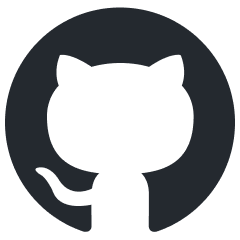
eval-dev-quality
DevQualityEval: An evaluation benchmark 📈 and framework to compare and evolve the quality of code generation of LLMs.
Stars: 159
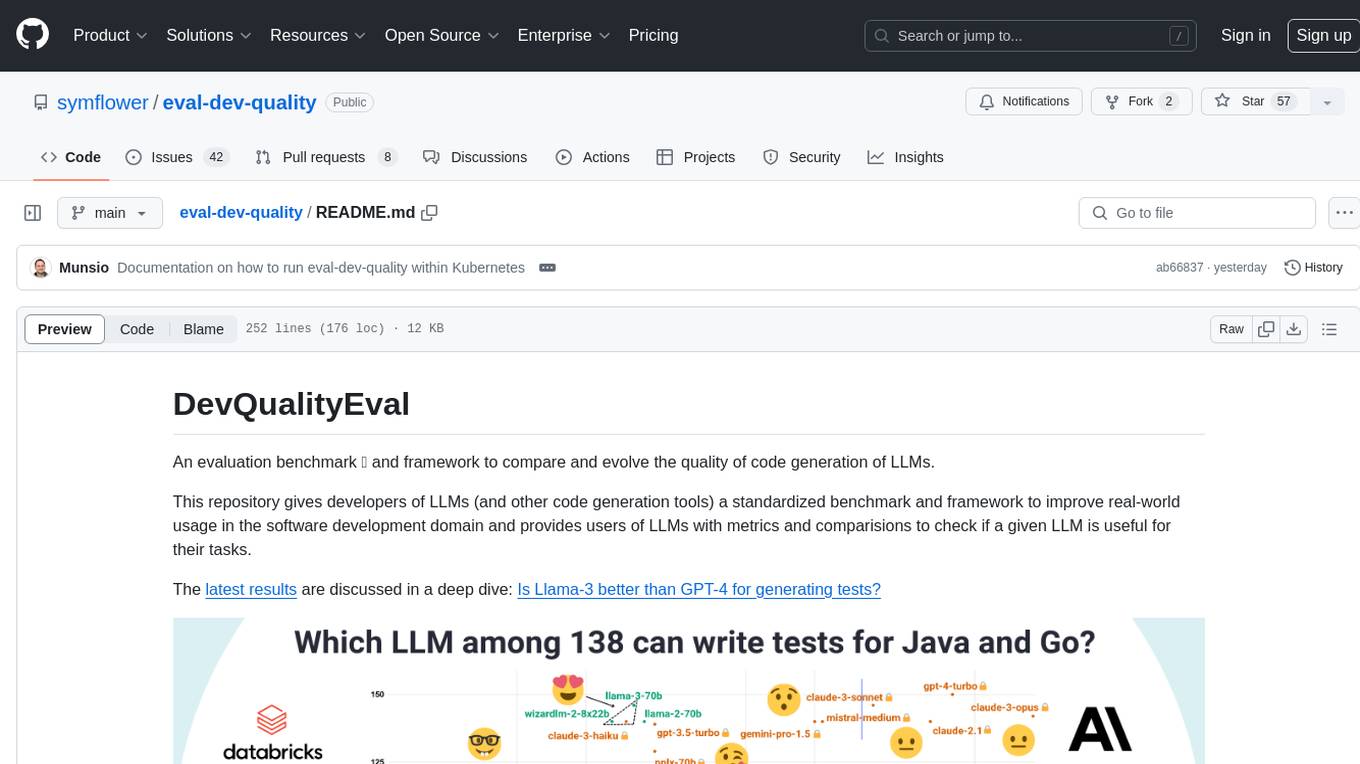
DevQualityEval is an evaluation benchmark and framework designed to compare and improve the quality of code generation of Language Model Models (LLMs). It provides developers with a standardized benchmark to enhance real-world usage in software development and offers users metrics and comparisons to assess the usefulness of LLMs for their tasks. The tool evaluates LLMs' performance in solving software development tasks and measures the quality of their results through a point-based system. Users can run specific tasks, such as test generation, across different programming languages to evaluate LLMs' language understanding and code generation capabilities.
README:
An evaluation benchmark 📈 and framework to compare and evolve the quality of code generation of LLMs.
This repository gives developers of LLMs (and other code generation tools) a standardized benchmark and framework to improve real-world usage in the software development domain and provides users of LLMs with metrics and comparisons to check if a given LLM is useful for their tasks.
The latest results are discussed in a deep dive: Anthropic's Claude 3.7 Sonnet is the new king 👑 of code generation (but only with help), and DeepSeek R1 disappoints (Deep dives from the DevQualityEval v1.0)
💰🍻 With this purchase you are mainly supporting DevQualityEval but you also receive access via your Google account to the detailed results of DevQualityEval v1.0 This includes: Access to the Google Sheet document with the leaderboard summary, as well as graphs, and exported metrics.
Since all deep dives build upon each other, it is worth taking a look at previous dives:
- OpenAI's o1-preview is the king 👑 of code generation but is super slow and expensive (Deep dives from the DevQualityEval v0.6)
- DeepSeek v2 Coder and Claude 3.5 Sonnet are more cost-effective at code generation than GPT-4o! (Deep dives from the DevQualityEval v0.5.0)
- Is Llama-3 better than GPT-4 for generating tests? And other deep dives of the DevQualityEval v0.4.0
- Can LLMs test a Go function that does nothing?
Install Git, install Go, and then execute the following commands:
git clone https://github.com/symflower/eval-dev-quality.git
cd eval-dev-quality
go install -v github.com/symflower/eval-dev-quality/cmd/eval-dev-quality
You can now use the eval-dev-quality
binary to execute the benchmark.
REMARK This project does not execute the LLM generated code in a sandbox by default. Make sure that you are running benchmarks only inside of an isolated environment, e.g. by using
--runtime docker
.
The easiest-to-use LLM provider is openrouter.ai. You need to create an access key and save it in an environment variable:
export PROVIDER_TOKEN=openrouter:${your-key}
Then you can run all benchmark tasks on all models and repositories:
eval-dev-quality evaluate
The output of the commands is a detailed log of all the requests and responses to the models and of all the commands executed. After the execution, you can find the final result saved to the file evaluation.csv
.
See eval-dev-quality --help
and especially eval-dev-quality evaluate --help
for options.
In the case you only want to evaluate only one or more models you can use the --model
option to define a model you want to use. You can use this option with as many models as you want.
Executing the following output:
eval-dev-quality evaluate --model=openrouter/meta-llama/llama-3-70b-instruct
Should return an evaluation log similar to this:
Log for the above command.
2024/05/02 10:01:58 Writing results to evaluation-2024-05-02-10:01:58
2024/05/02 10:01:58 Checking that models and languages can be used for evaluation
2024/05/02 10:01:58 Evaluating model "openrouter/meta-llama/llama-3-70b-instruct" using language "golang" and repository "golang/plain"
2024/05/02 10:01:58 Querying model "openrouter/meta-llama/llama-3-70b-instruct" with:
Given the following Go code file "plain.go" with package "plain", provide a test file for this code.
The tests should produce 100 percent code coverage and must compile.
The response must contain only the test code and nothing else.
```golang
package plain
func plain() {
return // This does not do anything but it gives us a line to cover.
}
```
2024/05/02 10:02:00 Model "openrouter/meta-llama/llama-3-70b-instruct" responded with:
```go
package plain
import "testing"
func TestPlain(t *testing.T) {
plain()
}
```
2024/05/02 10:02:00 $ symflower test --language golang --workspace /tmp/eval-dev-quality2330727502/plain
Checking for updates
There is a new version of symflower available! Please run `symflower update`.
=== RUN TestPlain
--- PASS: TestPlain (0.00s)
PASS
coverage: 100.0% of statements
ok plain 0.002s coverage: 100.0% of statements
DONE 1 tests in 0.348s
2024/05/02 10:02:01 Evaluated model "openrouter/meta-llama/llama-3-70b-instruct" using language "golang" and repository "golang/plain": encountered 0 problems: []
2024/05/02 10:02:01 Evaluating model "openrouter/meta-llama/llama-3-70b-instruct" using language "java" and repository "java/plain"
2024/05/02 10:02:01 Querying model "openrouter/meta-llama/llama-3-70b-instruct" with:
Given the following Java code file "src/main/java/com/eval/Plain.java" with package "com.eval", provide a test file for this code with JUnit 5 as a test framework.
The tests should produce 100 percent code coverage and must compile.
The response must contain only the test code and nothing else.
```java
package com.eval;
class Plain {
static void plain() {
}
}
```
2024/05/02 10:02:02 Model "openrouter/meta-llama/llama-3-70b-instruct" responded with:
```java
package com.eval;
import org.junit.jupiter.api.Test;
import static org.junit.jupiter.api.Assertions.assertFalse;
import static org.junit.jupiter.api.Assertions.assertTrue;
public class PlainTest {
@Test
void testPlain() {
Plain.plain();
// Since the method is empty, we can only assert that it doesn't throw an exception
assertTrue(true);
}
}
```
2024/05/02 10:02:02 $ symflower test --language java --workspace /tmp/eval-dev-quality1094965069/plain
Total coverage 100.000000%
2024/05/02 10:02:09 Evaluated model "openrouter/meta-llama/llama-3-70b-instruct" using language "java" and repository "java/plain": encountered 0 problems: []
2024/05/02 10:02:09 Evaluating models and languages
2024/05/02 10:02:09 Evaluation score for "openrouter/meta-llama/llama-3-70b-instruct": coverage=2, files-executed=2, response-no-error=2, response-no-excess=2, response-not-empty=2, response-with-code=2
The execution by default also creates a report file REPORT.md
that contains additional evaluation results and links to individual result files.
The following parameters do have a special behavior when using a containerized runtime.
-
--configuration
: Passing configuration files to the docker runtime is currently unsupported. -
--testdata
: The check if the path exists is ignored on the host system but still enforced inside the container because the paths of the host and inside the container might differ.
Ensure that docker is installed on the system.
docker build . -t eval-dev-quality:dev
docker pull ghcr.io/symflower/eval-dev-quality:main
The following command will run the model symflower/symbolic-execution
and stores the the results of the run inside the local directory evaluation
.
eval-dev-quality evaluate --runtime docker --runtime-image eval-dev-quality:dev --model symflower/symbolic-execution
Omitting the --runtime-image
parameter will default to the image from the main
branch. ghcr.io/symflower/eval-dev-quality:main
eval-dev-quality evaluate --runtime docker --model symflower/symbolic-execution
Please check the Kubernetes documentation.
The following providers (for model inference) are currently supported:
-
OpenRouter
- register API key via:
--tokens=openrouter:${key}
or environmentPROVIDER_TOKEN=openrouter:${key}
- select models via the
openrouter
prefix, i.e.--model openrouter/meta-llama/llama-3.1-8b-instruct
- register API key via:
-
Ollama
- the evaluation listens to the default Ollama port (
11434
), or will attempt to start an Ollama server if you have theollama
binary on your path - select models via the
ollama
prefix, i.e.--model ollama/llama3.1:8b
- the evaluation listens to the default Ollama port (
-
OpenAI API
- use any inference endpoint that conforms to the OpenAI chat completion API
- register the endpoint using
--urls=custom-${name}:${endpoint-url}
(mind thecustom-
prefix) or using the environmentPROVIDER_URL
- ensure to register your API key to the same
custom-${name}
- select models via the
custom-${name}
prefix - example for fireworks.ai:
eval-dev-quality evaluate --urls=custom-fw:https://api.fireworks.ai/inference/v1 --tokens=custom-fw:${your-api-token} --model custom-fw/accounts/fireworks/models/llama-v3p1-8b-instruct
With DevQualityEval
we answer answer the following questions:
- Which LLMs can solve software development tasks?
- How good is the quality of their results?
Programming is a non-trivial profession. Even writing tests for an empty function requires substantial knowledge of the used programming language and its conventions. We already investigated this challenge and how many LLMs failed at it in our first DevQualityEval
report. This highlights the need for a benchmarking framework for evaluating AI performance on software development task solving.
The models evaluated in DevQualityEval
have to solve programming tasks, not only in one, but in multiple programming languages.
Every task is a well-defined, abstract challenge that the model needs to complete (for example: writing a unit test for a given function).
Multiple concrete cases (or candidates) exist for a given task that each represent an actual real-world example that a model has to solve (i.e. for function abc() {...
write a unit test).
Completing a task-case rewards points depending on the quality of the result. This, of course, depends on which criteria make the solution to a task a "good" solution, but the general rule is that the more points - the better. For example, the unit tests generated by a model might actually be compiling, yielding points that set the model apart from other models that generate only non-compiling code.
Each repository can contain a configuration file repository.json
in its root directory specifying a list of tasks which are supposed to be run for this repository.
{
"tasks": ["write-tests"]
}
For the evaluation of the repository only the specified tasks are executed. If no repository.json
file exists, all tasks are executed.
Depending on the task, it can be beneficial to exclude parts of the repository from explicit evaluation. To give a concrete example: Spring controller tests can never be executed on their own but need a supporting Application
class. But such a file should never be used itself to prompt models for tests. Therefore, it can be excluded through the repository.json
configuration:
{
"tasks": ["write-tests"],
"ignore": ["src/main/java/com/example/Application.java"]
}
This ignore
setting is currently only applicable for generation task write-tests
.
It is possible to configure some model prompt parameters through repository.json
:
{
"tasks": ["write-tests"],
"prompt": {
"test-framework": "JUnit 5 for Spring Boot" // Overwrite the default test framework in the prompt.
}
}
This prompt.test-framework
setting is currently only applicable for the test generation task write-tests
.
When task results are validated, some repositories might require custom logic. For example: generating tests for a Spring Boot project requires ensuring that the tests used an actual Spring context (i.e. Spring Boot was initialized when the tests were executed). Therefore, the repository.json
supports adding rudimentary custom validation:
{
"tasks": ["write-tests"],
"validation": {
"execution": {
"stdout": "Initializing Spring" // Ensure the string "Initializing Spring" is contained in the execution output.
}
}
}
This validation.execution.stdout
setting is currently only applicable for the test generation task write-tests
.
Test generation is the task of generating a test suite for a given source code example.
The great thing about test generation is that it is easy to automatically check if the result is correct. It needs to compile and provide 100% coverage. A model can only write such tests if it understands the source, so implicitly we are evaluating the language understanding of a LLM.
On a high level, DevQualityEval
asks the model to produce tests for an example case, saves the response to a file and tries to execute the resulting tests together with the original source code.
Currently, the following cases are available for this task:
- Java
- Go
- Ruby
Code repair is the task of repairing source code with compilation errors.
For this task, we introduced the mistakes
repository, which includes examples of source code with compilation errors. Each example is isolated in its own package, along with a valid test suite. We compile each package in the mistakes
repository and provide the LLM's with both the source code and the list of compilation errors. The LLM's response is then validated with the predefined test suite.
Currently, the following cases are available for this task:
- Java
- Go
- Ruby
Transpile is the task of converting source code from one language to another.
The test data repository for this task consists of several packages. At the root of each package, there must be a folder called implementation
containing the implementation files (one per origin language), which are to be transpiled from. Each package must also contain a source file with a stub (a function signature so the LLM's know the signature of the function they need to generate code for) and a valid test suite. The LLM's response is then validated with the predefined test suite.
Currently, the following cases are available for this task:
- Java
- Go
- Ruby
Currently, the following points are awarded:
-
response-no-error
:+1
if the response did not encounter an error -
response-not-empty
:+1
if the response is not empty -
response-with-code
:+1
if the response contained source code -
compiled
:+1
if the source code compiled -
statement-coverage-reached
:+10
for each coverage object of executed code (disabled fortranspile
andcode-repair
, as models are writing the implementation code and could just add arbitrary statements to receive a higher score) -
no-excess
:+1
if the response did not contain more content than requested -
passing-tests
:+10
for each test that is passing (disabled forwrite-test
, as models are writing the test code and could just add arbitrary test cases to receive a higher score)
When interpreting the results, please keep the following in mind that LLMs are nondeterministic, so results may vary.
Furthermore, the choice of a "best" model for a task might depend on additional factors. For example, the needed computing resources or query cost of a cloud LLM endpoint differs greatly between models. Also, the open availability of model weights might change one's model choice. So in the end, there is no "perfect" overall winner, only the "perfect" model for a specific use case.
If you want to add new files to existing language repositories or new repositories to existing languages, install the evaluation binary of this repository and you are good to go.
To add new tasks to the benchmark, add features, or fix bugs, you'll need a development environment. The development environment comes with this repository and can be installed by executing make install-all
. Then you can run make
to see the documentation for all the available commands.
First of all, thank you for thinking about contributing! There are multiple ways to contribute:
- Add more files to existing language repositories.
- Add more repositories to languages.
- Implement another language and add repositories for it.
- Implement new tasks for existing languages and repositories.
- Add more features and fix bugs in the evaluation, development environment, or CI: best to have a look at the list of issues.
If you want to contribute but are unsure how: create a discussion or write us directly at [email protected].
Releasing a new version of DevQualityEval
and publishing content about it are two different things!
But, we plan development and releases to be "content-driven", i.e. we work on / add features that are interesting to write about (see "Release Roadmap" below).
Hence, for every release we also publish a deep dive blog post with our latest features and findings.
Our published content is aimed at giving insight into our work and educating the reader.
- new features that add value (i.e. change / add to the scoring / ranking) are always publish-worthy / release-worthy
- new tools / models are very often not release-worthy but still publish-worthy (i.e. how is new model
XYZ
doing in the benchmark) - insights, learnings, problems, surprises, achieved goals and experiments are always publish-worthy
- they need to be documented for later publishing (in a deep-dive blog post)
- they can also be published right away already (depending on the nature of the finding) as a small report / post
❗ Always publish / release / journal early:
- if something works, but is not merged yet: publish about it already
- if some feature is merged that is release-worthy, but we were planning on adding other things to that release: release anyways
- if something else is publish-worth: at least write down a few bullet-points immediately why it is interesting, including examples
The main
branch is always stable and could theoretically be used to form a new release at any given time.
To avoid having hundreds of releases for every merge to main
, we perform releases only when a (group of) publish-worthy feature(s) or important bugfix(es) is merged (see "Publishing Content" above).
Therefore, we plan releases in special Roadmap for vX.Y.Z
issues.
Such an issue contains a current list of publish-worthy goals that must be met for that release, a TODO
section with items not planned for the current but a future release, and instructions on how issues / PRs / tasks need to be groomed and what needs to be done for a release to happen.
The issue template for roadmap issues can be found at .github/ISSUE_TEMPLATE/roadmap.md
For Tasks:
Click tags to check more tools for each tasksFor Jobs:
Alternative AI tools for eval-dev-quality
Similar Open Source Tools
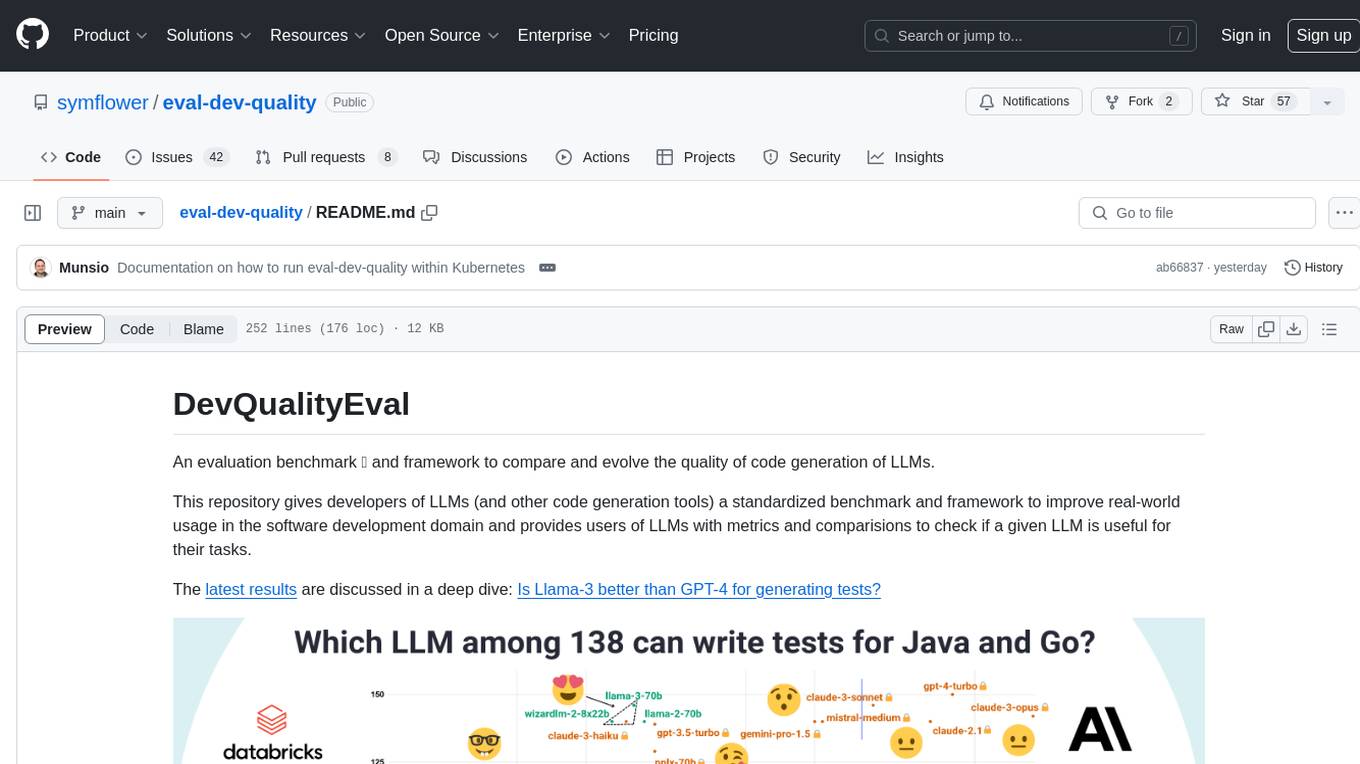
eval-dev-quality
DevQualityEval is an evaluation benchmark and framework designed to compare and improve the quality of code generation of Language Model Models (LLMs). It provides developers with a standardized benchmark to enhance real-world usage in software development and offers users metrics and comparisons to assess the usefulness of LLMs for their tasks. The tool evaluates LLMs' performance in solving software development tasks and measures the quality of their results through a point-based system. Users can run specific tasks, such as test generation, across different programming languages to evaluate LLMs' language understanding and code generation capabilities.
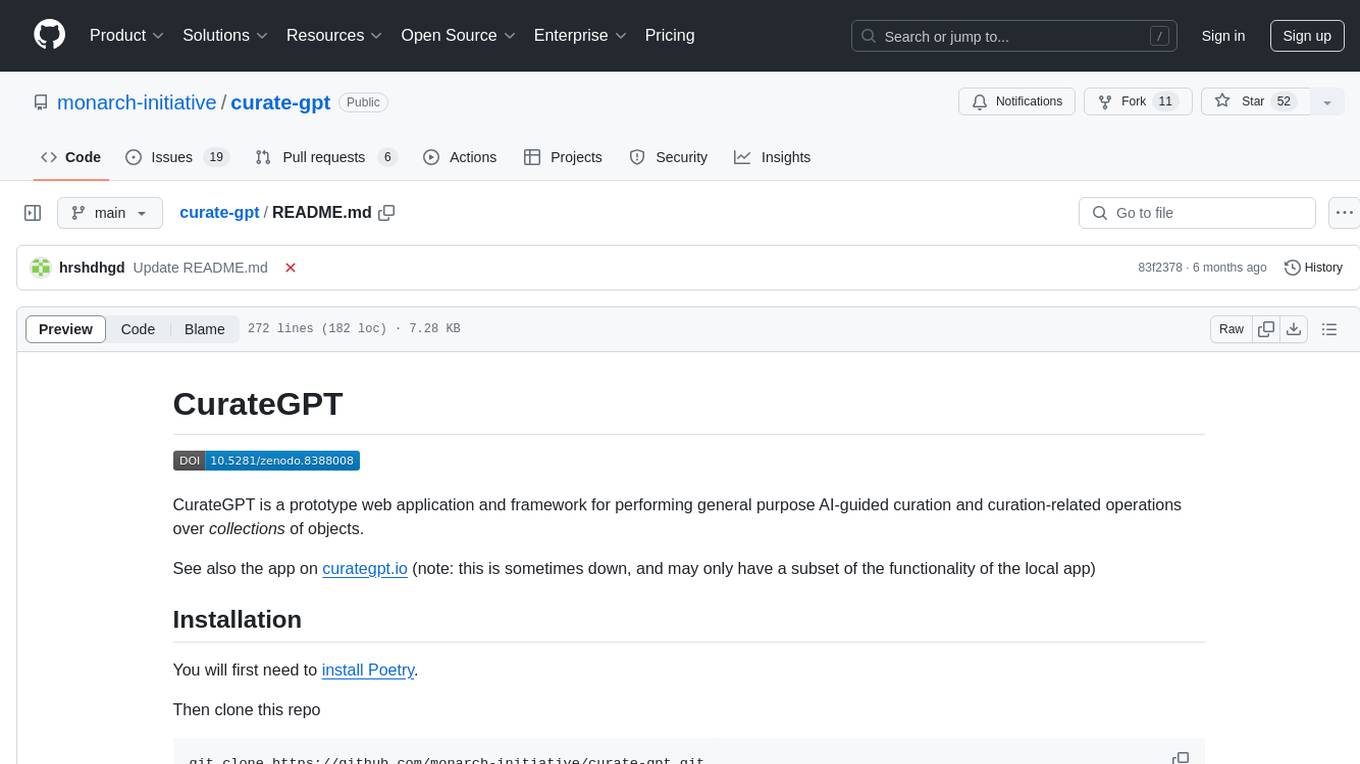
curate-gpt
CurateGPT is a prototype web application and framework for performing general purpose AI-guided curation and curation-related operations over collections of objects. It allows users to load JSON, YAML, or CSV data, build vector database indexes for ontologies, and interact with various data sources like GitHub, Google Drives, Google Sheets, and more. The tool supports ontology curation, knowledge base querying, term autocompletion, and all-by-all comparisons for objects in a collection.
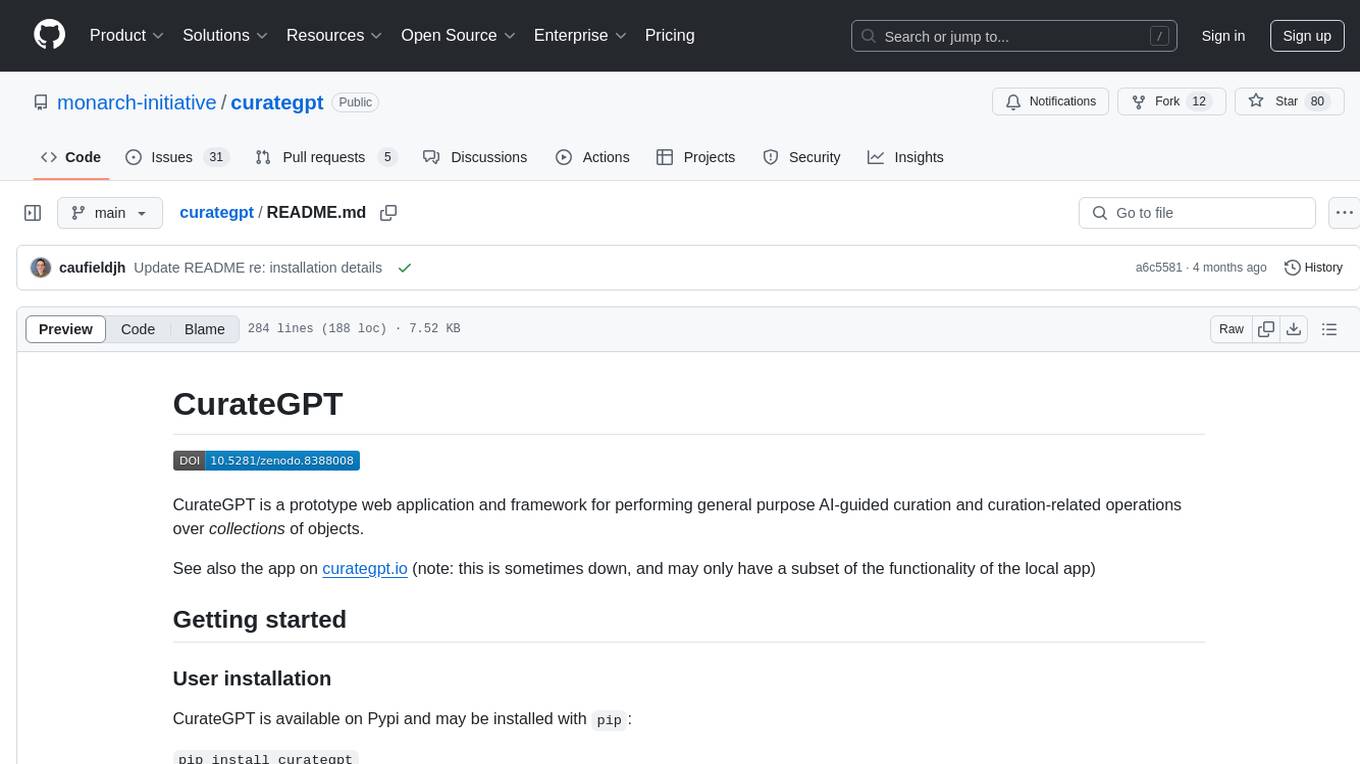
curategpt
CurateGPT is a prototype web application and framework designed for general purpose AI-guided curation and curation-related operations over collections of objects. It provides functionalities for loading example data, building indexes, interacting with knowledge bases, and performing tasks such as chatting with a knowledge base, querying Pubmed, interacting with a GitHub issue tracker, term autocompletion, and all-by-all comparisons. The tool is built to work best with the OpenAI gpt-4 model and OpenAI ada-text-embedding-002 for embedding, but also supports alternative models through a plugin architecture.
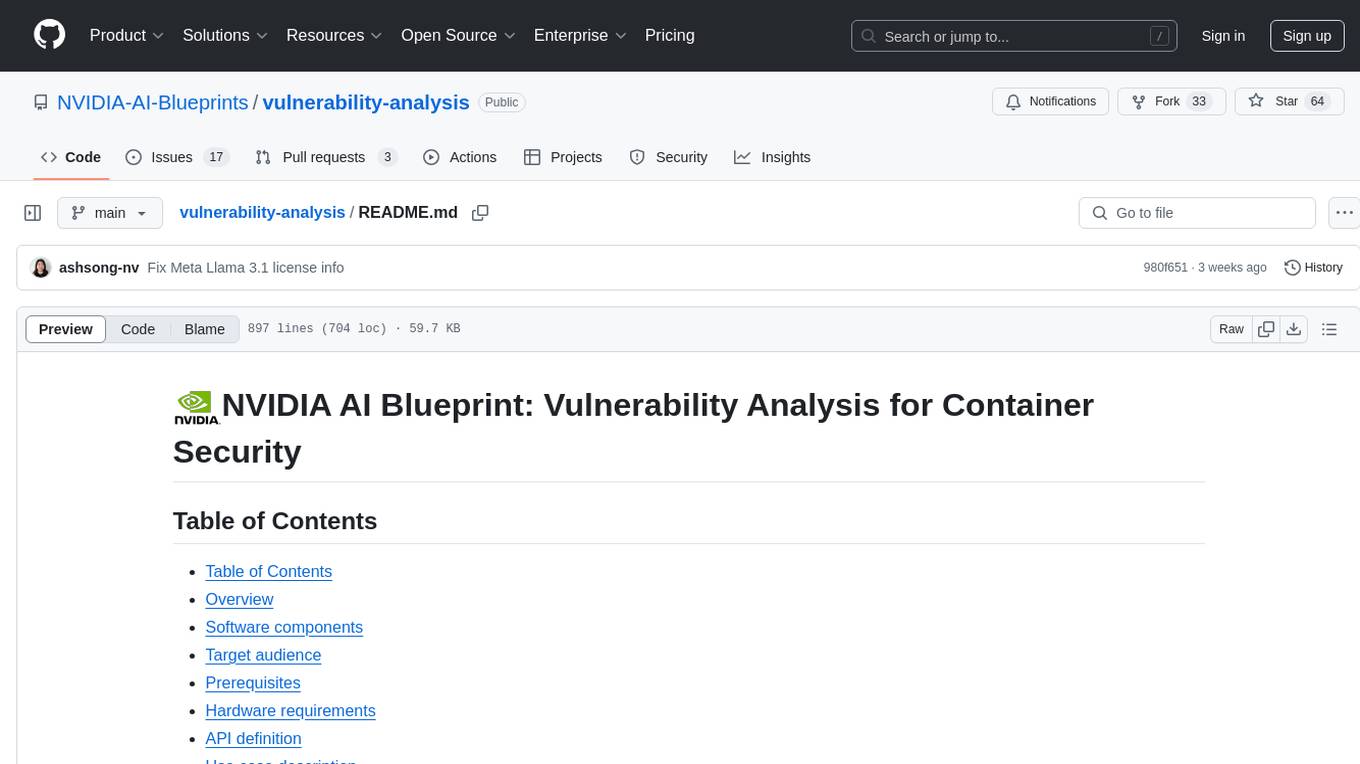
vulnerability-analysis
The NVIDIA AI Blueprint for Vulnerability Analysis for Container Security showcases accelerated analysis on common vulnerabilities and exposures (CVE) at an enterprise scale, reducing mitigation time from days to seconds. It enables security analysts to determine software package vulnerabilities using large language models (LLMs) and retrieval-augmented generation (RAG). The blueprint is designed for security analysts, IT engineers, and AI practitioners in cybersecurity. It requires NVAIE developer license and API keys for vulnerability databases, search engines, and LLM model services. Hardware requirements include L40 GPU for pipeline operation and optional LLM NIM and Embedding NIM. The workflow involves LLM pipeline for CVE impact analysis, utilizing LLM planner, agent, and summarization nodes. The blueprint uses NVIDIA NIM microservices and Morpheus Cybersecurity AI SDK for vulnerability analysis.
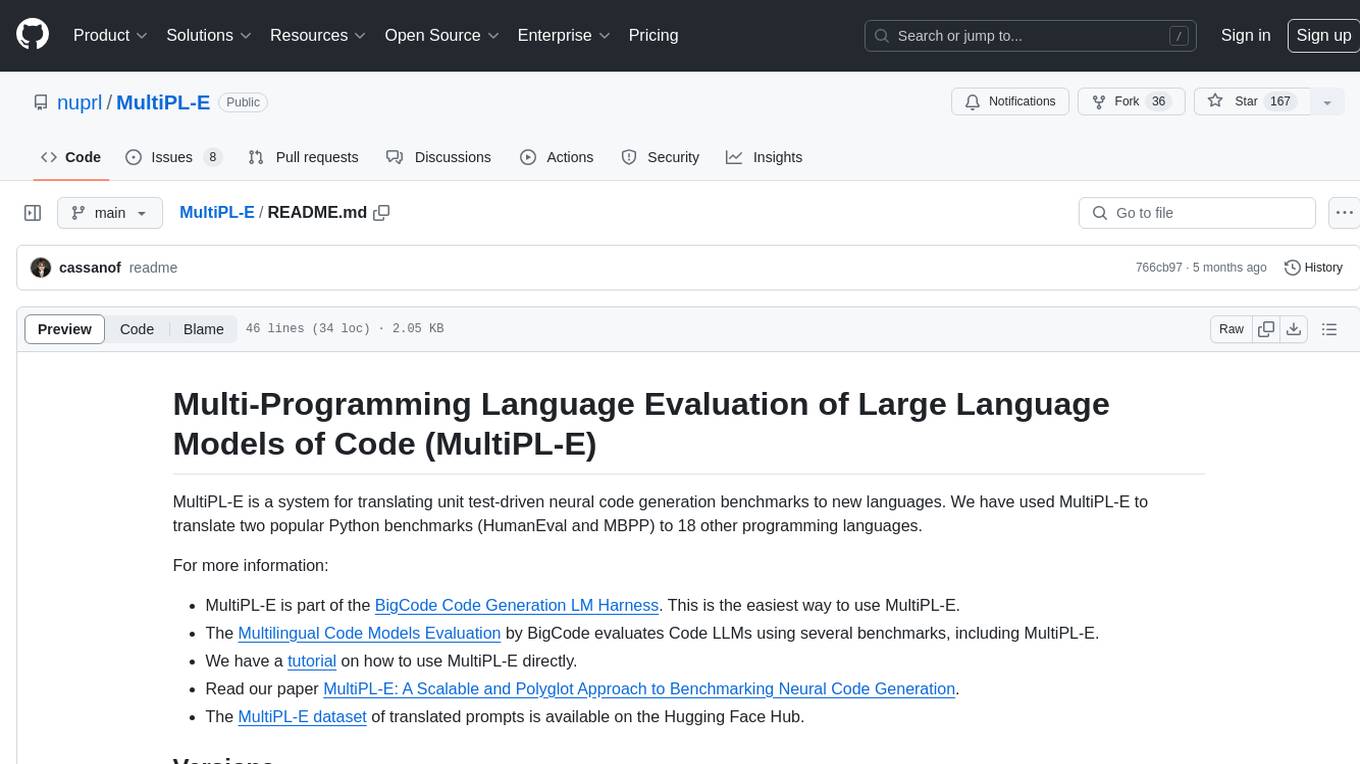
MultiPL-E
MultiPL-E is a system for translating unit test-driven neural code generation benchmarks to new languages. It is part of the BigCode Code Generation LM Harness and allows for evaluating Code LLMs using various benchmarks. The tool supports multiple versions with improvements and new language additions, providing a scalable and polyglot approach to benchmarking neural code generation. Users can access a tutorial for direct usage and explore the dataset of translated prompts on the Hugging Face Hub.
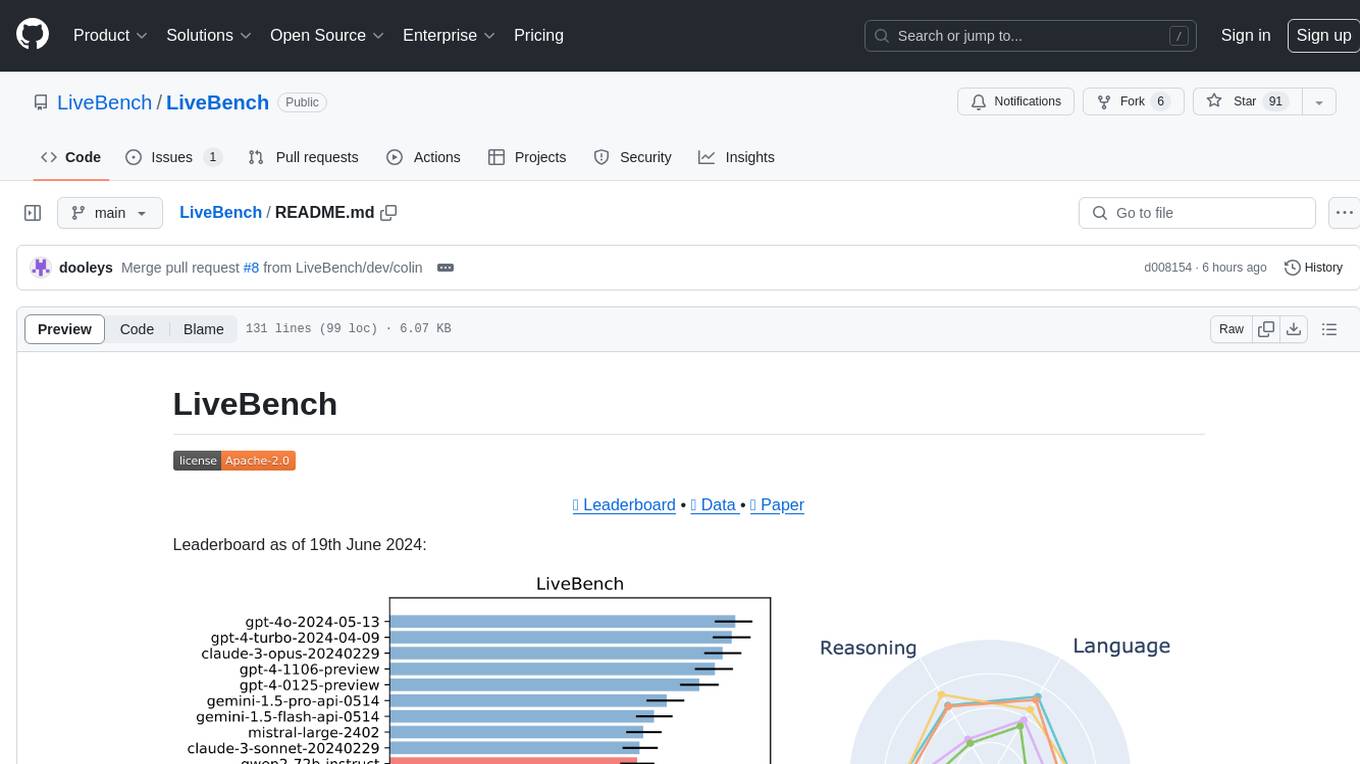
LiveBench
LiveBench is a benchmark tool designed for Language Model Models (LLMs) with a focus on limiting contamination through monthly new questions based on recent datasets, arXiv papers, news articles, and IMDb movie synopses. It provides verifiable, objective ground-truth answers for accurate scoring without an LLM judge. The tool offers 18 diverse tasks across 6 categories and promises to release more challenging tasks over time. LiveBench is built on FastChat's llm_judge module and incorporates code from LiveCodeBench and IFEval.
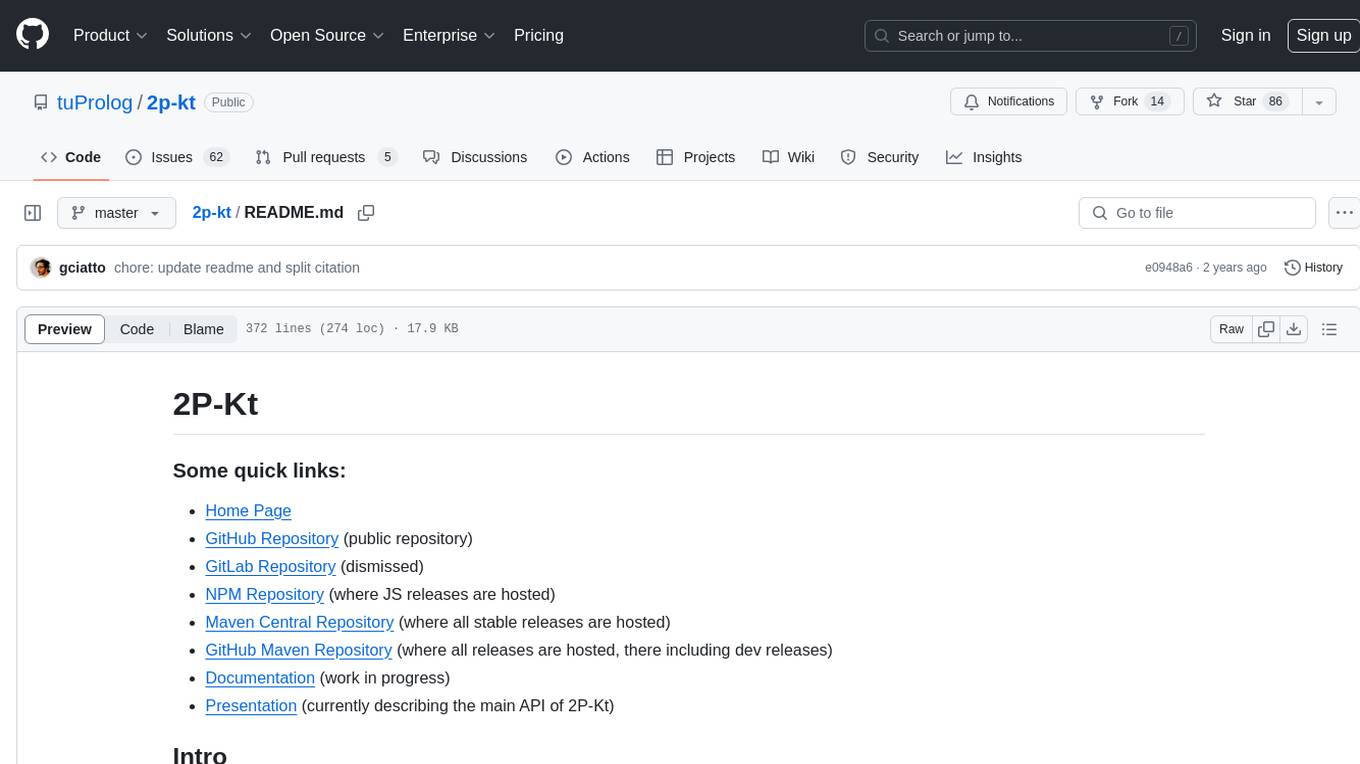
2p-kt
2P-Kt is a Kotlin-based and multi-platform reboot of tuProlog (2P), a multi-paradigm logic programming framework written in Java. It consists of an open ecosystem for Symbolic Artificial Intelligence (AI) with modules supporting logic terms, unification, indexing, resolution of logic queries, probabilistic logic programming, binary decision diagrams, OR-concurrent resolution, DSL for logic programming, parsing modules, serialisation modules, command-line interface, and graphical user interface. The tool is designed to support knowledge representation and automatic reasoning through logic programming in an extensible and flexible way, encouraging extensions towards other symbolic AI systems than Prolog. It is a pure, multi-platform Kotlin project supporting JVM, JS, Android, and Native platforms, with a lightweight library leveraging the Kotlin common library.
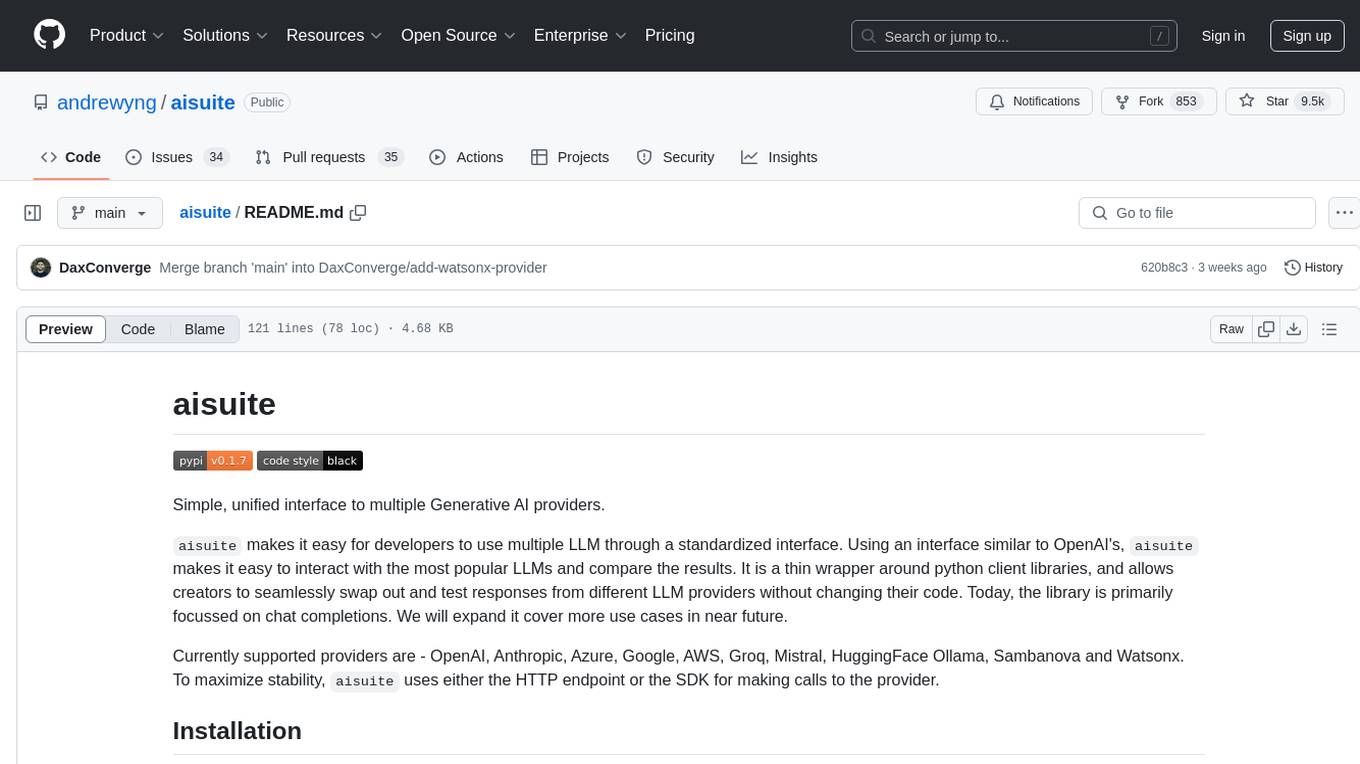
aisuite
Aisuite is a simple, unified interface to multiple Generative AI providers. It allows developers to easily interact with various Language Model (LLM) providers like OpenAI, Anthropic, Azure, Google, AWS, and more through a standardized interface. The library focuses on chat completions and provides a thin wrapper around python client libraries, enabling creators to test responses from different LLM providers without changing their code. Aisuite maximizes stability by using HTTP endpoints or SDKs for making calls to the providers. Users can install the base package or specific provider packages, set up API keys, and utilize the library to generate chat completion responses from different models.
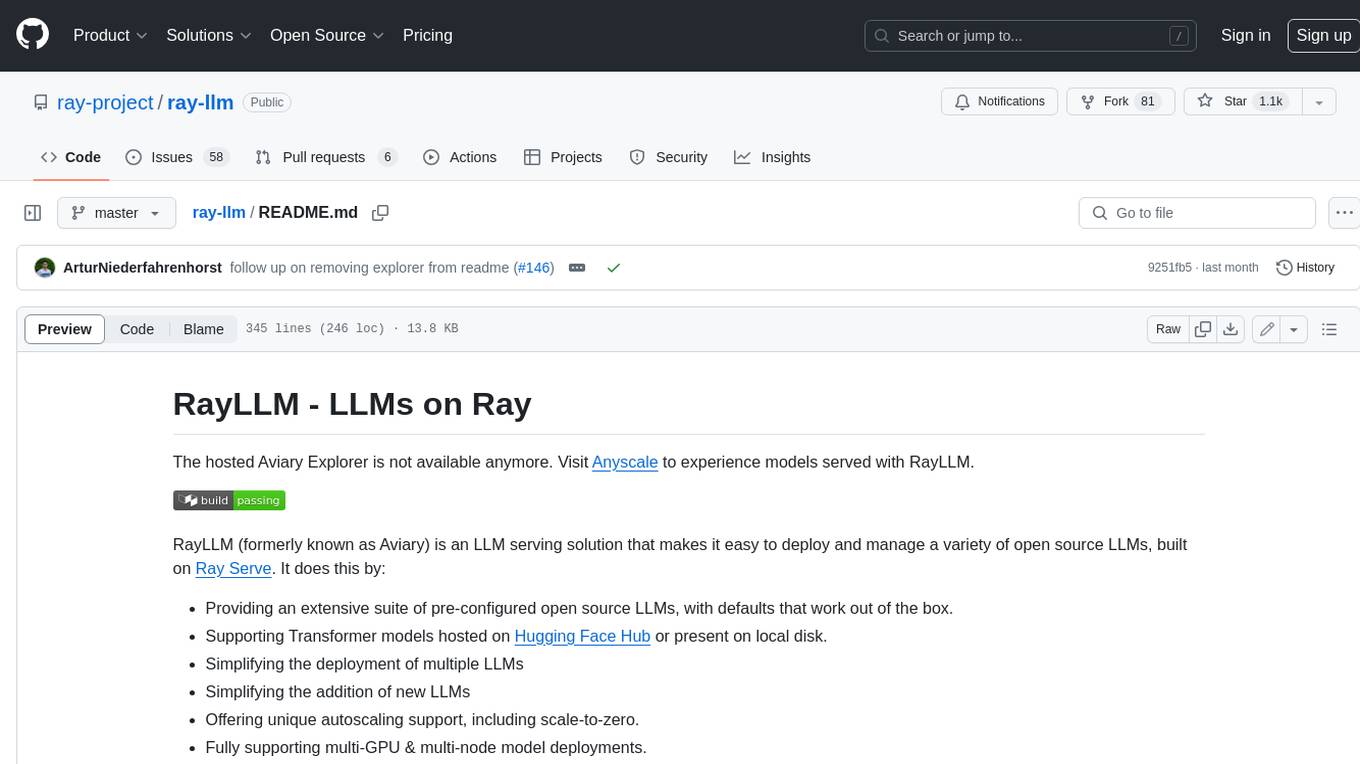
ray-llm
RayLLM (formerly known as Aviary) is an LLM serving solution that makes it easy to deploy and manage a variety of open source LLMs, built on Ray Serve. It provides an extensive suite of pre-configured open source LLMs, with defaults that work out of the box. RayLLM supports Transformer models hosted on Hugging Face Hub or present on local disk. It simplifies the deployment of multiple LLMs, the addition of new LLMs, and offers unique autoscaling support, including scale-to-zero. RayLLM fully supports multi-GPU & multi-node model deployments and offers high performance features like continuous batching, quantization and streaming. It provides a REST API that is similar to OpenAI's to make it easy to migrate and cross test them. RayLLM supports multiple LLM backends out of the box, including vLLM and TensorRT-LLM.
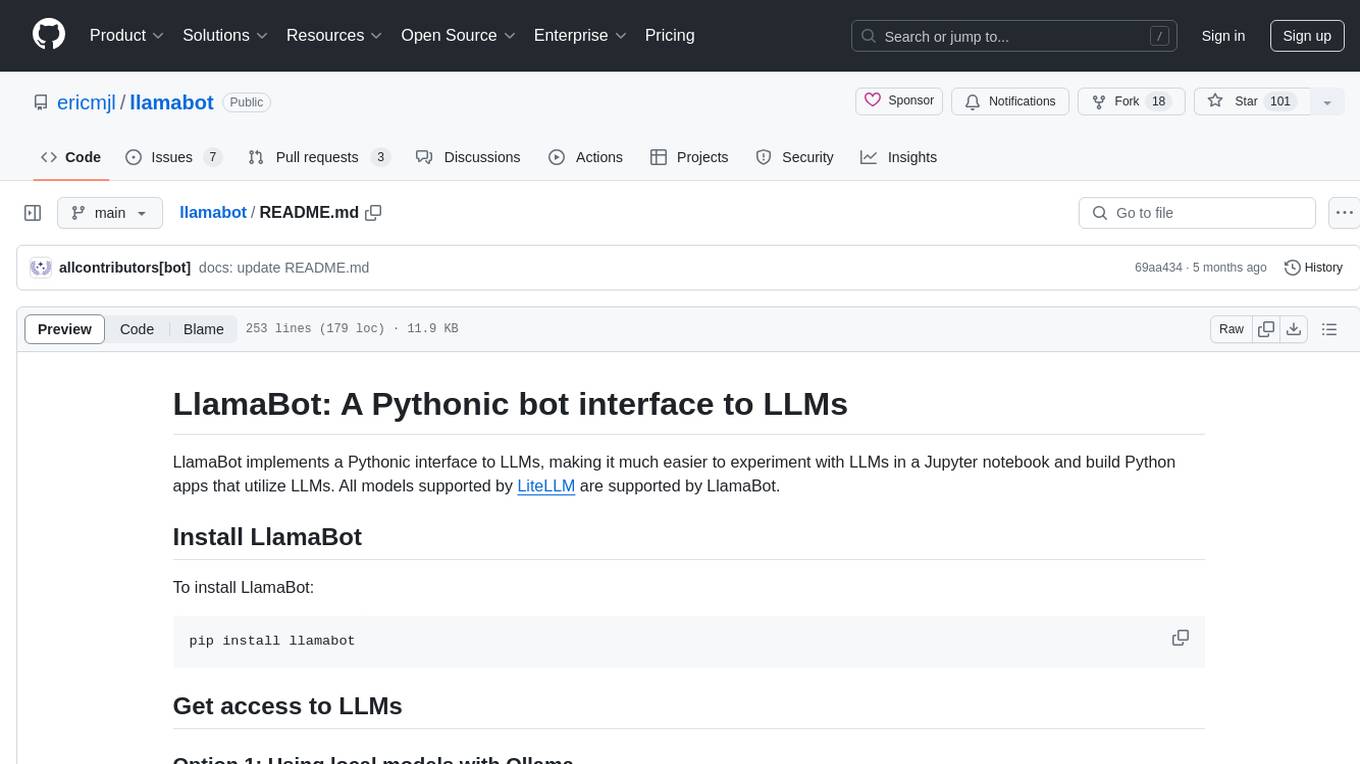
llamabot
LlamaBot is a Pythonic bot interface to Large Language Models (LLMs), providing an easy way to experiment with LLMs in Jupyter notebooks and build Python apps utilizing LLMs. It supports all models available in LiteLLM. Users can access LLMs either through local models with Ollama or by using API providers like OpenAI and Mistral. LlamaBot offers different bot interfaces like SimpleBot, ChatBot, QueryBot, and ImageBot for various tasks such as rephrasing text, maintaining chat history, querying documents, and generating images. The tool also includes CLI demos showcasing its capabilities and supports contributions for new features and bug reports from the community.
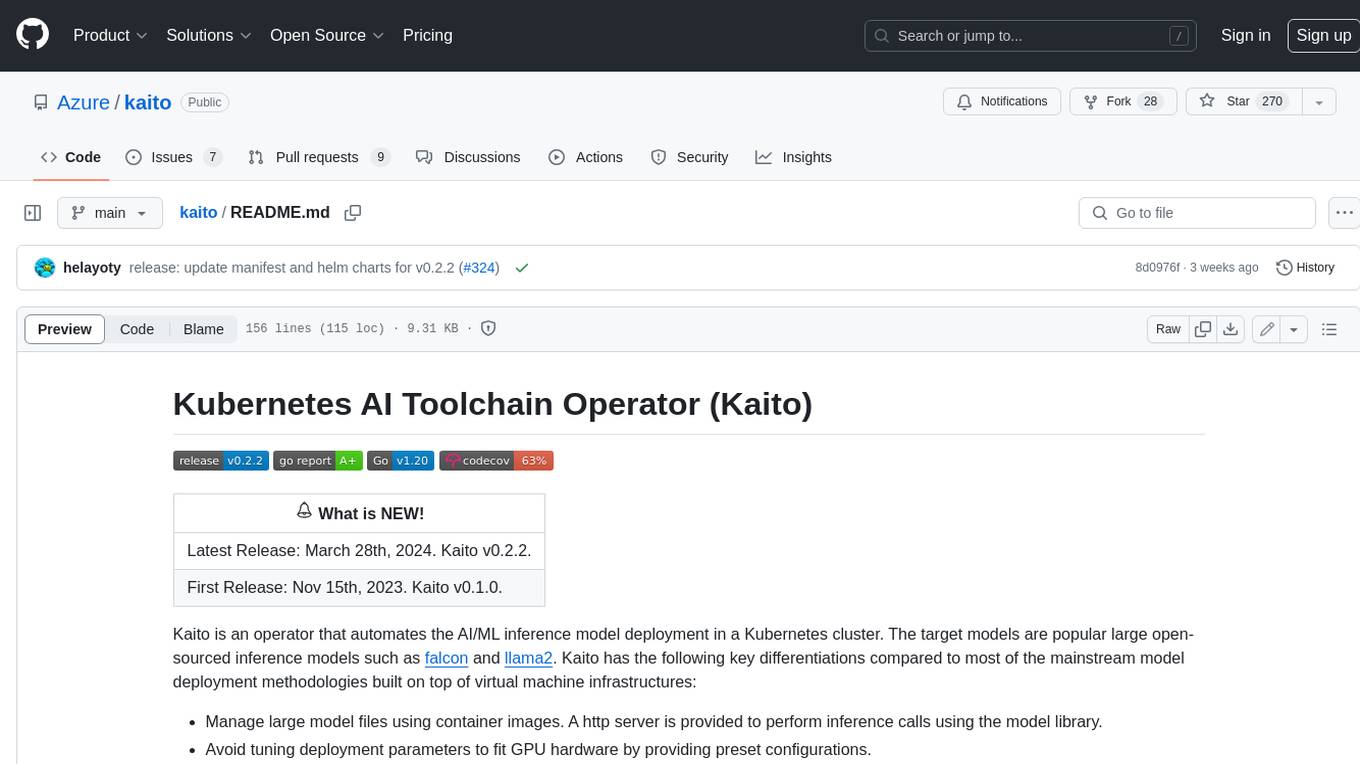
kaito
Kaito is an operator that automates the AI/ML inference model deployment in a Kubernetes cluster. It manages large model files using container images, avoids tuning deployment parameters to fit GPU hardware by providing preset configurations, auto-provisions GPU nodes based on model requirements, and hosts large model images in the public Microsoft Container Registry (MCR) if the license allows. Using Kaito, the workflow of onboarding large AI inference models in Kubernetes is largely simplified.
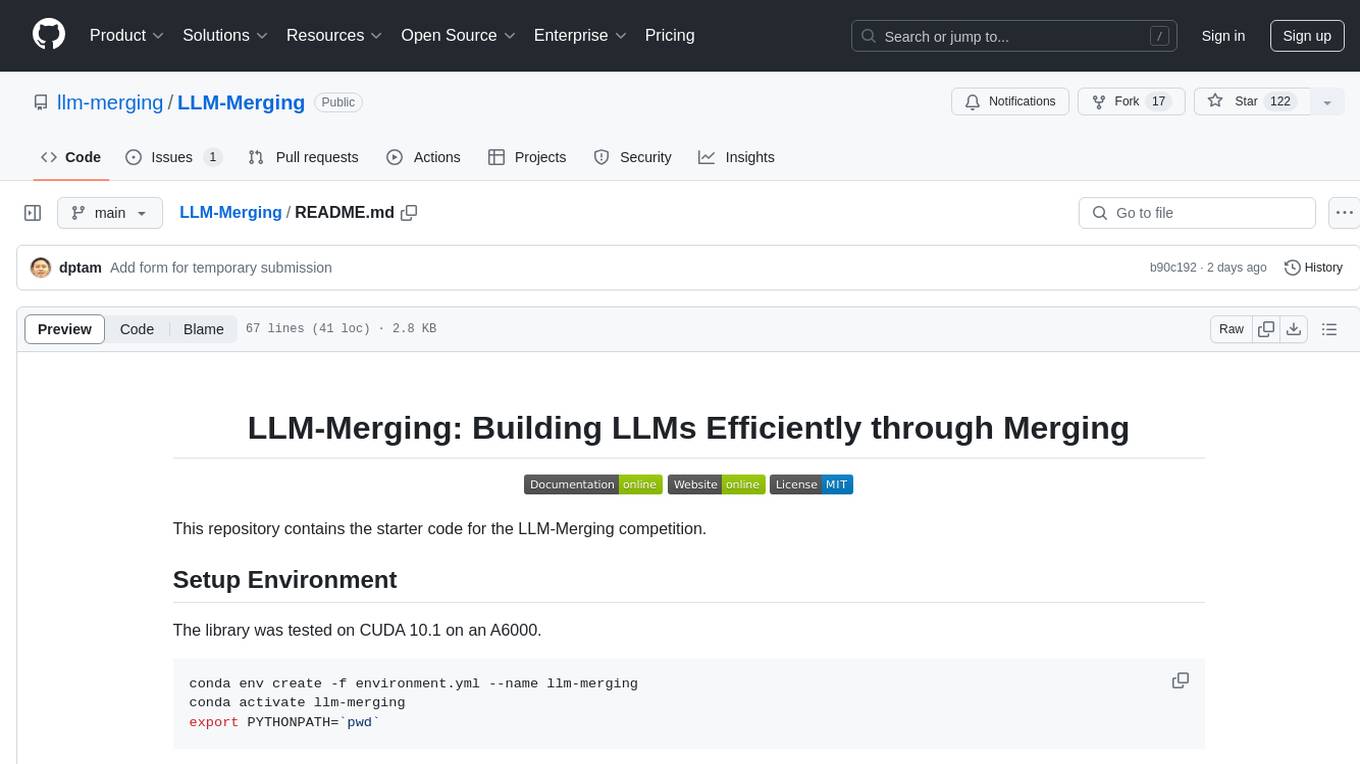
LLM-Merging
LLM-Merging is a repository containing starter code for the LLM-Merging competition. It provides a platform for efficiently building LLMs through merging methods. Users can develop new merging methods by creating new files in the specified directory and extending existing classes. The repository includes instructions for setting up the environment, developing new merging methods, testing the methods on specific datasets, and submitting solutions for evaluation. It aims to facilitate the development and evaluation of merging methods for LLMs.
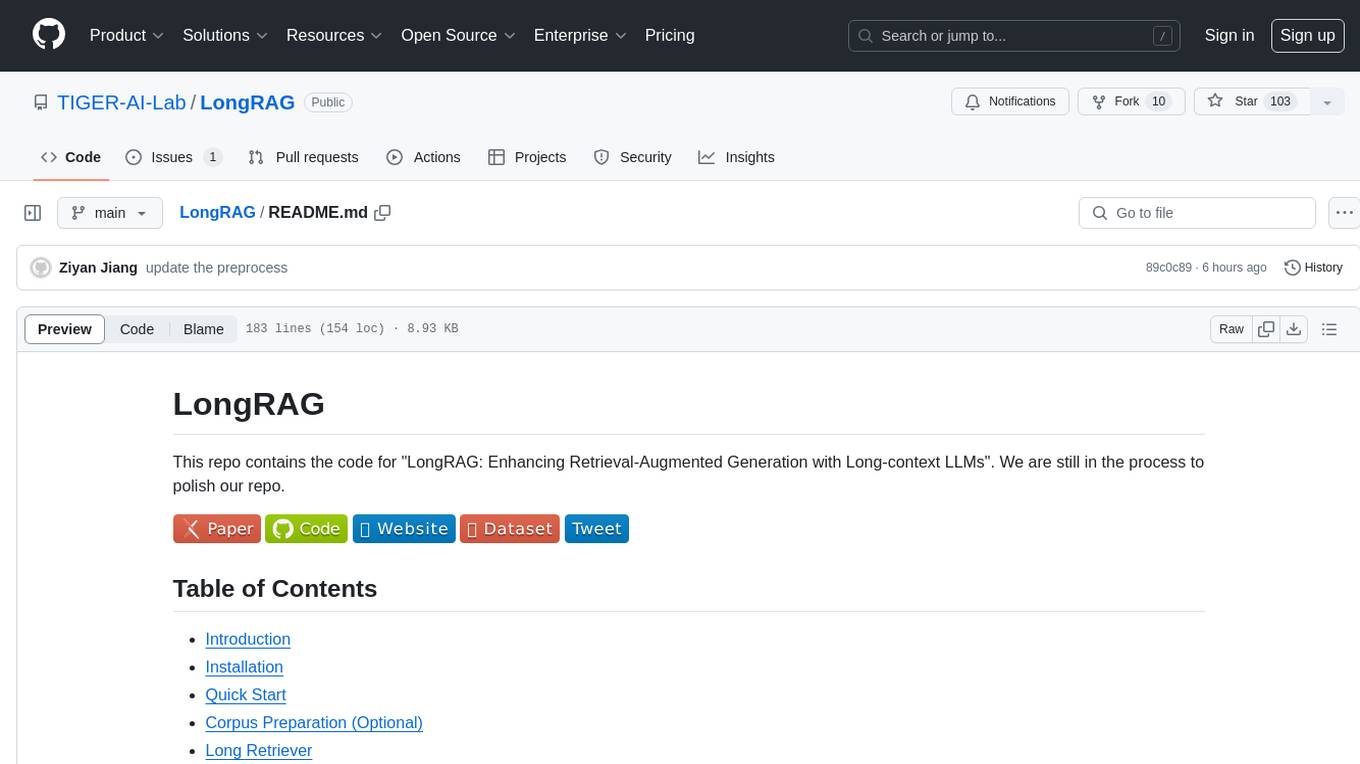
LongRAG
This repository contains the code for LongRAG, a framework that enhances retrieval-augmented generation with long-context LLMs. LongRAG introduces a 'long retriever' and a 'long reader' to improve performance by using a 4K-token retrieval unit, offering insights into combining RAG with long-context LLMs. The repo provides instructions for installation, quick start, corpus preparation, long retriever, and long reader.
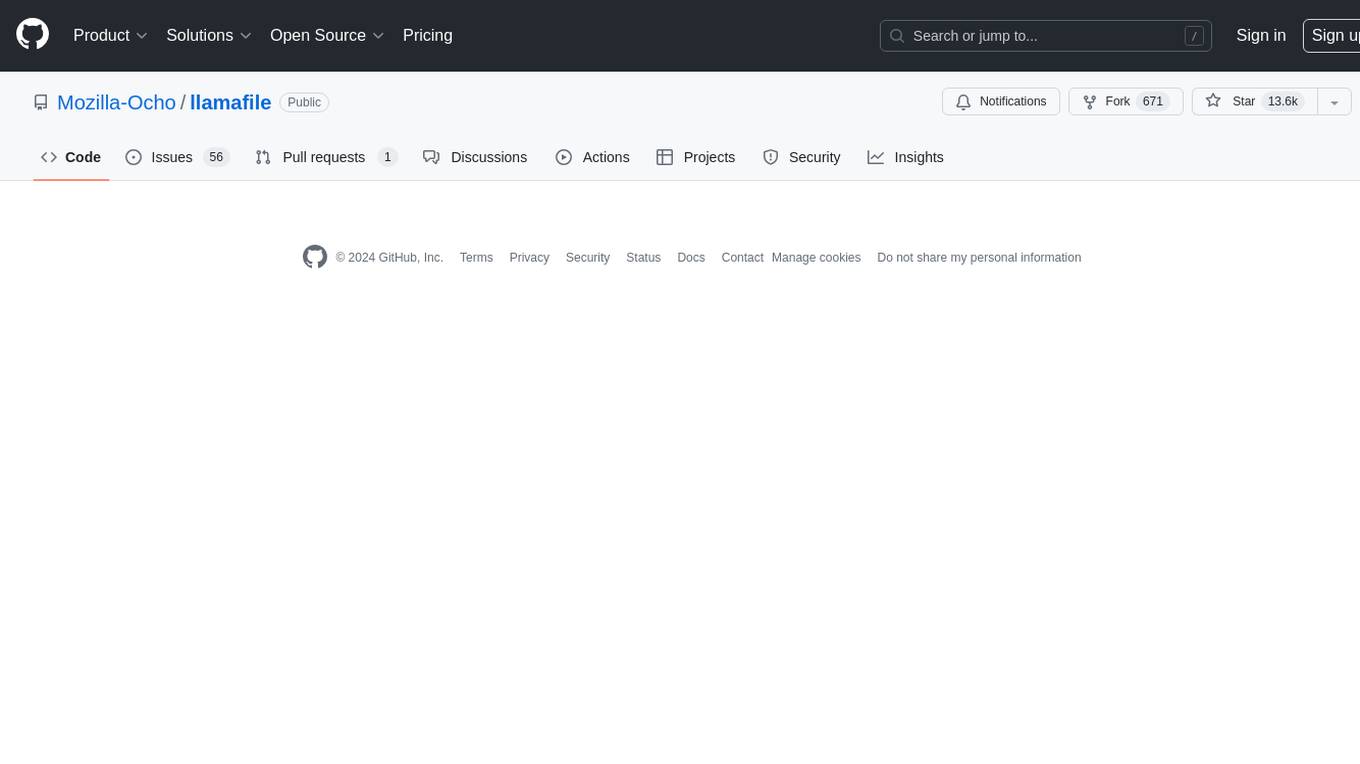
llamafile
llamafile is a tool that enables users to distribute and run Large Language Models (LLMs) with a single file. It combines llama.cpp with Cosmopolitan Libc to create a framework that simplifies the complexity of LLMs into a single-file executable called a 'llamafile'. Users can run these executable files locally on most computers without the need for installation, making open LLMs more accessible to developers and end users. llamafile also provides example llamafiles for various LLM models, allowing users to try out different LLMs locally. The tool supports multiple CPU microarchitectures, CPU architectures, and operating systems, making it versatile and easy to use.
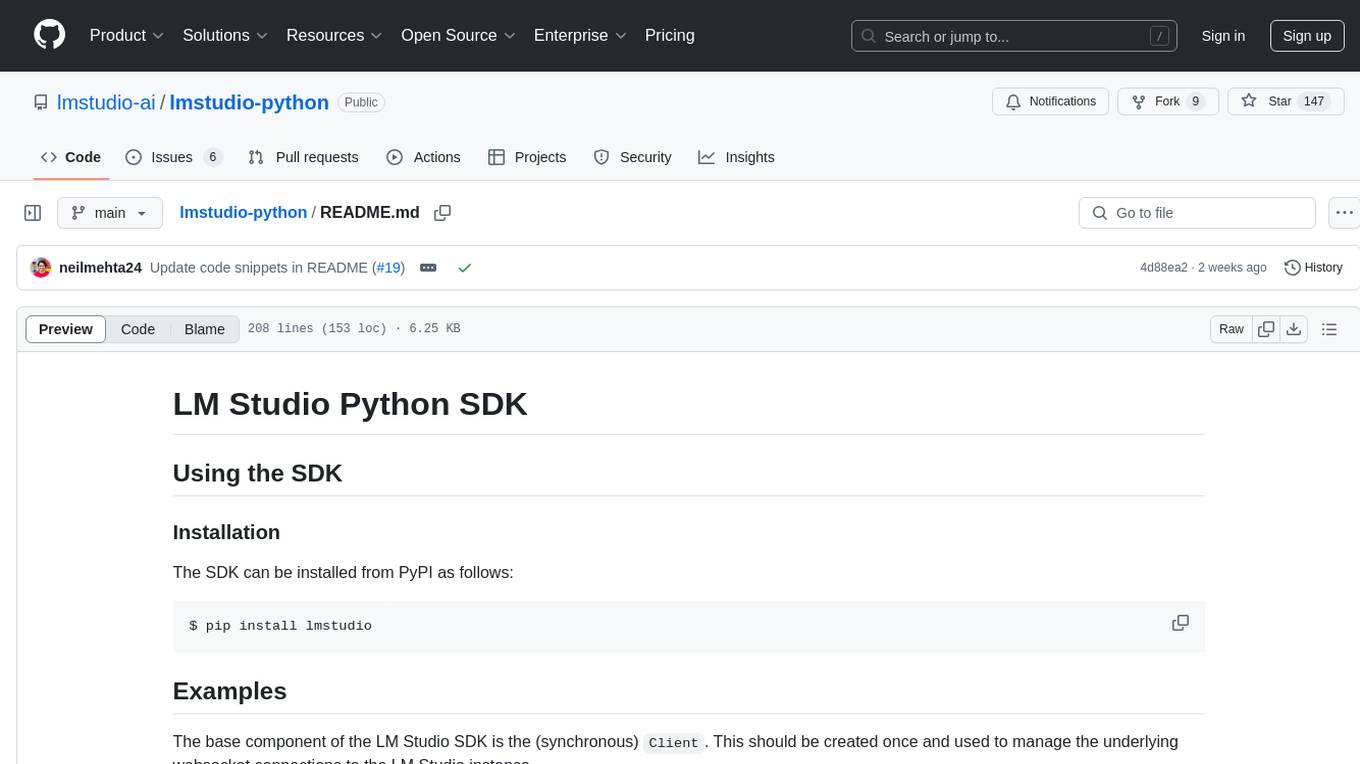
lmstudio-python
LM Studio Python SDK provides a convenient API for interacting with LM Studio instance, including text completion and chat response functionalities. The SDK allows users to manage websocket connections and chat history easily. It also offers tools for code consistency checks, automated testing, and expanding the API.
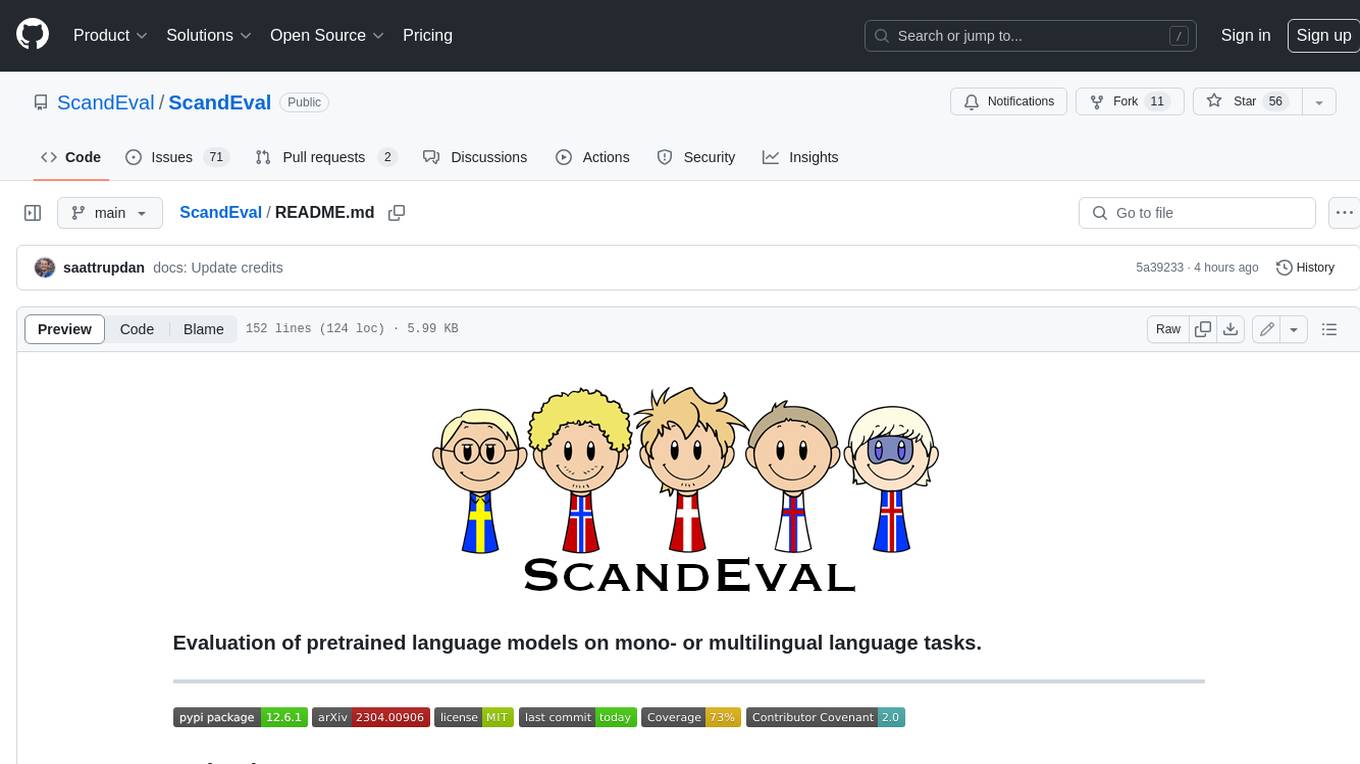
ScandEval
ScandEval is a framework for evaluating pretrained language models on mono- or multilingual language tasks. It provides a unified interface for benchmarking models on a variety of tasks, including sentiment analysis, question answering, and machine translation. ScandEval is designed to be easy to use and extensible, making it a valuable tool for researchers and practitioners alike.
For similar tasks
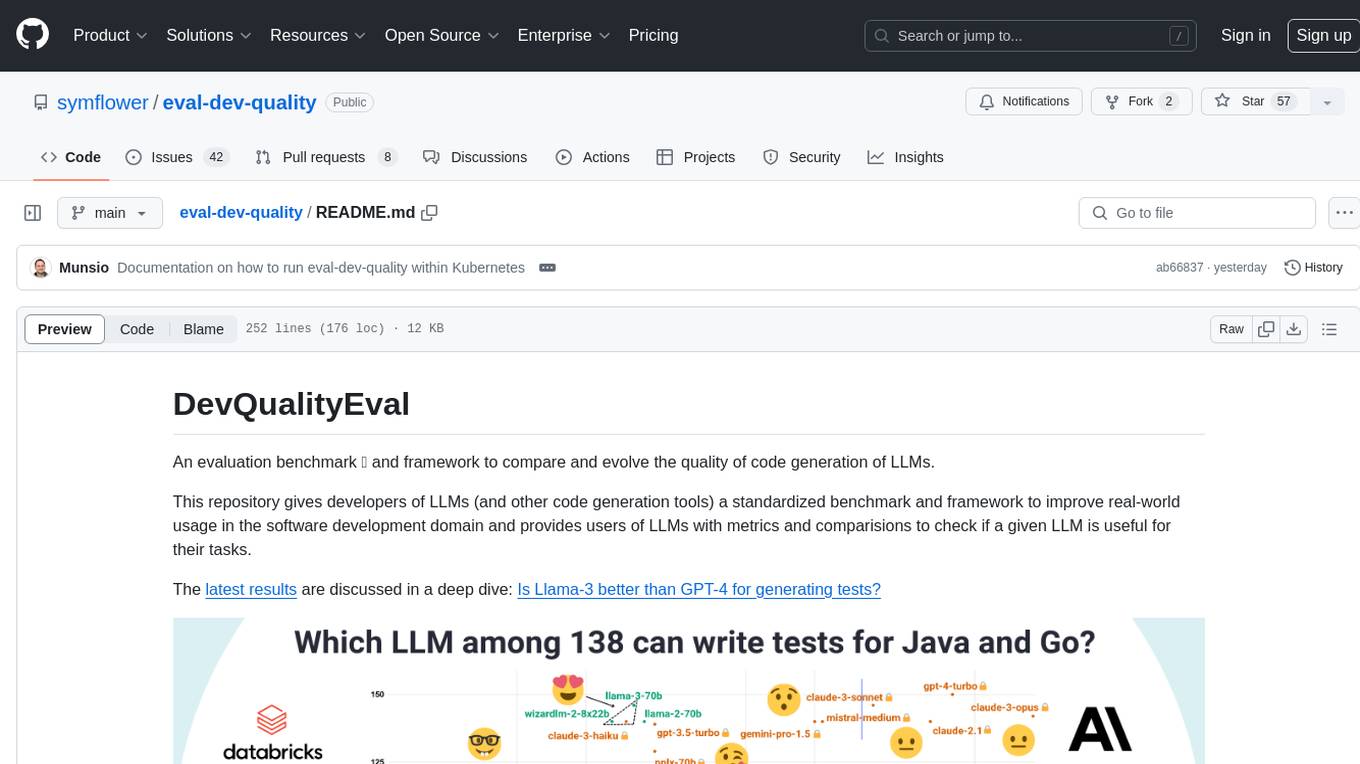
eval-dev-quality
DevQualityEval is an evaluation benchmark and framework designed to compare and improve the quality of code generation of Language Model Models (LLMs). It provides developers with a standardized benchmark to enhance real-world usage in software development and offers users metrics and comparisons to assess the usefulness of LLMs for their tasks. The tool evaluates LLMs' performance in solving software development tasks and measures the quality of their results through a point-based system. Users can run specific tasks, such as test generation, across different programming languages to evaluate LLMs' language understanding and code generation capabilities.
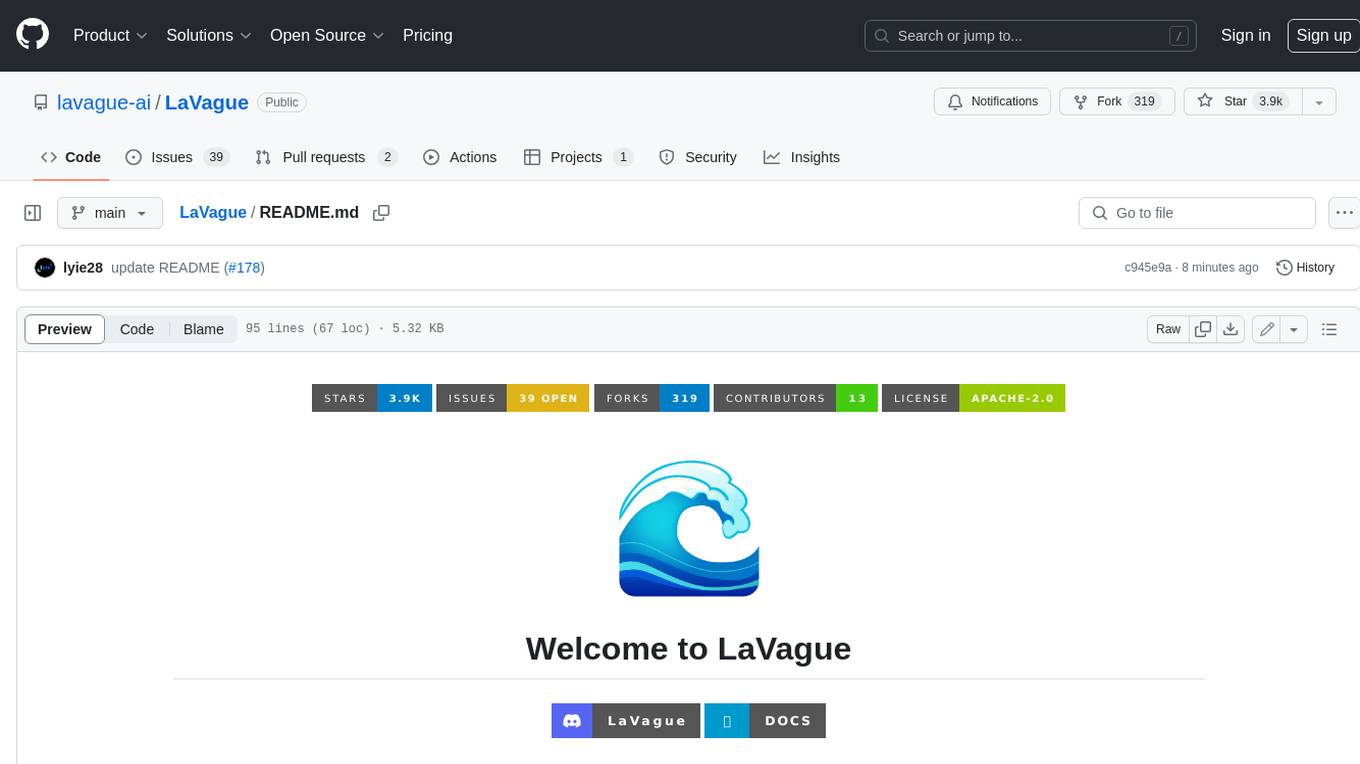
LaVague
LaVague is an open-source Large Action Model framework that uses advanced AI techniques to compile natural language instructions into browser automation code. It leverages Selenium or Playwright for browser actions. Users can interact with LaVague through an interactive Gradio interface to automate web interactions. The tool requires an OpenAI API key for default examples and offers a Playwright integration guide. Contributors can help by working on outlined tasks, submitting PRs, and engaging with the community on Discord. The project roadmap is available to track progress, but users should exercise caution when executing LLM-generated code using 'exec'.
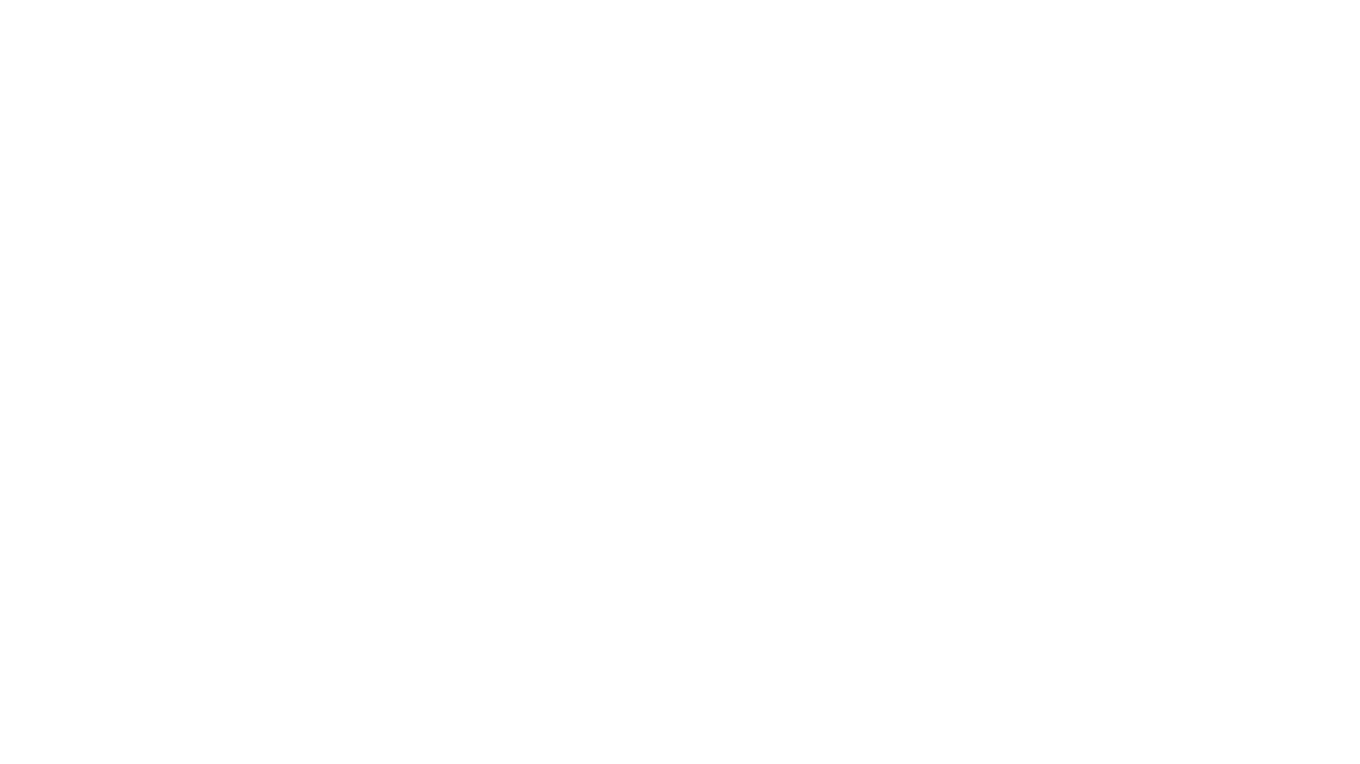
DocsGPT
DocsGPT is an open-source documentation assistant powered by GPT models. It simplifies the process of searching for information in project documentation by allowing developers to ask questions and receive accurate answers. With DocsGPT, users can say goodbye to manual searches and quickly find the information they need. The tool aims to revolutionize project documentation experiences and offers features like live previews, Discord community, guides, and contribution opportunities. It consists of a Flask app, Chrome extension, similarity search index creation script, and a frontend built with Vite and React. Users can quickly get started with DocsGPT by following the provided setup instructions and can contribute to its development by following the guidelines in the CONTRIBUTING.md file. The project follows a Code of Conduct to ensure a harassment-free community environment for all participants. DocsGPT is licensed under MIT and is built with LangChain.
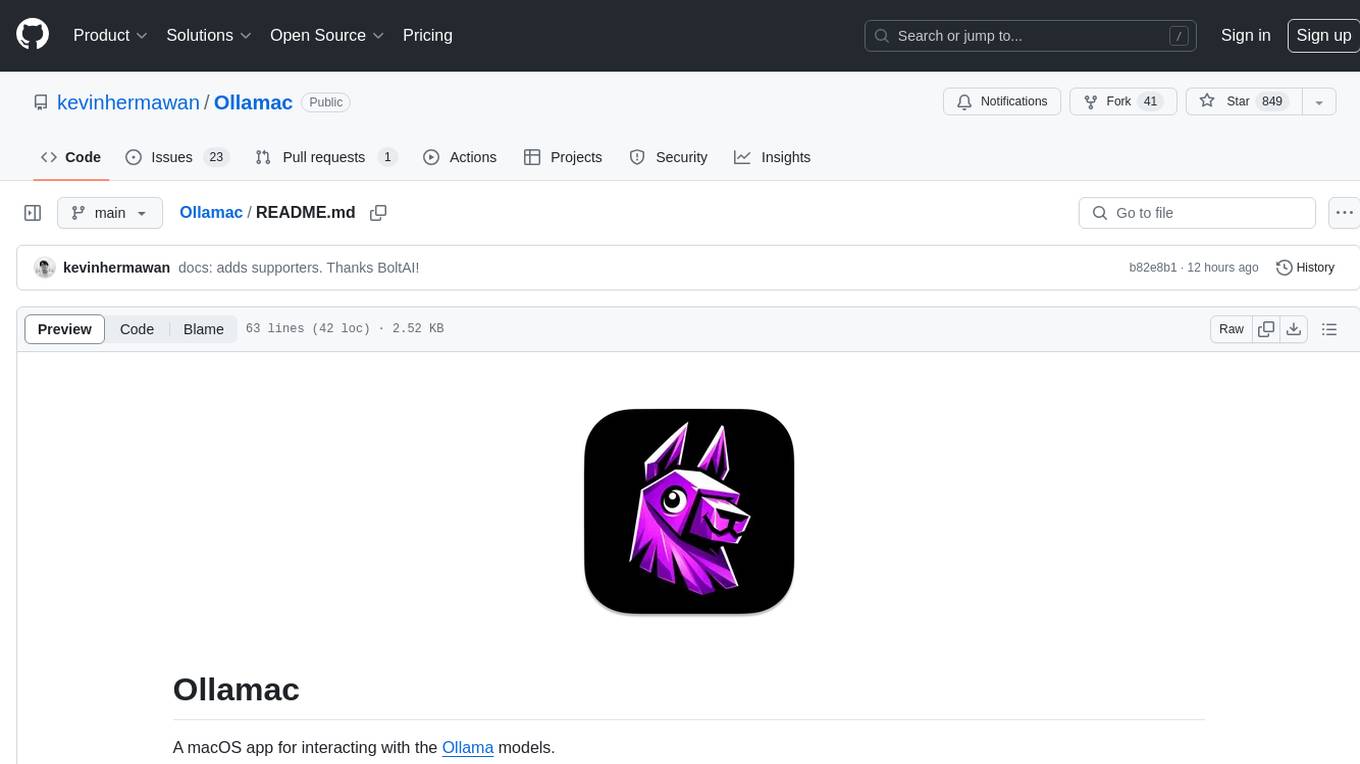
Ollamac
Ollamac is a macOS app designed for interacting with Ollama models. It is optimized for macOS, allowing users to easily use any model from the Ollama library. The app features a user-friendly interface, chat archive for saving interactions, and real-time communication using HTTP streaming technology. Ollamac is open-source, enabling users to contribute to its development and enhance its capabilities. It requires macOS 14 or later and the Ollama system to be installed on the user's Mac with at least one Ollama model downloaded.
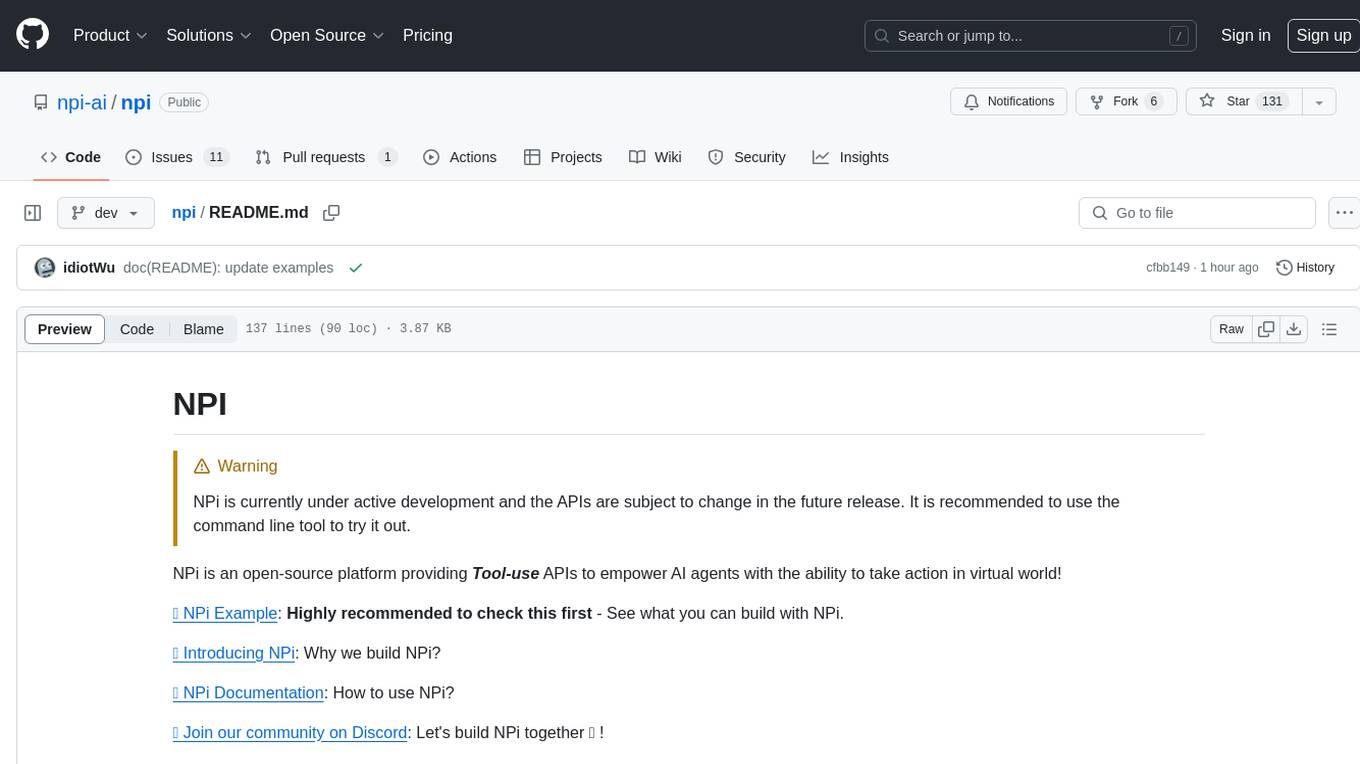
npi
NPi is an open-source platform providing Tool-use APIs to empower AI agents with the ability to take action in the virtual world. It is currently under active development, and the APIs are subject to change in future releases. NPi offers a command line tool for installation and setup, along with a GitHub app for easy access to repositories. The platform also includes a Python SDK and examples like Calendar Negotiator and Twitter Crawler. Join the NPi community on Discord to contribute to the development and explore the roadmap for future enhancements.
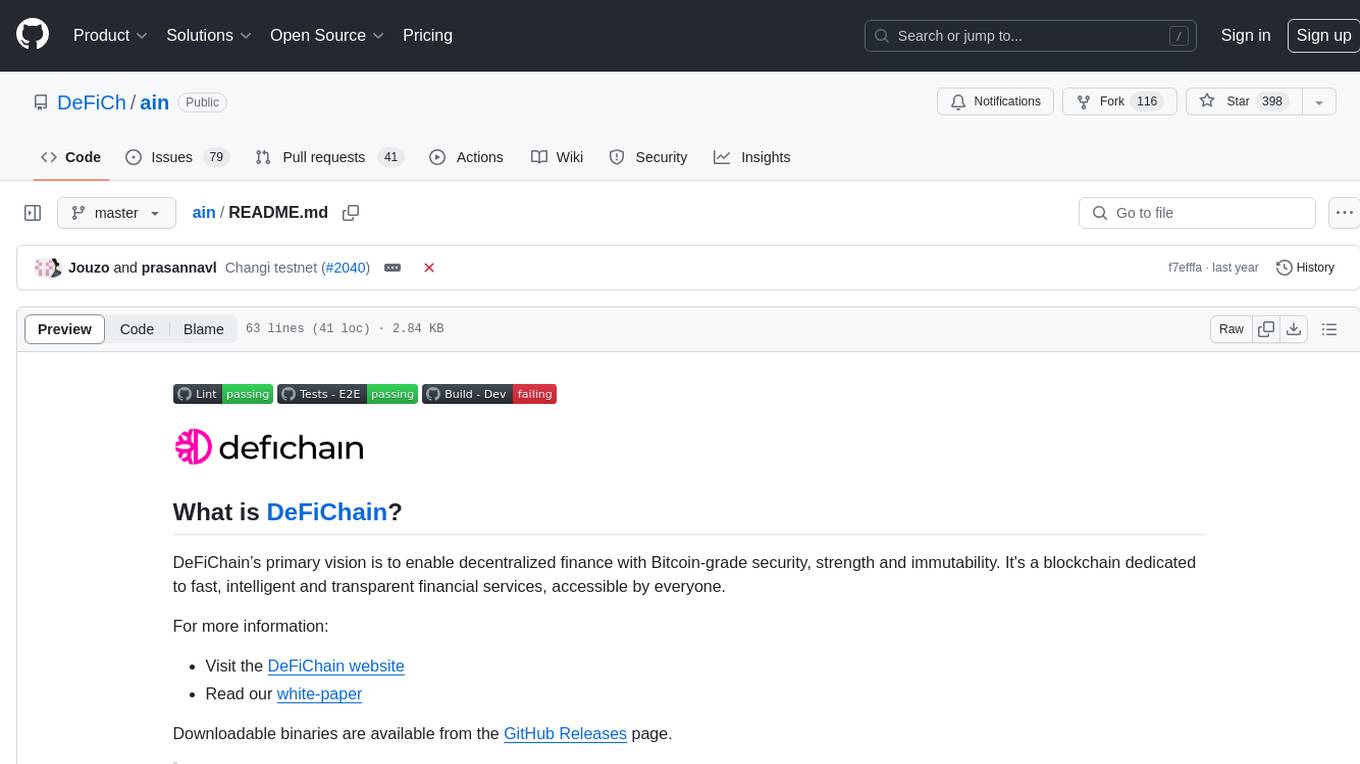
ain
DeFiChain is a blockchain platform dedicated to enabling decentralized finance with Bitcoin-grade security, strength, and immutability. It offers fast, intelligent, and transparent financial services accessible to everyone. DeFiChain has made significant modifications from Bitcoin Core, including moving to Proof-of-Stake, introducing a masternode model, supporting a community fund, anchoring to the Bitcoin blockchain, and enhancing decentralized financial transaction and opcode support. The platform is under active development with regular releases and contributions are welcomed.
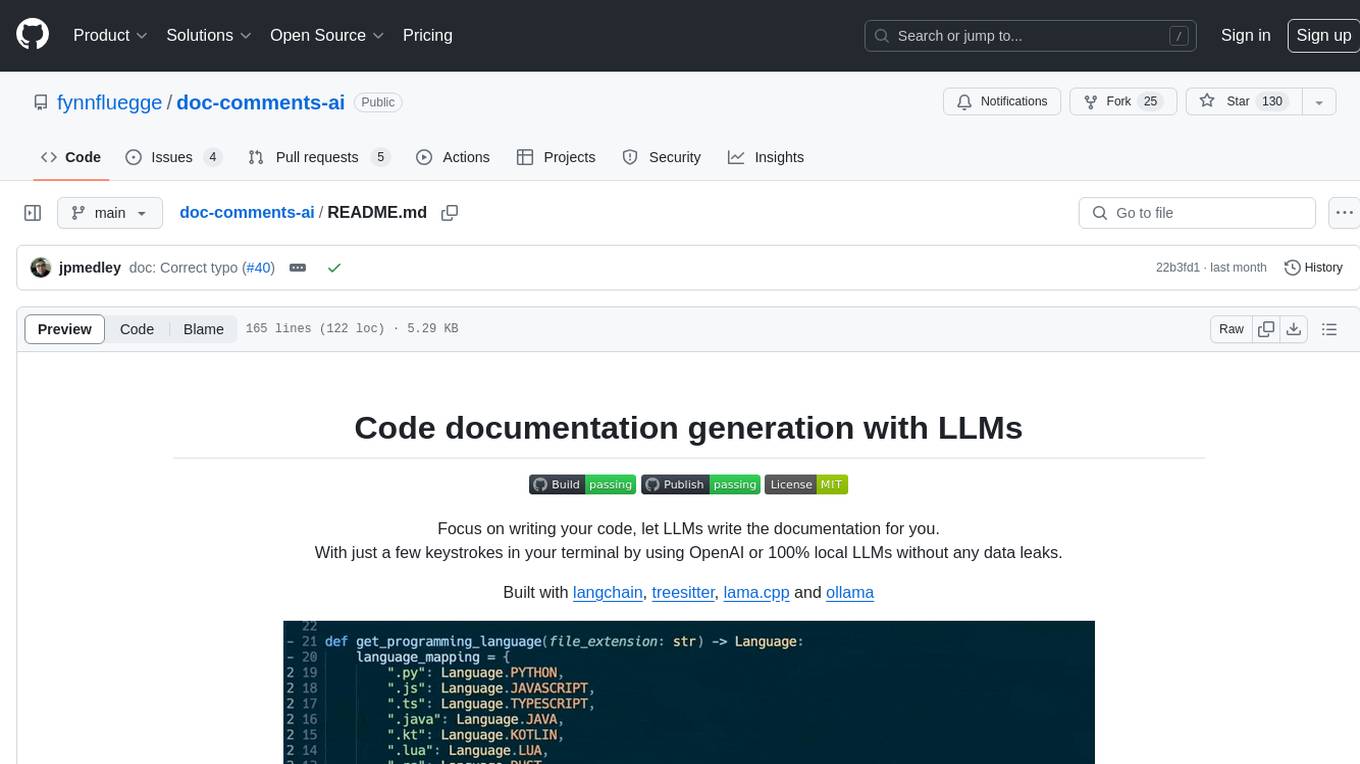
doc-comments-ai
doc-comments-ai is a tool designed to automatically generate code documentation using language models. It allows users to easily create documentation comment blocks for methods in various programming languages such as Python, Typescript, Javascript, Java, Rust, and more. The tool supports both OpenAI and local LLMs, ensuring data privacy and security. Users can generate documentation comments for methods in files, inline comments in method bodies, and choose from different models like GPT-3.5-Turbo, GPT-4, and Azure OpenAI. Additionally, the tool provides support for Treesitter integration and offers guidance on selecting the appropriate model for comprehensive documentation needs.
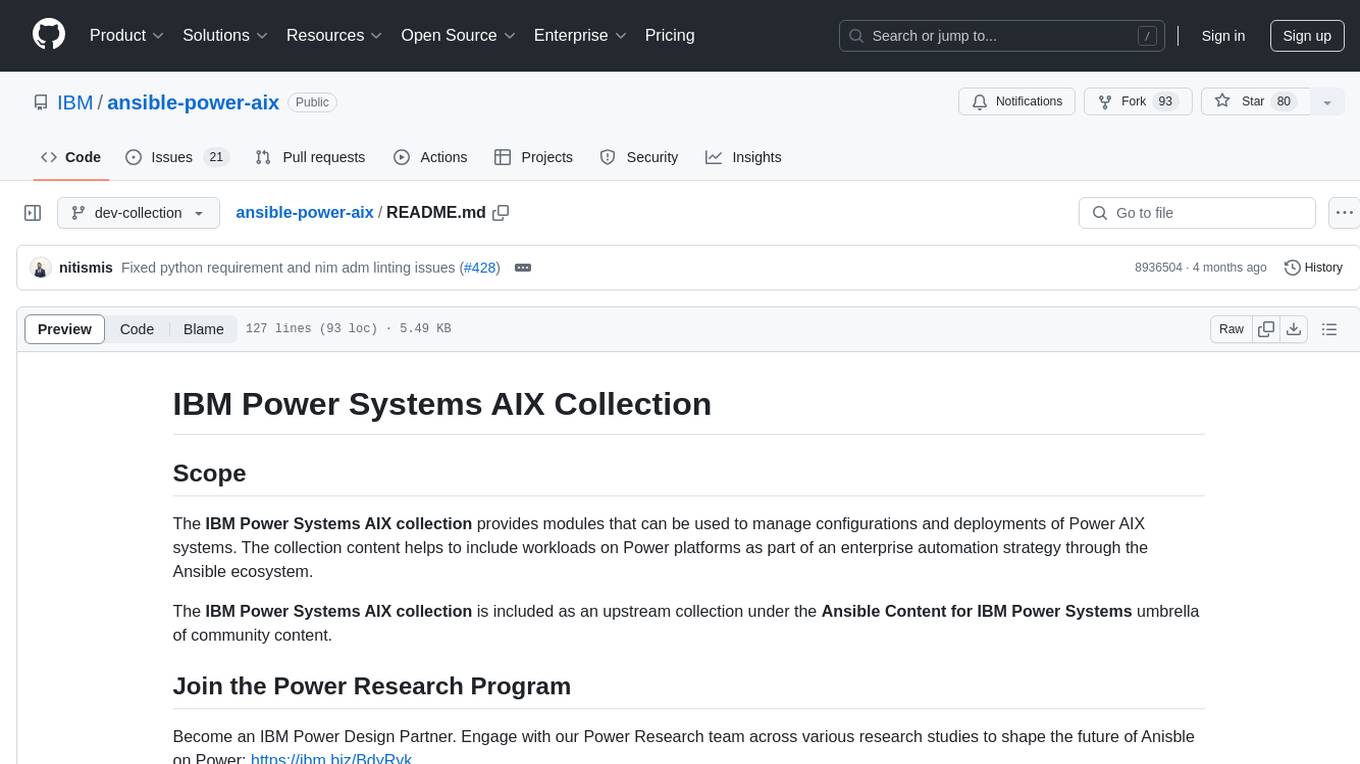
ansible-power-aix
The IBM Power Systems AIX Collection provides modules to manage configurations and deployments of Power AIX systems, enabling workloads on Power platforms as part of an enterprise automation strategy through the Ansible ecosystem. It includes example best practices, requirements for AIX versions, Ansible, and Python, along with resources for documentation and contribution.
For similar jobs
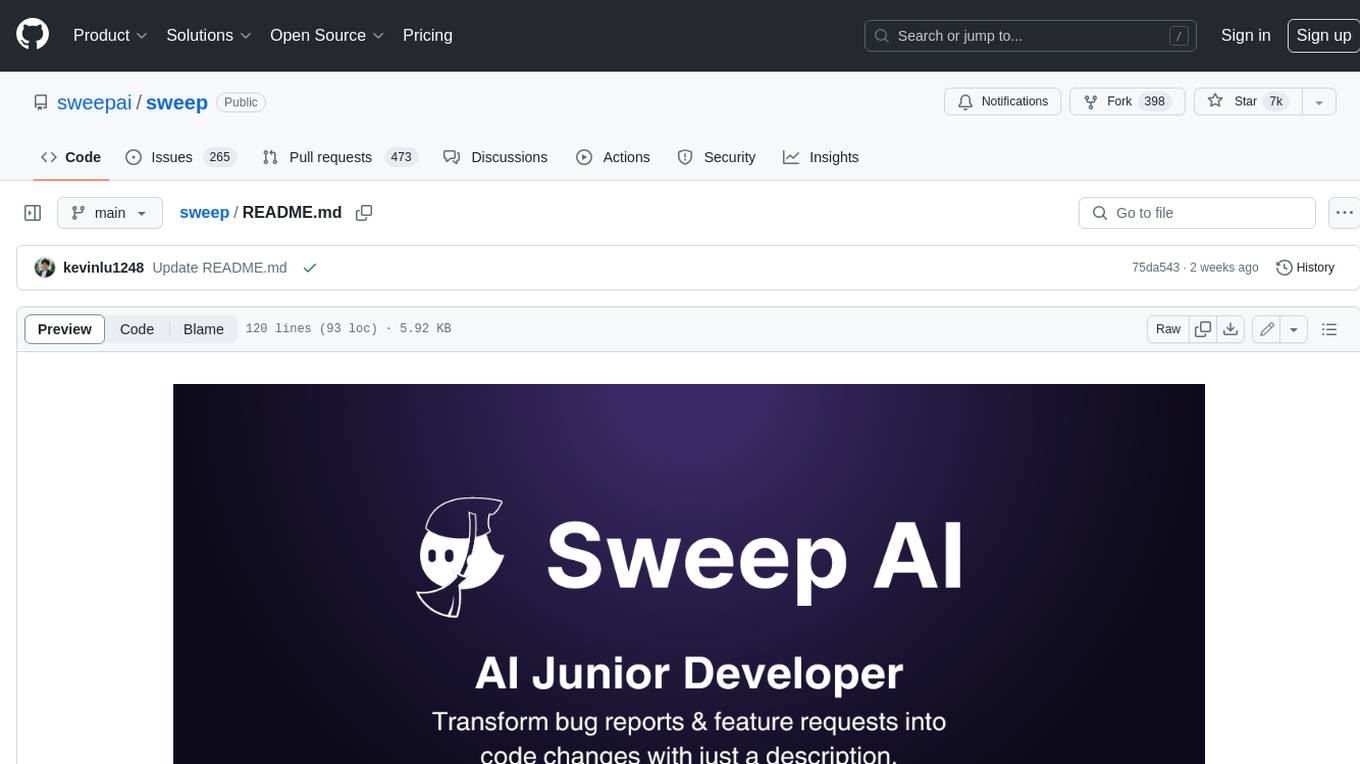
sweep
Sweep is an AI junior developer that turns bugs and feature requests into code changes. It automatically handles developer experience improvements like adding type hints and improving test coverage.
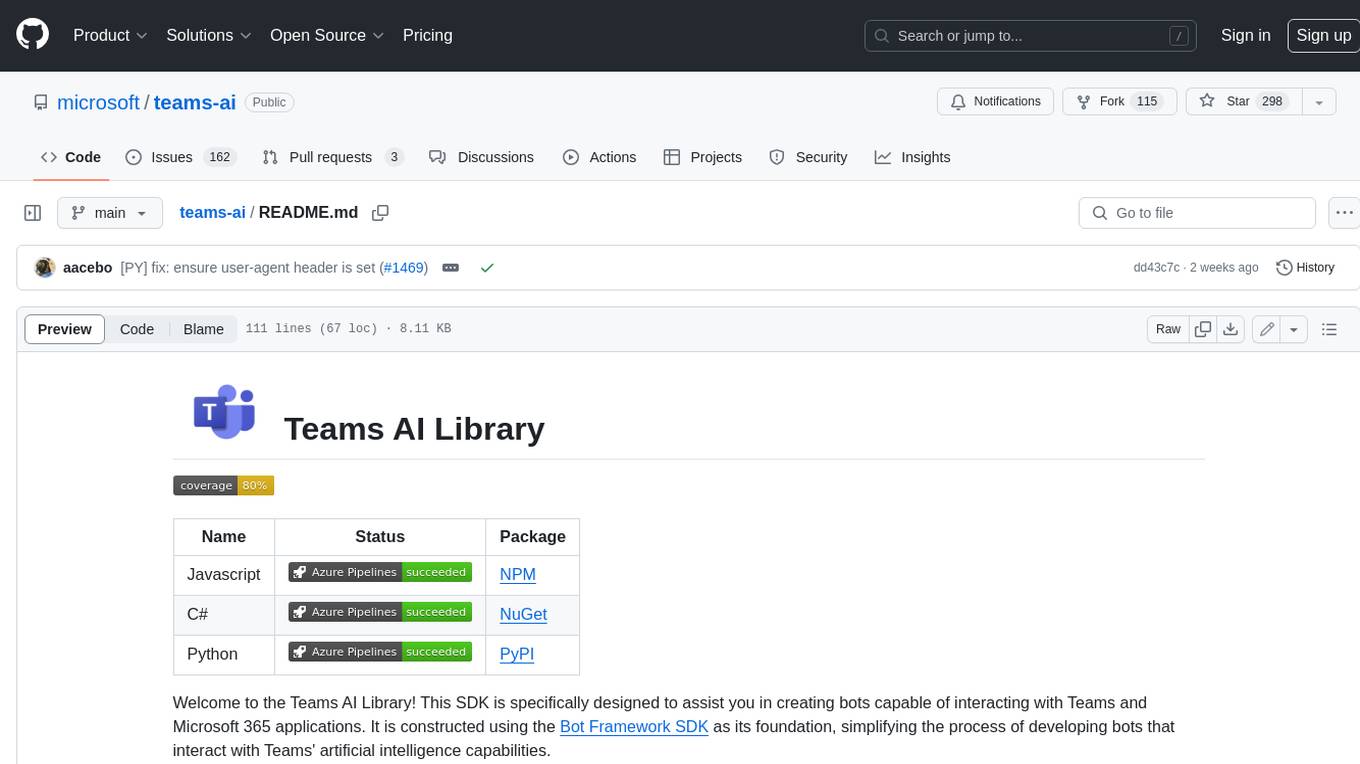
teams-ai
The Teams AI Library is a software development kit (SDK) that helps developers create bots that can interact with Teams and Microsoft 365 applications. It is built on top of the Bot Framework SDK and simplifies the process of developing bots that interact with Teams' artificial intelligence capabilities. The SDK is available for JavaScript/TypeScript, .NET, and Python.
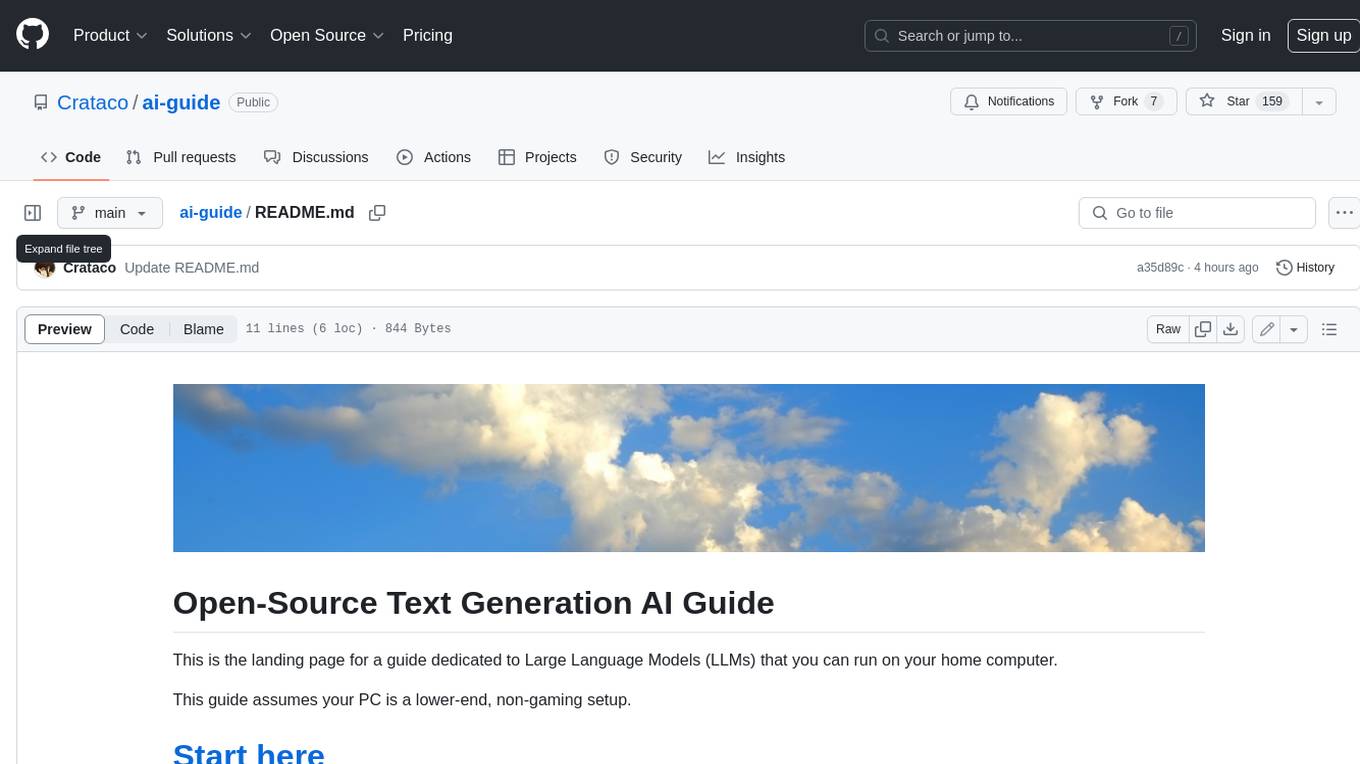
ai-guide
This guide is dedicated to Large Language Models (LLMs) that you can run on your home computer. It assumes your PC is a lower-end, non-gaming setup.
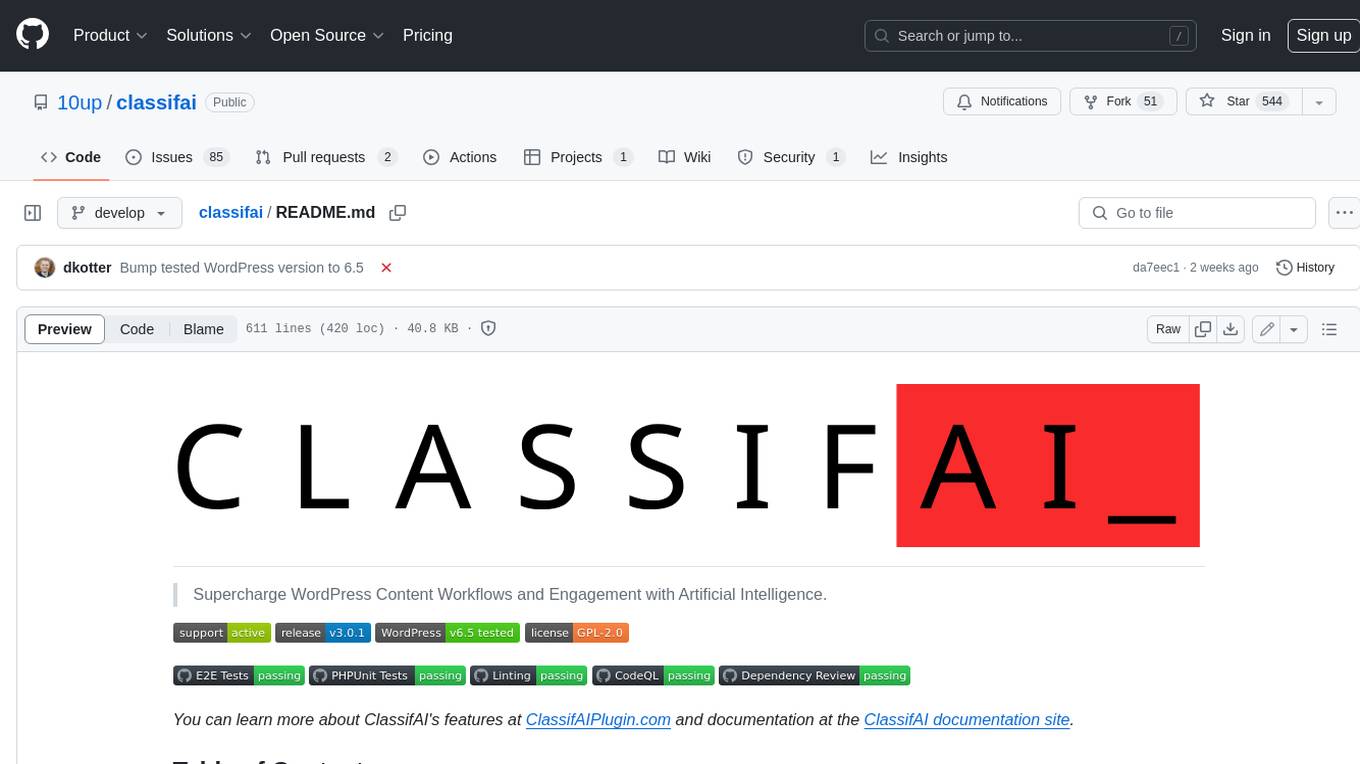
classifai
Supercharge WordPress Content Workflows and Engagement with Artificial Intelligence. Tap into leading cloud-based services like OpenAI, Microsoft Azure AI, Google Gemini and IBM Watson to augment your WordPress-powered websites. Publish content faster while improving SEO performance and increasing audience engagement. ClassifAI integrates Artificial Intelligence and Machine Learning technologies to lighten your workload and eliminate tedious tasks, giving you more time to create original content that matters.
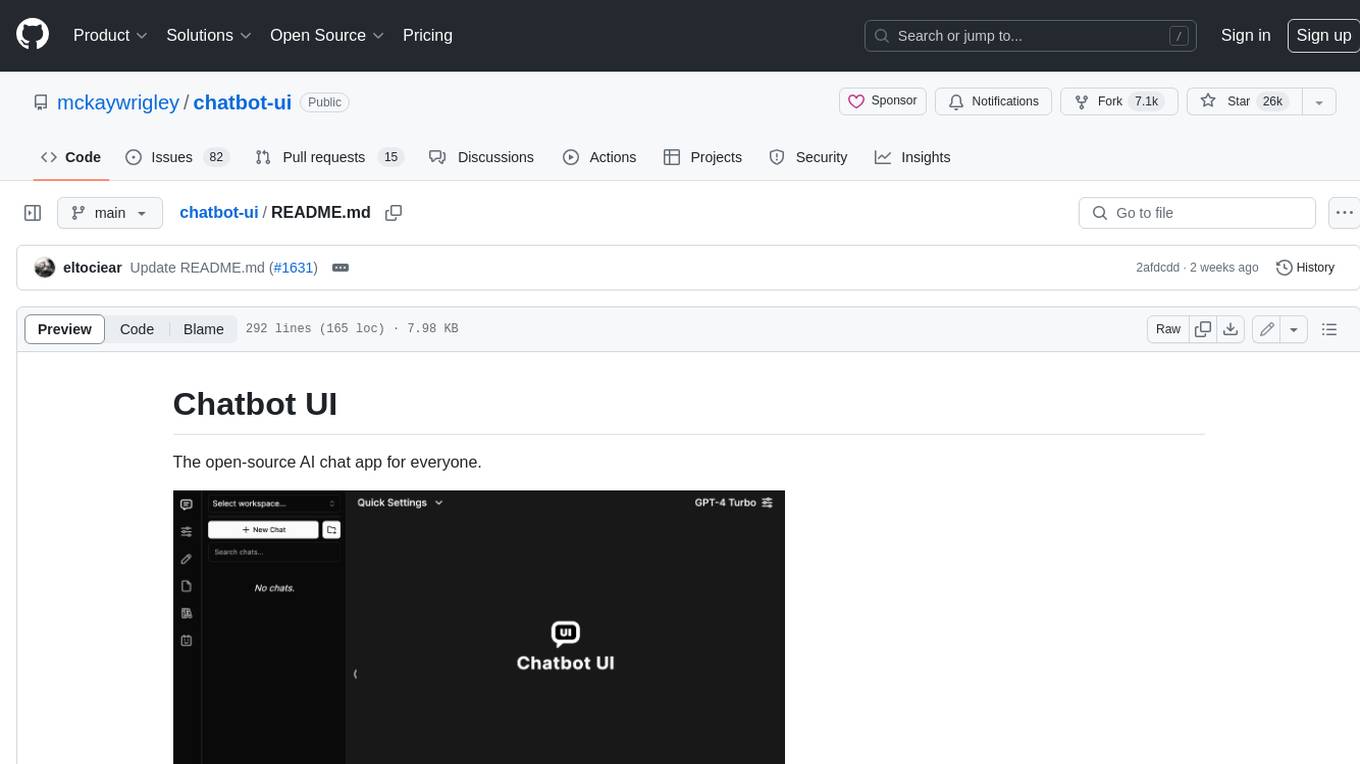
chatbot-ui
Chatbot UI is an open-source AI chat app that allows users to create and deploy their own AI chatbots. It is easy to use and can be customized to fit any need. Chatbot UI is perfect for businesses, developers, and anyone who wants to create a chatbot.
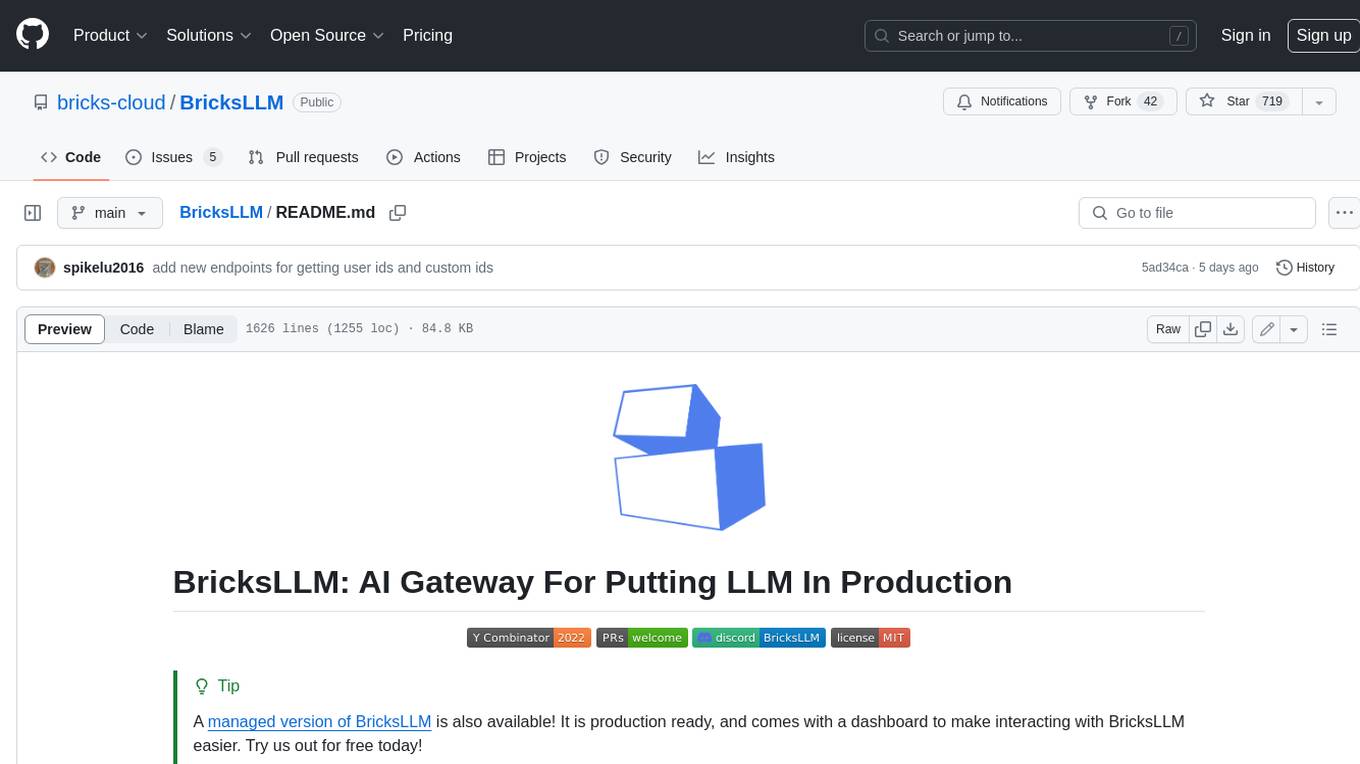
BricksLLM
BricksLLM is a cloud native AI gateway written in Go. Currently, it provides native support for OpenAI, Anthropic, Azure OpenAI and vLLM. BricksLLM aims to provide enterprise level infrastructure that can power any LLM production use cases. Here are some use cases for BricksLLM: * Set LLM usage limits for users on different pricing tiers * Track LLM usage on a per user and per organization basis * Block or redact requests containing PIIs * Improve LLM reliability with failovers, retries and caching * Distribute API keys with rate limits and cost limits for internal development/production use cases * Distribute API keys with rate limits and cost limits for students
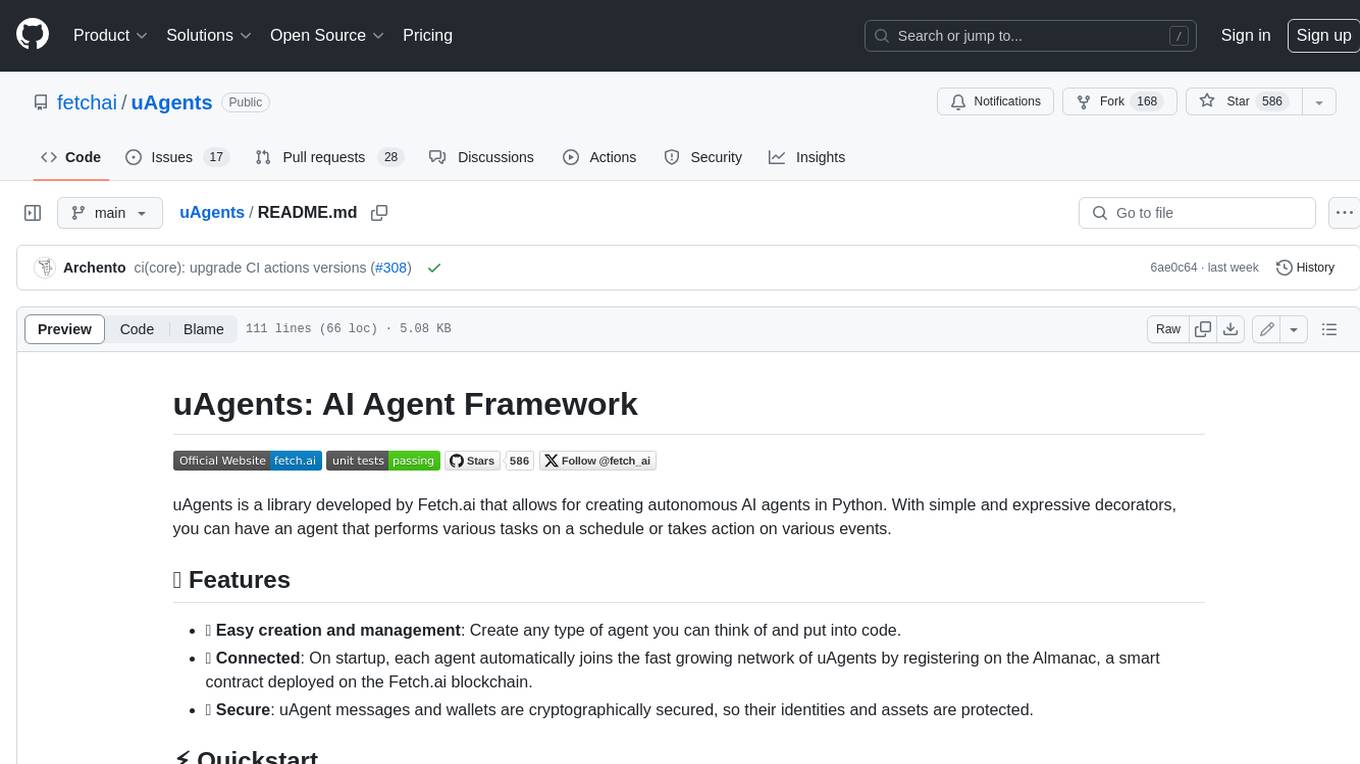
uAgents
uAgents is a Python library developed by Fetch.ai that allows for the creation of autonomous AI agents. These agents can perform various tasks on a schedule or take action on various events. uAgents are easy to create and manage, and they are connected to a fast-growing network of other uAgents. They are also secure, with cryptographically secured messages and wallets.
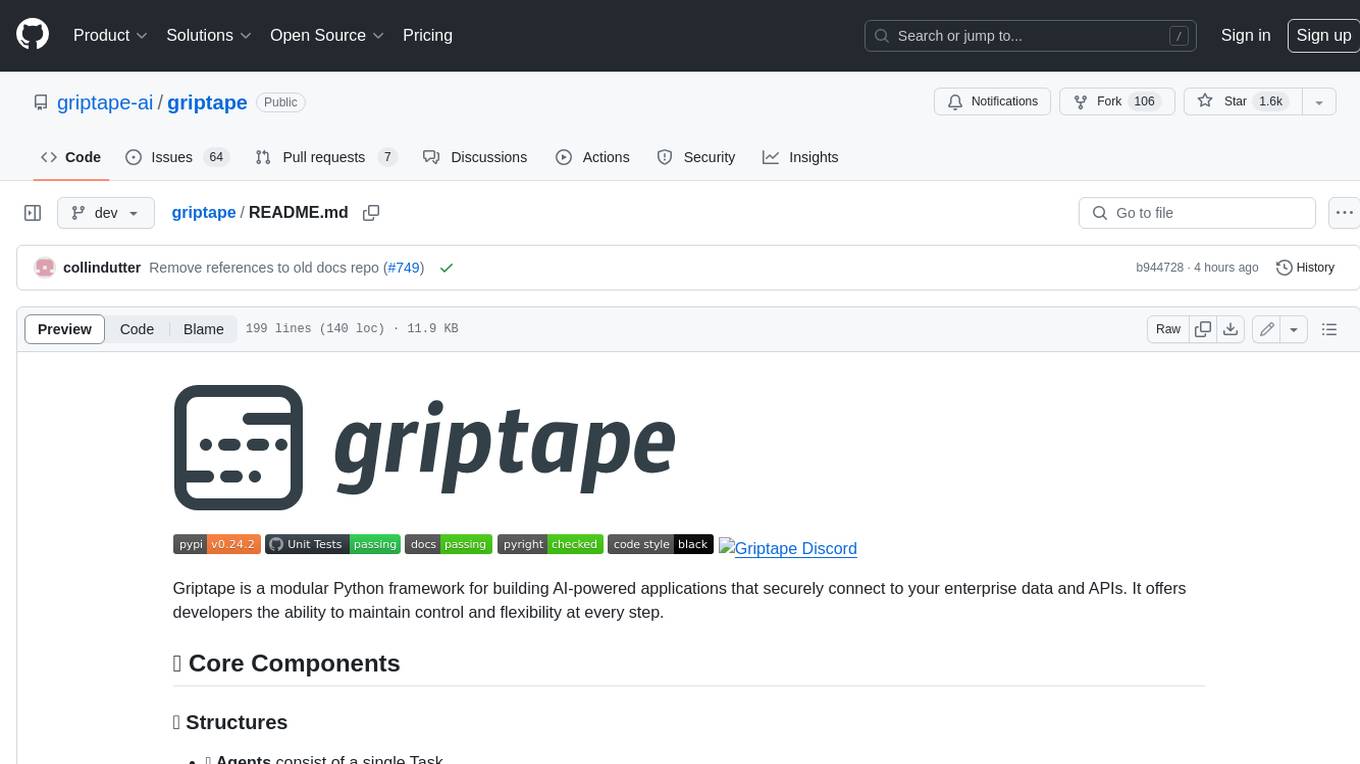
griptape
Griptape is a modular Python framework for building AI-powered applications that securely connect to your enterprise data and APIs. It offers developers the ability to maintain control and flexibility at every step. Griptape's core components include Structures (Agents, Pipelines, and Workflows), Tasks, Tools, Memory (Conversation Memory, Task Memory, and Meta Memory), Drivers (Prompt and Embedding Drivers, Vector Store Drivers, Image Generation Drivers, Image Query Drivers, SQL Drivers, Web Scraper Drivers, and Conversation Memory Drivers), Engines (Query Engines, Extraction Engines, Summary Engines, Image Generation Engines, and Image Query Engines), and additional components (Rulesets, Loaders, Artifacts, Chunkers, and Tokenizers). Griptape enables developers to create AI-powered applications with ease and efficiency.