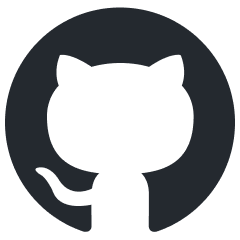
2p-kt
A Kotlin Multi-Platform ecosystem for symbolic AI
Stars: 86
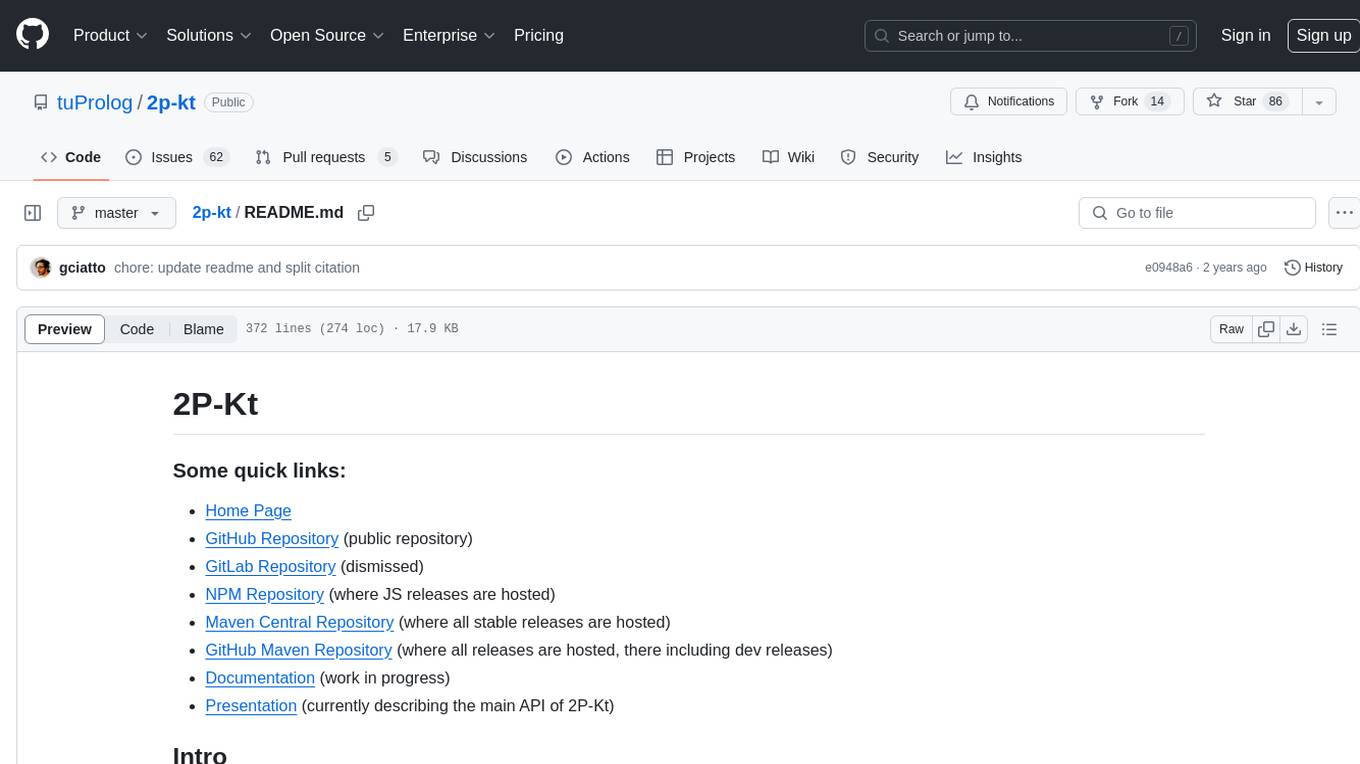
2P-Kt is a Kotlin-based and multi-platform reboot of tuProlog (2P), a multi-paradigm logic programming framework written in Java. It consists of an open ecosystem for Symbolic Artificial Intelligence (AI) with modules supporting logic terms, unification, indexing, resolution of logic queries, probabilistic logic programming, binary decision diagrams, OR-concurrent resolution, DSL for logic programming, parsing modules, serialisation modules, command-line interface, and graphical user interface. The tool is designed to support knowledge representation and automatic reasoning through logic programming in an extensible and flexible way, encouraging extensions towards other symbolic AI systems than Prolog. It is a pure, multi-platform Kotlin project supporting JVM, JS, Android, and Native platforms, with a lightweight library leveraging the Kotlin common library.
README:
- Home Page
- GitHub Repository (public repository)
- GitLab Repository (dismissed)
- NPM Repository (where JS releases are hosted)
- Maven Central Repository (where all stable releases are hosted)
- GitHub Maven Repository (where all releases are hosted, there including dev releases)
- Documentation (work in progress)
- Presentation (currently describing the main API of 2P-Kt)
tuProlog (2P henceforth) is a multi-paradigm logic programming framework written in Java.
2P-Kt is a Kotlin-based and multi-platform reboot of 2P. It consists of an open ecosystem for Symbolic Artificial Intelligence (AI). For this reason, 2P-Kt consists of a number of incrementally inter-dependent modules aimed at supporting symbolic manipulation and reasoning in an extensible and flexible way.
A complete overview about modules and their dependencies is provided by the following diagram:
As shown in the project map, 2P-Kt currently focuses on supporting knowledge representation and automatic reasoning through logic programming, by featuring:
-
a module for logic terms and clauses representation, namely
core
, -
a module for logic unification representation, namely
unify
, -
a module for in-memory indexing and storing logic theories, as well as other sorts of collections of logic clauses, namely
theory
, -
a module providing generic API for resolution of logic queries, namely
solve
, coming with several implementations (e.g.solve-classic
andsolve-streams
, targetting Prolog ISO Standard compliant resolution), -
a module providing generic API for the probabilistic resolution of logic queries via probabilistic logic programming (PLP), namely
solve-plp
, coming with an implementation targetting ProbLog (solve-problog
)- leveraging on module
:bdd
, which provides a multi-platform implementation of binary decision diagrams (BDD)
- leveraging on module
-
a module providing OR-concurrent resolution facilities, namely
solve-concurrent
, -
a number of modules (i.e., the many
dsl-*
modules) supporting a Prolog-like, Domain Specific Language (DSL) aimed at bridging the logic programming with the Kotlin object-oriented & functional environment,- further details are provided in this paper
-
two parsing modules: one aimed at parsing terms, namely
parser-core
, and the other aimed at parsing theories, namelyparser-theory
, -
two serialisation-related modules: one aimed at (de)serialising terms and clauses, namely
serialize-core
, and the other aimed at (de)serialising terms theories, namelyserialize-theory
, -
a module for using Prolog via a command-line interface, namely
repl
, -
a module for using Prolog via a graphical user interface (GUI), namely
ide
, -
a module for using PLP (and, in particular, ProbLob) via a GUI, namely
ide-plp
.
The modular, unopinionated architecture of 2P-Kt is deliberately aimed at supporting and encouraging extensions towards other sorts of symbolic AI systems than Prolog---such as ASP, tabled-Prolog, concurrent LP, etc.
Furthermore, 2P-Kt is developed as in pure, multi-platform Kotlin project. This brings two immediate advantages:
- it virtually supports several platforms, there including JVM, JS, Android, and Native (even if, currently, only JVM, JS and Android are supported),
- it consists of a very minimal and lightweight library, only leveraging on the Kotlin common library, as it cannot commit to any particular platform standard library.
2P-Kt can either be used as a command-line program or as a Kotlin, JVM, Android, or JS library.
The 2P-Kt executables are currently available for download on the Releases section of the GitHub repository.
The 2P-Kt modules for JVM, Android, or Kotlin users are currently available for import
on Maven Central, under the it.unibo.tuprolog
group ID (not
to be confused with the it.unibo.alice.tuprolog
, which contains the old Java-based implementation).
The same modules are available through an ad-hoc Maven repository as well,
hosted by GitHub.
The 2P-Kt modules for JS users, are available for import on NPM, under the @tuprolog
organization.
If you need a GUI for your Prolog interpreter, you can rely on the 2P-Kt IDE which is available on the Releases section of the GitHub repository.
The page of the latest release of 2P-Kt exposes a number of Assets. There, the one named:
2p-ide-VERSION-redist.jar
is the self-contained, executable Jar containing the 2P-Kt-based Prolog interpreter (VERSION
may vary depending on the
actual release version).
After you download the 2p-ide-VERSION-redist.jar
, you can simply launch it by running:
java -jar 2p-ide-VERSION-redist.jar
However, if you have properly configured the JVM on your system, it may be sufficient to just double-click on the aforementioned JAR to start the IDE. In any case, running the JAR should make the following window appear:
There, one may query the 2P-Kt Prolog interpreter against the currently opened theory file, which can of course be loaded from the user's file system by pressing File and then Open....
To issue a query, the user must write it in the query text field, at the center of the application. By either pressing Enter while the cursor is on the query text field, or by clicking on the Solve (resp. Solve all) button, the user can start a new resolution process, aimed at solving the provided query. Further solutions can be explored by clicking on the Next (resp. All next) button over and over again. The Next (resp. All next) button shall appear in place of Solve (resp. Solve all) if and when further solutions are available for the current query.
One may also compute all the unexplored solutions at once by clicking on the Solve all (resp. All next) button. Avoid this option in case of your query is expected to compute an unlimited amount of solutions.
To perform a novel query, they user may either:
- write the new query in the query text field, and then press Enter, or
- click on the Stop button, write the new query in the query text field, and then press the Solve (resp. SolveNext) button again.
The Reset button cleans up the status of the solver, clearing any side effect possibly provoked by previous queries (including assertions, retractions, prints, warnings, loading of libraries, operators, or flags).
Finally, users may inspect the current status of the solver by leveraging the many tabs laying at the bottom of the IDE. There,
- the Solutions tab is aimed at showing the Prolog interpreter's answers to the user's queries;
- the Stdin tab is aimed at letting the user provide some text the Prolog interpreter's standard input stream;
- the Stdout tab is aimed at showing the Prolog interpreter's standard output stream;
- the Stderr tab is aimed at showing the Prolog interpreter's standard error stream;
- the Warnings tab is aimed at showing any warning possibly generated by the Prolog interpreter while computing;
- the Operators tab is aimed at showing the current content Prolog interpreter's operator table;
- the Flags tab is aimed showing the actual values of all the flags currently defined with the Prolog interpreter;
- the Libraries tab is aimed at letting the user inspect the currently loaded libraries and the predicates, operators, and functions they import;
- the Static (resp. Dynamic) KB tab is aimed at letting the user inspect the current content of the Prolog interpreter's static (resp. dynamic) knowledge base.
Any of these tabs may be automatically updated after a solution to some query is computed. Whenever something changes w.r.t. the previous content of the tab, an asterisk will appear close to the tab name, to notify an update in that tab.
If you just need a command-line Prolog interpreter, you can rely on the 2P-Kt REPL which is available on the Releases section of the GitHub repository.
The page of the latest release of 2P-Kt exposes a number of Assets. There, the one named:
2p-repl-VERSION-redist.jar
is the self-contained, executable Jar containing the 2P-Kt-based Prolog interpreter (VERSION
may vary depending on the
actual release version).
After you download the 2p-repl-VERSION-redist.jar
, you can simply launch it by running:
java -jar 2p-repl-VERSION-redist.jar
This should start an interactive read-eval-print loop accepting Prolog queries. A normal output should be as follows:
# 2P-Kt version LAST_VERSION_HERE
?- <write your dot-terminated Prolog query here>.
For instance:
Other options or modes of execution are supported. One can explore them via the program help, which can be displayed by running:
java -jar 2p-repl-VERSION-redist.jar --help
This should display a message similar to the following one:
Usage: java -jar 2p-repl.jar [OPTIONS] COMMAND [ARGS]...
Start a Prolog Read-Eval-Print loop
Options:
-T, --theory TEXT Path of theory file to be loaded
-t, --timeout INT Maximum amount of time for computing a solution (default:
1000 ms)
--oop Loads the OOP library
-h, --help Show this message and exit
Commands:
solve Compute a particular query and then terminate
To import the 2P-Kt module named 2P_MODULE
(version 2P_VERSION
) into your Kotlin-based project leveraging on Gradle,
you simply need to declare the corresponding dependency in your build.gradle(.kts)
file:
// assumes Gradle's Kotlin DSL
dependencies {
implementation("it.unibo.tuprolog", "2P_MODULE", "2P_VERSION")
}
In this way, the dependencies of 2P_MODULE
should be automatically imported.
The step above, requires you to tell Gradle to either use Maven Central or our GitHub repository (or both) as a source for dependency lookup. You can do it as follows:
// assumes Gradle's Kotlin DSL
repositories {
maven("https://maven.pkg.github.com/tuProlog/2p-kt")
mavenCentral()
}
Authentication may be required in case the GitHub repository is exploited
Remember to add the -jvm
suffix to 2P_MODULE
in case your project only targets the JVM platform:
// assumes Gradle's Kotlin DSL
dependencies {
implementation("it.unibo.tuprolog", "2P_MODULE-jvm", "2P_VERSION")
}
To import the 2P-Kt module named 2P_MODULE
(version 2P_VERSION
) into your Kotlin-based project leveraging on Maven,
you simply need to declare the corresponding dependency in your pom.xml
file:
<dependency>
<groupId>it.unibo.tuprolog</groupId>
<artifactId>2P_MODULE</artifactId>
<version>2P_VERSION</version>
</dependency>
In this way, the dependencies of 2P_MODULE
should be automatically imported.
The step above, requires you to tell Maven to either use Maven Central or our GitHub repository (or both) as a source for dependency lookup. You can do it as follows:
<repositories>
<repository>
<id>github-2p-repo</id>
<url>https://maven.pkg.github.com/tuProlog/2p-kt</url>
</repository>
</repositories>
Authentication may be required in case the GitHub repository is exploited
Remember to add the -jvm
suffix to 2P_MODULE
in case your project only targets the JVM platform:
<dependency>
<groupId>it.unibo.tuprolog</groupId>
<artifactId>2P_MODULE-jvm</artifactId>
<version>2P_VERSION</version>
</dependency>
The 2P-Kt software is available as a JavaScript library as well, on NPM, under the @tuprolog
organization.
To import the 2P_MODULE
into your package.json
, it is sufficient to declare your dependency as follows:
{
"dependencies": {
"@tuprolog/2P_MODULE": "^2P_MODULE_VERSION"
}
}
Notice that the JS dependencies of 2P_MODULE
should be automatically imported.
Working with the 2P-Kt codebase requires a number of tools to be installed and properly configured on your system:
- JDK 11+ (please ensure the
JAVA_HOME
environment variable is properly) configured - Kotlin 1.5.10+
- Gradle 7.1+ (please ensure the
GRADLE_HOME
environment variable is properly configured) - Git 2.20+
To participate in the development of 2P-Kt, we suggest the IntelliJ Idea IDE. The free, Community version will be fine.
You will need the Kotlin plugin for IntelliJ Idea. This is usually installed upon Idea's very first setup wizard. However, one may easily late-install such plugin through the IDE's Plugins settings dialog. To open such dialog, use Ctrl+Shift+A, then search for "Plugins"
-
Clone this repository in a folder of your preference using
git clone
appropriately -
Open IntellJ Idea. If a project opens automatically, select "Close project". You should be on the welcome screen of IntelliJ idea, with an aspect similar to this image:
-
Select "Import Project"
-
Navigate your file system and find the folder where you cloned the repository. Do not select it. Open the folder, and you should find a lowercase
2p-in-kotlin
folder. That is the correct project folder, created bygit
in case you cloned without specifying a different folder name. Once the correct folder has been selected, click Ok -
Select "Import Project from external model"
-
Make sure "Gradle" is selected as external model tool
-
Click Finish
-
If prompted to override any
.idea
file, try to answer No. It's possible that IntelliJ refuses to proceed, in which case click Finish again, then select Yes -
A dialog stating that "IntelliJ IDEA found a Gradle build script" may appear, in such case answer Import Gradle Project
-
Wait for the IDE to import the project from Gradle. The process may take several minutes, due to the amount of dependencies. Should the synchronization fail, make sure that the IDE's Gradle is configured correctly:
-
In 'Settings -> Build, Execution, Deployment -> Build Tools > Gradle', for the option 'Use Gradle from' select 'gradle-wrapper.properties file'. Enabling auto-import is also recommended
Contributions to this project are welcome. Just some rules:
-
We use git flow, so if you write new features, please do so in a separate
feature/
branch -
We recommend forking the project, developing your stuff, then contributing back via pull request directly from the Web interface
-
Commit often. Do not throw pull requests with a single giant commit adding or changing the whole world. Split it in multiple commits and request a merge to the mainline often
-
Stay in sync with the
develop
branch: pull often fromdevelop
(if the build passes), so that you don't diverge too much from the main development line -
Do not introduce low quality or untested code. Merge requests will be reviewed before merge.
While developing, you can rely on IntelliJ to build the project, it will generally do a very good job. If you want to generate the artifacts, you can rely on Gradle. Just point a terminal on the project's root and issue
./gradlew build
This will trigger the creation of the artifacts the executions of the tests, the generation of the documentation and of the project reports.
The 2P project leverages on Semantic Versioning (SemVer, henceforth).
In particular, SemVer is enforced by the current Gradle configuration, which features DanySK's Git sensitive SemVer Gradle Plugin. This implies it is strictly forbidden in this project to create tags whose label is not a valid SemVar string.
Notice that the 2P project is still in its initial development stage---as proven by the major number equal to 0
in its version string.
According to SemVer, this implies anything may change at any time, as the public API should not be considered stable.
If you meet some problem in using or developing 2P, you are encouraged to signal it through the project "Issues" section on GitHub.
For Tasks:
Click tags to check more tools for each tasksFor Jobs:
Alternative AI tools for 2p-kt
Similar Open Source Tools
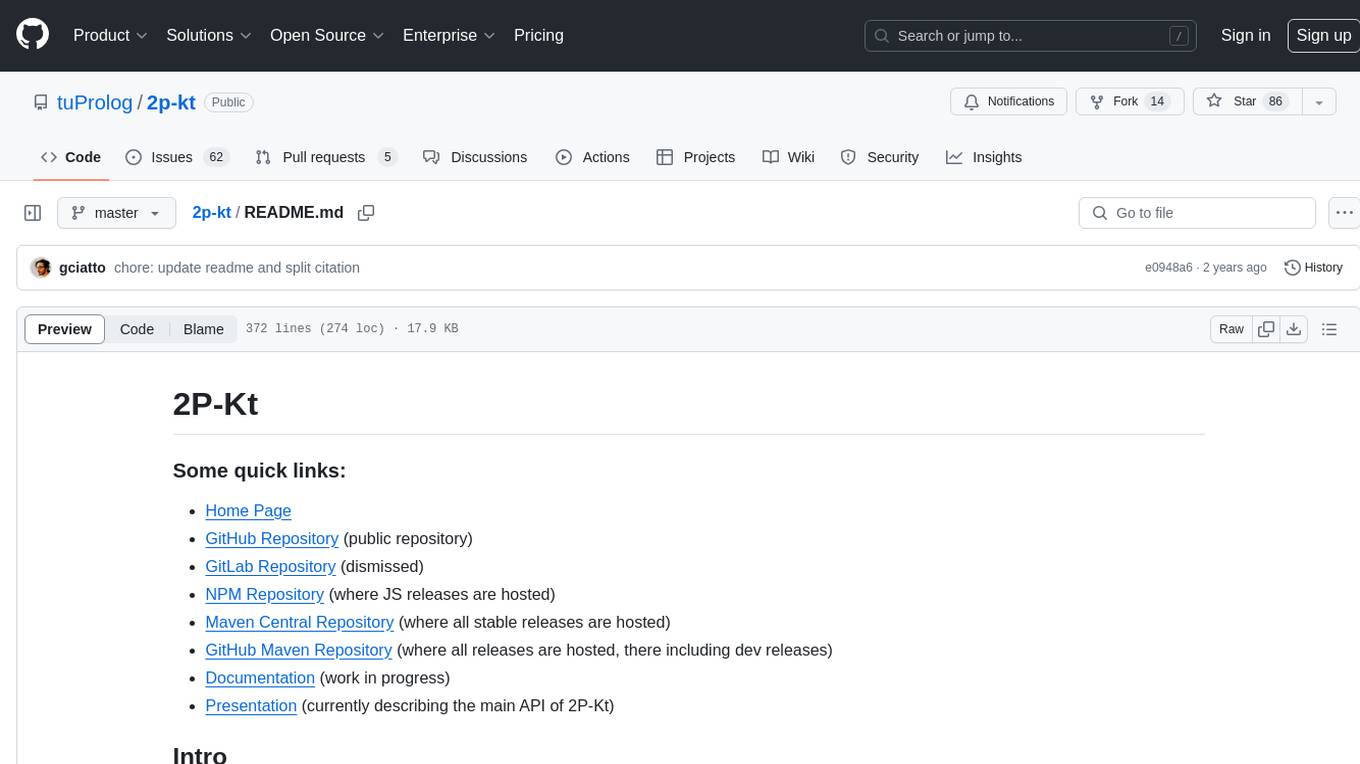
2p-kt
2P-Kt is a Kotlin-based and multi-platform reboot of tuProlog (2P), a multi-paradigm logic programming framework written in Java. It consists of an open ecosystem for Symbolic Artificial Intelligence (AI) with modules supporting logic terms, unification, indexing, resolution of logic queries, probabilistic logic programming, binary decision diagrams, OR-concurrent resolution, DSL for logic programming, parsing modules, serialisation modules, command-line interface, and graphical user interface. The tool is designed to support knowledge representation and automatic reasoning through logic programming in an extensible and flexible way, encouraging extensions towards other symbolic AI systems than Prolog. It is a pure, multi-platform Kotlin project supporting JVM, JS, Android, and Native platforms, with a lightweight library leveraging the Kotlin common library.
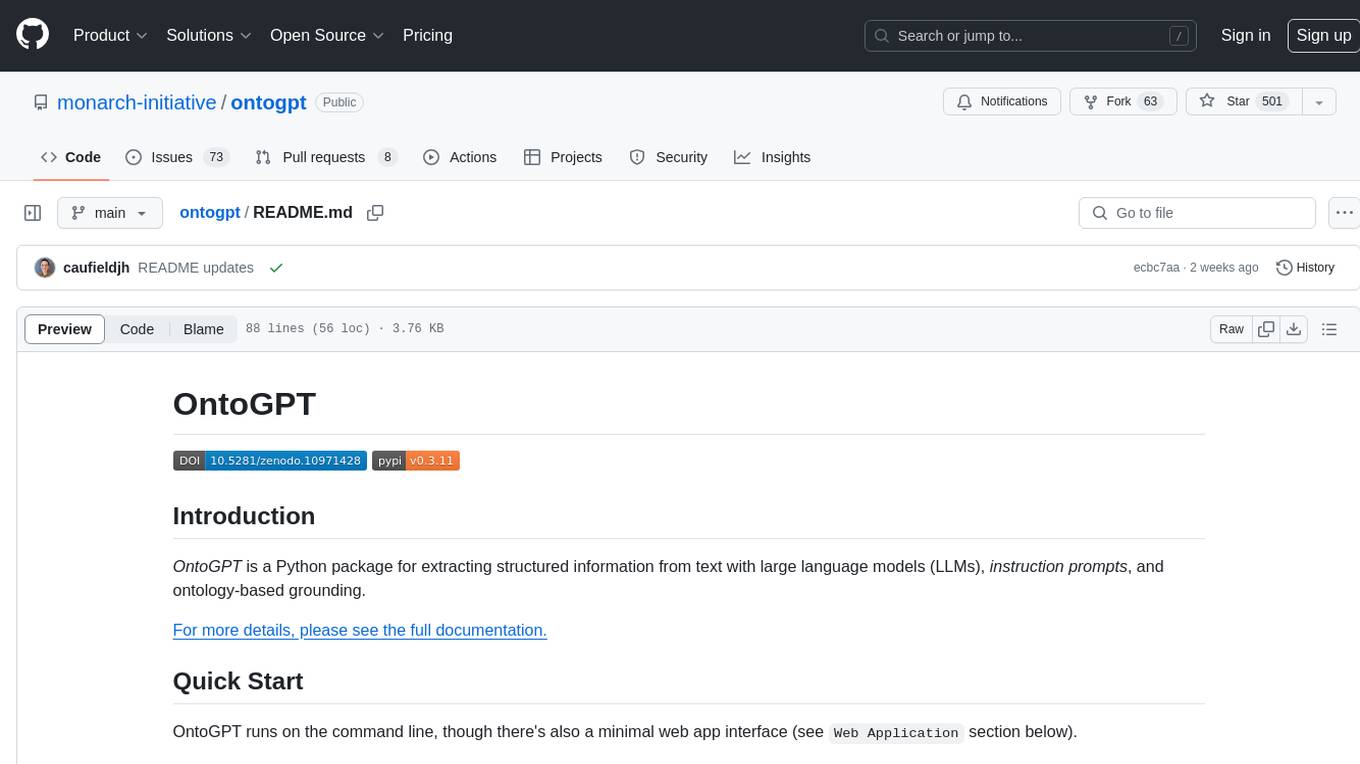
ontogpt
OntoGPT is a Python package for extracting structured information from text using large language models, instruction prompts, and ontology-based grounding. It provides a command line interface and a minimal web app for easy usage. The tool has been evaluated on test data and is used in related projects like TALISMAN for gene set analysis. OntoGPT enables users to extract information from text by specifying relevant terms and provides the extracted objects as output.
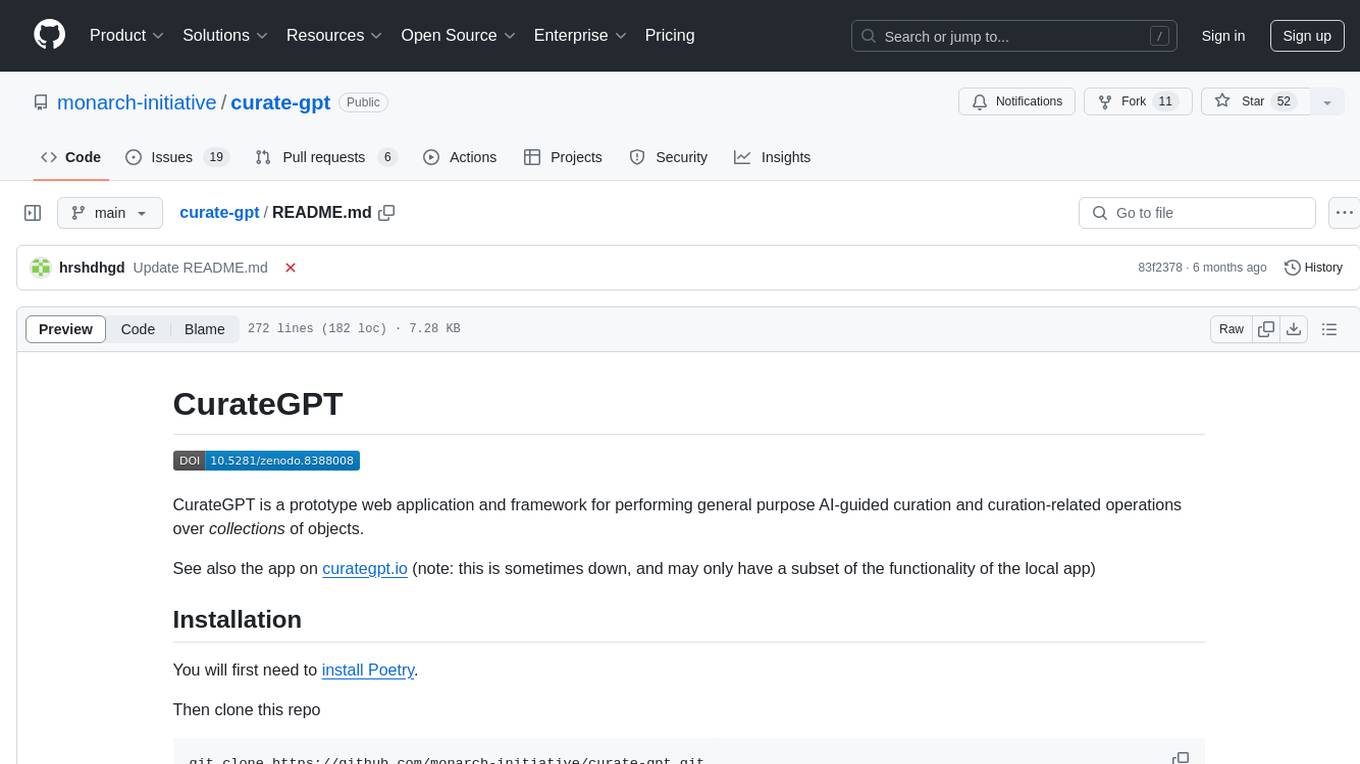
curate-gpt
CurateGPT is a prototype web application and framework for performing general purpose AI-guided curation and curation-related operations over collections of objects. It allows users to load JSON, YAML, or CSV data, build vector database indexes for ontologies, and interact with various data sources like GitHub, Google Drives, Google Sheets, and more. The tool supports ontology curation, knowledge base querying, term autocompletion, and all-by-all comparisons for objects in a collection.
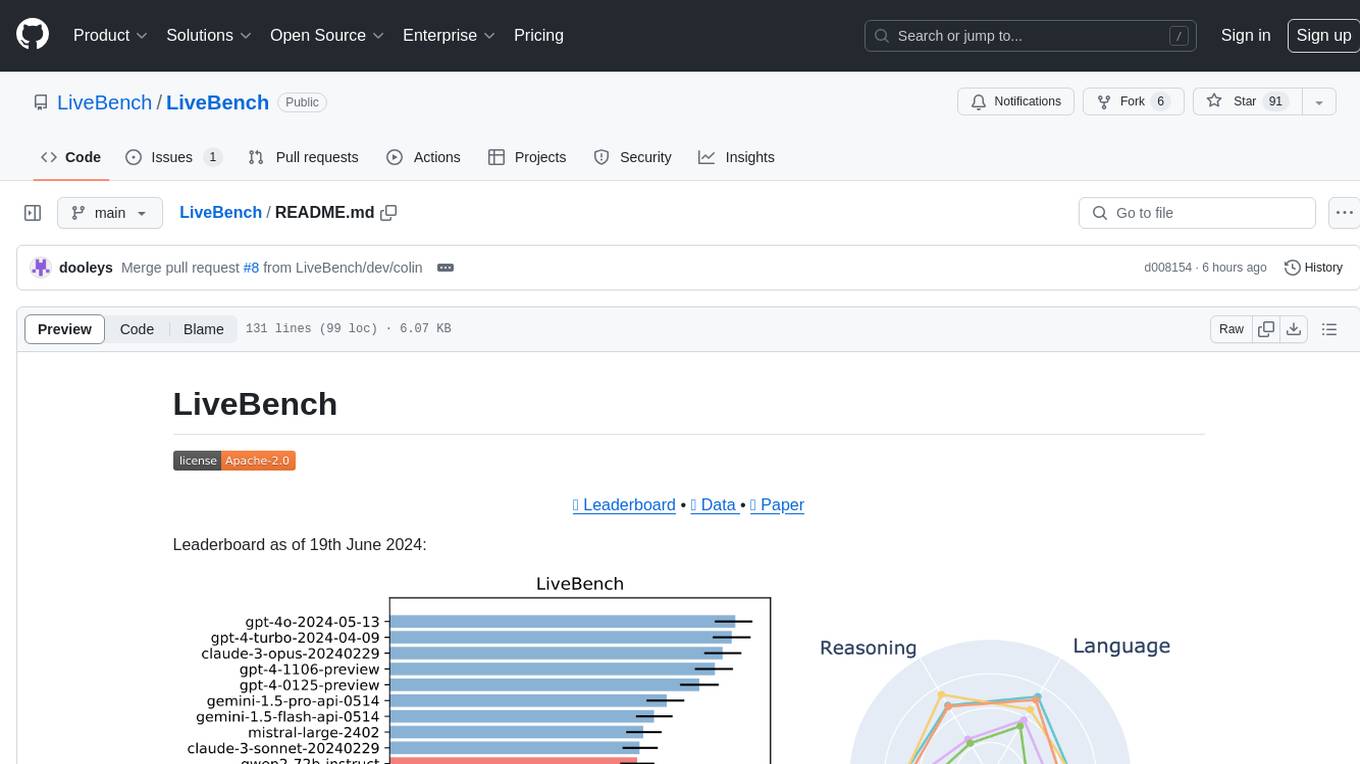
LiveBench
LiveBench is a benchmark tool designed for Language Model Models (LLMs) with a focus on limiting contamination through monthly new questions based on recent datasets, arXiv papers, news articles, and IMDb movie synopses. It provides verifiable, objective ground-truth answers for accurate scoring without an LLM judge. The tool offers 18 diverse tasks across 6 categories and promises to release more challenging tasks over time. LiveBench is built on FastChat's llm_judge module and incorporates code from LiveCodeBench and IFEval.
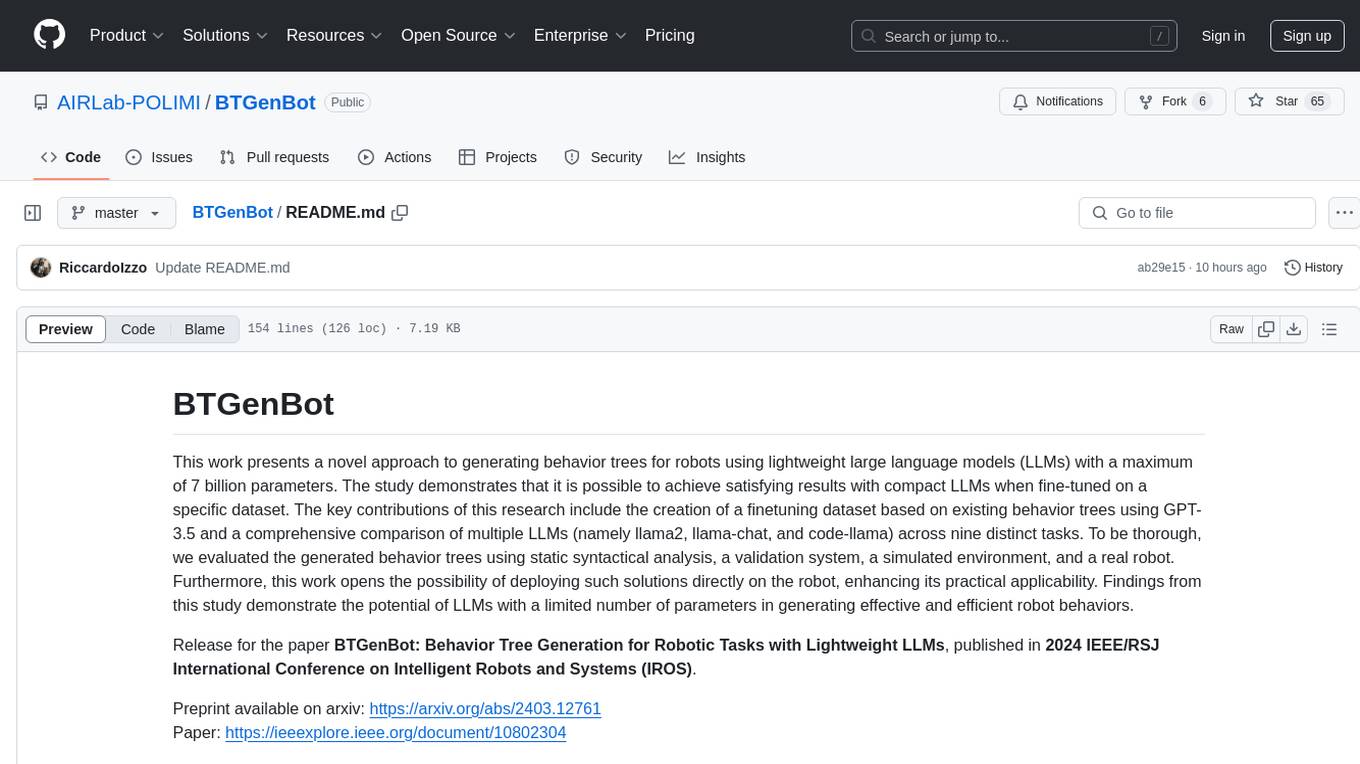
BTGenBot
BTGenBot is a tool that generates behavior trees for robots using lightweight large language models (LLMs) with a maximum of 7 billion parameters. It fine-tunes on a specific dataset, compares multiple LLMs, and evaluates generated behavior trees using various methods. The tool demonstrates the potential of LLMs with a limited number of parameters in creating effective and efficient robot behaviors.
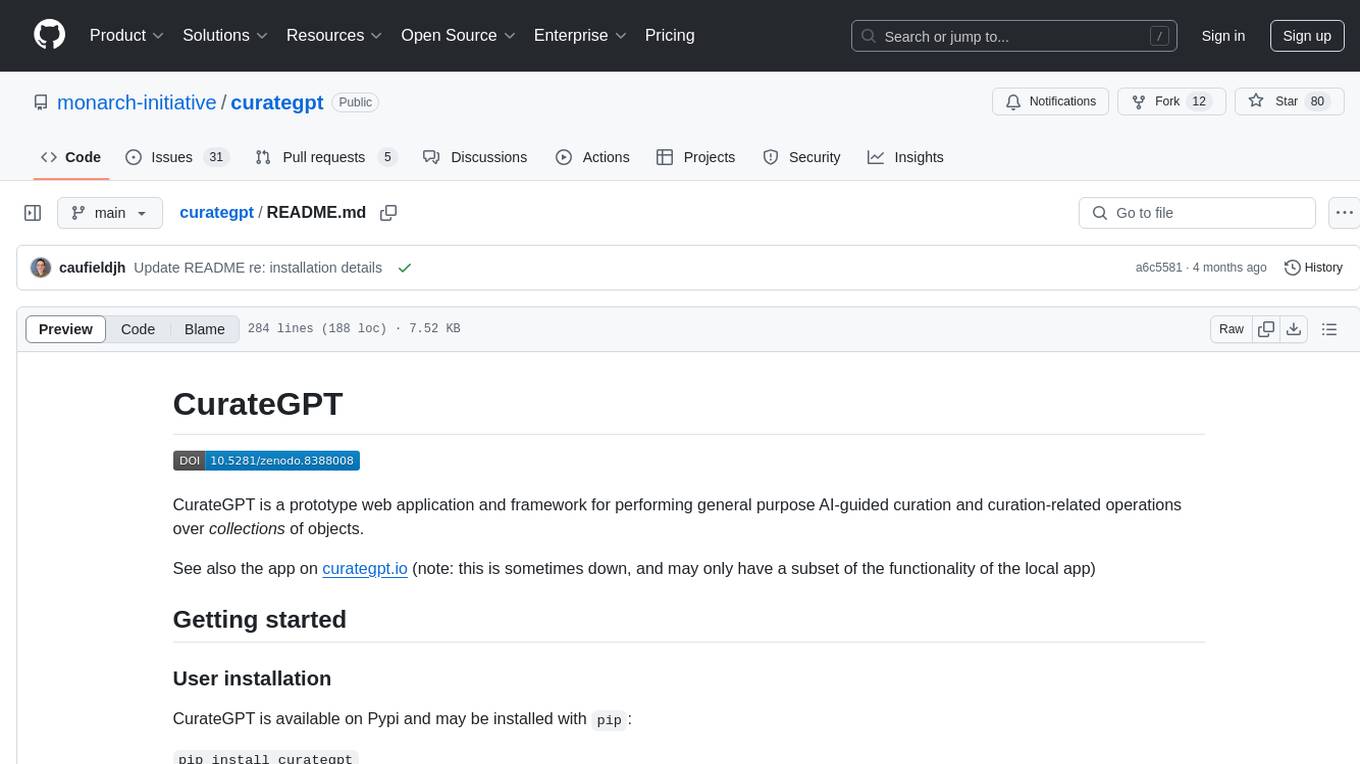
curategpt
CurateGPT is a prototype web application and framework designed for general purpose AI-guided curation and curation-related operations over collections of objects. It provides functionalities for loading example data, building indexes, interacting with knowledge bases, and performing tasks such as chatting with a knowledge base, querying Pubmed, interacting with a GitHub issue tracker, term autocompletion, and all-by-all comparisons. The tool is built to work best with the OpenAI gpt-4 model and OpenAI ada-text-embedding-002 for embedding, but also supports alternative models through a plugin architecture.
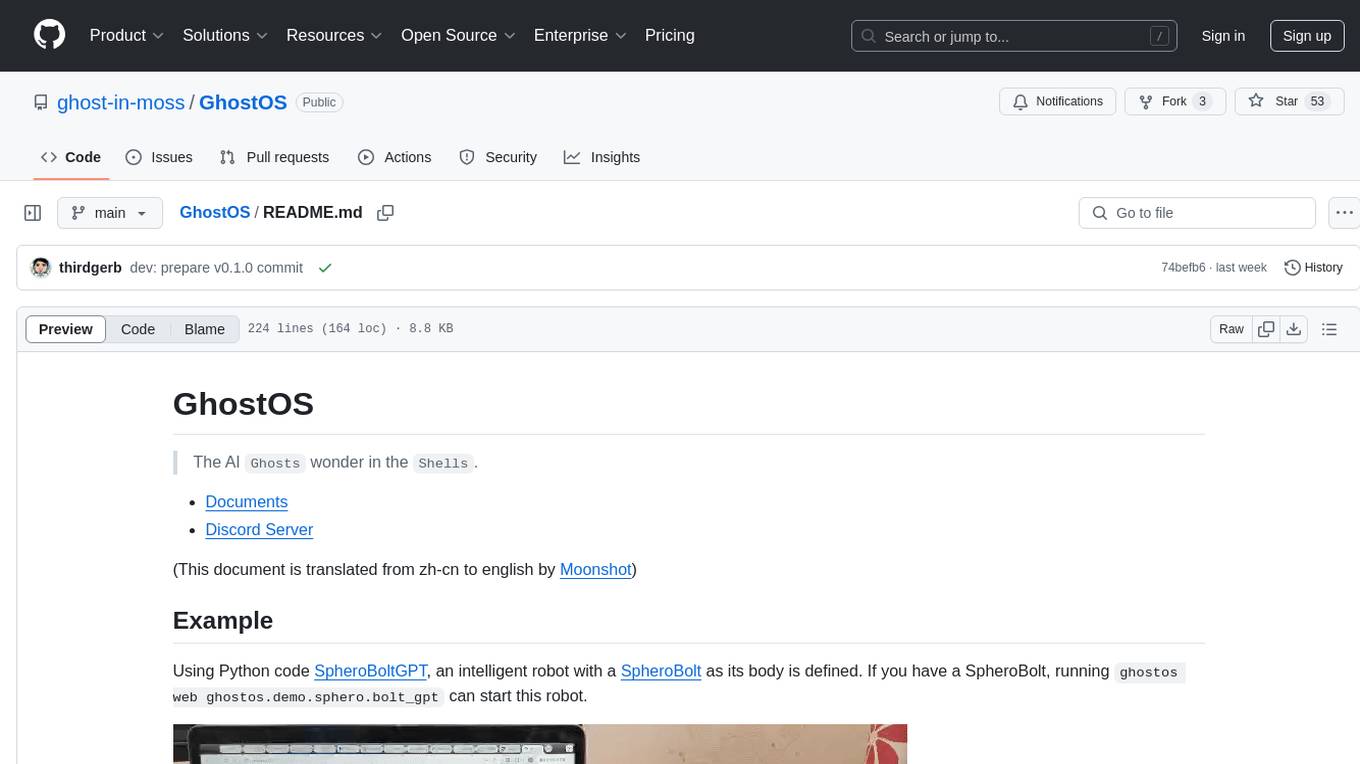
GhostOS
GhostOS is an AI Agent framework designed to replace JSON Schema with a Turing-complete code interaction interface (Moss Protocol). It aims to create intelligent entities capable of continuous learning and growth through code generation and project management. The framework supports various capabilities such as turning Python files into web agents, real-time voice conversation, body movements control, and emotion expression. GhostOS is still in early experimental development and focuses on out-of-the-box capabilities for AI agents.
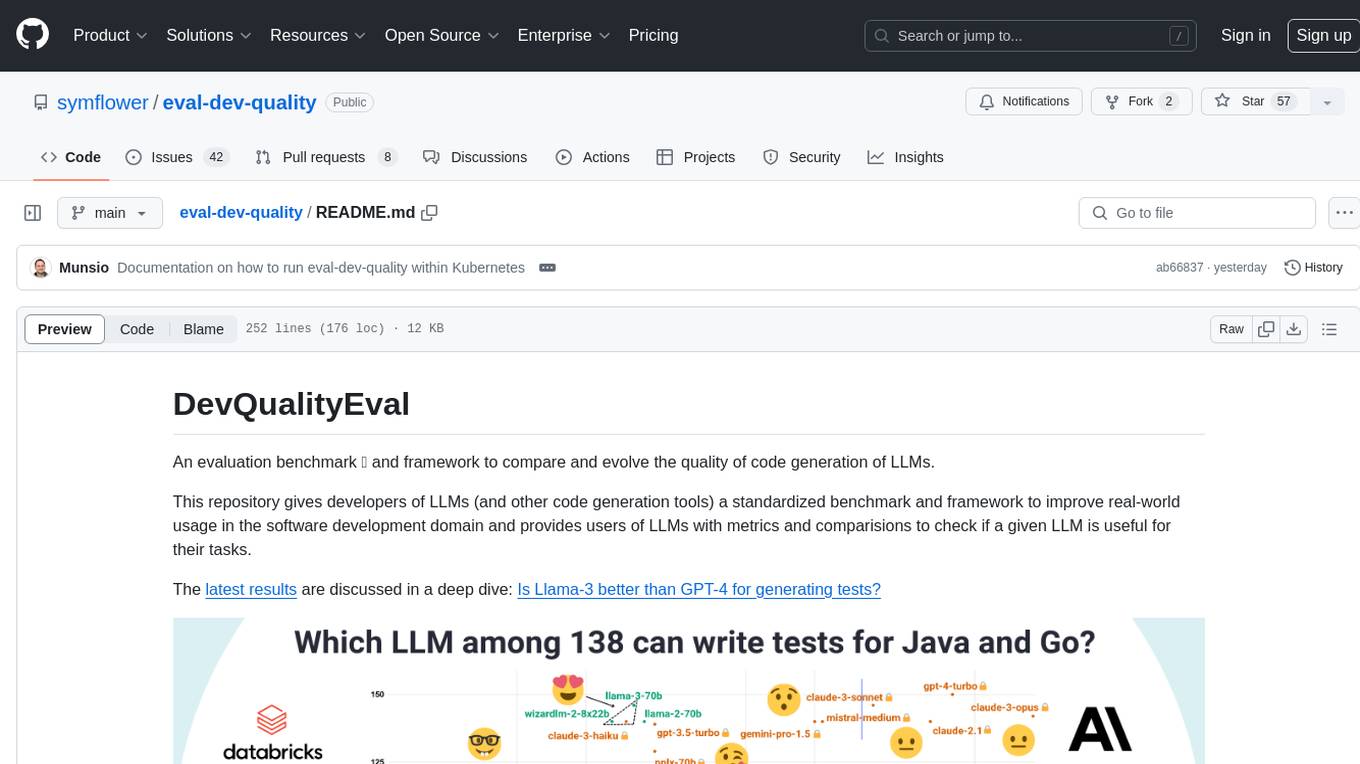
eval-dev-quality
DevQualityEval is an evaluation benchmark and framework designed to compare and improve the quality of code generation of Language Model Models (LLMs). It provides developers with a standardized benchmark to enhance real-world usage in software development and offers users metrics and comparisons to assess the usefulness of LLMs for their tasks. The tool evaluates LLMs' performance in solving software development tasks and measures the quality of their results through a point-based system. Users can run specific tasks, such as test generation, across different programming languages to evaluate LLMs' language understanding and code generation capabilities.
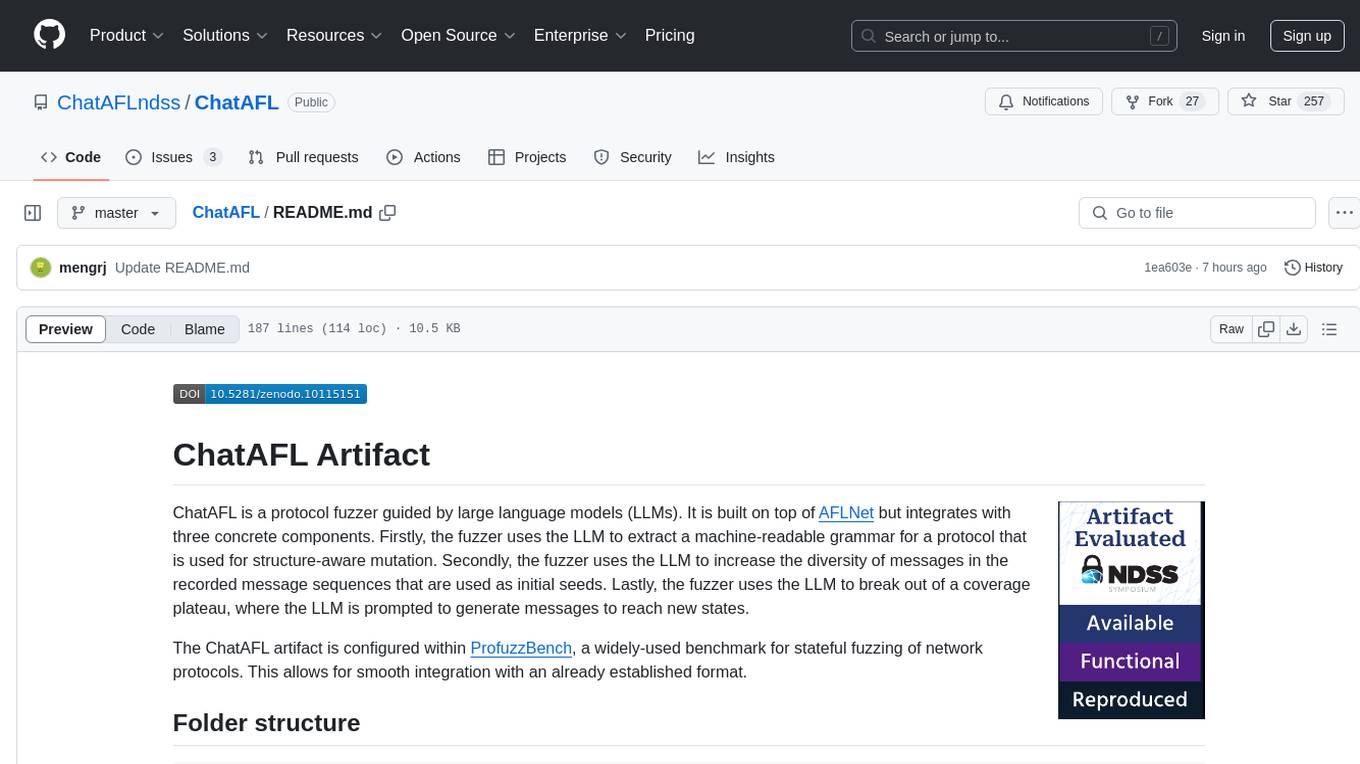
ChatAFL
ChatAFL is a protocol fuzzer guided by large language models (LLMs) that extracts machine-readable grammar for protocol mutation, increases message diversity, and breaks coverage plateaus. It integrates with ProfuzzBench for stateful fuzzing of network protocols, providing smooth integration. The artifact includes modified versions of AFLNet and ProfuzzBench, source code for ChatAFL with proposed strategies, and scripts for setup, execution, analysis, and cleanup. Users can analyze data, construct plots, examine LLM-generated grammars, enriched seeds, and state-stall responses, and reproduce results with downsized experiments. Customization options include modifying fuzzers, tuning parameters, adding new subjects, troubleshooting, and working on GPT-4. Limitations include interaction with OpenAI's Large Language Models and a hard limit of 150,000 tokens per minute.
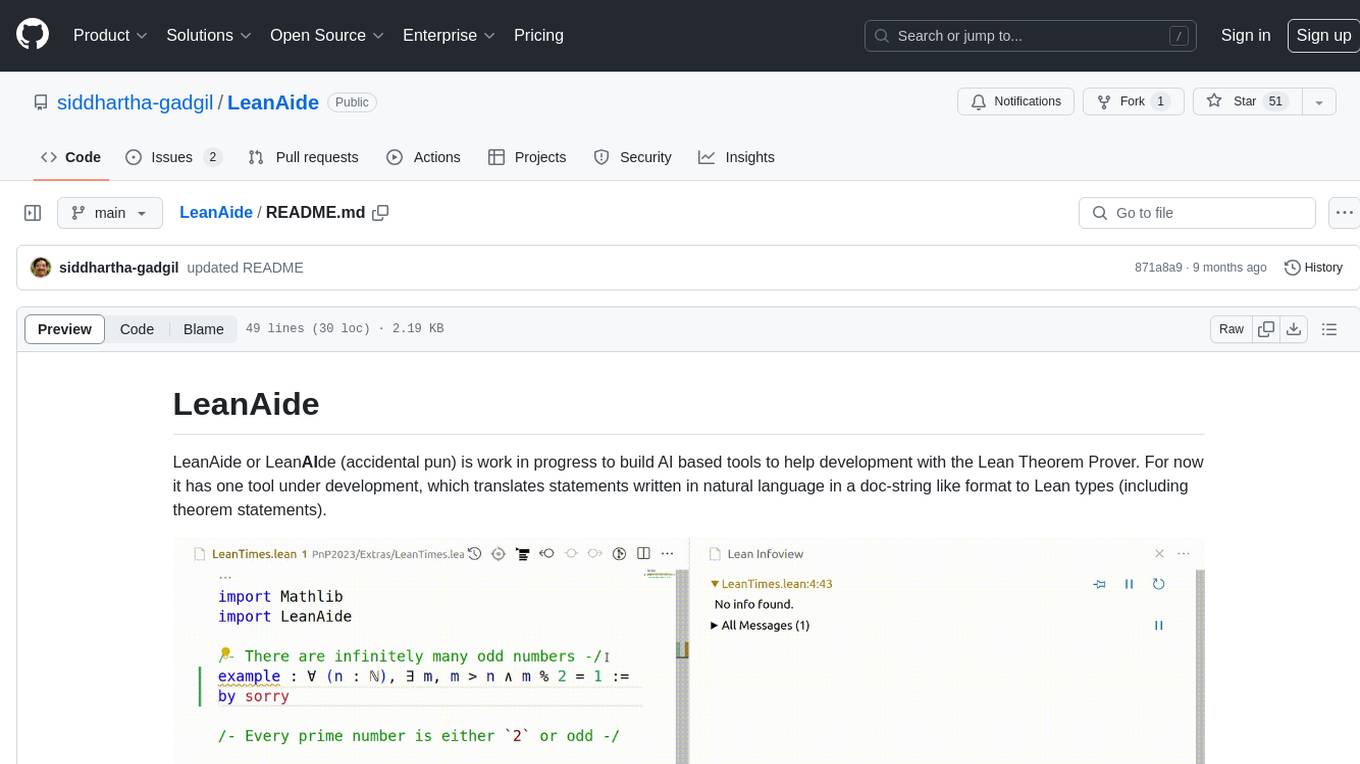
LeanAide
LeanAide is a work in progress AI tool designed to assist with development using the Lean Theorem Prover. It currently offers a tool that translates natural language statements to Lean types, including theorem statements. The tool is based on GPT 3.5-turbo/GPT 4 and requires an OpenAI key for usage. Users can include LeanAide as a dependency in their projects to access the translation functionality.
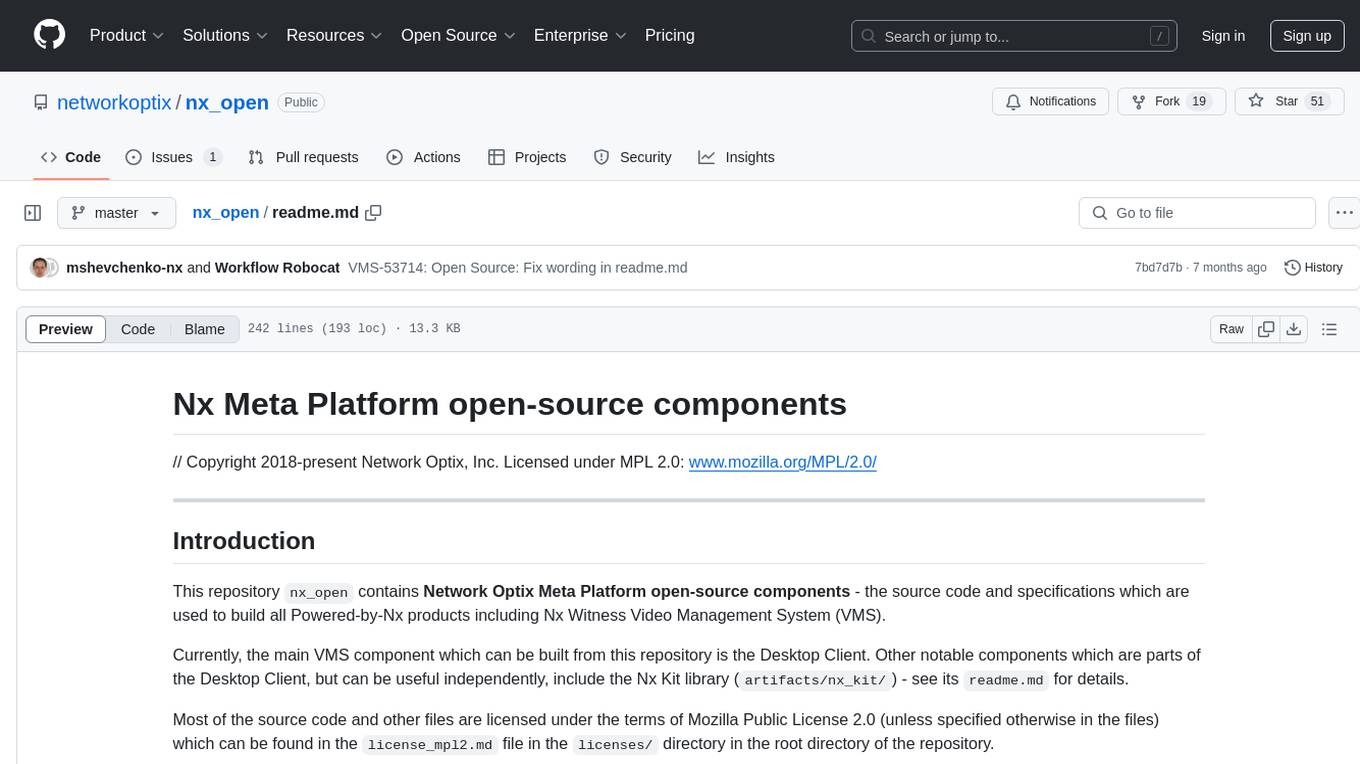
nx_open
The `nx_open` repository contains open-source components for the Network Optix Meta Platform, used to build products like Nx Witness Video Management System. It includes source code, specifications, and a Desktop Client. The repository is licensed under Mozilla Public License 2.0. Users can build the Desktop Client and customize it using a zip file. The build environment supports Windows, Linux, and macOS platforms with specific prerequisites. The repository provides scripts for building, signing executable files, and running the Desktop Client. Compatibility with VMS Server versions is crucial, and automatic VMS updates are disabled for the open-source Desktop Client.
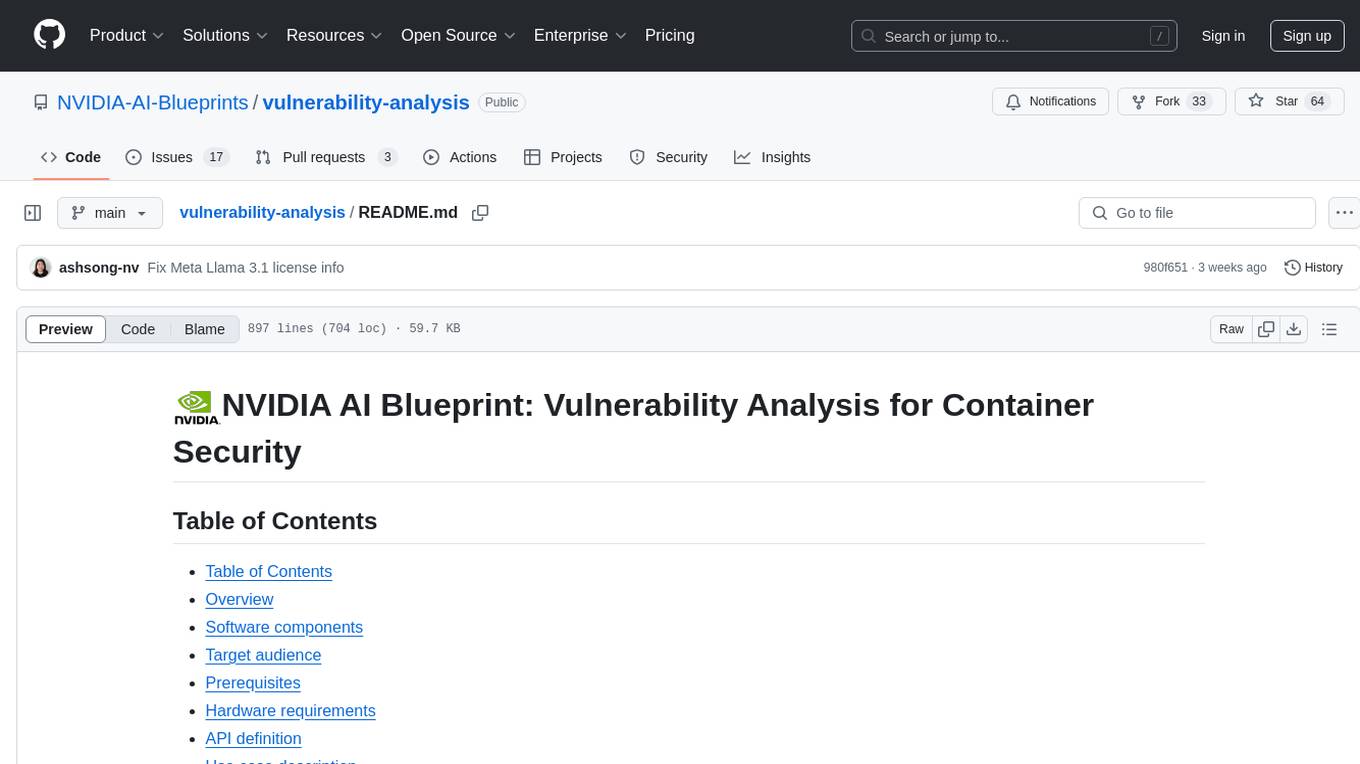
vulnerability-analysis
The NVIDIA AI Blueprint for Vulnerability Analysis for Container Security showcases accelerated analysis on common vulnerabilities and exposures (CVE) at an enterprise scale, reducing mitigation time from days to seconds. It enables security analysts to determine software package vulnerabilities using large language models (LLMs) and retrieval-augmented generation (RAG). The blueprint is designed for security analysts, IT engineers, and AI practitioners in cybersecurity. It requires NVAIE developer license and API keys for vulnerability databases, search engines, and LLM model services. Hardware requirements include L40 GPU for pipeline operation and optional LLM NIM and Embedding NIM. The workflow involves LLM pipeline for CVE impact analysis, utilizing LLM planner, agent, and summarization nodes. The blueprint uses NVIDIA NIM microservices and Morpheus Cybersecurity AI SDK for vulnerability analysis.
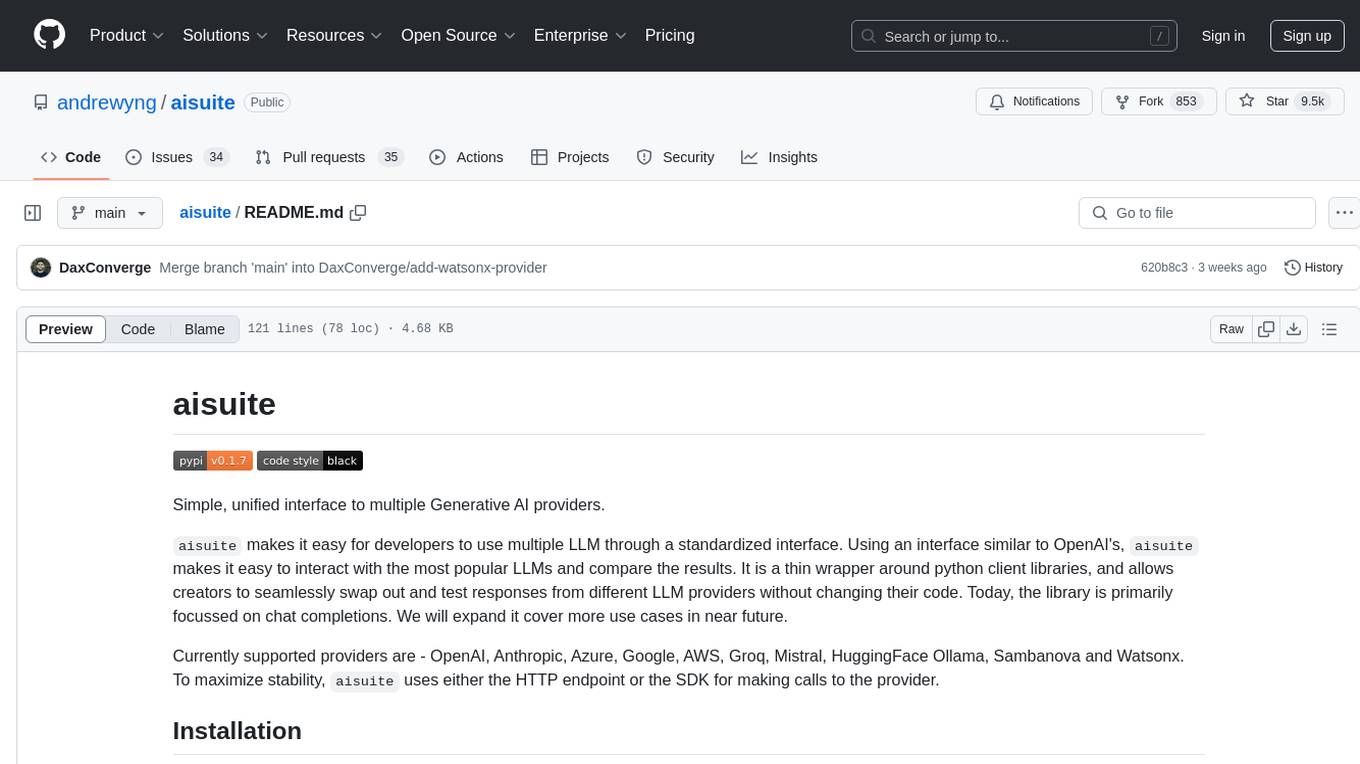
aisuite
Aisuite is a simple, unified interface to multiple Generative AI providers. It allows developers to easily interact with various Language Model (LLM) providers like OpenAI, Anthropic, Azure, Google, AWS, and more through a standardized interface. The library focuses on chat completions and provides a thin wrapper around python client libraries, enabling creators to test responses from different LLM providers without changing their code. Aisuite maximizes stability by using HTTP endpoints or SDKs for making calls to the providers. Users can install the base package or specific provider packages, set up API keys, and utilize the library to generate chat completion responses from different models.
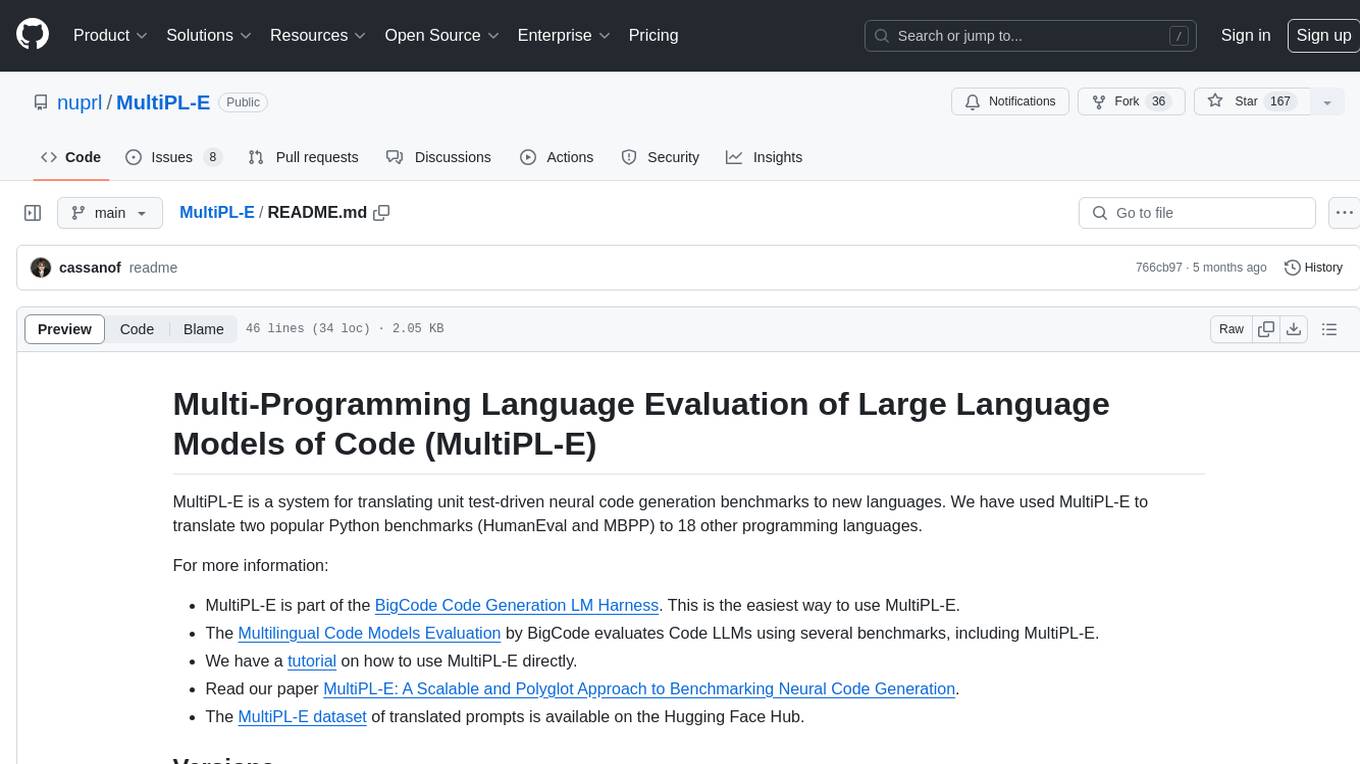
MultiPL-E
MultiPL-E is a system for translating unit test-driven neural code generation benchmarks to new languages. It is part of the BigCode Code Generation LM Harness and allows for evaluating Code LLMs using various benchmarks. The tool supports multiple versions with improvements and new language additions, providing a scalable and polyglot approach to benchmarking neural code generation. Users can access a tutorial for direct usage and explore the dataset of translated prompts on the Hugging Face Hub.
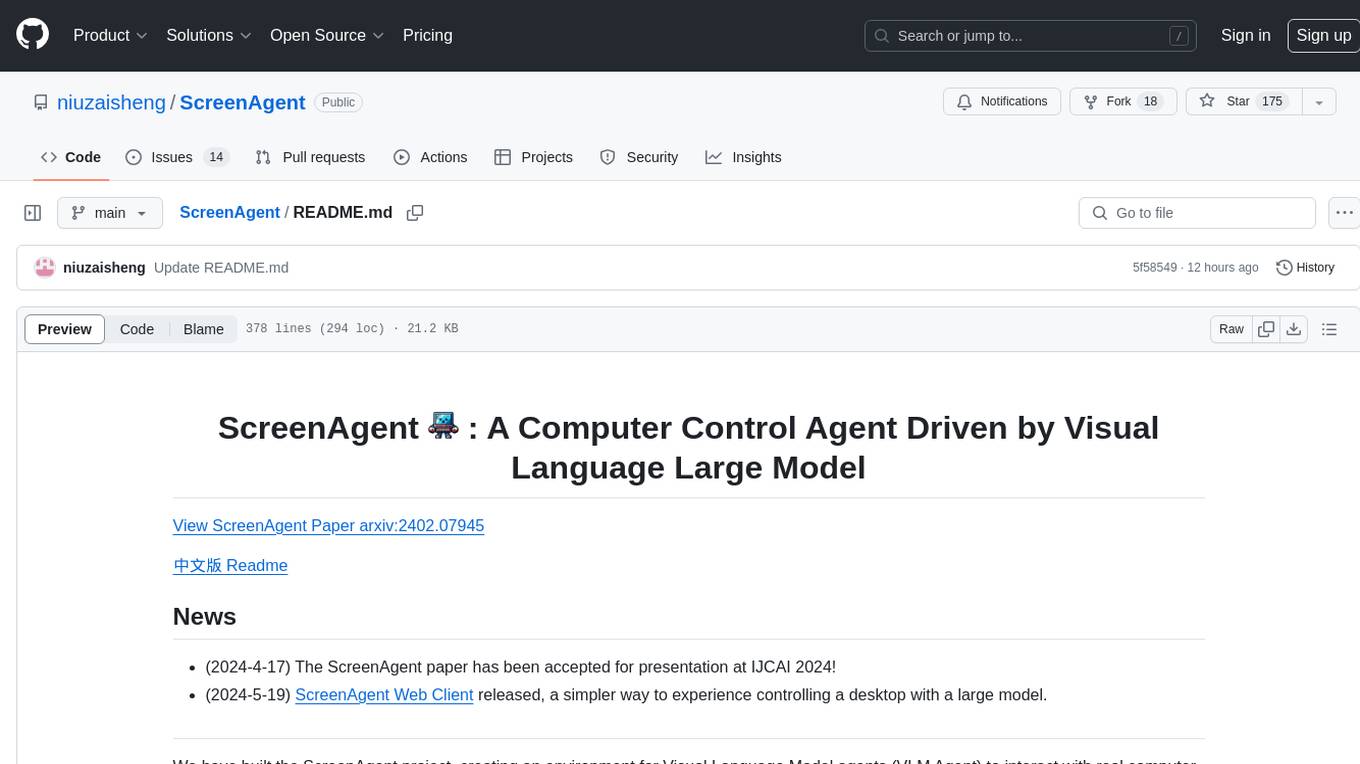
ScreenAgent
ScreenAgent is a project focused on creating an environment for Visual Language Model agents (VLM Agent) to interact with real computer screens. The project includes designing an automatic control process for agents to interact with the environment and complete multi-step tasks. It also involves building the ScreenAgent dataset, which collects screenshots and action sequences for various daily computer tasks. The project provides a controller client code, configuration files, and model training code to enable users to control a desktop with a large model.
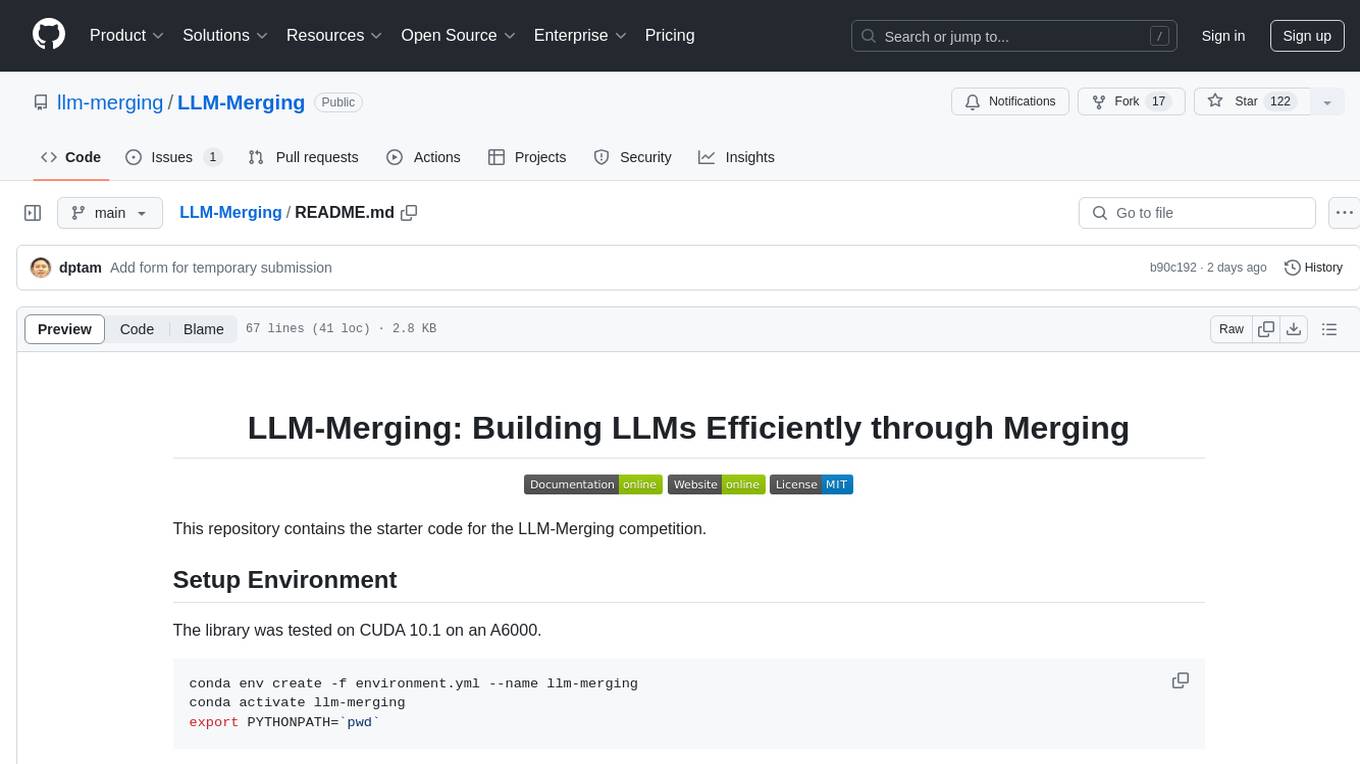
LLM-Merging
LLM-Merging is a repository containing starter code for the LLM-Merging competition. It provides a platform for efficiently building LLMs through merging methods. Users can develop new merging methods by creating new files in the specified directory and extending existing classes. The repository includes instructions for setting up the environment, developing new merging methods, testing the methods on specific datasets, and submitting solutions for evaluation. It aims to facilitate the development and evaluation of merging methods for LLMs.
For similar tasks
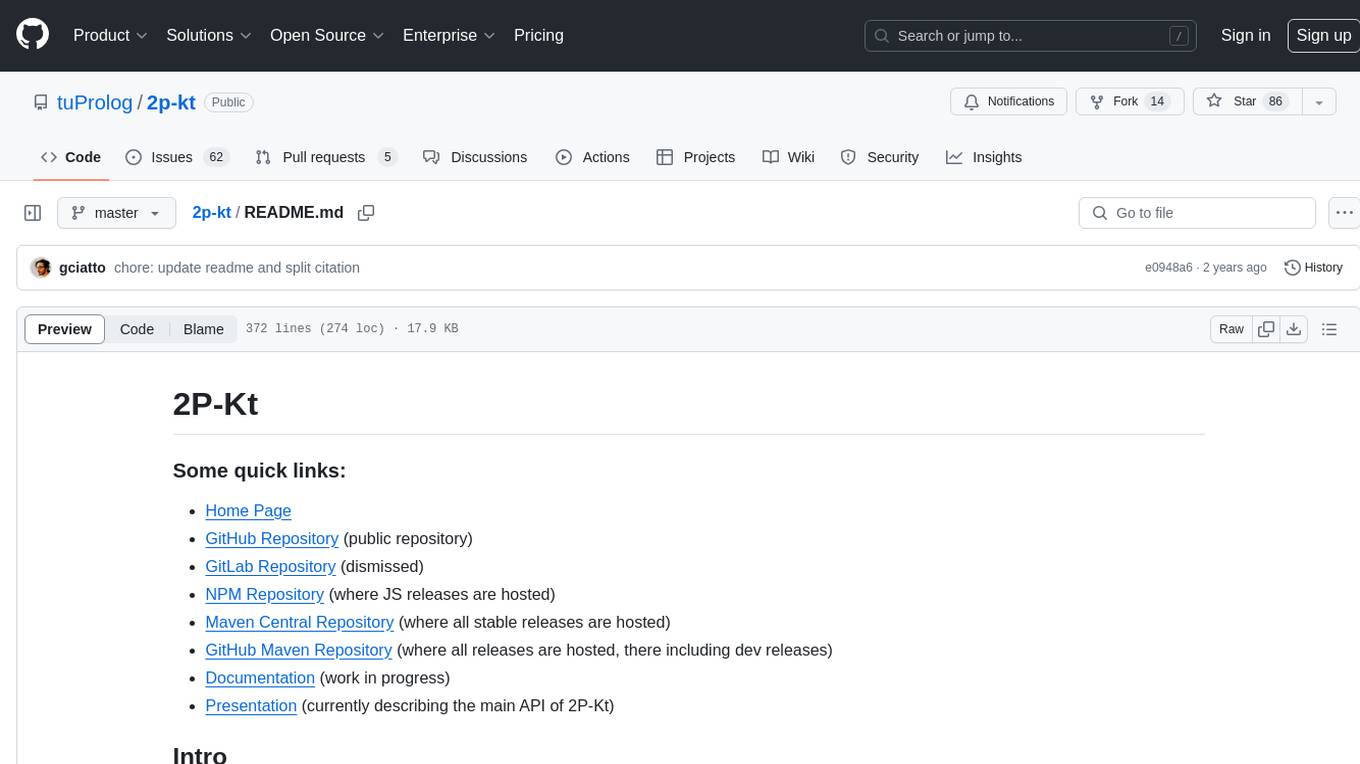
2p-kt
2P-Kt is a Kotlin-based and multi-platform reboot of tuProlog (2P), a multi-paradigm logic programming framework written in Java. It consists of an open ecosystem for Symbolic Artificial Intelligence (AI) with modules supporting logic terms, unification, indexing, resolution of logic queries, probabilistic logic programming, binary decision diagrams, OR-concurrent resolution, DSL for logic programming, parsing modules, serialisation modules, command-line interface, and graphical user interface. The tool is designed to support knowledge representation and automatic reasoning through logic programming in an extensible and flexible way, encouraging extensions towards other symbolic AI systems than Prolog. It is a pure, multi-platform Kotlin project supporting JVM, JS, Android, and Native platforms, with a lightweight library leveraging the Kotlin common library.
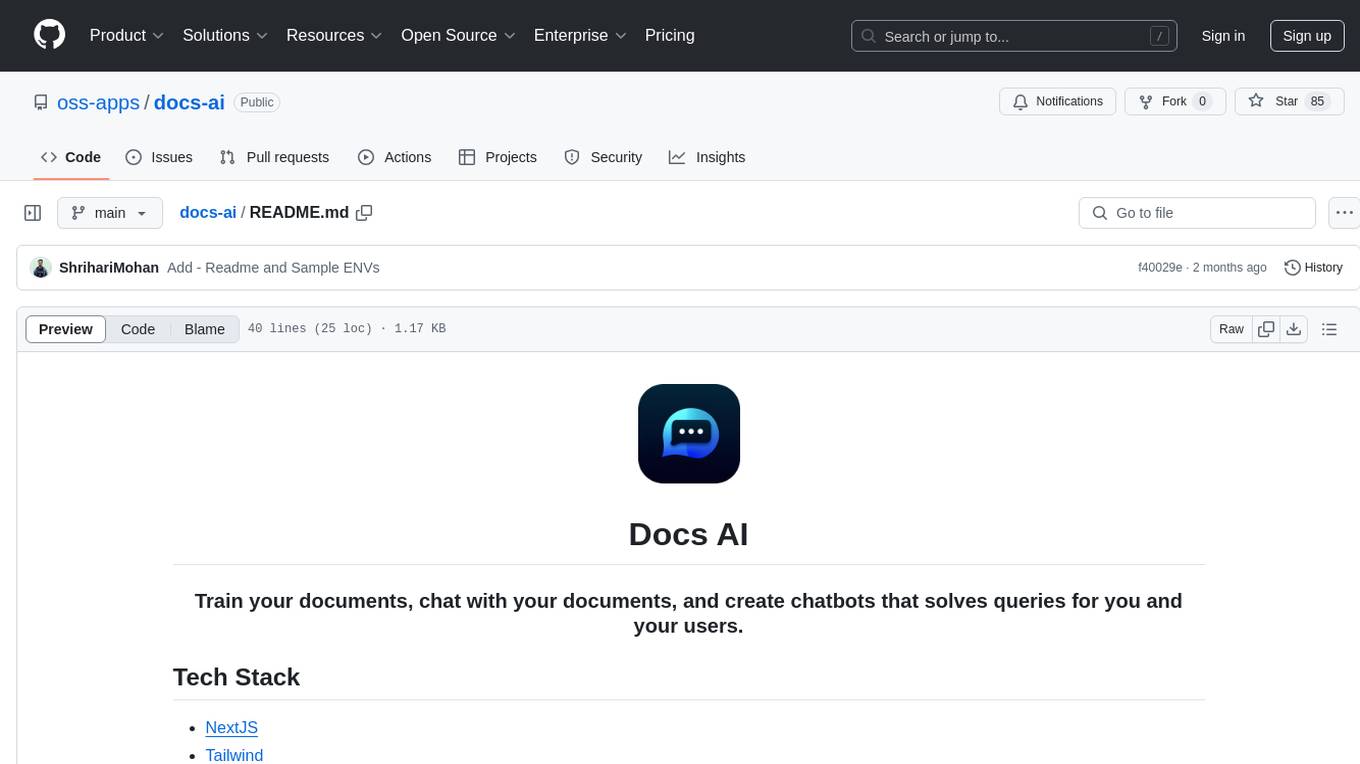
docs-ai
Docs AI is a platform that allows users to train their documents, chat with their documents, and create chatbots to solve queries. It is built using NextJS, Tailwind, tRPC, ShadcnUI, Prisma, Postgres, NextAuth, Pinecone, and Cloudflare R2. The platform requires Node.js (Version: >=18.x), PostgreSQL, and Redis for setup. Users can utilize Docker for development by using the provided `docker-compose.yml` file in the `/app` directory.
For similar jobs
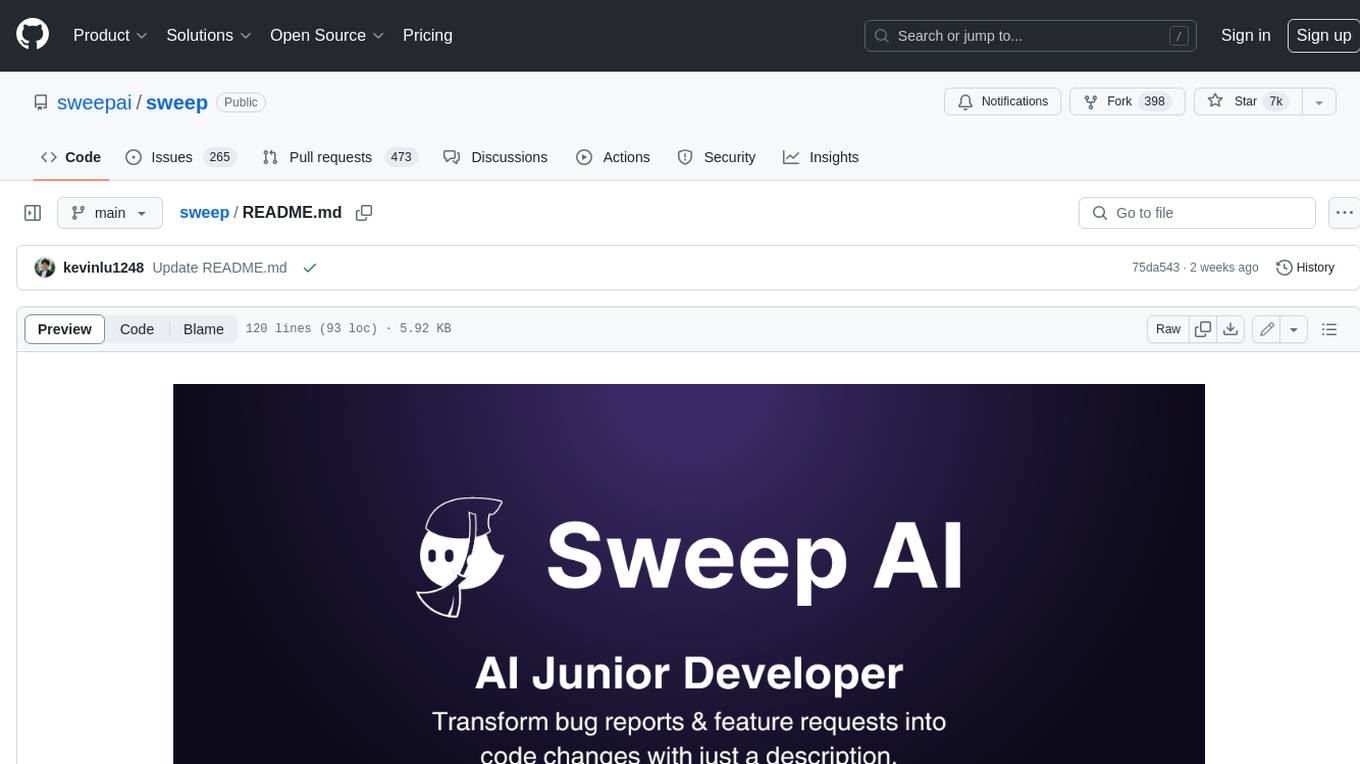
sweep
Sweep is an AI junior developer that turns bugs and feature requests into code changes. It automatically handles developer experience improvements like adding type hints and improving test coverage.
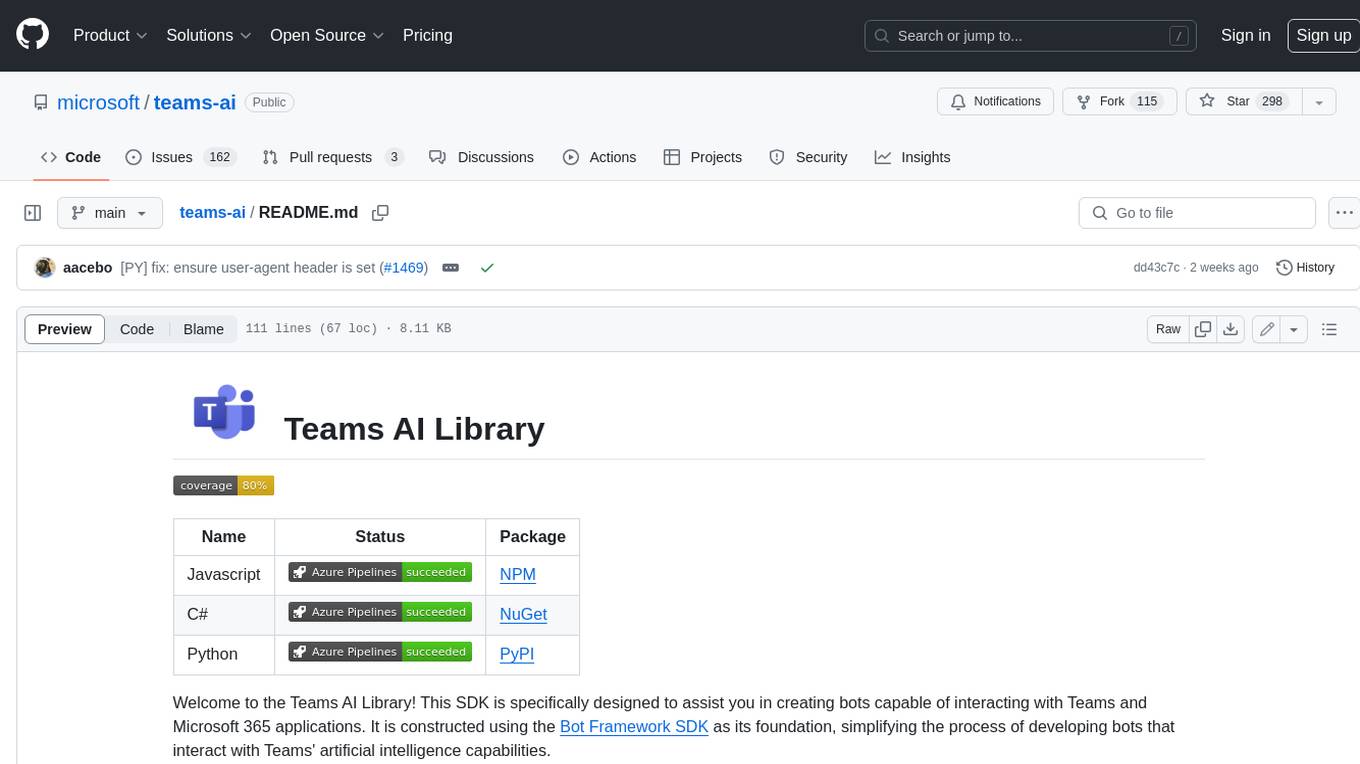
teams-ai
The Teams AI Library is a software development kit (SDK) that helps developers create bots that can interact with Teams and Microsoft 365 applications. It is built on top of the Bot Framework SDK and simplifies the process of developing bots that interact with Teams' artificial intelligence capabilities. The SDK is available for JavaScript/TypeScript, .NET, and Python.
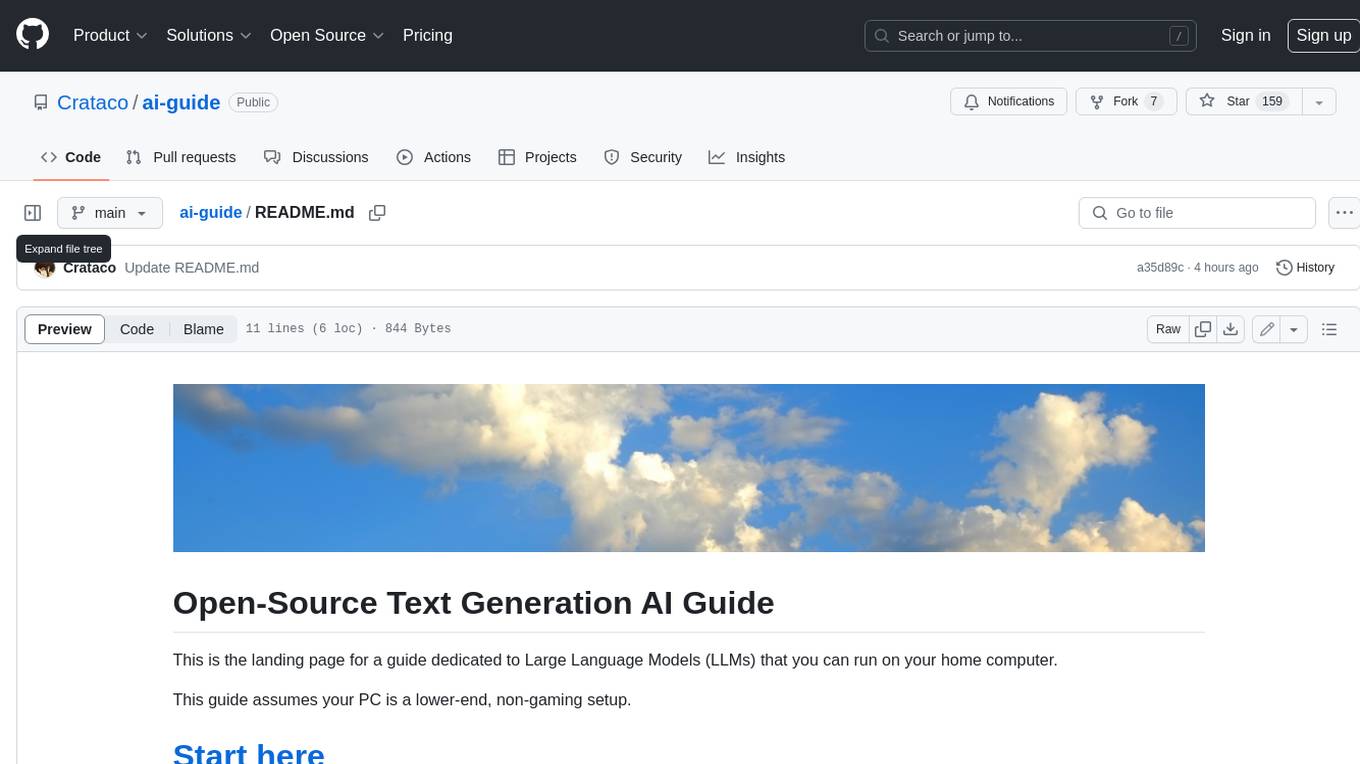
ai-guide
This guide is dedicated to Large Language Models (LLMs) that you can run on your home computer. It assumes your PC is a lower-end, non-gaming setup.
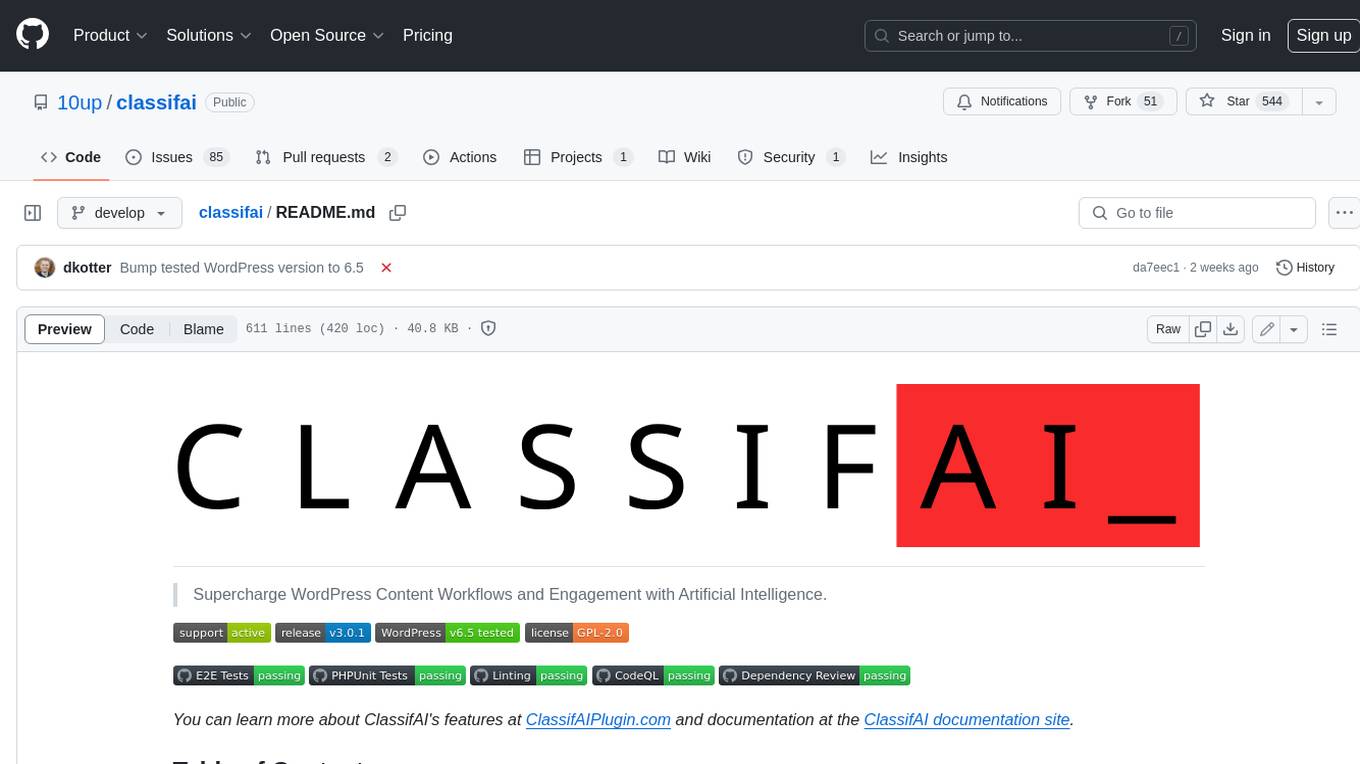
classifai
Supercharge WordPress Content Workflows and Engagement with Artificial Intelligence. Tap into leading cloud-based services like OpenAI, Microsoft Azure AI, Google Gemini and IBM Watson to augment your WordPress-powered websites. Publish content faster while improving SEO performance and increasing audience engagement. ClassifAI integrates Artificial Intelligence and Machine Learning technologies to lighten your workload and eliminate tedious tasks, giving you more time to create original content that matters.
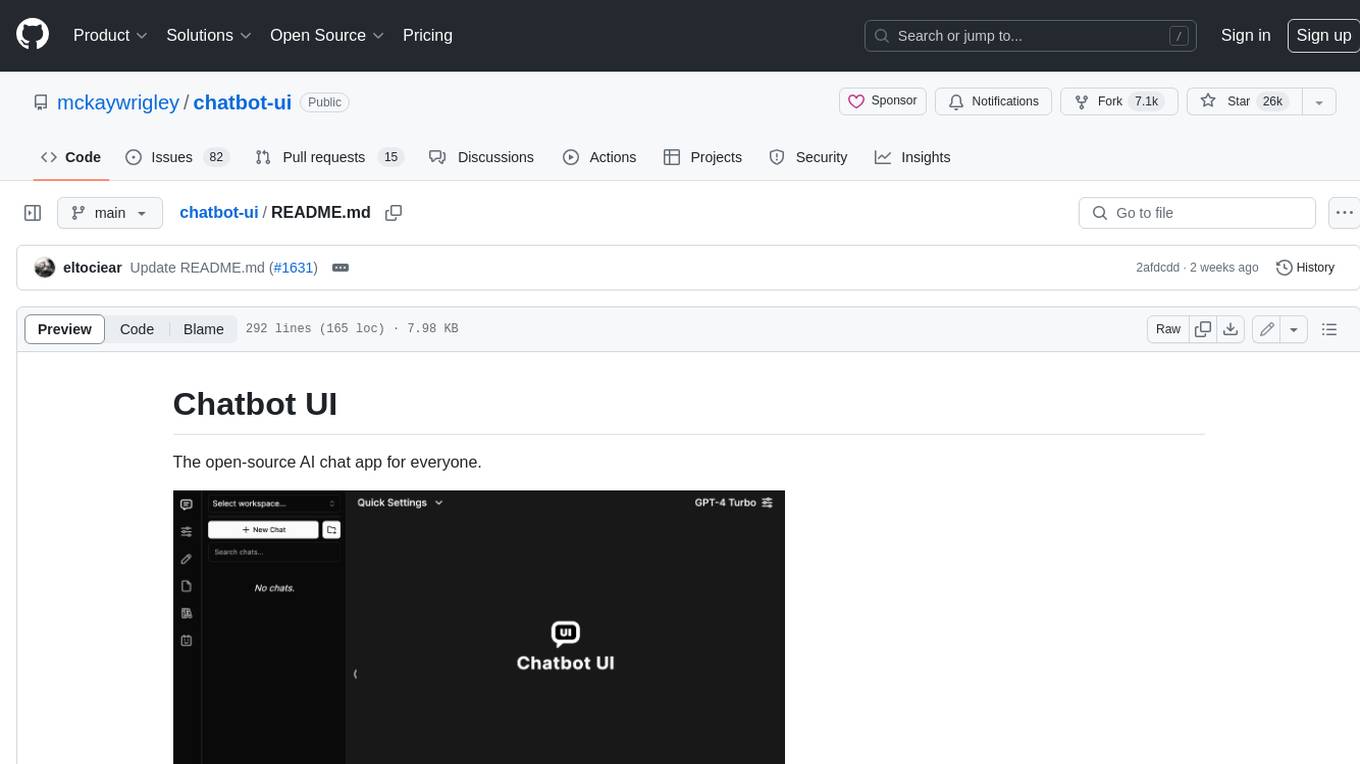
chatbot-ui
Chatbot UI is an open-source AI chat app that allows users to create and deploy their own AI chatbots. It is easy to use and can be customized to fit any need. Chatbot UI is perfect for businesses, developers, and anyone who wants to create a chatbot.
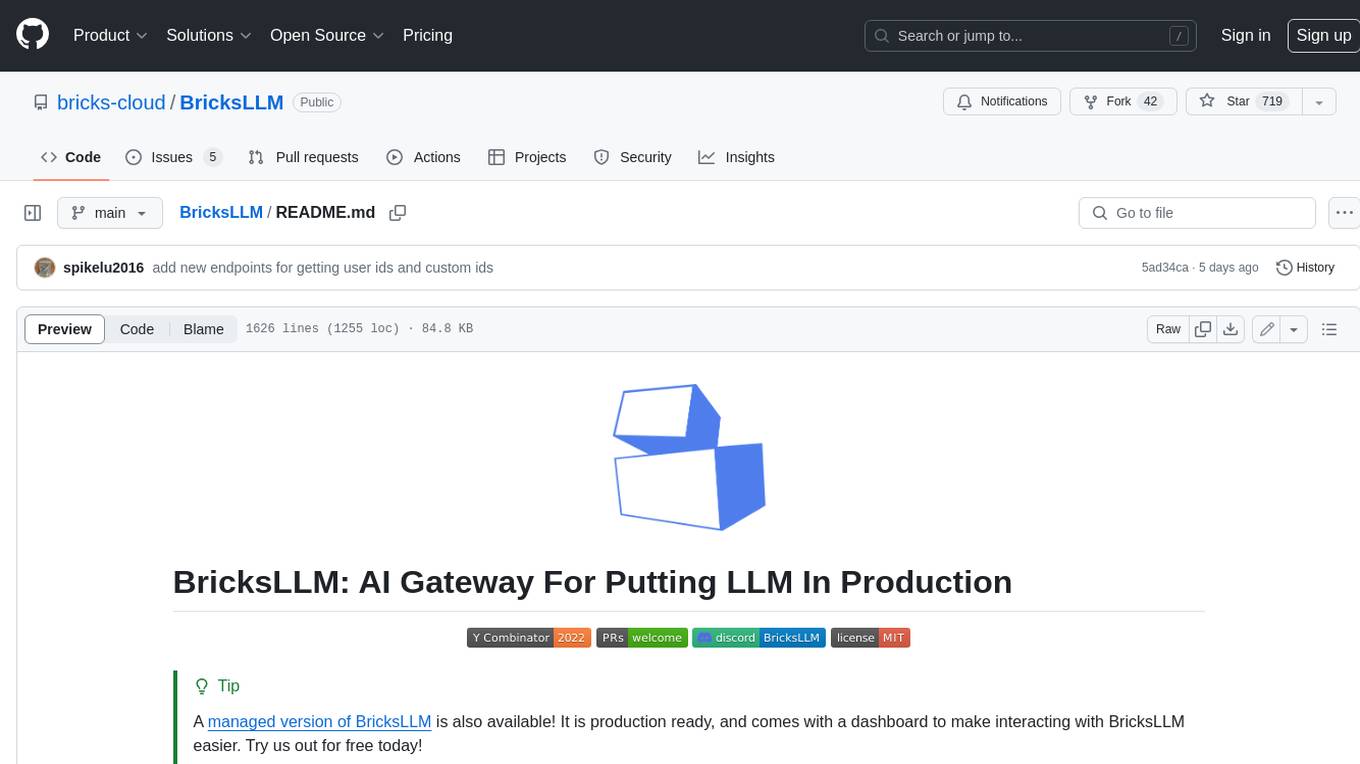
BricksLLM
BricksLLM is a cloud native AI gateway written in Go. Currently, it provides native support for OpenAI, Anthropic, Azure OpenAI and vLLM. BricksLLM aims to provide enterprise level infrastructure that can power any LLM production use cases. Here are some use cases for BricksLLM: * Set LLM usage limits for users on different pricing tiers * Track LLM usage on a per user and per organization basis * Block or redact requests containing PIIs * Improve LLM reliability with failovers, retries and caching * Distribute API keys with rate limits and cost limits for internal development/production use cases * Distribute API keys with rate limits and cost limits for students
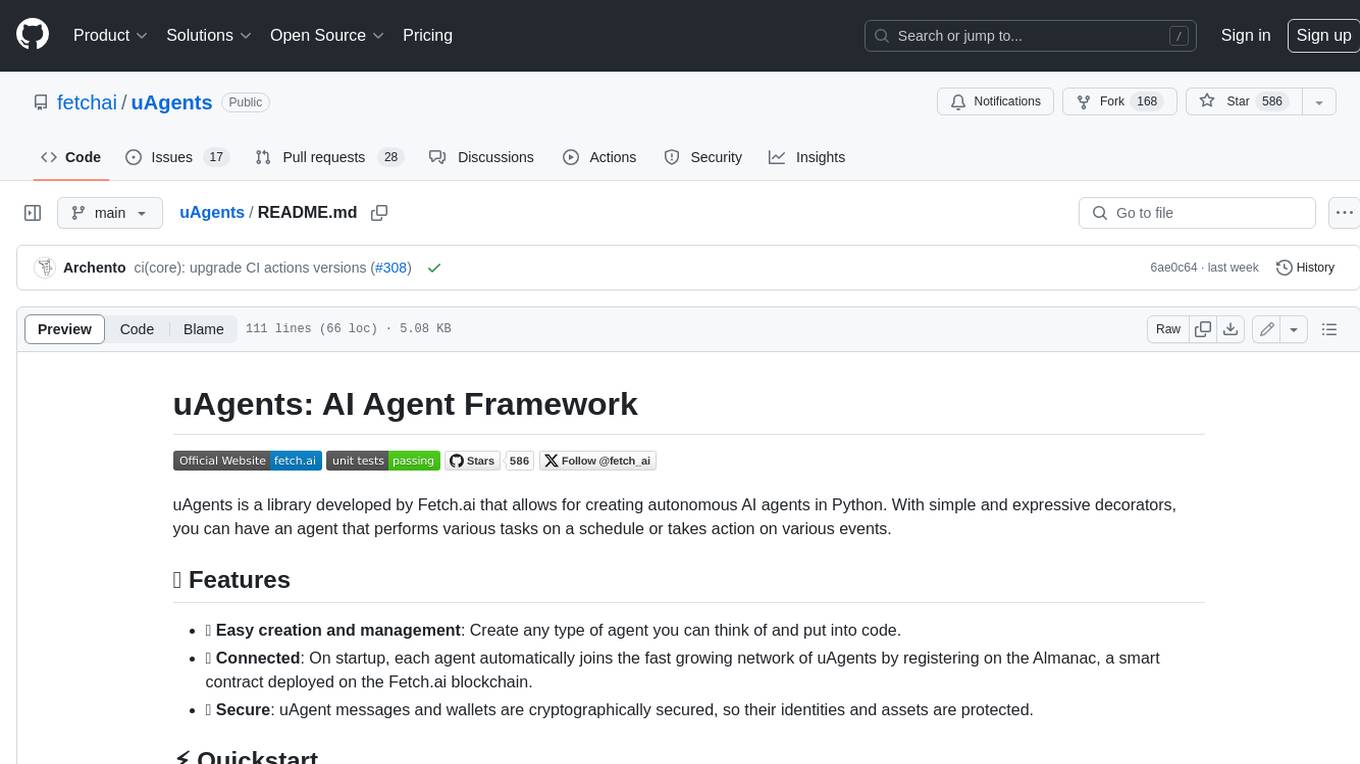
uAgents
uAgents is a Python library developed by Fetch.ai that allows for the creation of autonomous AI agents. These agents can perform various tasks on a schedule or take action on various events. uAgents are easy to create and manage, and they are connected to a fast-growing network of other uAgents. They are also secure, with cryptographically secured messages and wallets.
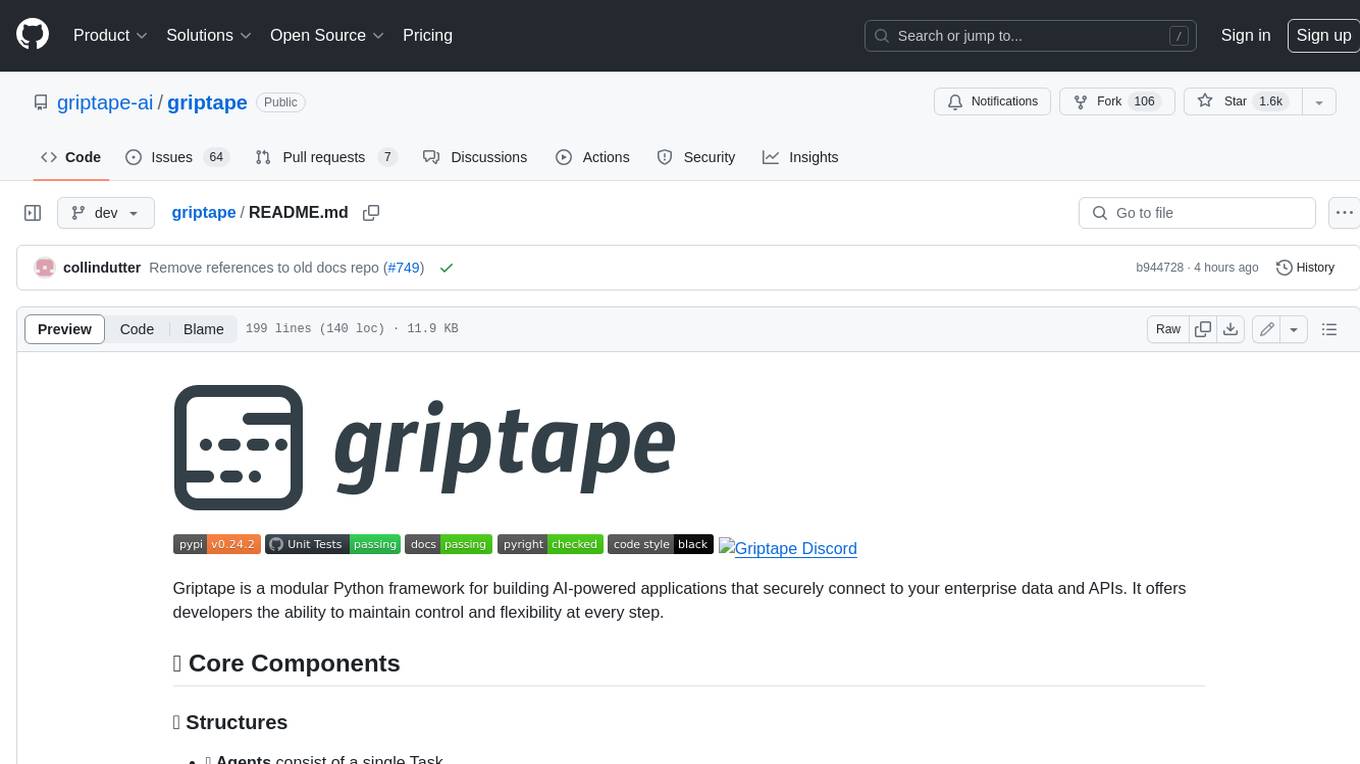
griptape
Griptape is a modular Python framework for building AI-powered applications that securely connect to your enterprise data and APIs. It offers developers the ability to maintain control and flexibility at every step. Griptape's core components include Structures (Agents, Pipelines, and Workflows), Tasks, Tools, Memory (Conversation Memory, Task Memory, and Meta Memory), Drivers (Prompt and Embedding Drivers, Vector Store Drivers, Image Generation Drivers, Image Query Drivers, SQL Drivers, Web Scraper Drivers, and Conversation Memory Drivers), Engines (Query Engines, Extraction Engines, Summary Engines, Image Generation Engines, and Image Query Engines), and additional components (Rulesets, Loaders, Artifacts, Chunkers, and Tokenizers). Griptape enables developers to create AI-powered applications with ease and efficiency.