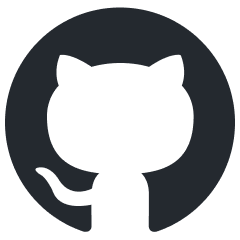
mosec
A high-performance ML model serving framework, offers dynamic batching and CPU/GPU pipelines to fully exploit your compute machine
Stars: 744
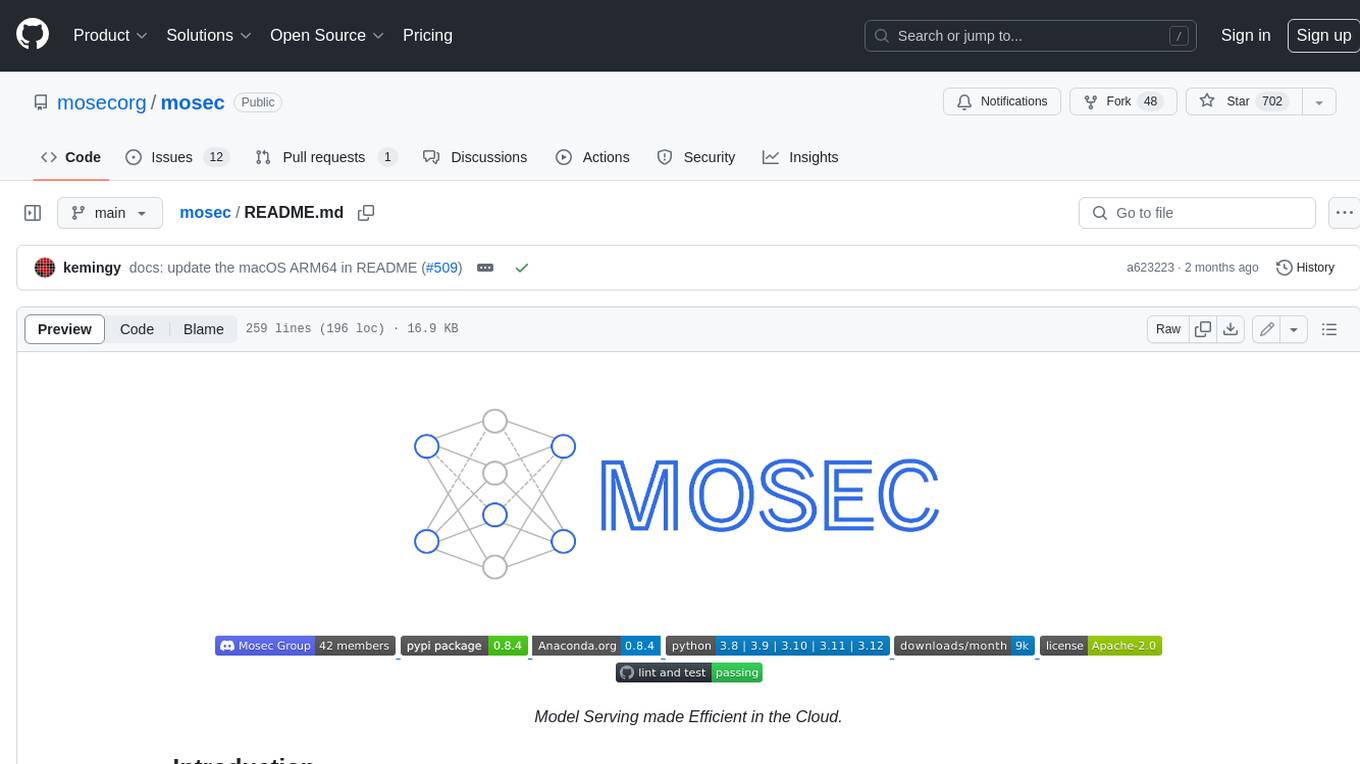
Mosec is a high-performance and flexible model serving framework for building ML model-enabled backend and microservices. It bridges the gap between any machine learning models you just trained and the efficient online service API. * **Highly performant** : web layer and task coordination built with Rust π¦, which offers blazing speed in addition to efficient CPU utilization powered by async I/O * **Ease of use** : user interface purely in Python π, by which users can serve their models in an ML framework-agnostic manner using the same code as they do for offline testing * **Dynamic batching** : aggregate requests from different users for batched inference and distribute results back * **Pipelined stages** : spawn multiple processes for pipelined stages to handle CPU/GPU/IO mixed workloads * **Cloud friendly** : designed to run in the cloud, with the model warmup, graceful shutdown, and Prometheus monitoring metrics, easily managed by Kubernetes or any container orchestration systems * **Do one thing well** : focus on the online serving part, users can pay attention to the model optimization and business logic
README:
Model Serving made Efficient in the Cloud.
Mosec is a high-performance and flexible model serving framework for building ML model-enabled backend and microservices. It bridges the gap between any machine learning models you just trained and the efficient online service API.
- Highly performant: web layer and task coordination built with Rust π¦, which offers blazing speed in addition to efficient CPU utilization powered by async I/O
- Ease of use: user interface purely in Python π, by which users can serve their models in an ML framework-agnostic manner using the same code as they do for offline testing
- Dynamic batching: aggregate requests from different users for batched inference and distribute results back
- Pipelined stages: spawn multiple processes for pipelined stages to handle CPU/GPU/IO mixed workloads
- Cloud friendly: designed to run in the cloud, with the model warmup, graceful shutdown, and Prometheus monitoring metrics, easily managed by Kubernetes or any container orchestration systems
- Do one thing well: focus on the online serving part, users can pay attention to the model optimization and business logic
Mosec requires Python 3.7 or above. Install the latest PyPI package for Linux x86_64 or macOS x86_64/ARM64 with:
pip install -U mosec
# or install with conda
conda install conda-forge::mosec
To build from the source code, install Rust and run the following command:
make package
You will get a mosec wheel file in the dist
folder.
We demonstrate how Mosec can help you easily host a pre-trained stable diffusion model as a service. You need to install diffusers and transformers as prerequisites:
pip install --upgrade diffusers[torch] transformers
Click me for server codes with explanations.
Firstly, we import the libraries and set up a basic logger to better observe what happens.
from io import BytesIO
from typing import List
import torch # type: ignore
from diffusers import StableDiffusionPipeline # type: ignore
from mosec import Server, Worker, get_logger
from mosec.mixin import MsgpackMixin
logger = get_logger()
Then, we build an API for clients to query a text prompt and obtain an image based on the stable-diffusion-v1-5 model in just 3 steps.
-
Define your service as a class which inherits
mosec.Worker
. Here we also inheritMsgpackMixin
to employ the msgpack serialization format(a). -
Inside the
__init__
method, initialize your model and put it onto the corresponding device. Optionally you can assignself.example
with some data to warm up(b) the model. Note that the data should be compatible with your handler's input format, which we detail next. -
Override the
forward
method to write your service handler(c), with the signatureforward(self, data: Any | List[Any]) -> Any | List[Any]
. Receiving/returning a single item or a tuple depends on whether dynamic batching(d) is configured.
class StableDiffusion(MsgpackMixin, Worker):
def __init__(self):
self.pipe = StableDiffusionPipeline.from_pretrained(
"runwayml/stable-diffusion-v1-5", torch_dtype=torch.float16
)
device = "cuda" if torch.cuda.is_available() else "cpu"
self.pipe = self.pipe.to(device)
self.example = ["useless example prompt"] * 4 # warmup (batch_size=4)
def forward(self, data: List[str]) -> List[memoryview]:
logger.debug("generate images for %s", data)
res = self.pipe(data)
logger.debug("NSFW: %s", res[1])
images = []
for img in res[0]:
dummy_file = BytesIO()
img.save(dummy_file, format="JPEG")
images.append(dummy_file.getbuffer())
return images
[!NOTE]
(a) In this example we return an image in the binary format, which JSON does not support (unless encoded with base64 that makes the payload larger). Hence, msgpack suits our need better. If we do not inherit
MsgpackMixin
, JSON will be used by default. In other words, the protocol of the service request/response can be either msgpack, JSON, or any other format (check our mixins).(b) Warm-up usually helps to allocate GPU memory in advance. If the warm-up example is specified, the service will only be ready after the example is forwarded through the handler. However, if no example is given, the first request's latency is expected to be longer. The
example
should be set as a single item or a tuple depending on whatforward
expects to receive. Moreover, in the case where you want to warm up with multiple different examples, you may setmulti_examples
(demo here).(c) This example shows a single-stage service, where the
StableDiffusion
worker directly takes in client's prompt request and responds the image. Thus theforward
can be considered as a complete service handler. However, we can also design a multi-stage service with workers doing different jobs (e.g., downloading images, model inference, post-processing) in a pipeline. In this case, the whole pipeline is considered as the service handler, with the first worker taking in the request and the last worker sending out the response. The data flow between workers is done by inter-process communication.(d) Since dynamic batching is enabled in this example, the
forward
method will wishfully receive a list of string, e.g.,['a cute cat playing with a red ball', 'a man sitting in front of a computer', ...]
, aggregated from different clients for batch inference, improving the system throughput.
Finally, we append the worker to the server to construct a single-stage workflow (multiple stages can be pipelined to further boost the throughput, see this example), and specify the number of processes we want it to run in parallel (num=1
), and the maximum batch size (max_batch_size=4
, the maximum number of requests dynamic batching will accumulate before timeout; timeout is defined with the max_wait_time=10
in milliseconds, meaning the longest time Mosec waits until sending the batch to the Worker).
if __name__ == "__main__":
server = Server()
# 1) `num` specifies the number of processes that will be spawned to run in parallel.
# 2) By configuring the `max_batch_size` with the value > 1, the input data in your
# `forward` function will be a list (batch); otherwise, it's a single item.
server.append_worker(StableDiffusion, num=1, max_batch_size=4, max_wait_time=10)
server.run()
Click me to see how to run and query the server.
The above snippets are merged in our example file. You may directly run at the project root level. We first have a look at the command line arguments (explanations here):
python examples/stable_diffusion/server.py --help
Then let's start the server with debug logs:
python examples/stable_diffusion/server.py --log-level debug --timeout 30000
Open http://127.0.0.1:8000/openapi/swagger/
in your browser to get the OpenAPI doc.
And in another terminal, test it:
python examples/stable_diffusion/client.py --prompt "a cute cat playing with a red ball" --output cat.jpg --port 8000
You will get an image named "cat.jpg" in the current directory.
You can check the metrics:
curl http://127.0.0.1:8000/metrics
That's it! You have just hosted your stable-diffusion model as a service! π
More ready-to-use examples can be found in the Example section. It includes:
- Pipeline: a simple echo demo even without any ML model.
- Request validation: validate the request with type annotation.
- Multiple route: serve multiple models in one service
- Embedding service: OpenAI compatible embedding service
- Reranking service: rerank a list of passages based on a query
- Shared memory IPC: inter-process communication with shared memory.
- Customized GPU allocation: deploy multiple replicas, each using different GPUs.
- Customized metrics: record your own metrics for monitoring.
- Jax jitted inference: just-in-time compilation speeds up the inference.
- PyTorch deep learning models:
- sentiment analysis: infer the sentiment of a sentence.
- image recognition: categorize a given image.
- stable diffusion: generate images based on texts, with msgpack serialization.
- Dynamic batching
-
max_batch_size
andmax_wait_time (millisecond)
are configured when you callappend_worker
. - Make sure inference with the
max_batch_size
value won't cause the out-of-memory in GPU. - Normally,
max_wait_time
should be less than the batch inference time. - If enabled, it will collect a batch either when the number of accumulated requests reaches
max_batch_size
or whenmax_wait_time
has elapsed. The service will benefit from this feature when the traffic is high.
-
- Check the arguments doc for other configurations.
- If you're looking for a GPU base image with
mosec
installed, you can check the official imagemosecorg/mosec
. For the complex use case, check out envd. - This service doesn't need Gunicorn or NGINX, but you can certainly use the ingress controller when necessary.
- This service should be the PID 1 process in the container since it controls multiple processes. If you need to run multiple processes in one container, you will need a supervisor. You may choose Supervisor or Horust.
- Remember to collect the metrics.
-
mosec_service_batch_size_bucket
shows the batch size distribution. -
mosec_service_batch_duration_second_bucket
shows the duration of dynamic batching for each connection in each stage (starts from receiving the first task). -
mosec_service_process_duration_second_bucket
shows the duration of processing for each connection in each stage (including the IPC time but excluding themosec_service_batch_duration_second_bucket
). -
mosec_service_remaining_task
shows the number of currently processing tasks. -
mosec_service_throughput
shows the service throughput.
-
- Stop the service with
SIGINT
(CTRL+C
) orSIGTERM
(kill {PID}
) since it has the graceful shutdown logic.
- Find out the best
max_batch_size
andmax_wait_time
for your inference service. The metrics will show the histograms of the real batch size and batch duration. Those are the key information to adjust these two parameters. - Try to split the whole inference process into separate CPU and GPU stages (ref DistilBERT). Different stages will be run in a data pipeline, which will keep the GPU busy.
- You can also adjust the number of workers in each stage. For example, if your pipeline consists of a CPU stage for preprocessing and a GPU stage for model inference, increasing the number of CPU-stage workers can help to produce more data to be batched for model inference at the GPU stage; increasing the GPU-stage workers can fully utilize the GPU memory and computation power. Both ways may contribute to higher GPU utilization, which consequently results in higher service throughput.
- For multi-stage services, note that the data passing through different stages will be serialized/deserialized by the
serialize_ipc/deserialize_ipc
methods, so extremely large data might make the whole pipeline slow. The serialized data is passed to the next stage through rust by default, you could enable shared memory to potentially reduce the latency (ref RedisShmIPCMixin). - You should choose appropriate
serialize/deserialize
methods, which are used to decode the user request and encode the response. By default, both are using JSON. However, images and embeddings are not well supported by JSON. You can choose msgpack which is faster and binary compatible (ref Stable Diffusion). - Configure the threads for OpenBLAS or MKL. It might not be able to choose the most suitable CPUs used by the current Python process. You can configure it for each worker by using the env (ref custom GPU allocation).
Here are some of the companies and individual users that are using Mosec:
- Modelz: Serverless platform for ML inference.
- MOSS: An open sourced conversational language model like ChatGPT.
- TencentCloud: Tencent Cloud Machine Learning Platform, using Mosec as the core inference server framework.
- TensorChord: Cloud native AI infrastructure company.
If you find this software useful for your research, please consider citing
@software{yang2021mosec,
title = {{MOSEC: Model Serving made Efficient in the Cloud}},
author = {Yang, Keming and Liu, Zichen and Cheng, Philip},
url = {https://github.com/mosecorg/mosec},
year = {2021}
}
We welcome any kind of contribution. Please give us feedback by raising issues or discussing on Discord. You could also directly contribute your code and pull request!
To start develop, you can use envd to create an isolated and clean Python & Rust environment. Check the envd-docs or build.envd for more information.
For Tasks:
Click tags to check more tools for each tasksFor Jobs:
Alternative AI tools for mosec
Similar Open Source Tools
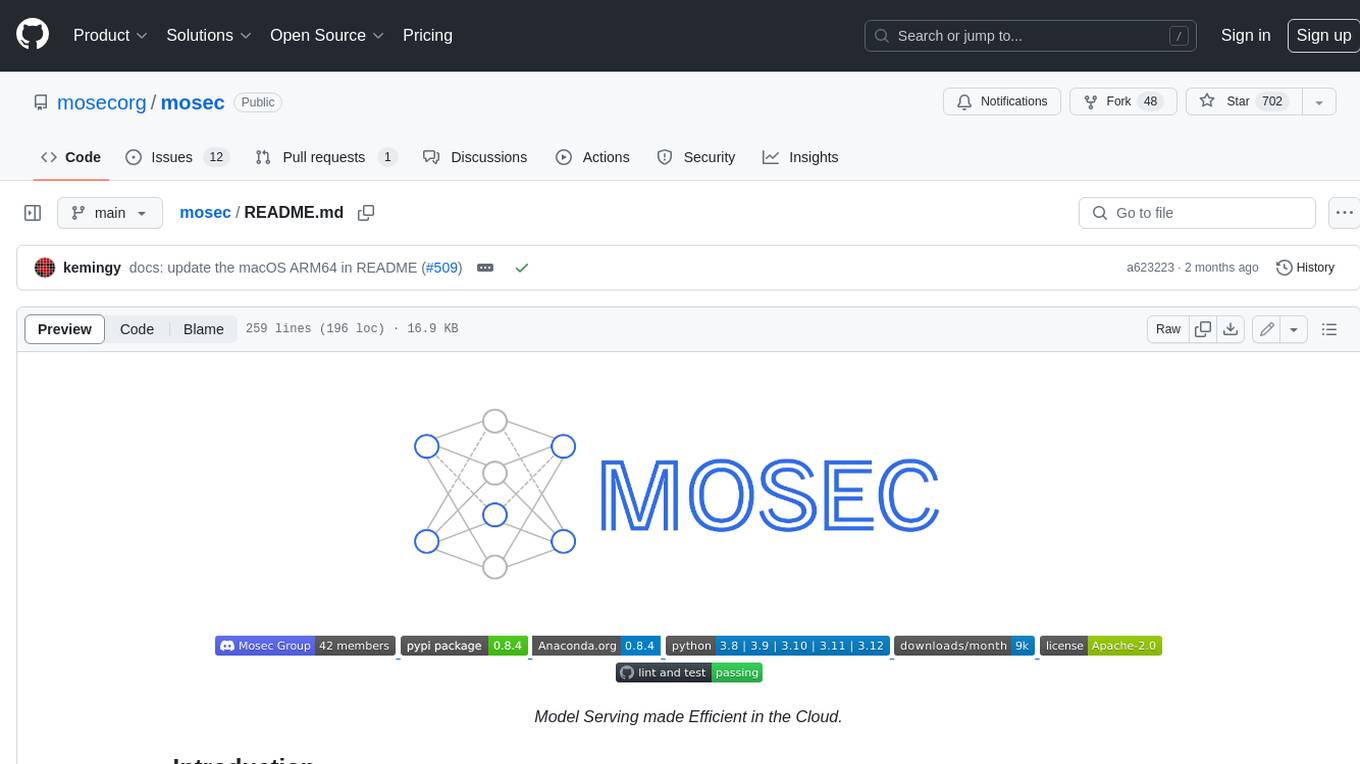
mosec
Mosec is a high-performance and flexible model serving framework for building ML model-enabled backend and microservices. It bridges the gap between any machine learning models you just trained and the efficient online service API. * **Highly performant** : web layer and task coordination built with Rust π¦, which offers blazing speed in addition to efficient CPU utilization powered by async I/O * **Ease of use** : user interface purely in Python π, by which users can serve their models in an ML framework-agnostic manner using the same code as they do for offline testing * **Dynamic batching** : aggregate requests from different users for batched inference and distribute results back * **Pipelined stages** : spawn multiple processes for pipelined stages to handle CPU/GPU/IO mixed workloads * **Cloud friendly** : designed to run in the cloud, with the model warmup, graceful shutdown, and Prometheus monitoring metrics, easily managed by Kubernetes or any container orchestration systems * **Do one thing well** : focus on the online serving part, users can pay attention to the model optimization and business logic
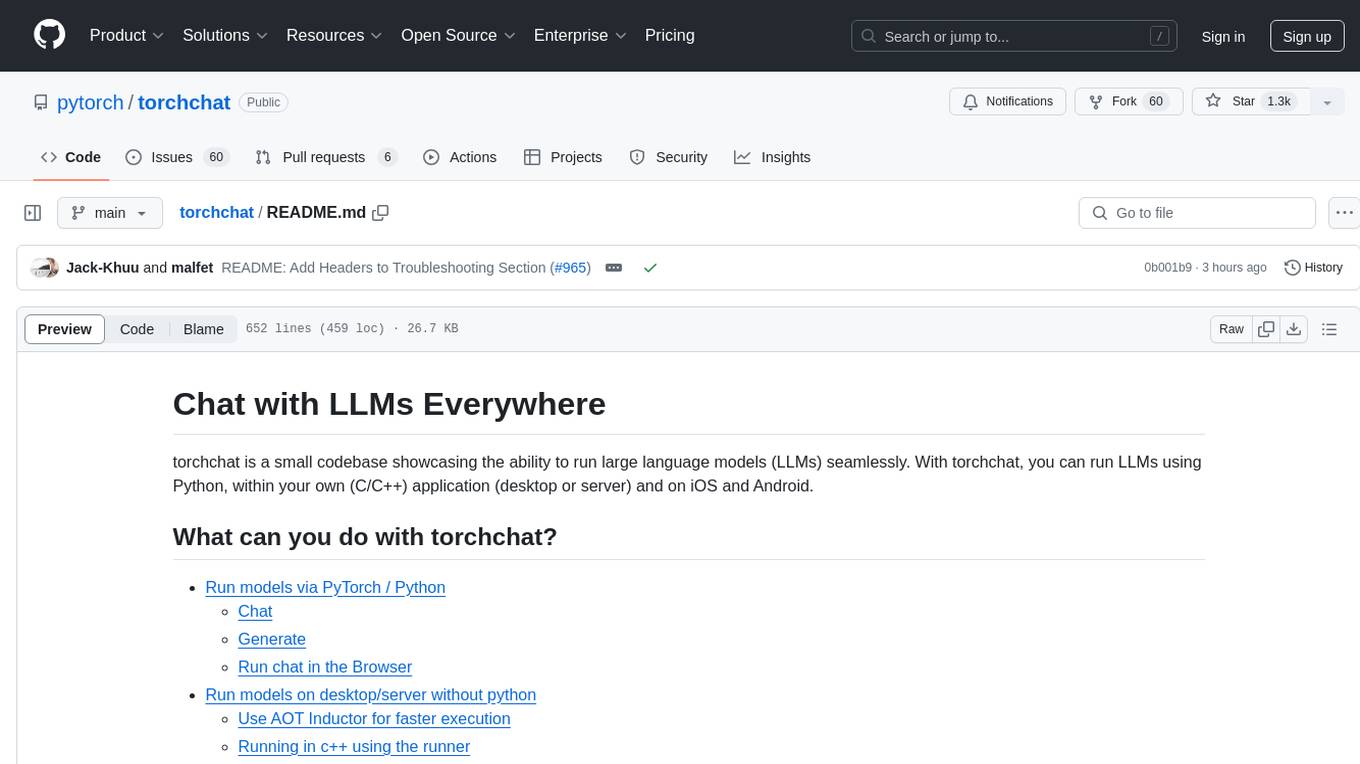
torchchat
torchchat is a codebase showcasing the ability to run large language models (LLMs) seamlessly. It allows running LLMs using Python in various environments such as desktop, server, iOS, and Android. The tool supports running models via PyTorch, chatting, generating text, running chat in the browser, and running models on desktop/server without Python. It also provides features like AOT Inductor for faster execution, running in C++ using the runner, and deploying and running on iOS and Android. The tool supports popular hardware and OS including Linux, Mac OS, Android, and iOS, with various data types and execution modes available.
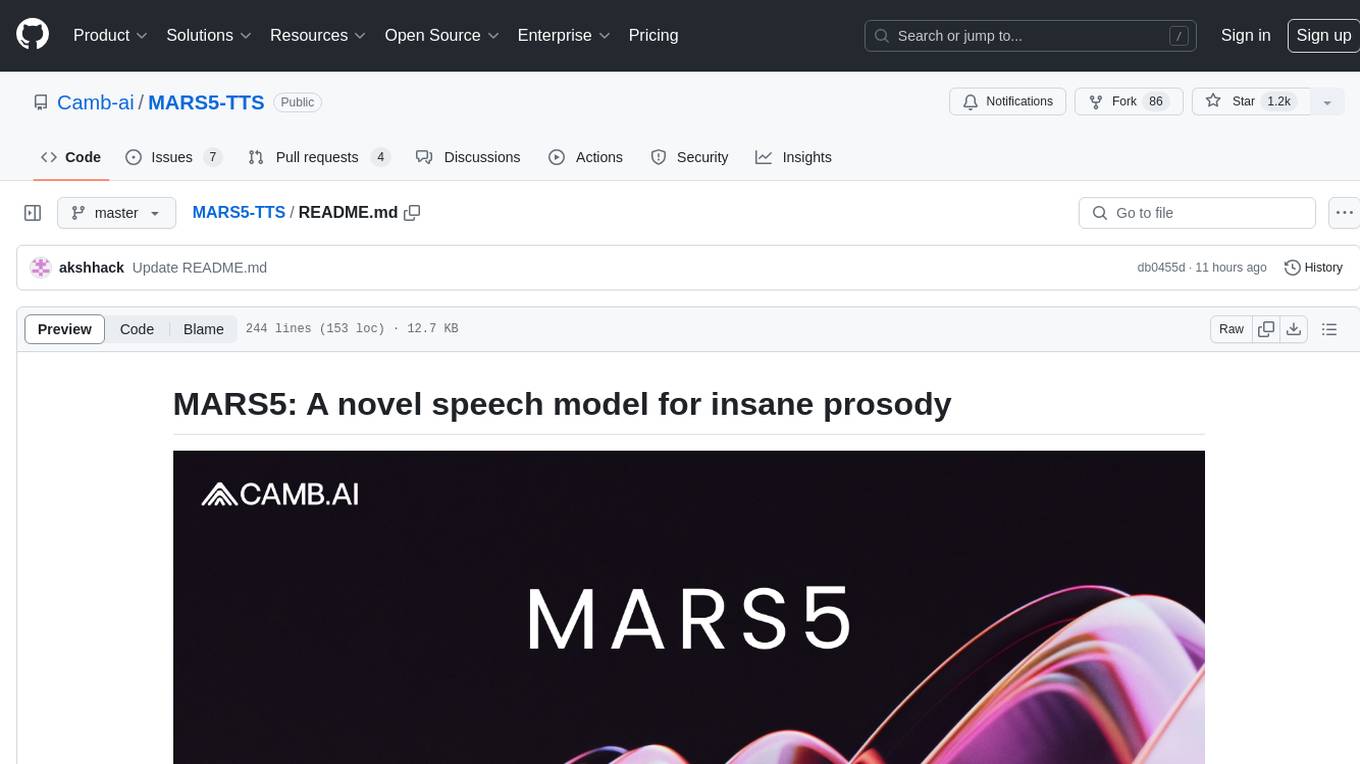
MARS5-TTS
MARS5 is a novel English speech model (TTS) developed by CAMB.AI, featuring a two-stage AR-NAR pipeline with a unique NAR component. The model can generate speech for various scenarios like sports commentary and anime with just 5 seconds of audio and a text snippet. It allows steering prosody using punctuation and capitalization in the transcript. Speaker identity is specified using an audio reference file, enabling 'deep clone' for improved quality. The model can be used via torch.hub or HuggingFace, supporting both shallow and deep cloning for inference. Checkpoints are provided for AR and NAR models, with hardware requirements of 750M+450M params on GPU. Contributions to improve model stability, performance, and reference audio selection are welcome.
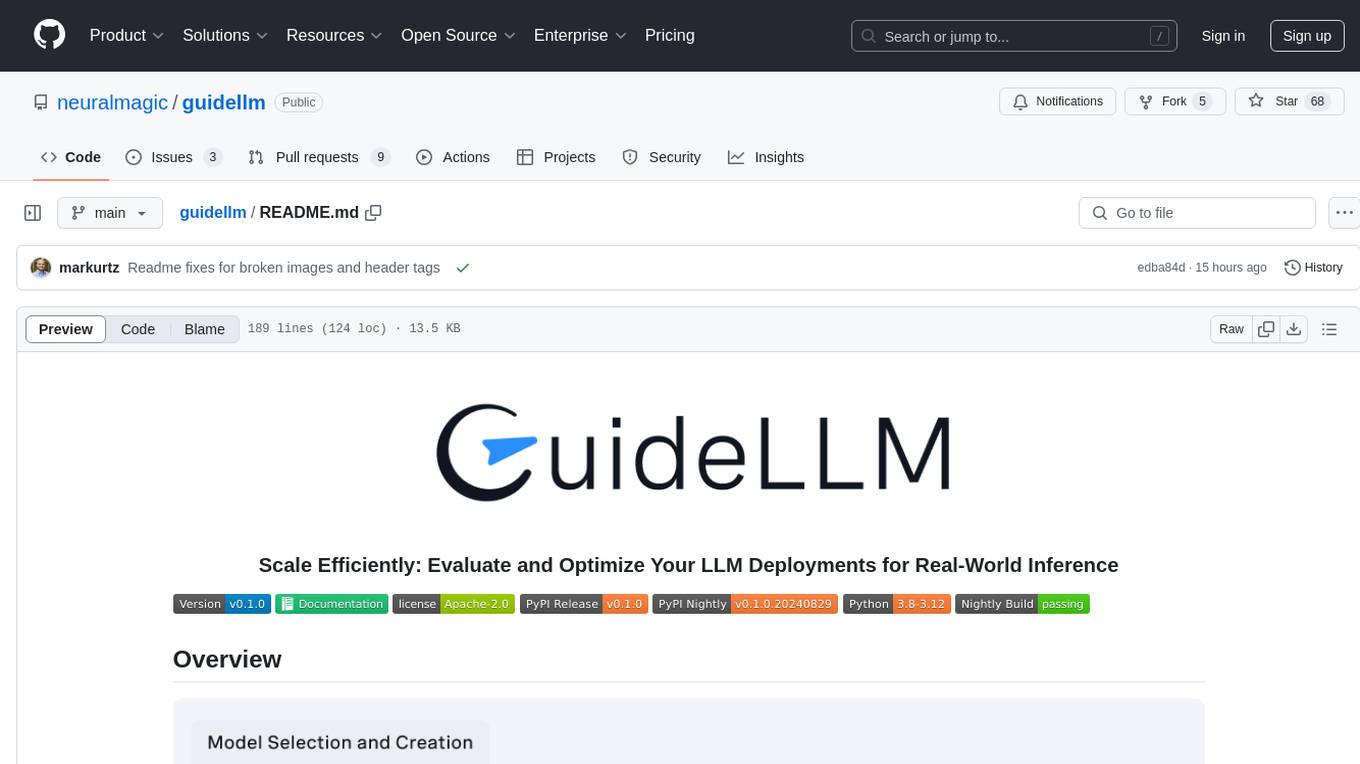
guidellm
GuideLLM is a powerful tool for evaluating and optimizing the deployment of large language models (LLMs). By simulating real-world inference workloads, GuideLLM helps users gauge the performance, resource needs, and cost implications of deploying LLMs on various hardware configurations. This approach ensures efficient, scalable, and cost-effective LLM inference serving while maintaining high service quality. Key features include performance evaluation, resource optimization, cost estimation, and scalability testing.
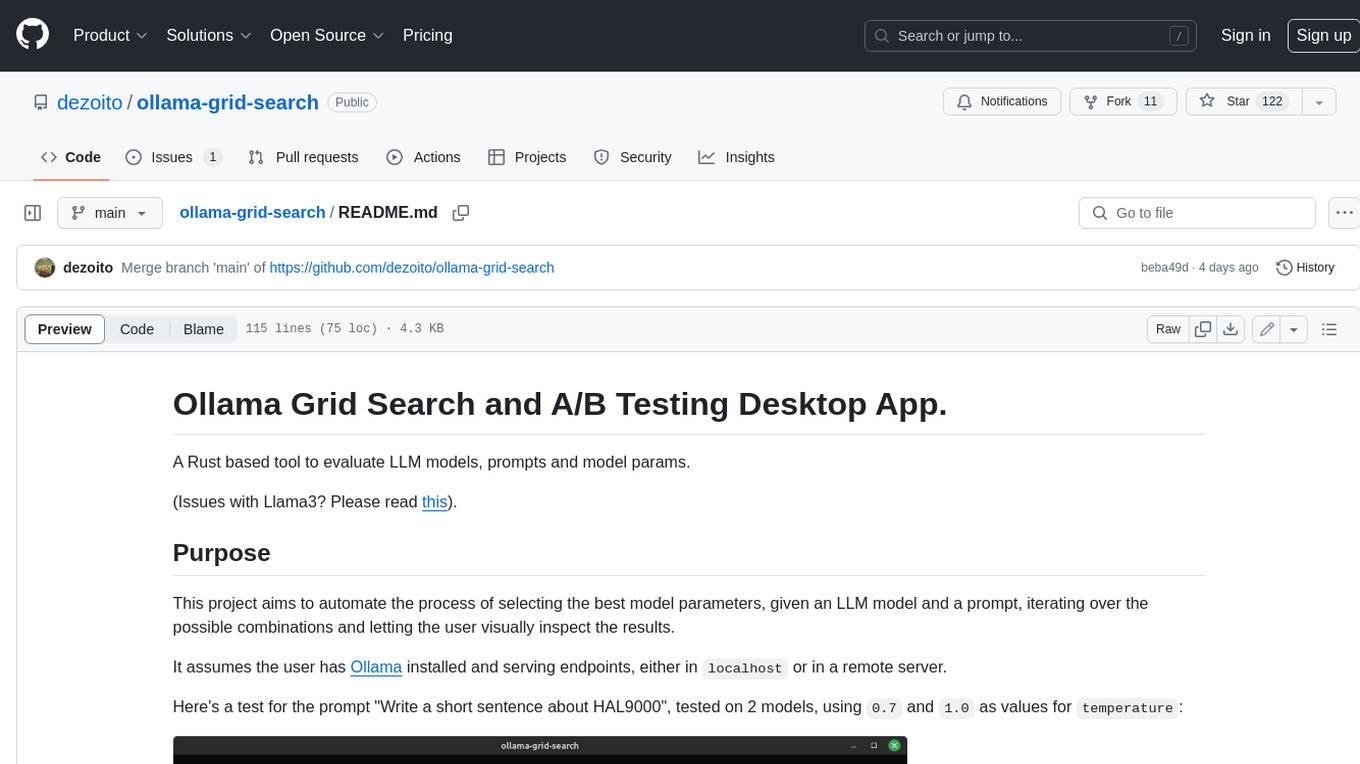
ollama-grid-search
A Rust based tool to evaluate LLM models, prompts and model params. It automates the process of selecting the best model parameters, given an LLM model and a prompt, iterating over the possible combinations and letting the user visually inspect the results. The tool assumes the user has Ollama installed and serving endpoints, either in `localhost` or in a remote server. Key features include: * Automatically fetches models from local or remote Ollama servers * Iterates over different models and params to generate inferences * A/B test prompts on different models simultaneously * Allows multiple iterations for each combination of parameters * Makes synchronous inference calls to avoid spamming servers * Optionally outputs inference parameters and response metadata (inference time, tokens and tokens/s) * Refetching of individual inference calls * Model selection can be filtered by name * List experiments which can be downloaded in JSON format * Configurable inference timeout * Custom default parameters and system prompts can be defined in settings
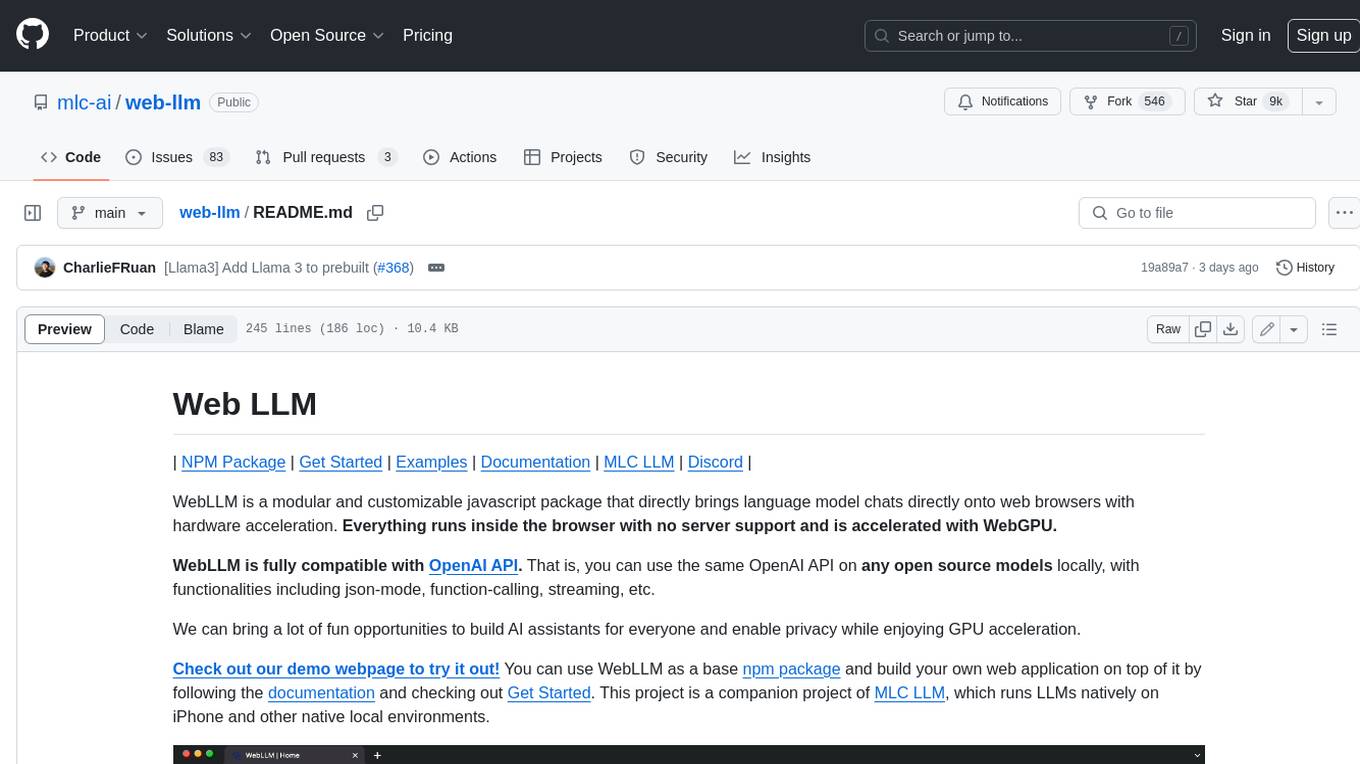
web-llm
WebLLM is a modular and customizable javascript package that directly brings language model chats directly onto web browsers with hardware acceleration. Everything runs inside the browser with no server support and is accelerated with WebGPU. WebLLM is fully compatible with OpenAI API. That is, you can use the same OpenAI API on any open source models locally, with functionalities including json-mode, function-calling, streaming, etc. We can bring a lot of fun opportunities to build AI assistants for everyone and enable privacy while enjoying GPU acceleration.
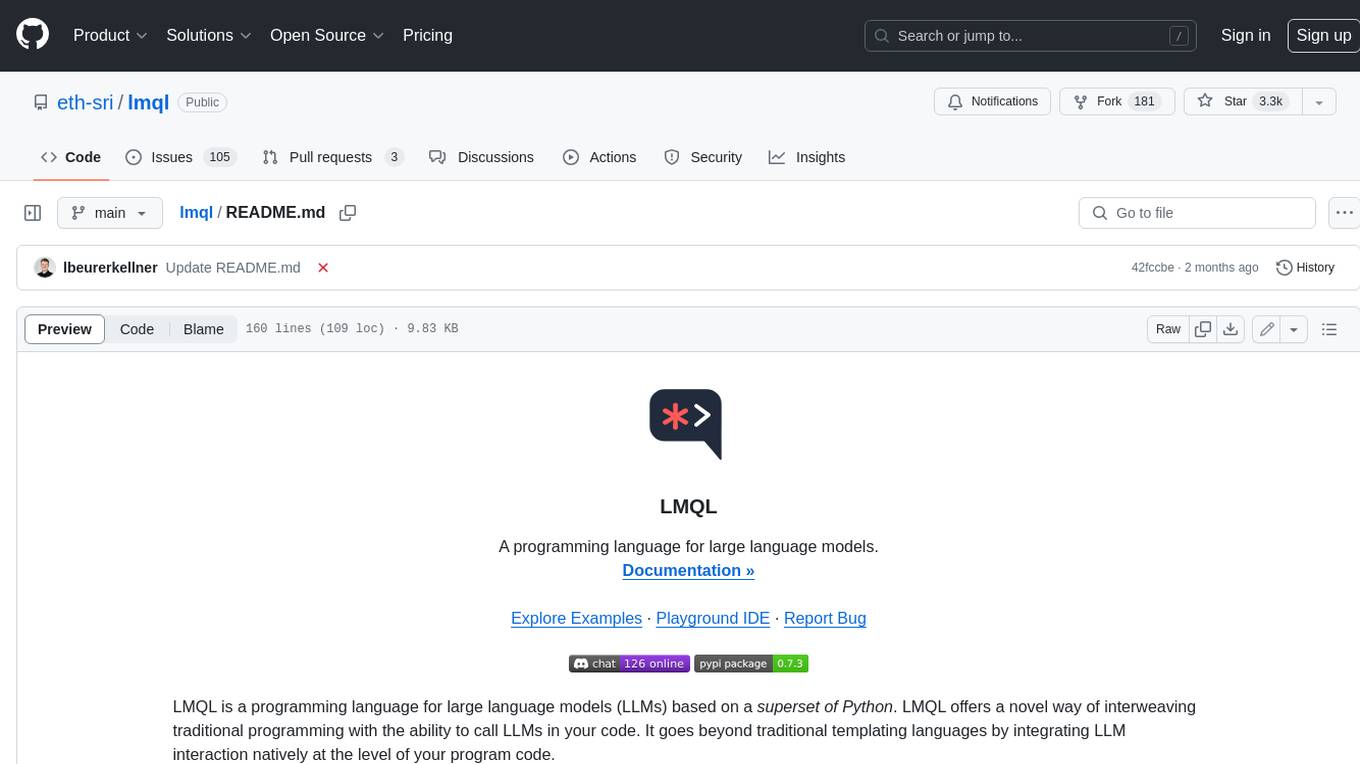
lmql
LMQL is a programming language designed for large language models (LLMs) that offers a unique way of integrating traditional programming with LLM interaction. It allows users to write programs that combine algorithmic logic with LLM calls, enabling model reasoning capabilities within the context of the program. LMQL provides features such as Python syntax integration, rich control-flow options, advanced decoding techniques, powerful constraints via logit masking, runtime optimization, sync and async API support, multi-model compatibility, and extensive applications like JSON decoding and interactive chat interfaces. The tool also offers library integration, flexible tooling, and output streaming options for easy model output handling.
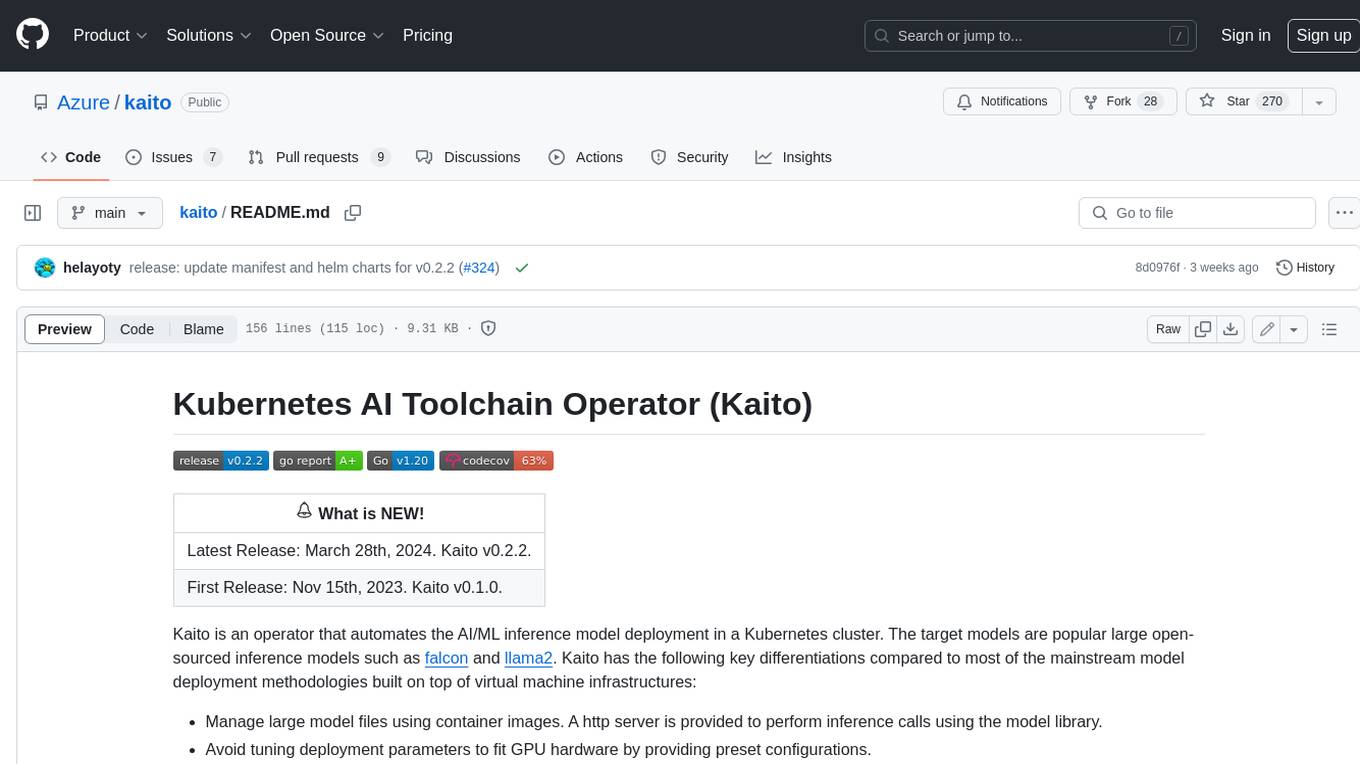
kaito
Kaito is an operator that automates the AI/ML inference model deployment in a Kubernetes cluster. It manages large model files using container images, avoids tuning deployment parameters to fit GPU hardware by providing preset configurations, auto-provisions GPU nodes based on model requirements, and hosts large model images in the public Microsoft Container Registry (MCR) if the license allows. Using Kaito, the workflow of onboarding large AI inference models in Kubernetes is largely simplified.
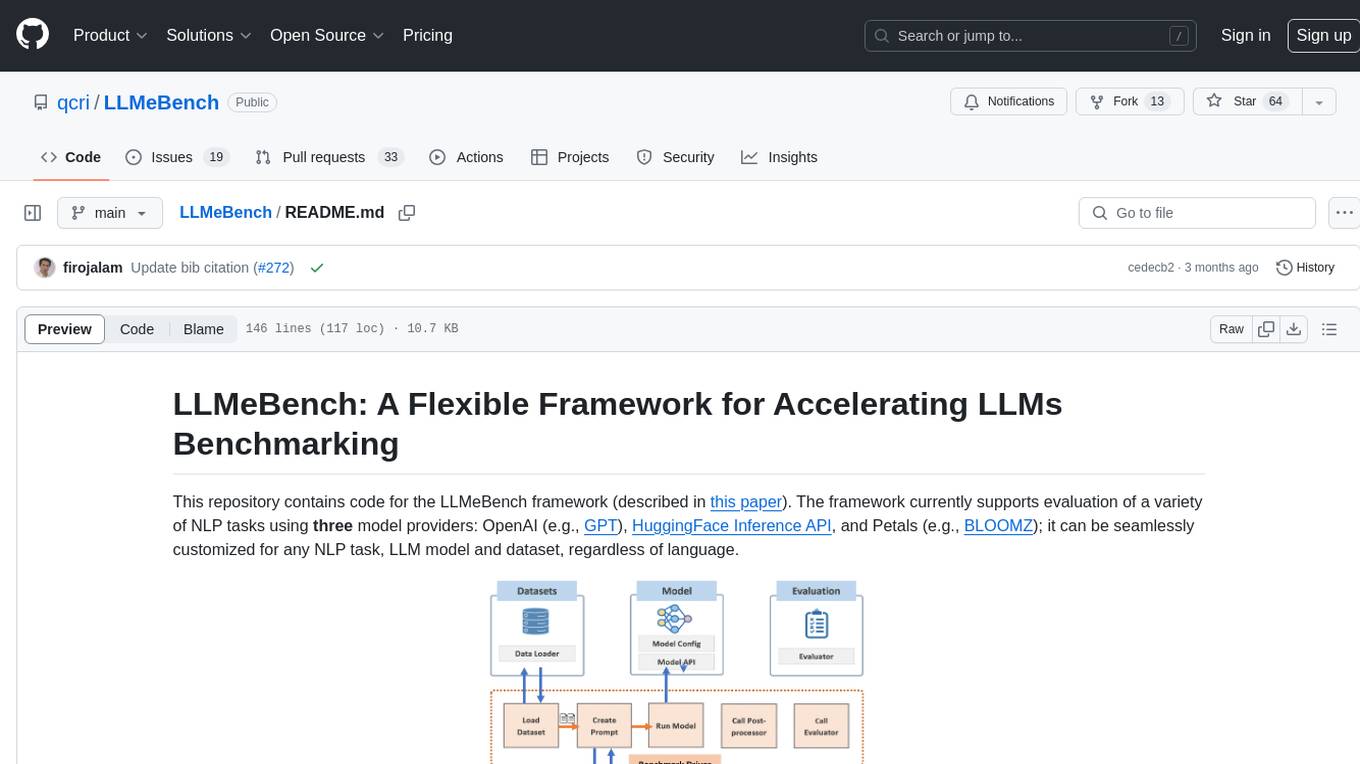
LLMeBench
LLMeBench is a flexible framework designed for accelerating benchmarking of Large Language Models (LLMs) in the field of Natural Language Processing (NLP). It supports evaluation of various NLP tasks using model providers like OpenAI, HuggingFace Inference API, and Petals. The framework is customizable for different NLP tasks, LLM models, and datasets across multiple languages. It features extensive caching capabilities, supports zero- and few-shot learning paradigms, and allows on-the-fly dataset download and caching. LLMeBench is open-source and continuously expanding to support new models accessible through APIs.
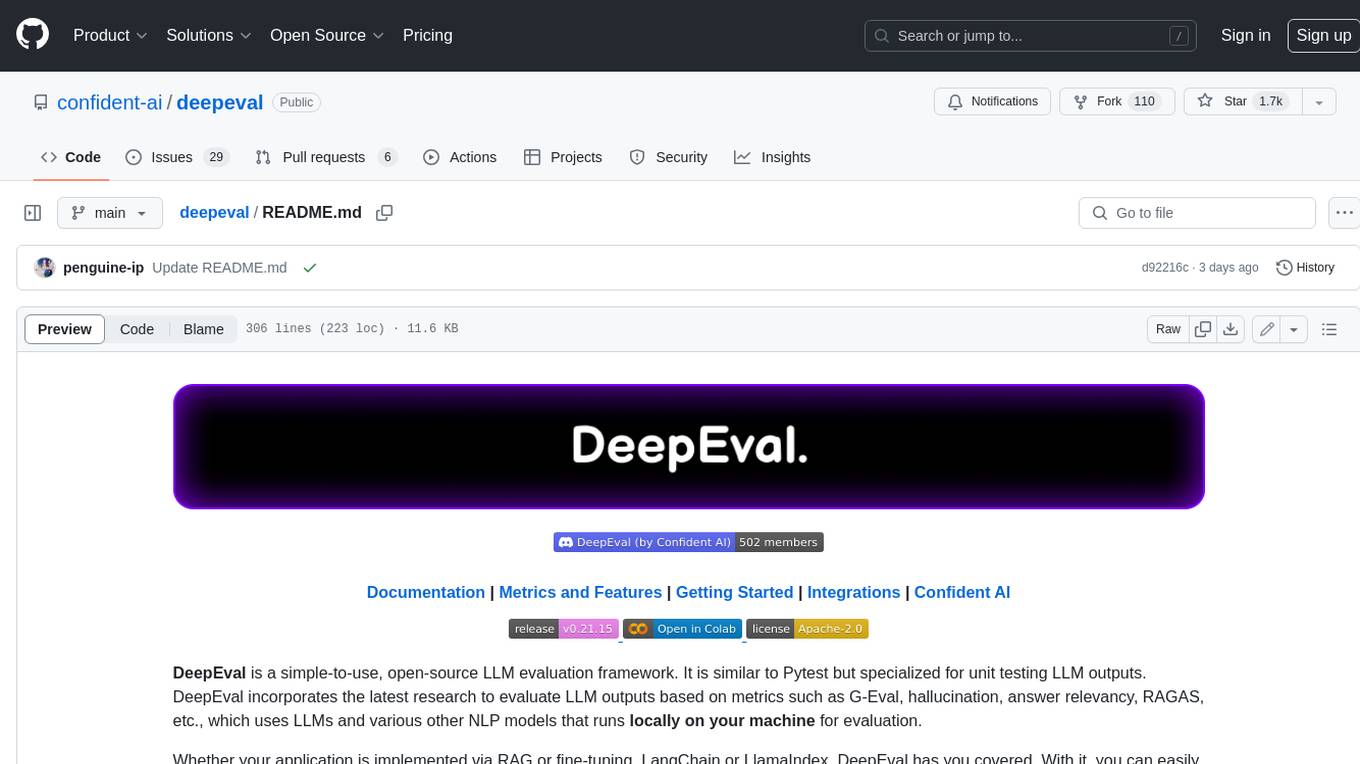
deepeval
DeepEval is a simple-to-use, open-source LLM evaluation framework specialized for unit testing LLM outputs. It incorporates various metrics such as G-Eval, hallucination, answer relevancy, RAGAS, etc., and runs locally on your machine for evaluation. It provides a wide range of ready-to-use evaluation metrics, allows for creating custom metrics, integrates with any CI/CD environment, and enables benchmarking LLMs on popular benchmarks. DeepEval is designed for evaluating RAG and fine-tuning applications, helping users optimize hyperparameters, prevent prompt drifting, and transition from OpenAI to hosting their own Llama2 with confidence.
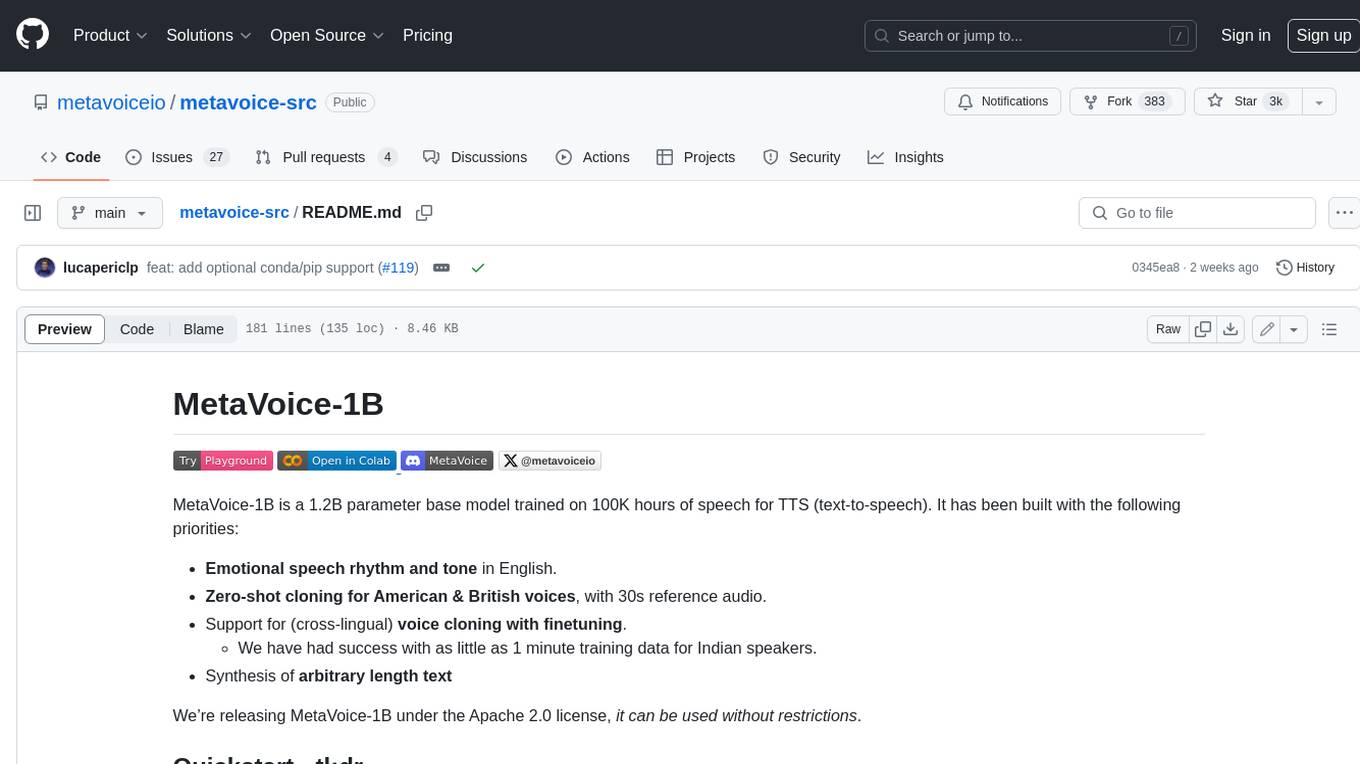
metavoice-src
MetaVoice-1B is a 1.2B parameter base model trained on 100K hours of speech for TTS (text-to-speech). It has been built with the following priorities: * Emotional speech rhythm and tone in English. * Zero-shot cloning for American & British voices, with 30s reference audio. * Support for (cross-lingual) voice cloning with finetuning. * We have had success with as little as 1 minute training data for Indian speakers. * Synthesis of arbitrary length text
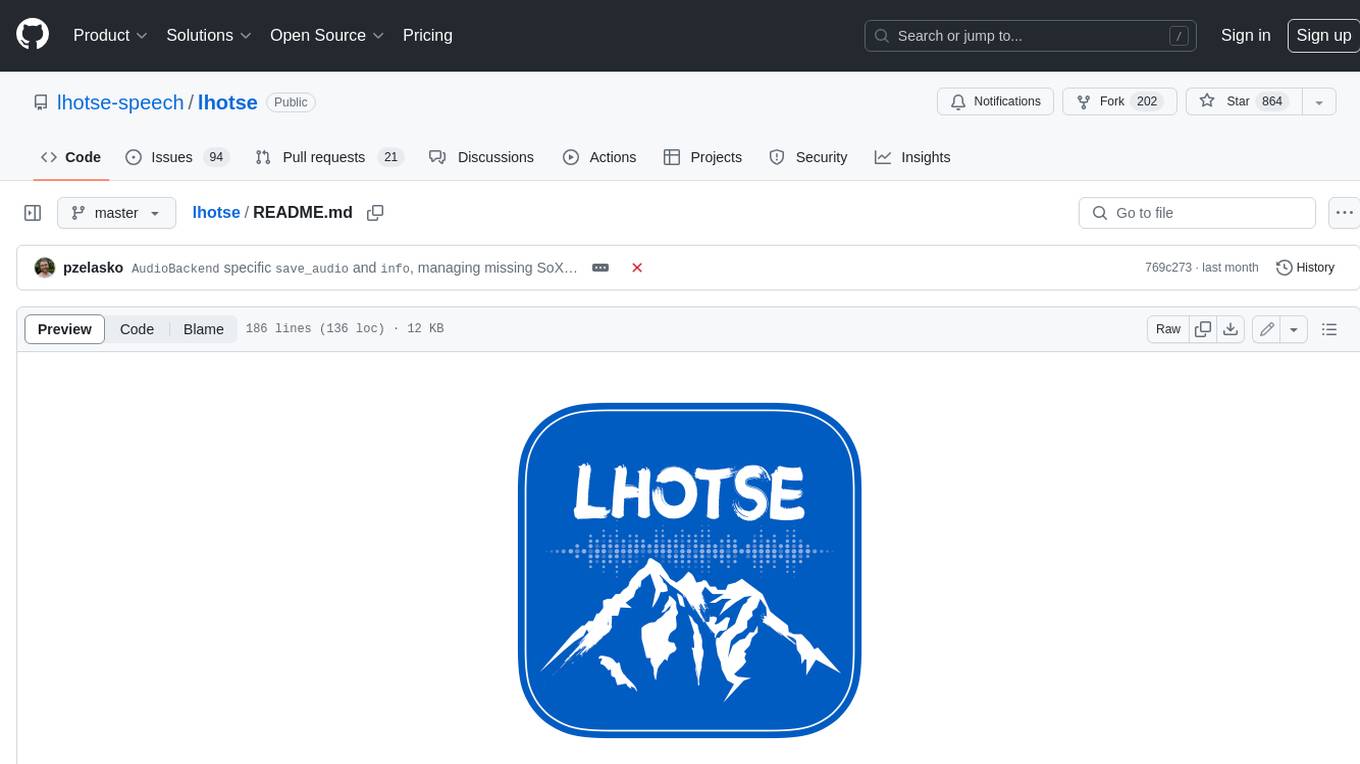
lhotse
Lhotse is a Python library designed to make speech and audio data preparation flexible and accessible. It aims to attract a wider community to speech processing tasks by providing a Python-centric design and an expressive command-line interface. Lhotse offers standard data preparation recipes, PyTorch Dataset classes for speech tasks, and efficient data preparation for model training with audio cuts. It supports data augmentation, feature extraction, and feature-space cut mixing. The tool extends Kaldi's data preparation recipes with seamless PyTorch integration, human-readable text manifests, and convenient Python classes.
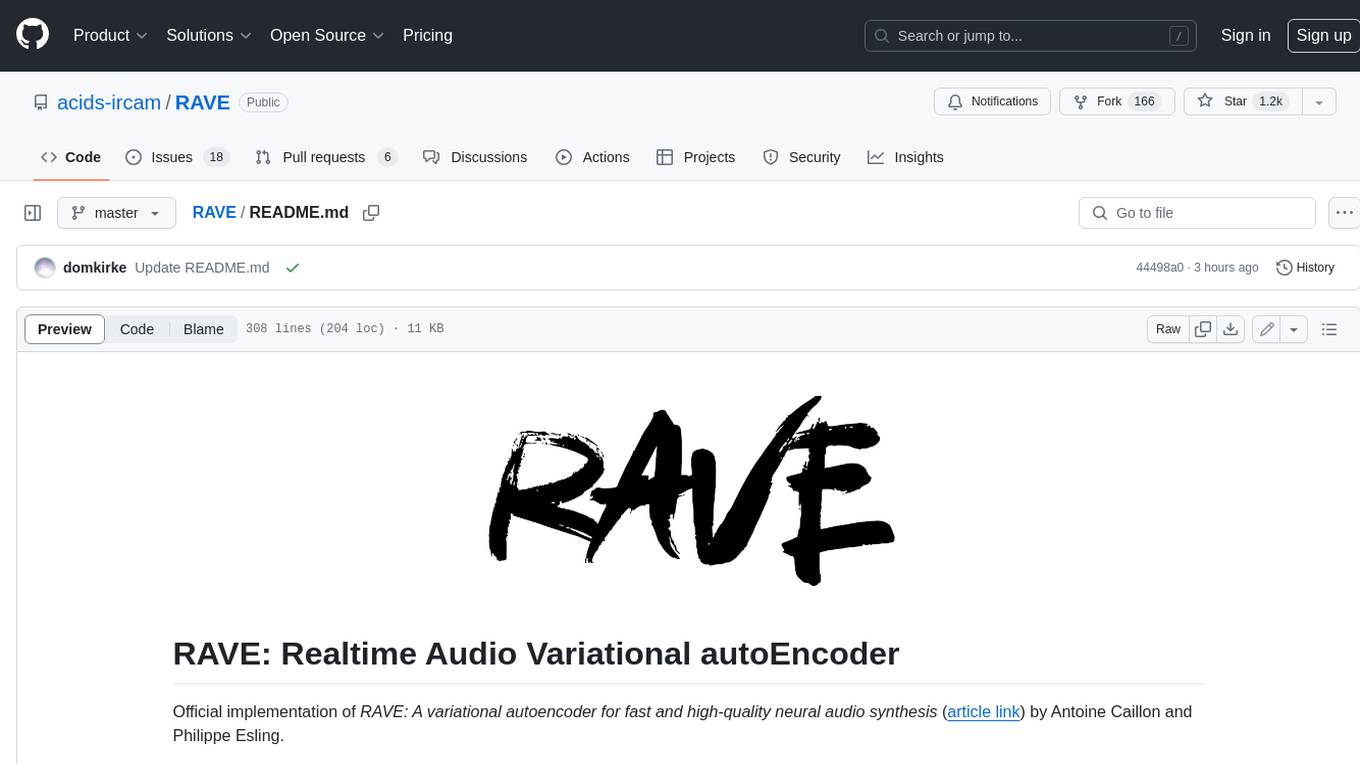
RAVE
RAVE is a variational autoencoder for fast and high-quality neural audio synthesis. It can be used to generate new audio samples from a given dataset, or to modify the style of existing audio samples. RAVE is easy to use and can be trained on a variety of audio datasets. It is also computationally efficient, making it suitable for real-time applications.
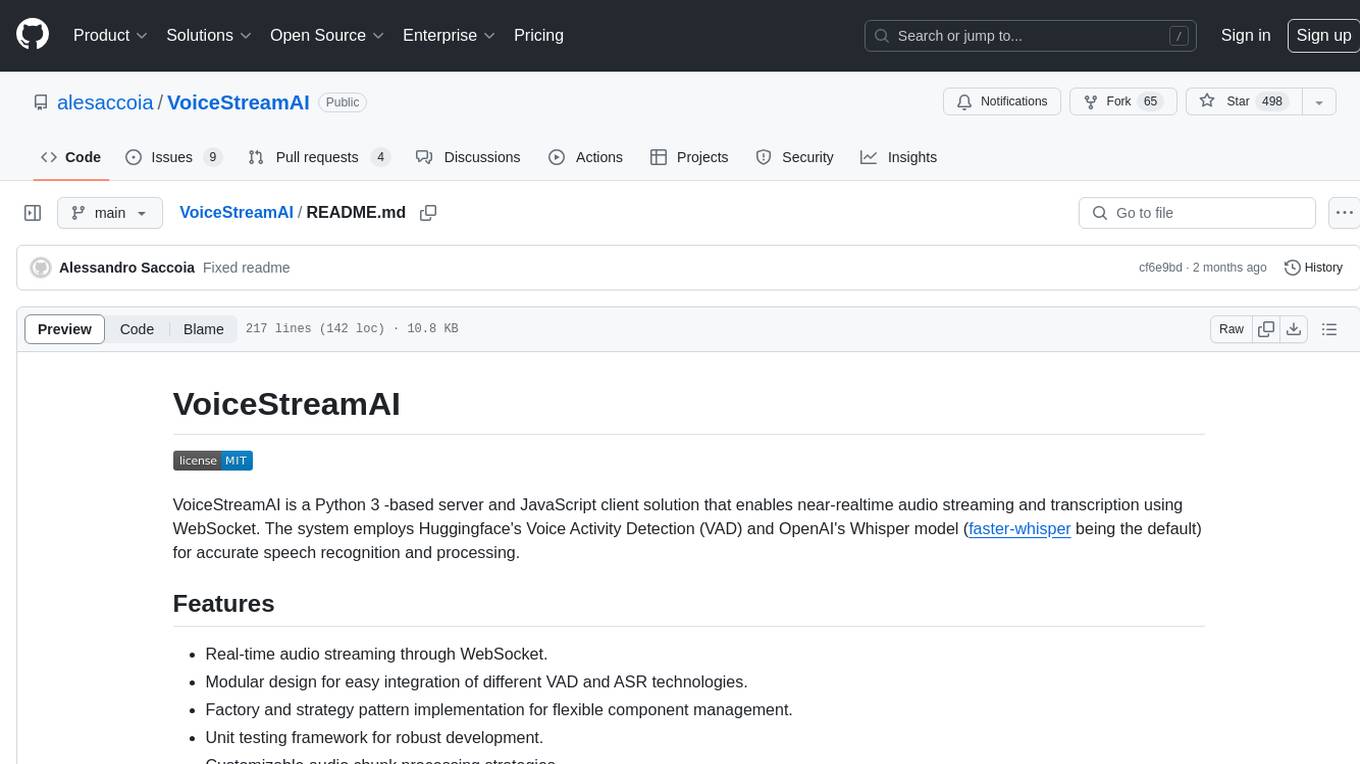
VoiceStreamAI
VoiceStreamAI is a Python 3-based server and JavaScript client solution for near-realtime audio streaming and transcription using WebSocket. It employs Huggingface's Voice Activity Detection (VAD) and OpenAI's Whisper model for accurate speech recognition. The system features real-time audio streaming, modular design for easy integration of VAD and ASR technologies, customizable audio chunk processing strategies, support for multilingual transcription, and secure sockets support. It uses a factory and strategy pattern implementation for flexible component management and provides a unit testing framework for robust development.
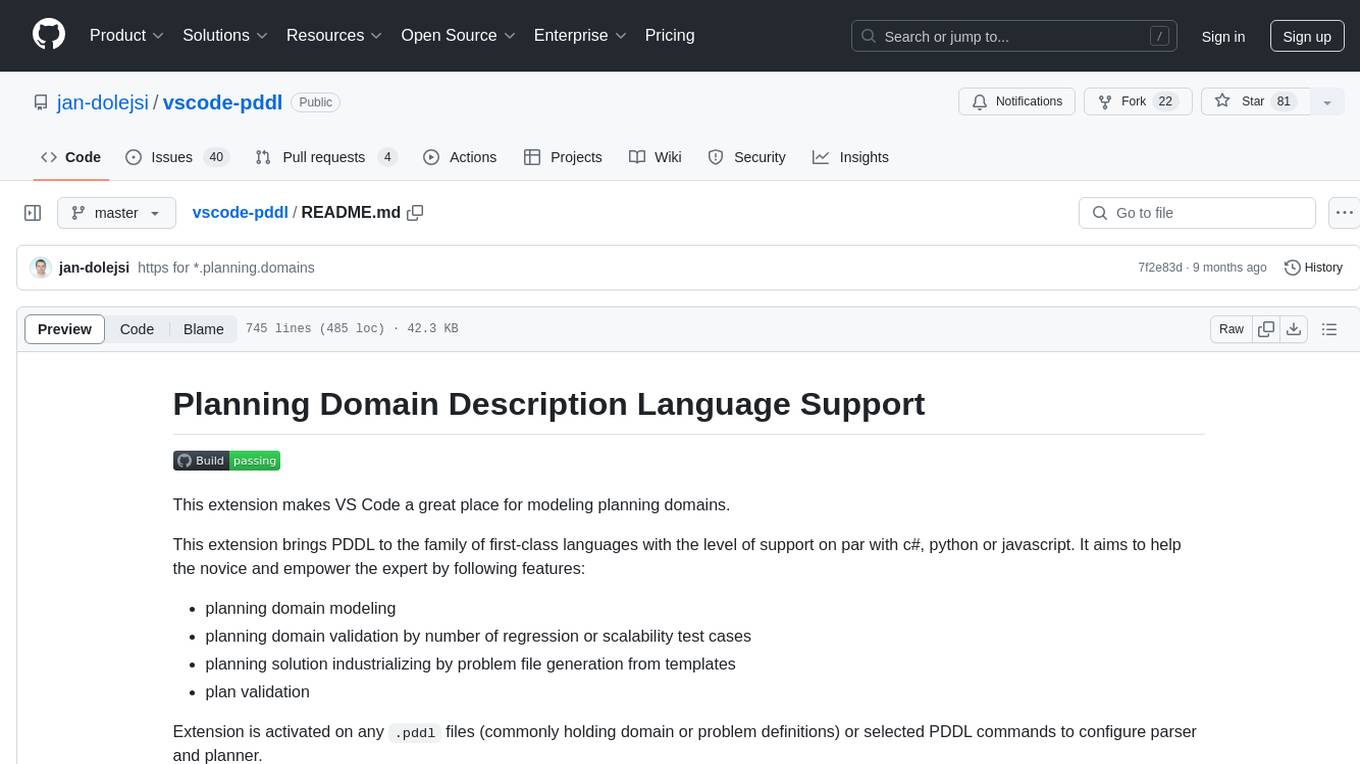
vscode-pddl
The vscode-pddl extension provides comprehensive support for Planning Domain Description Language (PDDL) in Visual Studio Code. It enables users to model planning domains, validate them, industrialize planning solutions, and run planners. The extension offers features like syntax highlighting, auto-completion, plan visualization, plan validation, plan happenings evaluation, search debugging, and integration with Planning.Domains. Users can create PDDL files, run planners, visualize plans, and debug search algorithms efficiently within VS Code.
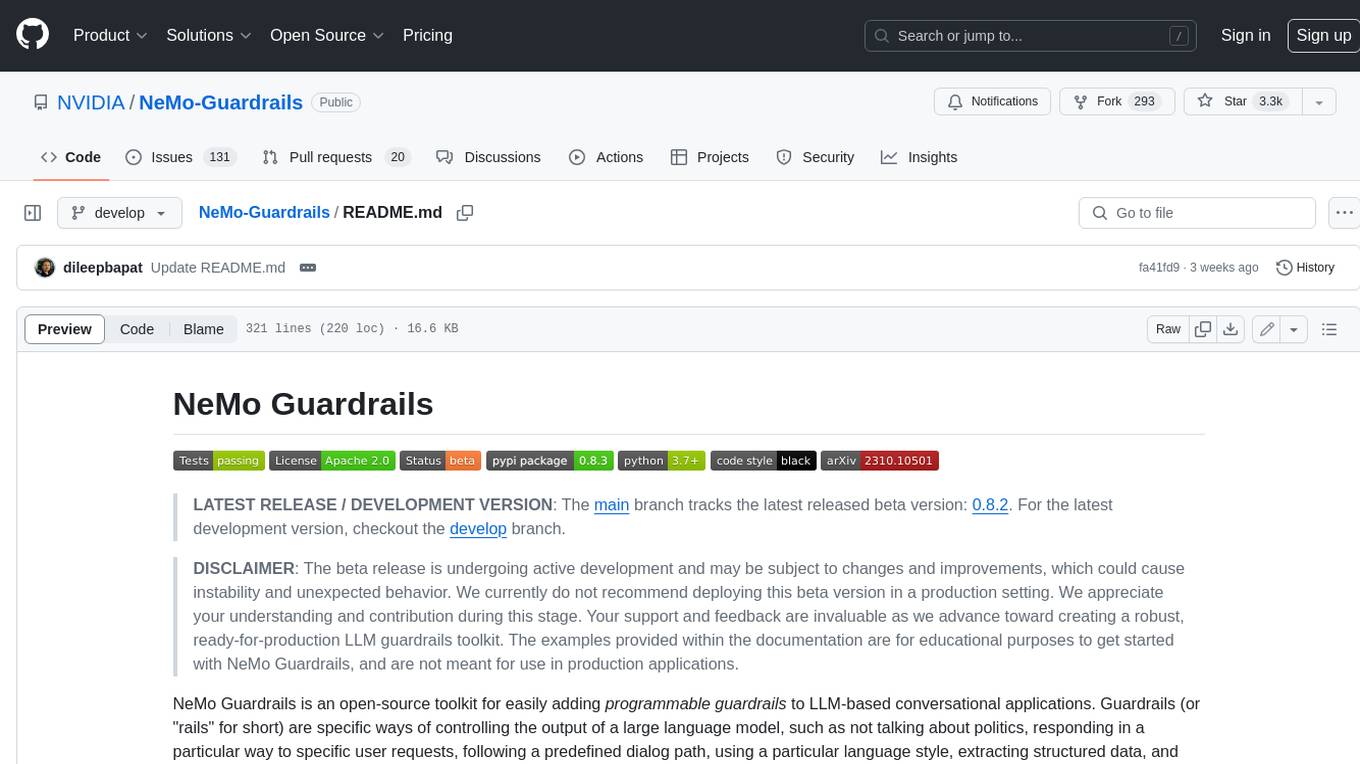
NeMo-Guardrails
NeMo Guardrails is an open-source toolkit for easily adding _programmable guardrails_ to LLM-based conversational applications. Guardrails (or "rails" for short) are specific ways of controlling the output of a large language model, such as not talking about politics, responding in a particular way to specific user requests, following a predefined dialog path, using a particular language style, extracting structured data, and more.
For similar tasks
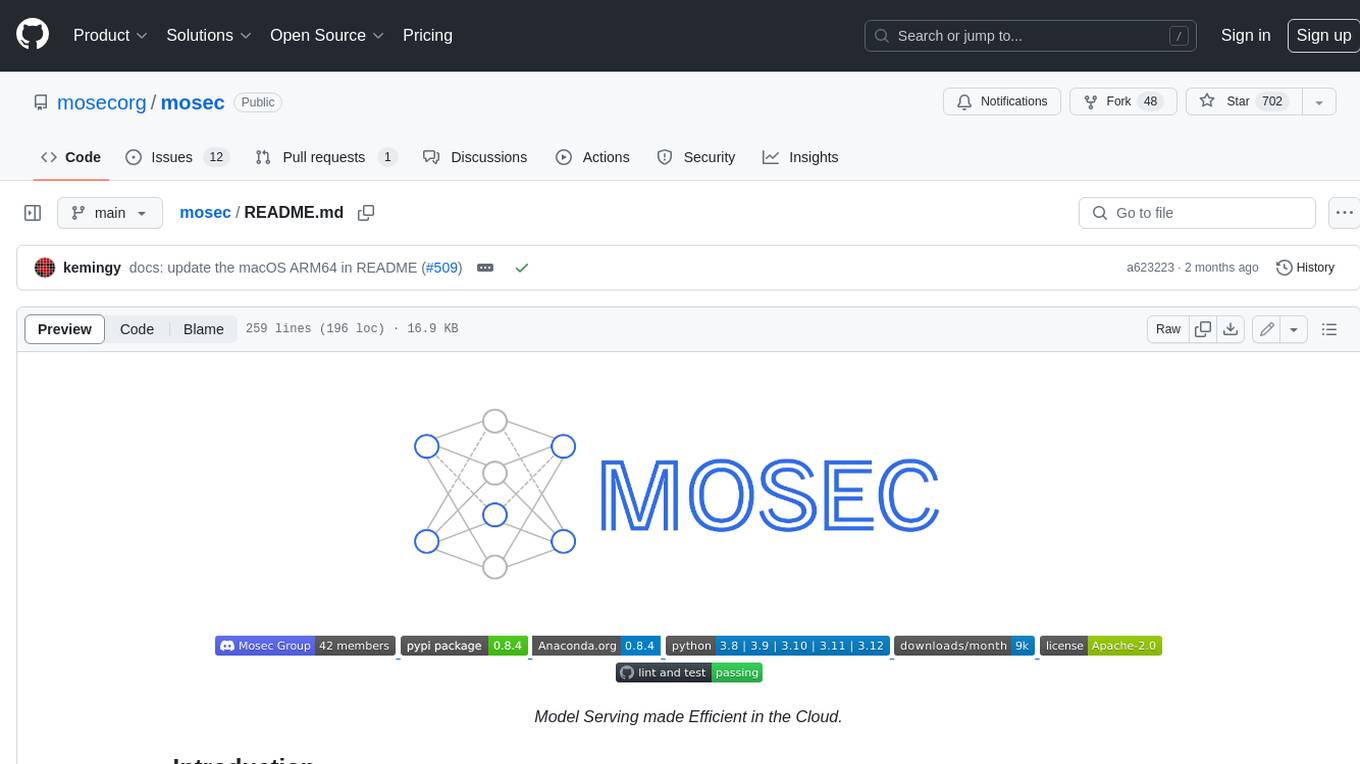
mosec
Mosec is a high-performance and flexible model serving framework for building ML model-enabled backend and microservices. It bridges the gap between any machine learning models you just trained and the efficient online service API. * **Highly performant** : web layer and task coordination built with Rust π¦, which offers blazing speed in addition to efficient CPU utilization powered by async I/O * **Ease of use** : user interface purely in Python π, by which users can serve their models in an ML framework-agnostic manner using the same code as they do for offline testing * **Dynamic batching** : aggregate requests from different users for batched inference and distribute results back * **Pipelined stages** : spawn multiple processes for pipelined stages to handle CPU/GPU/IO mixed workloads * **Cloud friendly** : designed to run in the cloud, with the model warmup, graceful shutdown, and Prometheus monitoring metrics, easily managed by Kubernetes or any container orchestration systems * **Do one thing well** : focus on the online serving part, users can pay attention to the model optimization and business logic
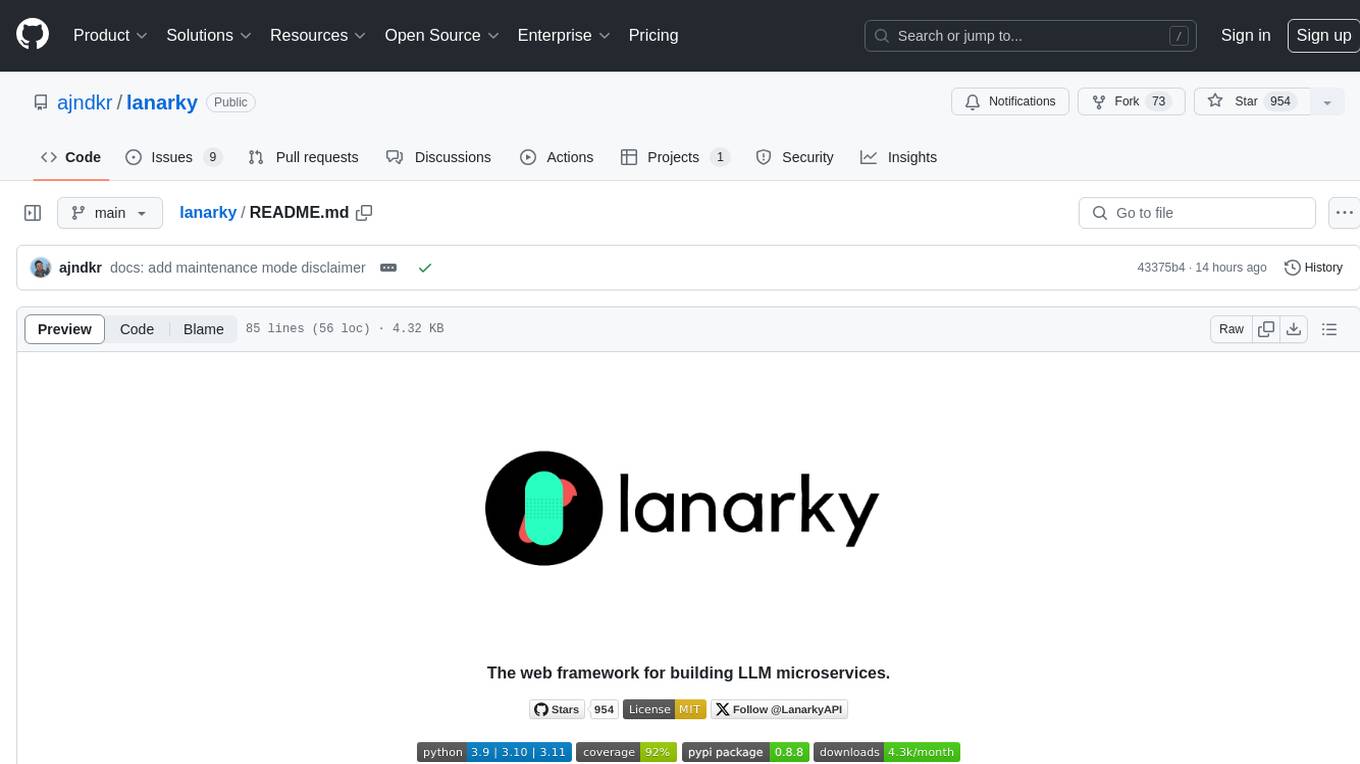
lanarky
Lanarky is a Python web framework designed for building microservices using Large Language Models (LLMs). It is LLM-first, fast, modern, supports streaming over HTTP and WebSockets, and is open-source. The framework provides an abstraction layer for developers to easily create LLM microservices. Lanarky guarantees zero vendor lock-in and is free to use. It is built on top of FastAPI and offers features familiar to FastAPI users. The project is now in maintenance mode, with no active development planned, but community contributions are encouraged.
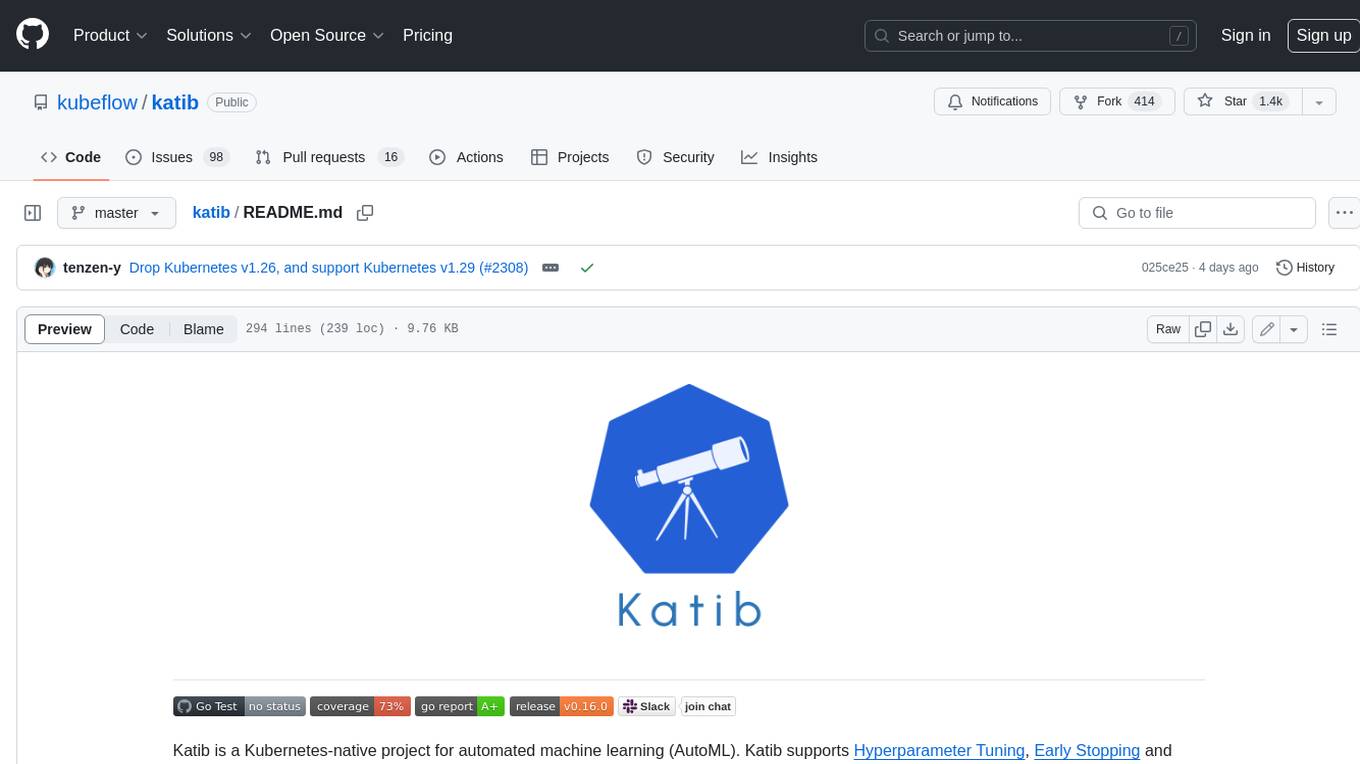
katib
Katib is a Kubernetes-native project for automated machine learning (AutoML). Katib supports Hyperparameter Tuning, Early Stopping and Neural Architecture Search. Katib is the project which is agnostic to machine learning (ML) frameworks. It can tune hyperparameters of applications written in any language of the usersβ choice and natively supports many ML frameworks, such as TensorFlow, Apache MXNet, PyTorch, XGBoost, and others. Katib can perform training jobs using any Kubernetes Custom Resources with out of the box support for Kubeflow Training Operator, Argo Workflows, Tekton Pipelines and many more.
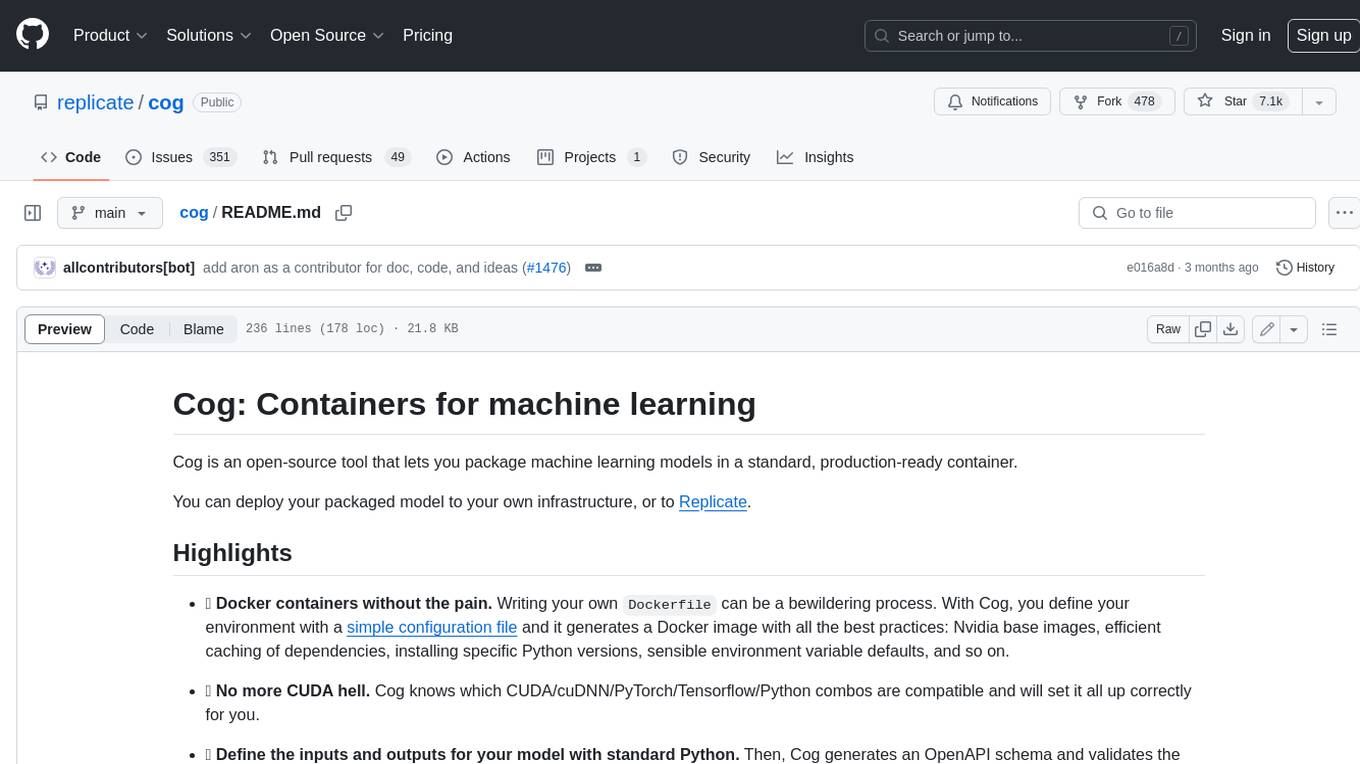
cog
Cog is an open-source tool that lets you package machine learning models in a standard, production-ready container. You can deploy your packaged model to your own infrastructure, or to Replicate.
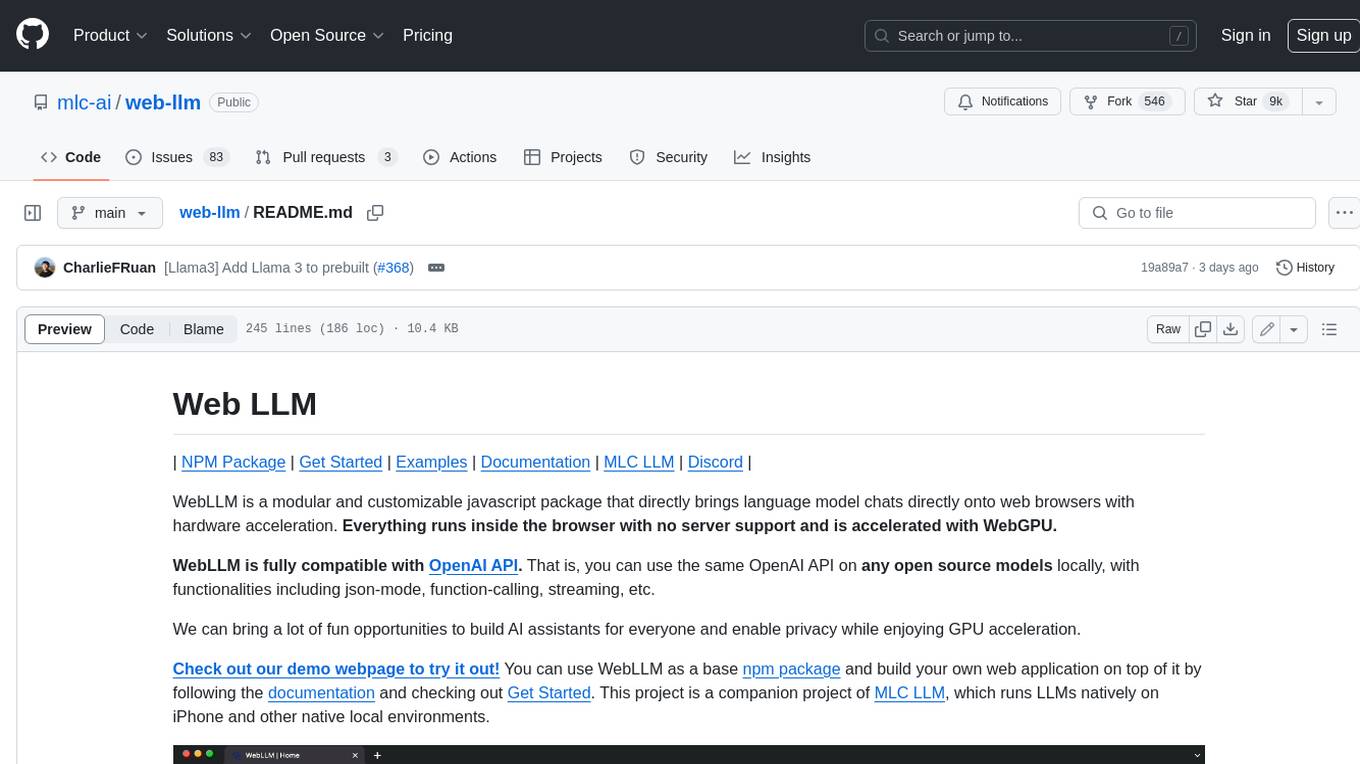
web-llm
WebLLM is a modular and customizable javascript package that directly brings language model chats directly onto web browsers with hardware acceleration. Everything runs inside the browser with no server support and is accelerated with WebGPU. WebLLM is fully compatible with OpenAI API. That is, you can use the same OpenAI API on any open source models locally, with functionalities including json-mode, function-calling, streaming, etc. We can bring a lot of fun opportunities to build AI assistants for everyone and enable privacy while enjoying GPU acceleration.
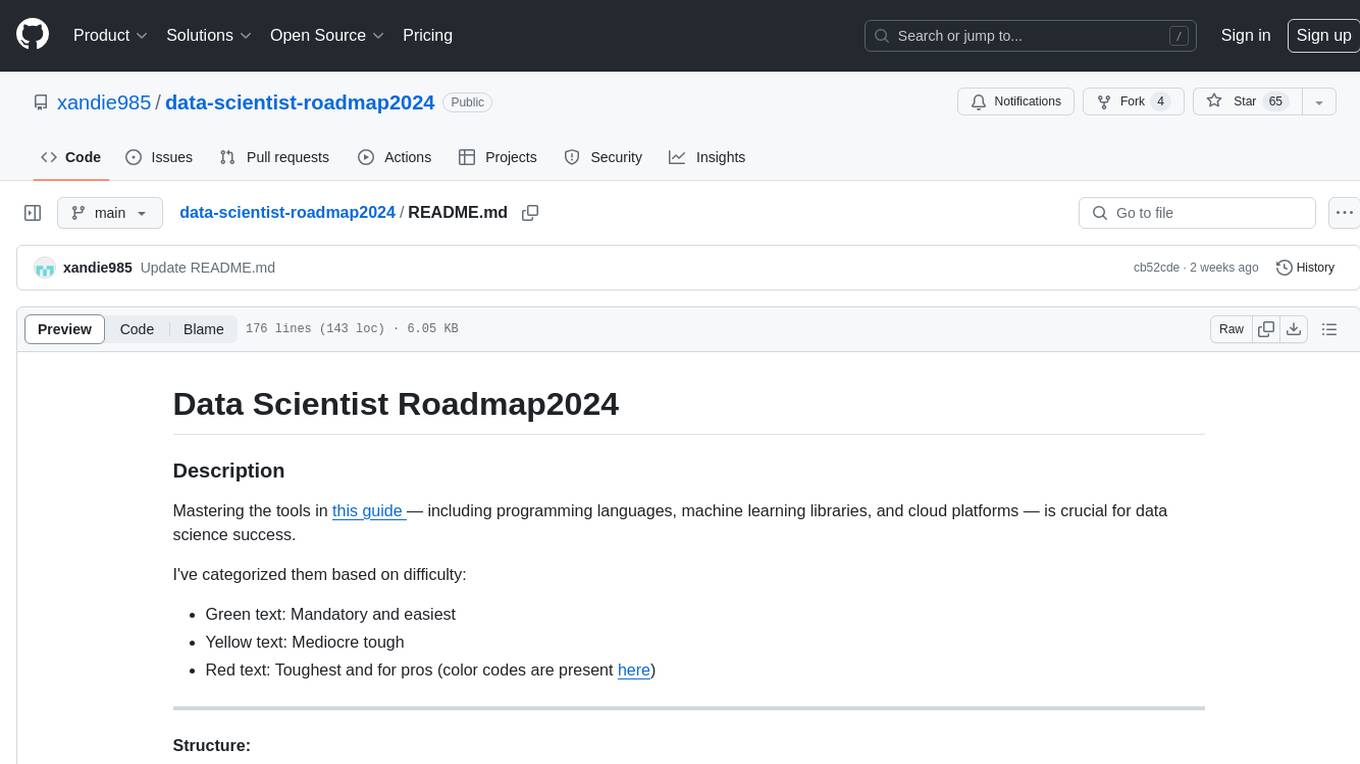
data-scientist-roadmap2024
The Data Scientist Roadmap2024 provides a comprehensive guide to mastering essential tools for data science success. It includes programming languages, machine learning libraries, cloud platforms, and concepts categorized by difficulty. The roadmap covers a wide range of topics from programming languages to machine learning techniques, data visualization tools, and DevOps/MLOps tools. It also includes web development frameworks and specific concepts like supervised and unsupervised learning, NLP, deep learning, reinforcement learning, and statistics. Additionally, it delves into DevOps tools like Airflow and MLFlow, data visualization tools like Tableau and Matplotlib, and other topics such as ETL processes, optimization algorithms, and financial modeling.
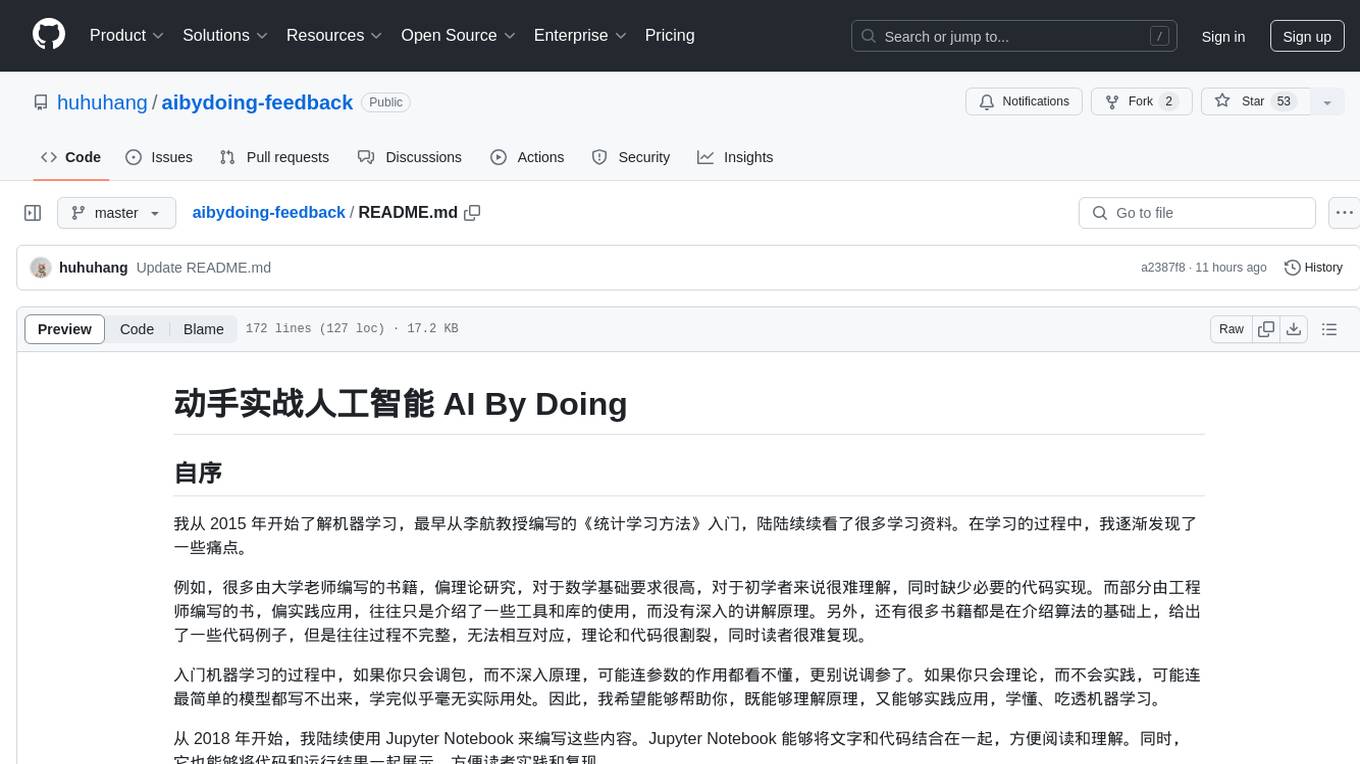
aibydoing-feedback
AI By Doing is a hands-on artificial intelligence tutorial series that aims to help beginners understand the principles of machine learning and deep learning while providing practical applications. The content covers various supervised and unsupervised learning algorithms, machine learning engineering, deep learning fundamentals, frameworks like TensorFlow and PyTorch, and applications in computer vision and natural language processing. The tutorials are written in Jupyter Notebook format, combining theory, mathematical derivations, and Python code implementations to facilitate learning and understanding.
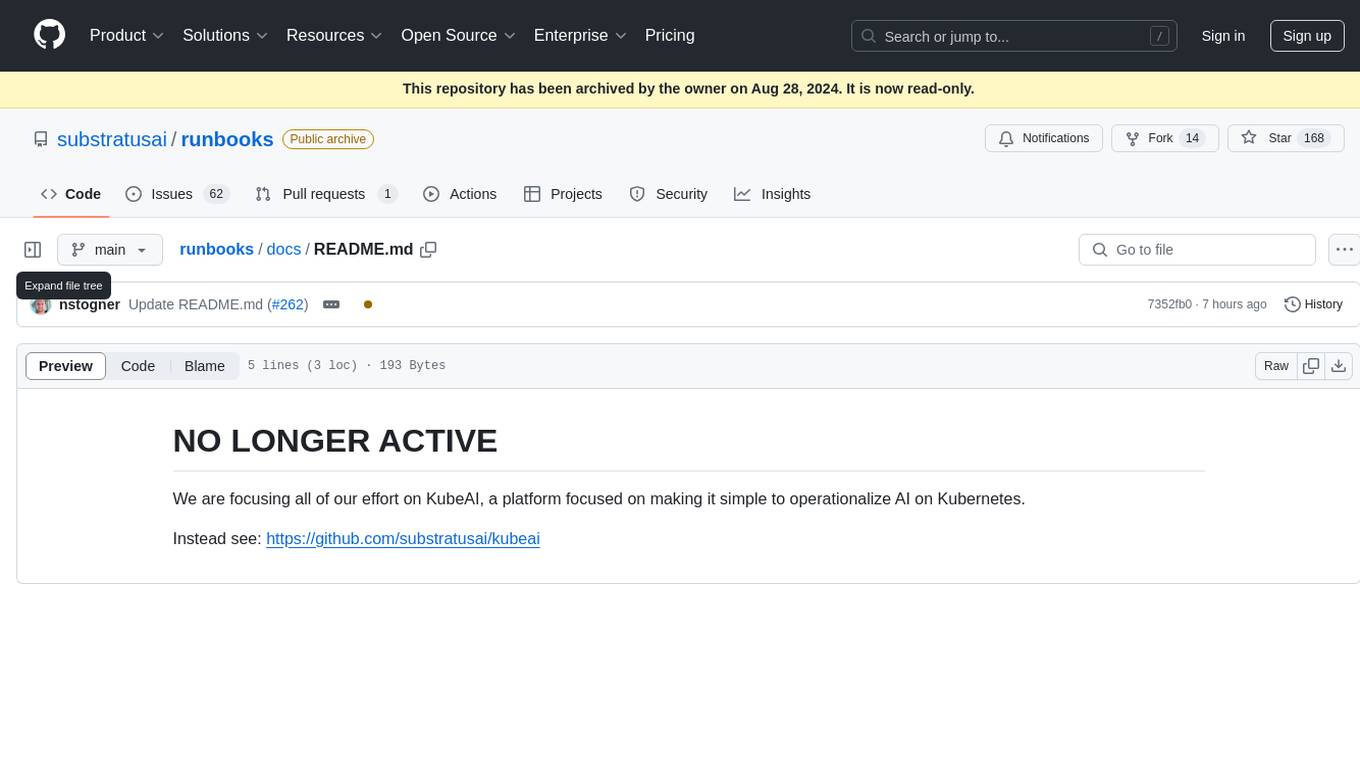
runbooks
Runbooks is a repository that is no longer active. The project has been deprecated in favor of KubeAI, a platform designed to simplify the operationalization of AI on Kubernetes. For more information, please refer to the new repository at https://github.com/substratusai/kubeai.
For similar jobs
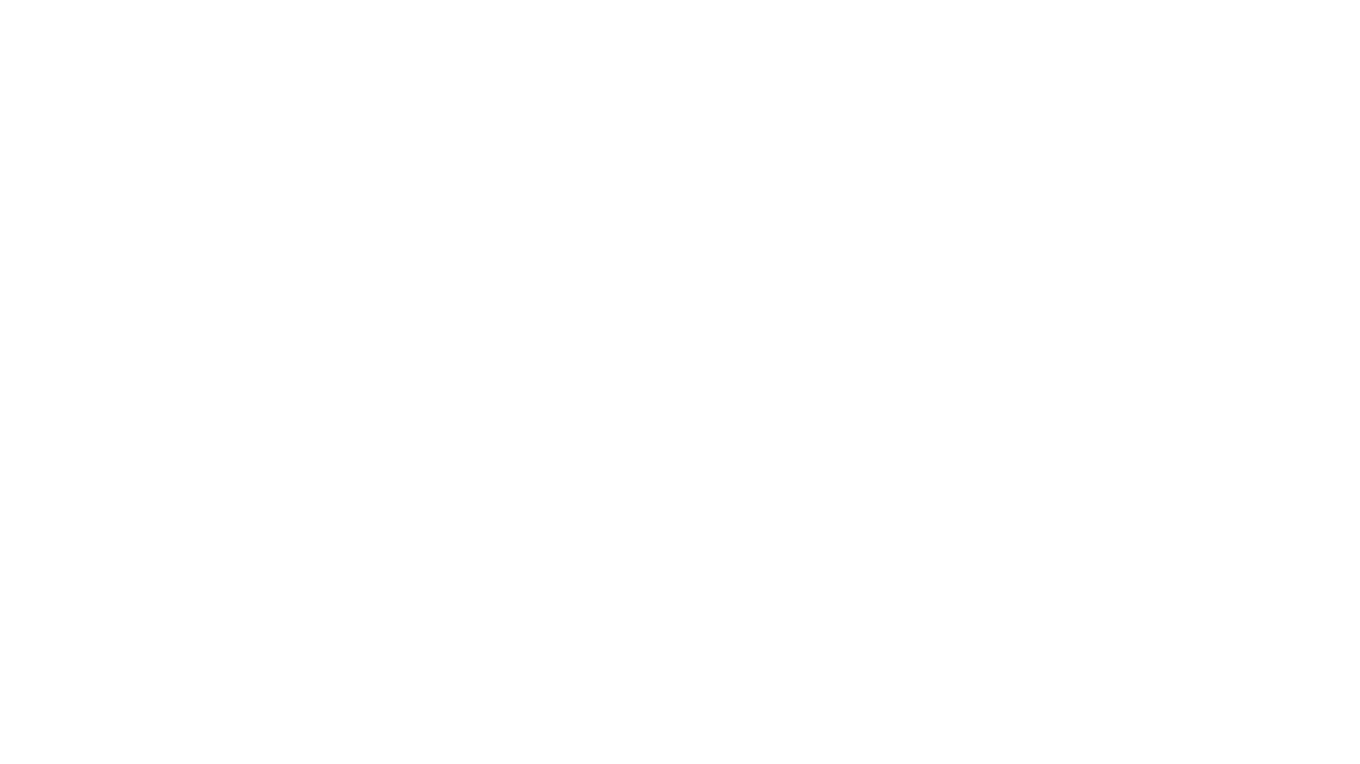
AirGo
AirGo is a front and rear end separation, multi user, multi protocol proxy service management system, simple and easy to use. It supports vless, vmess, shadowsocks, and hysteria2.
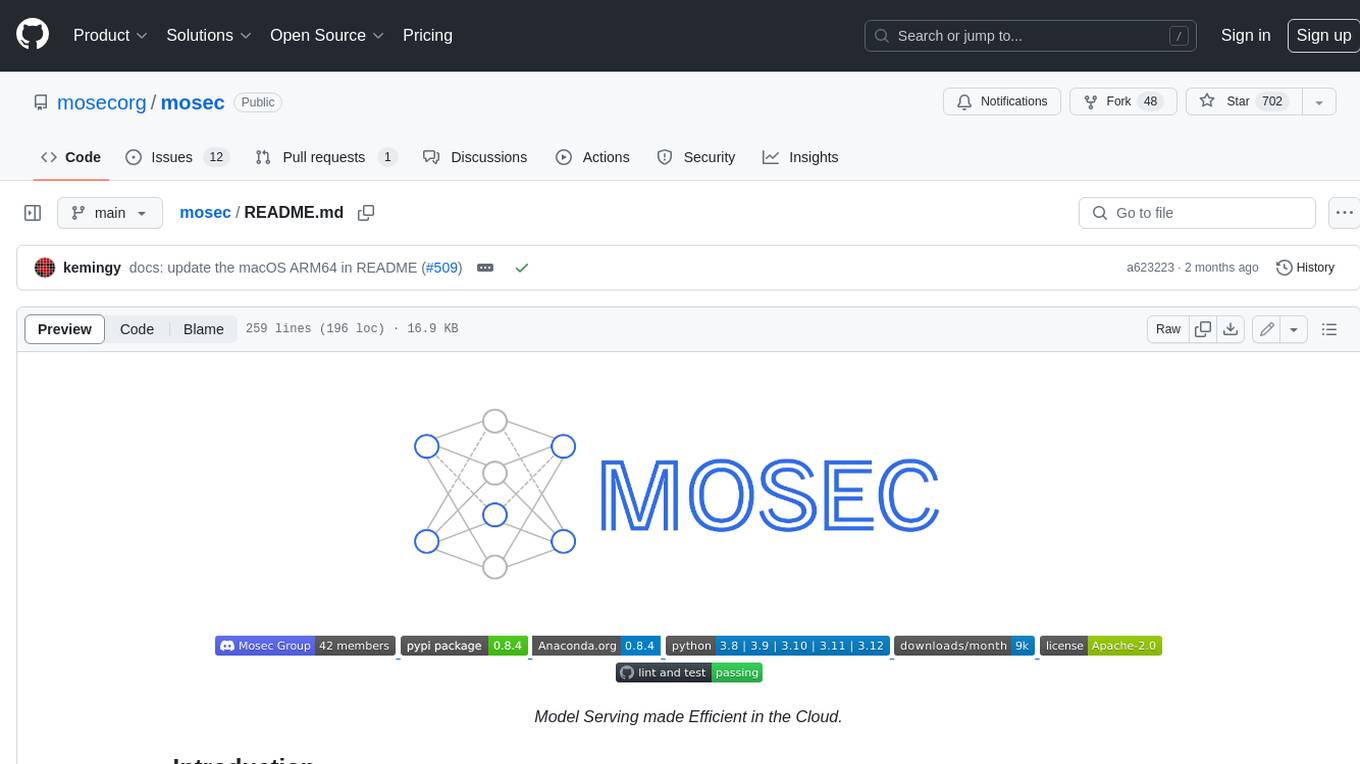
mosec
Mosec is a high-performance and flexible model serving framework for building ML model-enabled backend and microservices. It bridges the gap between any machine learning models you just trained and the efficient online service API. * **Highly performant** : web layer and task coordination built with Rust π¦, which offers blazing speed in addition to efficient CPU utilization powered by async I/O * **Ease of use** : user interface purely in Python π, by which users can serve their models in an ML framework-agnostic manner using the same code as they do for offline testing * **Dynamic batching** : aggregate requests from different users for batched inference and distribute results back * **Pipelined stages** : spawn multiple processes for pipelined stages to handle CPU/GPU/IO mixed workloads * **Cloud friendly** : designed to run in the cloud, with the model warmup, graceful shutdown, and Prometheus monitoring metrics, easily managed by Kubernetes or any container orchestration systems * **Do one thing well** : focus on the online serving part, users can pay attention to the model optimization and business logic
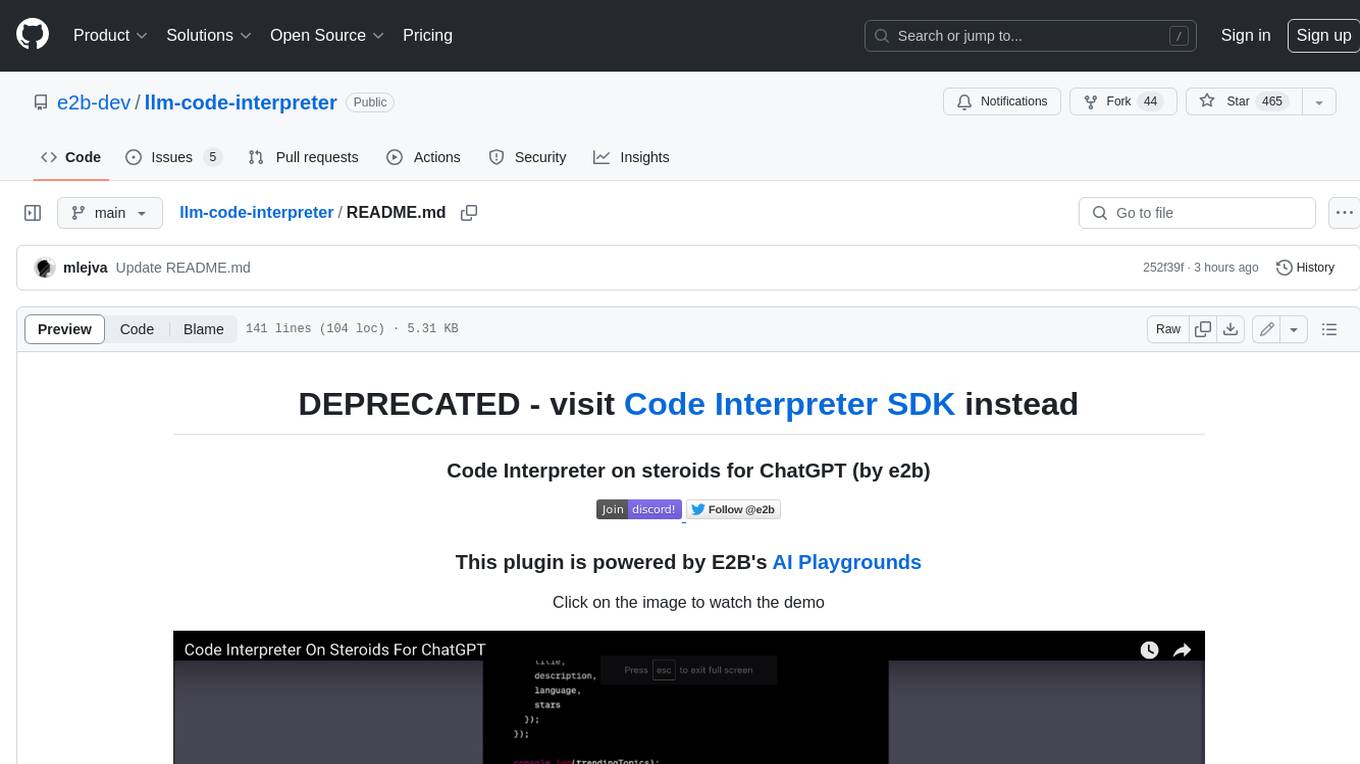
llm-code-interpreter
The 'llm-code-interpreter' repository is a deprecated plugin that provides a code interpreter on steroids for ChatGPT by E2B. It gives ChatGPT access to a sandboxed cloud environment with capabilities like running any code, accessing Linux OS, installing programs, using filesystem, running processes, and accessing the internet. The plugin exposes commands to run shell commands, read files, and write files, enabling various possibilities such as running different languages, installing programs, starting servers, deploying websites, and more. It is powered by the E2B API and is designed for agents to freely experiment within a sandboxed environment.
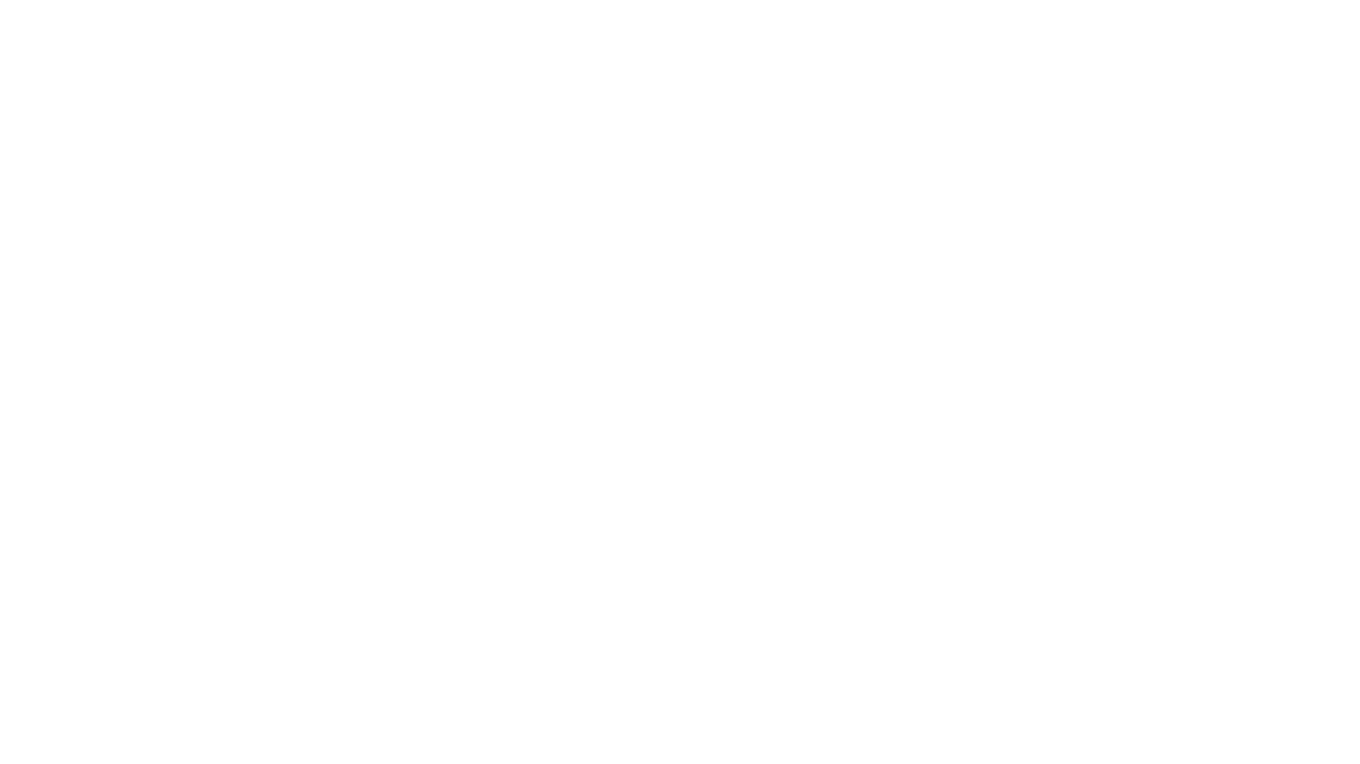
pezzo
Pezzo is a fully cloud-native and open-source LLMOps platform that allows users to observe and monitor AI operations, troubleshoot issues, save costs and latency, collaborate, manage prompts, and deliver AI changes instantly. It supports various clients for prompt management, observability, and caching. Users can run the full Pezzo stack locally using Docker Compose, with prerequisites including Node.js 18+, Docker, and a GraphQL Language Feature Support VSCode Extension. Contributions are welcome, and the source code is available under the Apache 2.0 License.
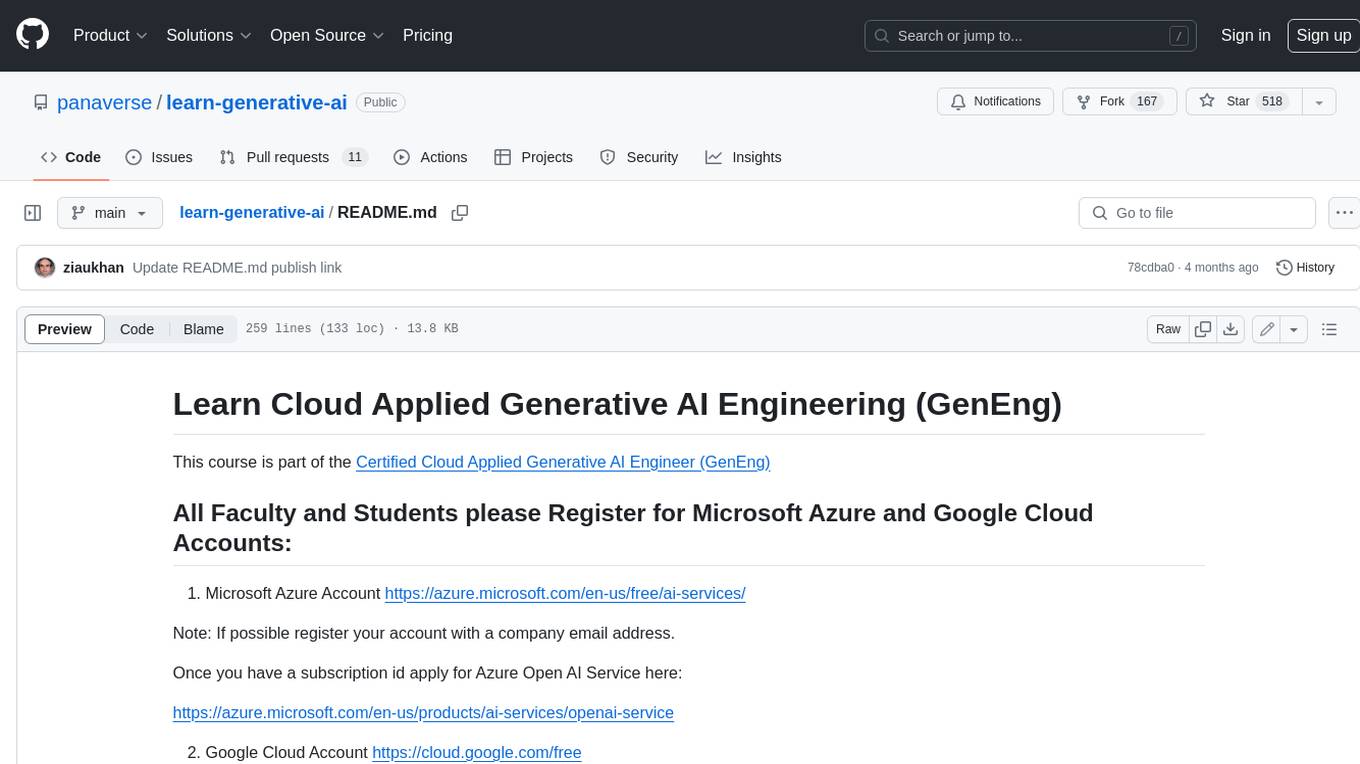
learn-generative-ai
Learn Cloud Applied Generative AI Engineering (GenEng) is a course focusing on the application of generative AI technologies in various industries. The course covers topics such as the economic impact of generative AI, the role of developers in adopting and integrating generative AI technologies, and the future trends in generative AI. Students will learn about tools like OpenAI API, LangChain, and Pinecone, and how to build and deploy Large Language Models (LLMs) for different applications. The course also explores the convergence of generative AI with Web 3.0 and its potential implications for decentralized intelligence.
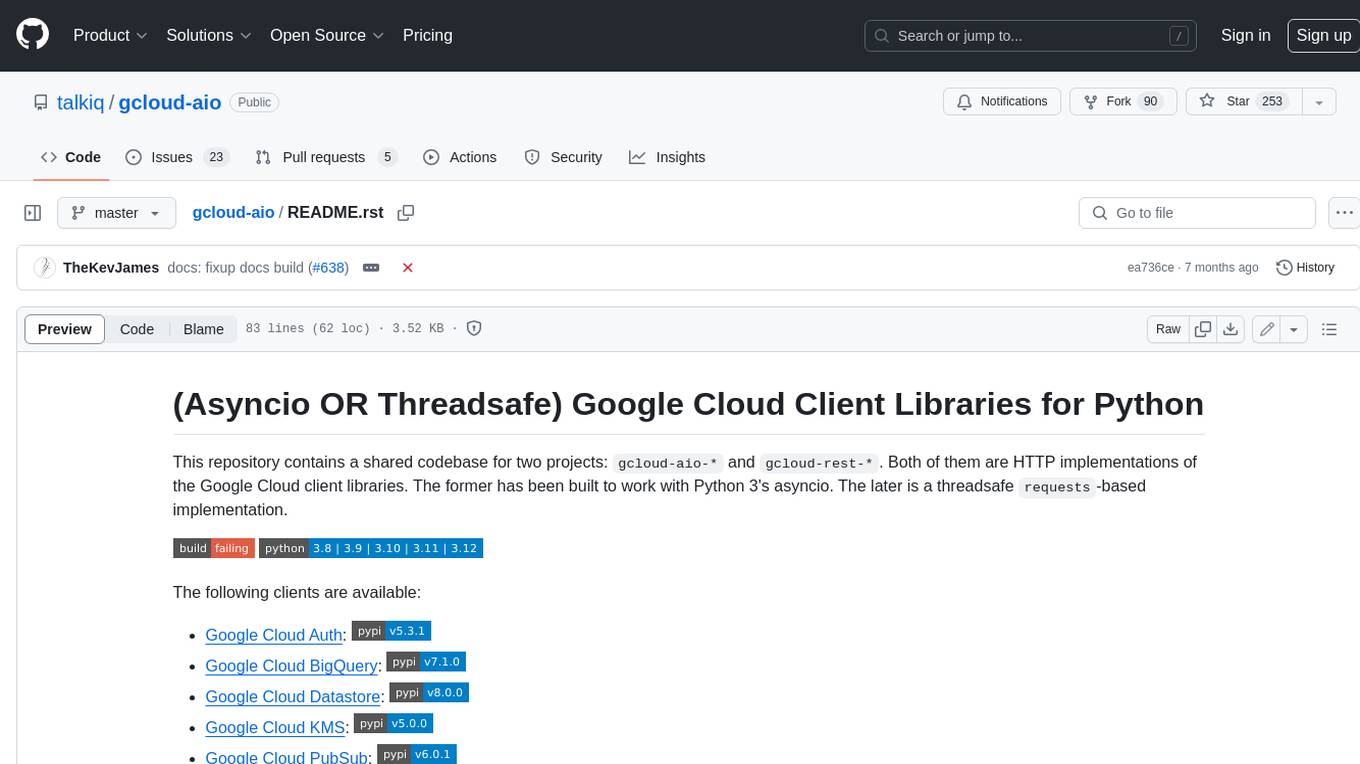
gcloud-aio
This repository contains shared codebase for two projects: gcloud-aio and gcloud-rest. gcloud-aio is built for Python 3's asyncio, while gcloud-rest is a threadsafe requests-based implementation. It provides clients for Google Cloud services like Auth, BigQuery, Datastore, KMS, PubSub, Storage, and Task Queue. Users can install the library using pip and refer to the documentation for usage details. Developers can contribute to the project by following the contribution guide.
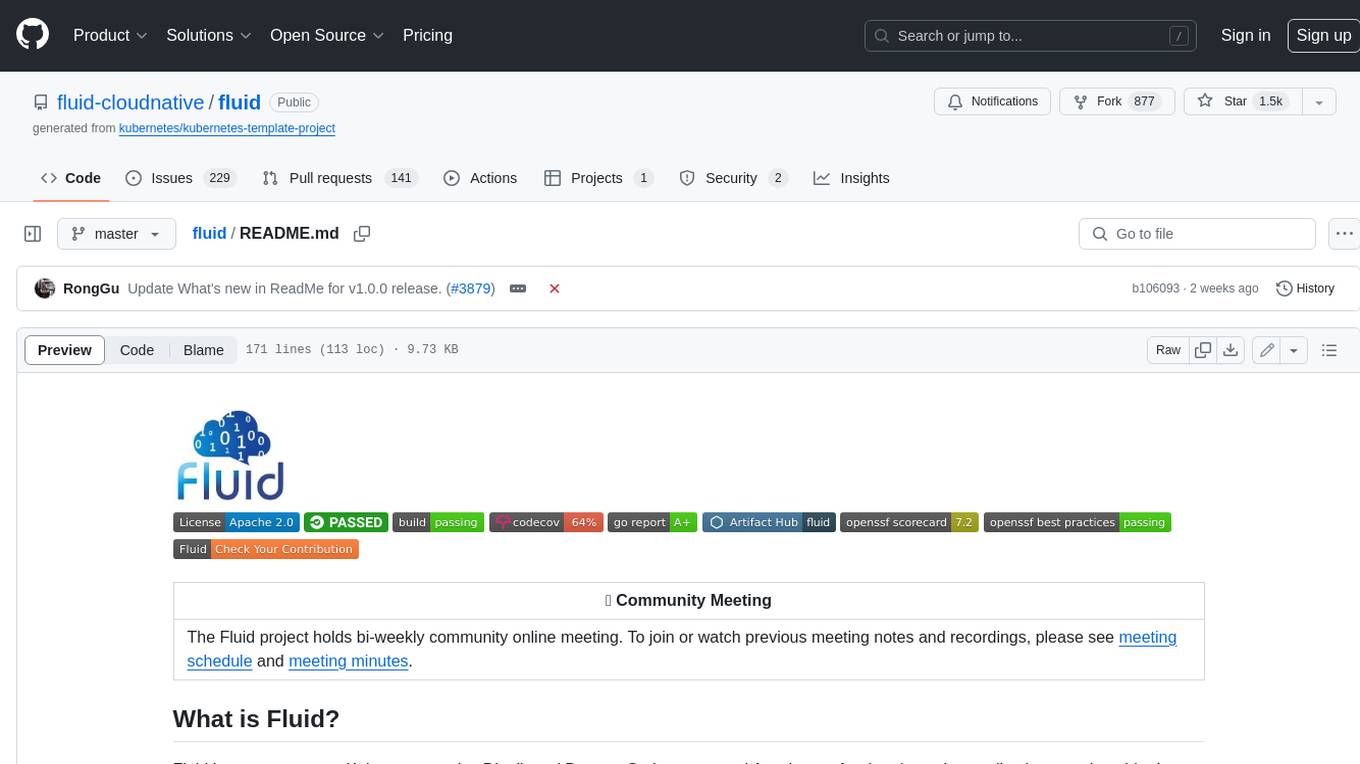
fluid
Fluid is an open source Kubernetes-native Distributed Dataset Orchestrator and Accelerator for data-intensive applications, such as big data and AI applications. It implements dataset abstraction, scalable cache runtime, automated data operations, elasticity and scheduling, and is runtime platform agnostic. Key concepts include Dataset and Runtime. Prerequisites include Kubernetes version > 1.16, Golang 1.18+, and Helm 3. The tool offers features like accelerating remote file accessing, machine learning, accelerating PVC, preloading dataset, and on-the-fly dataset cache scaling. Contributions are welcomed, and the project is under the Apache 2.0 license with a vendor-neutral approach.
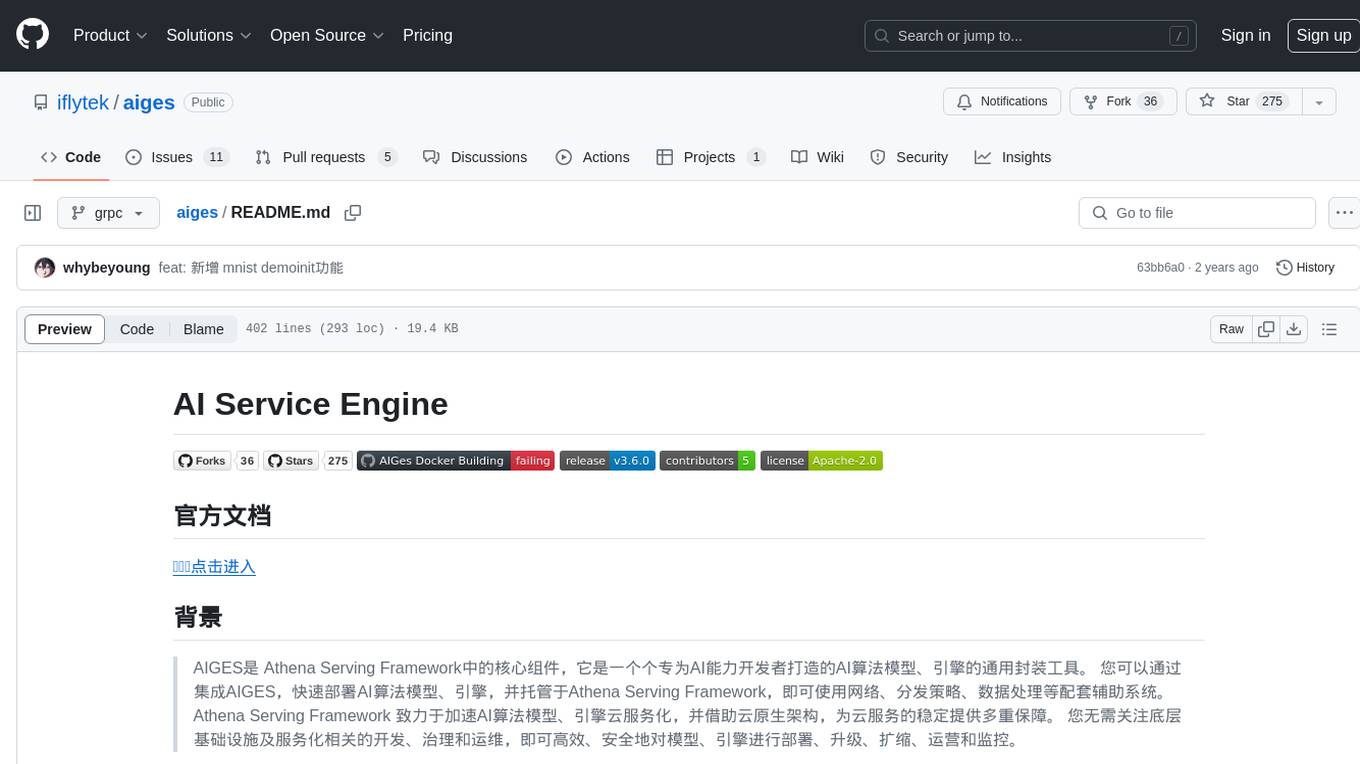
aiges
AIGES is a core component of the Athena Serving Framework, designed as a universal encapsulation tool for AI developers to deploy AI algorithm models and engines quickly. By integrating AIGES, you can deploy AI algorithm models and engines rapidly and host them on the Athena Serving Framework, utilizing supporting auxiliary systems for networking, distribution strategies, data processing, etc. The Athena Serving Framework aims to accelerate the cloud service of AI algorithm models and engines, providing multiple guarantees for cloud service stability through cloud-native architecture. You can efficiently and securely deploy, upgrade, scale, operate, and monitor models and engines without focusing on underlying infrastructure and service-related development, governance, and operations.