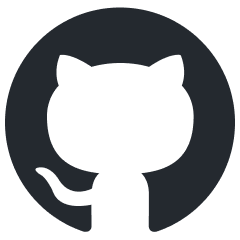
BTGenBot
BTGenBot: a system to generate behavior trees for robots using lightweight (~7 billion parameters) large language models (LLMs)
Stars: 65
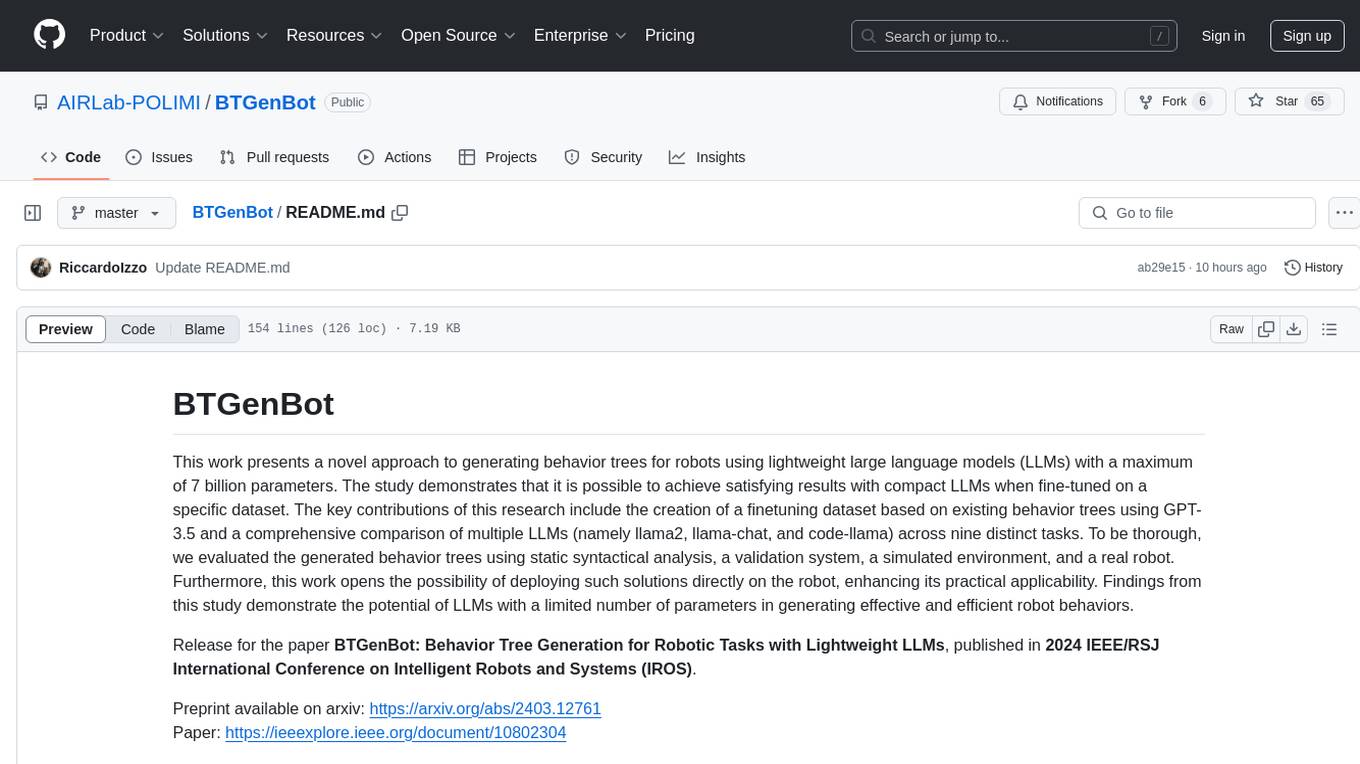
BTGenBot is a tool that generates behavior trees for robots using lightweight large language models (LLMs) with a maximum of 7 billion parameters. It fine-tunes on a specific dataset, compares multiple LLMs, and evaluates generated behavior trees using various methods. The tool demonstrates the potential of LLMs with a limited number of parameters in creating effective and efficient robot behaviors.
README:
This work presents a novel approach to generating behavior trees for robots using lightweight large language models (LLMs) with a maximum of 7 billion parameters. The study demonstrates that it is possible to achieve satisfying results with compact LLMs when fine-tuned on a specific dataset. The key contributions of this research include the creation of a finetuning dataset based on existing behavior trees using GPT-3.5 and a comprehensive comparison of multiple LLMs (namely llama2, llama-chat, and code-llama) across nine distinct tasks. To be thorough, we evaluated the generated behavior trees using static syntactical analysis, a validation system, a simulated environment, and a real robot. Furthermore, this work opens the possibility of deploying such solutions directly on the robot, enhancing its practical applicability. Findings from this study demonstrate the potential of LLMs with a limited number of parameters in generating effective and efficient robot behaviors.
Release for the paper BTGenBot: Behavior Tree Generation for Robotic Tasks with Lightweight LLMs, published in 2024 IEEE/RSJ International Conference on Intelligent Robots and Systems (IROS).
Preprint available on arxiv: https://arxiv.org/abs/2403.12761
Paper: https://ieeexplore.ieee.org/document/10802304
Dataset
, llama-2-7b-chat
and codellama-7b-instruct
LoRA adapters available on HuggingFace.
Authors: Riccardo Andrea Izzo, Gianluca Bardaro and Matteo Matteucci
Location: AIRLab (The Artificial Intelligence and Robotics Lab of Politecnico di Milano)
-
bt_client
: client designed to interpret and execute LLM-generated behavior trees directly on robot -
bt_generator
: demo notebook to load our fine-tuned models for generating behavior trees -
bt_validator
: validator that assess the overall correctness of the LLM-generated trees -
dataset
: our instruction-following dataset used to fine-tune the models -
lora_adapters
: LoRA adapters for the base models, used in the notebook to load the fine-tuned version -
prompt
: outcomes of prompts run on both LlamaChat and CodeLlama models, both in zero-shot and one-shot scenarios
Create a conda environment (or equivalent virtualenv):
conda create -n btgenbot python==3.10
Install dependencies:
pip install -r requirements.txt
Create a colcon workspace and clone this repository in your ROS2 workspace
Build:
colcon build
Required ROS2 dependencies:
-
BehaviorTree.CPP
: available here -
BehaviorTree.ROS2
: available here -
igus_rebel_commander
: available here, required only bybt_client
for the task involving arucos and arm activity -
aruco_interfaces
: available here, required only bybt_client
for the task involving arucos and arm activity
Tested on a Linux computer with Ubuntu 22.04 and ROS2 Humble
- Select the task and the corresponding behavior tree in XML format from the ones available in
/bt_xml
- Modify
config/tree.yaml
configuration file with the file name in thetree_name
field:
tree_name: "main_tree.xml"
To add a new behavior tree, follow these steps:
- Create an XML file representing the behavior tree
- Place the XML file in the
/bt_xml
folder - Specify the name of the XML file in the
config/tree.yaml
configuration file
- Build and source the package
colcon build
source install/setup.bash
- Launch the client and execute the selected behavior tree
ros2 launch bt_client bt.launch.py
Keep in mind that the system offers a range of pre-defined node functionalities. For instance, the "MoveTo" action facilitates the sending of a navigation goal to the Nav2 server, utilizing the goal specified within the behavior tree XML.
Moreover, locations for testing purposes are outlined in the location.yaml configuration file. These locations are pre-defined and serve as references for navigation tasks.
It is possible to add further actions with
factory.registerNodeType<ACTION_NAME>("ACTION_NAME");
- Launch the pipeline
ros2 launch bt_client monitor.launch.py
This command initiates the pipeline. Once the behavior tree specified in the config/tree.yaml
configuration file becomes available, the client will automatically execute it.
This behavior tree is intended to be the one generated by the LLM, for example with inference.ipynb
or btgenbot.py
.
Explore a demonstration notebook showcasing the generation of behavior trees utilizing llamachat and codellama, featuring both zero-shot and one-shot prompts.
Client application with GUI that generates a behavior tree given a new task description. After generating the behavior tree, the application saves it to a file and initiates its transmission to the remote location of a robot for immediate execution.
Two modes are available:
-
Standard Mode
: this mode requires a comprehensive one-shot example that includes a description and its corresponding behavior tree, in addition to the new task description. Recommended for new or specialized tasks. -
Automatic Retrieval Mode
: in this mode, only the new task description is required. Users can select the task domain to automatically infer a one-shot example from a list of predefined ones in a YAML file. Ideal for straightforward tasks similar to those demonstrated in the examples.
Steps:
- Update the
config/params.yaml
configuration file with SSH connection parameters and your HuggingFace access token. Define thelocal_dir
parameter to specify the local directory where XML behavior trees will be saved, and setremote_dir
to indicate the corresponding remote location on the robot where the trees should be stored. - Run the script
python3 btgenbot.py
- Build and source the package
colcon build
source install/setup.bash
- Launch the validator from the root directory
./build/bt_validator/main
If you use this work in your research, please consider citing our paper:
@INPROCEEDINGS{10802304,
author={Izzo, Riccardo Andrea and Bardaro, Gianluca and Matteucci, Matteo},
booktitle={2024 IEEE/RSJ International Conference on Intelligent Robots and Systems (IROS)},
title={BTGenBot: Behavior Tree Generation for Robotic Tasks with Lightweight LLMs},
year={2024},
volume={},
number={},
pages={9684-9690},
keywords={Accuracy;Service robots;Large language models;Semantics;Natural languages;XML;Syntactics;Robots;Intelligent robots;Logistics},
doi={10.1109/IROS58592.2024.10802304}}
For Tasks:
Click tags to check more tools for each tasksFor Jobs:
Alternative AI tools for BTGenBot
Similar Open Source Tools
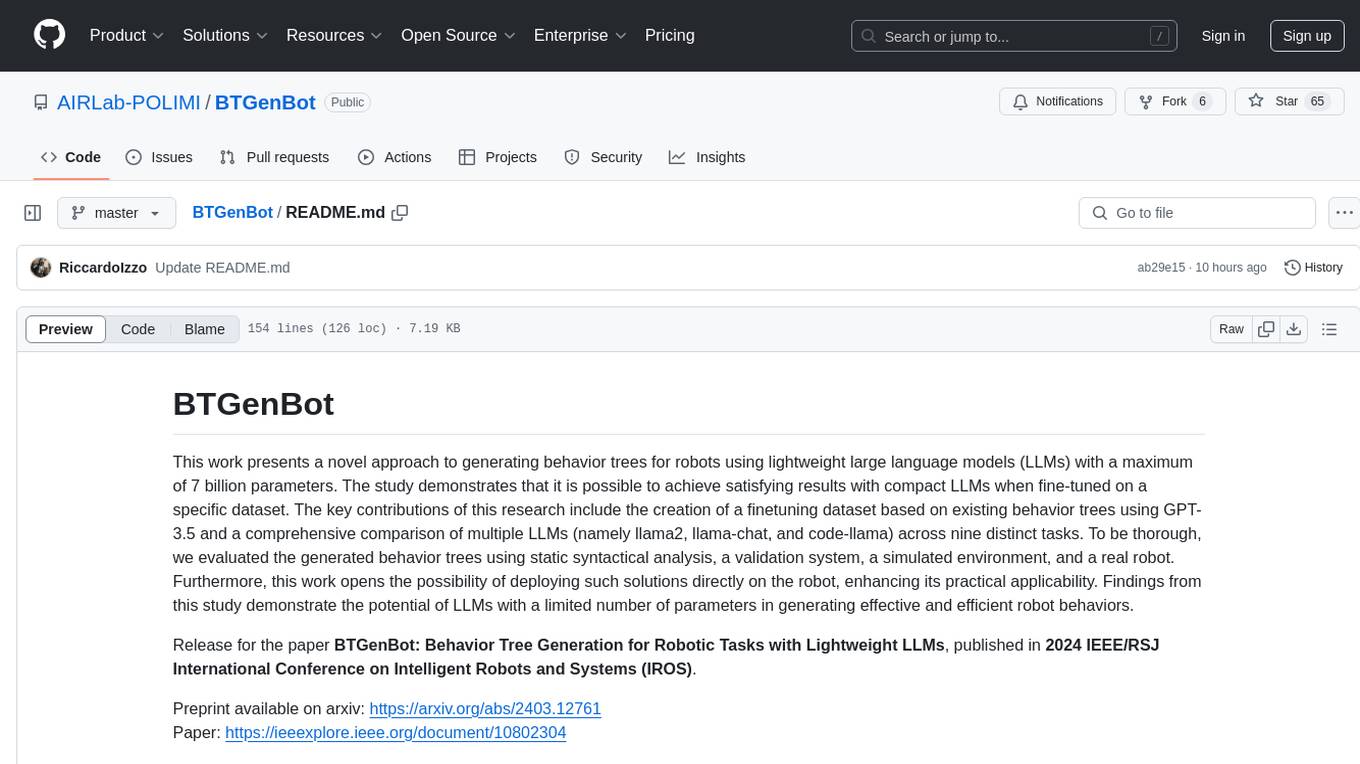
BTGenBot
BTGenBot is a tool that generates behavior trees for robots using lightweight large language models (LLMs) with a maximum of 7 billion parameters. It fine-tunes on a specific dataset, compares multiple LLMs, and evaluates generated behavior trees using various methods. The tool demonstrates the potential of LLMs with a limited number of parameters in creating effective and efficient robot behaviors.
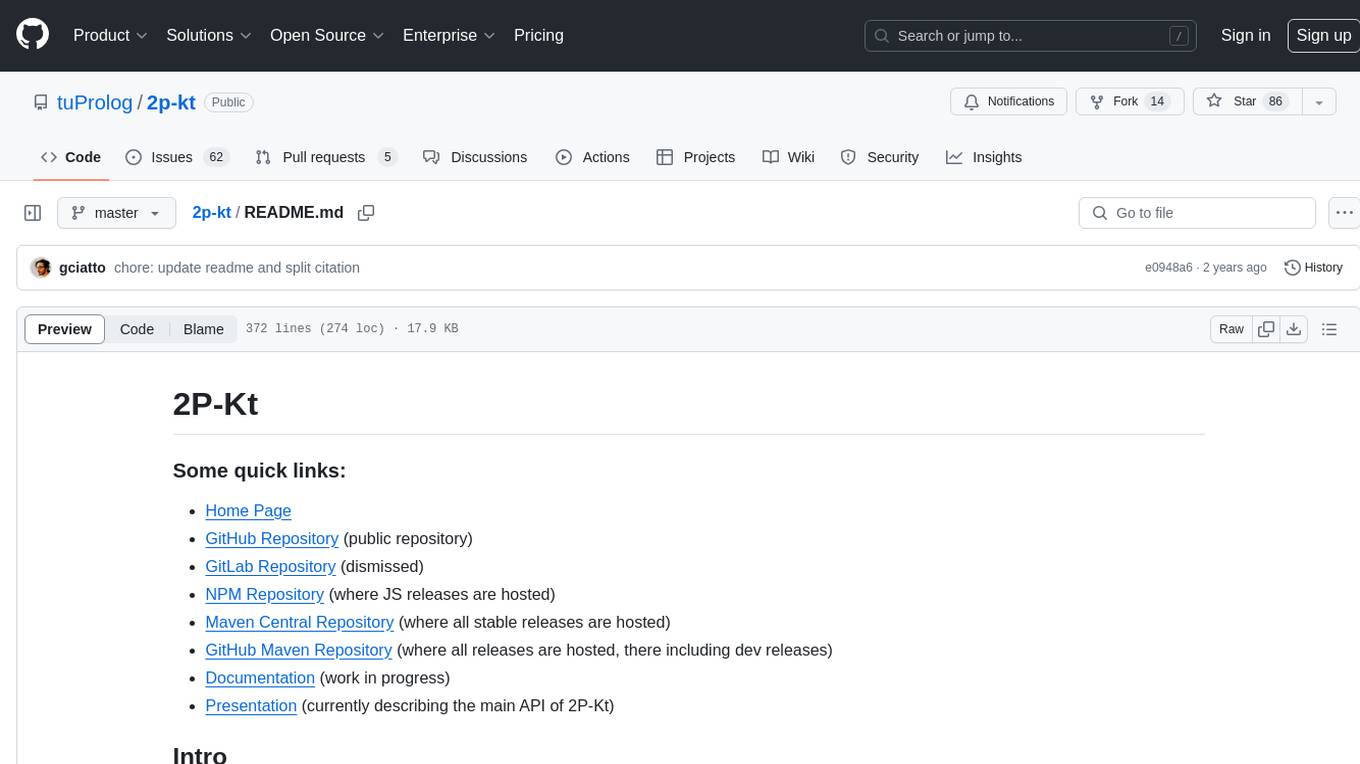
2p-kt
2P-Kt is a Kotlin-based and multi-platform reboot of tuProlog (2P), a multi-paradigm logic programming framework written in Java. It consists of an open ecosystem for Symbolic Artificial Intelligence (AI) with modules supporting logic terms, unification, indexing, resolution of logic queries, probabilistic logic programming, binary decision diagrams, OR-concurrent resolution, DSL for logic programming, parsing modules, serialisation modules, command-line interface, and graphical user interface. The tool is designed to support knowledge representation and automatic reasoning through logic programming in an extensible and flexible way, encouraging extensions towards other symbolic AI systems than Prolog. It is a pure, multi-platform Kotlin project supporting JVM, JS, Android, and Native platforms, with a lightweight library leveraging the Kotlin common library.
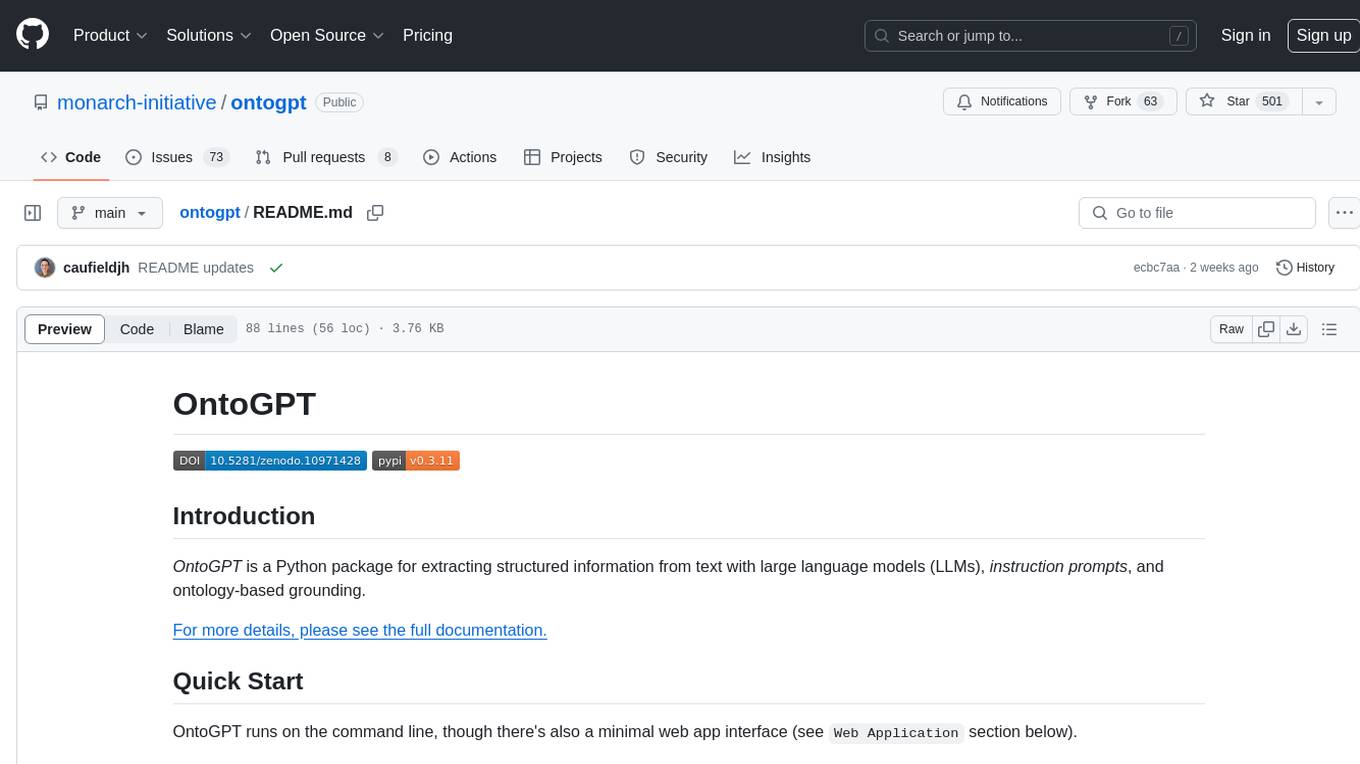
ontogpt
OntoGPT is a Python package for extracting structured information from text using large language models, instruction prompts, and ontology-based grounding. It provides a command line interface and a minimal web app for easy usage. The tool has been evaluated on test data and is used in related projects like TALISMAN for gene set analysis. OntoGPT enables users to extract information from text by specifying relevant terms and provides the extracted objects as output.
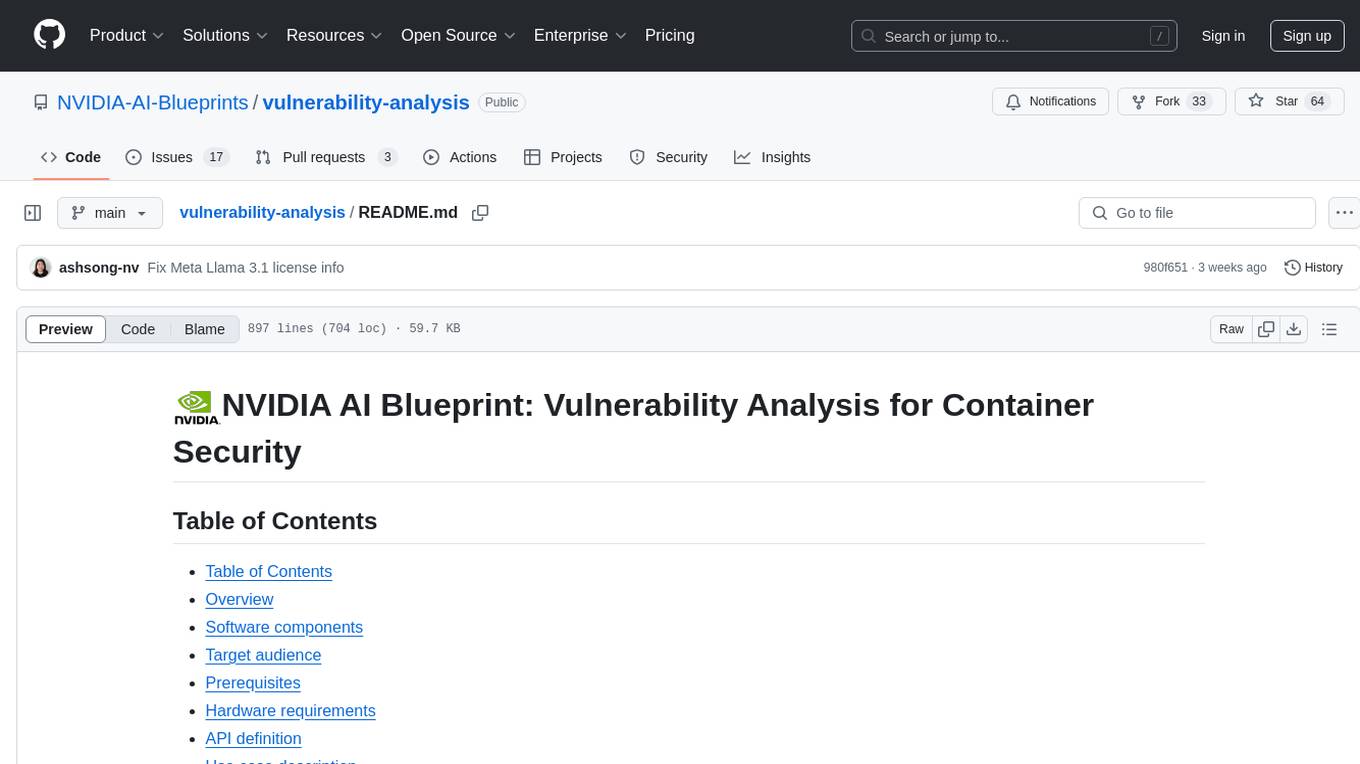
vulnerability-analysis
The NVIDIA AI Blueprint for Vulnerability Analysis for Container Security showcases accelerated analysis on common vulnerabilities and exposures (CVE) at an enterprise scale, reducing mitigation time from days to seconds. It enables security analysts to determine software package vulnerabilities using large language models (LLMs) and retrieval-augmented generation (RAG). The blueprint is designed for security analysts, IT engineers, and AI practitioners in cybersecurity. It requires NVAIE developer license and API keys for vulnerability databases, search engines, and LLM model services. Hardware requirements include L40 GPU for pipeline operation and optional LLM NIM and Embedding NIM. The workflow involves LLM pipeline for CVE impact analysis, utilizing LLM planner, agent, and summarization nodes. The blueprint uses NVIDIA NIM microservices and Morpheus Cybersecurity AI SDK for vulnerability analysis.
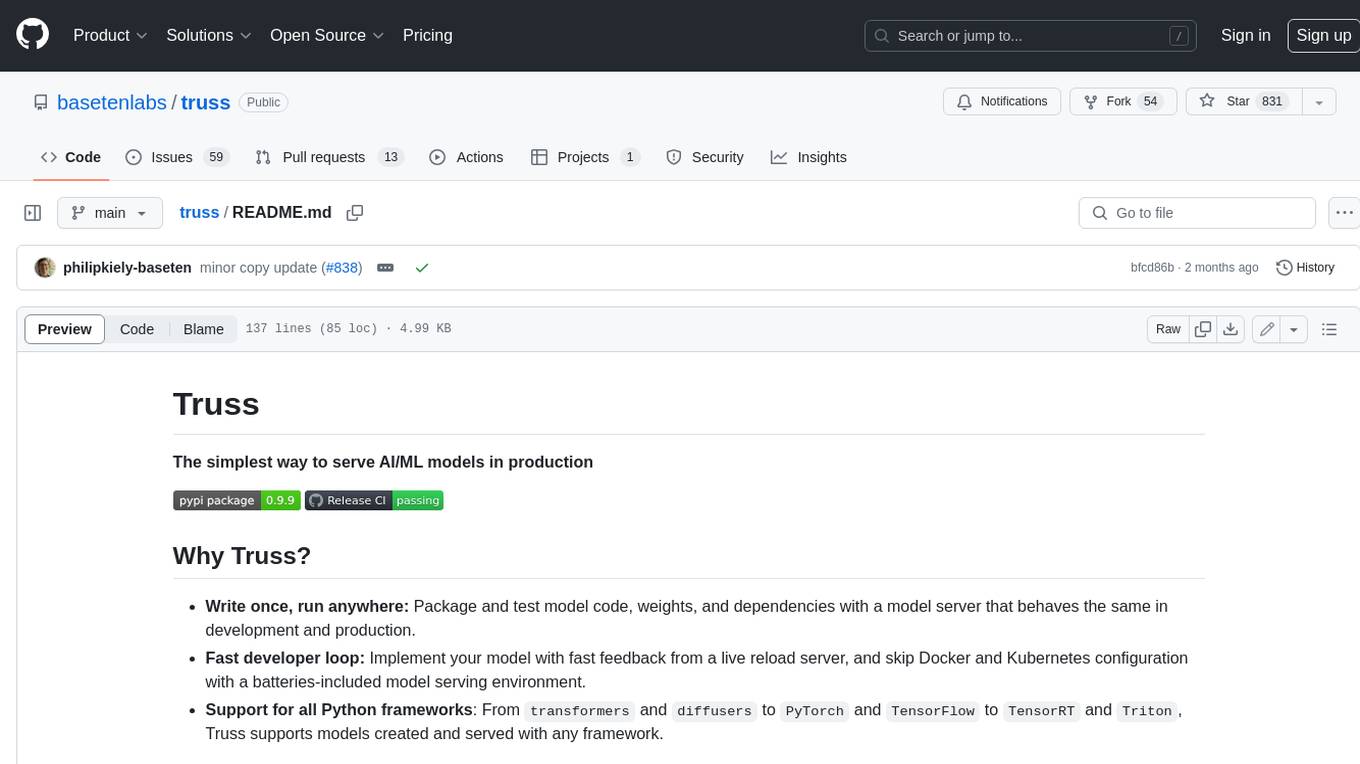
truss
Truss is a tool that simplifies the process of serving AI/ML models in production. It provides a consistent and easy-to-use interface for packaging, testing, and deploying models, regardless of the framework they were created with. Truss also includes a live reload server for fast feedback during development, and a batteries-included model serving environment that eliminates the need for Docker and Kubernetes configuration.
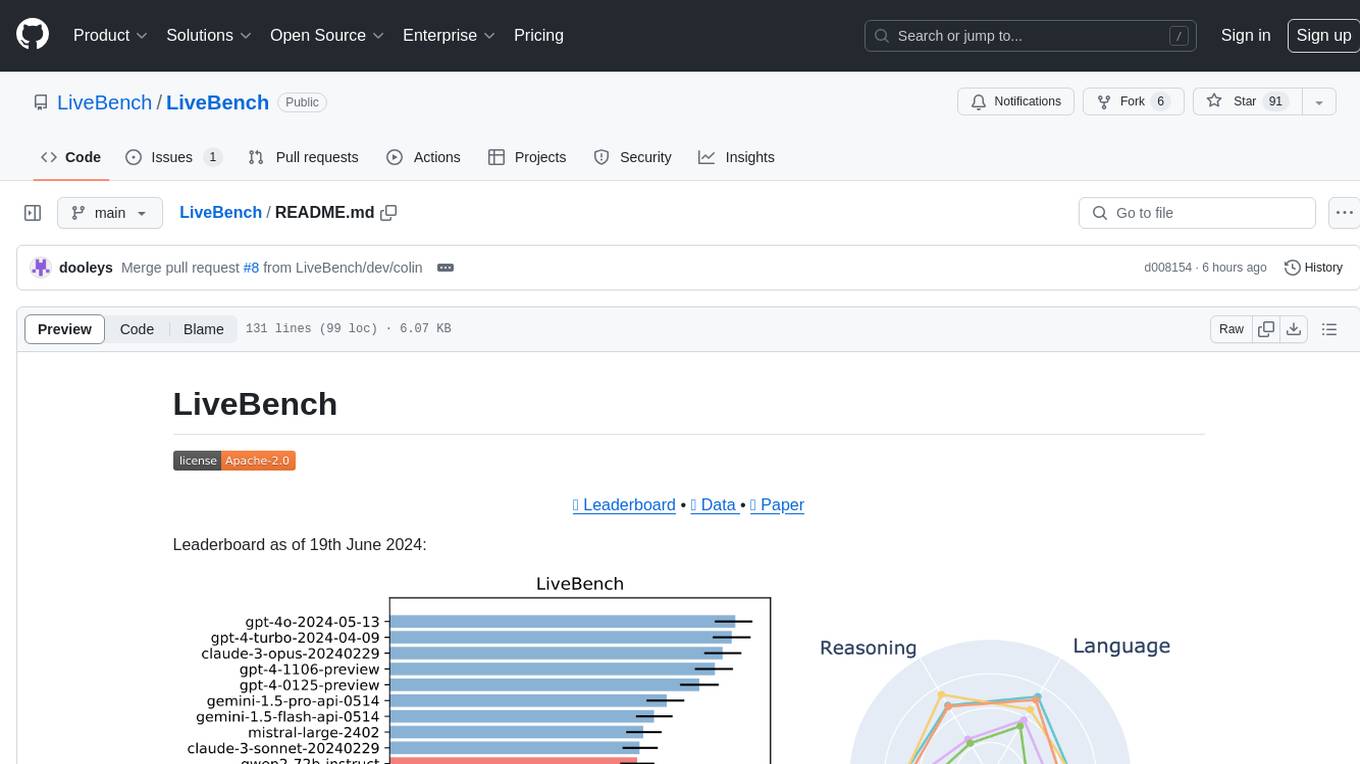
LiveBench
LiveBench is a benchmark tool designed for Language Model Models (LLMs) with a focus on limiting contamination through monthly new questions based on recent datasets, arXiv papers, news articles, and IMDb movie synopses. It provides verifiable, objective ground-truth answers for accurate scoring without an LLM judge. The tool offers 18 diverse tasks across 6 categories and promises to release more challenging tasks over time. LiveBench is built on FastChat's llm_judge module and incorporates code from LiveCodeBench and IFEval.
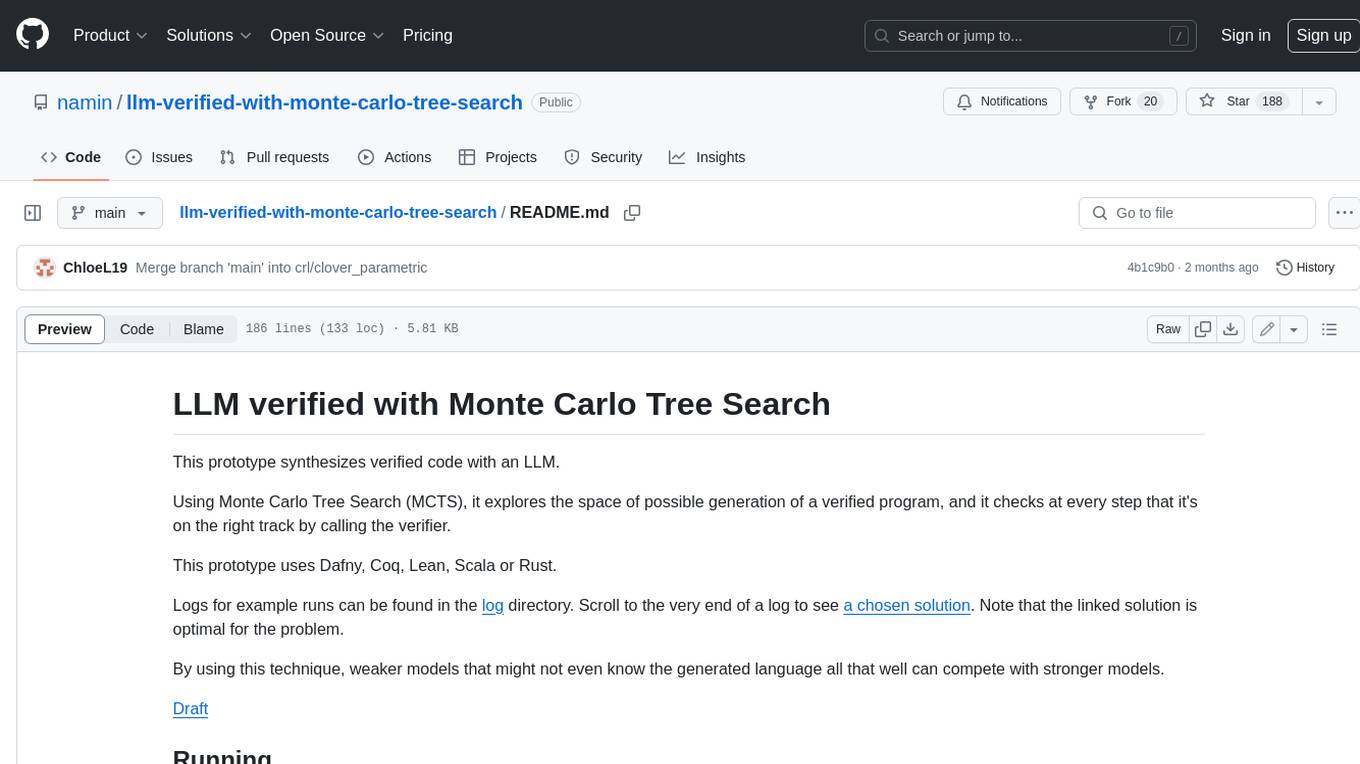
llm-verified-with-monte-carlo-tree-search
This prototype synthesizes verified code with an LLM using Monte Carlo Tree Search (MCTS). It explores the space of possible generation of a verified program and checks at every step that it's on the right track by calling the verifier. This prototype uses Dafny, Coq, Lean, Scala, or Rust. By using this technique, weaker models that might not even know the generated language all that well can compete with stronger models.
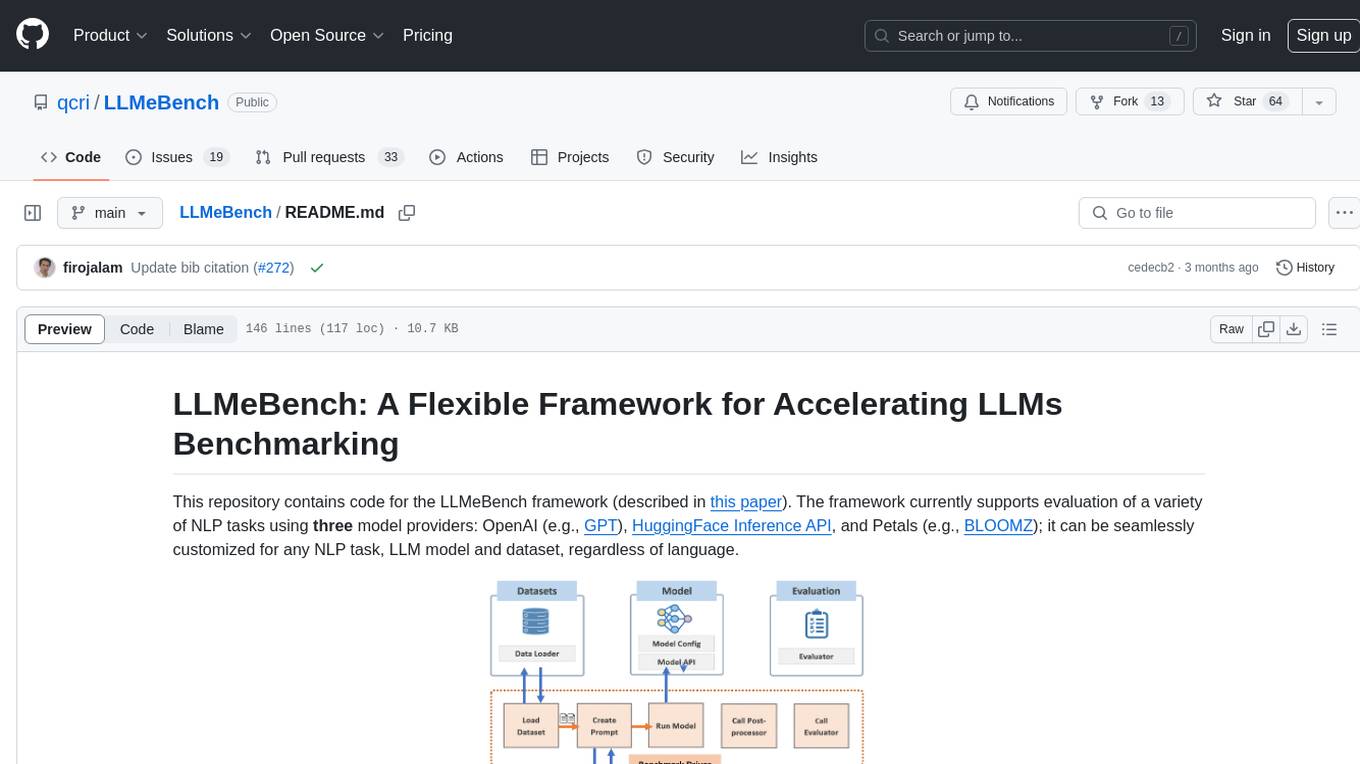
LLMeBench
LLMeBench is a flexible framework designed for accelerating benchmarking of Large Language Models (LLMs) in the field of Natural Language Processing (NLP). It supports evaluation of various NLP tasks using model providers like OpenAI, HuggingFace Inference API, and Petals. The framework is customizable for different NLP tasks, LLM models, and datasets across multiple languages. It features extensive caching capabilities, supports zero- and few-shot learning paradigms, and allows on-the-fly dataset download and caching. LLMeBench is open-source and continuously expanding to support new models accessible through APIs.
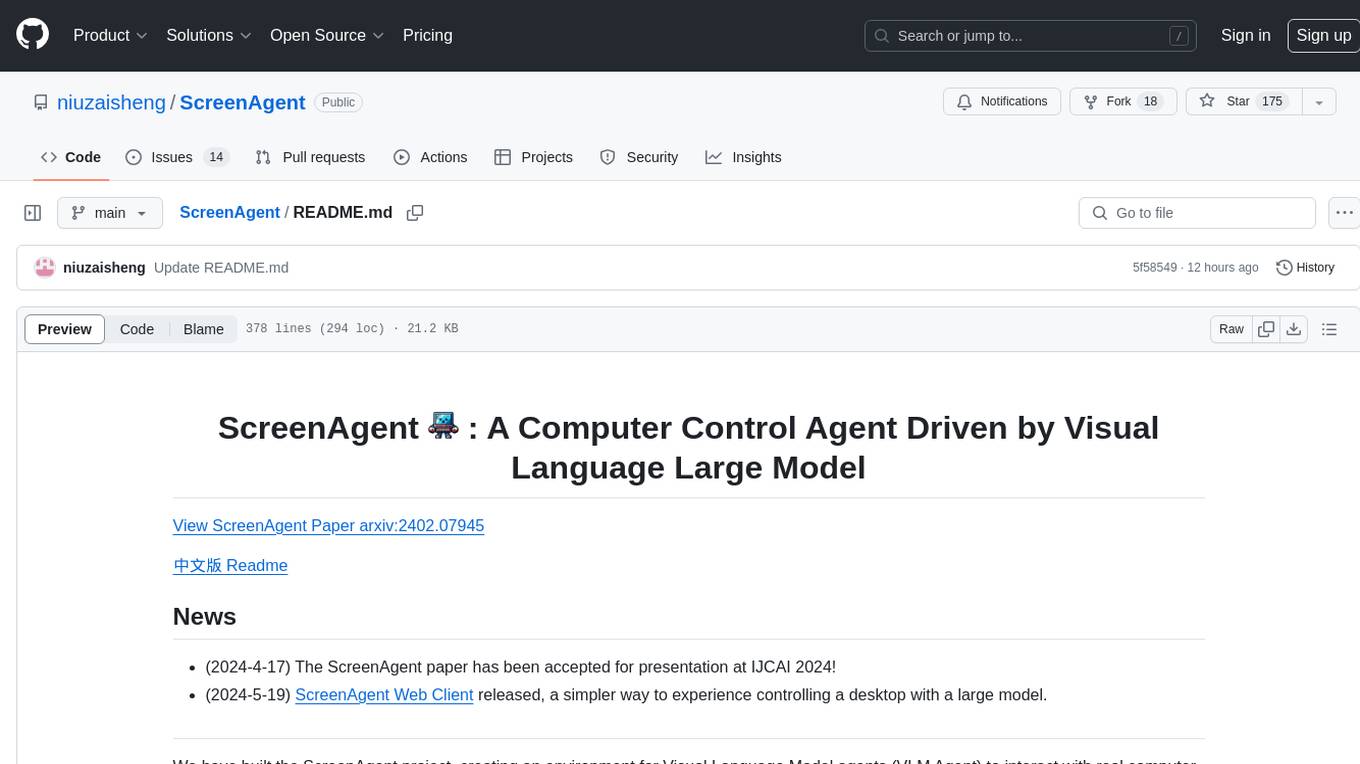
ScreenAgent
ScreenAgent is a project focused on creating an environment for Visual Language Model agents (VLM Agent) to interact with real computer screens. The project includes designing an automatic control process for agents to interact with the environment and complete multi-step tasks. It also involves building the ScreenAgent dataset, which collects screenshots and action sequences for various daily computer tasks. The project provides a controller client code, configuration files, and model training code to enable users to control a desktop with a large model.
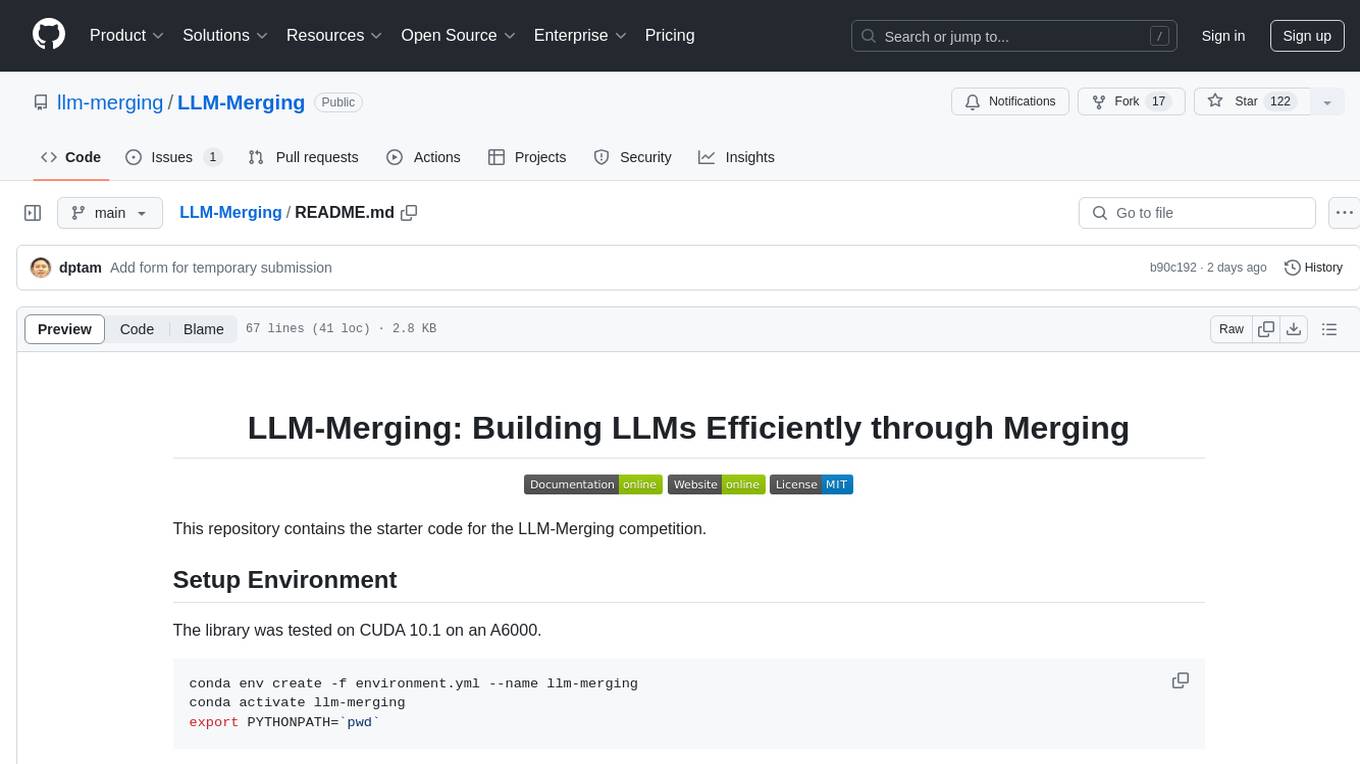
LLM-Merging
LLM-Merging is a repository containing starter code for the LLM-Merging competition. It provides a platform for efficiently building LLMs through merging methods. Users can develop new merging methods by creating new files in the specified directory and extending existing classes. The repository includes instructions for setting up the environment, developing new merging methods, testing the methods on specific datasets, and submitting solutions for evaluation. It aims to facilitate the development and evaluation of merging methods for LLMs.
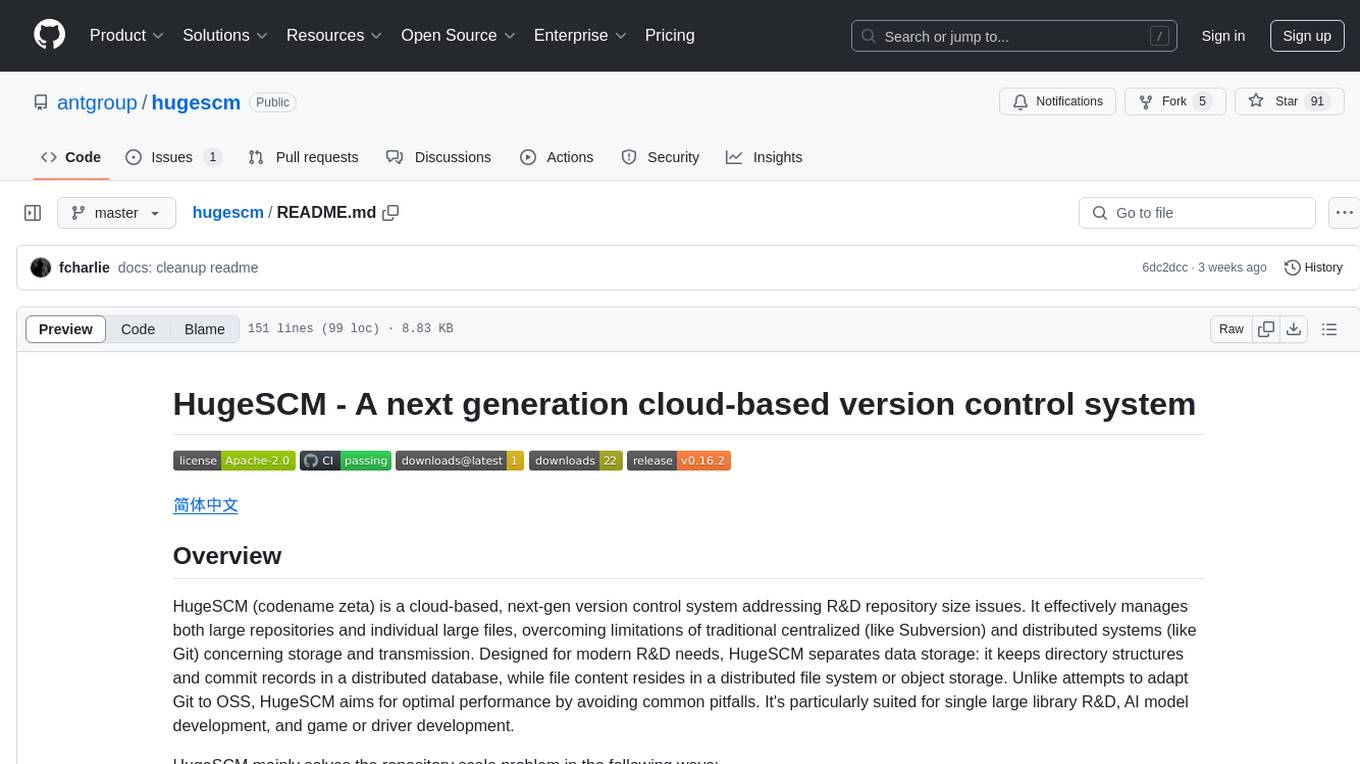
hugescm
HugeSCM is a cloud-based version control system designed to address R&D repository size issues. It effectively manages large repositories and individual large files by separating data storage and utilizing advanced algorithms and data structures. It aims for optimal performance in handling version control operations of large-scale repositories, making it suitable for single large library R&D, AI model development, and game or driver development.
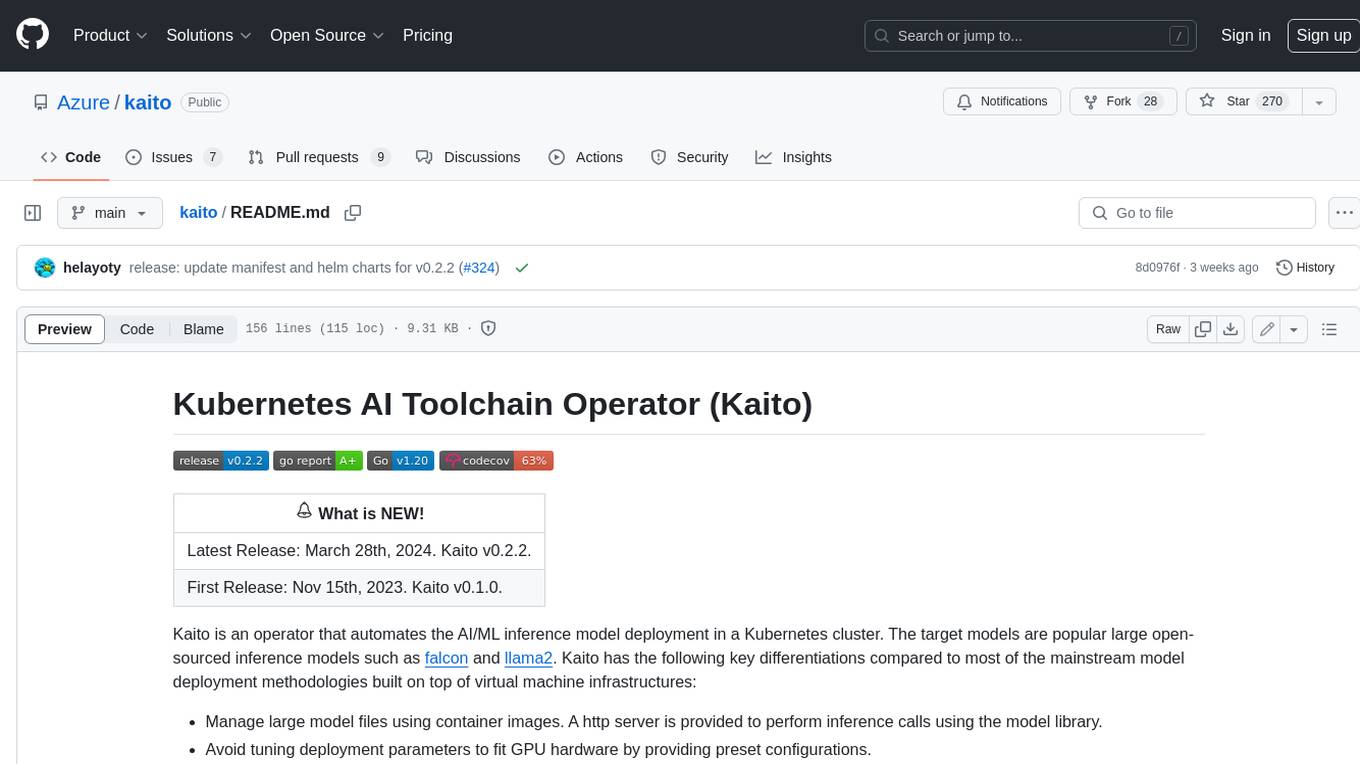
kaito
Kaito is an operator that automates the AI/ML inference model deployment in a Kubernetes cluster. It manages large model files using container images, avoids tuning deployment parameters to fit GPU hardware by providing preset configurations, auto-provisions GPU nodes based on model requirements, and hosts large model images in the public Microsoft Container Registry (MCR) if the license allows. Using Kaito, the workflow of onboarding large AI inference models in Kubernetes is largely simplified.
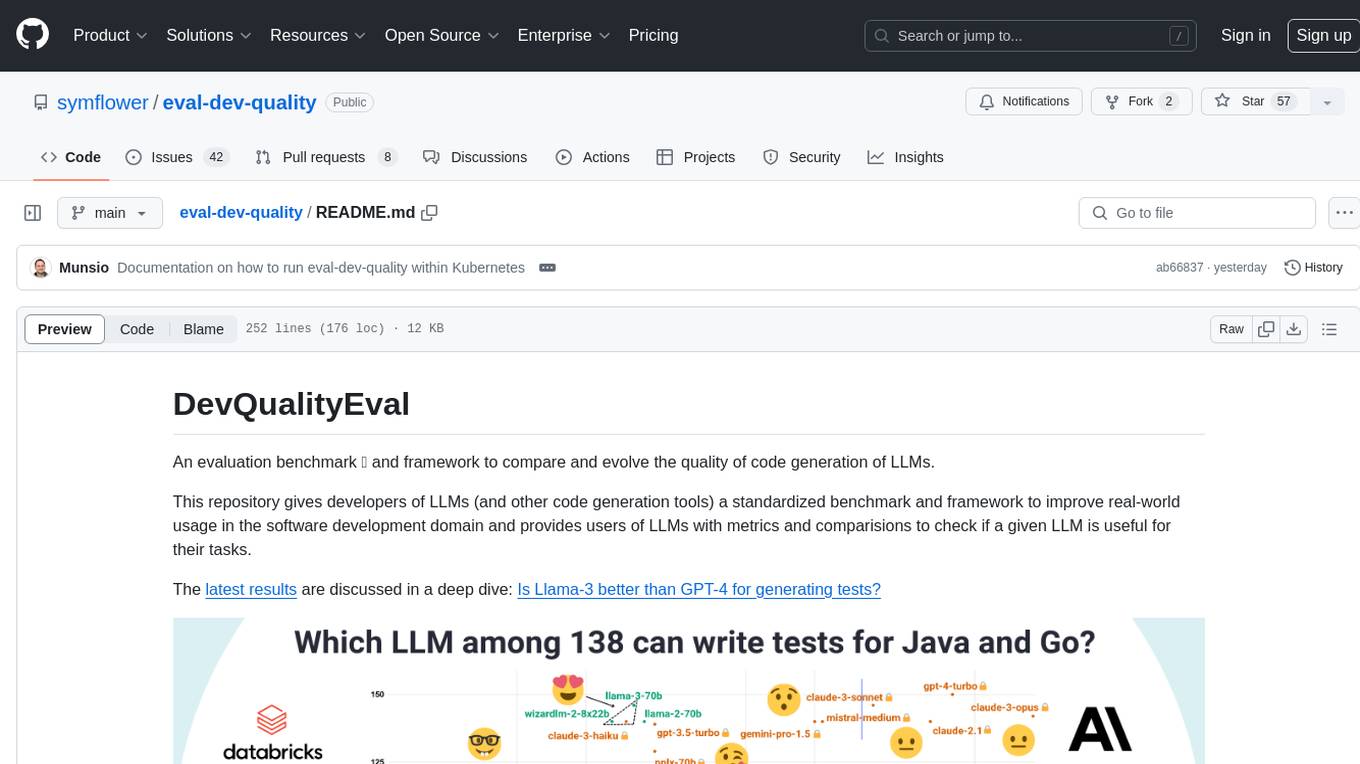
eval-dev-quality
DevQualityEval is an evaluation benchmark and framework designed to compare and improve the quality of code generation of Language Model Models (LLMs). It provides developers with a standardized benchmark to enhance real-world usage in software development and offers users metrics and comparisons to assess the usefulness of LLMs for their tasks. The tool evaluates LLMs' performance in solving software development tasks and measures the quality of their results through a point-based system. Users can run specific tasks, such as test generation, across different programming languages to evaluate LLMs' language understanding and code generation capabilities.
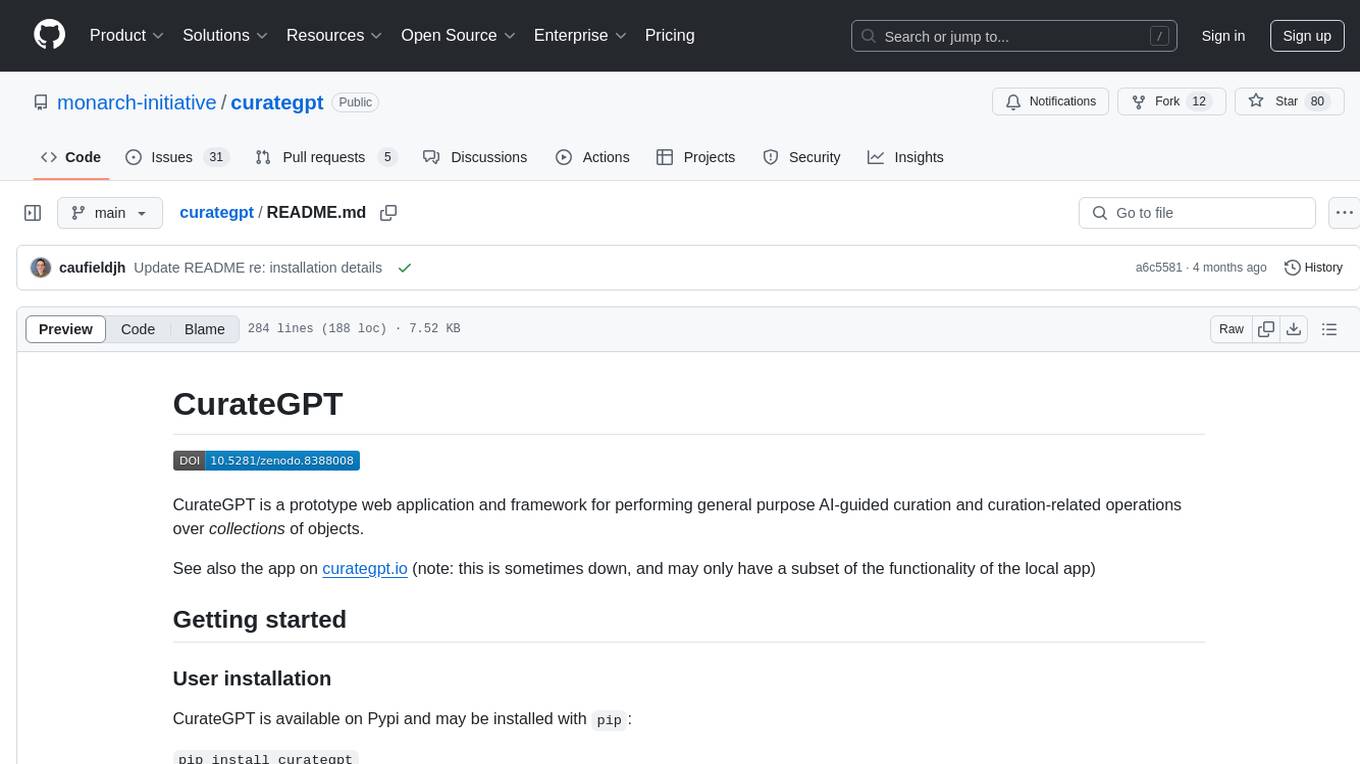
curategpt
CurateGPT is a prototype web application and framework designed for general purpose AI-guided curation and curation-related operations over collections of objects. It provides functionalities for loading example data, building indexes, interacting with knowledge bases, and performing tasks such as chatting with a knowledge base, querying Pubmed, interacting with a GitHub issue tracker, term autocompletion, and all-by-all comparisons. The tool is built to work best with the OpenAI gpt-4 model and OpenAI ada-text-embedding-002 for embedding, but also supports alternative models through a plugin architecture.
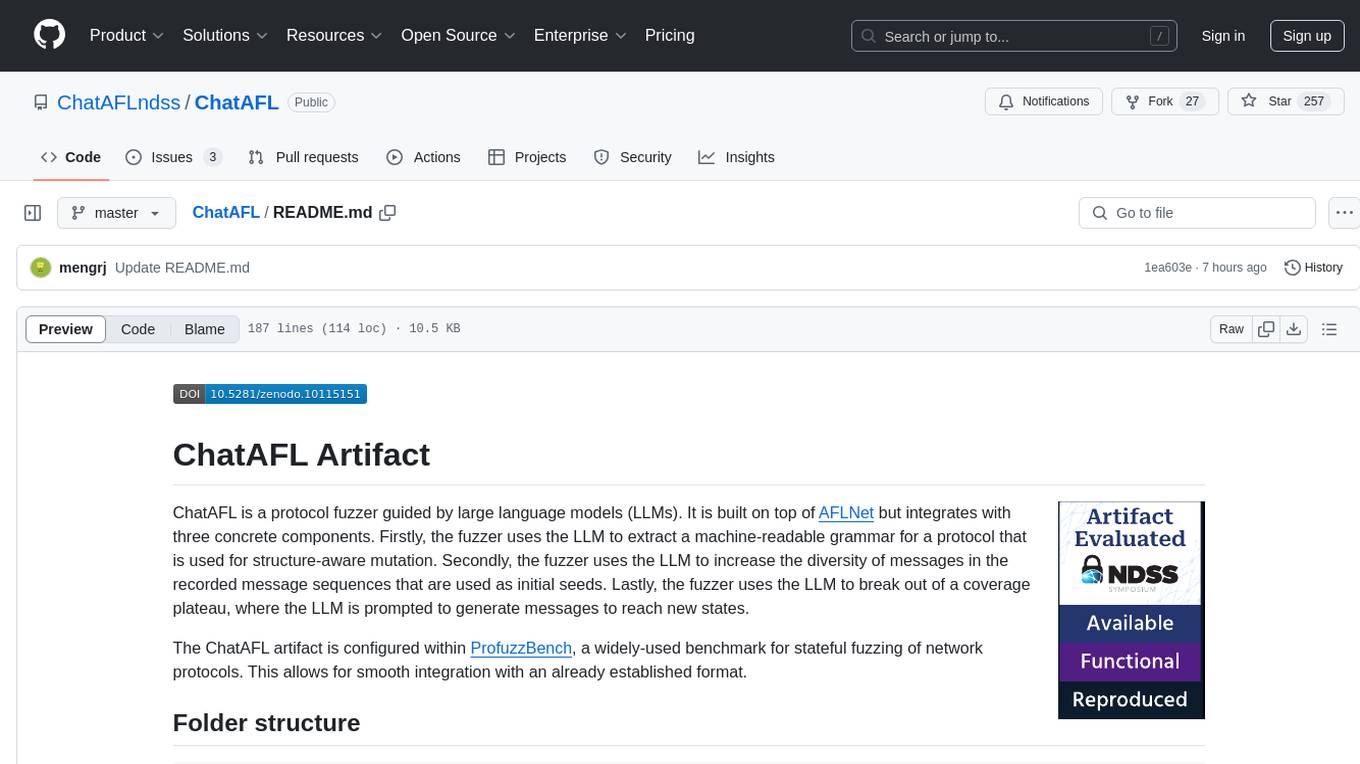
ChatAFL
ChatAFL is a protocol fuzzer guided by large language models (LLMs) that extracts machine-readable grammar for protocol mutation, increases message diversity, and breaks coverage plateaus. It integrates with ProfuzzBench for stateful fuzzing of network protocols, providing smooth integration. The artifact includes modified versions of AFLNet and ProfuzzBench, source code for ChatAFL with proposed strategies, and scripts for setup, execution, analysis, and cleanup. Users can analyze data, construct plots, examine LLM-generated grammars, enriched seeds, and state-stall responses, and reproduce results with downsized experiments. Customization options include modifying fuzzers, tuning parameters, adding new subjects, troubleshooting, and working on GPT-4. Limitations include interaction with OpenAI's Large Language Models and a hard limit of 150,000 tokens per minute.
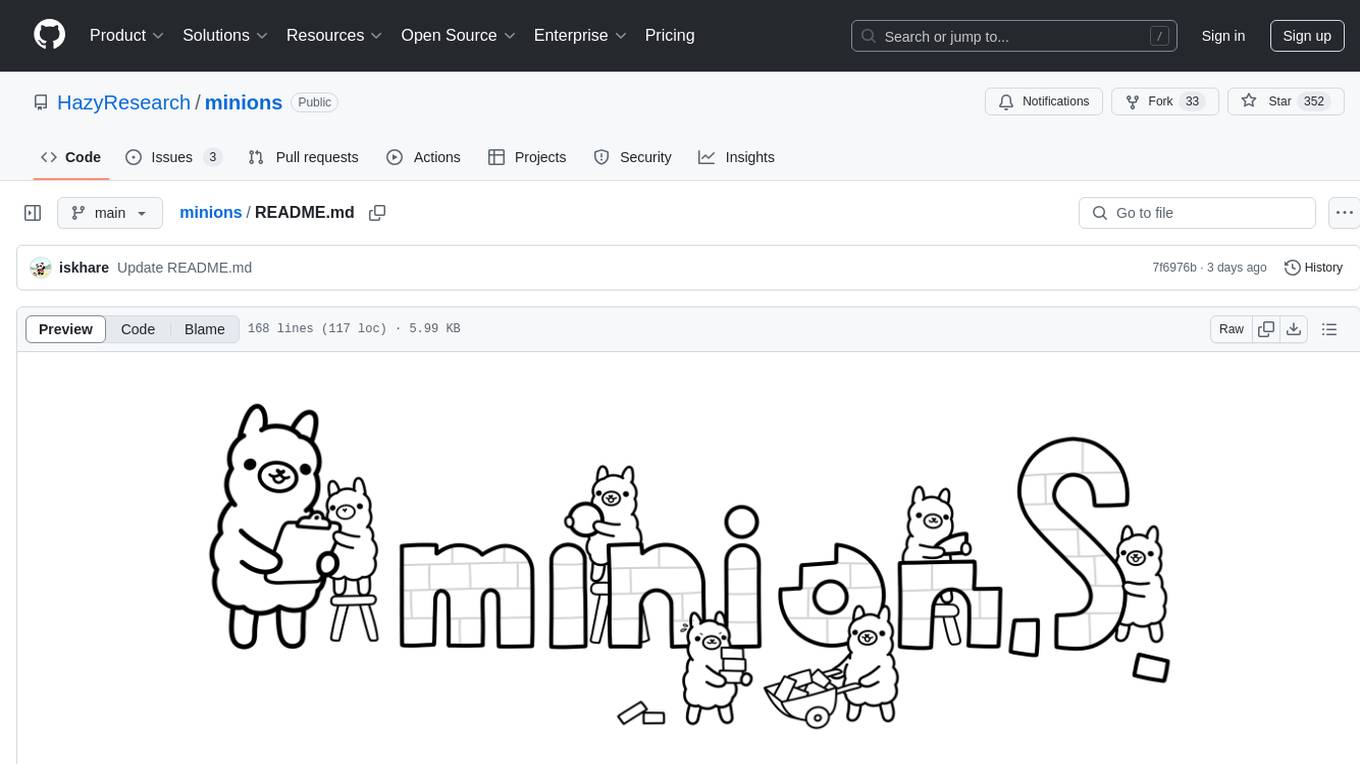
minions
Minions is a communication protocol that enables small on-device models to collaborate with frontier models in the cloud. By only reading long contexts locally, it reduces cloud costs with minimal or no quality degradation. The repository provides a demonstration of the protocol.
For similar tasks
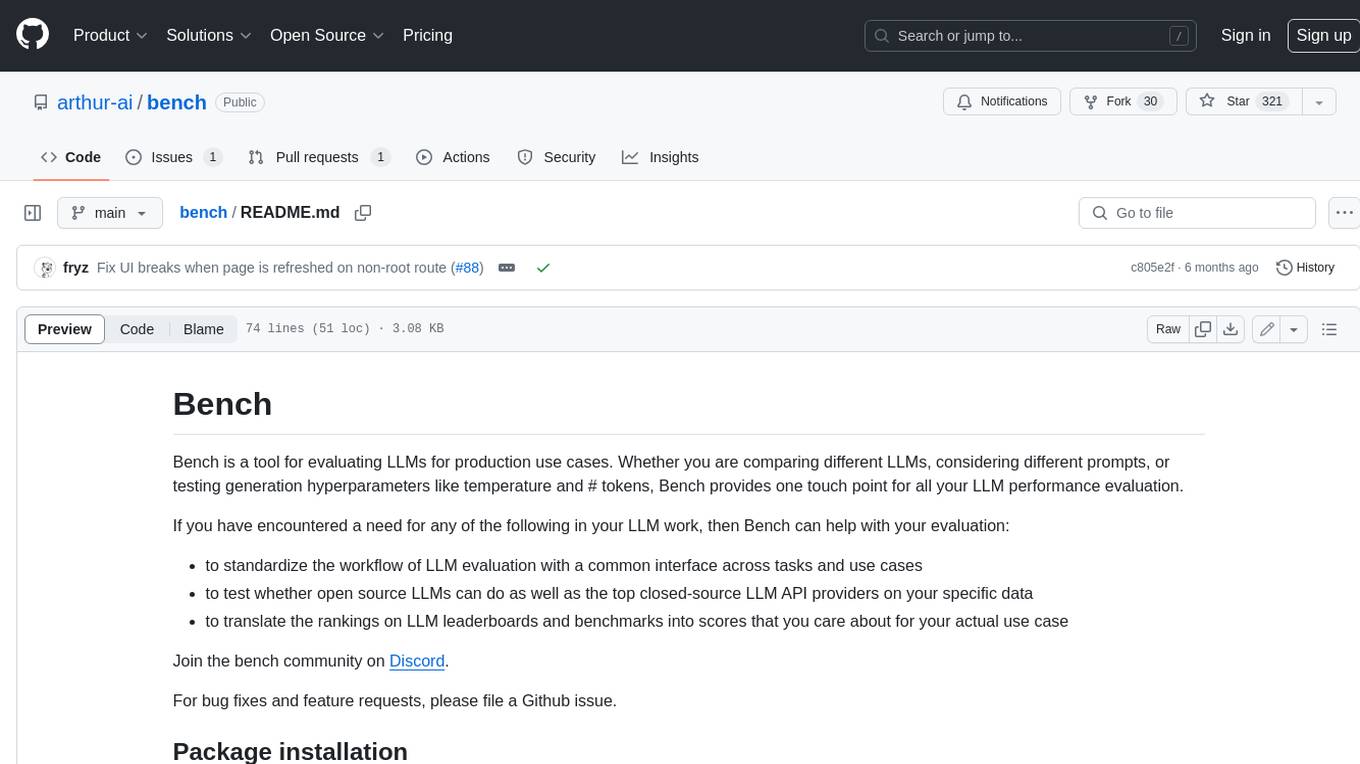
bench
Bench is a tool for evaluating LLMs for production use cases. It provides a standardized workflow for LLM evaluation with a common interface across tasks and use cases. Bench can be used to test whether open source LLMs can do as well as the top closed-source LLM API providers on specific data, and to translate the rankings on LLM leaderboards and benchmarks into scores that are relevant for actual use cases.
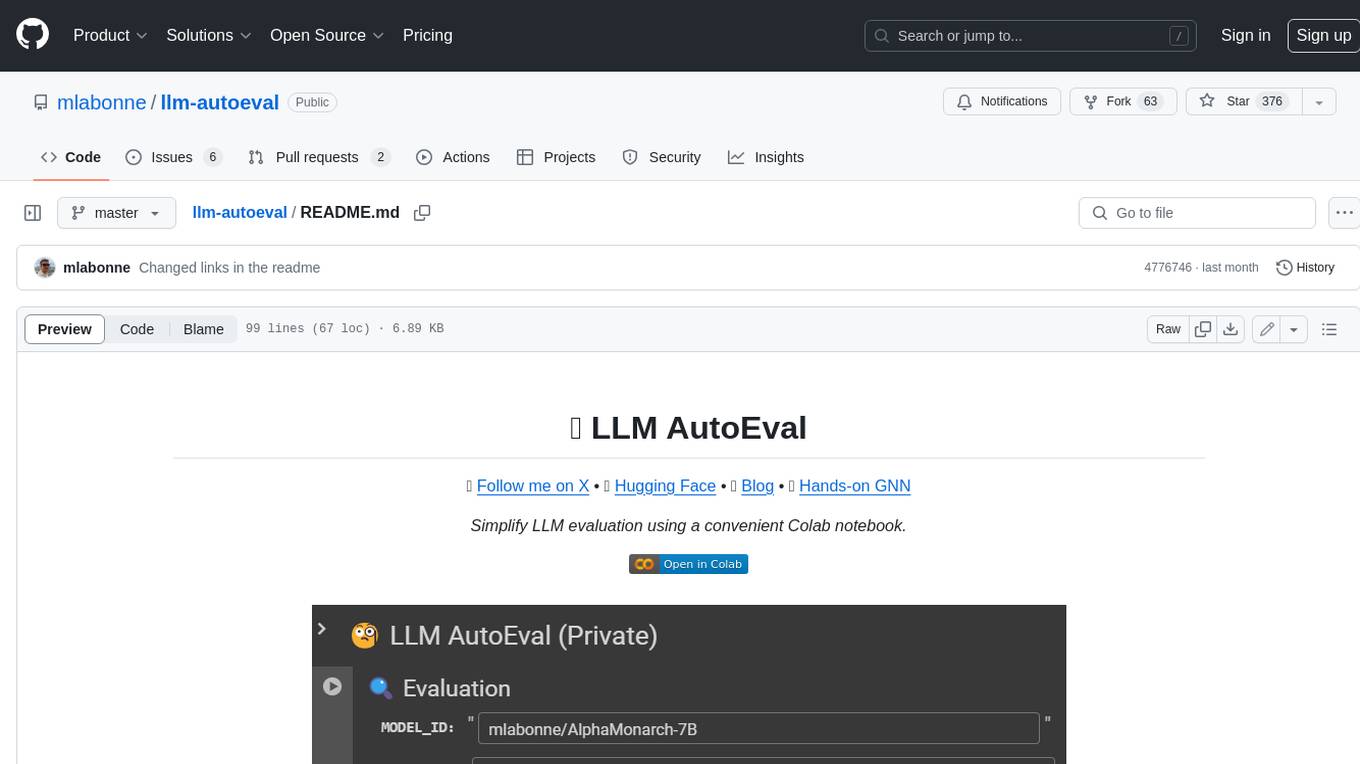
llm-autoeval
LLM AutoEval is a tool that simplifies the process of evaluating Large Language Models (LLMs) using a convenient Colab notebook. It automates the setup and execution of evaluations using RunPod, allowing users to customize evaluation parameters and generate summaries that can be uploaded to GitHub Gist for easy sharing and reference. LLM AutoEval supports various benchmark suites, including Nous, Lighteval, and Open LLM, enabling users to compare their results with existing models and leaderboards.
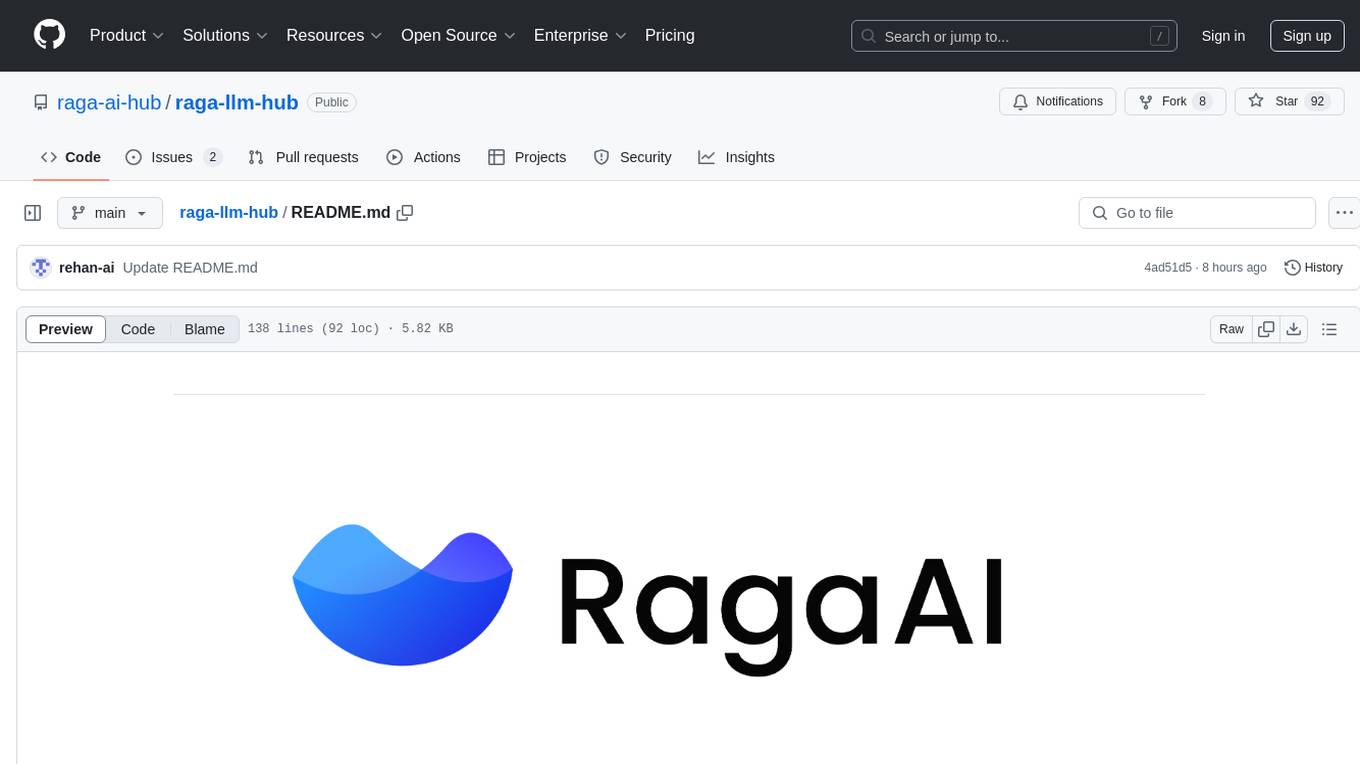
raga-llm-hub
Raga LLM Hub is a comprehensive evaluation toolkit for Language and Learning Models (LLMs) with over 100 meticulously designed metrics. It allows developers and organizations to evaluate and compare LLMs effectively, establishing guardrails for LLMs and Retrieval Augmented Generation (RAG) applications. The platform assesses aspects like Relevance & Understanding, Content Quality, Hallucination, Safety & Bias, Context Relevance, Guardrails, and Vulnerability scanning, along with Metric-Based Tests for quantitative analysis. It helps teams identify and fix issues throughout the LLM lifecycle, revolutionizing reliability and trustworthiness.
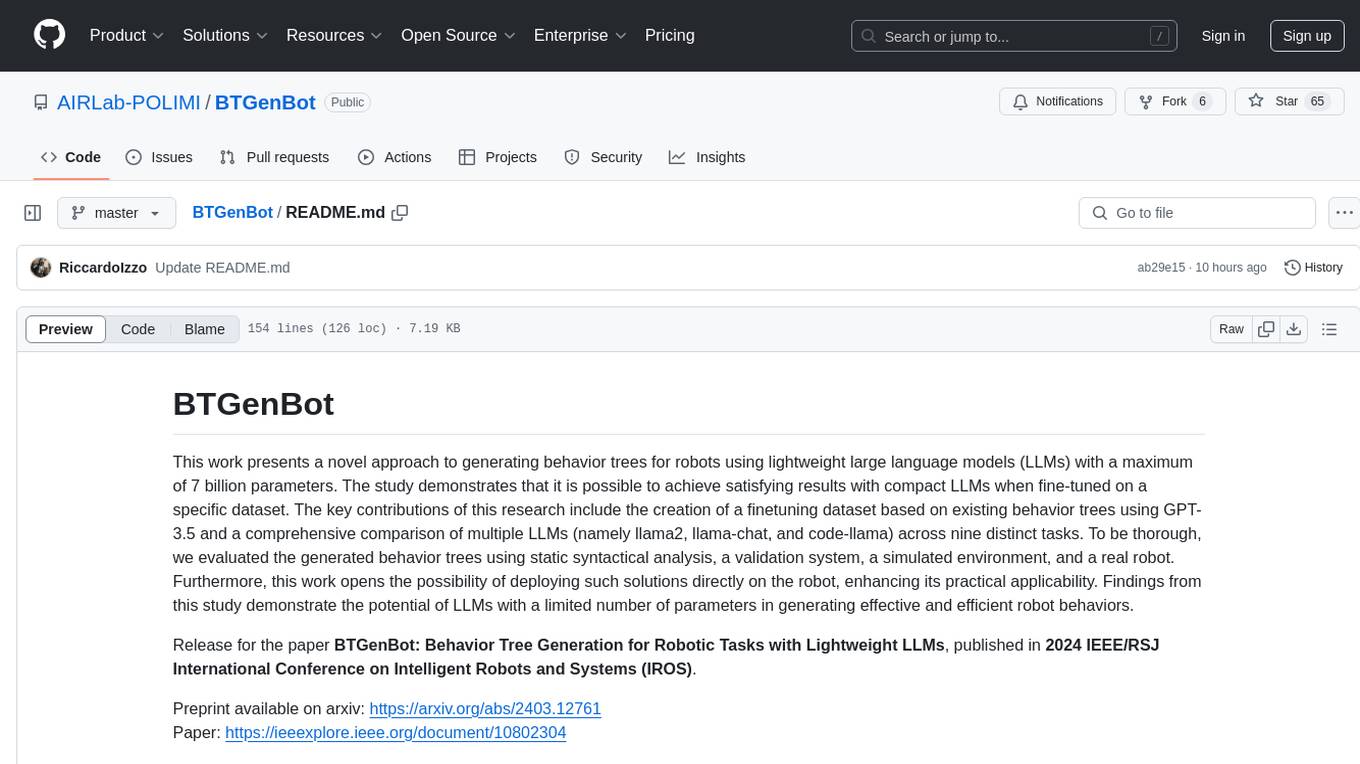
BTGenBot
BTGenBot is a tool that generates behavior trees for robots using lightweight large language models (LLMs) with a maximum of 7 billion parameters. It fine-tunes on a specific dataset, compares multiple LLMs, and evaluates generated behavior trees using various methods. The tool demonstrates the potential of LLMs with a limited number of parameters in creating effective and efficient robot behaviors.
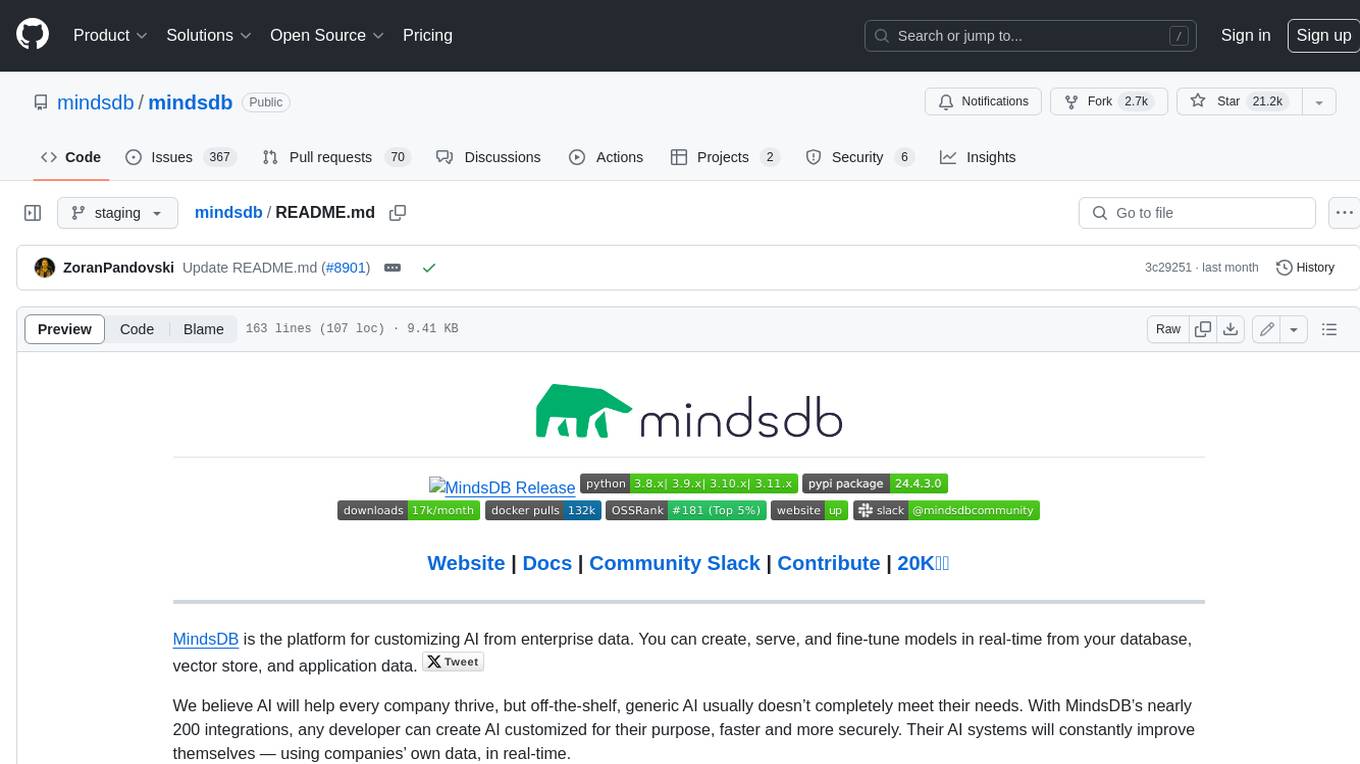
mindsdb
MindsDB is a platform for customizing AI from enterprise data. You can create, serve, and fine-tune models in real-time from your database, vector store, and application data. MindsDB "enhances" SQL syntax with AI capabilities to make it accessible for developers worldwide. With MindsDB’s nearly 200 integrations, any developer can create AI customized for their purpose, faster and more securely. Their AI systems will constantly improve themselves — using companies’ own data, in real-time.
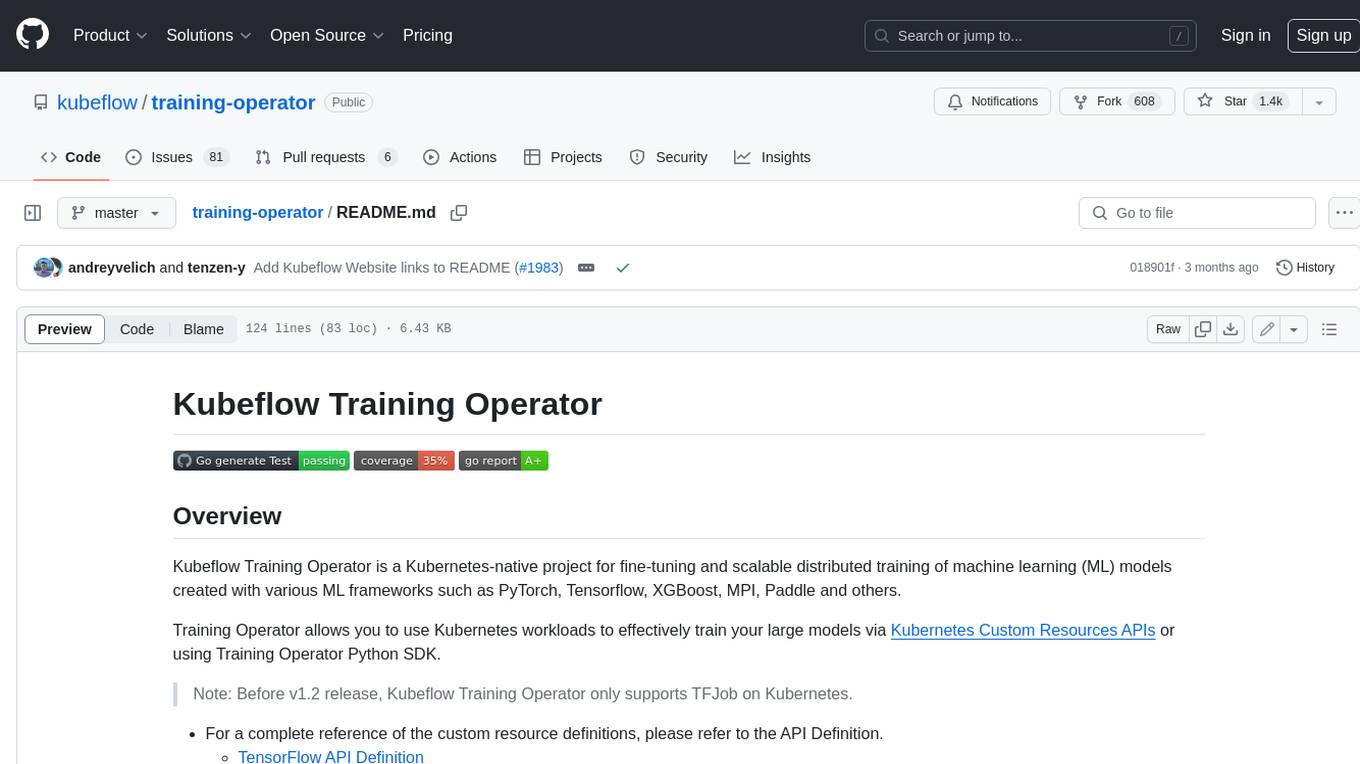
training-operator
Kubeflow Training Operator is a Kubernetes-native project for fine-tuning and scalable distributed training of machine learning (ML) models created with various ML frameworks such as PyTorch, Tensorflow, XGBoost, MPI, Paddle and others. Training Operator allows you to use Kubernetes workloads to effectively train your large models via Kubernetes Custom Resources APIs or using Training Operator Python SDK. > Note: Before v1.2 release, Kubeflow Training Operator only supports TFJob on Kubernetes. * For a complete reference of the custom resource definitions, please refer to the API Definition. * TensorFlow API Definition * PyTorch API Definition * Apache MXNet API Definition * XGBoost API Definition * MPI API Definition * PaddlePaddle API Definition * For details of all-in-one operator design, please refer to the All-in-one Kubeflow Training Operator * For details on its observability, please refer to the monitoring design doc.
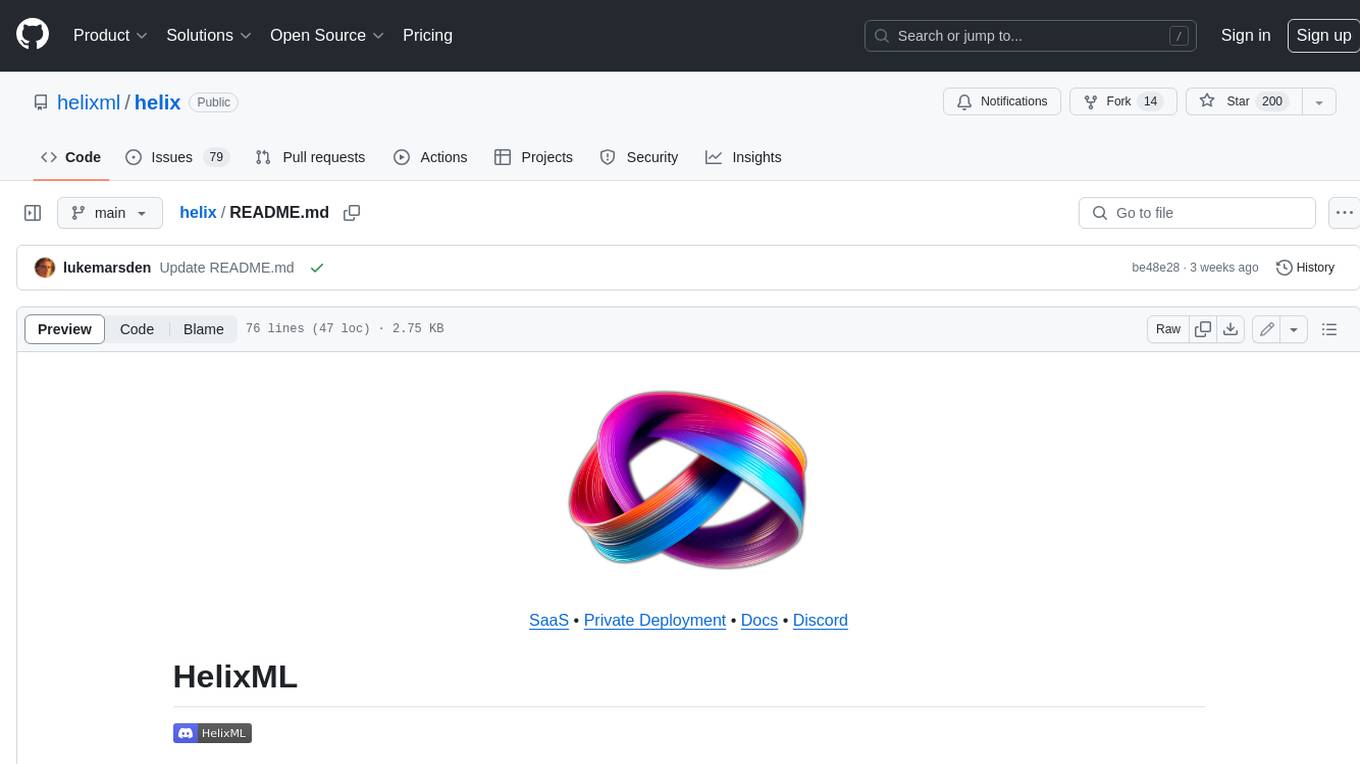
helix
HelixML is a private GenAI platform that allows users to deploy the best of open AI in their own data center or VPC while retaining complete data security and control. It includes support for fine-tuning models with drag-and-drop functionality. HelixML brings the best of open source AI to businesses in an ergonomic and scalable way, optimizing the tradeoff between GPU memory and latency.
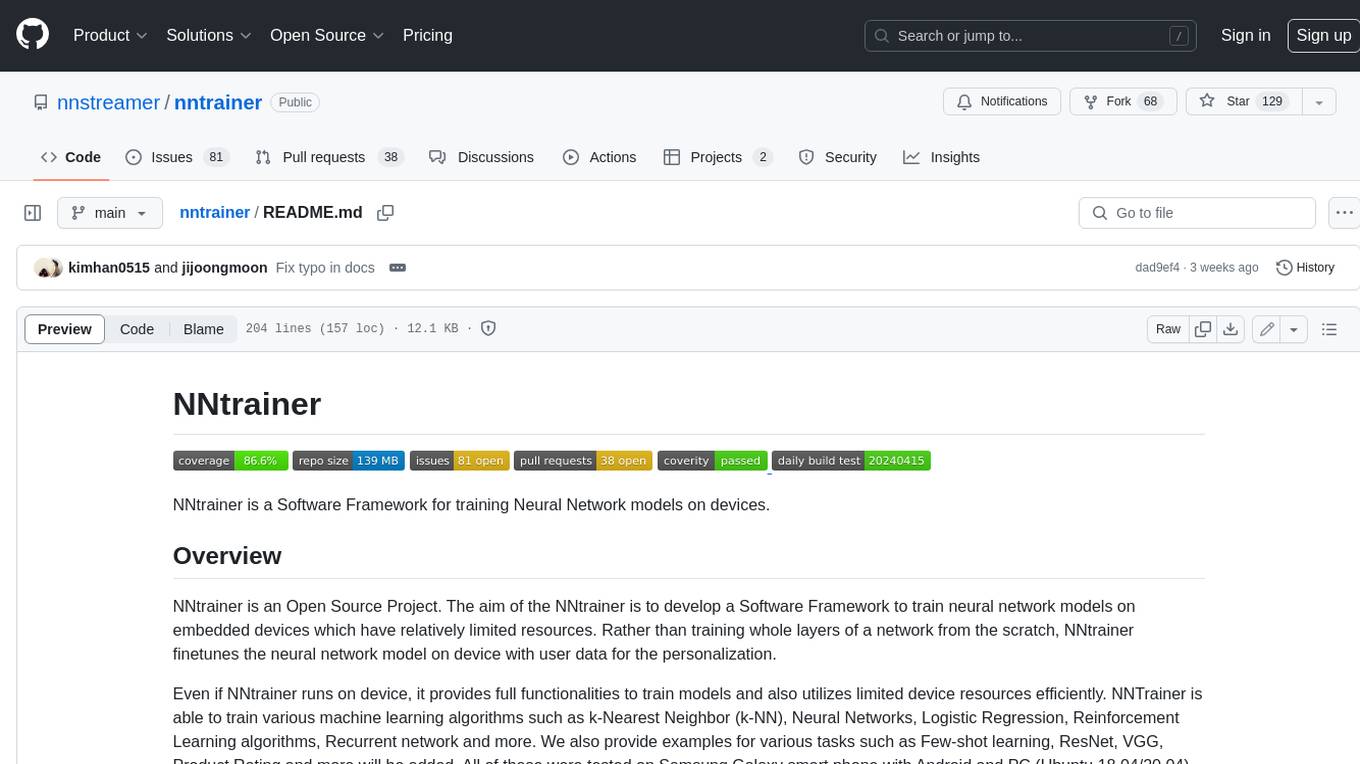
nntrainer
NNtrainer is a software framework for training neural network models on devices with limited resources. It enables on-device fine-tuning of neural networks using user data for personalization. NNtrainer supports various machine learning algorithms and provides examples for tasks such as few-shot learning, ResNet, VGG, and product rating. It is optimized for embedded devices and utilizes CBLAS and CUBLAS for accelerated calculations. NNtrainer is open source and released under the Apache License version 2.0.
For similar jobs
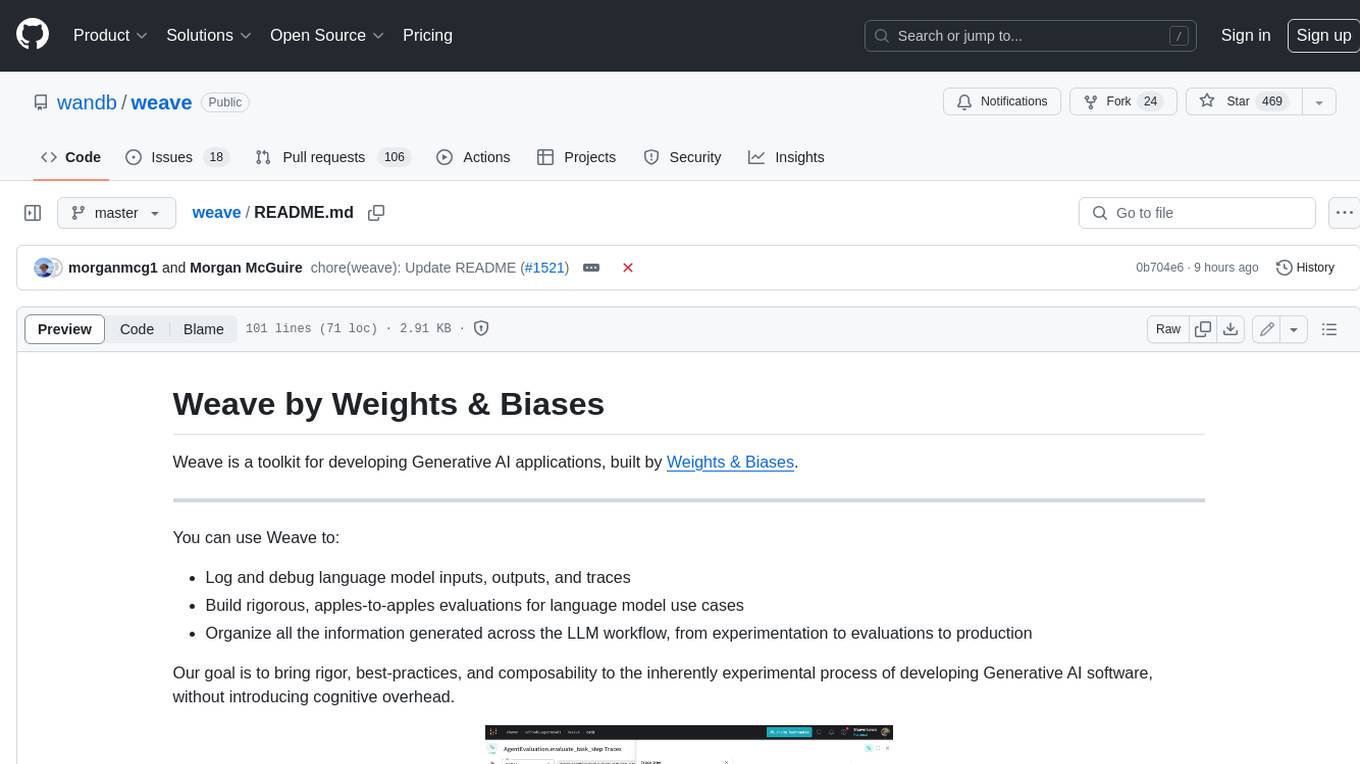
weave
Weave is a toolkit for developing Generative AI applications, built by Weights & Biases. With Weave, you can log and debug language model inputs, outputs, and traces; build rigorous, apples-to-apples evaluations for language model use cases; and organize all the information generated across the LLM workflow, from experimentation to evaluations to production. Weave aims to bring rigor, best-practices, and composability to the inherently experimental process of developing Generative AI software, without introducing cognitive overhead.
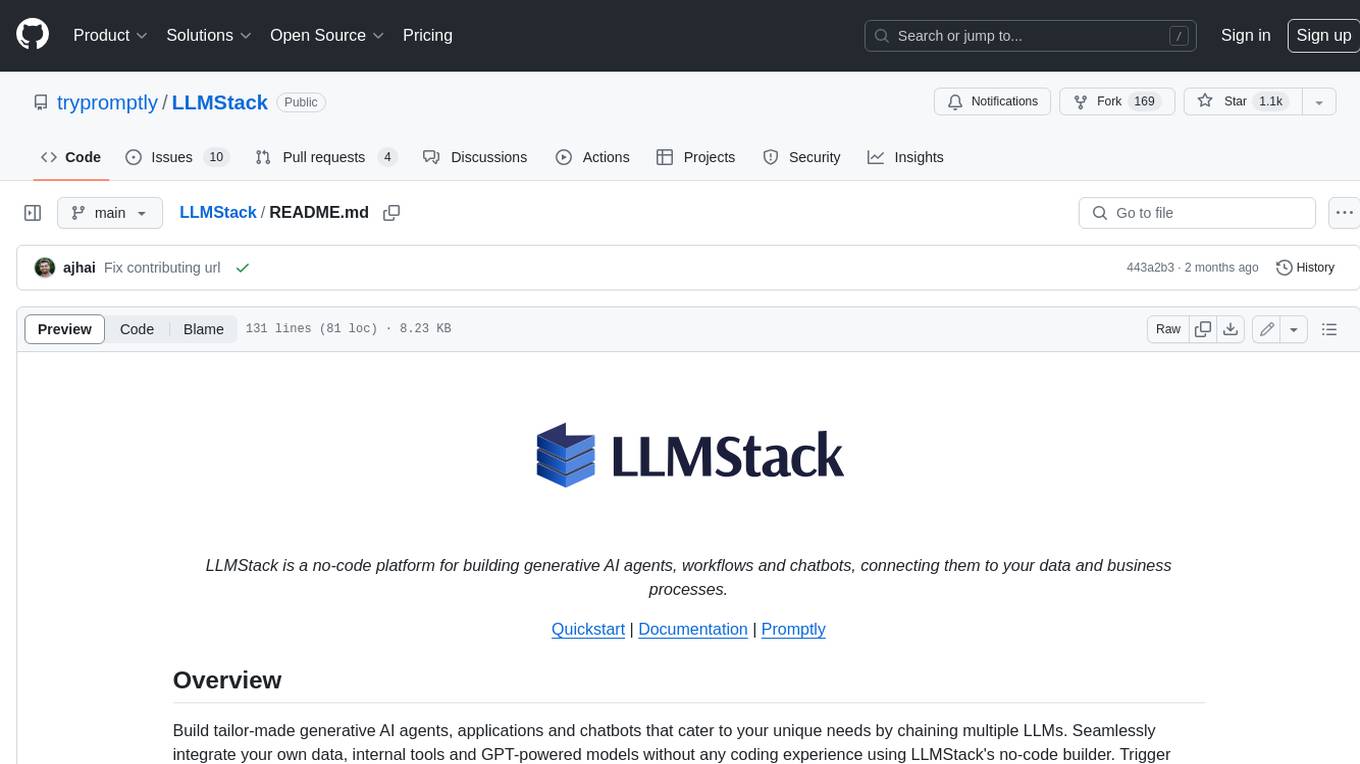
LLMStack
LLMStack is a no-code platform for building generative AI agents, workflows, and chatbots. It allows users to connect their own data, internal tools, and GPT-powered models without any coding experience. LLMStack can be deployed to the cloud or on-premise and can be accessed via HTTP API or triggered from Slack or Discord.
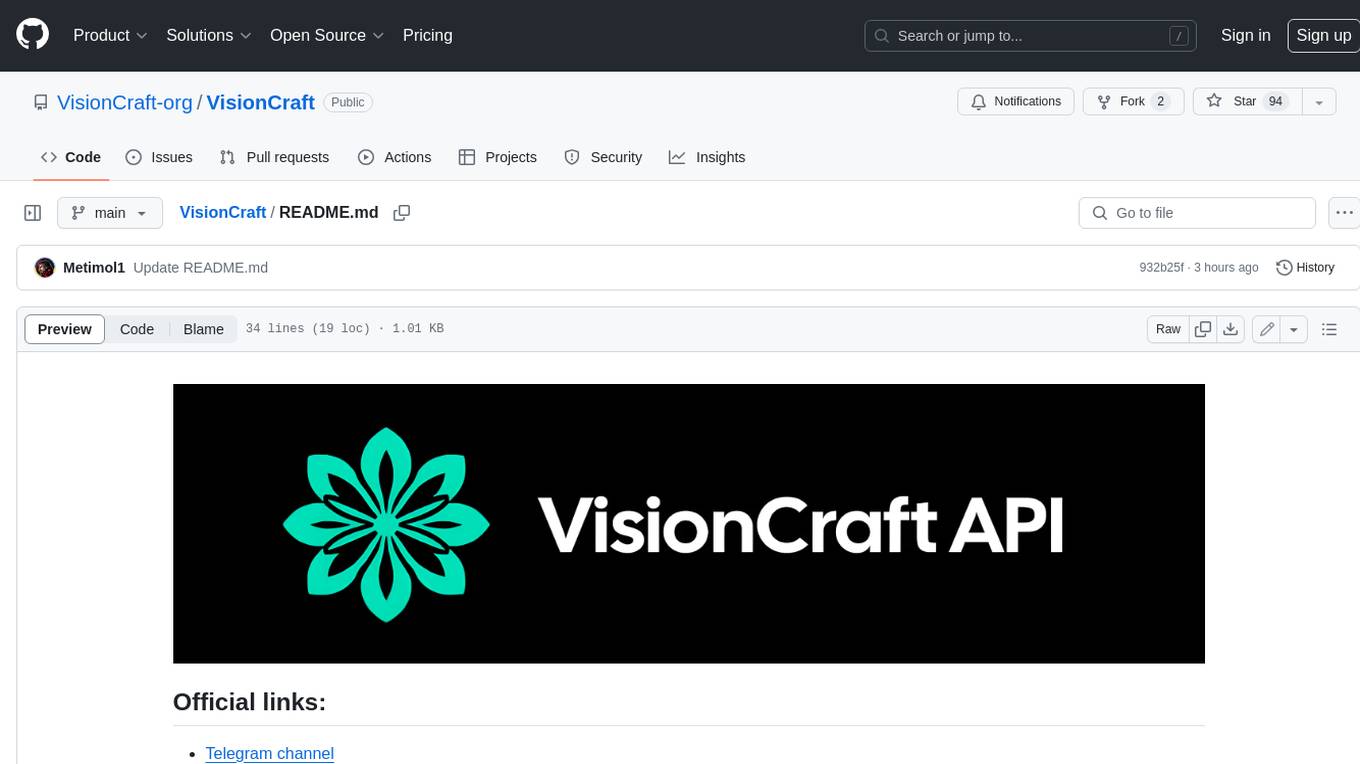
VisionCraft
The VisionCraft API is a free API for using over 100 different AI models. From images to sound.
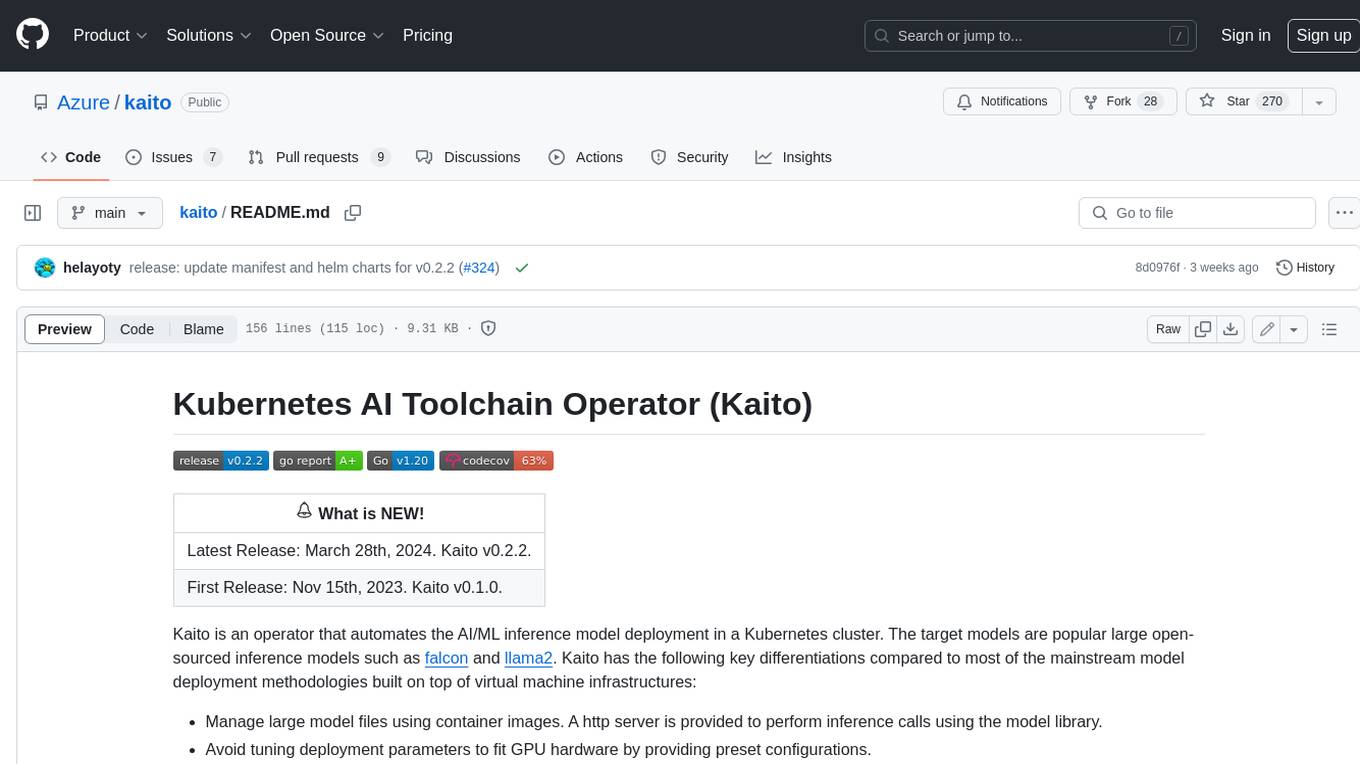
kaito
Kaito is an operator that automates the AI/ML inference model deployment in a Kubernetes cluster. It manages large model files using container images, avoids tuning deployment parameters to fit GPU hardware by providing preset configurations, auto-provisions GPU nodes based on model requirements, and hosts large model images in the public Microsoft Container Registry (MCR) if the license allows. Using Kaito, the workflow of onboarding large AI inference models in Kubernetes is largely simplified.
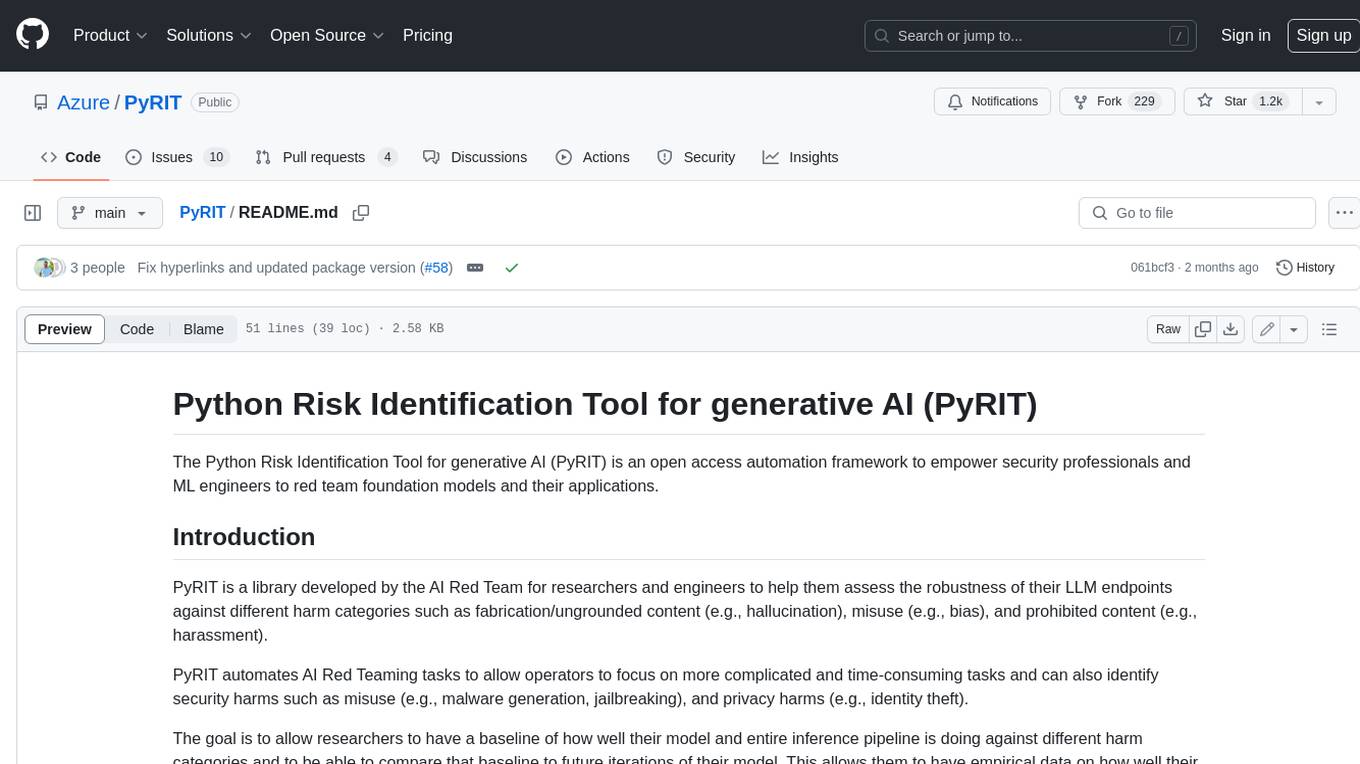
PyRIT
PyRIT is an open access automation framework designed to empower security professionals and ML engineers to red team foundation models and their applications. It automates AI Red Teaming tasks to allow operators to focus on more complicated and time-consuming tasks and can also identify security harms such as misuse (e.g., malware generation, jailbreaking), and privacy harms (e.g., identity theft). The goal is to allow researchers to have a baseline of how well their model and entire inference pipeline is doing against different harm categories and to be able to compare that baseline to future iterations of their model. This allows them to have empirical data on how well their model is doing today, and detect any degradation of performance based on future improvements.
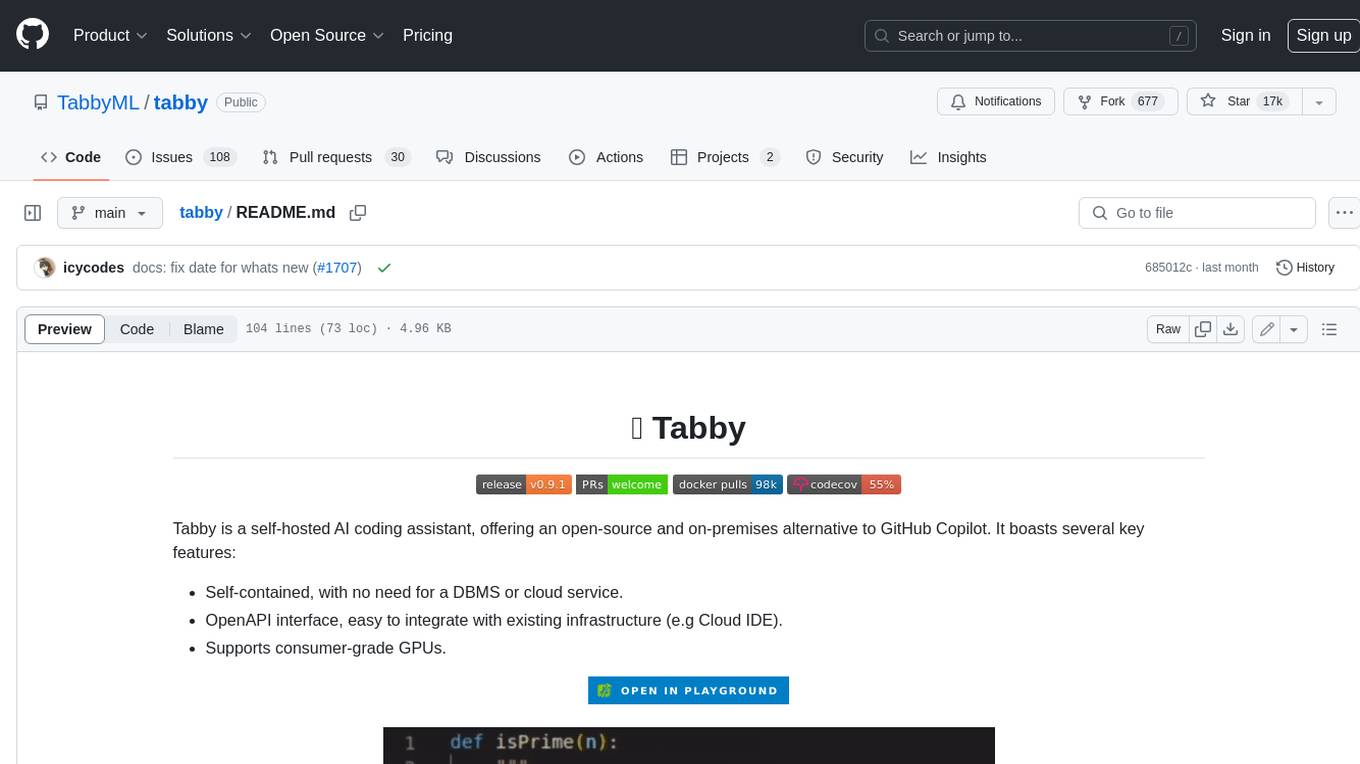
tabby
Tabby is a self-hosted AI coding assistant, offering an open-source and on-premises alternative to GitHub Copilot. It boasts several key features: * Self-contained, with no need for a DBMS or cloud service. * OpenAPI interface, easy to integrate with existing infrastructure (e.g Cloud IDE). * Supports consumer-grade GPUs.
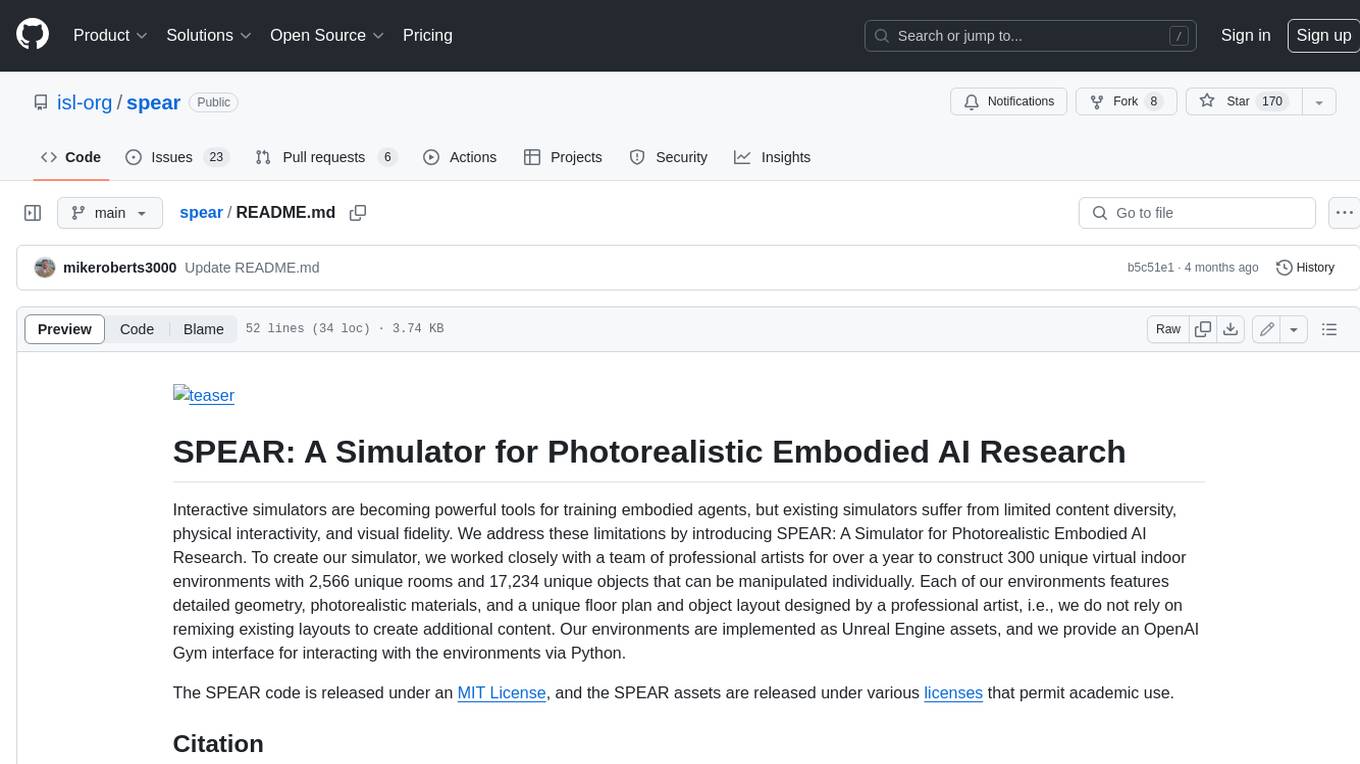
spear
SPEAR (Simulator for Photorealistic Embodied AI Research) is a powerful tool for training embodied agents. It features 300 unique virtual indoor environments with 2,566 unique rooms and 17,234 unique objects that can be manipulated individually. Each environment is designed by a professional artist and features detailed geometry, photorealistic materials, and a unique floor plan and object layout. SPEAR is implemented as Unreal Engine assets and provides an OpenAI Gym interface for interacting with the environments via Python.
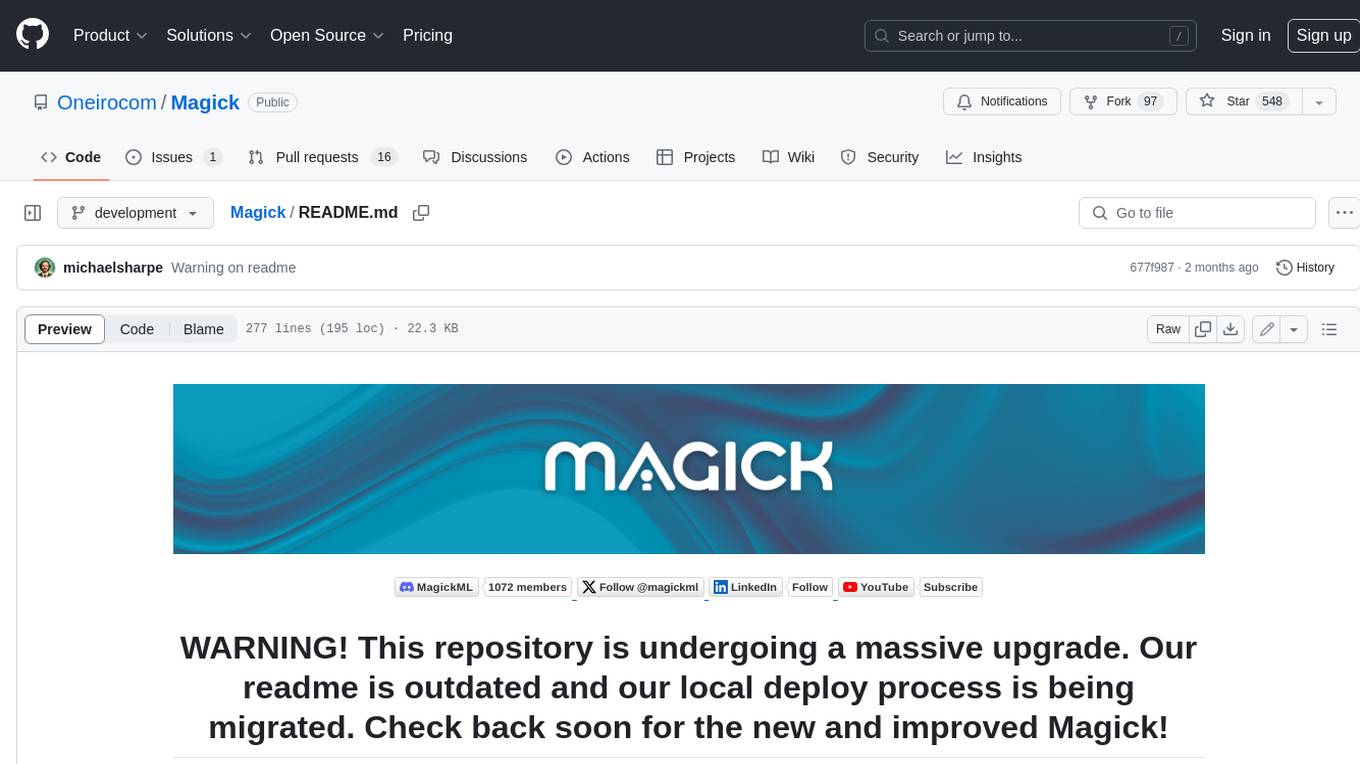
Magick
Magick is a groundbreaking visual AIDE (Artificial Intelligence Development Environment) for no-code data pipelines and multimodal agents. Magick can connect to other services and comes with nodes and templates well-suited for intelligent agents, chatbots, complex reasoning systems and realistic characters.