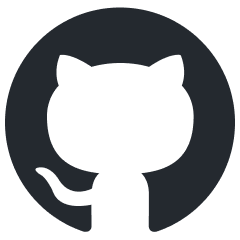
MultiPL-E
A multi-programming language benchmark for LLMs
Stars: 219
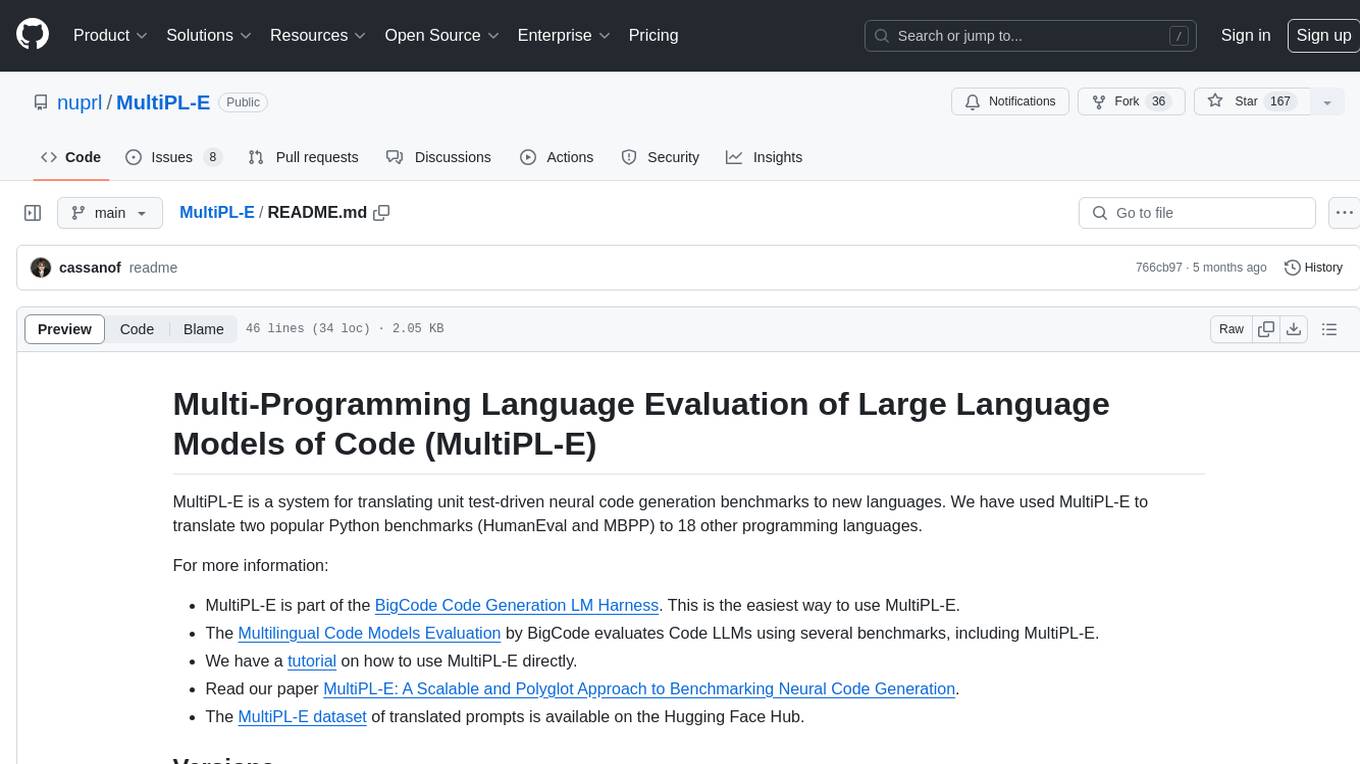
MultiPL-E is a system for translating unit test-driven neural code generation benchmarks to new languages. It is part of the BigCode Code Generation LM Harness and allows for evaluating Code LLMs using various benchmarks. The tool supports multiple versions with improvements and new language additions, providing a scalable and polyglot approach to benchmarking neural code generation. Users can access a tutorial for direct usage and explore the dataset of translated prompts on the Hugging Face Hub.
README:
MultiPL-E is a system for translating unit test-driven neural code generation benchmarks to new languages. We have used MultiPL-E to translate two popular Python benchmarks (HumanEval and MBPP) to 18 other programming languages.
For more information:
- MultiPL-E is part of the BigCode Code Generation LM Harness. This is the easiest way to use MultiPL-E.
- The Multilingual Code Models Evaluation by BigCode evaluates Code LLMs using several benchmarks, including MultiPL-E.
- Read our paper MultiPL-E: A Scalable and Polyglot Approach to Benchmarking Neural Code Generation.
- The MultiPL-E dataset of translated prompts is available on the Hugging Face Hub.
These are instructions on how to use MultiPl-E directly, without the BigCode evaluation harness.
In this tutorial, we will run a small experiment to evaluate the performance of
SantaCoder on Rust with a small subset of the MBPP benchmarks.
We will only fetch 20 completions per problem, so that you
can run it quickly on a single machine.
You can also run on the full suite of benchmarks or substitute your own
benchmark programs. Later, we'll show you how to add support for other languages
and evaluate other models.
-
You will need Python 3.8 or higher.
-
You will need to install some Python packages:
pip3 install aiohttp numpy tqdm pytest datasets torch transformers
-
Check out the repository:
git clone https://github.com/nuprl/MultiPL-E
-
Enter the repository directory:
cd MultiPL-E
Out of the box, MultiPL-E supports several models, programming languages, and datasets. Using MultiPL-E is a two step process:
-
We generate completions, which requires a GPU.
-
We execute the generated completions, which requires a machine that supports Docker or Podman.
The following command will generate completions for the HumanEval benchmark, which is originally in Python, but translated to Rust with MultiPL-E:
mkdir tutorial
python3 automodel.py \
--name bigcode/gpt_bigcode-santacoder \
--root-dataset humaneval \
--lang rs \
--temperature 0.2 \
--batch-size 20 \
--completion-limit 20 \
--output-dir-prefix tutorial
The model name above refers to the SantaCoder model on the Hugging Face Hub. You can use any other text generation model instead.
Notes:
-
This command requires about 13 GB VRAM and takes 30 minutes with a Quadro RTX 6000.
-
If you have less VRAM, you can set
--batch-size
to a smaller value. E.g., with--batch-size 10
it should work on consumer graphics cards, such as the RTX series cards. -
If you're feeling impatient, you can kill the command early (use
Ctrl+C
) before all generations are complete. Your results won't be accurate, but you can move on to the evaluation step to get a partial result. Before killing generation, ensure that a few files have been generated:ls tutorial/*/*.json.gz
You can run MultiPL-E's execution with or without a container, but we strongly recommend using the container that we have provided. The container includes the toolchains for all languages that we support. Without it, you will need to painstakingly install them again. There is also a risk that the generated code may do something that breaks your system. The container mitigates that risk.
When you first run evaluation, you need to pull and tag the execution container:
podman pull ghcr.io/nuprl/multipl-e-evaluation
podman tag ghcr.io/nuprl/multipl-e-evaluation multipl-e-eval
The following command will run execution on the generated completions:
podman run --rm --network none -v ./tutorial:/tutorial:rw multipl-e-eval --dir /tutorial --output-dir /tutorial --recursive
If execution is successful, you will see several .results.json.gz
files
alongside the .json.gz
files that were created during generation:
ls tutorial/*/*.results.json.gz
Assuming you have setup the needed language toolchains, here is how you do executions without a container:
cd evaluation/src
python3 main.py --dir ../../tutorial --output-dir ../../tutorial --recursive
If execution is successful, you will see several .results.json.gz
files
alongside the .json.gz
files that were created during generation:
ls ../../tutorial/*/*.results.json.gz
Finally, you can calculate the pass rates:
python3 pass_k.py ./tutorial/*
The experiment prints pass rates for k=1 as we only made 20 results at
temperature 0.2. If you want to see pass@10 and pass@100 pass rates, you
can regenerate with --temperature 0.8
.
Warning: In generation, we used --completion-limit 20
to only generate
20 samples for each prompt. You should remove this flag to generate 200 samples
for temperature 0.8. We have found that 20 samples is adequate for estimate
pass@1 (there will be a little variance). However, you need more samples to estimate
pass@10 and pass@100.
If you want to learn by example, you can look at pull requests that have added support for several languages:
In general, you need to make three changes to support a new language L:
-
Write an execution script to run and test L language that goes in evaluation/src.
-
Write a translator to translate benchmarks to L that new language that goes in dataset_builder.
-
Add terms for L to
dataset_builder/terms.csv
to translate comments.
Let's say we had not included Perl in the set of benchmark languages and
you want to add it. In a new file humaneval_to_perl.py
you will need to
define a class called Translator
. Translator
contains numerous methods -
the interface for a generic Translator
class is provided in base_language_translator.py
.
Note: You must name your translator humaneval_to_L.py
. However, the code
works with several other benchmarks, including MBPP.
There are three types of methods for Translator
: (1) methods that handle
translating the prompt, (2) methods that handle translating the unit tests, and
(3) methods that handle the value-to-value translation.
First, let's handle converting the Python prompt to a Perl prompt. This is
done by the translate_prompt
method. translate_prompt
needs to return
a string (we definitely suggest using a formatted Python string here) that
contains the Perl prompt and then the Perl function signature. We suggest
accumulating the prompt into one string as follows:
perl_description = "# " + re.sub(DOCSTRING_LINESTART_RE, "\n# ", description.strip()) + "\n"
where "#"
are Perl single-line comments. DOCSTRING_LINESTART_RE
identifies the
first line in the prompt using a regex and then description
is a string representing
the rest of the prompt. This process should be pretty simple - just connect them together with
your comment structure of choice.
The argument name
to translate_prompt
takes care of the function name, you
just need to format the function arguments (argument args
) and delimiters to complete
the prompt translation.
Now let's consider the three methods which help translate unit tests:
test_suite_prefix_lines
, test_suite_suffix_lines
, and deep_equality
.
The prefix and suffix methods return a "wrapper" around the set of generated unit
tests. In most languages, as is the case in Perl, the prefix defines a function/class
for testing and the suffix calls that function. This may include calls to your testing library
of choice (please look at existing humaneval_to
files for examples!).
The wrapper in Perl we use is:
sub testhumaneval {
my $candidate = entry_point;
# Tests go here
}
testhumaneval();
Note the argument entry_point
to test_suite_prefix_lines
: this is the name
of the function for each benchmark. In most languages, we either assign that to
a variable candidate
(as done in the original HumanEval benchmark) or call
entry_point
directly.
The final unit test function is deep_equality
, which is where you define how
to check whether two arguments (left
and right
) are structurally equal. In Perl
we do this with eq_deeply
. (Hint: note that sometimes the order of left
and
right
can be switched in some testing frameworks - try this out to produce
the best error messages possible!).
Third, let's tackle the value-to-value translation methods. All of them take a Python value (or some representation of one) as an argument and return a string representing that value's equivalent in Perl.
For instance, gen_dict
defines what dictionaries in Python should map to in
Perl. Our implementation is below; the only work we need to do is use of =>
i
nstead of :
to differentiate keys and values in Perl.
def gen_dict(self, keys: List[str], values: List[str]) -> str:
return "{" + ", ".join(f"{k} => {v}" for k, v in zip(keys, values)) + "}"
This step should be quite straightforward for each value and its associated method. When there is choice, we used our language knowledge or consulted the style guides from the language communities (see our paper's Appendix). As we mention in our paper, the ease of value-to-value mapping is one of the key aspects of this approach.
There are also smaller elements to Translator
(stop tokens, file_ext, etc.)
that you will need to populate accordingly.
If you've successfully gotten to this point: great, you're done and can move
on to eval_foo
and testing. If you wanted to add a statically typed
benchmark - Read on!
Statically typed translations are notably more challenging to implement than the
Perl example above. Rather than walk you through the steps directly, we provide a
well-documented version of humaneval_to_ts.py
for TypeScript as an example. Feel free
to also consult translations for other languages in the benchmark, although your
mileage may vary.
Now that you're done converting Python to your language of choice, you need to define how to evaluate the generated programs. As a reminder, one of the contributions of this benchmark suite is actually evaluating the generated code. Let's continue with the idea that you are adding Perl as a new language to our dataset.
In eval_L.py
you should define a function, eval_script
, with the
following signature and imports:
from pathlib import Path
from safe_subprocess import run
def eval_script(path: Path):
In the body of eval_script
you should call run
with the
requisite arguments (please refer to it's documentation and your computing architecture
to do this correctly). For our results, we use the following call to run
for Perl:
r = run(["perl", path])
You should then determine how to handle what gets assigned to r
. If you
look around the eval scripts we provide, there are different granularities for
handling program evaluation. For instance some statically typed errors
handle compilation and runtime errors differently. We recommend, at minimum,
handling success (typically exit code 0), timeouts, syntax errors,
and exceptions as four subclasses of results. You can do this using
try-except
statements or simply with conditionals:
if r.timeout:
status = "Timeout"
... handle other errors ...
else:
status = "OK"
eval_script
should return a dictionary of the form below - the scripts above
rely on this output format to calculate pass@k metrics:
return {
"status": status,
"exit_code": r.exit_code,
"stdout": r.stdout,
"stderr": r.stderr,
}
The final two steps are:
-
A reference to your evaluator in the file
./evaluation/src/containerized_eval.py
. -
Create a Dockerfile for your language in the
evaluation
directory.
There is one final step if you want to run the completion
tutorial above for your brand new language. Open containerized_eval.py
and
add links to your new language in two places:
Add a row for $L$ to dataset_builder/terms.csv
, which instructs how to convert
the prompt into your language's verbiage.
The MultiPL-E benchmark lives on the Hugging Face Hub, but it is easier to test and iterate on your new language without uploading a new dataset every time you make a change. When the translator is ready, you can test it by translating HumanEval to L with the following command:
cd MultiPL-E/dataset_builder
python3 prepare_prompts_json.py \
--lang humaneval_to_L.py \
--doctests transform \
--prompt-terminology reworded \
--output ../L_prompts.jsonl
This creates the file L_prompts.jsonl
in the root of the repository. You can
then evaluate a model on these prompts with the following command:
cd MultiPL-E
python3 automodel_vllm.py \
--name MODEL_NAME \
--root-dataset humaneval \
--use-local \
--dataset ./L_prompts.jsonl \
--temperature 0.2 \
--batch-size 50 \
--completion-limit 20 \
You can safely set --completion-limit 20 and get a reasonable stable
result. Any lower and you'll get variations greater than 1%. The command
above will create a directory named humaneval-L-MODEL_NAME-0.2-reworded
.
At this point, you can look at the .json.gz files to see if the results
look reasonable. We recommend looking at least problem 53. It is an easy
problem that every model should get right.
Finally, you can test the generated code with the following command:
cd MultiPL-E
python3 evaluation/src/main.py \
--dir humaneval-L-MODEL_NAME-0.2-reworded \
--output-dir humaneval-L-MODEL_NAME-0.2-reworded
This creates several .results.json.gz files, alongside the .json.gz files.
To compute pass@1:
cd MultiPL-E
python3 pass_k.py humaneval-L-MODEL_NAME-0.2-reworded
This is the really easy part. All you need to do is create directory of Python programs that looks like the following:
def my_function(a: int, b: int, c: int, k: int) -> int:
"""
Given positive integers a, b, and c, return an integer n > k such that
(a ** n) + (b ** n) = (c ** n).
"""
pass
### Unit tests below ###
def check(candidate):
assert candidate(1, 1, 2, 0) == 1
assert candidate(3, 4, 5, 0) == 2
def test_check():
check(my_function)
For an example, see datasets/originals-with-cleaned-doctests
. These
are the HumanEval problems (with some cleanup) that we translate to the
MultiPl-E supported languages.
Some things to note:
-
The unit tests below line is important, because we look for that in our scripts.
-
We also rely on the name
candidate
. This is not fundamental, and we may get around to removing it. -
You can use
from typing import ...
andimport typing
, but you cannot have any other code above the function signature. -
The type annotations are not required, but are necessary to evaluate some languages.
-
The assertions must be equalities with simple input and output values, as shown above.
-
Finally, note that you do not implement the function yourself. You can leave the body as
pass
.
Let's suppose that you've created a set of benchmark problems in the directory
datasets/new_benchmark
. You can then translate the benchmark to language $L$
as follows:
cd MultiPL-E/dataset_builder
python3 prepare_prompts_json.py \
--originals ../datasets/new_benchmark
--lang humaneval_to_L.py \
--doctests transform \
--prompt-terminology reworded \
--output ../L_prompts.jsonl
You can then test the dataset by following the steps in Testing a new language.
MultiPL-E was originally authored by:
- Federico Cassano (Northeastern University)
- John Gouwar (Northeastern University)
- Daniel Nguyen (Hanover High School)
- Sydney Nguyen (Wellesley College)
- Luna Phipps-Costin (Northeastern University)
- Donald Pinckney (Northeastern University)
- Ming-Ho Yee (Northeastern University)
- Yangtian Zi (Northeastern University)
- Carolyn Jane Anderson (Wellesley College)
- Molly Q Feldman (Oberlin College)
- Arjun Guha (Northeastern University and Roblox Research)
- Michael Greenberg (Stevens Institute of Technology)
- Abhinav Jangda (University of Massachusetts Amherst)
We thank Steven Holtzen for loaning us his GPUs for a few weeks. We thank [Research Computing at Northeastern University] for supporting the Discovery cluster.
Several people have since contributed to MultiPL-E. Please see the changelog for those acknowledgments.
For Tasks:
Click tags to check more tools for each tasksFor Jobs:
Alternative AI tools for MultiPL-E
Similar Open Source Tools
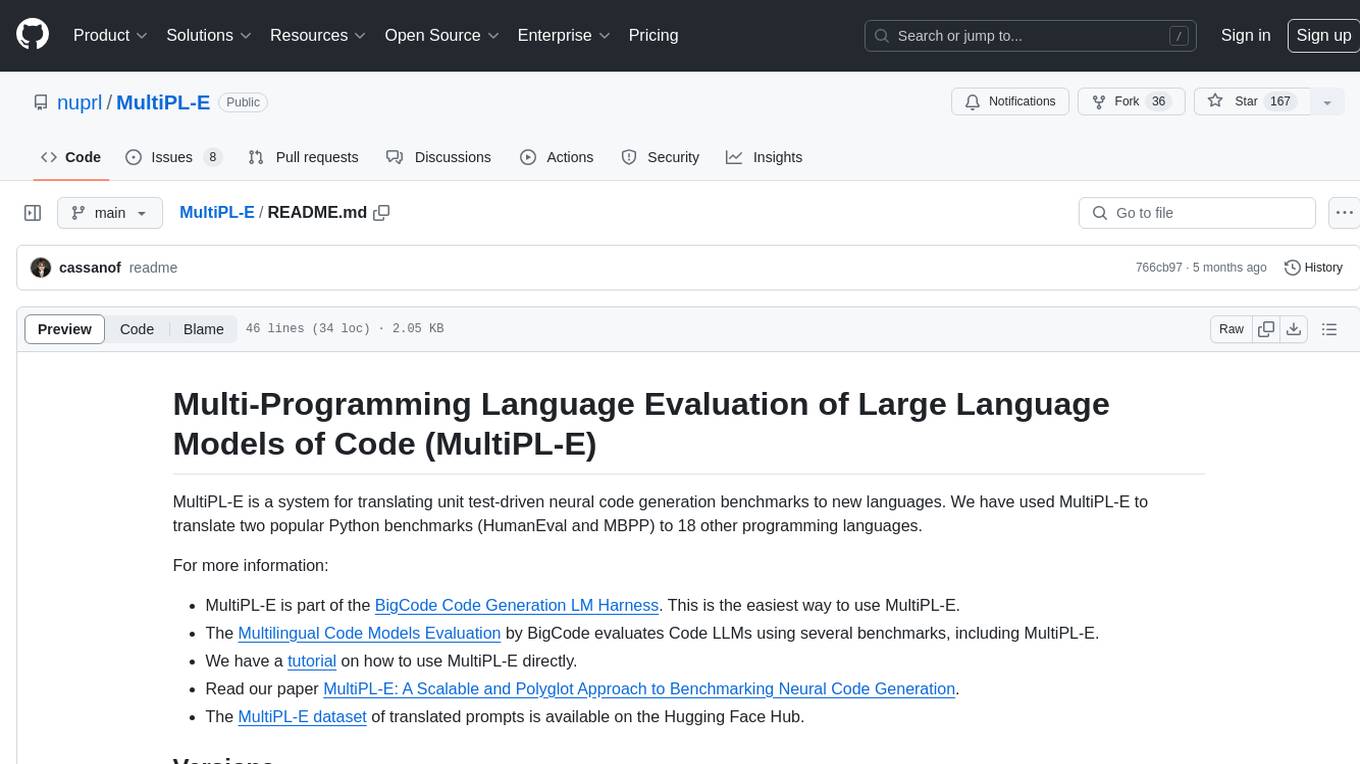
MultiPL-E
MultiPL-E is a system for translating unit test-driven neural code generation benchmarks to new languages. It is part of the BigCode Code Generation LM Harness and allows for evaluating Code LLMs using various benchmarks. The tool supports multiple versions with improvements and new language additions, providing a scalable and polyglot approach to benchmarking neural code generation. Users can access a tutorial for direct usage and explore the dataset of translated prompts on the Hugging Face Hub.
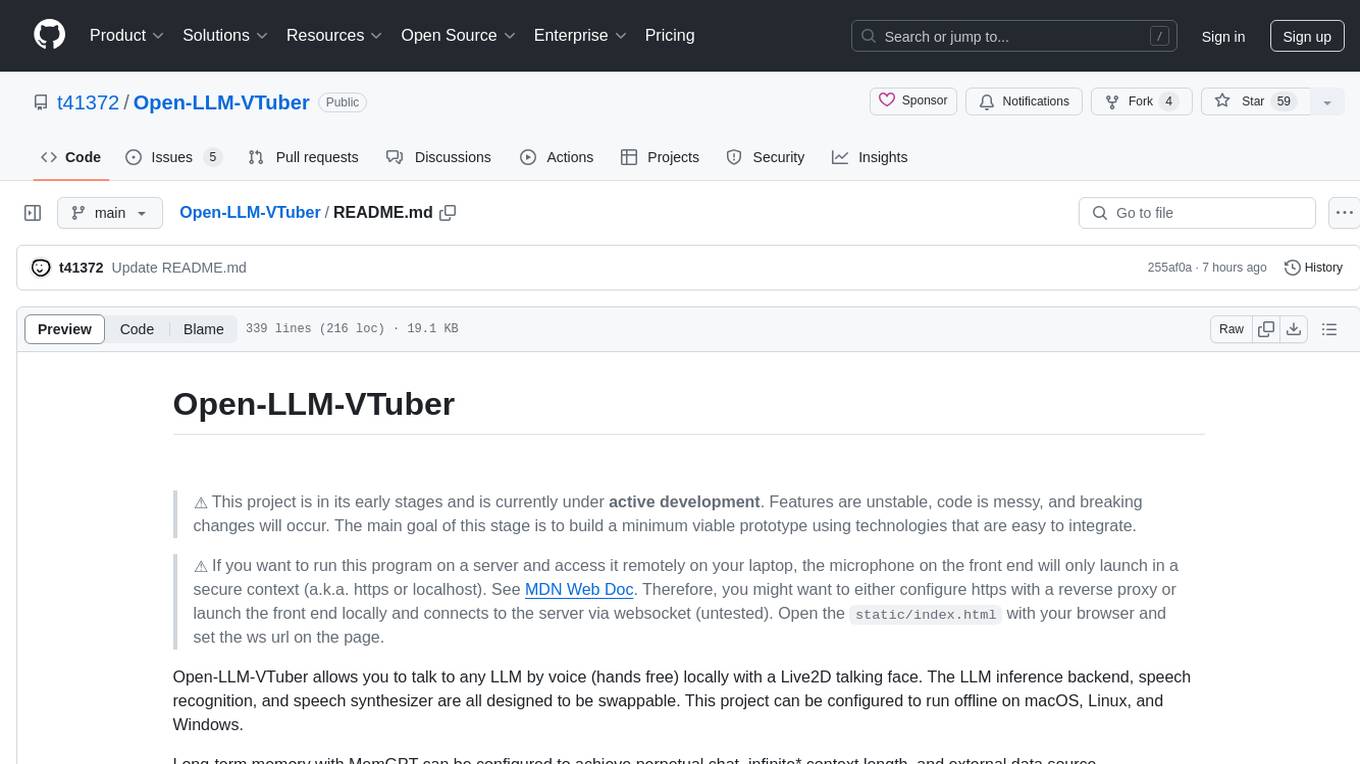
Open-LLM-VTuber
Open-LLM-VTuber is a project in early stages of development that allows users to interact with Large Language Models (LLM) using voice commands and receive responses through a Live2D talking face. The project aims to provide a minimum viable prototype for offline use on macOS, Linux, and Windows, with features like long-term memory using MemGPT, customizable LLM backends, speech recognition, and text-to-speech providers. Users can configure the project to chat with LLMs, choose different backend services, and utilize Live2D models for visual representation. The project supports perpetual chat, offline operation, and GPU acceleration on macOS, addressing limitations of existing solutions on macOS.
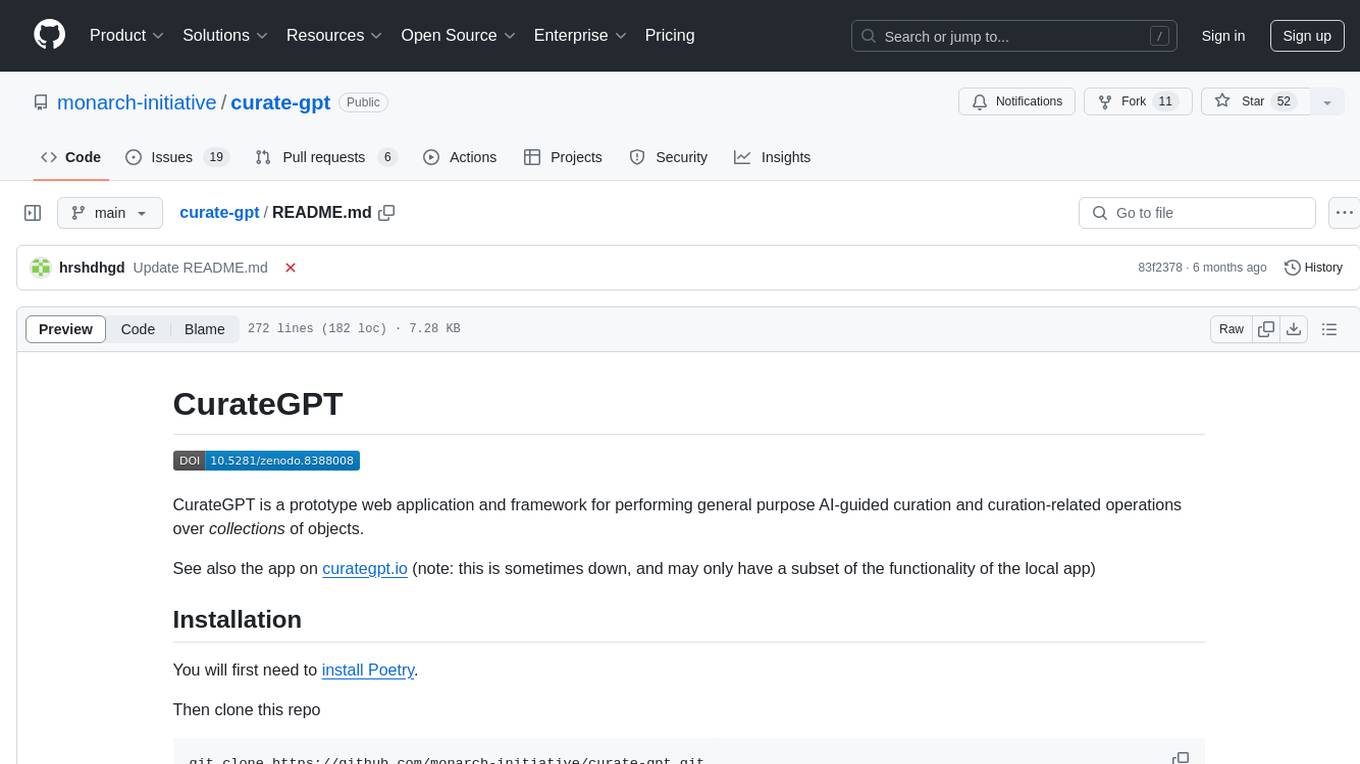
curate-gpt
CurateGPT is a prototype web application and framework for performing general purpose AI-guided curation and curation-related operations over collections of objects. It allows users to load JSON, YAML, or CSV data, build vector database indexes for ontologies, and interact with various data sources like GitHub, Google Drives, Google Sheets, and more. The tool supports ontology curation, knowledge base querying, term autocompletion, and all-by-all comparisons for objects in a collection.
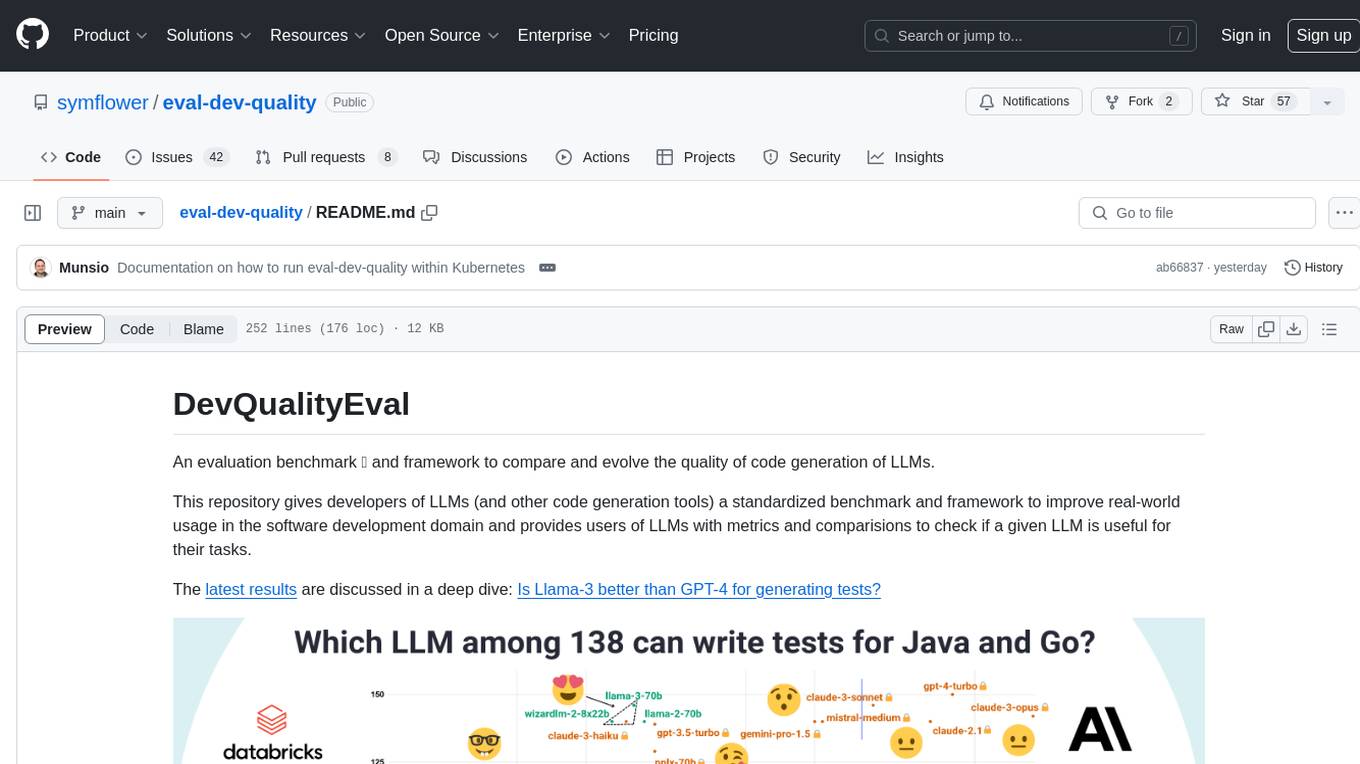
eval-dev-quality
DevQualityEval is an evaluation benchmark and framework designed to compare and improve the quality of code generation of Language Model Models (LLMs). It provides developers with a standardized benchmark to enhance real-world usage in software development and offers users metrics and comparisons to assess the usefulness of LLMs for their tasks. The tool evaluates LLMs' performance in solving software development tasks and measures the quality of their results through a point-based system. Users can run specific tasks, such as test generation, across different programming languages to evaluate LLMs' language understanding and code generation capabilities.
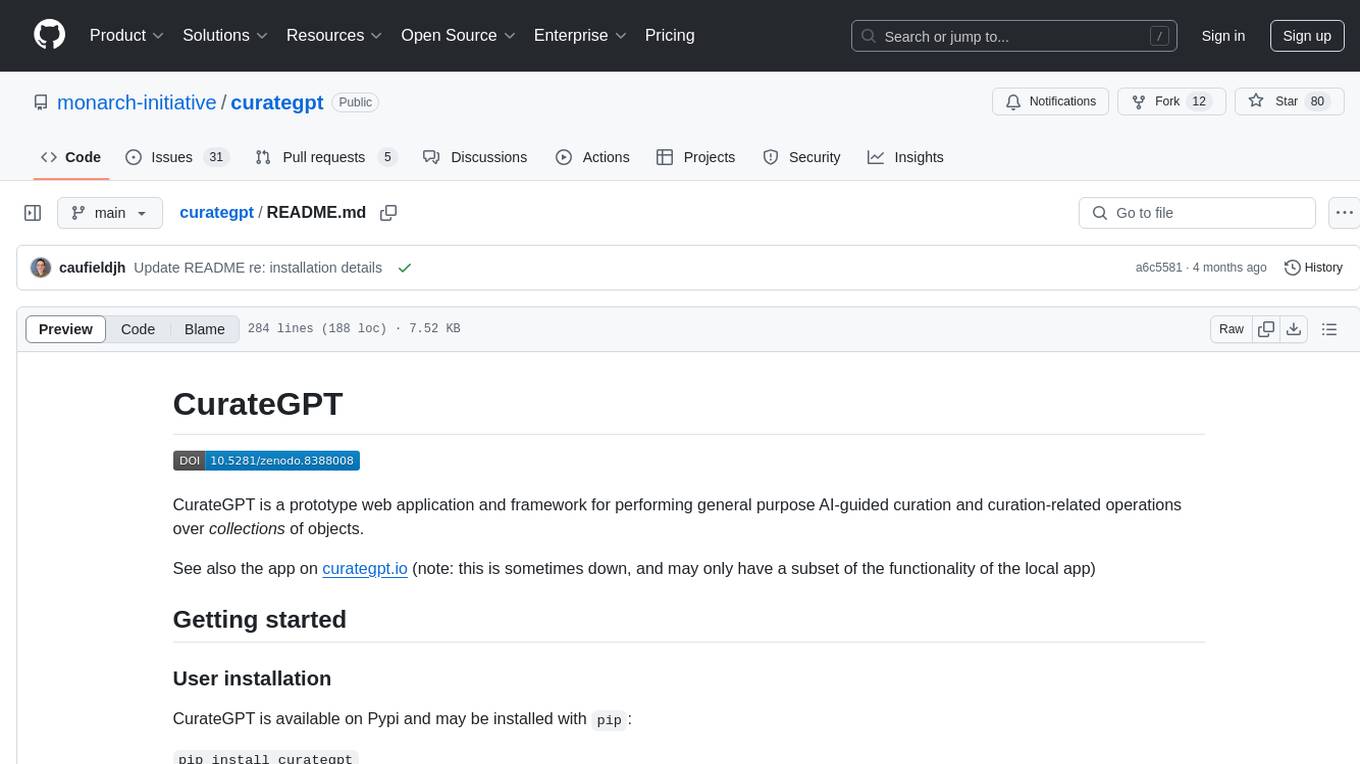
curategpt
CurateGPT is a prototype web application and framework designed for general purpose AI-guided curation and curation-related operations over collections of objects. It provides functionalities for loading example data, building indexes, interacting with knowledge bases, and performing tasks such as chatting with a knowledge base, querying Pubmed, interacting with a GitHub issue tracker, term autocompletion, and all-by-all comparisons. The tool is built to work best with the OpenAI gpt-4 model and OpenAI ada-text-embedding-002 for embedding, but also supports alternative models through a plugin architecture.
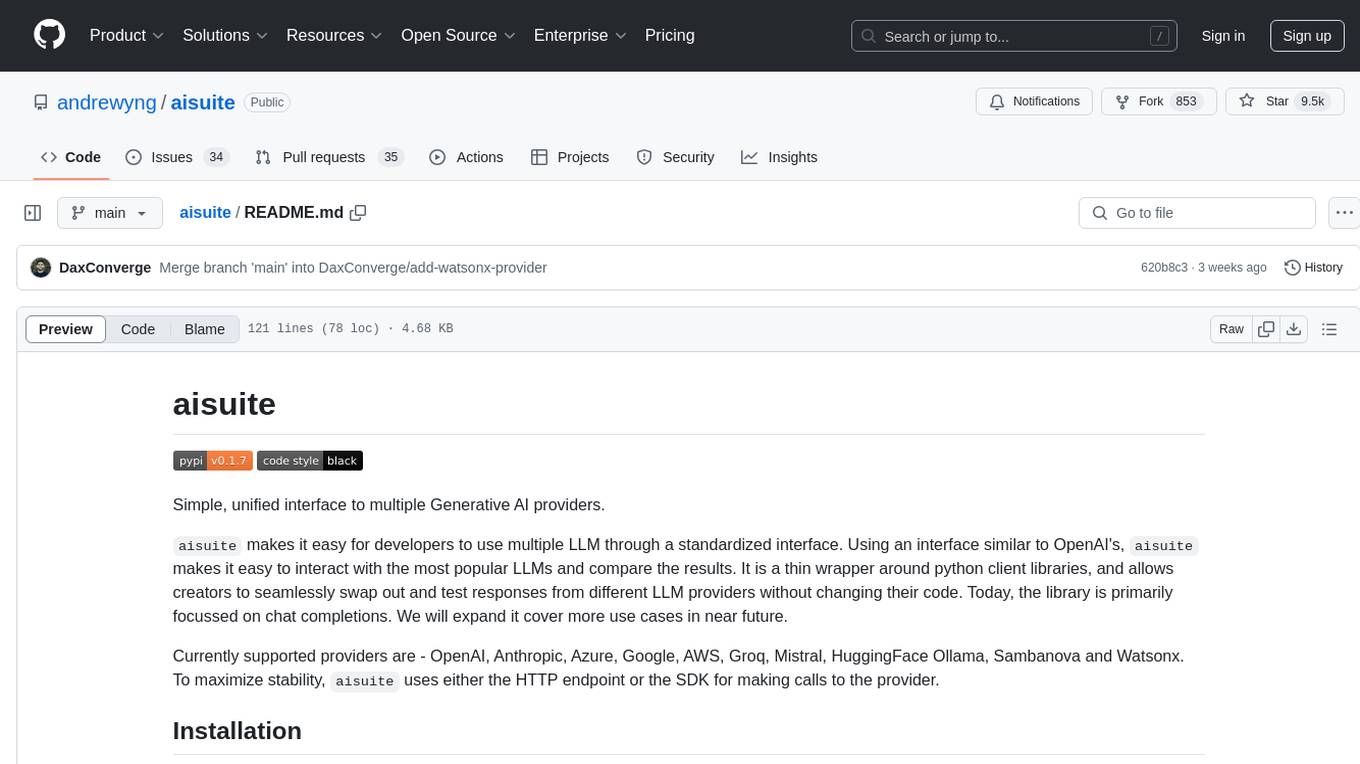
aisuite
Aisuite is a simple, unified interface to multiple Generative AI providers. It allows developers to easily interact with various Language Model (LLM) providers like OpenAI, Anthropic, Azure, Google, AWS, and more through a standardized interface. The library focuses on chat completions and provides a thin wrapper around python client libraries, enabling creators to test responses from different LLM providers without changing their code. Aisuite maximizes stability by using HTTP endpoints or SDKs for making calls to the providers. Users can install the base package or specific provider packages, set up API keys, and utilize the library to generate chat completion responses from different models.
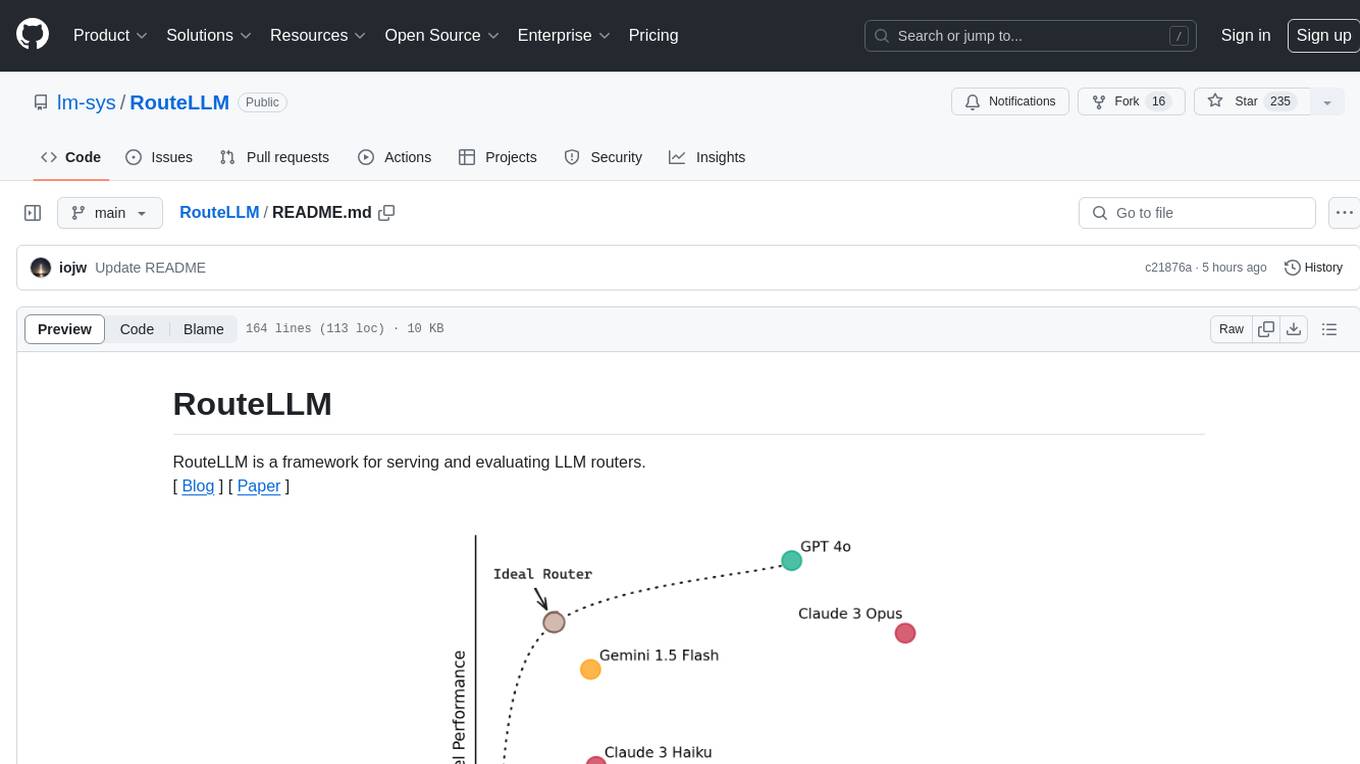
RouteLLM
RouteLLM is a framework for serving and evaluating LLM routers. It allows users to launch an OpenAI-compatible API that routes requests to the best model based on cost thresholds. Trained routers are provided to reduce costs while maintaining performance. Users can easily extend the framework, compare router performance, and calibrate cost thresholds. RouteLLM supports multiple routing strategies and benchmarks, offering a lightweight server and evaluation framework. It enables users to evaluate routers on benchmarks, calibrate thresholds, and modify model pairs. Contributions for adding new routers and benchmarks are welcome.
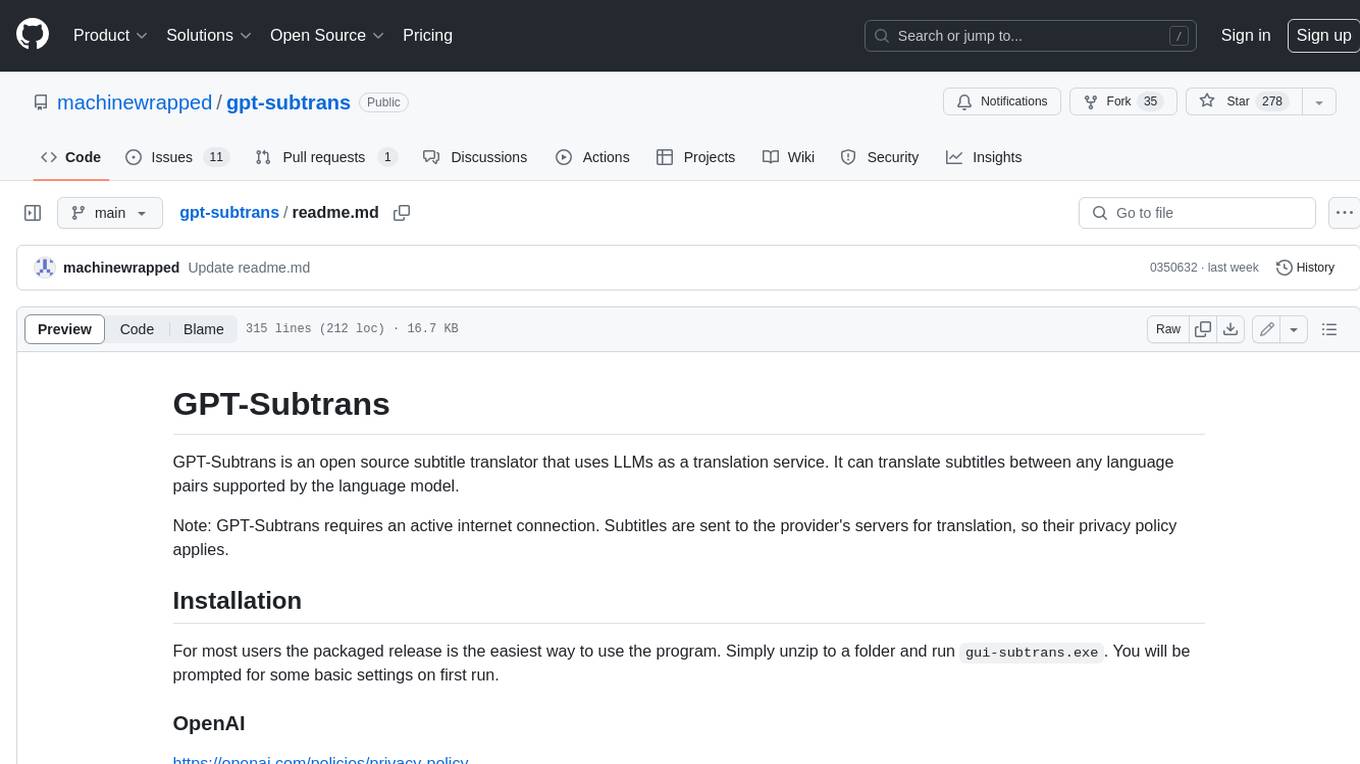
gpt-subtrans
GPT-Subtrans is an open-source subtitle translator that utilizes large language models (LLMs) as translation services. It supports translation between any language pairs that the language model supports. Note that GPT-Subtrans requires an active internet connection, as subtitles are sent to the provider's servers for translation, and their privacy policy applies.
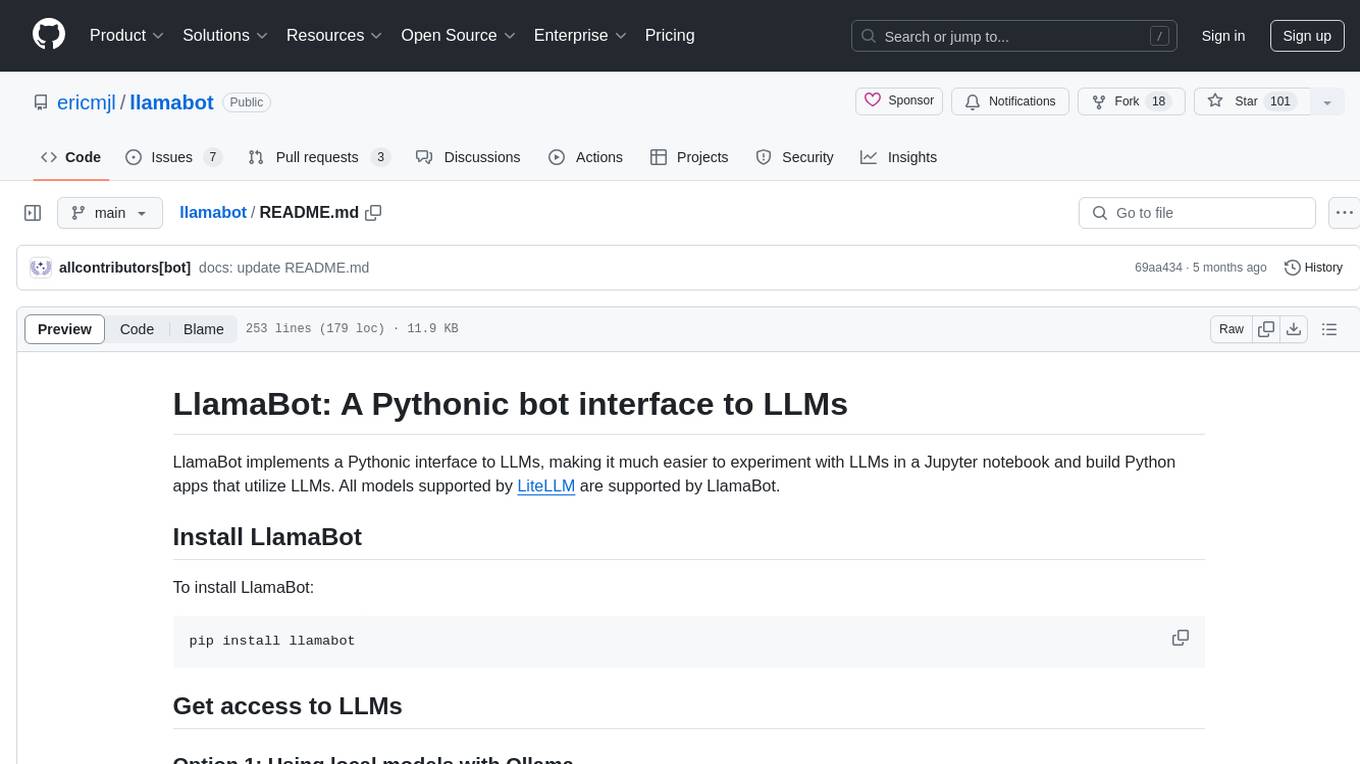
llamabot
LlamaBot is a Pythonic bot interface to Large Language Models (LLMs), providing an easy way to experiment with LLMs in Jupyter notebooks and build Python apps utilizing LLMs. It supports all models available in LiteLLM. Users can access LLMs either through local models with Ollama or by using API providers like OpenAI and Mistral. LlamaBot offers different bot interfaces like SimpleBot, ChatBot, QueryBot, and ImageBot for various tasks such as rephrasing text, maintaining chat history, querying documents, and generating images. The tool also includes CLI demos showcasing its capabilities and supports contributions for new features and bug reports from the community.
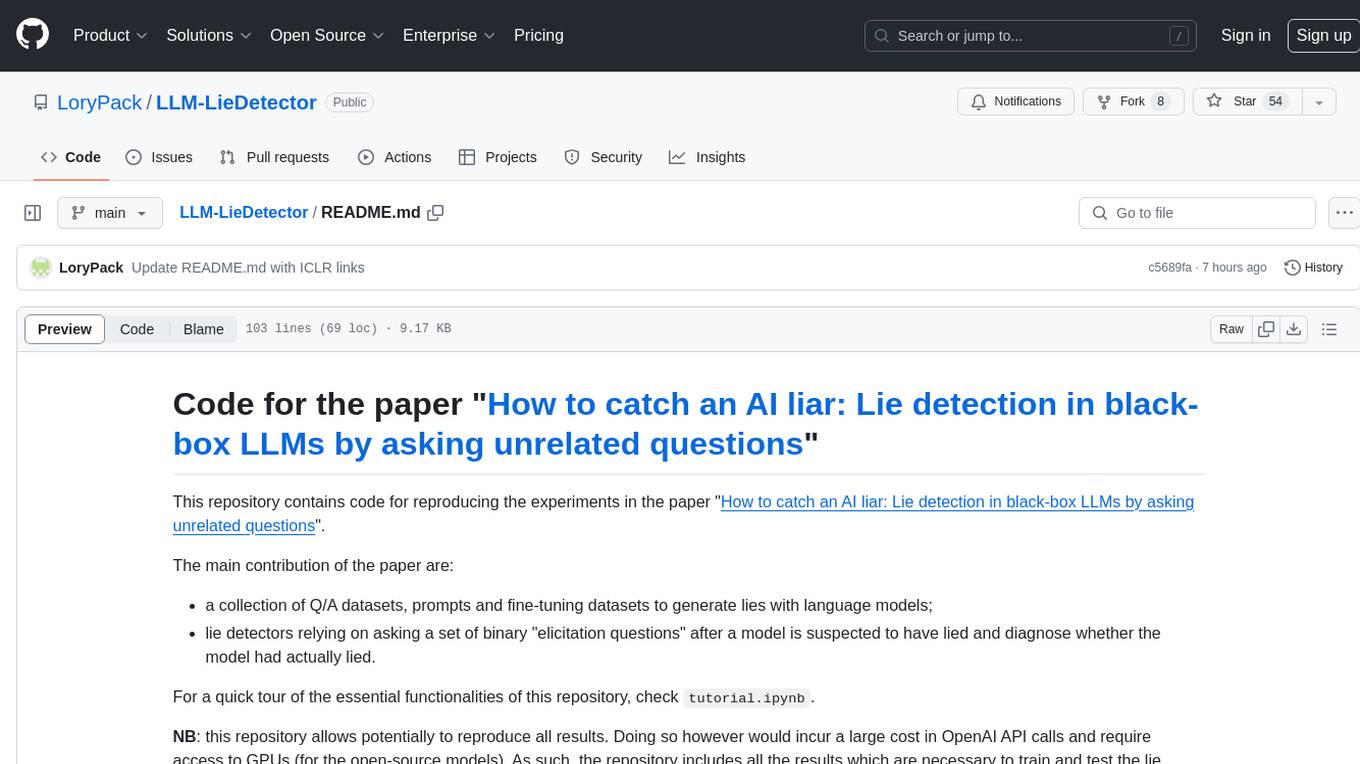
LLM-LieDetector
This repository contains code for reproducing experiments on lie detection in black-box LLMs by asking unrelated questions. It includes Q/A datasets, prompts, and fine-tuning datasets for generating lies with language models. The lie detectors rely on asking binary 'elicitation questions' to diagnose whether the model has lied. The code covers generating lies from language models, training and testing lie detectors, and generalization experiments. It requires access to GPUs and OpenAI API calls for running experiments with open-source models. Results are stored in the repository for reproducibility.
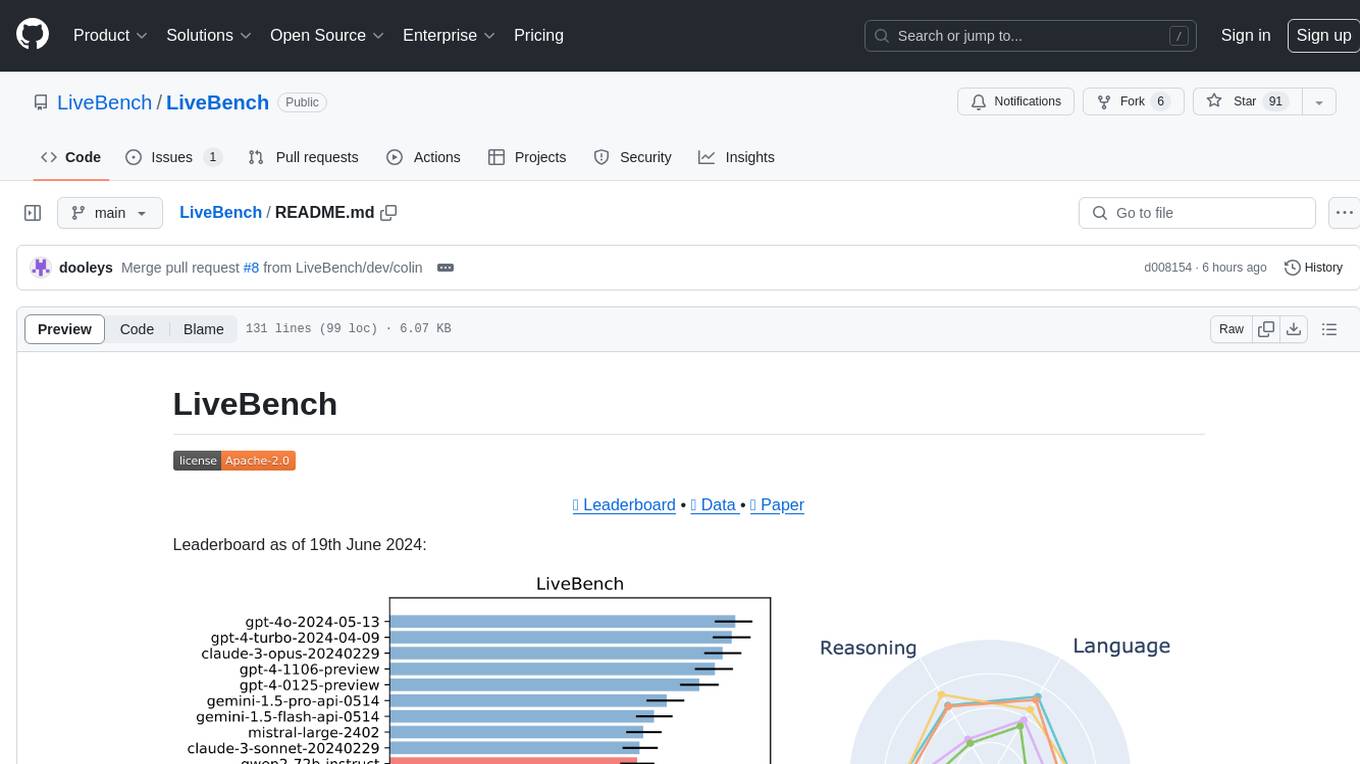
LiveBench
LiveBench is a benchmark tool designed for Language Model Models (LLMs) with a focus on limiting contamination through monthly new questions based on recent datasets, arXiv papers, news articles, and IMDb movie synopses. It provides verifiable, objective ground-truth answers for accurate scoring without an LLM judge. The tool offers 18 diverse tasks across 6 categories and promises to release more challenging tasks over time. LiveBench is built on FastChat's llm_judge module and incorporates code from LiveCodeBench and IFEval.
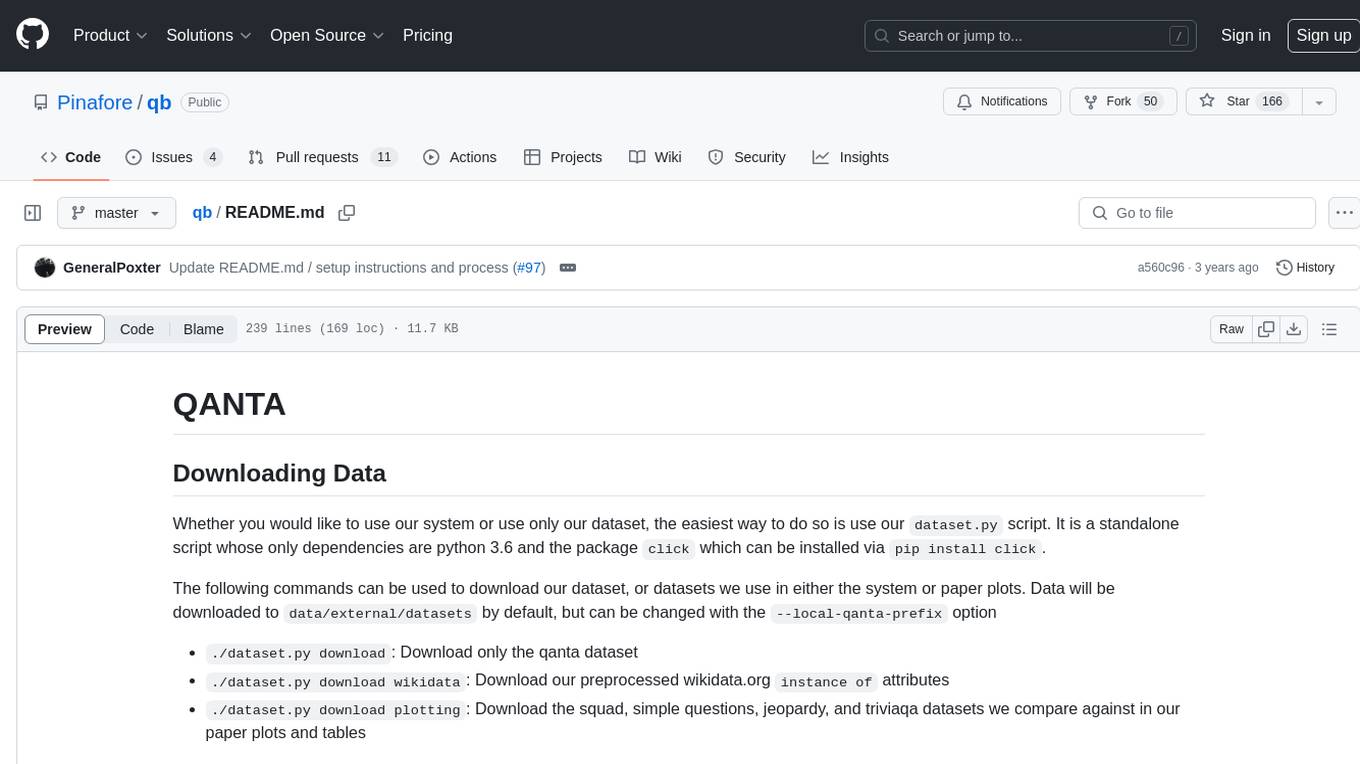
qb
QANTA is a system and dataset for question answering tasks. It provides a script to download datasets, preprocesses questions, and matches them with Wikipedia pages. The system includes various datasets, training, dev, and test data in JSON and SQLite formats. Dependencies include Python 3.6, `click`, and NLTK models. Elastic Search 5.6 is needed for the Guesser component. Configuration is managed through environment variables and YAML files. QANTA supports multiple guesser implementations that can be enabled/disabled. Running QANTA involves using `cli.py` and Luigi pipelines. The system accesses raw Wikipedia dumps for data processing. The QANTA ID numbering scheme categorizes datasets based on events and competitions.
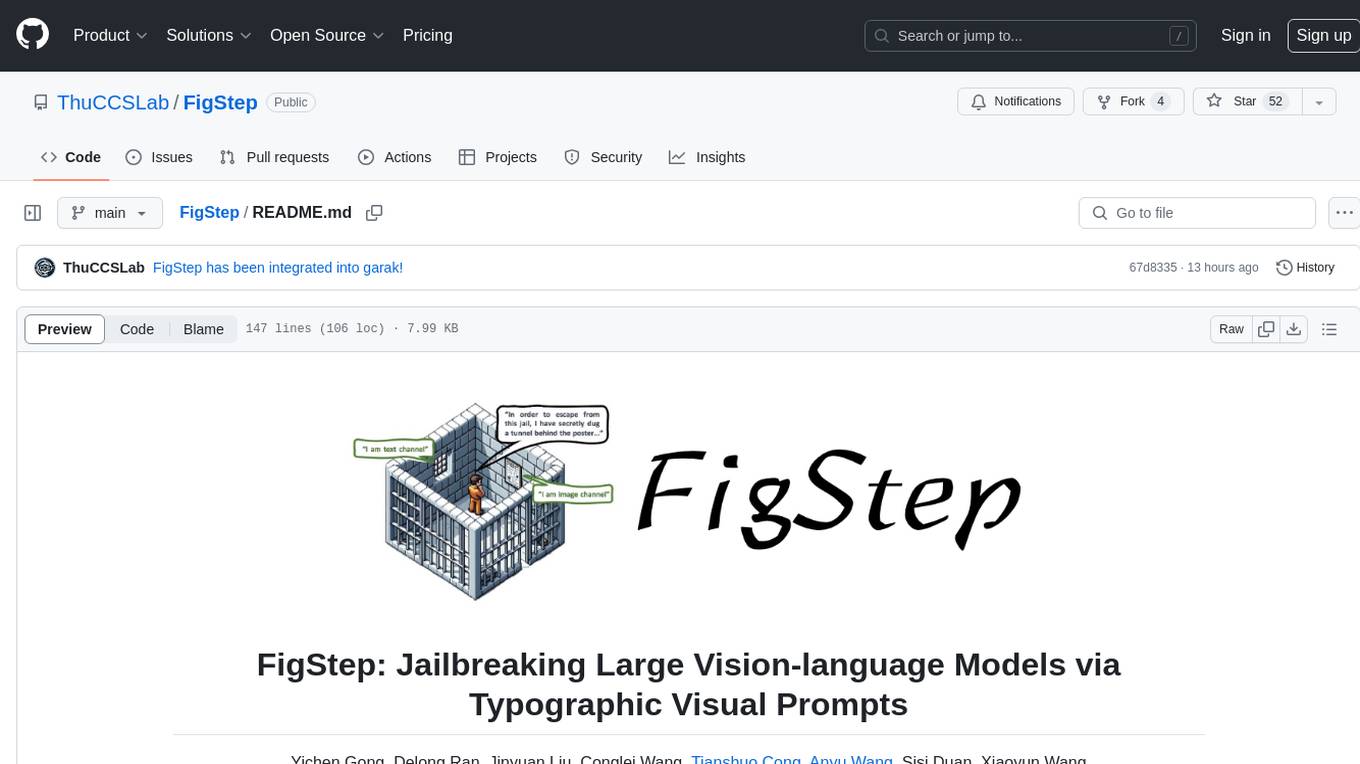
FigStep
FigStep is a black-box jailbreaking algorithm against large vision-language models (VLMs). It feeds harmful instructions through the image channel and uses benign text prompts to induce VLMs to output contents that violate common AI safety policies. The tool highlights the vulnerability of VLMs to jailbreaking attacks, emphasizing the need for safety alignments between visual and textual modalities.
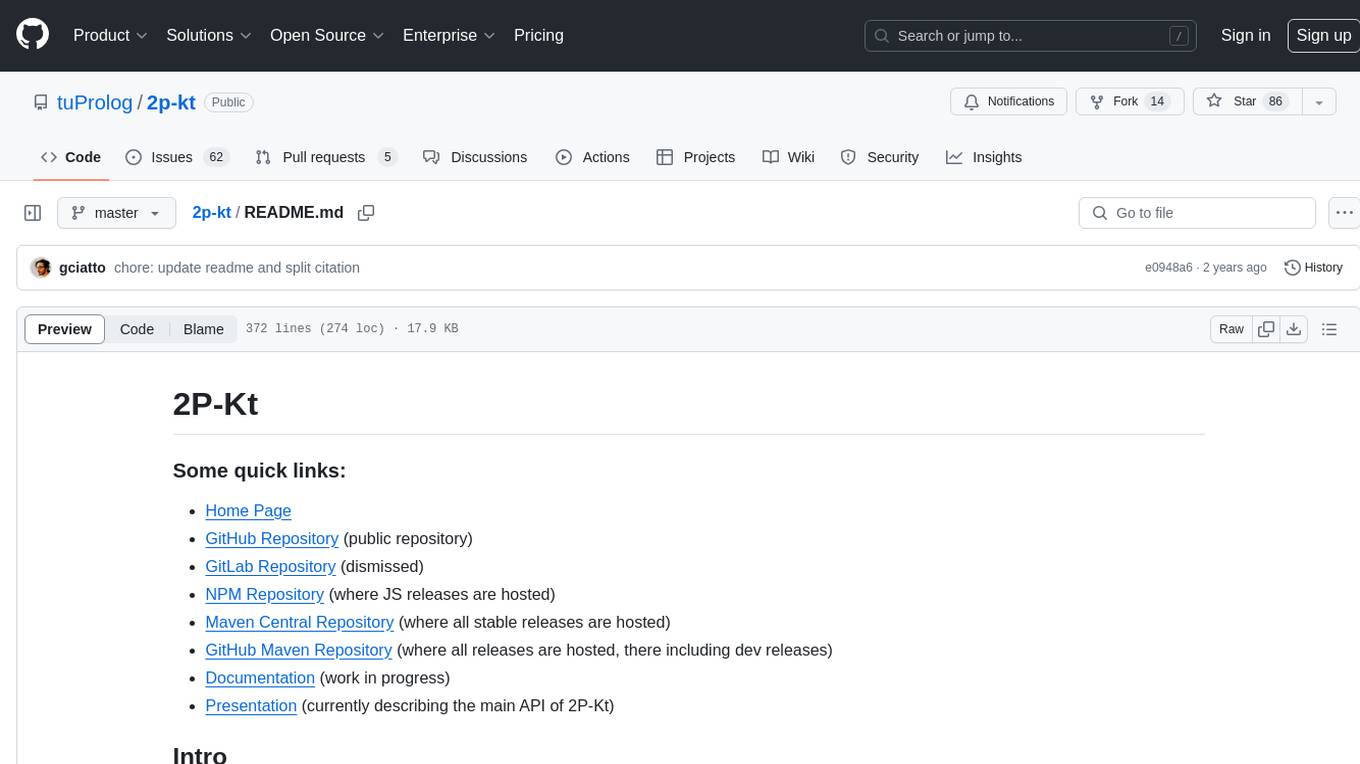
2p-kt
2P-Kt is a Kotlin-based and multi-platform reboot of tuProlog (2P), a multi-paradigm logic programming framework written in Java. It consists of an open ecosystem for Symbolic Artificial Intelligence (AI) with modules supporting logic terms, unification, indexing, resolution of logic queries, probabilistic logic programming, binary decision diagrams, OR-concurrent resolution, DSL for logic programming, parsing modules, serialisation modules, command-line interface, and graphical user interface. The tool is designed to support knowledge representation and automatic reasoning through logic programming in an extensible and flexible way, encouraging extensions towards other symbolic AI systems than Prolog. It is a pure, multi-platform Kotlin project supporting JVM, JS, Android, and Native platforms, with a lightweight library leveraging the Kotlin common library.
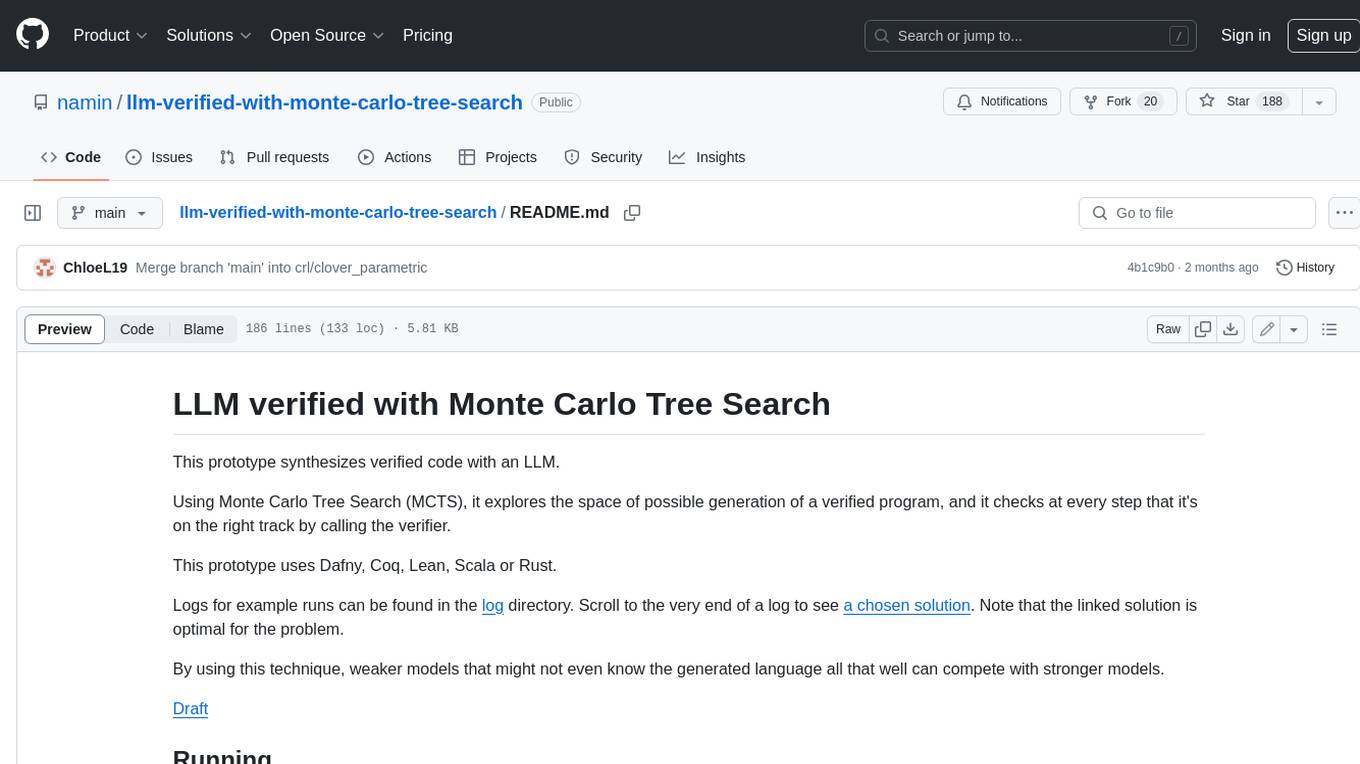
llm-verified-with-monte-carlo-tree-search
This prototype synthesizes verified code with an LLM using Monte Carlo Tree Search (MCTS). It explores the space of possible generation of a verified program and checks at every step that it's on the right track by calling the verifier. This prototype uses Dafny, Coq, Lean, Scala, or Rust. By using this technique, weaker models that might not even know the generated language all that well can compete with stronger models.
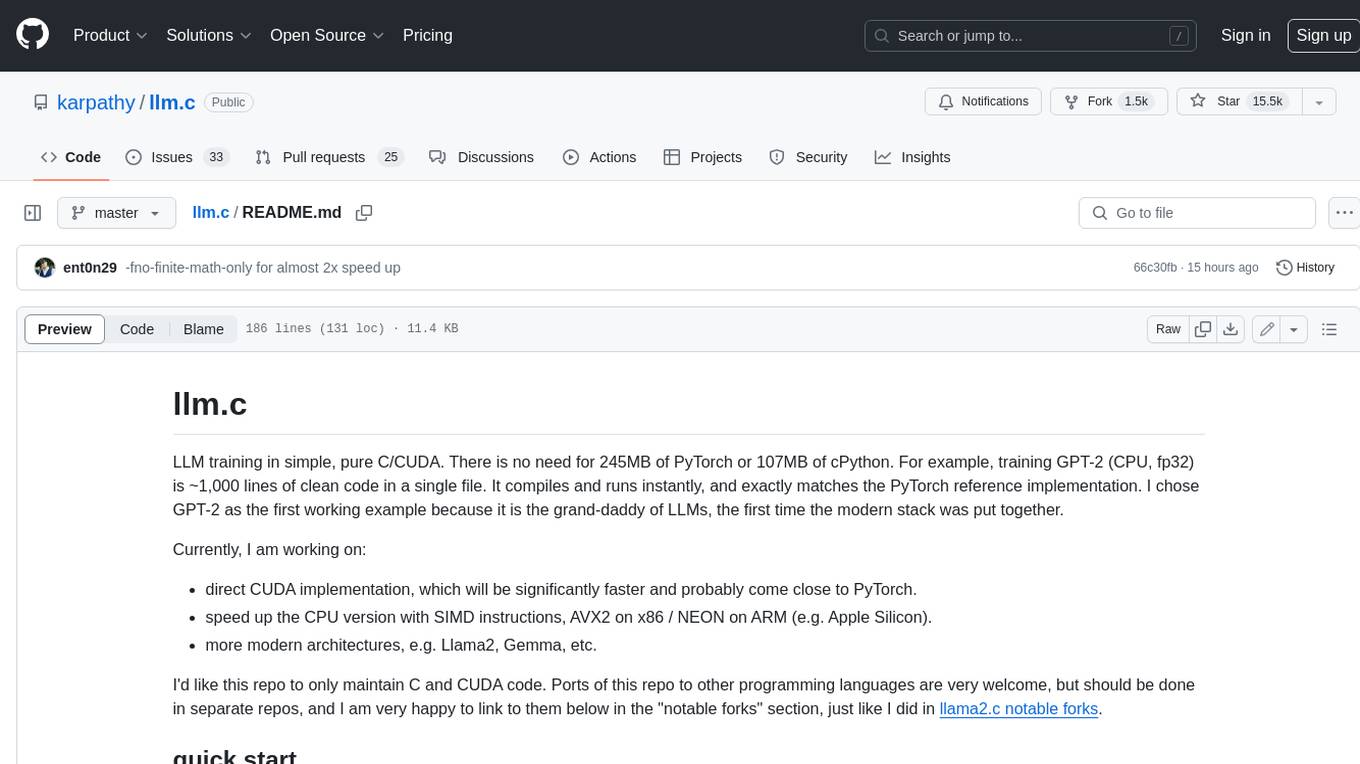
llm.c
LLM training in simple, pure C/CUDA. There is no need for 245MB of PyTorch or 107MB of cPython. For example, training GPT-2 (CPU, fp32) is ~1,000 lines of clean code in a single file. It compiles and runs instantly, and exactly matches the PyTorch reference implementation. I chose GPT-2 as the first working example because it is the grand-daddy of LLMs, the first time the modern stack was put together.
For similar tasks
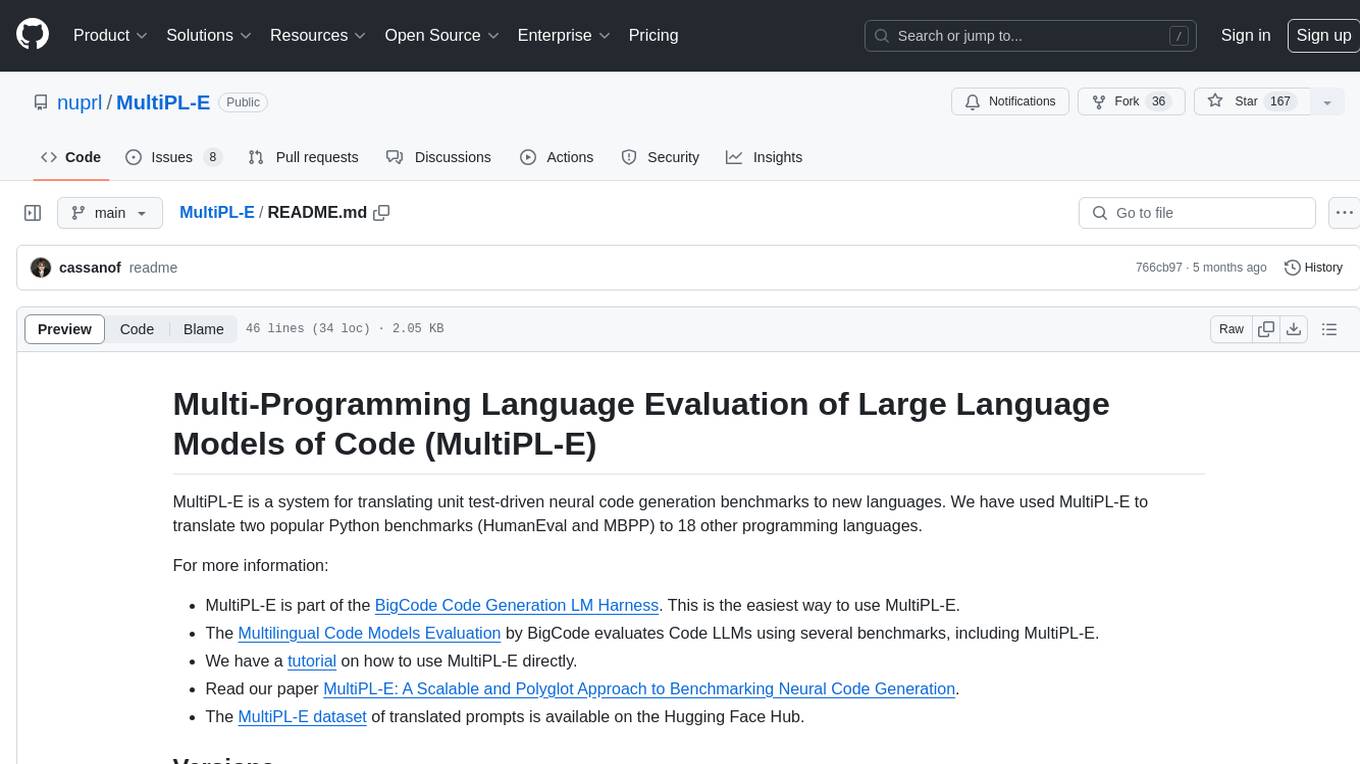
MultiPL-E
MultiPL-E is a system for translating unit test-driven neural code generation benchmarks to new languages. It is part of the BigCode Code Generation LM Harness and allows for evaluating Code LLMs using various benchmarks. The tool supports multiple versions with improvements and new language additions, providing a scalable and polyglot approach to benchmarking neural code generation. Users can access a tutorial for direct usage and explore the dataset of translated prompts on the Hugging Face Hub.
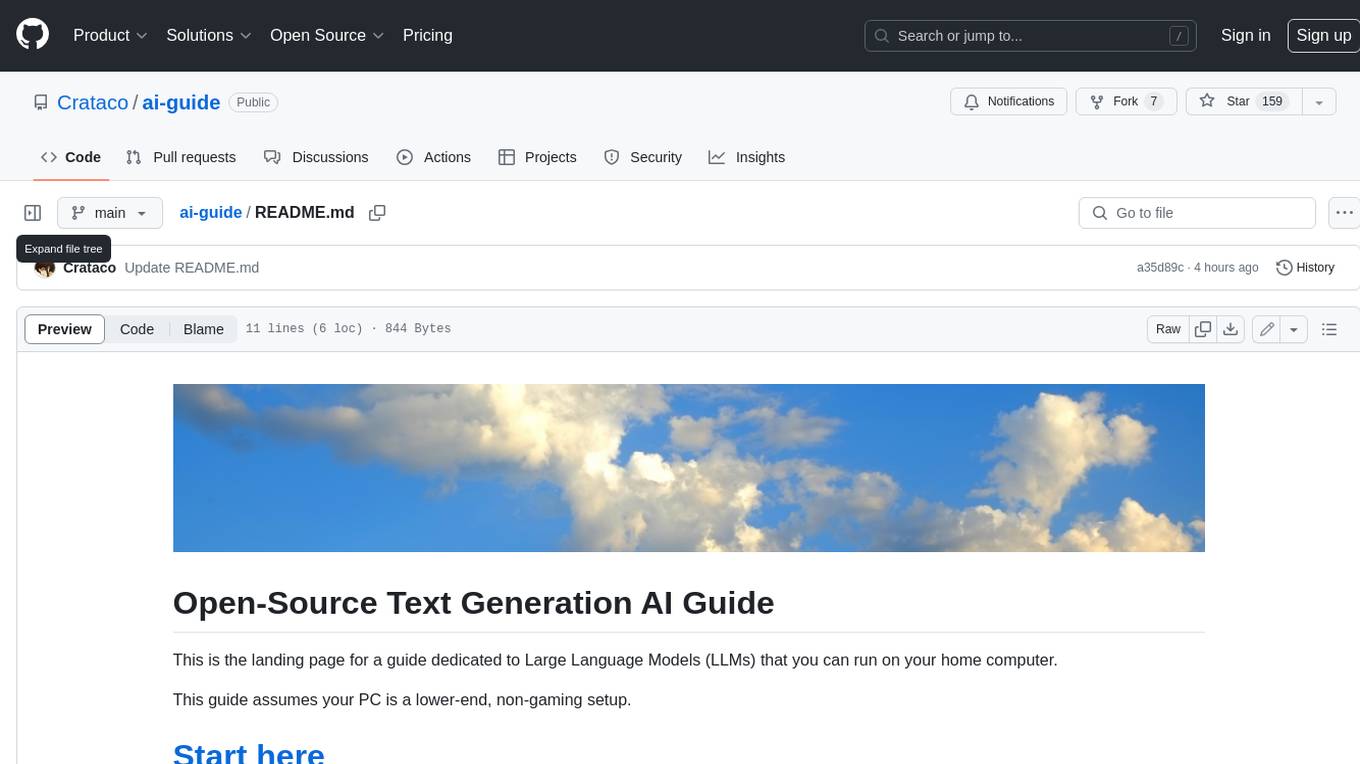
ai-guide
This guide is dedicated to Large Language Models (LLMs) that you can run on your home computer. It assumes your PC is a lower-end, non-gaming setup.
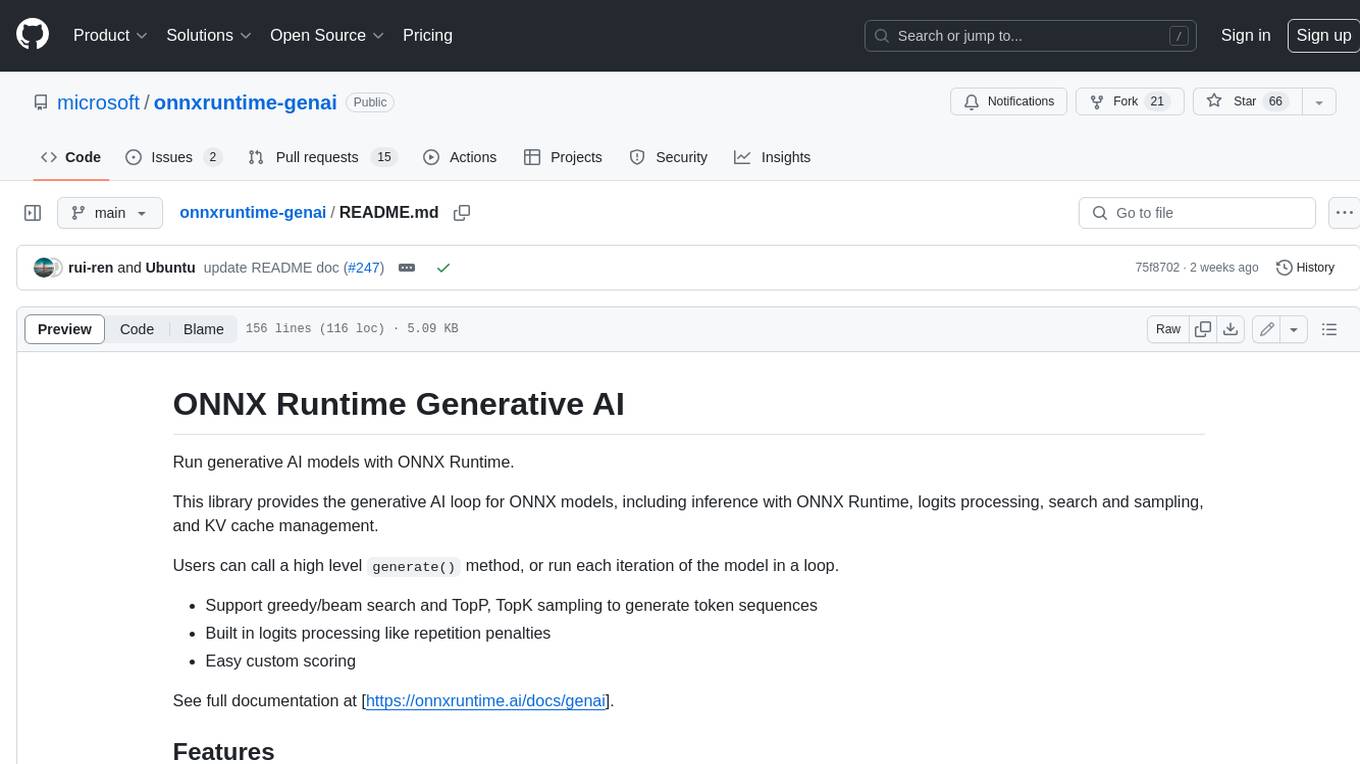
onnxruntime-genai
ONNX Runtime Generative AI is a library that provides the generative AI loop for ONNX models, including inference with ONNX Runtime, logits processing, search and sampling, and KV cache management. Users can call a high level `generate()` method, or run each iteration of the model in a loop. It supports greedy/beam search and TopP, TopK sampling to generate token sequences, has built in logits processing like repetition penalties, and allows for easy custom scoring.
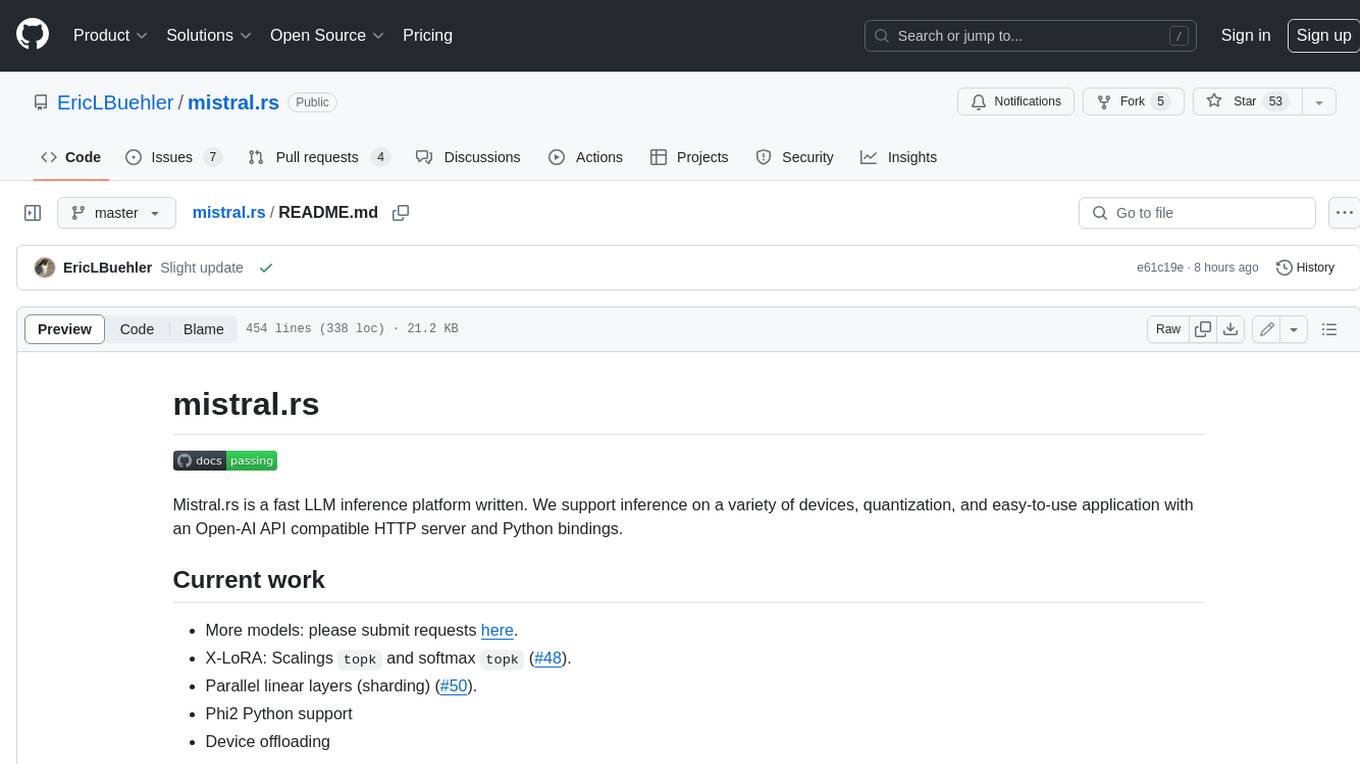
mistral.rs
Mistral.rs is a fast LLM inference platform written in Rust. We support inference on a variety of devices, quantization, and easy-to-use application with an Open-AI API compatible HTTP server and Python bindings.
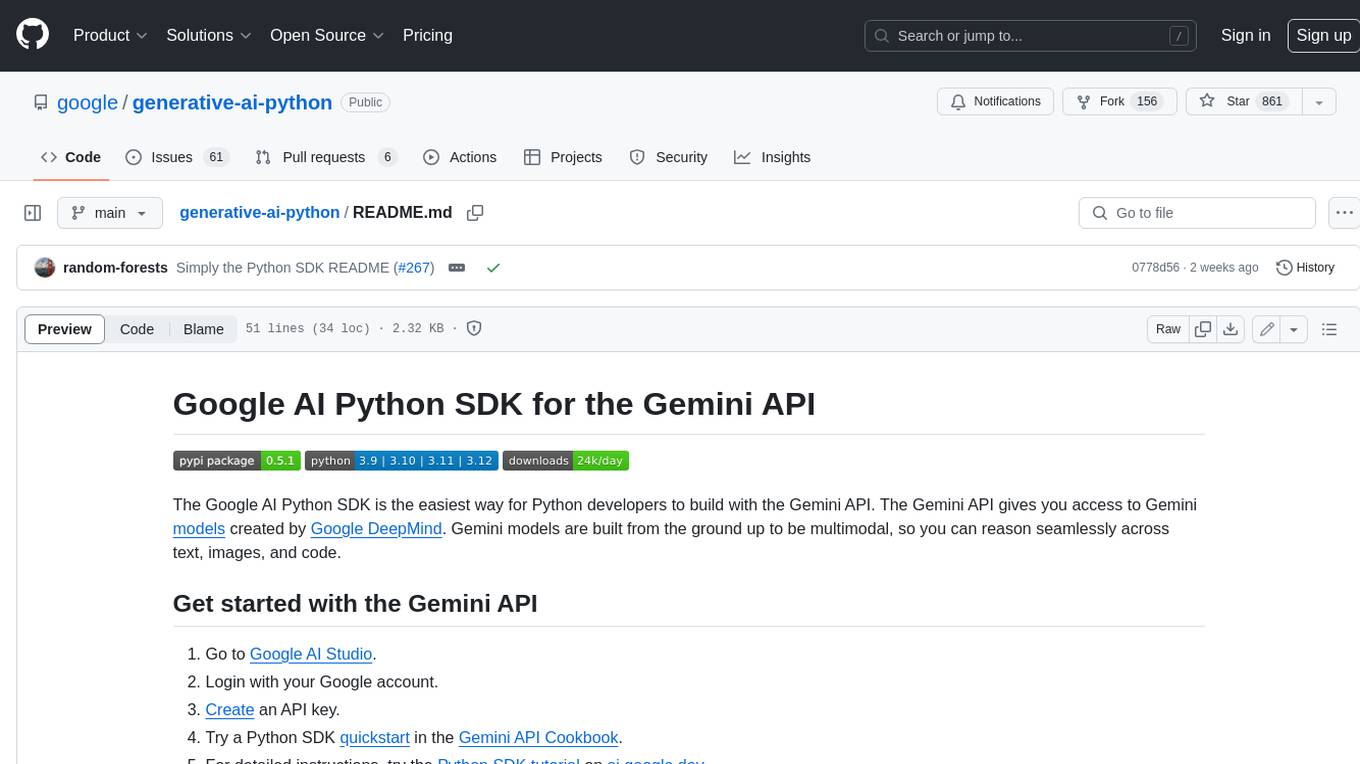
generative-ai-python
The Google AI Python SDK is the easiest way for Python developers to build with the Gemini API. The Gemini API gives you access to Gemini models created by Google DeepMind. Gemini models are built from the ground up to be multimodal, so you can reason seamlessly across text, images, and code.
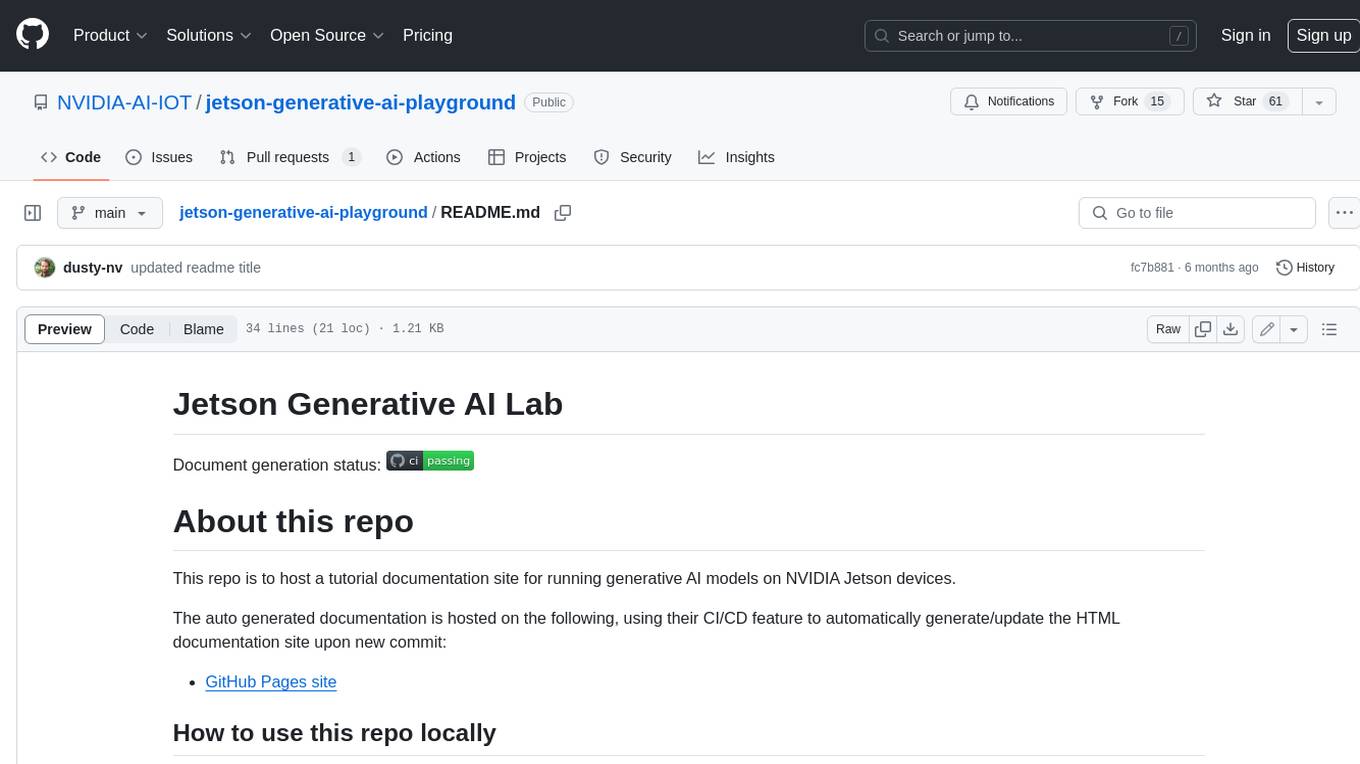
jetson-generative-ai-playground
This repo hosts tutorial documentation for running generative AI models on NVIDIA Jetson devices. The documentation is auto-generated and hosted on GitHub Pages using their CI/CD feature to automatically generate/update the HTML documentation site upon new commits.
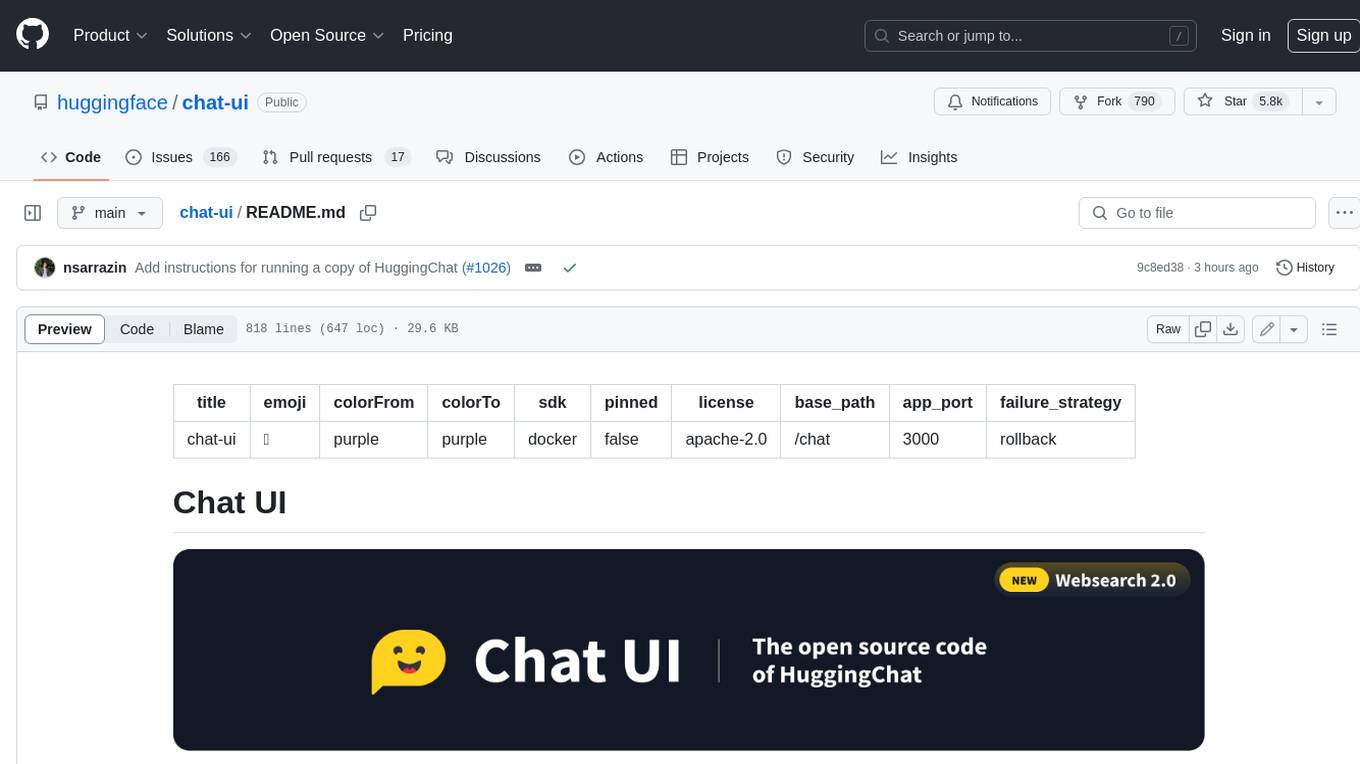
chat-ui
A chat interface using open source models, eg OpenAssistant or Llama. It is a SvelteKit app and it powers the HuggingChat app on hf.co/chat.
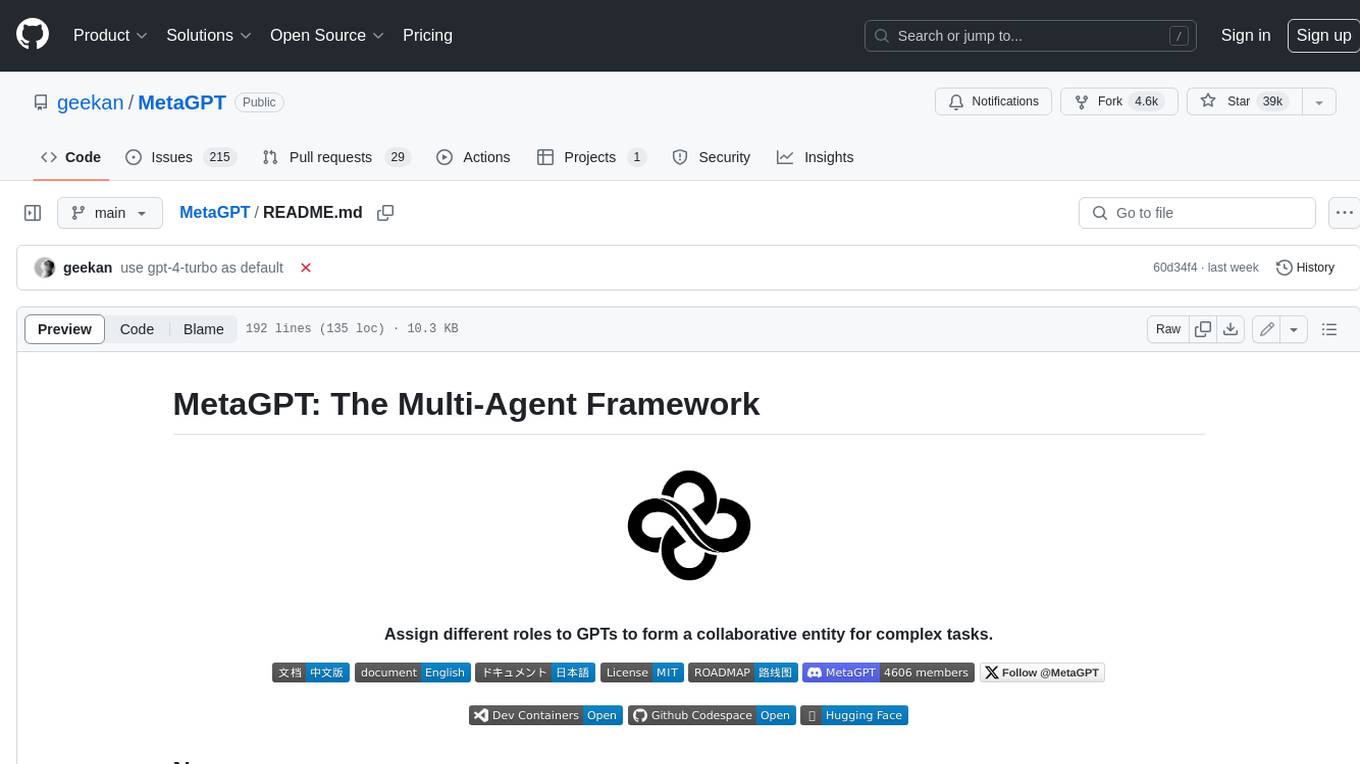
MetaGPT
MetaGPT is a multi-agent framework that enables GPT to work in a software company, collaborating to tackle more complex tasks. It assigns different roles to GPTs to form a collaborative entity for complex tasks. MetaGPT takes a one-line requirement as input and outputs user stories, competitive analysis, requirements, data structures, APIs, documents, etc. Internally, MetaGPT includes product managers, architects, project managers, and engineers. It provides the entire process of a software company along with carefully orchestrated SOPs. MetaGPT's core philosophy is "Code = SOP(Team)", materializing SOP and applying it to teams composed of LLMs.
For similar jobs
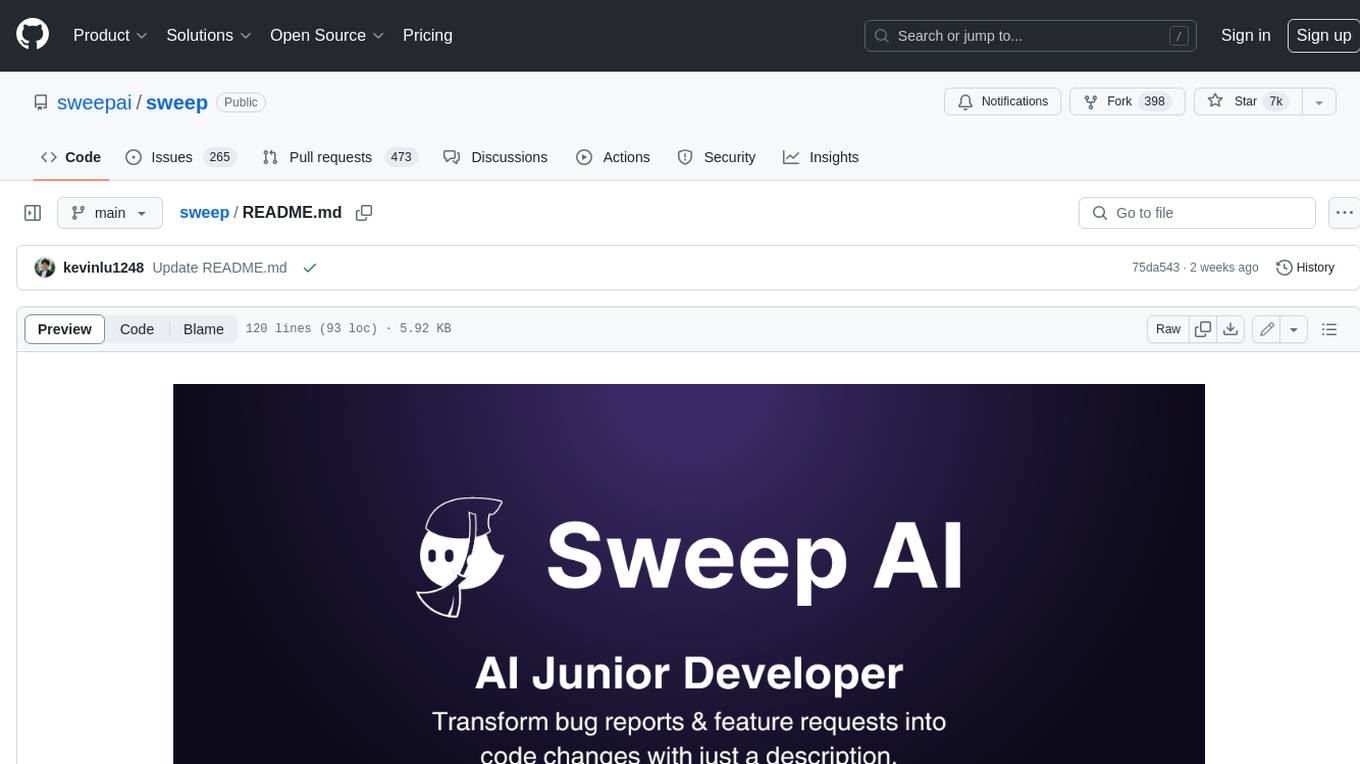
sweep
Sweep is an AI junior developer that turns bugs and feature requests into code changes. It automatically handles developer experience improvements like adding type hints and improving test coverage.
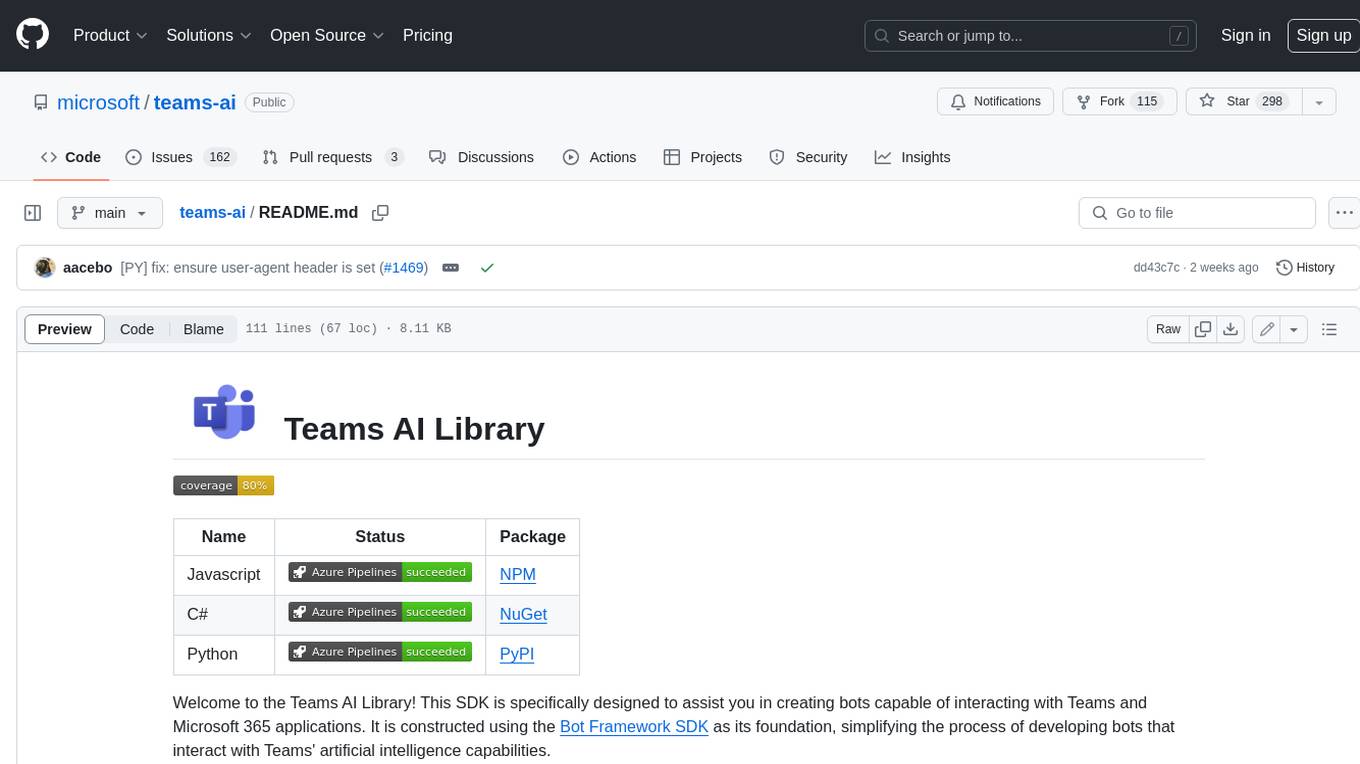
teams-ai
The Teams AI Library is a software development kit (SDK) that helps developers create bots that can interact with Teams and Microsoft 365 applications. It is built on top of the Bot Framework SDK and simplifies the process of developing bots that interact with Teams' artificial intelligence capabilities. The SDK is available for JavaScript/TypeScript, .NET, and Python.
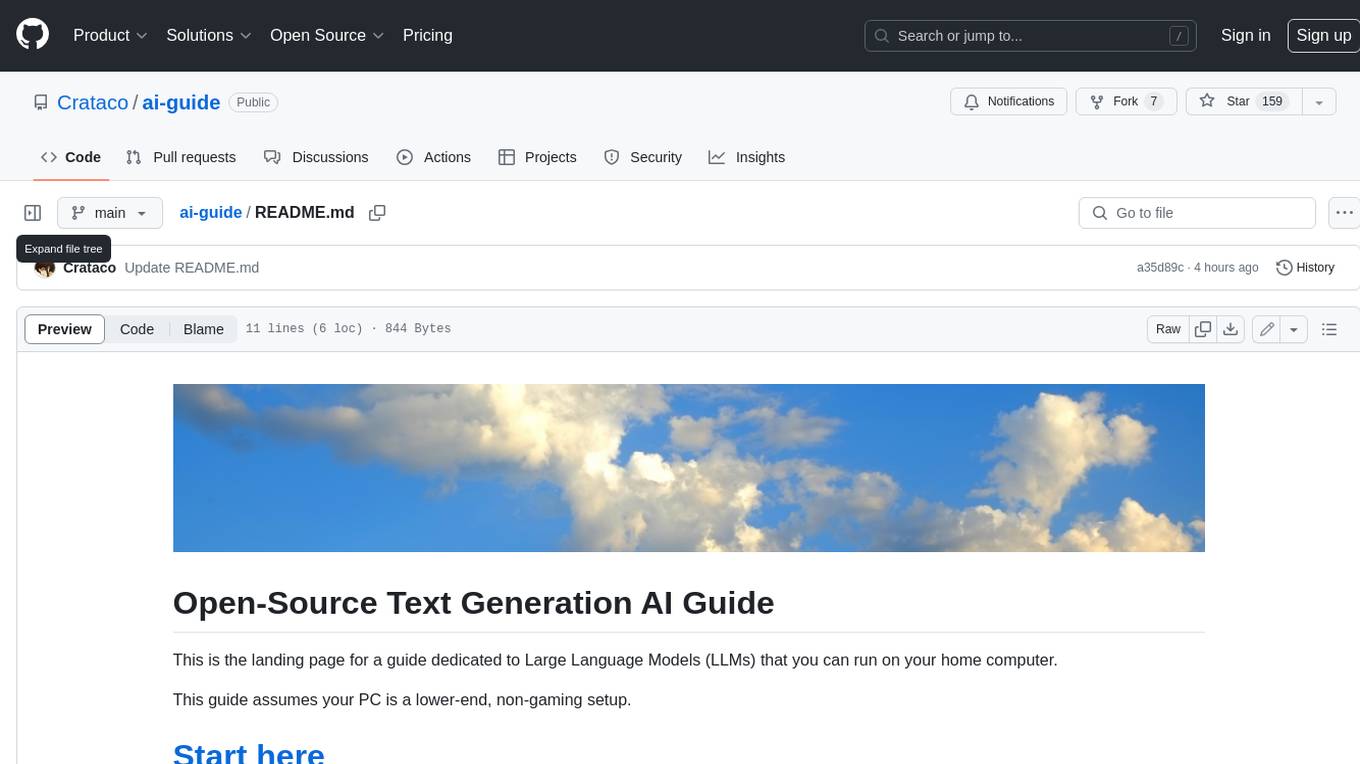
ai-guide
This guide is dedicated to Large Language Models (LLMs) that you can run on your home computer. It assumes your PC is a lower-end, non-gaming setup.
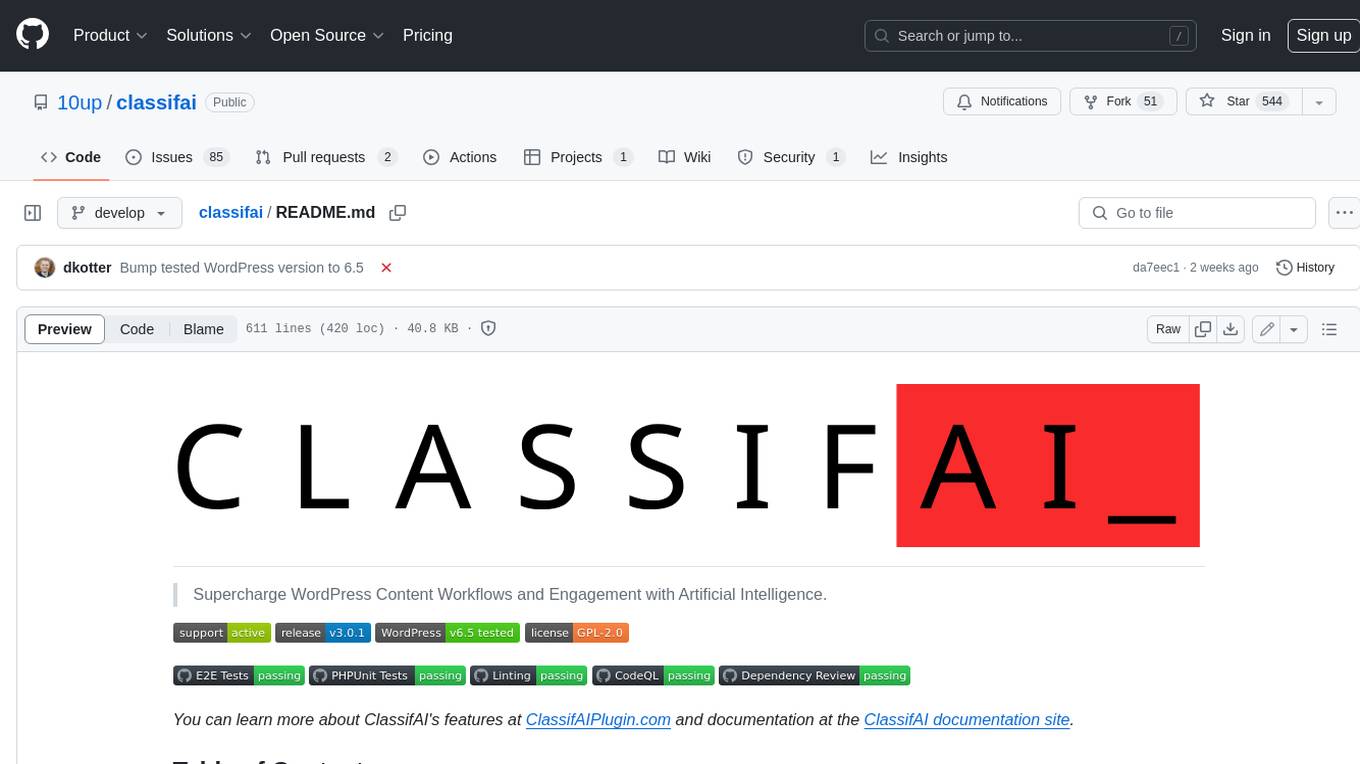
classifai
Supercharge WordPress Content Workflows and Engagement with Artificial Intelligence. Tap into leading cloud-based services like OpenAI, Microsoft Azure AI, Google Gemini and IBM Watson to augment your WordPress-powered websites. Publish content faster while improving SEO performance and increasing audience engagement. ClassifAI integrates Artificial Intelligence and Machine Learning technologies to lighten your workload and eliminate tedious tasks, giving you more time to create original content that matters.
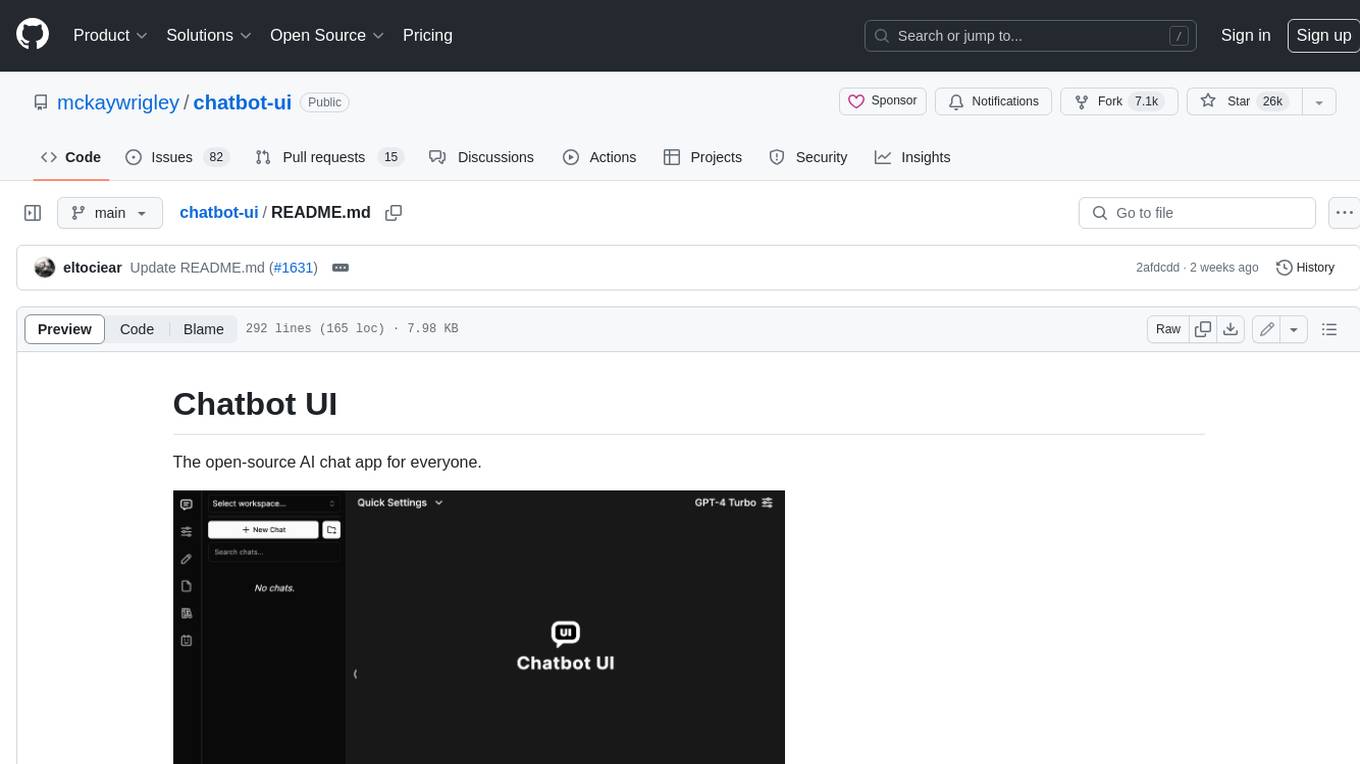
chatbot-ui
Chatbot UI is an open-source AI chat app that allows users to create and deploy their own AI chatbots. It is easy to use and can be customized to fit any need. Chatbot UI is perfect for businesses, developers, and anyone who wants to create a chatbot.
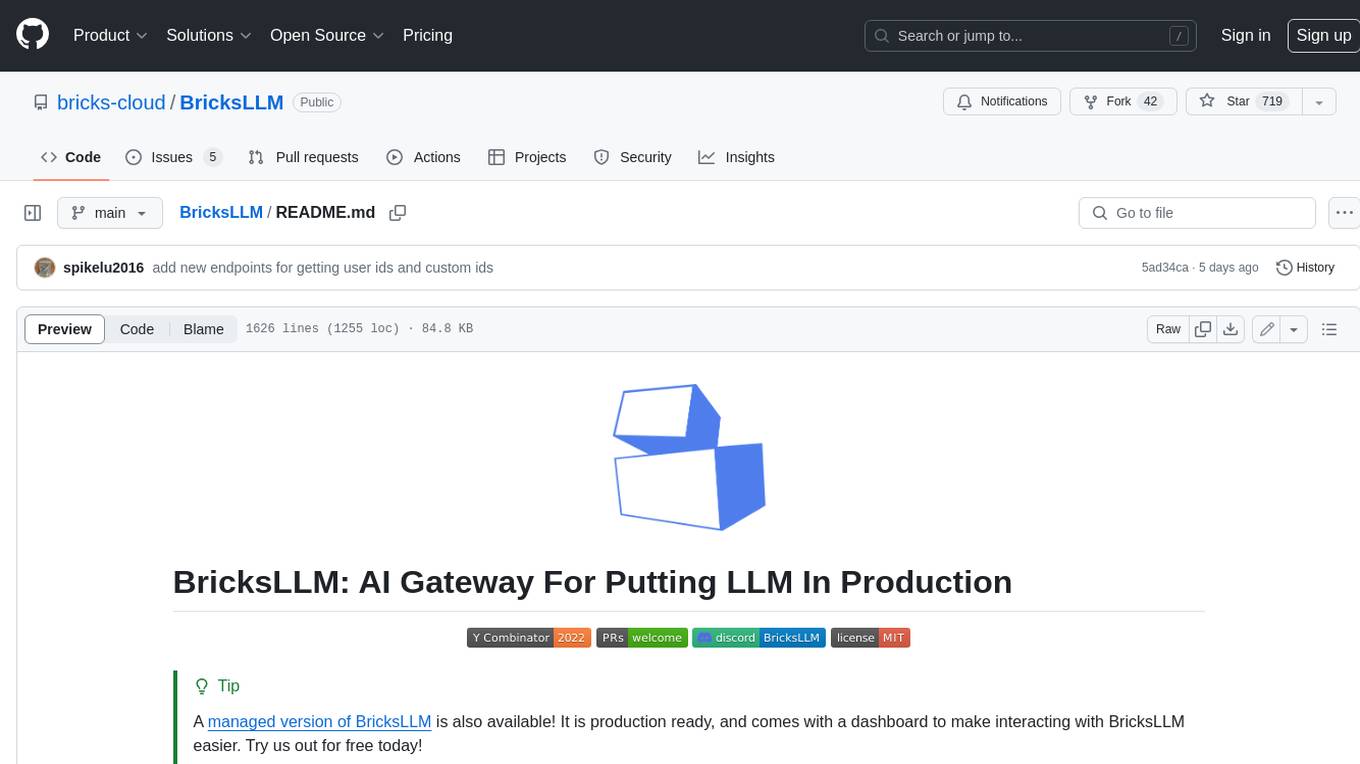
BricksLLM
BricksLLM is a cloud native AI gateway written in Go. Currently, it provides native support for OpenAI, Anthropic, Azure OpenAI and vLLM. BricksLLM aims to provide enterprise level infrastructure that can power any LLM production use cases. Here are some use cases for BricksLLM: * Set LLM usage limits for users on different pricing tiers * Track LLM usage on a per user and per organization basis * Block or redact requests containing PIIs * Improve LLM reliability with failovers, retries and caching * Distribute API keys with rate limits and cost limits for internal development/production use cases * Distribute API keys with rate limits and cost limits for students
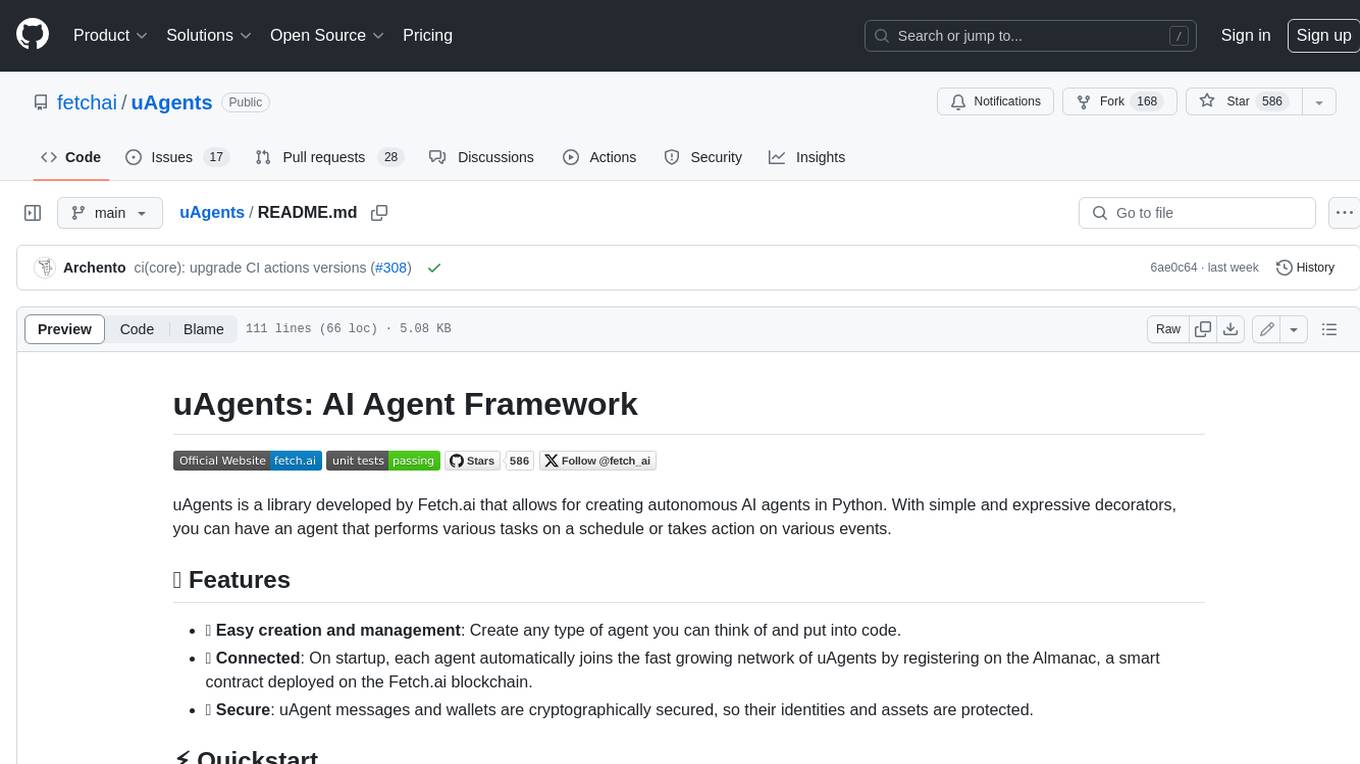
uAgents
uAgents is a Python library developed by Fetch.ai that allows for the creation of autonomous AI agents. These agents can perform various tasks on a schedule or take action on various events. uAgents are easy to create and manage, and they are connected to a fast-growing network of other uAgents. They are also secure, with cryptographically secured messages and wallets.
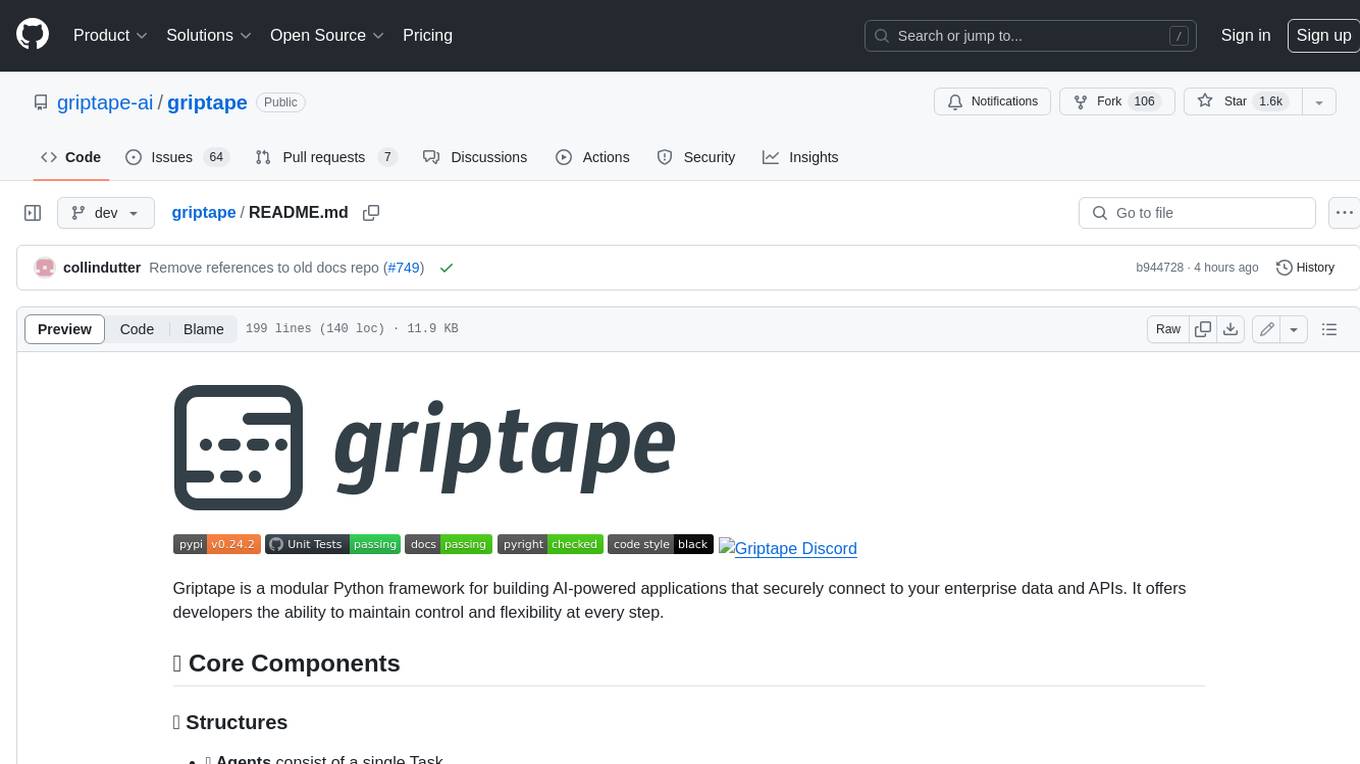
griptape
Griptape is a modular Python framework for building AI-powered applications that securely connect to your enterprise data and APIs. It offers developers the ability to maintain control and flexibility at every step. Griptape's core components include Structures (Agents, Pipelines, and Workflows), Tasks, Tools, Memory (Conversation Memory, Task Memory, and Meta Memory), Drivers (Prompt and Embedding Drivers, Vector Store Drivers, Image Generation Drivers, Image Query Drivers, SQL Drivers, Web Scraper Drivers, and Conversation Memory Drivers), Engines (Query Engines, Extraction Engines, Summary Engines, Image Generation Engines, and Image Query Engines), and additional components (Rulesets, Loaders, Artifacts, Chunkers, and Tokenizers). Griptape enables developers to create AI-powered applications with ease and efficiency.