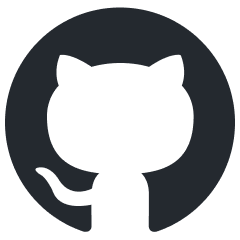
azure-search-openai-javascript
A TypeScript sample app for the Retrieval Augmented Generation pattern running on Azure, using Azure AI Search for retrieval and Azure OpenAI and LangChain large language models (LLMs) to power ChatGPT-style and Q&A experiences.
Stars: 277
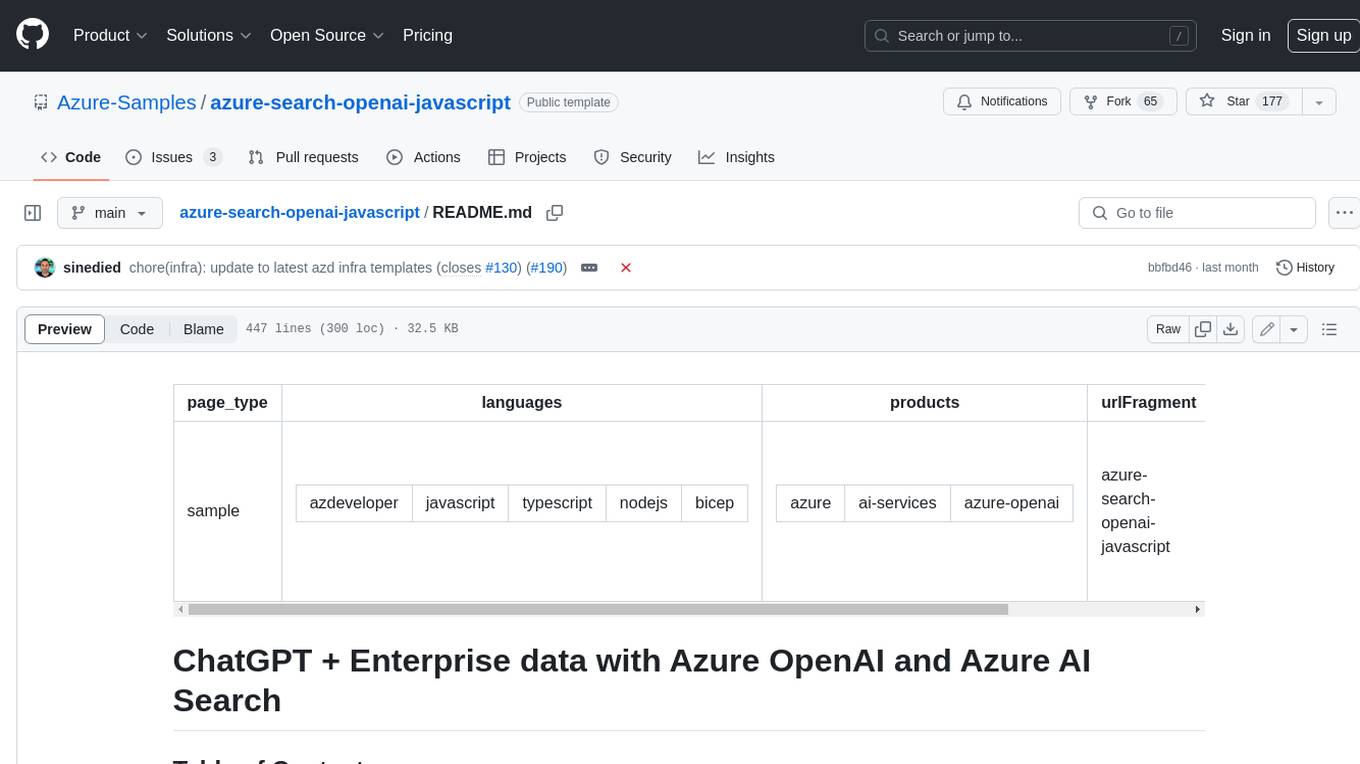
This sample demonstrates a few approaches for creating ChatGPT-like experiences over your own data using the Retrieval Augmented Generation pattern. It uses Azure OpenAI Service to access the ChatGPT model (gpt-35-turbo), and Azure AI Search for data indexing and retrieval.
README:
- Features
- Getting started
- Azure account requirements
- Azure deployment
- Sharing environments
- Clean up
- Enabling optional features
- Using the app
- Guidance
- Note
This sample demonstrates a few approaches for creating ChatGPT-like experiences over your own data using the Retrieval Augmented Generation pattern. It uses Azure OpenAI Service to access the ChatGPT model (gpt-4o-mini), and Azure AI Search for data indexing and retrieval.
The repo includes sample data so it's ready to try end to end. In this sample application we use a fictitious company called Contoso Real Estate, and the experience allows its customers to ask support questions about the usage of its products. The sample data includes a set of documents that describe its terms of service, privacy policy and a support guide.
The application is made from multiple components, including:
- Search service: the backend service that provides the search and retrieval capabilities.
- Indexer service: the service that indexes the data and creates the search indexes.
- Web app: the frontend web application that provides the user interface and orchestrates the interaction between the user and the backend services.
- Chat and Q&A interfaces
- Explores various options to help users evaluate the trustworthiness of responses with citations, tracking of source content, etc.
- Shows possible approaches for data preparation, prompt construction, and orchestration of interaction between model (ChatGPT) and retriever (Azure AI Search)
- Settings directly in the UX to tweak the behavior and experiment with options
- Optional performance tracing and monitoring with Application Insights
📺 Watch a video overview of the app
IMPORTANT: In order to deploy and run this sample, you'll need:
- Azure account. If you're new to Azure, get an Azure account for free to get free Azure credits to get started.
- Azure subscription with access enabled for the Azure OpenAI service. You can request access with this form.
-
Azure account permissions:
- Your Azure account must have
Microsoft.Authorization/roleAssignments/write
permissions, such as Role Based Access Control Administrator, User Access Administrator, or Owner. If you don't have subscription-level permissions, they must be granted to you with RBAC for an existing resource group and deploy to that existing group. - Your Azure account also needs
Microsoft.Resources/deployments/write
permissions at a subscription level.
- Your Azure account must have
Pricing may vary per region and usage. Exact costs cannot be estimated. You may try the Azure pricing calculator for the resources below.
- Azure Container Apps: Pay-as-you-go tier. Costs based on vCPU and memory used. Pricing
- Azure Static Web Apps: Free Tier. Pricing
- Azure OpenAI: Standard tier, ChatGPT and Ada models. Pricing per 1K tokens used, and at least 1K tokens are used per question. Pricing
- Azure AI Search: Standard tier, 1 replica, free level of semantic search*. Pricing per hour.Pricing (The pricing may vary or reflect an outdated tier model. Please visit the linked page for more accurate information)
- Azure Blob Storage: Standard tier with ZRS (Zone-redundant storage). Pricing per storage and read operations. Pricing
- Azure Monitor: Pay-as-you-go tier. Costs based on data ingested. Pricing
azd down --purge
.
There are multiple ways to successfully setup this project.
The easiest way to get started is with GitHub Codespaces that provides preconfigurations to setup all the tools for you. Read more below. Alternatively you can set up your local environment follwing the instructions below.
You can run this repo virtually by using GitHub Codespaces, which will open a web-based VS Code in your browser:
A similar option to Codespaces is VS Code Remote Containers, that will open the project in your local VS Code instance using the Dev Containers extension:
- Azure Developer CLI
- Node.js LTS
- Docker for Desktop
- Git
-
Powershell 7+ (pwsh) - For Windows users only.
-
Important: Ensure you can run
pwsh.exe
from a PowerShell command. If this fails, you likely need to upgrade PowerShell.
-
Important: Ensure you can run
Then get the project code:
- Create a new folder and switch to it in the terminal
- Run
azd auth login
- Run
azd init -t azure-search-openai-javascript
- note that this command will initialize a git repository and you do not need to clone this repository
Execute the following command, if you don't have any pre-existing Azure services and want to start from a fresh deployment.
- Run
azd up
- This will provision Azure resources and deploy this sample to those resources, including building the search index based on the files found in the./data
folder.- You will be prompted to select a location for the majority of resources, except for the OpenAI and Static Web App resources.
- By default, the OpenAI resource will be deployed to
eastus2
. You can set a different location withazd env set AZURE_OPENAI_RESOURCE_GROUP_LOCATION {location}
. Currently only a short list of locations is accepted. That location list is based on the OpenAI model availability table and may become outdated as availability changes. - By default, the Staic Web App resource will be deployed to
eastus2
. You can set a different location withazd env set AZURE_WEBAPP_LOCATION {location}
. Currently only a short list of locations is accepted. Note that Static Web App is a global service, and the location you choose will only affect the managed Functions App which is not used in this sample.
- After the application has been successfully deployed you will see a URL printed to the console. Click that URL to interact with the application in your browser.
It will look like the following:
NOTE: It can take 15+ minutes for the application to be fully deployed.
If you already have existing Azure resources, you can re-use those by setting azd
environment values.
- Run
azd env set AZURE_RESOURCE_GROUP {Name of existing resource group}
- Run
azd env set AZURE_LOCATION {Location of existing resource group}
- Run
azd env set AZURE_OPENAI_SERVICE {Name of existing OpenAI service}
- Run
azd env set AZURE_OPENAI_RESOURCE_GROUP {Name of existing resource group that OpenAI service is provisioned to}
- Run
azd env set AZURE_OPENAI_CHATGPT_DEPLOYMENT {Name of existing ChatGPT deployment}
. Only needed if your ChatGPT deployment is not the default 'chat'. - Run
azd env set AZURE_OPENAI_EMBEDDING_DEPLOYMENT {Name of existing GPT embedding deployment}
. Only needed if your embeddings deployment is not the default 'embedding'.
- Run
azd env set AZURE_SEARCH_SERVICE {Name of existing Azure AI Search service}
- Run
azd env set AZURE_SEARCH_SERVICE_RESOURCE_GROUP {Name of existing resource group with ACS service}
- If that resource group is in a different location than the one you'll pick for the
azd up
step, then runazd env set AZURE_SEARCH_SERVICE_LOCATION {Location of existing service}
- If the search service's SKU is not standard, then run
azd env set AZURE_SEARCH_SERVICE_SKU {Name of SKU}
. The free tier won't work as it doesn't support managed identity. (See other possible values)
You can also use an existing Storage Account. See ./infra/main.parameters.json
for list of environment variables to pass to azd env set
to configure those existing resources.
Now you can run azd up
, following the steps in Deploying from scratch above.
That will both provision resources and deploy the code.
If you've only changed the backend/frontend code in the app
folder, then you don't need to re-provision the Azure resources. You can just run:
azd deploy
If you've changed the infrastructure files (infra
folder or azure.yaml
), then you'll need to re-provision the Azure resources. You can do that by running:
azd up
To give someone else access to a completely deployed and existing environment, either you or they can follow these steps:
- Install the Azure Developer CLI
- Run
azd init -t azure-search-openai-javascript
or clone this repository. - Run
azd env refresh -e {environment name}
They will need the azd environment name, subscription ID, and location to run this command. You can find those values in your.azure/{env name}/.env
file. This will populate their azd environment's.env
file with all the settings needed to run the app locally. - Set the environment variable
AZURE_PRINCIPAL_ID
either in that.env
file or in the active shell to their Azure ID, which they can get withaz ad signed-in-user show
. - Run
./scripts/roles.ps1
or./scripts/roles.sh
to assign all of the necessary roles to the user. If they do not have the necessary permission to create roles in the subscription, then you may need to run this script for them. Once the script runs, they should be able to run the app locally.
To clean up all the resources created by this sample:
- Run
azd down --purge
- When asked if you are sure you want to continue, enter
y
- When asked if you want to permanently delete the resources, enter
y
The resource group and all the resources will be deleted.
By default, the deployed Azure web app will have no authentication or access restrictions enabled, meaning anyone with routable network access to the web app can chat with your indexed data. You can require authentication to your Azure Entra ID by following the Add app authentication tutorial and set it up against the deployed web app.
To then limit access to a specific set of users or groups, you can follow the steps from Restrict your Azure Entra app to a set of users by changing "Assignment Required?" option under the Enterprise Application, and then assigning users/groups access. Users not granted explicit access will receive the error message -AADSTS50105: Your administrator has configured the application <app_name> to block users unless they are specifically granted ('assigned') access to the application.-
We recommend deploying additional security mechanisms. When applicable, consider setting up a VNet or setting up a Proxy Policy.
By default, the deployed search API will only allow requests from the same origin as the deployed web app origin. To enable CORS for a frontend hosted on a different origin, run:
- Run
azd env set ALLOWED_ORIGIN https://<your-domain.com>
- Run
azd up
You can only run locally after having successfully run the azd up
command.
- Run
azd auth login
- Run
azd env get-values > .env
to get the environment variables for the app. - Run
./scripts/index-data.sh
or./scripts/index-data.ps1
to index the data. - Run
az login
to log in to your Azure account (needed for managed identity) - Run
npm start
or run the "VS Code Task: Start App" to start the project locally.
- In Azure: navigate to the Azure Static Web App deployed by azd. The URL is printed out when azd completes (as "Endpoint"), or you can find it in the Azure portal.
- Running locally: navigate to http://127.0.0.1:5173
Once in the web app:
- Try different topics in chat or Q&A context. For chat, try follow up questions, clarifications, ask to simplify or elaborate on answer, etc.
- Explore citations and sources
- Click on "settings" to try different options, tweak prompts, etc.
The Search API service implements the HTTP protocol for AI chat apps. It can be swapped with any service that implements the same protocol, like the Python backend client in this repository instead of the Node.js implementation featured in this repo.
To do so, follow these steps:
- Deploy this repository, following the steps above.
- Get the frontend URL:
- If you want to use the deployed web app, run
azd env get-values | grep WEBAPP_URI
to get the URL. - If you want to use the local web app, use
http://localhost:5173
. - If you want to use the Codespaces local web app, use
https://<your_codespace_base_url>-5173.app.github.dev
.
- Open the alternative backend repository your want to use, for example: https://github.com/Azure-Samples/azure-search-openai-demo
- Set the frontend URL as an allowed origin with
azd env set ALLOWED_ORIGIN <your_frontend_url>
. - Follow the steps to deploy the Python backend.
- Once the Python backend is fully deployed, get the backend URL with
azd env get-values | grep BACKEND_URI
. - Set the backend URL in this repo, running
azd env set BACKEND_URI <your_backend_url>
. - Depending on whether you want to use the deployed web app or the local web app:
-
If you want to use the deployed web app, run
azd up
to redeploy. -
If you want to use the local web app on your machine or in Codespaces, run:
# Export the environment variable. # The syntax may be different depending on your shell or if you're using Windows. export BACKEND_URI=<your_backend_url> # Start the app npm start --workspace=webapp
This sample is composed by two applications: a backend service and API, deployed to Azure Container Apps, and a frontend application, deployed to Azure Static Web Apps. By default, the deployed Azure Container App will have no authentication or access restrictions enabled, meaning anyone with routable network access to the container app can chat with your indexed data. You can require authentication to your Azure Entra ID by following the Add container app authentication tutorial and set it up against the deployed Azure Container App.
To limit access to a specific set of users or groups, you can follow the steps from Restrict your Azure Entra app to a set of users by changing "Assignment Required?" option under the Enterprise Application, and then assigning users/groups access. Users not granted explicit access will receive the error message -AADSTS50105: Your administrator has configured the application <app_name> to block users unless they are specifically granted ('assigned') access to the application.-
This sample is designed to be a starting point for your own production application, but you should do a thorough review of the security and performance before deploying to production. Here are some things to consider:
-
OpenAI Capacity: The default TPM (tokens per minute) is set to 30K. That is equivalent to approximately 30 conversations per minute (assuming 1K per user message/response). You can increase the capacity by changing the
chatGptDeploymentCapacity
andembeddingDeploymentCapacity
parameters ininfra/main.bicep
to your account's maximum capacity. You can also view the Quotas tab in Azure OpenAI studio to understand how much capacity you have. -
Azure Storage: The default storage account uses the
Standard_LRS
SKU. To improve your resiliency, we recommend usingStandard_ZRS
for production deployments, which you can specify using thesku
property under thestorage
module ininfra/main.bicep
. -
Azure AI Search: The default search service uses the
Standard
SKU with the free semantic search option, which gives you 1000 free queries a month. Assuming your app will experience more than 1000 questions, you should either changesemanticSearch
to "standard" or disable semantic search entirely in the request options. If you see errors about search service capacity being exceeded, you may find it helpful to increase the number of replicas by changingreplicaCount
ininfra/core/search/search-services.bicep
or manually scaling it from the Azure Portal. - Azure Container Apps: The default container app setup uses 1 vCPU core and 2 GB RAM per container, with autoscaling enabled. The minimum number of replicas is set to 1, and the maximum to 10. You can change vCPU and RAM capacity in the template, and define your own auto-scaling rules based on load. For more details, read Set scaling rules in Azure Container Apps.
- Authentication: By default, the deployed app is publicly accessible. We recommend restricting access to authenticated users. See Enabling authentication above for how to enable authentication.
- Networking: We recommend deploying inside a Virtual Network. If the app is only for internal enterprise use, use a private DNS zone. Also consider using Azure API Management (APIM) for firewalls and other forms of protection. For more details, read Azure OpenAI Landing Zone reference architecture.
- Generative AI For Beginners
- Revolutionize your Enterprise Data with ChatGPT: Next-gen Apps w/ Azure OpenAI and AI Search
- Azure AI Search
- Azure OpenAI Service
- Building ChatGPT-Like Experiences with Azure: A Guide to Retrieval Augmented Generation for JavaScript applications
Why do we need to break up the documents into chunks when Azure AI Search supports searching large documents?
Chunking allows us to limit the amount of information we send to OpenAI due to token limits. By breaking up the content, it allows us to easily find potential chunks of text that we can inject into OpenAI. The method of chunking we use leverages a sliding window of text such that sentences that end one chunk will start the next. This allows us to reduce the chance of losing the context of the text.
How can we upload additional documents without redeploying everything?
To upload more documents, put them in the data/ folder and run ./scripts/index-data.sh
or ./scripts/index-data.ps1
.
How does this sample compare to other Chat with Your Data samples?
Another popular repository for this use case is here: https://github.com/Microsoft/sample-app-aoai-chatGPT/
That repository is designed for use by customers using Azure OpenAI studio and Azure Portal for setup. It also includes azd
support for folks who want to deploy it completely from scratch.
The primary differences:
- This repository includes multiple RAG (retrieval-augmented generation) approaches that chain the results of multiple API calls (to Azure OpenAI and ACS) together in different ways. The other repository uses only the built-in data sources option for the ChatCompletions API, which uses a RAG approach on the specified ACS index. That should work for most uses, but if you needed more flexibility, this sample may be a better option.
- This repository is also a bit more experimental in other ways, since it's not tied to the Azure OpenAI Studio like the other repository.
Feature comparison:
Feature | azure-search-openai-javascript | sample-app-aoai-chatGPT |
---|---|---|
RAG approach | Multiple approaches | Only via ChatCompletion API data_sources |
Vector support | âś… Yes | âś… Yes |
Data ingestion | âś… Yes (MD) | âś… Yes (PDF, TXT, MD, HTML) |
Persistent chat history | ❌ No (browser tab only) | ✅ Yes, in CosmosDB |
Technology comparison:
Tech | azure-search-openai-javascript | sample-app-aoai-chatGPT |
---|---|---|
Frontend | React/Lit | React |
Backend | Node.js (Fastify) | Python (Flask) |
Vector DB | Azure AI Search | Azure AI Search |
Deployment | Azure Developer CLI (azd) | Azure Portal, az, azd |
How do you use GPT-4 with this sample?
Run these commands:
azd env set AZURE_OPENAI_CHATGPT_MODEL gpt-4
You may also need to adjust the capacity in infra/main.bicep
file, depending on how much TPM your account is allowed.
What is the difference between the Chat and Ask tabs?
The chat tab uses the approach programmed in chat-read-retrieve-read.ts. The ask tab uses the approach programmed in ask-retrieve-then-read.ts.
What does the `azd up` command do?
The azd up
command comes from the Azure Developer CLI, and takes care of both provisioning the Azure resources and deploying code to the selected Azure hosts.
The azd up
command uses the azure.yaml
file combined with the infrastructure-as-code .bicep
files in the infra/
folder. The azure.yaml
file for this project declares several "hooks" for the prepackage step and postprovision steps. The up
command first runs the prepackage
hook which installs Node dependencies and builds the React.JS-based JavaScript files. It then packages all the code (both frontend and backend services) into a zip file which it will deploy later.
Next, it provisions the resources based on main.bicep
and main.parameters.json
. At that point, since there is no default value for the OpenAI resource location, it asks you to pick a location from a short list of available regions. Then it will send requests to Azure to provision all the required resources. With everything provisioned, it runs the postprovision
hook to process the local data and add it to an Azure AI Search index.
Finally, it looks at azure.yaml
to determine the Azure host (Container Apps and Static Web Apps, in this case) and uploads the zip to Azure App Service. The azd up
command is now complete, but it may take a few minutes for the app to be fully available and working after the initial deploy.
Related commands are azd provision
for just provisioning (if infra files change) and azd deploy
for just deploying updated app code.
Here are the most common failure scenarios and solutions:
-
The subscription (
AZURE_SUBSCRIPTION_ID
) doesn't have access to the Azure OpenAI service. Please ensureAZURE_SUBSCRIPTION_ID
matches the ID specified in the OpenAI access request process. -
You're attempting to create resources in regions not enabled for Azure OpenAI (e.g. East US 2 instead of East US), or where the model you're trying to use isn't enabled. See this matrix of model availability.
-
You've exceeded a quota, most often number of resources per region. See this article on quotas and limits.
-
You're getting "same resource name not allowed" conflicts. That's likely because you've run the sample multiple times and deleted the resources you've been creating each time, but are forgetting to purge them. Azure keeps resources for 48 hours unless you purge from soft delete. See this article on purging resources.
-
After running
azd up
and visiting the website, you see a '404 Not Found' in the browser. Wait 10 minutes and try again, as it might be still starting up. Then try runningazd deploy
and wait again. If you still encounter errors with the deployed app, consult these tips for debugging App Service app deployments and file an issue if the error logs don't help you resolve the issue. -
You're getting an error
401 Principal does not have access to API/Operation
while running the project locally or trying to deploy. That's likely because your environment variables includeAZURE_TENANT_ID
,AZURE_CLIENT_ID
andAZURE_CLIENT_SECRET
. You should either grant permissions to the related Service Principal or remove these variables from your environment to ensure normal access. For more details, please refer to Azure identity SDK.
See this doc for reducing costs.
Note: The documents used in this demo contain information generated using a language model (Azure OpenAI Service). The information contained in these documents is only for demonstration purposes and does not reflect the opinions or beliefs of Microsoft. Microsoft makes no representations or warranties of any kind, express or implied, about the completeness, accuracy, reliability, suitability or availability with respect to the information contained in this document. All rights reserved to Microsoft.
For Tasks:
Click tags to check more tools for each tasksFor Jobs:
Alternative AI tools for azure-search-openai-javascript
Similar Open Source Tools
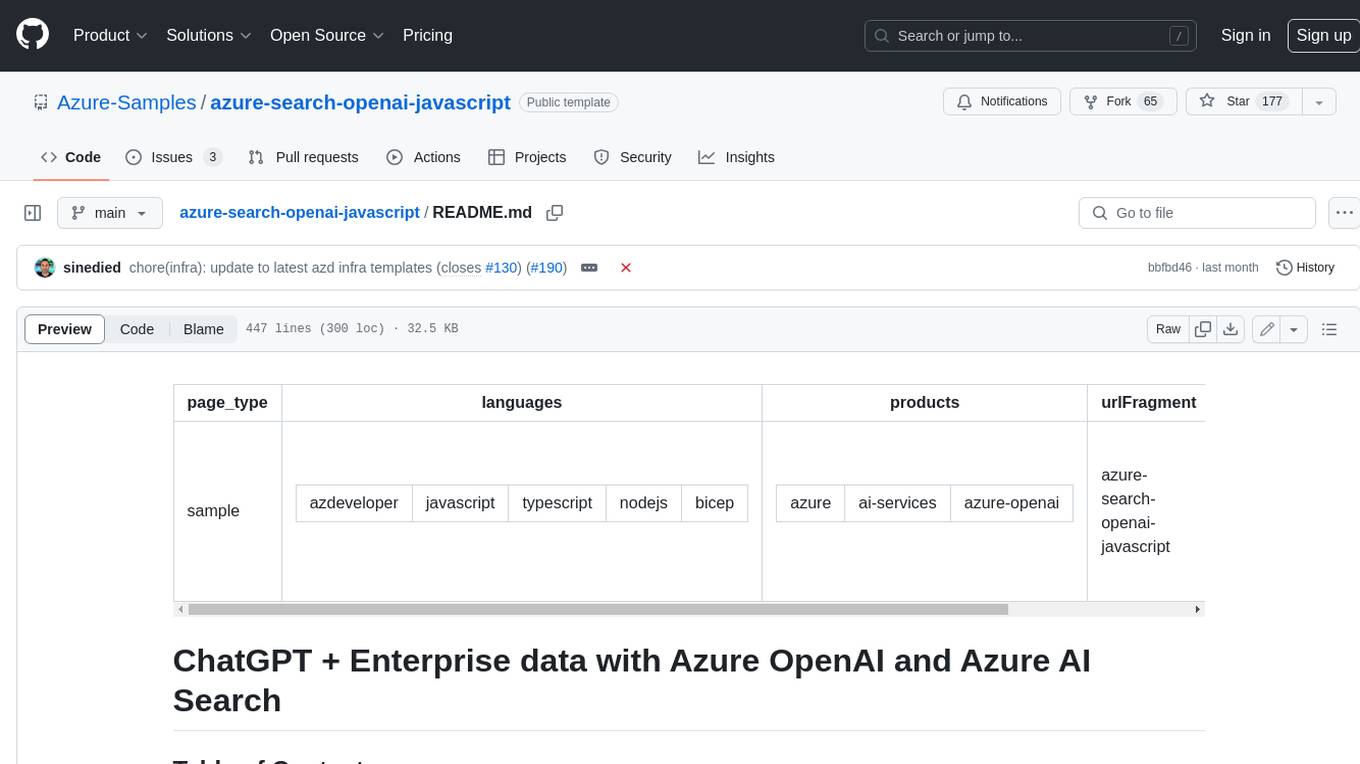
azure-search-openai-javascript
This sample demonstrates a few approaches for creating ChatGPT-like experiences over your own data using the Retrieval Augmented Generation pattern. It uses Azure OpenAI Service to access the ChatGPT model (gpt-35-turbo), and Azure AI Search for data indexing and retrieval.
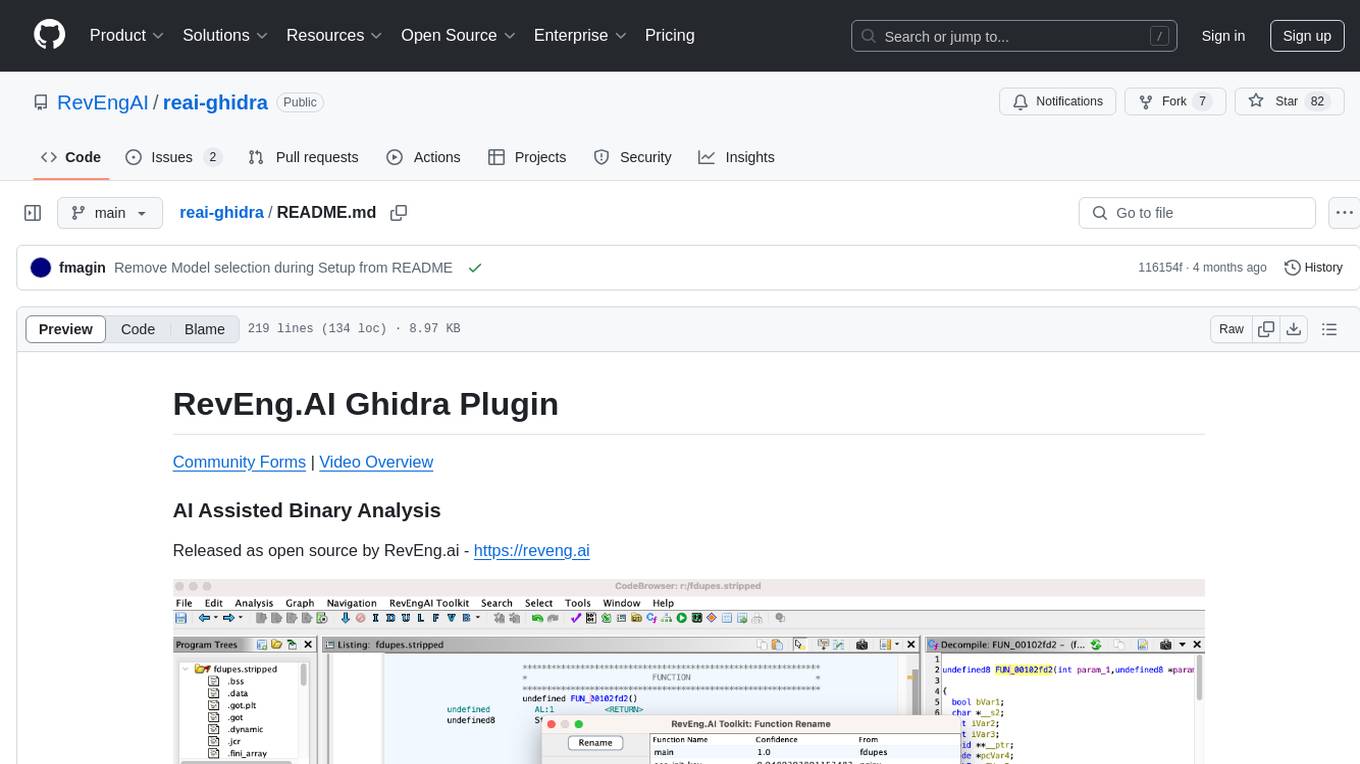
reai-ghidra
The RevEng.AI Ghidra Plugin by RevEng.ai allows users to interact with their API within Ghidra for Binary Code Similarity analysis to aid in Reverse Engineering stripped binaries. Users can upload binaries, rename functions above a confidence threshold, and view similar functions for a selected function.
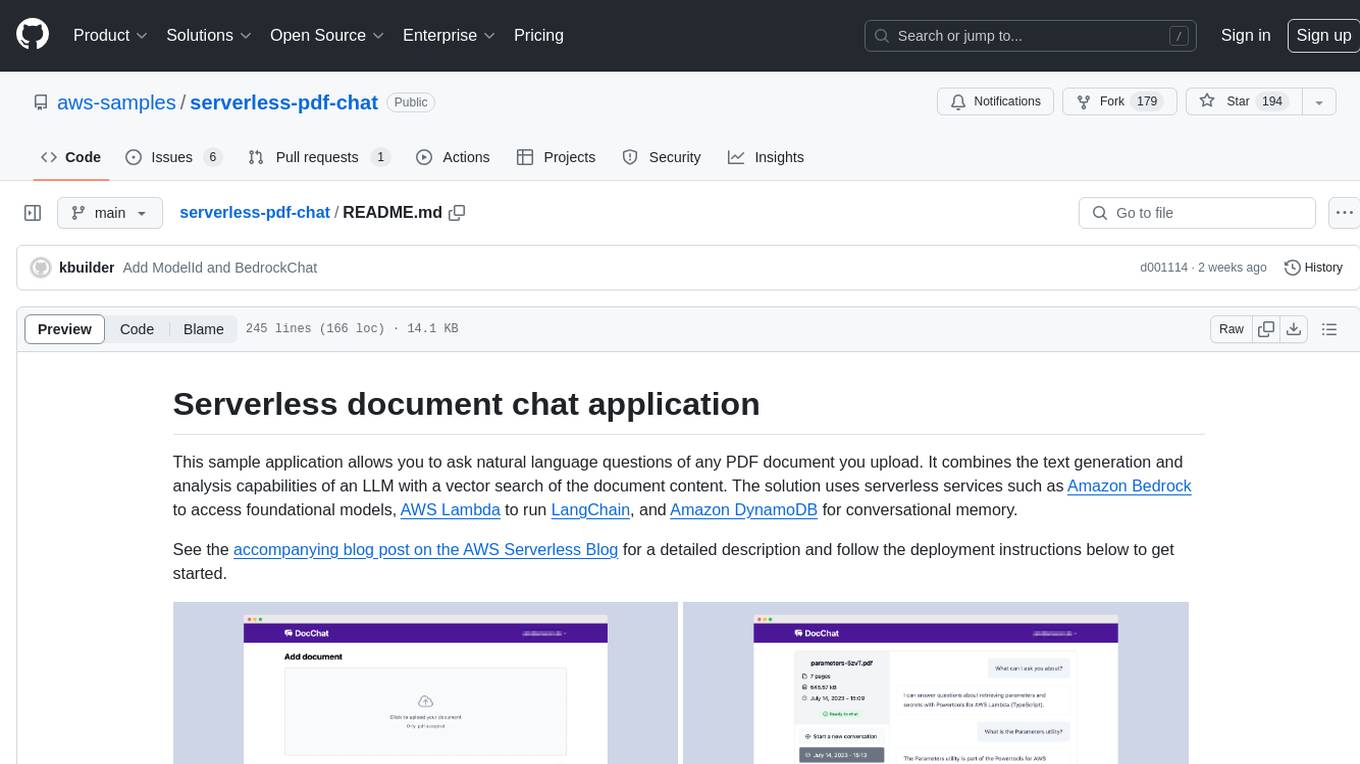
serverless-pdf-chat
The serverless-pdf-chat repository contains a sample application that allows users to ask natural language questions of any PDF document they upload. It leverages serverless services like Amazon Bedrock, AWS Lambda, and Amazon DynamoDB to provide text generation and analysis capabilities. The application architecture involves uploading a PDF document to an S3 bucket, extracting metadata, converting text to vectors, and using a LangChain to search for information related to user prompts. The application is not intended for production use and serves as a demonstration and educational tool.
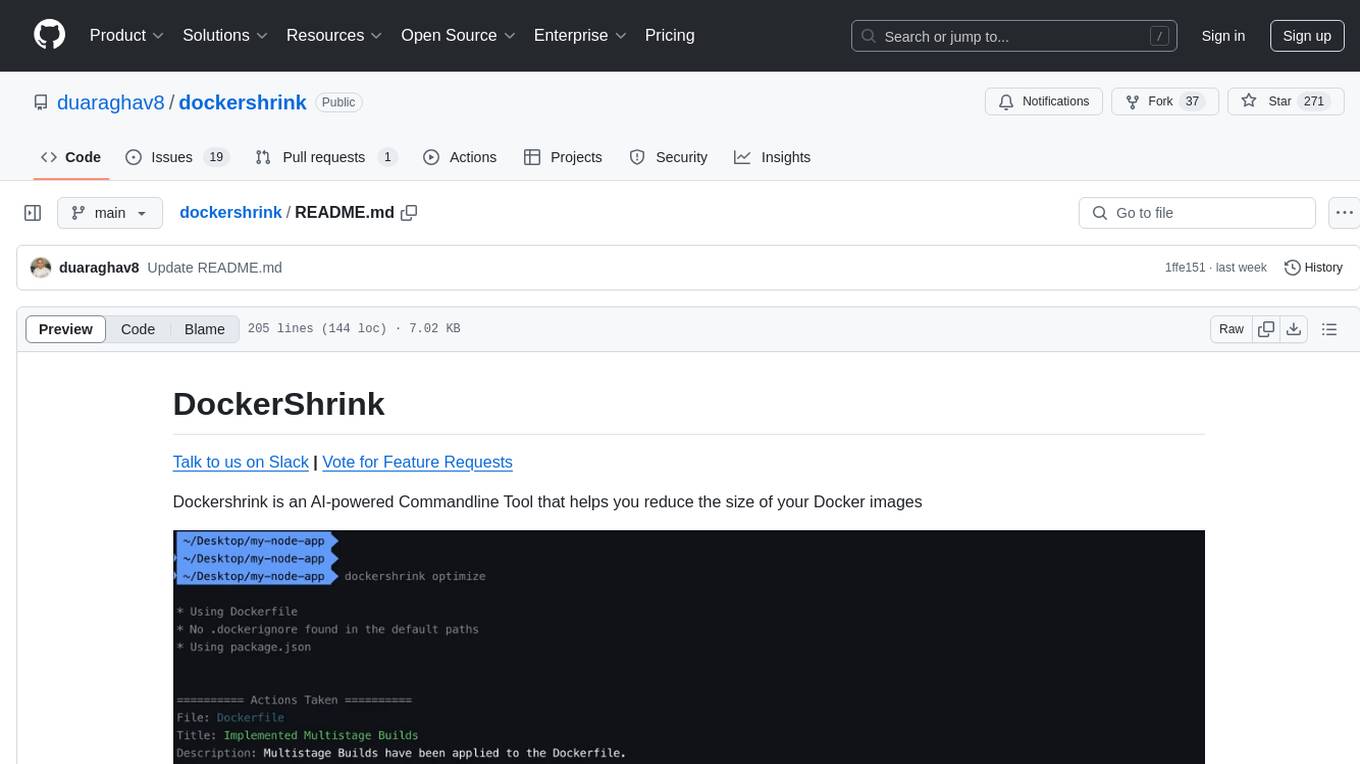
dockershrink
Dockershrink is an AI-powered Commandline Tool designed to help reduce the size of Docker images. It combines traditional Rule-based analysis with Generative AI techniques to optimize Image configurations. The tool supports NodeJS applications and aims to save costs on storage, data transfer, and build times while increasing developer productivity. By automatically applying advanced optimization techniques, Dockershrink simplifies the process for engineers and organizations, resulting in significant savings and efficiency improvements.
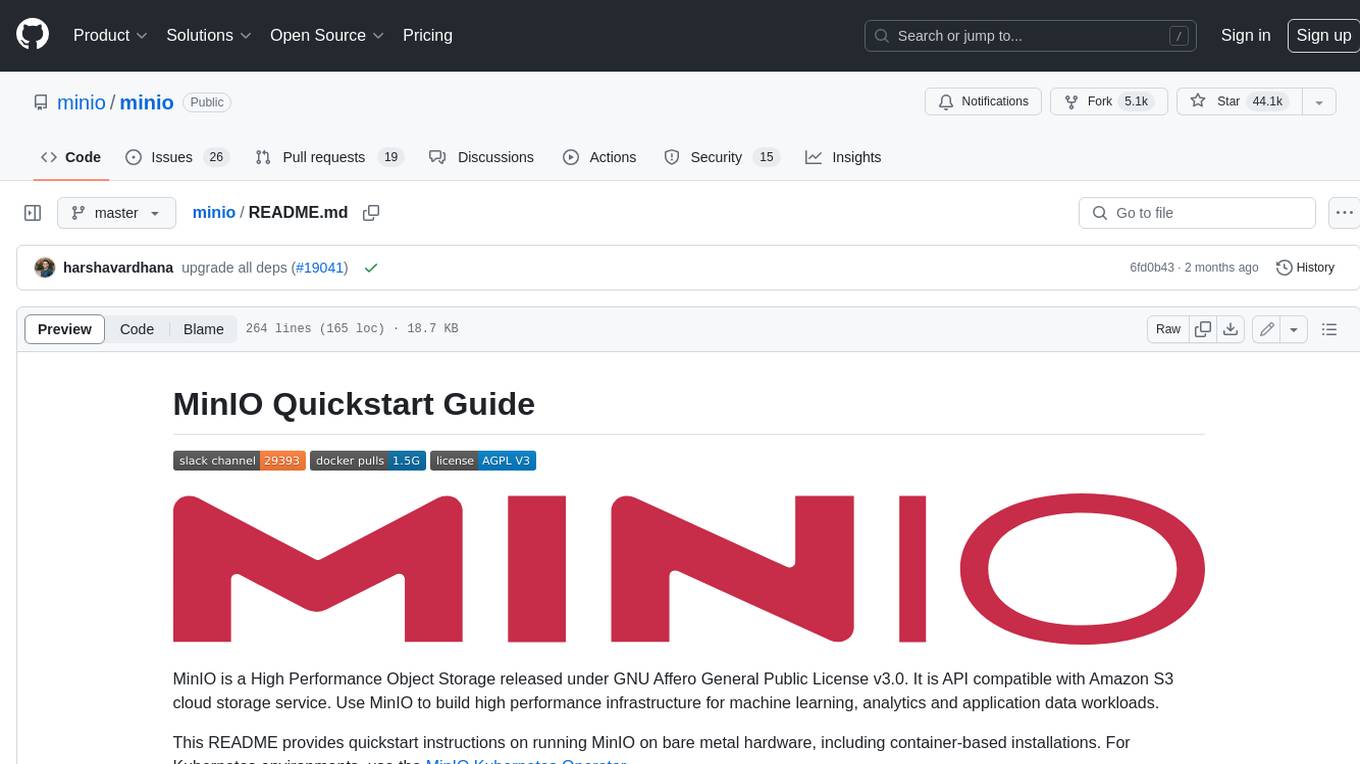
minio
MinIO is a High Performance Object Storage released under GNU Affero General Public License v3.0. It is API compatible with Amazon S3 cloud storage service. Use MinIO to build high performance infrastructure for machine learning, analytics and application data workloads.
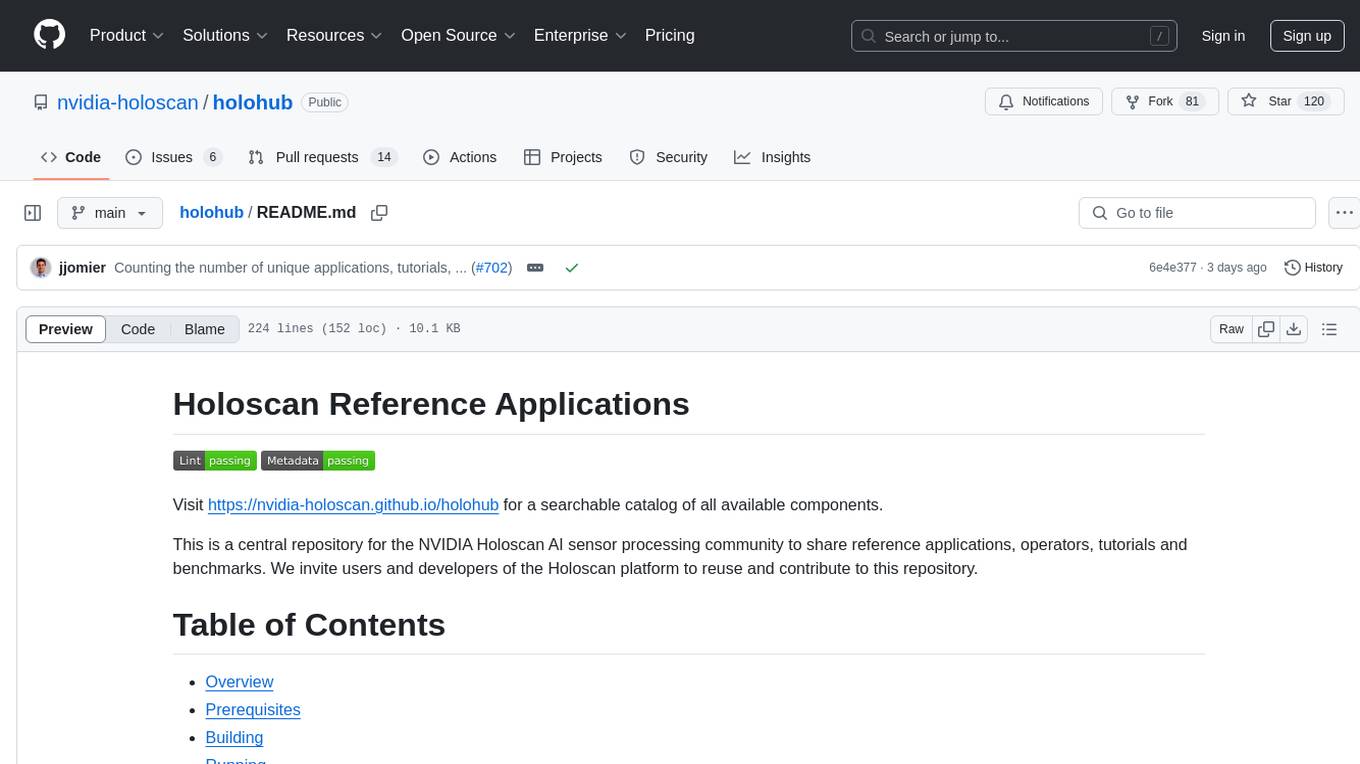
holohub
Holohub is a central repository for the NVIDIA Holoscan AI sensor processing community to share reference applications, operators, tutorials, and benchmarks. It includes example applications, community components, package configurations, and tutorials. Users and developers of the Holoscan platform are invited to reuse and contribute to this repository. The repository provides detailed instructions on prerequisites, building, running applications, contributing, and glossary terms. It also offers a searchable catalog of available components on the Holoscan SDK User Guide website.
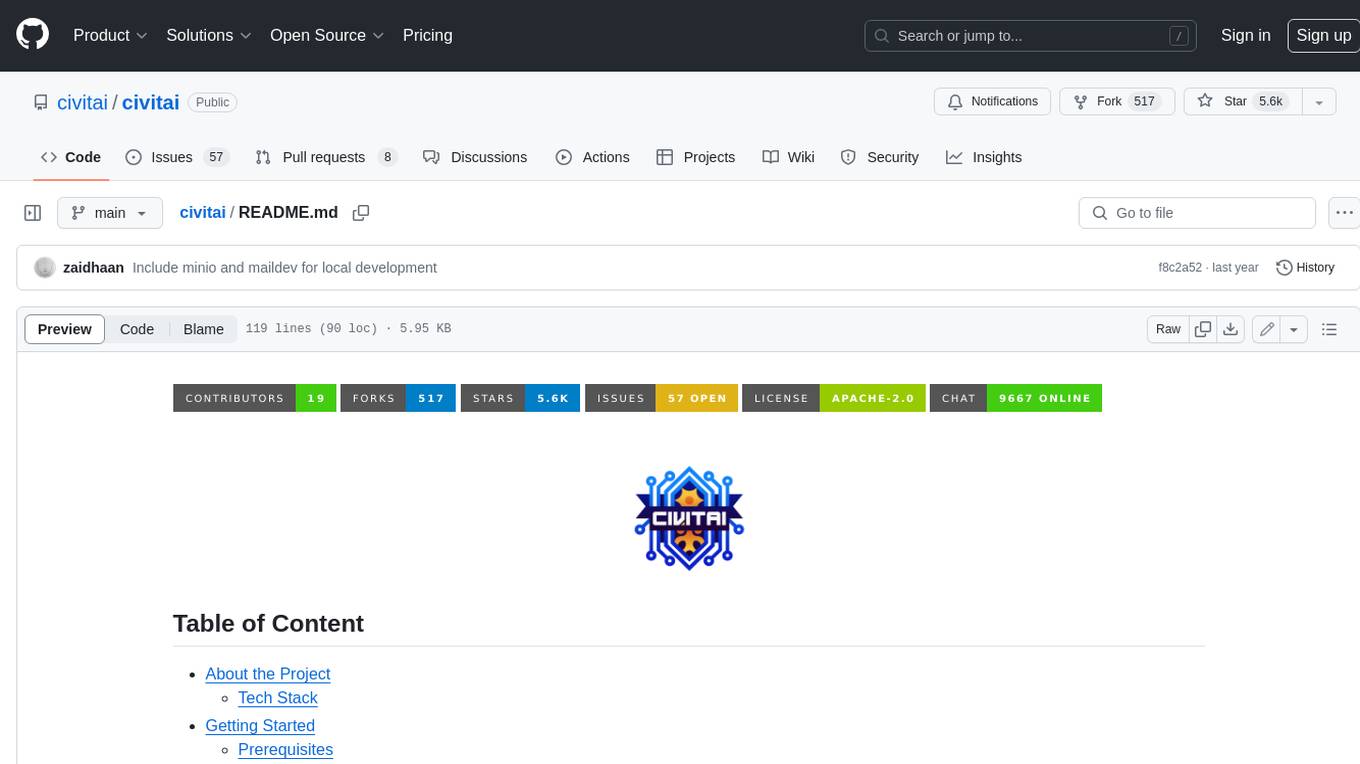
civitai
Civitai is a platform where people can share their stable diffusion models (textual inversions, hypernetworks, aesthetic gradients, VAEs, and any other crazy stuff people do to customize their AI generations), collaborate with others to improve them, and learn from each other's work. The platform allows users to create an account, upload their models, and browse models that have been shared by others. Users can also leave comments and feedback on each other's models to facilitate collaboration and knowledge sharing.
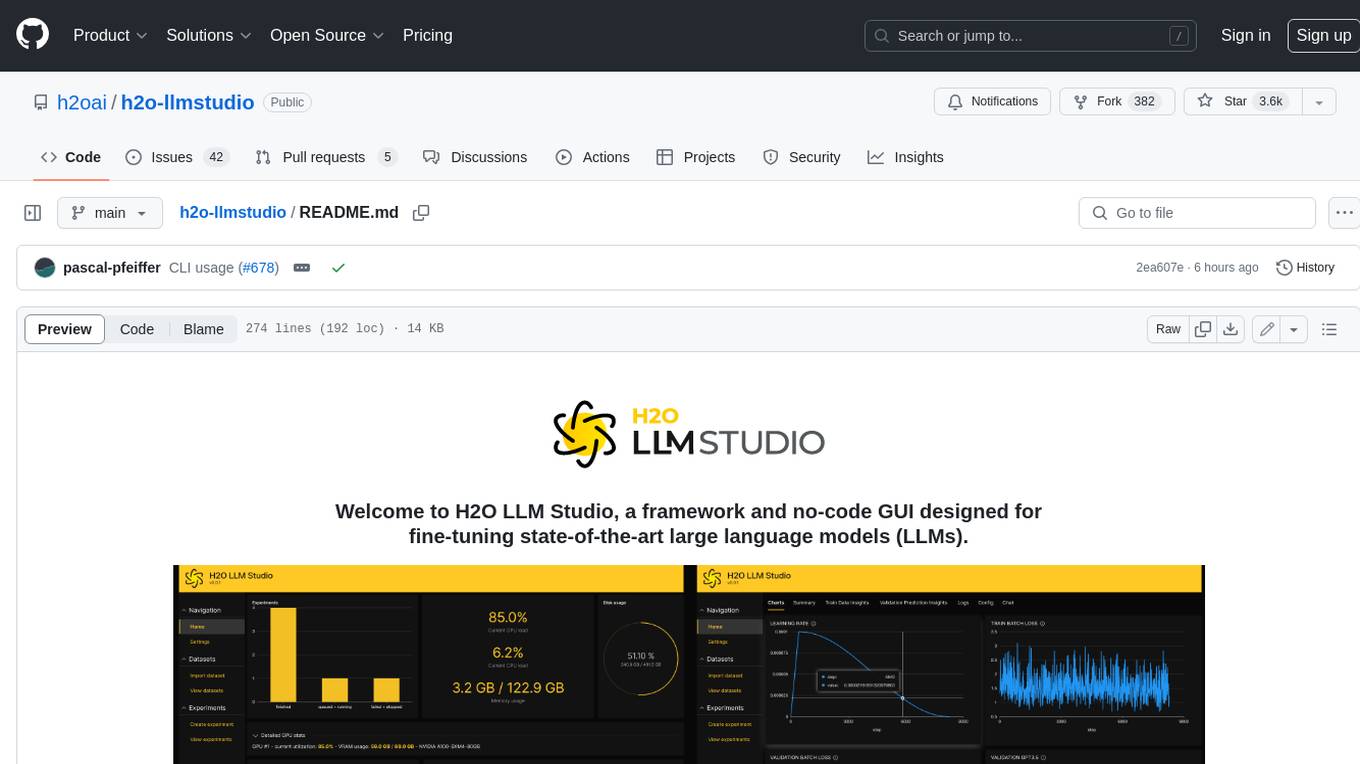
h2o-llmstudio
H2O LLM Studio is a framework and no-code GUI designed for fine-tuning state-of-the-art large language models (LLMs). With H2O LLM Studio, you can easily and effectively fine-tune LLMs without the need for any coding experience. The GUI is specially designed for large language models, and you can finetune any LLM using a large variety of hyperparameters. You can also use recent finetuning techniques such as Low-Rank Adaptation (LoRA) and 8-bit model training with a low memory footprint. Additionally, you can use Reinforcement Learning (RL) to finetune your model (experimental), use advanced evaluation metrics to judge generated answers by the model, track and compare your model performance visually, and easily export your model to the Hugging Face Hub and share it with the community.
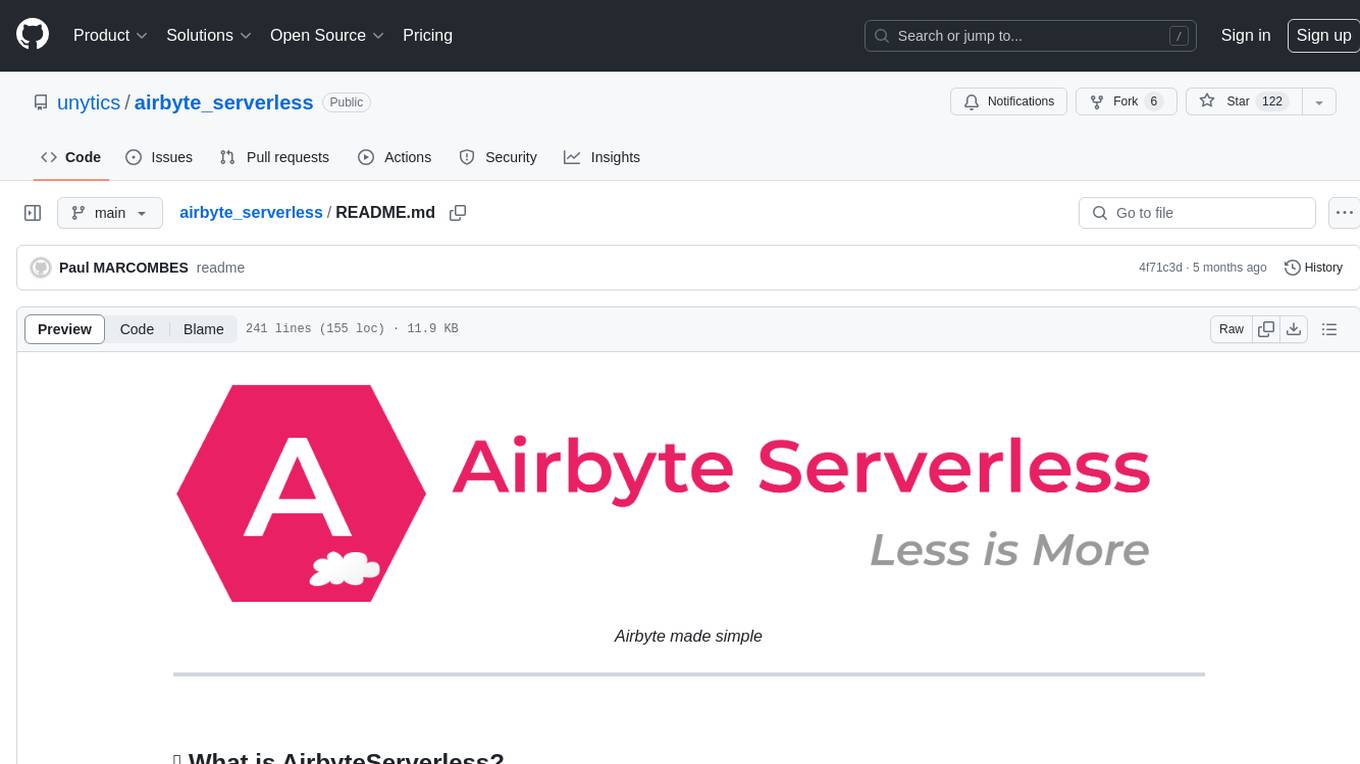
airbyte_serverless
AirbyteServerless is a lightweight tool designed to simplify the management of Airbyte connectors. It offers a serverless mode for running connectors, allowing users to easily move data from any source to their data warehouse. Unlike the full Airbyte-Open-Source-Platform, AirbyteServerless focuses solely on the Extract-Load process without a UI, database, or transform layer. It provides a CLI tool, 'abs', for managing connectors, creating connections, running jobs, selecting specific data streams, handling secrets securely, and scheduling remote runs. The tool is scalable, allowing independent deployment of multiple connectors. It aims to streamline the connector management process and provide a more agile alternative to the comprehensive Airbyte platform.
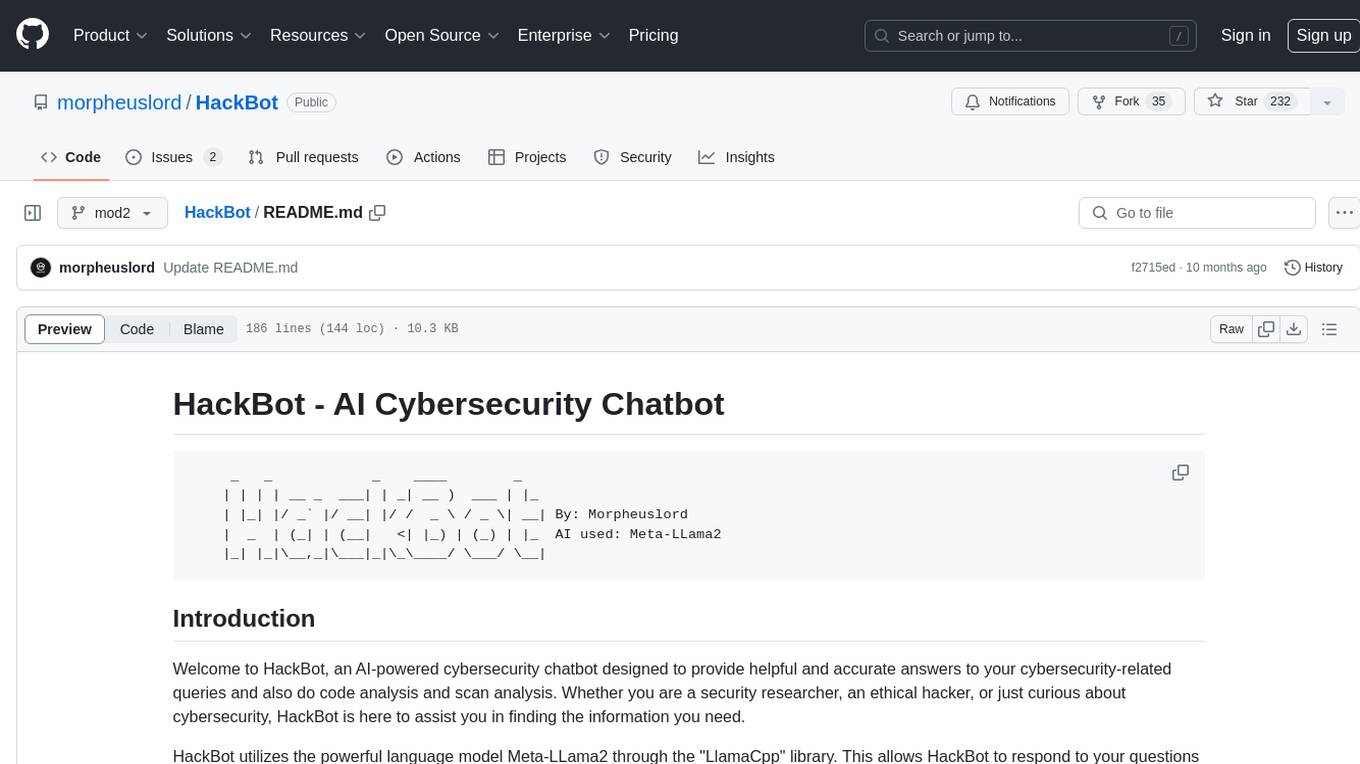
HackBot
HackBot is an AI-powered cybersecurity chatbot designed to provide accurate answers to cybersecurity-related queries, conduct code analysis, and scan analysis. It utilizes the Meta-LLama2 AI model through the 'LlamaCpp' library to respond coherently. The chatbot offers features like local AI/Runpod deployment support, cybersecurity chat assistance, interactive interface, clear output presentation, static code analysis, and vulnerability analysis. Users can interact with HackBot through a command-line interface and utilize it for various cybersecurity tasks.
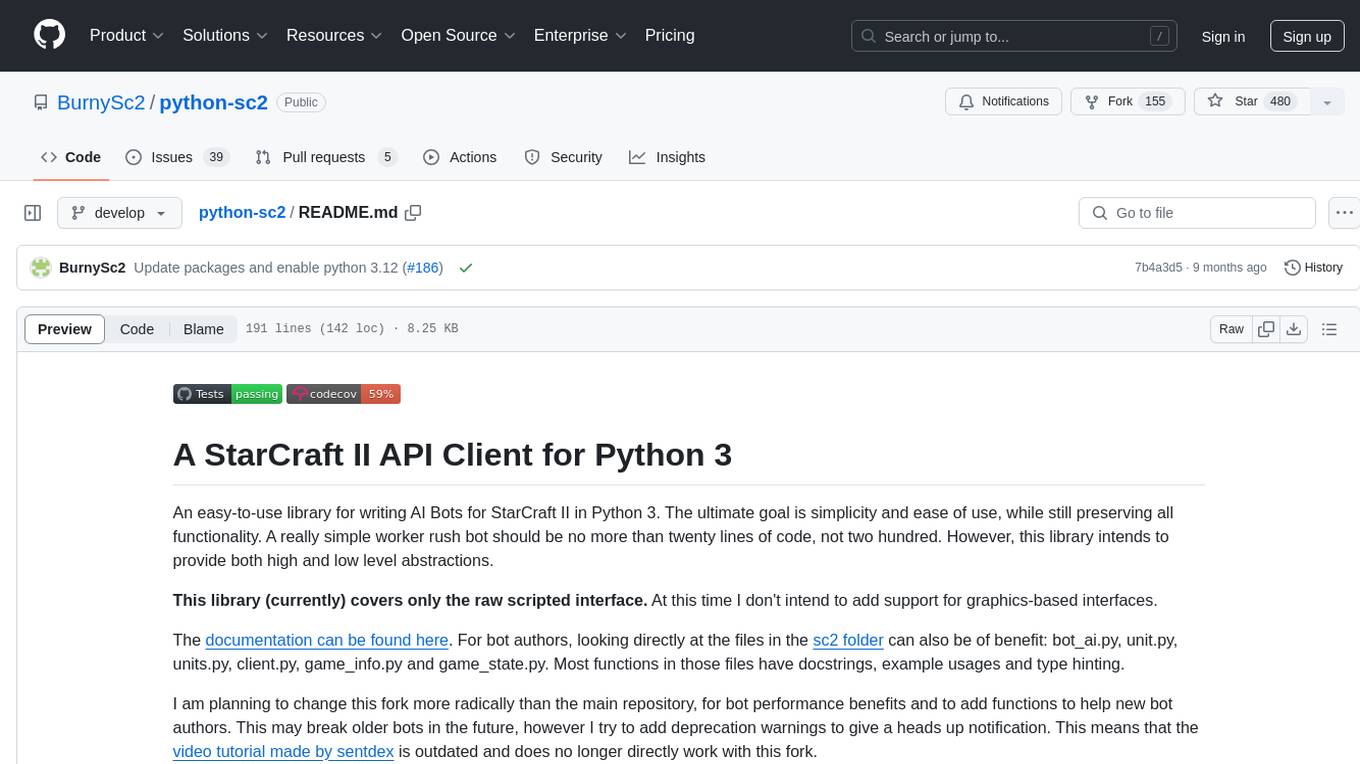
python-sc2
python-sc2 is an easy-to-use library for writing AI Bots for StarCraft II in Python 3. It aims for simplicity and ease of use while providing both high and low level abstractions. The library covers only the raw scripted interface and intends to help new bot authors with added functions. Users can install the library using pip and need a StarCraft II executable to run bots. The API configuration options allow users to customize bot behavior and performance. The community provides support through Discord servers, and users can contribute to the project by creating new issues or pull requests following style guidelines.
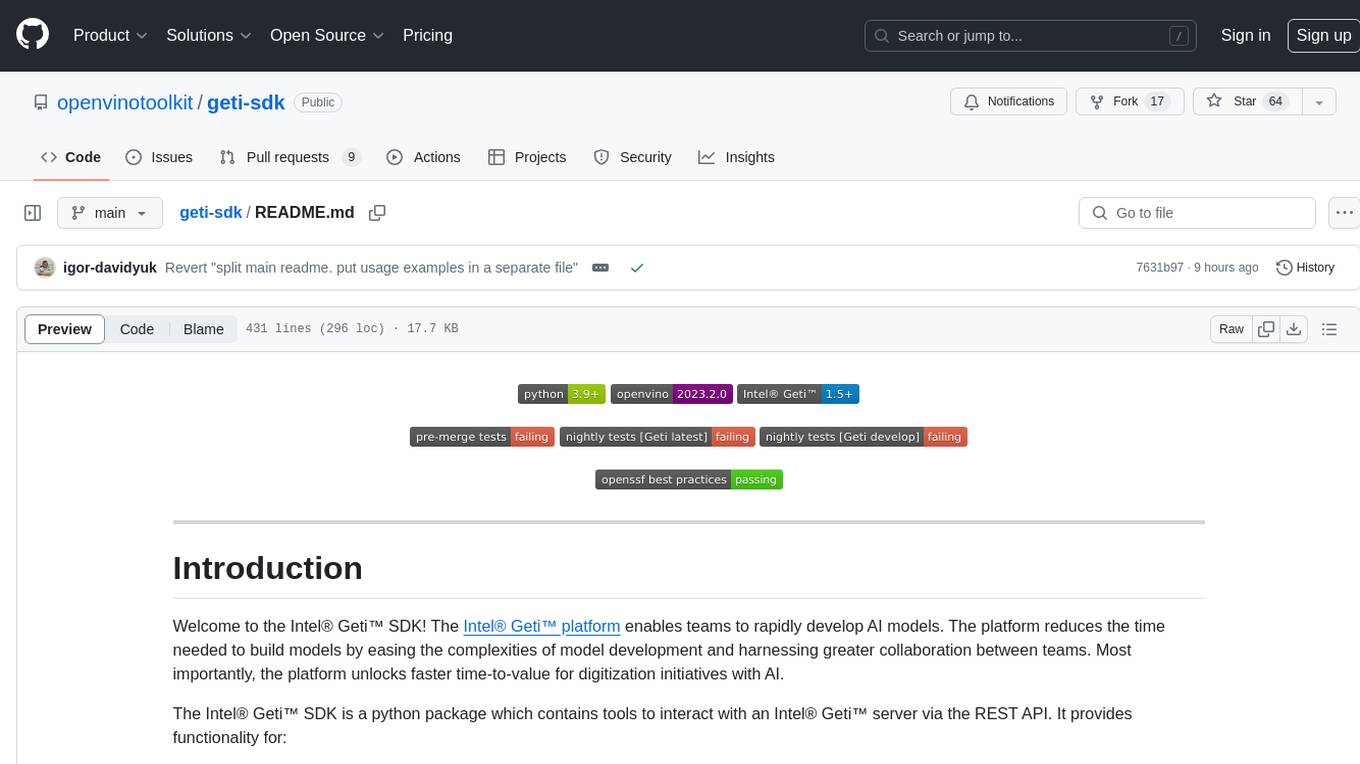
geti-sdk
The Intel® Geti™ SDK is a python package that enables teams to rapidly develop AI models by easing the complexities of model development and enhancing collaboration between teams. It provides tools to interact with an Intel® Geti™ server via the REST API, allowing for project creation, downloading, uploading, deploying for local inference with OpenVINO, setting project and model configuration, launching and monitoring training jobs, and media upload and prediction. The SDK also includes tutorial-style Jupyter notebooks demonstrating its usage.
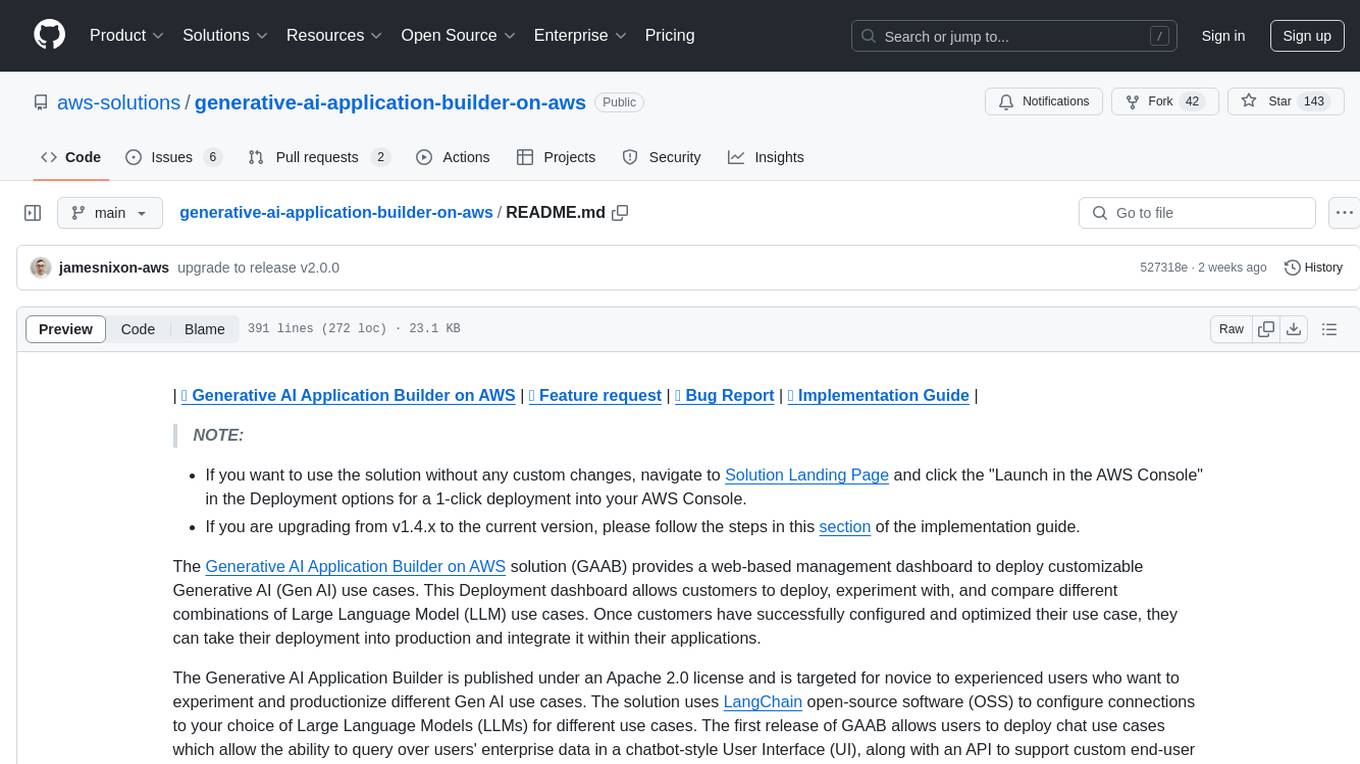
generative-ai-application-builder-on-aws
The Generative AI Application Builder on AWS (GAAB) is a solution that provides a web-based management dashboard for deploying customizable Generative AI (Gen AI) use cases. Users can experiment with and compare different combinations of Large Language Model (LLM) use cases, configure and optimize their use cases, and integrate them into their applications for production. The solution is targeted at novice to experienced users who want to experiment and productionize different Gen AI use cases. It uses LangChain open-source software to configure connections to Large Language Models (LLMs) for various use cases, with the ability to deploy chat use cases that allow querying over users' enterprise data in a chatbot-style User Interface (UI) and support custom end-user implementations through an API.
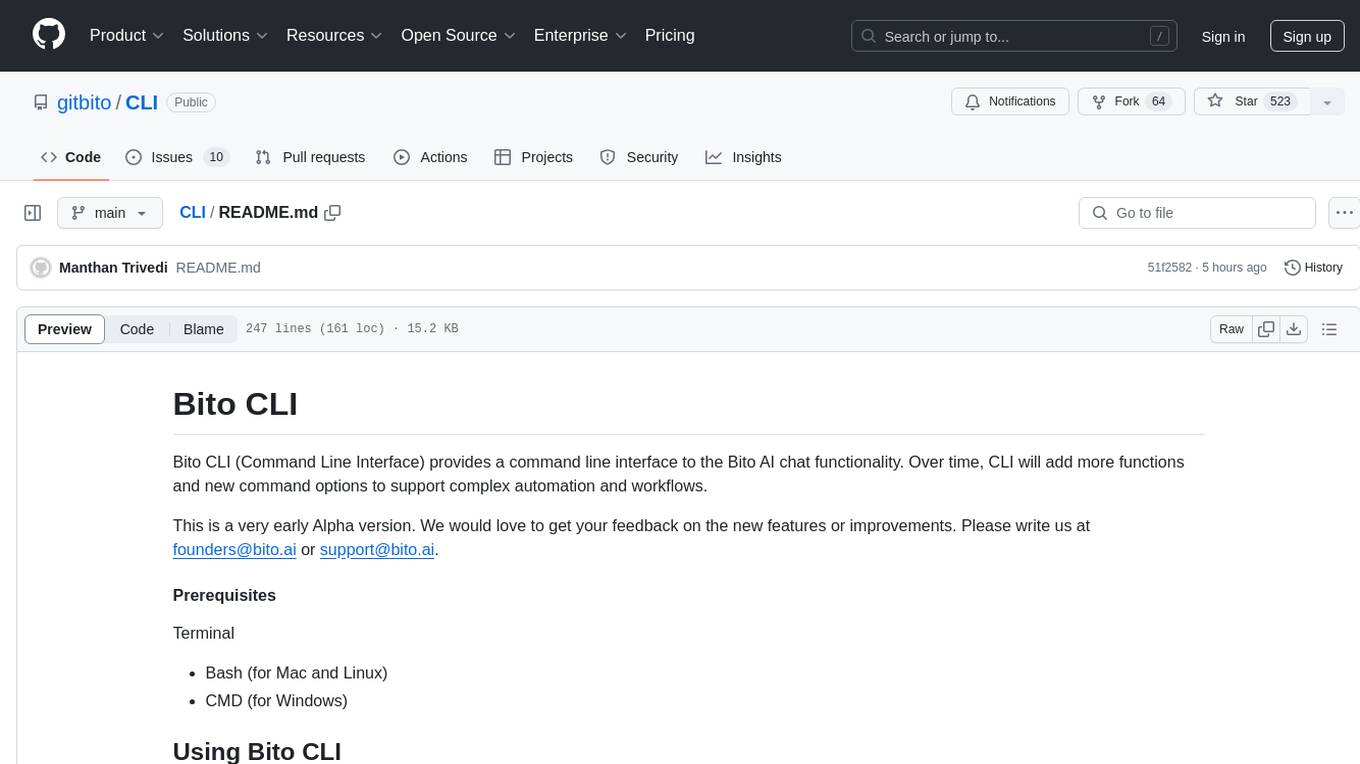
CLI
Bito CLI provides a command line interface to the Bito AI chat functionality, allowing users to interact with the AI through commands. It supports complex automation and workflows, with features like long prompts and slash commands. Users can install Bito CLI on Mac, Linux, and Windows systems using various methods. The tool also offers configuration options for AI model type, access key management, and output language customization. Bito CLI is designed to enhance user experience in querying AI models and automating tasks through the command line interface.
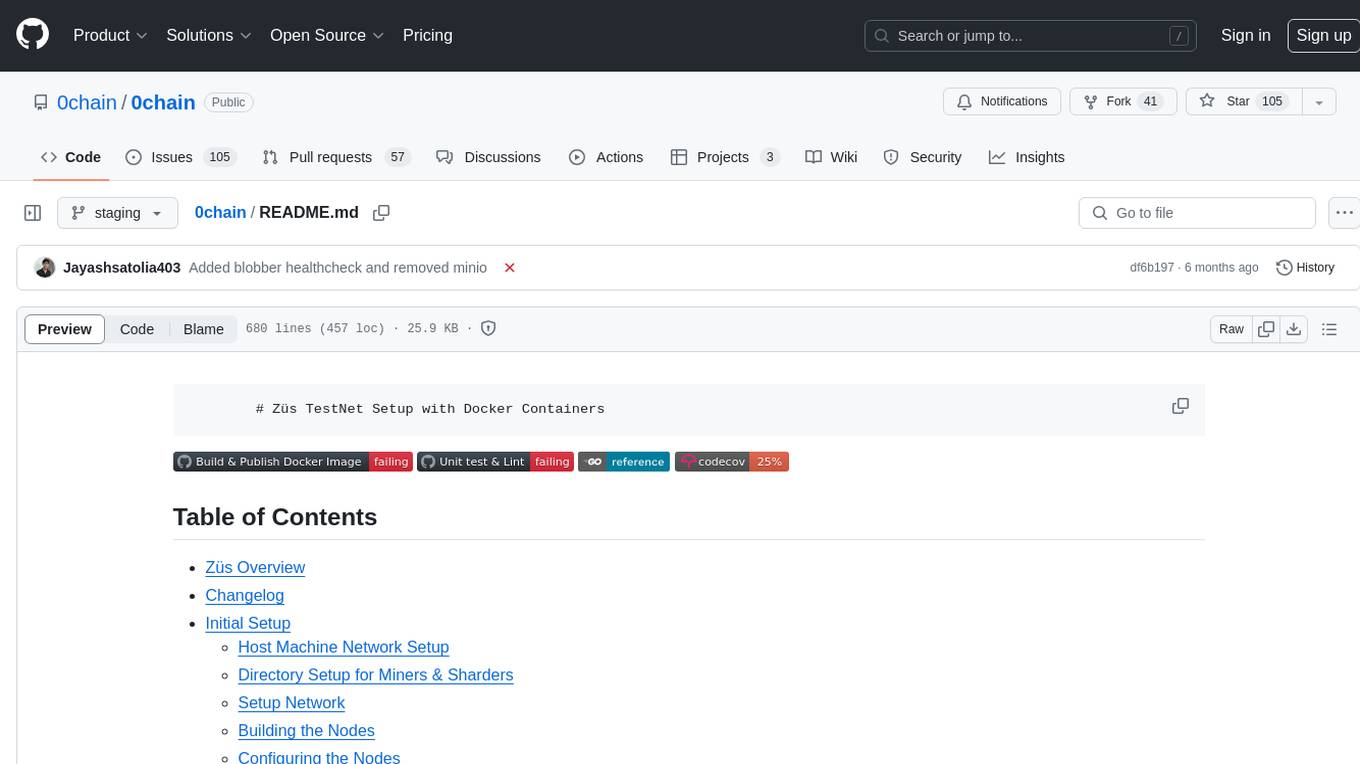
0chain
ZĂĽs is a high-performance cloud on a fast blockchain offering privacy and configurable uptime. It uses erasure code to distribute data between data and parity servers, allowing flexibility for IT managers to design for security and uptime. Users can easily share encrypted data with business partners through a proxy key sharing protocol. The ecosystem includes apps like Blimp for cloud migration, Vult for personal cloud storage, and Chalk for NFT artists. Other apps include Bolt for secure wallet and staking, Atlus for blockchain explorer, and Chimney for network participation. The QoS protocol challenges providers based on response time, while the privacy protocol enables secure data sharing. ZĂĽs supports hybrid and multi-cloud architectures, allowing users to improve regulatory compliance and security requirements.
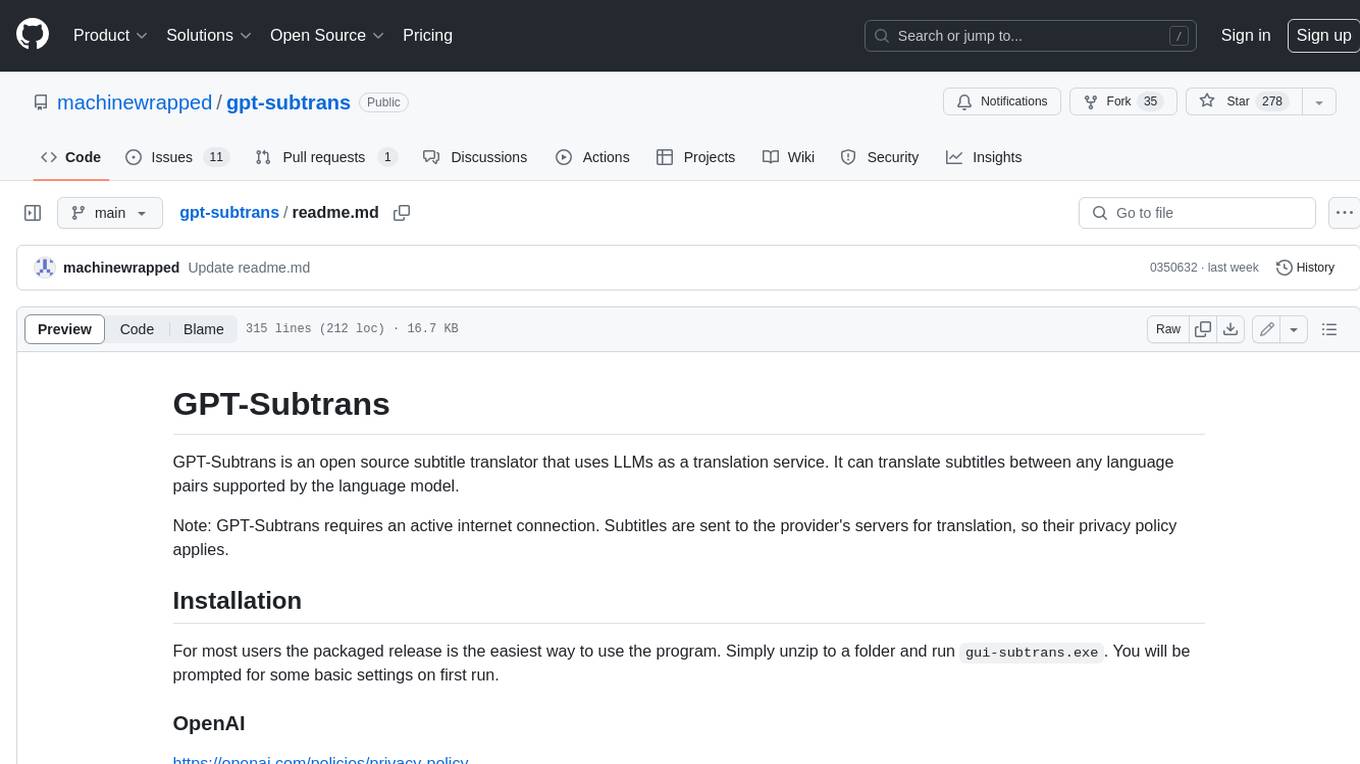
gpt-subtrans
GPT-Subtrans is an open-source subtitle translator that utilizes large language models (LLMs) as translation services. It supports translation between any language pairs that the language model supports. Note that GPT-Subtrans requires an active internet connection, as subtitles are sent to the provider's servers for translation, and their privacy policy applies.
For similar tasks
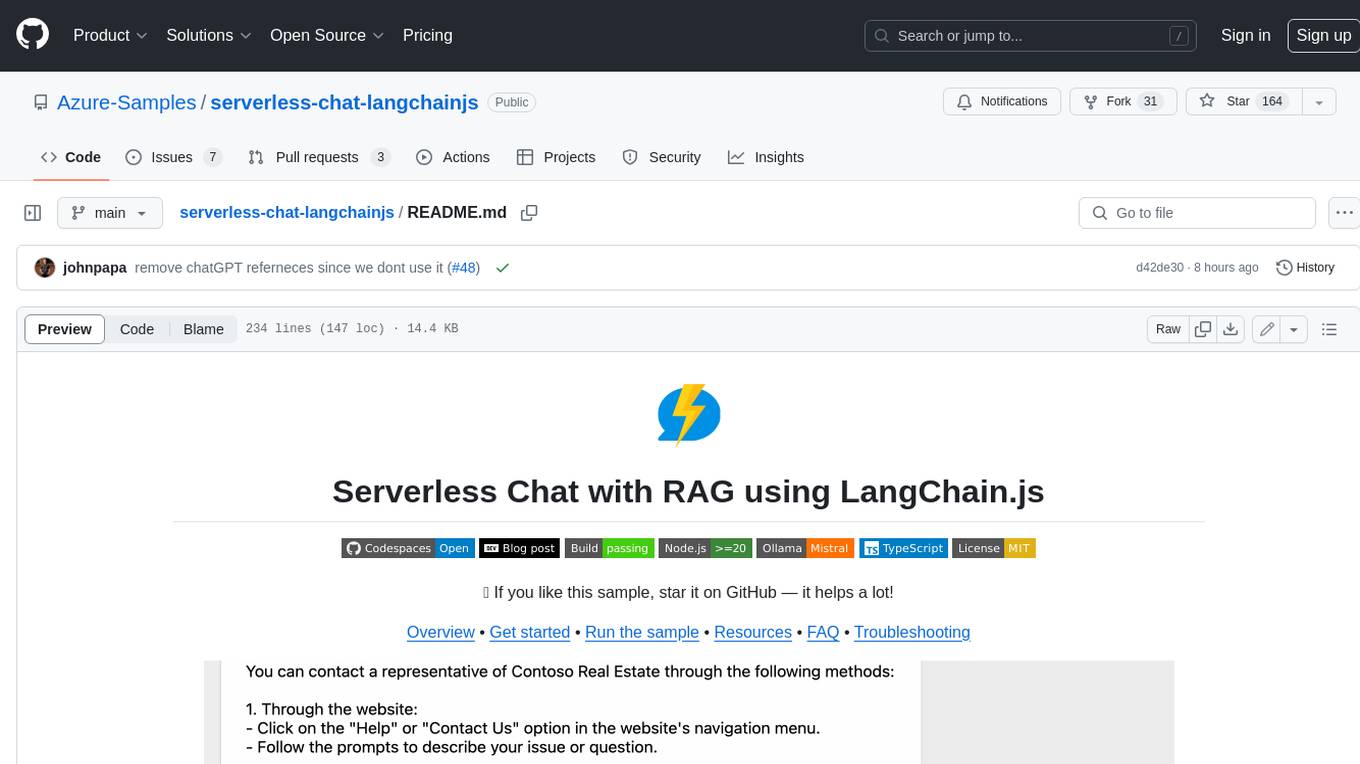
serverless-chat-langchainjs
This sample shows how to build a serverless chat experience with Retrieval-Augmented Generation using LangChain.js and Azure. The application is hosted on Azure Static Web Apps and Azure Functions, with Azure Cosmos DB for MongoDB vCore as the vector database. You can use it as a starting point for building more complex AI applications.
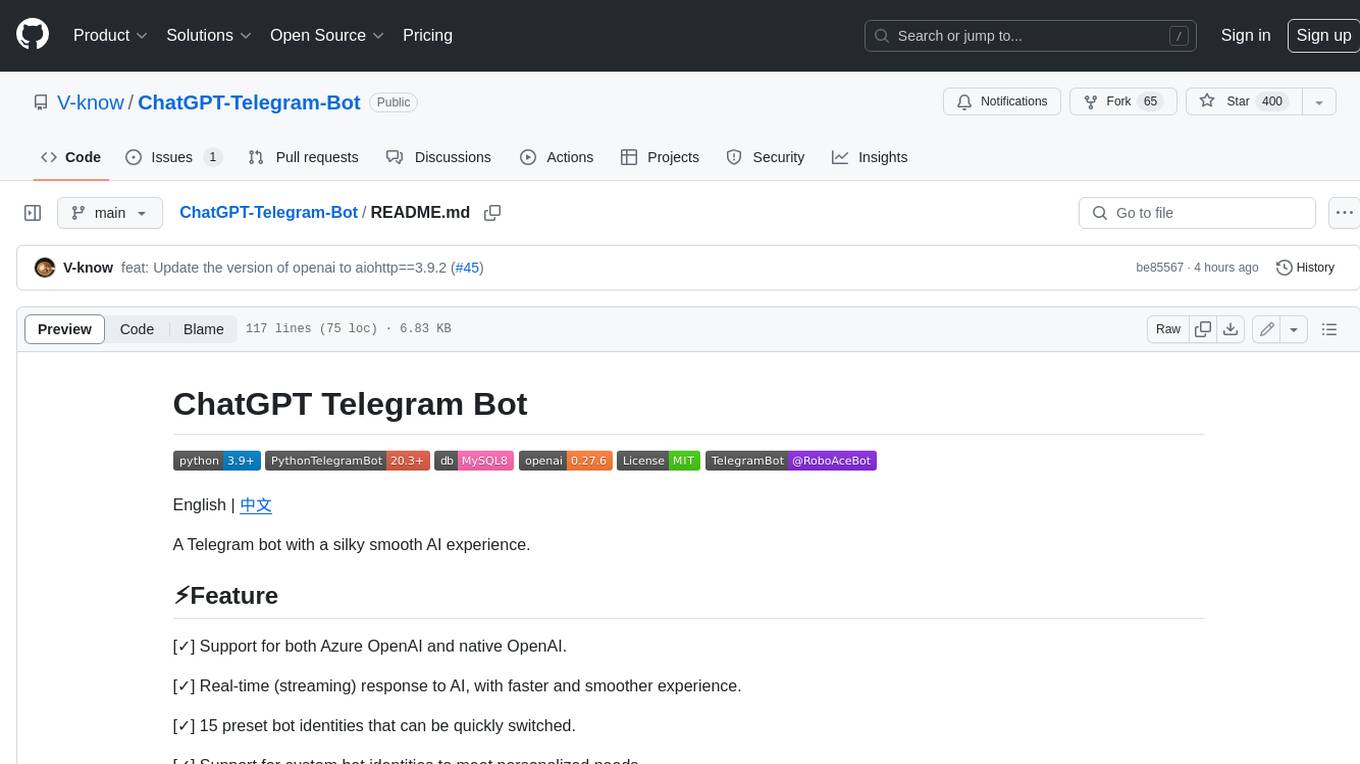
ChatGPT-Telegram-Bot
ChatGPT Telegram Bot is a Telegram bot that provides a smooth AI experience. It supports both Azure OpenAI and native OpenAI, and offers real-time (streaming) response to AI, with a faster and smoother experience. The bot also has 15 preset bot identities that can be quickly switched, and supports custom bot identities to meet personalized needs. Additionally, it supports clearing the contents of the chat with a single click, and restarting the conversation at any time. The bot also supports native Telegram bot button support, making it easy and intuitive to implement required functions. User level division is also supported, with different levels enjoying different single session token numbers, context numbers, and session frequencies. The bot supports English and Chinese on UI, and is containerized for easy deployment.
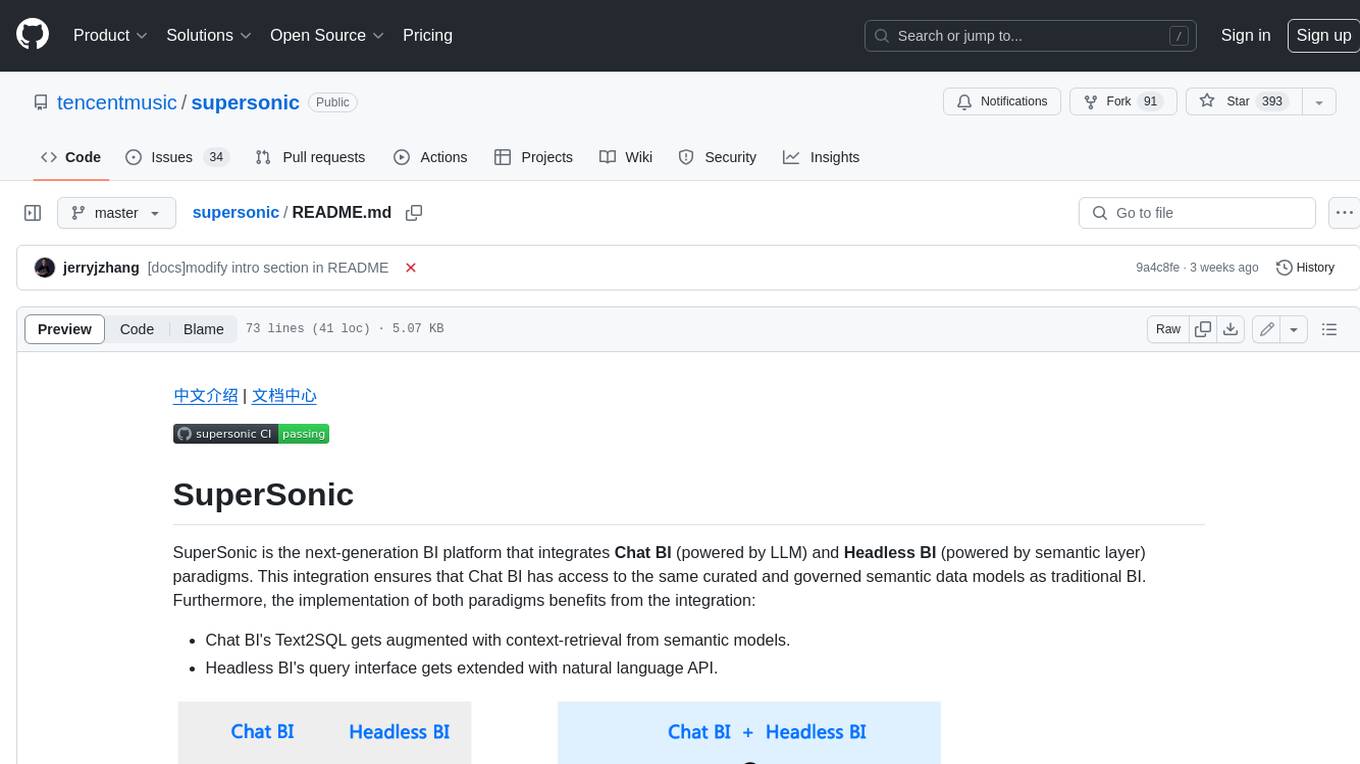
supersonic
SuperSonic is a next-generation BI platform that integrates Chat BI (powered by LLM) and Headless BI (powered by semantic layer) paradigms. This integration ensures that Chat BI has access to the same curated and governed semantic data models as traditional BI. Furthermore, the implementation of both paradigms benefits from the integration: * Chat BI's Text2SQL gets augmented with context-retrieval from semantic models. * Headless BI's query interface gets extended with natural language API. SuperSonic provides a Chat BI interface that empowers users to query data using natural language and visualize the results with suitable charts. To enable such experience, the only thing necessary is to build logical semantic models (definition of metric/dimension/tag, along with their meaning and relationships) through a Headless BI interface. Meanwhile, SuperSonic is designed to be extensible and composable, allowing custom implementations to be added and configured with Java SPI. The integration of Chat BI and Headless BI has the potential to enhance the Text2SQL generation in two dimensions: 1. Incorporate data semantics (such as business terms, column values, etc.) into the prompt, enabling LLM to better understand the semantics and reduce hallucination. 2. Offload the generation of advanced SQL syntax (such as join, formula, etc.) from LLM to the semantic layer to reduce complexity. With these ideas in mind, we develop SuperSonic as a practical reference implementation and use it to power our real-world products. Additionally, to facilitate further development we decide to open source SuperSonic as an extensible framework.
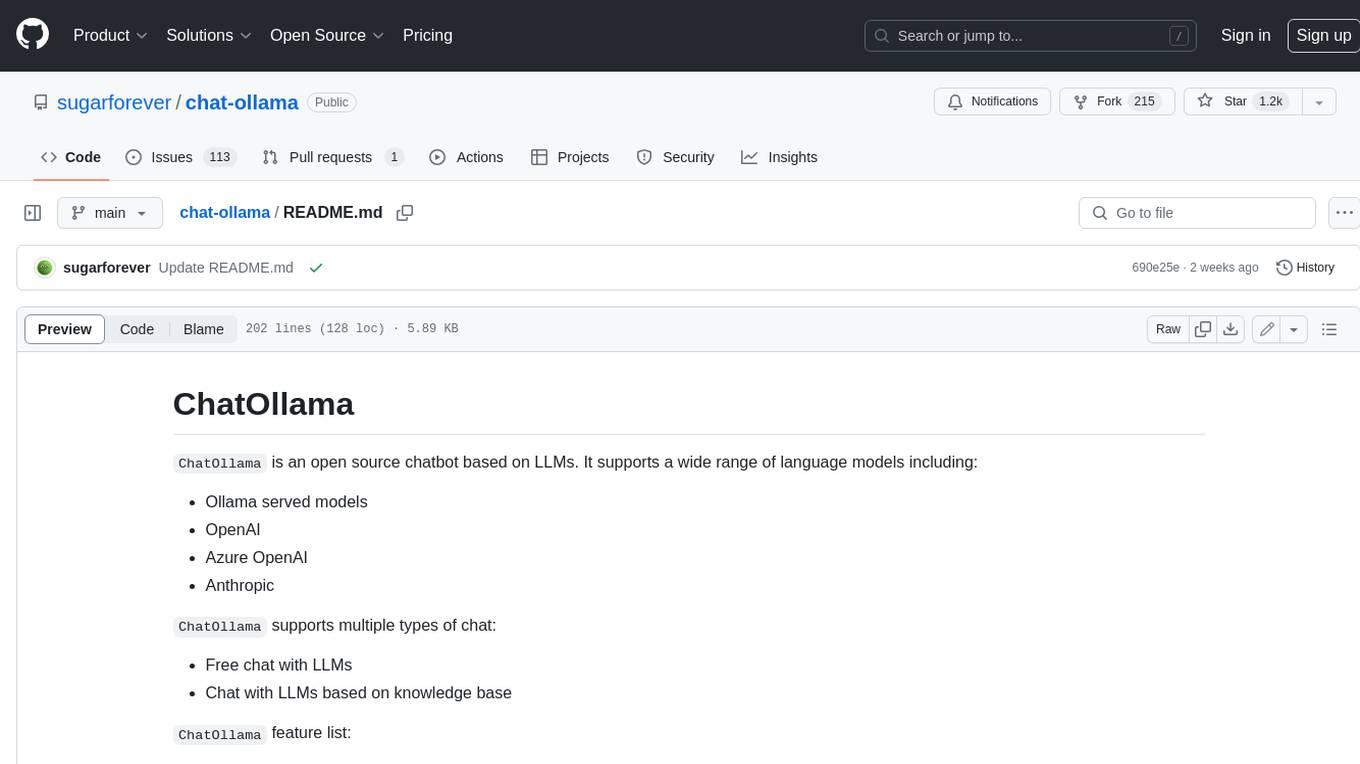
chat-ollama
ChatOllama is an open-source chatbot based on LLMs (Large Language Models). It supports a wide range of language models, including Ollama served models, OpenAI, Azure OpenAI, and Anthropic. ChatOllama supports multiple types of chat, including free chat with LLMs and chat with LLMs based on a knowledge base. Key features of ChatOllama include Ollama models management, knowledge bases management, chat, and commercial LLMs API keys management.
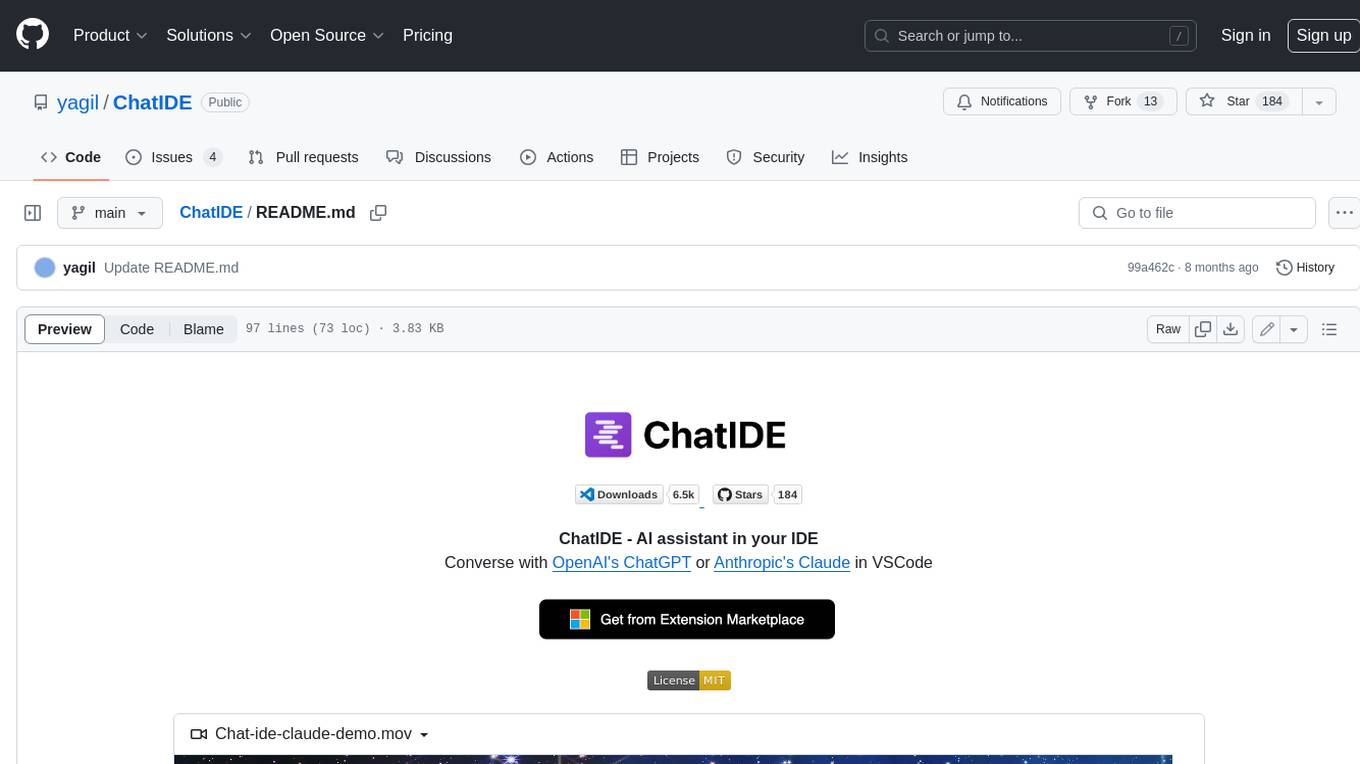
ChatIDE
ChatIDE is an AI assistant that integrates with your IDE, allowing you to converse with OpenAI's ChatGPT or Anthropic's Claude within your development environment. It provides a seamless way to access AI-powered assistance while coding, enabling you to get real-time help, generate code snippets, debug errors, and brainstorm ideas without leaving your IDE.
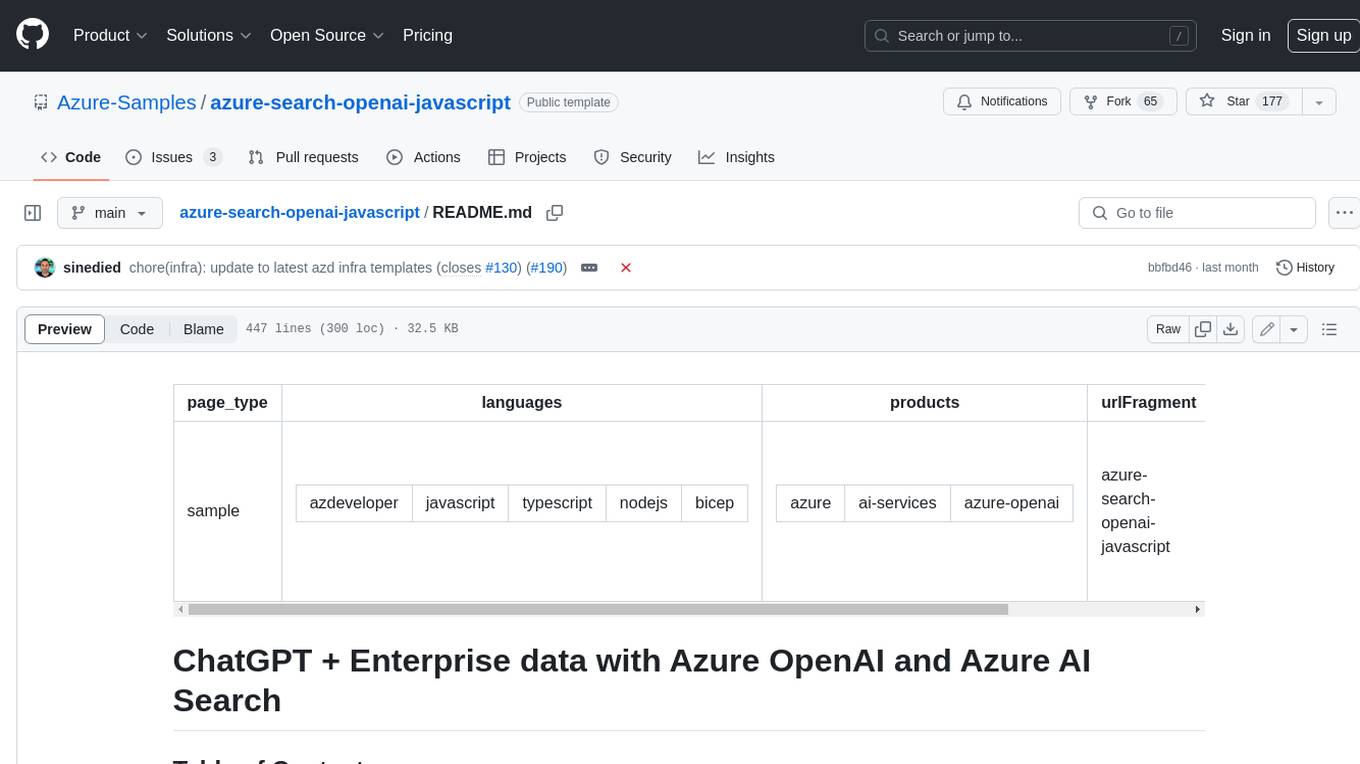
azure-search-openai-javascript
This sample demonstrates a few approaches for creating ChatGPT-like experiences over your own data using the Retrieval Augmented Generation pattern. It uses Azure OpenAI Service to access the ChatGPT model (gpt-35-turbo), and Azure AI Search for data indexing and retrieval.
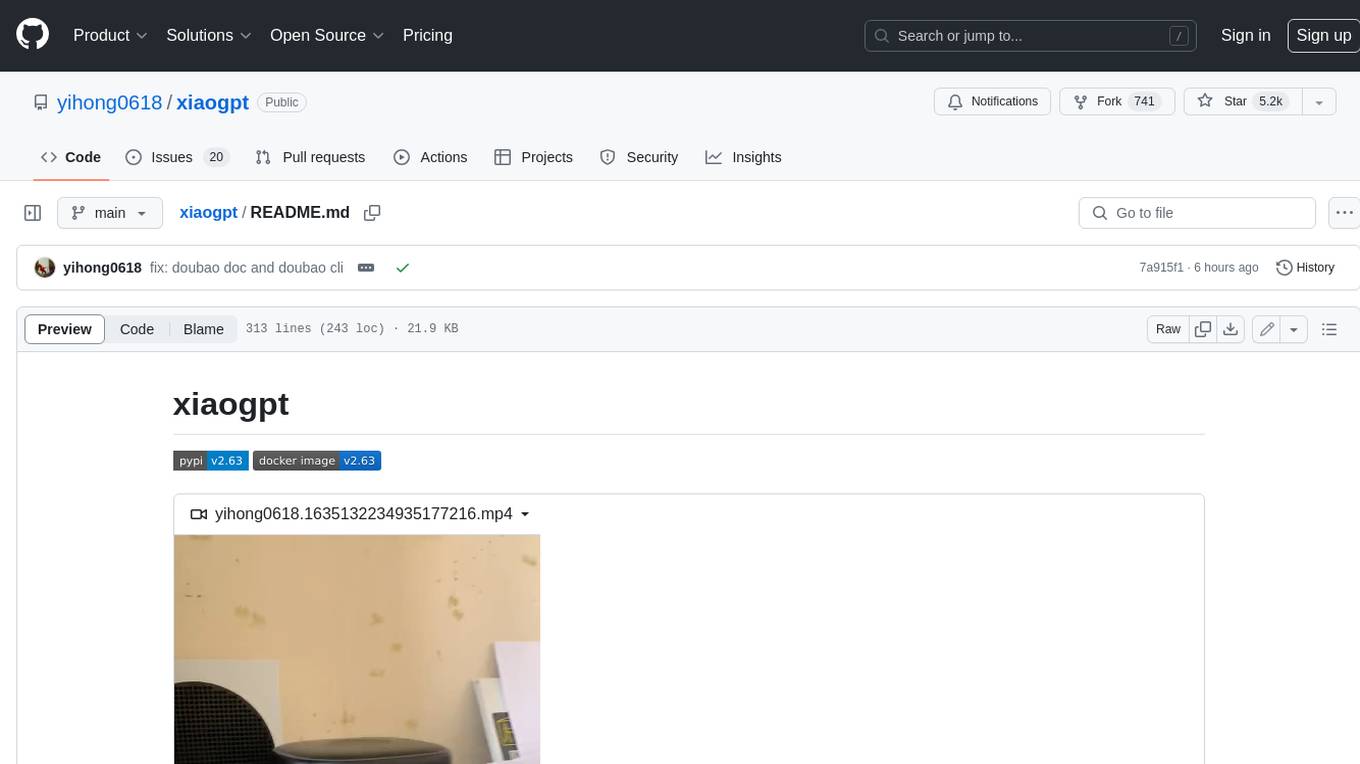
xiaogpt
xiaogpt is a tool that allows you to play ChatGPT and other LLMs with Xiaomi AI Speaker. It supports ChatGPT, New Bing, ChatGLM, Gemini, Doubao, and Tongyi Qianwen. You can use it to ask questions, get answers, and have conversations with AI assistants. xiaogpt is easy to use and can be set up in a few minutes. It is a great way to experience the power of AI and have fun with your Xiaomi AI Speaker.
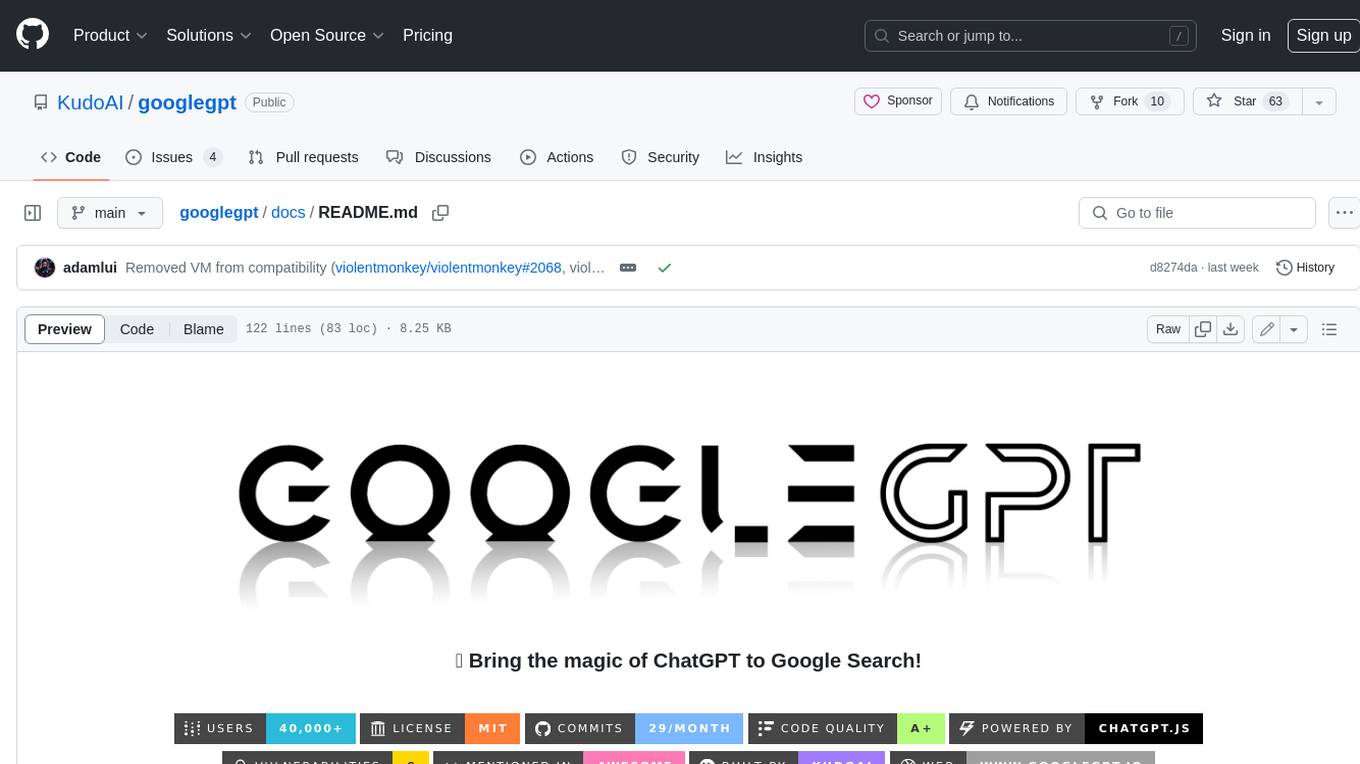
googlegpt
GoogleGPT is a browser extension that brings the power of ChatGPT to Google Search. With GoogleGPT, you can ask ChatGPT questions and get answers directly in your search results. You can also use GoogleGPT to generate text, translate languages, and more. GoogleGPT is compatible with all major browsers, including Chrome, Firefox, Edge, and Safari.
For similar jobs
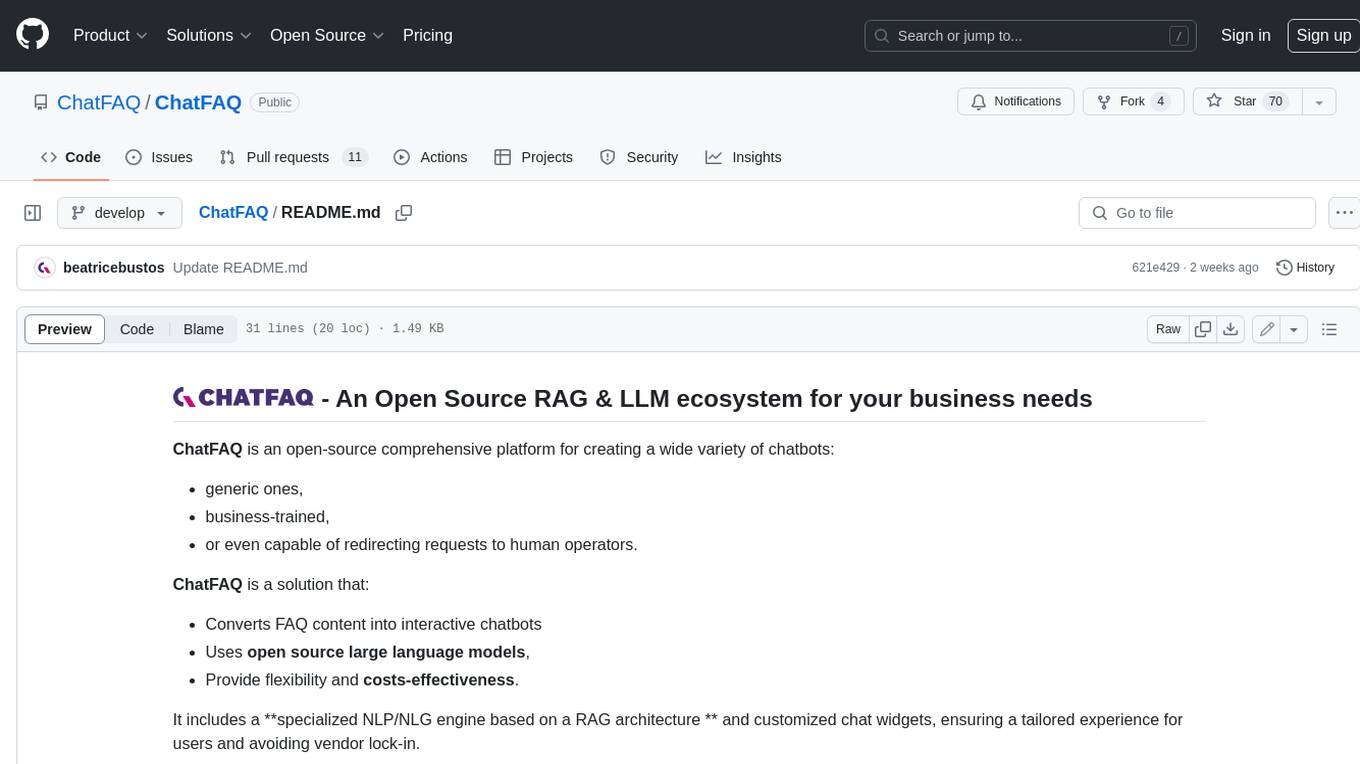
ChatFAQ
ChatFAQ is an open-source comprehensive platform for creating a wide variety of chatbots: generic ones, business-trained, or even capable of redirecting requests to human operators. It includes a specialized NLP/NLG engine based on a RAG architecture and customized chat widgets, ensuring a tailored experience for users and avoiding vendor lock-in.
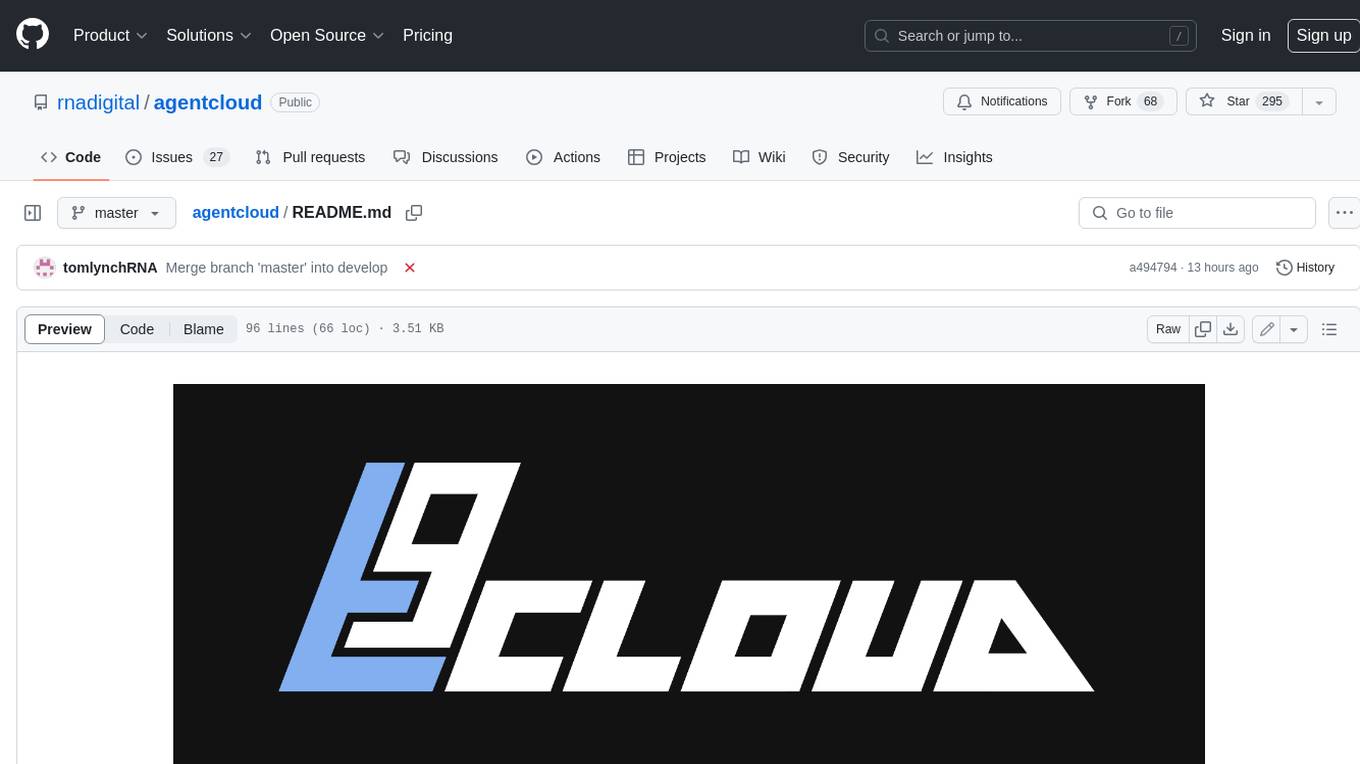
agentcloud
AgentCloud is an open-source platform that enables companies to build and deploy private LLM chat apps, empowering teams to securely interact with their data. It comprises three main components: Agent Backend, Webapp, and Vector Proxy. To run this project locally, clone the repository, install Docker, and start the services. The project is licensed under the GNU Affero General Public License, version 3 only. Contributions and feedback are welcome from the community.
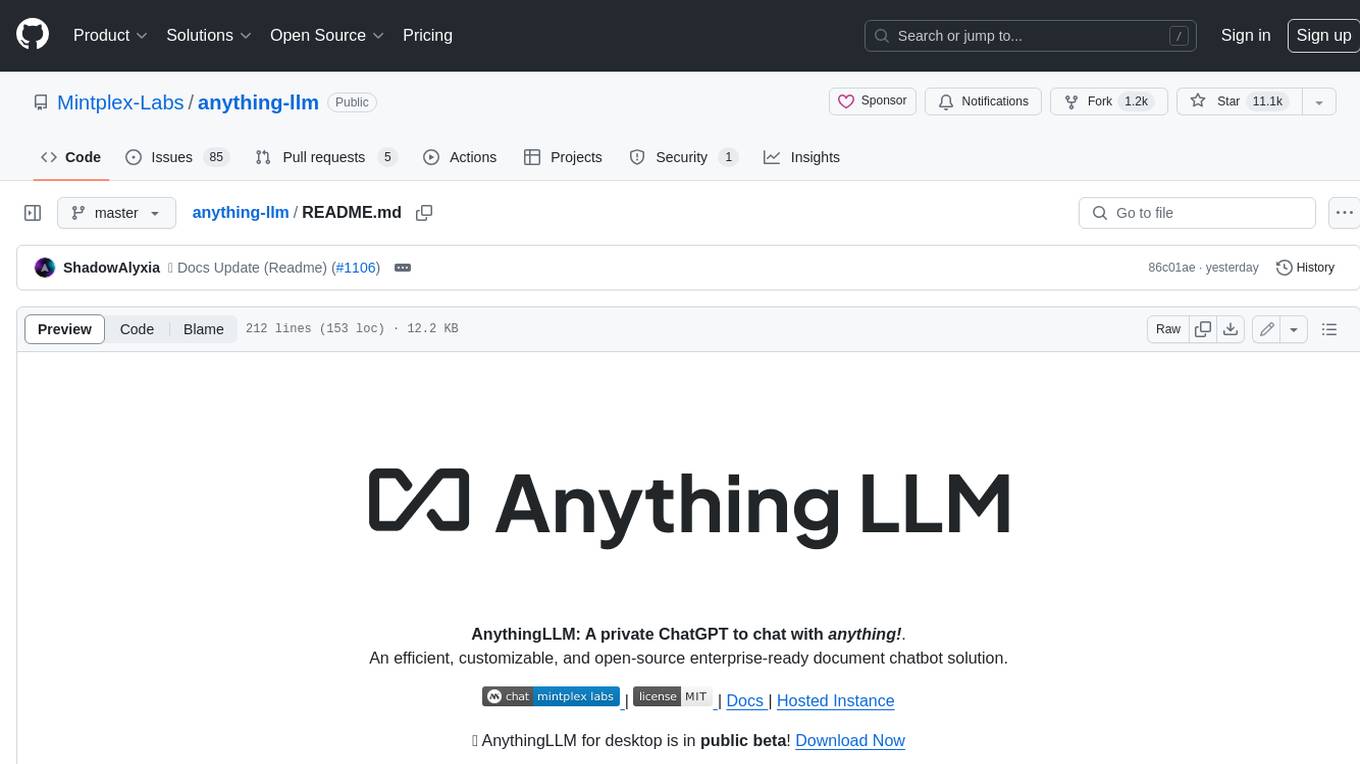
anything-llm
AnythingLLM is a full-stack application that enables you to turn any document, resource, or piece of content into context that any LLM can use as references during chatting. This application allows you to pick and choose which LLM or Vector Database you want to use as well as supporting multi-user management and permissions.
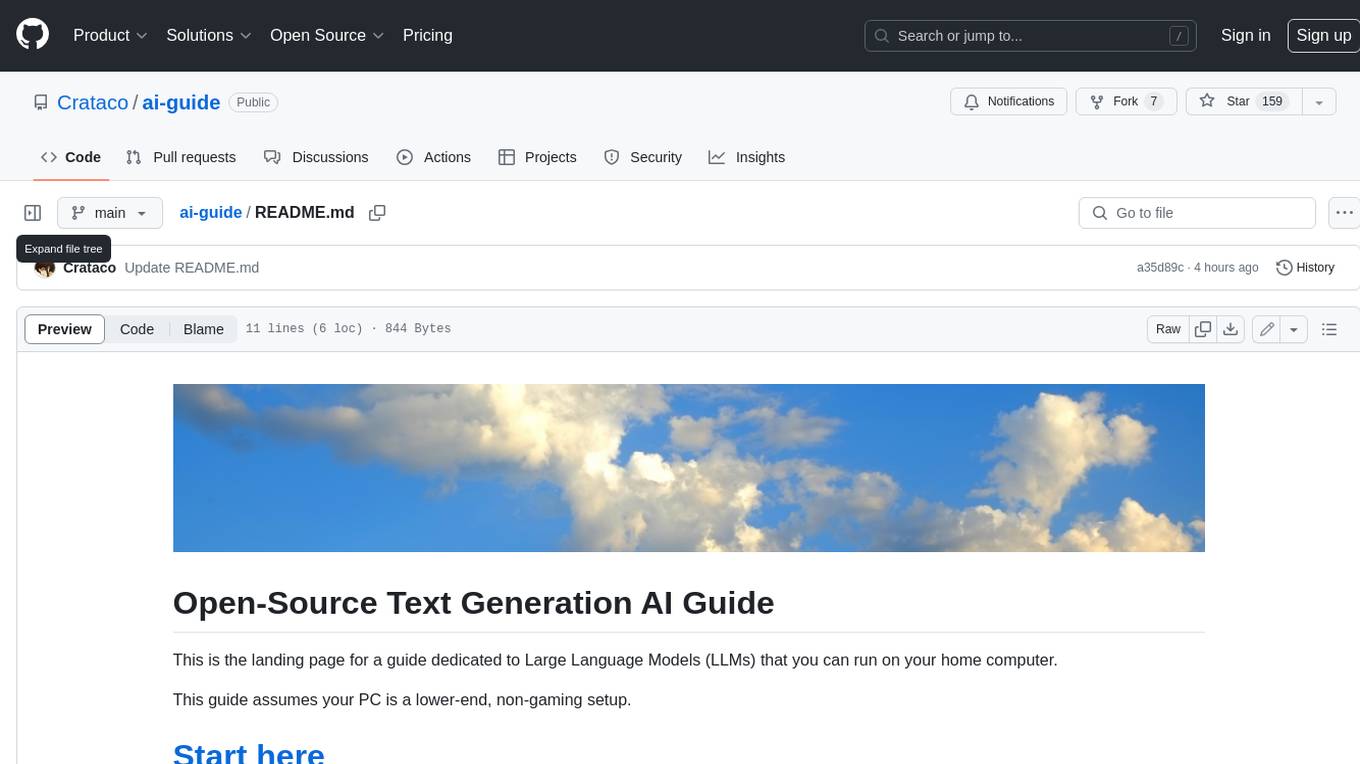
ai-guide
This guide is dedicated to Large Language Models (LLMs) that you can run on your home computer. It assumes your PC is a lower-end, non-gaming setup.
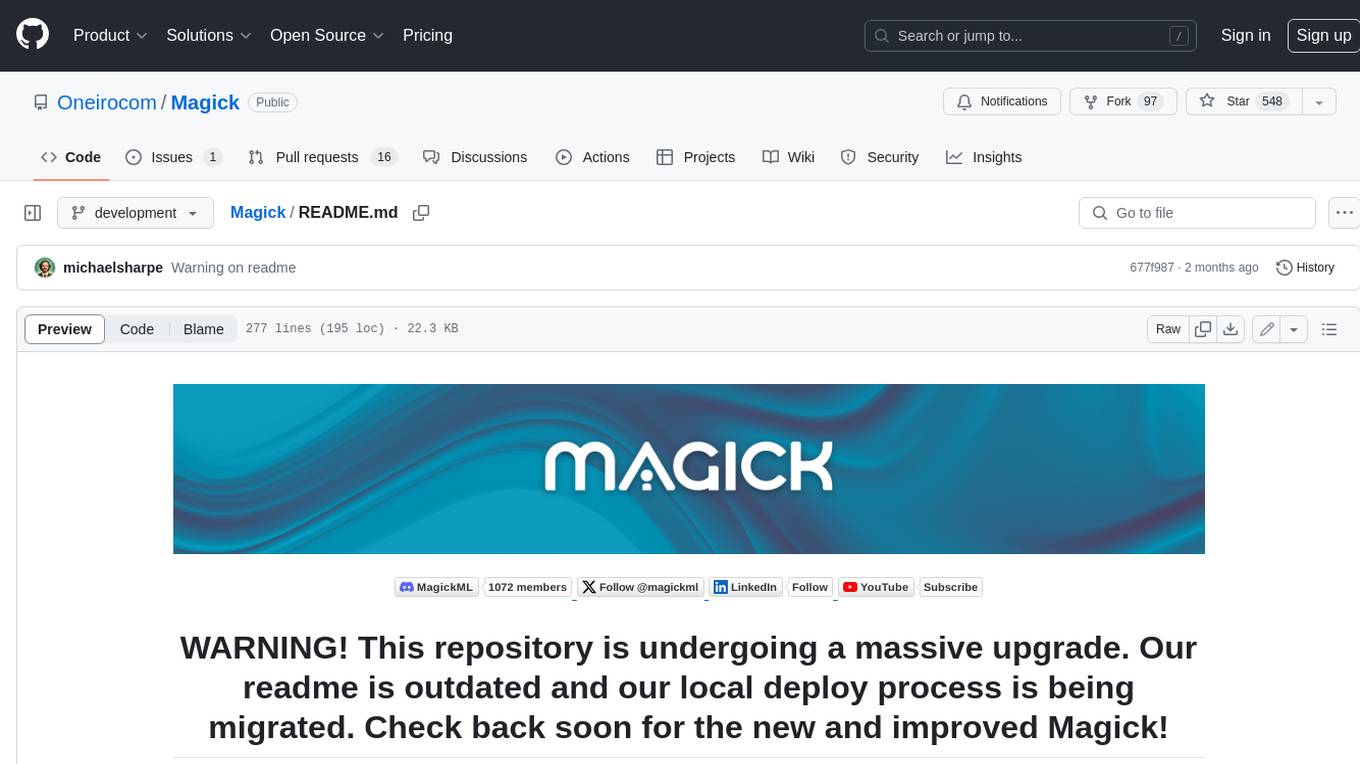
Magick
Magick is a groundbreaking visual AIDE (Artificial Intelligence Development Environment) for no-code data pipelines and multimodal agents. Magick can connect to other services and comes with nodes and templates well-suited for intelligent agents, chatbots, complex reasoning systems and realistic characters.
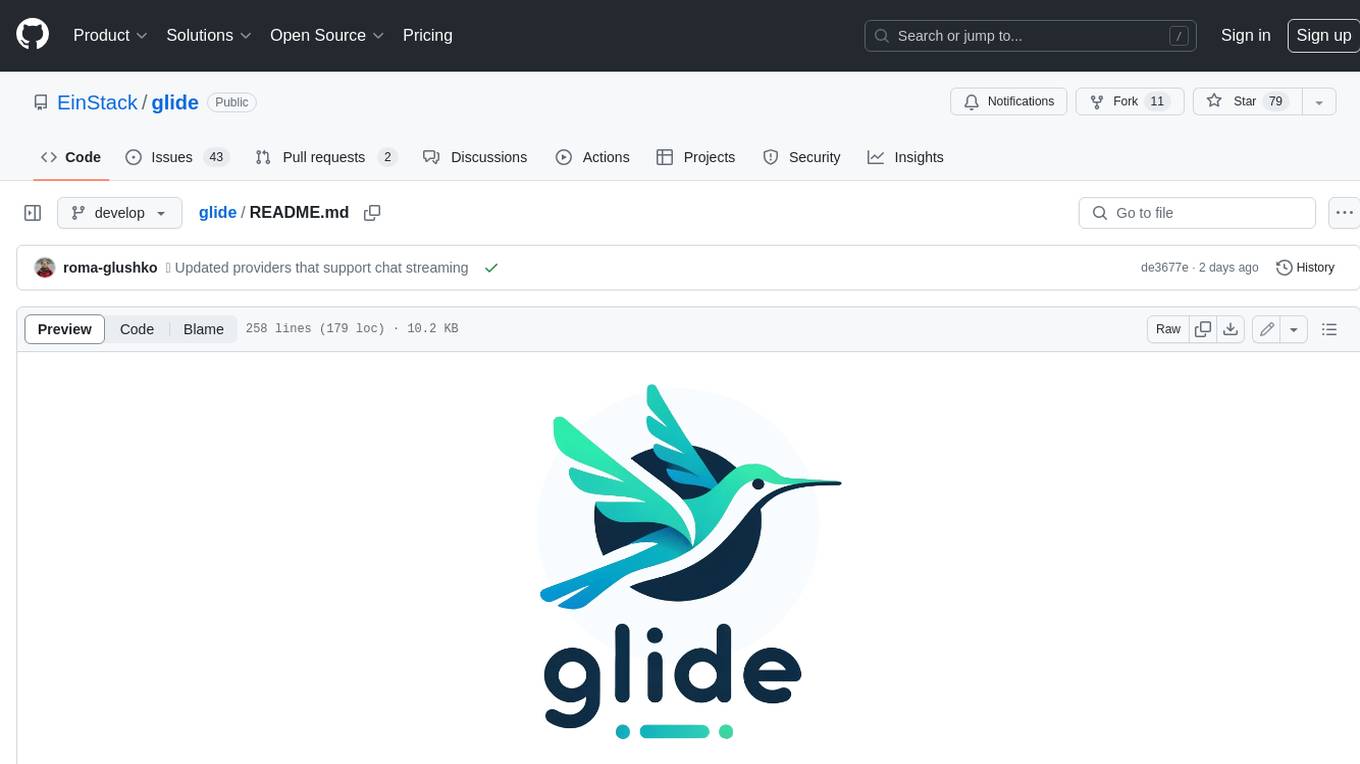
glide
Glide is a cloud-native LLM gateway that provides a unified REST API for accessing various large language models (LLMs) from different providers. It handles LLMOps tasks such as model failover, caching, key management, and more, making it easy to integrate LLMs into applications. Glide supports popular LLM providers like OpenAI, Anthropic, Azure OpenAI, AWS Bedrock (Titan), Cohere, Google Gemini, OctoML, and Ollama. It offers high availability, performance, and observability, and provides SDKs for Python and NodeJS to simplify integration.
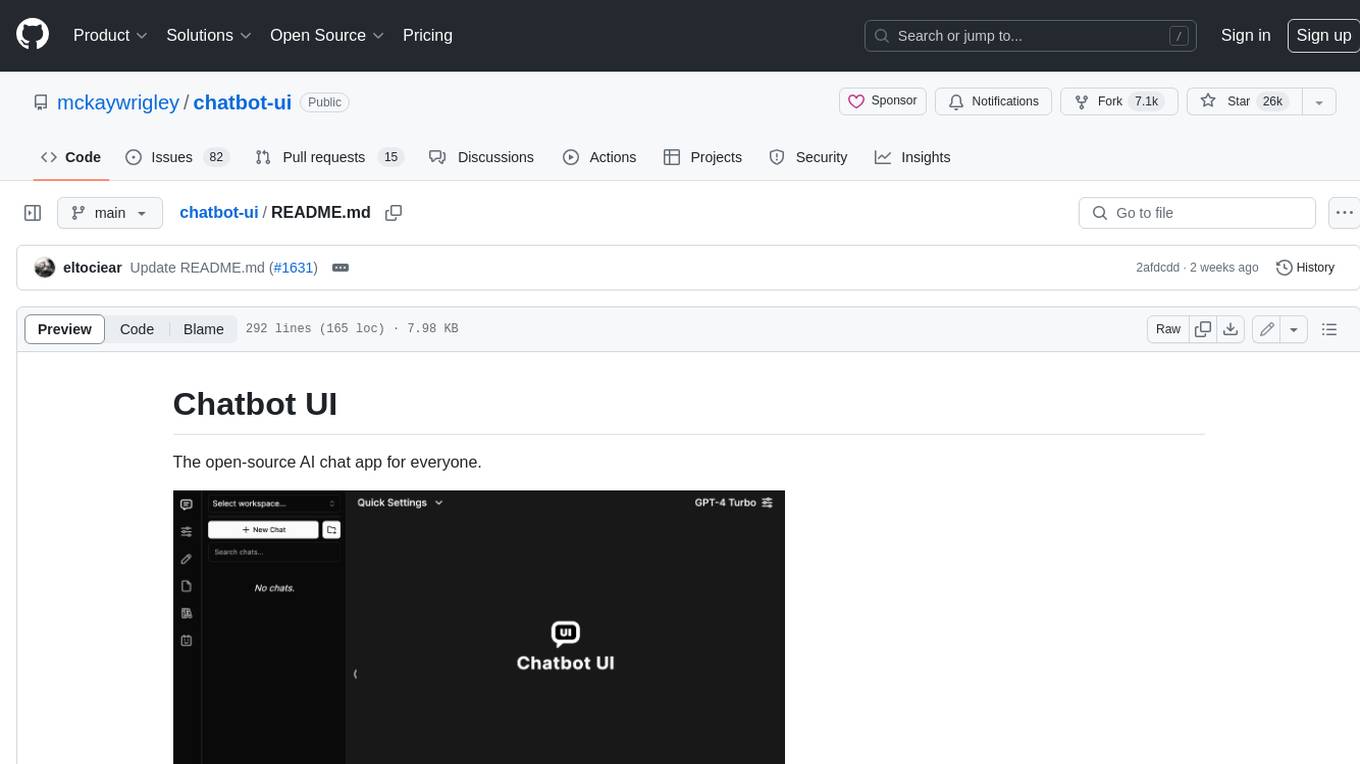
chatbot-ui
Chatbot UI is an open-source AI chat app that allows users to create and deploy their own AI chatbots. It is easy to use and can be customized to fit any need. Chatbot UI is perfect for businesses, developers, and anyone who wants to create a chatbot.
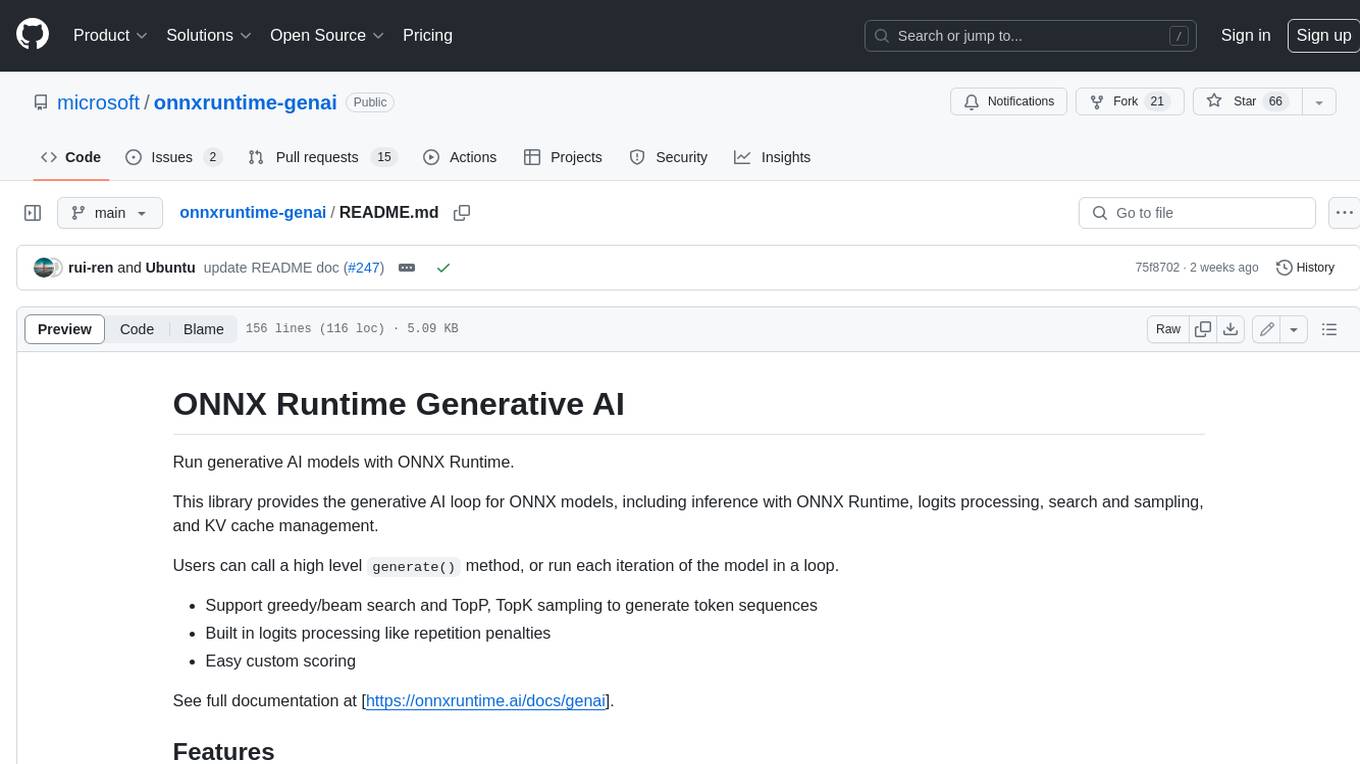
onnxruntime-genai
ONNX Runtime Generative AI is a library that provides the generative AI loop for ONNX models, including inference with ONNX Runtime, logits processing, search and sampling, and KV cache management. Users can call a high level `generate()` method, or run each iteration of the model in a loop. It supports greedy/beam search and TopP, TopK sampling to generate token sequences, has built in logits processing like repetition penalties, and allows for easy custom scoring.