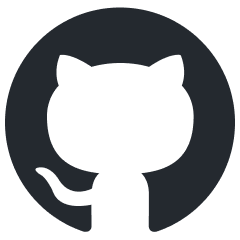
rag-experiment-accelerator
The RAG Experiment Accelerator is a versatile tool designed to expedite and facilitate the process of conducting experiments and evaluations using Azure Cognitive Search and RAG pattern.
Stars: 242
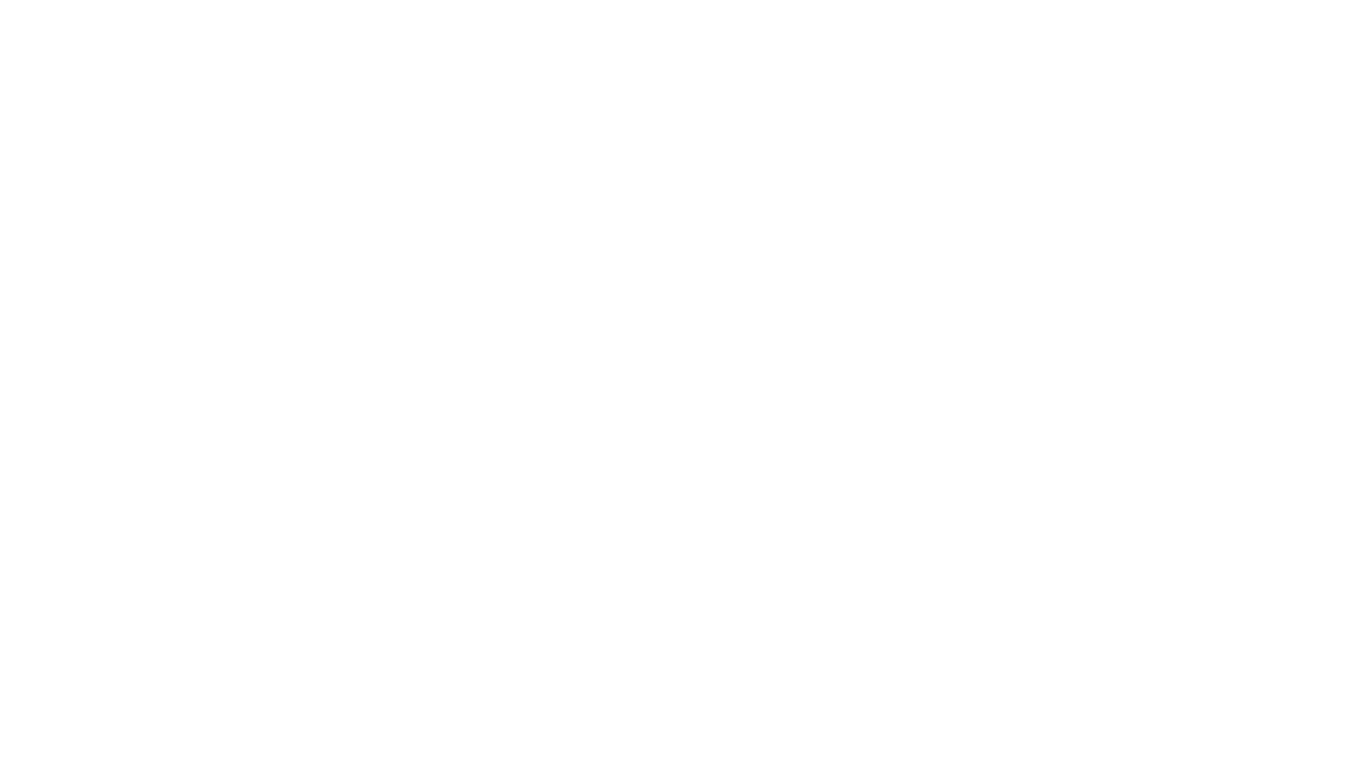
The RAG Experiment Accelerator is a versatile tool that helps you conduct experiments and evaluations using Azure AI Search and RAG pattern. It offers a rich set of features, including experiment setup, integration with Azure AI Search, Azure Machine Learning, MLFlow, and Azure OpenAI, multiple document chunking strategies, query generation, multiple search types, sub-querying, re-ranking, metrics and evaluation, report generation, and multi-lingual support. The tool is designed to make it easier and faster to run experiments and evaluations of search queries and quality of response from OpenAI, and is useful for researchers, data scientists, and developers who want to test the performance of different search and OpenAI related hyperparameters, compare the effectiveness of various search strategies, fine-tune and optimize parameters, find the best combination of hyperparameters, and generate detailed reports and visualizations from experiment results.
README:
The RAG Experiment Accelerator is a versatile tool that helps you conduct experiments and evaluations using Azure AI Search and RAG pattern. This document provides a comprehensive guide that covers everything you need to know about this tool, such as its purpose, features, installation, usage, and more.
The main goal of the RAG Experiment Accelerator is to make it easier and faster to run experiments and evaluations of search queries and quality of response from OpenAI. This tool is useful for researchers, data scientists, and developers who want to:
- Test the performance of different Search and OpenAI related hyperparameters.
- Compare the effectiveness of various search strategies.
- Fine-tune and optimize parameters.
- Find the best combination of hyperparameters.
- Generate detailed reports and visualizations from experiment results.
18 March 2024: Content sampling has been added. This functionality will allow the dataset to be sampled by a specified percentage. The data is clustered by content and then the sample percentage is taken across each cluster to attempt even distribution of the sampled data.
This is done to ensure representative results in the sample that one would get across the entire dataset.
Note: It is recommended to rebuild your environment if you have used this tool before due to new dependencies.
The RAG Experiment Accelerator is config driven and offers a rich set of features to support its purpose:
-
Experiment Setup: You can define and configure experiments by specifying a range of search engine parameters, search types, query sets, and evaluation metrics.
-
Integration: It integrates seamlessly with Azure AI Search, Azure Machine Learning, MLFlow and Azure OpenAI.
-
Rich Search Index: It creates multiple search indexes based on hyperparameter configurations available in the config file.
-
Multiple Document Loaders: The tool supports multiple document loaders, including loading via Azure Document Intelligence and basic LangChain loaders. This gives you the flexibility to experiment with different extraction methods and evaluate their effectiveness.
-
Custom Document Intelligence Loader: When selecting the 'prebuilt-layout' API model for Document Intelligence, the tool utilizes a custom Document Intelligence loader to load the data. This custom loader supports formatting of tables with column headers into key-value pairs (to enhance readability for the LLM), excludes irrelevant parts of the file for the LLM (such as page numbers and footers), removes recurring patterns in the file using regex, and more. Since each table row is transformed into a text line, to avoid breaking a row in the middle, chunking is done recursively by paragraph and line. The custom loader resorts to the simpler 'prebuilt-layout' API model as a fallback when the 'prebuilt-layout' fails. Any other API model will utilize LangChain's implementation, which returns the raw response from Document Intelligence's API.
-
Query Generation: The tool can generate a variety of diverse and customizable query sets, which can be tailored for specific experimentation needs.
-
Multiple Search Types: It supports multiple search types, including pure text, pure vector, cross-vector, multi-vector, hybrid, and more. This gives you the ability to conduct comprehensive analysis on search capabilities and results.
-
Sub-Querying: The pattern evaluates the user query and if it finds it complex enough, it breaks it down into smaller sub-queries to generate relevant context.
-
Re-Ranking: The query responses from Azure AI Search are re-evaluated using LLM and ranked according to the relevance between the query and the context.
-
Metrics and Evaluation: It supports end-to-end metrics comparing the generated answers (actual) against the ground-truth answers (expected), including distance-based, cosine and semantic similarity metrics. It also includes component-based metrics to assess retrieval and generation performance using LLMs as judges, such as context recall or answer relevance, as well as retrieval metrics to assess search results (e.g. MAP@k).
-
Report Generation: The RAG Experiment Accelerator automates the process of report generation, complete with visualizations that make it easy to analyze and share experiment findings.
-
Multi-Lingual: The tool supports language analyzers for linguistic support on individual languages and specialized (language-agnostic) analyzers for user-defined patterns on search indexes. For more information, see Types of Analyzers.
-
Sampling: If you have a large dataset and/or want to speed up the experimentation, a sampling process is available to create a small but representative sample of the data for the percentage specified. The data will be clustered by content and a percentage of each cluster will be selected as part of the sample. Results obtained should be roughly indicative of the full dataset within a ~10% margin. Once an approach has been identified, running on the full dataset is recommended for accurate results.
- Azure AI Search Service (Note: Semantic Search is available in Azure AI Search, at Basic tier or higher.)
- Azure OpenAI Service or access to the OpenAI API
- Azure Machine Learning Resources
At the moment, the RAG Experiment Accelerator can be run locally leveraging one of the following:
Using a development container will mean that all of the required software is installed for you. This will require WSL. For more information about development containers visit containers.dev
Install the following software on the host machine you will perform the deployment from:
- For Windows - Windows Store Ubuntu 22.04.3 LTS
- Docker Desktop
- Visual Studio Code
- VS Code Extension: Remote-Containers
Further guidance of setting up WSL can be found here. Now you have the prerequisites, you can:
- Clone the repository: Clone the accelerator's repository in your WSL terminal.
git clone https://github.com/microsoft/rag-experiment-accelerator.git
code .
Once the project opens in vscode it should ask you if you would like to "Reopen this in a development container". Say yes.
You can of course run the RAG Experiment Accelerator on a Windows/Mac machine if you like; you are responsible for installing the correct tooling. Follow these installation steps:
- Clone the repository: Clone the accelerator's repository from [GitHub].
git clone https://github.com/microsoft/rag-experiment-accelerator.git
- Execute the requirements.txt in a conda (first install Anaconda/Miniconda) or virtual environment (then install a couple of dependencies - prompted on the run) to install the dependencies.
conda create -n rag-experiment python=3.11
conda init bash
Close your terminal, open a new one, and run:
conda activate rag-experiment
pip install .
- Install Azure CLI and authorize:
az login
az account set --subscription="<your_subscription_guid>"
az account show
There are 3 options to install all the required azure services:
This project supports Azure Developer CLI.
azd provision
- You can also use
azd up
if you prefer as this callsazd provision
anyway - Please use the up/down arrows to select your Subscription and Region
Once this has completed you can use the launch configuration to run, or debug the 4 steps and the current environment provisioned by azd
will be loaded with the correct values.
If you want to deploy the infrastructure yourself from template you can also click here:
If you don't want to use azd
you can use the normal az
cli too.
Use following command to deploy.
az login
az deployment sub create --subscription <subscription-id> --location <location> --template-file infra/main.bicep
Or
To deploy with isolated network use following command. Replace the parameter values with the specifics of your isolated network. You must supply all three parameters (i.e. vnetAddressSpace
, proxySubnetAddressSpace
and subnetAddressSpace
) if you wish to deploy to an isolated network.
az login
az deployment sub create --location <location> --template-file infra/main.bicep \
--parameters vnetAddressSpace=<vnet-address-space> \
--parameters proxySubnetAddressSpace=<proxy-subnet-address-space> \
--parameters subnetAddressSpace=<azure-subnet-address-space>
here is an example with parameter values:
az deployment sub create --location uksouth --template-file infra/main.bicep \
--parameters vnetAddressSpace='10.0.0.0/16' \
--parameters proxySubnetAddressSpace='10.0.1.0/24' \
--parameters subnetAddressSpace='10.0.2.0/24'
To use the RAG Experiment Accelerator locally, follow these steps:
-
Copy the provided
.env.template
file to a file named.env
and update all of the required values. Many of the required values for the.env
file will come from resources which have previously been configured and/or can be gathered from resources provisioned in the Provision Infrastructure section. Also note, by default,LOGGING_LEVEL
is set toINFO
but can be changed to any of the following levels:NOTSET
,DEBUG
,INFO
,WARN
,ERROR
,CRITICAL
.cp .env.template .env # change parameters manually
-
Copy the provided
config.sample.json
file to a file namedconfig.json
and change any hyperparameters to tailor to your experiment.cp config.sample.json config.json # change parameters manually
-
Copy any files for ingestion (PDF, HTML, Markdown, Text, JSON or DOCX format) into the
data
folder. -
Run
01_index.py
(python 01_index.py) to create Azure AI Search indexes and load data into them.python 01_index.py -d "The directory holding the configuration files and data. Defaults to current working directory" -dd "The directory holding the data. Defaults to data" -cf "JSON config filename. Defaults to config.json"
-
Run
02_qa_generation.py
(python 02_qa_generation.py) to generate question-answer pairs using Azure OpenAI.python 02_qa_generation.py -d "The directory holding the configuration files and data. Defaults to current working directory" -dd "The directory holding the data. Defaults to data" -cf "JSON config filename. Defaults to config.json"
-
Run
03_querying.py
(python 03_querying.py) to query Azure AI Search to generate context, re-rank items in context, and get response from Azure OpenAI using the new context.python 03_querying.py -d "The directory holding the configuration files and data. Defaults to current working directory" -cf "JSON config filename. Defaults to config.json"
-
Run
04_evaluation.py
(python 04_evaluation.py) to calculate metrics using various methods and generate charts and reports in Azure Machine Learning using MLFlow integration.python 04_evaluation.py -d "The directory holding the configuration files and data. Defaults to current working directory" -cf "JSON config filename. Defaults to config.json"
Alternatively, you can run the above steps (apart from 02_qa_generation.py
) using an Azure ML pipeline. To do so, follow the guide here.
Sampling will be run locally to create a small but representative slice of the data. This helps with rapid experimentation and keeps costs down. Results obtained should be roughly indicative of the full dataset within a ~10% margin. Once an approach has been identified, running on the full dataset is recommended for accurate results.
Note: Sampling can only be run locally, at this stage it is not supported on a distributed AML compute cluster. So the process would be to run sampling locally and then use the generated sample dataset to run on AML.
If you have a very large dataset and want to run a similar approach to sample the data, you can use the pyspark in-memory distributed implementation in the Data Discovery Toolkit for Microsoft Fabric or Azure Synapse Analytics.
"sampling": {
"sample_data": "Set to true to enable sampling",
"only_run_sampling": "If set to true, this will only run the sampling step and will not create an index or any subsequent steps, use this if you want to build a small sampled dataset to run in AML",
"sample_percentage": "Percentage of the document corpus to sample",
"optimum_k": "Set to 'auto' to automatically determine the optimum cluster number or set to a specific value e.g. 15",
"min_cluster": "Used by the automated optimum cluster process, this is the minimum number of clusters e.g. 2",
"max_cluster": "Used by the automated optimum cluster process, this is the maximum number of clusters e.g. 30",
},
The sampling process will produce the following artifacts in the sampling directory:
- A directory named after the config value
job_name
containing the subset of files sampled, these can be specified as--data_dir
argument when running the entire process on AML. - A 2 dimensional scatter plot of the clustered files (by content) selected as the sampled dataset in the sampling folder.
- A .cvs file of the entire dataset with cluster predictions named "all_cluster_predictions..." and a cvs file with the sampled cluster predictions named "sampled_cluster_predictions...". This can be used for further enriching the dataset, for example, creating a meaningful label per cluster and updates all record. See the Heuristics classifier in the Data Discovery Toolkit as an example or Pixplotml for image data.
- If the
"optimum_k": auto
config value is set to auto, the sampling process will attempt to set the optimum number of clusters automatically. This can be overridden if you know roughly how many broad buckets of content exist in your data. An elbow graph will be generated in the sampling folder.
Two options exist for running sampling, namely:
- Run the entire process locally with sampling, including the index generation and subsequent steps
- Run only the sampling locally and then use the created sampled dataset to execute on AML
Set the following values to run the indexing process locally:
"sampling": {
"sample_data": true,
"only_run_sampling": false,
"sample_percentage": 10,
"optimum_k": auto,
"min_cluster": 2,
"max_cluster": 30
},
If only_run_sampling
config value is set to true, this will only run the sampling step, no index will be created and any other subsequent steps will not executed. Set the --data_dir
argument to directory created by the sampling process which will be:
artifacts/sampling/config.[job_name]
and execute the AML pipeline step.
All the values can be lists of elements. Including the nested configurations.
Every array will produce the combinations of flat configurations when the method flatten()
is called on a particular node, to select 1 random combination - call the method sample()
.
{
"experiment_name": "If provided, this will be the experiment name in Azure ML and it will group all job run under the same experiment, otherwise (if left empty) index_name_prefix will be used and there may be more than one experiment",
"job_name": "If provided, all jobs runs in Azure ML will be named with this property value plus timestamp, otherwise (if left empty) each job with be named only with timestamp",
"job_description": "You may provide a description for the current job run which describes in words what you are about to experiment with",
"data_formats": "Specifies the supported data formats for the application. You can choose from a variety of formats such as JSON, CSV, PDF, and more. [*] - means all formats included",
"main_instruction": "Defines the main instruction prompt coming with queries to LLM",
"use_checkpoints": "A boolean. If true, enables use of checkpoints to load data and skip processing that was already done in previous executions.",
"index": {
"index_name_prefix": "Search index name prefix",
"ef_construction": "ef_construction value determines the value of Azure AI Search vector configuration.",
"ef_search": "ef_search value determines the value of Azure AI Search vector configuration.",
"chunking": {
"preprocess": "A boolean. If true, preprocess documents, split into smaller chunks, embed and enrich them, and finally upload documents chunks for retrieval into Azure Search Index.",
"chunk_size": "Size of each chunk e.g. [500, 1000, 2000]",
"overlap_size": "Overlap Size for each chunk e.g. [100, 200, 300]",
"generate_title": "A boolean. If true, a title is generated for the chunk of content and an embedding is created for it",
"generate_summary": "A boolean. If true, a summary is generated for the chunk of content and an embedding is created for it",
"override_content_with_summary": "A boolean. If true, The chunk content is replaced with its summary",
"chunking_strategy": "determines the chunking strategy. Valid values are 'azure-document-intelligence' or 'basic'",
"azure_document_intelligence_model": "represents the Azure Document Intelligence Model. Used when chunking strategy is 'azure-document-intelligence'. When set to 'prebuilt-layout', provides additional features (see above)"
},
"embedding_model": "see 'Description of embedding models config' below",
"sampling": {
"sample_data": "Set to true to enable sampling",
"percentage": "Percentage of the document corpus to sample",
"optimum_k": "Set to 'auto' to automatically determine the optimum cluster number or set to a specific value e.g. 15",
"min_cluster": "Used by the automated optimum cluster process, this is the minimum number of clusters e.g. 2",
"max_cluster": "Used by the automated optimum cluster process, this is the maximum number of clusters e.g. 30"
}
},
"language": {
"analyzer": {
"analyzer_name": "name of the analyzer to use for the field. This option can be used only with searchable fields and it can't be set together with either searchAnalyzer or indexAnalyzer.",
"index_analyzer_name": "name of the analyzer used at indexing time for the field. This option can be used only with searchable fields. It must be set together with searchAnalyzer and it cannot be set together with the analyzer option.",
"search_analyzer_name": "name of the analyzer used at search time for the field. This option can be used only with searchable fields. It must be set together with indexAnalyzer and it cannot be set together with the analyzer option. This property cannot be set to the name of a language analyzer; use the analyzer property instead if you need a language analyzer.",
"char_filters": "The character filters for the index",
"tokenizers": "The tokenizers for the index",
"token_filters": "The token filters for the index"
},
"query_language": "The language of the query. Possible values: en-us, en-gb, fr-fr etc."
},
"rerank": {
"enabled": "determines if search results should be re-ranked. Value values are TRUE or FALSE",
"type": "determines the type of re-ranking. Value values are llm or cross_encoder",
"llm_rerank_threshold": "determines the threshold when using llm re-ranking. Chunks with rank above this number are selected in range from 1 - 10.",
"cross_encoder_at_k": "determines the threshold when using cross-encoding re-ranking. Chunks with given rank value are selected.",
"cross_encoder_model": "determines the model used for cross-encoding re-ranking step. Valid value is cross-encoder/stsb-roberta-base"
},
"search": {
"retrieve_num_of_documents": "determines the number of chunks to retrieve from the search index",
"search_type": "determines the search types used for experimentation. Valid value are search_for_match_semantic, search_for_match_Hybrid_multi, search_for_match_Hybrid_cross, search_for_match_text, search_for_match_pure_vector, search_for_match_pure_vector_multi, search_for_match_pure_vector_cross, search_for_manual_hybrid. e.g. ['search_for_manual_hybrid', 'search_for_match_Hybrid_multi','search_for_match_semantic']",
"search_relevancy_threshold": "the similarity threshold to determine if a doc is relevant. Valid ranges are from 0.0 to 1.0"
},
"query_expansion": {
"expand_to_multiple_questions": "whether the system should expand a single question into multiple related questions. By enabling this feature, you can generate a set of alternative related questions that may improve the retrieval process and provide more accurate results".,
"query_expansion": "determines if query expansion feature is on. Value values are TRUE or FALSE",
"hyde": "this feature allows you to experiment with various query expansion approaches which may improve the retrieval metrics. The possible values are 'disabled' (default), 'generated_hypothetical_answer', 'generated_hypothetical_document_to_answer' reference article - Precise Zero-Shot Dense Retrieval without Relevance Labels (HyDE - Hypothetical Document Embeddings) - https://arxiv.org/abs/2212.10496",
"min_query_expansion_related_question_similarity_score": "minimum similarity score in percentage between LLM generated related queries to the original query using cosine similarly score. default 90%"
},
"openai": {
"azure_oai_chat_deployment_name": "determines the Azure OpenAI deployment name",
"azure_oai_eval_deployment_name": "determines the Azure OpenAI deployment name used for evaluation",
"temperature": "determines the OpenAI temperature. Valid value ranges from 0 to 1."
},
"eval": {
"metric_types": "determines the metrics used for evaluation (end-to-end or component-wise metrics using LLMs). Valid values for end-to-end metrics are lcsstr, lcsseq, cosine, jaro_winkler, hamming, jaccard, levenshtein, fuzzy_score, cosine_ochiai, bert_all_MiniLM_L6_v2, bert_base_nli_mean_tokens, bert_large_nli_mean_tokens, bert_large_nli_stsb_mean_tokens, bert_distilbert_base_nli_stsb_mean_tokens, bert_paraphrase_multilingual_MiniLM_L12_v2. Valid values for component-wise LLM-based metrics are llm_answer_relevance, llm_context_precision and llm_context_recall. e.g ['fuzzy_score','bert_all_MiniLM_L6_v2','cosine_ochiai','bert_distilbert_base_nli_stsb_mean_tokens', 'llm_answer_relevance']",
}
}
NOTE: When changing the config, remember to change:
-
config.sample.json
(the example config to be copied by others) - Github actions config file to be used by tests on CI
- Unit tests config file
embedding_model
is an array containing the configuration for the embedding models to use. Embedding model type
must be azure
for Azure OpenAI models and sentence-transformer
for HuggingFace sentence transformer models.
{
"type": "azure",
"model_name": "the name of the Azure OpenAI model",
"dimension": "the dimension of the embedding model. For example, 1536 which is the dimension of text-embedding-ada-002"
}
If you are using a model other than text-embedding-ada-002
, you must specify the corresponding dimension for the model in the dimension
field; for example:
{
"type": "azure",
"model_name": "text-embedding-3-large",
"dimension": 3072
}
The dimensions for the different Azure OpenAI embeddings models can be found in the Azure OpenAI Service models documentation.
When using the newer embeddings models (v3), you can also leverage their support for shortening embeddings. In this case, specify the number of dimensions you require, and add the shorten_dimensions
flag to indicate that you want to shorten the embeddings. For example:
{
"type": "azure",
"model_name": "text-embedding-3-large",
"dimension": 256,
"shorten_dimensions": true
}
{
"type": "sentence-transformer",
"model_name": "the name of the sentence transformer model",
"dimension": "the dimension of the model. This field is not required if model name is one of ['all-MiniLM-L6-v2', 'all-mpnet-base-v2', 'bert-large-nli-mean-tokens]"
}
Giving an example of an hypothetical answer for the question in query, an hypothetical passage which holds an answer to the query, or generate few alternative related question might improve retrieval and thus get more accurate chunks of docs to pass into LLM context. Based on the reference article - Precise Zero-Shot Dense Retrieval without Relevance Labels (HyDE - Hypothetical Document Embeddings).
The following configuration options turns on this experimentation approaches:
{
"hyde": "generated_hypothetical_answer"
}
{
"hyde": "generated_hypothetical_document_to_answer"
}
This feature will generate fine related questions, filter out those which are less than min_query_expansion_related_question_similarity_score
percent from the original query (using cosine similarity score), and search documents for each one of them along with the original query,
deduplicate results and return them to the reranker and top k steps.
default value for min_query_expansion_related_question_similarity_score
is set to 90%, you may change this in the config.json
{
"query_expansion": true,
"min_query_expansion_related_question_similarity_score": 90
}
The solution integrates with Azure Machine Learning and uses MLFlow to manage experiments, jobs, and artifacts. You can view the following reports as part of the evaluation process:
all_metrics_current_run.html
shows average scores across questions and search types for each selected metric:
The computation of each metric and fields used for evaluation are tracked for each question and search type in the output csv file:
Metrics can be compared across runs:
Metrics can be compared across different search strategies:
Mean average precision scores are tracked and average MAP scores can be compared across search type:
This section outlines common gotchas or pitfalls that engineers/developers/data scientists may encounter while working with RAG Experiment Accelerator.
To successfully utilize this solution, you must first authenticate yourself by logging in to your Azure account. This essential step ensures you have the required permissions to access and manage Azure resources used by it. You might errors related to storing QnA data into Azure Machine Learning Data Assets, executing the query and evaluation step as a result of inappropriate authorization and authentication to Azure. Refer to Point 4 in this document for authentication and authorization.
There might be situations in which the solution would still generate errors despite of valid authentication and authorization. In such cases, start a new session with a brand new terminal instance, login to Azure using steps mentioned in step 4 and also check if the user has contribute access to the Azure resources related to the solution.
This solution utilizes several configuration parameters in config.json
that directly impact its functionality and performance. Please pay close attention to these settings:
retrieve_num_of_documents: This config controls the initial number of documents retrieved for analysis. Excessively high or low values can lead to "index out of range" errors due to rank processing of Search AI results.
cross_encoder_at_k: This config influences the ranking process. A high value might result in irrelevant documents being included in the final results.
llm_rerank_threshold: This config determines which documents are passed to the language model (LLM) for further processing. Setting this value too high could create an overly large context for the LLM to handle, potentially leading to processing errors or degraded results. This might also result in exception from Azure OpenAI endpoint.
Before running this solution, please ensure you've correctly set up both your Azure OpenAI deployment name within config.json file and add relevant secrets to environment variables (.env file). This information is crucial for the application to connect to the appropriate Azure OpenAI resources and function as designed. If you're unsure about the configuration data, please refer to .env.template and config.json file. The solution has been tested with GPT 3.5 turbo model and needs further tests for any other model.
During the QnA generation step, you may occasionally encounter errors related to the JSON output received from Azure OpenAI. These errors can prevent the successful generation of few questions and answers. Here's what you need to know:
Incorrect Formatting: The JSON output from Azure OpenAI may not adhere to the expected format, causing issues with the QnA generation process. Content Filtering: Azure OpenAI has content filters in place. If the input text or generated responses are deemed inappropriate, it could lead to errors. API Limitations: The Azure OpenAI service have token and rate limitations that affect the output.
End-to-end evaluation metrics: not all the metrics comparing the generated and ground-truth answers are able to capture differences in semantics. For example, metrics such as levenshtein
or jaro_winkler
only measure edit distances. The cosine
metric doesn't allow the comparison of semantics either: it uses the textdistance token-based implementation based on term frequency vectors. To calculate the semantic similarity between the generated answers and the expected responses, consider using embedding-based metrics such as Bert scores (bert_
).
Component-wise evaluation metrics: evaluation metrics using LLM-as-judges aren't deterministic. The llm_
metrics included in the accelerator use the model indicated in the azure_oai_eval_deployment_name
config field. The prompts used for evaluation instruction can be adjusted and are included in the prompts.py
file (llm_answer_relevance_instruction
, llm_context_recall_instruction
, llm_context_precision_instruction
).
Retrieval-based metrics: MAP scores are computed by comparing each retrieved chunk against the question and the chunk used to generate the qna pair. To assess whether a retrieved chunk is relevant or not, the similarity between the retrieved chunk and the concatenation of the end user question and the chunk used in the qna step (02_qa_generation.py
) is computed using the SpacyEvaluator. Spacy similarity defaults to the average of the token vectors, meaning that the computation is insensitive to the order of the words. By default, the similarity threshold is set to 80% (spacy_evaluator.py
).
We welcome your contributions and suggestions. To contribute, you need to agree to a Contributor License Agreement (CLA) that confirms you have the right to, and actually do, grant us the rights to use your contribution. For details, visit [https://cla.opensource.microsoft.com].
When you submit a pull request, a CLA bot will automatically check whether you need to provide a CLA and give you instructions (for example, status check, comment). Follow the instructions from the bot. You only need to do this once for all repos that use our CLA.
Before you contribute, make sure to run
pip install -e .
pre-commit install
This project follows the Microsoft Open Source Code of Conduct. For more information, see the Code of Conduct FAQ or contact [email protected] with any questions or comments.
-
Branch Naming Convention:
- Use the GitHub UI to include a tag in the branch name, then create the branch directly from the UI. Here are some examples:
bug/11-short-description
feature/22-short-description
- Use the GitHub UI to include a tag in the branch name, then create the branch directly from the UI. Here are some examples:
-
Merging Changes:
- When merging, squash your commits to have up to 3 incremental commits for Pull Requests (PRs) and merges.
- Project maintainers can merge accepted code changes from contributors or contributors can request Write Permissions to the repo to merge a pull request once it has been reviewed by project maintainers.
- Project Maintainers can be contacted via email.
-
Branch Hygiene:
- Delete the branch after it has been merged.
-
Testing Changes Locally:
- Before merging, test your changes locally.
-
Naming Conventions:
- Use snake case for metric names and configuration variables, like
example_snake_case
. - Set up your Git username to be your first and last name, like this:
git config --global user.name "First Last"
- Use snake case for metric names and configuration variables, like
-
Issue Tracking:
- Working on a contribution to the RAG Experiment Accelerator? Before opening a new issue, make sure to check if the feature has already been requested by searching for it in the associated project issue tracker, and consider adding to that discussion instead. Otherwise, please open an issue for it using the feature request template or create a PR and make sure it is associated to the project.
This project might contain trademarks or logos for projects, products, or services. You must follow Microsoft's Trademark & Brand Guidelines to use Microsoft trademarks or logos correctly. Don't use Microsoft trademarks or logos in modified versions of this project in a way that causes confusion or implies Microsoft sponsorship. Follow the policies of any third-party trademarks or logos that this project contains.
For Tasks:
Click tags to check more tools for each tasksFor Jobs:
Alternative AI tools for rag-experiment-accelerator
Similar Open Source Tools
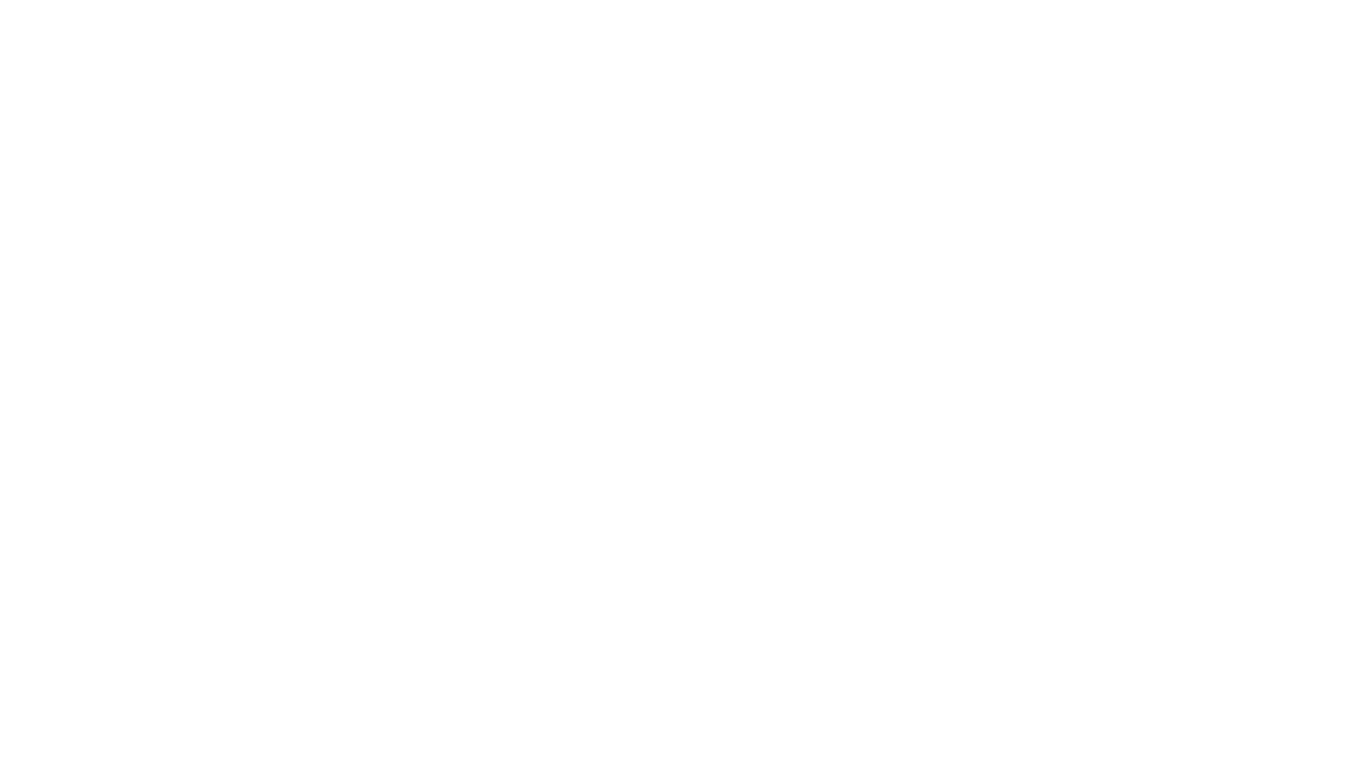
rag-experiment-accelerator
The RAG Experiment Accelerator is a versatile tool that helps you conduct experiments and evaluations using Azure AI Search and RAG pattern. It offers a rich set of features, including experiment setup, integration with Azure AI Search, Azure Machine Learning, MLFlow, and Azure OpenAI, multiple document chunking strategies, query generation, multiple search types, sub-querying, re-ranking, metrics and evaluation, report generation, and multi-lingual support. The tool is designed to make it easier and faster to run experiments and evaluations of search queries and quality of response from OpenAI, and is useful for researchers, data scientists, and developers who want to test the performance of different search and OpenAI related hyperparameters, compare the effectiveness of various search strategies, fine-tune and optimize parameters, find the best combination of hyperparameters, and generate detailed reports and visualizations from experiment results.
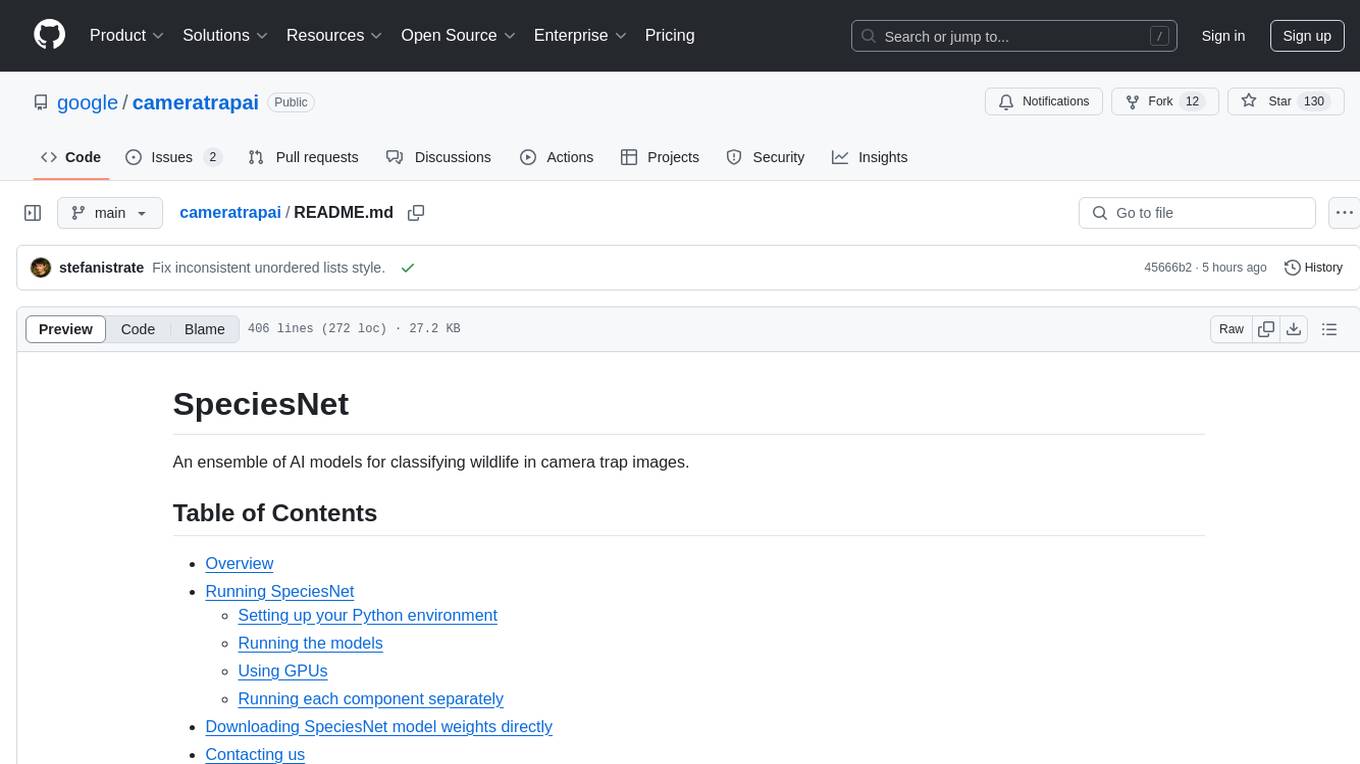
cameratrapai
SpeciesNet is an ensemble of AI models designed for classifying wildlife in camera trap images. It consists of an object detector that finds objects of interest in wildlife camera images and an image classifier that classifies those objects to the species level. The ensemble combines these two models using heuristics and geographic information to assign each image to a single category. The models have been trained on a large dataset of camera trap images and are used for species recognition in the Wildlife Insights platform.
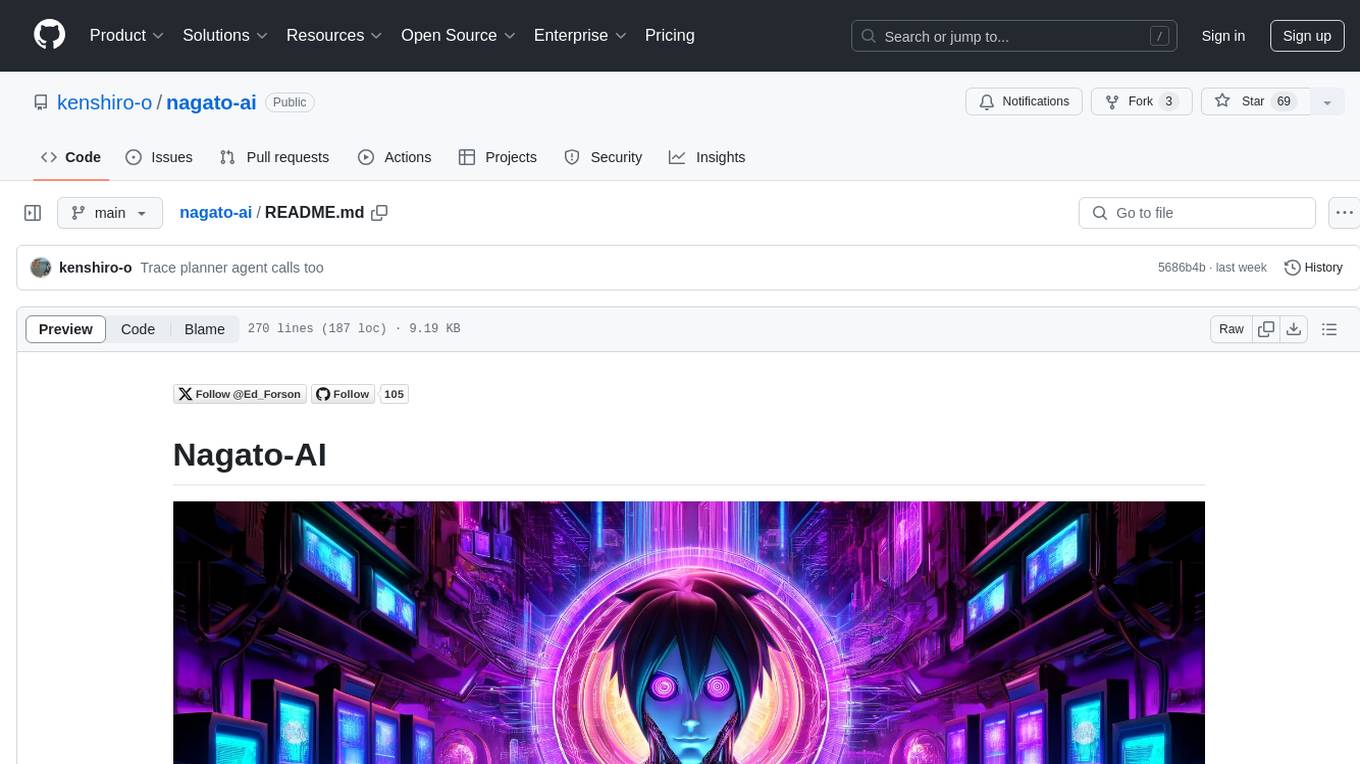
nagato-ai
Nagato-AI is an intuitive AI Agent library that supports multiple LLMs including OpenAI's GPT, Anthropic's Claude, Google's Gemini, and Groq LLMs. Users can create agents from these models and combine them to build an effective AI Agent system. The library is named after the powerful ninja Nagato from the anime Naruto, who can control multiple bodies with different abilities. Nagato-AI acts as a linchpin to summon and coordinate AI Agents for specific missions. It provides flexibility in programming and supports tools like Coordinator, Researcher, Critic agents, and HumanConfirmInputTool.
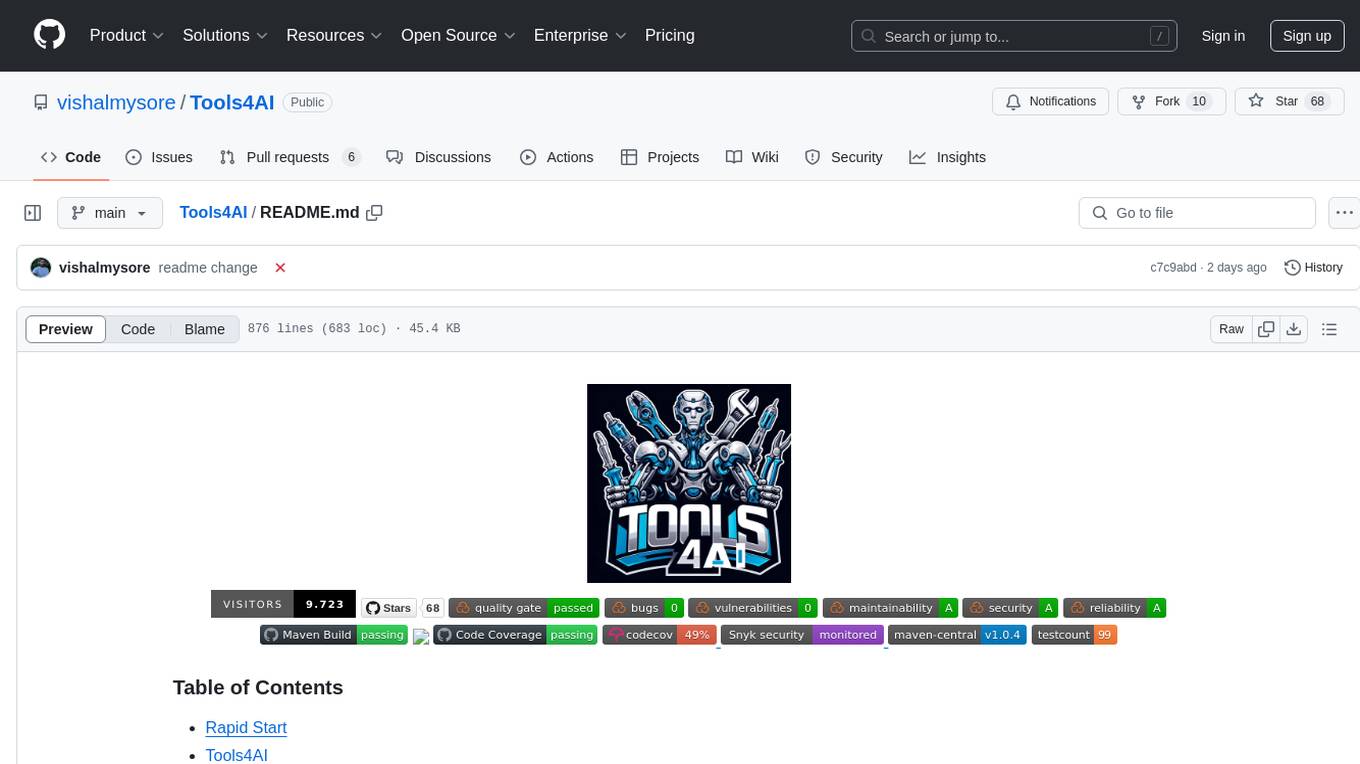
Tools4AI
Tools4AI is a Java-based Agentic Framework for building AI agents to integrate with enterprise Java applications. It enables the conversion of natural language prompts into actionable behaviors, streamlining user interactions with complex systems. By leveraging AI capabilities, it enhances productivity and innovation across diverse applications. The framework allows for seamless integration of AI with various systems, such as customer service applications, to interpret user requests, trigger actions, and streamline workflows. Prompt prediction anticipates user actions based on input prompts, enhancing user experience by proactively suggesting relevant actions or services based on context.
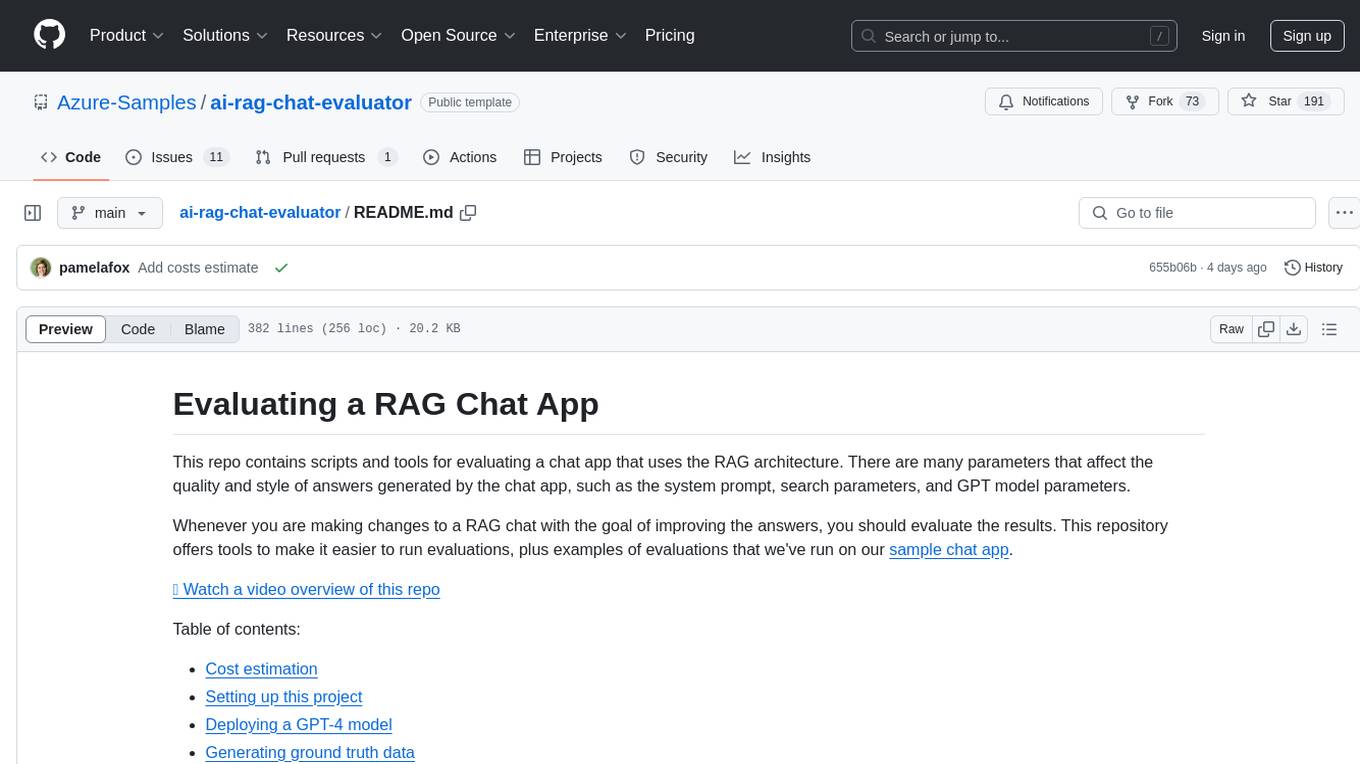
ai-rag-chat-evaluator
This repository contains scripts and tools for evaluating a chat app that uses the RAG architecture. It provides parameters to assess the quality and style of answers generated by the chat app, including system prompt, search parameters, and GPT model parameters. The tools facilitate running evaluations, with examples of evaluations on a sample chat app. The repo also offers guidance on cost estimation, setting up the project, deploying a GPT-4 model, generating ground truth data, running evaluations, and measuring the app's ability to say 'I don't know'. Users can customize evaluations, view results, and compare runs using provided tools.
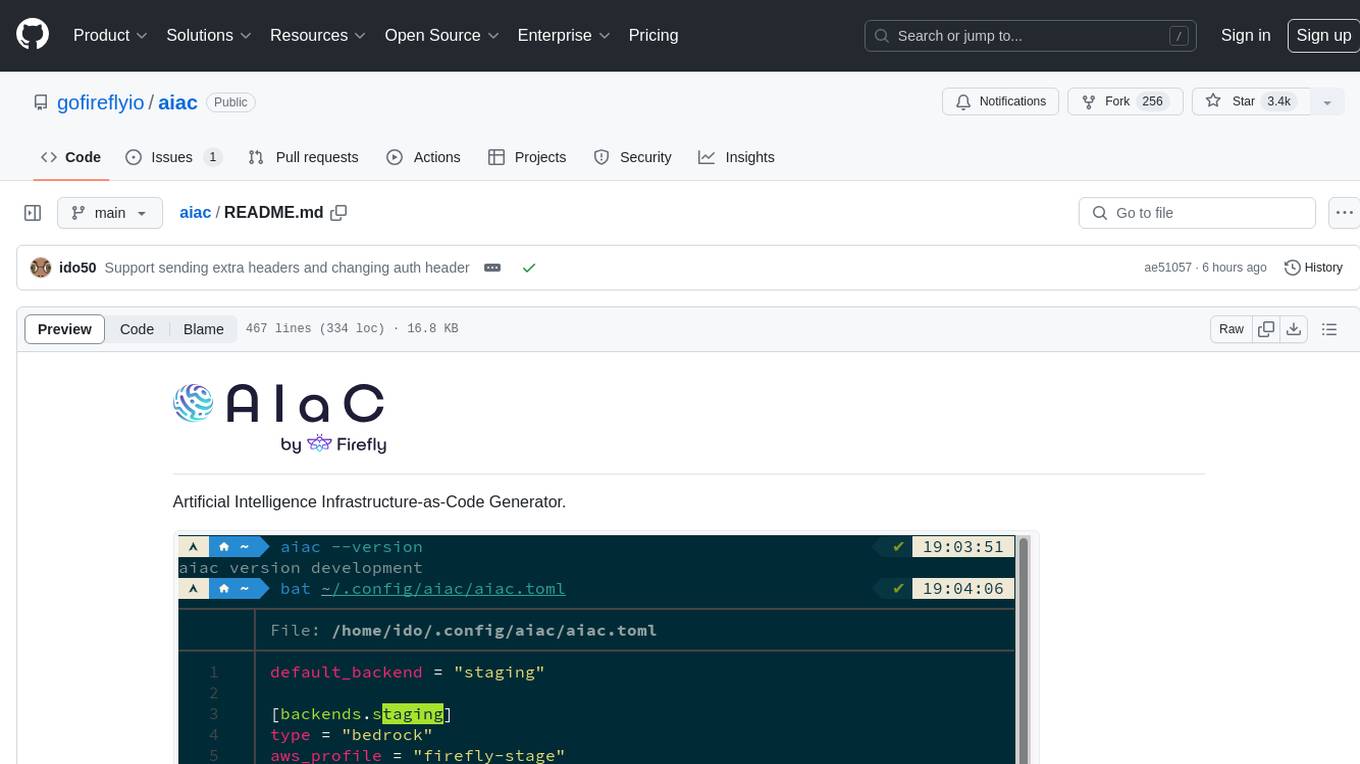
aiac
AIAC is a library and command line tool to generate Infrastructure as Code (IaC) templates, configurations, utilities, queries, and more via LLM providers such as OpenAI, Amazon Bedrock, and Ollama. Users can define multiple 'backends' targeting different LLM providers and environments using a simple configuration file. The tool allows users to ask a model to generate templates for different scenarios and composes an appropriate request to the selected provider, storing the resulting code to a file and/or printing it to standard output.
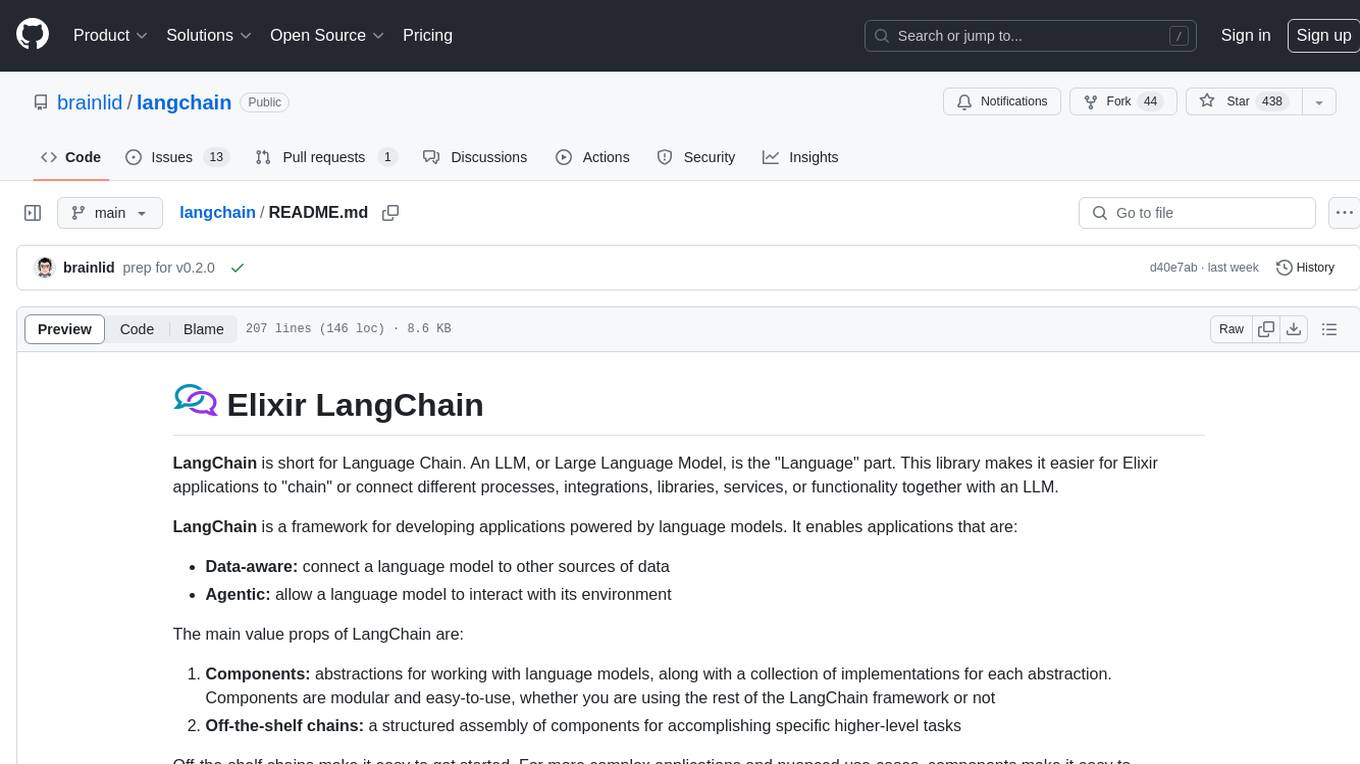
langchain
LangChain is a framework for developing Elixir applications powered by language models. It enables applications to connect language models to other data sources and interact with the environment. The library provides components for working with language models and off-the-shelf chains for specific tasks. It aims to assist in building applications that combine large language models with other sources of computation or knowledge. LangChain is written in Elixir and is not aimed for parity with the JavaScript and Python versions due to differences in programming paradigms and design choices. The library is designed to make it easy to integrate language models into applications and expose features, data, and functionality to the models.
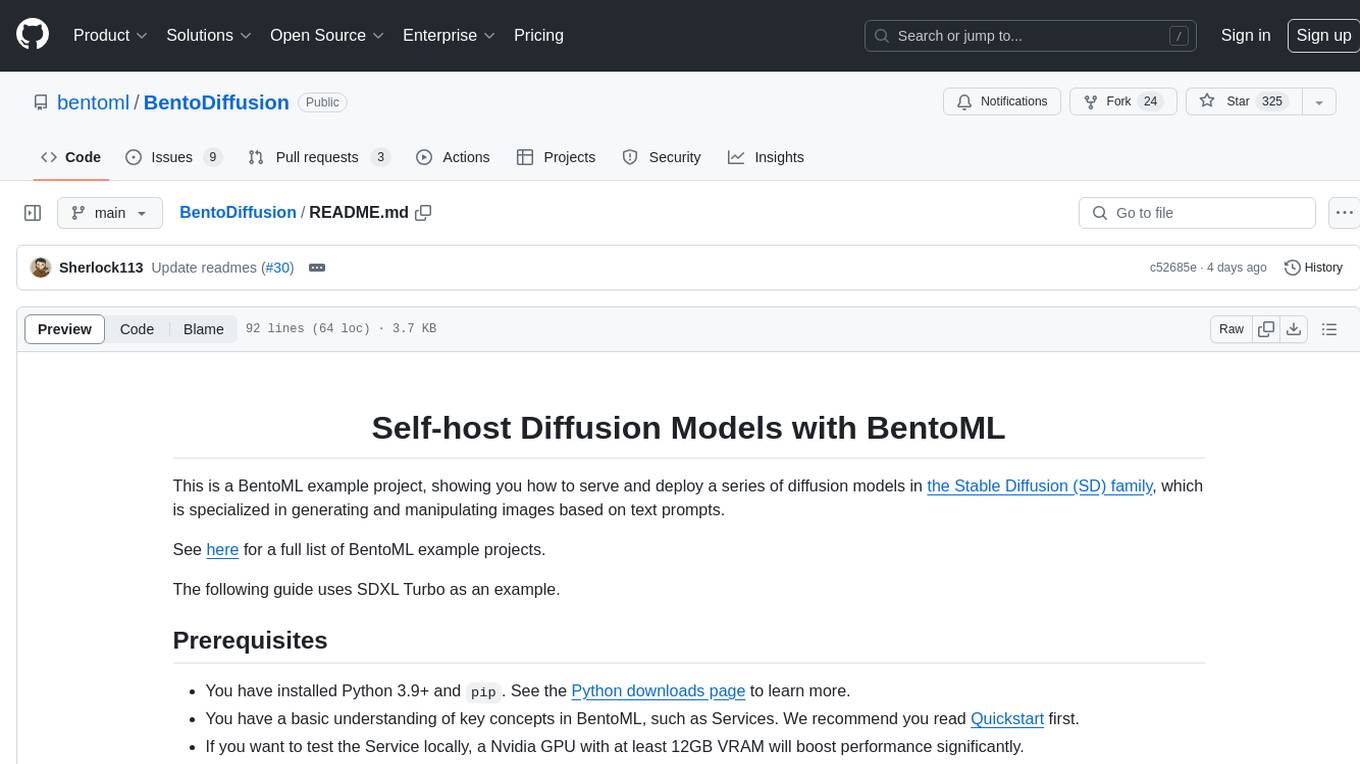
BentoDiffusion
BentoDiffusion is a BentoML example project that demonstrates how to serve and deploy diffusion models in the Stable Diffusion (SD) family. These models are specialized in generating and manipulating images based on text prompts. The project provides a guide on using SDXL Turbo as an example, along with instructions on prerequisites, installing dependencies, running the BentoML service, and deploying to BentoCloud. Users can interact with the deployed service using Swagger UI or other methods. Additionally, the project offers the option to choose from various diffusion models available in the repository for deployment.
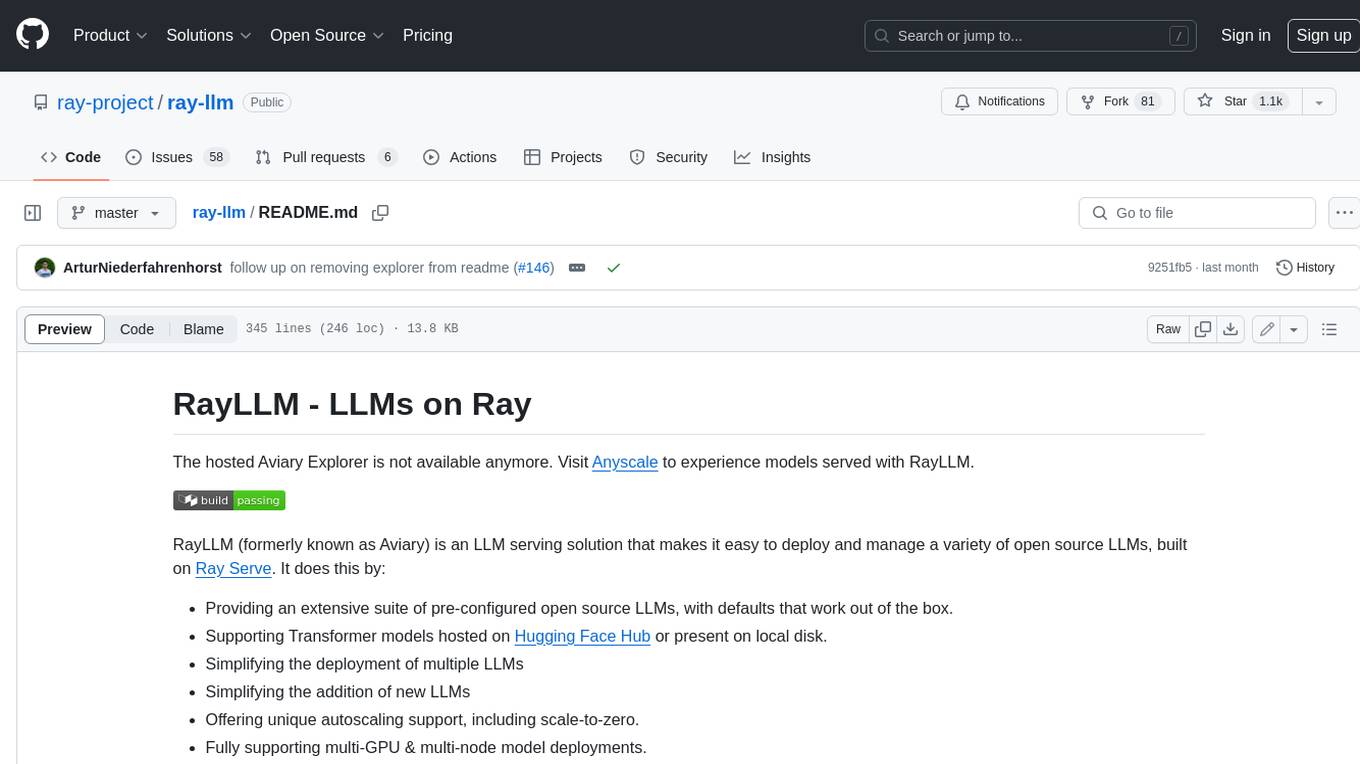
ray-llm
RayLLM (formerly known as Aviary) is an LLM serving solution that makes it easy to deploy and manage a variety of open source LLMs, built on Ray Serve. It provides an extensive suite of pre-configured open source LLMs, with defaults that work out of the box. RayLLM supports Transformer models hosted on Hugging Face Hub or present on local disk. It simplifies the deployment of multiple LLMs, the addition of new LLMs, and offers unique autoscaling support, including scale-to-zero. RayLLM fully supports multi-GPU & multi-node model deployments and offers high performance features like continuous batching, quantization and streaming. It provides a REST API that is similar to OpenAI's to make it easy to migrate and cross test them. RayLLM supports multiple LLM backends out of the box, including vLLM and TensorRT-LLM.
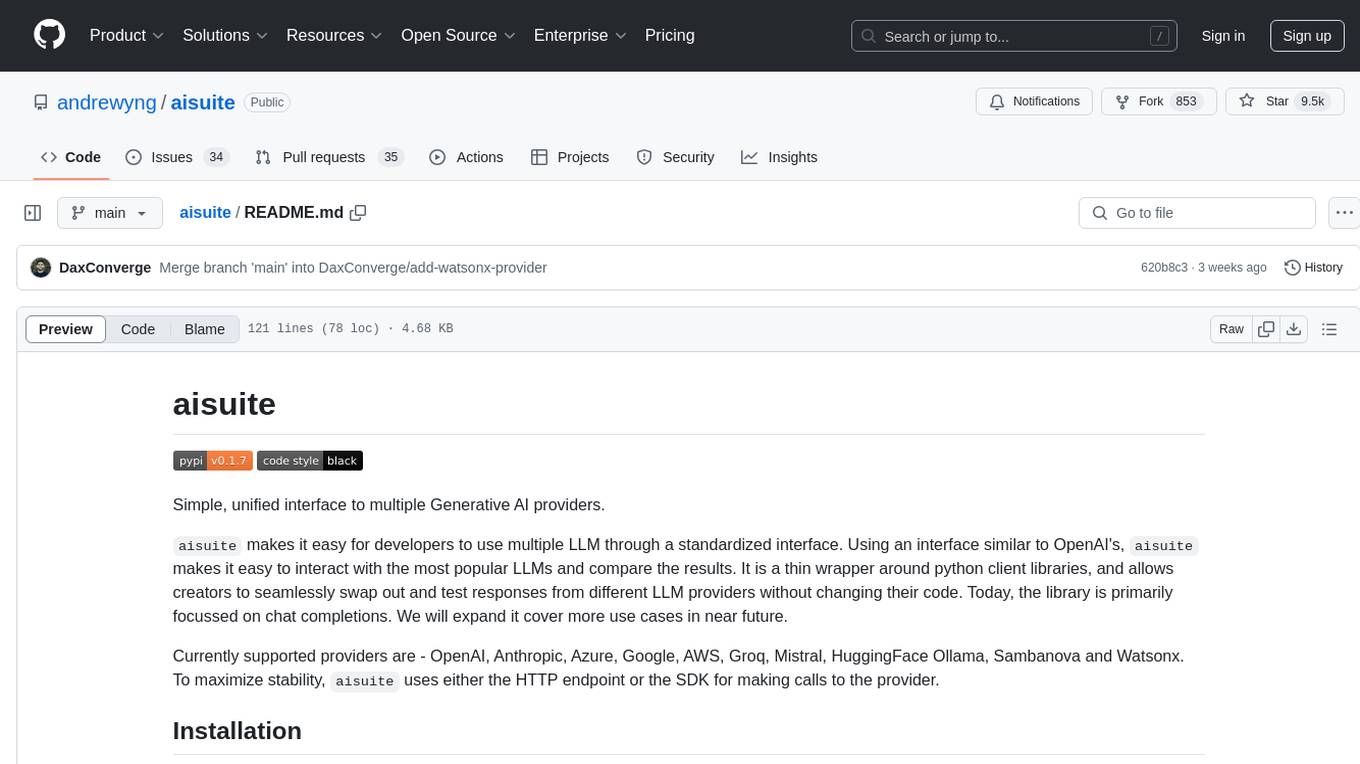
aisuite
Aisuite is a simple, unified interface to multiple Generative AI providers. It allows developers to easily interact with various Language Model (LLM) providers like OpenAI, Anthropic, Azure, Google, AWS, and more through a standardized interface. The library focuses on chat completions and provides a thin wrapper around python client libraries, enabling creators to test responses from different LLM providers without changing their code. Aisuite maximizes stability by using HTTP endpoints or SDKs for making calls to the providers. Users can install the base package or specific provider packages, set up API keys, and utilize the library to generate chat completion responses from different models.
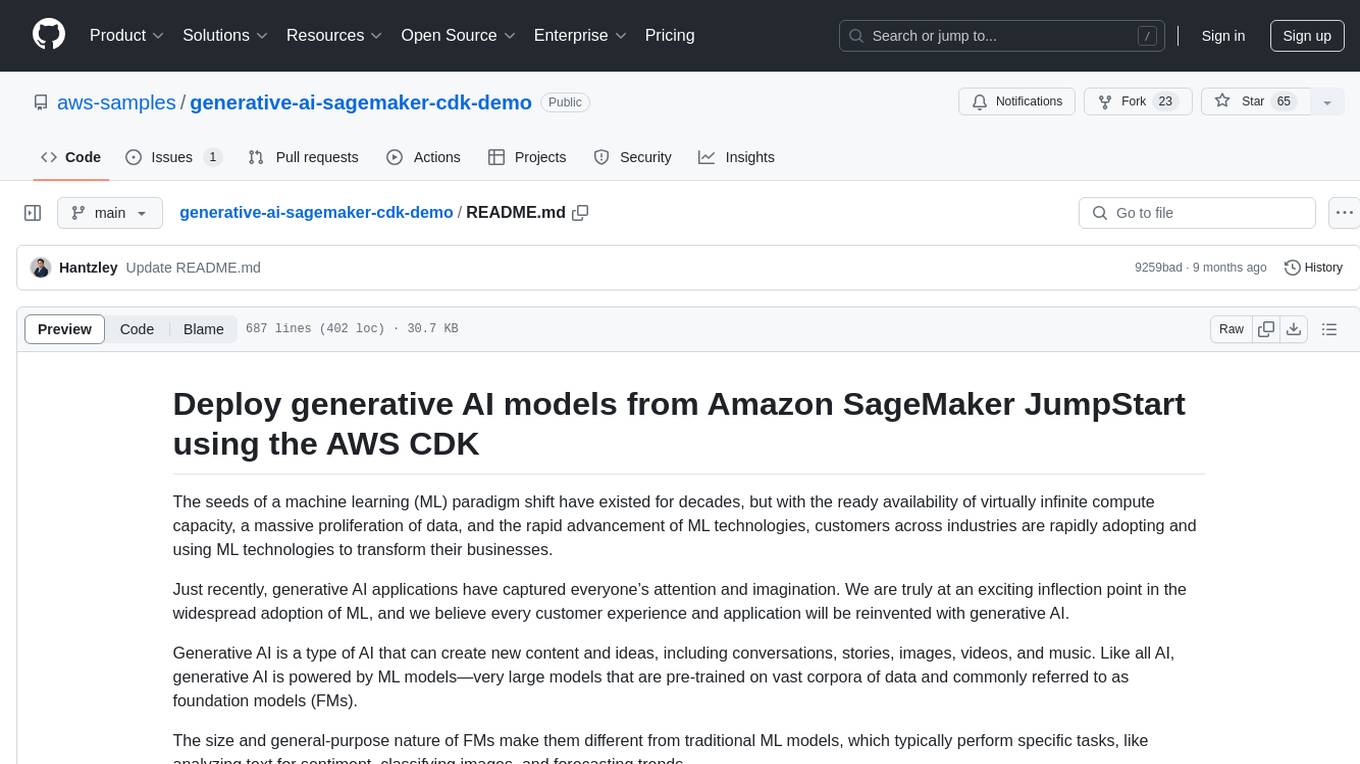
generative-ai-sagemaker-cdk-demo
This repository showcases how to deploy generative AI models from Amazon SageMaker JumpStart using the AWS CDK. Generative AI is a type of AI that can create new content and ideas, such as conversations, stories, images, videos, and music. The repository provides a detailed guide on deploying image and text generative AI models, utilizing pre-trained models from SageMaker JumpStart. The web application is built on Streamlit and hosted on Amazon ECS with Fargate. It interacts with the SageMaker model endpoints through Lambda functions and Amazon API Gateway. The repository also includes instructions on setting up the AWS CDK application, deploying the stacks, using the models, and viewing the deployed resources on the AWS Management Console.
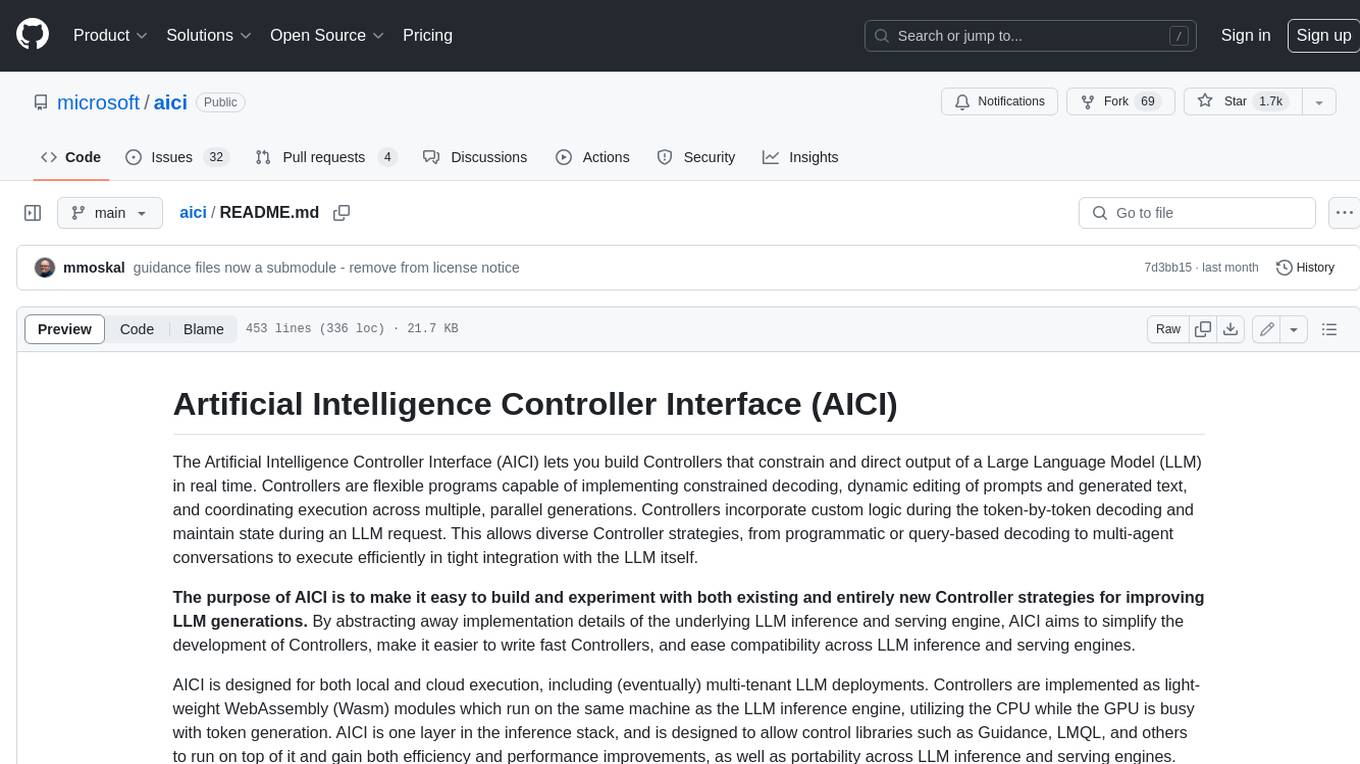
aici
The Artificial Intelligence Controller Interface (AICI) lets you build Controllers that constrain and direct output of a Large Language Model (LLM) in real time. Controllers are flexible programs capable of implementing constrained decoding, dynamic editing of prompts and generated text, and coordinating execution across multiple, parallel generations. Controllers incorporate custom logic during the token-by-token decoding and maintain state during an LLM request. This allows diverse Controller strategies, from programmatic or query-based decoding to multi-agent conversations to execute efficiently in tight integration with the LLM itself.
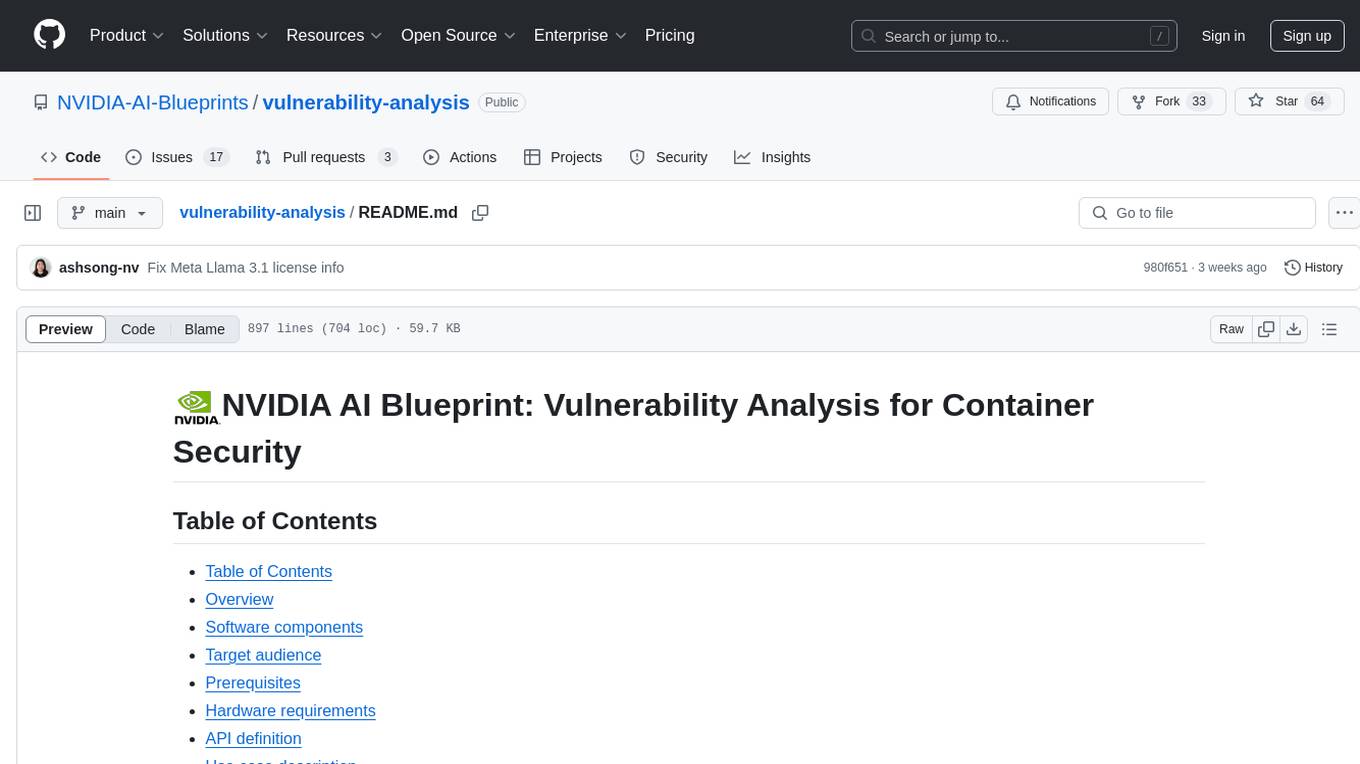
vulnerability-analysis
The NVIDIA AI Blueprint for Vulnerability Analysis for Container Security showcases accelerated analysis on common vulnerabilities and exposures (CVE) at an enterprise scale, reducing mitigation time from days to seconds. It enables security analysts to determine software package vulnerabilities using large language models (LLMs) and retrieval-augmented generation (RAG). The blueprint is designed for security analysts, IT engineers, and AI practitioners in cybersecurity. It requires NVAIE developer license and API keys for vulnerability databases, search engines, and LLM model services. Hardware requirements include L40 GPU for pipeline operation and optional LLM NIM and Embedding NIM. The workflow involves LLM pipeline for CVE impact analysis, utilizing LLM planner, agent, and summarization nodes. The blueprint uses NVIDIA NIM microservices and Morpheus Cybersecurity AI SDK for vulnerability analysis.
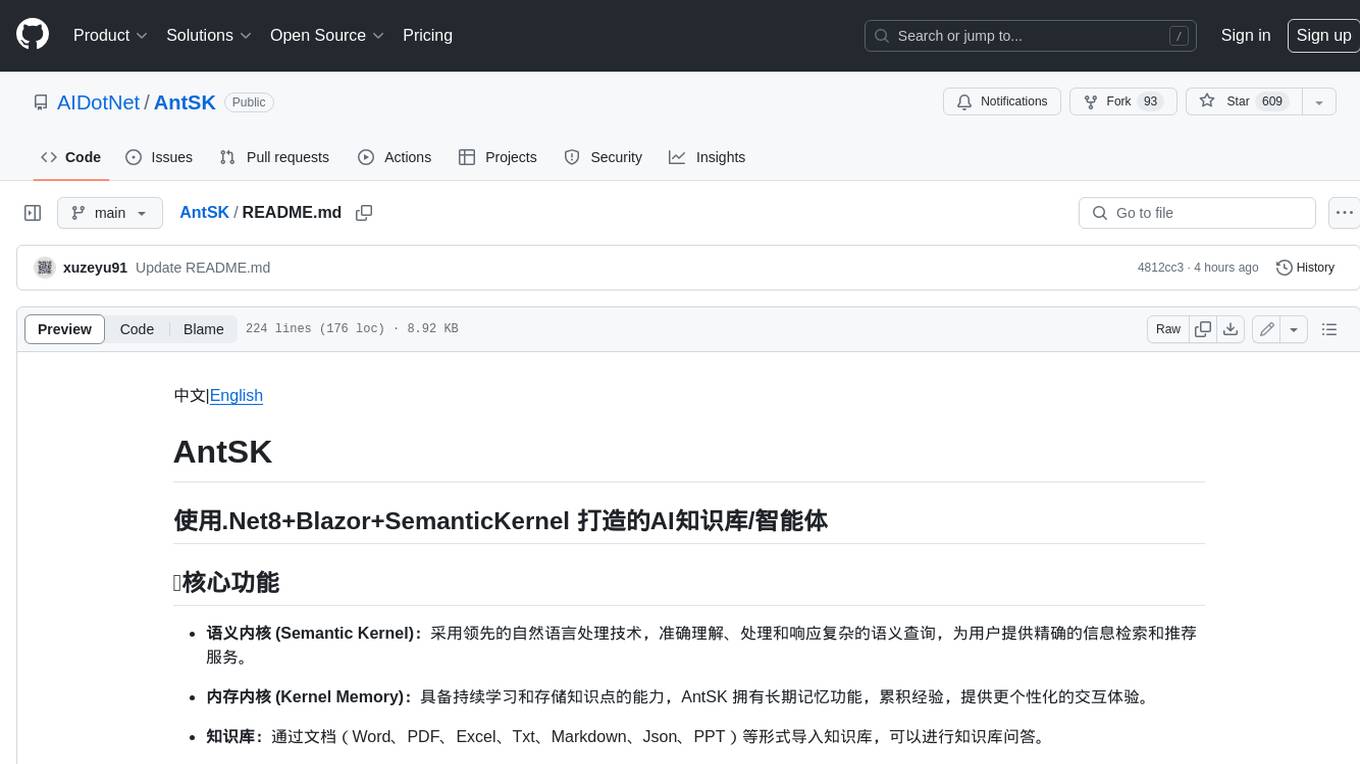
AntSK
AntSK is an AI knowledge base/agent built with .Net8+Blazor+SemanticKernel. It features a semantic kernel for accurate natural language processing, a memory kernel for continuous learning and knowledge storage, a knowledge base for importing and querying knowledge from various document formats, a text-to-image generator integrated with StableDiffusion, GPTs generation for creating personalized GPT models, API interfaces for integrating AntSK into other applications, an open API plugin system for extending functionality, a .Net plugin system for integrating business functions, real-time information retrieval from the internet, model management for adapting and managing different models from different vendors, support for domestic models and databases for operation in a trusted environment, and planned model fine-tuning based on llamafactory.
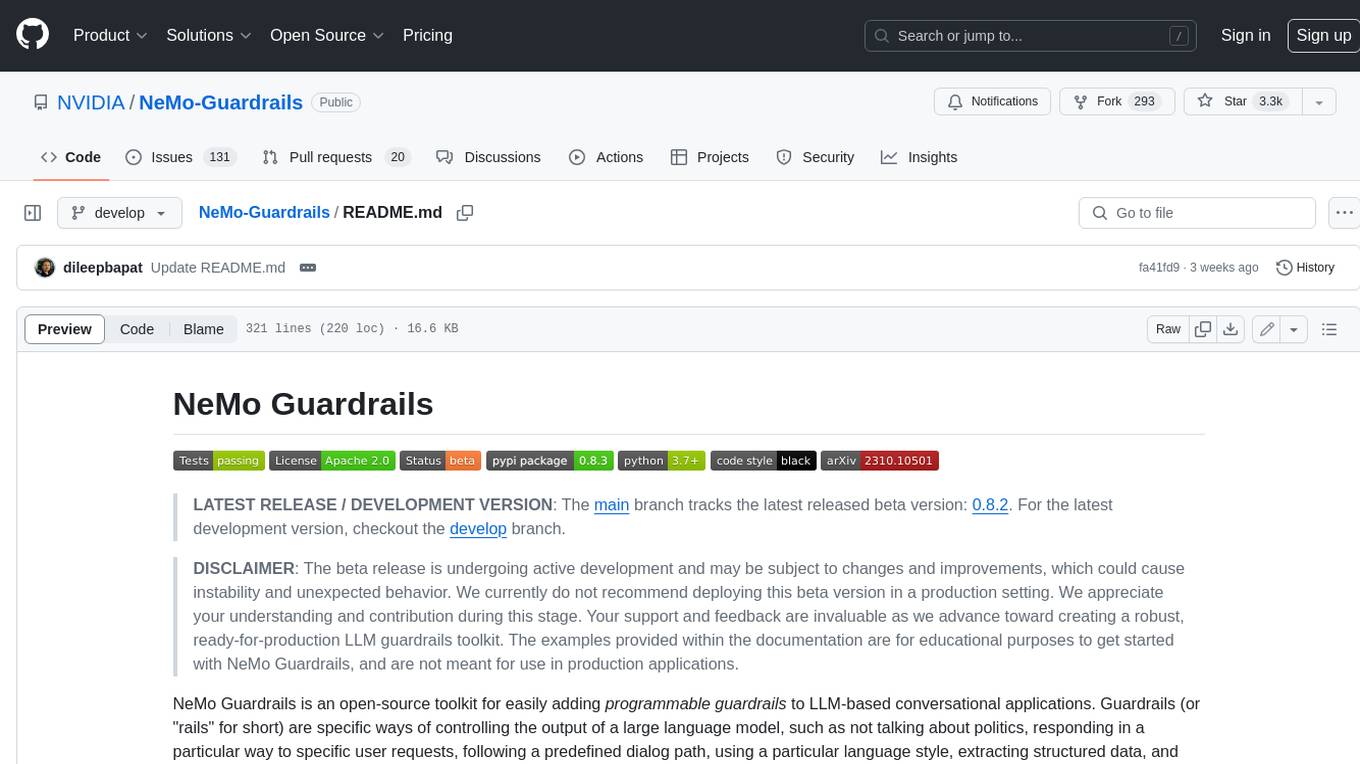
NeMo-Guardrails
NeMo Guardrails is an open-source toolkit for easily adding _programmable guardrails_ to LLM-based conversational applications. Guardrails (or "rails" for short) are specific ways of controlling the output of a large language model, such as not talking about politics, responding in a particular way to specific user requests, following a predefined dialog path, using a particular language style, extracting structured data, and more.
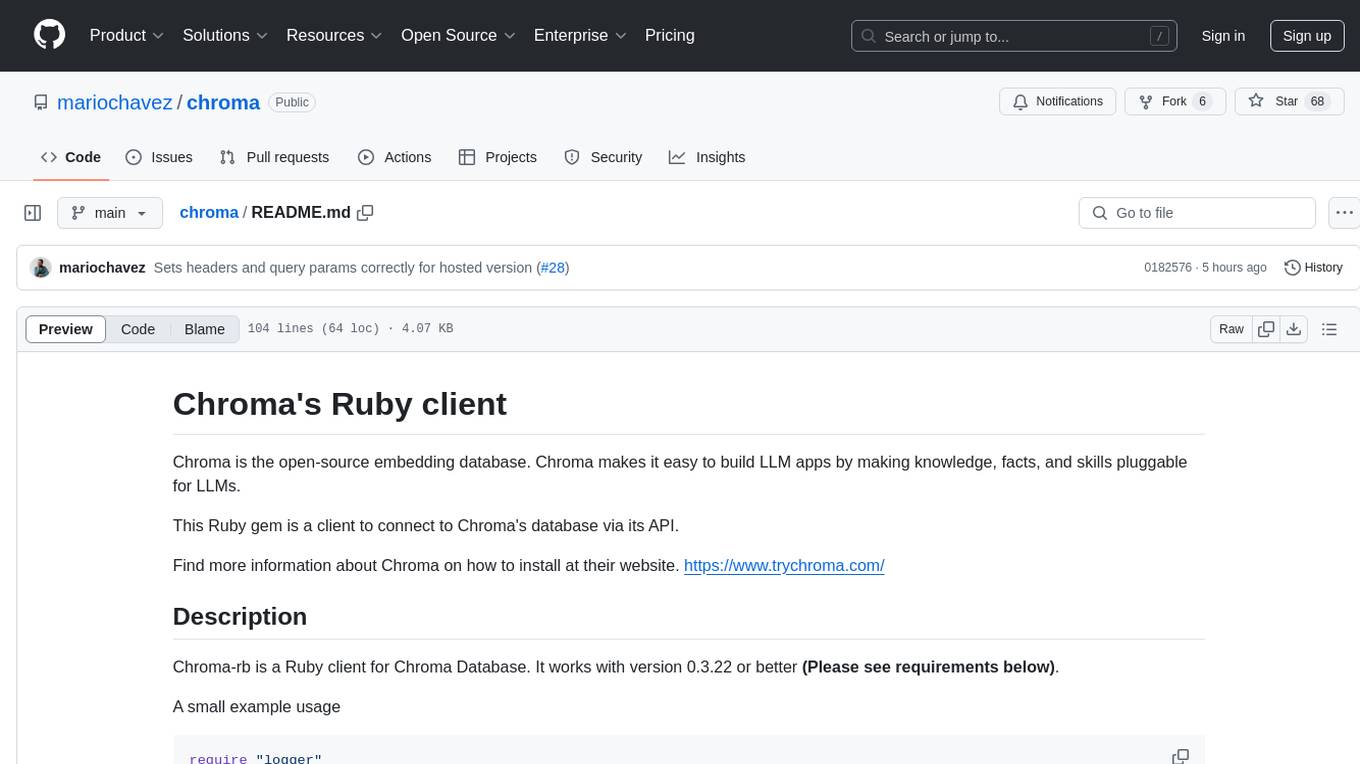
chroma
Chroma is an open-source embedding database that simplifies building LLM apps by enabling the integration of knowledge, facts, and skills for LLMs. The Ruby client for Chroma Database, chroma-rb, facilitates connecting to Chroma's database via its API. Users can configure the host, check server version, create collections, and add embeddings. The gem supports Chroma Database version 0.3.22 or newer, requiring Ruby 3.1.4 or later. It can be used with the hosted Chroma service at trychroma.com by setting configuration options like api_key, tenant, and database. Additionally, the gem provides integration with Jupyter Notebook for creating embeddings using Ollama and Nomic embed text with a Ruby HTTP client.
For similar tasks
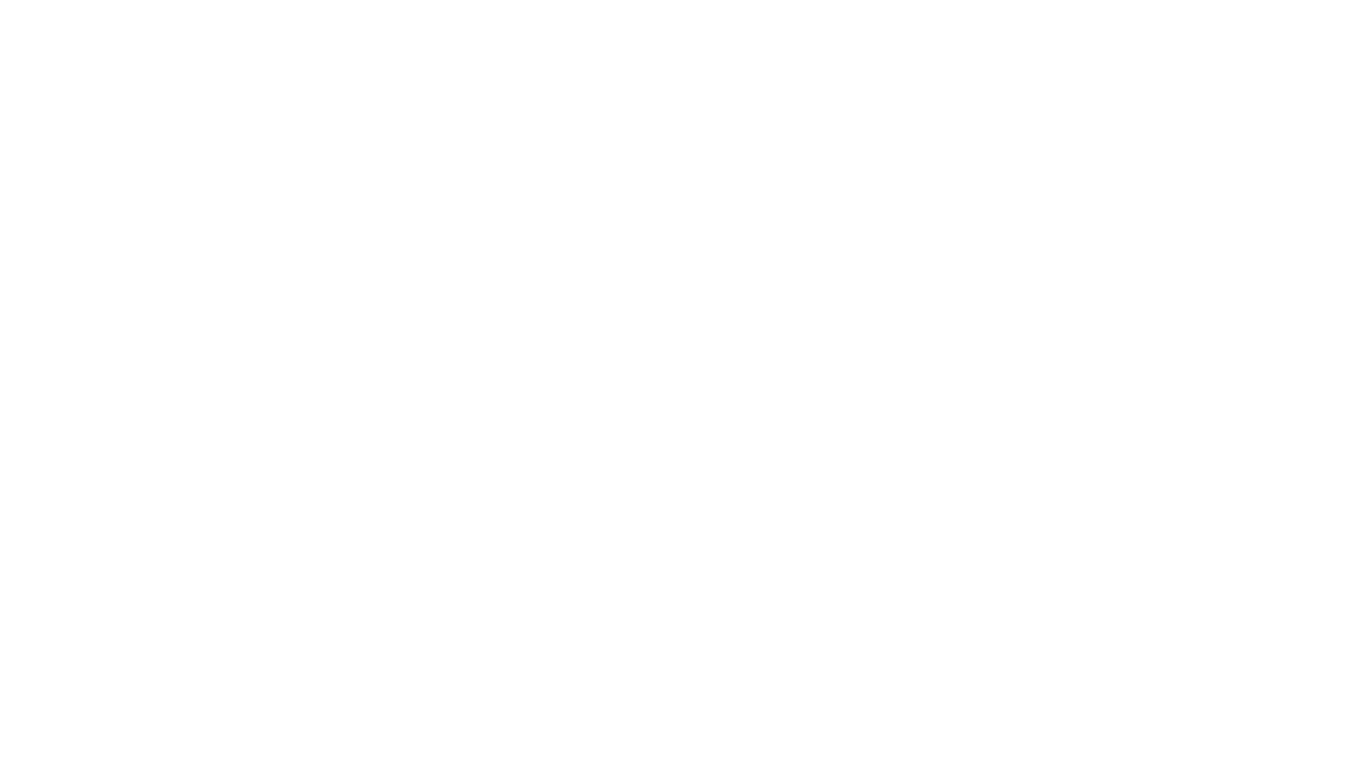
rag-experiment-accelerator
The RAG Experiment Accelerator is a versatile tool that helps you conduct experiments and evaluations using Azure AI Search and RAG pattern. It offers a rich set of features, including experiment setup, integration with Azure AI Search, Azure Machine Learning, MLFlow, and Azure OpenAI, multiple document chunking strategies, query generation, multiple search types, sub-querying, re-ranking, metrics and evaluation, report generation, and multi-lingual support. The tool is designed to make it easier and faster to run experiments and evaluations of search queries and quality of response from OpenAI, and is useful for researchers, data scientists, and developers who want to test the performance of different search and OpenAI related hyperparameters, compare the effectiveness of various search strategies, fine-tune and optimize parameters, find the best combination of hyperparameters, and generate detailed reports and visualizations from experiment results.
For similar jobs
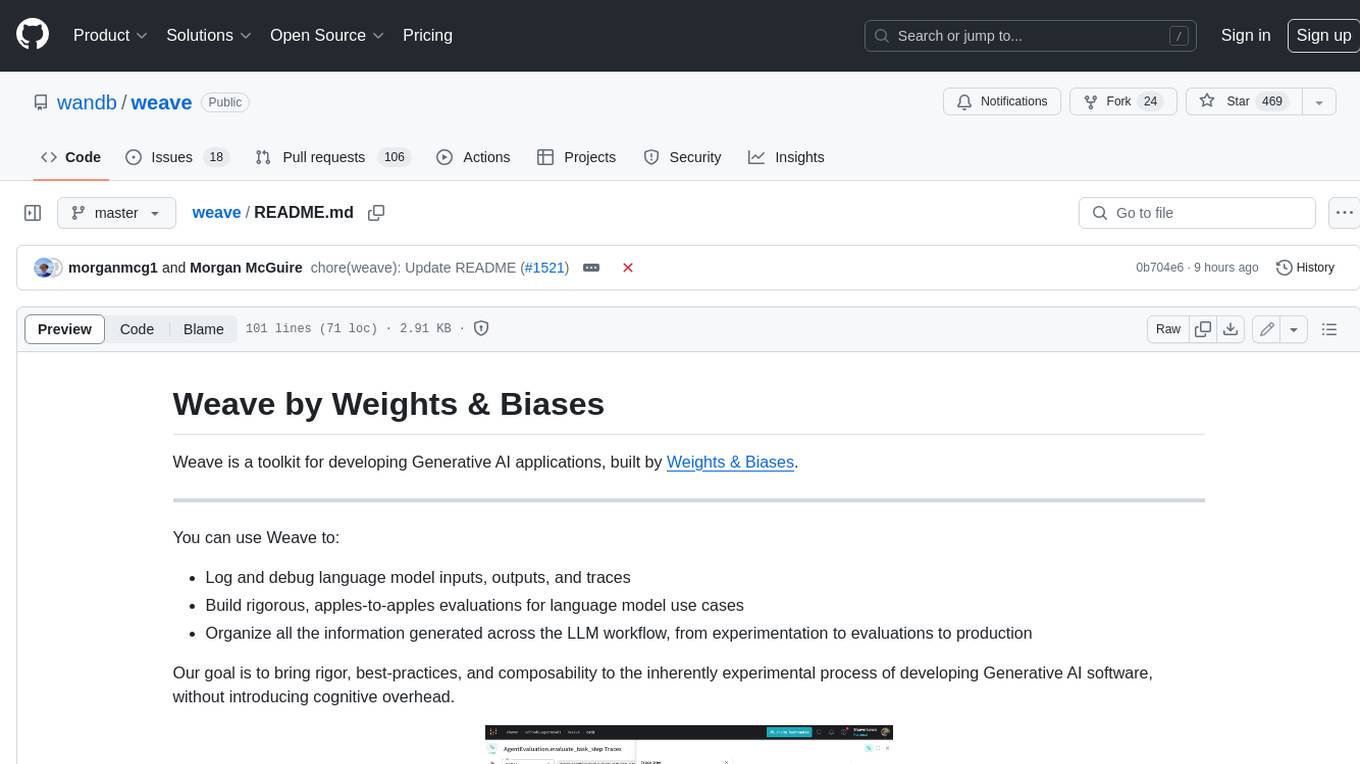
weave
Weave is a toolkit for developing Generative AI applications, built by Weights & Biases. With Weave, you can log and debug language model inputs, outputs, and traces; build rigorous, apples-to-apples evaluations for language model use cases; and organize all the information generated across the LLM workflow, from experimentation to evaluations to production. Weave aims to bring rigor, best-practices, and composability to the inherently experimental process of developing Generative AI software, without introducing cognitive overhead.
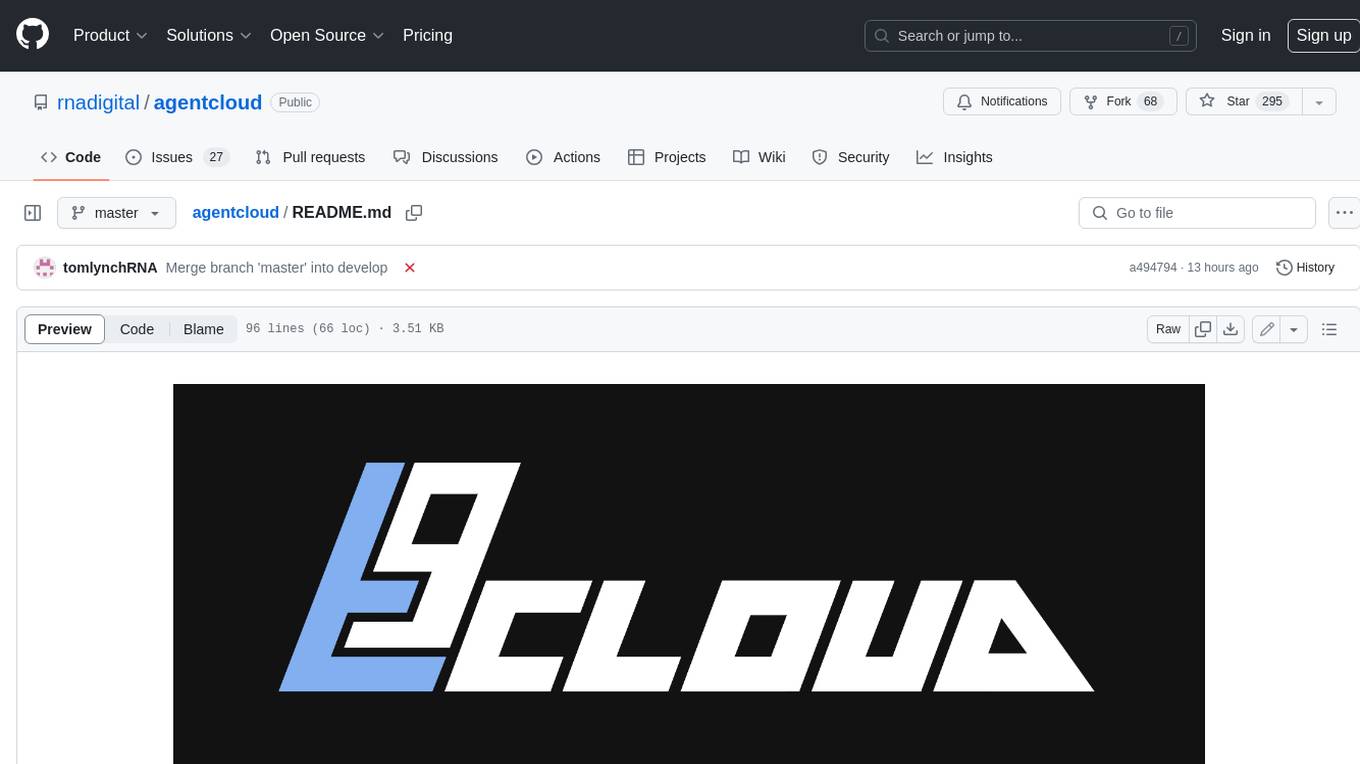
agentcloud
AgentCloud is an open-source platform that enables companies to build and deploy private LLM chat apps, empowering teams to securely interact with their data. It comprises three main components: Agent Backend, Webapp, and Vector Proxy. To run this project locally, clone the repository, install Docker, and start the services. The project is licensed under the GNU Affero General Public License, version 3 only. Contributions and feedback are welcome from the community.
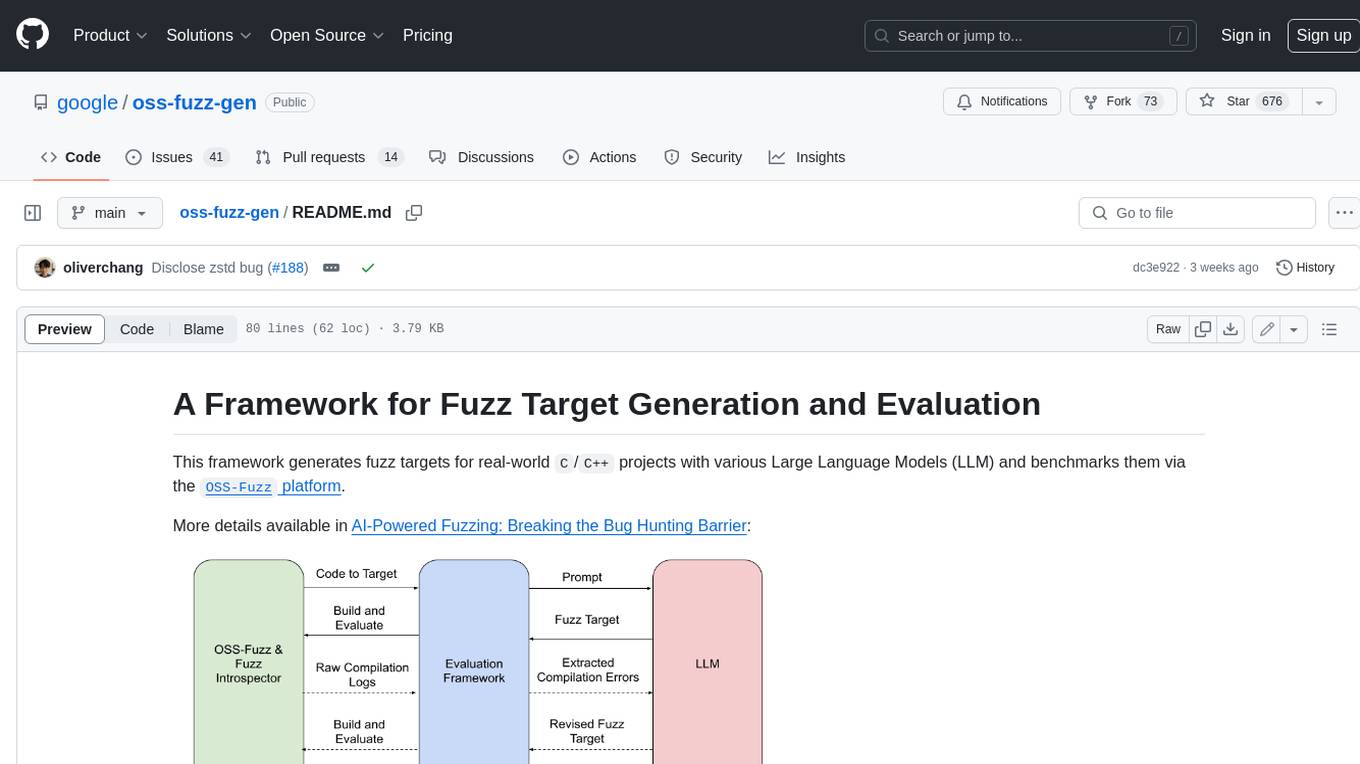
oss-fuzz-gen
This framework generates fuzz targets for real-world `C`/`C++` projects with various Large Language Models (LLM) and benchmarks them via the `OSS-Fuzz` platform. It manages to successfully leverage LLMs to generate valid fuzz targets (which generate non-zero coverage increase) for 160 C/C++ projects. The maximum line coverage increase is 29% from the existing human-written targets.
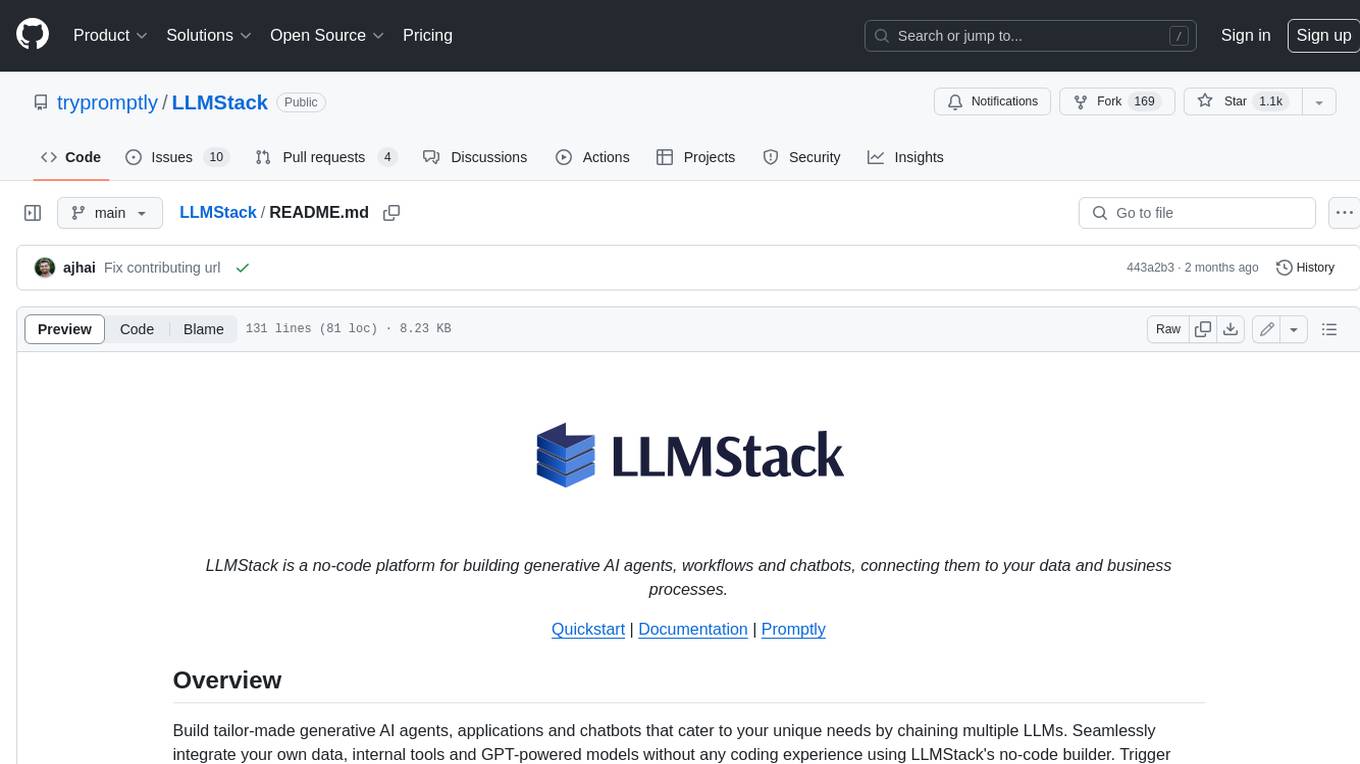
LLMStack
LLMStack is a no-code platform for building generative AI agents, workflows, and chatbots. It allows users to connect their own data, internal tools, and GPT-powered models without any coding experience. LLMStack can be deployed to the cloud or on-premise and can be accessed via HTTP API or triggered from Slack or Discord.
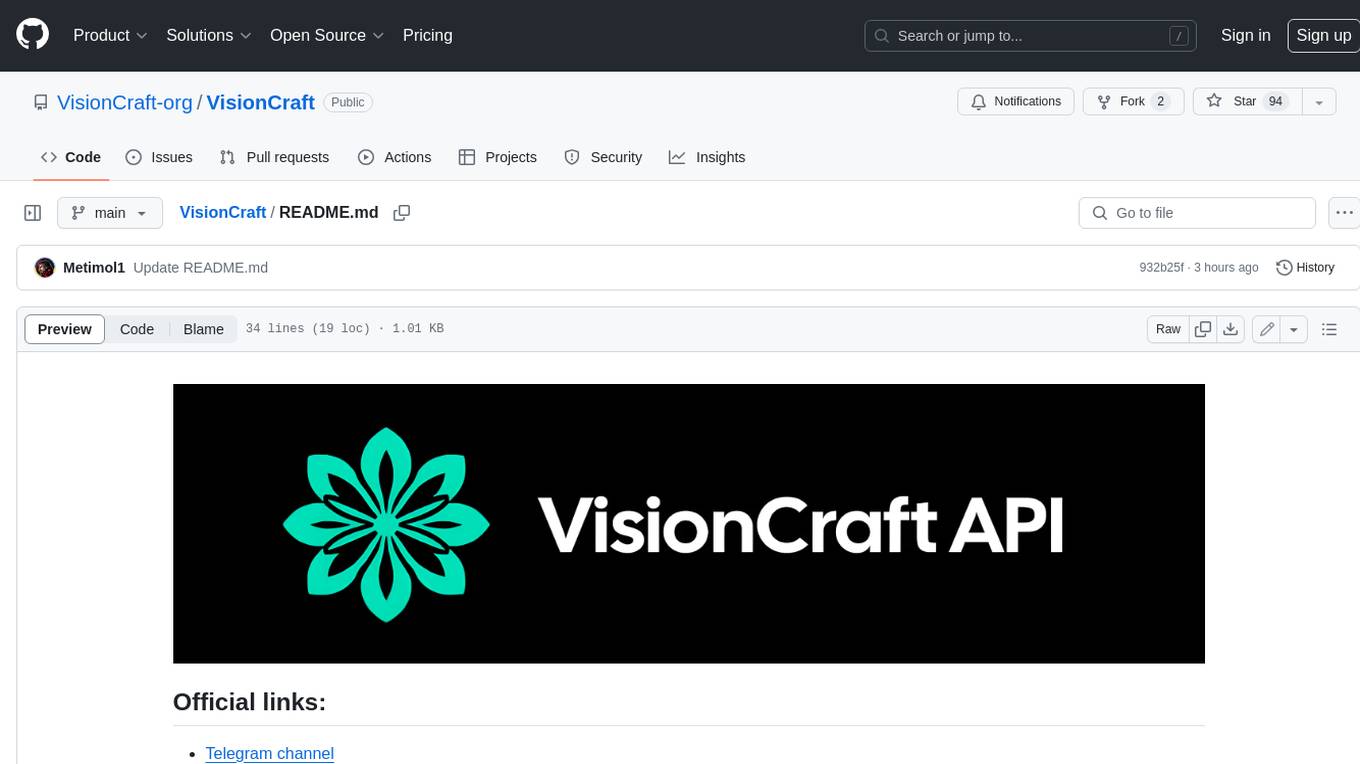
VisionCraft
The VisionCraft API is a free API for using over 100 different AI models. From images to sound.
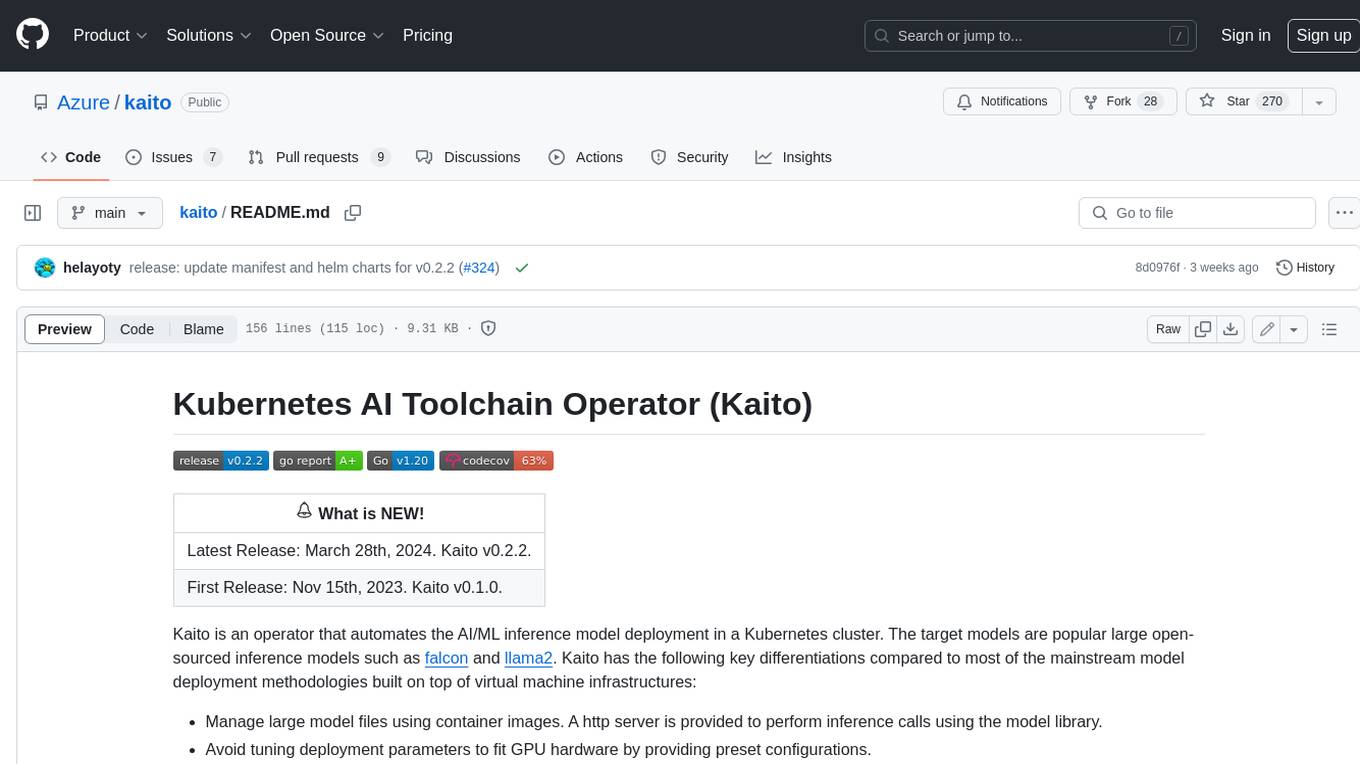
kaito
Kaito is an operator that automates the AI/ML inference model deployment in a Kubernetes cluster. It manages large model files using container images, avoids tuning deployment parameters to fit GPU hardware by providing preset configurations, auto-provisions GPU nodes based on model requirements, and hosts large model images in the public Microsoft Container Registry (MCR) if the license allows. Using Kaito, the workflow of onboarding large AI inference models in Kubernetes is largely simplified.
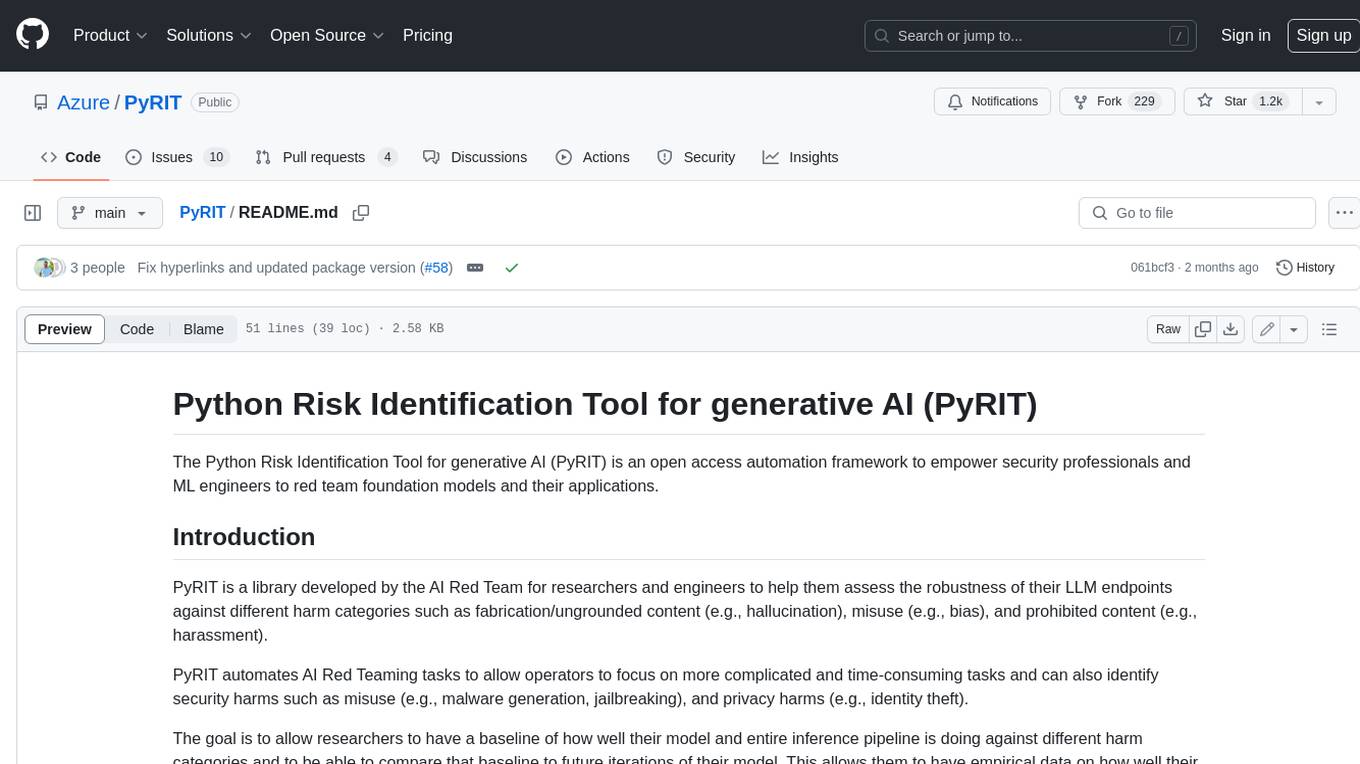
PyRIT
PyRIT is an open access automation framework designed to empower security professionals and ML engineers to red team foundation models and their applications. It automates AI Red Teaming tasks to allow operators to focus on more complicated and time-consuming tasks and can also identify security harms such as misuse (e.g., malware generation, jailbreaking), and privacy harms (e.g., identity theft). The goal is to allow researchers to have a baseline of how well their model and entire inference pipeline is doing against different harm categories and to be able to compare that baseline to future iterations of their model. This allows them to have empirical data on how well their model is doing today, and detect any degradation of performance based on future improvements.
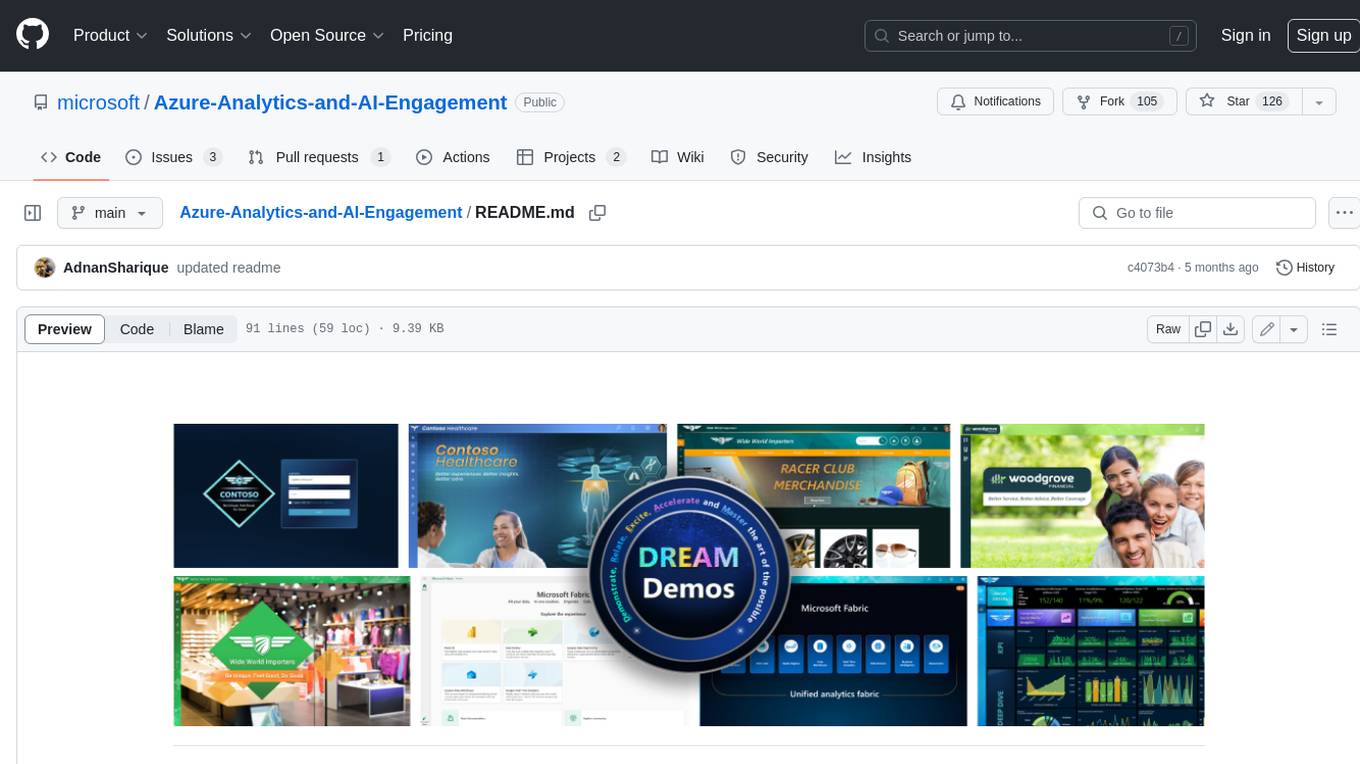
Azure-Analytics-and-AI-Engagement
The Azure-Analytics-and-AI-Engagement repository provides packaged Industry Scenario DREAM Demos with ARM templates (Containing a demo web application, Power BI reports, Synapse resources, AML Notebooks etc.) that can be deployed in a customer’s subscription using the CAPE tool within a matter of few hours. Partners can also deploy DREAM Demos in their own subscriptions using DPoC.