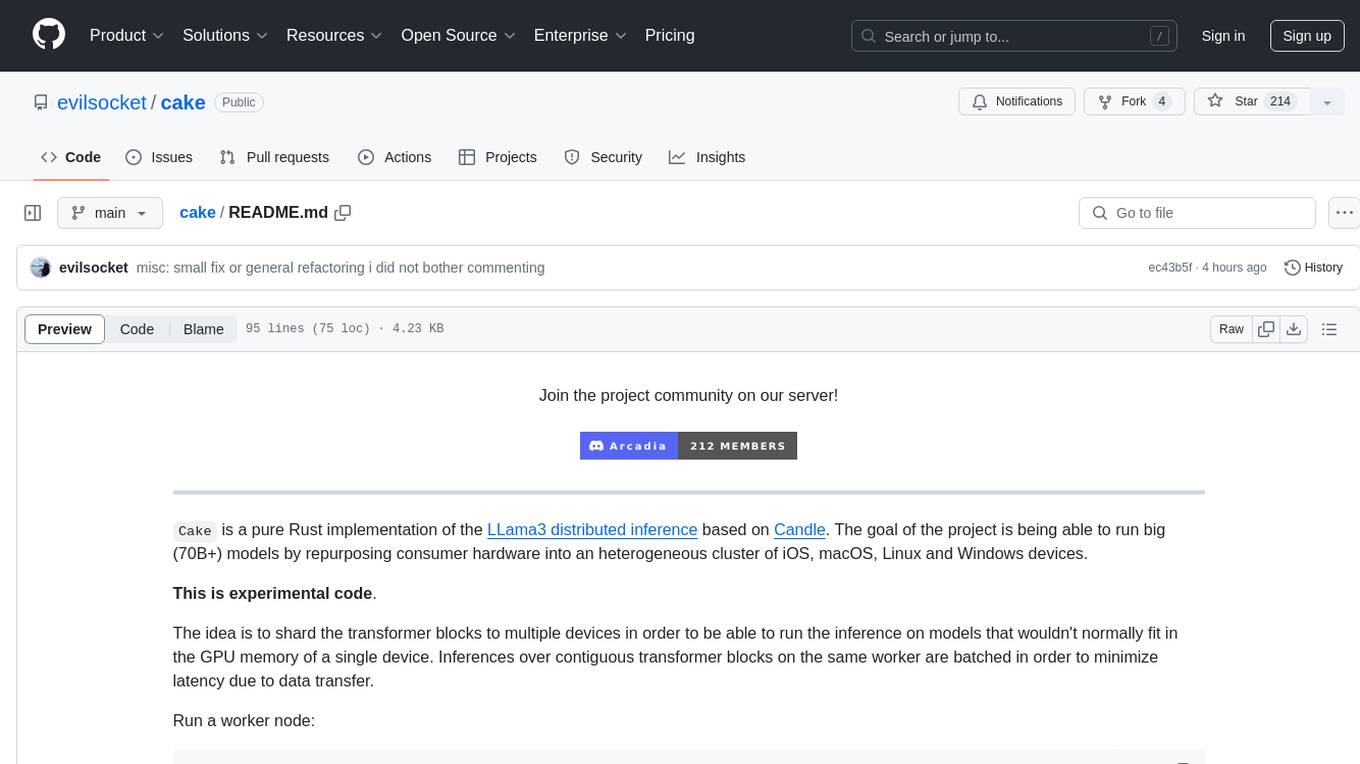
cake
cake is a pure Rust implementation of the llama3 LLM distributed inference based on Candle. The project aims to enable running large models on consumer hardware clusters of iOS, macOS, Linux, and Windows devices by sharding transformer blocks. It allows running inferences on models that wouldn't fit in a single device's GPU memory by batching contiguous transformer blocks on the same worker to minimize latency. The tool provides a way to optimize memory and disk space by splitting the model into smaller bundles for workers, ensuring they only have the necessary data. cake supports various OS, architectures, and accelerations, with different statuses for each configuration.
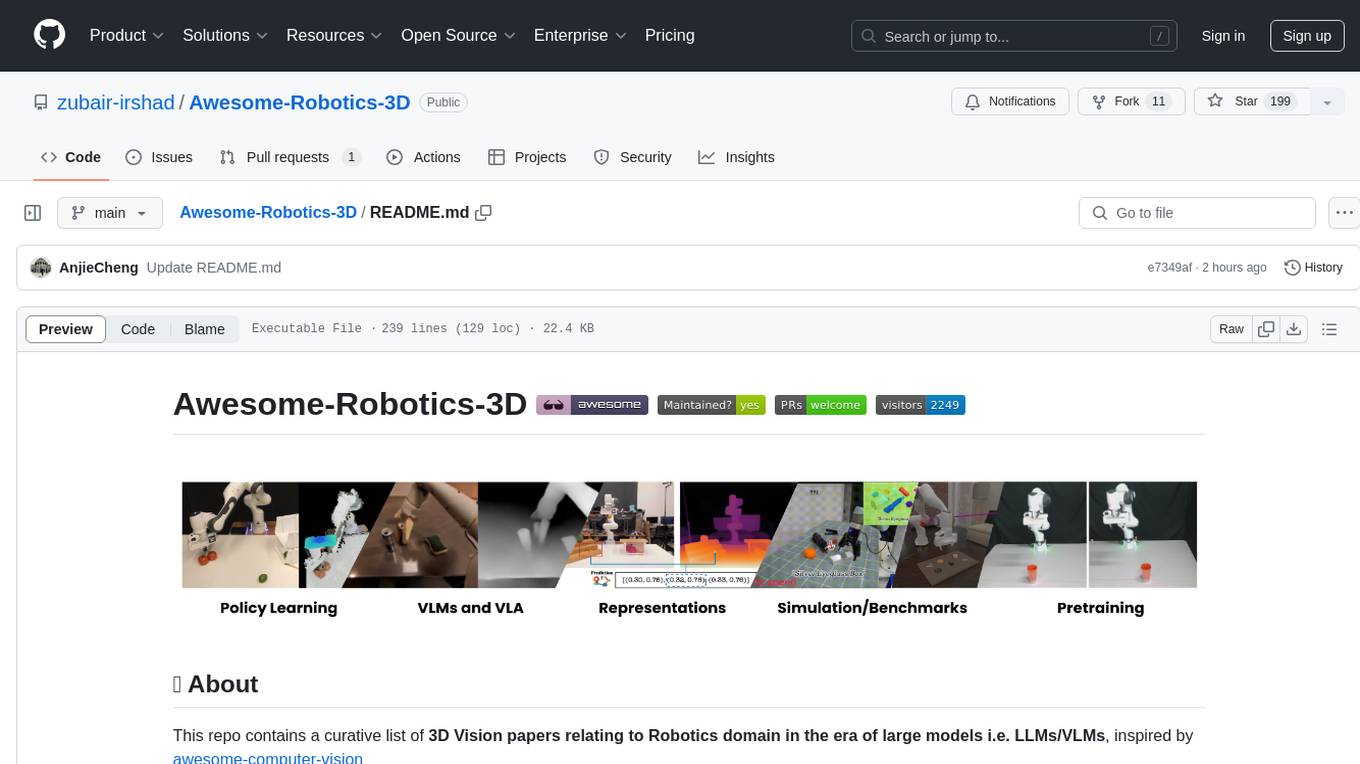
Awesome-Robotics-3D
Awesome-Robotics-3D is a curated list of 3D Vision papers related to Robotics domain, focusing on large models like LLMs/VLMs. It includes papers on Policy Learning, Pretraining, VLM and LLM, Representations, and Simulations, Datasets, and Benchmarks. The repository is maintained by Zubair Irshad and welcomes contributions and suggestions for adding papers. It serves as a valuable resource for researchers and practitioners in the field of Robotics and Computer Vision.
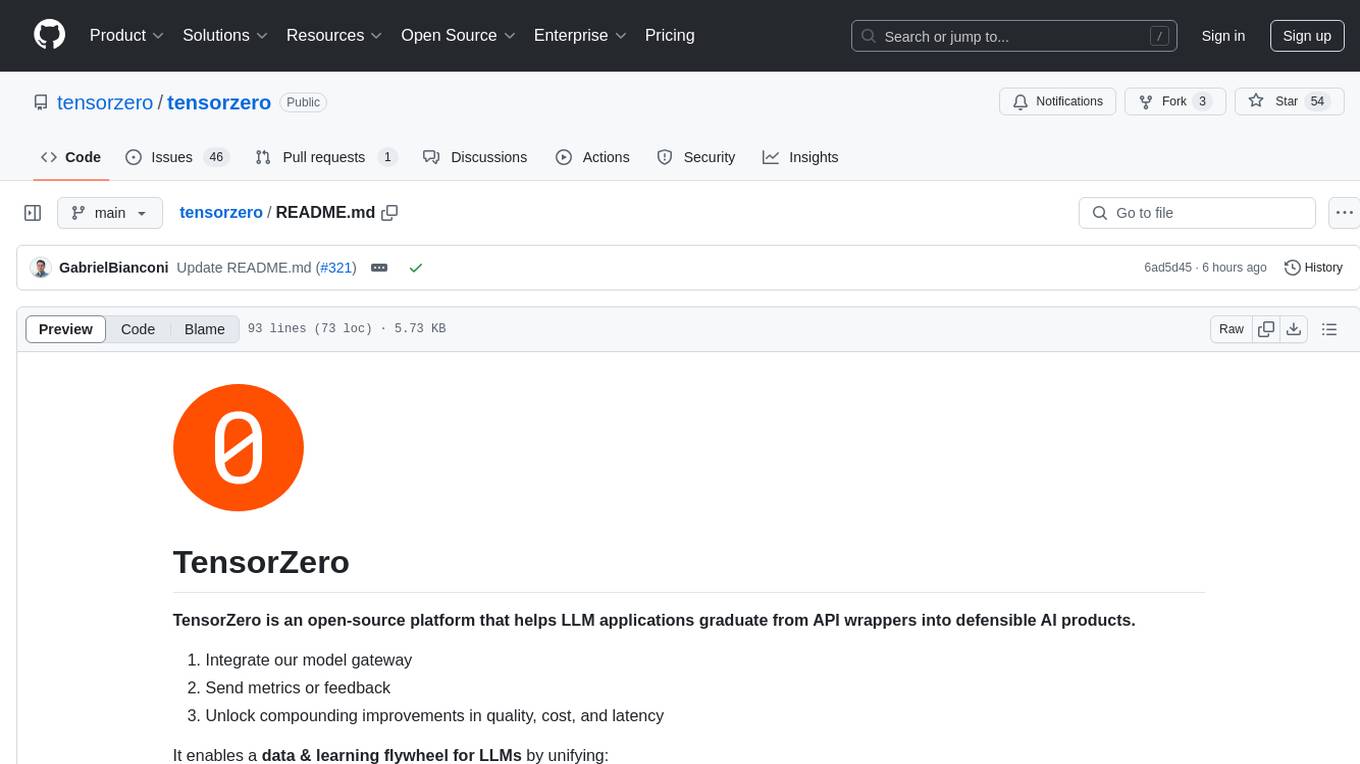
tensorzero
TensorZero is an open-source platform that helps LLM applications graduate from API wrappers into defensible AI products. It enables a data & learning flywheel for LLMs by unifying inference, observability, optimization, and experimentation. The platform includes a high-performance model gateway, structured schema-based inference, observability, experimentation, and data warehouse for analytics. TensorZero Recipes optimize prompts and models, and the platform supports experimentation features and GitOps orchestration for deployment.
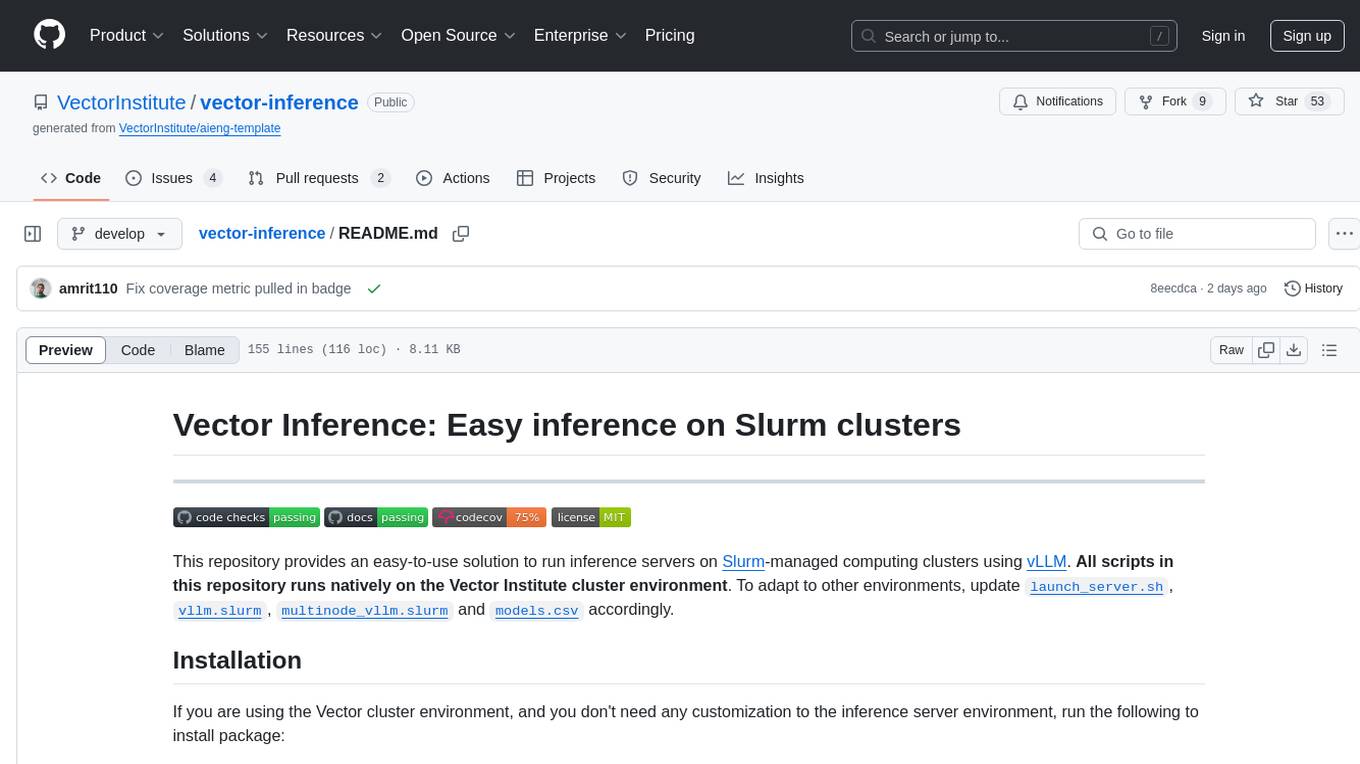
vector-inference
This repository provides an easy-to-use solution for running inference servers on Slurm-managed computing clusters using vLLM. All scripts in this repository run natively on the Vector Institute cluster environment. Users can deploy models as Slurm jobs, check server status and performance metrics, and shut down models. The repository also supports launching custom models with specific configurations. Additionally, users can send inference requests and set up an SSH tunnel to run inference from a local device.
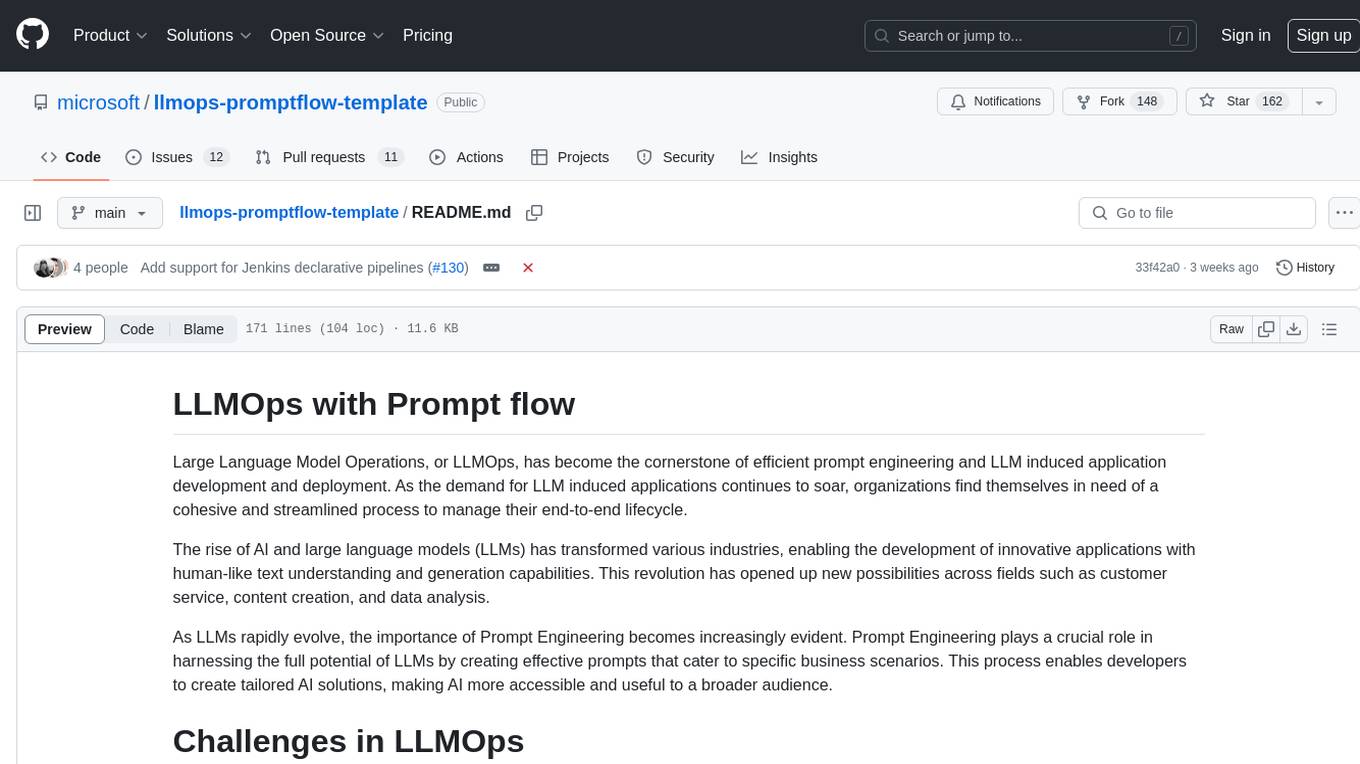
llmops-promptflow-template
LLMOps with Prompt flow is a template and guidance for building LLM-infused apps using Prompt flow. It provides centralized code hosting, lifecycle management, variant and hyperparameter experimentation, A/B deployment, many-to-many dataset/flow relationships, multiple deployment targets, comprehensive reporting, BYOF capabilities, configuration-based development, local prompt experimentation and evaluation, endpoint testing, and optional Human-in-loop validation. The tool is customizable to suit various application needs.