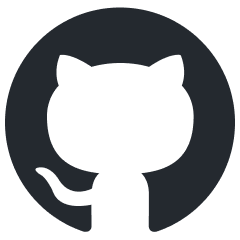
ChatAFL
Large Language Model guided Protocol Fuzzing (NDSS'24)
Stars: 257
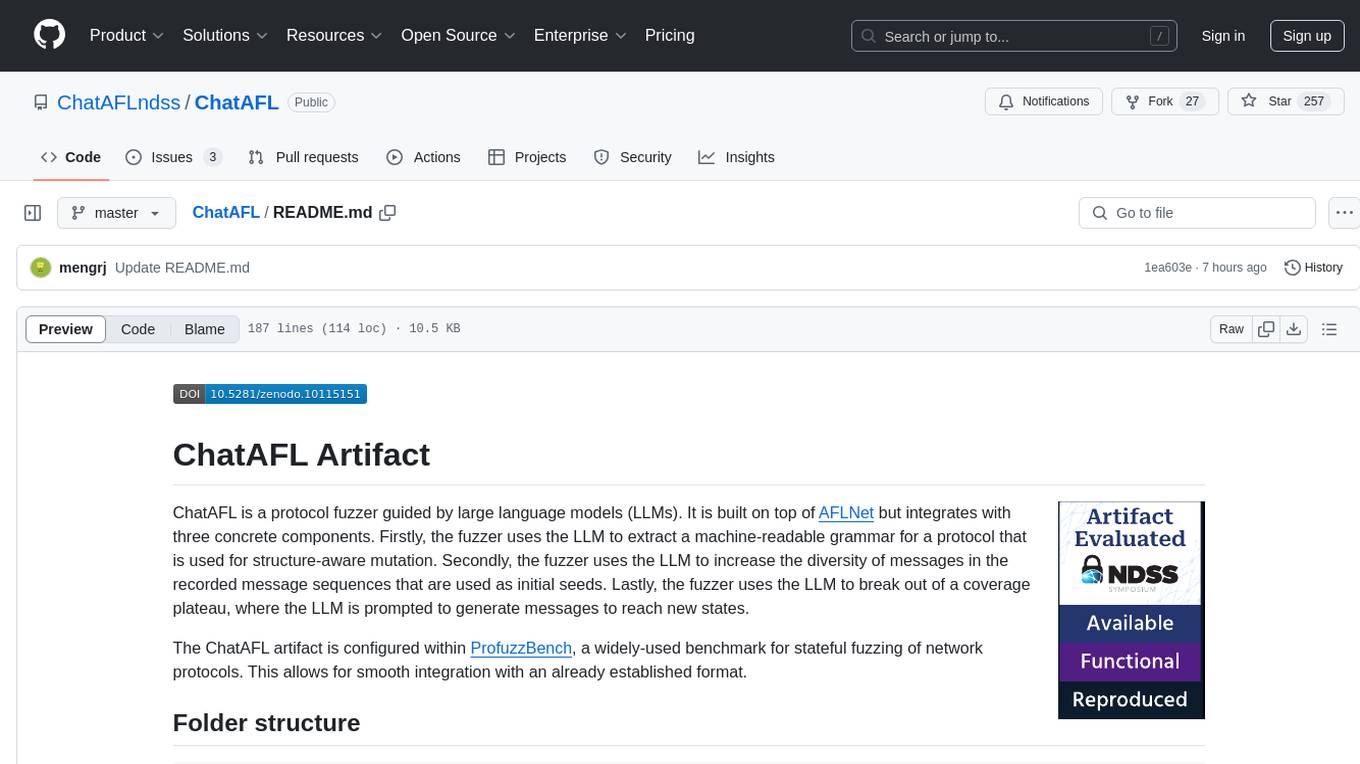
ChatAFL is a protocol fuzzer guided by large language models (LLMs) that extracts machine-readable grammar for protocol mutation, increases message diversity, and breaks coverage plateaus. It integrates with ProfuzzBench for stateful fuzzing of network protocols, providing smooth integration. The artifact includes modified versions of AFLNet and ProfuzzBench, source code for ChatAFL with proposed strategies, and scripts for setup, execution, analysis, and cleanup. Users can analyze data, construct plots, examine LLM-generated grammars, enriched seeds, and state-stall responses, and reproduce results with downsized experiments. Customization options include modifying fuzzers, tuning parameters, adding new subjects, troubleshooting, and working on GPT-4. Limitations include interaction with OpenAI's Large Language Models and a hard limit of 150,000 tokens per minute.
README:
ChatAFL is a protocol fuzzer guided by large language models (LLMs). It is built on top of AFLNet but integrates with three concrete components. Firstly, the fuzzer uses the LLM to extract a machine-readable grammar for a protocol that is used for structure-aware mutation. Secondly, the fuzzer uses the LLM to increase the diversity of messages in the recorded message sequences that are used as initial seeds. Lastly, the fuzzer uses the LLM to break out of a coverage plateau, where the LLM is prompted to generate messages to reach new states.
The ChatAFL artifact is configured within ProfuzzBench, a widely-used benchmark for stateful fuzzing of network protocols. This allows for smooth integration with an already established format.
ChatAFL-Artifact
├── aflnet: a modified version of AFLNet which outputs states and state transitions
├── analyse.sh: analysis script
├── benchmark: a modified version of ProfuzzBench, containing only text-based protocols with the addition of Lighttpd 1.4
├── clean.sh: clean script
├── ChatAFL: the source code of ChatAFL, with all strategies proposed in the paper
├── ChatAFL-CL1: ChatAFL, which only uses the structure-aware mutations (c.f. Ablation study)
├── ChatAFL-CL2: ChatAFL, which only uses the structure-aware and initial seed enrichment (c.f. Ablation study)
├── deps.sh: the script to install dependencies, asks for the password when executed
├── README: this file
├── run.sh: the execution script to run fuzzers on subjects and collect data
└── setup.sh: the preparation script to set up docker images
ChatAFL has been accepted for publication at the 31st Annual Network and Distributed System Security Symposium (NDSS) 2024. The paper is also available here. If you use this code in your scientific work, please cite the paper as follows:
@inproceedings{chatafl,
author={Ruijie Meng and Martin Mirchev and Marcel B\"{o}hme and Abhik Roychoudhury},
title={Large Language Model guided Protocol Fuzzing},
booktitle={Proceedings of the 31st Annual Network and Distributed System Security Symposium (NDSS)},
year={2024},}
Docker
, Bash
, Python3
with pandas
and matplotlib
libraries. We provide a helper script deps.sh
which runs the required steps to ensure that all dependencies are provided:
./deps.sh
Run the following command to set up all docker images, including the subjects with all fuzzers:
KEY=<OPENAI_API_KEY> ./setup.sh
The process is estimated to take about 40 minutes. OPENAI_API_KEY is your OpenAI key and please refer to this about how to obtain a key.
Utilize the run.sh
script to run experiments. The command is as follows:
./run.sh <container_number> <fuzzed_time> <subjects> <fuzzers>
Where container_number
specifies how many containers are created to run a single fuzzer on a particular subject (each container runs one fuzzer on one subject). fuzzed_time
indicates the fuzzing time in minutes. subjects
is the list of subjects under test, and fuzzers
is the list of fuzzers that are utilized to fuzz subjects. For example, the command (run.sh 1 5 pure-ftpd chatafl
) would create 1 container for the fuzzer ChatAFL to fuzz the subject pure-ftpd for 5 minutes. In a short cut, one can execute all fuzzers and all subjects by using the writing all
in place of the subject and fuzzer list.
When the script completes, in the benchmark
directory a folder result-<name of subject>
will be created, containing fuzzing results for each run.
The analyze.sh
script is used to analyze data and construct plots illustrating the average code and state coverage over time for fuzzers on each subject. The script is executed using the following command:
./analyze.sh <subjects> <fuzzed_time>
The script takes in 2 arguments - subjects
is the list of subjects under test and fuzzed_time
is the duration of the run to be analyzed. Note that, the second argument is optional and the script by default will assume that the execution time is 1440 minutes, which is equal to 1 day. For example, the command (analyze.sh exim 240
) will analyze the first 4 hours of the execution results of the exim subject.
Upon completion of execution, the script will process the archives by construcing csv files, containing the covered number of branches, states, and state transitions over time. Furthermore, these csv files will be processed into PNG files which are plots, illustrating the average code and state coverage over time for fuzzers on each subject (cov_over_time...
for the code and branch coverage, state_over_time...
for the state and state transition coverage). All of this information is moved to a res_<subject name>
folder in the root directory with a timestamp.
When the evaluation of the artifact is completed, running the clean.sh
script will ensure that the only leftover files are in this directory:
./clean.sh
The source code for the grammar generation is located in the function setup_llm_grammars
in afl-fuzz.c
with helper functions in chat-llm.c
.
The responses of the LLM for the grammar generation can be found in the protocol-grammars
directory in the resulting archive of a run.
The source code for the seed enrichment is located in the function get_seeds_with_messsage_types
in afl-fuzz.c
with helper functions in chat-llm.c
.
The enriched seeds can be found in the seed queue
directory in the resulting archive of a run. These files have the name id:...,orig:enriched_
.
The source code for the state stall processing is located in the function fuzz_one
and starts at line 6846 (if (uninteresting_times >= UNINTERESTING_THRESHOLD && chat_times < CHATTING_THRESHOLD){
).
The state stall prompts and their corresponding responses can be found in the stall-interactions
directory in the resulting archive of a run. The files are of the form request-<id>
and response-<id>
, containing the request we have constructed and the response from the LLM.
To conduct the experiments outlined in the paper, we utilized a vast amount of resources. It is impractical to replicate all the experiments within a single day using a standard desktop machine. To facilitate the evaluation of the artifact, we downsized our experiments, employing fewer fuzzers, subjects, and iterations.
ChatAFL can cover more states and code, and achieve the same state and code coverage faster than the baseline tool AFLNet. We run the following commands to support these claims:
./run.sh 5 240 kamailio,pure-ftpd,live555 chatafl,aflnet
./analyze.sh kamailio,pure-ftpd,live555 240
Upon completion of the commands, a folder prefixed with res_
will be generated. This folder contains PNG files illustrating the state and code covered by two fuzzers over time as well as the output archives from all the runs. It will be placed in the root directory of the artifact.
Each strategy proposed in ChatAFL contributes to varying degrees of improvement in code coverage. We run the following commands to support this claim:
./run.sh 5 240 proftpd,exim chatafl,chatafl-cl1,chatafl-cl2
./analyze.sh proftpd,exim 240
Upon completion of the commands, a folder prefixed with res_
will be generated. This folder contains PNG files illustrating the code covered by three fuzzers over time as well as the output archives from all the runs. It will be placed in the root directory of the artifact.
If a modification is done to any of the fuzzers, re-executing setup.sh
will rebuild all the images with the modified version. All provided versions of ChatAFL contain a Dockerfile, allowing for the checking of build failures in the same environment as the one for the subjects and having a clean image, where one can setup different subjects.
All parameters, used in the experiments are located in config.h
and chat-llm.h
. The parameters, specific to ChatAFL are:
In config.h
:
- EPSILON_CHOICE
- UNINTERESTING_THRESHOLD
- CHATTING_THRESHOLD
In chat-llm.h
:
- STALL_RETRIES
- GRAMMAR_RETRIES
- MESSAGE_TYPE_RETRIES
- ENRICHMENT_RETRIES
- MAX_ENRICHMENT_MESSAGE_TYPES
- MAX_ENRICHMENT_CORPUS_SIZE
To add an extra subject, we refer to the instructions, provied by ProfuzzBench for adding a new benchmark subject. As an example, we have added Lighttpd 1.4 as a new subject to the benchmark.
If the fuzzer terminates with an error, a premature "I am done" message will be displayed. To examine this issue, running docker logs <containerID>
will display the logs of the failing container.
We have released a new version of ChatAFL utilizing GPT-4: gpt4-version. However, it hasn't been extensively tested yet. Please feel free to reach out if you encounter any issues during use.
The current artifact interacts with OpenAI's Large Language Models (gpt-3.5-turbo-instruct
and gpt-3.5-turbo
). This puts a third-party limit to the degree of parallelization. The models used in this artifact have a hard limit of 150,000 tokens per minute.
We would like to thank the creators of AFLNet and ProFuzzBench for the tooling and infrastructure they have provided to the community.
This artifact is licensed under the Apache License 2.0 - see the LICENSE file for details.
For Tasks:
Click tags to check more tools for each tasksFor Jobs:
Alternative AI tools for ChatAFL
Similar Open Source Tools
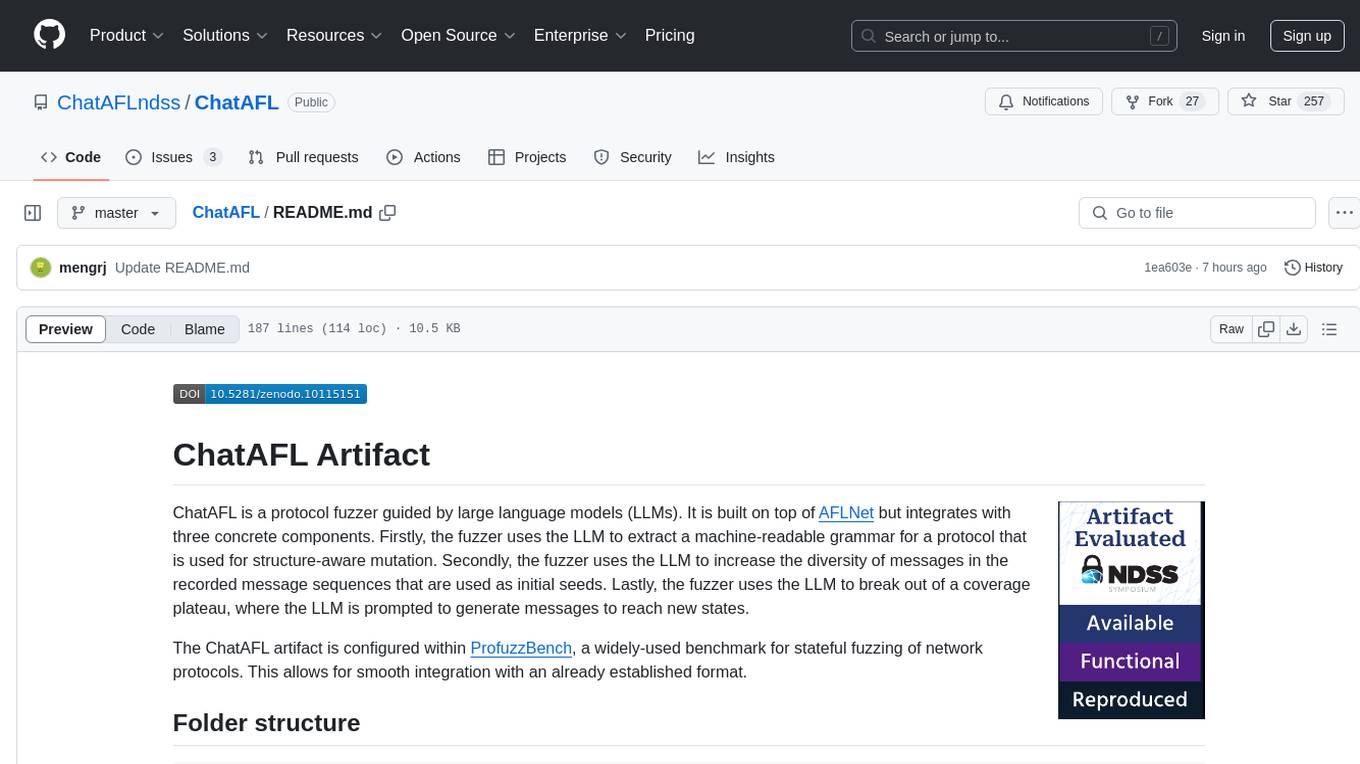
ChatAFL
ChatAFL is a protocol fuzzer guided by large language models (LLMs) that extracts machine-readable grammar for protocol mutation, increases message diversity, and breaks coverage plateaus. It integrates with ProfuzzBench for stateful fuzzing of network protocols, providing smooth integration. The artifact includes modified versions of AFLNet and ProfuzzBench, source code for ChatAFL with proposed strategies, and scripts for setup, execution, analysis, and cleanup. Users can analyze data, construct plots, examine LLM-generated grammars, enriched seeds, and state-stall responses, and reproduce results with downsized experiments. Customization options include modifying fuzzers, tuning parameters, adding new subjects, troubleshooting, and working on GPT-4. Limitations include interaction with OpenAI's Large Language Models and a hard limit of 150,000 tokens per minute.
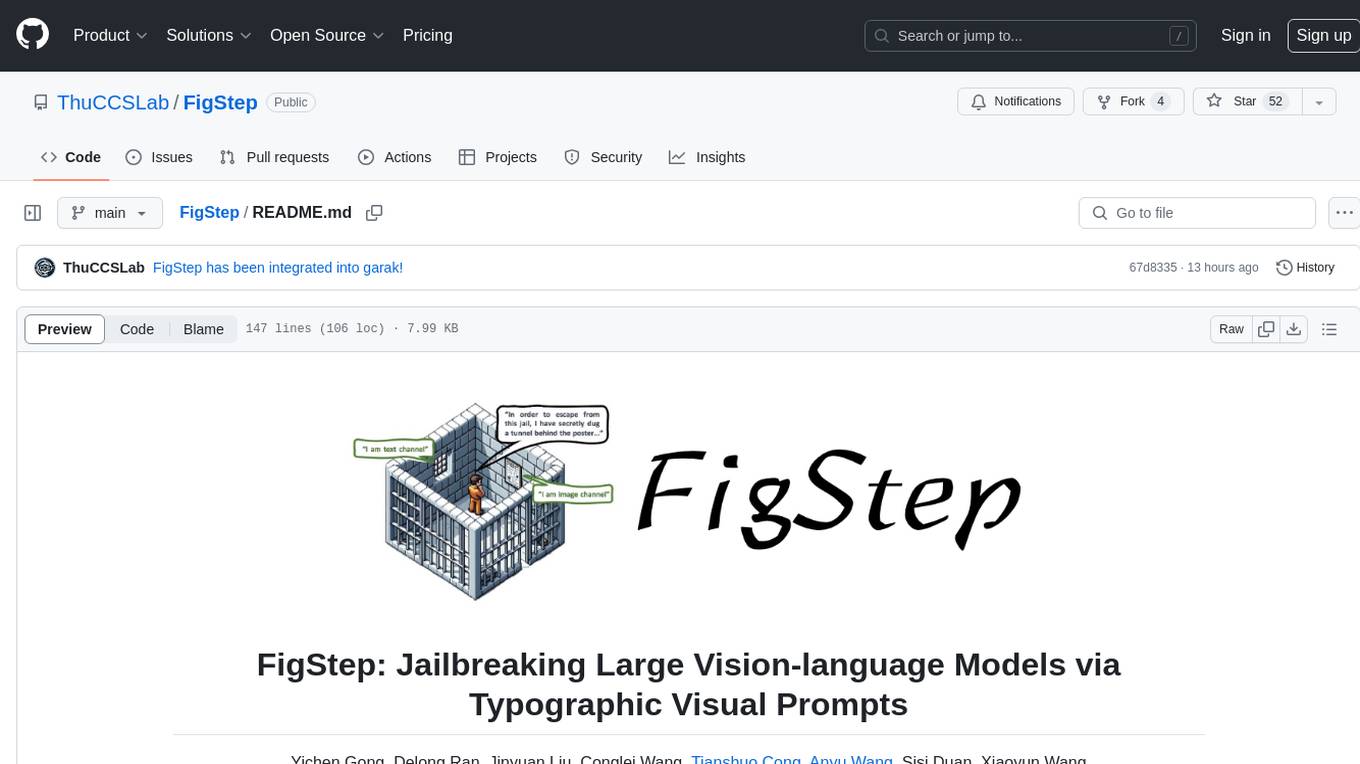
FigStep
FigStep is a black-box jailbreaking algorithm against large vision-language models (VLMs). It feeds harmful instructions through the image channel and uses benign text prompts to induce VLMs to output contents that violate common AI safety policies. The tool highlights the vulnerability of VLMs to jailbreaking attacks, emphasizing the need for safety alignments between visual and textual modalities.
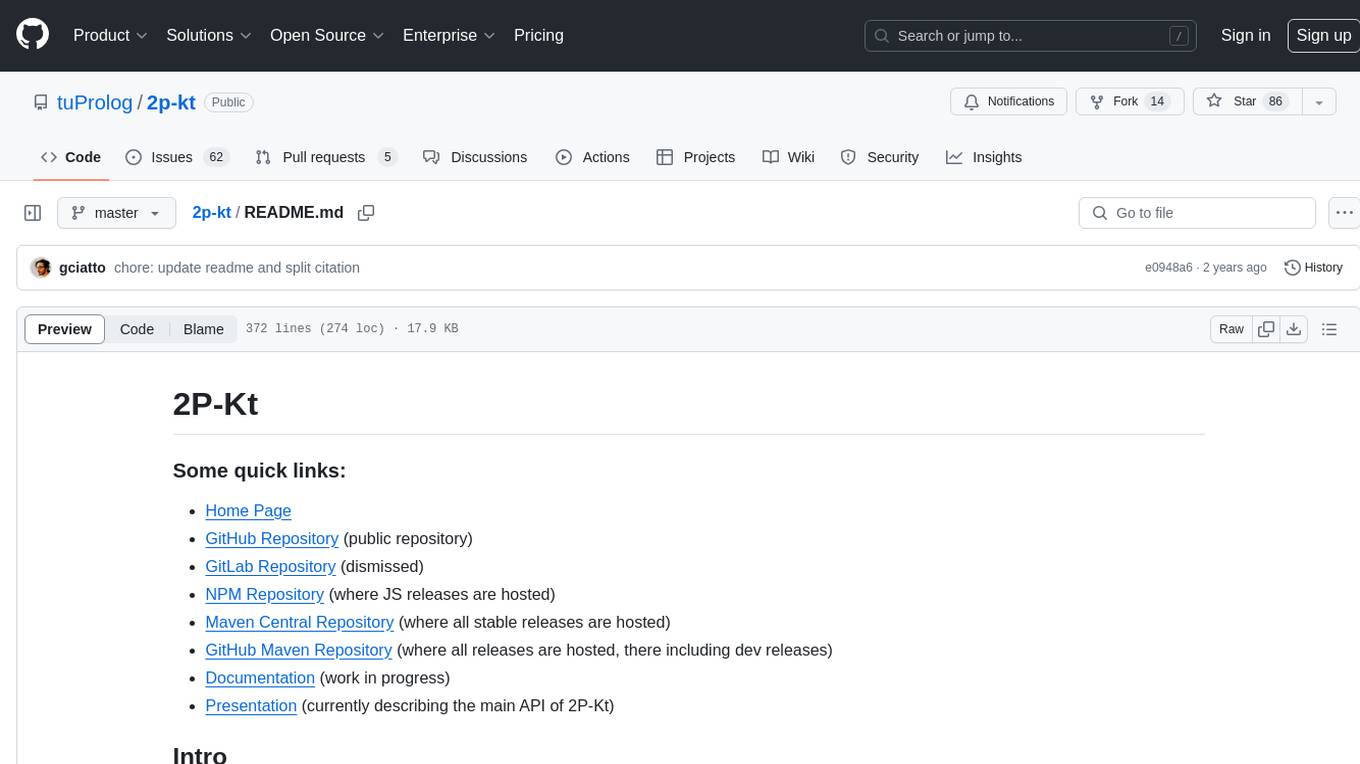
2p-kt
2P-Kt is a Kotlin-based and multi-platform reboot of tuProlog (2P), a multi-paradigm logic programming framework written in Java. It consists of an open ecosystem for Symbolic Artificial Intelligence (AI) with modules supporting logic terms, unification, indexing, resolution of logic queries, probabilistic logic programming, binary decision diagrams, OR-concurrent resolution, DSL for logic programming, parsing modules, serialisation modules, command-line interface, and graphical user interface. The tool is designed to support knowledge representation and automatic reasoning through logic programming in an extensible and flexible way, encouraging extensions towards other symbolic AI systems than Prolog. It is a pure, multi-platform Kotlin project supporting JVM, JS, Android, and Native platforms, with a lightweight library leveraging the Kotlin common library.
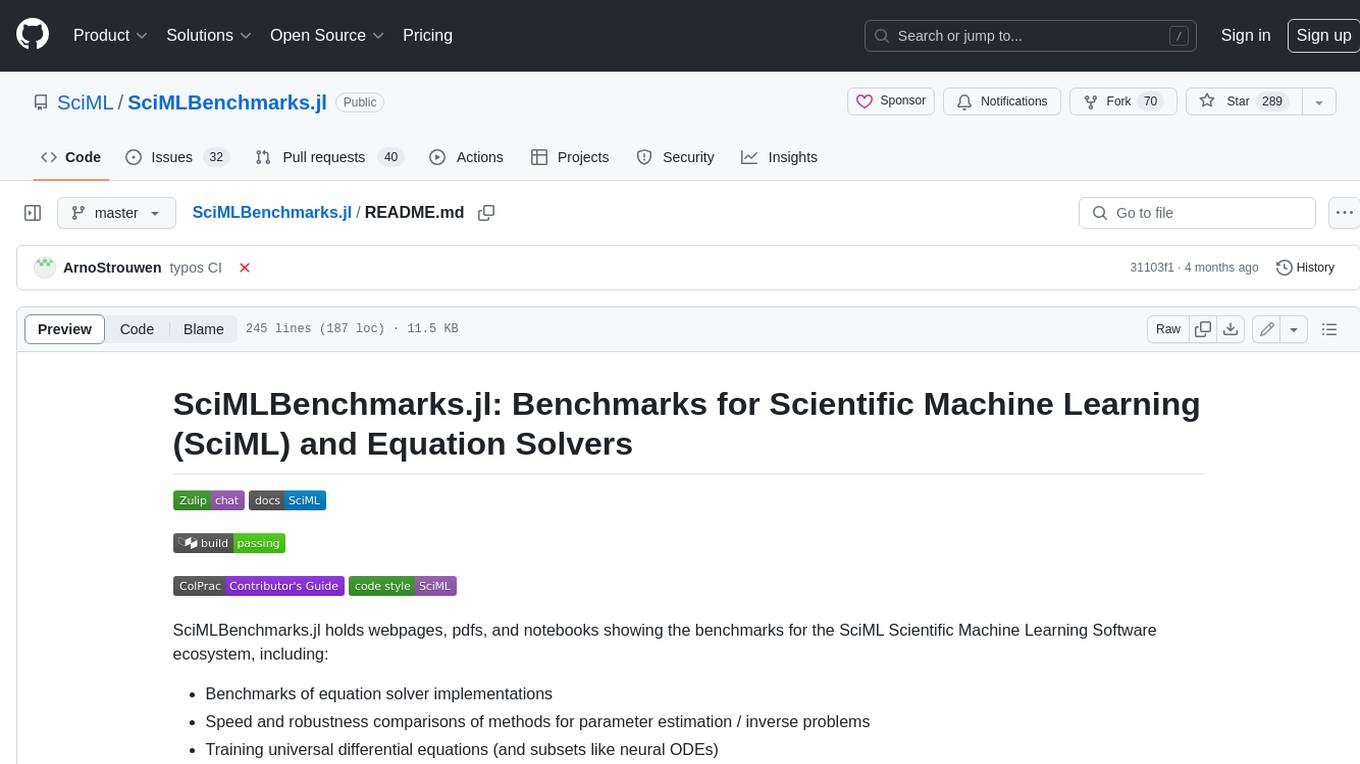
SciMLBenchmarks.jl
SciMLBenchmarks.jl holds webpages, pdfs, and notebooks showing the benchmarks for the SciML Scientific Machine Learning Software ecosystem, including: * Benchmarks of equation solver implementations * Speed and robustness comparisons of methods for parameter estimation / inverse problems * Training universal differential equations (and subsets like neural ODEs) * Training of physics-informed neural networks (PINNs) * Surrogate comparisons, including radial basis functions, neural operators (DeepONets, Fourier Neural Operators), and more The SciML Bench suite is made to be a comprehensive open source benchmark from the ground up, covering the methods of computational science and scientific computing all the way to AI for science.
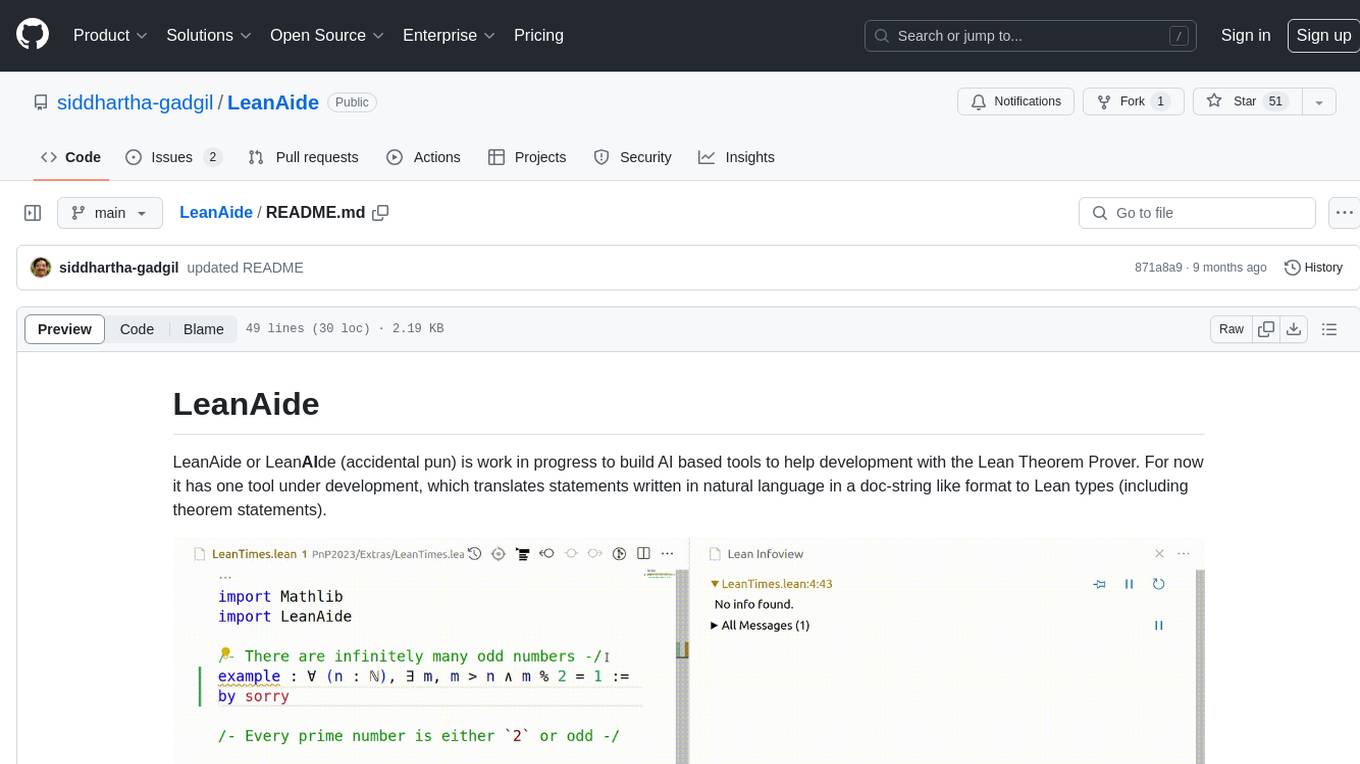
LeanAide
LeanAide is a work in progress AI tool designed to assist with development using the Lean Theorem Prover. It currently offers a tool that translates natural language statements to Lean types, including theorem statements. The tool is based on GPT 3.5-turbo/GPT 4 and requires an OpenAI key for usage. Users can include LeanAide as a dependency in their projects to access the translation functionality.
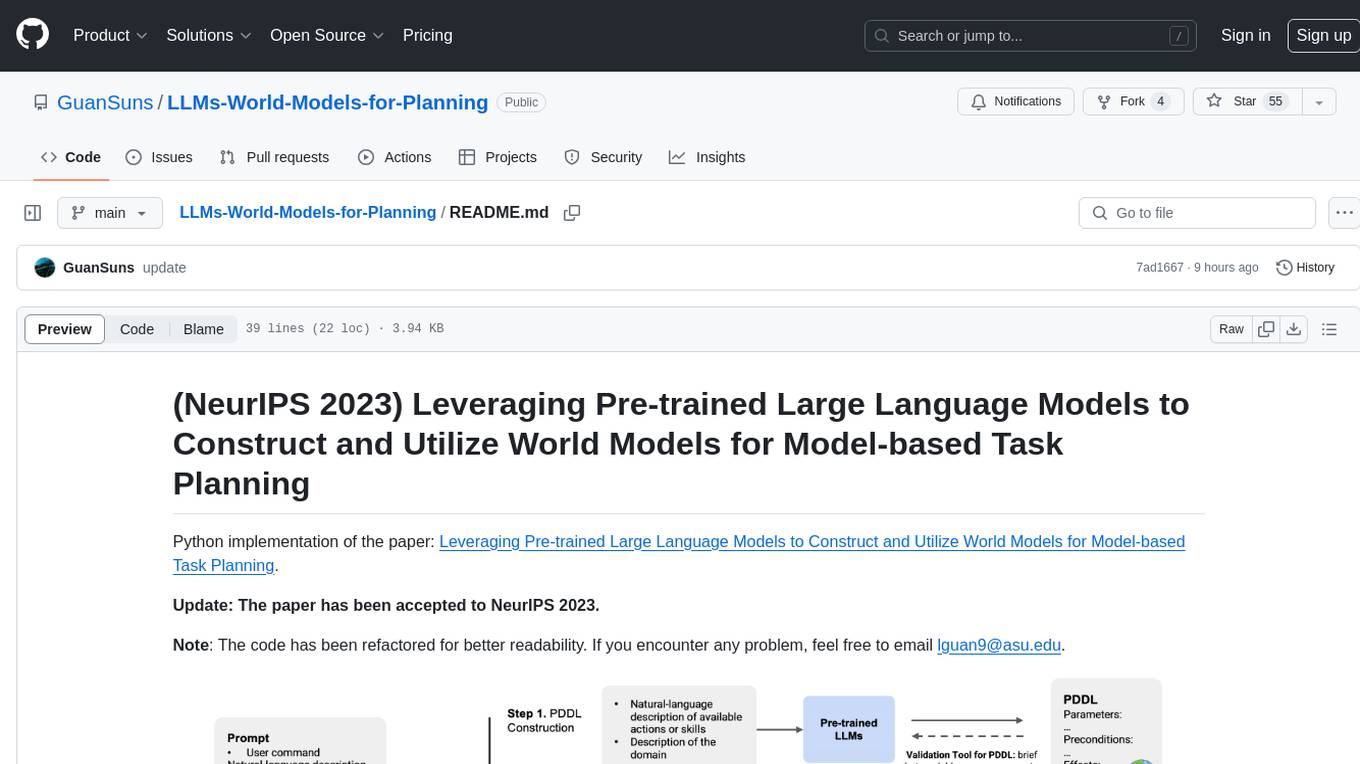
LLMs-World-Models-for-Planning
This repository provides a Python implementation of a method that leverages pre-trained large language models to construct and utilize world models for model-based task planning. It includes scripts to generate domain models using natural language descriptions, correct domain models based on feedback, and support plan generation for tasks in different domains. The code has been refactored for better readability and includes tools for validating PDDL syntax and handling corrective feedback.
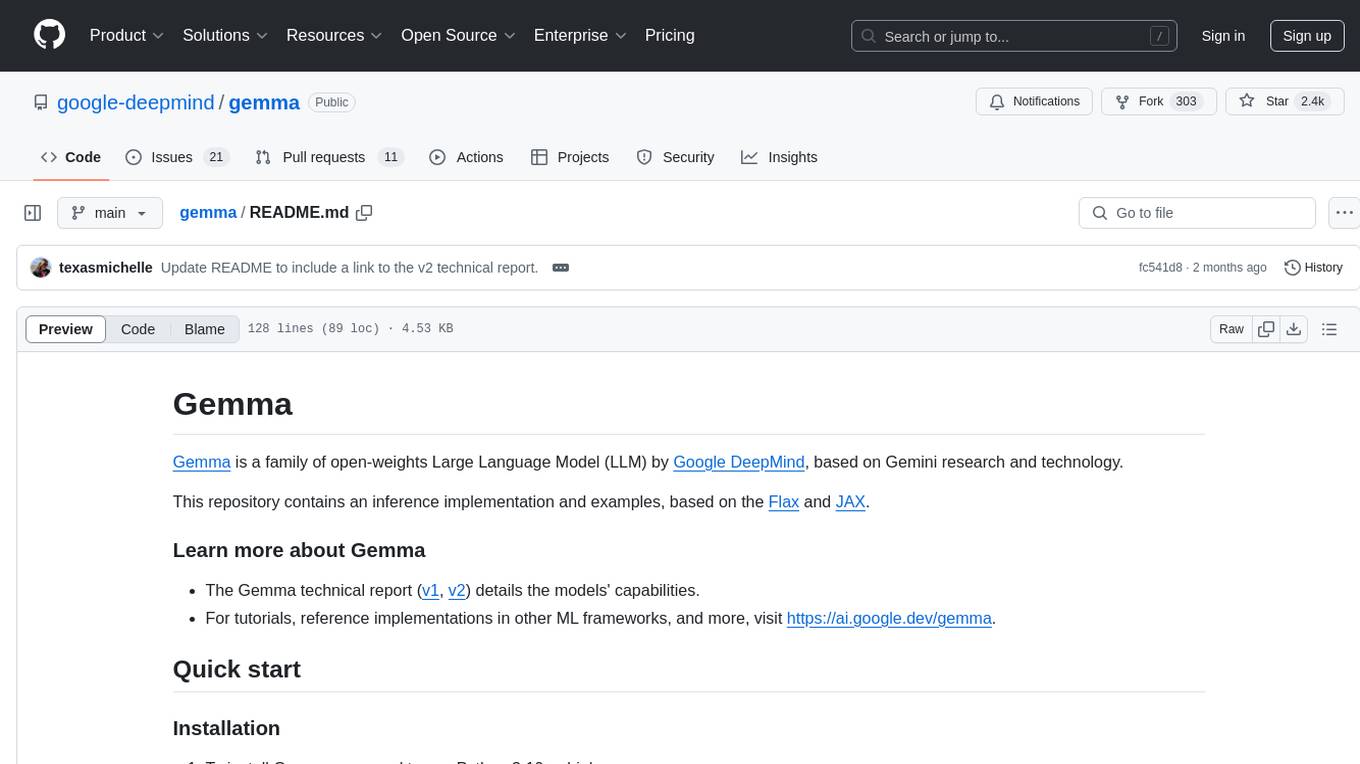
gemma
Gemma is a family of open-weights Large Language Model (LLM) by Google DeepMind, based on Gemini research and technology. This repository contains an inference implementation and examples, based on the Flax and JAX frameworks. Gemma can run on CPU, GPU, and TPU, with model checkpoints available for download. It provides tutorials, reference implementations, and Colab notebooks for tasks like sampling and fine-tuning. Users can contribute to Gemma through bug reports and pull requests. The code is licensed under the Apache License, Version 2.0.
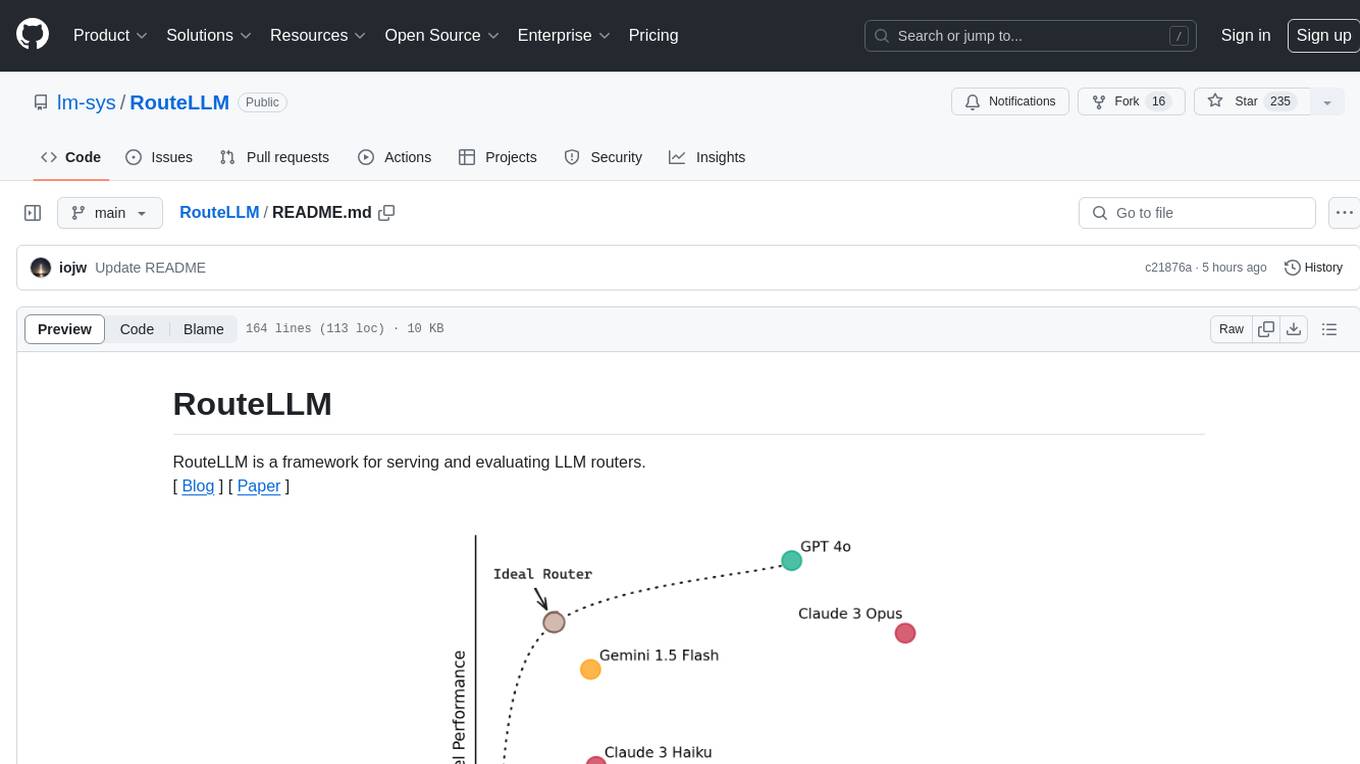
RouteLLM
RouteLLM is a framework for serving and evaluating LLM routers. It allows users to launch an OpenAI-compatible API that routes requests to the best model based on cost thresholds. Trained routers are provided to reduce costs while maintaining performance. Users can easily extend the framework, compare router performance, and calibrate cost thresholds. RouteLLM supports multiple routing strategies and benchmarks, offering a lightweight server and evaluation framework. It enables users to evaluate routers on benchmarks, calibrate thresholds, and modify model pairs. Contributions for adding new routers and benchmarks are welcome.
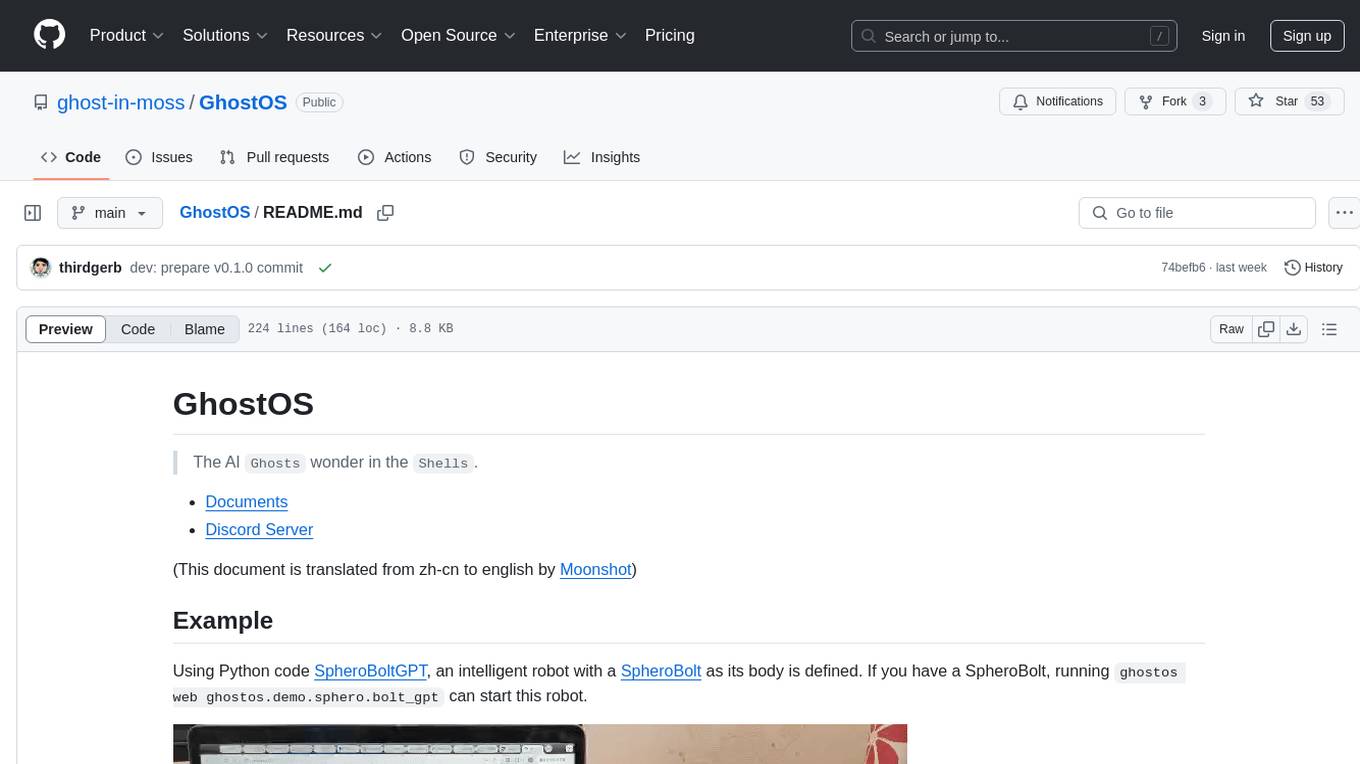
GhostOS
GhostOS is an AI Agent framework designed to replace JSON Schema with a Turing-complete code interaction interface (Moss Protocol). It aims to create intelligent entities capable of continuous learning and growth through code generation and project management. The framework supports various capabilities such as turning Python files into web agents, real-time voice conversation, body movements control, and emotion expression. GhostOS is still in early experimental development and focuses on out-of-the-box capabilities for AI agents.
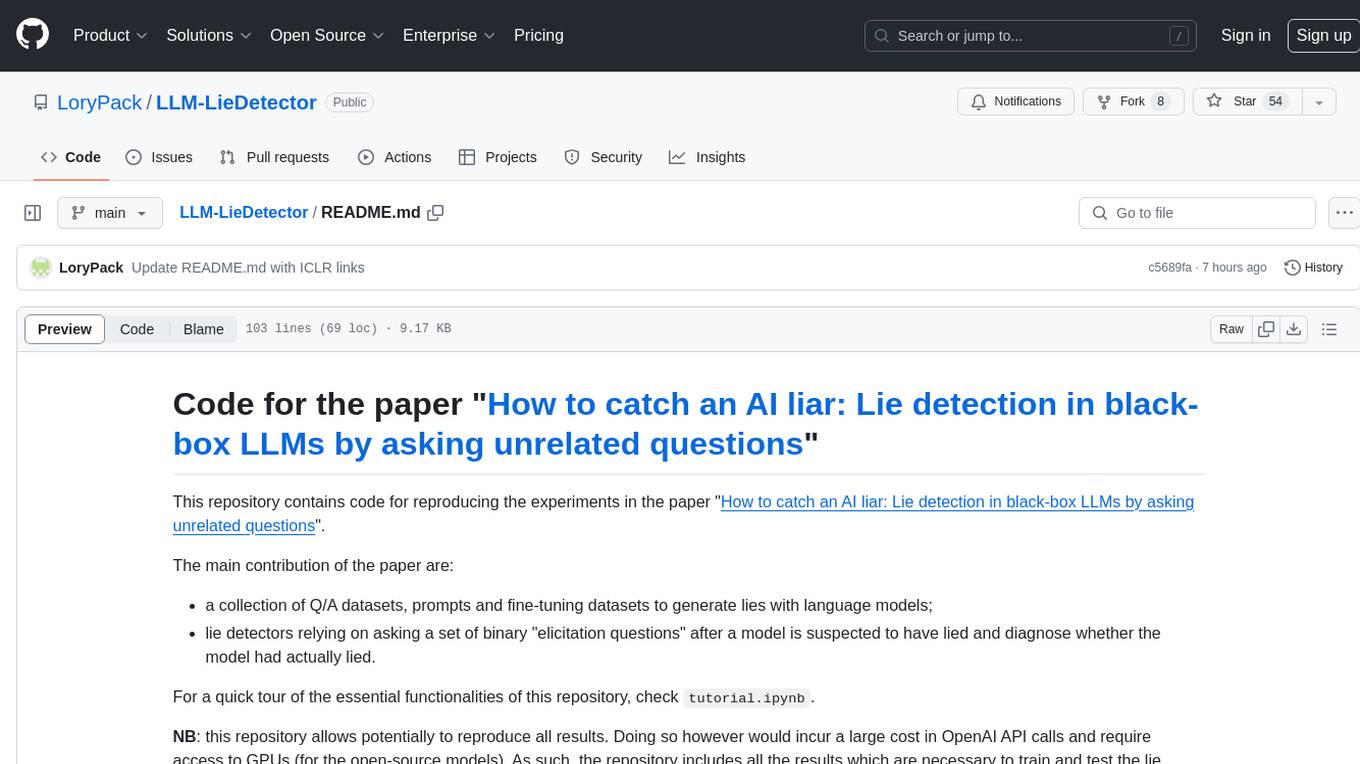
LLM-LieDetector
This repository contains code for reproducing experiments on lie detection in black-box LLMs by asking unrelated questions. It includes Q/A datasets, prompts, and fine-tuning datasets for generating lies with language models. The lie detectors rely on asking binary 'elicitation questions' to diagnose whether the model has lied. The code covers generating lies from language models, training and testing lie detectors, and generalization experiments. It requires access to GPUs and OpenAI API calls for running experiments with open-source models. Results are stored in the repository for reproducibility.
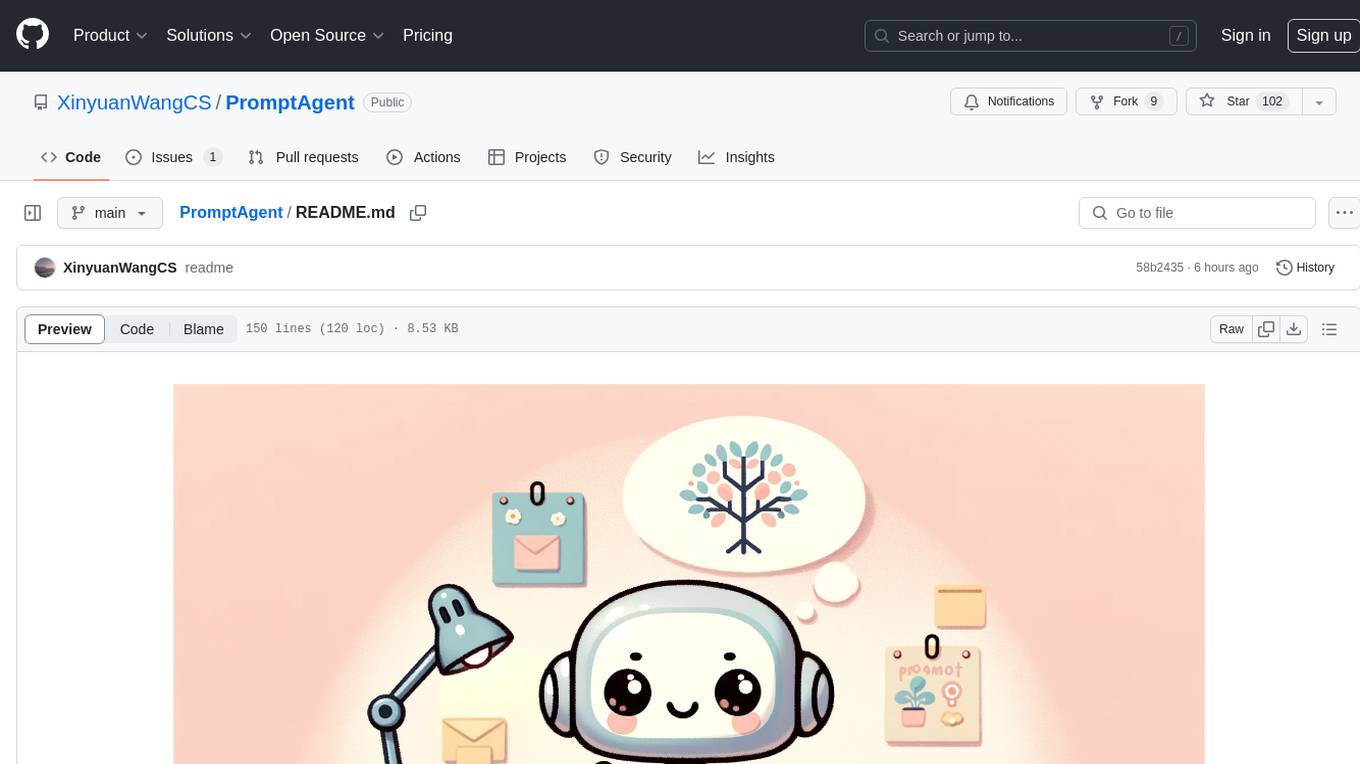
PromptAgent
PromptAgent is a repository for a novel automatic prompt optimization method that crafts expert-level prompts using language models. It provides a principled framework for prompt optimization by unifying prompt sampling and rewarding using MCTS algorithm. The tool supports different models like openai, palm, and huggingface models. Users can run PromptAgent to optimize prompts for specific tasks by strategically sampling model errors, generating error feedbacks, simulating future rewards, and searching for high-reward paths leading to expert prompts.
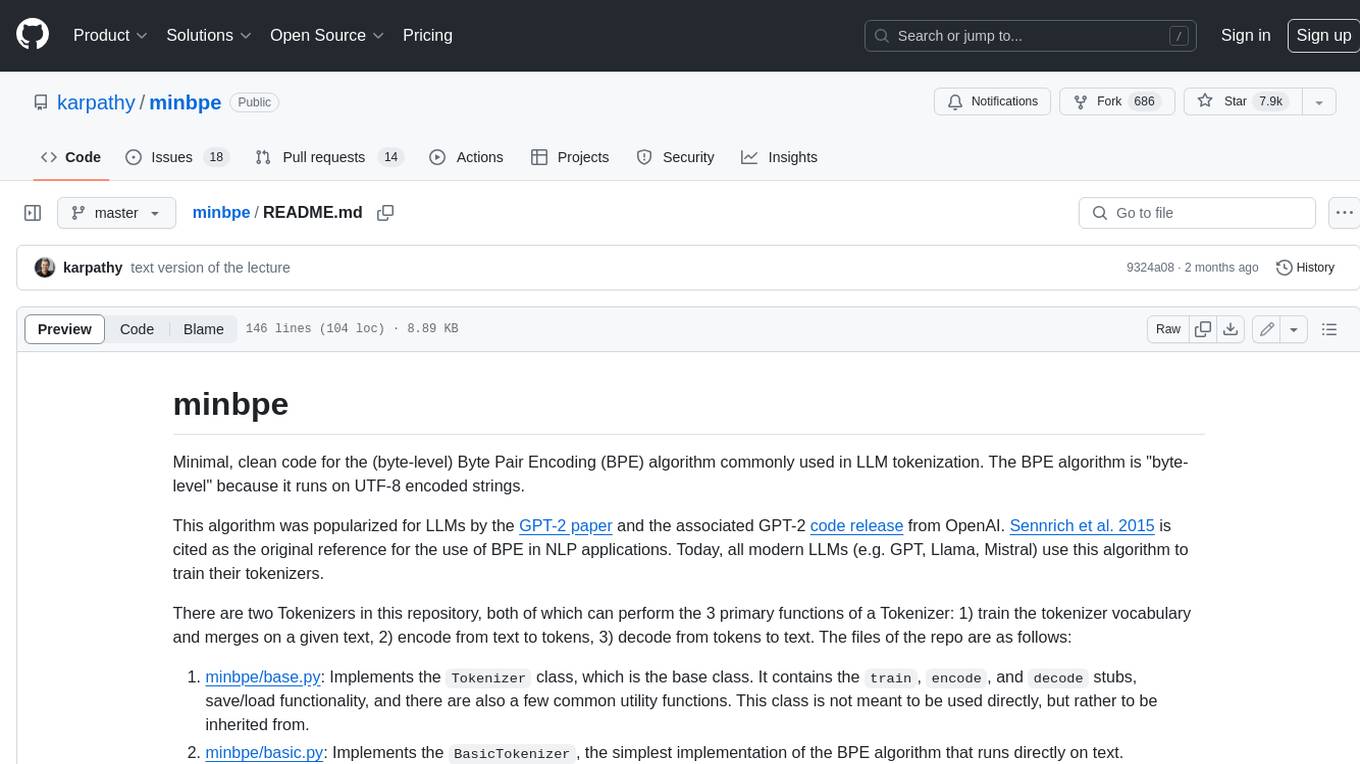
minbpe
This repository contains a minimal, clean code implementation of the Byte Pair Encoding (BPE) algorithm, commonly used in LLM tokenization. The BPE algorithm is "byte-level" because it runs on UTF-8 encoded strings. This algorithm was popularized for LLMs by the GPT-2 paper and the associated GPT-2 code release from OpenAI. Sennrich et al. 2015 is cited as the original reference for the use of BPE in NLP applications. Today, all modern LLMs (e.g. GPT, Llama, Mistral) use this algorithm to train their tokenizers. There are two Tokenizers in this repository, both of which can perform the 3 primary functions of a Tokenizer: 1) train the tokenizer vocabulary and merges on a given text, 2) encode from text to tokens, 3) decode from tokens to text. The files of the repo are as follows: 1. minbpe/base.py: Implements the `Tokenizer` class, which is the base class. It contains the `train`, `encode`, and `decode` stubs, save/load functionality, and there are also a few common utility functions. This class is not meant to be used directly, but rather to be inherited from. 2. minbpe/basic.py: Implements the `BasicTokenizer`, the simplest implementation of the BPE algorithm that runs directly on text. 3. minbpe/regex.py: Implements the `RegexTokenizer` that further splits the input text by a regex pattern, which is a preprocessing stage that splits up the input text by categories (think: letters, numbers, punctuation) before tokenization. This ensures that no merges will happen across category boundaries. This was introduced in the GPT-2 paper and continues to be in use as of GPT-4. This class also handles special tokens, if any. 4. minbpe/gpt4.py: Implements the `GPT4Tokenizer`. This class is a light wrapper around the `RegexTokenizer` (2, above) that exactly reproduces the tokenization of GPT-4 in the tiktoken library. The wrapping handles some details around recovering the exact merges in the tokenizer, and the handling of some unfortunate (and likely historical?) 1-byte token permutations. Finally, the script train.py trains the two major tokenizers on the input text tests/taylorswift.txt (this is the Wikipedia entry for her kek) and saves the vocab to disk for visualization. This script runs in about 25 seconds on my (M1) MacBook. All of the files above are very short and thoroughly commented, and also contain a usage example on the bottom of the file.
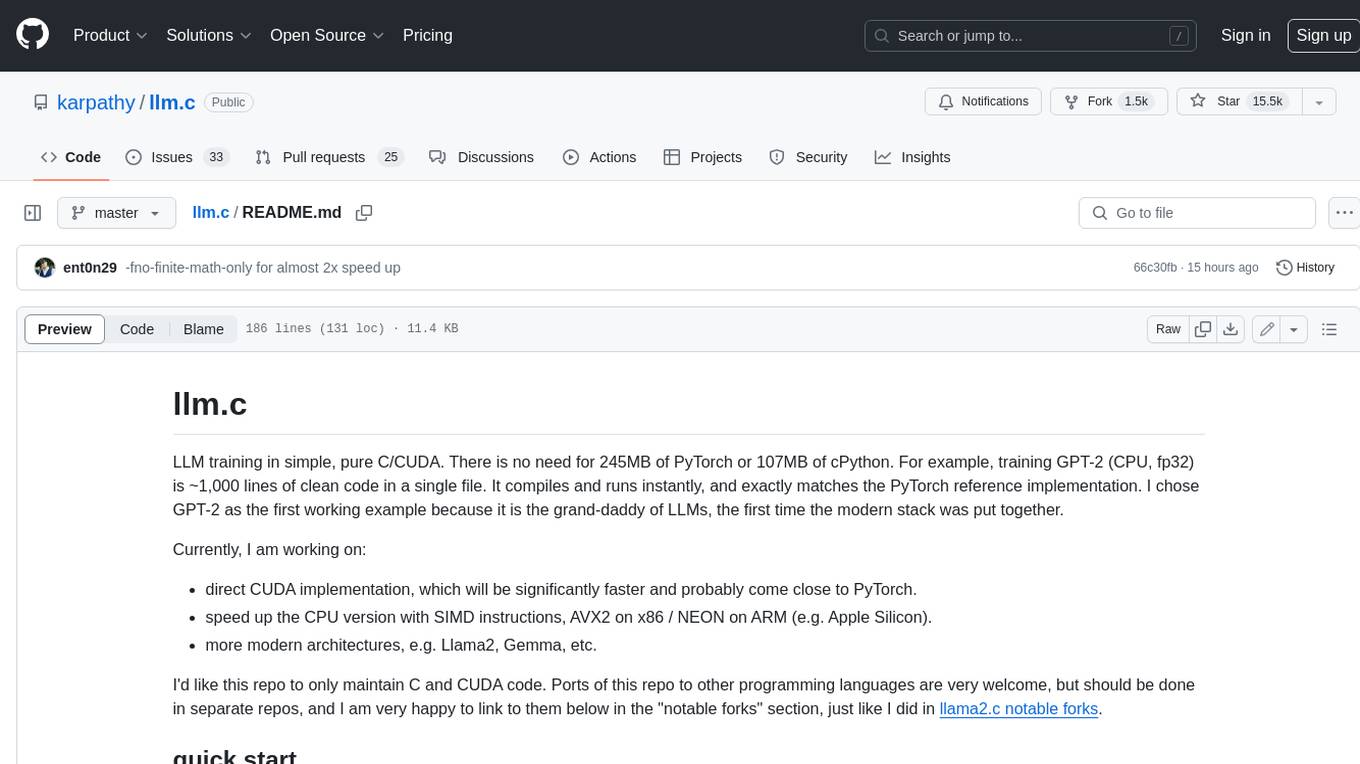
llm.c
LLM training in simple, pure C/CUDA. There is no need for 245MB of PyTorch or 107MB of cPython. For example, training GPT-2 (CPU, fp32) is ~1,000 lines of clean code in a single file. It compiles and runs instantly, and exactly matches the PyTorch reference implementation. I chose GPT-2 as the first working example because it is the grand-daddy of LLMs, the first time the modern stack was put together.
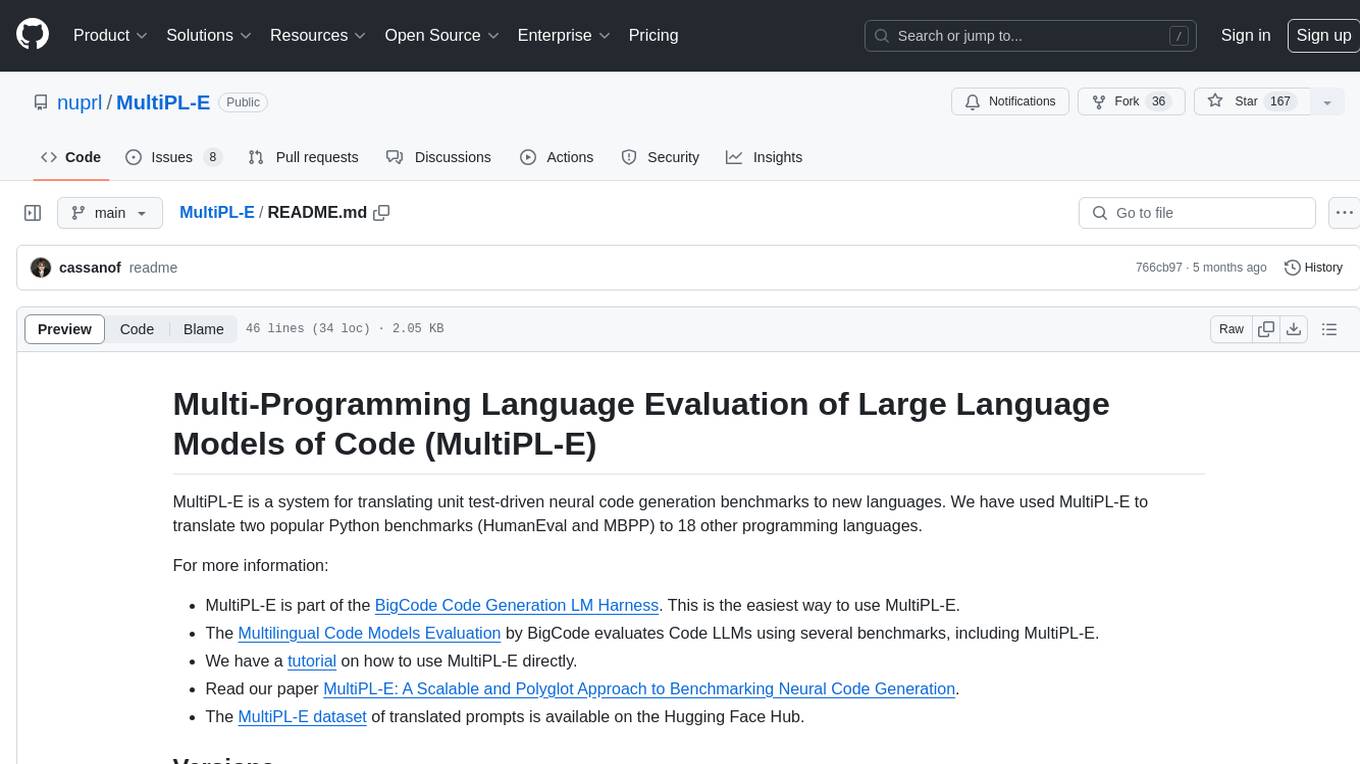
MultiPL-E
MultiPL-E is a system for translating unit test-driven neural code generation benchmarks to new languages. It is part of the BigCode Code Generation LM Harness and allows for evaluating Code LLMs using various benchmarks. The tool supports multiple versions with improvements and new language additions, providing a scalable and polyglot approach to benchmarking neural code generation. Users can access a tutorial for direct usage and explore the dataset of translated prompts on the Hugging Face Hub.
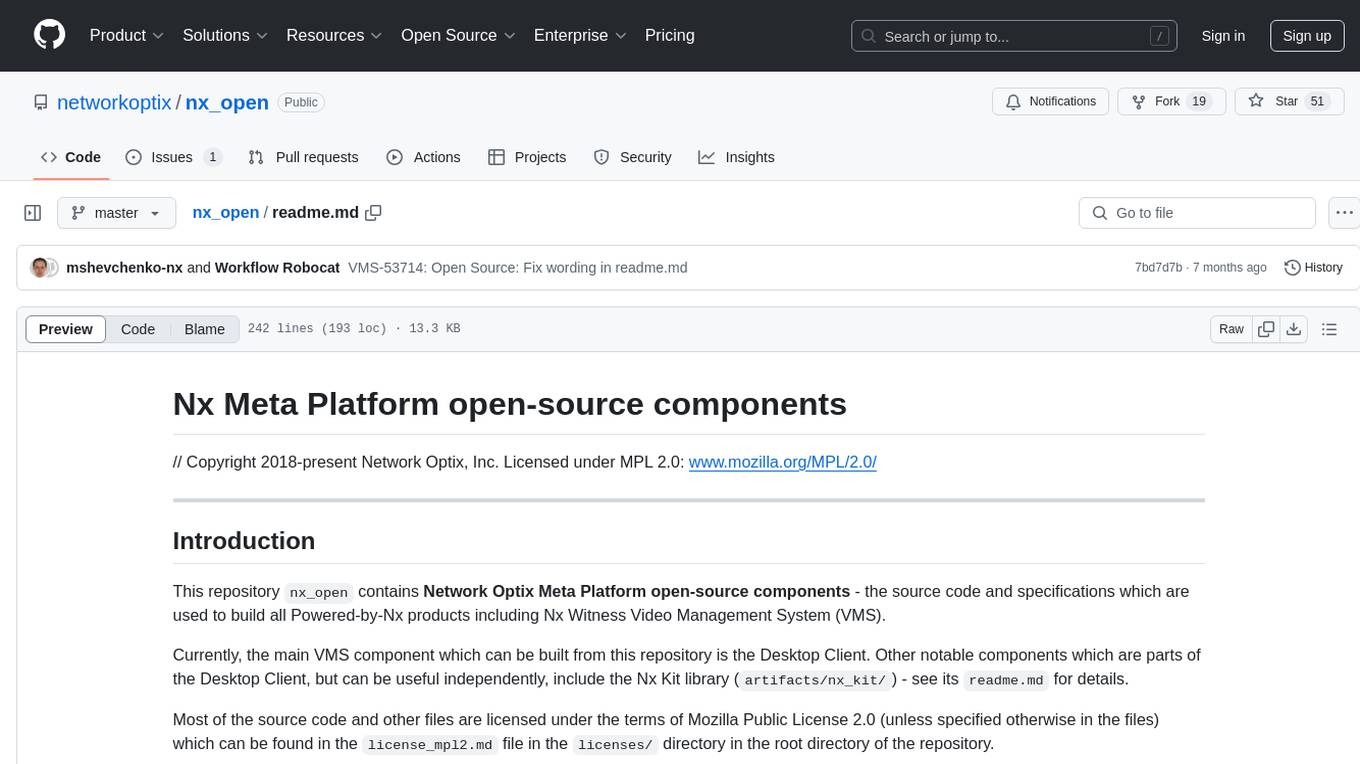
nx_open
The `nx_open` repository contains open-source components for the Network Optix Meta Platform, used to build products like Nx Witness Video Management System. It includes source code, specifications, and a Desktop Client. The repository is licensed under Mozilla Public License 2.0. Users can build the Desktop Client and customize it using a zip file. The build environment supports Windows, Linux, and macOS platforms with specific prerequisites. The repository provides scripts for building, signing executable files, and running the Desktop Client. Compatibility with VMS Server versions is crucial, and automatic VMS updates are disabled for the open-source Desktop Client.
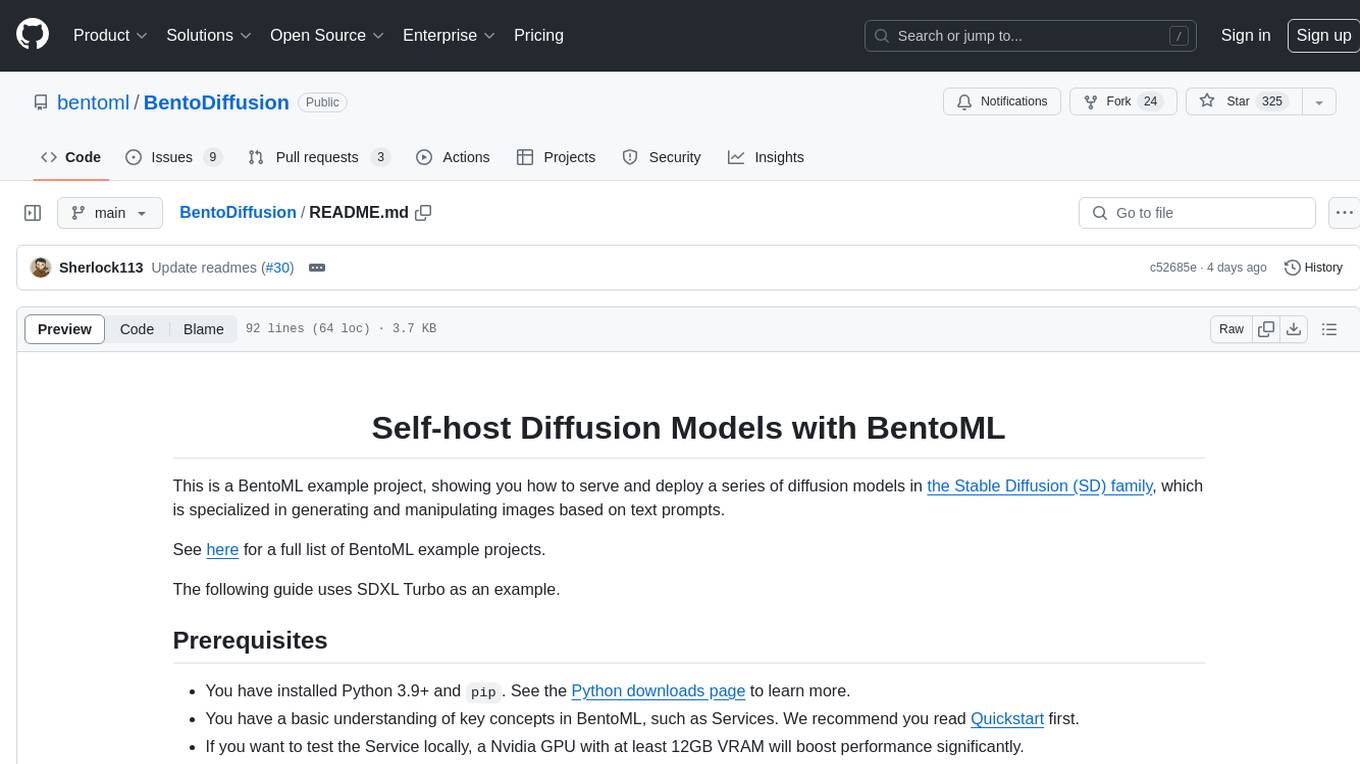
BentoDiffusion
BentoDiffusion is a BentoML example project that demonstrates how to serve and deploy diffusion models in the Stable Diffusion (SD) family. These models are specialized in generating and manipulating images based on text prompts. The project provides a guide on using SDXL Turbo as an example, along with instructions on prerequisites, installing dependencies, running the BentoML service, and deploying to BentoCloud. Users can interact with the deployed service using Swagger UI or other methods. Additionally, the project offers the option to choose from various diffusion models available in the repository for deployment.
For similar tasks
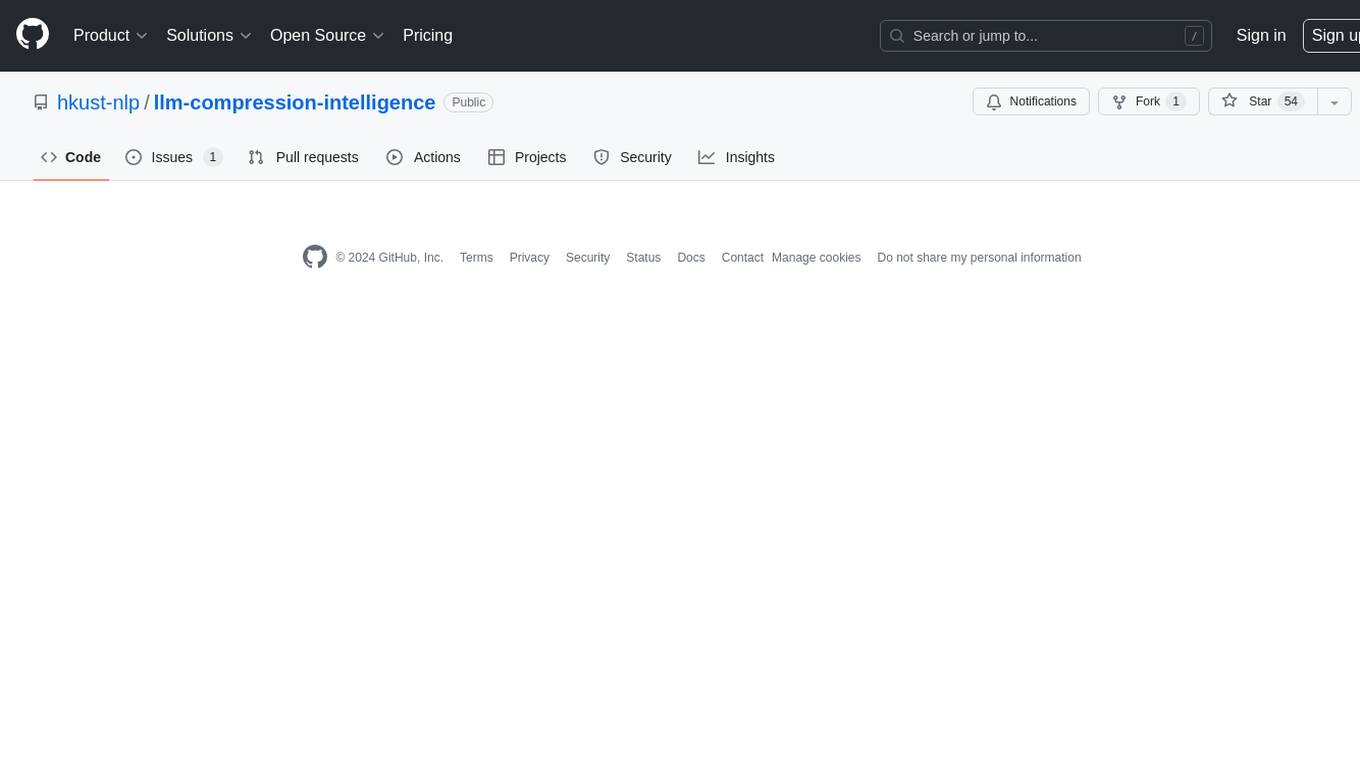
llm-compression-intelligence
This repository presents the findings of the paper "Compression Represents Intelligence Linearly". The study reveals a strong linear correlation between the intelligence of LLMs, as measured by benchmark scores, and their ability to compress external text corpora. Compression efficiency, derived from raw text corpora, serves as a reliable evaluation metric that is linearly associated with model capabilities. The repository includes the compression corpora used in the paper, code for computing compression efficiency, and data collection and processing pipelines.
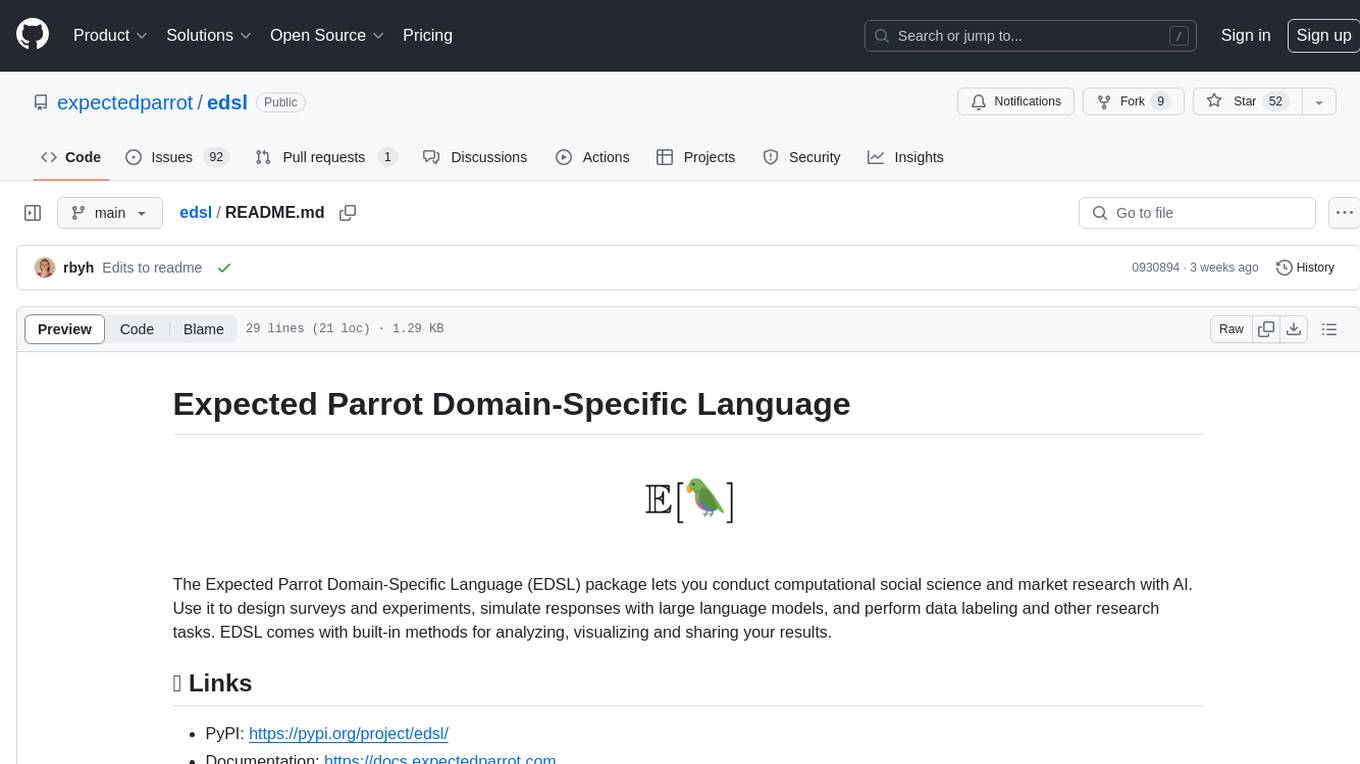
edsl
The Expected Parrot Domain-Specific Language (EDSL) package enables users to conduct computational social science and market research with AI. It facilitates designing surveys and experiments, simulating responses using large language models, and performing data labeling and other research tasks. EDSL includes built-in methods for analyzing, visualizing, and sharing research results. It is compatible with Python 3.9 - 3.11 and requires API keys for LLMs stored in a `.env` file.
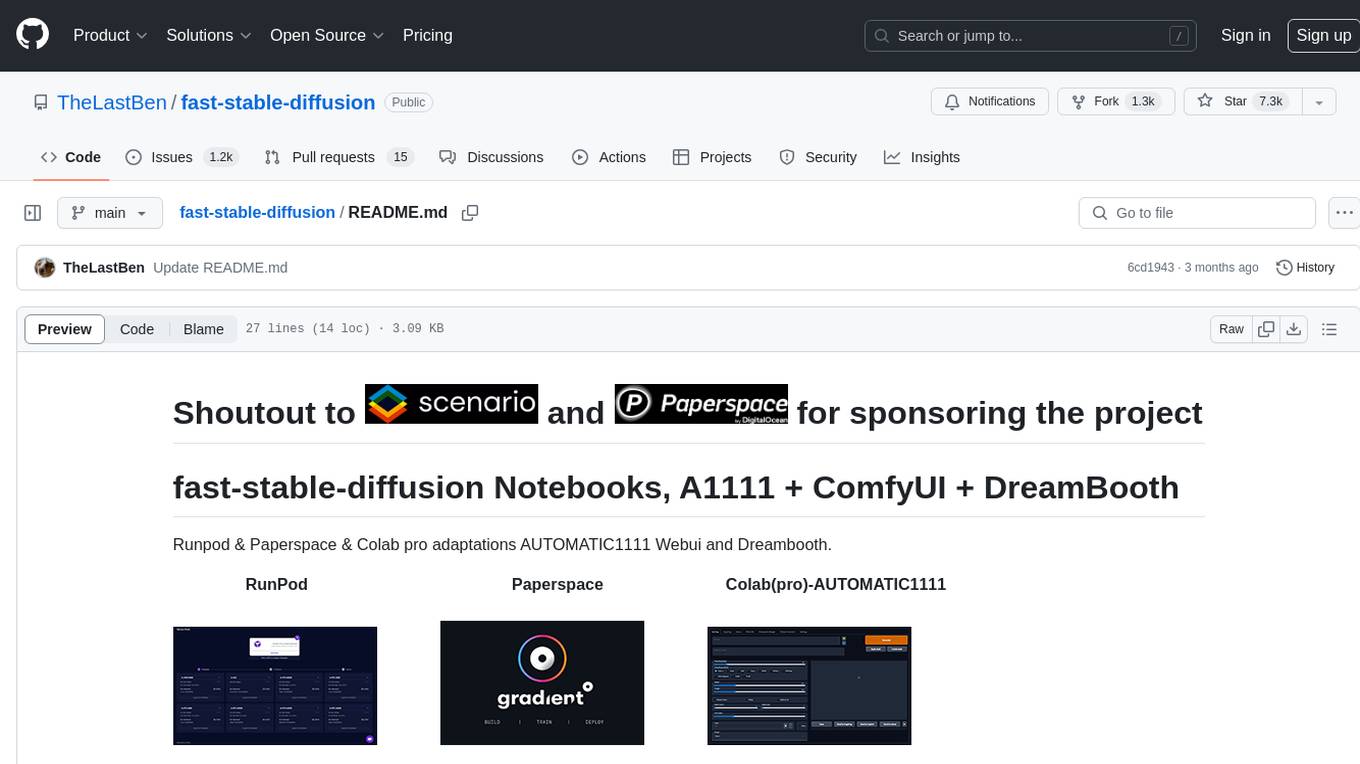
fast-stable-diffusion
Fast-stable-diffusion is a project that offers notebooks for RunPod, Paperspace, and Colab Pro adaptations with AUTOMATIC1111 Webui and Dreambooth. It provides tools for running and implementing Dreambooth, a stable diffusion project. The project includes implementations by XavierXiao and is sponsored by Runpod, Paperspace, and Colab Pro.
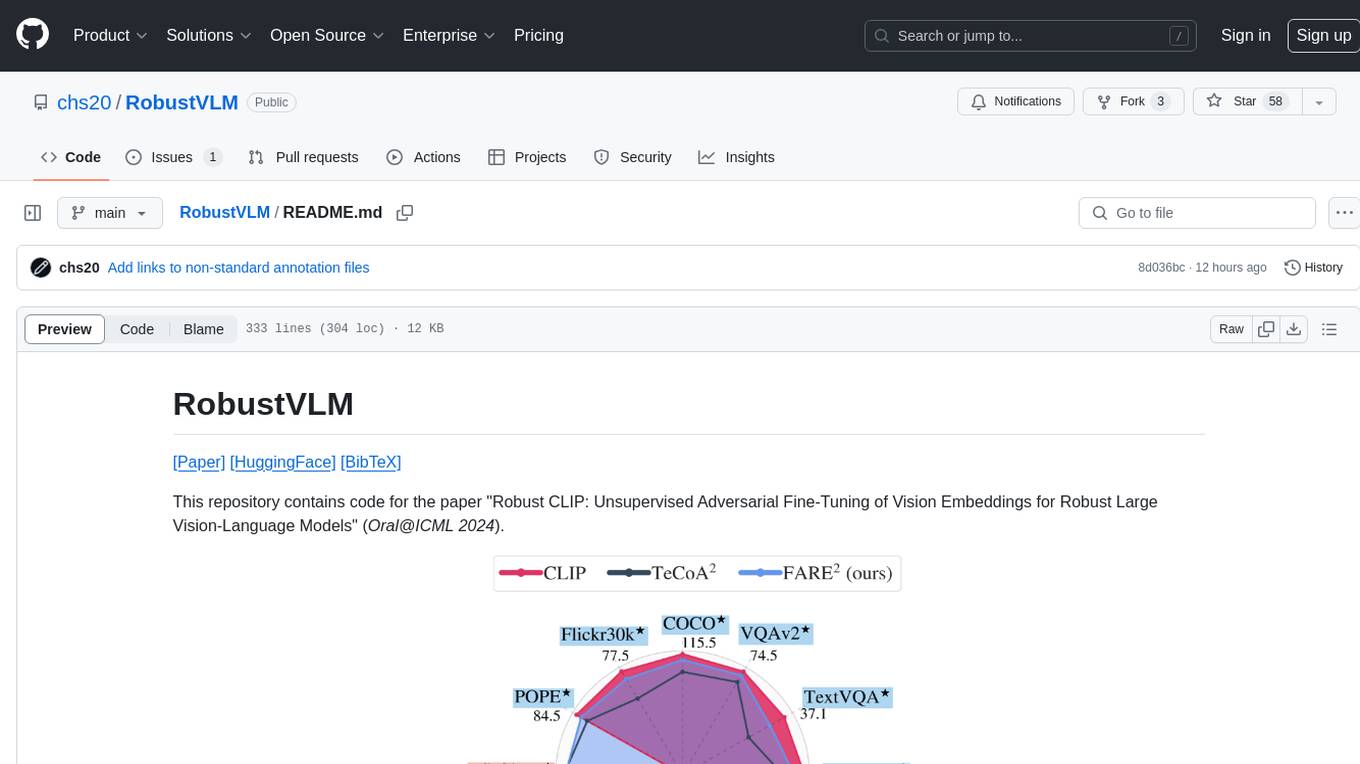
RobustVLM
This repository contains code for the paper 'Robust CLIP: Unsupervised Adversarial Fine-Tuning of Vision Embeddings for Robust Large Vision-Language Models'. It focuses on fine-tuning CLIP in an unsupervised manner to enhance its robustness against visual adversarial attacks. By replacing the vision encoder of large vision-language models with the fine-tuned CLIP models, it achieves state-of-the-art adversarial robustness on various vision-language tasks. The repository provides adversarially fine-tuned ViT-L/14 CLIP models and offers insights into zero-shot classification settings and clean accuracy improvements.
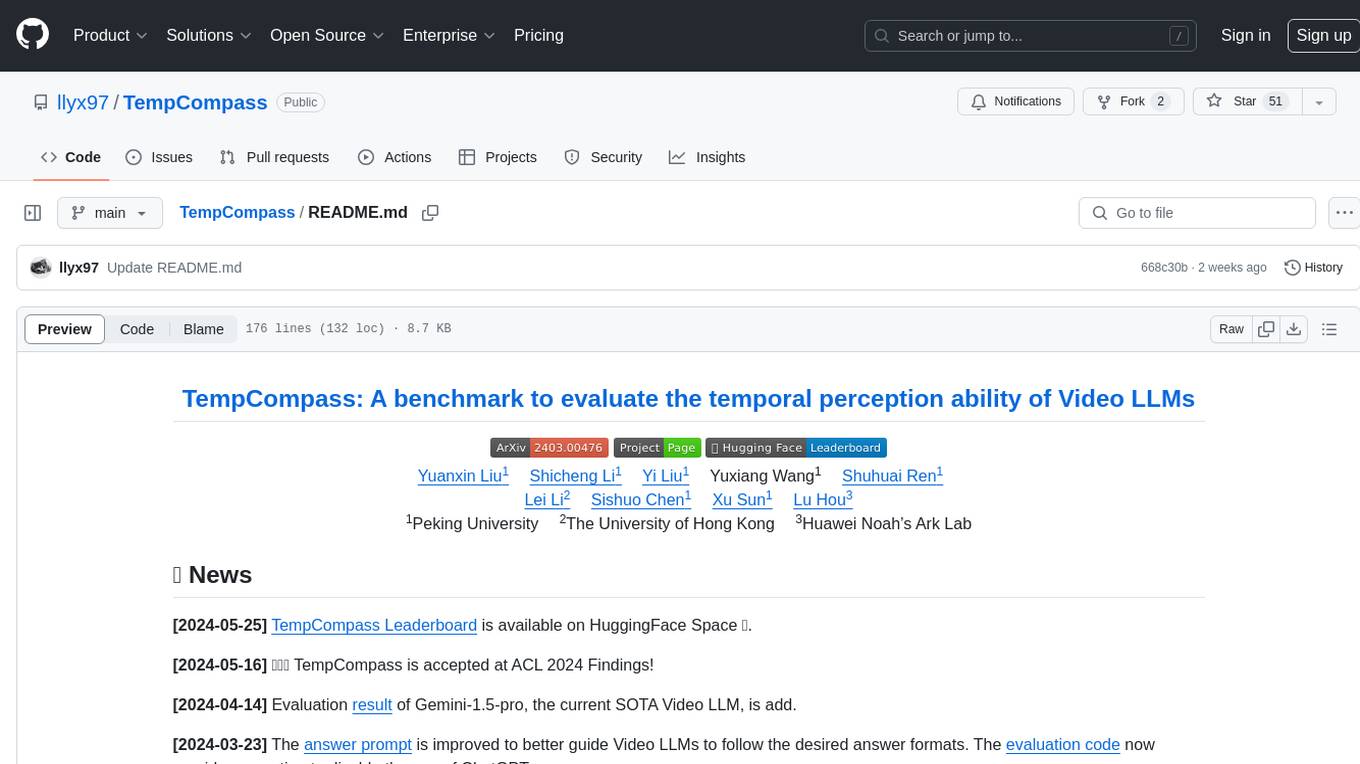
TempCompass
TempCompass is a benchmark designed to evaluate the temporal perception ability of Video LLMs. It encompasses a diverse set of temporal aspects and task formats to comprehensively assess the capability of Video LLMs in understanding videos. The benchmark includes conflicting videos to prevent models from relying on single-frame bias and language priors. Users can clone the repository, install required packages, prepare data, run inference using examples like Video-LLaVA and Gemini, and evaluate the performance of their models across different tasks such as Multi-Choice QA, Yes/No QA, Caption Matching, and Caption Generation.
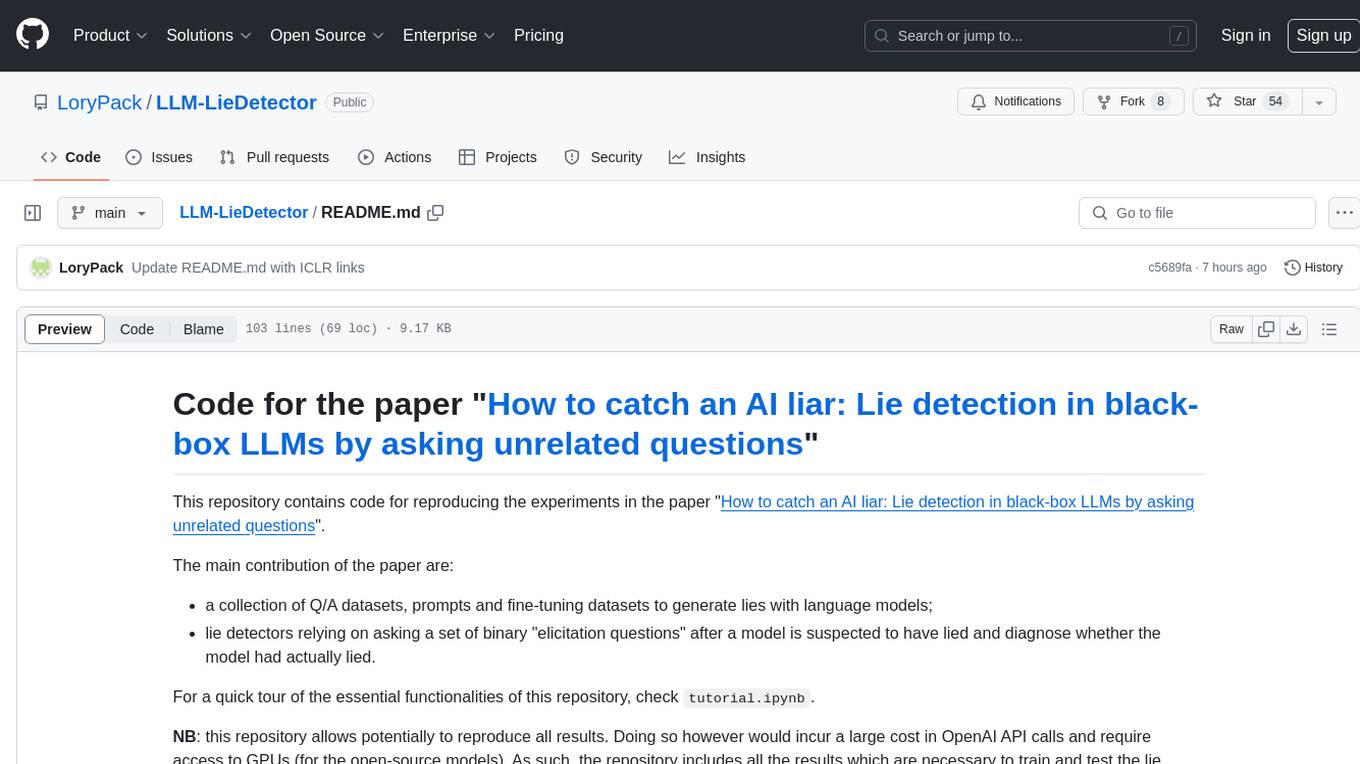
LLM-LieDetector
This repository contains code for reproducing experiments on lie detection in black-box LLMs by asking unrelated questions. It includes Q/A datasets, prompts, and fine-tuning datasets for generating lies with language models. The lie detectors rely on asking binary 'elicitation questions' to diagnose whether the model has lied. The code covers generating lies from language models, training and testing lie detectors, and generalization experiments. It requires access to GPUs and OpenAI API calls for running experiments with open-source models. Results are stored in the repository for reproducibility.
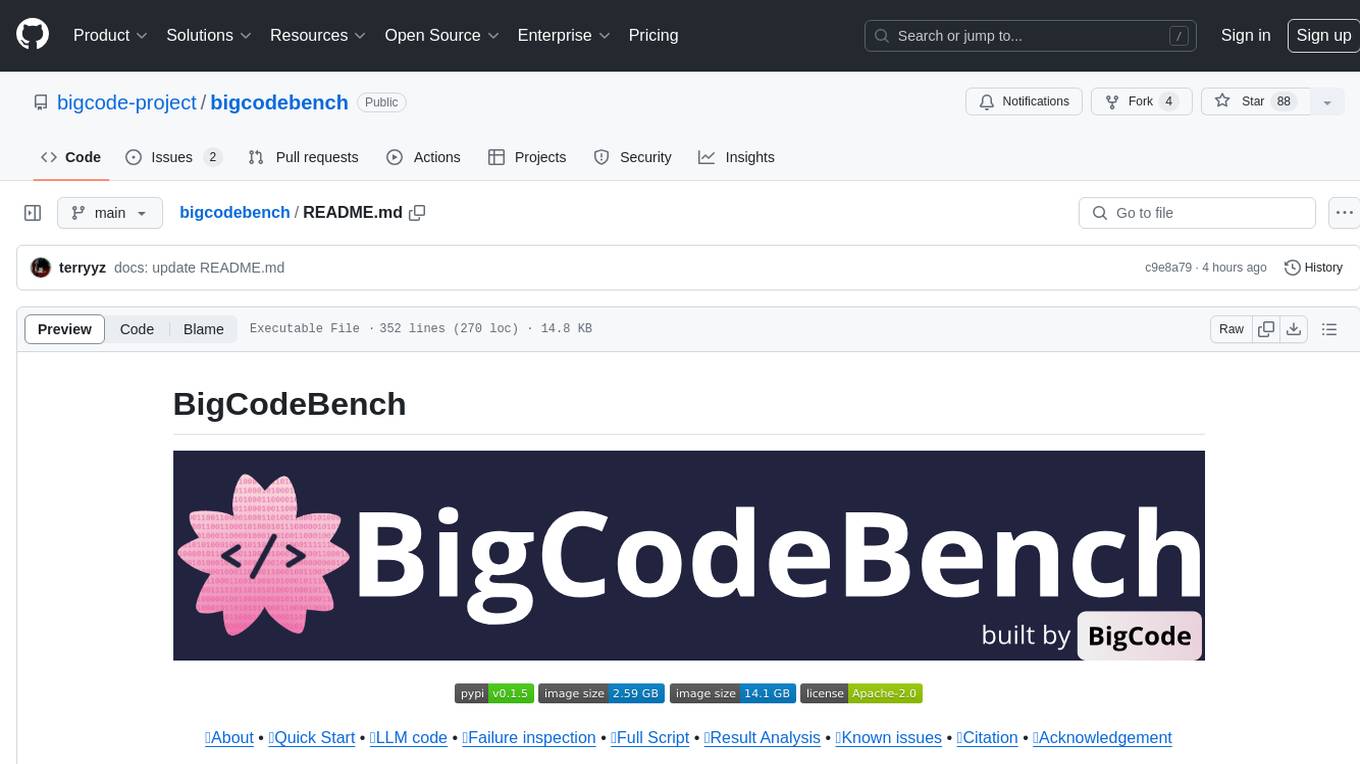
bigcodebench
BigCodeBench is an easy-to-use benchmark for code generation with practical and challenging programming tasks. It aims to evaluate the true programming capabilities of large language models (LLMs) in a more realistic setting. The benchmark is designed for HumanEval-like function-level code generation tasks, but with much more complex instructions and diverse function calls. BigCodeBench focuses on the evaluation of LLM4Code with diverse function calls and complex instructions, providing precise evaluation & ranking and pre-generated samples to accelerate code intelligence research. It inherits the design of the EvalPlus framework but differs in terms of execution environment and test evaluation.
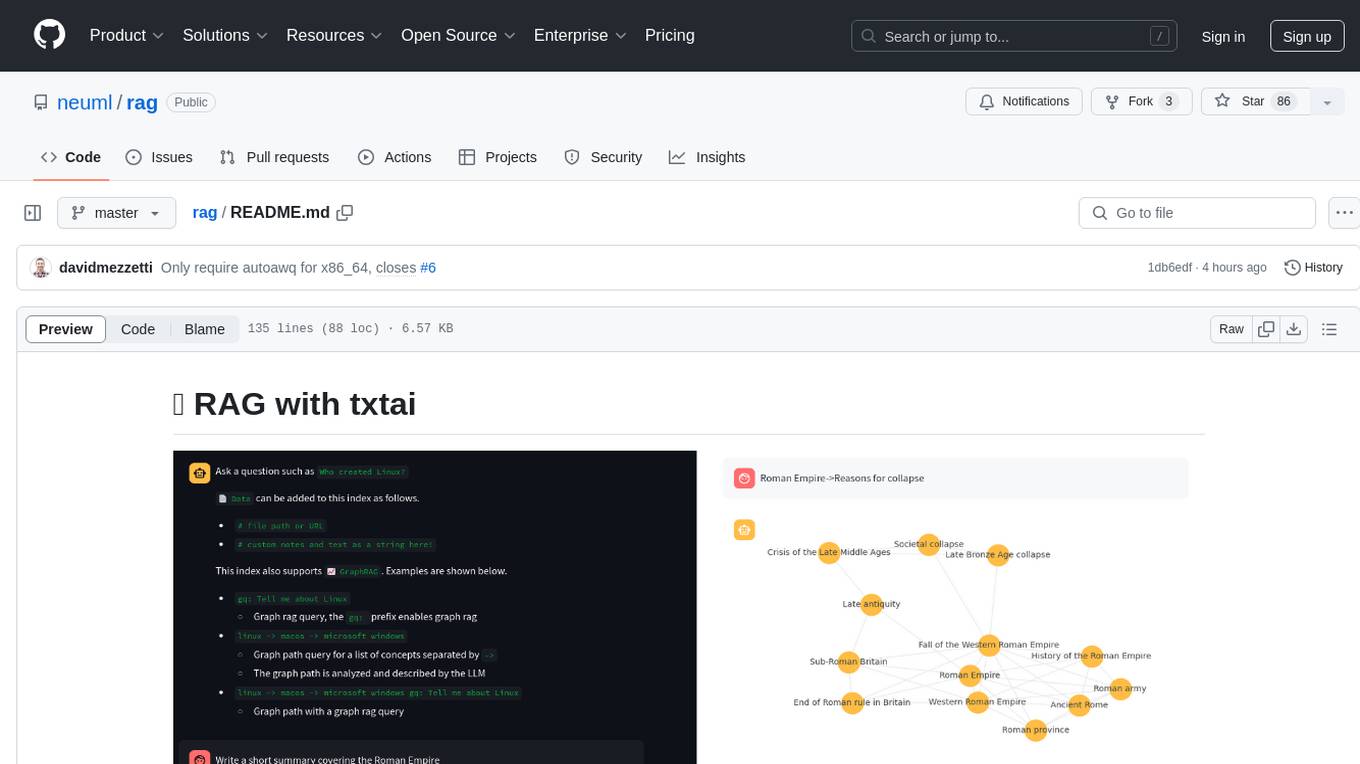
rag
RAG with txtai is a Retrieval Augmented Generation (RAG) Streamlit application that helps generate factually correct content by limiting the context in which a Large Language Model (LLM) can generate answers. It supports two categories of RAG: Vector RAG, where context is supplied via a vector search query, and Graph RAG, where context is supplied via a graph path traversal query. The application allows users to run queries, add data to the index, and configure various parameters to control its behavior.
For similar jobs
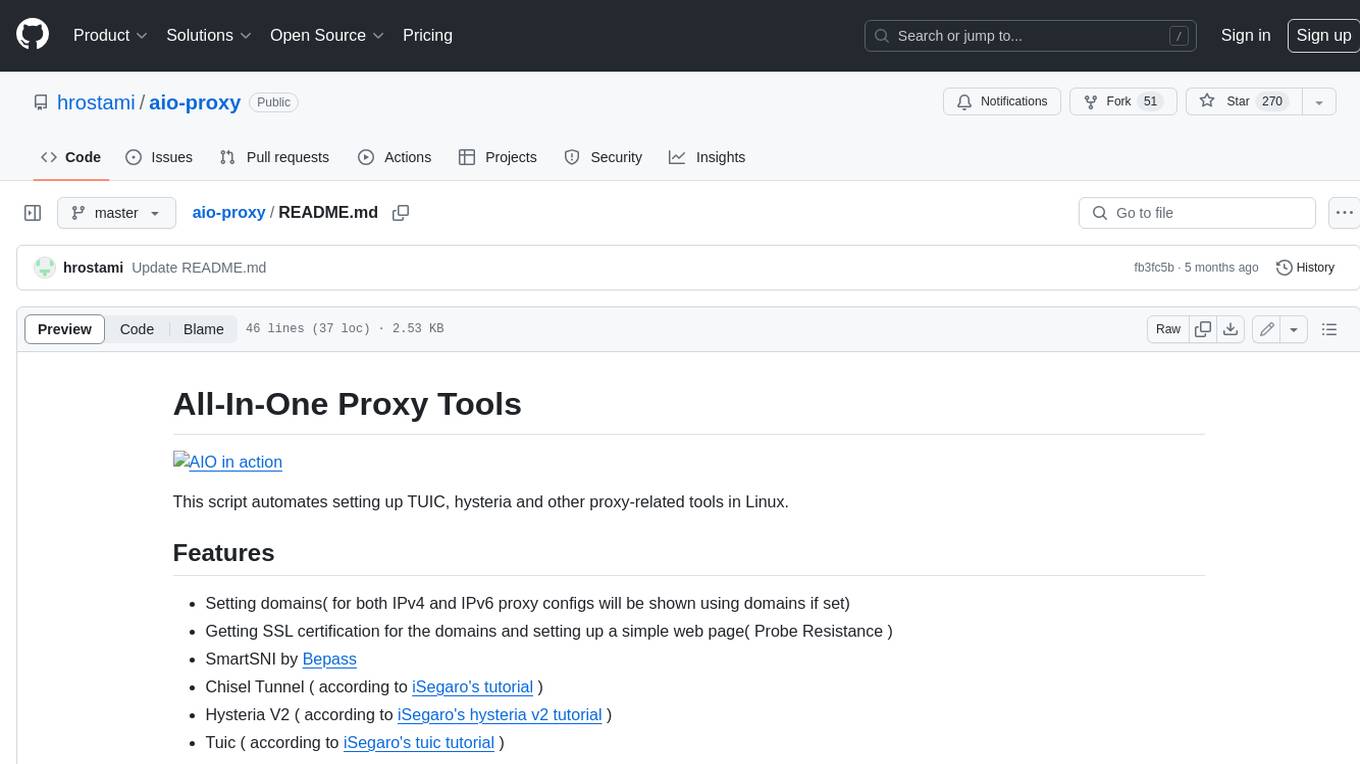
aio-proxy
This script automates setting up TUIC, hysteria and other proxy-related tools in Linux. It features setting domains, getting SSL certification, setting up a simple web page, SmartSNI by Bepass, Chisel Tunnel, Hysteria V2, Tuic, Hiddify Reality Scanner, SSH, Telegram Proxy, Reverse TLS Tunnel, different panels, installing, disabling, and enabling Warp, Sing Box 4-in-1 script, showing ports in use and their corresponding processes, and an Android script to use Chisel tunnel.
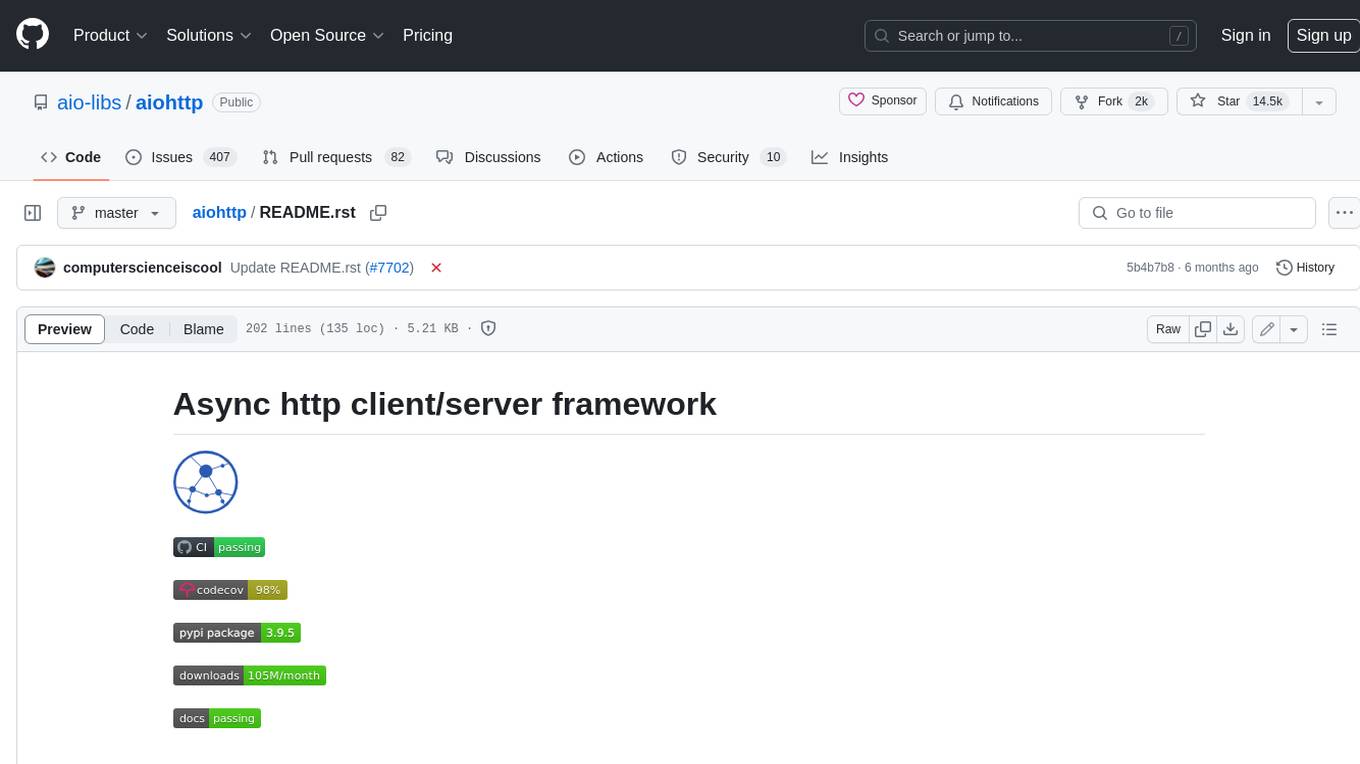
aiohttp
aiohttp is an async http client/server framework that supports both client and server side of HTTP protocol. It also supports both client and server Web-Sockets out-of-the-box and avoids Callback Hell. aiohttp provides a Web-server with middleware and pluggable routing.
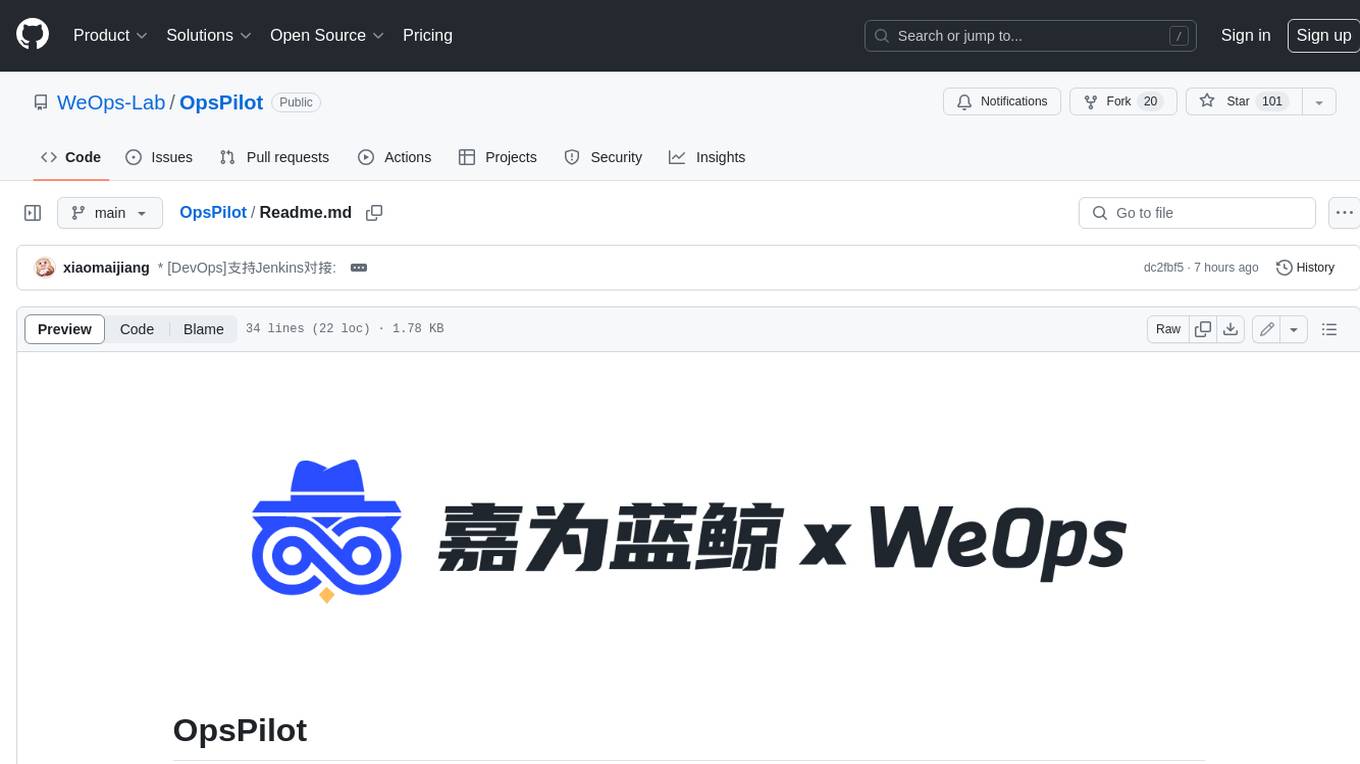
OpsPilot
OpsPilot is an AI-powered operations navigator developed by the WeOps team. It leverages deep learning and LLM technologies to make operations plans interactive and generalize and reason about local operations knowledge. OpsPilot can be integrated with web applications in the form of a chatbot and primarily provides the following capabilities: 1. Operations capability precipitation: By depositing operations knowledge, operations skills, and troubleshooting actions, when solving problems, it acts as a navigator and guides users to solve operations problems through dialogue. 2. Local knowledge Q&A: By indexing local knowledge and Internet knowledge and combining the capabilities of LLM, it answers users' various operations questions. 3. LLM chat: When the problem is beyond the scope of OpsPilot's ability to handle, it uses LLM's capabilities to solve various long-tail problems.
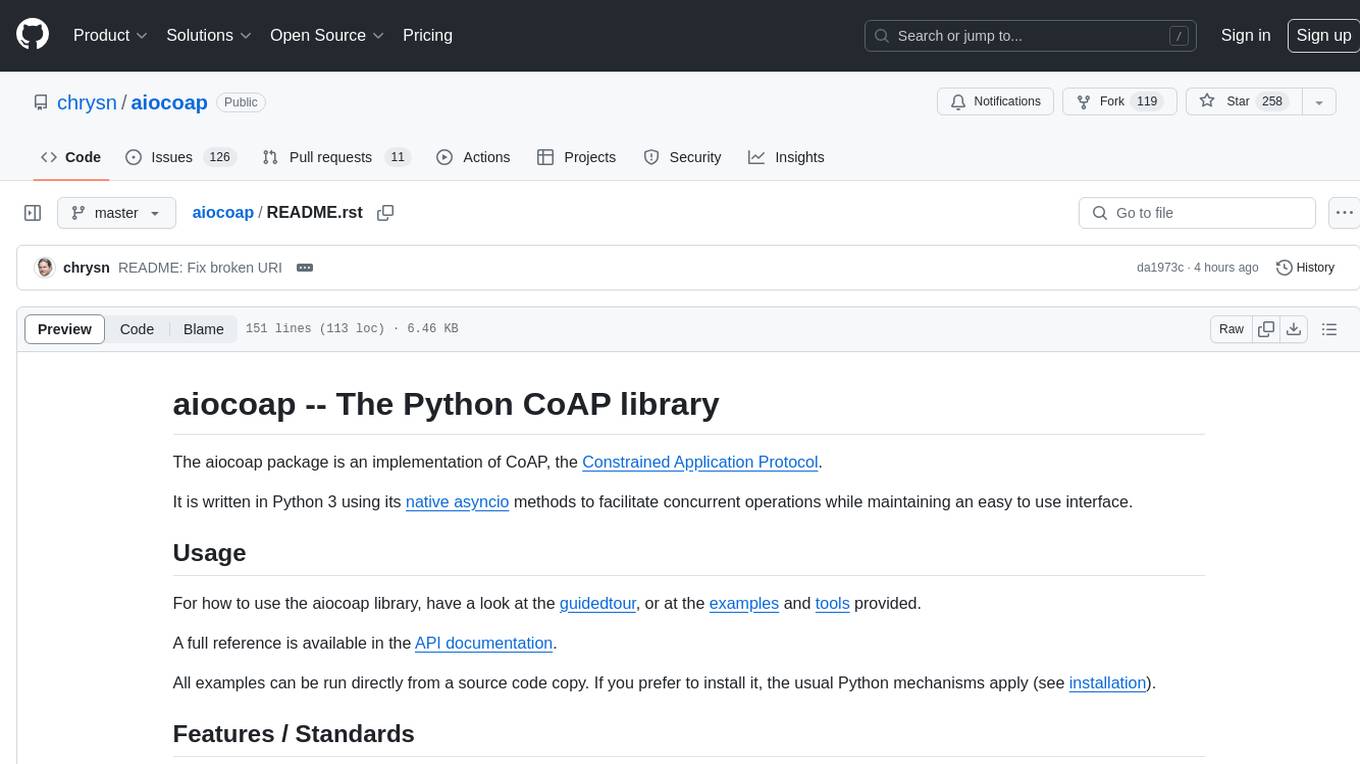
aiocoap
aiocoap is a Python library that implements the Constrained Application Protocol (CoAP) using native asyncio methods in Python 3. It supports various CoAP standards such as RFC7252, RFC7641, RFC7959, RFC8323, RFC7967, RFC8132, RFC9176, RFC8613, and draft-ietf-core-oscore-groupcomm-17. The library provides features for clients and servers, including multicast support, blockwise transfer, CoAP over TCP, TLS, and WebSockets, No-Response, PATCH/FETCH, OSCORE, and Group OSCORE. It offers an easy-to-use interface for concurrent operations and is suitable for IoT applications.
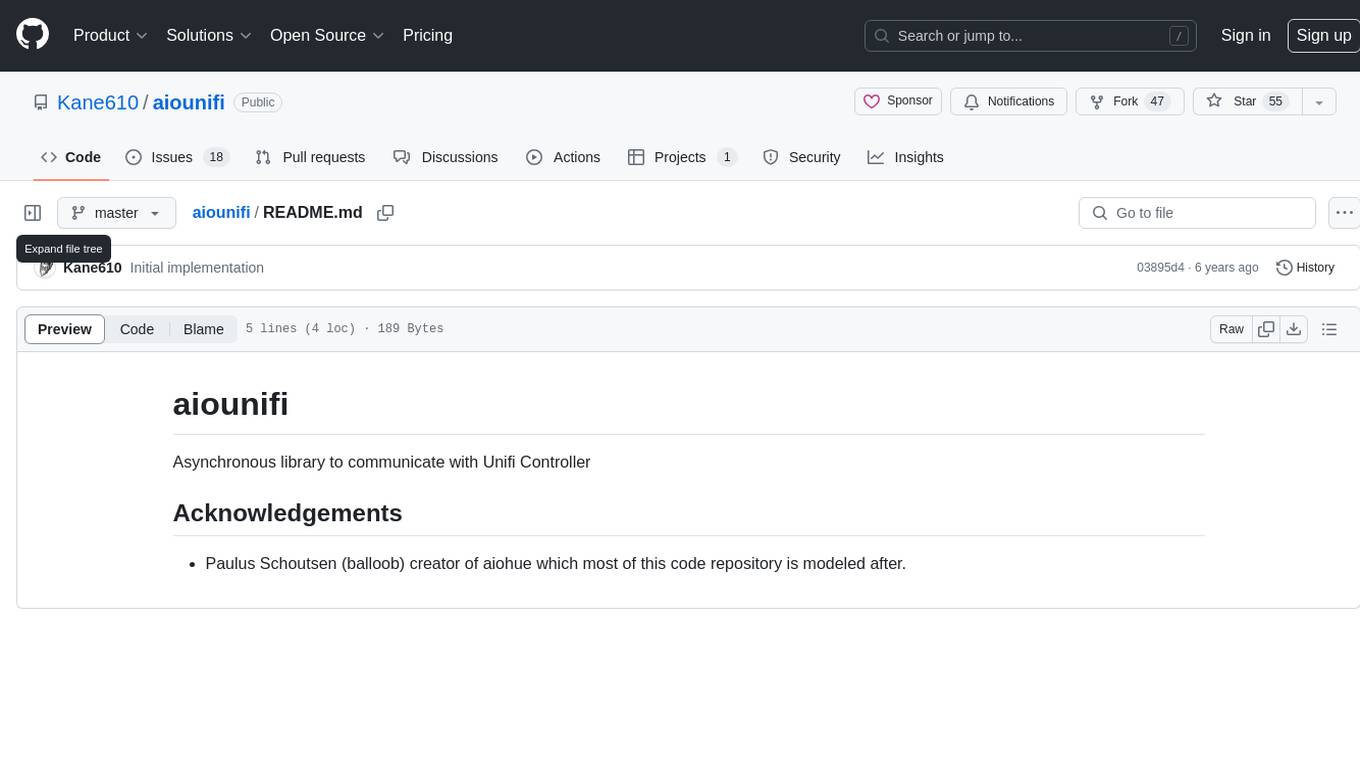
aiounifi
Aiounifi is a Python library that provides a simple interface for interacting with the Unifi Controller API. It allows users to easily manage their Unifi network devices, such as access points, switches, and gateways, through automated scripts or applications. With Aiounifi, users can retrieve device information, perform configuration changes, monitor network performance, and more, all through a convenient and efficient API wrapper. This library simplifies the process of integrating Unifi network management into custom solutions, making it ideal for network administrators, developers, and enthusiasts looking to automate and streamline their network operations.
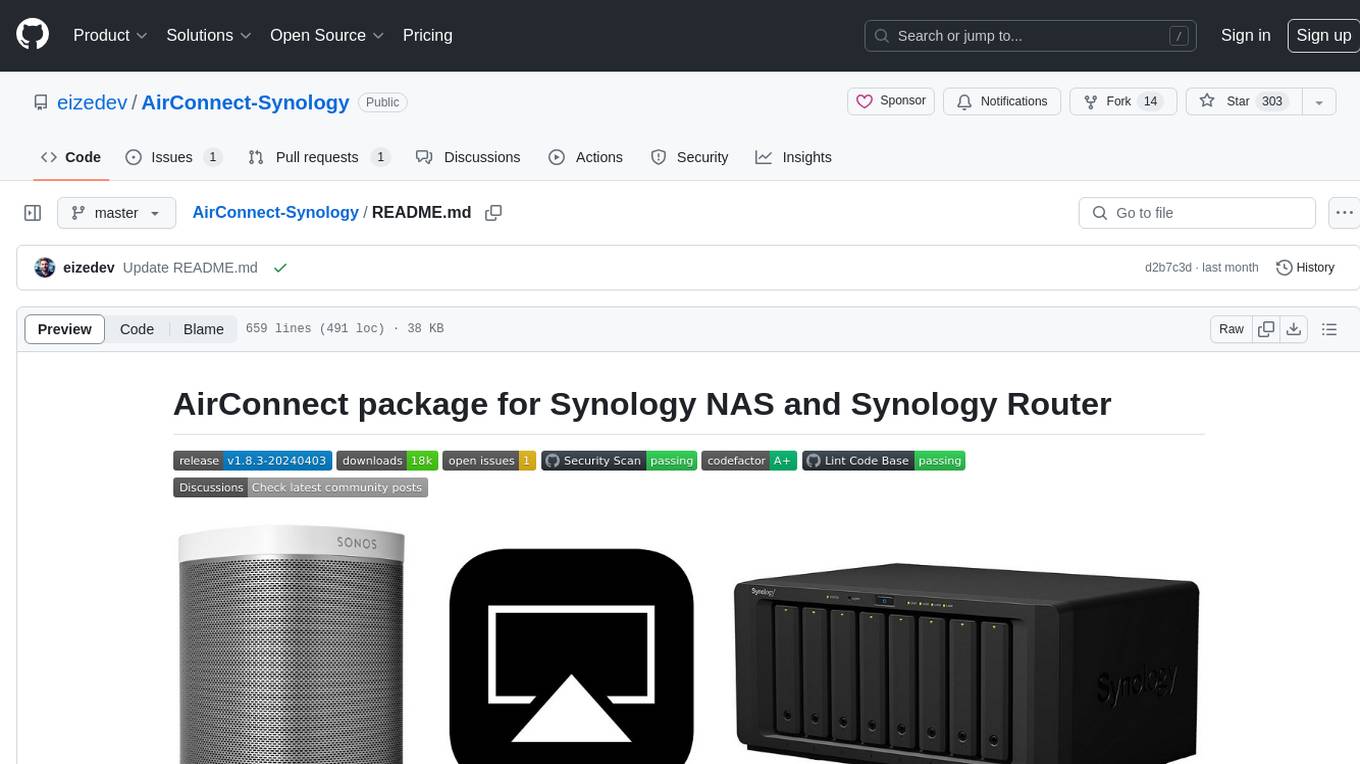
AirConnect-Synology
AirConnect-Synology is a minimal Synology package that allows users to use AirPlay to stream to UPnP/Sonos & Chromecast devices that do not natively support AirPlay. It is compatible with DSM 7.0 and DSM 7.1, and provides detailed information on installation, configuration, supported devices, troubleshooting, and more. The package automates the installation and usage of AirConnect on Synology devices, ensuring compatibility with various architectures and firmware versions. Users can customize the configuration using the airconnect.conf file and adjust settings for specific speakers like Sonos, Bose SoundTouch, and Pioneer/Phorus/Play-Fi.
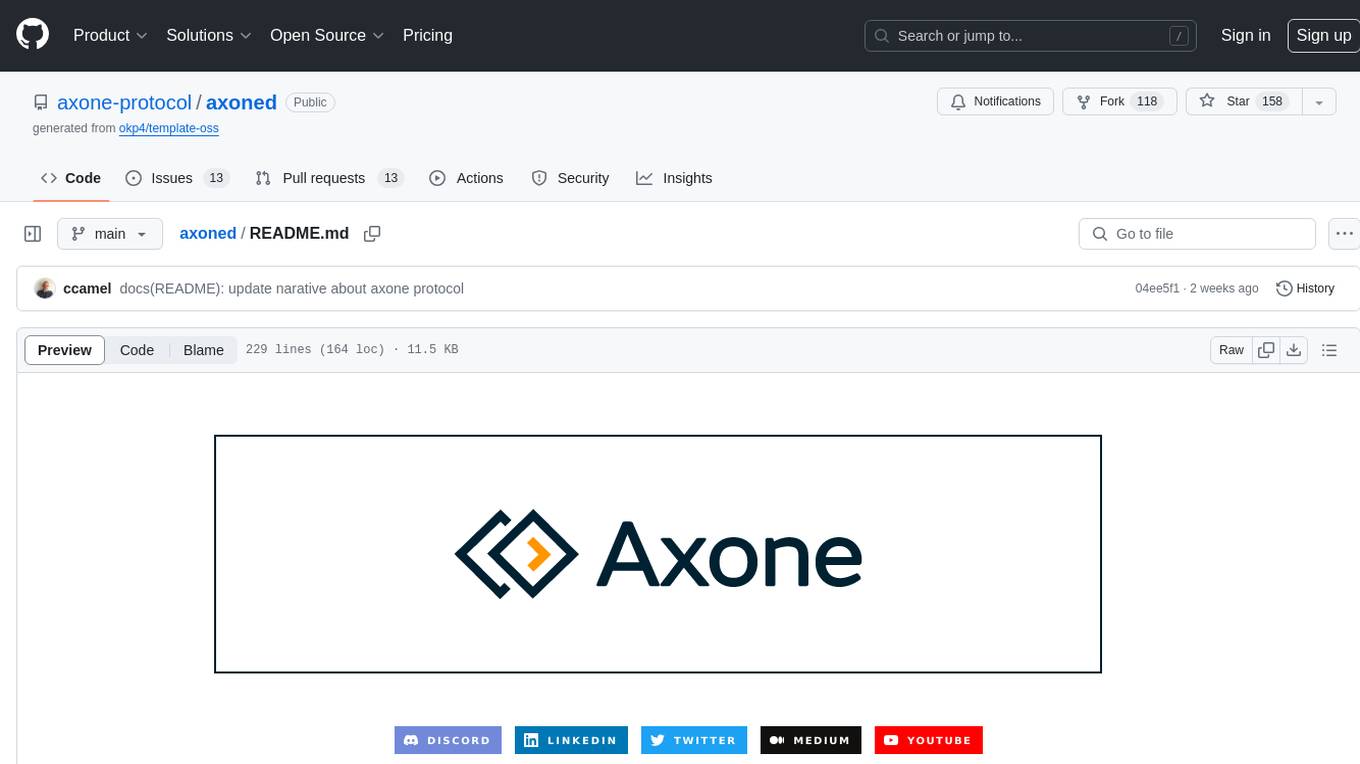
axoned
Axone is a public dPoS layer 1 designed for connecting, sharing, and monetizing resources in the AI stack. It is an open network for collaborative AI workflow management compatible with any data, model, or infrastructure, allowing sharing of data, algorithms, storage, compute, APIs, both on-chain and off-chain. The 'axoned' node of the AXONE network is built on Cosmos SDK & Tendermint consensus, enabling companies & individuals to define on-chain rules, share off-chain resources, and create new applications. Validators secure the network by maintaining uptime and staking $AXONE for rewards. The blockchain supports various platforms and follows Semantic Versioning 2.0.0. A docker image is available for quick start, with documentation on querying networks, creating wallets, starting nodes, and joining networks. Development involves Go and Cosmos SDK, with smart contracts deployed on the AXONE blockchain. The project provides a Makefile for building, installing, linting, and testing. Community involvement is encouraged through Discord, open issues, and pull requests.
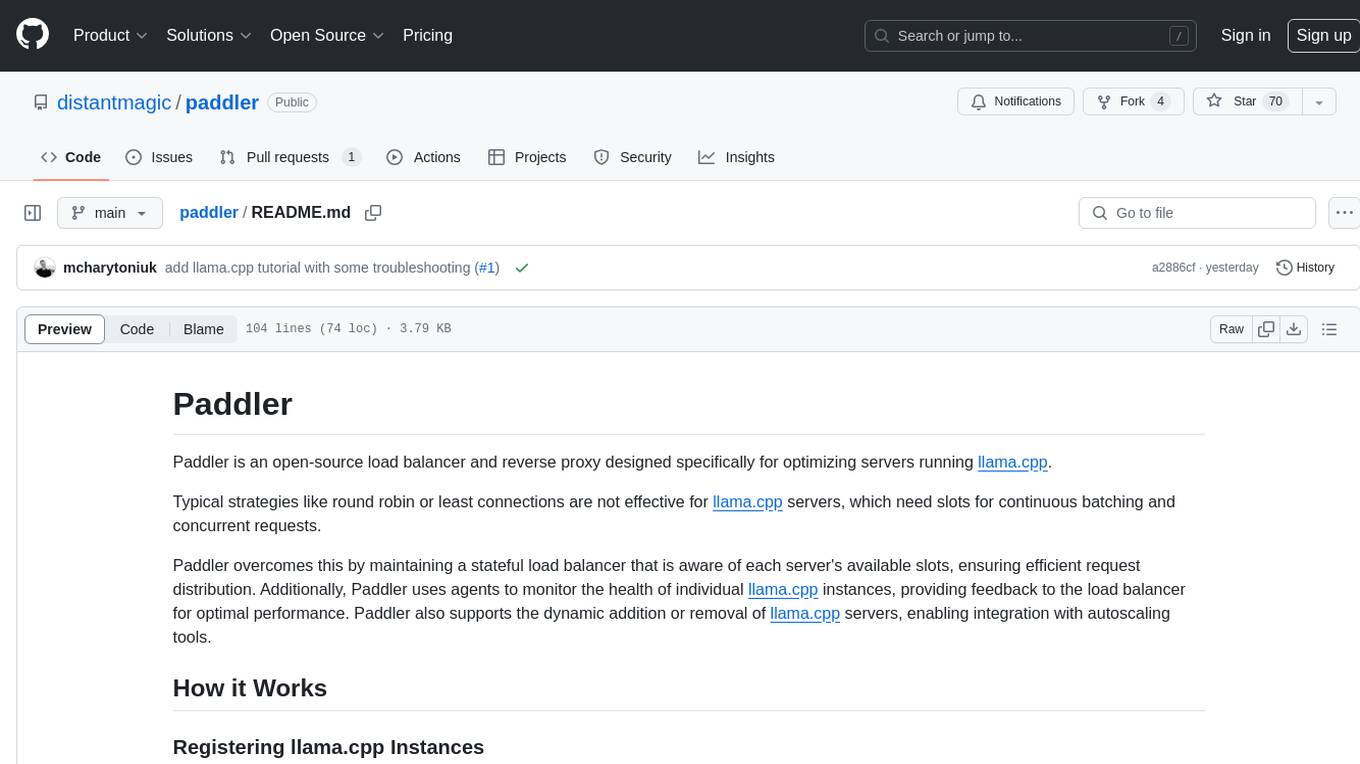
paddler
Paddler is an open-source load balancer and reverse proxy designed specifically for optimizing servers running llama.cpp. It overcomes typical load balancing challenges by maintaining a stateful load balancer that is aware of each server's available slots, ensuring efficient request distribution. Paddler also supports dynamic addition or removal of servers, enabling integration with autoscaling tools.