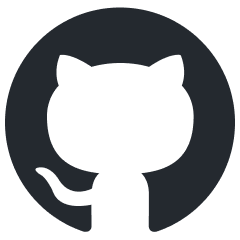
aws-ai-stack
AWS AI Stack – A ready-to-use, full-stack boilerplate project for building serverless AI applications on AWS
Stars: 882
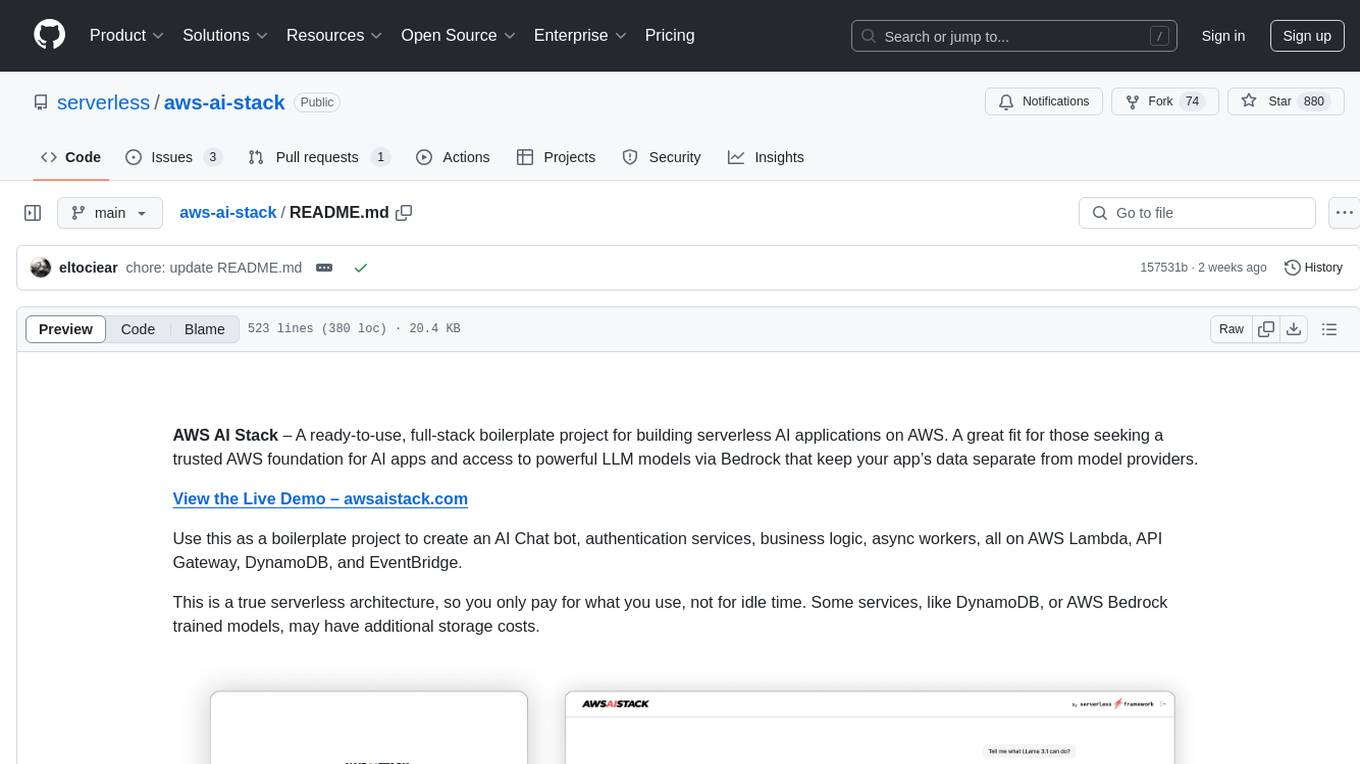
AWS AI Stack is a full-stack boilerplate project designed for building serverless AI applications on AWS. It provides a trusted AWS foundation for AI apps with access to powerful LLM models via Bedrock. The architecture is serverless, ensuring cost-efficiency by only paying for usage. The project includes features like AI Chat & Streaming Responses, Multiple AI Models & Data Privacy, Custom Domain Names, API & Event-Driven architecture, Built-In Authentication, Multi-Environment support, and CI/CD with Github Actions. Users can easily create AI Chat bots, authentication services, business logic, and async workers using AWS Lambda, API Gateway, DynamoDB, and EventBridge.
README:
AWS AI Stack – A ready-to-use, full-stack boilerplate project for building serverless AI applications on AWS. A great fit for those seeking a trusted AWS foundation for AI apps and access to powerful LLM models via Bedrock that keep your app’s data separate from model providers.
View the Live Demo – awsaistack.com
Use this as a boilerplate project to create an AI Chat bot, authentication services, business logic, async workers, all on AWS Lambda, API Gateway, DynamoDB, and EventBridge.
This is a true serverless architecture, so you only pay for what you use, not for idle time. Some services, like DynamoDB, or AWS Bedrock trained models, may have additional storage costs.
-
Full-Stack Application
- Backend: API (AWS API Gateway V2, AWS Lambda), Event-driven architecture (AWS Event-Bridge, AWS Lambda), Database (AWS DynamoDB), AI (AWS Bedrock)
- Frontend: Vanilla React app.
-
AI Chat & Streaming Responses
- Full serverless AI Chat architecture w/ streaming responses on AWS Lambda.
-
Multiple AI Models & Data Privacy
- Use one or multiple models via AWS Bedrock: Claude 3.5 Sonnet, Llama3.1, Mistral Large 2, and many more.
- App data never leaves AWS and is not sent to model providers.
-
100% Serverless
- This is a true serverless architecture. It auto-scales and you only pay when users use it. Some services may have additional storage costs.
-
Custom Domain Names
- Custom domain names for API Gateway services using the
serverless-domain-manager
plugin - Custom domain names for Lambda services using CloudFront Distributions
- Custom domain names for API Gateway services using the
-
API & Event-Driven
- Express.js API placeholder service for your business logic
- Shared EventBridge to public & subscribe to events
- Worker service to process events from EventBridge
-
Built-In Authentication
- API Gateway authorizer
- Login & Registration API on Lambda with Express.js
- DynamoDB table to store user information
- Shared library to provide JWT token authentication
- Frontend website that uses login & registration API
-
Multi-Environment
- Shared configuration for all services.
- Separated configuration for different environments.
-
Domain Oriented Architecture
- This project is domain-oriented so you can easily remove the pieces you don't need, like AI Chat, authentication, etc.
-
CI/CD with Github Action
- Github Actions to deploy the services to prod.
- Github Actions to deploy PRs & remove services after merge.
Install Serverless Framework
npm i -g serverless
Install NPM dependencies
This project is structured as a monorepo with multiple services. Each service
has its own package.json
file, so you must install the dependencies for each
service. Running npm install
in the root directory will install the
dependencies for all services.
npm install
Setup AWS Credentials
If you haven't already, setup your AWS Credentials. You can follow the AWS Credentials doc for step-by-step instructions.
This example requires the meta.llama3-70b-instruct-v1:0
AWS Bedrock
Model to be enabled. By default, AWS does not enable these models, you must go
to the AWS Console
and individually request access to the AI Models.
There is no cost to enable the models, but you must request access to use them.
Upon request, it may take a few minutes for AWS to enable the model. Once they are enabled, you will receive an email from AWS confirming the model is enabled.
Some users have reported issues with getting models enabled on AWS Bedrock. Make sure you have sufficient permissions in AWS to enable the models first. Often, AWS accounts that are new or have not historically had a monthly invoice over a few dollars may require contacting AWS to enable models.
Now you are ready to deploy the services. This will deploy all the services
to your AWS account. You can deploy the services to the default
stage, which
is the default stage for development.
Deploy the services
serverless deploy
At this point the service is live. When running the serverless deploy
command,
you will see the output of the services that were deployed. One of those
services is the web
service, which is the website service. To view the app,
go to the URL in the endpoint: ANY -
section for the web
service.
Deploying "web" to stage "dev" (us-east-1)
endpoint: ANY - https://ps5s7dd634.execute-api.us-east-1.amazonaws.com
functions:
app: web-dev-app (991 kB)
Once you start developing it is easier to run the service locally for faster iteration. We recommend using Serverless Dev Mode. You can run Dev Mode for individual services. This emulates Lambda locally and proxies requests to the real service.
serverless auth dev
Once done, you can redeploy individual services using the serverless
command
with the service name.
serverless auth deploy
The website
service is a static website that is served from an AWS Lambda
function. As such, it can run locally without needing to use Dev Mode. However,
it has a dependency on the AI Chat service and the Auth service, so you must
configure environment variables locally.
# If you have the jq CLI command installed you can use that with the --json flag
# on serverless info to get the URLs from the deployed services. If you do not
# have jq installed, you can get the URLs by running "serverless auth info" and
# "serverless ai-chat info" and copying the URLs manually into the environment
# variables.
export VITE_CHAT_API_URL=$(serverless aiChatApi info --json | jq -r '.outputs[] | select(.OutputKey == "ChatApiUrl") | .OutputValue')
export VITE_AUTH_API_URL=$(serverless auth info --json | jq -r '.outputs[] | select(.OutputKey == "AuthApiUrl") | .OutputValue')
# now you can run the local development server
cd website/app
npm run build
Now that the app is up and running in a development environment, lets get it ready for production by setting up a custom domain name, and setting a new shared secret for JWT token authentication.
This project is configured to use custom domain names. For non prod
deployments this is disabled. Deployments to prod
are designed to use a custom
domain name and require additional setup:
Register the domain name & create a Route53 hosted zone
If you haven't already, register a domain name, and create a Route53 hosted zone for the domain name.
https://us-east-1.console.aws.amazon.com/route53/v2/hostedzones?region=us-east-1#
Create a Certificate in AWS Certificate Manager
A Certificate is required in order to use SSL (https
) with a custom domain
name. AWS Certificate Manager (ACM) provides free SSL certificates for use with
your custom domain name. A certificate must first be requested, which requires
verification, and may take a few minutes.
https://us-east-1.console.aws.amazon.com/acm/home?region=us-east-1#/certificates/list
After you have created the certificate, you must validate the certificate by following the instructions in the AWS Console. This may require adding a CNAME record to your DNS provider.
This example uses a Certificate with the following full qualified domain names:
awsaistack.com
\*.awsaistack.com
The base domain name, awsaistack.com
is used for the website service
to host the static website. The wildcard domain name,
*.awsaistack.com
is used for the API services,
api.awsaistack.com
, and chat.awsaistack.com
.
Update serverless-compose.yml
- Update the
stages.prod.params.customDomainName
to your custom domain name. - Update the
stages.prod.params.customDomainCertificateARN
to the ARN of the certificate you created in ACM.
Authentication is implemented using JWT tokens. A shared secret is used to sign the JWT tokens when a user logs in. The secret is also used to verify the JWT tokens when a user makes a request to the API. It is important that this secret is kept secure and not shared.
In the serverless-compose.yml
file, you'll see that the sharedTokenSecret
is
set to "DEFAULT"
in the stages.default.params
section. This is a placeholder
value that is used when the secret is not provided in non-prod environments.
The prod
stage uses the ${ssm}
parameter to retrieve the secret from AWS
Systems Manager Parameter Store.
Generate a random secret and store it in the AWS Systems Manager Parameter Store
with a key like /serverless-ai-service/shared-token
, and set it in the
stages.prod.params.sharedTokenSecret
parameter in the serverless-compose.yml
file:
sharedTokenSecret: ${ssm:/serverless-ai-service/shared-token}
Once you've setup the custom domain name (optional), and created the secret, you are ready to deploy the service to prod.
serverless deploy --stage prod
Now you can use the service by visiting your domain name, or https://awsaistack.com. This uses the Auth service to login and register users, the AI Chat service to interact with the AI Chat bot.
This example uses serverless services like AWS Lambda, API Gateway, DynamoDB, EventBridge, and CloudFront. These services are designed to scale with usage, and you only pay for what you use. This means you do not pay for idle, and only pay for the resources you consume. If you have 0 usage, you will have $0 cost.
If you are using the custom domain names, it will require Route53 which has a fixed monthly cost.
This example uses Serverless Compose to share configuration across all services.
It defines the global parameters in the serverless-compose.yml
file under
stages.default.params
and stages.prod.params
. These parameters are used
across all services to provide shared configuration.
It also uses CloudFormation from services to set parameters on other services.
For example, the auth
service publishes the CloudFormation Output
AuthApiUrl
, which is used by the website service.
web:
path: ./website
params:
authApiUrl: ${auth.AuthApiUrl}
Using Serverless Compose also allows you to deploy all services with a single
command, serverless deploy
.
The auth
service contains a shared client library that is used by the other
services to validate the JWT token. This library is defined as an NPM package
and is used by the ai-chat-api
and business-api
services and included using
relative paths in the package.json
file.
The auth
service is an Express.js-based API service that provides login and
registration endpoints. It uses a DynamoDB table to store user information and
uses JWT tokens for authentication.
Upon login or registration, the service returns a JWT token. These APIs are used
by the website service to authenticate users. The token is stored in
localstorage and is used to authenticate requests to the ai-chat-api
and
business-api
services.
The ai-chat-api
service uses AWS Lambda Function URLs instead of API Gateway,
in order to support streaming responses. As such, it uses the Auth
class from
auth-sdk
to validate the JWT token, instead of using an API Gateway
authorizer.
The auth
service also publishes the CloudFormation Output AuthApiUrl
, which
is used by the website service to make requests to the auth
service.
In most cases APIs on AWS Lambda use the API Gateway to expose the API. However,
the ai-chat-api
service uses Lambda Function URLs instead of API Gateway, in
order to support streaming responses as streaming responses are not supported by
API Gateway.
Since the ai-chat-api
service does not use API Gateway, it does not support
custom domain names natively. Instead, it uses a CloudFront Distribution to
support a custom domain name.
To provide the AI Chat functionality, the service uses the AWS Bedrock Models service to interact with the AI Chat bot. The requests from the frontend (via the API) are sent to the AWS Bedrock Models service, and the streaming response from Bedrock is sent back to the frontend via the streaming response.
The AWS Bedrock AI Model is selected using the modelId
parameter in the
ai-chat-api/serverless.yml
file.
stages:
default:
params:
modelId: meta.llama3-70b-instruct-v1:0
The AI Chat service also implements a simple throttling schema to limit cost exposure when using AWS Bedrock. It implements a monthly limit for the number of requests per user and a global monthly limit for all users. It uses a DynamoDB Table to persist the request counts and other AI usage metrics.
The inline comments provider more details on this mechanism as well as ways to customize it to use other metrics, like token usage.
stages:
default:
params:
throttleMonthlyLimitUser: 10
throttleMonthlyLimitGlobal: 100
The website service is a simple Lambda function which uses Express to serve
static assets. The service uses the serverless-plugin-scripts
plugin to
run the npm run build
command to build the website before deploying.
The build command uses the parameters to set the REACT_APP_*
environment
variables, which are used in the React app to configure the API URLs.
The frontend website is built using React. It uses the auth
service to
login and register uses, and uses the ai-chat-api
to interact with the
AI Chat bot API.
This is an Express.js-based API service that provides a placeholder for your
business logic. It is configured to use the same custom domain name as the
auth
service, but with a different base path (/business
).
The endpoints are protected using the express-jwt
middleware, which uses the
JWT token provided by the auth
service to authenticate the user.
This is a placeholder function for your business logic for processing asynchronous events. It subscribes to events on the EventBridge and processes the events.
Currently this subscribes to the auth.register
event, which is published by
the auth
service when a user registers.
Both the Business Worker and the Auth service therefore depend on the
EventBridge which is provisioned in the event-bus
service.
The services which use API Gateway use the serverless-domain-manager
plugin to
setup the custom domain name. More details about the plugin can be found on the
serverless-domain-manager plugin page.
The api-ai-chat
service uses Lambda Function URLs instead of API Gateway, so
custom domain name is supported by creating a CloudFront Distribution with the
custom domain name and the Lambda Function URL as the origin.
The business-api
and auth
APIs both use the same custom domain name. Instead
of sharing an API Gateway, they are configured to use the same domain name
with different base paths, one for each service.
Below are a few simple API requests using the curl
command.
curl -X POST https://api.awsaistack.com/auth/register \
-H 'Content-Type: application/json' \
-d '{"email": "[email protected]", "password": "password"}'
curl -X POST https://api.awsaistack.com/auth/login \
-H 'Content-Type: application/json' \
-d '{"email": "[email protected]", "password": "password"}'
If you have jq
installed, you can wrap the login request in a command to set
the token as an environment variable so you can use the token in subsequent
requests.
export SERVERLESS_EXAMPLE_TOKEN=$(curl -X POST https://api.awsaistack.com/auth/login \
-H 'Content-Type: application/json' \
-d '{"email": "[email protected]", "password": "password"}' \
| jq -r '.token')
You can also use the Chat API directly; however, the response payload is a a stream of JSON objects containing the response and other metadata. Each buffer may also contain multiple JSON objects.
This endpoint is authenticated and requires the JWT token from the login API.
curl -N -X POST https://chat.awsaistack.com/ \
-H 'Content-Type: application/json' \
-H "Authorization: Bearer $SERVERLESS_EXAMPLE_TOKEN" \
-d '[{"role":"user","content":[{"text":"What makes the serverless framework so great?"}]}]'
This endpoint is also authenticated and requires the JWT token from the login API. The response is a simple message.
curl -X GET https://api.awsaistack.com/business/ \
-H 'Content-Type: application/json' \
-H "Authorization: Bearer $SERVERLESS_EXAMPLE_TOKEN"
The Chat API uses CloudFront Distributions to add support for custom domain names to the AWS Lambda Function URL, as it is not natively supported. The Auth & Business APIs on the other hand use API Gateway which supports custom domain names natively. However, an API Gateway and a CloudFront Distribution do not support using the same hostname as they both require a CNAME record.
For these two services to share the same domain name, consider using the CloudFront distribution to proxy the API Gateway requests. This would allow both services to use the same domain name, and would also allow the Chat API to use the same domain name as the other services.
In this configuration, the Auth and Business APIs use the paths /auth
and
/business
respectively on api.awsaistack.com
. The Custom Domain
Name Path Mapping was used in the Custom Domain Name support in API Gateway
to use the same domain name but shared across multiple API Gateway instances.
Alternatively, you can use a single API Gateway and map the paths to the respective services. This would allow you to use the same domain name for multiple services, and would also allow you to use the same authorizer for all the services. However, sharing an API Gateway instance may have performance implications at scale, which is why this example uses separate API Gateway instances for each service.
The auth
, business-api
, and chat-api
all validate the user input, and in
the case of chat-api
use Zod to validate the schema. Consider including
schema validation on all API requests using a library like Zod, and/or
Express.js middleware.
This example, for simplicity, hosts the static assets from an AWS Lambda Function. This is not recommended for production, and you should consider using a static website hosting service like S3 or CloudFront to host your website. Consider using one of the following plugins to deploy your website:
This example uses a custom authorization method using JWT tokens for the
ai-chat-api
service, which doesn't use API Gateway.
The business-api
is based on Express.js and uses the authMiddleware
method
in the auth-sdk
to validate the JWT token.
API Gateway supports Lambda Authorizers which can be used to validate JWT tokens
before the request is passed to the Lambda Function. This is a more robust
solution than the custom method used in this example, and should be considered
for production services. This method will not work for the ai-chat-api
service
as it does not use API Gateway.
Using Github Actions this example deploys all the services using Serverless
Compose. This ensures that any changes to the individual services or the
serverless-compose.yml
will reevaluate the interdependent parameters. However,
all services are redeployed on any change in the repo, which may not be
necessary.
Consider using a more fine-grained approach to deploying services, such as
only deploying the services that have changed by using the serverless <service> deploy
command.
For Tasks:
Click tags to check more tools for each tasksFor Jobs:
Alternative AI tools for aws-ai-stack
Similar Open Source Tools
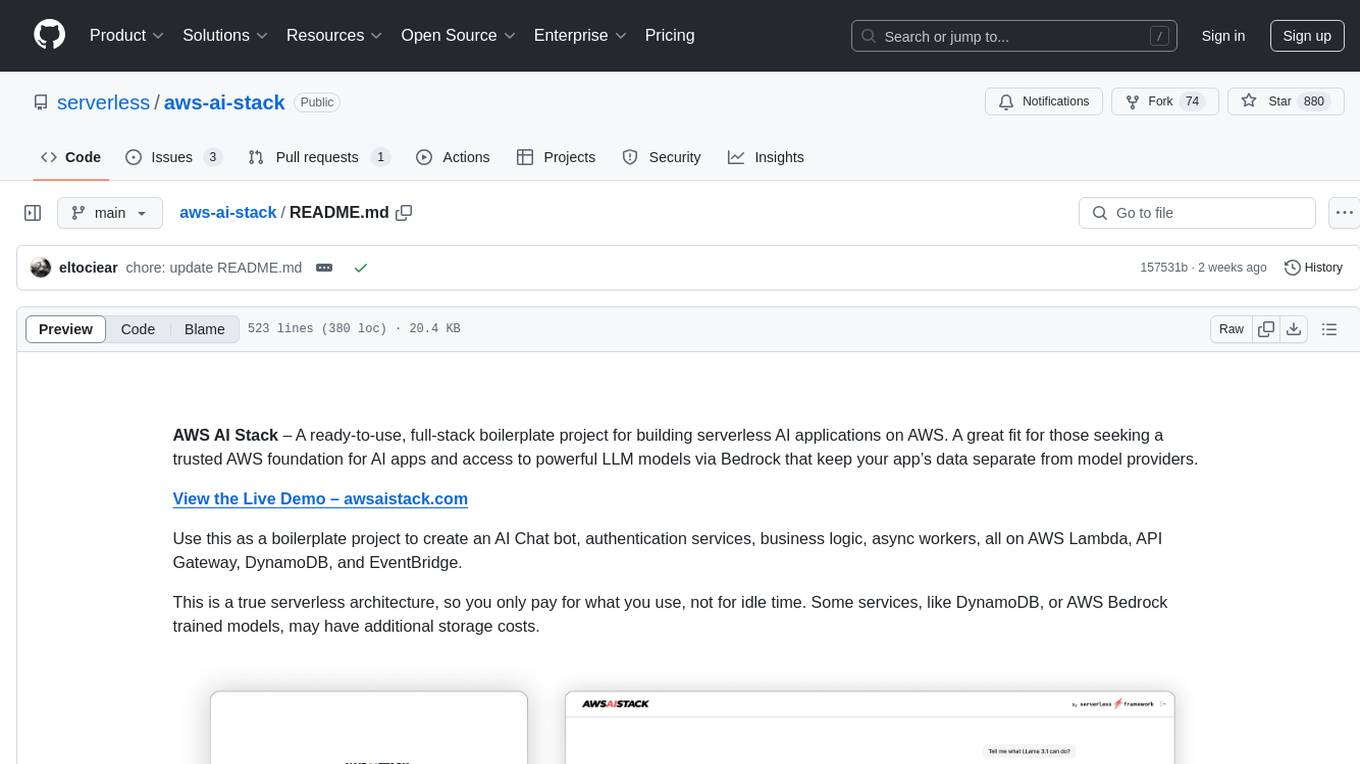
aws-ai-stack
AWS AI Stack is a full-stack boilerplate project designed for building serverless AI applications on AWS. It provides a trusted AWS foundation for AI apps with access to powerful LLM models via Bedrock. The architecture is serverless, ensuring cost-efficiency by only paying for usage. The project includes features like AI Chat & Streaming Responses, Multiple AI Models & Data Privacy, Custom Domain Names, API & Event-Driven architecture, Built-In Authentication, Multi-Environment support, and CI/CD with Github Actions. Users can easily create AI Chat bots, authentication services, business logic, and async workers using AWS Lambda, API Gateway, DynamoDB, and EventBridge.
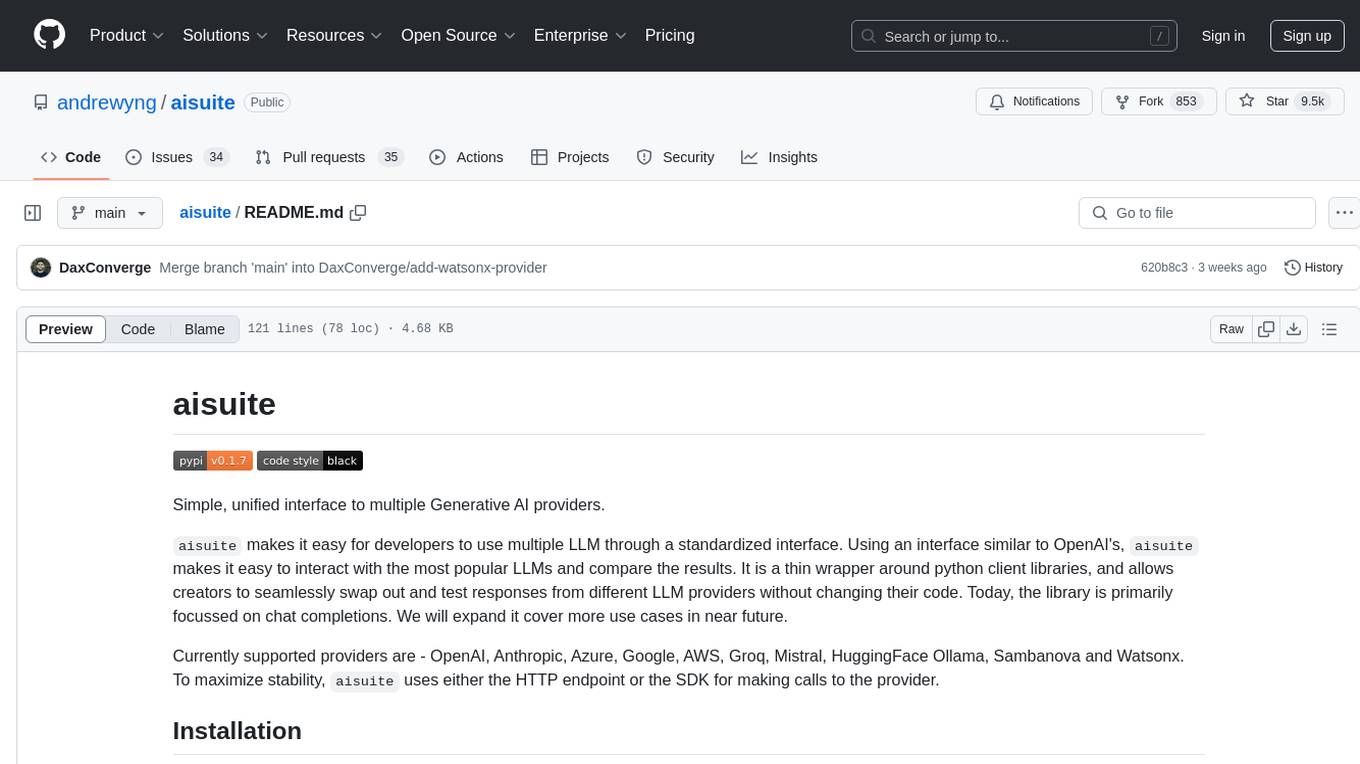
aisuite
Aisuite is a simple, unified interface to multiple Generative AI providers. It allows developers to easily interact with various Language Model (LLM) providers like OpenAI, Anthropic, Azure, Google, AWS, and more through a standardized interface. The library focuses on chat completions and provides a thin wrapper around python client libraries, enabling creators to test responses from different LLM providers without changing their code. Aisuite maximizes stability by using HTTP endpoints or SDKs for making calls to the providers. Users can install the base package or specific provider packages, set up API keys, and utilize the library to generate chat completion responses from different models.
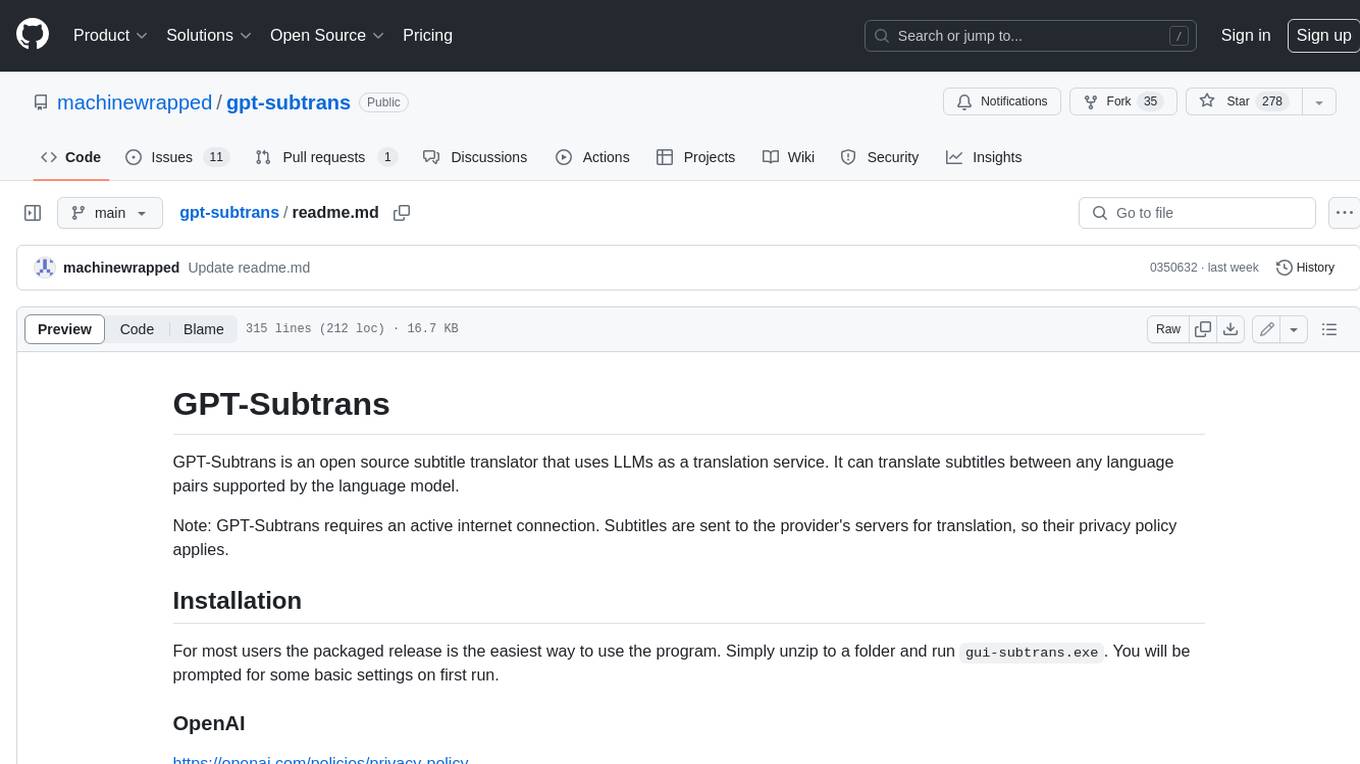
gpt-subtrans
GPT-Subtrans is an open-source subtitle translator that utilizes large language models (LLMs) as translation services. It supports translation between any language pairs that the language model supports. Note that GPT-Subtrans requires an active internet connection, as subtitles are sent to the provider's servers for translation, and their privacy policy applies.
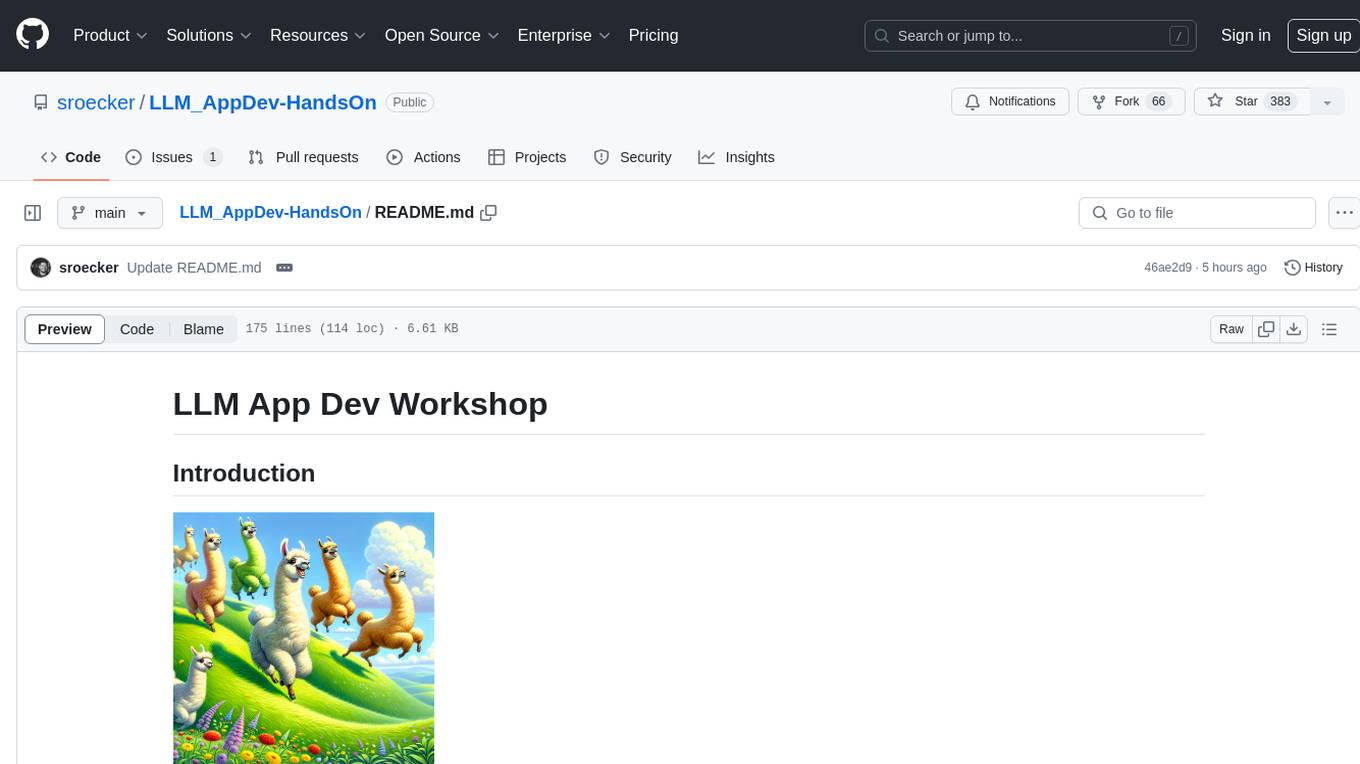
LLM_AppDev-HandsOn
This repository showcases how to build a simple LLM-based chatbot for answering questions based on documents using retrieval augmented generation (RAG) technique. It also provides guidance on deploying the chatbot using Podman or on the OpenShift Container Platform. The workshop associated with this repository introduces participants to LLMs & RAG concepts and demonstrates how to customize the chatbot for specific purposes. The software stack relies on open-source tools like streamlit, LlamaIndex, and local open LLMs via Ollama, making it accessible for GPU-constrained environments.
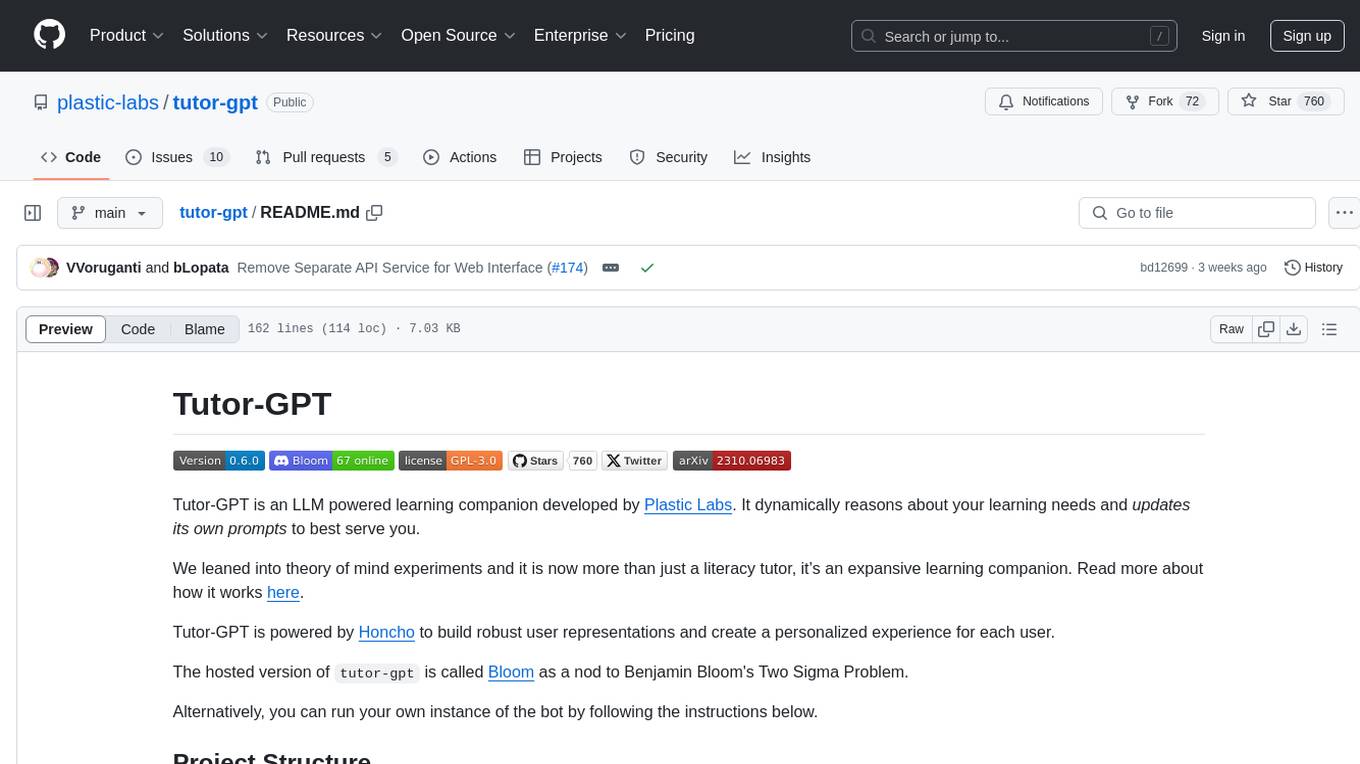
tutor-gpt
Tutor-GPT is an LLM powered learning companion developed by Plastic Labs. It dynamically reasons about your learning needs and updates its own prompts to best serve you. It is an expansive learning companion that uses theory of mind experiments to provide personalized learning experiences. The project is split into different modules for backend logic, including core logic, discord bot implementation, FastAPI API interface, NextJS web front end, common utilities, and SQL scripts for setting up local supabase. Tutor-GPT is powered by Honcho to build robust user representations and create personalized experiences for each user. Users can run their own instance of the bot by following the provided instructions.
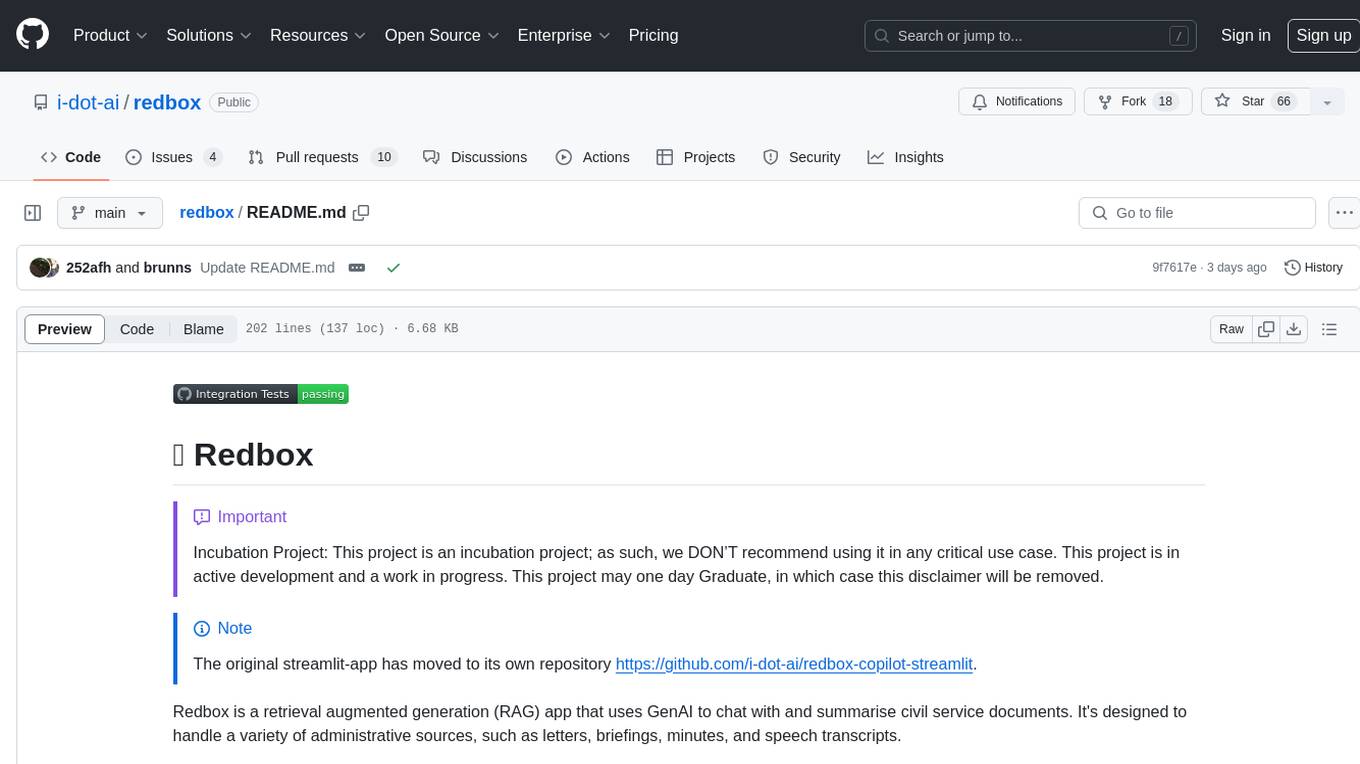
redbox
Redbox is a retrieval augmented generation (RAG) app that uses GenAI to chat with and summarise civil service documents. It increases organisational memory by indexing documents and can summarise reports read months ago, supplement them with current work, and produce a first draft that lets civil servants focus on what they do best. The project uses a microservice architecture with each microservice running in its own container defined by a Dockerfile. Dependencies are managed using Python Poetry. Contributions are welcome, and the project is licensed under the MIT License. Security measures are in place to ensure user data privacy and considerations are being made to make the core-api secure.
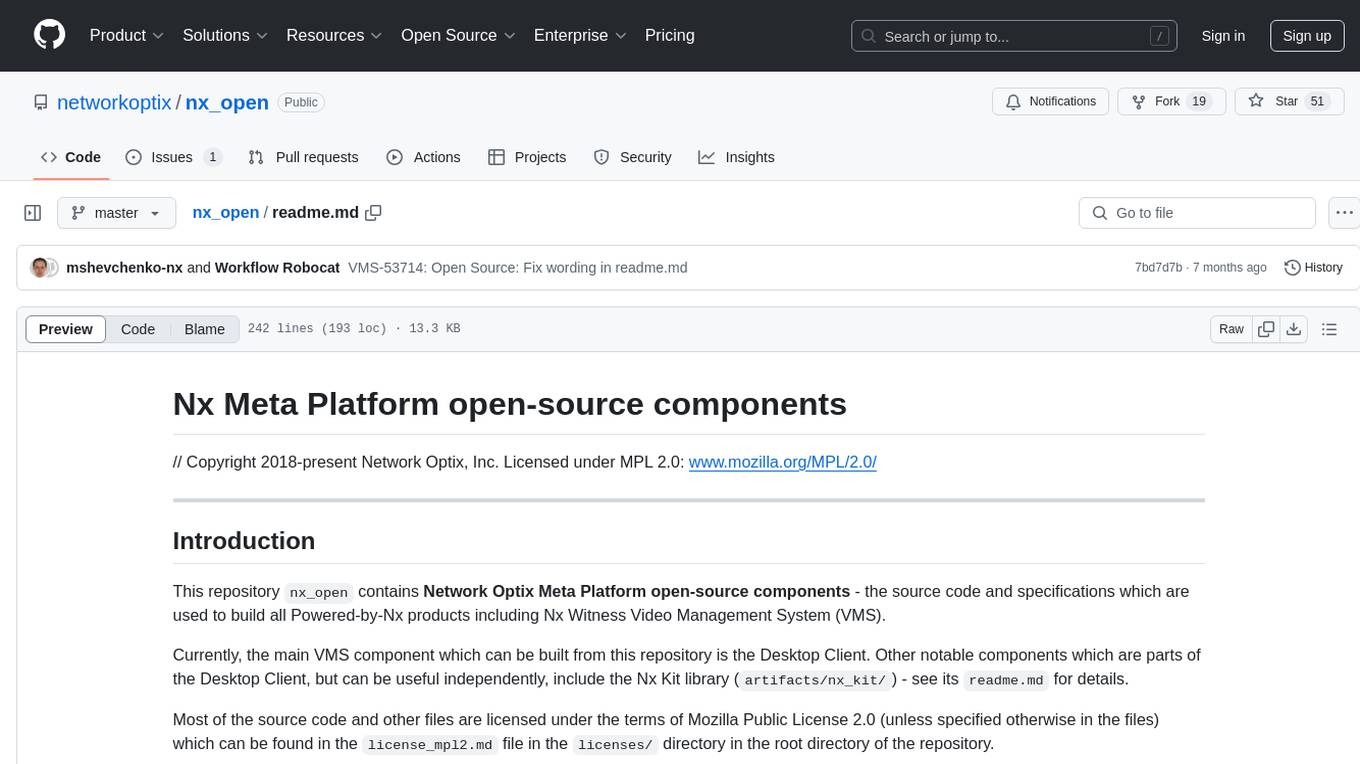
nx_open
The `nx_open` repository contains open-source components for the Network Optix Meta Platform, used to build products like Nx Witness Video Management System. It includes source code, specifications, and a Desktop Client. The repository is licensed under Mozilla Public License 2.0. Users can build the Desktop Client and customize it using a zip file. The build environment supports Windows, Linux, and macOS platforms with specific prerequisites. The repository provides scripts for building, signing executable files, and running the Desktop Client. Compatibility with VMS Server versions is crucial, and automatic VMS updates are disabled for the open-source Desktop Client.
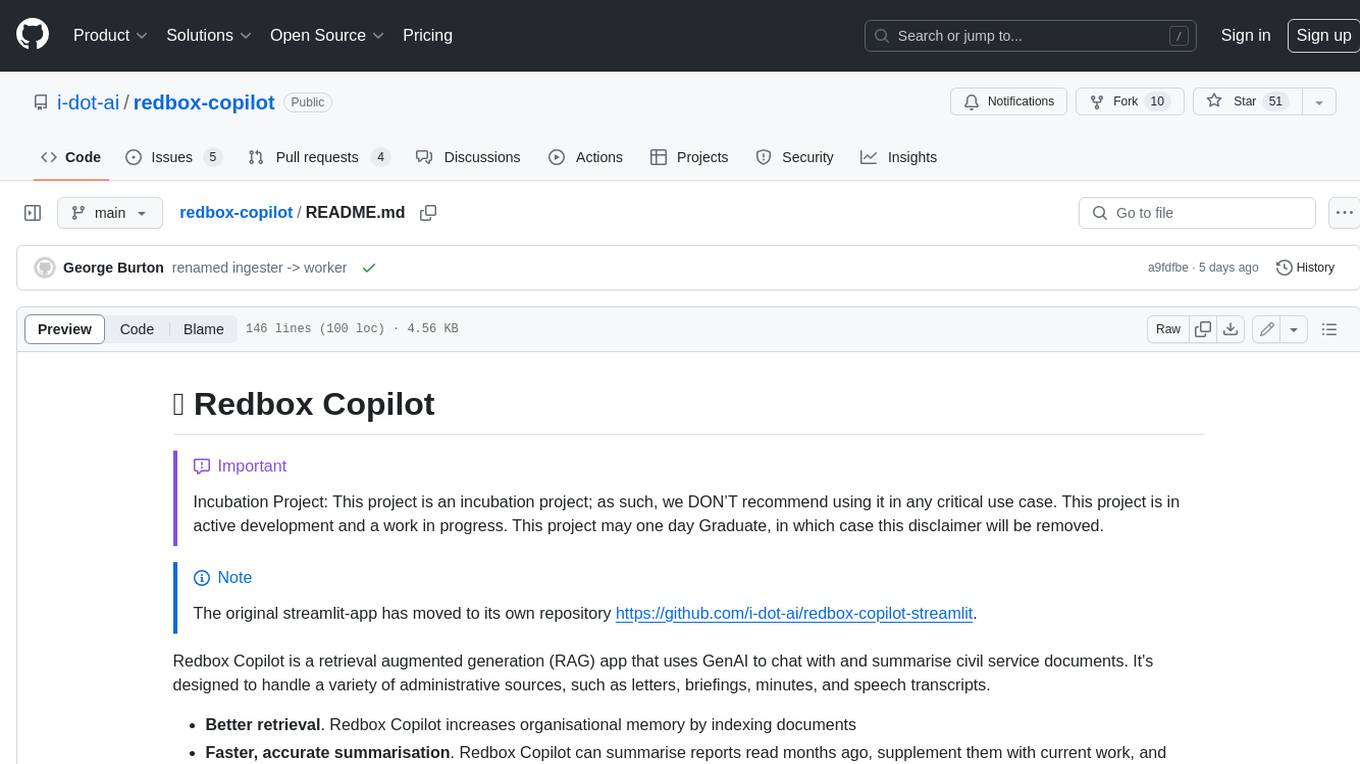
redbox-copilot
Redbox Copilot is a retrieval augmented generation (RAG) app that uses GenAI to chat with and summarise civil service documents. It increases organisational memory by indexing documents and can summarise reports read months ago, supplement them with current work, and produce a first draft that lets civil servants focus on what they do best. The project uses a microservice architecture with each microservice running in its own container defined by a Dockerfile. Dependencies are managed using Python Poetry. Contributions are welcome, and the project is licensed under the MIT License.
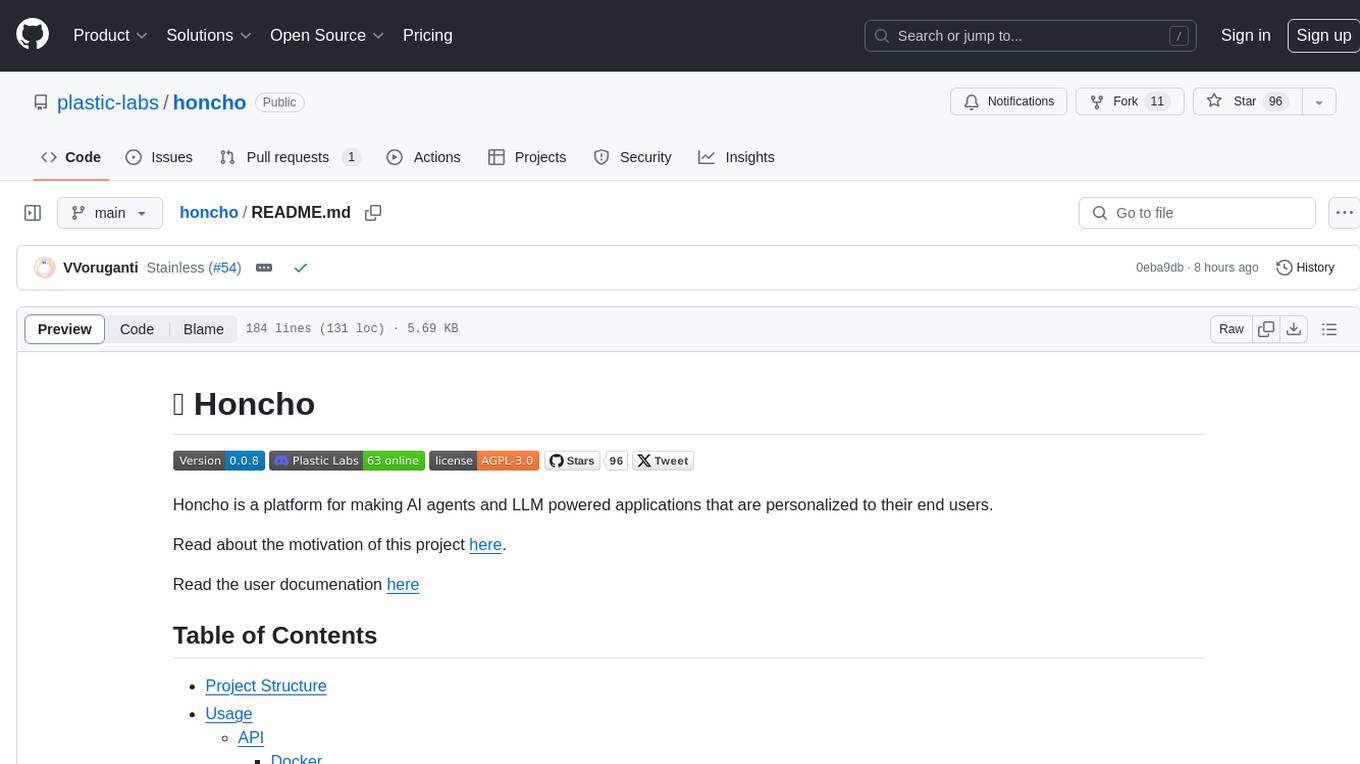
honcho
Honcho is a platform for creating personalized AI agents and LLM powered applications for end users. The repository is a monorepo containing the server/API for managing database interactions and storing application state, along with a Python SDK. It utilizes FastAPI for user context management and Poetry for dependency management. The API can be run using Docker or manually by setting environment variables. The client SDK can be installed using pip or Poetry. The project is open source and welcomes contributions, following a fork and PR workflow. Honcho is licensed under the AGPL-3.0 License.
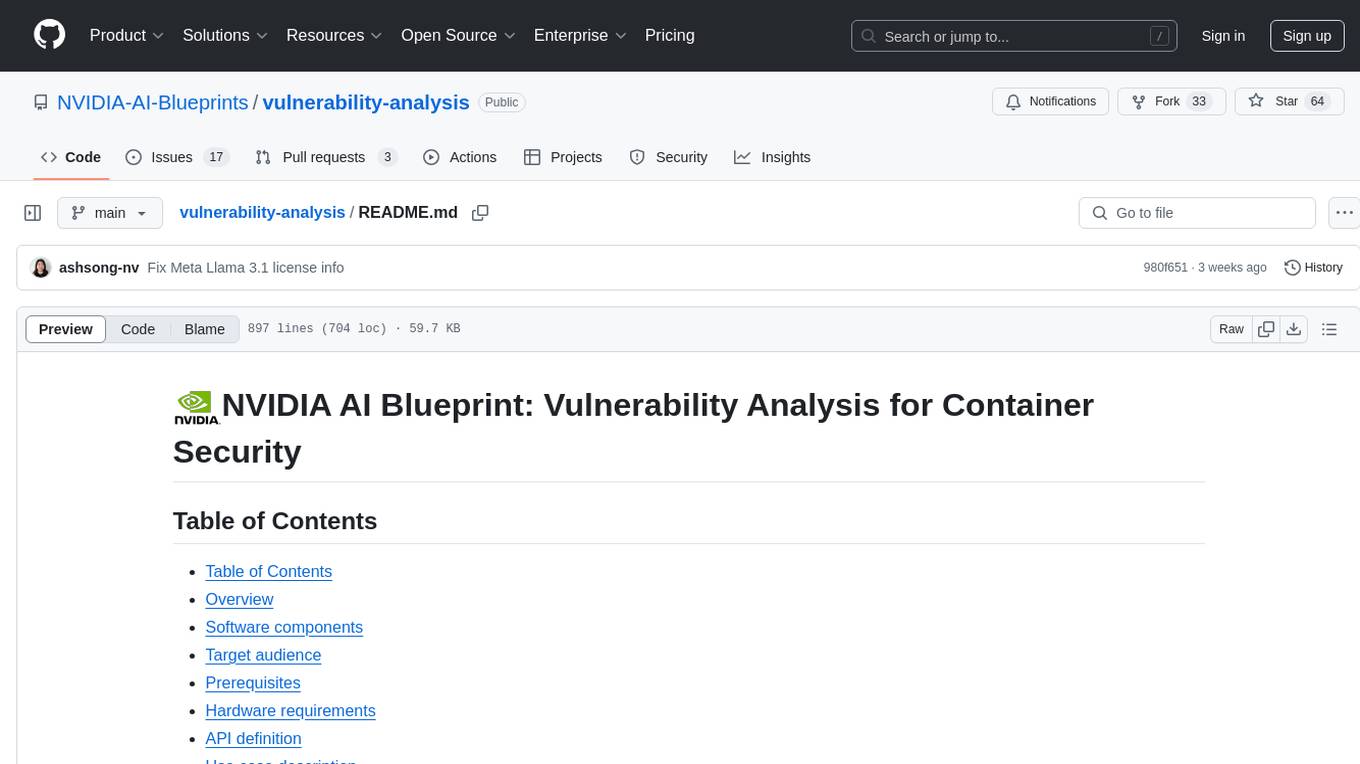
vulnerability-analysis
The NVIDIA AI Blueprint for Vulnerability Analysis for Container Security showcases accelerated analysis on common vulnerabilities and exposures (CVE) at an enterprise scale, reducing mitigation time from days to seconds. It enables security analysts to determine software package vulnerabilities using large language models (LLMs) and retrieval-augmented generation (RAG). The blueprint is designed for security analysts, IT engineers, and AI practitioners in cybersecurity. It requires NVAIE developer license and API keys for vulnerability databases, search engines, and LLM model services. Hardware requirements include L40 GPU for pipeline operation and optional LLM NIM and Embedding NIM. The workflow involves LLM pipeline for CVE impact analysis, utilizing LLM planner, agent, and summarization nodes. The blueprint uses NVIDIA NIM microservices and Morpheus Cybersecurity AI SDK for vulnerability analysis.
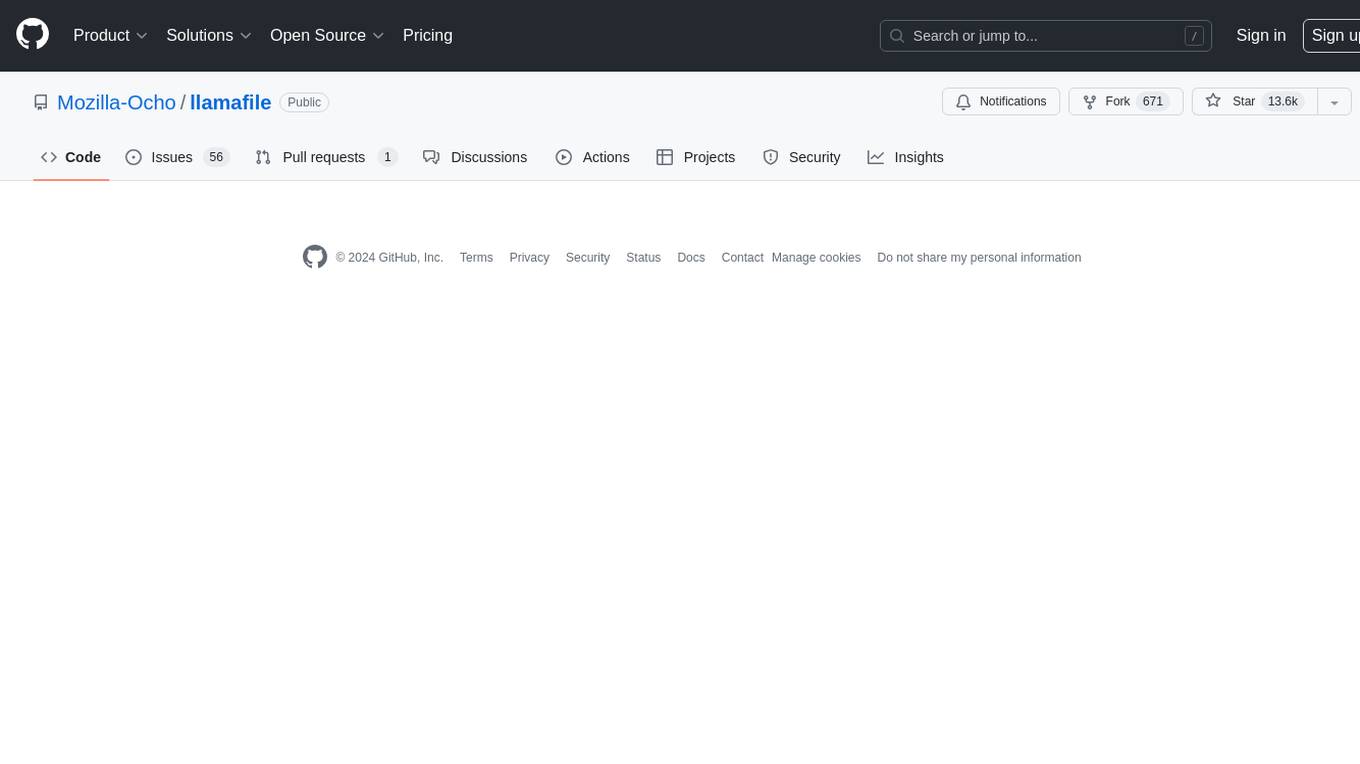
llamafile
llamafile is a tool that enables users to distribute and run Large Language Models (LLMs) with a single file. It combines llama.cpp with Cosmopolitan Libc to create a framework that simplifies the complexity of LLMs into a single-file executable called a 'llamafile'. Users can run these executable files locally on most computers without the need for installation, making open LLMs more accessible to developers and end users. llamafile also provides example llamafiles for various LLM models, allowing users to try out different LLMs locally. The tool supports multiple CPU microarchitectures, CPU architectures, and operating systems, making it versatile and easy to use.
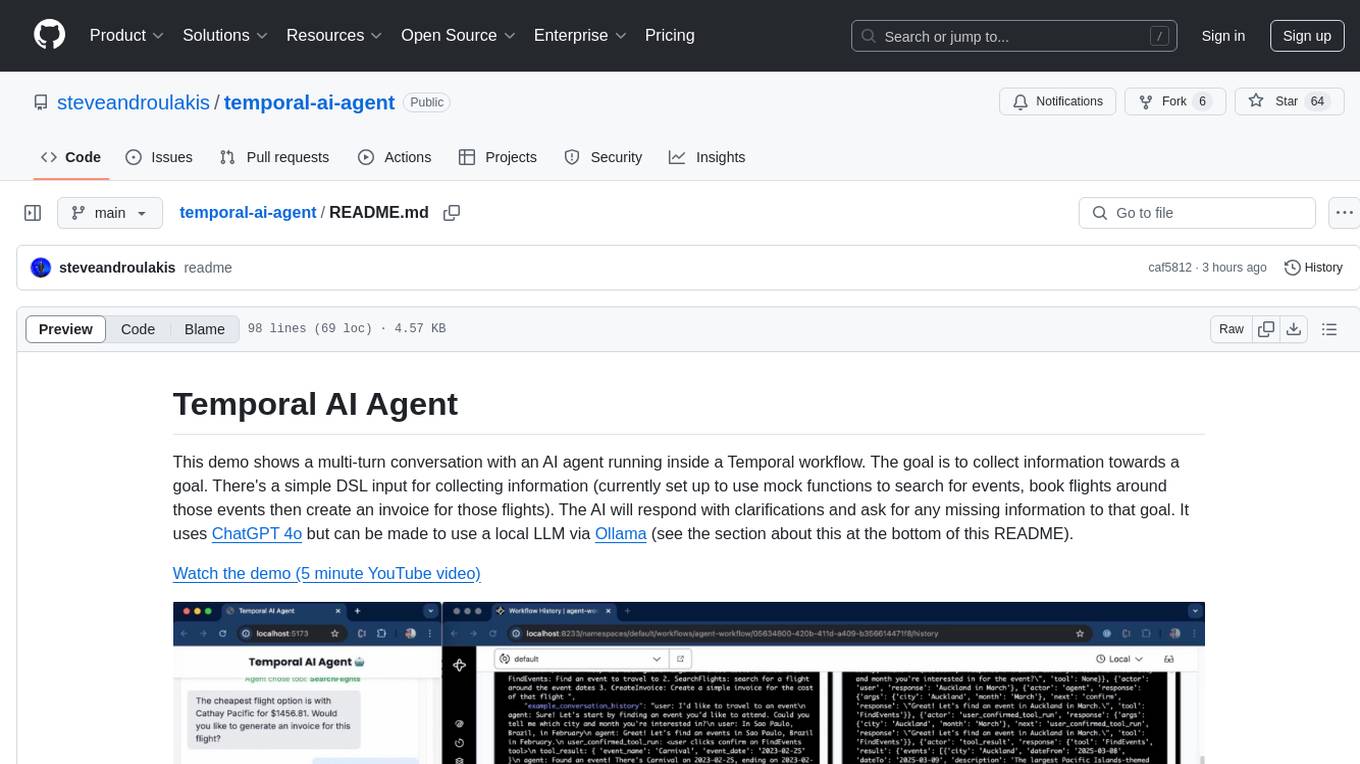
temporal-ai-agent
Temporal AI Agent is a demo showcasing a multi-turn conversation with an AI agent running inside a Temporal workflow. The agent collects information towards a goal using a simple DSL input. It is currently set up to search for events, book flights around those events, and create an invoice for those flights. The AI agent responds with clarifications and prompts for missing information. Users can configure the agent to use ChatGPT 4o or a local LLM via Ollama. The tool requires Rapidapi key for sky-scrapper to find flights and a Stripe key for creating invoices. Users can customize the agent by modifying tool and goal definitions in the codebase.
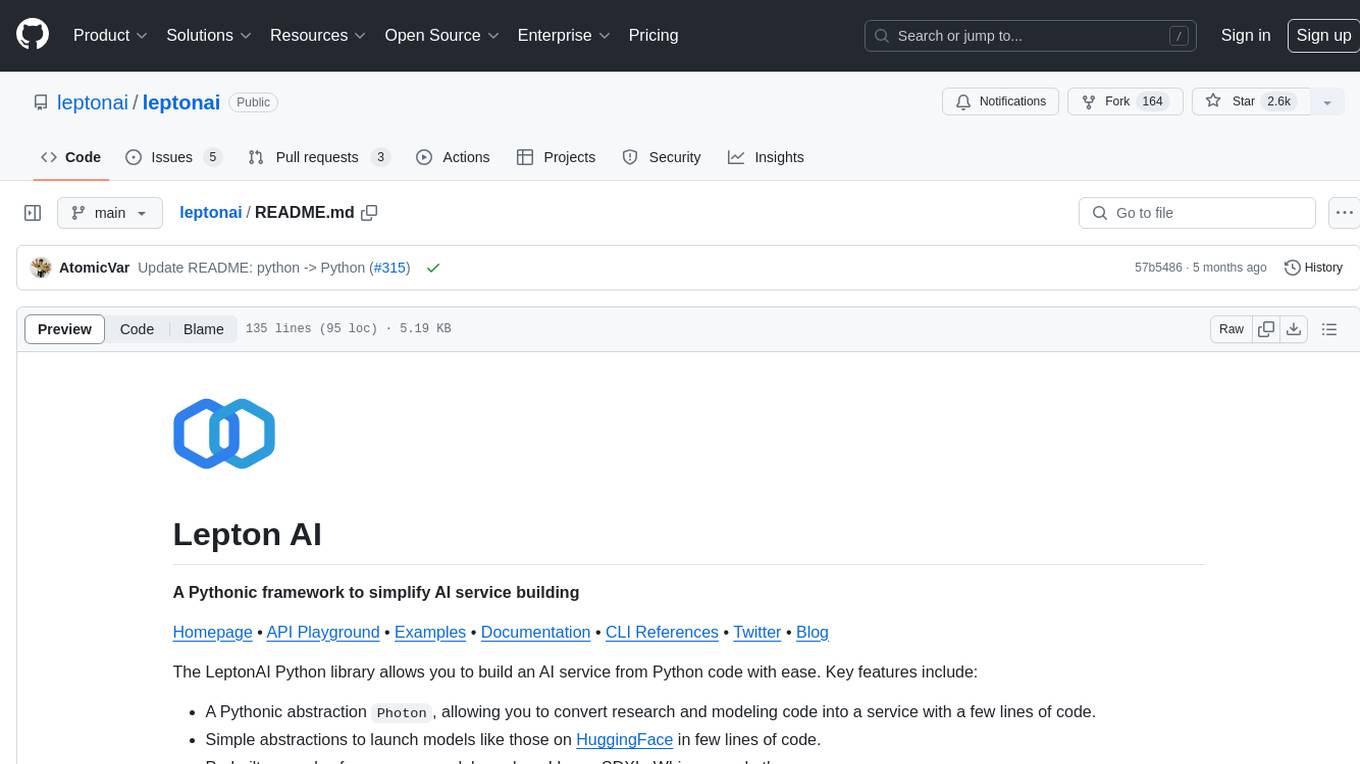
leptonai
A Pythonic framework to simplify AI service building. The LeptonAI Python library allows you to build an AI service from Python code with ease. Key features include a Pythonic abstraction Photon, simple abstractions to launch models like those on HuggingFace, prebuilt examples for common models, AI tailored batteries, a client to automatically call your service like native Python functions, and Pythonic configuration specs to be readily shipped in a cloud environment.
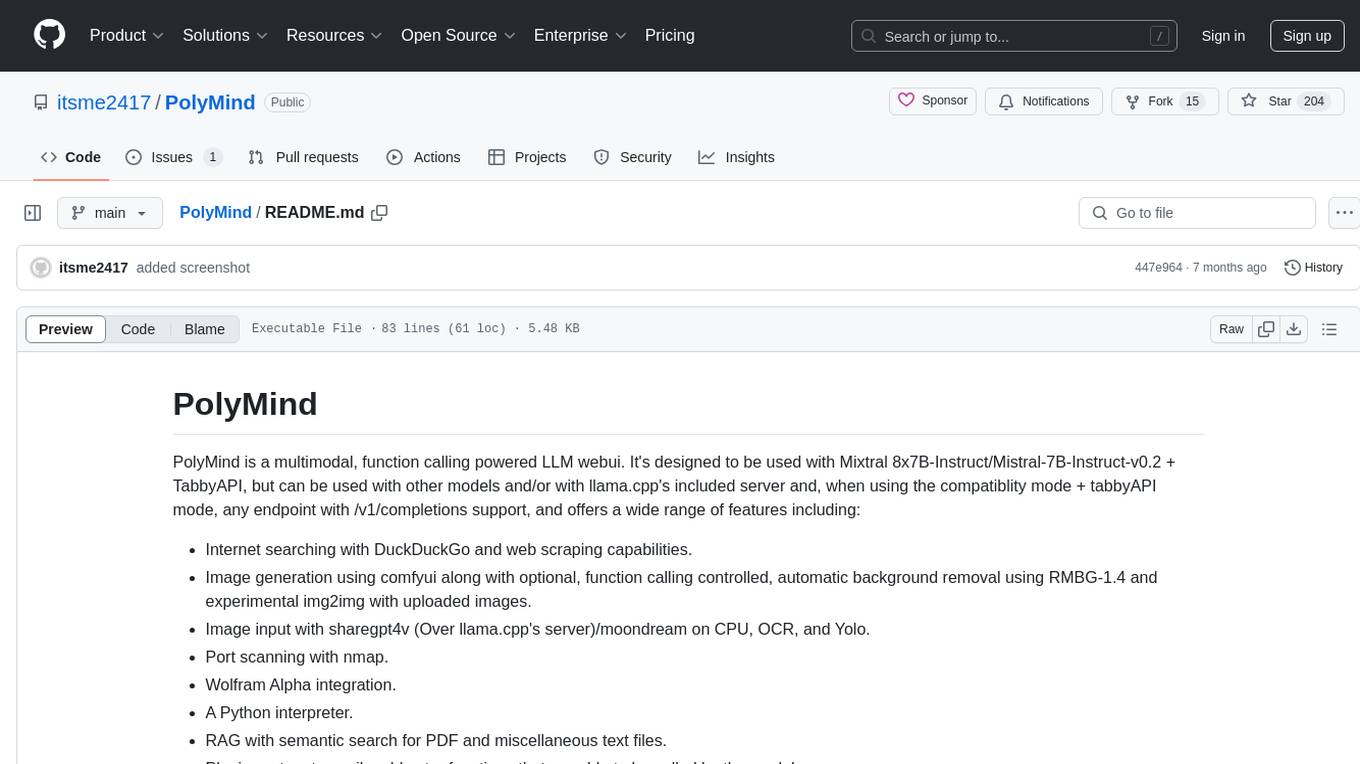
PolyMind
PolyMind is a multimodal, function calling powered LLM webui designed for various tasks such as internet searching, image generation, port scanning, Wolfram Alpha integration, Python interpretation, and semantic search. It offers a plugin system for adding extra functions and supports different models and endpoints. The tool allows users to interact via function calling and provides features like image input, image generation, and text file search. The application's configuration is stored in a `config.json` file with options for backend selection, compatibility mode, IP address settings, API key, and enabled features.
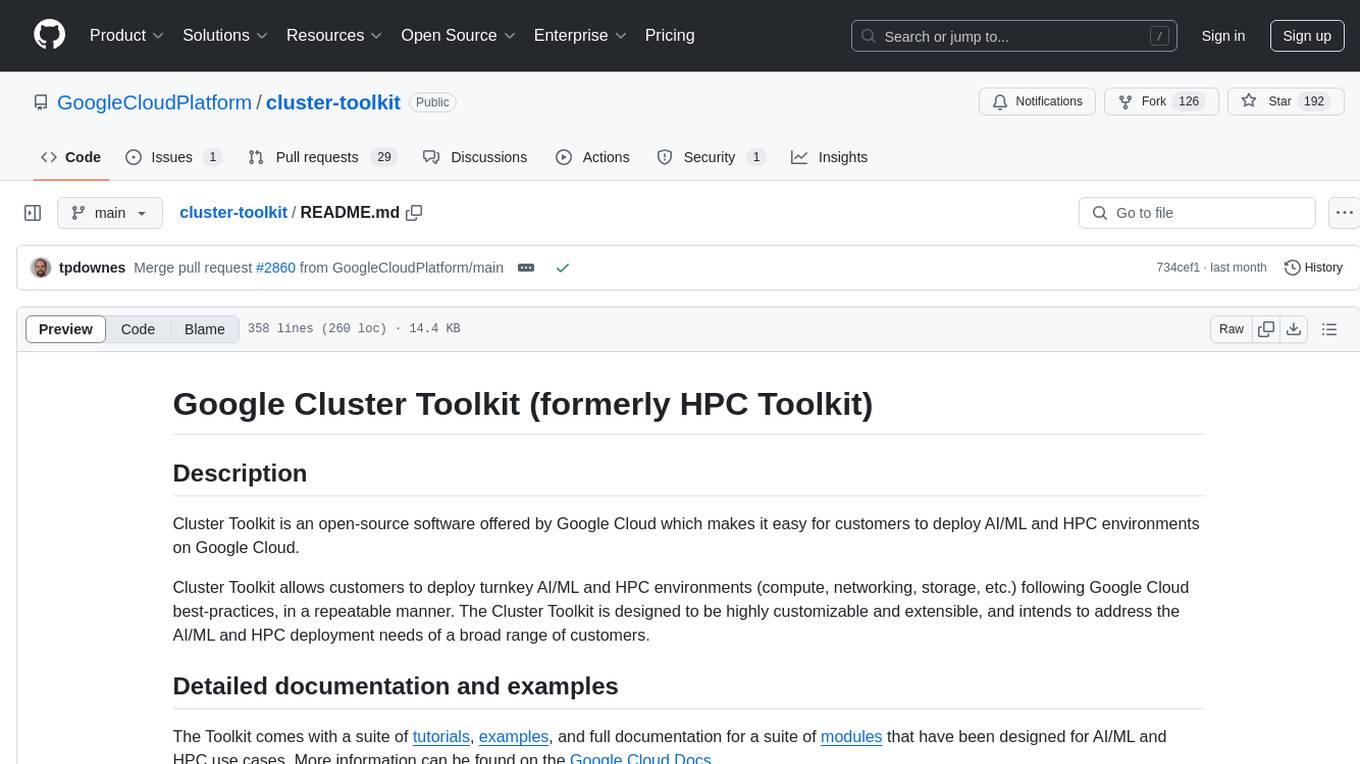
cluster-toolkit
Cluster Toolkit is an open-source software by Google Cloud for deploying AI/ML and HPC environments on Google Cloud. It allows easy deployment following best practices, with high customization and extensibility. The toolkit includes tutorials, examples, and documentation for various modules designed for AI/ML and HPC use cases.
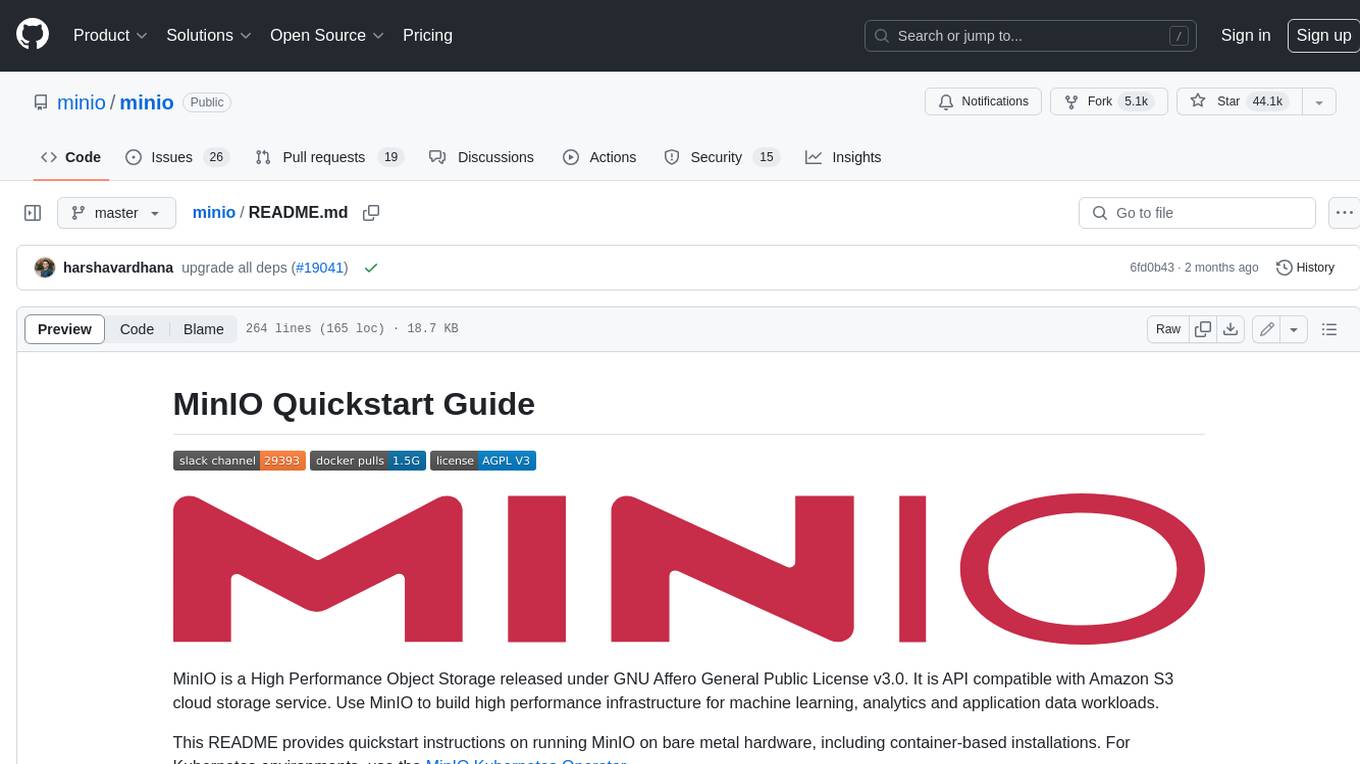
minio
MinIO is a High Performance Object Storage released under GNU Affero General Public License v3.0. It is API compatible with Amazon S3 cloud storage service. Use MinIO to build high performance infrastructure for machine learning, analytics and application data workloads.
For similar tasks
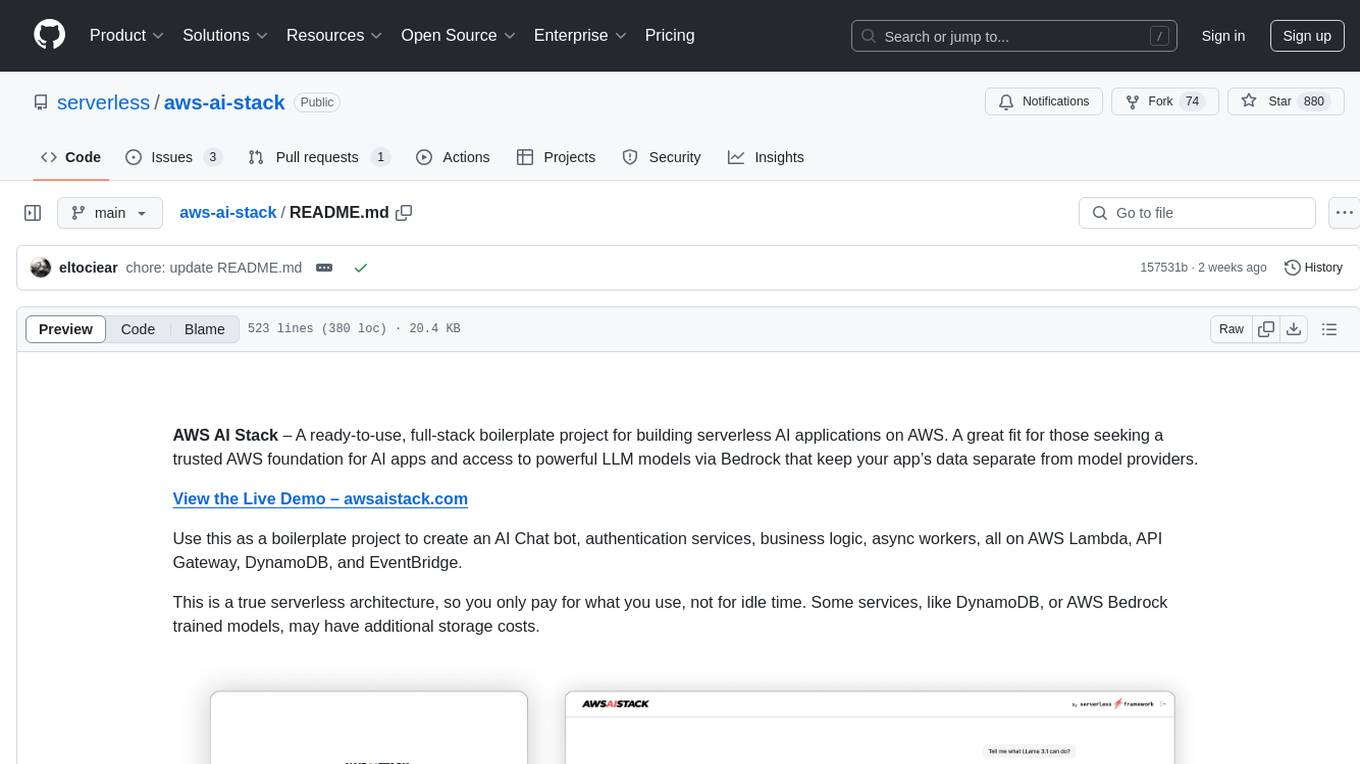
aws-ai-stack
AWS AI Stack is a full-stack boilerplate project designed for building serverless AI applications on AWS. It provides a trusted AWS foundation for AI apps with access to powerful LLM models via Bedrock. The architecture is serverless, ensuring cost-efficiency by only paying for usage. The project includes features like AI Chat & Streaming Responses, Multiple AI Models & Data Privacy, Custom Domain Names, API & Event-Driven architecture, Built-In Authentication, Multi-Environment support, and CI/CD with Github Actions. Users can easily create AI Chat bots, authentication services, business logic, and async workers using AWS Lambda, API Gateway, DynamoDB, and EventBridge.
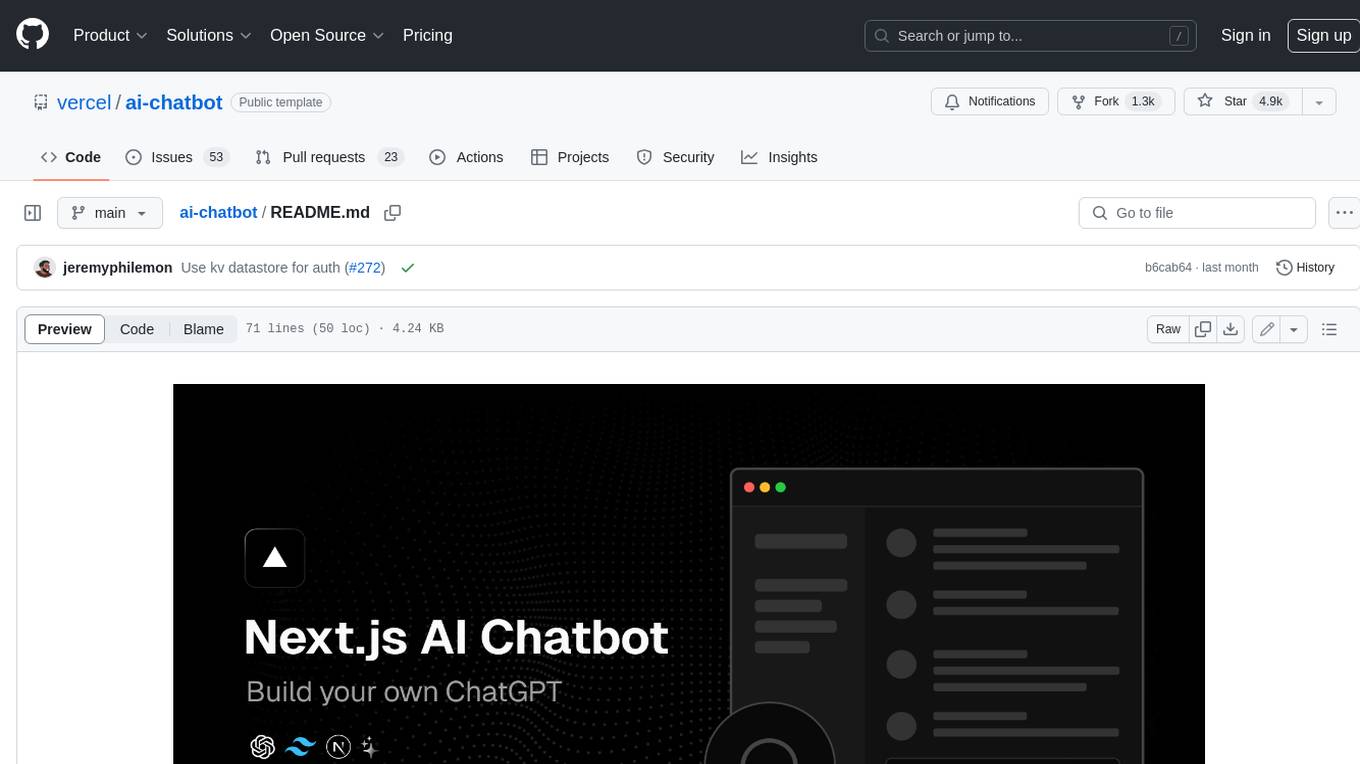
ai-chatbot
Next.js AI Chatbot is an open-source app template for building AI chatbots using Next.js, Vercel AI SDK, OpenAI, and Vercel KV. It includes features like Next.js App Router, React Server Components, Vercel AI SDK for streaming chat UI, support for various AI models, Tailwind CSS styling, Radix UI for headless components, chat history management, rate limiting, session storage with Vercel KV, and authentication with NextAuth.js. The template allows easy deployment to Vercel and customization of AI model providers.
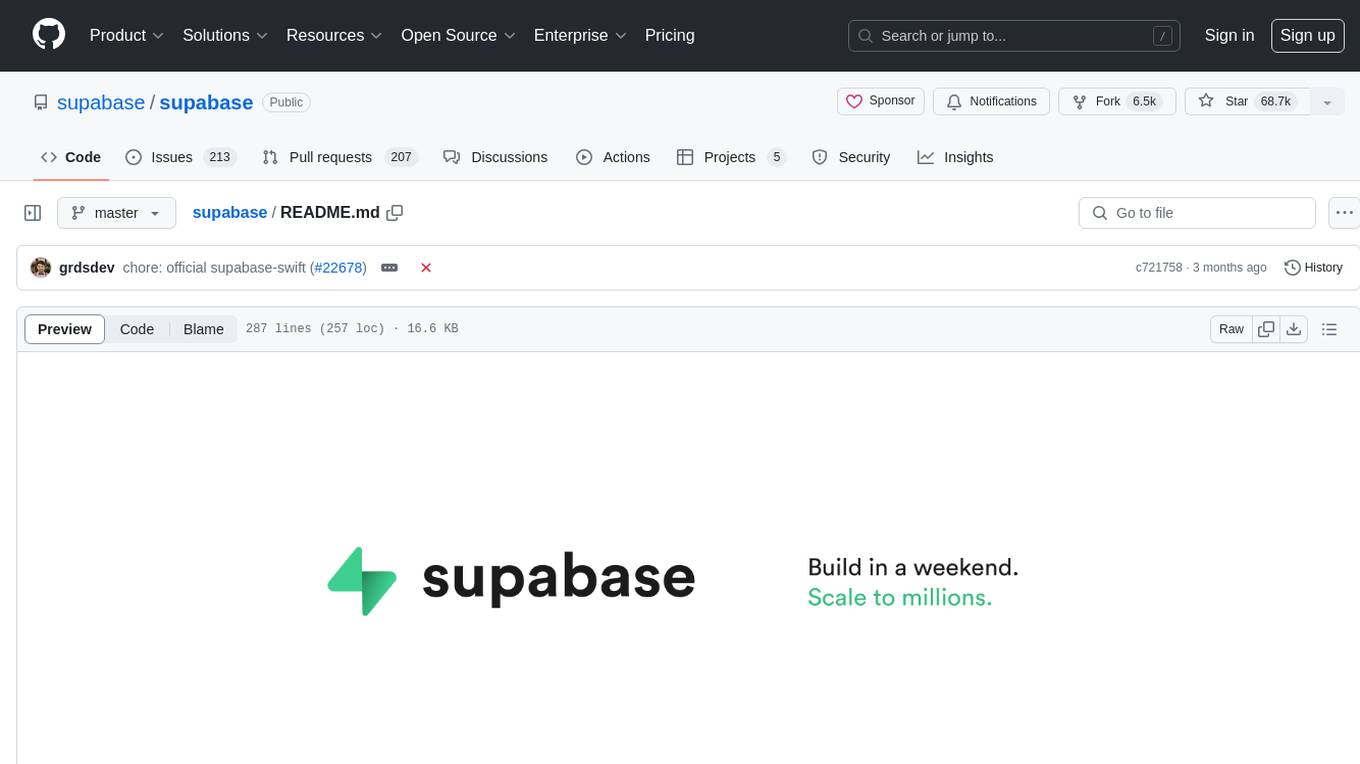
supabase
Supabase is an open source Firebase alternative that provides a wide range of features including a hosted Postgres database, authentication and authorization, auto-generated APIs, REST and GraphQL support, realtime subscriptions, functions, file storage, AI and vector/embeddings toolkit, and a dashboard. It aims to offer developers a Firebase-like experience using enterprise-grade open source tools.
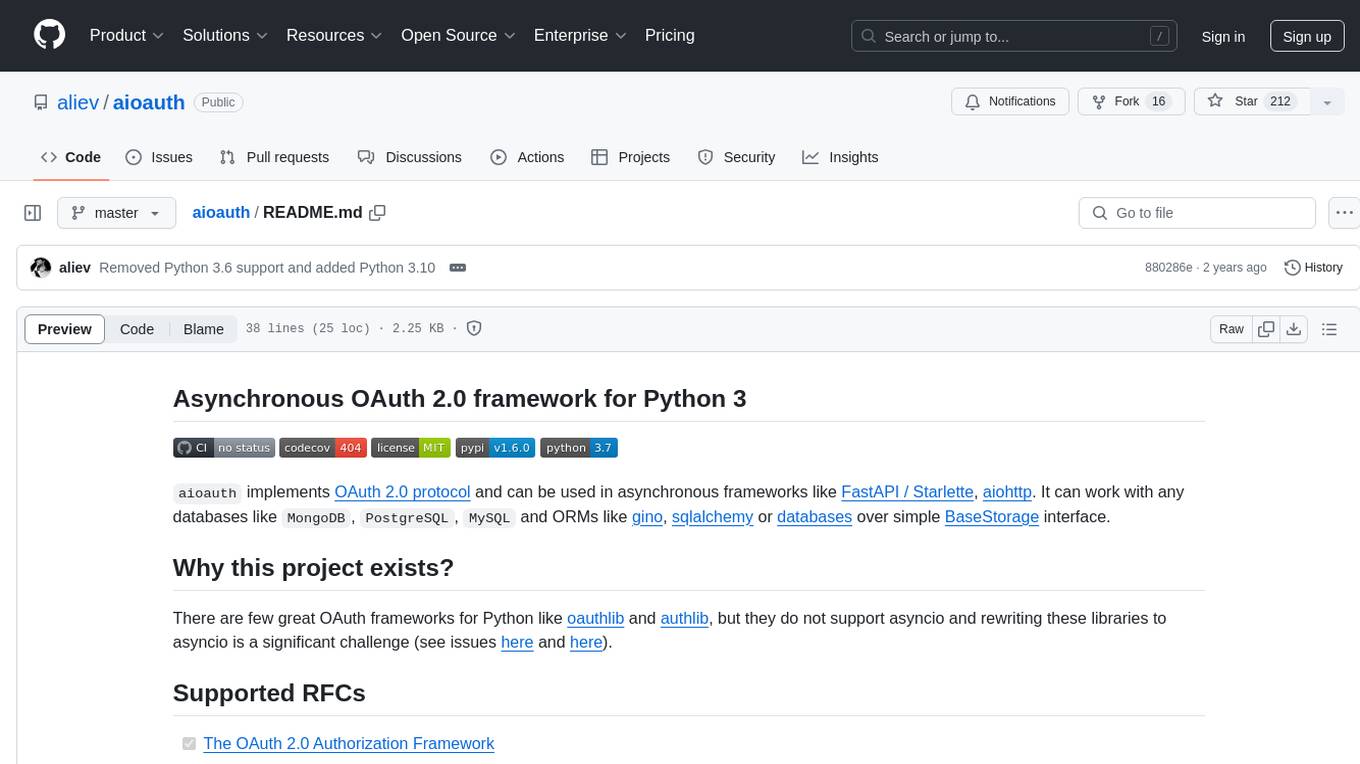
aioauth
Aioauth is an asynchronous OAuth 2.0 framework for Python 3 that implements the OAuth 2.0 protocol and can be used in asynchronous frameworks like FastAPI, Starlette, and aiohttp. It supports various databases such as MongoDB, PostgreSQL, MySQL, and ORMs like gino and sqlalchemy through a simple BaseStorage interface.
For similar jobs
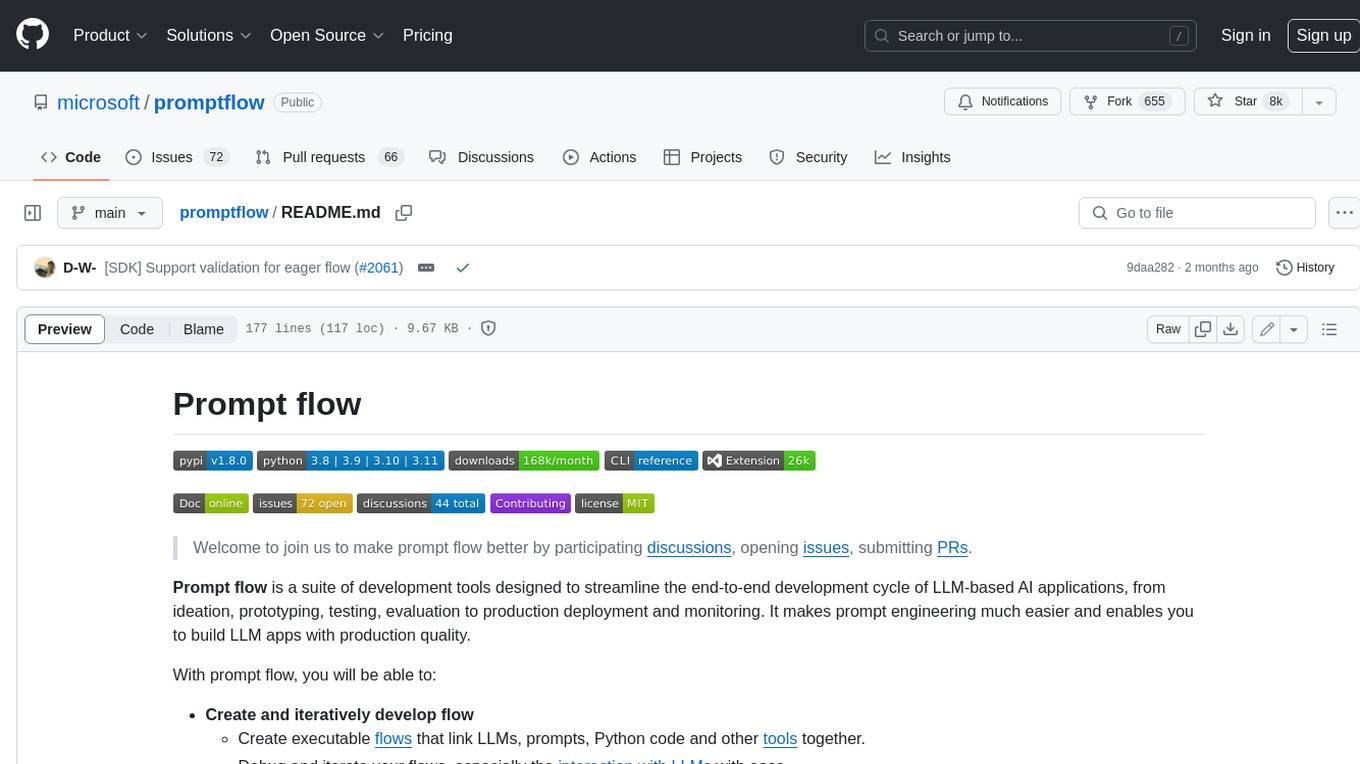
promptflow
**Prompt flow** is a suite of development tools designed to streamline the end-to-end development cycle of LLM-based AI applications, from ideation, prototyping, testing, evaluation to production deployment and monitoring. It makes prompt engineering much easier and enables you to build LLM apps with production quality.
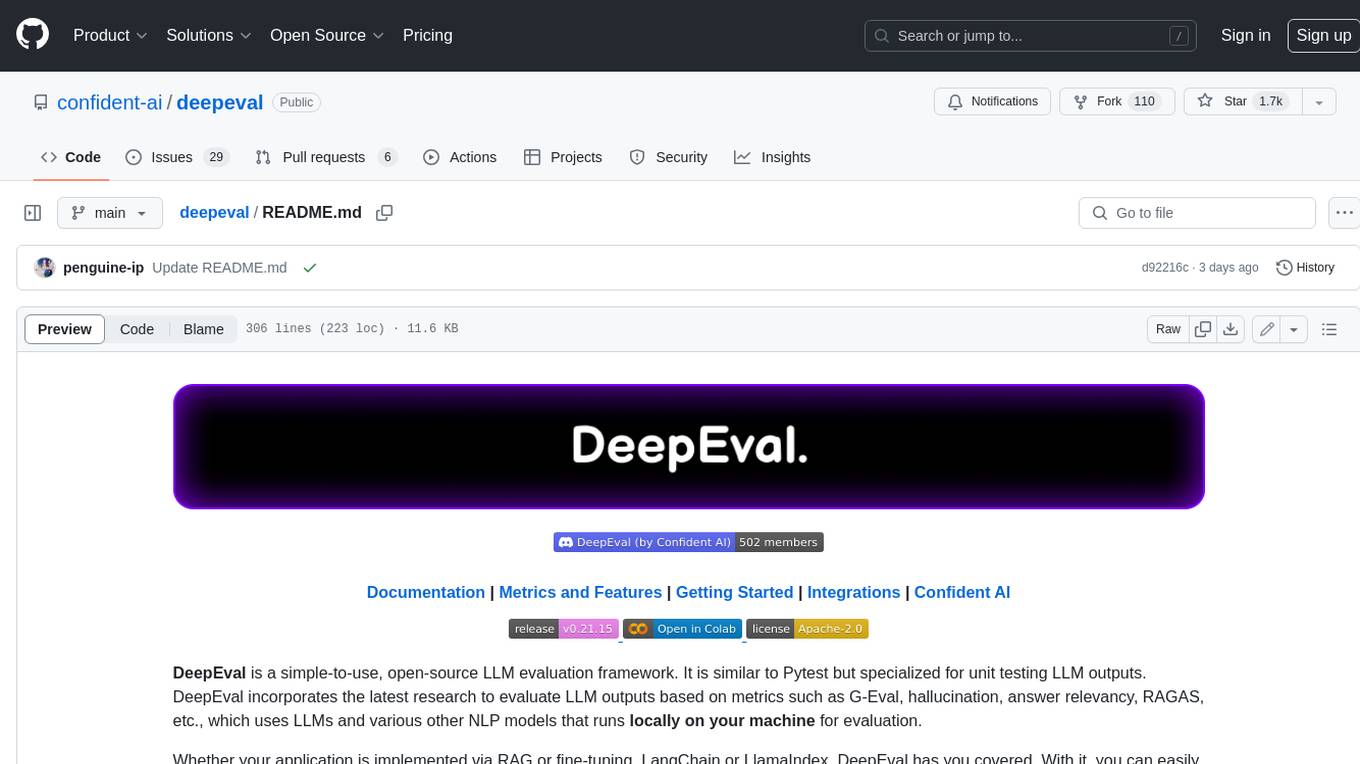
deepeval
DeepEval is a simple-to-use, open-source LLM evaluation framework specialized for unit testing LLM outputs. It incorporates various metrics such as G-Eval, hallucination, answer relevancy, RAGAS, etc., and runs locally on your machine for evaluation. It provides a wide range of ready-to-use evaluation metrics, allows for creating custom metrics, integrates with any CI/CD environment, and enables benchmarking LLMs on popular benchmarks. DeepEval is designed for evaluating RAG and fine-tuning applications, helping users optimize hyperparameters, prevent prompt drifting, and transition from OpenAI to hosting their own Llama2 with confidence.
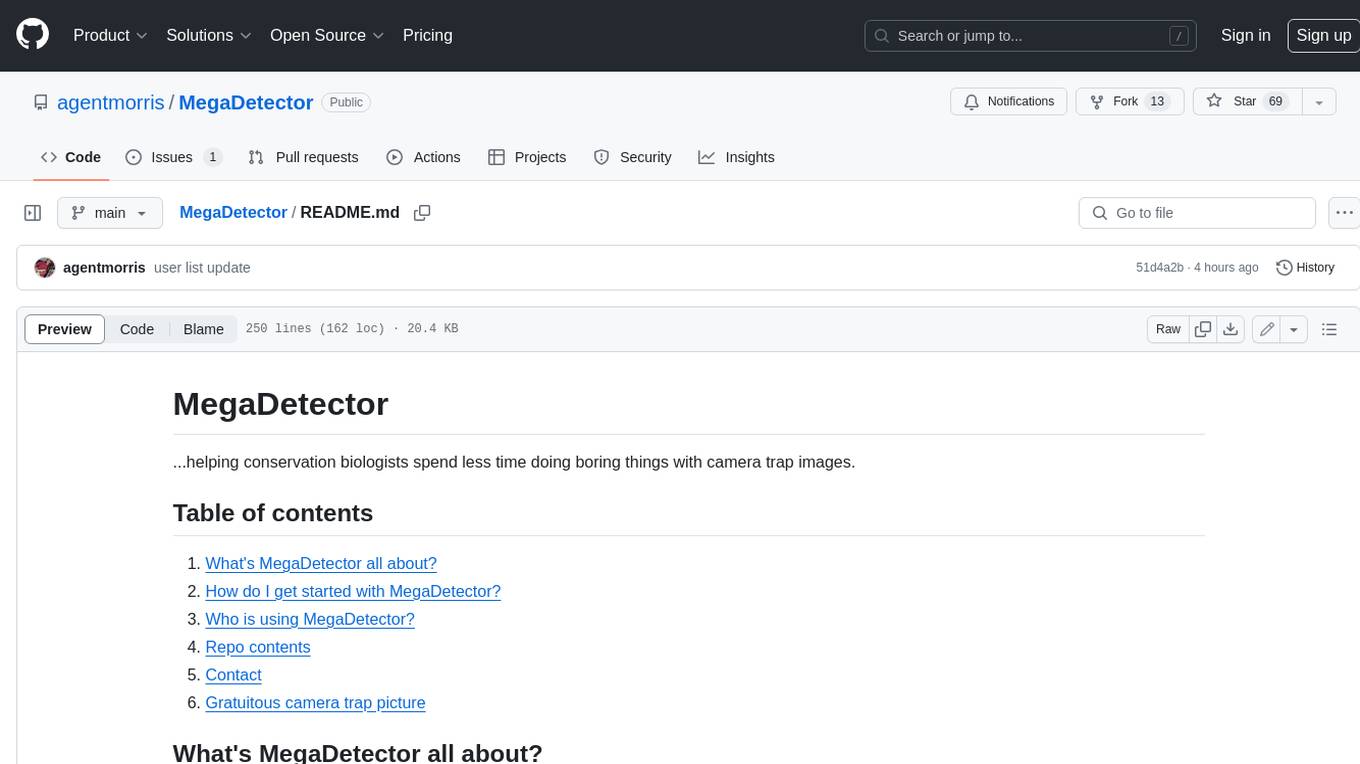
MegaDetector
MegaDetector is an AI model that identifies animals, people, and vehicles in camera trap images (which also makes it useful for eliminating blank images). This model is trained on several million images from a variety of ecosystems. MegaDetector is just one of many tools that aims to make conservation biologists more efficient with AI. If you want to learn about other ways to use AI to accelerate camera trap workflows, check out our of the field, affectionately titled "Everything I know about machine learning and camera traps".
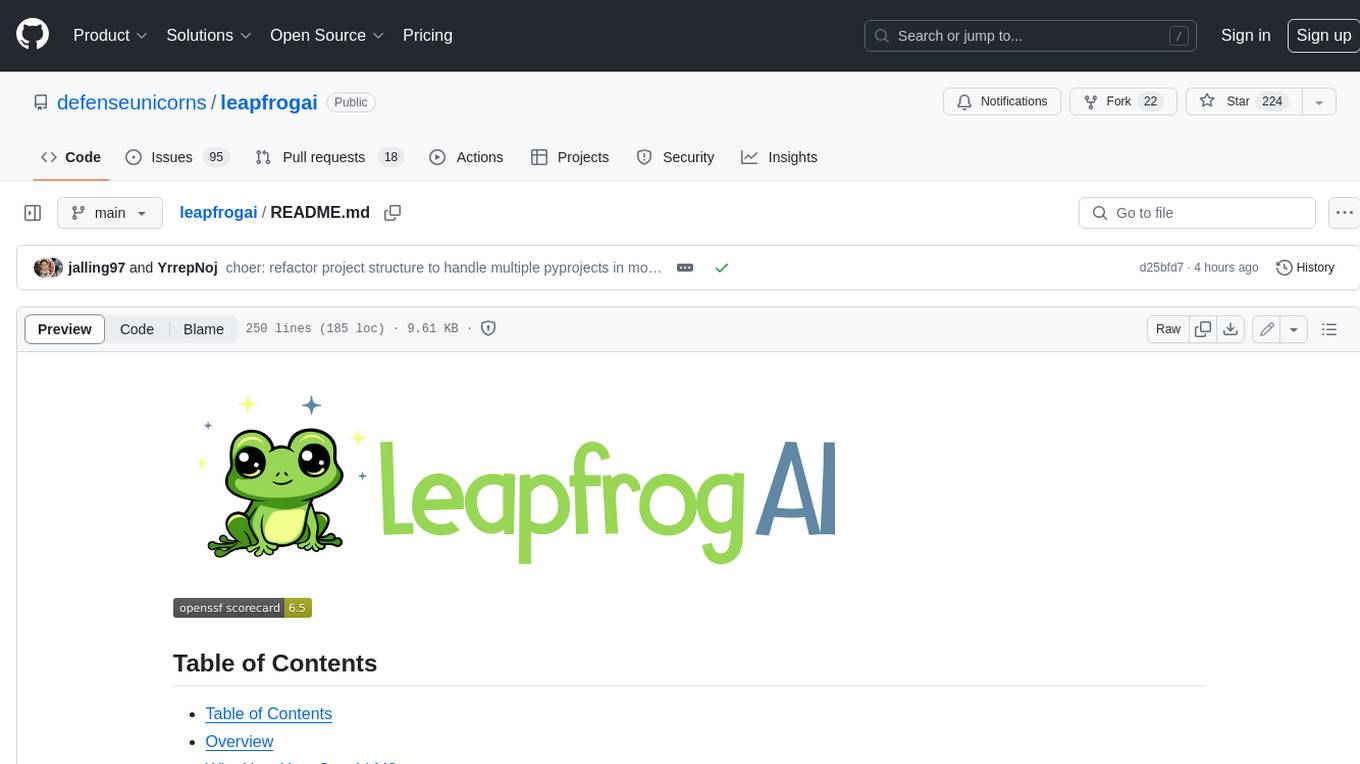
leapfrogai
LeapfrogAI is a self-hosted AI platform designed to be deployed in air-gapped resource-constrained environments. It brings sophisticated AI solutions to these environments by hosting all the necessary components of an AI stack, including vector databases, model backends, API, and UI. LeapfrogAI's API closely matches that of OpenAI, allowing tools built for OpenAI/ChatGPT to function seamlessly with a LeapfrogAI backend. It provides several backends for various use cases, including llama-cpp-python, whisper, text-embeddings, and vllm. LeapfrogAI leverages Chainguard's apko to harden base python images, ensuring the latest supported Python versions are used by the other components of the stack. The LeapfrogAI SDK provides a standard set of protobuffs and python utilities for implementing backends and gRPC. LeapfrogAI offers UI options for common use-cases like chat, summarization, and transcription. It can be deployed and run locally via UDS and Kubernetes, built out using Zarf packages. LeapfrogAI is supported by a community of users and contributors, including Defense Unicorns, Beast Code, Chainguard, Exovera, Hypergiant, Pulze, SOSi, United States Navy, United States Air Force, and United States Space Force.
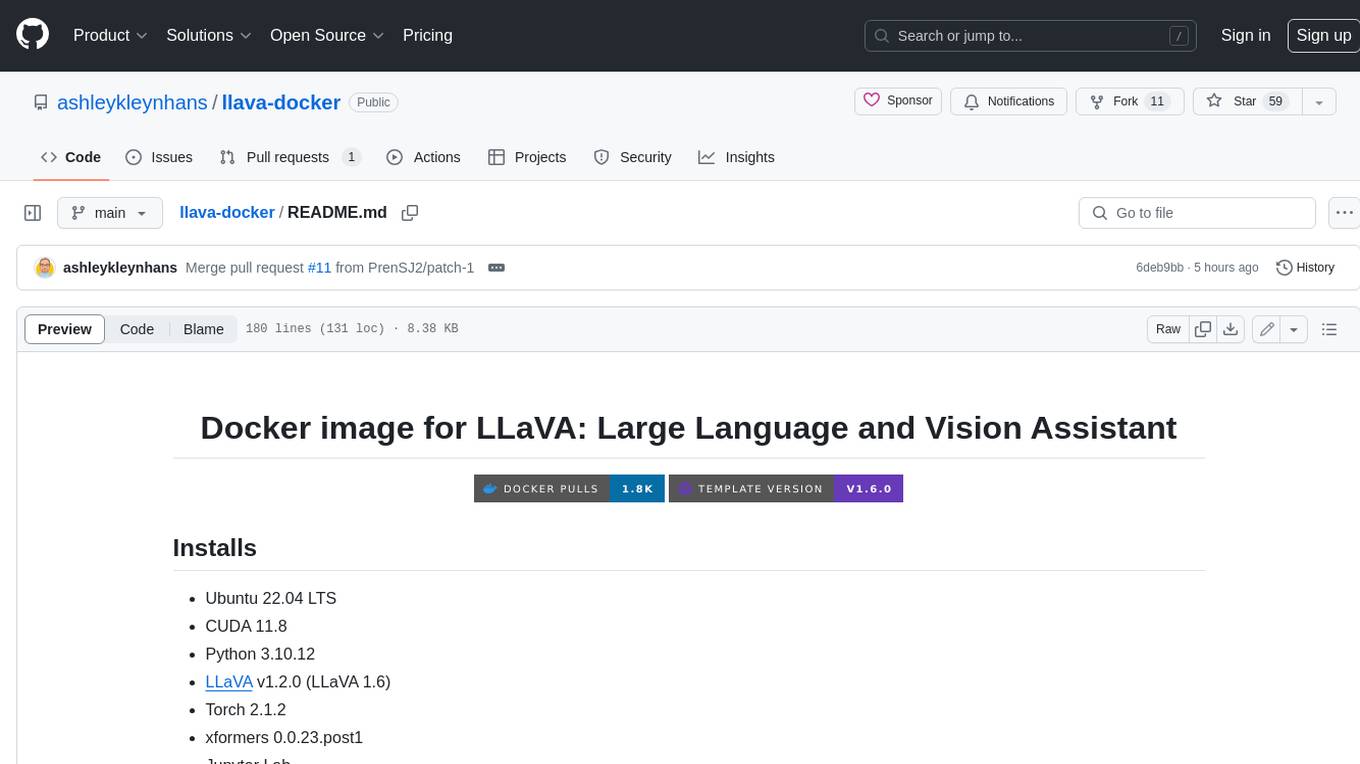
llava-docker
This Docker image for LLaVA (Large Language and Vision Assistant) provides a convenient way to run LLaVA locally or on RunPod. LLaVA is a powerful AI tool that combines natural language processing and computer vision capabilities. With this Docker image, you can easily access LLaVA's functionalities for various tasks, including image captioning, visual question answering, text summarization, and more. The image comes pre-installed with LLaVA v1.2.0, Torch 2.1.2, xformers 0.0.23.post1, and other necessary dependencies. You can customize the model used by setting the MODEL environment variable. The image also includes a Jupyter Lab environment for interactive development and exploration. Overall, this Docker image offers a comprehensive and user-friendly platform for leveraging LLaVA's capabilities.
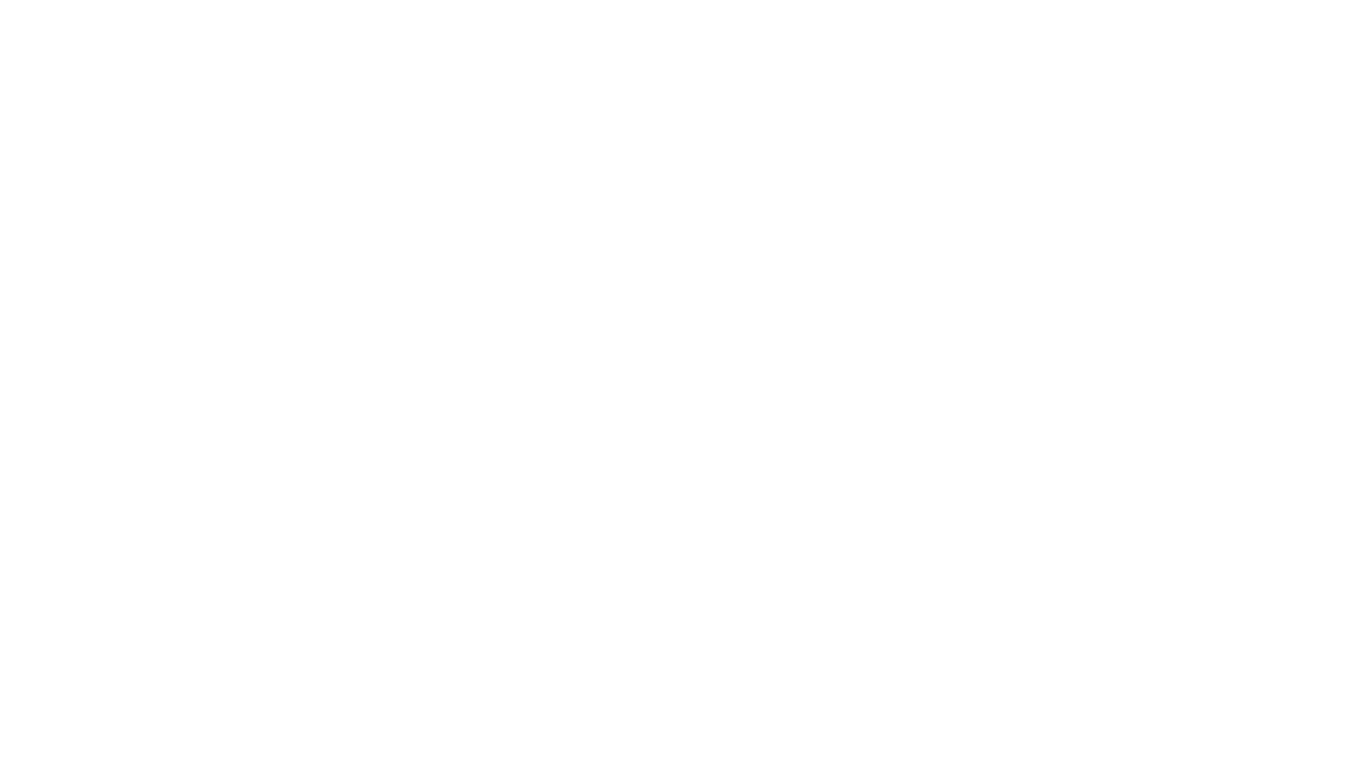
carrot
The 'carrot' repository on GitHub provides a list of free and user-friendly ChatGPT mirror sites for easy access. The repository includes sponsored sites offering various GPT models and services. Users can find and share sites, report errors, and access stable and recommended sites for ChatGPT usage. The repository also includes a detailed list of ChatGPT sites, their features, and accessibility options, making it a valuable resource for ChatGPT users seeking free and unlimited GPT services.
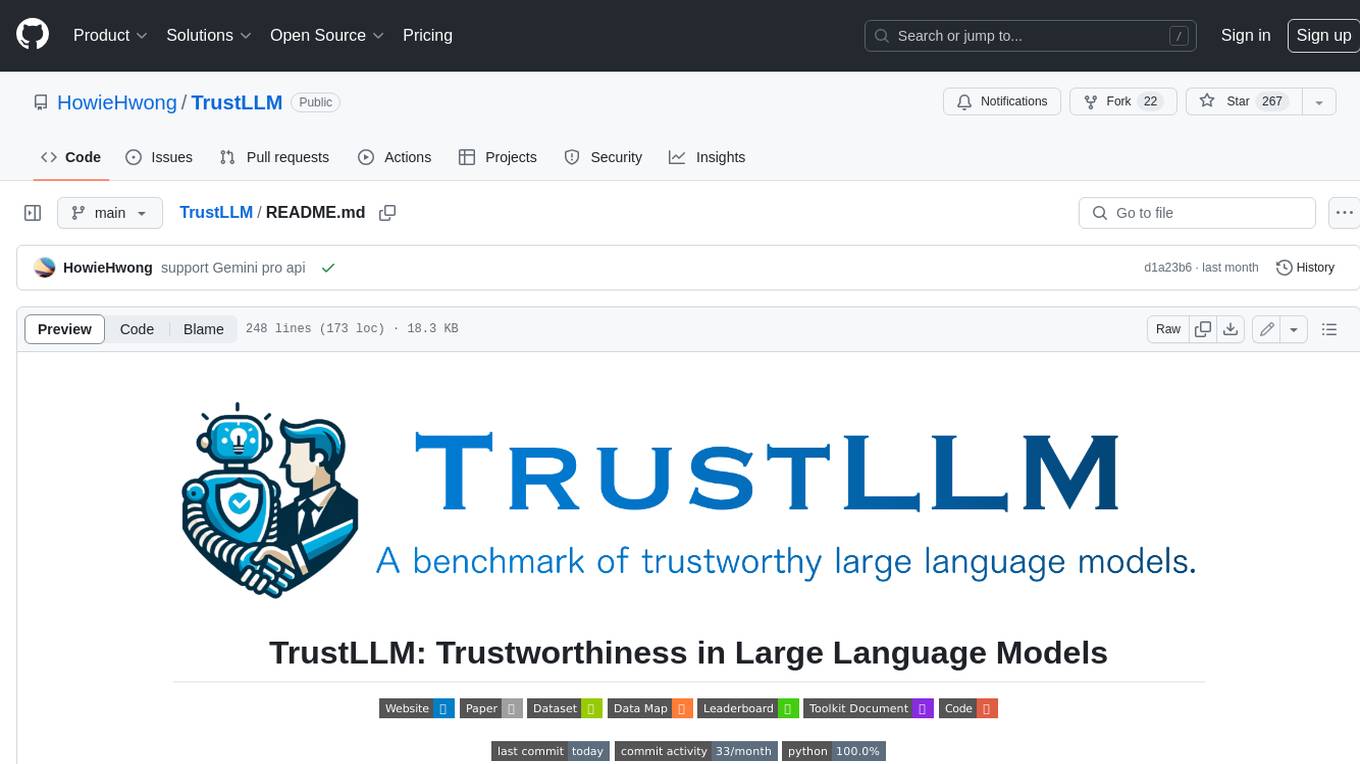
TrustLLM
TrustLLM is a comprehensive study of trustworthiness in LLMs, including principles for different dimensions of trustworthiness, established benchmark, evaluation, and analysis of trustworthiness for mainstream LLMs, and discussion of open challenges and future directions. Specifically, we first propose a set of principles for trustworthy LLMs that span eight different dimensions. Based on these principles, we further establish a benchmark across six dimensions including truthfulness, safety, fairness, robustness, privacy, and machine ethics. We then present a study evaluating 16 mainstream LLMs in TrustLLM, consisting of over 30 datasets. The document explains how to use the trustllm python package to help you assess the performance of your LLM in trustworthiness more quickly. For more details about TrustLLM, please refer to project website.
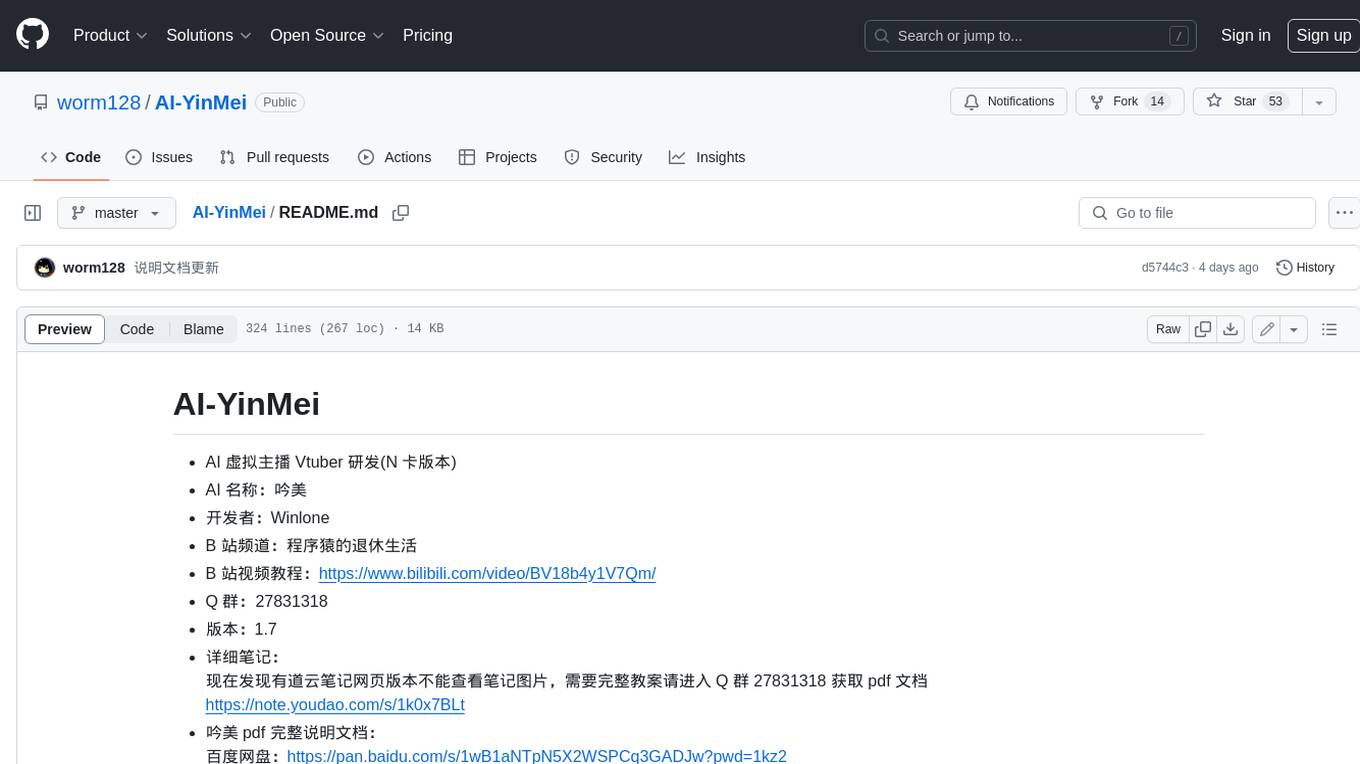
AI-YinMei
AI-YinMei is an AI virtual anchor Vtuber development tool (N card version). It supports fastgpt knowledge base chat dialogue, a complete set of solutions for LLM large language models: [fastgpt] + [one-api] + [Xinference], supports docking bilibili live broadcast barrage reply and entering live broadcast welcome speech, supports Microsoft edge-tts speech synthesis, supports Bert-VITS2 speech synthesis, supports GPT-SoVITS speech synthesis, supports expression control Vtuber Studio, supports painting stable-diffusion-webui output OBS live broadcast room, supports painting picture pornography public-NSFW-y-distinguish, supports search and image search service duckduckgo (requires magic Internet access), supports image search service Baidu image search (no magic Internet access), supports AI reply chat box [html plug-in], supports AI singing Auto-Convert-Music, supports playlist [html plug-in], supports dancing function, supports expression video playback, supports head touching action, supports gift smashing action, supports singing automatic start dancing function, chat and singing automatic cycle swing action, supports multi scene switching, background music switching, day and night automatic switching scene, supports open singing and painting, let AI automatically judge the content.