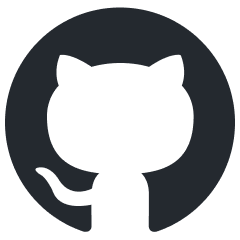
serverless-pdf-chat
LLM-powered document chat using Amazon Bedrock and AWS Serverless
Stars: 221
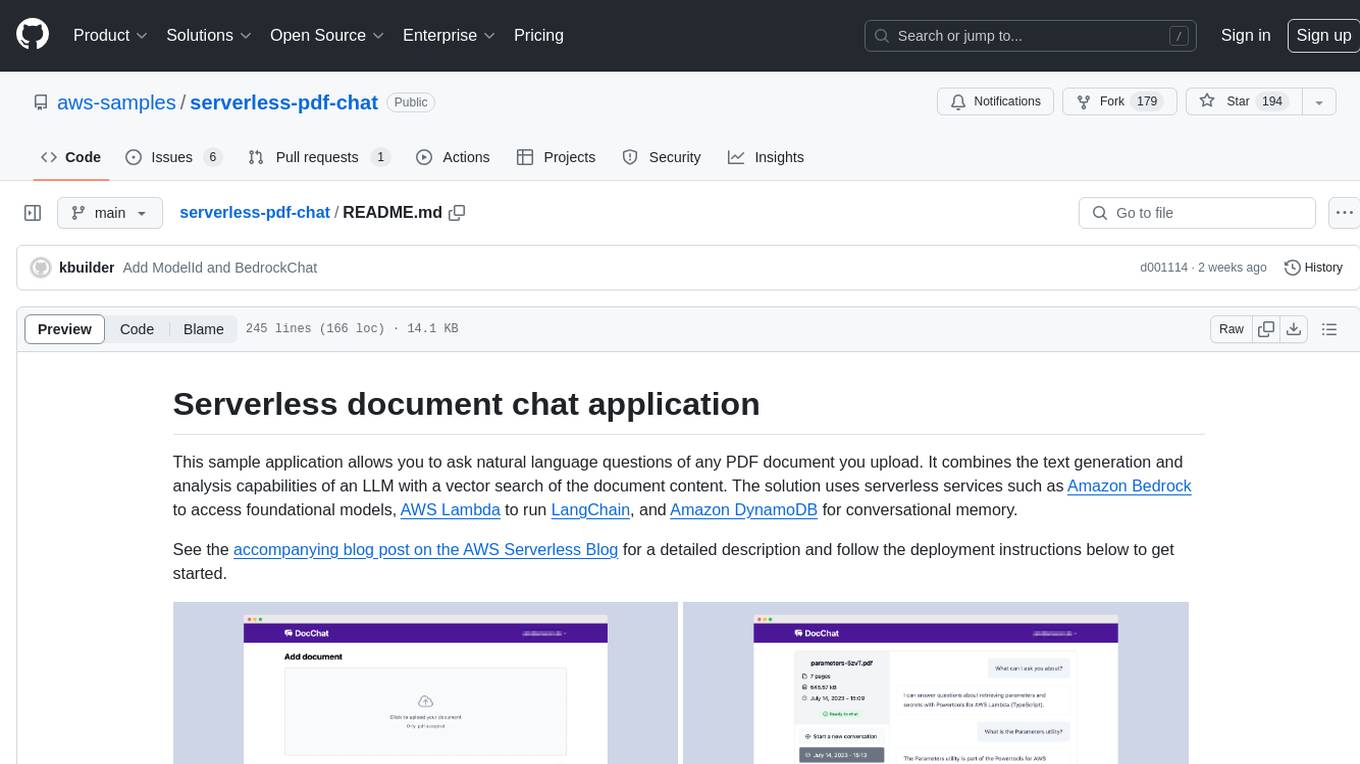
The serverless-pdf-chat repository contains a sample application that allows users to ask natural language questions of any PDF document they upload. It leverages serverless services like Amazon Bedrock, AWS Lambda, and Amazon DynamoDB to provide text generation and analysis capabilities. The application architecture involves uploading a PDF document to an S3 bucket, extracting metadata, converting text to vectors, and using a LangChain to search for information related to user prompts. The application is not intended for production use and serves as a demonstration and educational tool.
README:
This sample application allows you to ask natural language questions of any PDF document you upload. It combines the text generation and analysis capabilities of an LLM with a vector search of the document content. The solution uses serverless services such as Amazon Bedrock to access foundational models, AWS Lambda to run LangChain, and Amazon DynamoDB for conversational memory.
See the accompanying blog post on the AWS Serverless Blog for a detailed description and follow the deployment instructions below to get started.
Warning This application is not ready for production use. It was written for demonstration and educational purposes. Review the Security section of this README and consult with your security team before deploying this stack. No warranty is implied in this example.
Note This architecture creates resources that have costs associated with them. Please see the AWS Pricing page for details and make sure to understand the costs before deploying this stack.
- Amazon Bedrock for serverless embedding and inference
- LangChain to orchestrate a Q&A LLM chain
- FAISS vector store
- Amazon DynamoDB for serverless conversational memory
- AWS Lambda for serverless compute
- Frontend built in React, TypeScript, TailwindCSS, and Vite.
- Run locally or deploy to AWS Amplify Hosting
- Amazon Cognito for authentication
- A user uploads a PDF document into an Amazon Simple Storage Service (S3) bucket through a static web application frontend.
- This upload triggers a metadata extraction and document embedding process. The process converts the text in the document into vectors. The vectors are loaded into a vector index and stored in S3 for later use.
- When a user chats with a PDF document and sends a prompt to the backend, a Lambda function retrieves the index from S3 and searches for information related to the prompt.
- A LLM then uses the results of this vector search, previous messages in the conversation, and its general-purpose capabilities to formulate a response to the user.
- AWS SAM CLI
- Python 3.11 or greater
Clone this repository:
git clone https://github.com/aws-samples/serverless-pdf-chat.git
This application can be used with a variety of Amazon Bedrock models. See Supported models in Amazon Bedrock for a complete list.
By default, this application uses Titan Embeddings G1 - Text to generate embeddings and Anthropic Claude v3 Sonnet for responses.
Important - Before you can use these models with this application, you must request access in the Amazon Bedrock console. See the Model access section of the Bedrock User Guide for detailed instructions. By default, this application is configured to use Amazon Bedrock in the
us-east-1
Region, make sure you request model access in that Region (this does not have to be the same Region that you deploy this stack to).
To select your Bedrock model, specify the ModelId
parameter during the AWS SAM deployment, such as anthropic.claude-3-sonnet-20240229-v1:0
. See Amazon Bedrock model IDs for a complete list.
The ModelId
parameter is used in the GenerateResponseFunction Lambda function of your AWS SAM template to instantiate LangChain BedrockChat and ConversationalRetrievalChain objects, providing efficient retrieval of relevant context from large PDF datasets to enable the Bedrock model-generated response.
def bedrock_chain(faiss_index, memory, human_input, bedrock_runtime):
chat = BedrockChat(
model_id=MODEL_ID,
model_kwargs={'temperature': 0.0}
)
chain = ConversationalRetrievalChain.from_llm(
llm=chat,
chain_type="stuff",
retriever=faiss_index.as_retriever(),
memory=memory,
return_source_documents=True,
)
response = chain.invoke({"question": human_input})
return response
AWS Amplify Hosting enables a fully-managed deployment of the application's React frontend in an AWS-managed account using Amazon S3 and Amazon CloudFront. You can optionally run the React frontend locally by skipping to Deploy the application with AWS SAM.
To set up Amplify Hosting:
-
Fork this GitHub repository and take note of your repository URL, for example
https://github.com/user/serverless-pdf-chat/
. -
Create a GitHub fine-grained access token for the new repository by following this guide. For the Repository permissions, select Read and write for Content and Webhooks.
-
Create a new secret called
serverless-pdf-chat-github-token
in AWS Secrets Manager and input your fine-grained access token as plaintext. Select the Plaintext tab and confirm your secret looks like this:github_pat_T2wyo------------------------------------------------------------------------rs0Pp
-
Change to the
backend
directory and build the application:cd backend sam build
-
Deploy the application into your AWS account:
sam deploy --guided
-
For Stack Name, choose
serverless-pdf-chat
. -
For Frontend, specify the environment ("local", "amplify") for the frontend of the application.
-
If you selected "amplify", specify the URL of the forked Git repository containing the application code.
-
Specify the Amazon Bedrock model ID. For example,
anthropic.claude-3-sonnet-20240229-v1:0
. -
For the remaining options, keep the defaults by pressing the enter key.
AWS SAM will now provision the AWS resources defined in the backend/template.yaml
template. Once the deployment is completed successfully, you will see a set of output values similar to the following:
CloudFormation outputs from deployed stack
-------------------------------------------------------------------------------
Outputs
-------------------------------------------------------------------------------
Key CognitoUserPool
Description -
Value us-east-1_gxKtRocFs
Key CognitoUserPoolClient
Description -
Value 874ghcej99f8iuo0lgdpbrmi76k
Key ApiGatewayBaseUrl
Description -
Value https://abcd1234.execute-api.us-east-1.amazonaws.com/dev/
-------------------------------------------------------------------------------
If you selected to deploy the React frontend using Amplify Hosting, navigate to the Amplify console to check the build status. If the build does not start automatically, trigger it through the Amplify console.
If you selected to run the React frontend locally and connect to the deployed resources in AWS, you will use the CloudFormation stack outputs in the following section.
Create a file named .env.development
in the frontend
directory. Vite will use this file to set up environment variables when we run the application locally.
Copy the following file content and replace the values with the outputs provided by AWS SAM:
VITE_REGION=us-east-1
VITE_API_ENDPOINT=https://abcd1234.execute-api.us-east-1.amazonaws.com/dev/
VITE_USER_POOL_ID=us-east-1_gxKtRocFs
VITE_USER_POOL_CLIENT_ID=874ghcej99f8iuo0lgdpbrmi76k
Next, install the frontend's dependencies by running the following command in the frontend
directory:
npm ci
Finally, to start the application locally, run the following command in the frontend
directory:
npm run dev
Vite will now start the application under http://localhost:5173
.
The application uses Amazon Cognito to authenticate users through a login screen. In this step, you will create a user to access the application.
Perform the following steps to create a user in the Cognito user pool:
- Navigate to the Amazon Cognito console.
- Find the user pool with an ID matching the output provided by AWS SAM above.
- Under Users, choose Create user.
- Enter an email address and a password that adheres to the password requirements.
- Choose Create user.
Navigate back to your Amplify website URL or local host address to log in with the new user's credentials.
-
Delete any secrets in AWS Secrets Manager created as part of this walkthrough.
-
Empty the Amazon S3 bucket created as part of the AWS SAM template.
-
Run the following command in the
backend
directory of the project to delete all associated resources resources:sam delete
If you are experiencing issues when running the sam build
command, try setting the --use-container
flag (requires Docker):
sam build --use-container
If you are still experiencing issues despite using --use-container
, try switching the AWS Lambda functions from arm64
to x86_64
in the backend/template.yaml
(as well as switching to the x_86_64
version of Powertools):
Globals:
Function:
Runtime: python3.11
Handler: main.lambda_handler
Architectures:
- x86_64
Tracing: Active
Environment:
Variables:
LOG_LEVEL: INFO
Layers:
- !Sub arn:aws:lambda:${AWS::Region}:017000801446:layer:AWSLambdaPowertoolsPythonV2:51
This application was written for demonstration and educational purposes and not for production use. The Security Pillar of the AWS Well-Architected Framework can support you in further adopting the sample into a production deployment in addition to your own established processes. Take note of the following:
-
The application uses encryption in transit and at rest with AWS-managed keys where applicable. Optionally, use AWS KMS with DynamoDB, SQS, and S3 for more control over encryption keys.
-
This application uses Powertools for AWS Lambda (Python) to log to inputs and ouputs to CloudWatch Logs. Per default, this can include sensitive data contained in user input. Adjust the log level and remove log statements to fit your security requirements.
-
API Gateway access logging and usage plans are not activiated in this code sample. Similarly, S3 access logging is currently not enabled.
-
In order to simplify the setup of the demo, this solution uses AWS managed policies associated to IAM roles that contain wildcards on resources. Please consider to further scope down the policies as you see fit according to your needs. Please note that there is a resource wildcard on the AWS managed
AWSLambdaSQSQueueExecutionRole
. This is a known behaviour, see this GitHub issue for details. -
If your security controls require inspecting network traffic, consider adjusting the AWS SAM template to attach the Lambda functions to a VPC via its
VpcConfig
.
See CONTRIBUTING for more information.
This library is licensed under the MIT-0 License. See the LICENSE file.
For Tasks:
Click tags to check more tools for each tasksFor Jobs:
Alternative AI tools for serverless-pdf-chat
Similar Open Source Tools
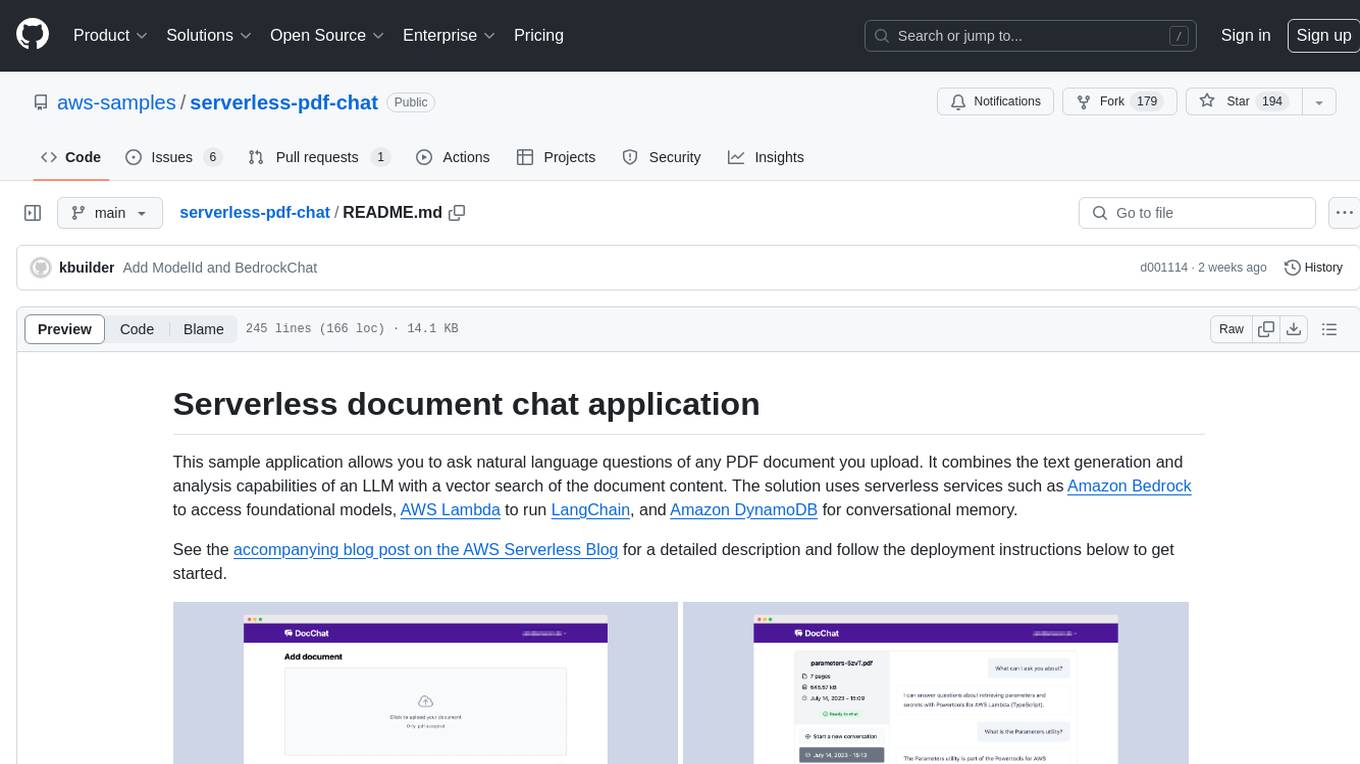
serverless-pdf-chat
The serverless-pdf-chat repository contains a sample application that allows users to ask natural language questions of any PDF document they upload. It leverages serverless services like Amazon Bedrock, AWS Lambda, and Amazon DynamoDB to provide text generation and analysis capabilities. The application architecture involves uploading a PDF document to an S3 bucket, extracting metadata, converting text to vectors, and using a LangChain to search for information related to user prompts. The application is not intended for production use and serves as a demonstration and educational tool.
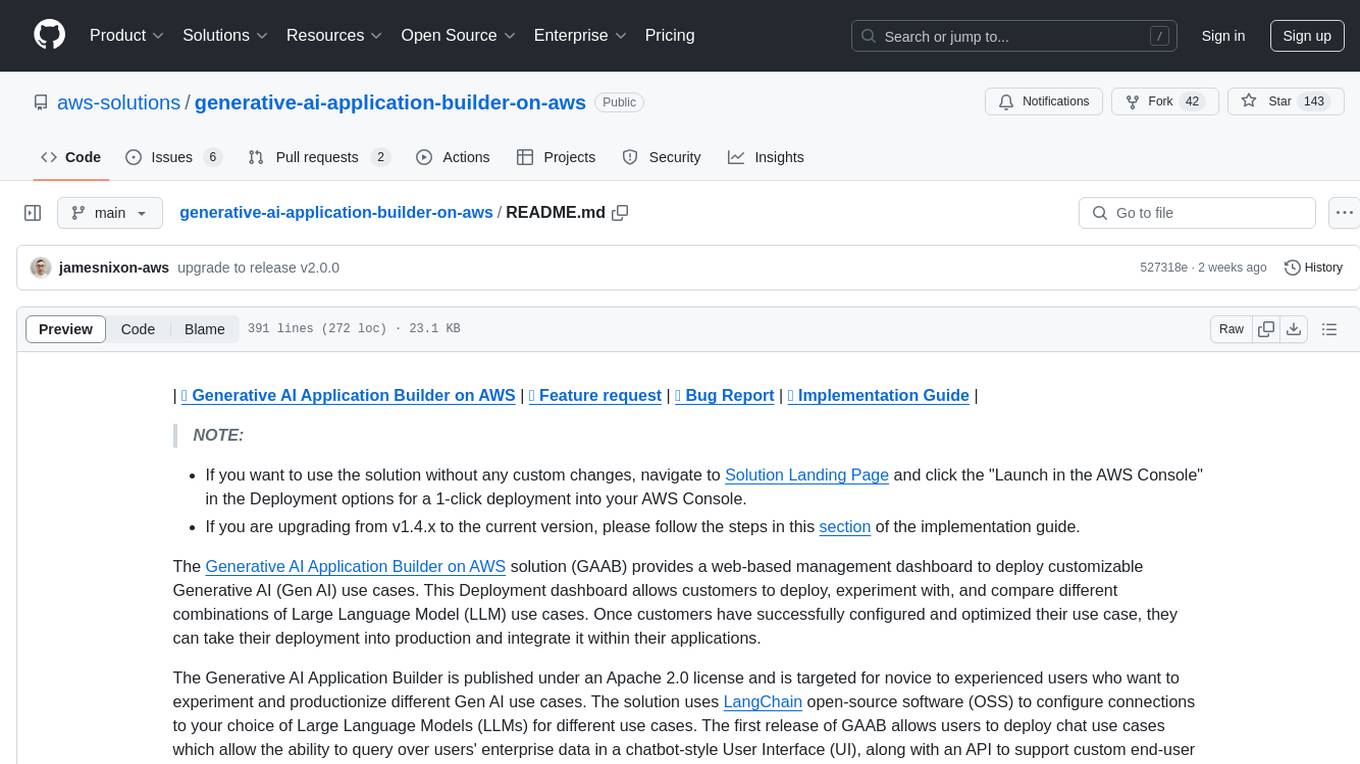
generative-ai-application-builder-on-aws
The Generative AI Application Builder on AWS (GAAB) is a solution that provides a web-based management dashboard for deploying customizable Generative AI (Gen AI) use cases. Users can experiment with and compare different combinations of Large Language Model (LLM) use cases, configure and optimize their use cases, and integrate them into their applications for production. The solution is targeted at novice to experienced users who want to experiment and productionize different Gen AI use cases. It uses LangChain open-source software to configure connections to Large Language Models (LLMs) for various use cases, with the ability to deploy chat use cases that allow querying over users' enterprise data in a chatbot-style User Interface (UI) and support custom end-user implementations through an API.
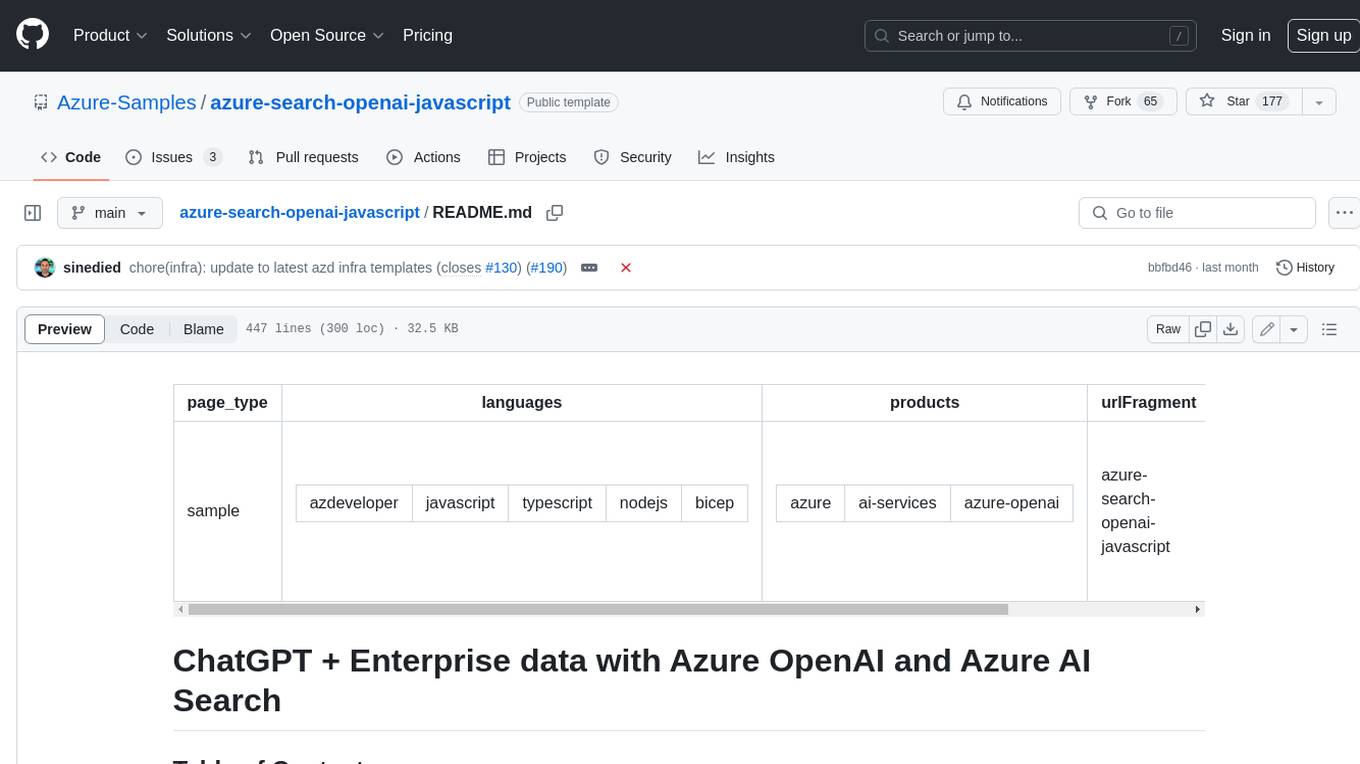
azure-search-openai-javascript
This sample demonstrates a few approaches for creating ChatGPT-like experiences over your own data using the Retrieval Augmented Generation pattern. It uses Azure OpenAI Service to access the ChatGPT model (gpt-35-turbo), and Azure AI Search for data indexing and retrieval.
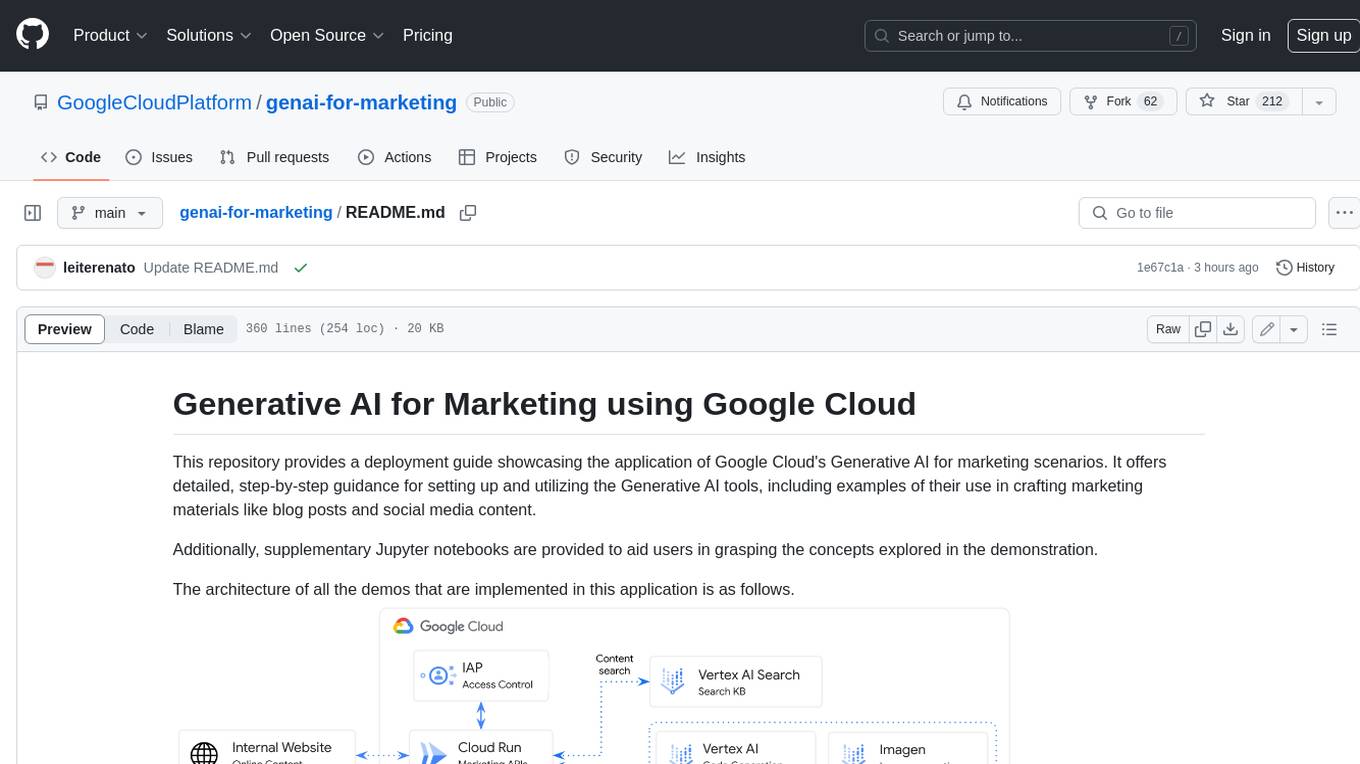
genai-for-marketing
This repository provides a deployment guide for utilizing Google Cloud's Generative AI tools in marketing scenarios. It includes step-by-step instructions, examples of crafting marketing materials, and supplementary Jupyter notebooks. The demos cover marketing insights, audience analysis, trendspotting, content search, content generation, and workspace integration. Users can access and visualize marketing data, analyze trends, improve search experience, and generate compelling content. The repository structure includes backend APIs, frontend code, sample notebooks, templates, and installation scripts.
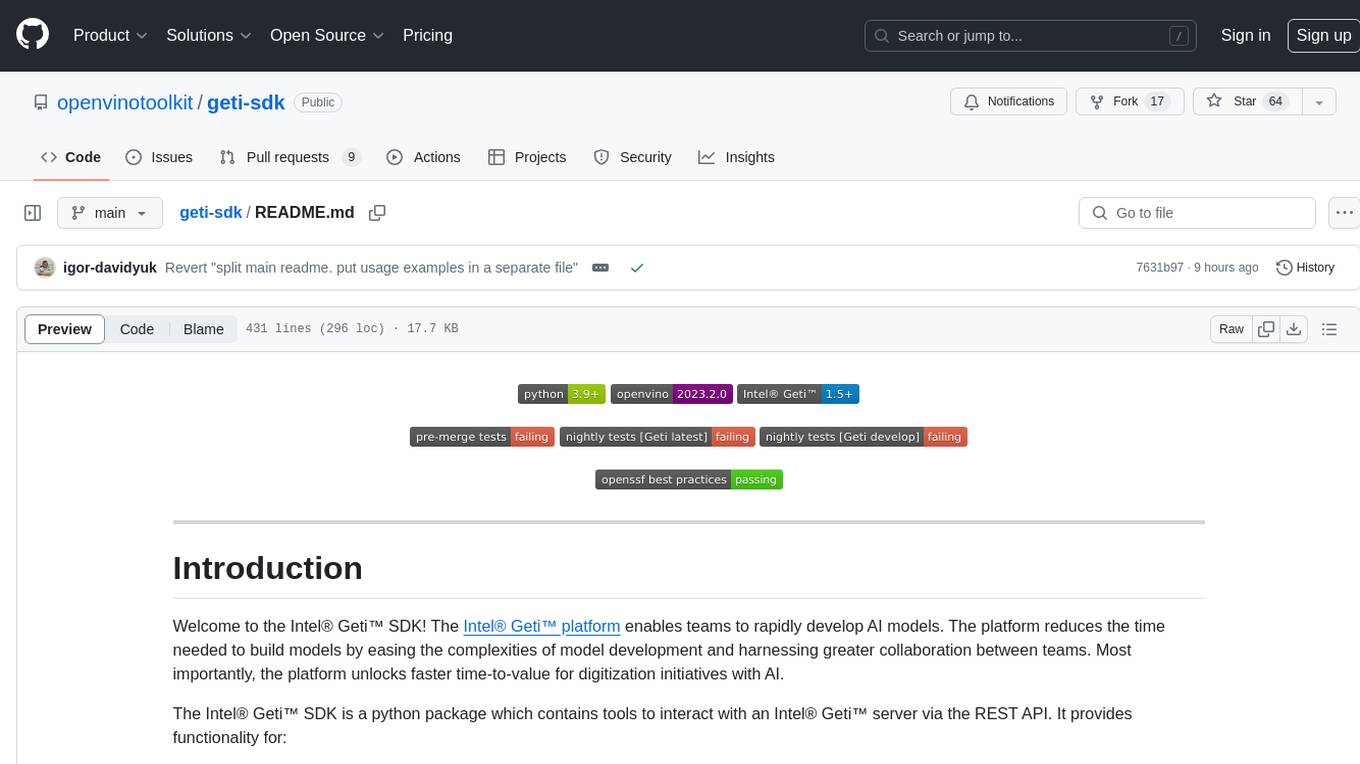
geti-sdk
The Intel® Geti™ SDK is a python package that enables teams to rapidly develop AI models by easing the complexities of model development and enhancing collaboration between teams. It provides tools to interact with an Intel® Geti™ server via the REST API, allowing for project creation, downloading, uploading, deploying for local inference with OpenVINO, setting project and model configuration, launching and monitoring training jobs, and media upload and prediction. The SDK also includes tutorial-style Jupyter notebooks demonstrating its usage.
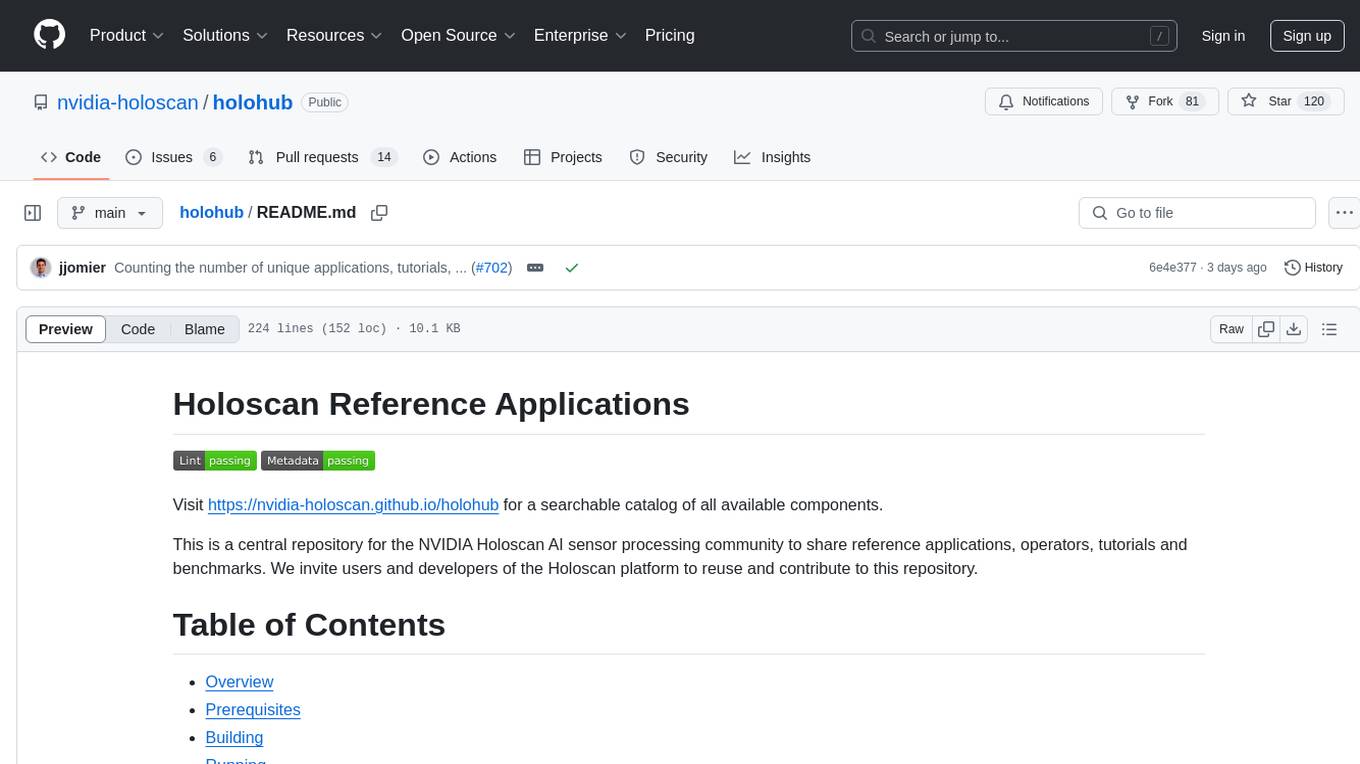
holohub
Holohub is a central repository for the NVIDIA Holoscan AI sensor processing community to share reference applications, operators, tutorials, and benchmarks. It includes example applications, community components, package configurations, and tutorials. Users and developers of the Holoscan platform are invited to reuse and contribute to this repository. The repository provides detailed instructions on prerequisites, building, running applications, contributing, and glossary terms. It also offers a searchable catalog of available components on the Holoscan SDK User Guide website.
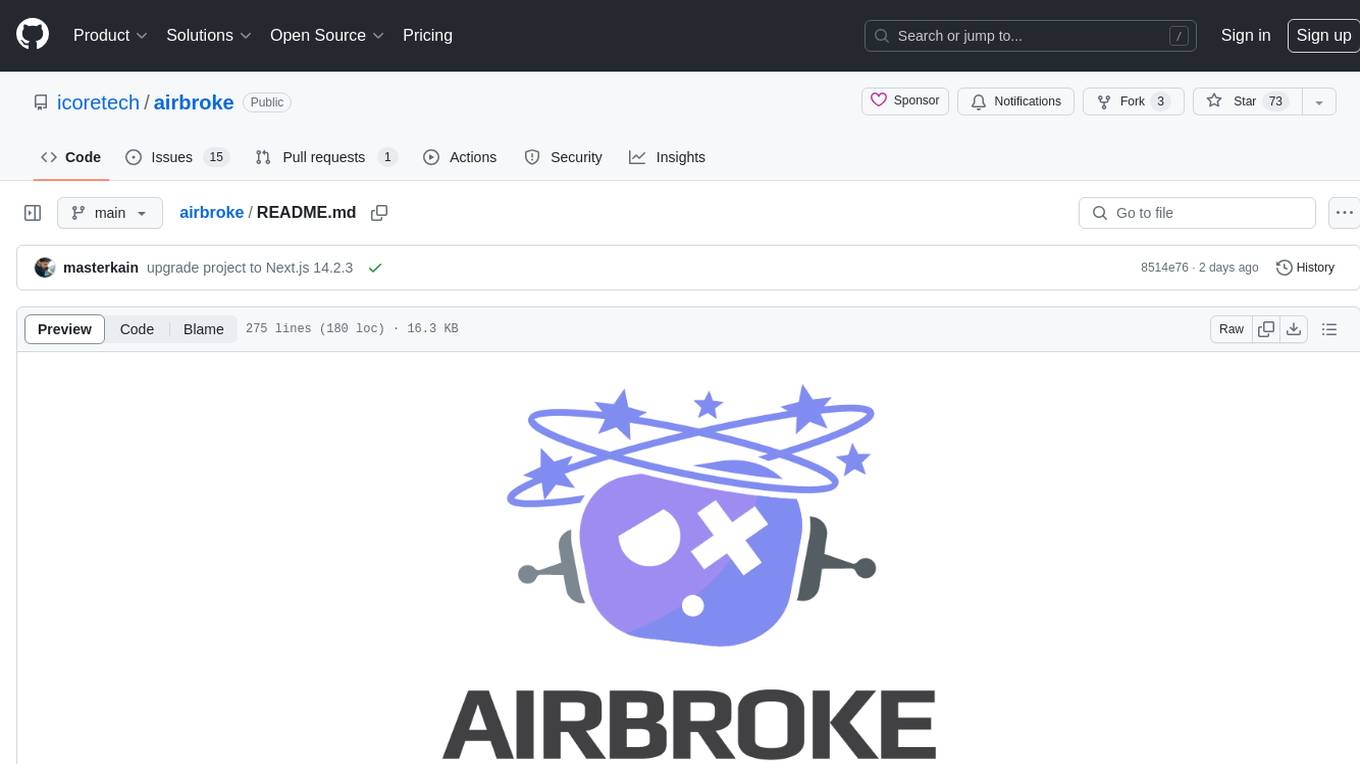
airbroke
Airbroke is an open-source error catcher tool designed for modern web applications. It provides a PostgreSQL-based backend with an Airbrake-compatible HTTP collector endpoint and a React-based frontend for error management. The tool focuses on simplicity, maintaining a small database footprint even under heavy data ingestion. Users can ask AI about issues, replay HTTP exceptions, and save/manage bookmarks for important occurrences. Airbroke supports multiple OAuth providers for secure user authentication and offers occurrence charts for better insights into error occurrences. The tool can be deployed in various ways, including building from source, using Docker images, deploying on Vercel, Render.com, Kubernetes with Helm, or Docker Compose. It requires Node.js, PostgreSQL, and specific system resources for deployment.
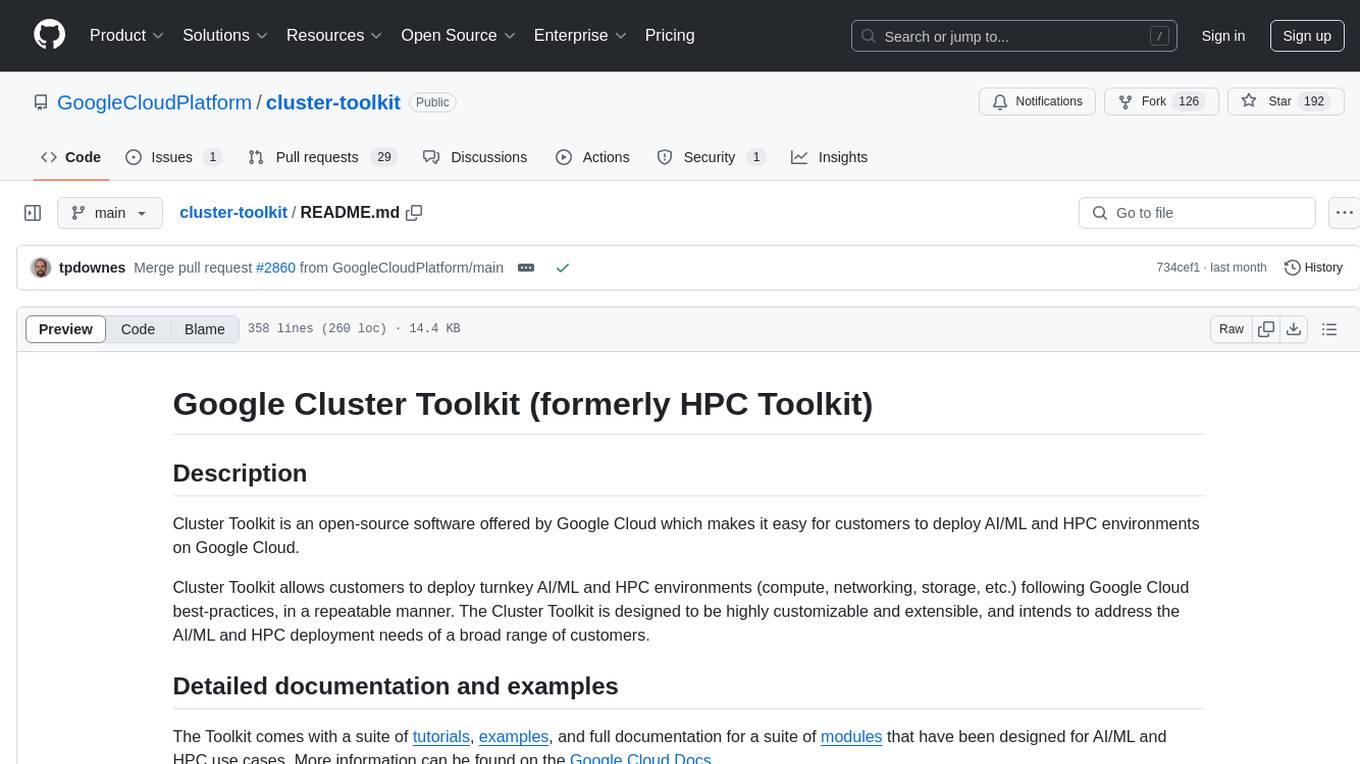
cluster-toolkit
Cluster Toolkit is an open-source software by Google Cloud for deploying AI/ML and HPC environments on Google Cloud. It allows easy deployment following best practices, with high customization and extensibility. The toolkit includes tutorials, examples, and documentation for various modules designed for AI/ML and HPC use cases.
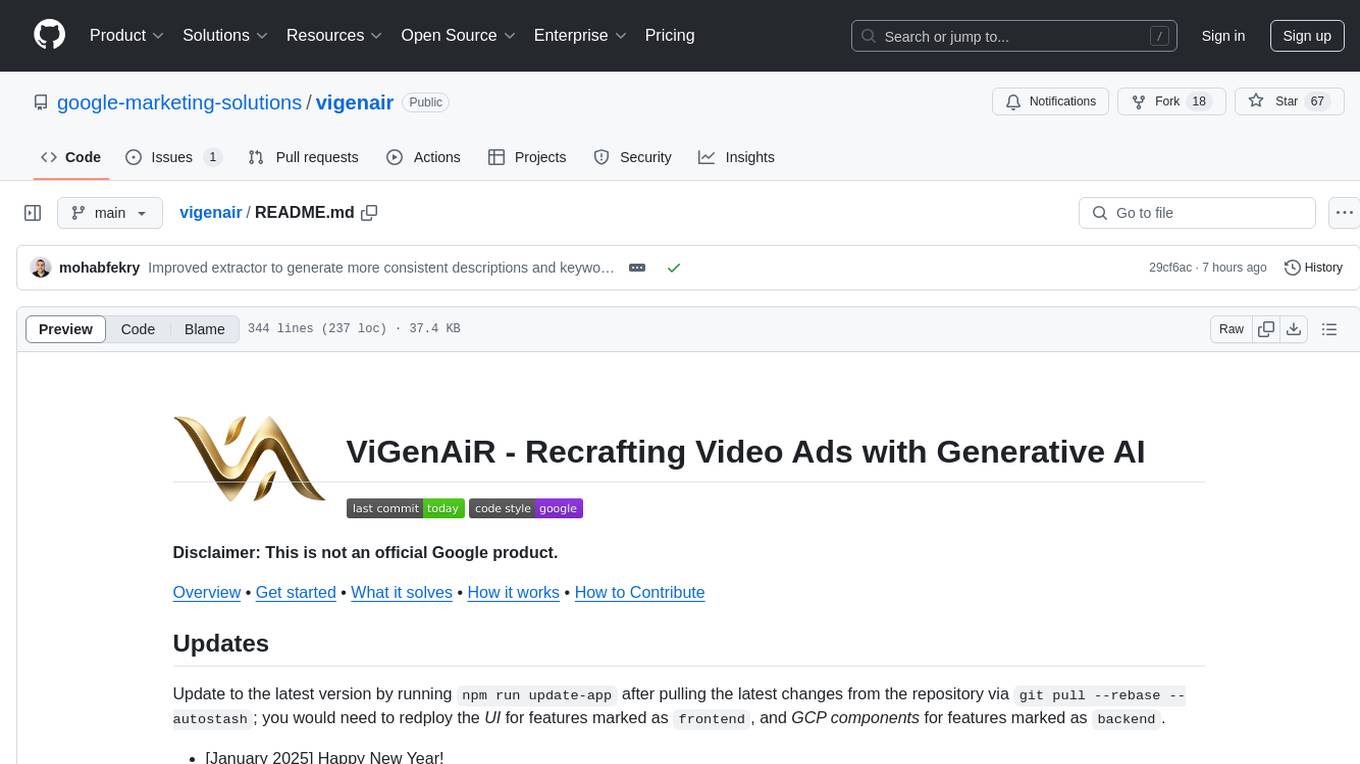
vigenair
ViGenAiR is a tool that harnesses the power of Generative AI models on Google Cloud Platform to automatically transform long-form Video Ads into shorter variants, targeting different audiences. It generates video, image, and text assets for Demand Gen and YouTube video campaigns. Users can steer the model towards generating desired videos, conduct A/B testing, and benefit from various creative features. The tool offers benefits like diverse inventory, compelling video ads, creative excellence, user control, and performance insights. ViGenAiR works by analyzing video content, splitting it into coherent segments, and generating variants following Google's best practices for effective ads.
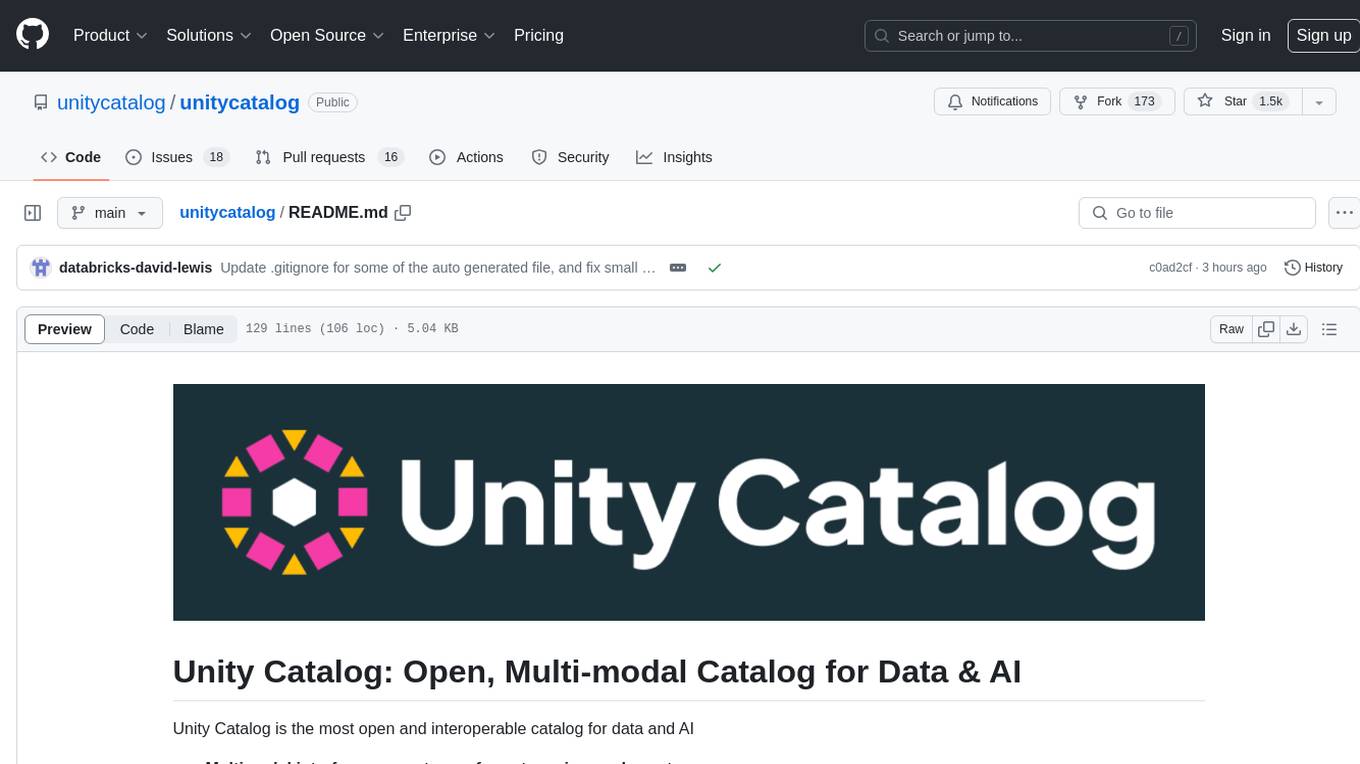
unitycatalog
Unity Catalog is an open and interoperable catalog for data and AI, supporting multi-format tables, unstructured data, and AI assets. It offers plugin support for extensibility and interoperates with Delta Sharing protocol. The catalog is fully open with OpenAPI spec and OSS implementation, providing unified governance for data and AI with asset-level access control enforced through REST APIs.
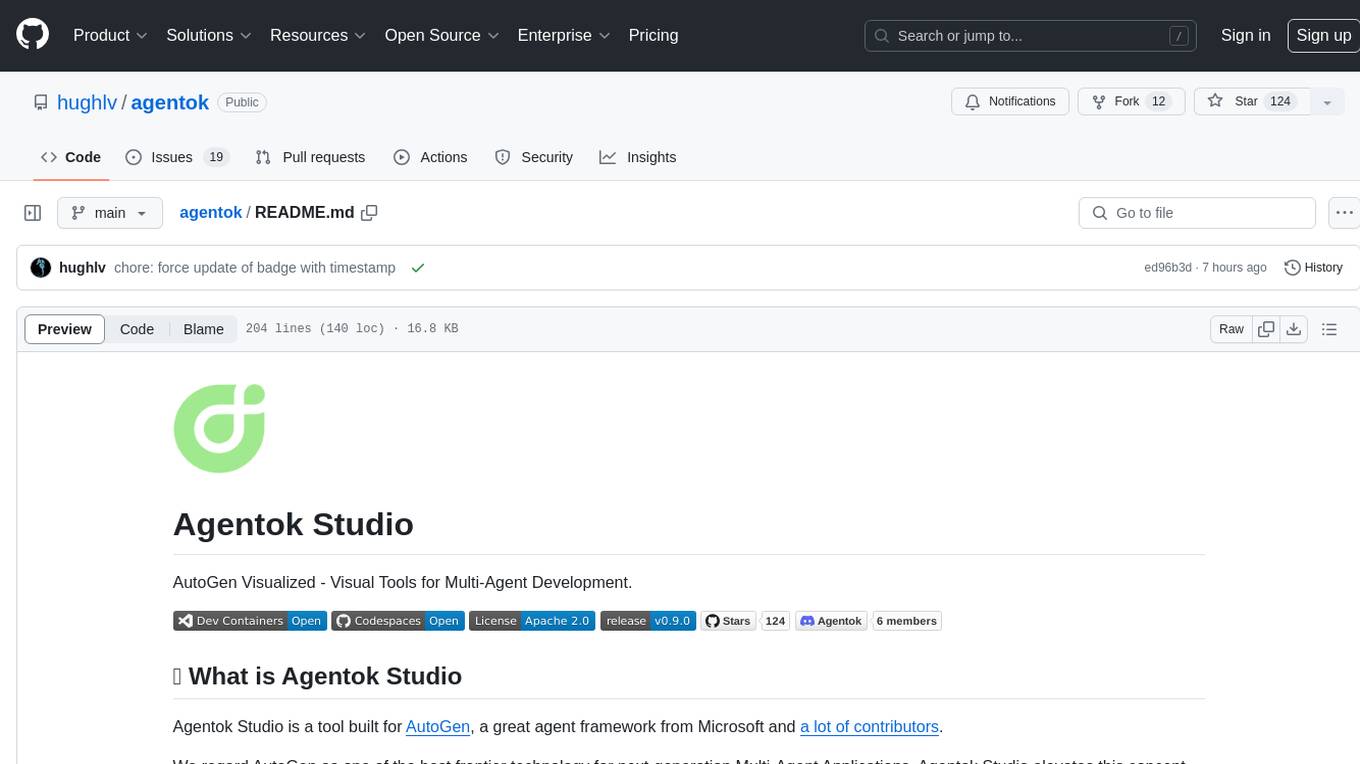
agentok
Agentok Studio is a visual tool built for AutoGen, a cutting-edge agent framework from Microsoft and various contributors. It offers intuitive visual tools to simplify the construction and management of complex agent-based workflows. Users can create workflows visually as graphs, chat with agents, and share flow templates. The tool is designed to streamline the development process for creators and developers working on next-generation Multi-Agent Applications.
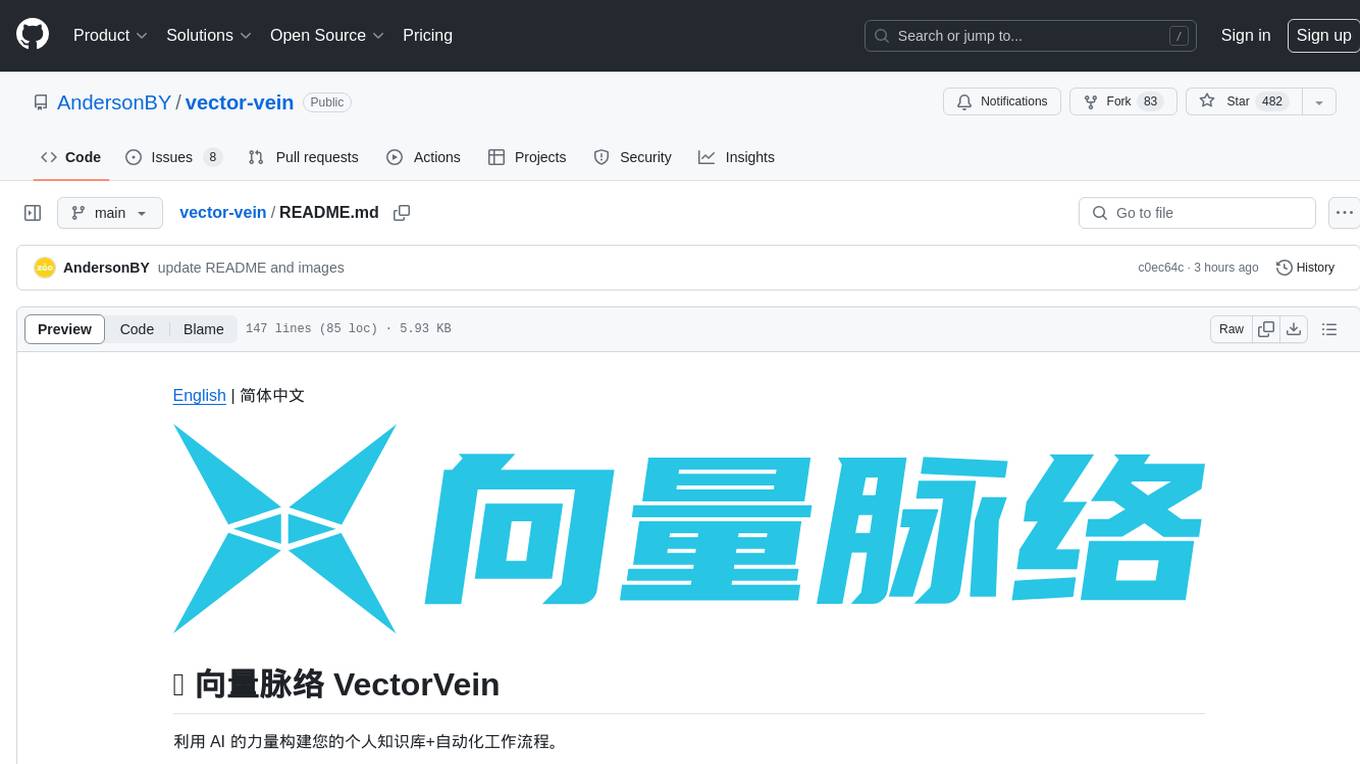
vector-vein
VectorVein is a no-code AI workflow software inspired by LangChain and langflow, aiming to combine the powerful capabilities of large language models and enable users to achieve intelligent and automated daily workflows through simple drag-and-drop actions. Users can create powerful workflows without the need for programming, automating all tasks with ease. The software allows users to define inputs, outputs, and processing methods to create customized workflow processes for various tasks such as translation, mind mapping, summarizing web articles, and automatic categorization of customer reviews.
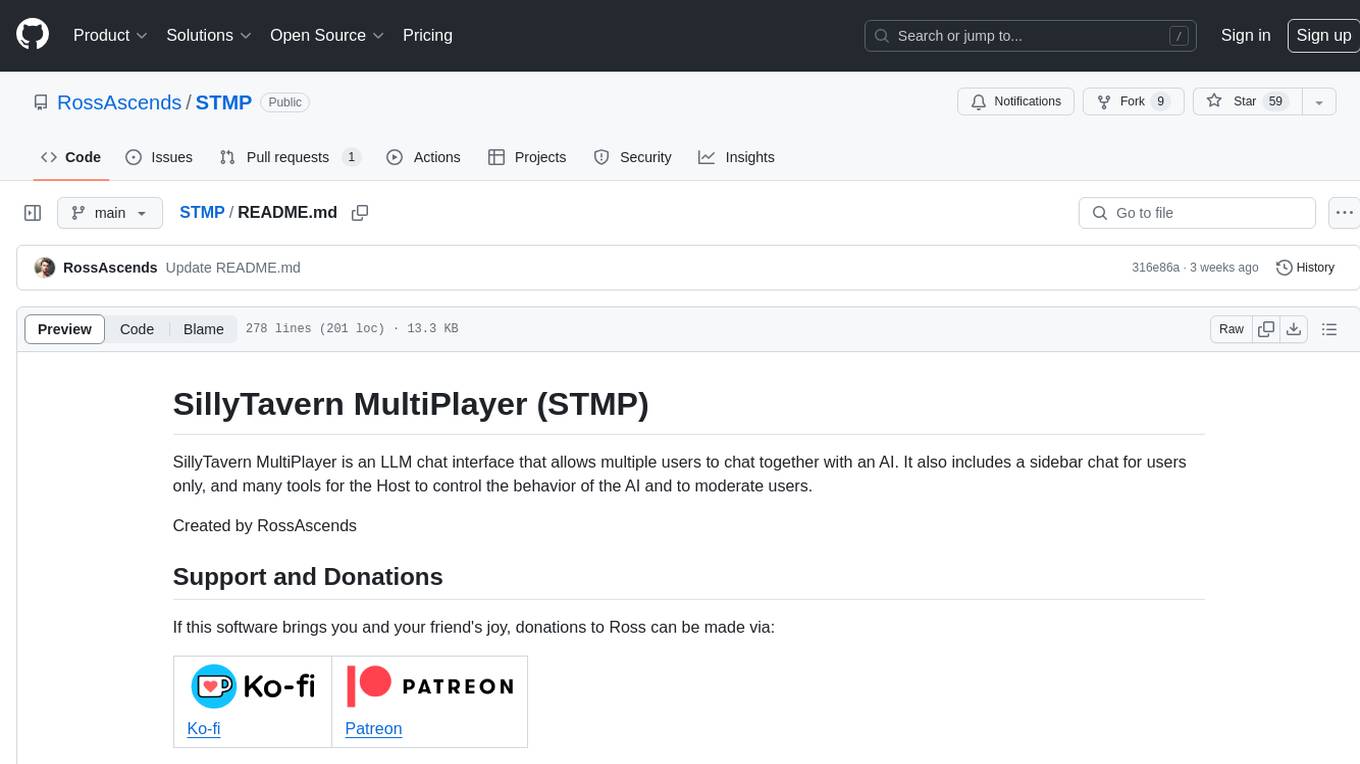
STMP
SillyTavern MultiPlayer (STMP) is an LLM chat interface that enables multiple users to chat with an AI. It features a sidebar chat for users, tools for the Host to manage the AI's behavior and moderate users. Users can change display names, chat in different windows, and the Host can control AI settings. STMP supports Text Completions, Chat Completions, and HordeAI. Users can add/edit APIs, manage past chats, view user lists, and control delays. Hosts have access to various controls, including AI configuration, adding presets, and managing characters. Planned features include smarter retry logic, host controls enhancements, and quality of life improvements like user list fading and highlighting exact usernames in AI responses.
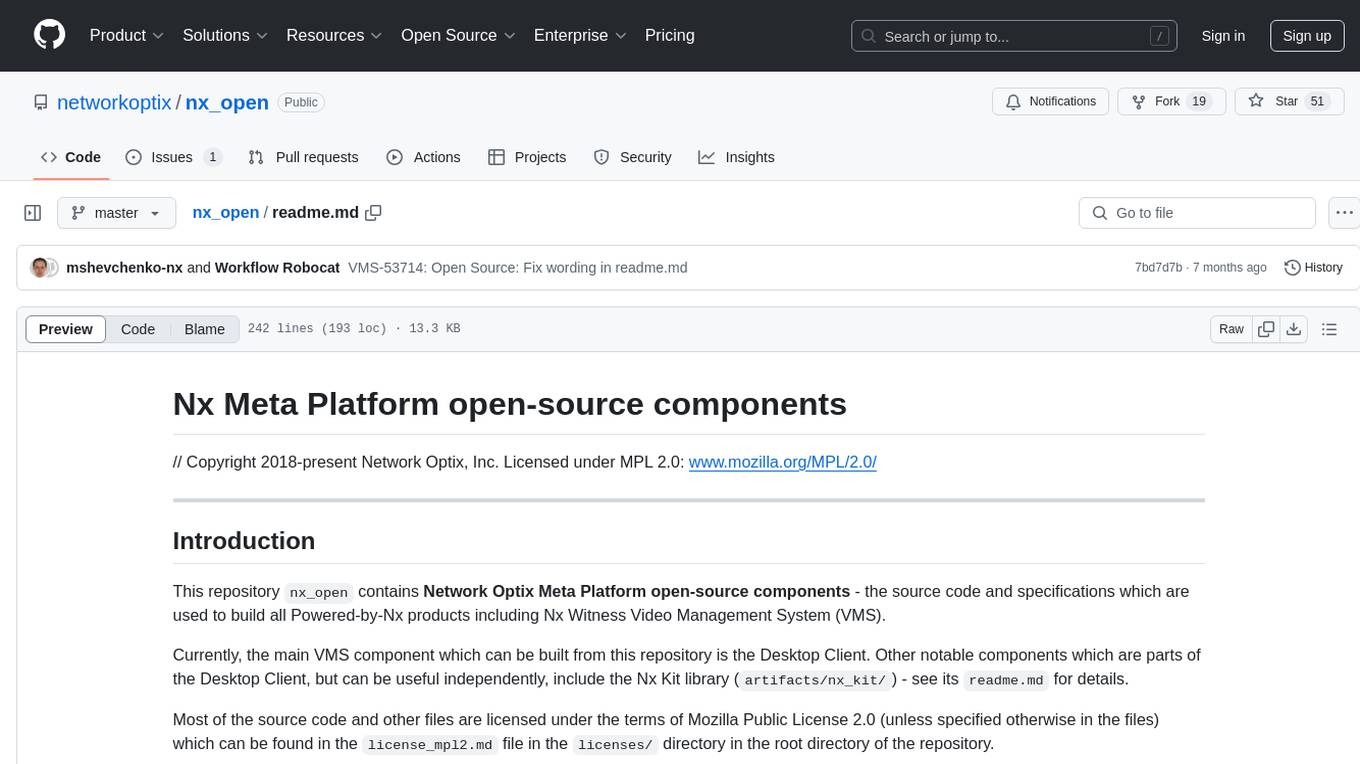
nx_open
The `nx_open` repository contains open-source components for the Network Optix Meta Platform, used to build products like Nx Witness Video Management System. It includes source code, specifications, and a Desktop Client. The repository is licensed under Mozilla Public License 2.0. Users can build the Desktop Client and customize it using a zip file. The build environment supports Windows, Linux, and macOS platforms with specific prerequisites. The repository provides scripts for building, signing executable files, and running the Desktop Client. Compatibility with VMS Server versions is crucial, and automatic VMS updates are disabled for the open-source Desktop Client.
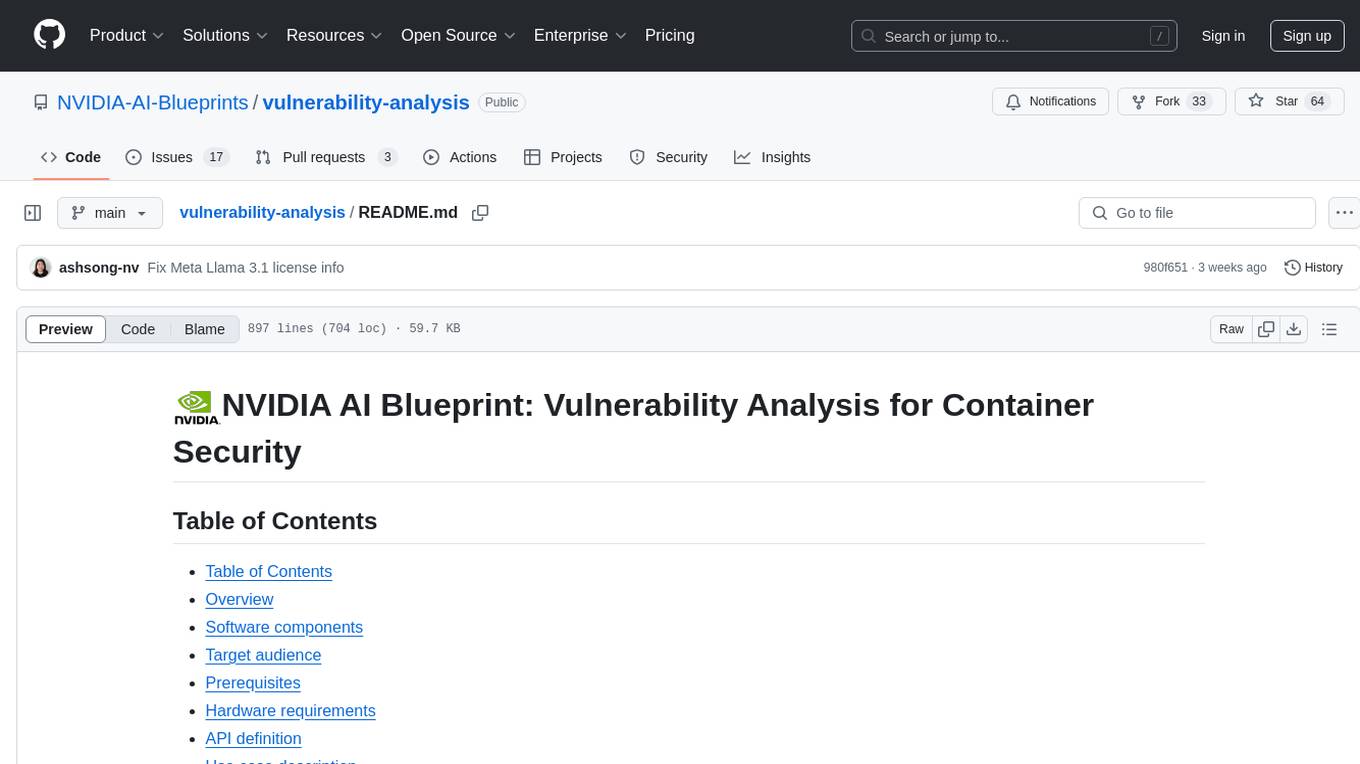
vulnerability-analysis
The NVIDIA AI Blueprint for Vulnerability Analysis for Container Security showcases accelerated analysis on common vulnerabilities and exposures (CVE) at an enterprise scale, reducing mitigation time from days to seconds. It enables security analysts to determine software package vulnerabilities using large language models (LLMs) and retrieval-augmented generation (RAG). The blueprint is designed for security analysts, IT engineers, and AI practitioners in cybersecurity. It requires NVAIE developer license and API keys for vulnerability databases, search engines, and LLM model services. Hardware requirements include L40 GPU for pipeline operation and optional LLM NIM and Embedding NIM. The workflow involves LLM pipeline for CVE impact analysis, utilizing LLM planner, agent, and summarization nodes. The blueprint uses NVIDIA NIM microservices and Morpheus Cybersecurity AI SDK for vulnerability analysis.
For similar tasks
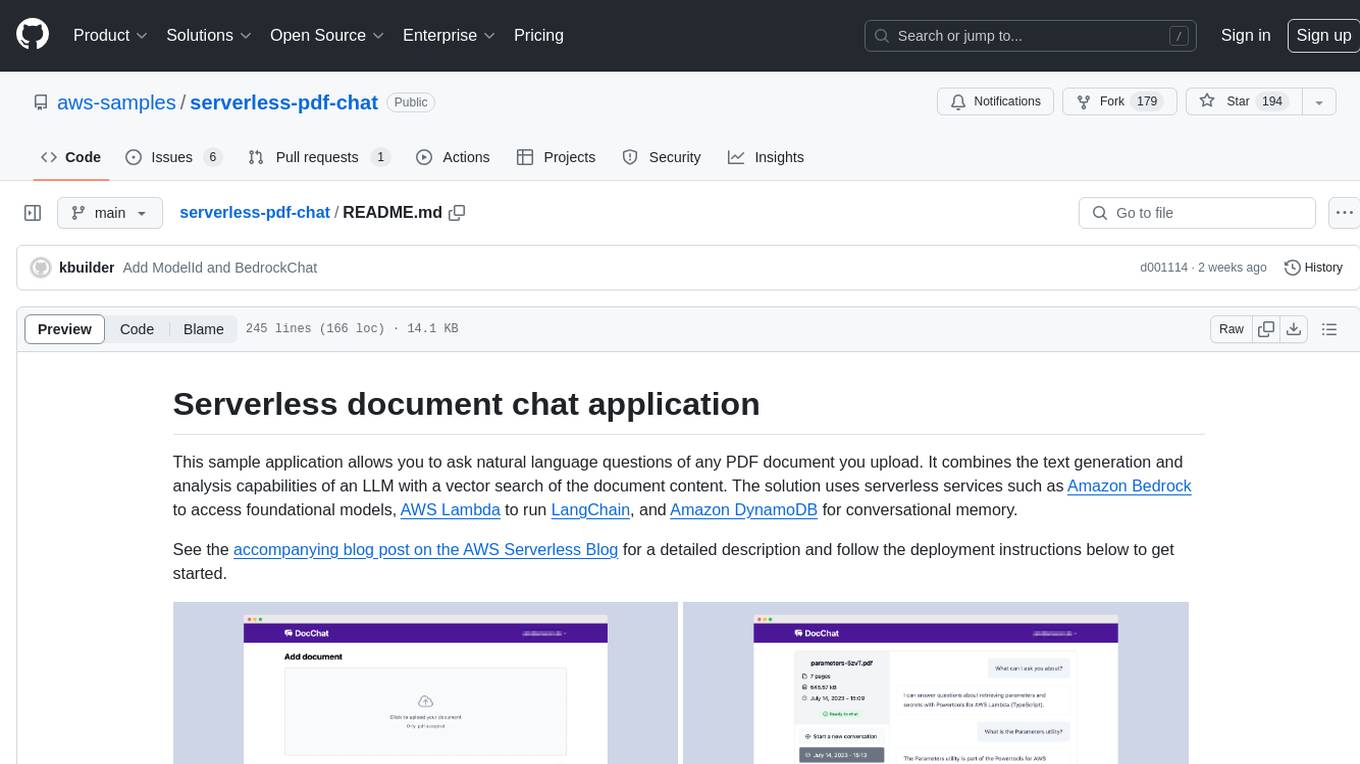
serverless-pdf-chat
The serverless-pdf-chat repository contains a sample application that allows users to ask natural language questions of any PDF document they upload. It leverages serverless services like Amazon Bedrock, AWS Lambda, and Amazon DynamoDB to provide text generation and analysis capabilities. The application architecture involves uploading a PDF document to an S3 bucket, extracting metadata, converting text to vectors, and using a LangChain to search for information related to user prompts. The application is not intended for production use and serves as a demonstration and educational tool.
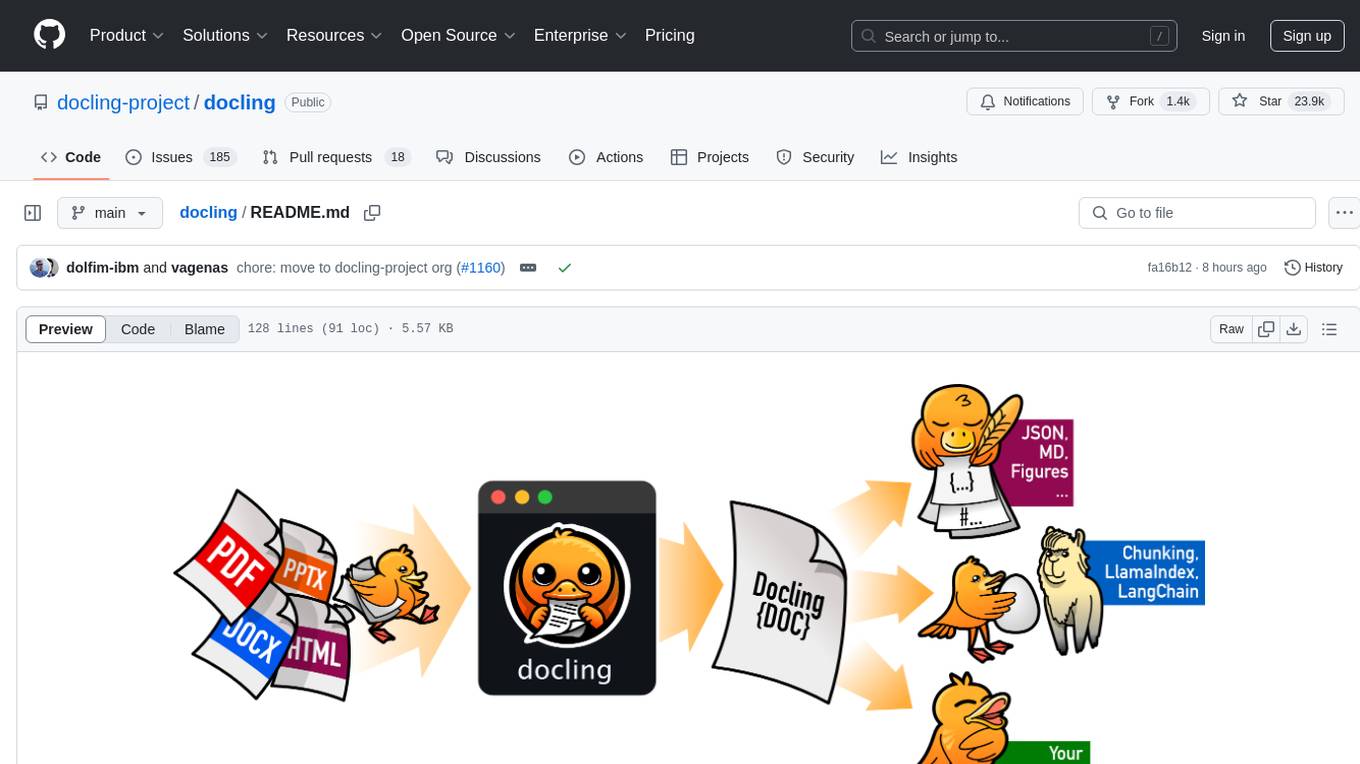
docling
Docling simplifies document processing, parsing diverse formats including advanced PDF understanding, and providing seamless integrations with the general AI ecosystem. It offers features such as parsing multiple document formats, advanced PDF understanding, unified DoclingDocument representation format, various export formats, local execution capabilities, plug-and-play integrations with agentic AI tools, extensive OCR support, and a simple CLI. Coming soon features include metadata extraction, visual language models, chart understanding, and complex chemistry understanding. Docling is installed via pip and works on macOS, Linux, and Windows environments. It provides detailed documentation, examples, integrations with popular frameworks, and support through the discussion section. The codebase is under the MIT license and has been developed by IBM.
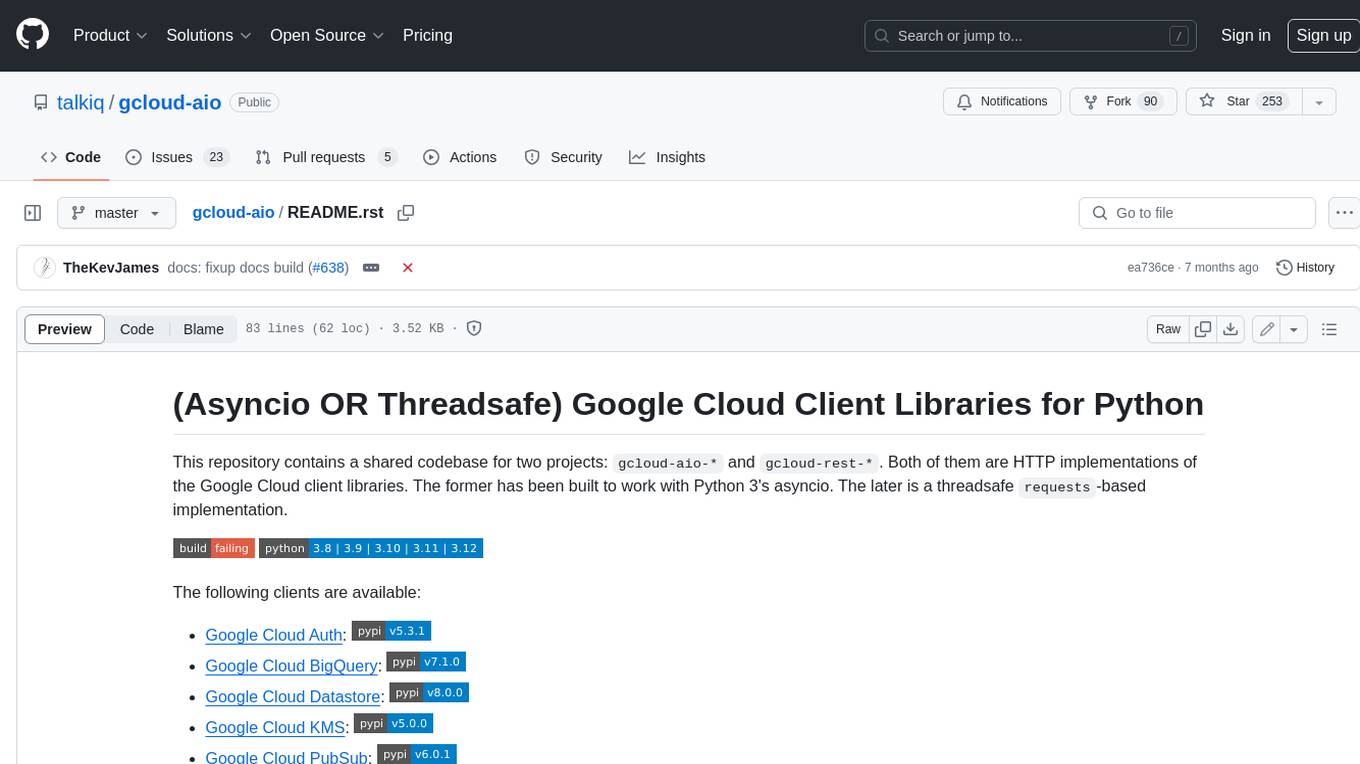
gcloud-aio
This repository contains shared codebase for two projects: gcloud-aio and gcloud-rest. gcloud-aio is built for Python 3's asyncio, while gcloud-rest is a threadsafe requests-based implementation. It provides clients for Google Cloud services like Auth, BigQuery, Datastore, KMS, PubSub, Storage, and Task Queue. Users can install the library using pip and refer to the documentation for usage details. Developers can contribute to the project by following the contribution guide.
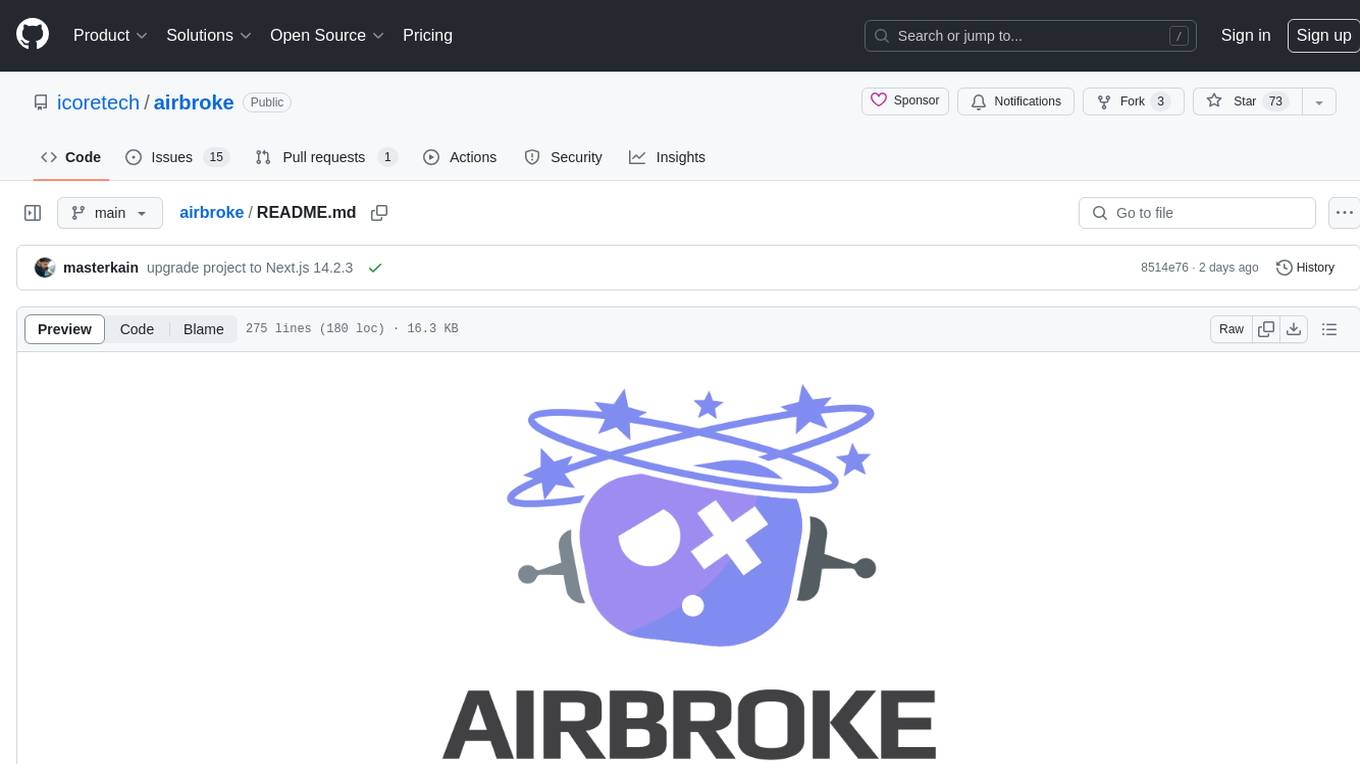
airbroke
Airbroke is an open-source error catcher tool designed for modern web applications. It provides a PostgreSQL-based backend with an Airbrake-compatible HTTP collector endpoint and a React-based frontend for error management. The tool focuses on simplicity, maintaining a small database footprint even under heavy data ingestion. Users can ask AI about issues, replay HTTP exceptions, and save/manage bookmarks for important occurrences. Airbroke supports multiple OAuth providers for secure user authentication and offers occurrence charts for better insights into error occurrences. The tool can be deployed in various ways, including building from source, using Docker images, deploying on Vercel, Render.com, Kubernetes with Helm, or Docker Compose. It requires Node.js, PostgreSQL, and specific system resources for deployment.
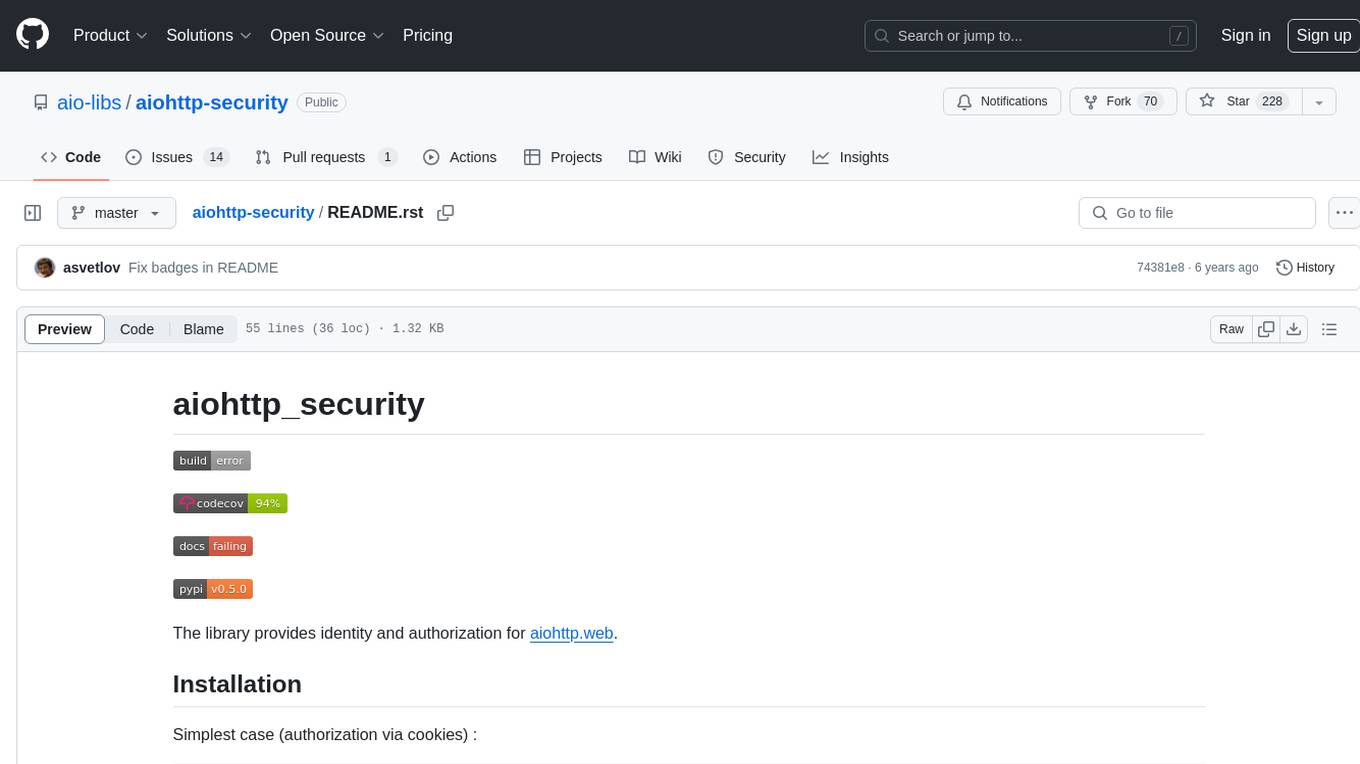
aiohttp-security
aiohttp_security is a library that provides identity and authorization for aiohttp.web. It offers features for handling authorization via cookies and supports aiohttp-session. The library includes examples for basic usage and database authentication, along with demos in the demo directory. For development, the library requires installation of specific requirements listed in the requirements-dev.txt file. aiohttp_security is licensed under the Apache 2 license.
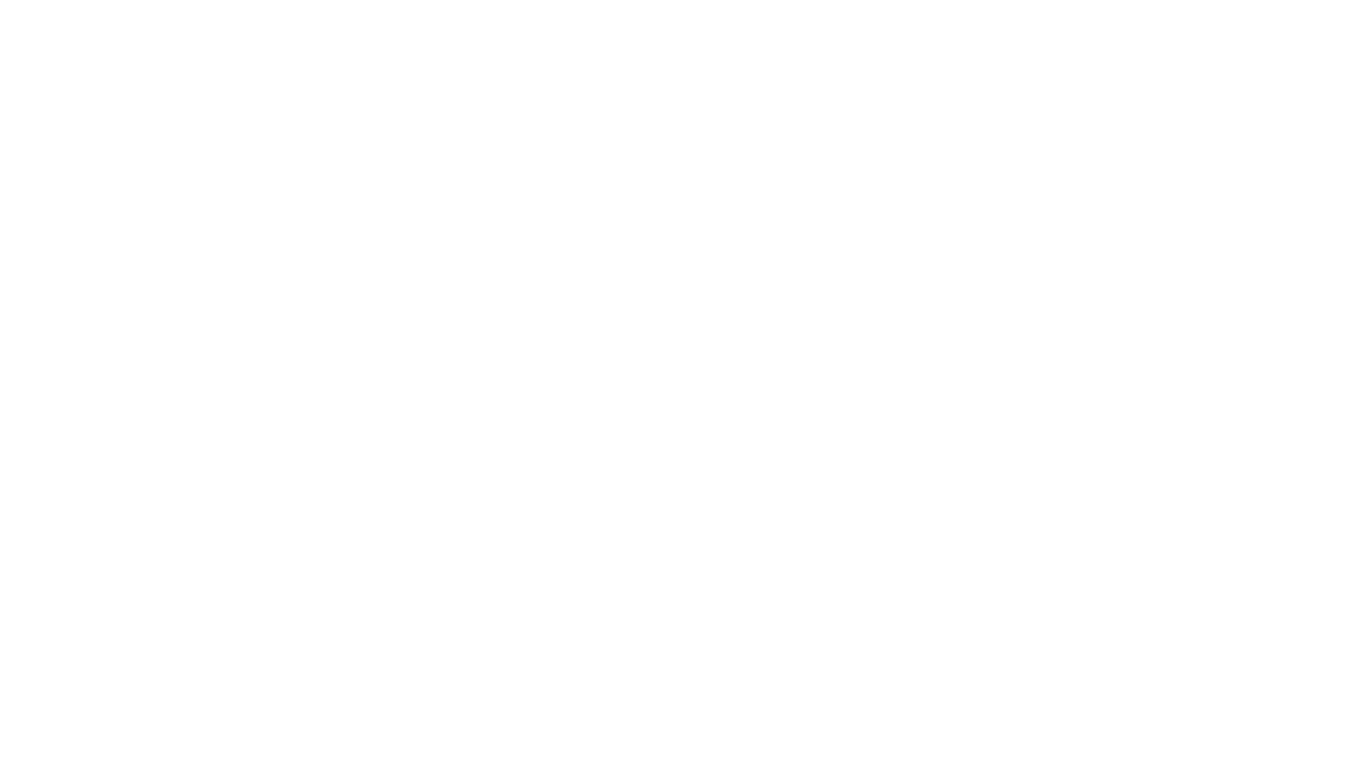
EvoMaster
EvoMaster is an open-source AI-driven tool that automatically generates system-level test cases for web/enterprise applications. It uses Evolutionary Algorithm and Dynamic Program Analysis to evolve test cases, maximizing code coverage and fault detection. It supports REST, GraphQL, and RPC APIs, with whitebox testing for JVM-compiled APIs. The tool generates JUnit tests in Java or Kotlin, focusing on fault detection, self-contained tests, SQL handling, and authentication. Known limitations include manual driver creation for whitebox testing and longer execution times for better results. EvoMaster has been funded by ERC and RCN grants.
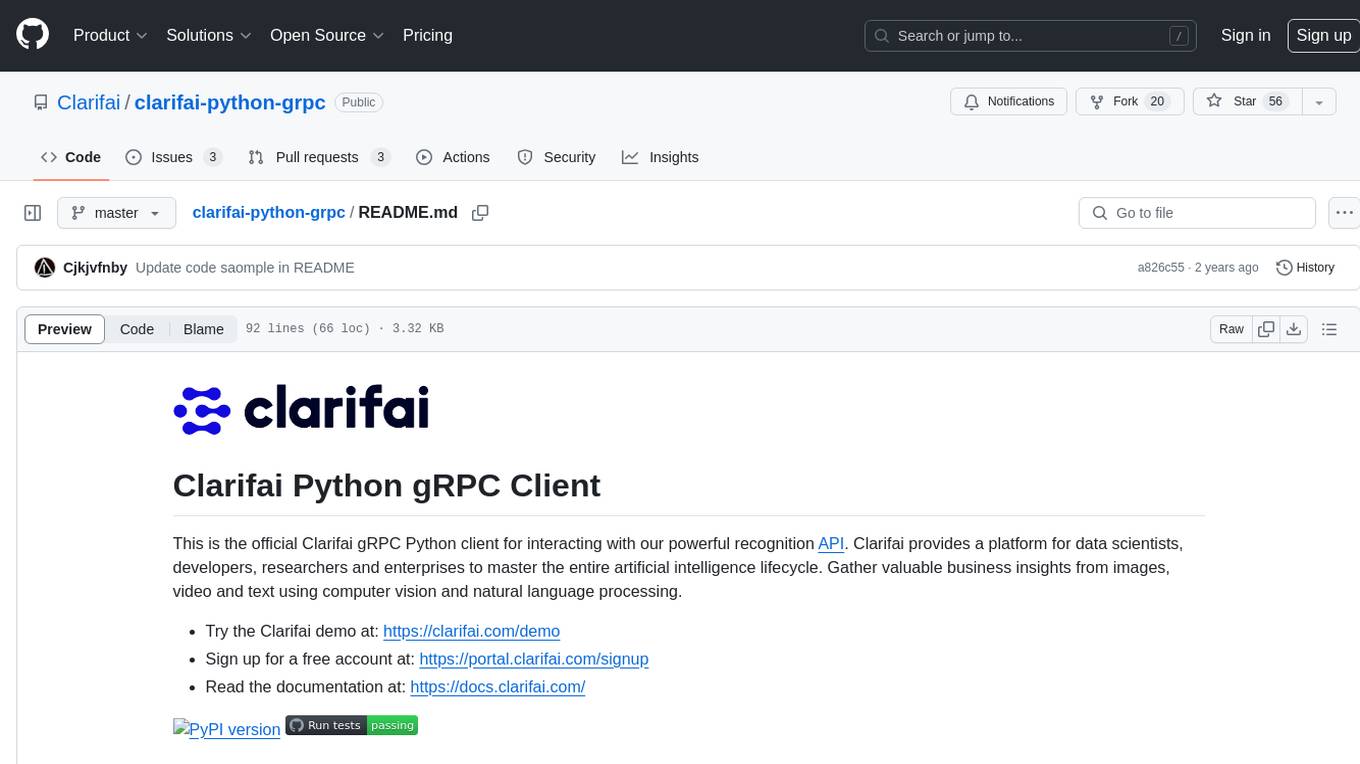
clarifai-python-grpc
This is the official Clarifai gRPC Python client for interacting with their recognition API. Clarifai offers a platform for data scientists, developers, researchers, and enterprises to utilize artificial intelligence for image, video, and text analysis through computer vision and natural language processing. The client allows users to authenticate, predict concepts in images, and access various functionalities provided by the Clarifai API. It follows a versioning scheme that aligns with the backend API updates and includes specific instructions for installation and troubleshooting. Users can explore the Clarifai demo, sign up for an account, and refer to the documentation for detailed information.
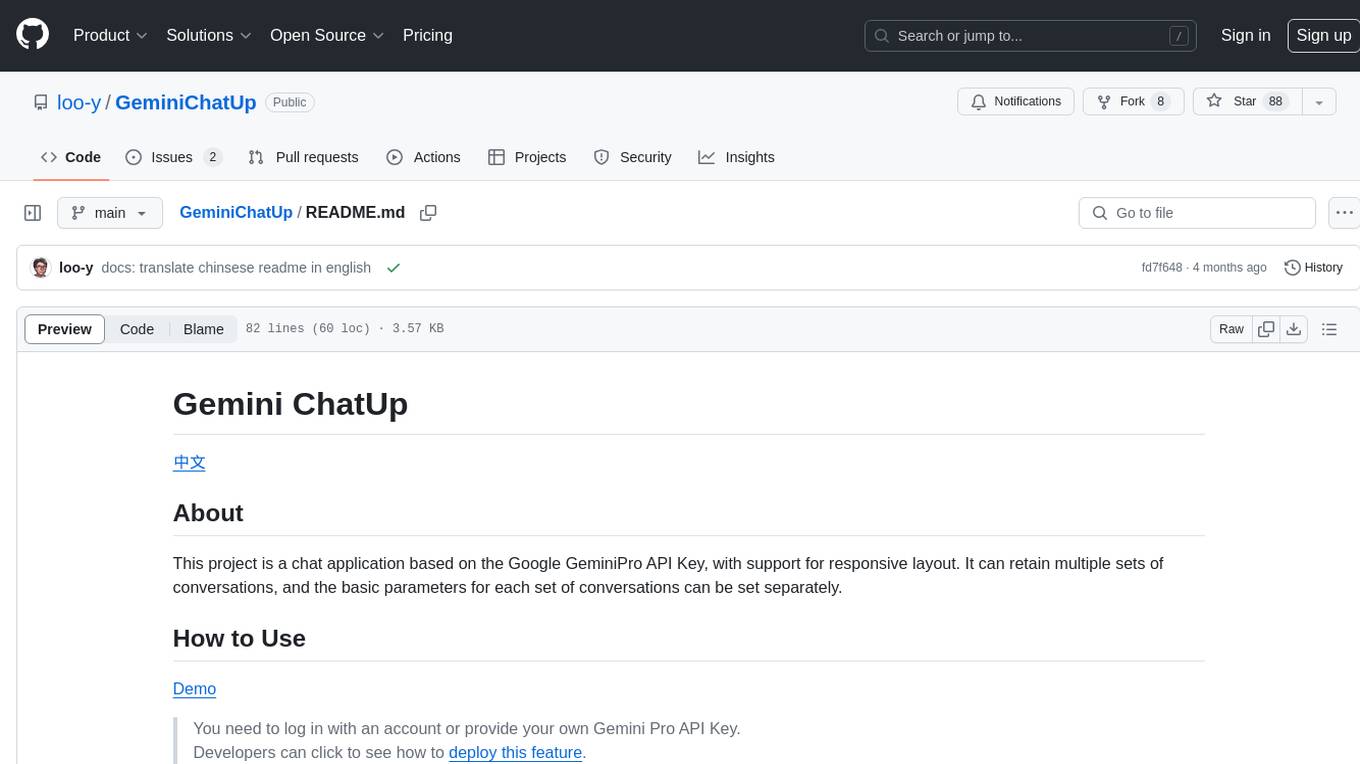
GeminiChatUp
Gemini ChatUp is a chat application utilizing the Google GeminiPro API Key. It supports responsive layout and can store multiple sets of conversations with customizable parameters for each set. Users can log in with a test account or provide their own API Key to deploy the feature. The application also offers user authentication through Edge config in Vercel, allowing users to add usernames and passwords in JSON format. Local deployment is possible by installing dependencies, setting up environment variables, and running the application locally.
For similar jobs
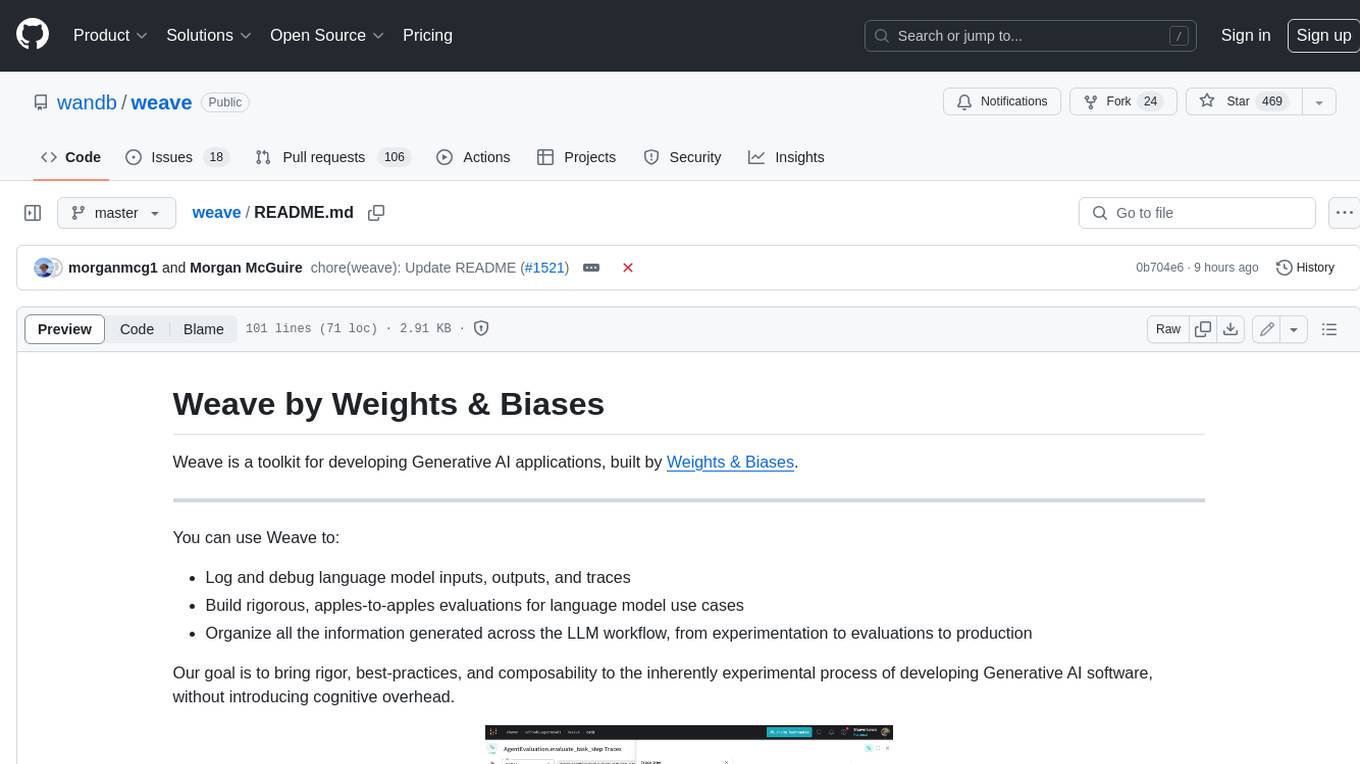
weave
Weave is a toolkit for developing Generative AI applications, built by Weights & Biases. With Weave, you can log and debug language model inputs, outputs, and traces; build rigorous, apples-to-apples evaluations for language model use cases; and organize all the information generated across the LLM workflow, from experimentation to evaluations to production. Weave aims to bring rigor, best-practices, and composability to the inherently experimental process of developing Generative AI software, without introducing cognitive overhead.
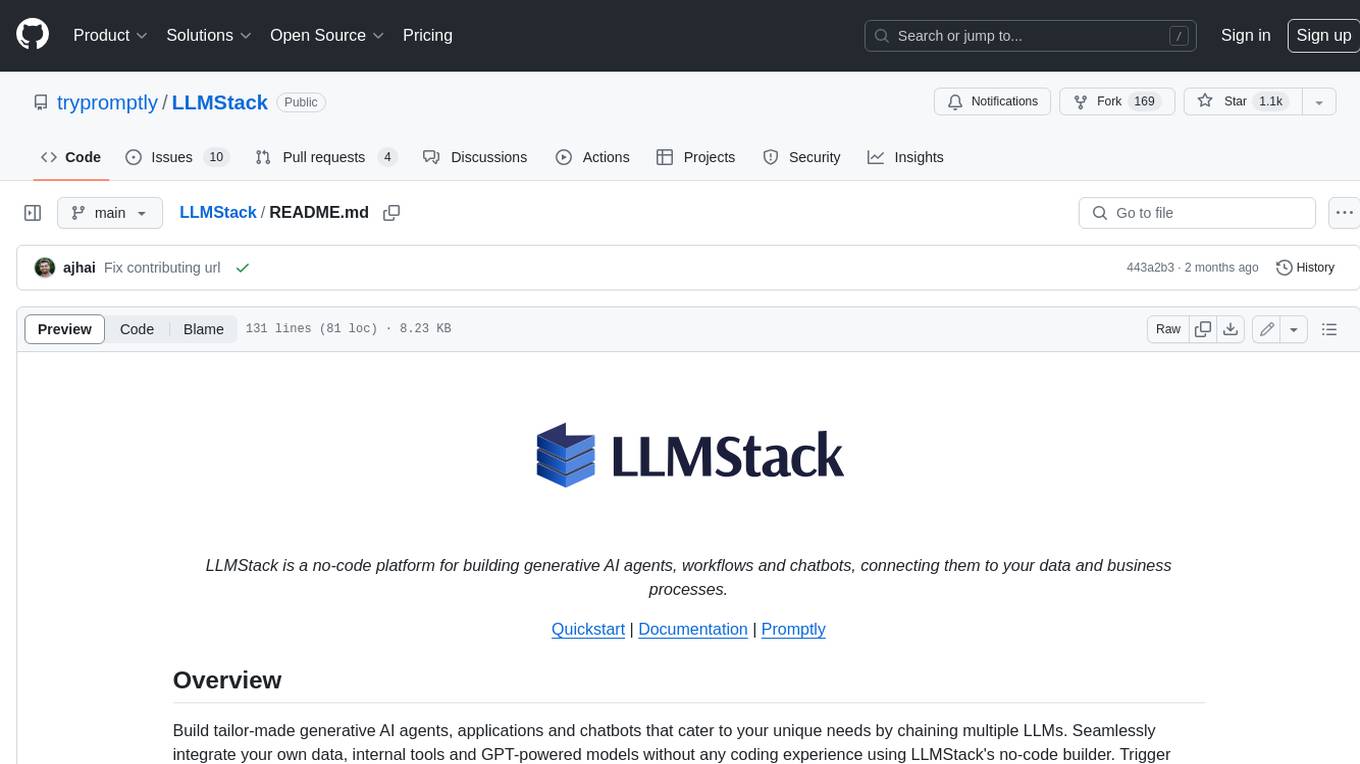
LLMStack
LLMStack is a no-code platform for building generative AI agents, workflows, and chatbots. It allows users to connect their own data, internal tools, and GPT-powered models without any coding experience. LLMStack can be deployed to the cloud or on-premise and can be accessed via HTTP API or triggered from Slack or Discord.
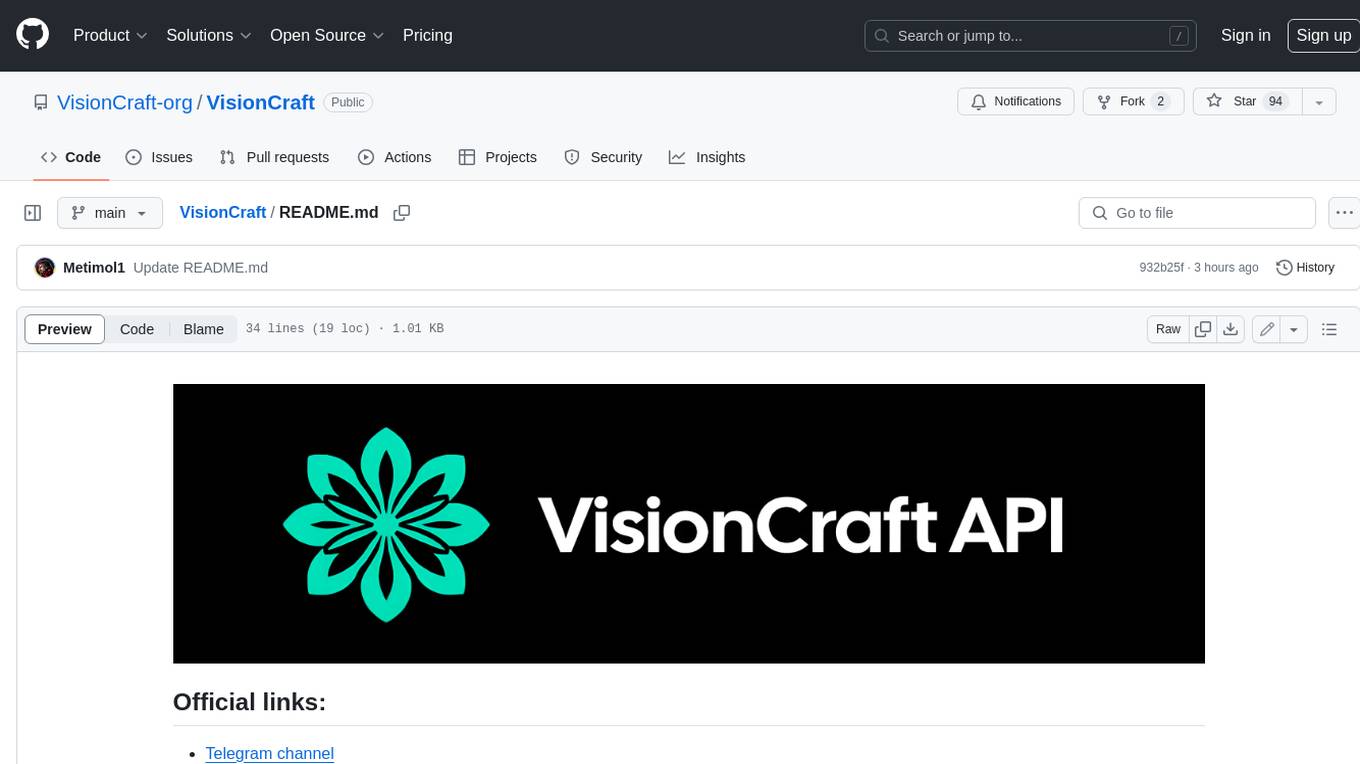
VisionCraft
The VisionCraft API is a free API for using over 100 different AI models. From images to sound.
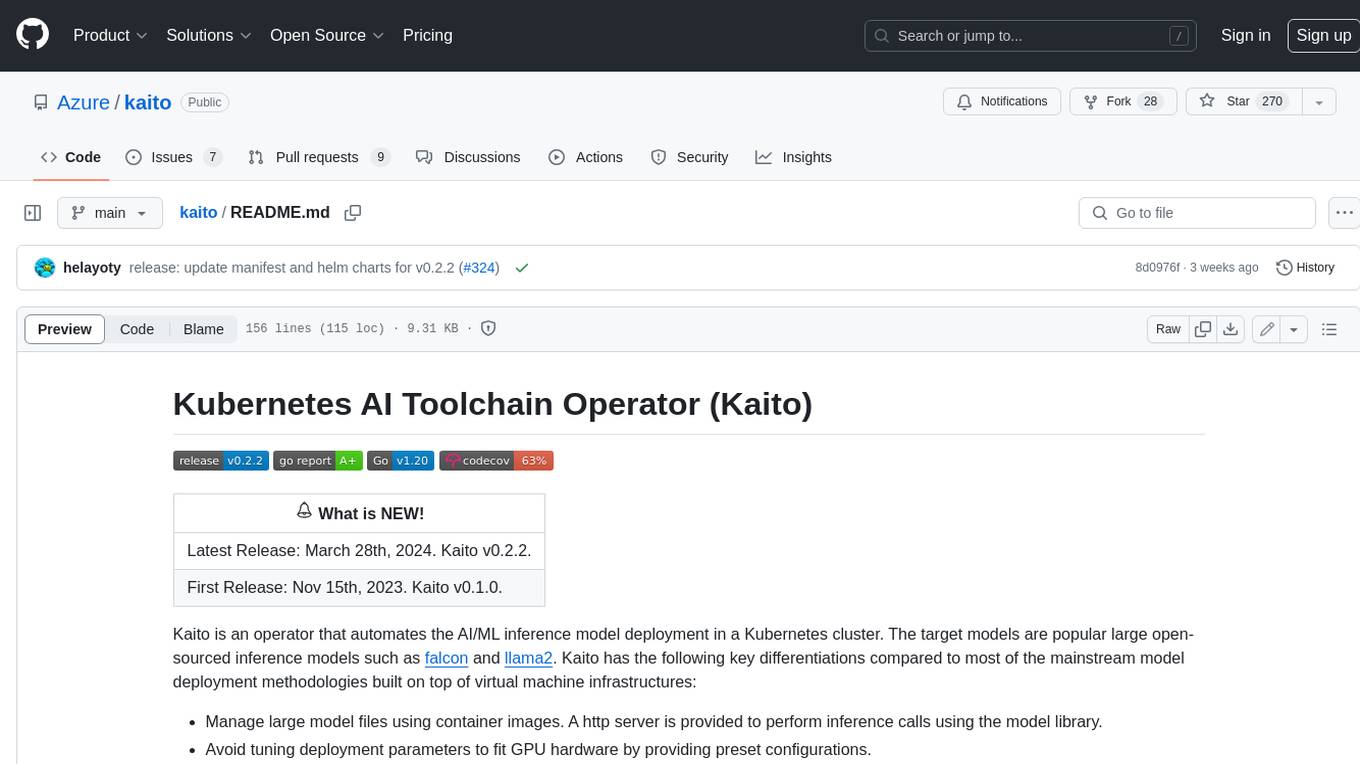
kaito
Kaito is an operator that automates the AI/ML inference model deployment in a Kubernetes cluster. It manages large model files using container images, avoids tuning deployment parameters to fit GPU hardware by providing preset configurations, auto-provisions GPU nodes based on model requirements, and hosts large model images in the public Microsoft Container Registry (MCR) if the license allows. Using Kaito, the workflow of onboarding large AI inference models in Kubernetes is largely simplified.
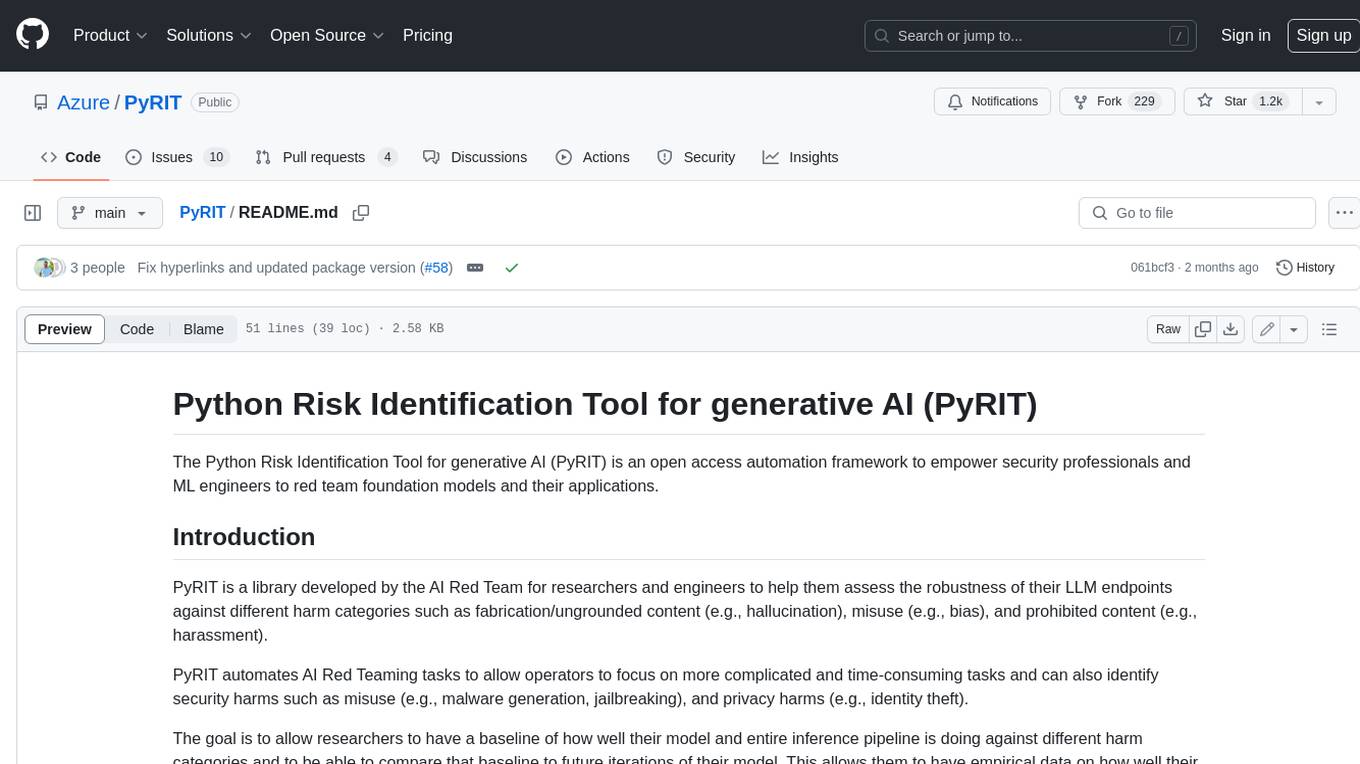
PyRIT
PyRIT is an open access automation framework designed to empower security professionals and ML engineers to red team foundation models and their applications. It automates AI Red Teaming tasks to allow operators to focus on more complicated and time-consuming tasks and can also identify security harms such as misuse (e.g., malware generation, jailbreaking), and privacy harms (e.g., identity theft). The goal is to allow researchers to have a baseline of how well their model and entire inference pipeline is doing against different harm categories and to be able to compare that baseline to future iterations of their model. This allows them to have empirical data on how well their model is doing today, and detect any degradation of performance based on future improvements.
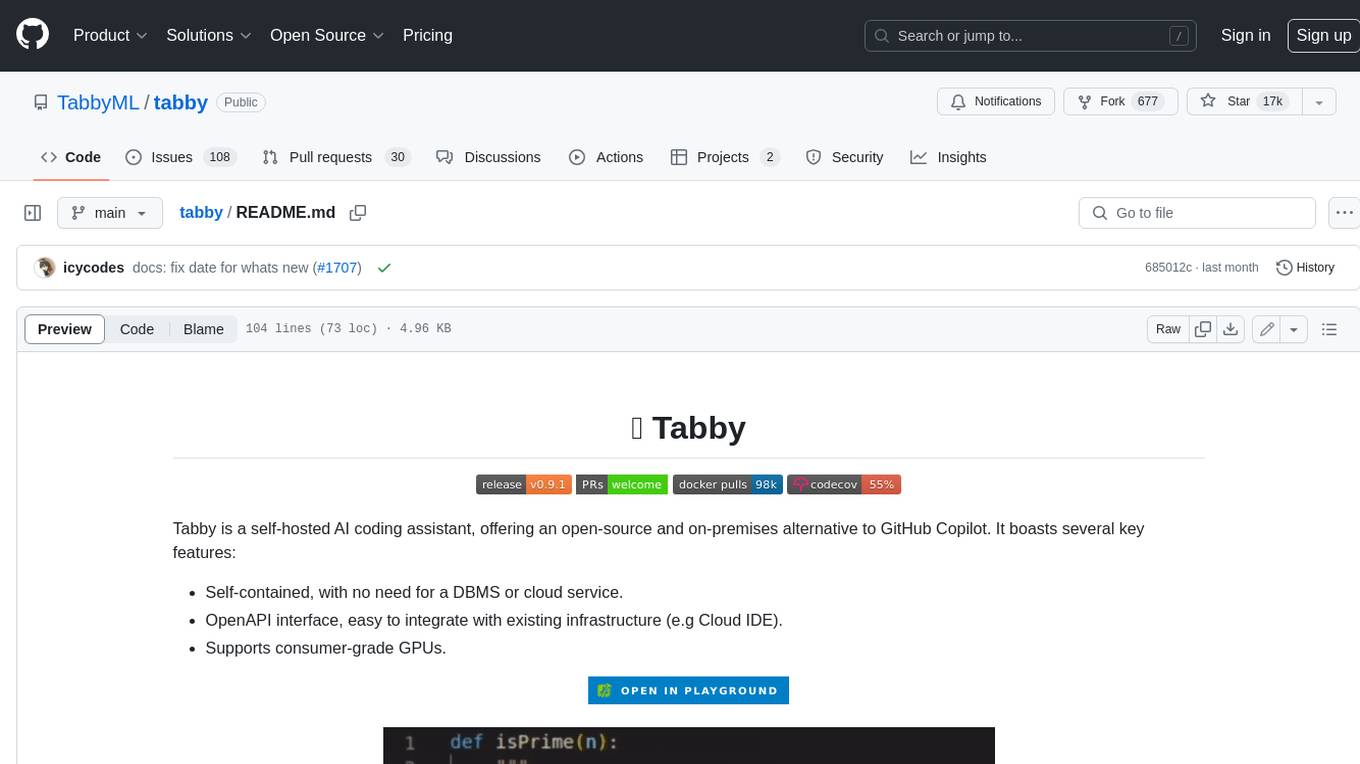
tabby
Tabby is a self-hosted AI coding assistant, offering an open-source and on-premises alternative to GitHub Copilot. It boasts several key features: * Self-contained, with no need for a DBMS or cloud service. * OpenAPI interface, easy to integrate with existing infrastructure (e.g Cloud IDE). * Supports consumer-grade GPUs.
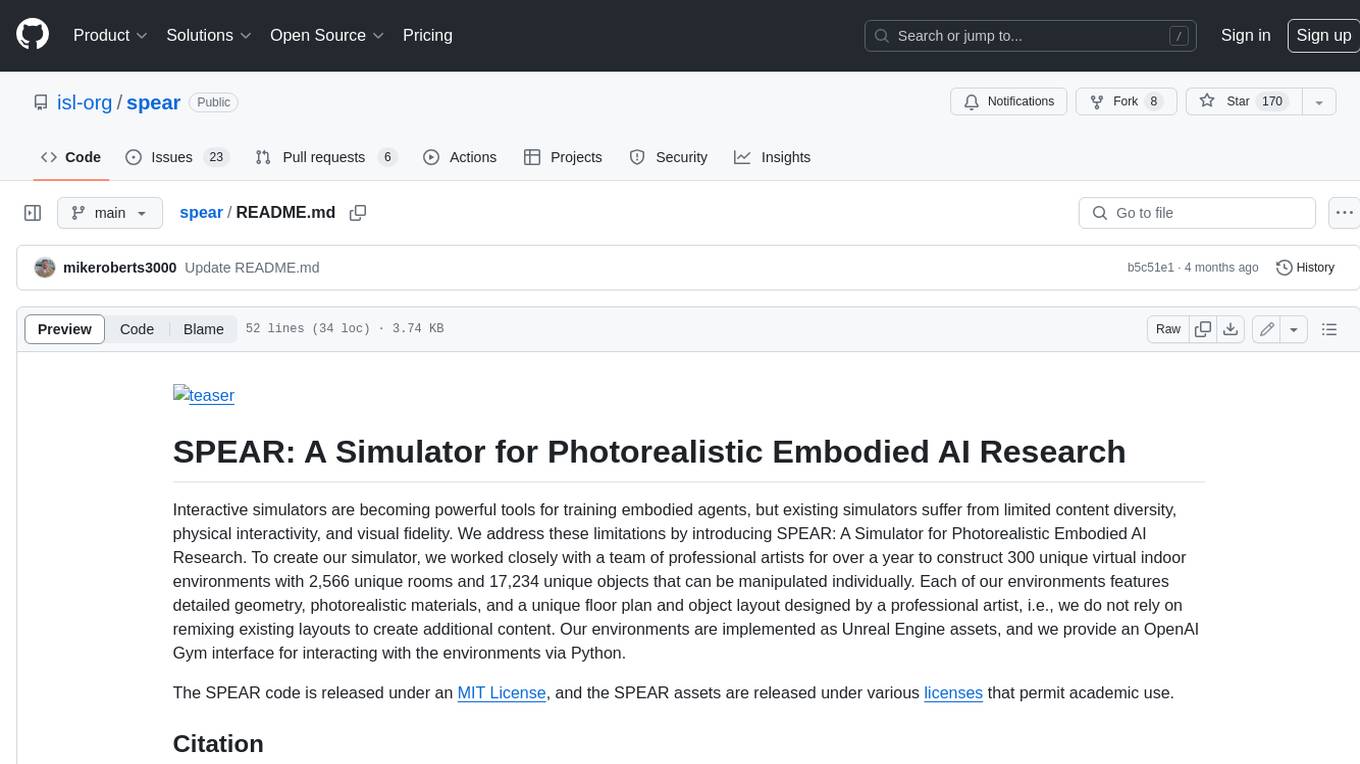
spear
SPEAR (Simulator for Photorealistic Embodied AI Research) is a powerful tool for training embodied agents. It features 300 unique virtual indoor environments with 2,566 unique rooms and 17,234 unique objects that can be manipulated individually. Each environment is designed by a professional artist and features detailed geometry, photorealistic materials, and a unique floor plan and object layout. SPEAR is implemented as Unreal Engine assets and provides an OpenAI Gym interface for interacting with the environments via Python.
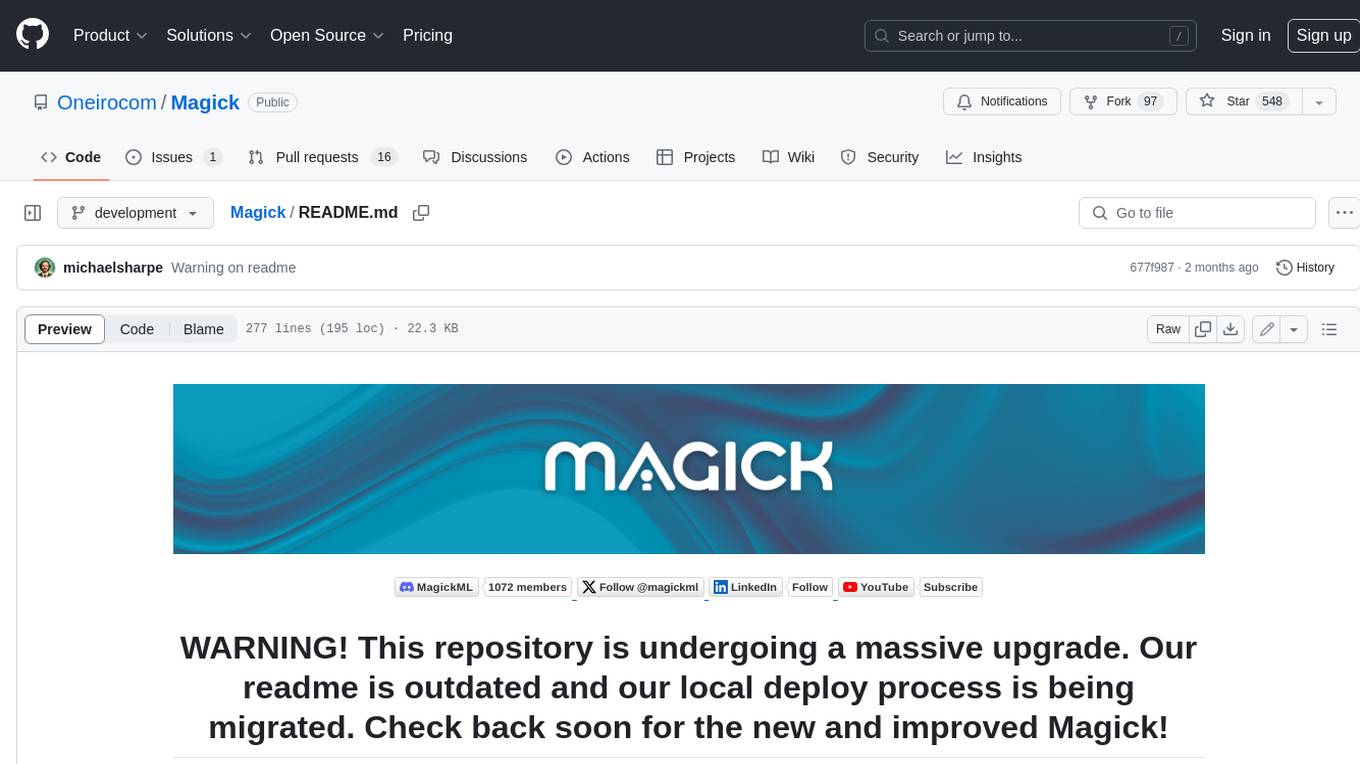
Magick
Magick is a groundbreaking visual AIDE (Artificial Intelligence Development Environment) for no-code data pipelines and multimodal agents. Magick can connect to other services and comes with nodes and templates well-suited for intelligent agents, chatbots, complex reasoning systems and realistic characters.