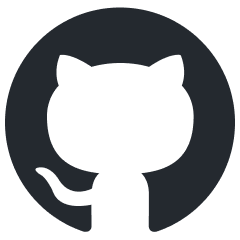
airbyte_serverless
Airbyte made simple (no UI, no database, no cluster)
Stars: 135
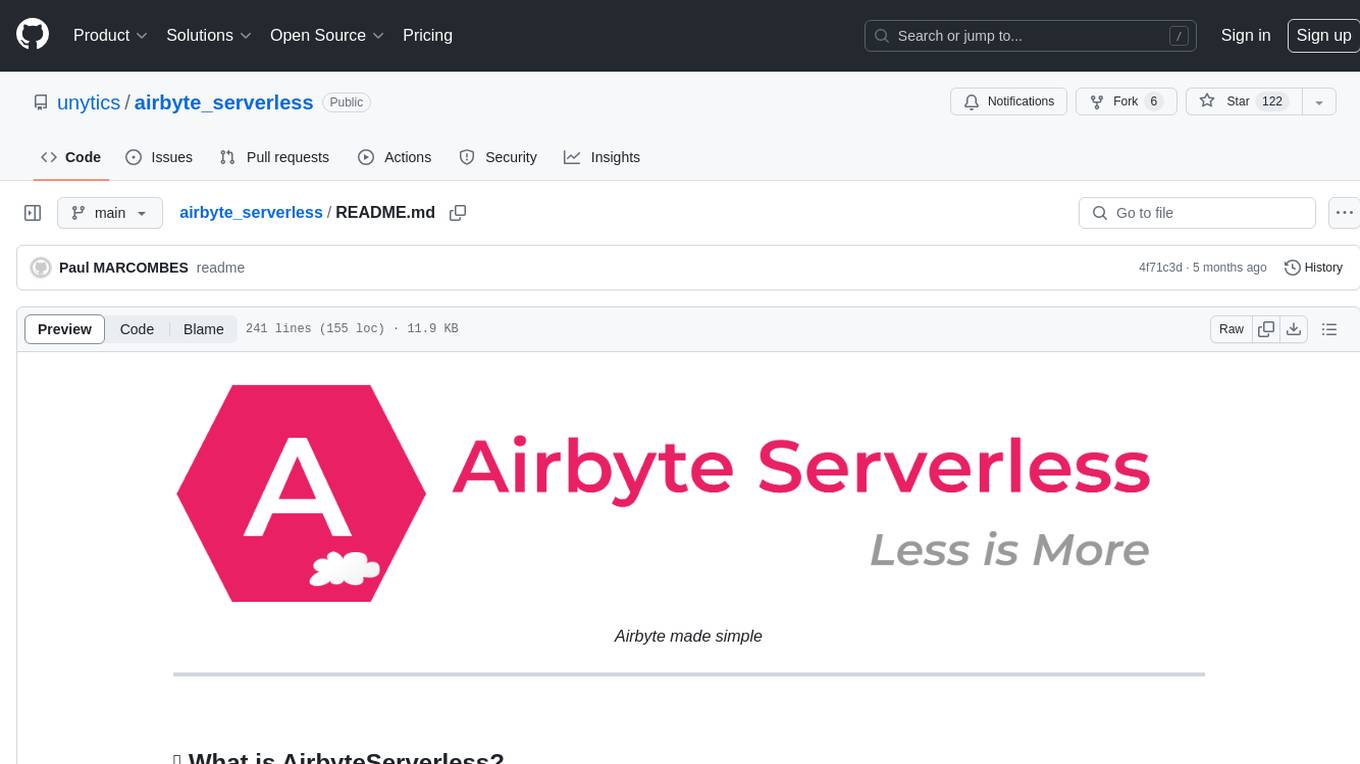
AirbyteServerless is a lightweight tool designed to simplify the management of Airbyte connectors. It offers a serverless mode for running connectors, allowing users to easily move data from any source to their data warehouse. Unlike the full Airbyte-Open-Source-Platform, AirbyteServerless focuses solely on the Extract-Load process without a UI, database, or transform layer. It provides a CLI tool, 'abs', for managing connectors, creating connections, running jobs, selecting specific data streams, handling secrets securely, and scheduling remote runs. The tool is scalable, allowing independent deployment of multiple connectors. It aims to streamline the connector management process and provide a more agile alternative to the comprehensive Airbyte platform.
README:
Airbyte made simple
AirbyteServerless is a simple tool to manage Airbyte connectors, run them locally or deploy them in serverless mode.
Airbyte is a must-have in your data-stack with its catalog of open-source connectors to move your data from any source to your data-warehouse.
To manage these connectors, Airbyte offers Airbyte-Open-Source-Platform which includes a server, workers, database, UI, orchestrator, connectors, secret manager, logs manager, etc.
AirbyteServerless aims at offering a lightweight alternative to Airbyte-Open-Source-Platform to simplify connectors management.
Airbyte-Open-Source-Platform | AirbyteServerless |
---|---|
Has a UI |
Has NO UI Connections configurations are managed by documented yaml files |
Has a database |
Has NO database - Configurations files are versioned in git - The destination stores the state (the checkpoint of where sync stops) and logs which can then be visualized with your preferred BI tool |
Has a transform layer Airbyte loads your data in a raw format but then enables you to perform basic transform such as replace, upsert, schema normalization |
Has NO transform layer - Data is appended in your destination in raw format. - airbyte_serverless is dedicated to do one thing and do it well: Extract-Load . |
NOT Serverless - Can be deployed on a VM or Kubernetes Cluster. - The platform is made of tens of dependent containers that you CANNOT deploy with serverless |
Serverless - An Airbyte source docker image is upgraded with a destination connector - The upgraded docker image can then be deployed as an isolated Cloud Run Job (or Cloud Run Service )- Cloud Run is natively monitored with metrics, dashboards, logs, error reporting, alerting, etc - It can be scheduled or triggered by events |
Is scalable with conditions Scalable if deployed on autoscaled Kubernetes Cluster and if you are skilled enough. ๐ Check that you are skilled enough with Kubernetes by watching this video ๐. |
Is scalable Each connector is deployed independently of each other. You can have as many as you want. |
abs
is the CLI (command-line-interface) of AirbyteServerless which facilitates connectors management.
pip install airbyte-serverless
abs create my_first_connection --source="airbyte/source-faker:0.1.4" --destination="bigquery" --remote-runner "cloud_run_job"
- Docker is required. Make sure you have it installed. (IF YOU DON'T HAVE DOCKER AND WANT TO RUN A PYTHON CONNECTOR, READ NEXT SECTION)
source
param can be any Public Docker Airbyte Source (here is the list). We recomend that you use faker source to get started.destination
param must be one of the following:
bigquery
- contributions are welcome to offer more destinations ๐ค
remote-runner
param must becloud_run_job
. More integrations will come in the future. This remote-runner is only used if you want to run the connection on a remote runner and schedule it.- The command will create a configuration file
./connections/my_first_connection.yaml
with initialized configuration.- Update this configuration file to suit your needs.
Actually, source
argument can be a docker image as above or any command.
Below, we use pipx
tool to run airbyte-source-faker
python package available on pypi.
abs create my_first_connection --source="pipx run airbyte-source-faker==0.1.4"
The value just after pipx run
can be any Airbyte Python Source available on pypi. For security reasons, beware to check that the source you are going to install is really from Airbyte.
The other arguments are the same as before.
abs run my_first_connection
- This will launch an Extract-Load Job from the source to the destination.
- The
run
commmand will only work if you have correctly edited./connections/my_first_connection.yaml
configuration file.- If you chose
bigquery
destination, you must:
- have
gcloud
installed on your machine with default credentials initialized with the commandgcloud auth application-default login
.- have correctly edited the
destination
section of./connections/my_first_connection.yaml
configuration file. You must havedataEditor
permission on the chosen BigQuery dataset.- Data is always appended at destination (not replaced nor upserted). It will be in raw format.
- If the connector supports incremental extract (extract only new or recently modified data) then this mode is chosen.
You may not want to copy all the data that the source can get. To see all available streams
run:
abs list-available-streams my_first_connection
If you want to configure your connection with only some of these streams, run:
abs set-streams my_first_connection "stream1,stream2"
Next run
executions will extract selected streams only.
For security reasons, you do NOT want to store secrets such as api tokens in your yaml files. Instead, add your secrets in Google Secret Manager by following this documentation. Then you can add the secret resource name in the yaml file such as below:
source:
docker_image: "..."
config:
api_token: GCP_SECRET({SECRET_RESOURCE_NAME})
Replace {SECRET_RESOURCE_NAME}
by your secret resource name which must have the format: projects/{PROJECT_ID}/secrets/{SECRET_ID}/versions/{SECRET_VERSION}
. To get this path:
- Go to the Secret Manager page in the Google Cloud console.
- Go to the Secret Manager page
- On the Secret Manager page, click on the Name of a secret.
- On the Secret details page, in the Versions table, locate a secret version to access.
- In the Actions column, click on the three dots.
- Click on 'Copy Resource Name' from the menu.
WARNING: THIS ONLY WORKS FOR NOW WITH A DOCKER SOURCE in python language.
abs remote-run my_first_connection
- The
remote-run
commmand will only work if you have correctly edited./connections/my_first_connection.yaml
configuration file including theremote_runner
part.
- This command will launch an Extract-Load Job like the
abs run
command. The main difference is that the command will be run on a remote deployed container (we use Cloud Run Job as the only container runner for now).- If you chose
bigquery
destination, the service account you put inservice_account
field ofremote_runner
section of the yaml must bebigquery.dataEditor
on the target dataset and have permission to create some BigQuery jobs in the project.- If your yaml config contains some Google Secrets, the service account you put in
service_account
field ofremote_runner
section of the yaml must have read access to the secrets.
When you create a connection using abs create my_connection --source "SOURCE"
, you can put any docker image you have access to as SOURCE
. So SOURCE
can be:
- a public docker image from Docker Hub
- a local docker image that you built
- a docker image that you built and pushed on Google Artifact Registry.
To run remotely on a cloud run job, the image must be available to Cloud Run (so cannot be local). It must be either public from Docker Hub or from Google Artifact Registry.
abs schedule-remote-run my_first_connection "0 * * * *"
โ ๏ธ THIS IS NOT IMPLEMENTED YET
$ abs --help
Usage: abs [OPTIONS] COMMAND [ARGS]...
Options:
--help Show this message and exit.
Commands:
create Create CONNECTION
list List created connections
list-available-streams List available streams of CONNECTION
remote-run Run CONNECTION Extract-Load Job from remote runner
run Run CONNECTION Extract-Load Job
run-env-vars Run Extract-Load Job configured by environment...
set-streams Set STREAMS to retrieve for CONNECTION (STREAMS...
Is it easy to migrate from/to Airbyte?
- AirbyteServerless uses Airbyte source connectors. Then, the same config is used. If it works on AirbyteServerless, it will work on Airbyte. The reverse may be sometimes a bit harder if for some sources you created credentials using oauth2 (with a pop-up window from the source opened by Airbyte UI). Indeed, Airbyte may not give you a way to read these created credentials.
- Airbyte jobs have two steps: extract-load of raw data and optional transform (transform can be replace, upsert, basic normalization). The extract-load of raw data is exactly the same but AirbyteServerless does not do transform. It only appends raw data at the destination. This is for purpose as AirbyteServerless was made to do only one thing and do it well and we believe it makes it resilient to schema changes. Then,
- if you create your transforms from raw data on dbt, you will be able to migrate from AirbyteServerless to Airbyte and vice-versa and still use your transforms.
- if you use Airbyte and rely on Airbyte transforms, you will need to re-create them in dbt if you switch to AirbyteServerless
- When migrating from/to Airbyte Cloud โ Airbyte OSS self-deployed โ AirbyteServerless, you won't be able to copy the state (which stores where incremental jobs stop). Then you will need to make a full refresh.
Why cannot we use usual Airbyte destination connectors?
Airbyte-Serverless destination connectors are indeed specific to AirbyteServerless and can NOT be the ones from Airbyte. This is because, in AirbyteServerless, destination connectors manage the states and logs while in Airbyte this is handled by the platform. Thanks to this, we don't need a database ๐ฅณ!
This being said, AirbyteServerless destination connectors are very light. You'll find here that the BigQuery destination connector is only 50 lines of code.
Join our Slack for any question, to get help for getting started, to speak about a bug, to suggest improvements, or simply if you want to have a chat ๐.
Any contribution is more than welcome ๐ค!
- Add a โญ on the repo to show your support
- Join our Slack and talk with us
- Raise an issue to raise a bug or suggest improvements
- Open a PR! Below are some suggestions of work to be done:
- implements a scheduler
- create a very light python Airbyte source / add a tutorial to use it in abs
- implement the
get_logs
method ofBigQueryDestination
- use the new BigQuery Storage Write API for bigquery destination
- enable updating cloud run job instead of deleting/creating when it already exists
- add a new destination connector (Cloud Storage?)
- add more remote runners such compute instances.
- implements vpc access
- implement optional post-processing (replace, upsert data at destination instead of append?)
- Big kudos to Airbyte for all the hard work on connectors!
- The generation of the sample connector configuration in yaml is heavily inspired from the code of
octavia
CLI developed by Airbyte.
For Tasks:
Click tags to check more tools for each tasksFor Jobs:
Alternative AI tools for airbyte_serverless
Similar Open Source Tools
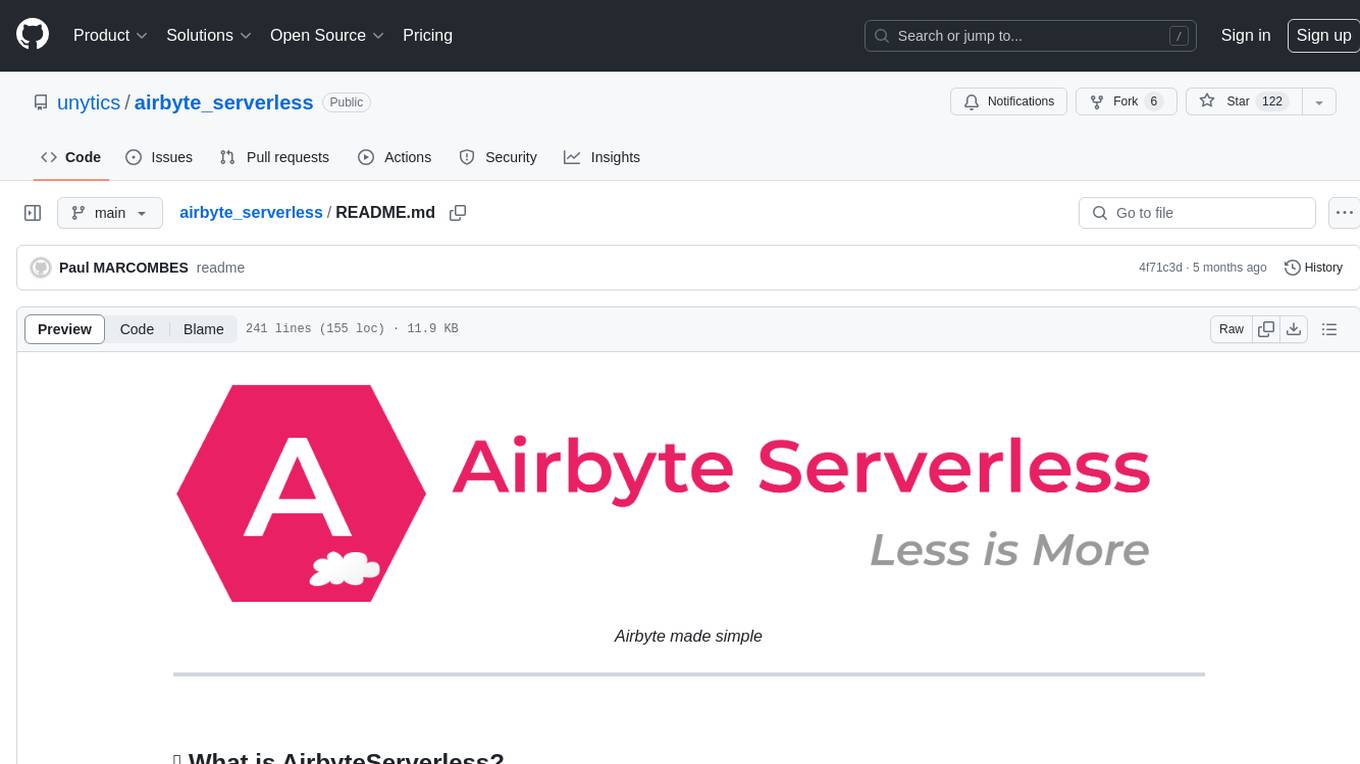
airbyte_serverless
AirbyteServerless is a lightweight tool designed to simplify the management of Airbyte connectors. It offers a serverless mode for running connectors, allowing users to easily move data from any source to their data warehouse. Unlike the full Airbyte-Open-Source-Platform, AirbyteServerless focuses solely on the Extract-Load process without a UI, database, or transform layer. It provides a CLI tool, 'abs', for managing connectors, creating connections, running jobs, selecting specific data streams, handling secrets securely, and scheduling remote runs. The tool is scalable, allowing independent deployment of multiple connectors. It aims to streamline the connector management process and provide a more agile alternative to the comprehensive Airbyte platform.
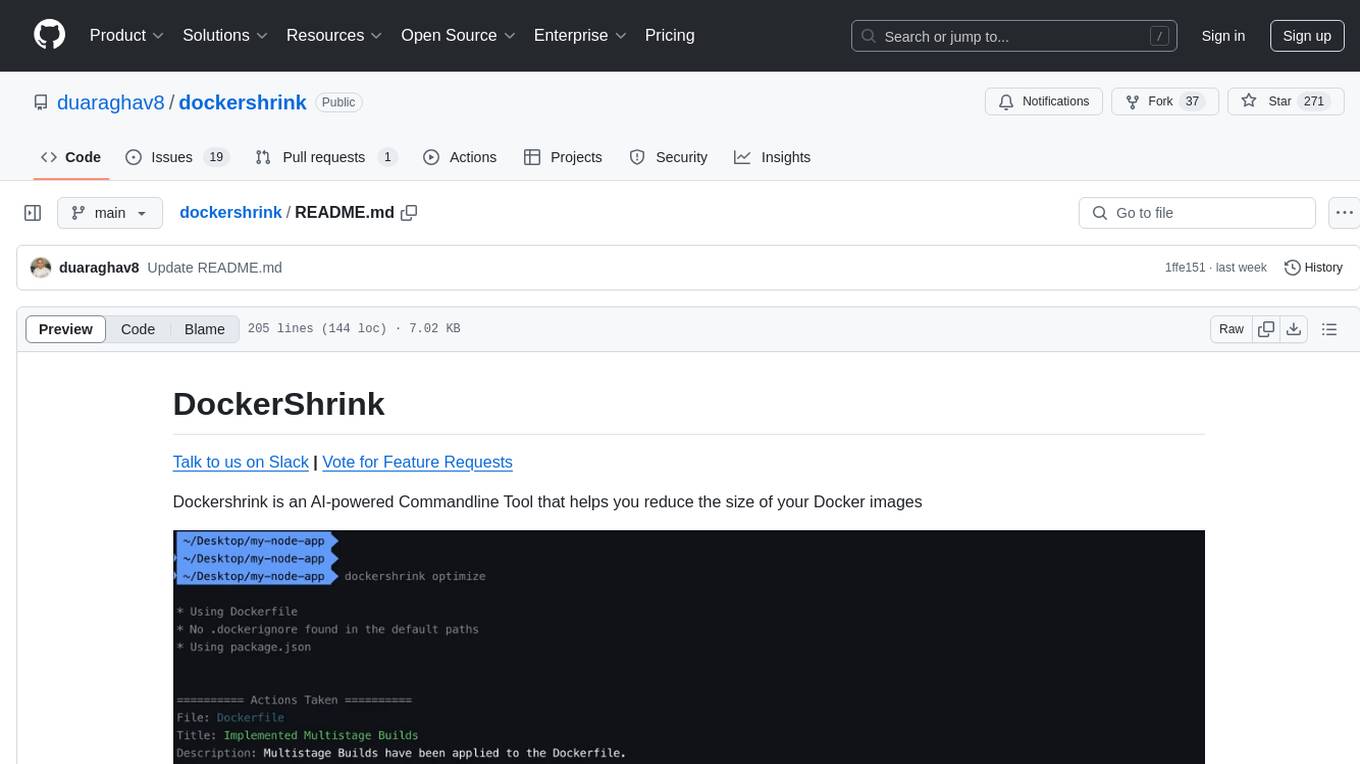
dockershrink
Dockershrink is an AI-powered Commandline Tool designed to help reduce the size of Docker images. It combines traditional Rule-based analysis with Generative AI techniques to optimize Image configurations. The tool supports NodeJS applications and aims to save costs on storage, data transfer, and build times while increasing developer productivity. By automatically applying advanced optimization techniques, Dockershrink simplifies the process for engineers and organizations, resulting in significant savings and efficiency improvements.
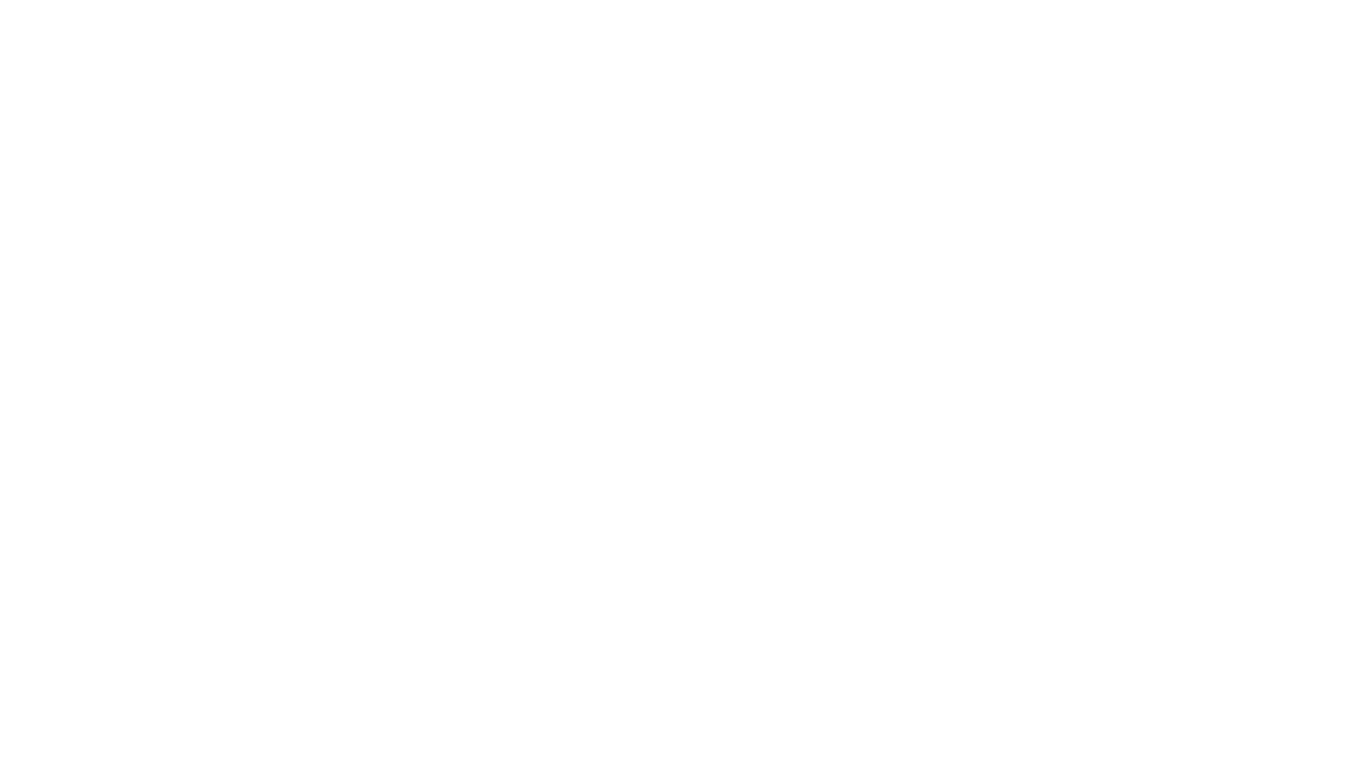
cognita
Cognita is an open-source framework to organize your RAG codebase along with a frontend to play around with different RAG customizations. It provides a simple way to organize your codebase so that it becomes easy to test it locally while also being able to deploy it in a production ready environment. The key issues that arise while productionizing RAG system from a Jupyter Notebook are: 1. **Chunking and Embedding Job** : The chunking and embedding code usually needs to be abstracted out and deployed as a job. Sometimes the job will need to run on a schedule or be trigerred via an event to keep the data updated. 2. **Query Service** : The code that generates the answer from the query needs to be wrapped up in a api server like FastAPI and should be deployed as a service. This service should be able to handle multiple queries at the same time and also autoscale with higher traffic. 3. **LLM / Embedding Model Deployment** : Often times, if we are using open-source models, we load the model in the Jupyter notebook. This will need to be hosted as a separate service in production and model will need to be called as an API. 4. **Vector DB deployment** : Most testing happens on vector DBs in memory or on disk. However, in production, the DBs need to be deployed in a more scalable and reliable way. Cognita makes it really easy to customize and experiment everything about a RAG system and still be able to deploy it in a good way. It also ships with a UI that makes it easier to try out different RAG configurations and see the results in real time. You can use it locally or with/without using any Truefoundry components. However, using Truefoundry components makes it easier to test different models and deploy the system in a scalable way. Cognita allows you to host multiple RAG systems using one app. ### Advantages of using Cognita are: 1. A central reusable repository of parsers, loaders, embedders and retrievers. 2. Ability for non-technical users to play with UI - Upload documents and perform QnA using modules built by the development team. 3. Fully API driven - which allows integration with other systems. > If you use Cognita with Truefoundry AI Gateway, you can get logging, metrics and feedback mechanism for your user queries. ### Features: 1. Support for multiple document retrievers that use `Similarity Search`, `Query Decompostion`, `Document Reranking`, etc 2. Support for SOTA OpenSource embeddings and reranking from `mixedbread-ai` 3. Support for using LLMs using `Ollama` 4. Support for incremental indexing that ingests entire documents in batches (reduces compute burden), keeps track of already indexed documents and prevents re-indexing of those docs.
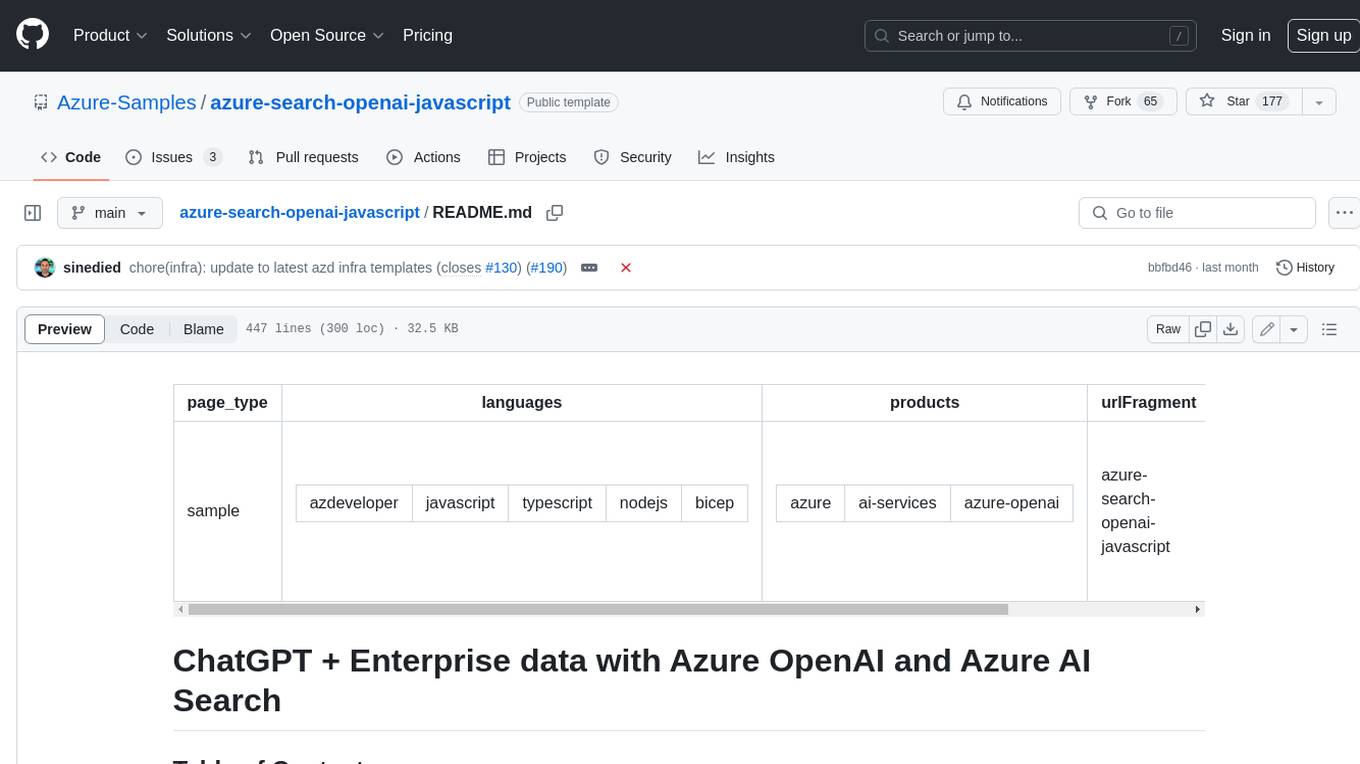
azure-search-openai-javascript
This sample demonstrates a few approaches for creating ChatGPT-like experiences over your own data using the Retrieval Augmented Generation pattern. It uses Azure OpenAI Service to access the ChatGPT model (gpt-35-turbo), and Azure AI Search for data indexing and retrieval.
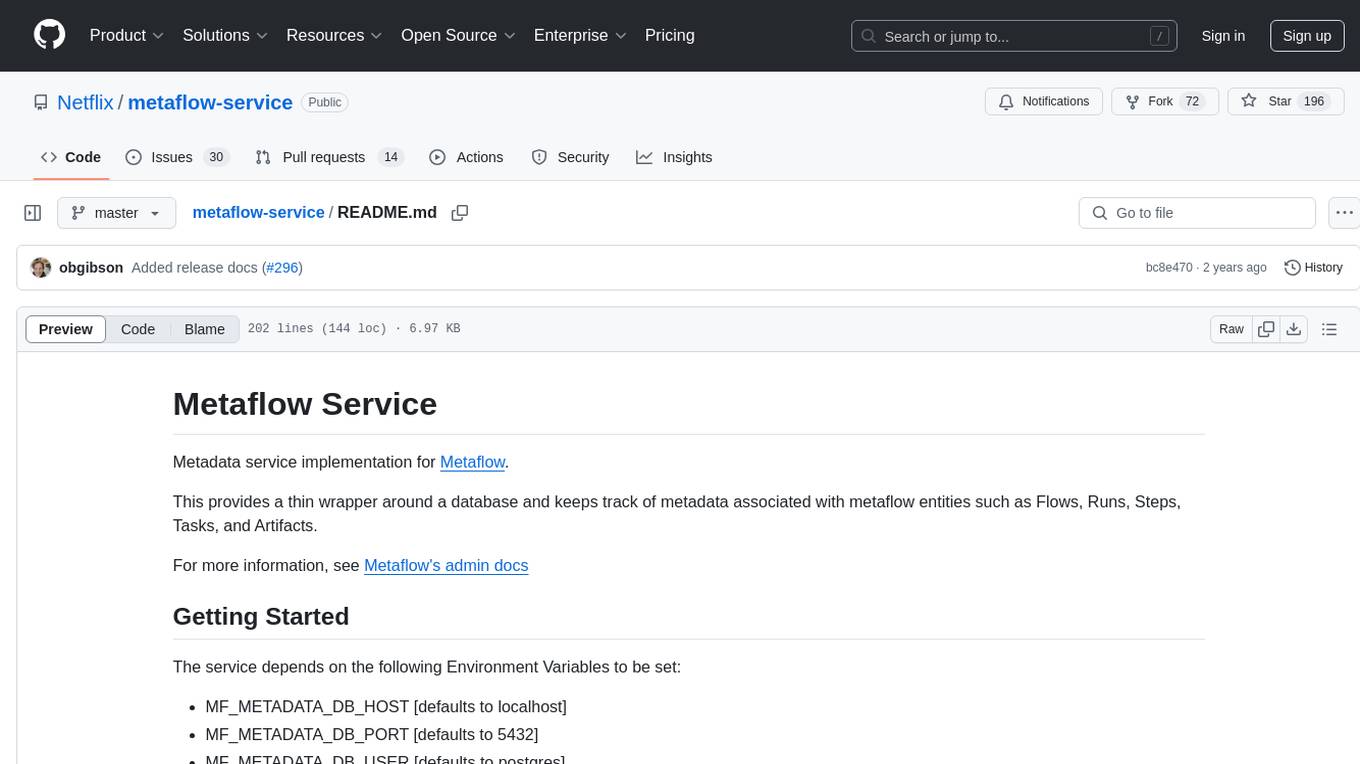
metaflow-service
Metaflow Service is a metadata service implementation for Metaflow, providing a thin wrapper around a database to keep track of metadata associated with Flows, Runs, Steps, Tasks, and Artifacts. It includes features for managing DB migrations, launching compatible versions of the metadata service, and executing flows locally. The service can be run using Docker or as a standalone service, with options for testing and running unit/integration tests. Users can interact with the service via API endpoints or utility CLI tools.
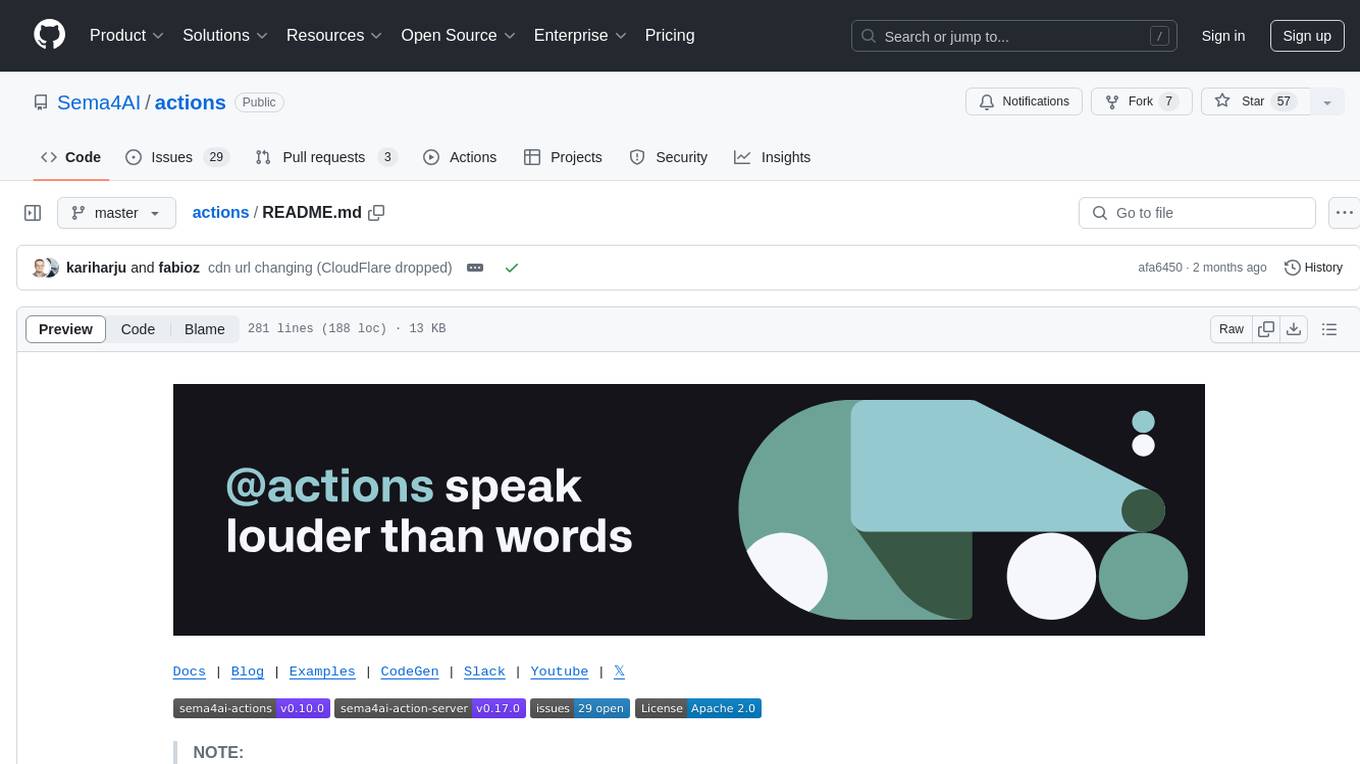
actions
Sema4.ai Action Server is a tool that allows users to build semantic actions in Python to connect AI agents with real-world applications. It enables users to create custom actions, skills, loaders, and plugins that securely connect any AI Assistant platform to data and applications. The tool automatically creates and exposes an API based on function declaration, type hints, and docstrings by adding '@action' to Python scripts. It provides an end-to-end stack supporting various connections between AI and user's apps and data, offering ease of use, security, and scalability.
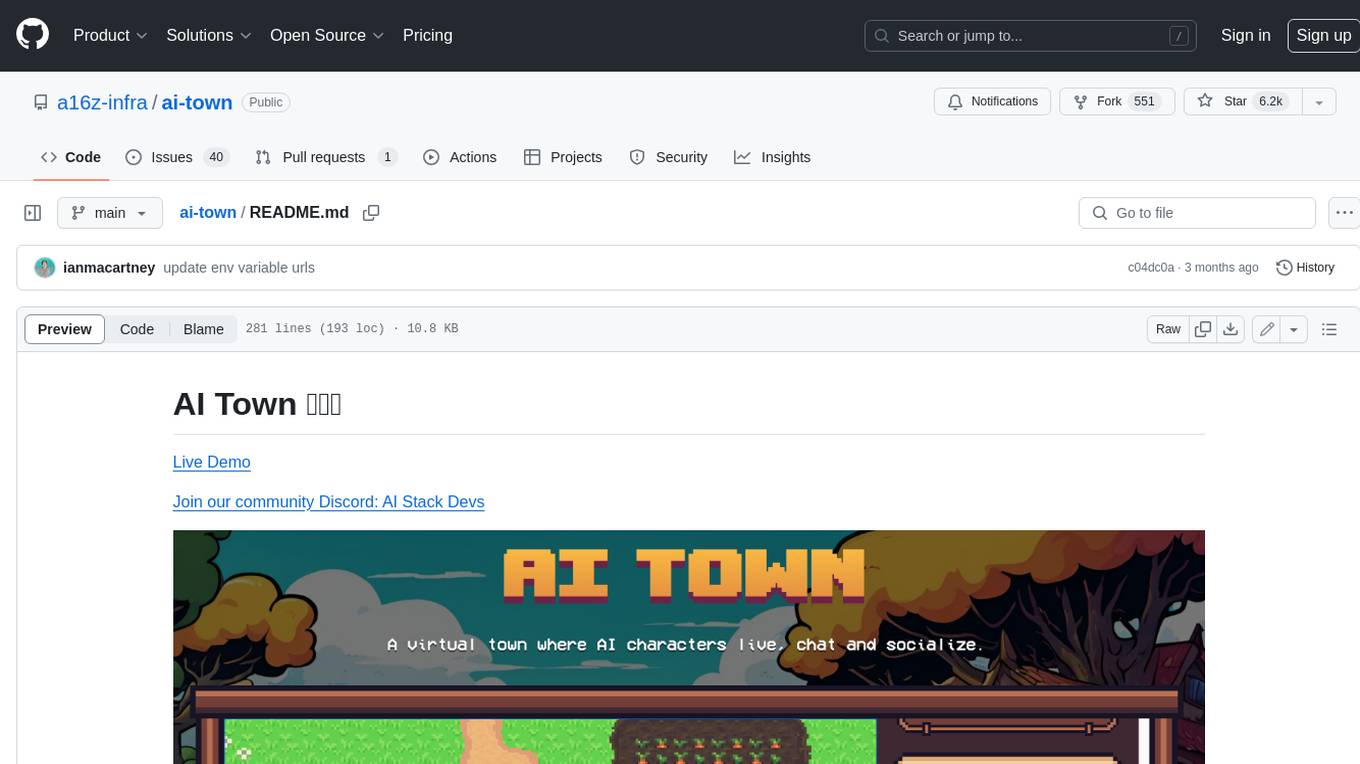
ai-town
AI Town is a virtual town where AI characters live, chat, and socialize. This project provides a deployable starter kit for building and customizing your own version of AI Town. It features a game engine, database, vector search, auth, text model, deployment, pixel art generation, background music generation, and local inference. You can customize your own simulation by creating characters and stories, updating spritesheets, changing the background, and modifying the background music.
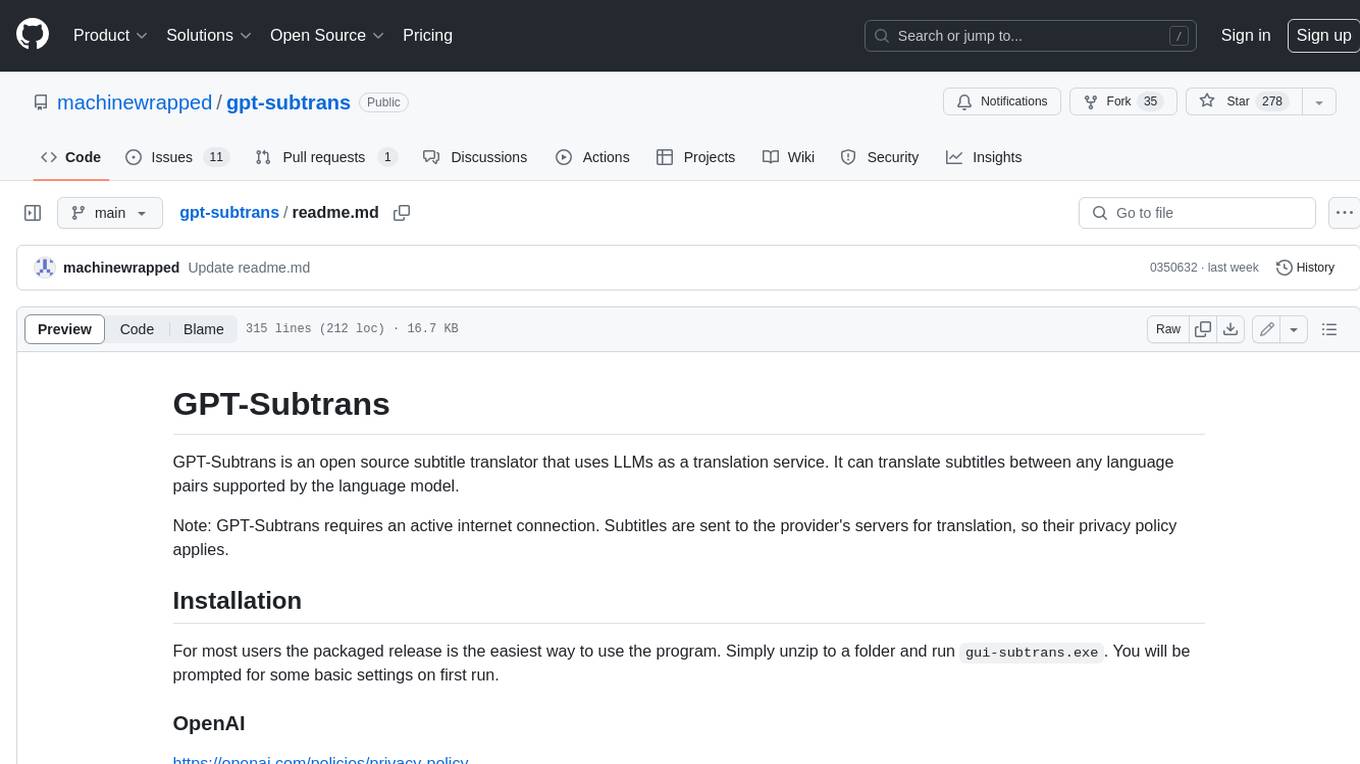
gpt-subtrans
GPT-Subtrans is an open-source subtitle translator that utilizes large language models (LLMs) as translation services. It supports translation between any language pairs that the language model supports. Note that GPT-Subtrans requires an active internet connection, as subtitles are sent to the provider's servers for translation, and their privacy policy applies.
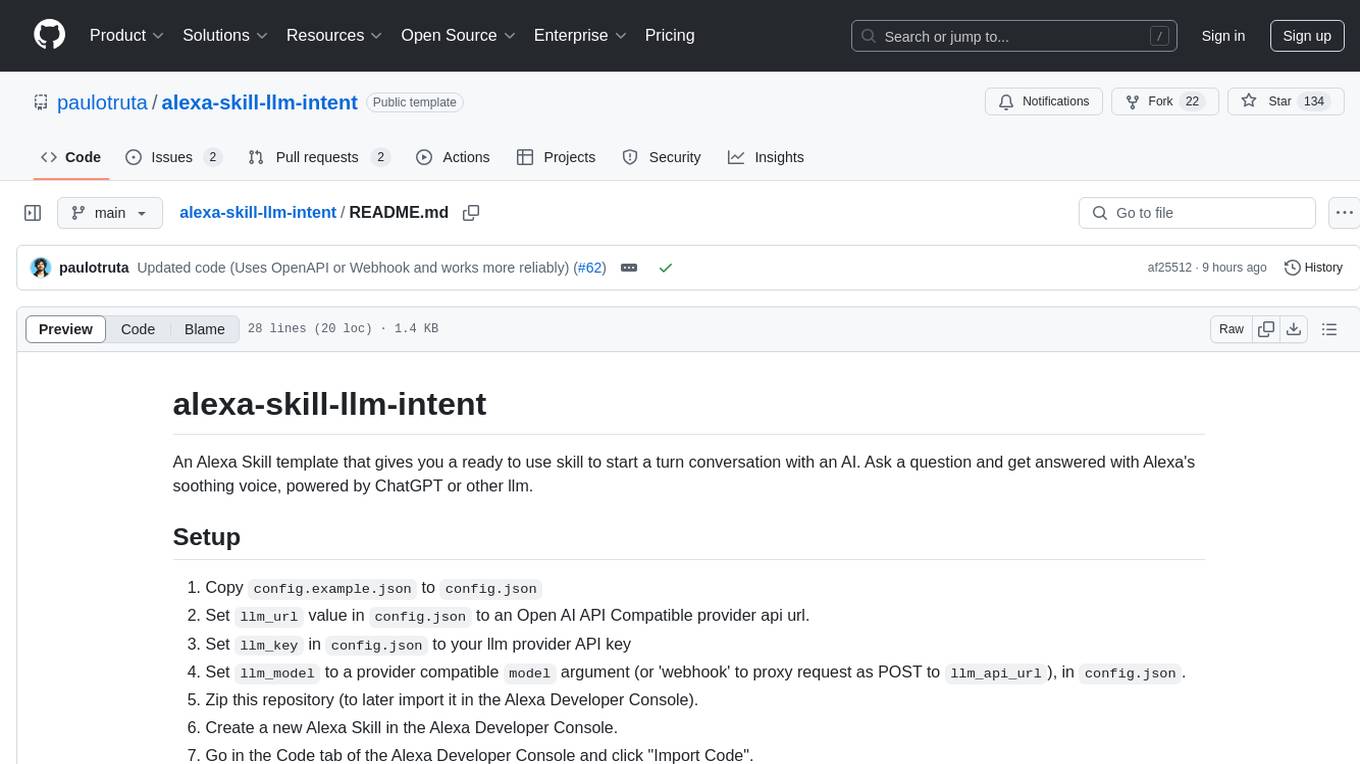
alexa-skill-llm-intent
An Alexa Skill template that provides a ready-to-use skill for starting a conversation with an AI. Users can ask questions and receive answers in Alexa's voice, powered by ChatGPT or other llm. The template includes setup instructions for configuring the AI provider API and model, as well as usage commands for interacting with the skill. It serves as a starting point for creating custom Alexa Skills and should be used at the user's own risk.
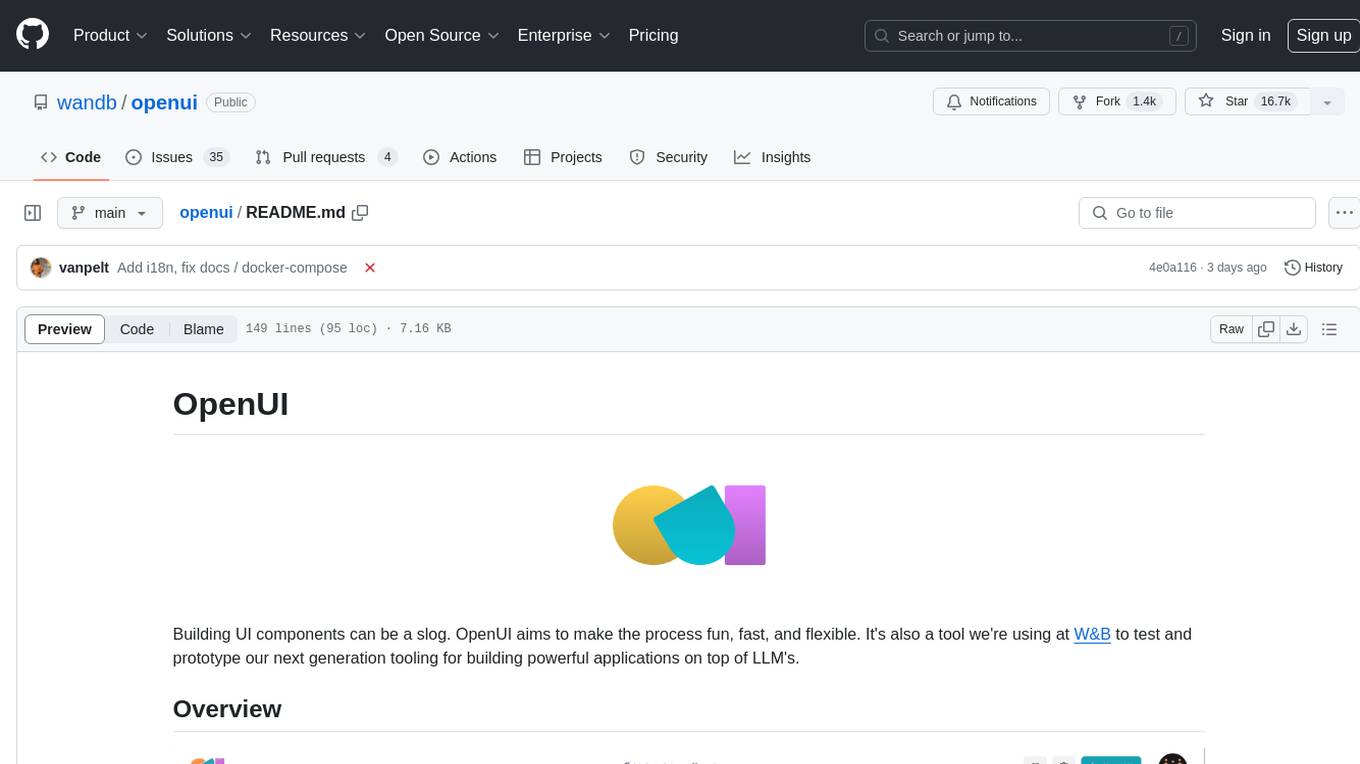
openui
OpenUI is a tool designed to simplify the process of building UI components by allowing users to describe UI using their imagination and see it rendered live. It supports converting HTML to React, Svelte, Web Components, etc. The tool is open source and aims to make UI development fun, fast, and flexible. It integrates with various AI services like OpenAI, Groq, Gemini, Anthropic, Cohere, and Mistral, providing users with the flexibility to use different models. OpenUI also supports LiteLLM for connecting to various LLM services and allows users to create custom proxy configs. The tool can be run locally using Docker or Python, and it offers a development environment for quick setup and testing.
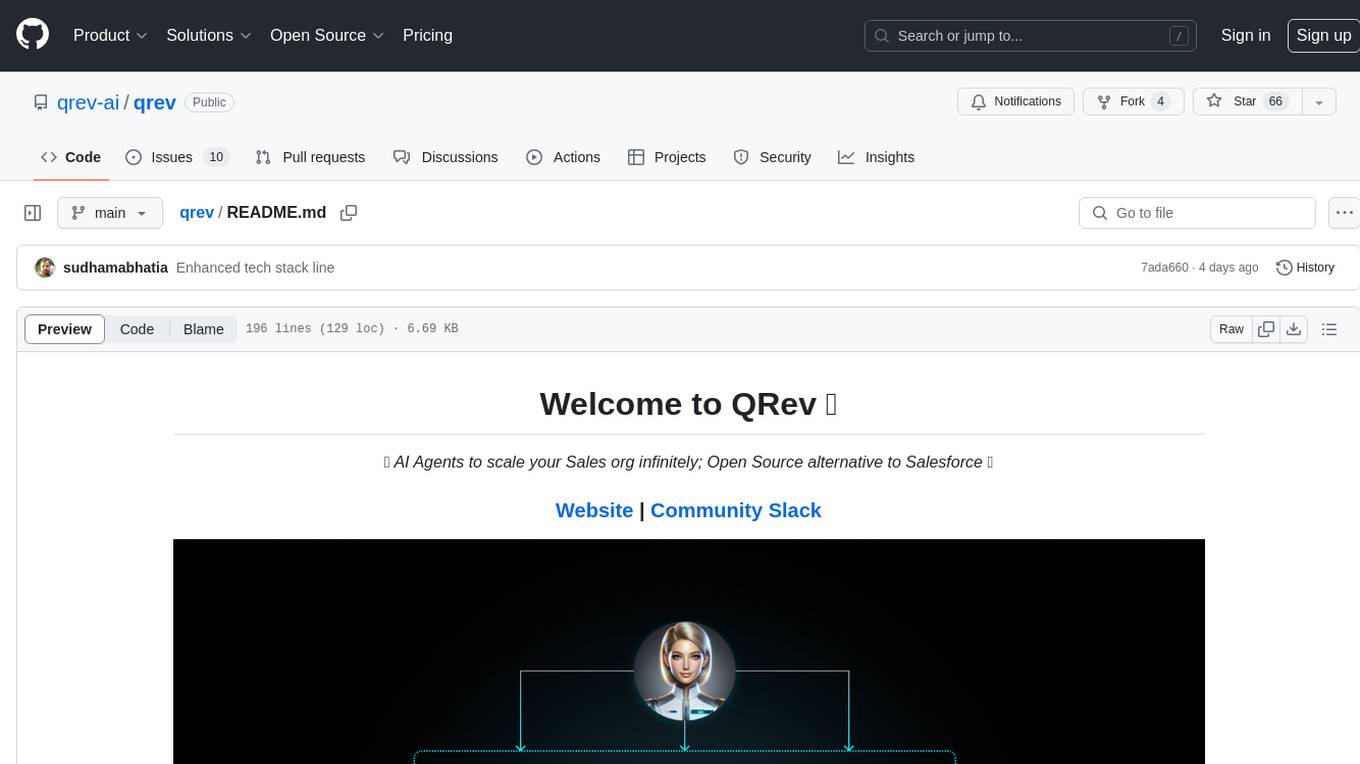
qrev
QRev is an open-source alternative to Salesforce, offering AI agents to scale sales organizations infinitely. It aims to provide digital workers for various sales roles or a superagent named Qai. The tech stack includes TypeScript for frontend, NodeJS for backend, MongoDB for app server database, ChromaDB for vector database, SQLite for AI server SQL relational database, and Langchain for LLM tooling. The tool allows users to run client app, app server, and AI server components. It requires Node.js and MongoDB to be installed, and provides detailed setup instructions in the README file.
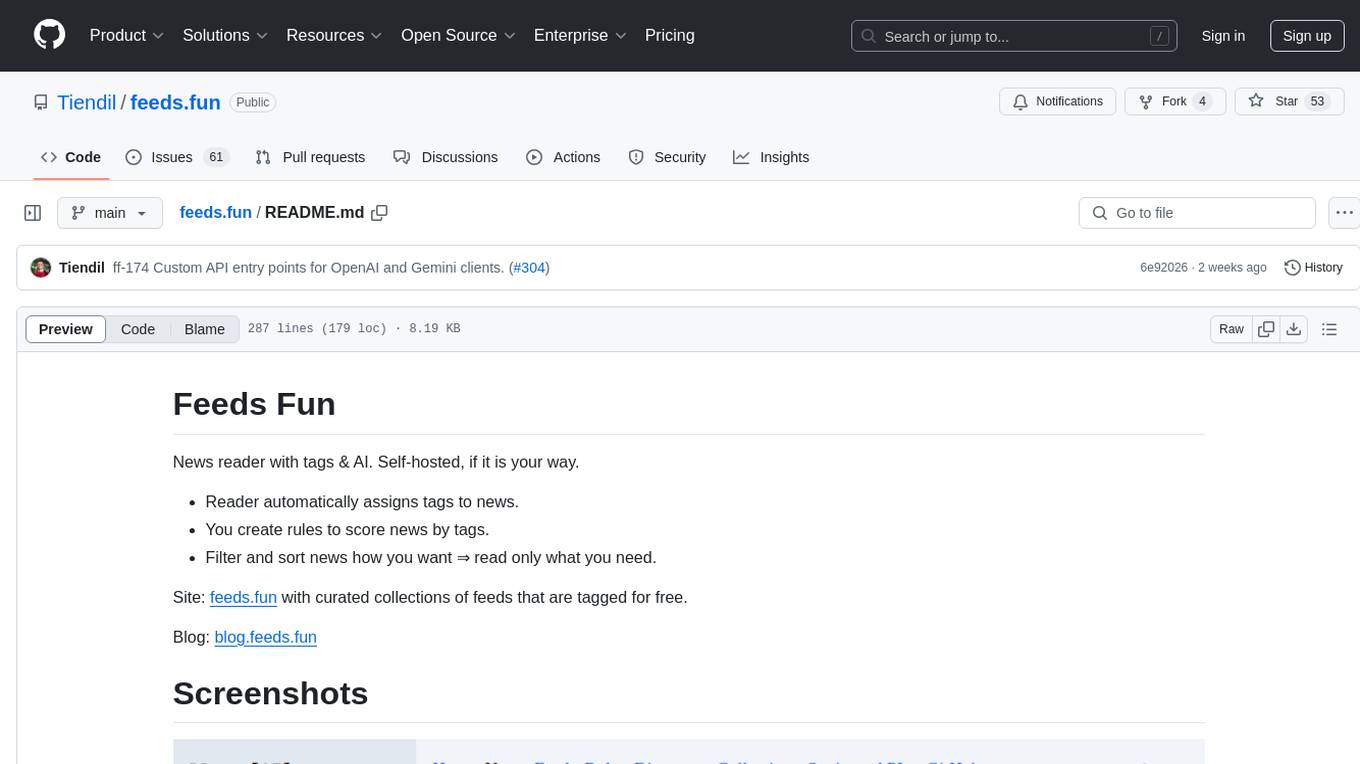
feeds.fun
Feeds Fun is a self-hosted news reader tool that automatically assigns tags to news entries. Users can create rules to score news based on tags, filter and sort news as needed, and track read news. The tool offers multi/single-user support, feeds management, and various features for personalized news consumption. Users can access the tool's backend as the ffun package on PyPI and the frontend as the feeds-fun package on NPM. Feeds Fun requires setting up OpenAI or Gemini API keys for full tag generation capabilities. The tool uses tag processors to detect tags for news entries, with options for simple and complex processors. Feeds Fun primarily relies on LLM tag processors from OpenAI and Google for tag generation.
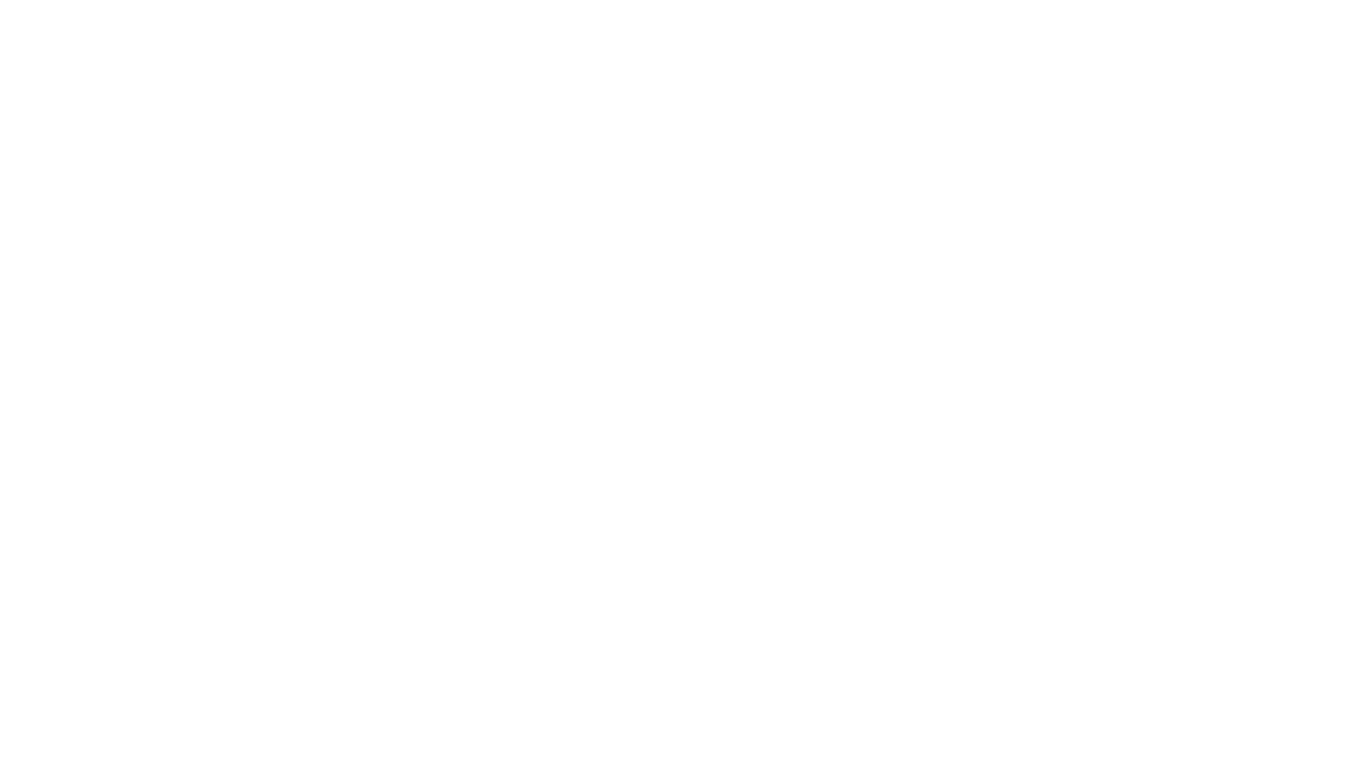
aider-composer
Aider Composer is a VSCode extension that integrates Aider into your development workflow. It allows users to easily add and remove files, toggle between read-only and editable modes, review code changes, use different chat modes, and reference files in the chat. The extension supports multiple models, code generation, code snippets, and settings customization. It has limitations such as lack of support for multiple workspaces, Git repository features, linting, testing, voice features, in-chat commands, and configuration options.
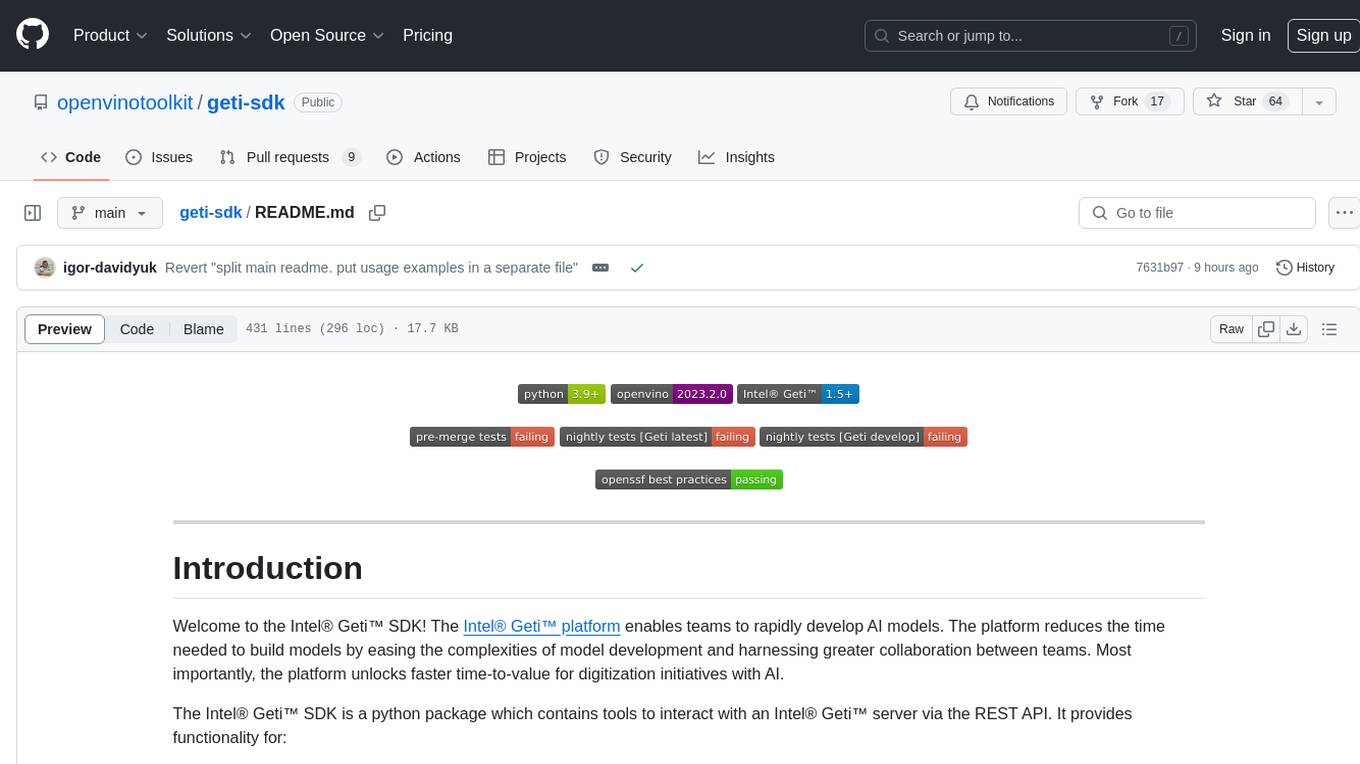
geti-sdk
The Intelยฎ Getiโข SDK is a python package that enables teams to rapidly develop AI models by easing the complexities of model development and enhancing collaboration between teams. It provides tools to interact with an Intelยฎ Getiโข server via the REST API, allowing for project creation, downloading, uploading, deploying for local inference with OpenVINO, setting project and model configuration, launching and monitoring training jobs, and media upload and prediction. The SDK also includes tutorial-style Jupyter notebooks demonstrating its usage.
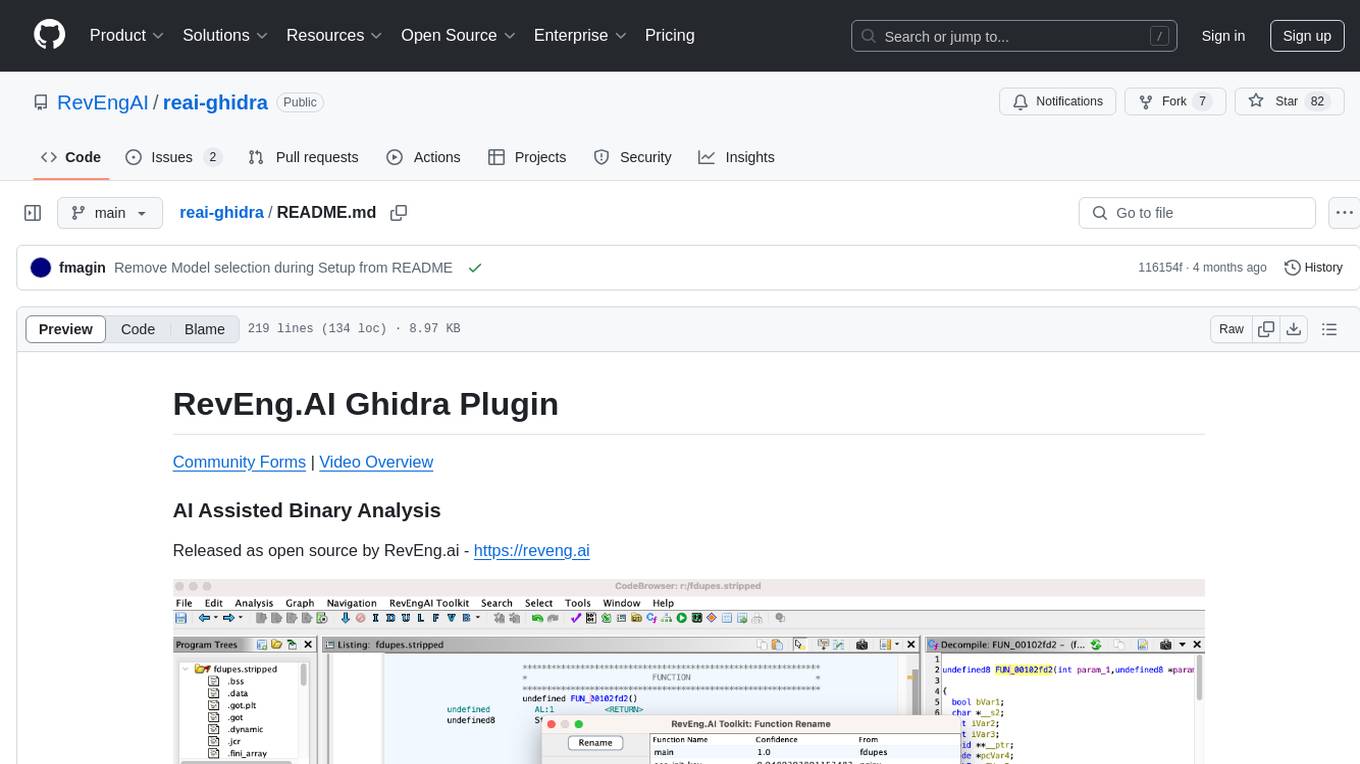
reai-ghidra
The RevEng.AI Ghidra Plugin by RevEng.ai allows users to interact with their API within Ghidra for Binary Code Similarity analysis to aid in Reverse Engineering stripped binaries. Users can upload binaries, rename functions above a confidence threshold, and view similar functions for a selected function.
For similar tasks
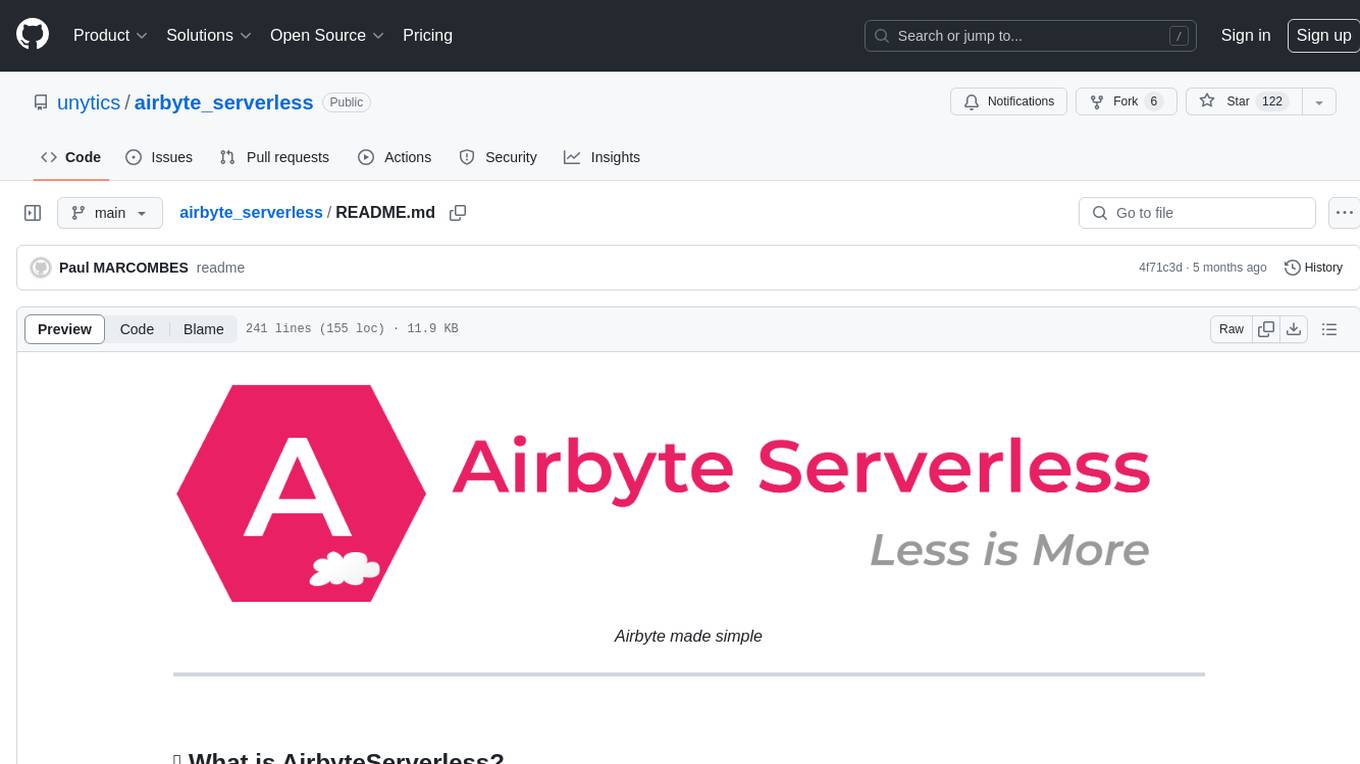
airbyte_serverless
AirbyteServerless is a lightweight tool designed to simplify the management of Airbyte connectors. It offers a serverless mode for running connectors, allowing users to easily move data from any source to their data warehouse. Unlike the full Airbyte-Open-Source-Platform, AirbyteServerless focuses solely on the Extract-Load process without a UI, database, or transform layer. It provides a CLI tool, 'abs', for managing connectors, creating connections, running jobs, selecting specific data streams, handling secrets securely, and scheduling remote runs. The tool is scalable, allowing independent deployment of multiple connectors. It aims to streamline the connector management process and provide a more agile alternative to the comprehensive Airbyte platform.
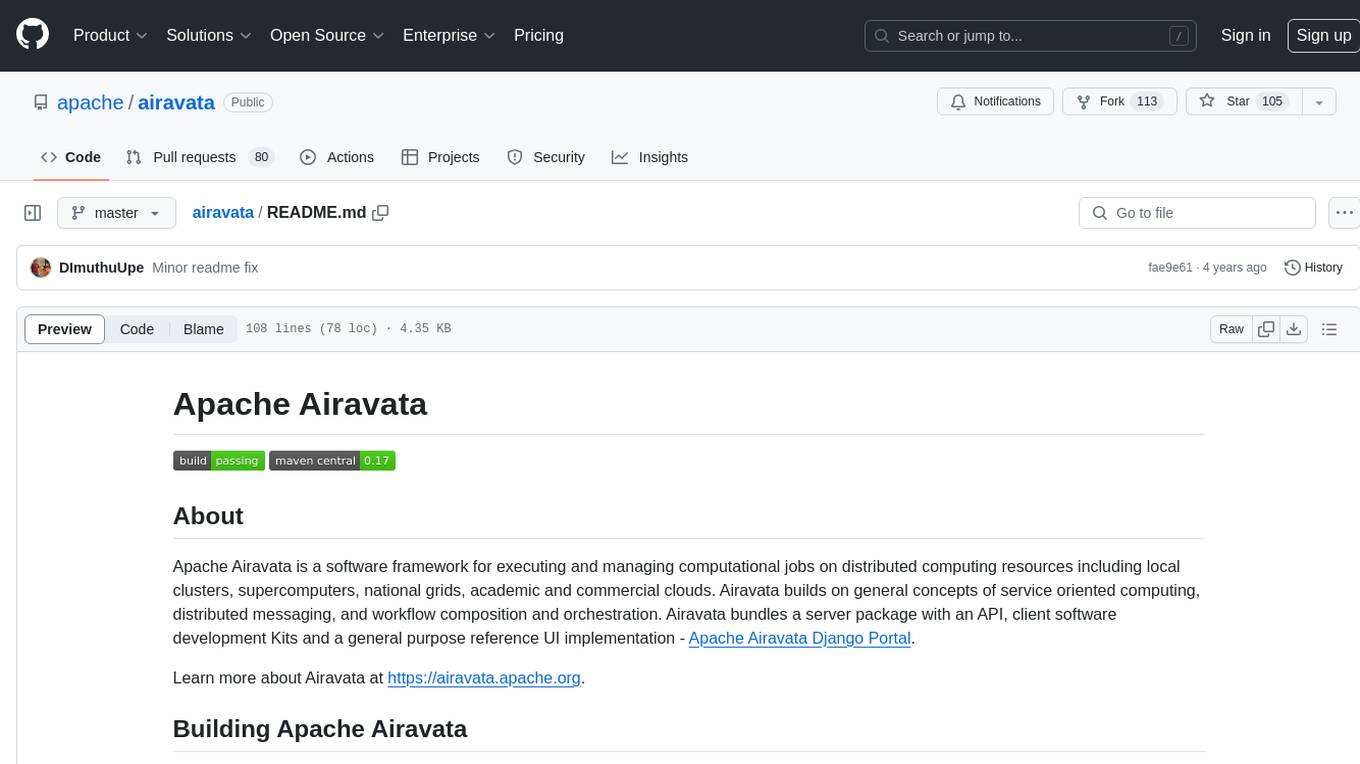
airavata
Apache Airavata is a software framework for executing and managing computational jobs on distributed computing resources. It supports local clusters, supercomputers, national grids, academic and commercial clouds. Airavata utilizes service-oriented computing, distributed messaging, and workflow composition. It includes a server package with an API, client SDKs, and a general-purpose UI implementation called Apache Airavata Django Portal.
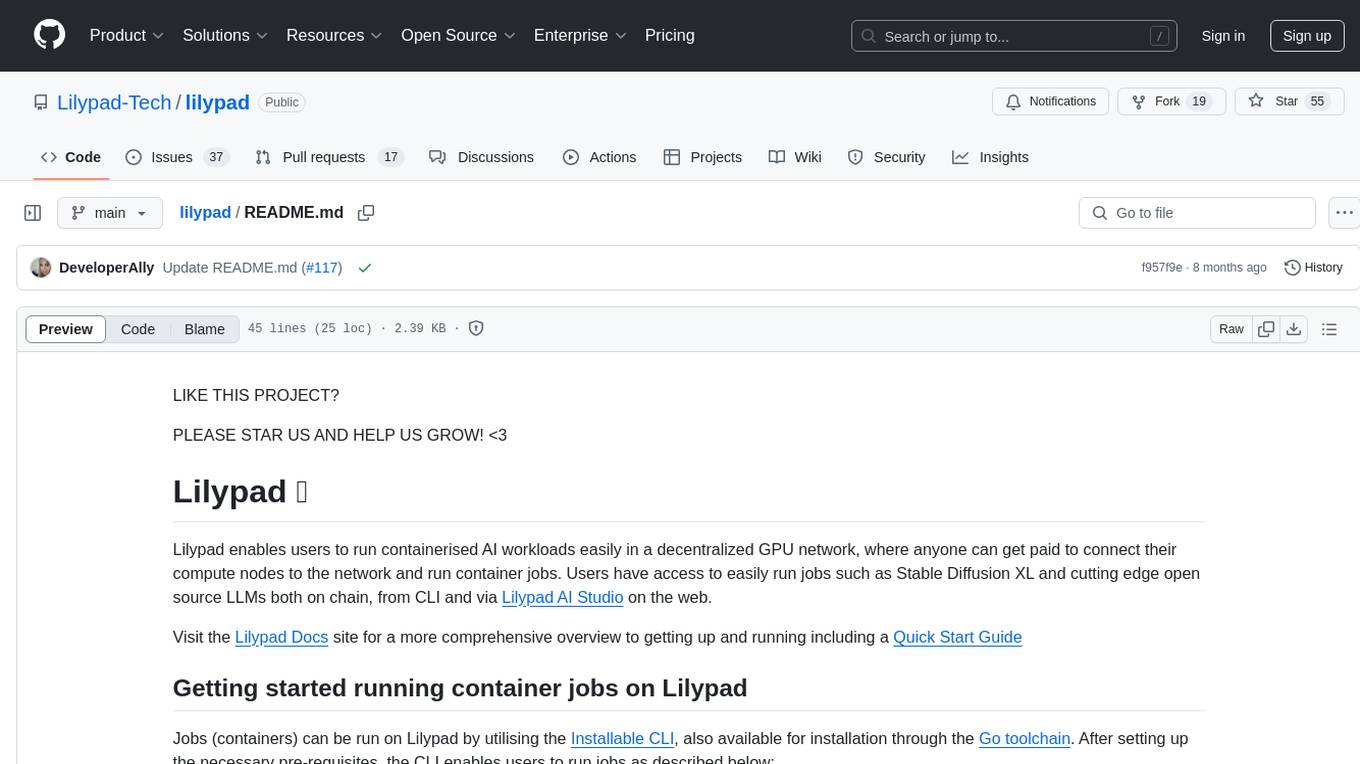
lilypad
Lilypad enables users to run containerised AI workloads easily in a decentralized GPU network. Users can get paid to connect their compute nodes to the network and run container jobs. It provides access to run jobs such as Stable Diffusion XL and cutting edge open source LLMs both on chain, from CLI, and via Lilypad AI Studio on the web.
For similar jobs
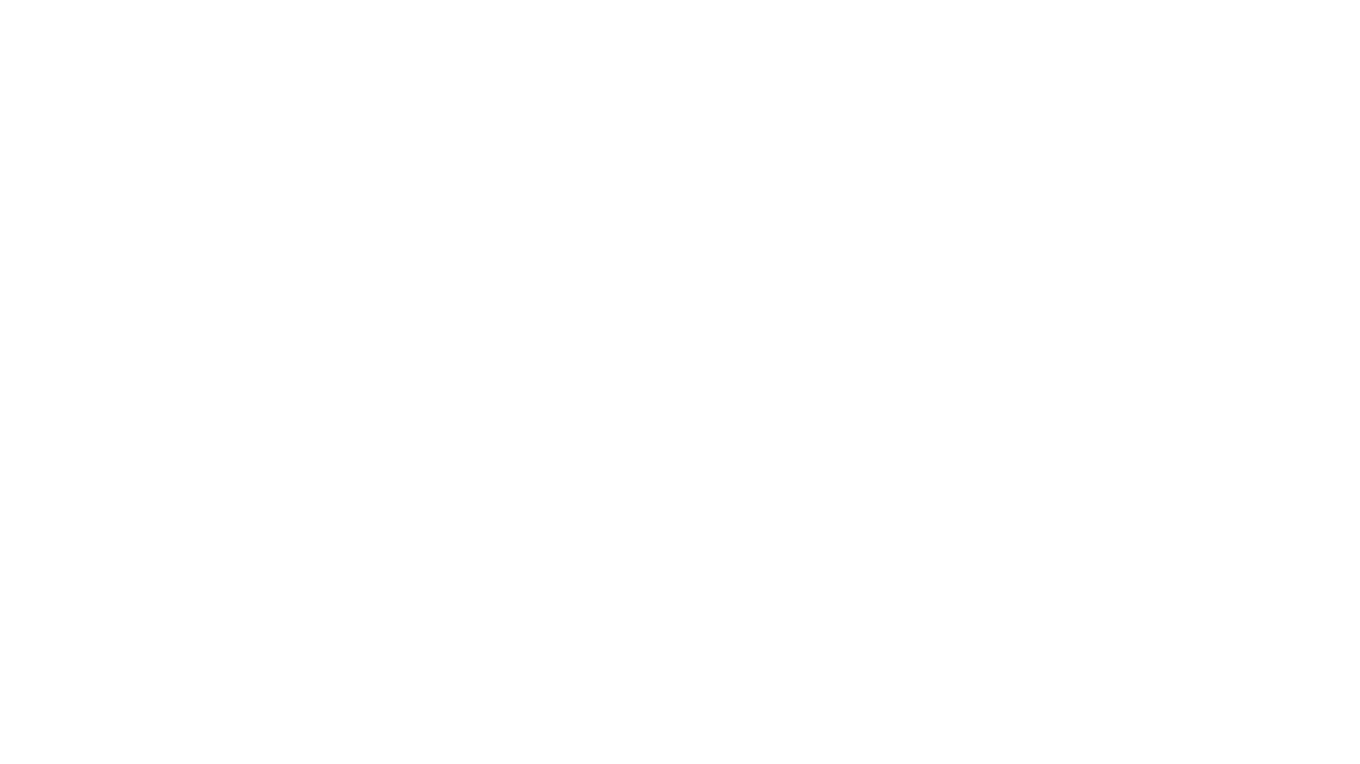
AirGo
AirGo is a front and rear end separation, multi user, multi protocol proxy service management system, simple and easy to use. It supports vless, vmess, shadowsocks, and hysteria2.
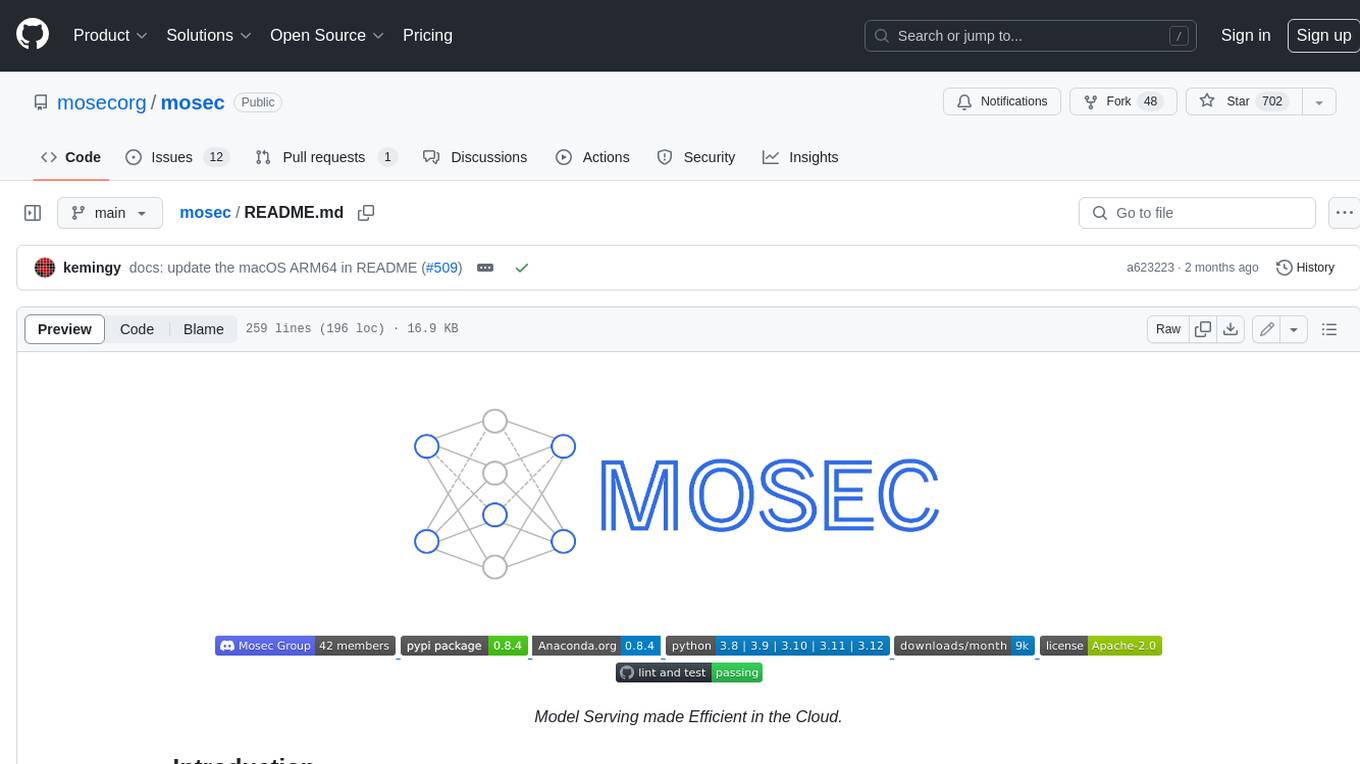
mosec
Mosec is a high-performance and flexible model serving framework for building ML model-enabled backend and microservices. It bridges the gap between any machine learning models you just trained and the efficient online service API. * **Highly performant** : web layer and task coordination built with Rust ๐ฆ, which offers blazing speed in addition to efficient CPU utilization powered by async I/O * **Ease of use** : user interface purely in Python ๐, by which users can serve their models in an ML framework-agnostic manner using the same code as they do for offline testing * **Dynamic batching** : aggregate requests from different users for batched inference and distribute results back * **Pipelined stages** : spawn multiple processes for pipelined stages to handle CPU/GPU/IO mixed workloads * **Cloud friendly** : designed to run in the cloud, with the model warmup, graceful shutdown, and Prometheus monitoring metrics, easily managed by Kubernetes or any container orchestration systems * **Do one thing well** : focus on the online serving part, users can pay attention to the model optimization and business logic
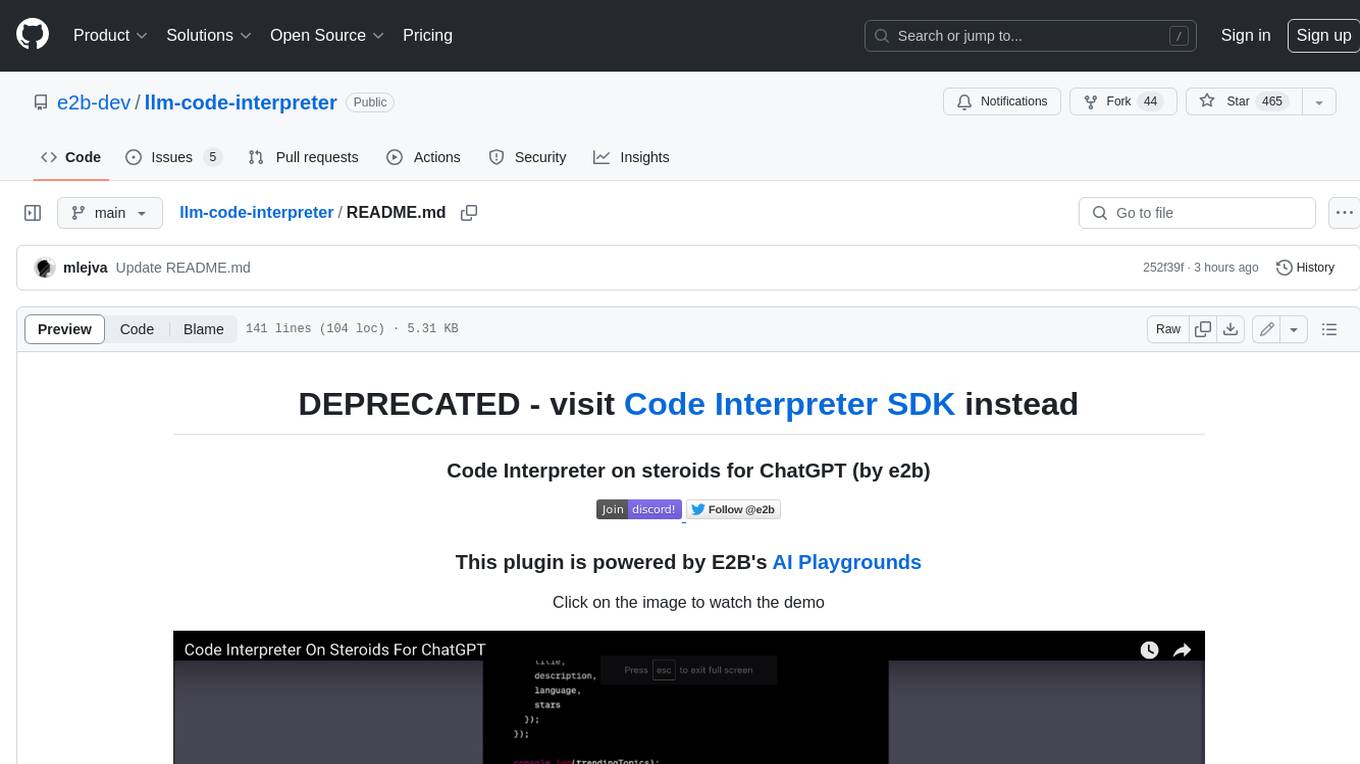
llm-code-interpreter
The 'llm-code-interpreter' repository is a deprecated plugin that provides a code interpreter on steroids for ChatGPT by E2B. It gives ChatGPT access to a sandboxed cloud environment with capabilities like running any code, accessing Linux OS, installing programs, using filesystem, running processes, and accessing the internet. The plugin exposes commands to run shell commands, read files, and write files, enabling various possibilities such as running different languages, installing programs, starting servers, deploying websites, and more. It is powered by the E2B API and is designed for agents to freely experiment within a sandboxed environment.
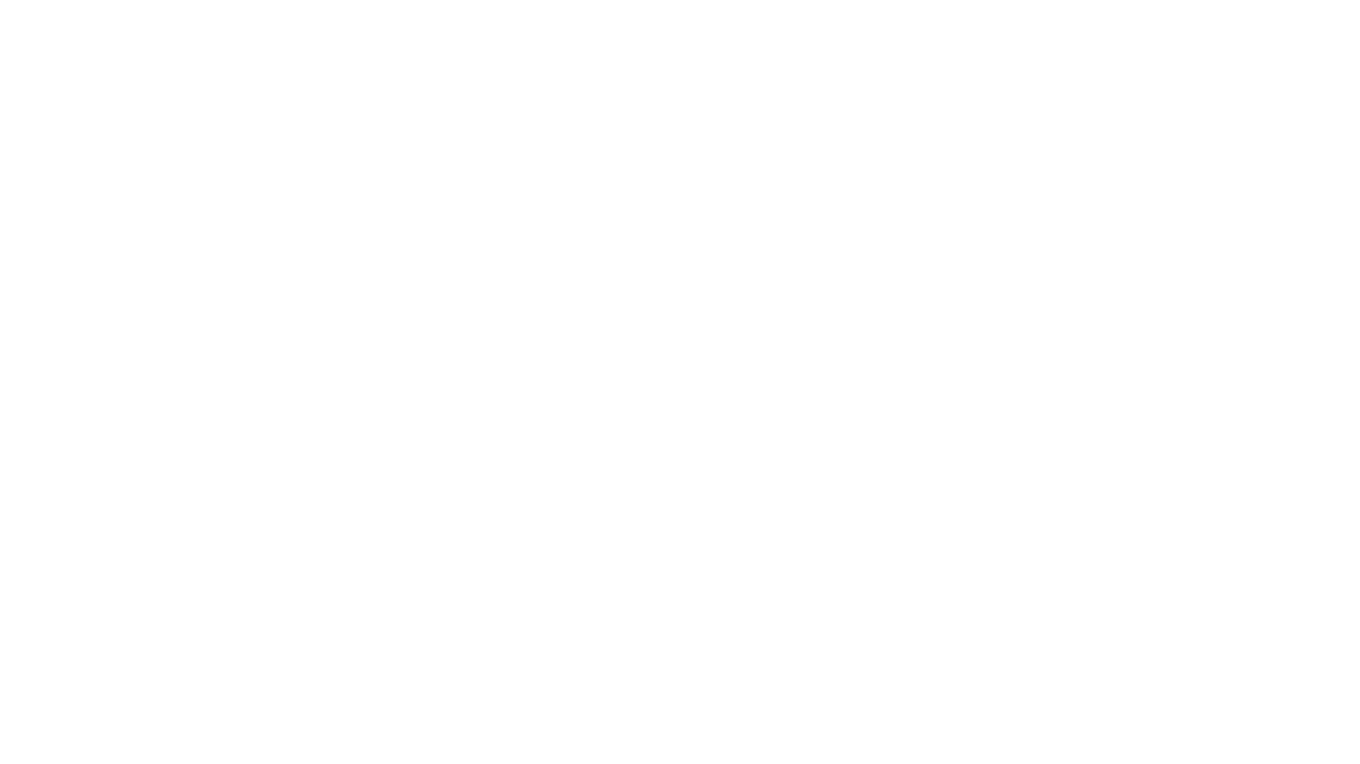
pezzo
Pezzo is a fully cloud-native and open-source LLMOps platform that allows users to observe and monitor AI operations, troubleshoot issues, save costs and latency, collaborate, manage prompts, and deliver AI changes instantly. It supports various clients for prompt management, observability, and caching. Users can run the full Pezzo stack locally using Docker Compose, with prerequisites including Node.js 18+, Docker, and a GraphQL Language Feature Support VSCode Extension. Contributions are welcome, and the source code is available under the Apache 2.0 License.
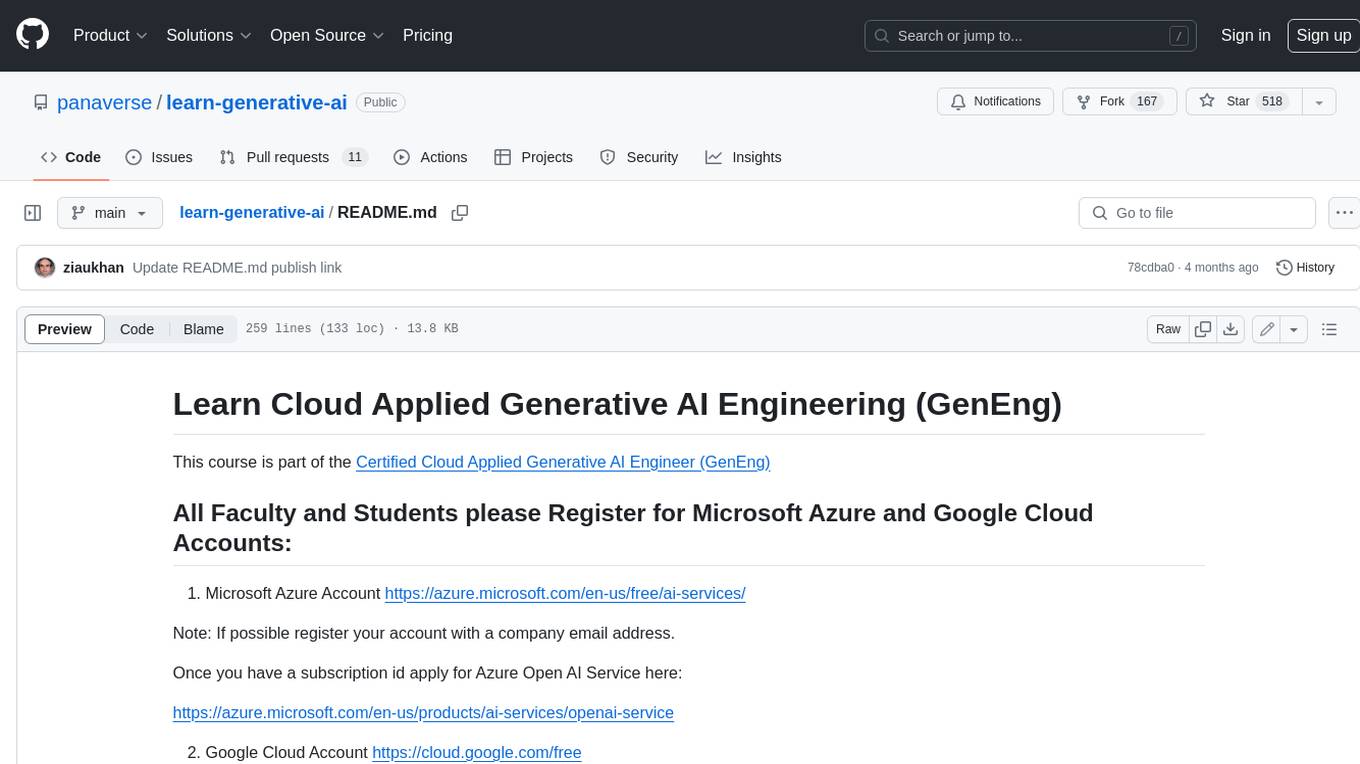
learn-generative-ai
Learn Cloud Applied Generative AI Engineering (GenEng) is a course focusing on the application of generative AI technologies in various industries. The course covers topics such as the economic impact of generative AI, the role of developers in adopting and integrating generative AI technologies, and the future trends in generative AI. Students will learn about tools like OpenAI API, LangChain, and Pinecone, and how to build and deploy Large Language Models (LLMs) for different applications. The course also explores the convergence of generative AI with Web 3.0 and its potential implications for decentralized intelligence.
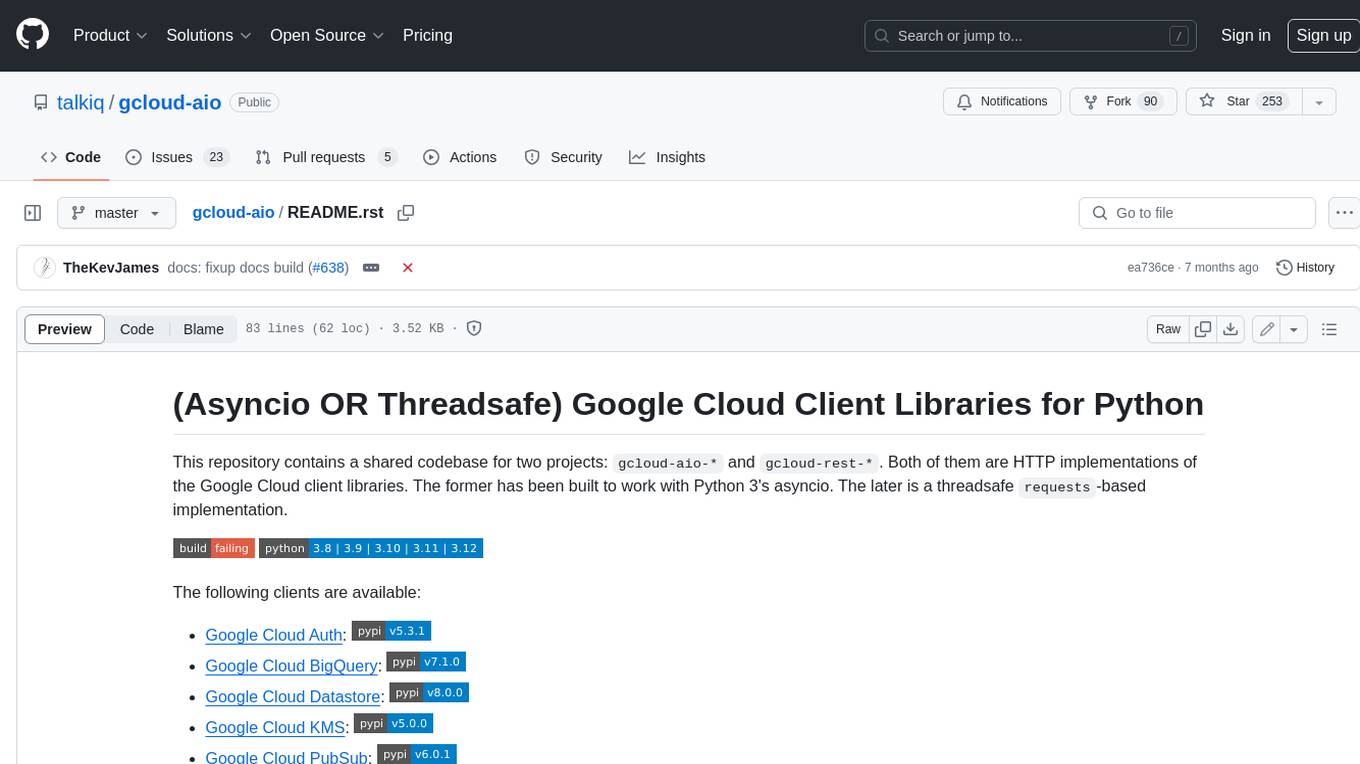
gcloud-aio
This repository contains shared codebase for two projects: gcloud-aio and gcloud-rest. gcloud-aio is built for Python 3's asyncio, while gcloud-rest is a threadsafe requests-based implementation. It provides clients for Google Cloud services like Auth, BigQuery, Datastore, KMS, PubSub, Storage, and Task Queue. Users can install the library using pip and refer to the documentation for usage details. Developers can contribute to the project by following the contribution guide.
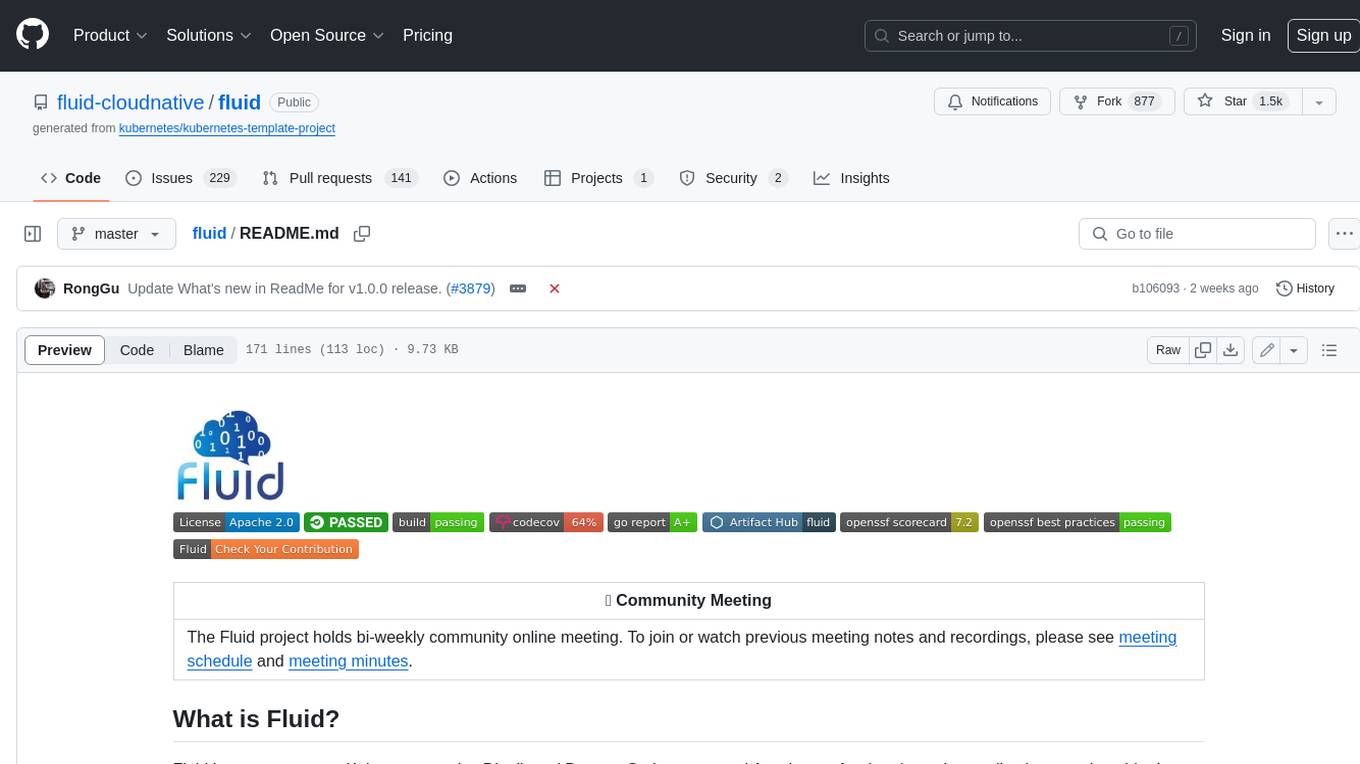
fluid
Fluid is an open source Kubernetes-native Distributed Dataset Orchestrator and Accelerator for data-intensive applications, such as big data and AI applications. It implements dataset abstraction, scalable cache runtime, automated data operations, elasticity and scheduling, and is runtime platform agnostic. Key concepts include Dataset and Runtime. Prerequisites include Kubernetes version > 1.16, Golang 1.18+, and Helm 3. The tool offers features like accelerating remote file accessing, machine learning, accelerating PVC, preloading dataset, and on-the-fly dataset cache scaling. Contributions are welcomed, and the project is under the Apache 2.0 license with a vendor-neutral approach.
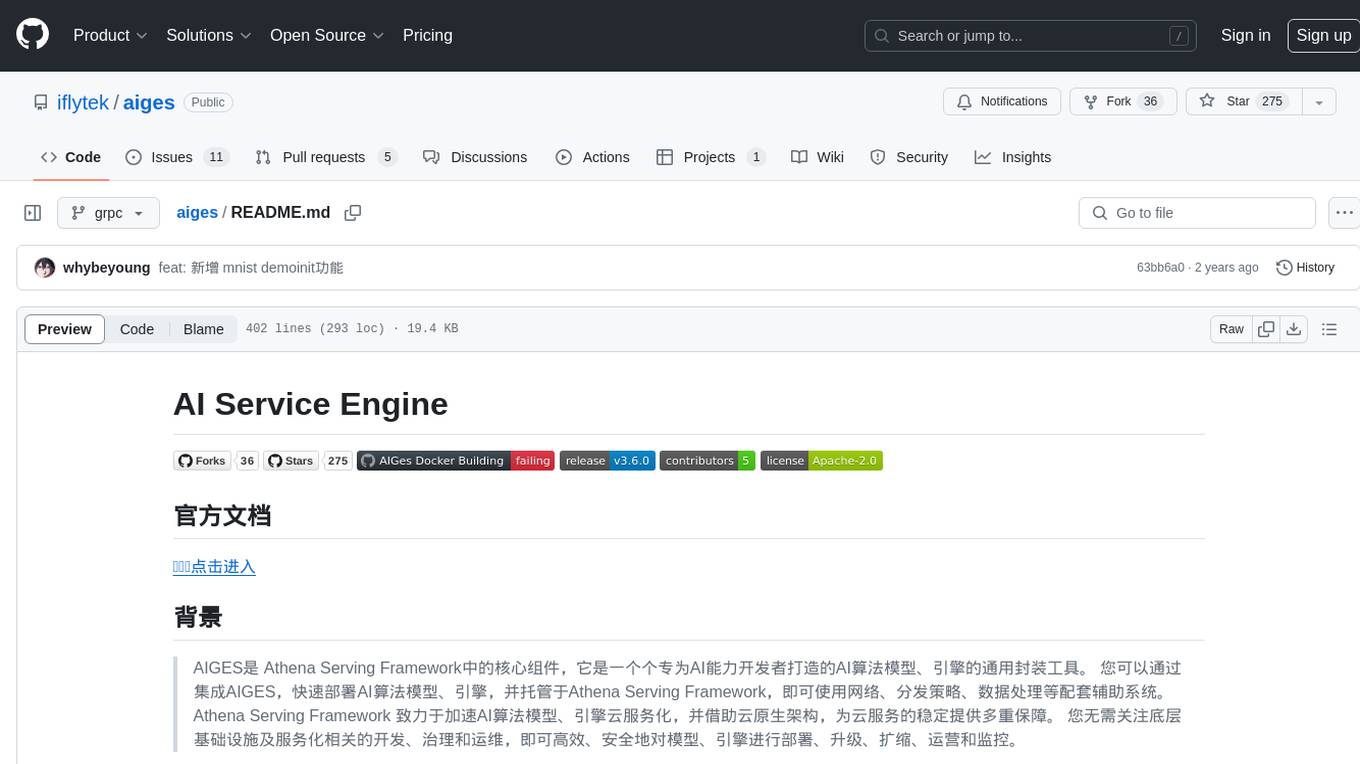
aiges
AIGES is a core component of the Athena Serving Framework, designed as a universal encapsulation tool for AI developers to deploy AI algorithm models and engines quickly. By integrating AIGES, you can deploy AI algorithm models and engines rapidly and host them on the Athena Serving Framework, utilizing supporting auxiliary systems for networking, distribution strategies, data processing, etc. The Athena Serving Framework aims to accelerate the cloud service of AI algorithm models and engines, providing multiple guarantees for cloud service stability through cloud-native architecture. You can efficiently and securely deploy, upgrade, scale, operate, and monitor models and engines without focusing on underlying infrastructure and service-related development, governance, and operations.