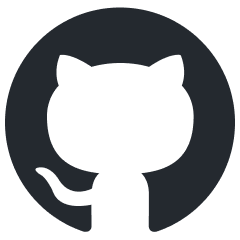
aiac
Artificial Intelligence Infrastructure-as-Code Generator.
Stars: 3355
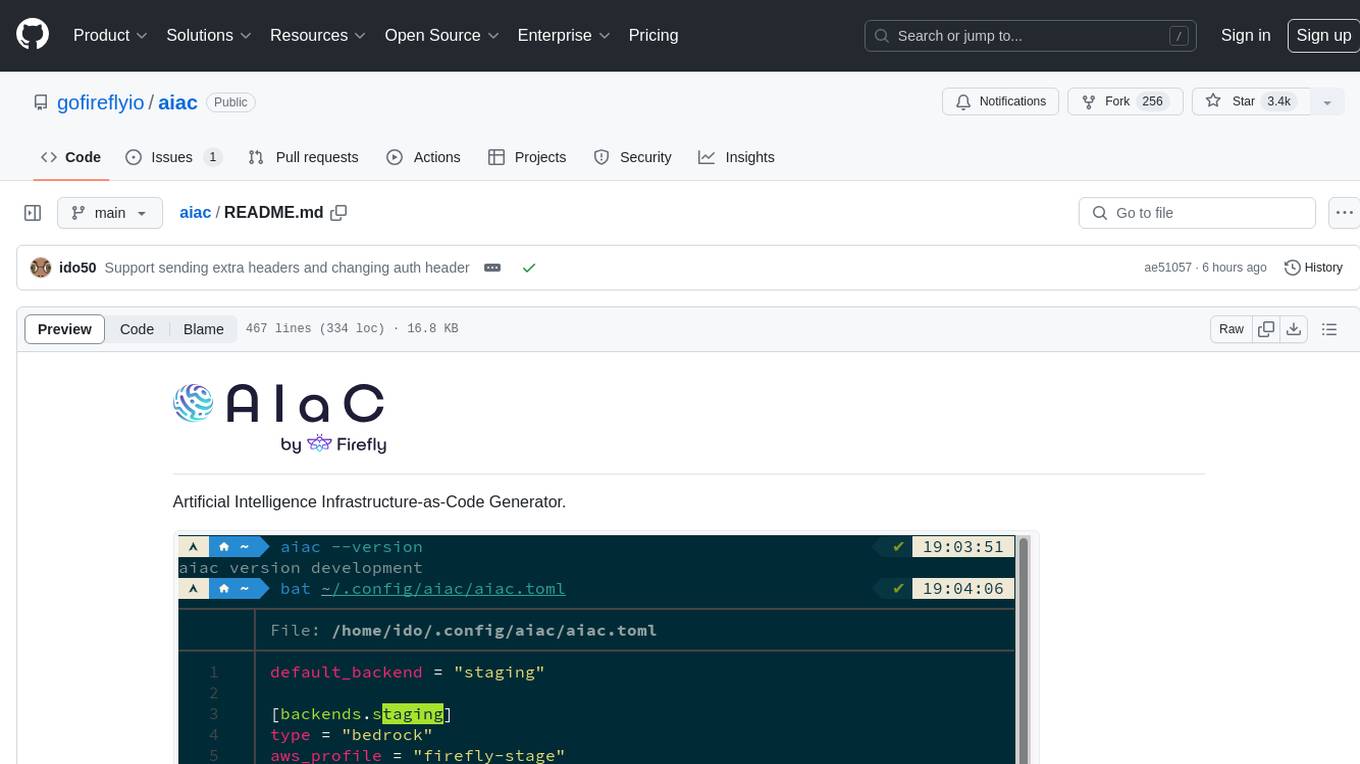
AIAC is a library and command line tool to generate Infrastructure as Code (IaC) templates, configurations, utilities, queries, and more via LLM providers such as OpenAI, Amazon Bedrock, and Ollama. Users can define multiple 'backends' targeting different LLM providers and environments using a simple configuration file. The tool allows users to ask a model to generate templates for different scenarios and composes an appropriate request to the selected provider, storing the resulting code to a file and/or printing it to standard output.
README:
Artificial Intelligence Infrastructure-as-Code Generator.
aiac
is a library and command line tool to generate IaC (Infrastructure as Code)
templates, configurations, utilities, queries and more via LLM providers such
as OpenAI, Amazon Bedrock and Ollama.
The CLI allows you to ask a model to generate templates for different scenarios (e.g. "get terraform for AWS EC2"). It composes an appropriate request to the selected provider, and stores the resulting code to a file, and/or prints it to standard output.
Users can define multiple "backends" targeting different LLM providers and environments using a simple configuration file.
aiac terraform for a highly available eks
aiac pulumi golang for an s3 with sns notification
aiac cloudformation for a neptundb
aiac dockerfile for a secured nginx
aiac k8s manifest for a mongodb deployment
aiac jenkins pipeline for building nodejs
aiac github action that plans and applies terraform and sends a slack notification
aiac opa policy that enforces readiness probe at k8s deployments
aiac python code that scans all open ports in my network
aiac bash script that kills all active terminal sessions
aiac kubectl that gets ExternalIPs of all nodes
aiac awscli that lists instances with public IP address and Name
aiac mongo query that aggregates all documents by created date
aiac elastic query that applies a condition on a value greater than some value in aggregation
aiac sql query that counts the appearances of each row in one table in another table based on an id column
Before installing/running aiac
, you may need to configure your LLM providers
or collect some information.
For OpenAI, you will need an API key in order for aiac
to work. Refer to
OpenAI's pricing model for more information. If you're not using the API hosted
by OpenAI (for example, you may be using Azure OpenAI), you will also need to
provide the API URL endpoint.
For Amazon Bedrock, you will need an AWS account with Bedrock enabled, and access to relevant models. Refer to the Bedrock documentation for more information.
For Ollama, you only need the URL to the local Ollama API server, including
the /api path prefix. This defaults to http://localhost:11434/api. Ollama does
not provide an authentication mechanism, but one may be in place in case of a
proxy server being used. This scenario is not currently supported by aiac
.
Via brew
:
brew tap gofireflyio/aiac https://github.com/gofireflyio/aiac
brew install aiac
Using docker
:
docker pull ghcr.io/gofireflyio/aiac
Using go install
:
go install github.com/gofireflyio/aiac/v5@latest
Alternatively, clone the repository and build from source:
git clone https://github.com/gofireflyio/aiac.git
go build
aiac
is also available in the Arch Linux user repository (AUR) as aiac (which
compiles from source) and aiac-bin (which downloads a compiled executable).
aiac
is configured via a TOML configuration file. Unless a specific path is
provided, aiac
looks for a configuration file in the user's XDG_CONFIG_HOME
directory, specifically ${XDG_CONFIG_HOME}/aiac/aiac.toml
. On Unix-like
operating systems, this will default to "~/.config/aiac/aiac.toml". If you want
to use a different path, provide the --config
or -c
flag with the file's path.
The configuration file defines one or more named backends. Each backend has a type identifying the LLM provider (e.g. "openai", "bedrock", "ollama"), and various settings relevant to that provider. Multiple backends of the same LLM provider can be configured, for example for "staging" and "production" environments.
Here's an example configuration file:
default_backend = "official_openai" # Default backend when one is not selected
[backends.official_openai]
type = "openai"
api_key = "API KEY"
default_model = "gpt-4o" # Default model to use for this backend
[backends.azure_openai]
type = "openai"
url = "https://tenant.openai.azure.com/openai/deployments/test"
api_key = "API KEY"
api_version = "2023-05-15" # Optional
auth_header = "api-key" # Default is "Authorization"
extra_headers = { X-Header-1 = "one", X-Header-2 = "two" }
[backends.aws_staging]
type = "bedrock"
aws_profile = "staging"
aws_region = "eu-west-2"
[backends.aws_prod]
type = "bedrock"
aws_profile = "production"
aws_region = "us-east-1"
default_model = "amazon.titan-text-express-v1"
[backends.localhost]
type = "ollama"
url = "http://localhost:11434/api" # This is the default
Notes:
- Every backend can have a default model (via configuration key
default_model
). If not provided, calls that do not define a model will fail. - Backends of type "openai" can change the header used for authorization by
providing the
auth_header
setting. This defaults to "Authorization", but Azure OpenAI uses "api-key" instead. When the header is either "Authorization" or "Proxy-Authorization", the header's value for requests will be "Bearer API_KEY". If it's anything else, it'll simply be "API_KEY". - Backends of type "openai" and "ollama" support adding extra headers to every
request issued by aiac, by utilizing the
extra_headers
setting.
Once a configuration file is created, you can start generating code and you only
need to refer to the name of the backend. You can use aiac
from the command
line, or as a Go library.
Before starting to generate code, you can list all models available in a backend:
aiac -b aws_prod --list-models
This will return a list of all available models. Note that depending on the LLM provider, this may list models that aren't accessible or enabled for the specific account.
By default, aiac prints the extracted code to standard output and opens an interactive shell that allows conversing with the model, retrying requests, saving output to files, copying code to clipboard, and more:
aiac terraform for AWS EC2
This will use the default backend in the configuration file and the default
model for that backend, assuming they are indeed defined. To use a specific
backend, provide the --backend
or -b
flag:
aiac -b aws_prod terraform for AWS EC2
To use a specific model, provide the --model
or -m
flag:
aiac -m gpt-4-turbo terraform for AWS EC2
You can ask aiac
to save the resulting code to a specific file:
aiac terraform for eks --output-file=eks.tf
You can use a flag to save the full Markdown output as well:
aiac terraform for eks --output-file=eks.tf --readme-file=eks.md
If you prefer aiac to print the full Markdown output to standard output rather
than the extracted code, use the -f
or --full
flag:
aiac terraform for eks -f
You can use aiac in non-interactive mode, simply printing the generated code
to standard output, and optionally saving it to files with the above flags,
by providing the -q
or --quiet
flag:
aiac terraform for eks -q
In quiet mode, you can also send the resulting code to the clipboard by
providing the --clipboard
flag:
aiac terraform for eks -q --clipboard
Note that aiac will not exit in this case until the contents of the clipboard changes. This is due to the mechanics of the clipboard.
All the same instructions apply, except you execute a docker
image:
docker run \
-it \
-v ~/.config/aiac/aiac.toml:~/.config/aiac/aiac.toml \
ghcr.io/gofireflyio/aiac terraform for ec2
You can use aiac
as a Go library:
package main
import (
"context"
"log"
"os"
"github.com/gofireflyio/aiac/v5/libaiac"
)
func main() {
aiac, err := libaiac.New() // Will load default configuration path.
// You can also do libaiac.New("/path/to/aiac.toml")
if err != nil {
log.Fatalf("Failed creating aiac object: %s", err)
}
ctx := context.TODO()
models, err := aiac.ListModels(ctx, "backend name")
if err != nil {
log.Fatalf("Failed listing models: %s", err)
}
chat, err := aiac.Chat(ctx, "backend name", "model name")
if err != nil {
log.Fatalf("Failed starting chat: %s", err)
}
res, err = chat.Send(ctx, "generate terraform for eks")
res, err = chat.Send(ctx, "region must be eu-central-1")
}
Version 5.0.0 introduced a significant change to the aiac
API in both the
command line and library forms, as per feedback from the community.
Before v5, there was no concept of a configuration file or named backends. Users had to provide all the information necessary to contact a specific LLM provider via command line flags or environment variables, and the library allowed creating a "client" object that could only talk with one LLM provider.
Backends are now configured only via the configuration file. Refer to the
Configuration section for instructions. Provider-specific flags such as
--api-key
, --aws-profile
, etc. (and their respective environment variables,
if any) are no longer accepted.
Since v5, backends are also named. Previously, the --backend
and -b
flags
referred to the name of the LLM provider (e.g. "openai", "bedrock", "ollama").
Now they refer to whatever name you've defined in the configuration file:
[backends.my_local_llm]
type = "ollama"
url = "http://localhost:11434/api"
Here we configure an Ollama backend named "my_local_llm". When you want to
generate code with this backend, you will use -b my_local_llm
rather than
-b ollama
, as multiple backends may exist for the same LLM provider.
Before v5, the command line was split into three subcommands: get
,
list-models
and version
. Due to this hierarchical nature of the CLI, flags may
not have been accepted if they were provided in the "wrong location". For
example, the --model
flag had to be provided after the word "get", otherwise
it would not be accepted. In v5, there are no subcommands, so the position of
the flags no longer matters.
The list-models
subcommand is replaced with the flag --list-models
, and the
version
subcommand is replaced with the flag --version
.
Before v5:
aiac -b ollama list-models
Since v5:
aiac -b my_local_llm --list-models
In earlier versions, the word "get" was actually a subcommand and not truly part of the prompt sent to the LLM provider. Since v5, there is no "get" subcommand, so you no longer need to add this word to your prompts.
Before v5:
aiac get terraform for S3 bucket
Since v5:
aiac terraform for S3 bucket
That said, adding either the word "get" or "generate" will not hurt, as v5 will simply remove it if provided.
Before v5, the models for each LLM provider were hardcoded in each backend
implementation, and each provider had a hardcoded default model. This
significantly limited the usability of the project, and required us to update
aiac
whenever new models were added or deprecated. On the other hand, we could
provide extra information about each model, such as its context lengths and
type, as we manually extracted them from the provider documentation.
Since v5, aiac
no longer hardcodes any models, including default ones. It
will not attempt to verify the model you select actually exists. The
--list-models
flag will now directly contact the chosen backend API to get a
list of supported models. Setting a model when generating code simply sends its
name to the API as-is. Also, instead of hardcoding a default model for each
backend, users can define their own default models in the configuration file:
[backends.my_local_llm]
type = "ollama"
url = "http://localhost:11434/api"
default_model = "mistral:latest"
Before v5, aiac
supported both completion models and chat models. Since v5,
it only supports chat models. Since none of the LLM provider APIs actually
note whether a model is a completion model or a chat model (or even an image
or video model), the --list-models
flag may list models which are not actually
usable, and attempting to use them will result in an error being returned from
the provider API. The reason we've decided to drop support for completion models
was that they require setting a maximum amount of tokens for the API to
generate (at least in OpenAI), which we can no longer do without knowing the
context length. Chat models are not only a lot more useful, but they do not have
this limitation.
Most LLM provider APIs, when returning a response to a prompt, will include a
"reason" for why the response ended where it did. Generally, the response should
end because the model finished generating a response, but sometimes the response
may be truncated due to the model's context length or the user's token
utilization. When the response did not "stop" because it finished generation,
the response is said to be "truncated". Before v5, if the API returned that the
response was truncated, aiac
returned an error. Since v5, an error is no longer
returned, as it seems that some providers do not return an accurate stop reason.
Instead, the library returns the stop reason as part of its output for users to
decide how to proceed.
Command line prompt:
aiac dockerfile for nodejs with comments
Output:
FROM node:latest
# Create app directory
WORKDIR /usr/src/app
# Install app dependencies
# A wildcard is used to ensure both package.json AND package-lock.json are copied
# where available (npm@5+)
COPY package*.json ./
RUN npm install
# If you are building your code for production
# RUN npm ci --only=production
# Bundle app source
COPY . .
EXPOSE 8080
CMD [ "node", "index.js" ]
Most errors that you are likely to encounter are coming from the LLM provider API, e.g. OpenAI or Amazon Bedrock. Some common errors you may encounter are:
-
"[insufficient_quota] You exceeded your current quota, please check your plan and billing details": As described in the Instructions section, OpenAI is a paid API with a certain amount of free credits given. This error means you have exceeded your quota, whether free or paid. You will need to top up to continue usage.
-
"[tokens] Rate limit reached...": The OpenAI API employs rate limiting as described here.
aiac
only performs individual requests and cannot workaround or prevent these rate limits. If you are usingaiac
in programmatically, you will have to implement throttling yourself. See here for tips.
This code is published under the terms of the Apache License 2.0.
For Tasks:
Click tags to check more tools for each tasksFor Jobs:
Alternative AI tools for aiac
Similar Open Source Tools
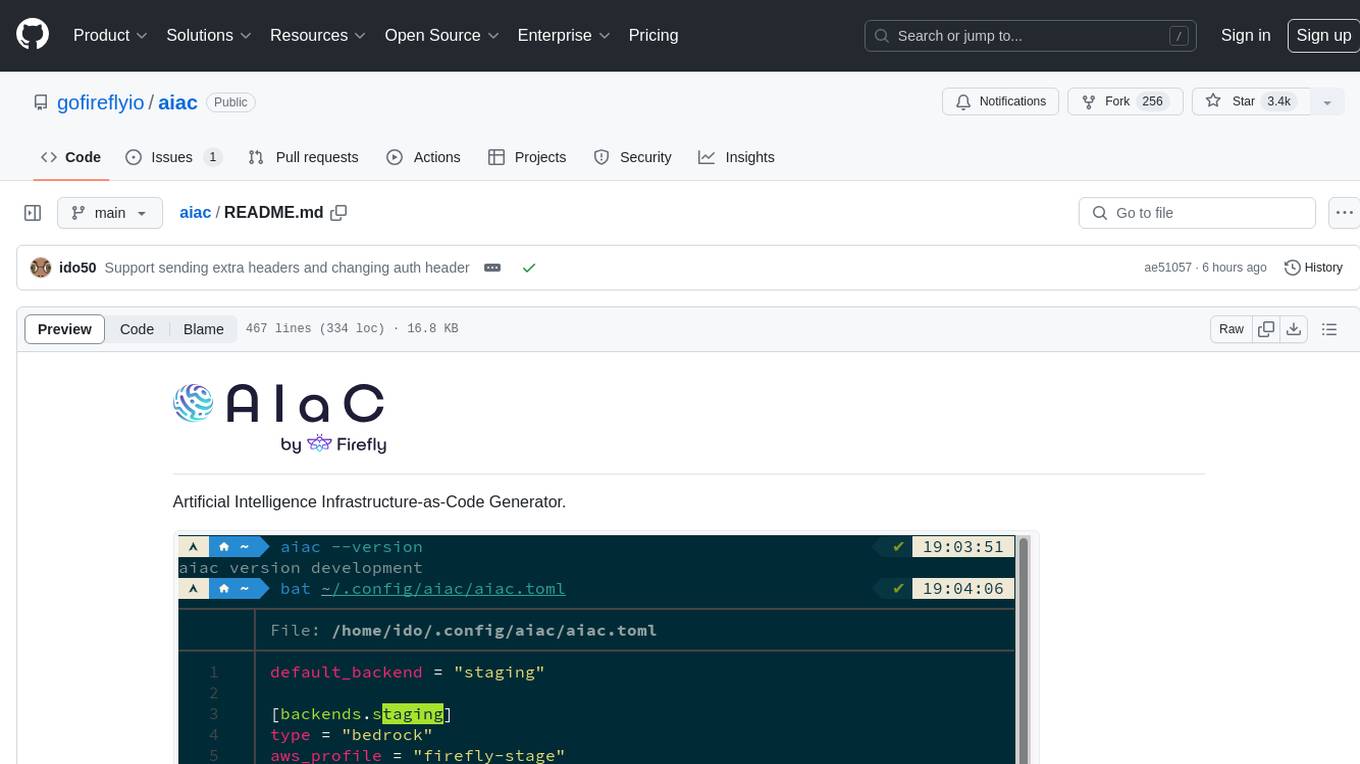
aiac
AIAC is a library and command line tool to generate Infrastructure as Code (IaC) templates, configurations, utilities, queries, and more via LLM providers such as OpenAI, Amazon Bedrock, and Ollama. Users can define multiple 'backends' targeting different LLM providers and environments using a simple configuration file. The tool allows users to ask a model to generate templates for different scenarios and composes an appropriate request to the selected provider, storing the resulting code to a file and/or printing it to standard output.
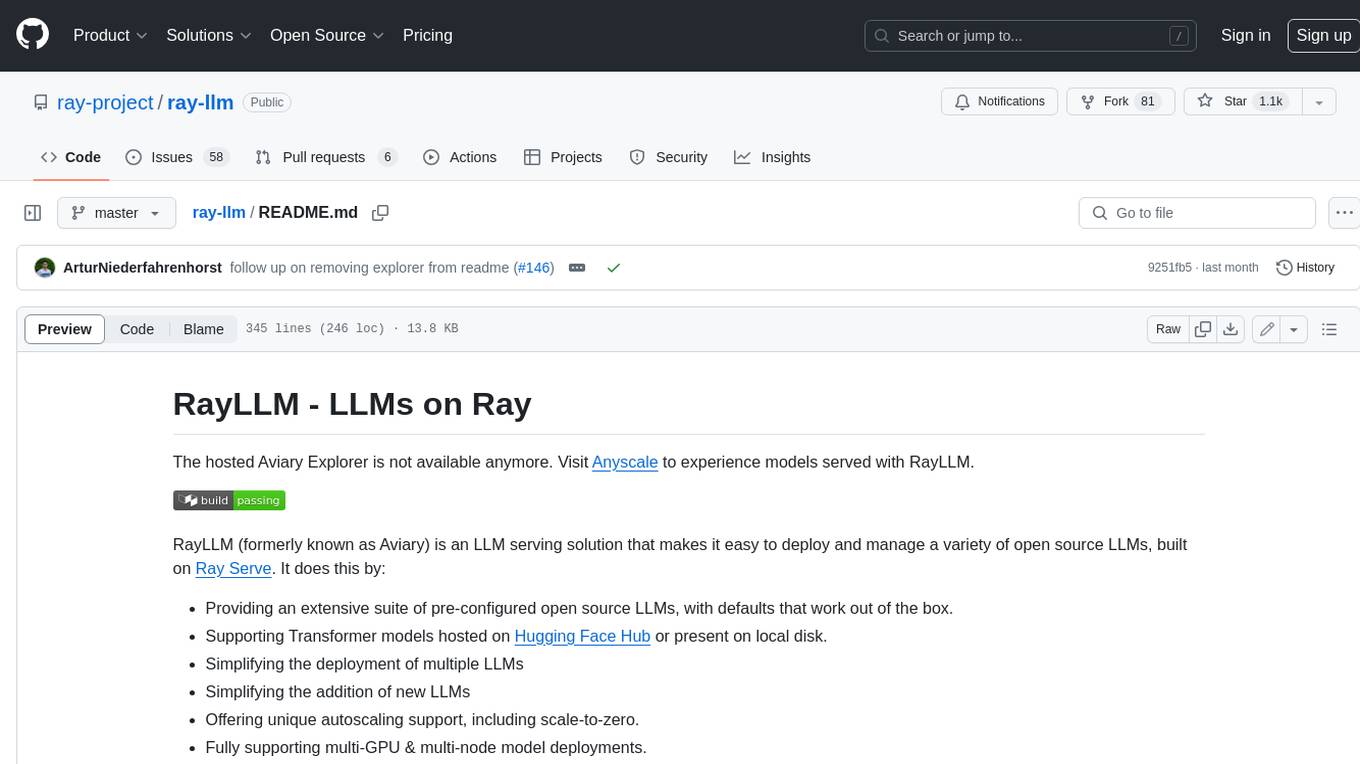
ray-llm
RayLLM (formerly known as Aviary) is an LLM serving solution that makes it easy to deploy and manage a variety of open source LLMs, built on Ray Serve. It provides an extensive suite of pre-configured open source LLMs, with defaults that work out of the box. RayLLM supports Transformer models hosted on Hugging Face Hub or present on local disk. It simplifies the deployment of multiple LLMs, the addition of new LLMs, and offers unique autoscaling support, including scale-to-zero. RayLLM fully supports multi-GPU & multi-node model deployments and offers high performance features like continuous batching, quantization and streaming. It provides a REST API that is similar to OpenAI's to make it easy to migrate and cross test them. RayLLM supports multiple LLM backends out of the box, including vLLM and TensorRT-LLM.
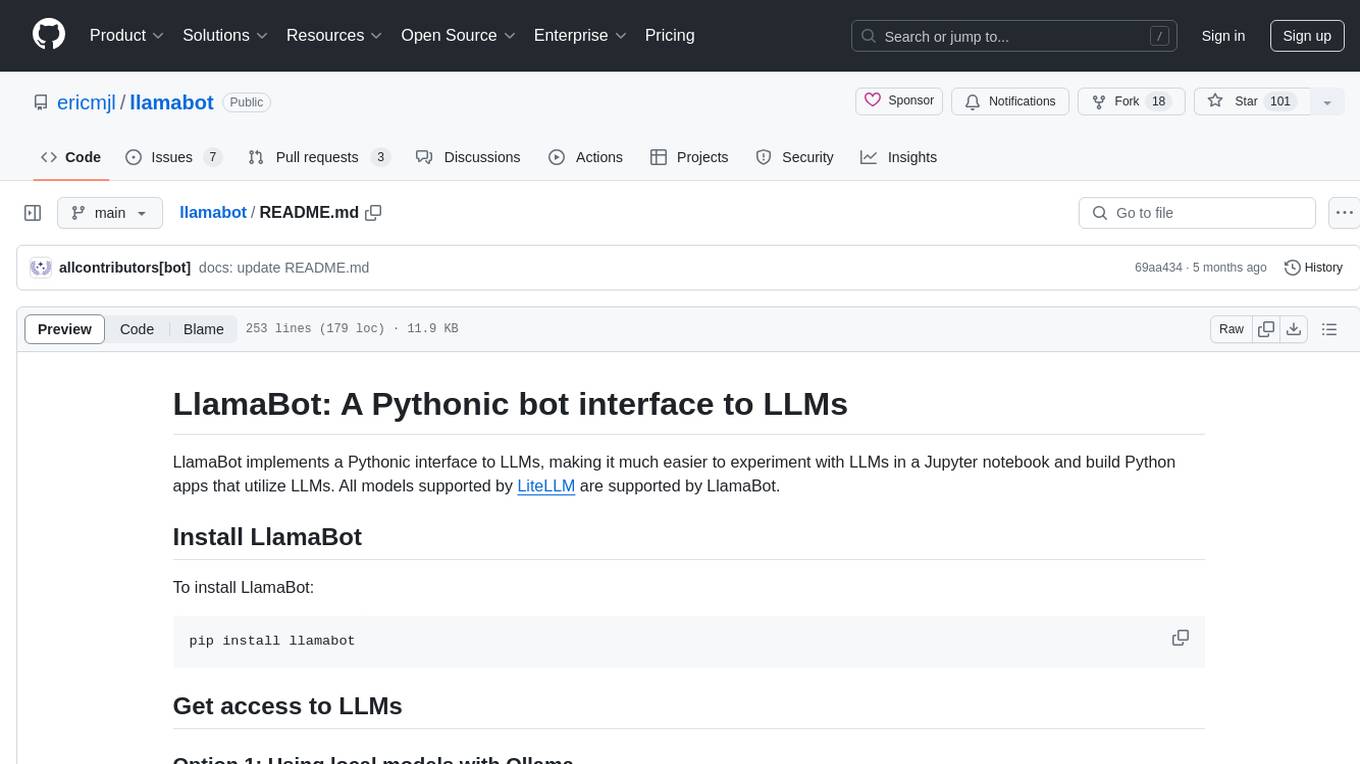
llamabot
LlamaBot is a Pythonic bot interface to Large Language Models (LLMs), providing an easy way to experiment with LLMs in Jupyter notebooks and build Python apps utilizing LLMs. It supports all models available in LiteLLM. Users can access LLMs either through local models with Ollama or by using API providers like OpenAI and Mistral. LlamaBot offers different bot interfaces like SimpleBot, ChatBot, QueryBot, and ImageBot for various tasks such as rephrasing text, maintaining chat history, querying documents, and generating images. The tool also includes CLI demos showcasing its capabilities and supports contributions for new features and bug reports from the community.
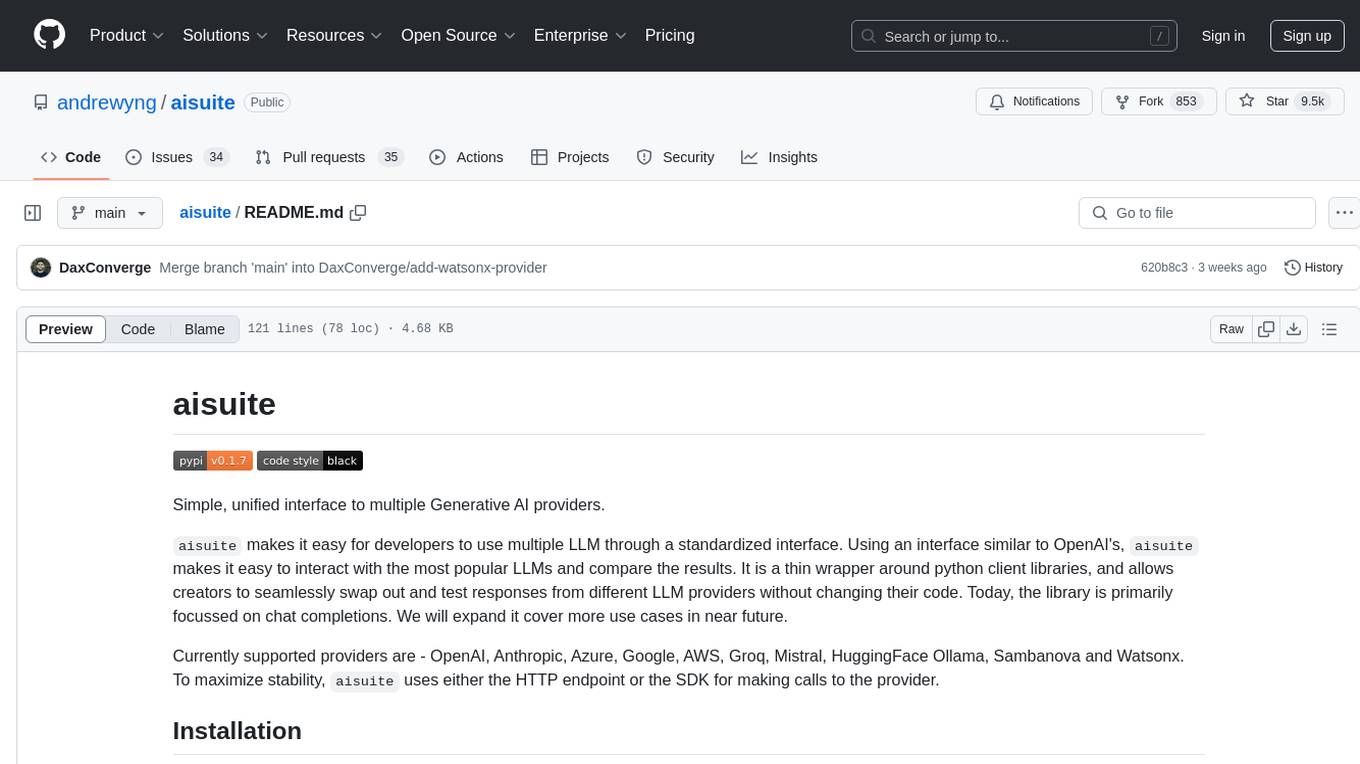
aisuite
Aisuite is a simple, unified interface to multiple Generative AI providers. It allows developers to easily interact with various Language Model (LLM) providers like OpenAI, Anthropic, Azure, Google, AWS, and more through a standardized interface. The library focuses on chat completions and provides a thin wrapper around python client libraries, enabling creators to test responses from different LLM providers without changing their code. Aisuite maximizes stability by using HTTP endpoints or SDKs for making calls to the providers. Users can install the base package or specific provider packages, set up API keys, and utilize the library to generate chat completion responses from different models.
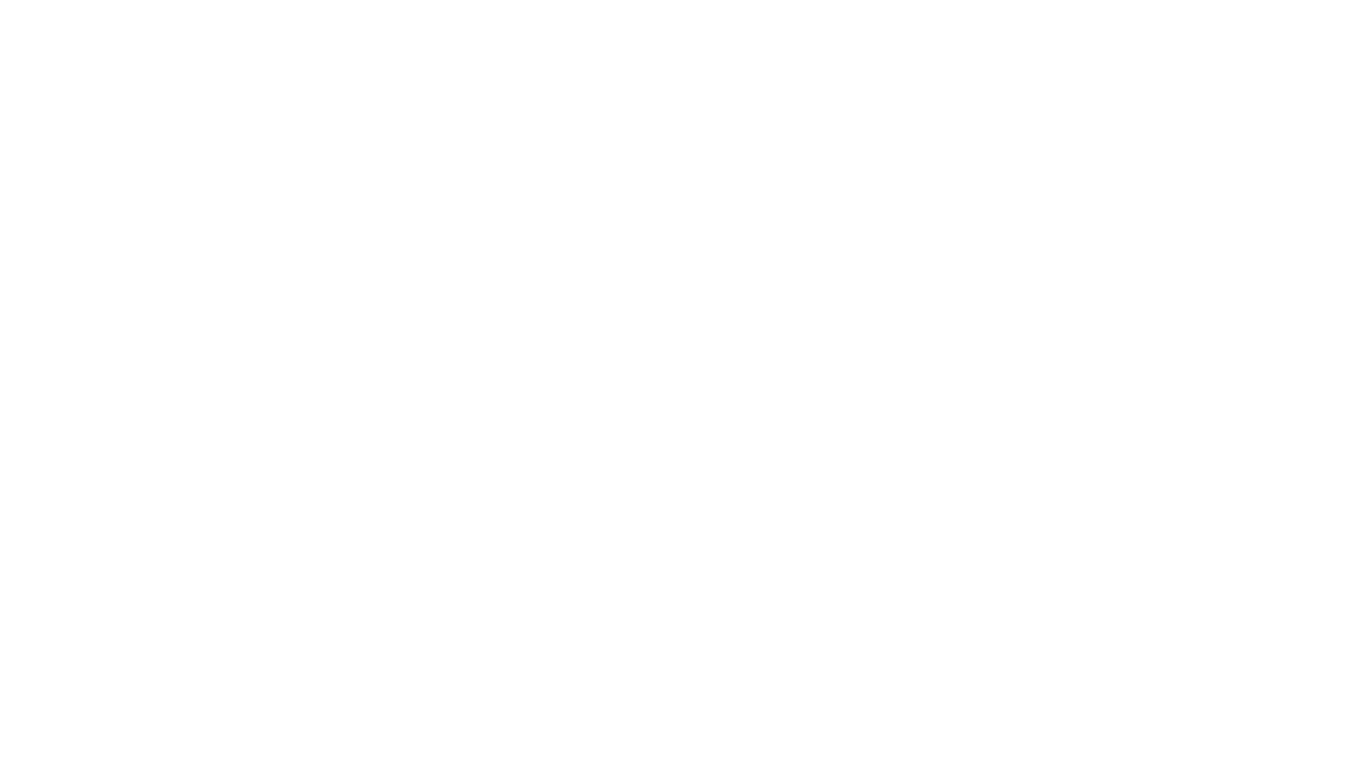
rag-experiment-accelerator
The RAG Experiment Accelerator is a versatile tool that helps you conduct experiments and evaluations using Azure AI Search and RAG pattern. It offers a rich set of features, including experiment setup, integration with Azure AI Search, Azure Machine Learning, MLFlow, and Azure OpenAI, multiple document chunking strategies, query generation, multiple search types, sub-querying, re-ranking, metrics and evaluation, report generation, and multi-lingual support. The tool is designed to make it easier and faster to run experiments and evaluations of search queries and quality of response from OpenAI, and is useful for researchers, data scientists, and developers who want to test the performance of different search and OpenAI related hyperparameters, compare the effectiveness of various search strategies, fine-tune and optimize parameters, find the best combination of hyperparameters, and generate detailed reports and visualizations from experiment results.
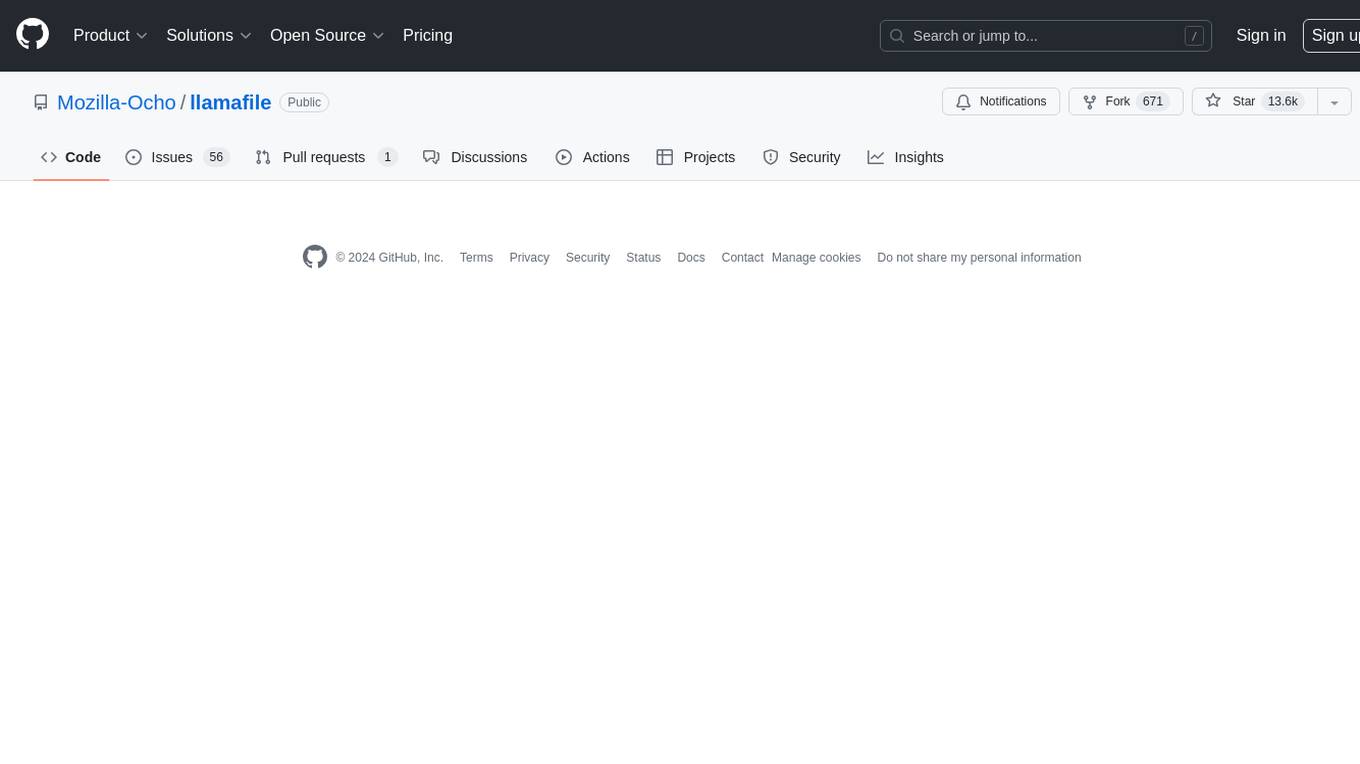
llamafile
llamafile is a tool that enables users to distribute and run Large Language Models (LLMs) with a single file. It combines llama.cpp with Cosmopolitan Libc to create a framework that simplifies the complexity of LLMs into a single-file executable called a 'llamafile'. Users can run these executable files locally on most computers without the need for installation, making open LLMs more accessible to developers and end users. llamafile also provides example llamafiles for various LLM models, allowing users to try out different LLMs locally. The tool supports multiple CPU microarchitectures, CPU architectures, and operating systems, making it versatile and easy to use.
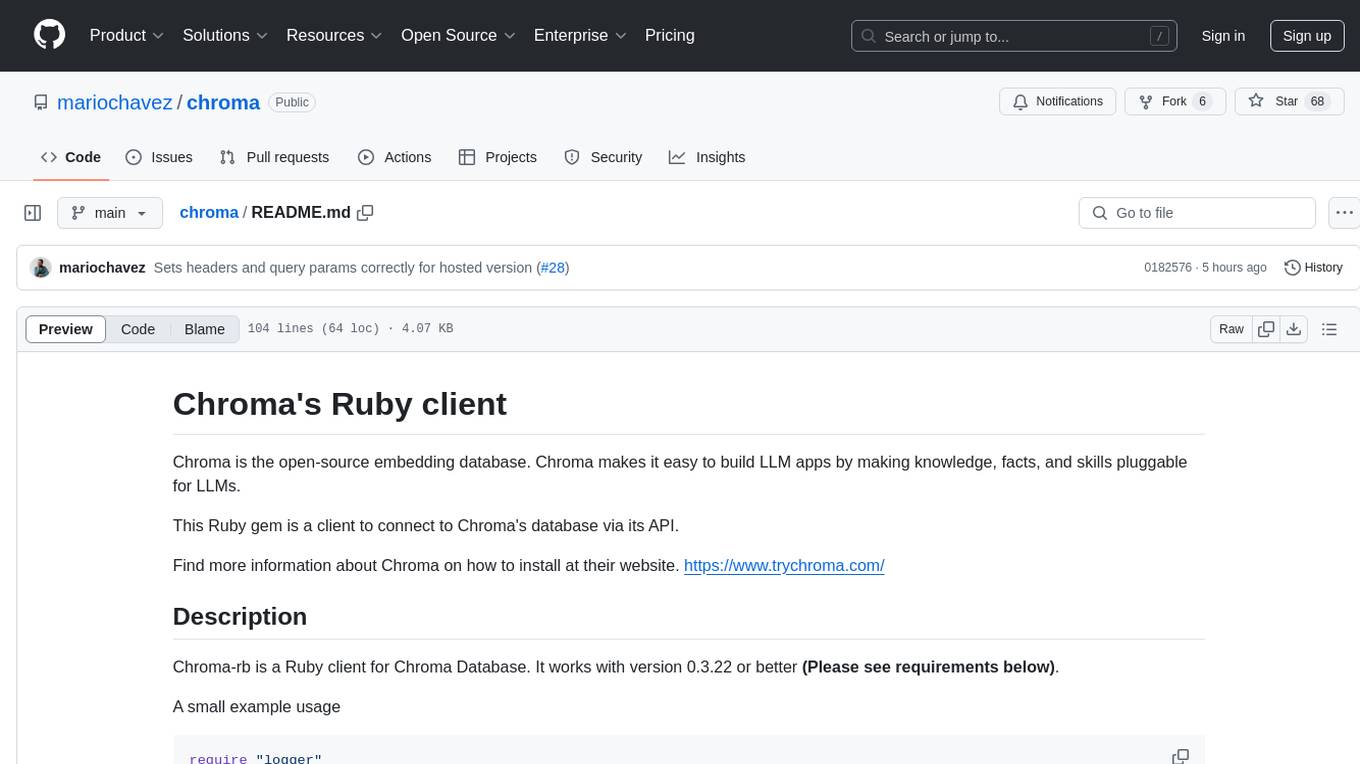
chroma
Chroma is an open-source embedding database that simplifies building LLM apps by enabling the integration of knowledge, facts, and skills for LLMs. The Ruby client for Chroma Database, chroma-rb, facilitates connecting to Chroma's database via its API. Users can configure the host, check server version, create collections, and add embeddings. The gem supports Chroma Database version 0.3.22 or newer, requiring Ruby 3.1.4 or later. It can be used with the hosted Chroma service at trychroma.com by setting configuration options like api_key, tenant, and database. Additionally, the gem provides integration with Jupyter Notebook for creating embeddings using Ollama and Nomic embed text with a Ruby HTTP client.
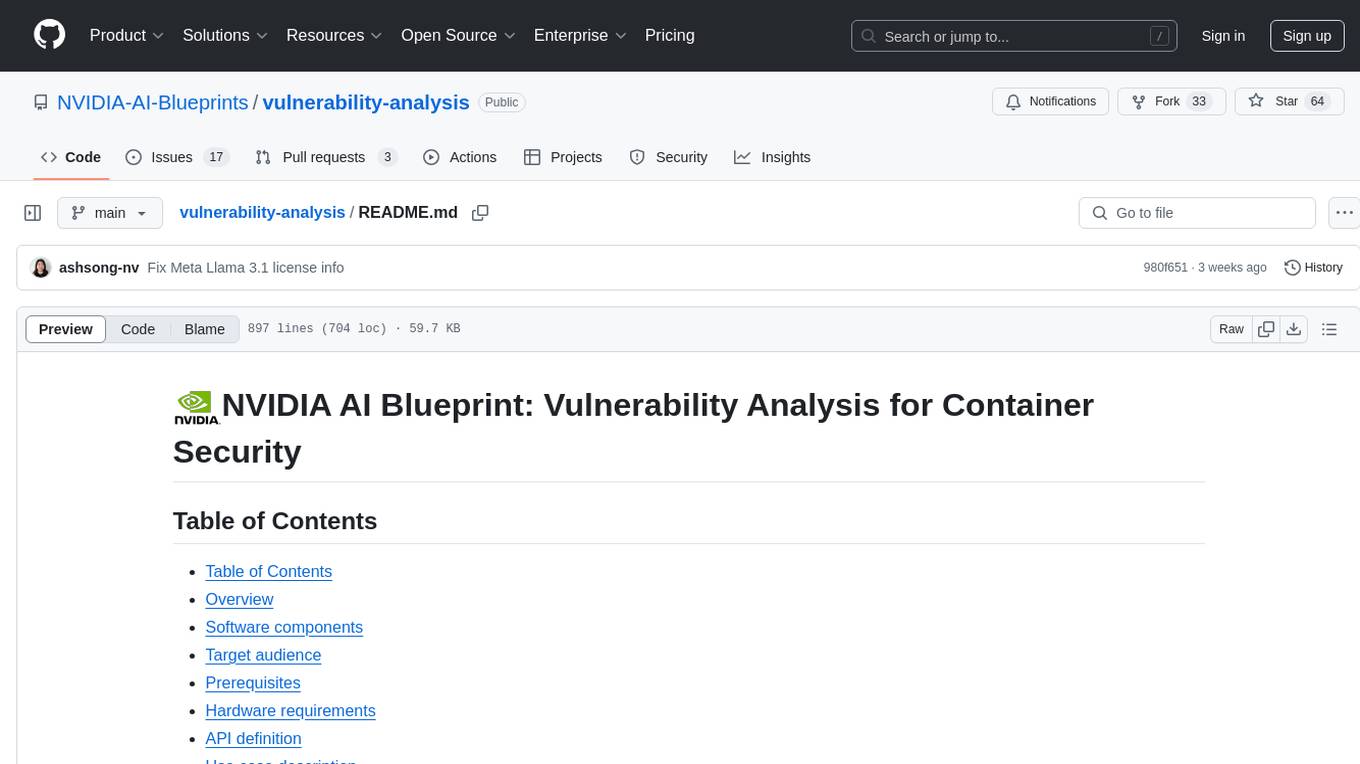
vulnerability-analysis
The NVIDIA AI Blueprint for Vulnerability Analysis for Container Security showcases accelerated analysis on common vulnerabilities and exposures (CVE) at an enterprise scale, reducing mitigation time from days to seconds. It enables security analysts to determine software package vulnerabilities using large language models (LLMs) and retrieval-augmented generation (RAG). The blueprint is designed for security analysts, IT engineers, and AI practitioners in cybersecurity. It requires NVAIE developer license and API keys for vulnerability databases, search engines, and LLM model services. Hardware requirements include L40 GPU for pipeline operation and optional LLM NIM and Embedding NIM. The workflow involves LLM pipeline for CVE impact analysis, utilizing LLM planner, agent, and summarization nodes. The blueprint uses NVIDIA NIM microservices and Morpheus Cybersecurity AI SDK for vulnerability analysis.
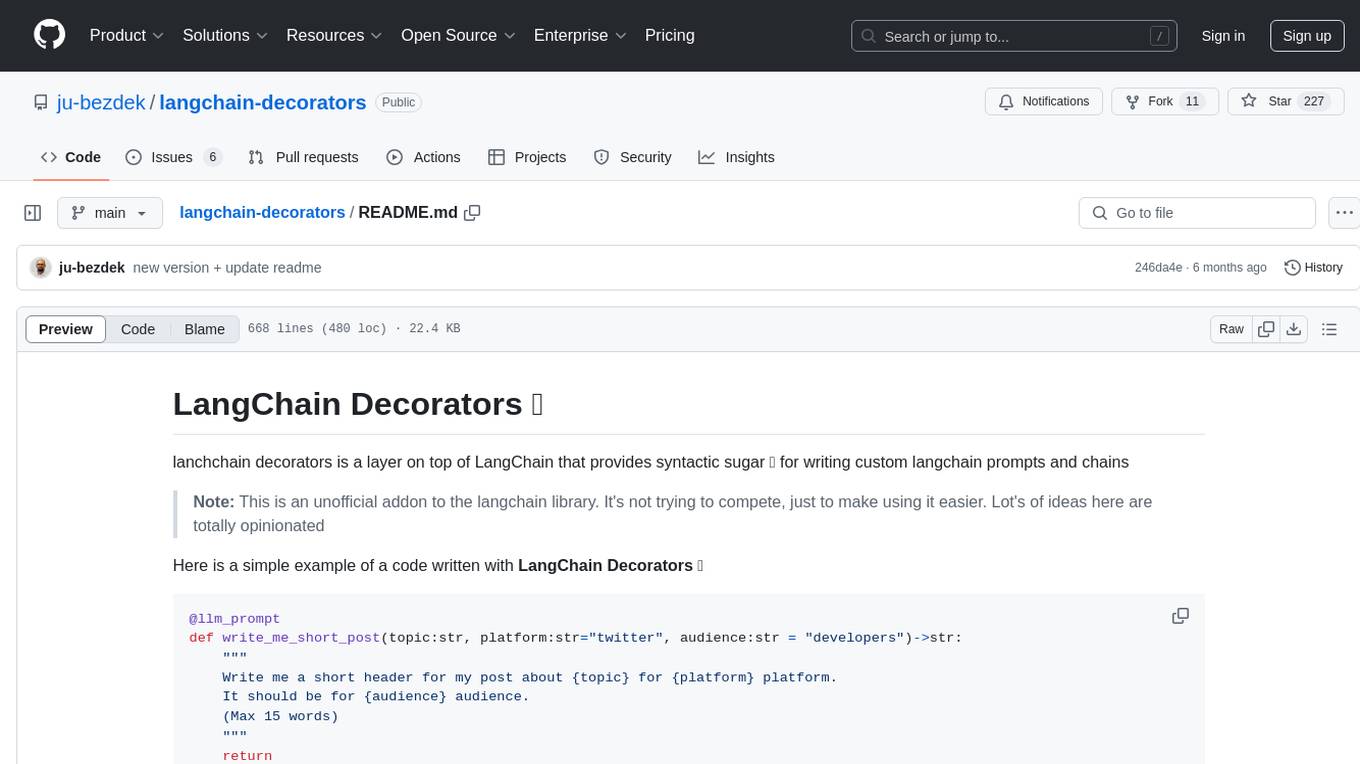
langchain-decorators
LangChain Decorators is a layer on top of LangChain that provides syntactic sugar for writing custom langchain prompts and chains. It offers a more pythonic way of writing code, multiline prompts without breaking code flow, IDE support for hinting and type checking, leveraging LangChain ecosystem, support for optional parameters, and sharing parameters between prompts. It simplifies streaming, automatic LLM selection, defining custom settings, debugging, and passing memory, callback, stop, etc. It also provides functions provider, dynamic function schemas, binding prompts to objects, defining custom settings, and debugging options. The project aims to enhance the LangChain library by making it easier to use and more efficient for writing custom prompts and chains.
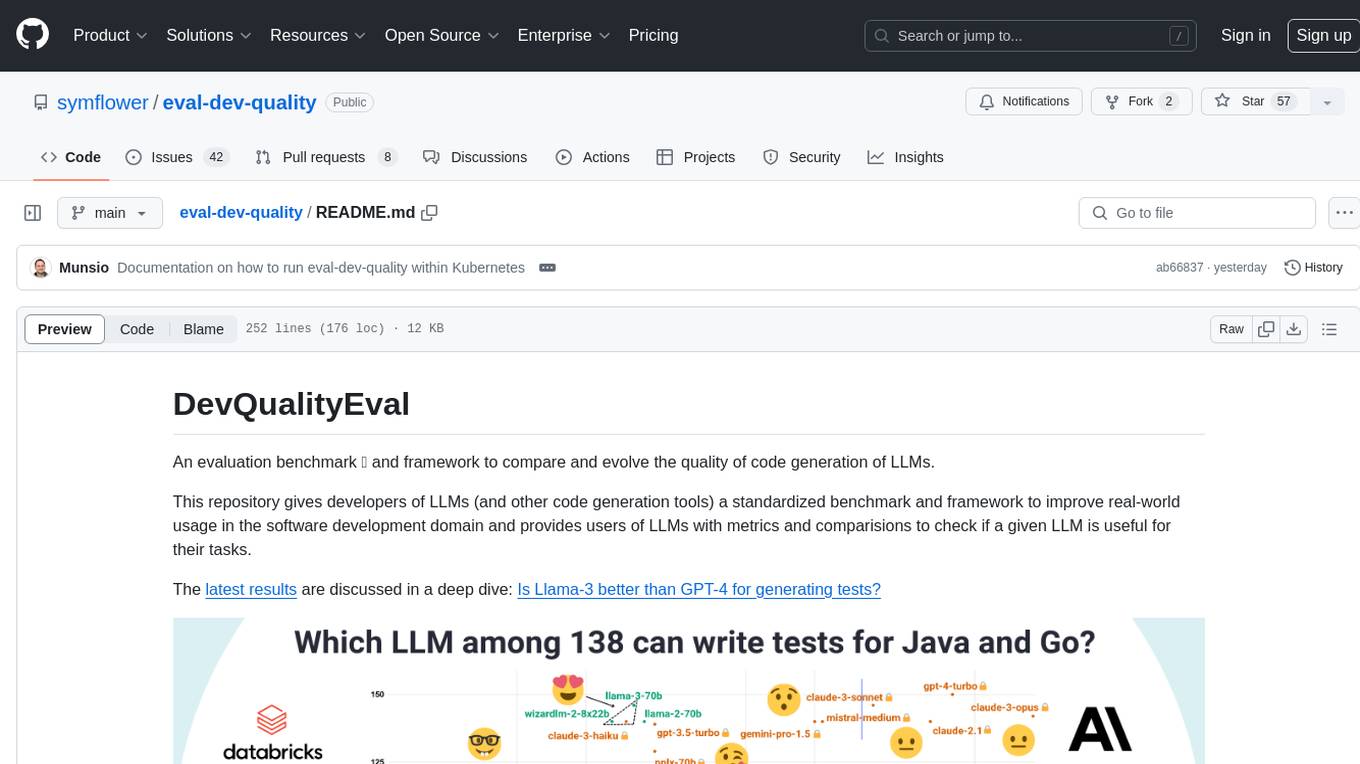
eval-dev-quality
DevQualityEval is an evaluation benchmark and framework designed to compare and improve the quality of code generation of Language Model Models (LLMs). It provides developers with a standardized benchmark to enhance real-world usage in software development and offers users metrics and comparisons to assess the usefulness of LLMs for their tasks. The tool evaluates LLMs' performance in solving software development tasks and measures the quality of their results through a point-based system. Users can run specific tasks, such as test generation, across different programming languages to evaluate LLMs' language understanding and code generation capabilities.
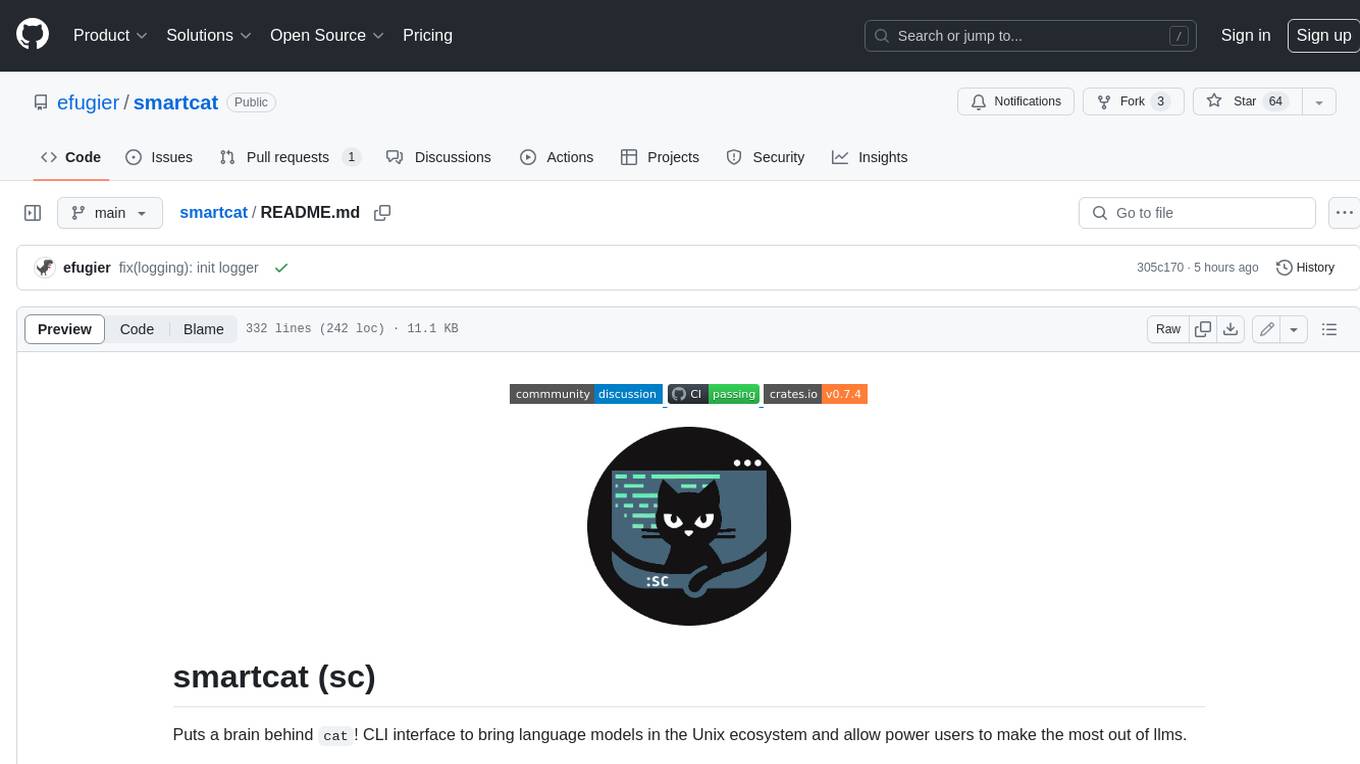
smartcat
Smartcat is a CLI interface that brings language models into the Unix ecosystem, allowing power users to leverage the capabilities of LLMs in their daily workflows. It features a minimalist design, seamless integration with terminal and editor workflows, and customizable prompts for specific tasks. Smartcat currently supports OpenAI, Mistral AI, and Anthropic APIs, providing access to a range of language models. With its ability to manipulate file and text streams, integrate with editors, and offer configurable settings, Smartcat empowers users to automate tasks, enhance code quality, and explore creative possibilities.
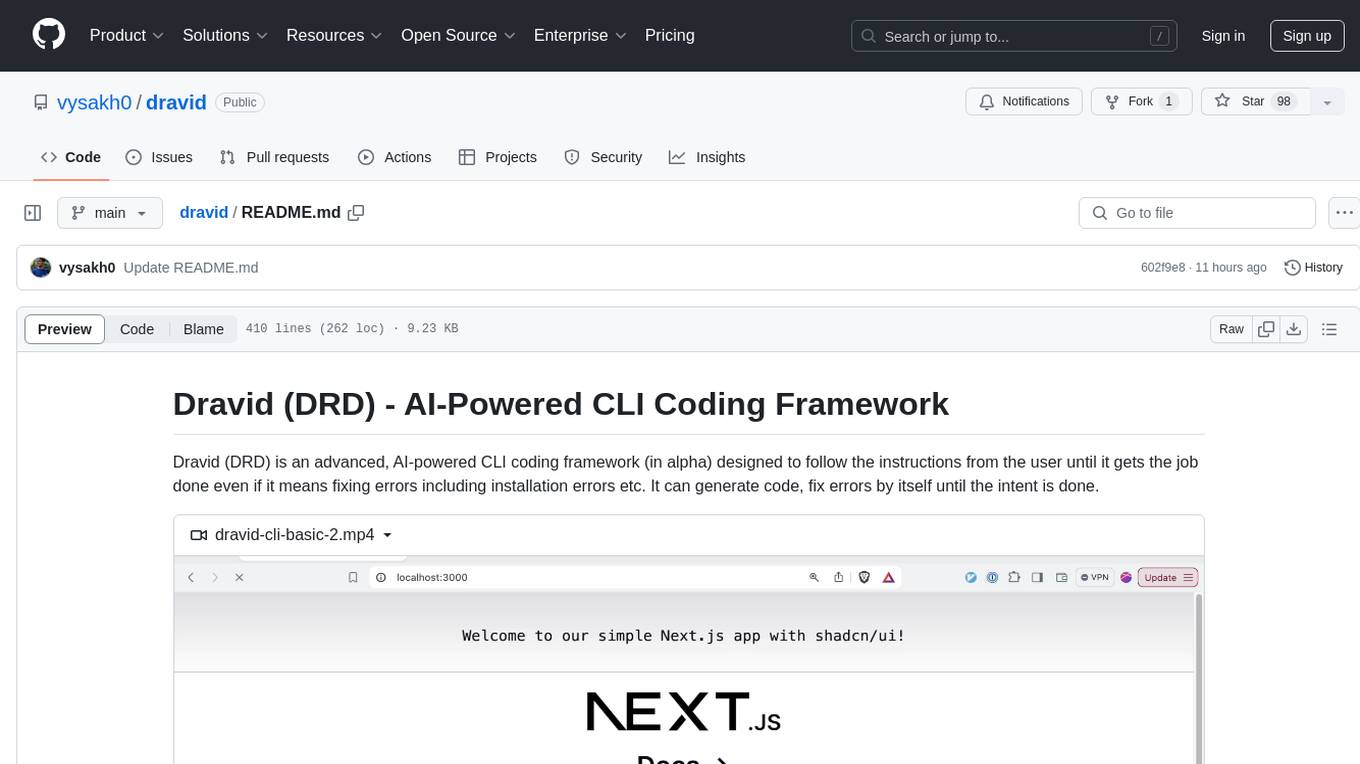
dravid
Dravid (DRD) is an advanced, AI-powered CLI coding framework designed to follow user instructions until the job is completed, including fixing errors. It can generate code, fix errors, handle image queries, manage file operations, integrate with external APIs, and provide a development server with error handling. Dravid is extensible and requires Python 3.7+ and CLAUDE_API_KEY. Users can interact with Dravid through CLI commands for various tasks like creating projects, asking questions, generating content, handling metadata, and file-specific queries. It supports use cases like Next.js project development, working with existing projects, exploring new languages, Ruby on Rails project development, and Python project development. Dravid's project structure includes directories for source code, CLI modules, API interaction, utility functions, AI prompt templates, metadata management, and tests. Contributions are welcome, and development setup involves cloning the repository, installing dependencies with Poetry, setting up environment variables, and using Dravid for project enhancements.
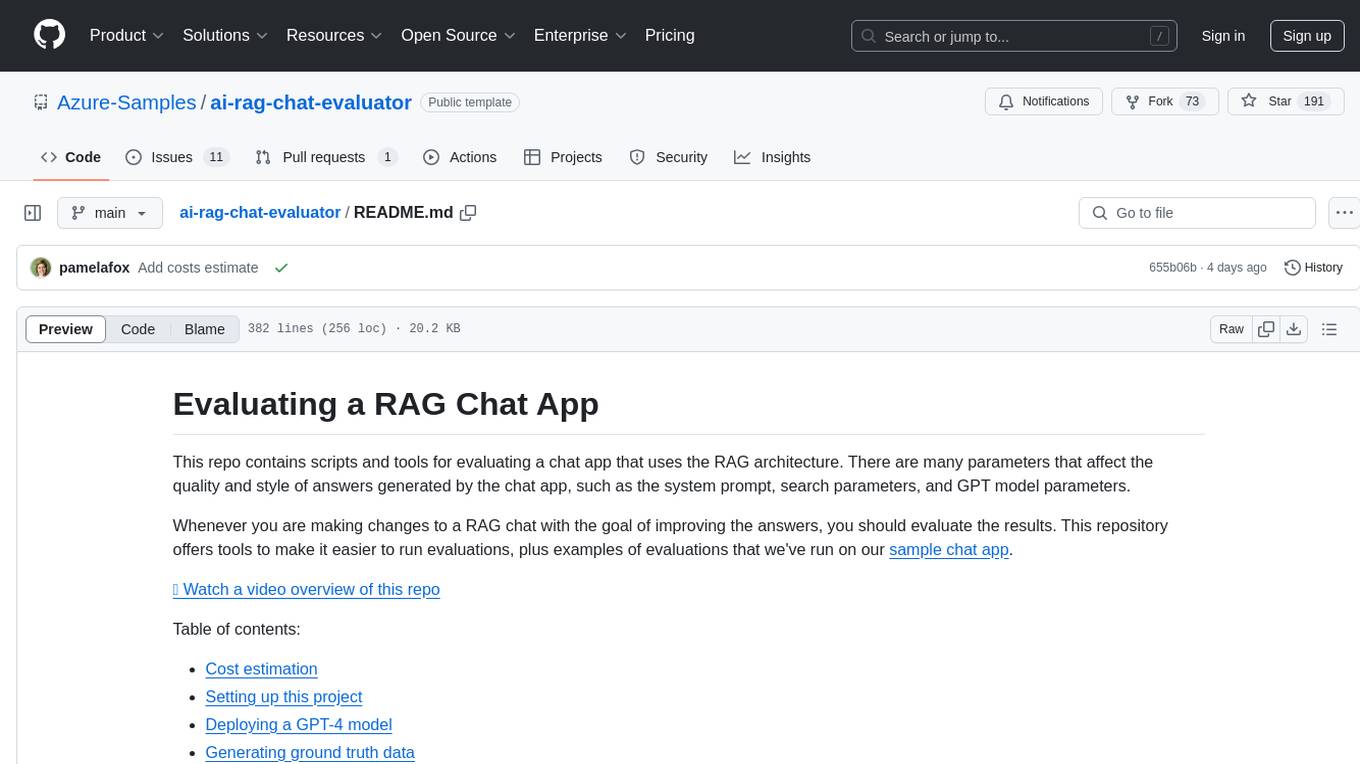
ai-rag-chat-evaluator
This repository contains scripts and tools for evaluating a chat app that uses the RAG architecture. It provides parameters to assess the quality and style of answers generated by the chat app, including system prompt, search parameters, and GPT model parameters. The tools facilitate running evaluations, with examples of evaluations on a sample chat app. The repo also offers guidance on cost estimation, setting up the project, deploying a GPT-4 model, generating ground truth data, running evaluations, and measuring the app's ability to say 'I don't know'. Users can customize evaluations, view results, and compare runs using provided tools.
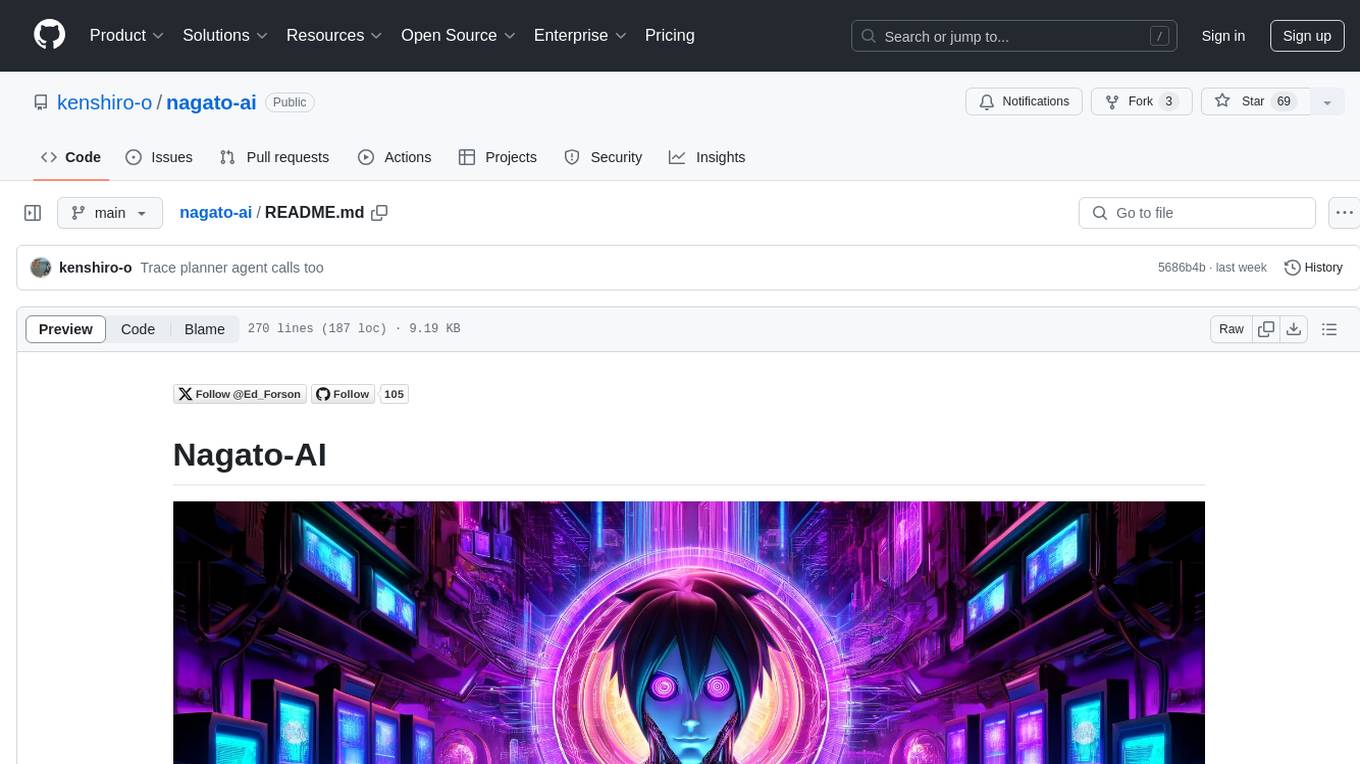
nagato-ai
Nagato-AI is an intuitive AI Agent library that supports multiple LLMs including OpenAI's GPT, Anthropic's Claude, Google's Gemini, and Groq LLMs. Users can create agents from these models and combine them to build an effective AI Agent system. The library is named after the powerful ninja Nagato from the anime Naruto, who can control multiple bodies with different abilities. Nagato-AI acts as a linchpin to summon and coordinate AI Agents for specific missions. It provides flexibility in programming and supports tools like Coordinator, Researcher, Critic agents, and HumanConfirmInputTool.
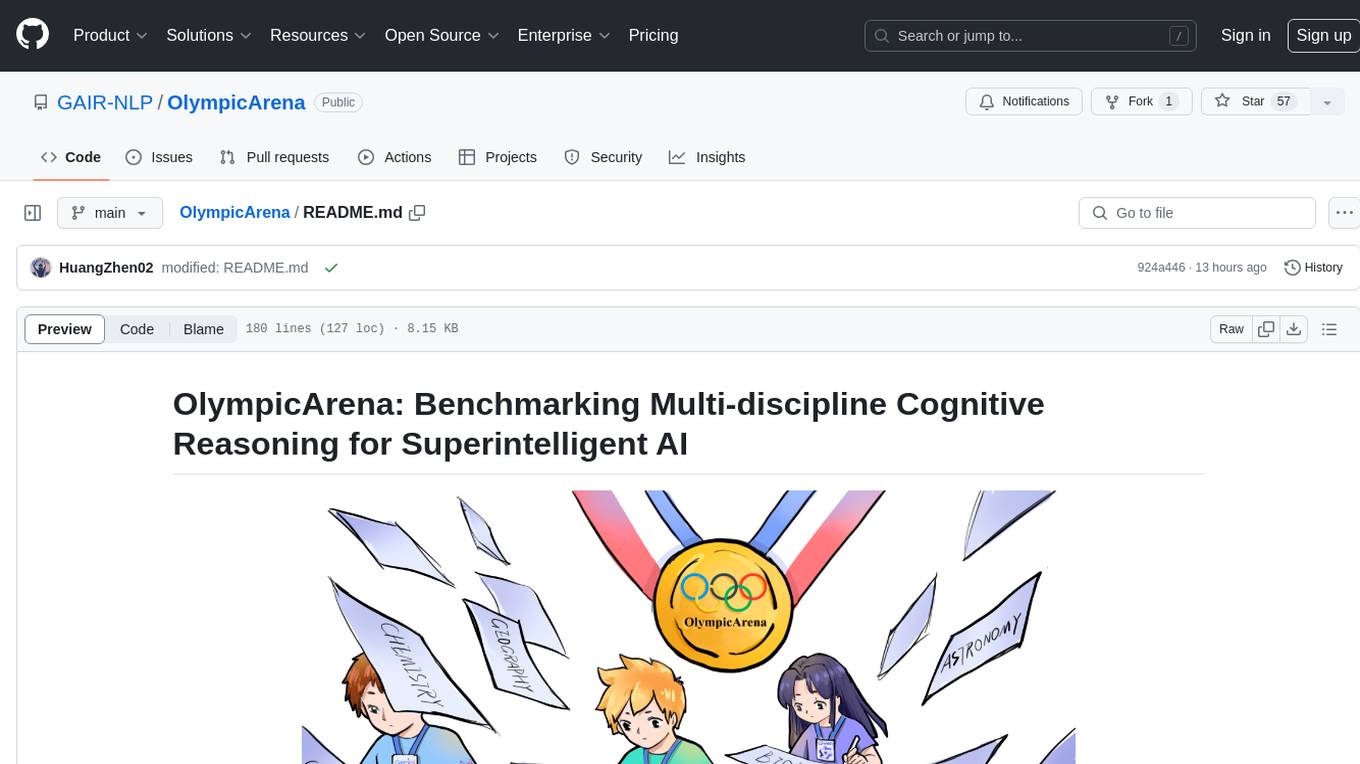
OlympicArena
OlympicArena is a comprehensive benchmark designed to evaluate advanced AI capabilities across various disciplines. It aims to push AI towards superintelligence by tackling complex challenges in science and beyond. The repository provides detailed data for different disciplines, allows users to run inference and evaluation locally, and offers a submission platform for testing models on the test set. Additionally, it includes an annotation interface and encourages users to cite their paper if they find the code or dataset helpful.
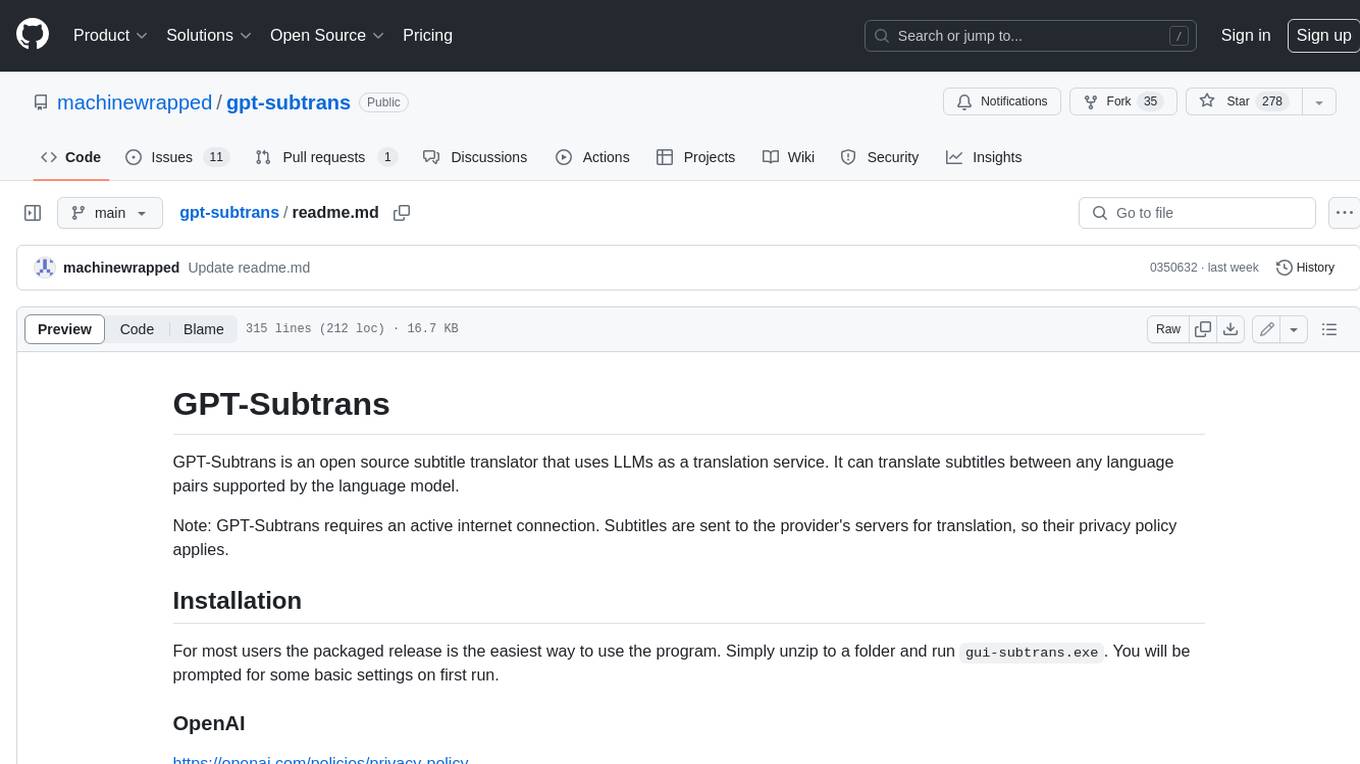
gpt-subtrans
GPT-Subtrans is an open-source subtitle translator that utilizes large language models (LLMs) as translation services. It supports translation between any language pairs that the language model supports. Note that GPT-Subtrans requires an active internet connection, as subtitles are sent to the provider's servers for translation, and their privacy policy applies.
For similar tasks
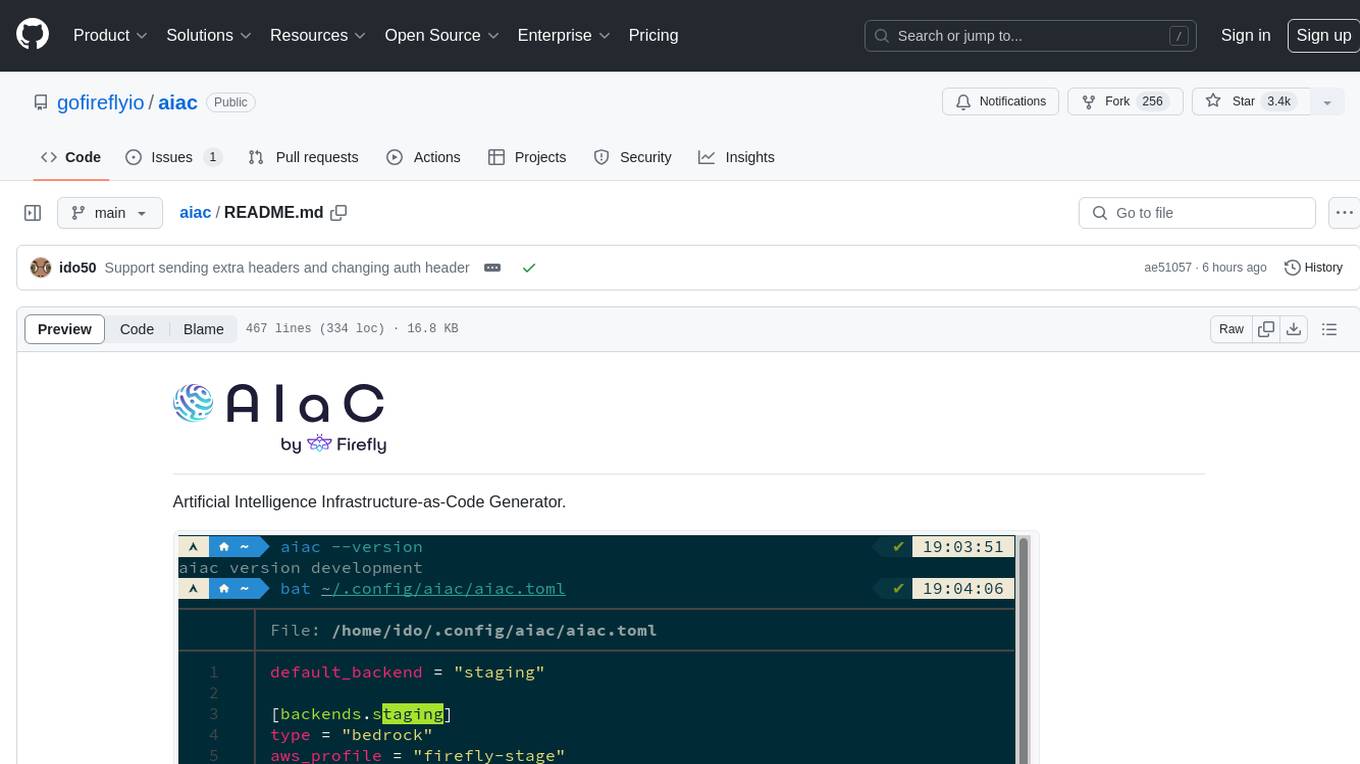
aiac
AIAC is a library and command line tool to generate Infrastructure as Code (IaC) templates, configurations, utilities, queries, and more via LLM providers such as OpenAI, Amazon Bedrock, and Ollama. Users can define multiple 'backends' targeting different LLM providers and environments using a simple configuration file. The tool allows users to ask a model to generate templates for different scenarios and composes an appropriate request to the selected provider, storing the resulting code to a file and/or printing it to standard output.
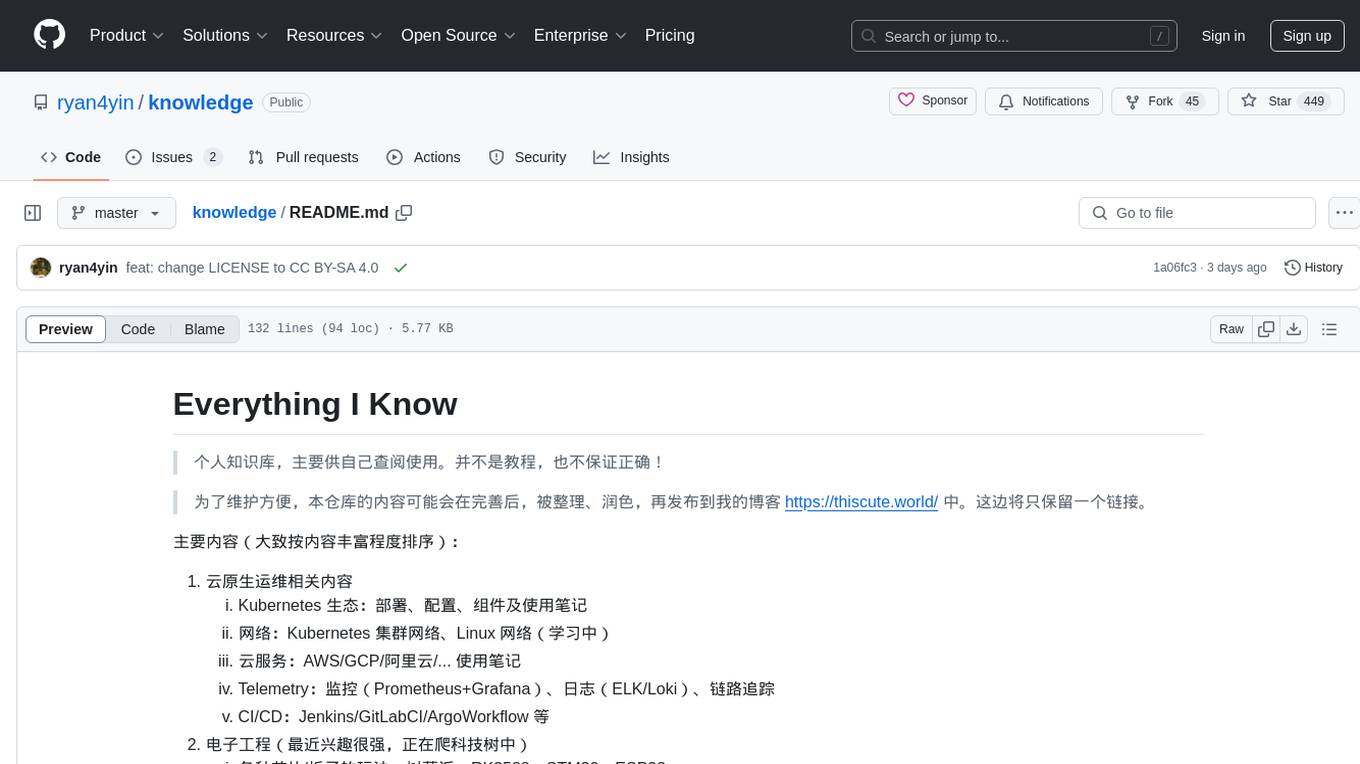
knowledge
This repository serves as a personal knowledge base for the owner's reference and use. It covers a wide range of topics including cloud-native operations, Kubernetes ecosystem, networking, cloud services, telemetry, CI/CD, electronic engineering, hardware projects, operating systems, homelab setups, high-performance computing applications, openwrt router usage, programming languages, music theory, blockchain, distributed systems principles, and various other knowledge domains. The content is periodically refined and published on the owner's blog for maintenance purposes.
For similar jobs
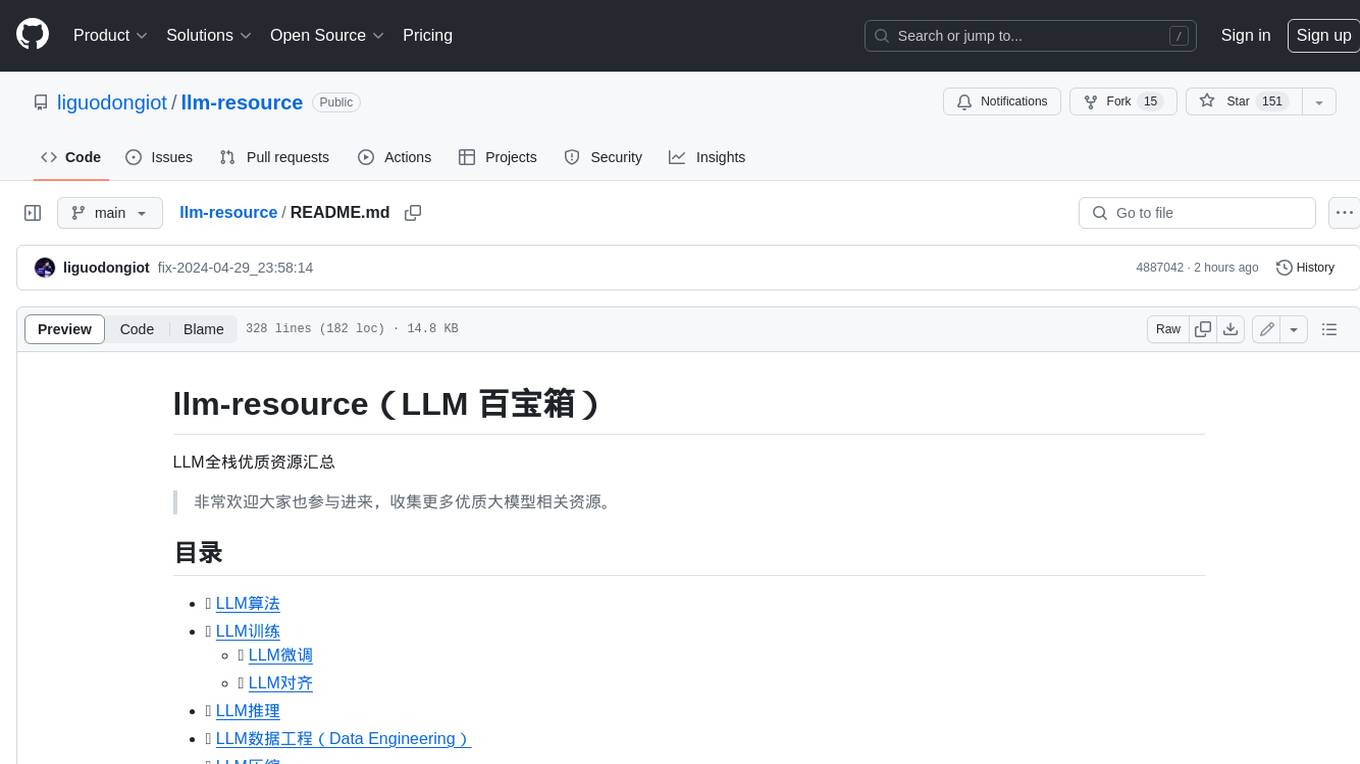
llm-resource
llm-resource is a comprehensive collection of high-quality resources for Large Language Models (LLM). It covers various aspects of LLM including algorithms, training, fine-tuning, alignment, inference, data engineering, compression, evaluation, prompt engineering, AI frameworks, AI basics, AI infrastructure, AI compilers, LLM application development, LLM operations, AI systems, and practical implementations. The repository aims to gather and share valuable resources related to LLM for the community to benefit from.
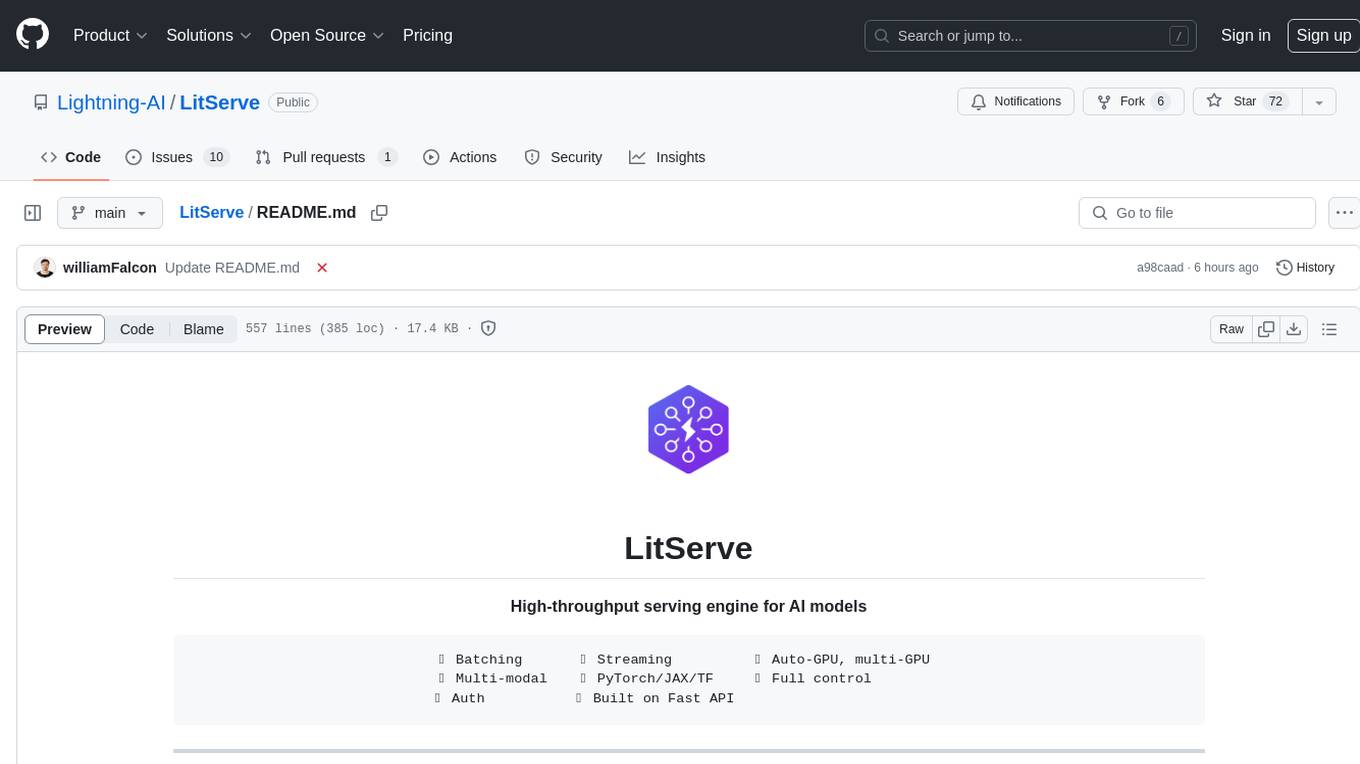
LitServe
LitServe is a high-throughput serving engine designed for deploying AI models at scale. It generates an API endpoint for models, handles batching, streaming, and autoscaling across CPU/GPUs. LitServe is built for enterprise scale with a focus on minimal, hackable code-base without bloat. It supports various model types like LLMs, vision, time-series, and works with frameworks like PyTorch, JAX, Tensorflow, and more. The tool allows users to focus on model performance rather than serving boilerplate, providing full control and flexibility.
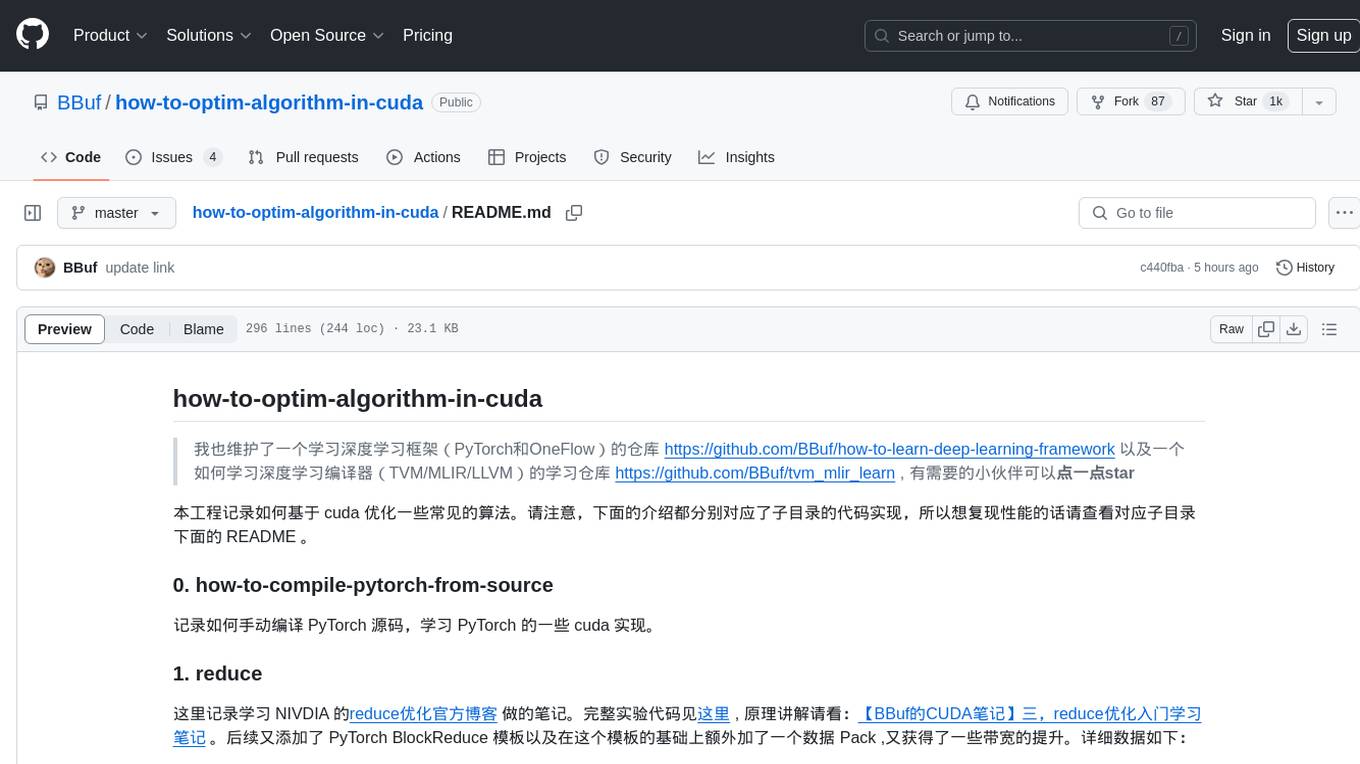
how-to-optim-algorithm-in-cuda
This repository documents how to optimize common algorithms based on CUDA. It includes subdirectories with code implementations for specific optimizations. The optimizations cover topics such as compiling PyTorch from source, NVIDIA's reduce optimization, OneFlow's elementwise template, fast atomic add for half data types, upsample nearest2d optimization in OneFlow, optimized indexing in PyTorch, OneFlow's softmax kernel, linear attention optimization, and more. The repository also includes learning resources related to deep learning frameworks, compilers, and optimization techniques.
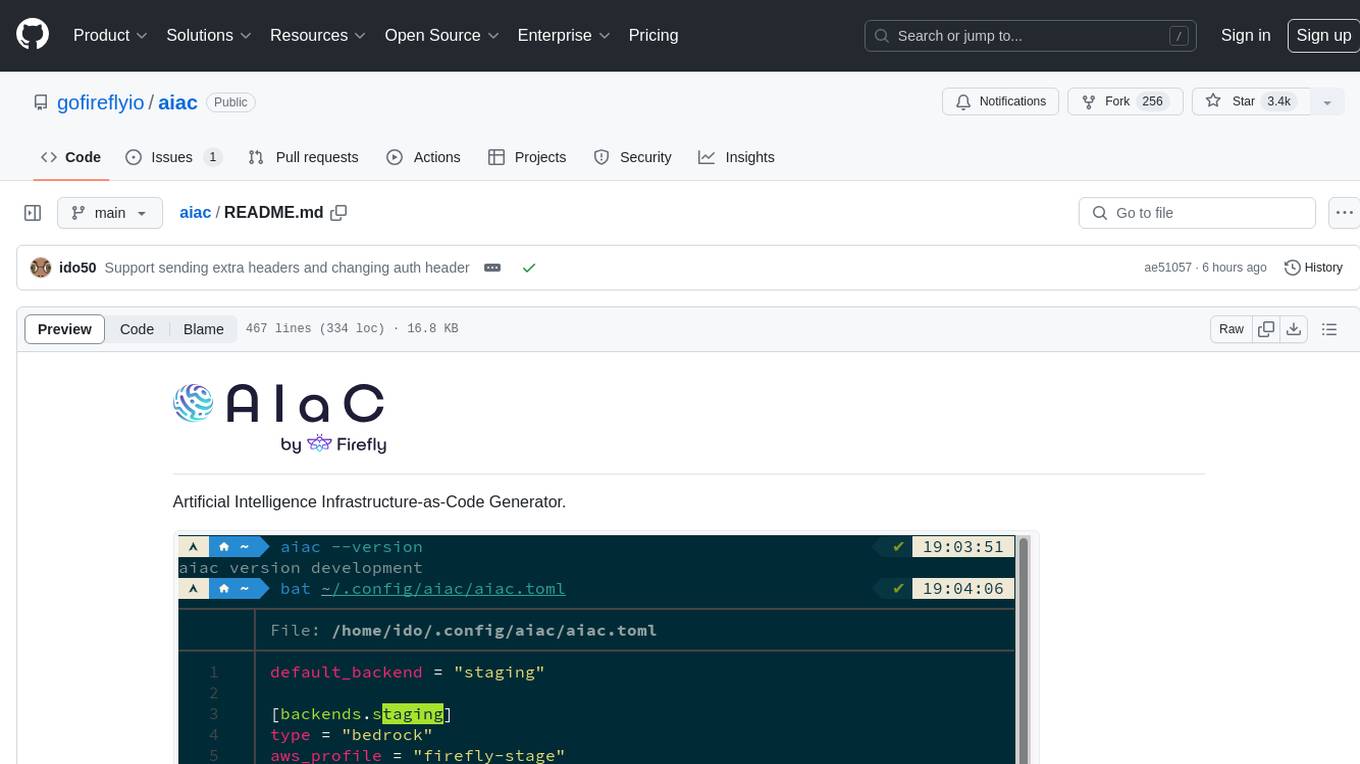
aiac
AIAC is a library and command line tool to generate Infrastructure as Code (IaC) templates, configurations, utilities, queries, and more via LLM providers such as OpenAI, Amazon Bedrock, and Ollama. Users can define multiple 'backends' targeting different LLM providers and environments using a simple configuration file. The tool allows users to ask a model to generate templates for different scenarios and composes an appropriate request to the selected provider, storing the resulting code to a file and/or printing it to standard output.
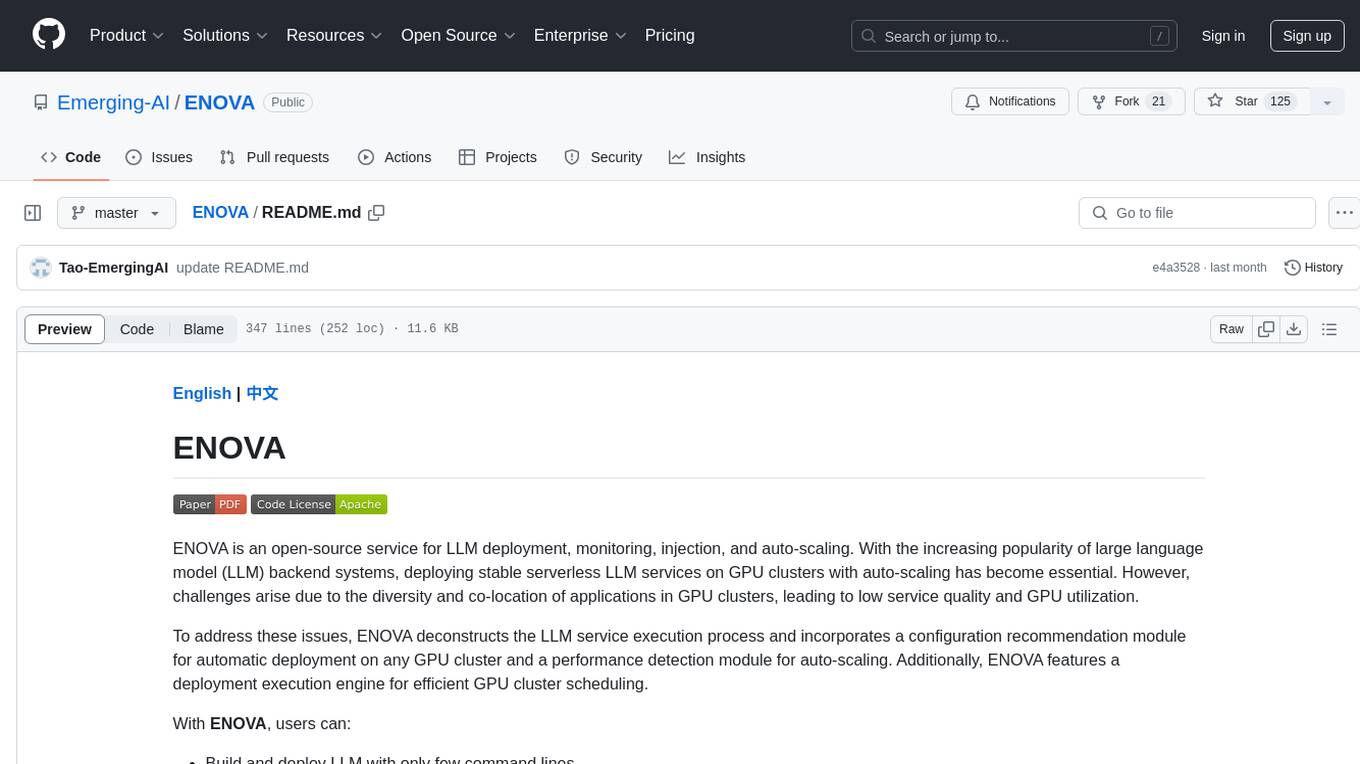
ENOVA
ENOVA is an open-source service for Large Language Model (LLM) deployment, monitoring, injection, and auto-scaling. It addresses challenges in deploying stable serverless LLM services on GPU clusters with auto-scaling by deconstructing the LLM service execution process and providing configuration recommendations and performance detection. Users can build and deploy LLM with few command lines, recommend optimal computing resources, experience LLM performance, observe operating status, achieve load balancing, and more. ENOVA ensures stable operation, cost-effectiveness, efficiency, and strong scalability of LLM services.
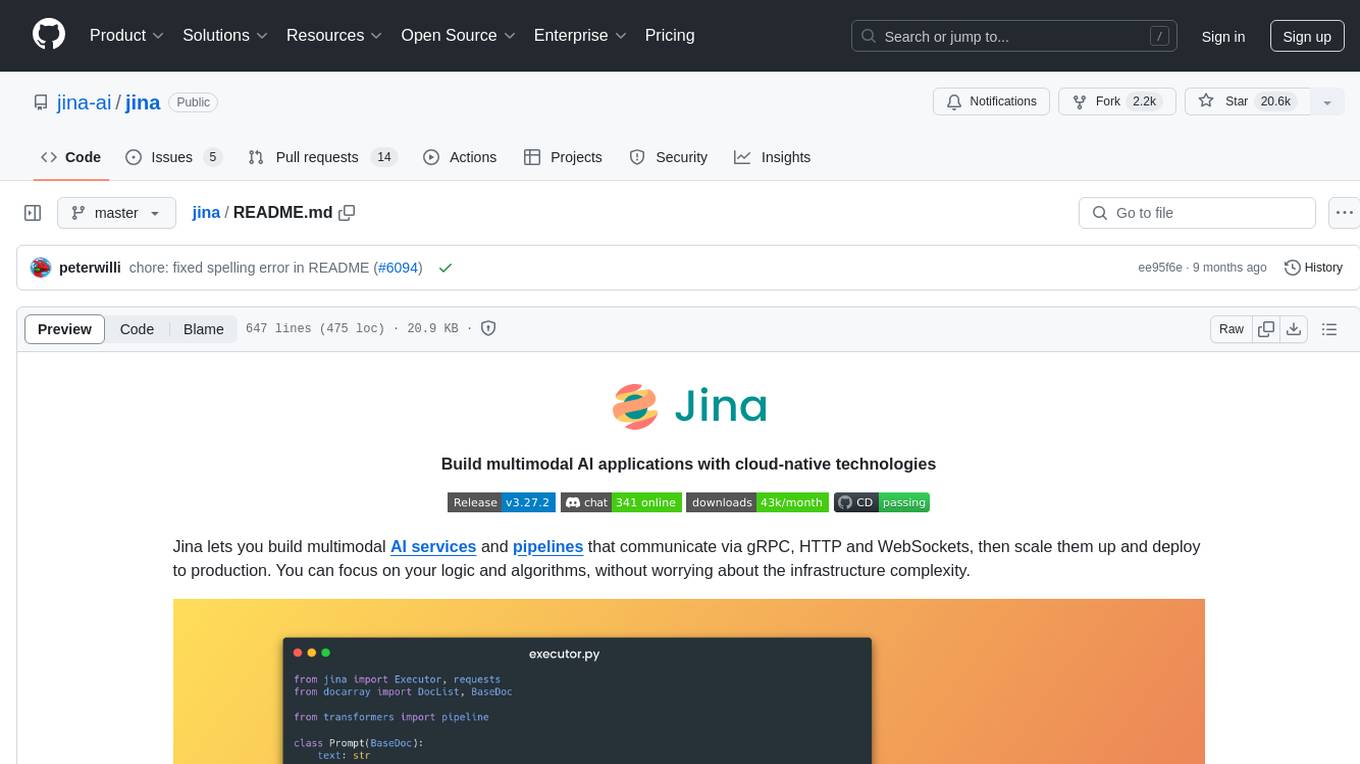
jina
Jina is a tool that allows users to build multimodal AI services and pipelines using cloud-native technologies. It provides a Pythonic experience for serving ML models and transitioning from local deployment to advanced orchestration frameworks like Docker-Compose, Kubernetes, or Jina AI Cloud. Users can build and serve models for any data type and deep learning framework, design high-performance services with easy scaling, serve LLM models while streaming their output, integrate with Docker containers via Executor Hub, and host on CPU/GPU using Jina AI Cloud. Jina also offers advanced orchestration and scaling capabilities, a smooth transition to the cloud, and easy scalability and concurrency features for applications. Users can deploy to their own cloud or system with Kubernetes and Docker Compose integration, and even deploy to JCloud for autoscaling and monitoring.
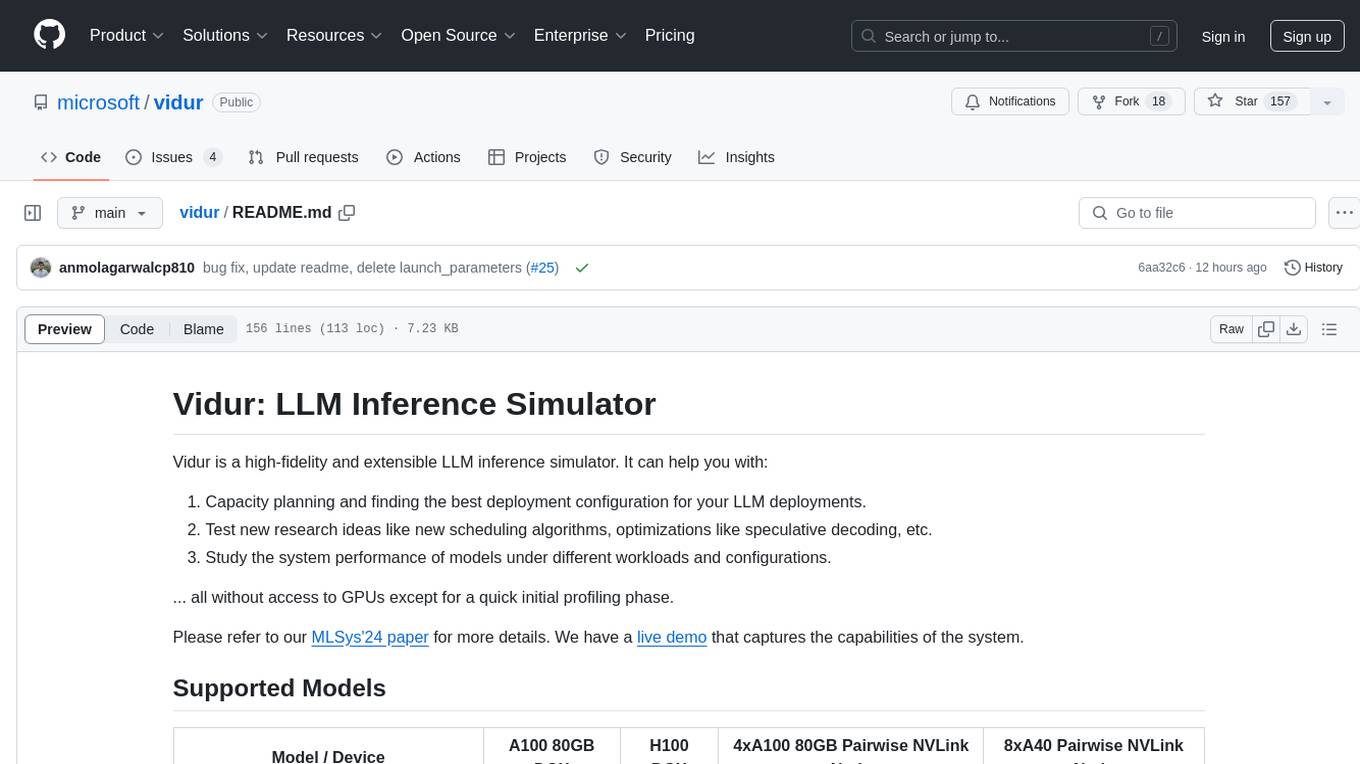
vidur
Vidur is a high-fidelity and extensible LLM inference simulator designed for capacity planning, deployment configuration optimization, testing new research ideas, and studying system performance of models under different workloads and configurations. It supports various models and devices, offers chrome trace exports, and can be set up using mamba, venv, or conda. Users can run the simulator with various parameters and monitor metrics using wandb. Contributions are welcome, subject to a Contributor License Agreement and adherence to the Microsoft Open Source Code of Conduct.
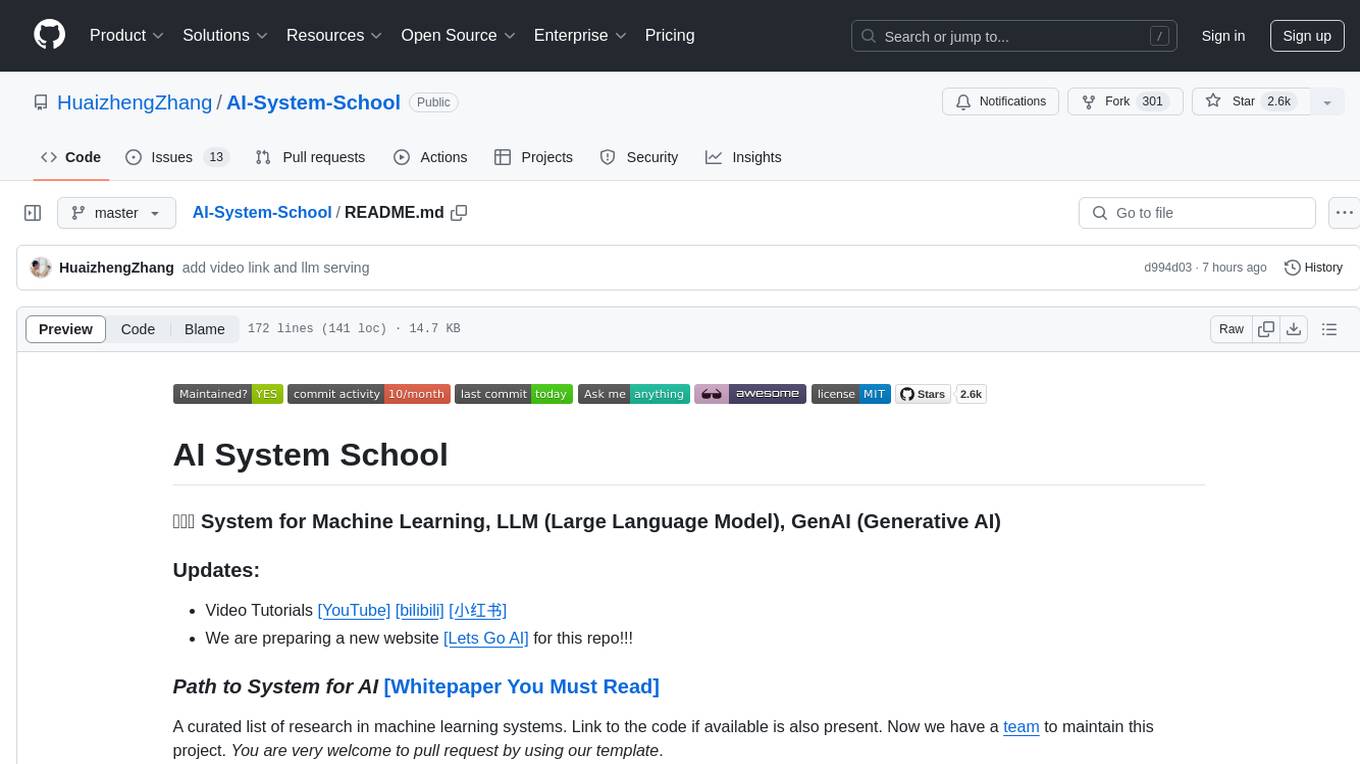
AI-System-School
AI System School is a curated list of research in machine learning systems, focusing on ML/DL infra, LLM infra, domain-specific infra, ML/LLM conferences, and general resources. It provides resources such as data processing, training systems, video systems, autoML systems, and more. The repository aims to help users navigate the landscape of AI systems and machine learning infrastructure, offering insights into conferences, surveys, books, videos, courses, and blogs related to the field.