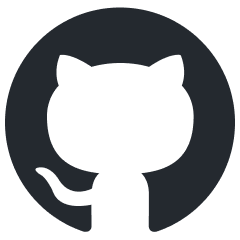
gpt-subtrans
Open Source project using LLMs to translate SRT subtitles
Stars: 418
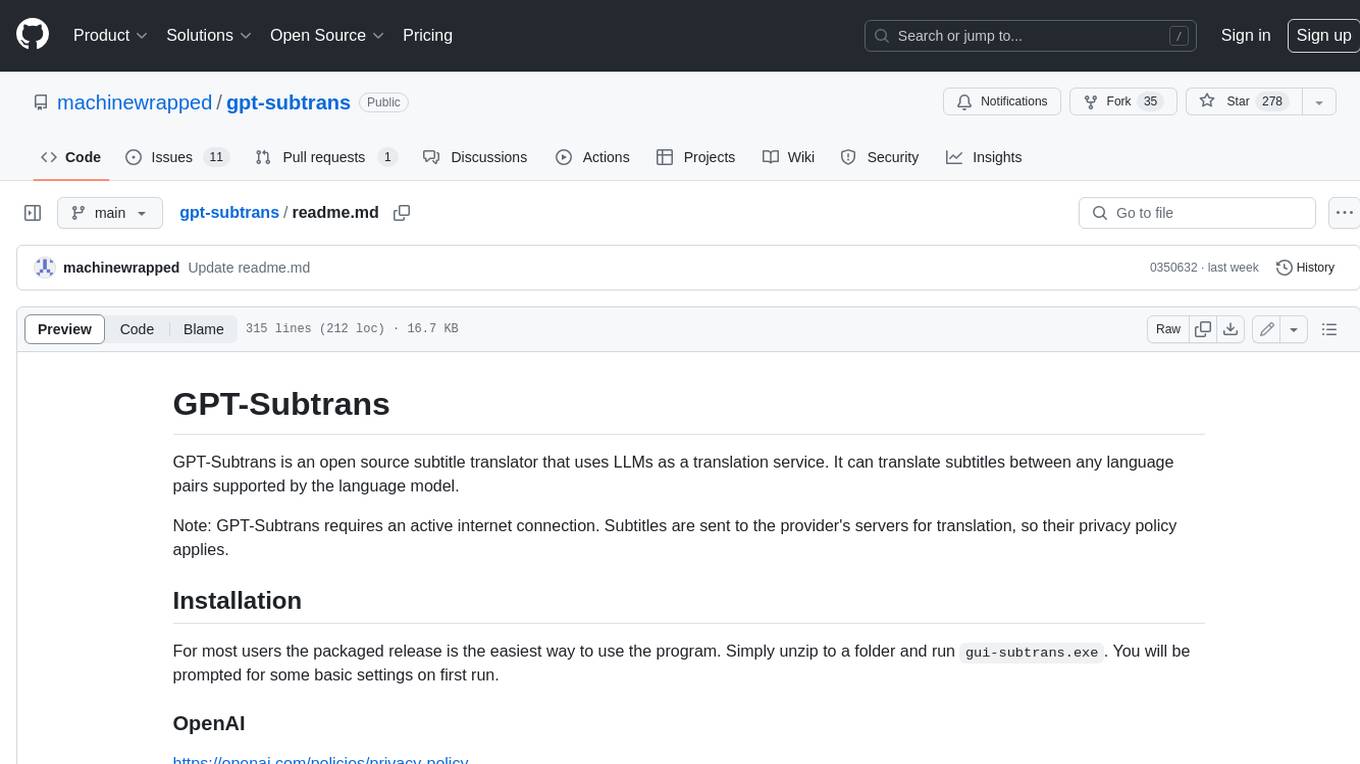
GPT-Subtrans is an open-source subtitle translator that utilizes large language models (LLMs) as translation services. It supports translation between any language pairs that the language model supports. Note that GPT-Subtrans requires an active internet connection, as subtitles are sent to the provider's servers for translation, and their privacy policy applies.
README:
GPT-Subtrans is an open source subtitle translator that uses LLMs as a translation service. It can translate subtitles between any language pairs supported by the language model.
Note: GPT-Subtrans requires an active internet connection. Subtitles are sent to the provider's servers for translation, so their privacy policy applies.
For most users the packaged release is the easiest way to use the program. Download a package from the releases page, unzip to a folder and run gui-subtrans.exe
. You will be prompted for some basic settings on first run.
Every release is packaged for Windows (gui-subtrans-x.x.x.zip). MacOS packages are provided when possible (gui-subtrans-x.x.x.macos-arm64.zip), but are sometimes blocked by PyInstaller issues. If the latest release does not have a macos-arm64 package you can download an earlier release or install from source.
https://openai.com/policies/privacy-policy
You will need an OpenAI API key from https://platform.openai.com/account/api-keys to use OpenAI's GPT models as a translator.
If the API key is associated with a free trial account the translation speed will be severely restricted.
You can use the custom api_base parameter to access a custom OpenAI instance or other providers with an OpenAI compatible API (which is most of them).
You can use an OpenAI Azure installation as a translation provider, but this is only advisable if you know what you're doing - in which case hopefully it will be clear how to configure the Azure provider settings.
Please note that regions restrictions may apply: https://ai.google.dev/available_regions
You will need a Google Gemini API key from https://ai.google.dev/ or from a project created on https://console.cloud.google.com/. You must ensure that Generative AI is enabled for the api key and project.
The Gemini 2.0 Flash Experimental model is perhaps the leading model for translation speed and fluency at time of writing, and is currently free to use.
https://support.anthropic.com/en/collections/4078534-privacy-legal
You will need an Anthropic API key from https://console.anthropic.com/settings/keys to use Claude as a provider. The Anthropic SDK does not provide a way to retrieve available models, so the latest Claude 3 model names are currently hardcoded.
The API has very strict rate limits based on your credit tier, both on requests per minutes and tokens per day. The free credit tier should be sufficient to translate approximately one full movie per day.
https://platform.deepseek.com/downloads/DeepSeek%20Open%20Platform%20Terms%20of%20Service.html
You will need a DeepSeek API key from https://platform.deepseek.com/api_keys to use this provider.
-
API Base: You can optionally specify a custom URL, e.g. if you are hosting your own DeepSeek instance. If this is not set, the official DeepSeek API endpoint will be used.
-
Model: The default model is
deepseek-chat
, which is recommended for translation tasks.deepseek-reasoner
may produce better results for source subtitles with OCR or transcription errors as it will spend longer trying to guess what the error is.
DeepSeek is quite simple to set up and offers reasonable performance at a very low price, though translation does not seem to be its strongest point.
You will need a Mistral API key from https://console.mistral.ai/api-keys/ to use this provider.
-
Server URL: If you are using a custom deployment of the Mistral API, you can specify the server URL using the
--server_url
argument. -
Model:
mistral-large-latest
is recommended for translation. Smaller models tend to perform poorly and may not follow the system instructions well.
Mistral AI is straightforward to set up, but its performance as a translator is not particularly good.
GPT-Subtrans can interface directly with any server that supports an OpenAI compatible API, including locally hosted models e.g. LM Studio.
This is mainly for research and you should not expect particularly good results. LLMs derive much of their power from their size, so the small, quantized models you can run on a GPU are likely to produce poor translations, fail to generate valid responses or get stuck in endless loops. If you find a model that reliably producess good results, please post about it in the Discussions area!
Chat and completion endpoints are supported, you should configure the settings and endpoint based on the model the server is running (e.g. instruction tuned models will probably produce better results using the completions endpoint rather than chat/conversation). The prompt template can be edited in the GUI if you are using a model that requires a particular format - make sure to include at least the {prompt} tag in the template, as this is where the subtitles that need translating in each batch will be provided.
https://aws.amazon.com/service-terms/
Bedrock is not recommended for most users: The setup process is complex, requiring AWS credentials, proper IAM permissions, and region configuration. Additionally, not all models on Bedrock support translation tasks or offer reliable results. Bedrock support will not be included in pre-packaged versions - if you can handle setting up AWS, you can handle installing gpt-subtrans from source!
To use Bedrock, you must:
- Create an IAM user or role with appropriate permissions (e.g.,
bedrock:InvokeModel
,bedrock:ListFoundationModels
). - Ensure the model you wish to use is accessible in your selected AWS region and enabled for the IAM user.
Building MacOS universal binaries with PyInstaller has not worked for some time so releases are only provided for Apple Silicon. If you have an Intel Mac you will need to install from source. If anybody would like to volunteer to maintain Intel releases, please get in touch.
Prebuilt Linux packages are not provided so you will need to install from source.
For other platforms, or if you want to modify the program, you will need to have Python 3.10+ and pip installed on your system, then follow these steps.
-
Clone the GPT-Subtrans repository onto your local machine using the following command:
git clone https://github.com/machinewrapped/gpt-subtrans.git
The easiest setup method is to run an installation script, e.g. install-openai.bat
or install-gemini.bat
. This will create a virtual environment and install all the required packages for the provider, and generate command scripts to launch the specified provider. MacOS and Linux users should run install.sh
instead (this should work on any unix-like system).
During the installing process, input the apikey for the selected provider if requested. It will be saved in a .env file so that you don't need to provide it every time you run the program.
If you ran an install script you can skip the remaining steps. Continue reading if you want to configure the environment manually instead.
-
Create a new file named .env in the root directory of the project. Add any required settings for your chosen provider to the .env file like this:
OPENAI_API_KEY=<your_openai_api_key> GEMINI_API_KEY=<your_gemini_api_key> AZURE_API_KEY=<your_azure_api_key> CLAUDE_API_KEY=<your_claude_api_key>
If you are using Azure:
AZURE_API_BASE=<your api_base, such as https://something.openai.azure.com> AZURE_DEPLOYMENT_NAME=<deployment_name>
If you are using Bedrock:
AWS_ACCESS_KEY_ID=your-access-key-id AWS_SECRET_ACCESS_KEY=your-secret-access-key AWS_REGION=your-region
For OpenAI reasoning models you can set the reasoning effort (default is low):
OPENAI_REASONING_EFFORT=low/medium/high
-
Create a virtual environment for the project by running the following command in the root folder to create a local environment for the Python interpreter.:
python -m venv envsubtrans
notice: For linux user, the environment has already prepared during the installing process.
-
Activate the virtual environment by running the appropriate command for your operating system:
.\envsubtrans\Scripts\activate .\envsubtrans\bin\activate source path/to/gpt-subtrans/envsubtrans/bin/activate # for linux user
-
Install the required libraries using pip by running the following command in your terminal to install the project dependencies (listed in the requirements.txt file):
pip install -r requirements.txt
-
Install the SDKs for the provider(s) you intend to use
pip install openai pip install google-genai pip install anthropic
Note that steps 3 and 4 are optional, but they can help prevent conflicts with other Python applications.
The program works by dividing the subtitles up into small batches and sending each one to the translation service in turn. It is likely to take time to complete, and can potentially make many API calls for each subtitle file.
By default The translated subtitles will be written to a new SRT file in the same directory with the target langugage appended to the original filename.
Subtitle Edit's (https://www.nikse.dk/subtitleedit) "Fix Common Errors" can help to clean up the translated subtitles, though some of its functionality is now covered by the post-process option (--postprocess
) in GPT-Subtrans.
The Subtrans GUI is the best and easiest way to use the program. After installation, launch the GUI with the gui-subtrans
command or shell script, and hopefully the rest should be self-explanatory.
See the project wiki for further details on how to use the program.
GPT-Subtrans can be used as a console command or shell script. The install scripts create a cmd or sh file in the project root for each provider, which will take care of activating the virtual environment and calling the corresponding translation script.
The most basic usage is:
gpt-subtrans <path_to_srt_file> --target_language <target_language>
gemini-subtrans <path_to_srt_file> --target_language <target_language>
claude-subtrans <path_to_srt_file> --target_language <target_language>
llm-subtrans -s <server_address> -e <endpoint> -l <language> <path_to_srt_file>
python3 batch_process.py # process files in different folders
If the target language is not specified the default is English. Other options that can be specified on the command line are detailed below.
There are a number of command-line arguments that offer more control over the translation process.
Default values for many settings can be set in the .env file, using a NAME_IN_CAPS with format. See Options.py for the full list.
To use any of these arguments, add them to the command-line after the path to the SRT file. For example:
gpt-subtrans path/to/my/subtitles.srt --moviename "My Awesome Movie" --ratelimit 10 --substitution cat::dog
-
-l
,--target_language
: The language to translate the subtitles to. -
-o
,--output
: Specify a filename for the translated subtitles. -
--project
: Read or Write a project file for the subtitles being translated. More on this below. -
--ratelimit
: Maximum number of requests to the translation service per minute (mainly relevant if you are using an OpenAI free trial account). -
--moviename
: Optionally identify the source material to give context to the translator. -
--description
: A brief description of the source material to give further context. Less is generally more here, or the AI can start improvising. -
--name
,--names
: Optionally provide (a list of) names to use in the translation (more powerful AI models are more likely to actually use them). -
--substitution
: A pair of strings separated by::
, to substitute in either source or translation, or the name of a file containing a list of such pairs. -
--scenethreshold
: Number of seconds between lines to consider it a new scene. -
--minbatchsize
: Minimum number of lines to consider starting a new batch to send to the translator. Higher values typically result in faster and cheaper translations but increase the risk of desyncs. -
--maxbatchsize
: Maximum number of lines before starting a new batch is compulsory. This needs to take into account the token limit for the model being used, but the "optimal" value depends on many factors, so experimentation is encouraged. Larger batches are more cost-effective but increase the risk of the AI desynchronising, triggering expensive retries. -
--preprocess
: Preprocess the subtitles prior to batching. This performs various actions to prepare the subtitles for more efficient translation, e.g. splitting long (duration) lines into multiple lines. Mainly intended for subtitles that have been automatically transcribed with e.g. Whisper. -
--postprocess
: Post-process translated subtitles. Performs various actions like adding line breaks to long lines and normalising dialogue tags after a translation request. -
--instruction
: An additional instruction for the AI indicating how it should approach the translation. -
--instructionfile
: Name/path of a file to load AI system instructions from (otherwise the default instructions.txt is used). -
--maxlines
: Maximum number of batches to process. To end the translation after a certain number of lines, e.g. to check the results. -
--temperature
: A higher temperature increases the random variance of translations. Default 0.
Some additional arguments are available for specific providers.
-
-k
,--apikey
: Your OpenAI API Key. -
-b
,--apibase
: API base URL if you are using a custom instance. if it is not set, the default URL will be used. -
'--httpx': Use the HTTPX library for requests (only supported if apibase is specified)
-
-m
,--model
: Specify the AI model to use for translation
-
-k
,--apikey
: Your Google Gemini API Key. Not required if it is set in the .env file. -
-m
,--model
: Specify the AI model to use for translation
-
-k
,--apikey
: Your Anthropic API Key. Not required if it is set in the .env file. -
-m
,--model
: Specify the AI model to use for translation. This should be the full model name, e.g.claude-3-haiku-20240307
-
--deploymentname
: Azure deployment name -
-k
,--apikey
: API key for your deployment. -
-b
,--apibase
: API backend base address. -
-a
,--apiversion
: Azure API version.
-
-k
,--apikey
: Your DeepSeek API Key. -
-b
,--apibase
: Base URL if you are using a custom deployment of DeepSeek. if it is not set, the official URL will be used. -
-m
,--model
: Specify the model to use for translation. deepseek-chat is probably the only sensible choice (and default).
-
-k
,--apikey
: Your DeepSeek API Key. -
--server_url
: URL if you are using a custom deployment of Mistral. if unset, the official URL will be used. -
-m
,--model
: Specify the model to use for translation. mistral-large-latest is recommended, the small models are not very reliable.
-
-k
,--accesskey
: Your AWS Access Key ID. Not required if it is set in the.env
file. -
-s
,--secretkey
: Your AWS Secret Access Key. Not required if it is set in the.env
file. -
-r
,--region
: AWS Region where Bedrock is available. You can check the list of regions here. For example:us-east-1
oreu-west-1
. -
-m
,--model
: The ID of the Bedrock model to use for translation. Examples includeamazon.titan-text-lite-v1
oramazon.titan-text-express-v1
.
-
-s
,--server
: The address the server is running on, including port (e.g. http://localhost:1234). Should be provided by the server -
-e
,--endpoint
: The API function to call on the server, e.g./v1/completions
. Choose an appropriate endpoint for the model running on the server. -
--chat
: Specify this argument if the endpoint expects requests in a conversation format - otherwise it is assumed to be a completion endpoint. -
--systemmessages
: If using a conversation endpoint, translation instructions will be sent as the "system" user if this flag is specified. -
-k
,--apikey
: Local servers shouldn't need an api key, but the option is provided in case it is needed for your setup. -
-m
,--model
: The model will usually be determined by the server, but the option is provided in case you need to specify it.
If you need to use proxy in your location, you can use socks proxy by using command line
python3 gpt-subtrans.py <path_to_srt_file> --target_language <target_language> --proxy socks://127.0.0.1:1089
Remember to change the local port to yours and turn on your proxy tools such as v2ray, naiveproxy and clash.
you can process files with the following struct:
# -SRT
# --fold1
# ---1.srt
# ---2.srt
# ...
# --fold2
# ---1.srt
# ---2.srt
# ...
python3 batch_process.py # process files in different folders
You need to modify the command line in batch_process.py accordingly.
It is recommended to use an IDE such as Visual Studio Code to run the program when installed from source, and set up a launch.json file to specify the arguments.
Note: Remember to activate the virtual environment every time you work on the project.
Note If you are using the GUI a project file is created automatically when you open a subtitle file for the first time, and updated automatically.
The --project
argument or PROJECT
.env setting can take a number of values, which control whether and when an intermediate file will be written to disc.
The default setting is None
, which means the project file is neither written nor read, the only output of the program is the final translation.
If the argument is set to True
then a project file will be created with the .subtrans
extension, containing details of the translation process,
and it will be updated as the translation progresses. Writing a project file allows, amongst other things, resuming a translation that was interrupted.
Other valid options include preview
, resume
, reparse
and retranslate
. These are probably only useful if you're modifying the code, in which case
you should be able to see what they do.
Version 1.0 is (ironically) a minor update, updating the major version to 1.0 because the project has been stable for some time.
Version 0.7 introduced optional post-processing of translated subtitles to try to fix some of the common issues with LLM-translated subtitles (e.g. adding line breaks), along with new default instructions that tend to produce fewer errors.
Version 0.6 changes the architecture to a provider-based system, allowing multiple AI services to be used as translators. Settings are compartmentalised for each provider. For the intial release the only supported provider is OpenAI.
Version 0.5 adds support for gpt-instruct models and a refactored code base to support different translation engines. For most users, the recommendation is still to use the gpt-3.5-turbo-16k model with batch sizes of between (10,100) lines, for the best combination of performance/cost and translation quality.
Version 0.4 features significant optimisations to the GUI making it more responsive and usable, along with numerous bug fixes.
Version 0.3 featured a major effort to bring the GUI up to full functionality and usability, including adding options dialogs and more, plus many bug fixes.
Version 0.2 employs a new prompting approach that greatly reduces desyncs caused by GPT merging together source lines in the translation. This can reduce the naturalness of the translation when the source and target languages have very different grammar, but it provides a better base for a human to polish the output.
The instructions have also been made more detailed, with multiple examples of correct output for GPT to reference, and the generation of summaries has been improved so that GPT is better able to understand the context of the batch it is translating. Additionally, double-clicking a scene or batch now allows the summary to be edited by hand, which can greatly improve the results of a retranslation and of subsequent batches or scenes. Individually lines can also be edited by double-clicking them.
Contributions from the community are welcome! To contribute, follow these steps:
Fork the repository onto your own GitHub account.
Clone the repository onto your local machine using the following command:
git clone https://github.com/your_username/GPT-Subtrans.git
Create a new branch for your changes using the following command:
git checkout -b feature/your-new-feature
Make your changes to the code and commit them with a descriptive commit message.
Push your changes to your forked repository.
Submit a pull request to the main GPT-Subtrans repository.
This project uses several useful libraries:
- srt (https://github.com/cdown/srt)
- requests (https://github.com/psf/requests)
- regex (https://github.com/mrabarnett/mrab-regex)
- httpx (https://github.com/projectdiscovery/httpx)
Translation providers:
- openai (https://platform.openai.com/docs/libraries/python-bindings)
- google-genai (https://github.com/googleapis/python-genai)
- anthropic (https://github.com/anthropics/anthropic-sdk-python)
- mistralai (https://github.com/mistralai/client-python)
- boto3 (Amazon Bedrock) (https://github.com/boto/boto3)
For the GUI:
- pyside6 (https://wiki.qt.io/Qt_for_Python)
- events (https://pypi.org/project/Events/)
- darkdetect (https://github.com/albertosottile/darkdetect)
- appdirs (https://github.com/ActiveState/appdirs)
For bundled versions:
- python (https://www.python.org/)
- pyinstaller (https://pyinstaller.org/)
GPT-Subtrans is licensed under the MIT License. See LICENSE for the 3rd party library licenses.
For Tasks:
Click tags to check more tools for each tasksFor Jobs:
Alternative AI tools for gpt-subtrans
Similar Open Source Tools
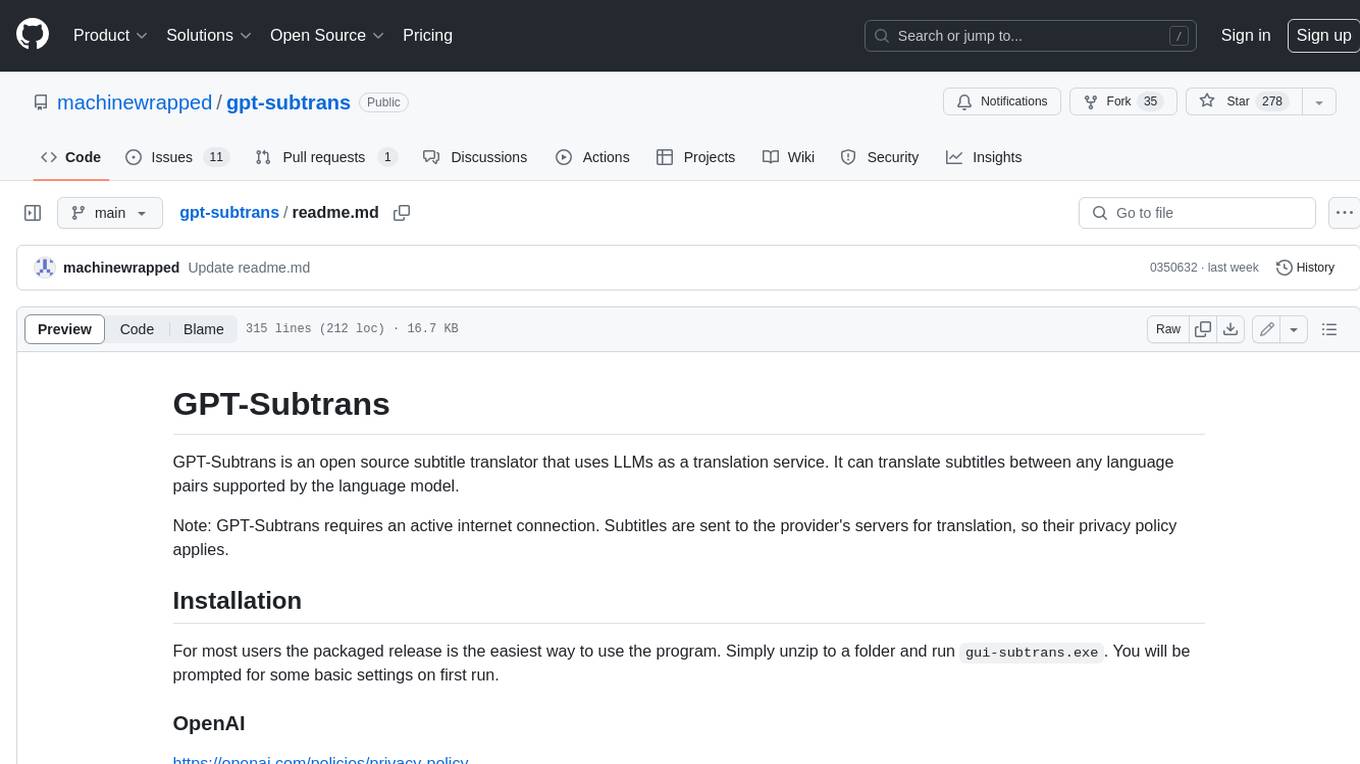
gpt-subtrans
GPT-Subtrans is an open-source subtitle translator that utilizes large language models (LLMs) as translation services. It supports translation between any language pairs that the language model supports. Note that GPT-Subtrans requires an active internet connection, as subtitles are sent to the provider's servers for translation, and their privacy policy applies.
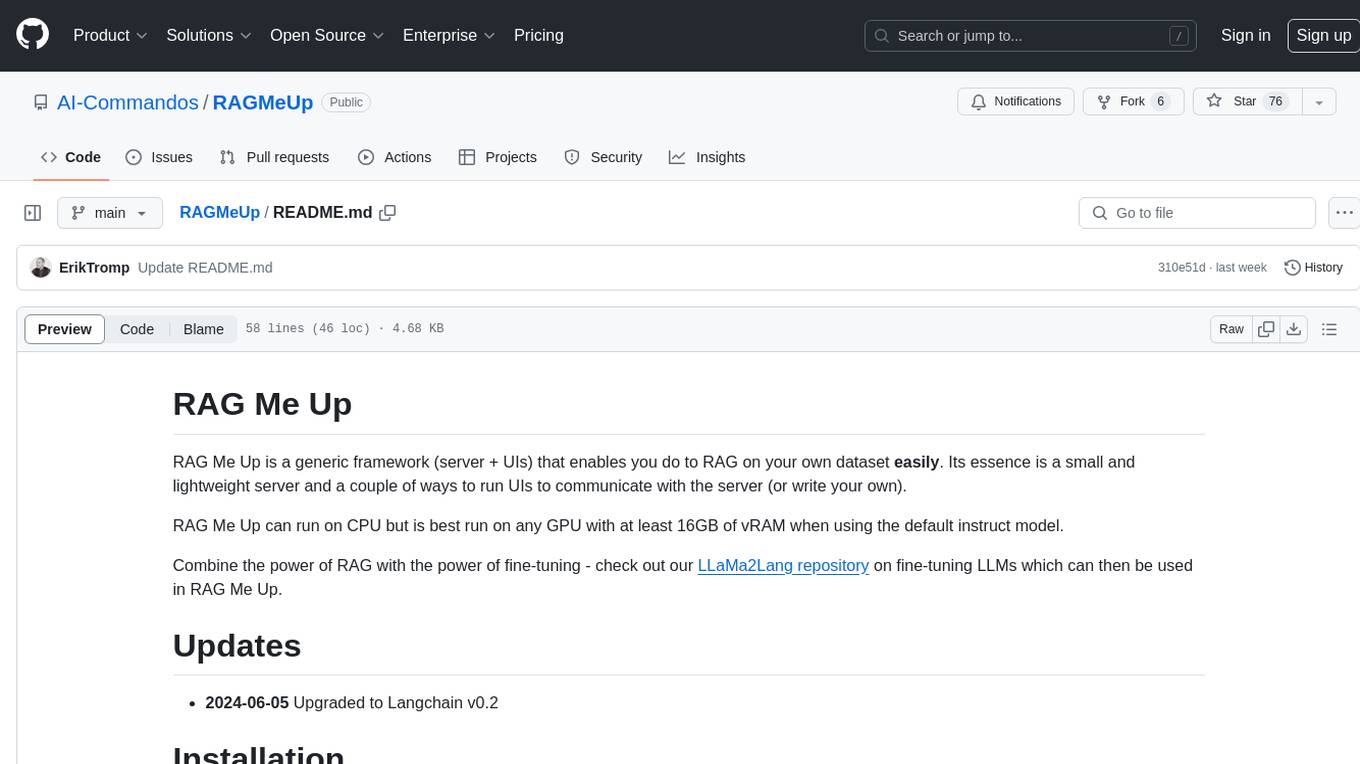
RAGMeUp
RAG Me Up is a generic framework that enables users to perform Retrieve and Generate (RAG) on their own dataset easily. It consists of a small server and UIs for communication. Best run on GPU with 16GB vRAM. Users can combine RAG with fine-tuning using LLaMa2Lang repository. The tool allows configuration for LLM, data, LLM parameters, prompt, and document splitting. Funding is sought to democratize AI and advance its applications.
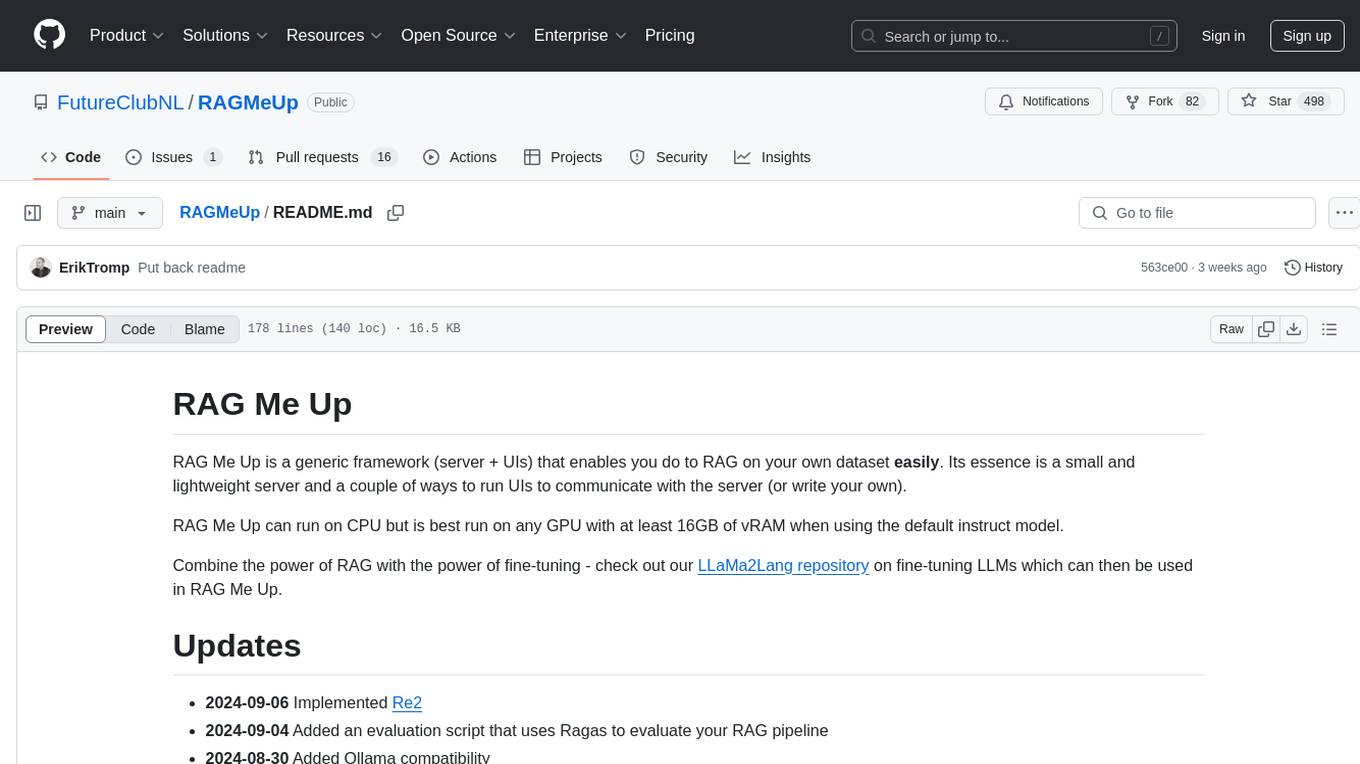
RAGMeUp
RAG Me Up is a generic framework that enables users to perform Retrieve, Answer, Generate (RAG) on their own dataset easily. It consists of a small server and UIs for communication. The tool can run on CPU but is optimized for GPUs with at least 16GB of vRAM. Users can combine RAG with fine-tuning using the LLaMa2Lang repository. The tool provides a configurable RAG pipeline without the need for coding, utilizing indexing and inference steps to accurately answer user queries.
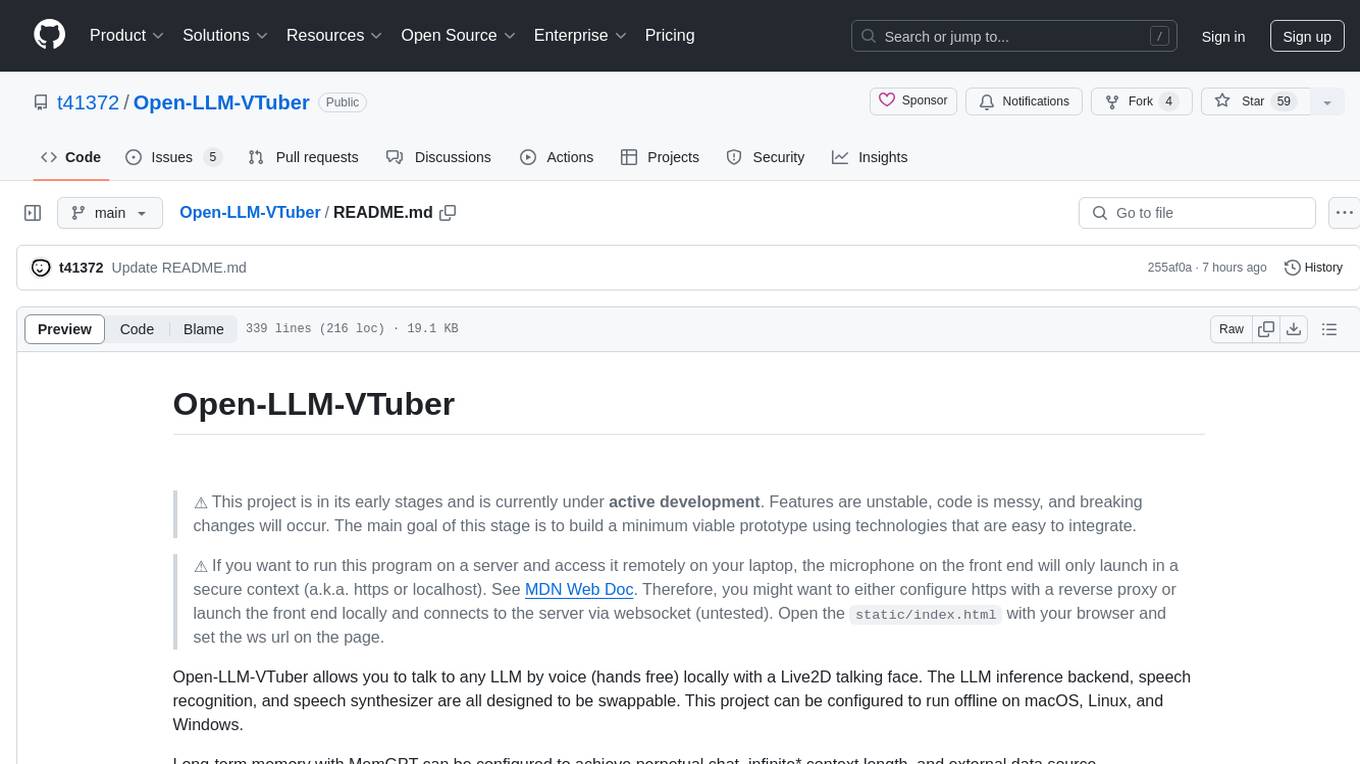
Open-LLM-VTuber
Open-LLM-VTuber is a project in early stages of development that allows users to interact with Large Language Models (LLM) using voice commands and receive responses through a Live2D talking face. The project aims to provide a minimum viable prototype for offline use on macOS, Linux, and Windows, with features like long-term memory using MemGPT, customizable LLM backends, speech recognition, and text-to-speech providers. Users can configure the project to chat with LLMs, choose different backend services, and utilize Live2D models for visual representation. The project supports perpetual chat, offline operation, and GPU acceleration on macOS, addressing limitations of existing solutions on macOS.
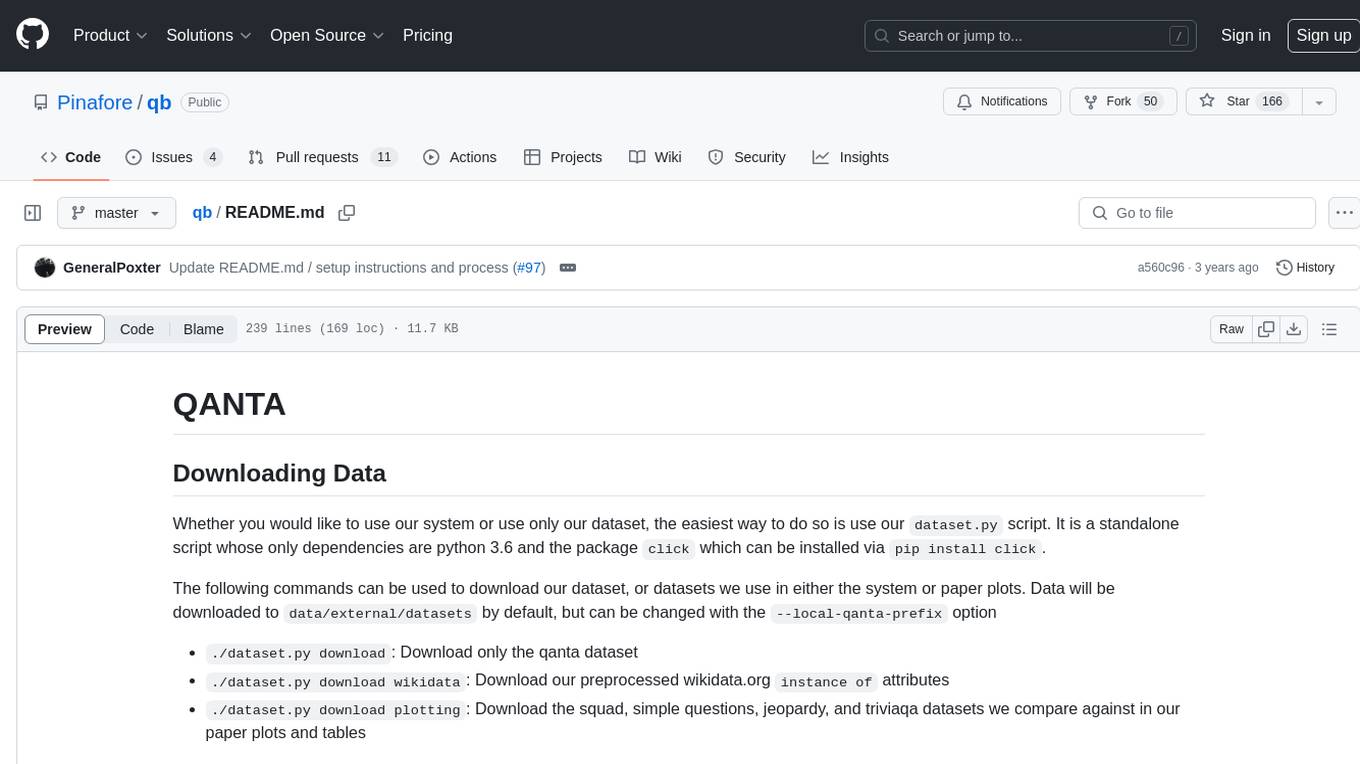
qb
QANTA is a system and dataset for question answering tasks. It provides a script to download datasets, preprocesses questions, and matches them with Wikipedia pages. The system includes various datasets, training, dev, and test data in JSON and SQLite formats. Dependencies include Python 3.6, `click`, and NLTK models. Elastic Search 5.6 is needed for the Guesser component. Configuration is managed through environment variables and YAML files. QANTA supports multiple guesser implementations that can be enabled/disabled. Running QANTA involves using `cli.py` and Luigi pipelines. The system accesses raw Wikipedia dumps for data processing. The QANTA ID numbering scheme categorizes datasets based on events and competitions.
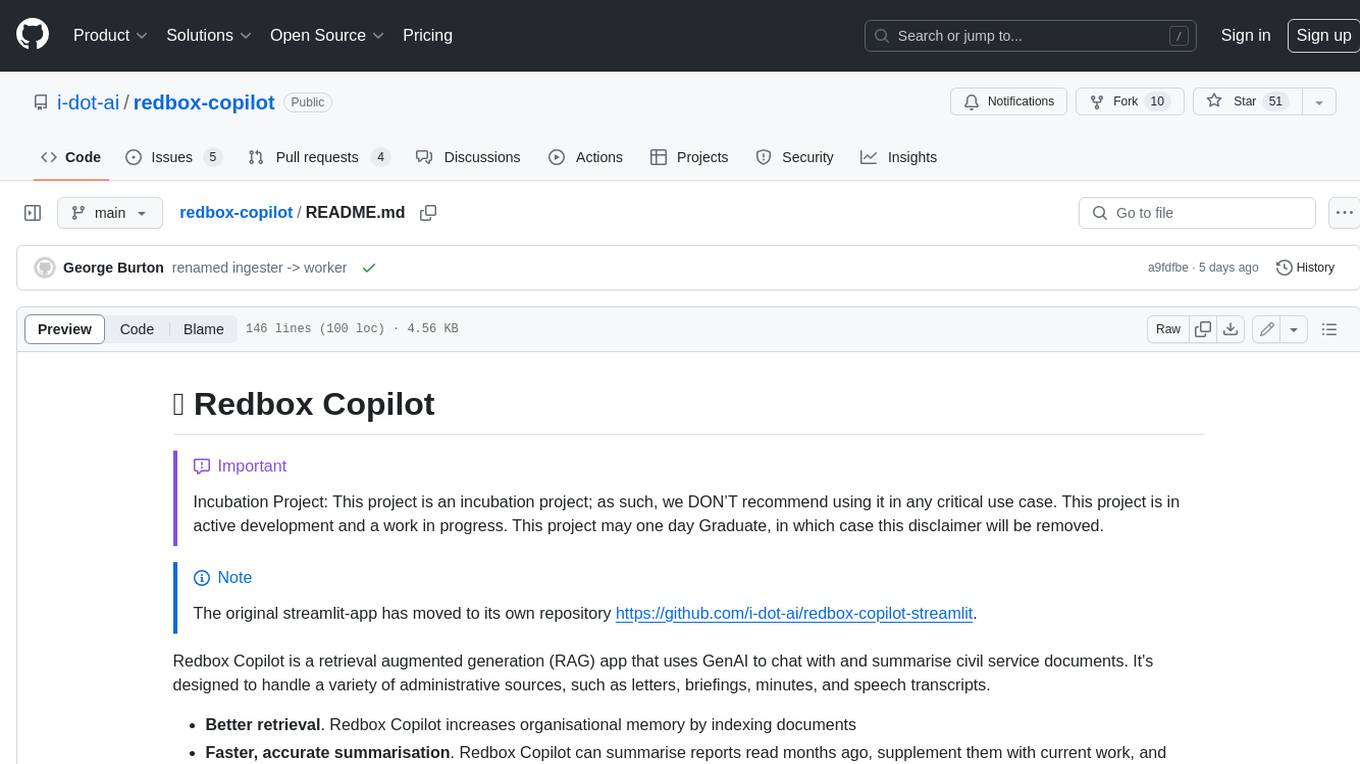
redbox-copilot
Redbox Copilot is a retrieval augmented generation (RAG) app that uses GenAI to chat with and summarise civil service documents. It increases organisational memory by indexing documents and can summarise reports read months ago, supplement them with current work, and produce a first draft that lets civil servants focus on what they do best. The project uses a microservice architecture with each microservice running in its own container defined by a Dockerfile. Dependencies are managed using Python Poetry. Contributions are welcome, and the project is licensed under the MIT License.
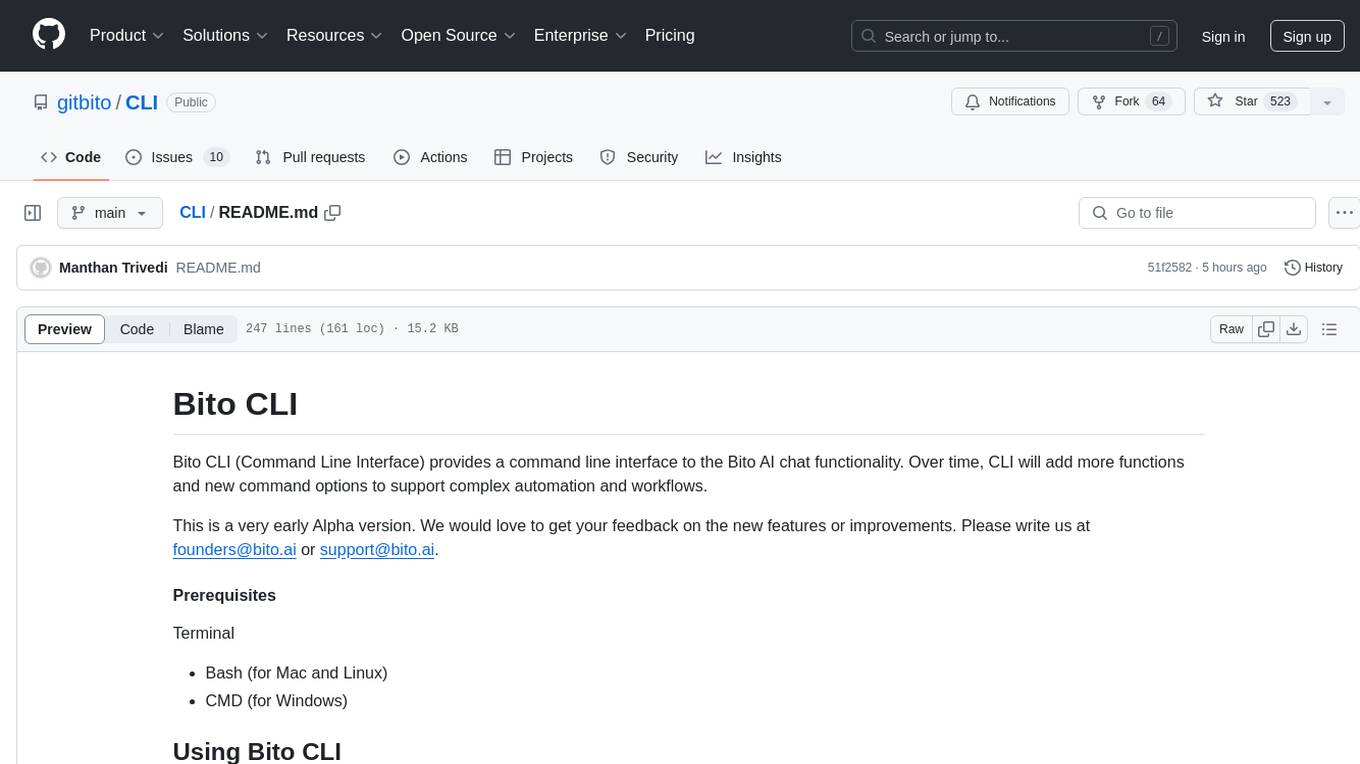
CLI
Bito CLI provides a command line interface to the Bito AI chat functionality, allowing users to interact with the AI through commands. It supports complex automation and workflows, with features like long prompts and slash commands. Users can install Bito CLI on Mac, Linux, and Windows systems using various methods. The tool also offers configuration options for AI model type, access key management, and output language customization. Bito CLI is designed to enhance user experience in querying AI models and automating tasks through the command line interface.
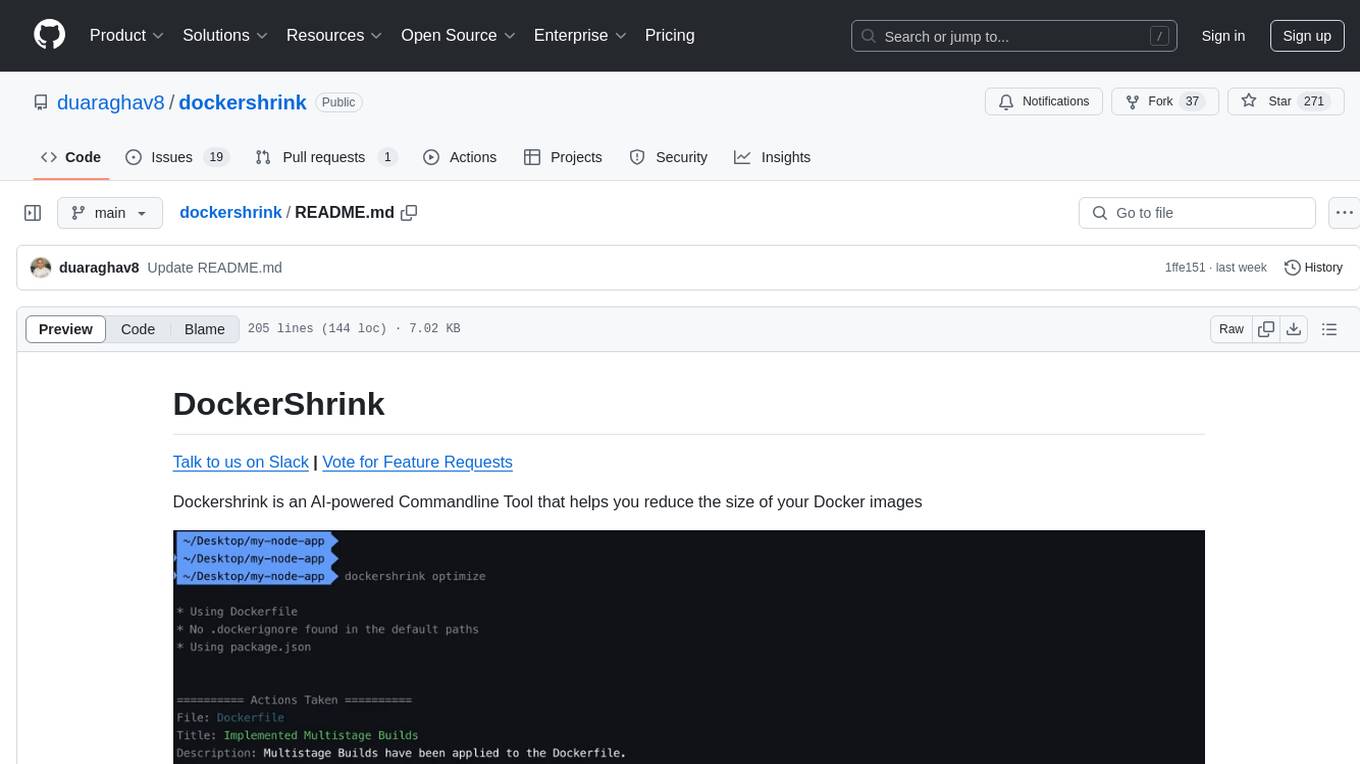
dockershrink
Dockershrink is an AI-powered Commandline Tool designed to help reduce the size of Docker images. It combines traditional Rule-based analysis with Generative AI techniques to optimize Image configurations. The tool supports NodeJS applications and aims to save costs on storage, data transfer, and build times while increasing developer productivity. By automatically applying advanced optimization techniques, Dockershrink simplifies the process for engineers and organizations, resulting in significant savings and efficiency improvements.
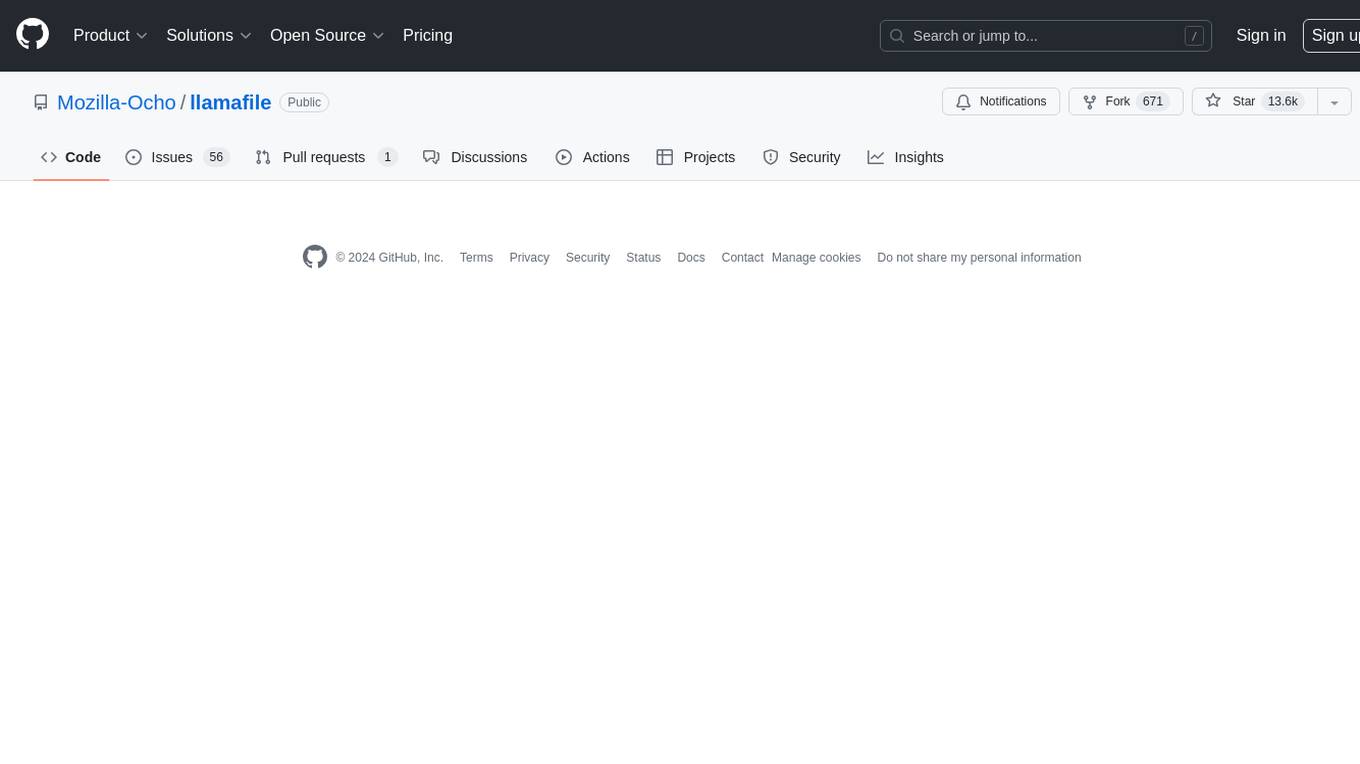
llamafile
llamafile is a tool that enables users to distribute and run Large Language Models (LLMs) with a single file. It combines llama.cpp with Cosmopolitan Libc to create a framework that simplifies the complexity of LLMs into a single-file executable called a 'llamafile'. Users can run these executable files locally on most computers without the need for installation, making open LLMs more accessible to developers and end users. llamafile also provides example llamafiles for various LLM models, allowing users to try out different LLMs locally. The tool supports multiple CPU microarchitectures, CPU architectures, and operating systems, making it versatile and easy to use.
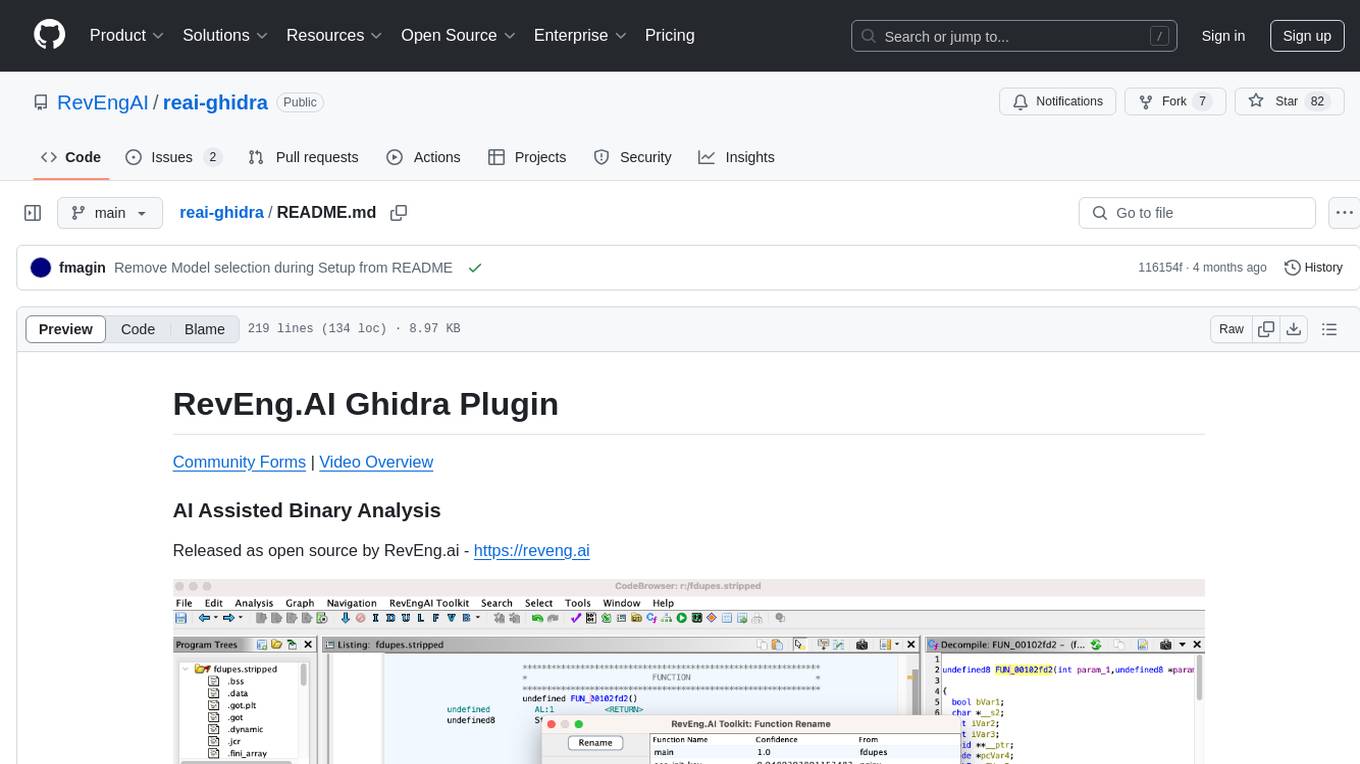
reai-ghidra
The RevEng.AI Ghidra Plugin by RevEng.ai allows users to interact with their API within Ghidra for Binary Code Similarity analysis to aid in Reverse Engineering stripped binaries. Users can upload binaries, rename functions above a confidence threshold, and view similar functions for a selected function.
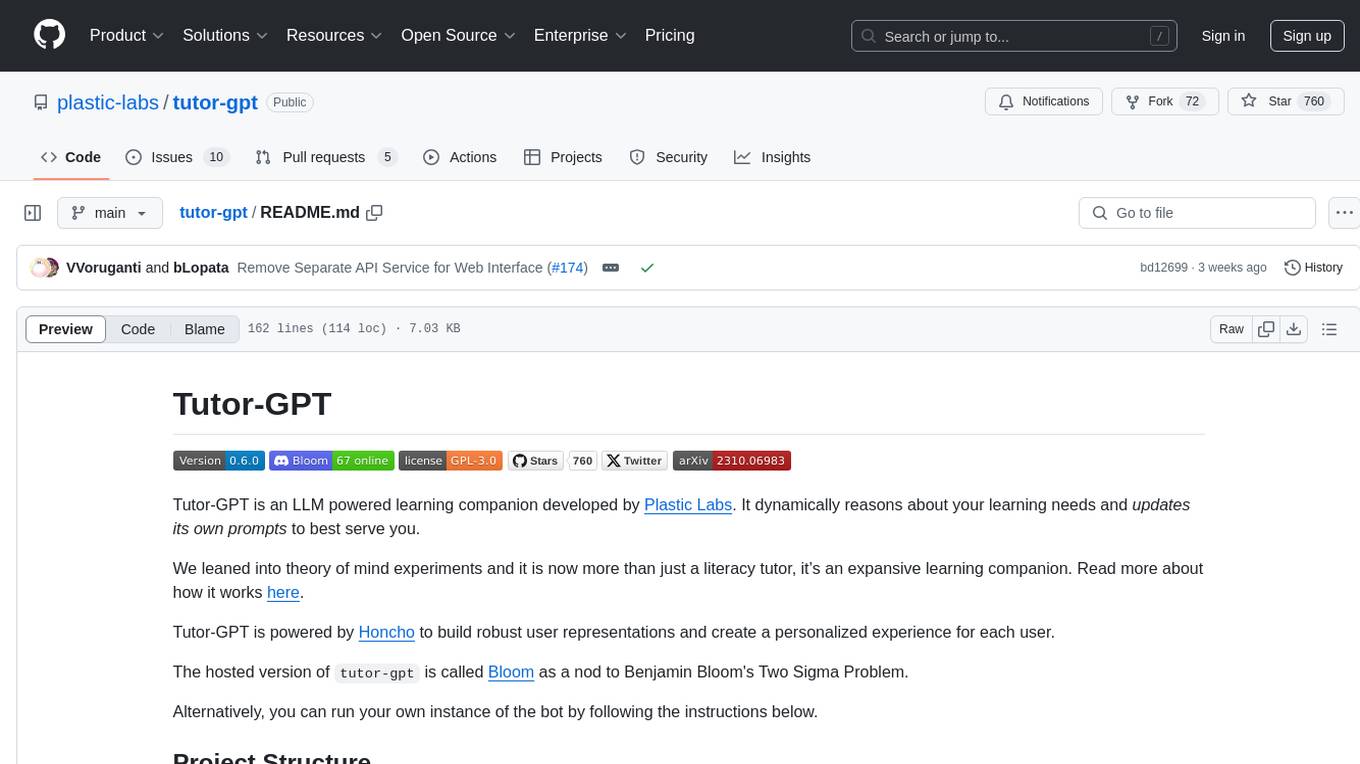
tutor-gpt
Tutor-GPT is an LLM powered learning companion developed by Plastic Labs. It dynamically reasons about your learning needs and updates its own prompts to best serve you. It is an expansive learning companion that uses theory of mind experiments to provide personalized learning experiences. The project is split into different modules for backend logic, including core logic, discord bot implementation, FastAPI API interface, NextJS web front end, common utilities, and SQL scripts for setting up local supabase. Tutor-GPT is powered by Honcho to build robust user representations and create personalized experiences for each user. Users can run their own instance of the bot by following the provided instructions.
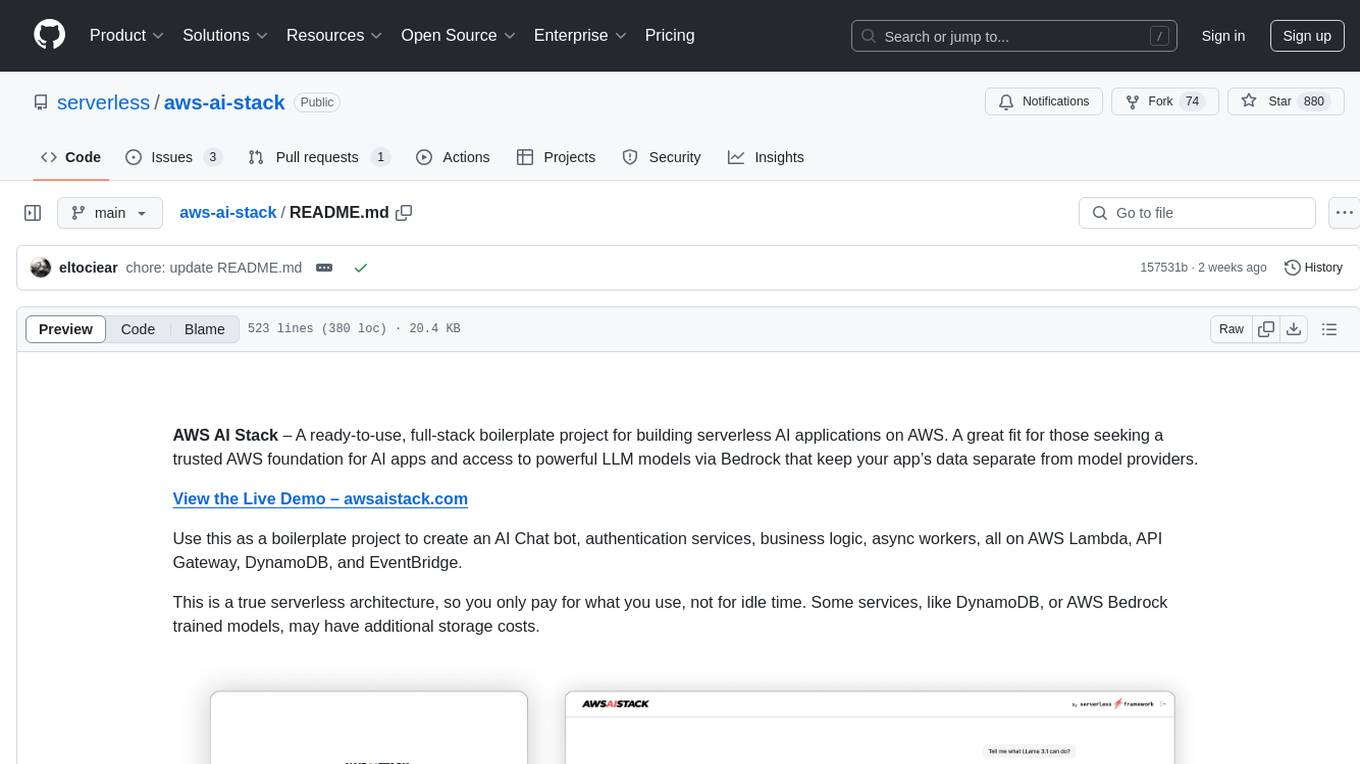
aws-ai-stack
AWS AI Stack is a full-stack boilerplate project designed for building serverless AI applications on AWS. It provides a trusted AWS foundation for AI apps with access to powerful LLM models via Bedrock. The architecture is serverless, ensuring cost-efficiency by only paying for usage. The project includes features like AI Chat & Streaming Responses, Multiple AI Models & Data Privacy, Custom Domain Names, API & Event-Driven architecture, Built-In Authentication, Multi-Environment support, and CI/CD with Github Actions. Users can easily create AI Chat bots, authentication services, business logic, and async workers using AWS Lambda, API Gateway, DynamoDB, and EventBridge.
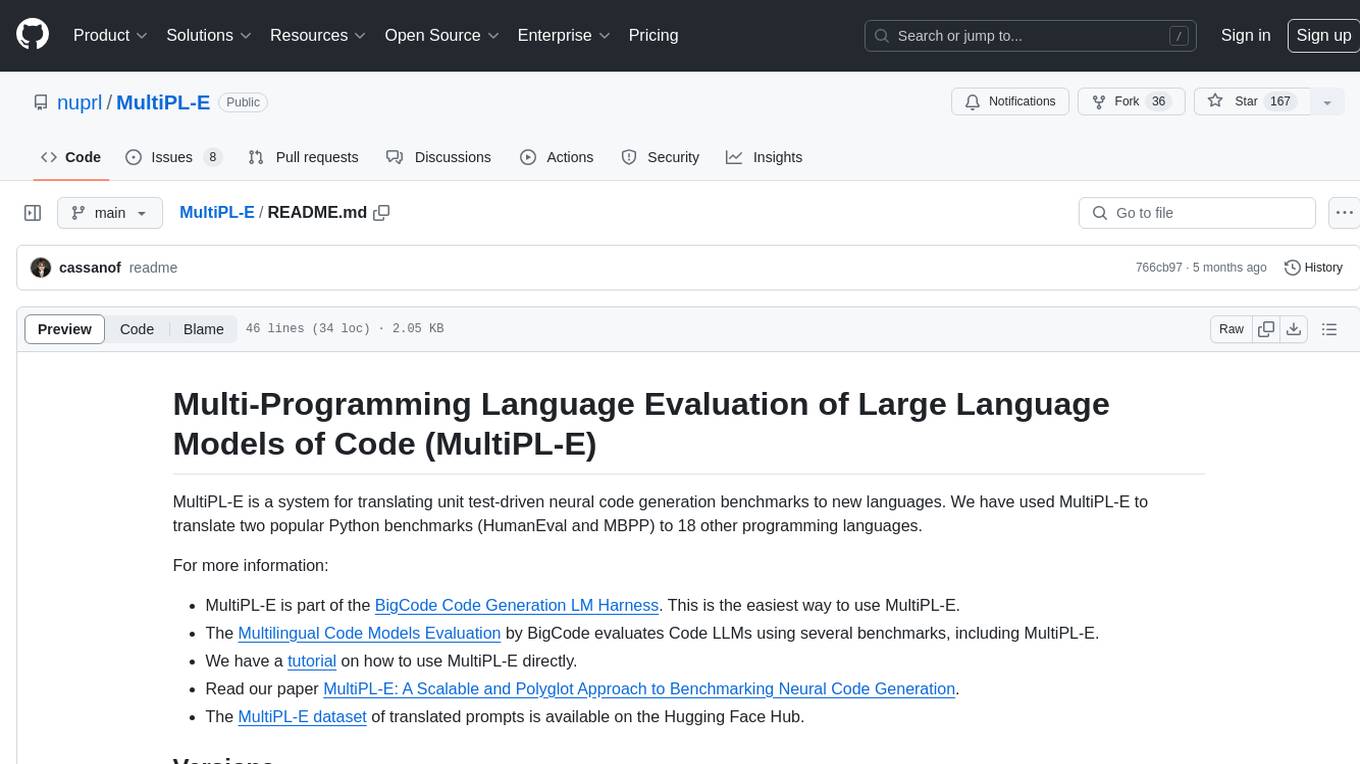
MultiPL-E
MultiPL-E is a system for translating unit test-driven neural code generation benchmarks to new languages. It is part of the BigCode Code Generation LM Harness and allows for evaluating Code LLMs using various benchmarks. The tool supports multiple versions with improvements and new language additions, providing a scalable and polyglot approach to benchmarking neural code generation. Users can access a tutorial for direct usage and explore the dataset of translated prompts on the Hugging Face Hub.
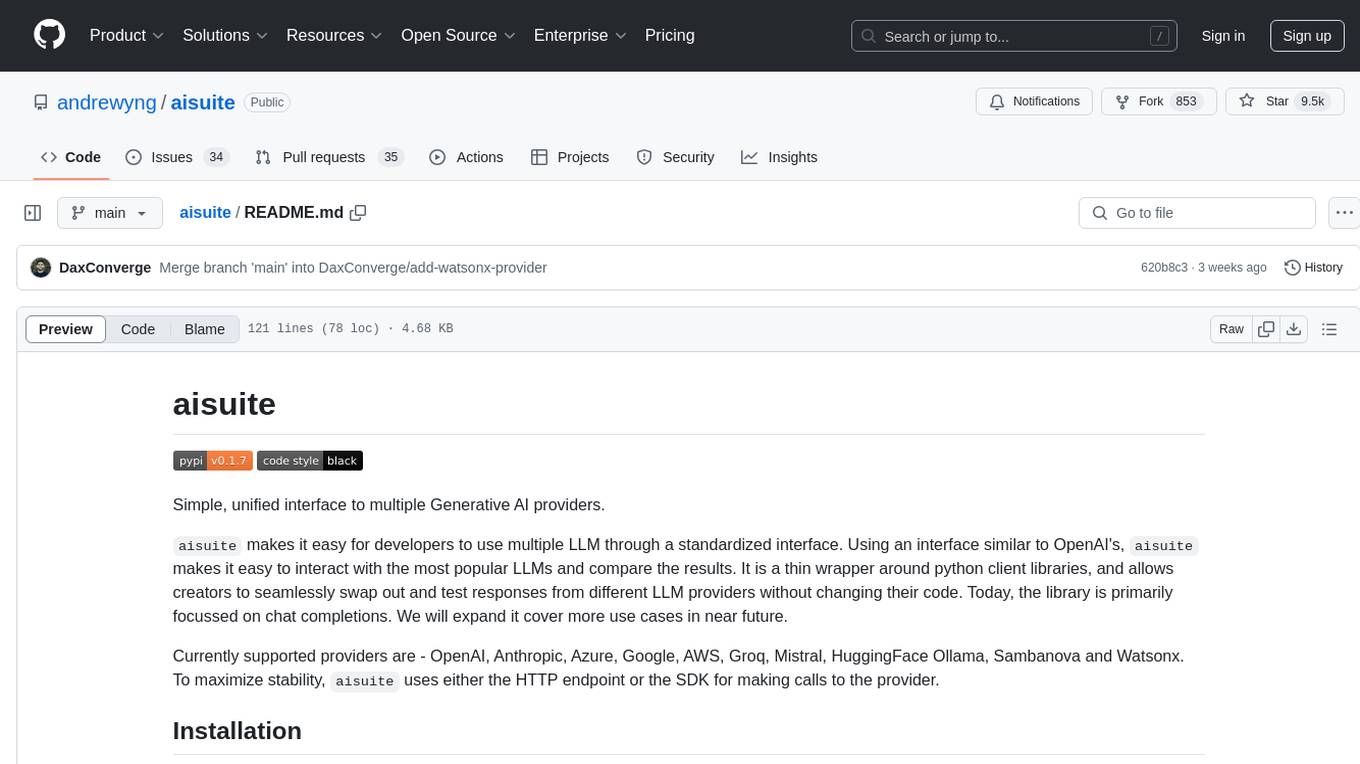
aisuite
Aisuite is a simple, unified interface to multiple Generative AI providers. It allows developers to easily interact with various Language Model (LLM) providers like OpenAI, Anthropic, Azure, Google, AWS, and more through a standardized interface. The library focuses on chat completions and provides a thin wrapper around python client libraries, enabling creators to test responses from different LLM providers without changing their code. Aisuite maximizes stability by using HTTP endpoints or SDKs for making calls to the providers. Users can install the base package or specific provider packages, set up API keys, and utilize the library to generate chat completion responses from different models.
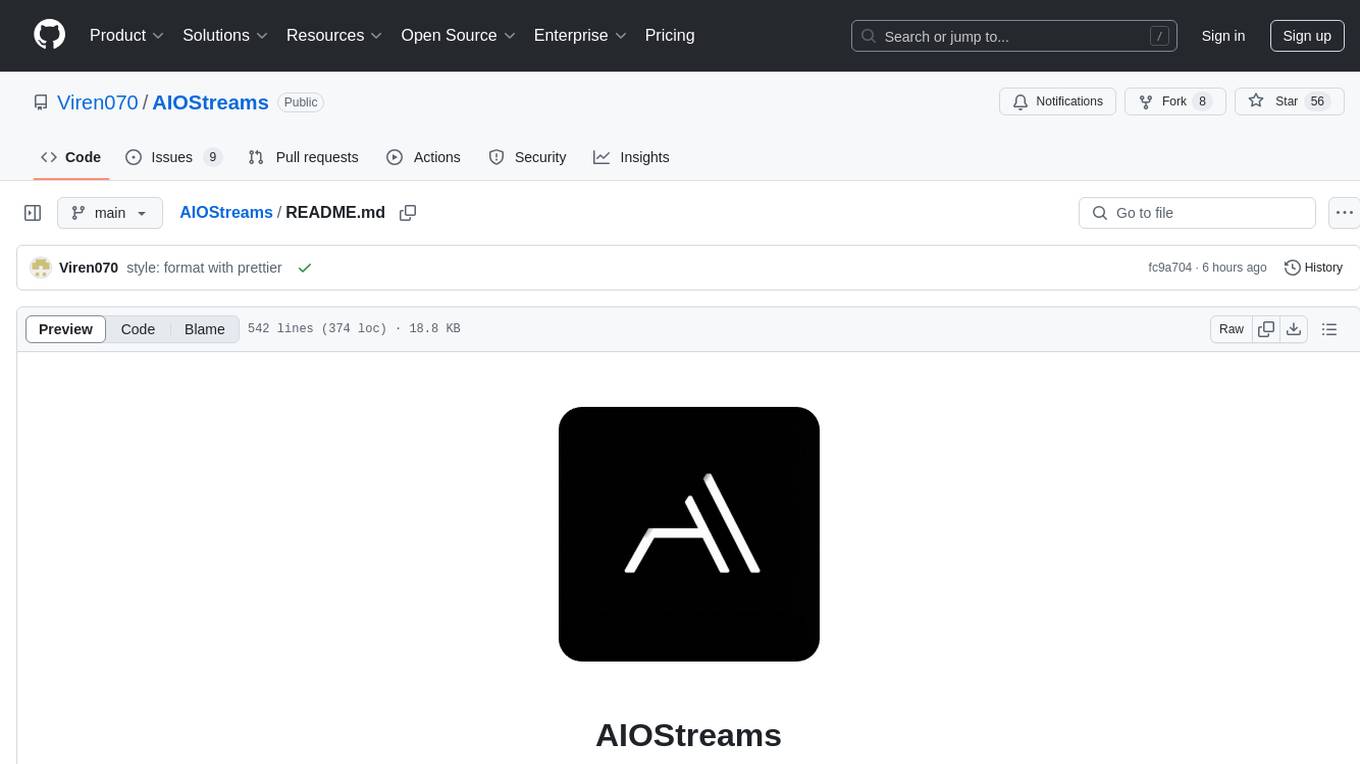
AIOStreams
AIOStreams is a versatile tool that combines streams from various addons into one platform, offering extensive customization options. Users can change result formats, filter results by various criteria, remove duplicates, prioritize services, sort results, specify size limits, and more. The tool scrapes results from selected addons, applies user configurations, and presents the results in a unified manner. It simplifies the process of finding and accessing desired content from multiple sources, enhancing user experience and efficiency.
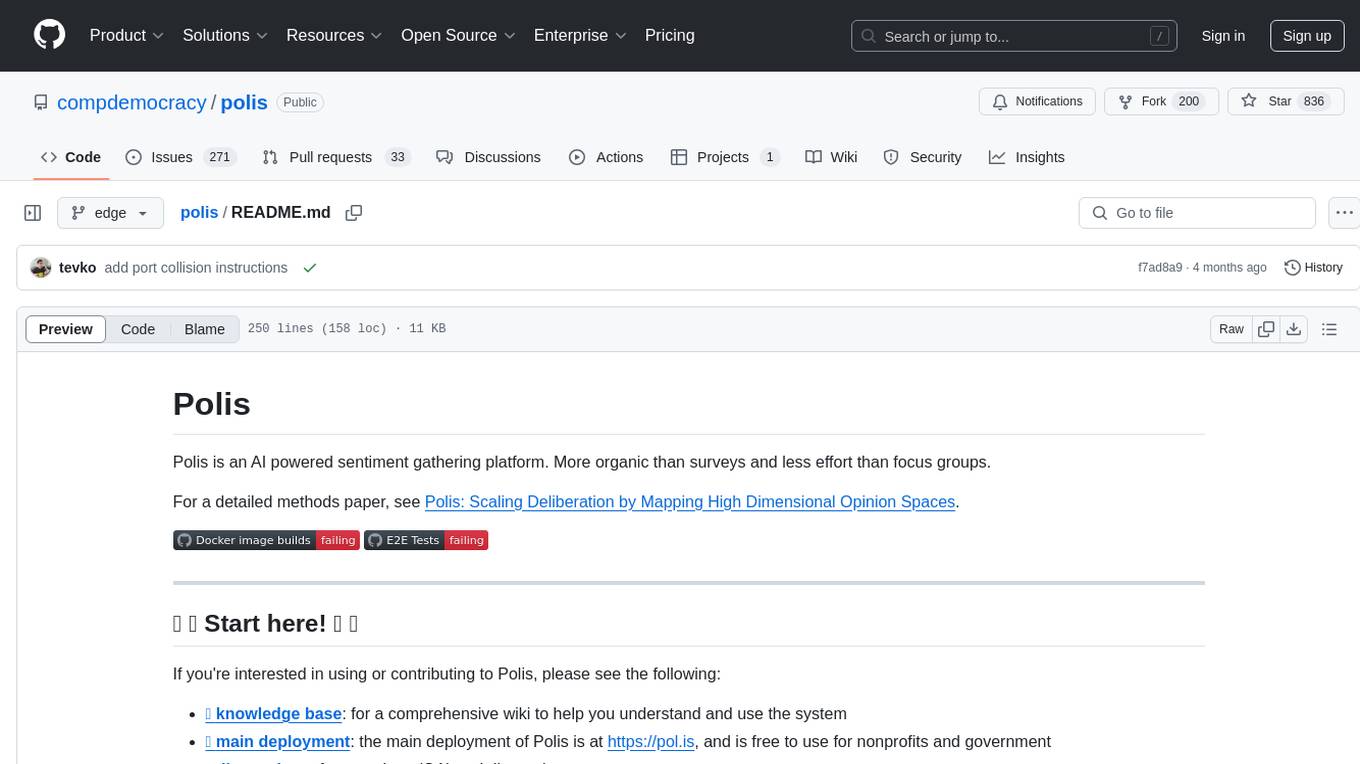
polis
Polis is an AI powered sentiment gathering platform that offers a more organic approach than surveys and requires less effort than focus groups. It provides a comprehensive wiki, main deployment at https://pol.is, discussions, issue tracking, and project board for users. Polis can be set up using Docker infrastructure and offers various commands for building and running containers. Users can test their instance, update the system, and deploy Polis for production. The tool also provides developer conveniences for code reloading, type checking, and database connections. Additionally, Polis supports end-to-end browser testing using Cypress and offers troubleshooting tips for common Docker and npm issues.
For similar tasks
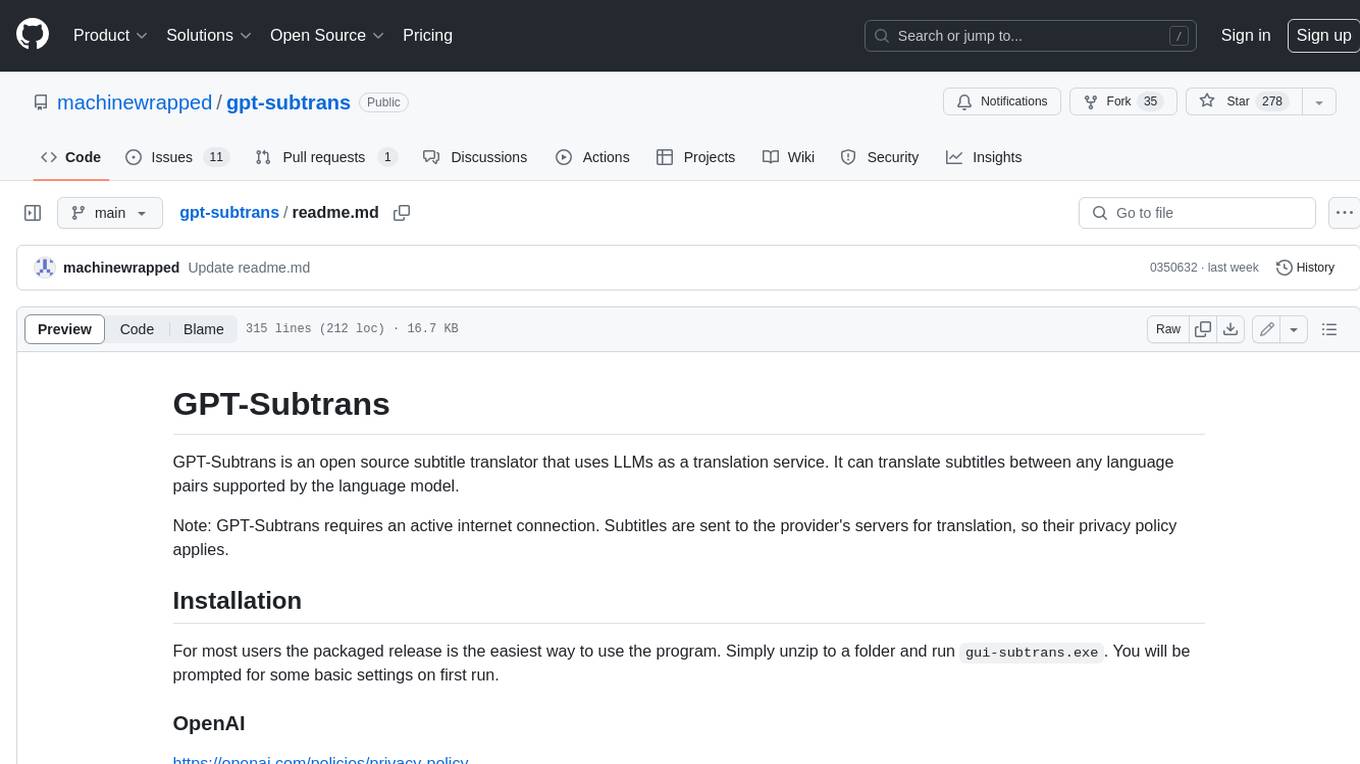
gpt-subtrans
GPT-Subtrans is an open-source subtitle translator that utilizes large language models (LLMs) as translation services. It supports translation between any language pairs that the language model supports. Note that GPT-Subtrans requires an active internet connection, as subtitles are sent to the provider's servers for translation, and their privacy policy applies.
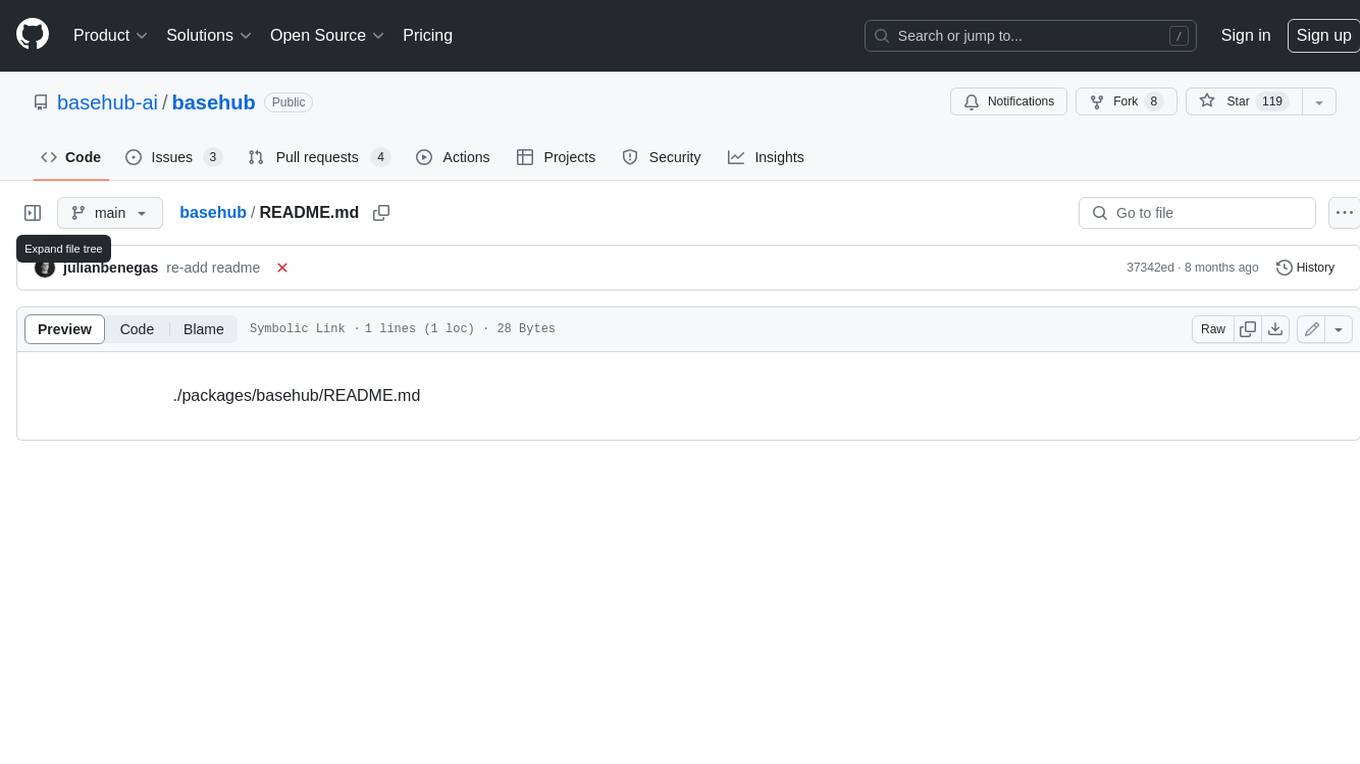
basehub
JavaScript / TypeScript SDK for BaseHub, the first AI-native content hub. **Features:** * ✨ Infers types from your BaseHub repository... _meaning IDE autocompletion works great._ * 🏎️ No dependency on graphql... _meaning your bundle is more lightweight._ * 🌐 Works everywhere `fetch` is supported... _meaning you can use it anywhere._
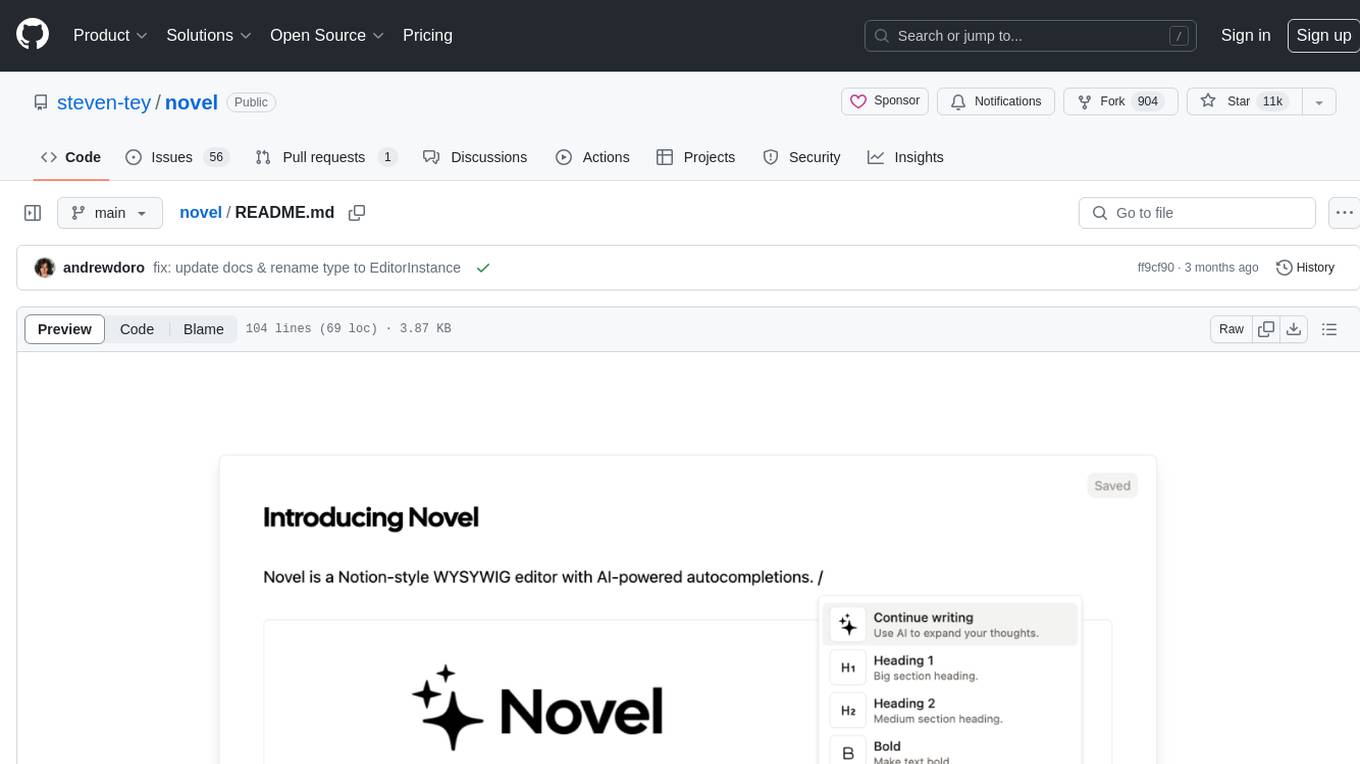
novel
Novel is an open-source Notion-style WYSIWYG editor with AI-powered autocompletions. It allows users to easily create and edit content with the help of AI suggestions. The tool is built on a modern tech stack and supports cross-framework development. Users can deploy their own version of Novel to Vercel with one click and contribute to the project by reporting bugs or making feature enhancements through pull requests.
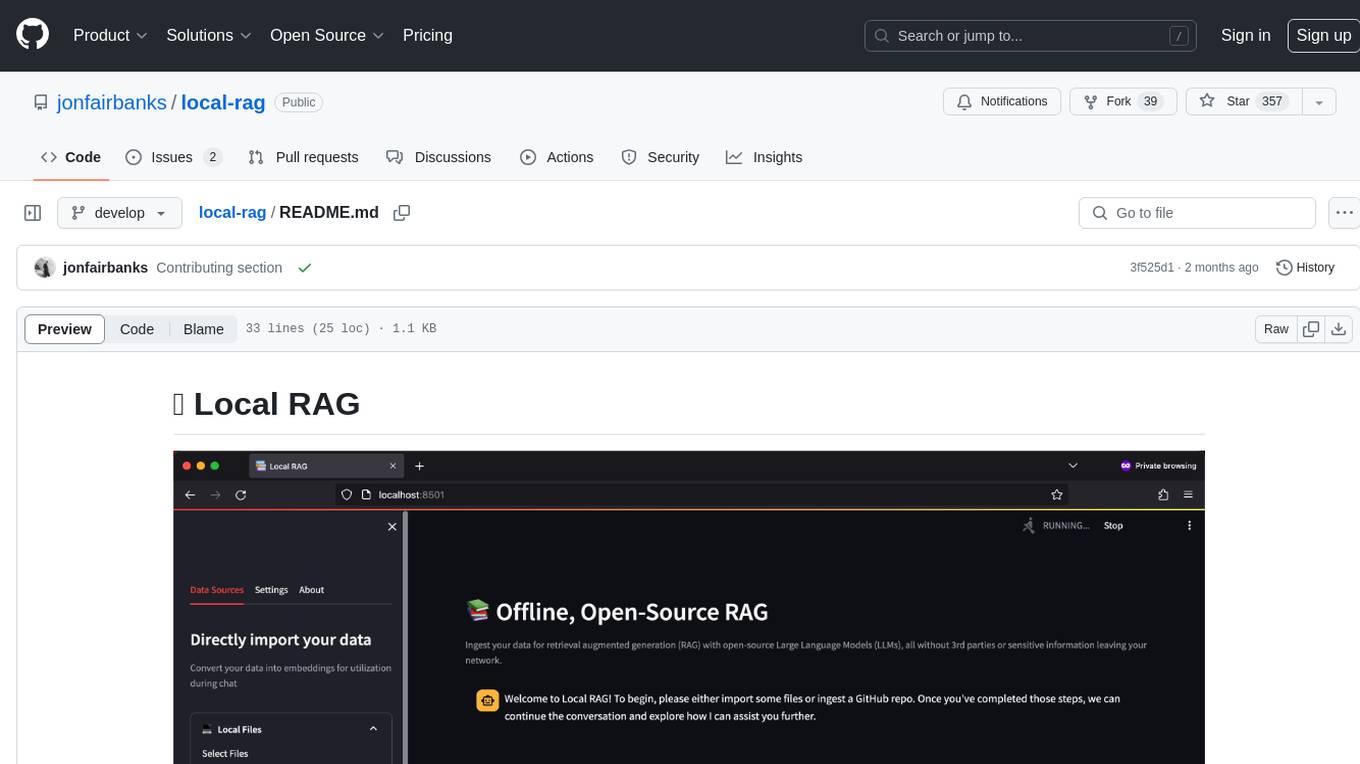
local-rag
Local RAG is an offline, open-source tool that allows users to ingest files for retrieval augmented generation (RAG) using large language models (LLMs) without relying on third parties or exposing sensitive data. It supports offline embeddings and LLMs, multiple sources including local files, GitHub repos, and websites, streaming responses, conversational memory, and chat export. Users can set up and deploy the app, learn how to use Local RAG, explore the RAG pipeline, check planned features, known bugs and issues, access additional resources, and contribute to the project.
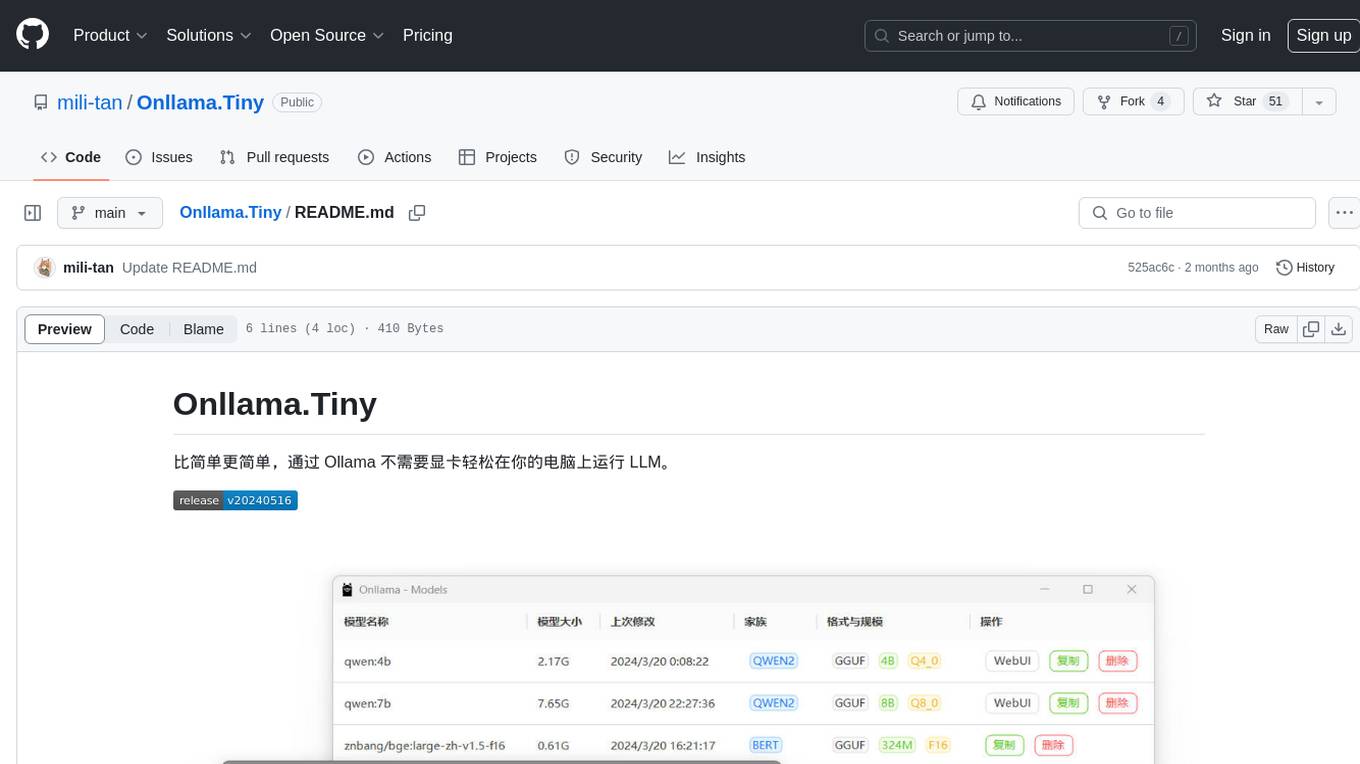
Onllama.Tiny
Onllama.Tiny is a lightweight tool that allows you to easily run LLM on your computer without the need for a dedicated graphics card. It simplifies the process of running LLM, making it more accessible for users. The tool provides a user-friendly interface and streamlines the setup and configuration required to run LLM on your machine. With Onllama.Tiny, users can quickly set up and start using LLM for various applications and projects.
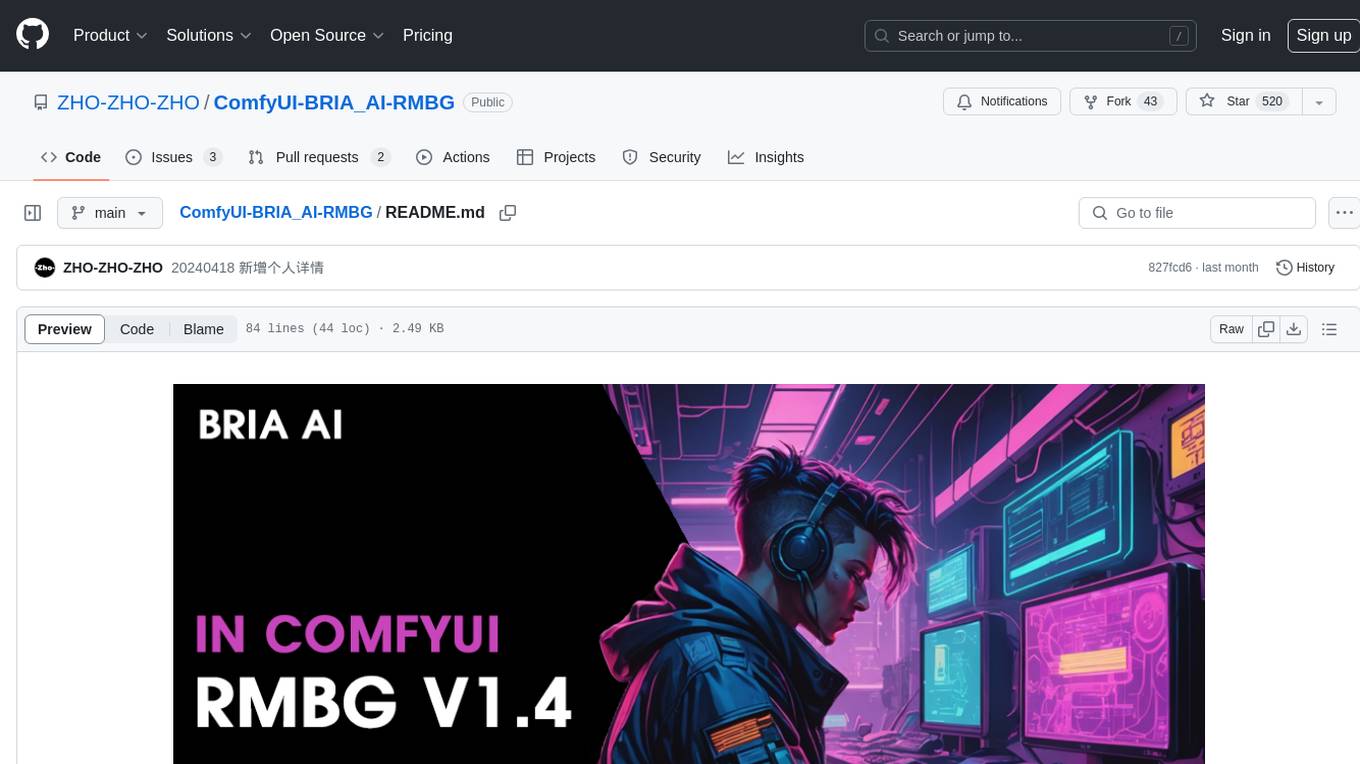
ComfyUI-BRIA_AI-RMBG
ComfyUI-BRIA_AI-RMBG is an unofficial implementation of the BRIA Background Removal v1.4 model for ComfyUI. The tool supports batch processing, including video background removal, and introduces a new mask output feature. Users can install the tool using ComfyUI Manager or manually by cloning the repository. The tool includes nodes for automatically loading the Removal v1.4 model and removing backgrounds. Updates include support for batch processing and the addition of a mask output feature.
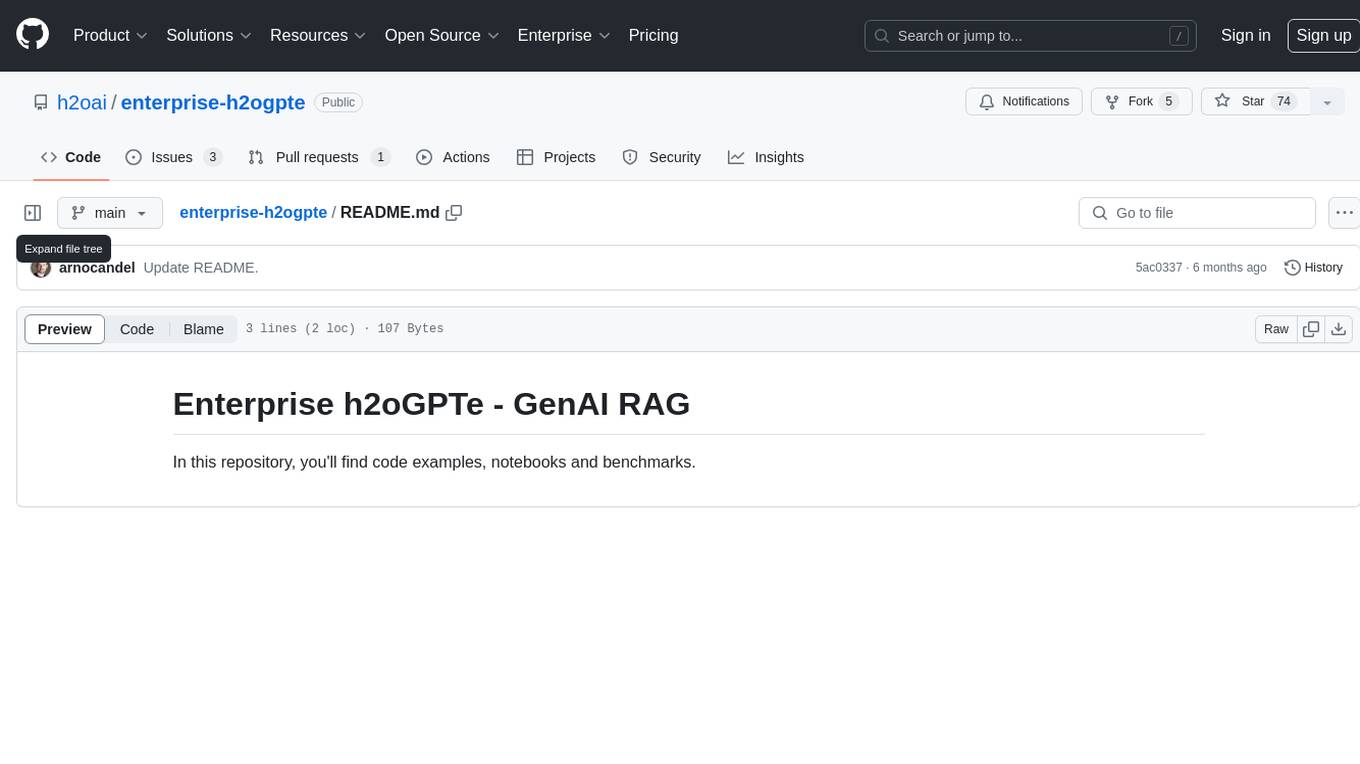
enterprise-h2ogpte
Enterprise h2oGPTe - GenAI RAG is a repository containing code examples, notebooks, and benchmarks for the enterprise version of h2oGPTe, a powerful AI tool for generating text based on the RAG (Retrieval-Augmented Generation) architecture. The repository provides resources for leveraging h2oGPTe in enterprise settings, including implementation guides, performance evaluations, and best practices. Users can explore various applications of h2oGPTe in natural language processing tasks, such as text generation, content creation, and conversational AI.
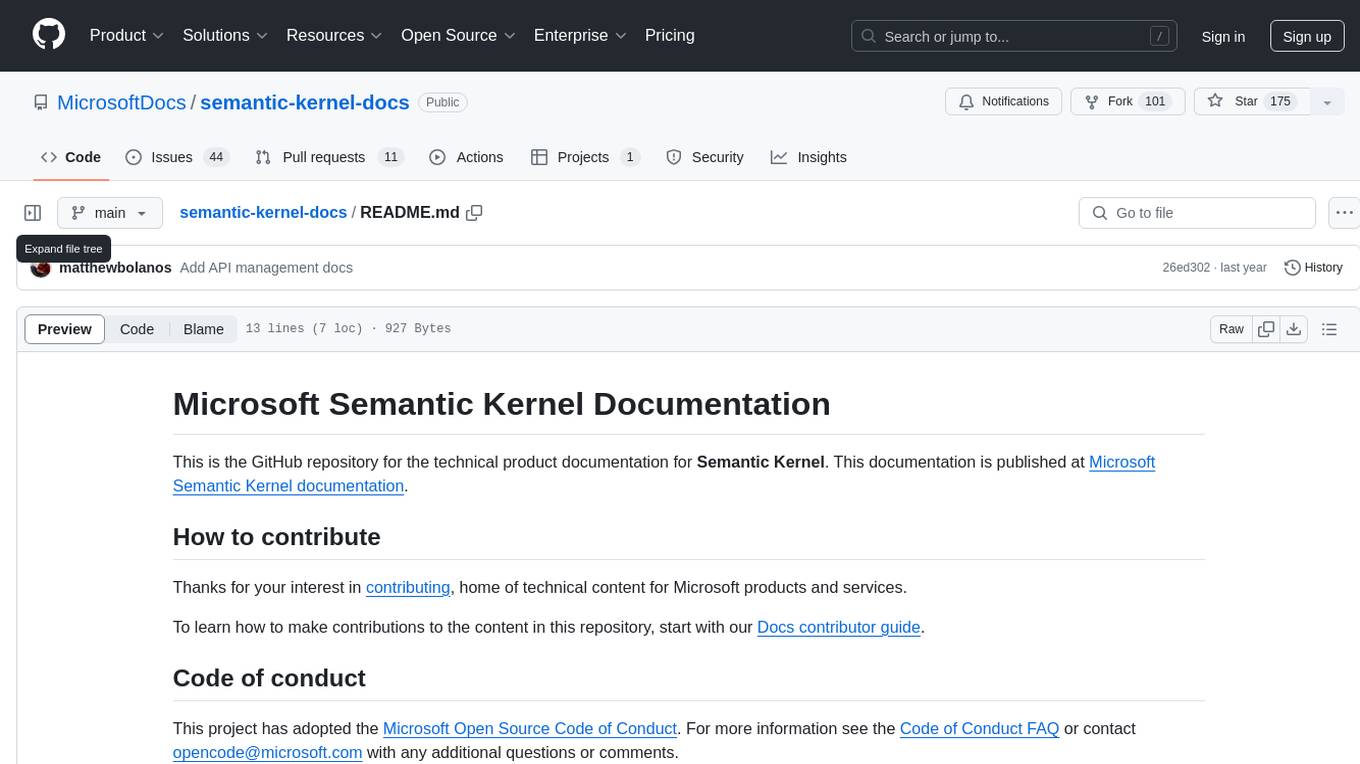
semantic-kernel-docs
The Microsoft Semantic Kernel Documentation GitHub repository contains technical product documentation for Semantic Kernel. It serves as the home of technical content for Microsoft products and services. Contributors can learn how to make contributions by following the Docs contributor guide. The project follows the Microsoft Open Source Code of Conduct.
For similar jobs
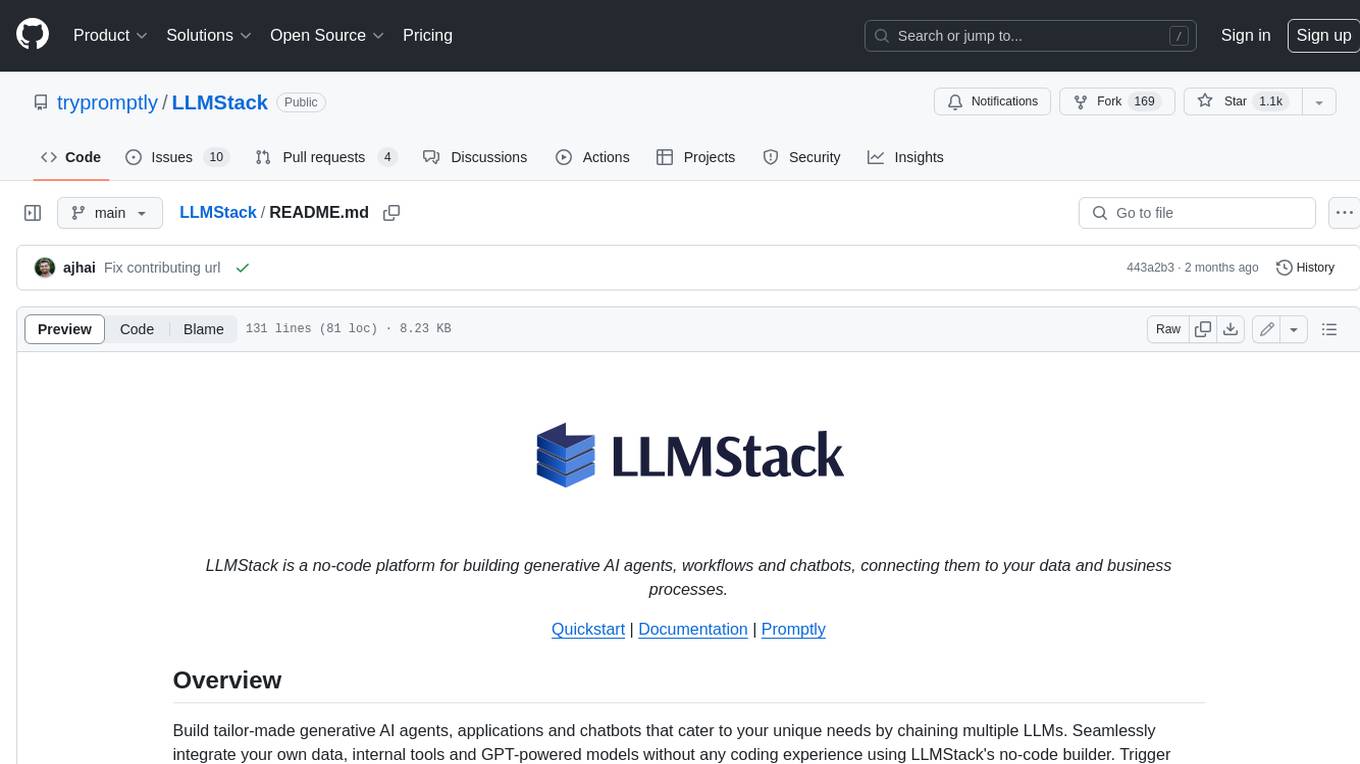
LLMStack
LLMStack is a no-code platform for building generative AI agents, workflows, and chatbots. It allows users to connect their own data, internal tools, and GPT-powered models without any coding experience. LLMStack can be deployed to the cloud or on-premise and can be accessed via HTTP API or triggered from Slack or Discord.
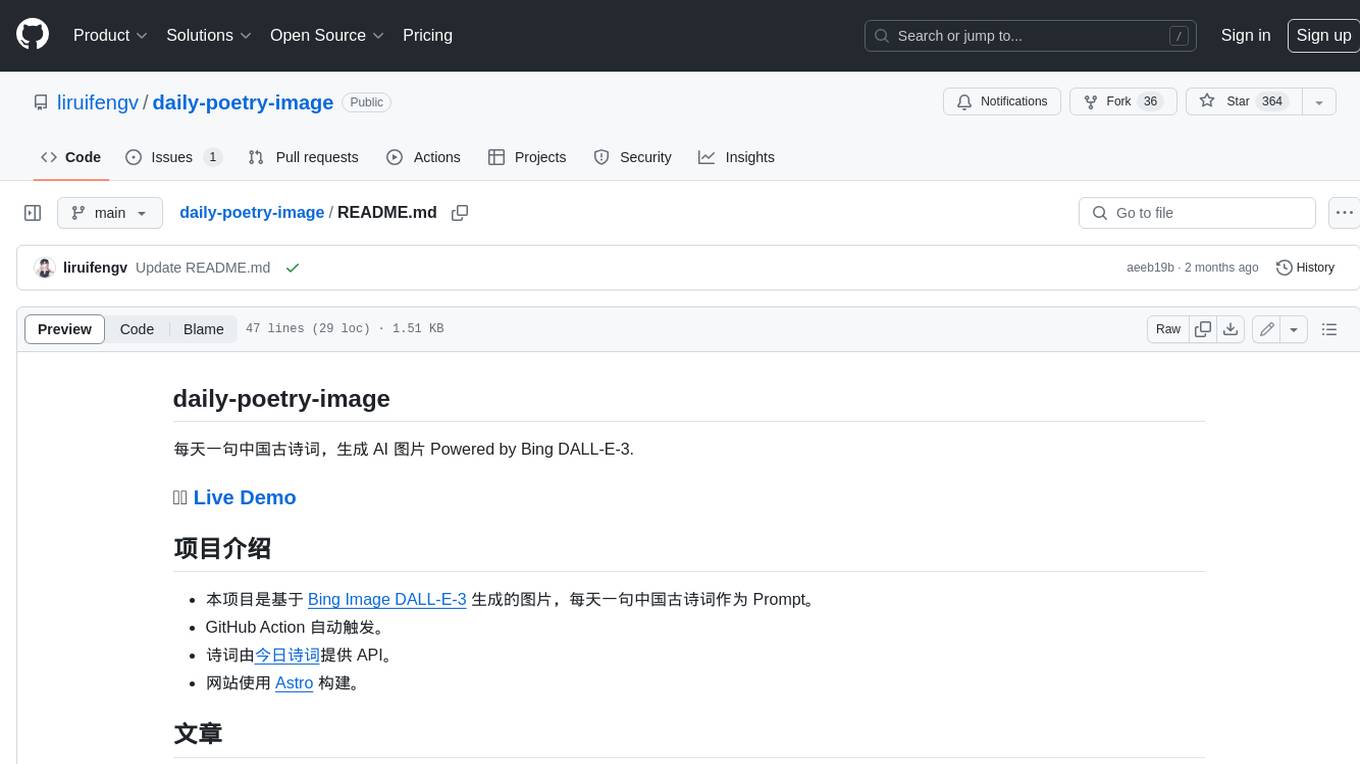
daily-poetry-image
Daily Chinese ancient poetry and AI-generated images powered by Bing DALL-E-3. GitHub Action triggers the process automatically. Poetry is provided by Today's Poem API. The website is built with Astro.
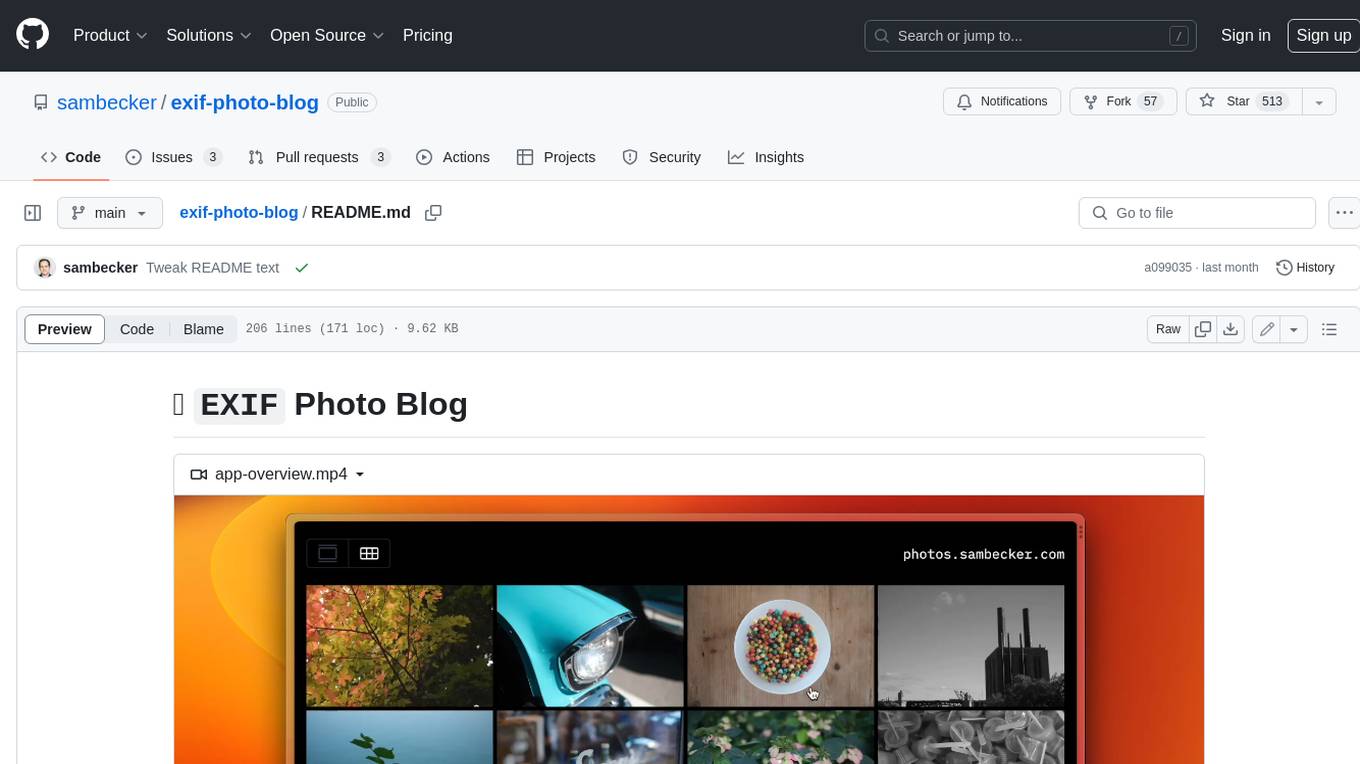
exif-photo-blog
EXIF Photo Blog is a full-stack photo blog application built with Next.js, Vercel, and Postgres. It features built-in authentication, photo upload with EXIF extraction, photo organization by tag, infinite scroll, light/dark mode, automatic OG image generation, a CMD-K menu with photo search, experimental support for AI-generated descriptions, and support for Fujifilm simulations. The application is easy to deploy to Vercel with just a few clicks and can be customized with a variety of environment variables.
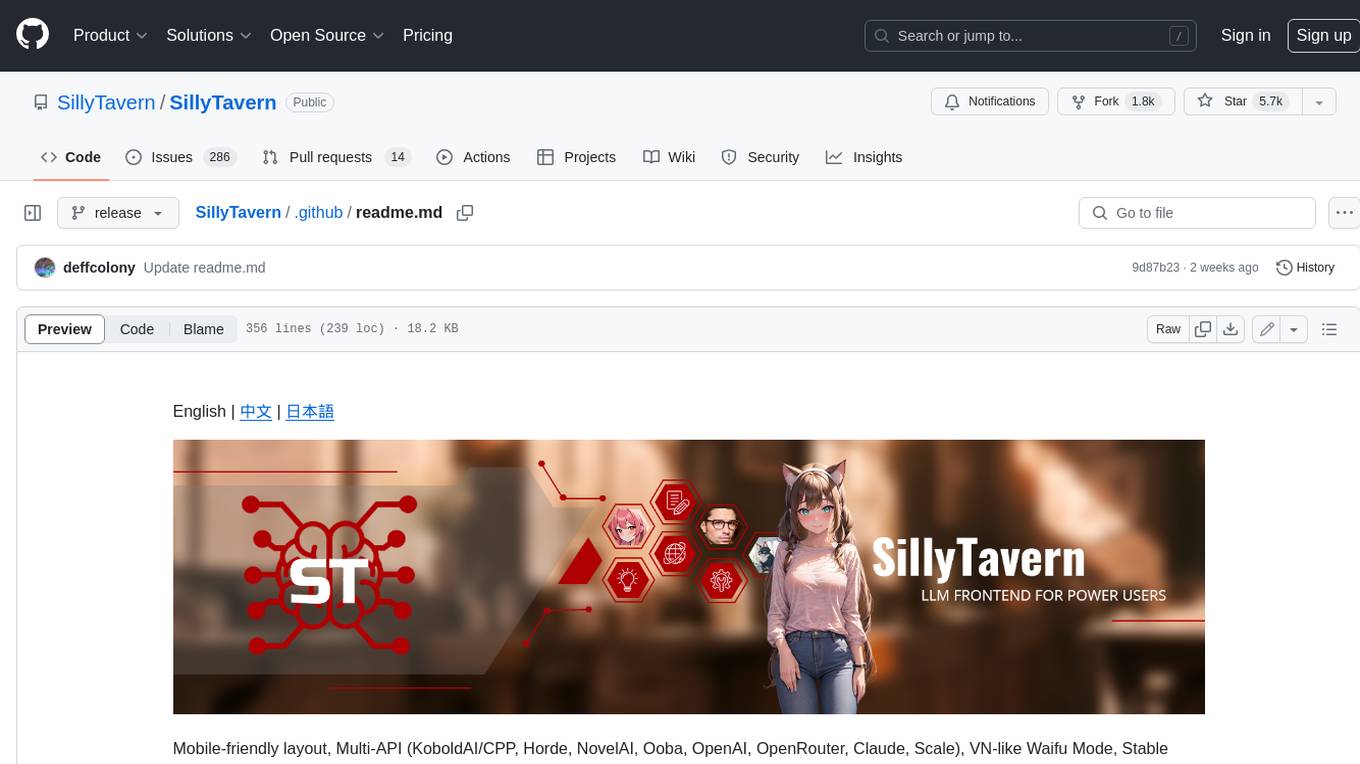
SillyTavern
SillyTavern is a user interface you can install on your computer (and Android phones) that allows you to interact with text generation AIs and chat/roleplay with characters you or the community create. SillyTavern is a fork of TavernAI 1.2.8 which is under more active development and has added many major features. At this point, they can be thought of as completely independent programs.
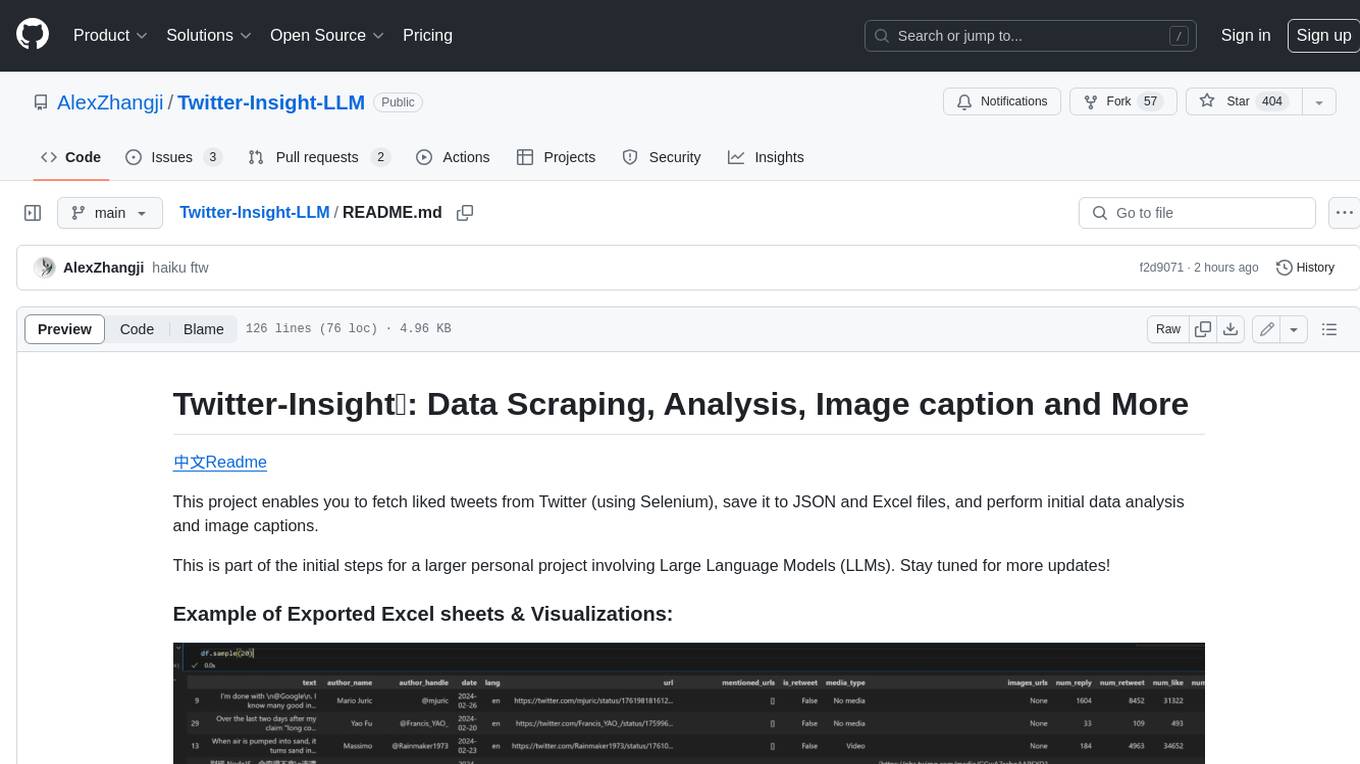
Twitter-Insight-LLM
This project enables you to fetch liked tweets from Twitter (using Selenium), save it to JSON and Excel files, and perform initial data analysis and image captions. This is part of the initial steps for a larger personal project involving Large Language Models (LLMs).
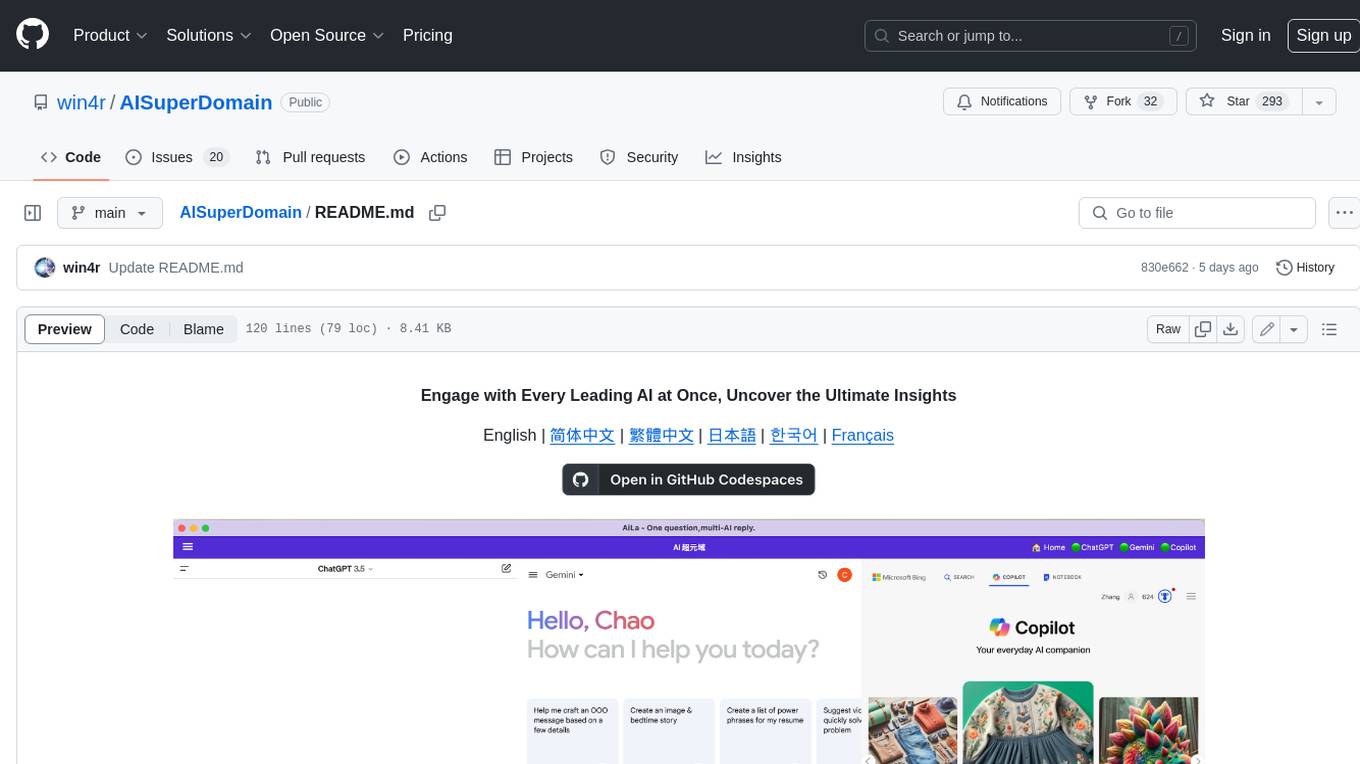
AISuperDomain
Aila Desktop Application is a powerful tool that integrates multiple leading AI models into a single desktop application. It allows users to interact with various AI models simultaneously, providing diverse responses and insights to their inquiries. With its user-friendly interface and customizable features, Aila empowers users to engage with AI seamlessly and efficiently. Whether you're a researcher, student, or professional, Aila can enhance your AI interactions and streamline your workflow.
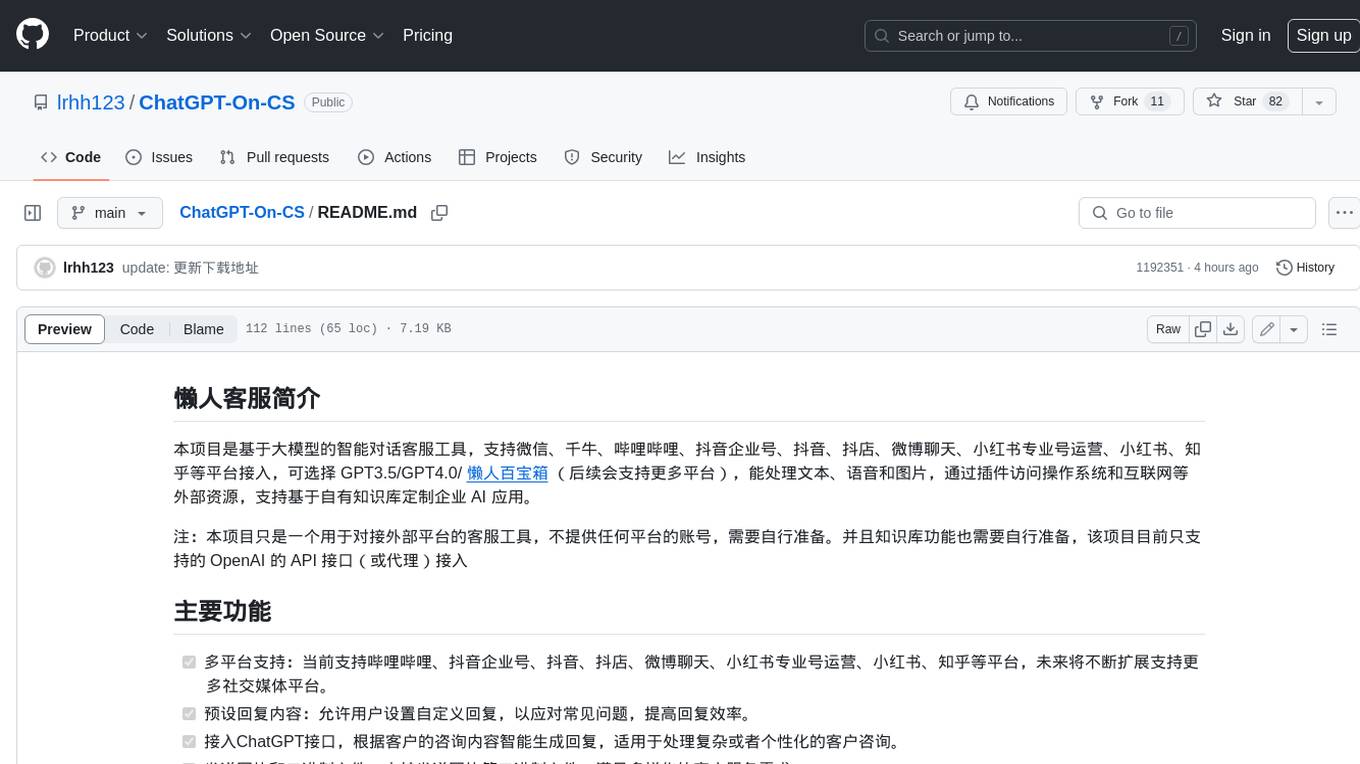
ChatGPT-On-CS
This project is an intelligent dialogue customer service tool based on a large model, which supports access to platforms such as WeChat, Qianniu, Bilibili, Douyin Enterprise, Douyin, Doudian, Weibo chat, Xiaohongshu professional account operation, Xiaohongshu, Zhihu, etc. You can choose GPT3.5/GPT4.0/ Lazy Treasure Box (more platforms will be supported in the future), which can process text, voice and pictures, and access external resources such as operating systems and the Internet through plug-ins, and support enterprise AI applications customized based on their own knowledge base.
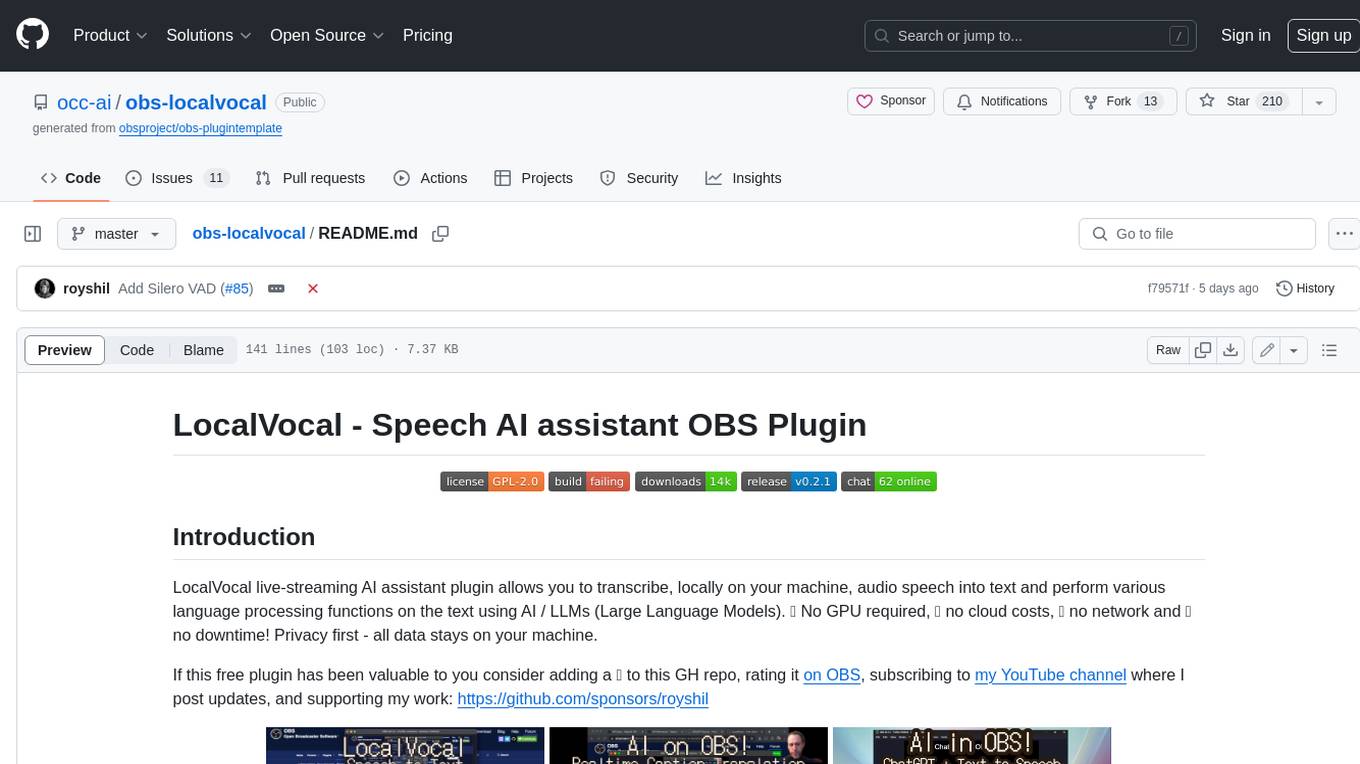
obs-localvocal
LocalVocal is a live-streaming AI assistant plugin for OBS that allows you to transcribe audio speech into text and perform various language processing functions on the text using AI / LLMs (Large Language Models). It's privacy-first, with all data staying on your machine, and requires no GPU, cloud costs, network, or downtime.