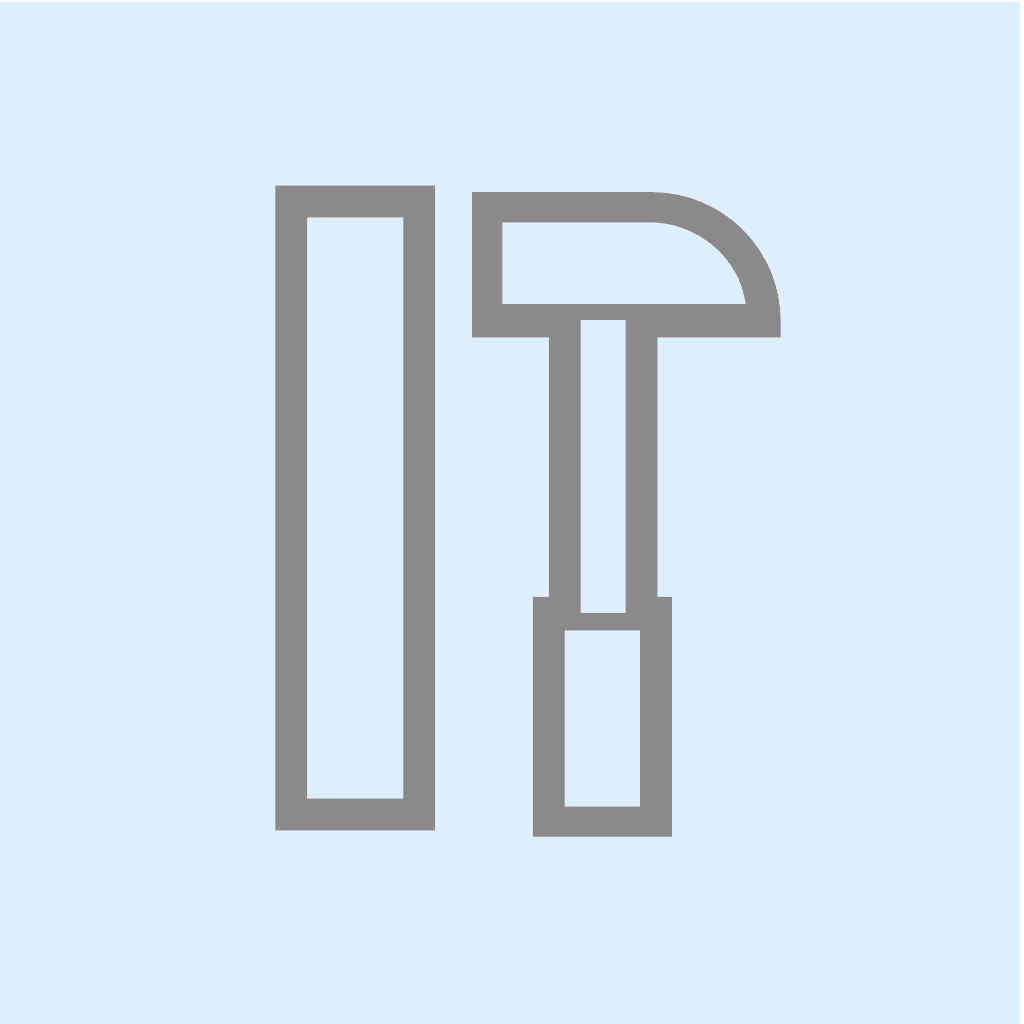
lmql
A language for constraint-guided and efficient LLM programming.
Stars: 3413
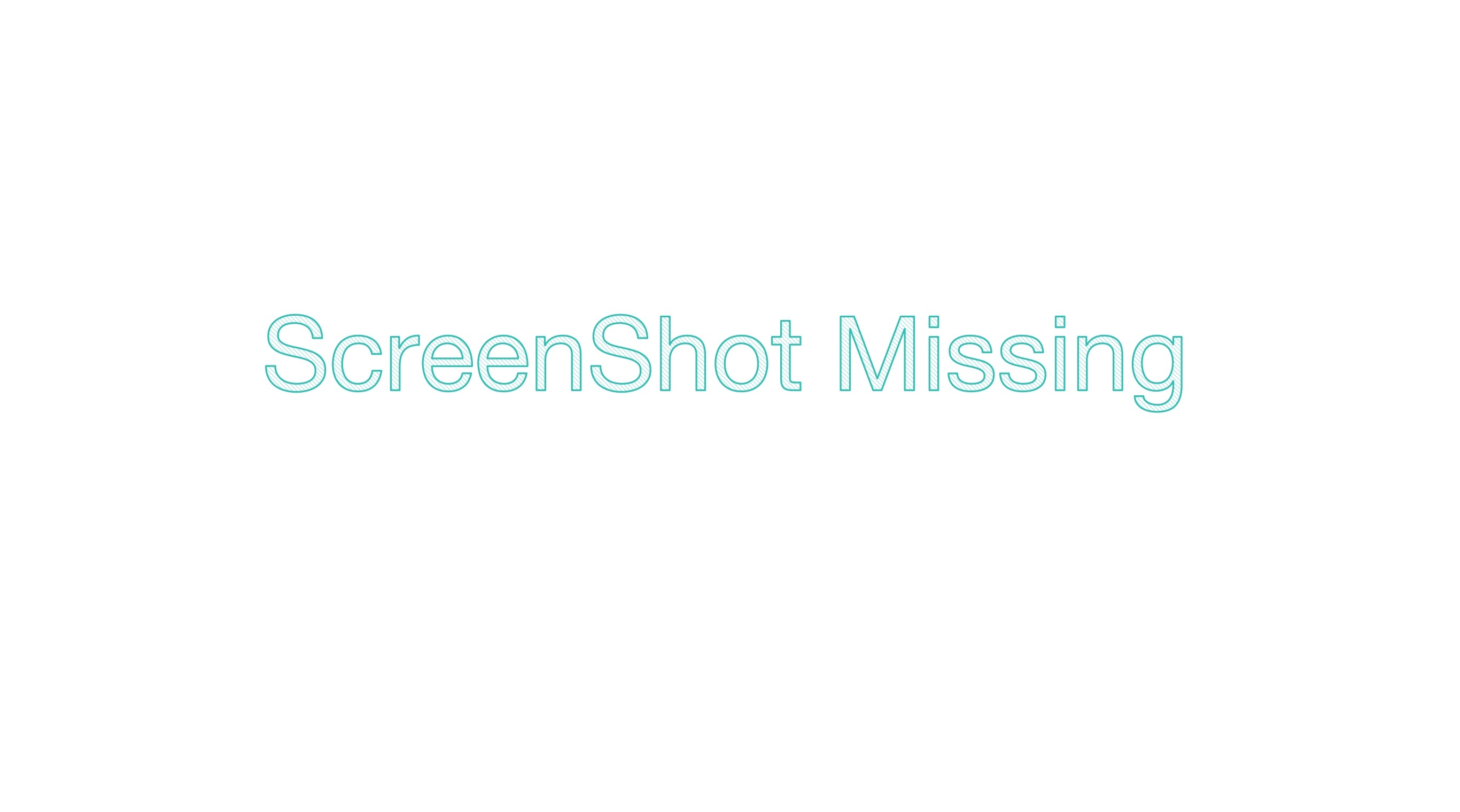
LMQL is a programming language designed for large language models (LLMs) that offers a unique way of integrating traditional programming with LLM interaction. It allows users to write programs that combine algorithmic logic with LLM calls, enabling model reasoning capabilities within the context of the program. LMQL provides features such as Python syntax integration, rich control-flow options, advanced decoding techniques, powerful constraints via logit masking, runtime optimization, sync and async API support, multi-model compatibility, and extensive applications like JSON decoding and interactive chat interfaces. The tool also offers library integration, flexible tooling, and output streaming options for easy model output handling.
README:
A programming language for large language models.
Documentation »
Explore Examples
·
Playground IDE
·
Report Bug
LMQL is a programming language for large language models (LLMs) based on a superset of Python. LMQL offers a novel way of interweaving traditional programming with the ability to call LLMs in your code. It goes beyond traditional templating languages by integrating LLM interaction natively at the level of your program code.
![]()
Help us shape the next major version of LMQL by filling out the LMQL developer survey: https://forms.gle/pGvAicNpUhS1rAkK9
An LMQL program reads like standard Python, but top-level strings are interpreted as query strings: They are passed to an LLM, where template variables like [GREETINGS]
are automatically completed by the model:
"Greet LMQL:[GREETINGS]\n" where stops_at(GREETINGS, ".") and not "\n" in GREETINGS
if "Hi there" in GREETINGS:
"Can you reformulate your greeting in the speech of \
victorian-era English: [VIC_GREETINGS]\n" where stops_at(VIC_GREETINGS, ".")
"Analyse what part of this response makes it typically victorian:\n"
for i in range(4):
"-[THOUGHT]\n" where stops_at(THOUGHT, ".")
"To summarize:[SUMMARY]"
Program Output:
LMQL allows you to express programs that contain both, traditional algorithmic logic, and LLM calls. At any point during execution, you can prompt an LLM on program variables in combination with standard natural language prompting, to leverage model reasoning capabilities in the context of your program.
To better control LLM behavior, you can use the where
keyword to specify constraints and data types of the generated text. This enables guidance of the model's reasoning process, and constraining of intermediate outputs using an expressive constraint language.
Beyond this linear form of scripting, LMQL also supports a number of decoding algorithms to execute your program, such as argmax
, sample
or even advanced branching decoders like beam search and best_k
.
Learn more about LMQL by exploring thne Example Showcase, by running your own programs in our browser-based Playground IDE or by reading the documentation.
LMQL is designed to make working with language models like OpenAI and 🤗 Transformers more efficient and powerful through its advanced functionality, including multi-variable templates, conditional distributions, constraints, datatypes and control flow.
- [X] Python Syntax: Write your queries using familiar Python syntax, fully integrated with your Python environment (classes, variable captures, etc.)
- [X] Rich Control-Flow: LMQL offers full Python support, enabling powerful control flow and logic in your prompting logic.
- [X] Advanced Decoding: Take advantage of advanced decoding techniques like beam search, best_k, and more.
- [X] Powerful Constraints Via Logit Masking: Apply constraints to model output, e.g. to specify token length, character-level constraints, datatype and stopping phrases to get more control of model behavior.
- [X] Optimizing Runtime: LMQL leverages speculative execution to enable faster inference, constraint short-circuiting, more efficient token use and tree-based caching.
- [X] Sync and Async API: Execute hundreds of queries in parallel with LMQL's asynchronous API, which enables cross-query batching.
- [X] Multi-Model Support: Seamlessly use LMQL with OpenAI API, Azure OpenAI, and 🤗 Transformers models.
- [X] Extensive Applications: Use LMQL to implement advanced applications like schema-safe JSON decoding, algorithmic prompting, interactive chat interfaces, and inline tool use.
- [X] Library Integration: Easily employ LMQL in your existing stack leveraging LangChain or LlamaIndex.
- [X] Flexible Tooling: Enjoy an interactive development experience with LMQL's Interactive Playground IDE, and Visual Studio Code Extension.
- [X] Output Streaming: Stream model output easily via WebSocket, REST endpoint, or Server-Sent Event streaming.
To install the latest version of LMQL run the following command with Python ==3.10 installed.
pip install lmql
Local GPU Support: If you want to run models on a local GPU, make sure to install LMQL in an environment with a GPU-enabled installation of PyTorch >= 1.11 (cf. https://pytorch.org/get-started/locally/) and install via pip install lmql[hf]
.
After installation, you can launch the LMQL playground IDE with the following command:
lmql playground
Using the LMQL playground requires an installation of Node.js. If you are in a conda-managed environment you can install node.js via
conda install nodejs=14.20 -c conda-forge
. Otherwise, please see the official Node.js website https://nodejs.org/en/download/ for instructions how to install it on your system.
This launches a browser-based playground IDE, including a showcase of many exemplary LMQL programs. If the IDE does not launch automatically, go to http://localhost:3000
.
Alternatively, lmql run
can be used to execute local .lmql
files. Note that when using local HuggingFace Transformers models in the Playground IDE or via lmql run
, you have to first launch an instance of the LMQL Inference API for the corresponding model via the command lmql serve-model
.
If you want to use OpenAI models, you have to configure your API credentials. To do so you can either define the OPENAI_API_KEY
environment variable or create a file api.env
in the active working directory, with the following contents:
openai-org: <org identifier>
openai-secret: <api secret>
For system-wide configuration, you can also create an api.env
file at $HOME/.lmql/api.env
or at the project root of your LMQL distribution (e.g. src/
in a development copy).
Alternatively, you can use LMQL-specific env variables LMQL_OPENAI_SECRET
and LMQL_OPENAI_ORG
.
To install the latest (bleeding-edge) version of LMQL, you can also run the following command:
pip install git+https://github.com/eth-sri/lmql
This will install the lmql
package directly from the main
branch of this repository. We do not continously test the main
version, so it may be less stable than the latest PyPI release.
LMQL is a community-centric project. If you are interested in contributing to LMQL, please see the contributing guidelines for more information, and reach out to us via Discord. We are looking forward to your contributions!
To setup a conda
environment for local LMQL development with GPU support, run the following commands:
# prepare conda environment
conda env create -f scripts/conda/requirements.yml -n lmql
conda activate lmql
# registers the `lmql` command in the current shell
source scripts/activate-dev.sh
Operating System: The GPU-enabled version of LMQL was tested to work on Ubuntu 22.04 with CUDA 12.0 and Windows 10 via WSL2 and CUDA 11.7. The no-GPU version (see below) was tested to work on Ubuntu 22.04 and macOS 13.2 Ventura or Windows 10 via WSL2.
This section outlines how to setup an LMQL development environment without local GPU support. Note that LMQL without local GPU support only supports the use of API-integrated models like openai/text-davinci-003
. Please see the OpenAI API documentation (https://platform.openai.com/docs/models/gpt-3-5) to learn more about the set of available models.
To setup a conda
environment for LMQL with no GPU support, run the following commands:
# prepare conda environment
conda env create -f scripts/conda/requirements-no-gpu.yml -n lmql-no-gpu
conda activate lmql-no-gpu
# registers the `lmql` command in the current shell
source scripts/activate-dev.sh
For Tasks:
Click tags to check more tools for each tasksFor Jobs:
Alternative AI tools for lmql
Similar Open Source Tools
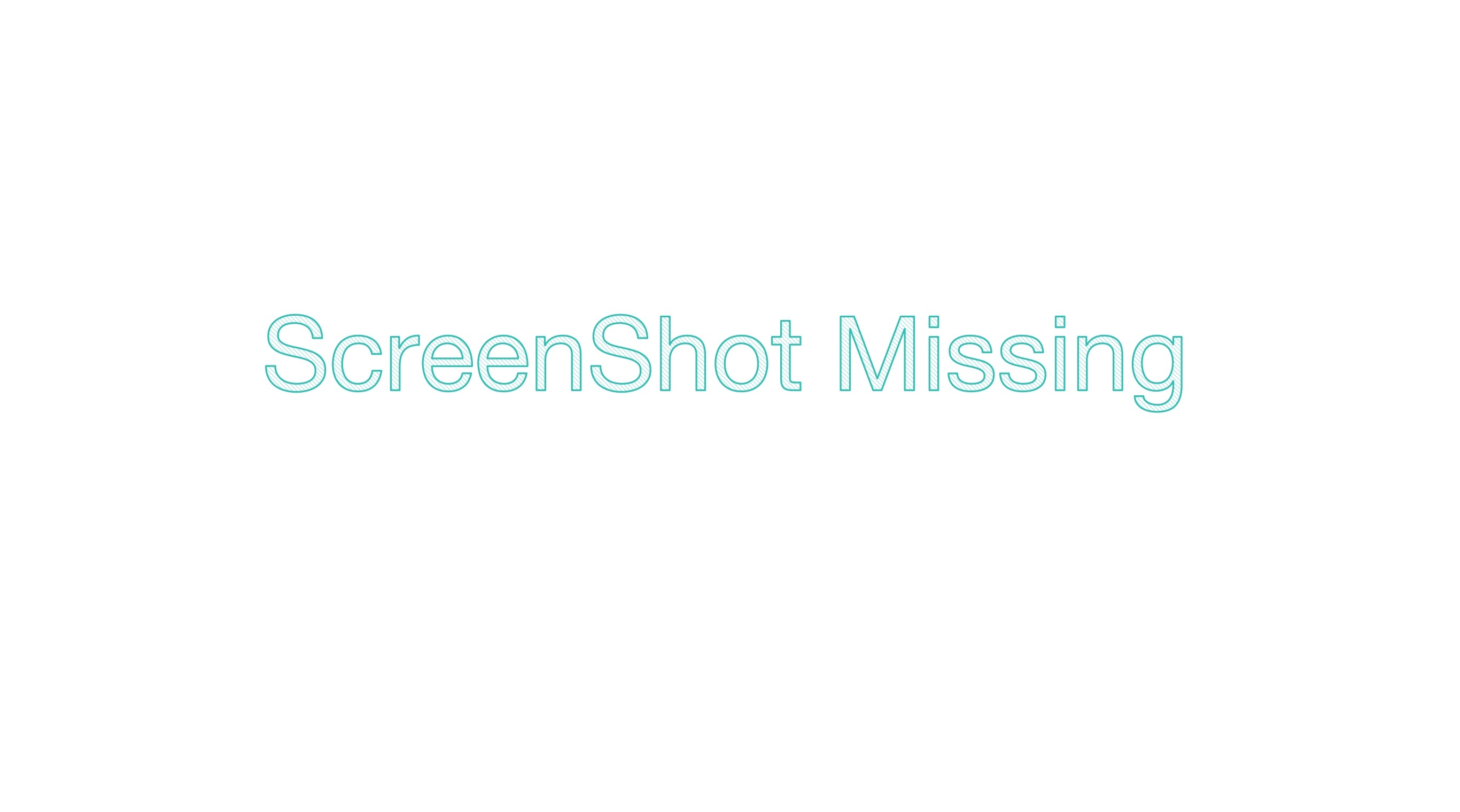
lmql
LMQL is a programming language designed for large language models (LLMs) that offers a unique way of integrating traditional programming with LLM interaction. It allows users to write programs that combine algorithmic logic with LLM calls, enabling model reasoning capabilities within the context of the program. LMQL provides features such as Python syntax integration, rich control-flow options, advanced decoding techniques, powerful constraints via logit masking, runtime optimization, sync and async API support, multi-model compatibility, and extensive applications like JSON decoding and interactive chat interfaces. The tool also offers library integration, flexible tooling, and output streaming options for easy model output handling.
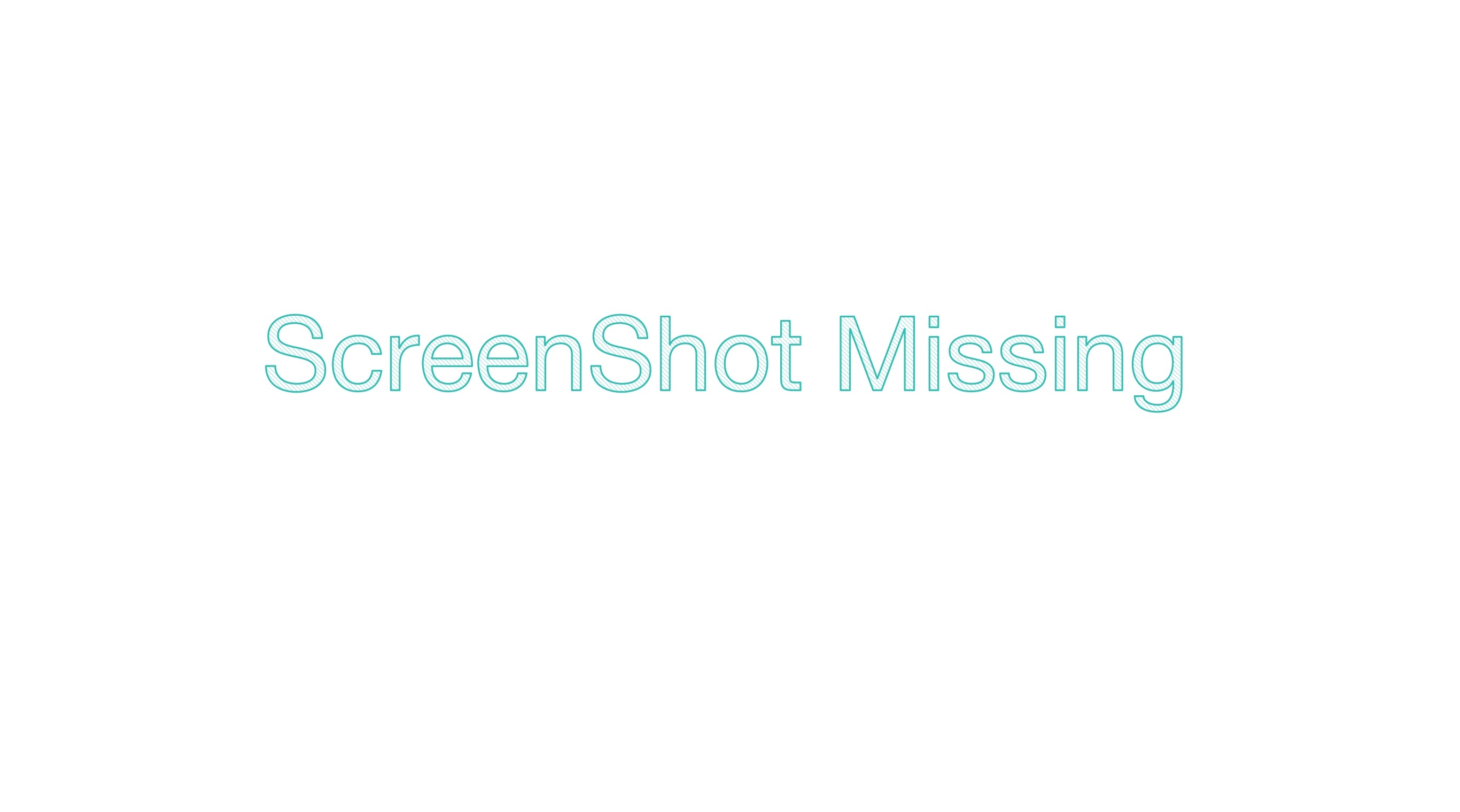
torchchat
torchchat is a codebase showcasing the ability to run large language models (LLMs) seamlessly. It allows running LLMs using Python in various environments such as desktop, server, iOS, and Android. The tool supports running models via PyTorch, chatting, generating text, running chat in the browser, and running models on desktop/server without Python. It also provides features like AOT Inductor for faster execution, running in C++ using the runner, and deploying and running on iOS and Android. The tool supports popular hardware and OS including Linux, Mac OS, Android, and iOS, with various data types and execution modes available.
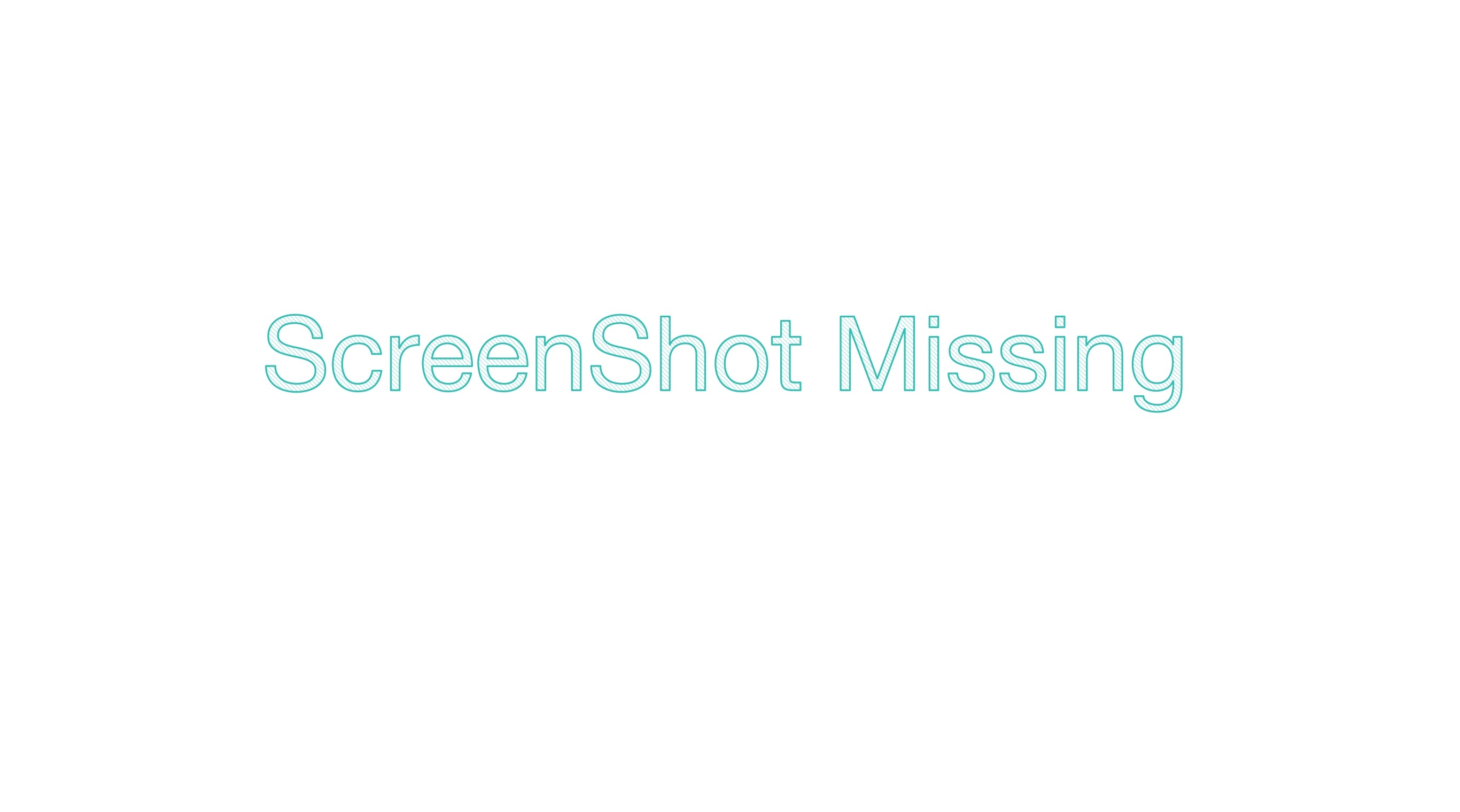
rosa
ROSA is an AI Agent designed to interact with ROS-based robotics systems using natural language queries. It can generate system reports, read and parse ROS log files, adapt to new robots, and run various ROS commands using natural language. The tool is versatile for robotics research and development, providing an easy way to interact with robots and the ROS environment.
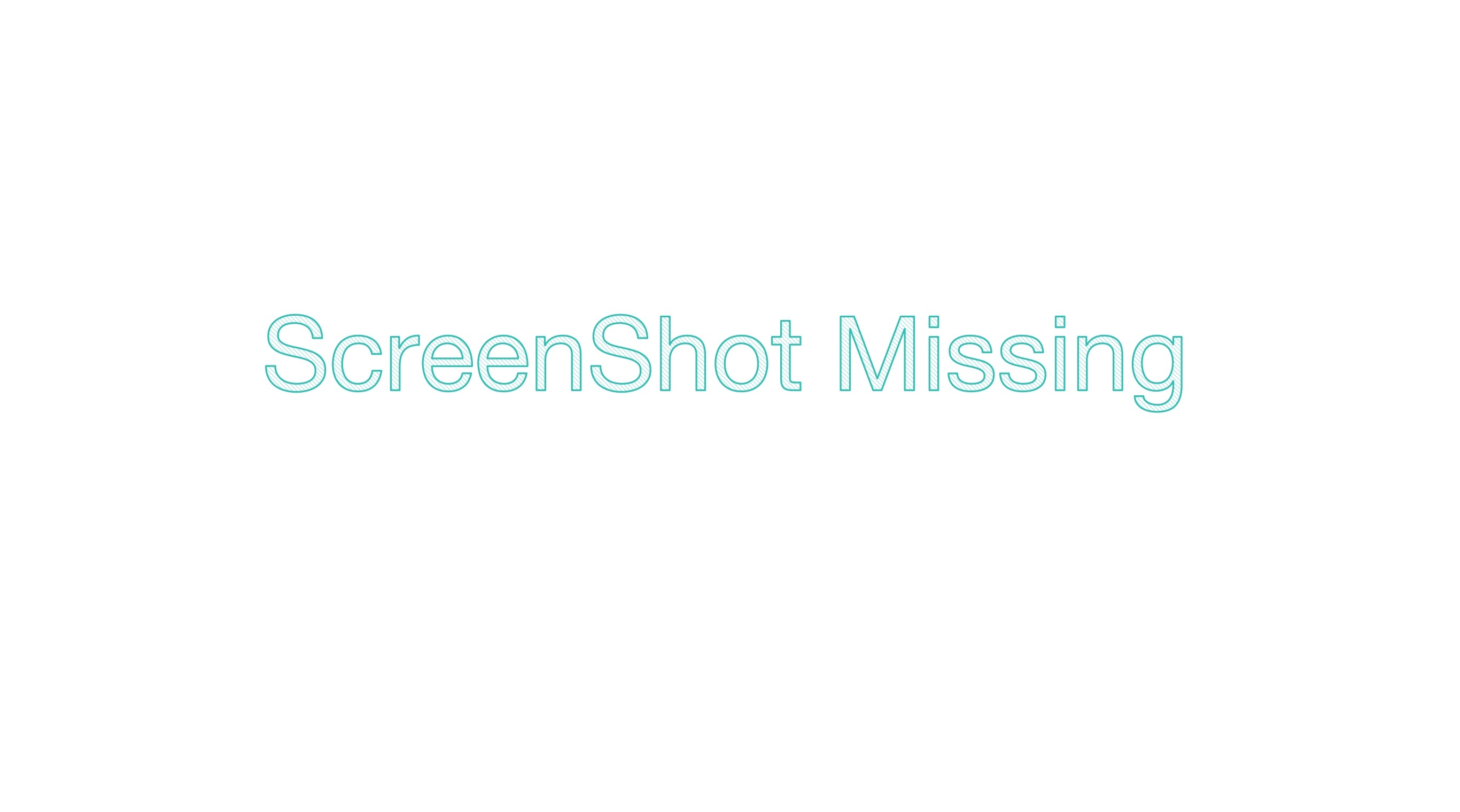
RepoAgent
RepoAgent is an LLM-powered framework designed for repository-level code documentation generation. It automates the process of detecting changes in Git repositories, analyzing code structure through AST, identifying inter-object relationships, replacing Markdown content, and executing multi-threaded operations. The tool aims to assist developers in understanding and maintaining codebases by providing comprehensive documentation, ultimately improving efficiency and saving time.
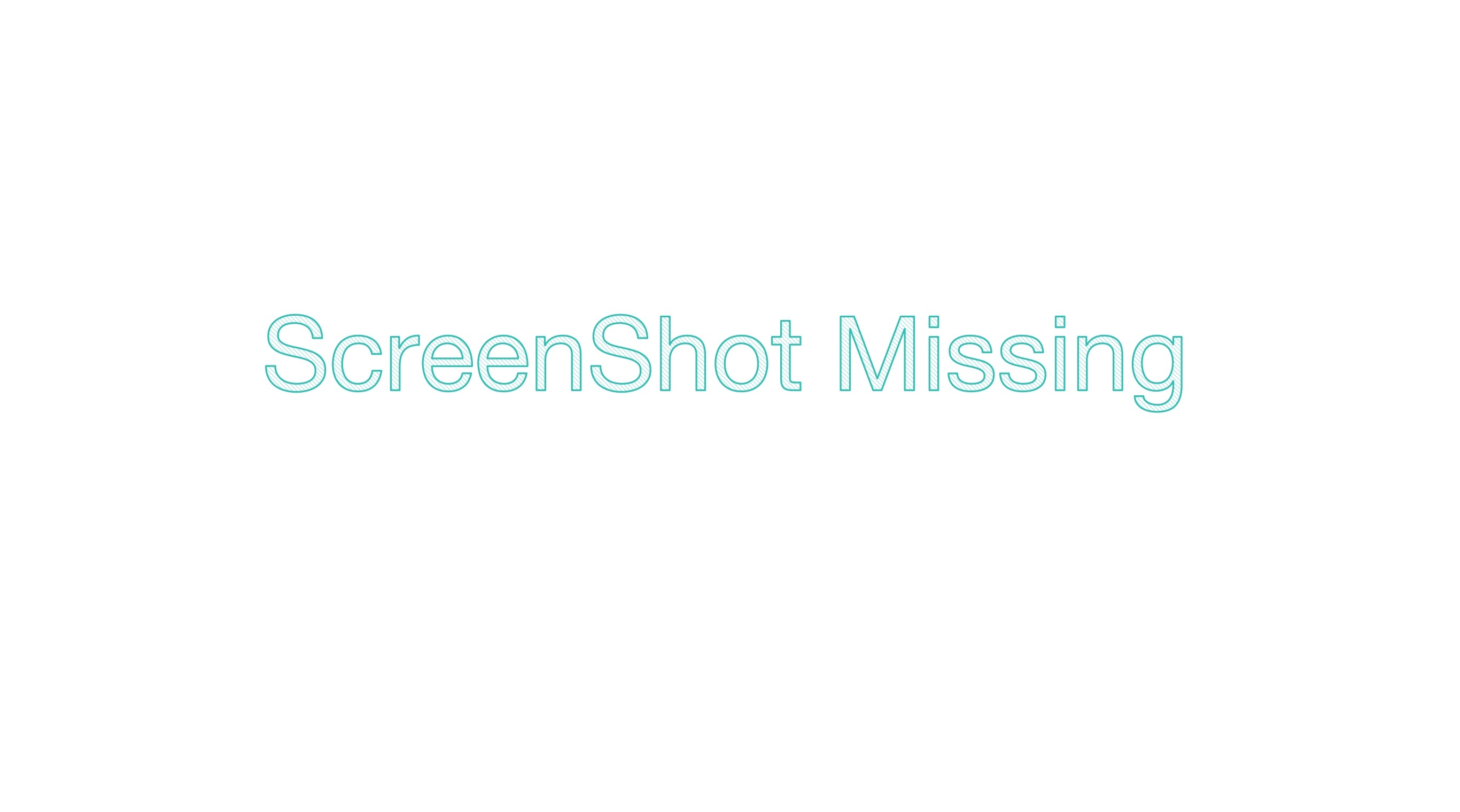
mosec
Mosec is a high-performance and flexible model serving framework for building ML model-enabled backend and microservices. It bridges the gap between any machine learning models you just trained and the efficient online service API. * **Highly performant** : web layer and task coordination built with Rust 🦀, which offers blazing speed in addition to efficient CPU utilization powered by async I/O * **Ease of use** : user interface purely in Python 🐍, by which users can serve their models in an ML framework-agnostic manner using the same code as they do for offline testing * **Dynamic batching** : aggregate requests from different users for batched inference and distribute results back * **Pipelined stages** : spawn multiple processes for pipelined stages to handle CPU/GPU/IO mixed workloads * **Cloud friendly** : designed to run in the cloud, with the model warmup, graceful shutdown, and Prometheus monitoring metrics, easily managed by Kubernetes or any container orchestration systems * **Do one thing well** : focus on the online serving part, users can pay attention to the model optimization and business logic
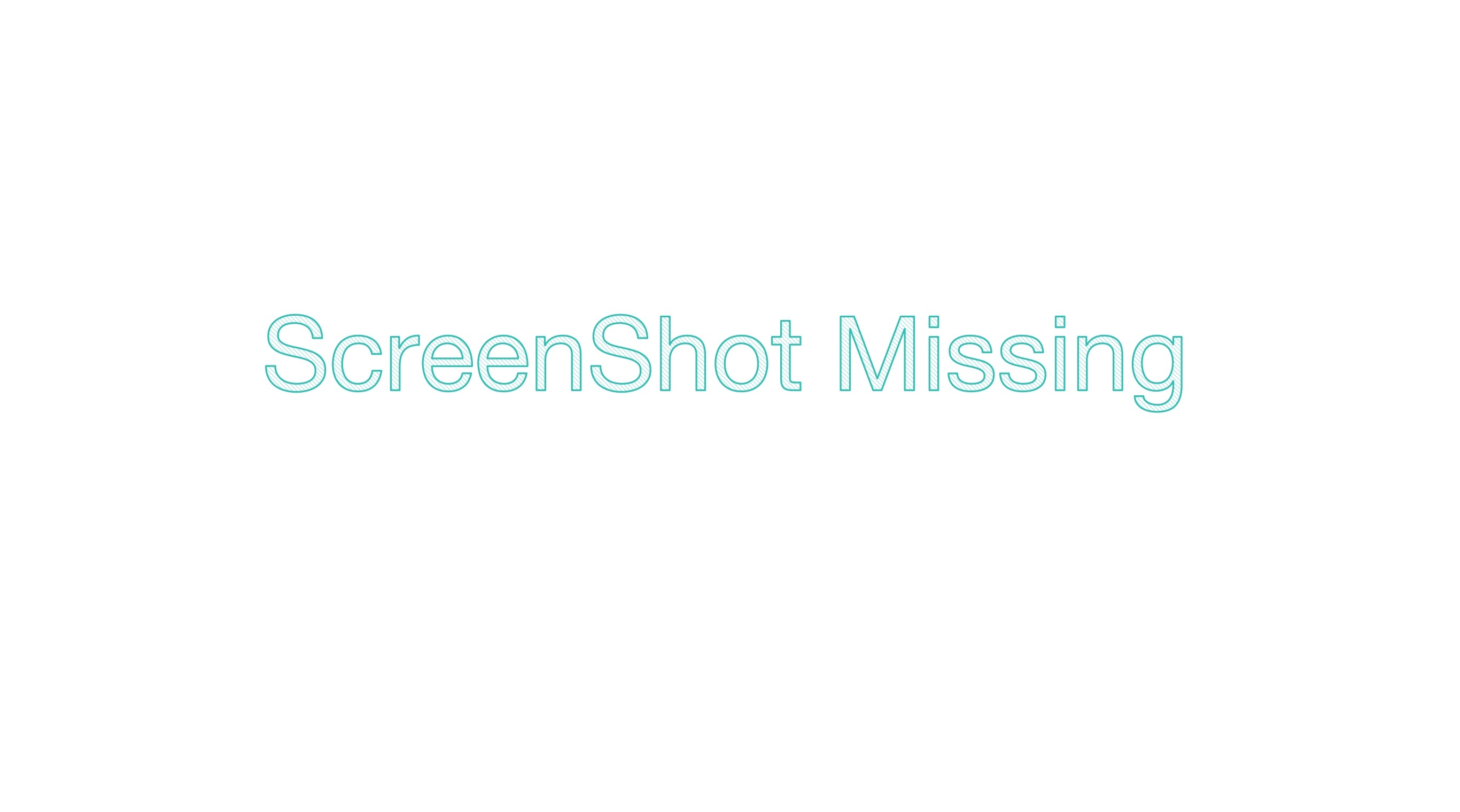
open-source-slack-ai
This repository provides a ready-to-run basic Slack AI solution that allows users to summarize threads and channels using OpenAI. Users can generate thread summaries, channel overviews, channel summaries since a specific time, and full channel summaries. The tool is powered by GPT-3.5-Turbo and an ensemble of NLP models. It requires Python 3.8 or higher, an OpenAI API key, Slack App with associated API tokens, Poetry package manager, and ngrok for local development. Users can customize channel and thread summaries, run tests with coverage using pytest, and contribute to the project for future enhancements.
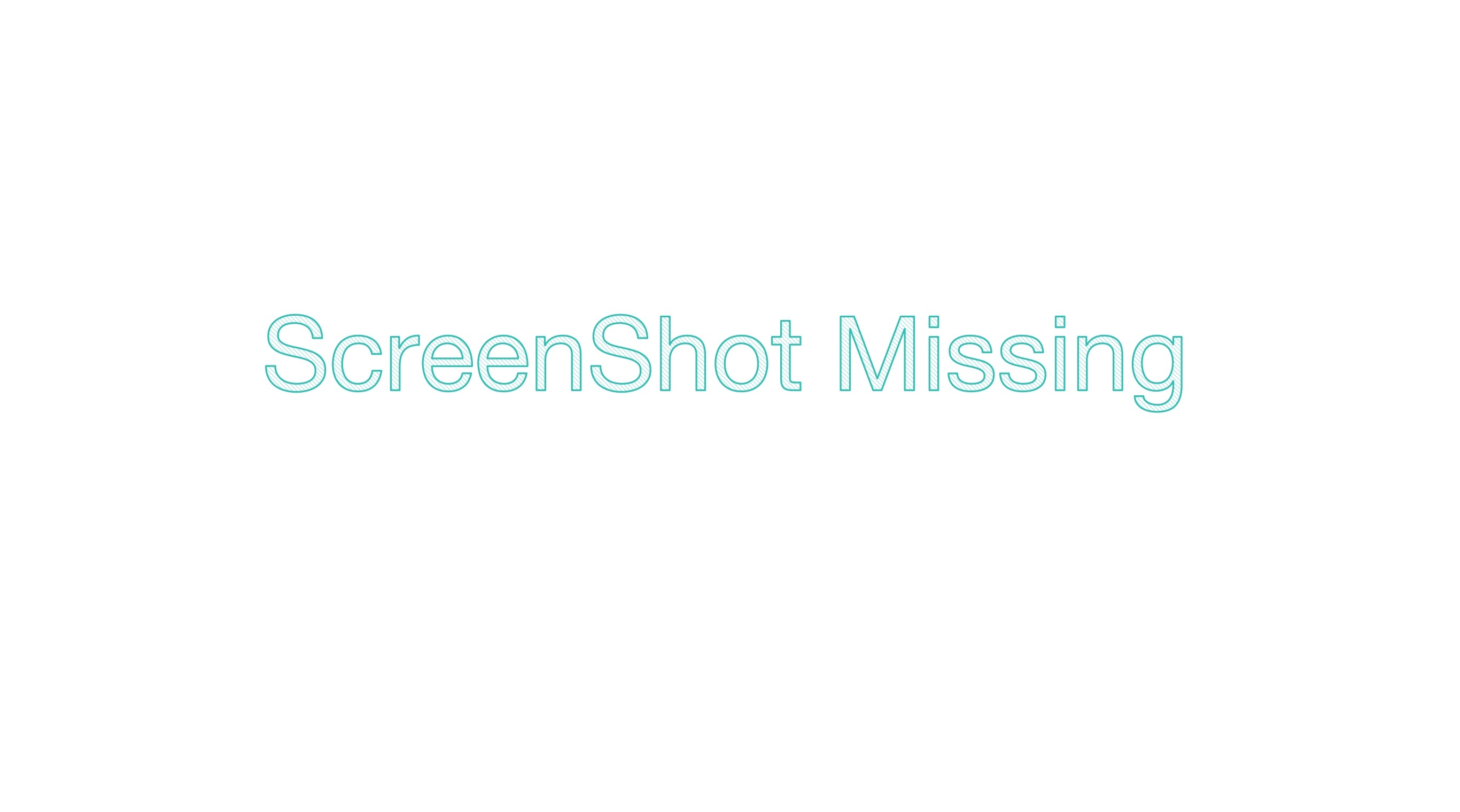
NeMo-Guardrails
NeMo Guardrails is an open-source toolkit for easily adding _programmable guardrails_ to LLM-based conversational applications. Guardrails (or "rails" for short) are specific ways of controlling the output of a large language model, such as not talking about politics, responding in a particular way to specific user requests, following a predefined dialog path, using a particular language style, extracting structured data, and more.
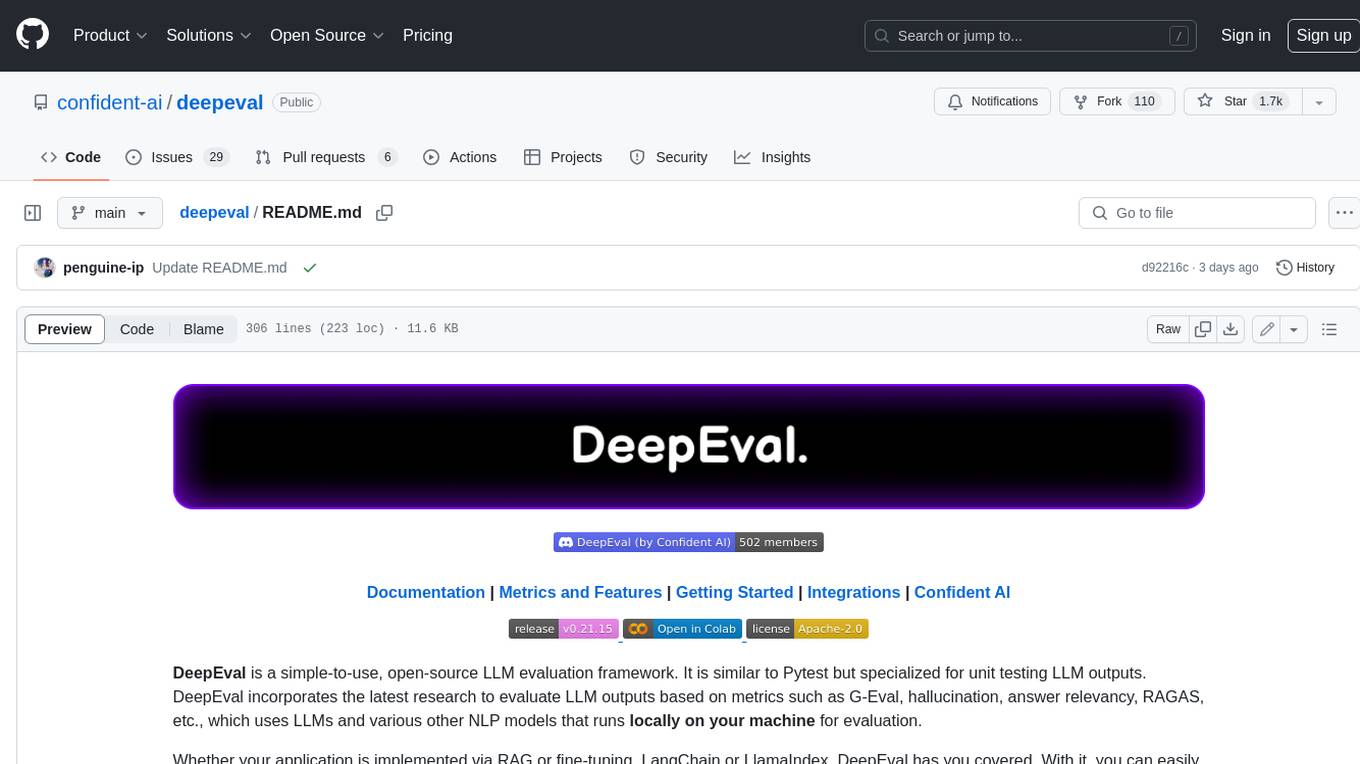
deepeval
DeepEval is a simple-to-use, open-source LLM evaluation framework specialized for unit testing LLM outputs. It incorporates various metrics such as G-Eval, hallucination, answer relevancy, RAGAS, etc., and runs locally on your machine for evaluation. It provides a wide range of ready-to-use evaluation metrics, allows for creating custom metrics, integrates with any CI/CD environment, and enables benchmarking LLMs on popular benchmarks. DeepEval is designed for evaluating RAG and fine-tuning applications, helping users optimize hyperparameters, prevent prompt drifting, and transition from OpenAI to hosting their own Llama2 with confidence.
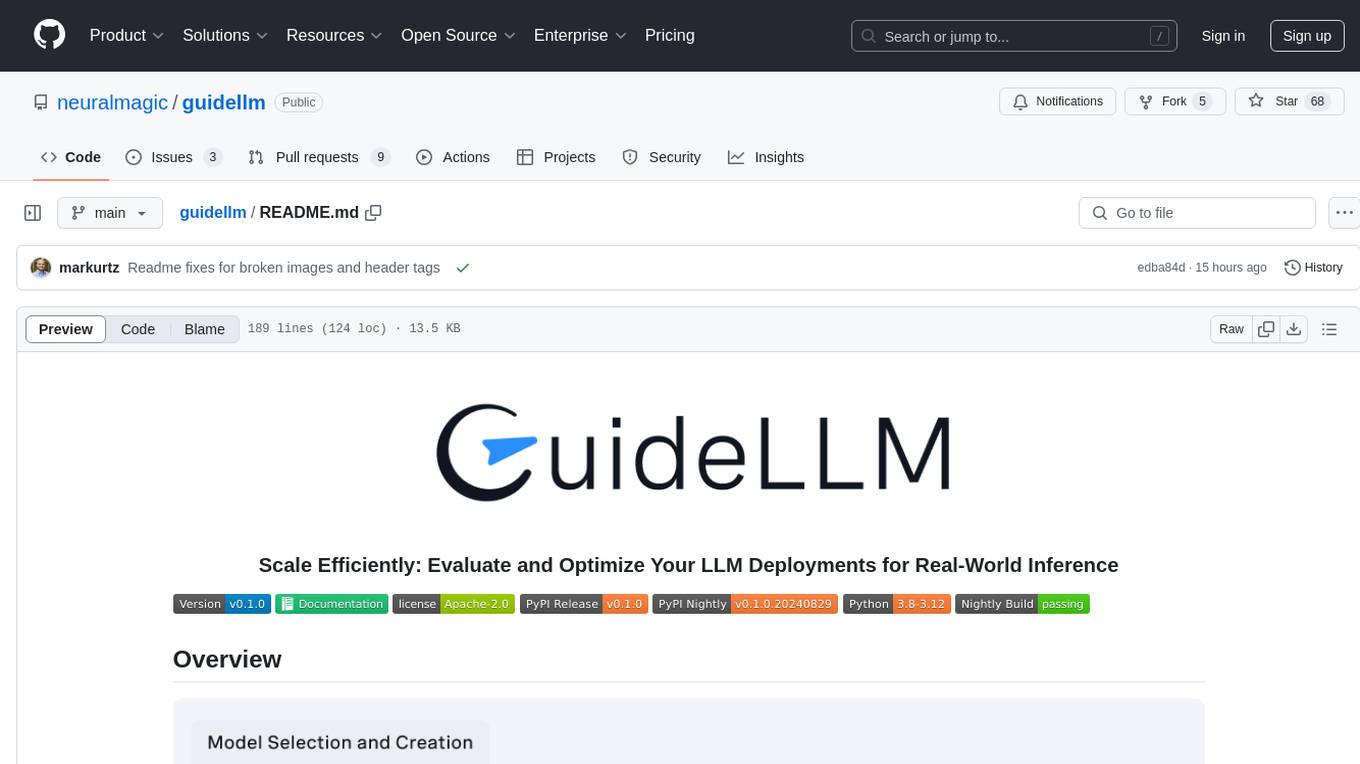
guidellm
GuideLLM is a powerful tool for evaluating and optimizing the deployment of large language models (LLMs). By simulating real-world inference workloads, GuideLLM helps users gauge the performance, resource needs, and cost implications of deploying LLMs on various hardware configurations. This approach ensures efficient, scalable, and cost-effective LLM inference serving while maintaining high service quality. Key features include performance evaluation, resource optimization, cost estimation, and scalability testing.
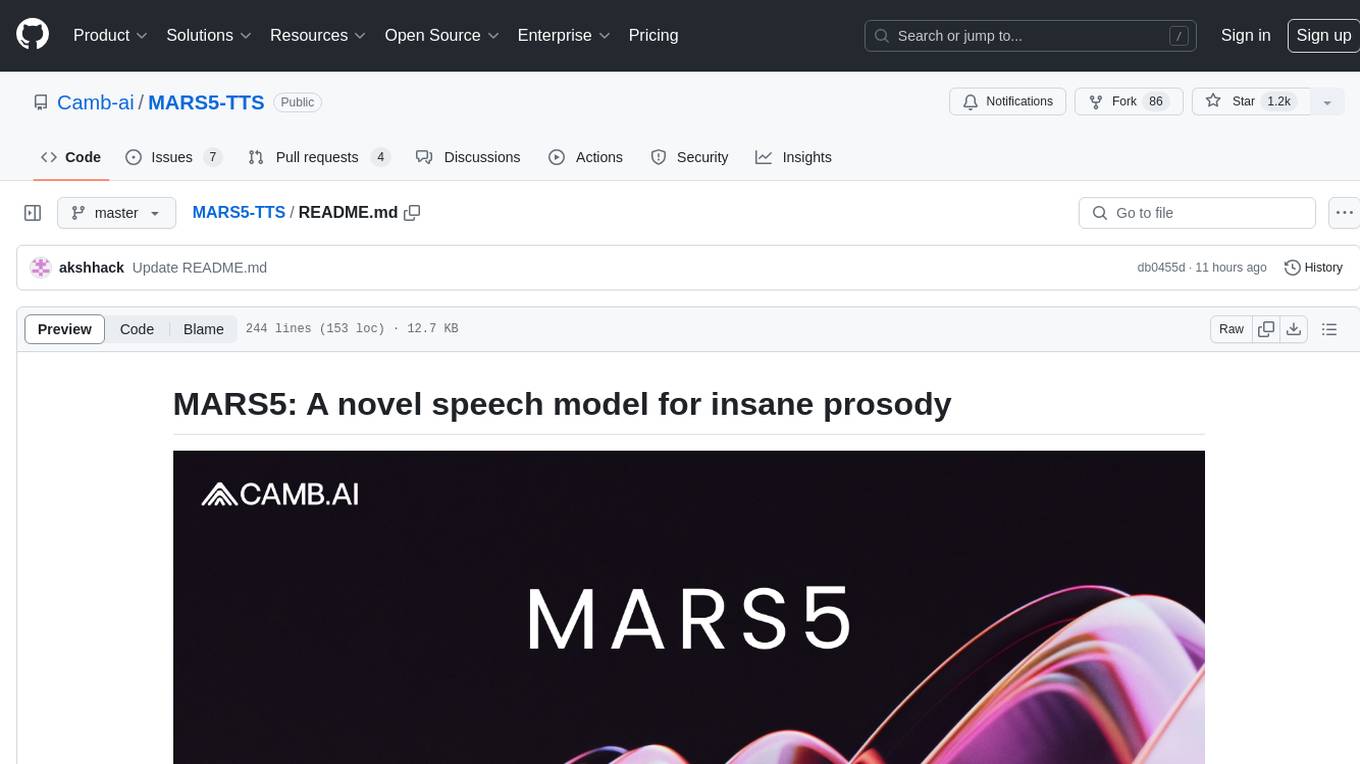
MARS5-TTS
MARS5 is a novel English speech model (TTS) developed by CAMB.AI, featuring a two-stage AR-NAR pipeline with a unique NAR component. The model can generate speech for various scenarios like sports commentary and anime with just 5 seconds of audio and a text snippet. It allows steering prosody using punctuation and capitalization in the transcript. Speaker identity is specified using an audio reference file, enabling 'deep clone' for improved quality. The model can be used via torch.hub or HuggingFace, supporting both shallow and deep cloning for inference. Checkpoints are provided for AR and NAR models, with hardware requirements of 750M+450M params on GPU. Contributions to improve model stability, performance, and reference audio selection are welcome.
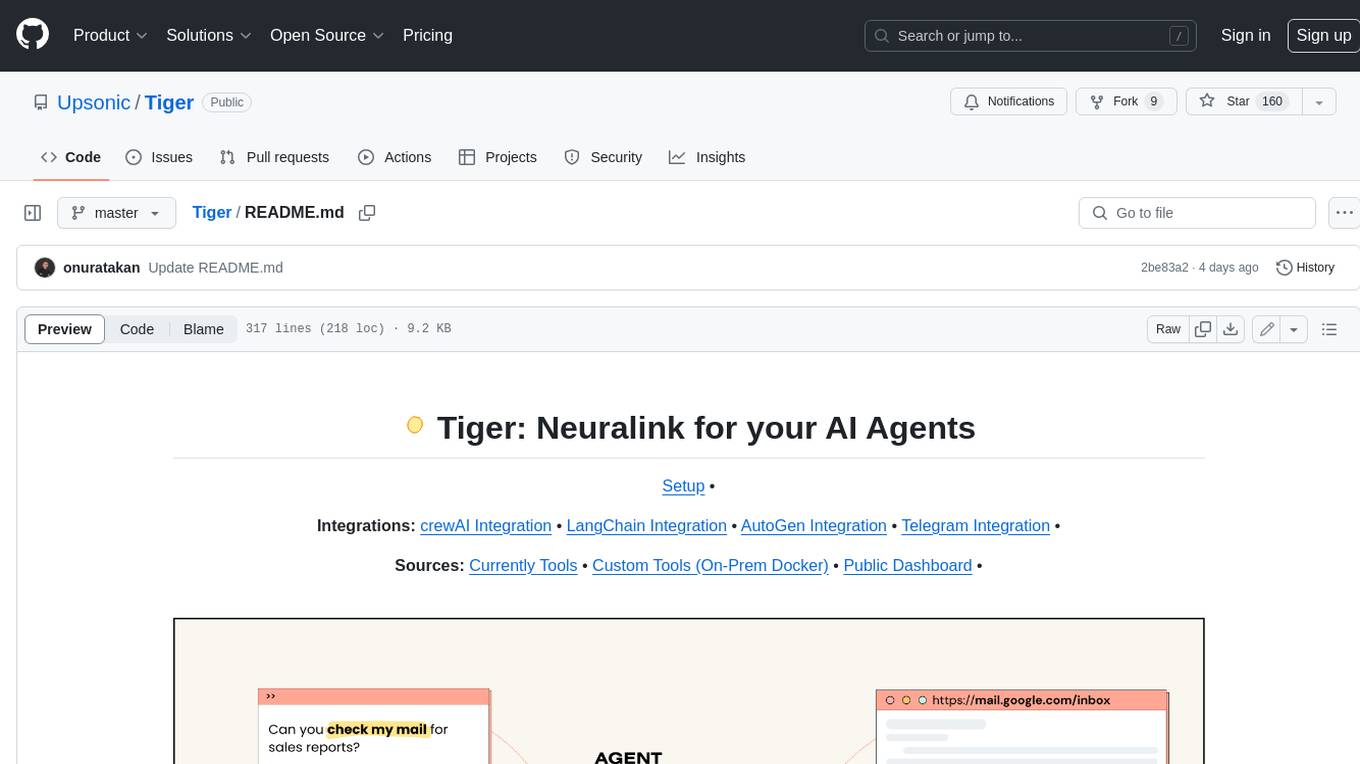
Tiger
Tiger is a community-driven project developing a reusable and integrated tool ecosystem for LLM Agent Revolution. It utilizes Upsonic for isolated tool storage, profiling, and automatic document generation. With Tiger, you can create a customized environment for your agents or leverage the robust and publicly maintained Tiger curated by the community itself.
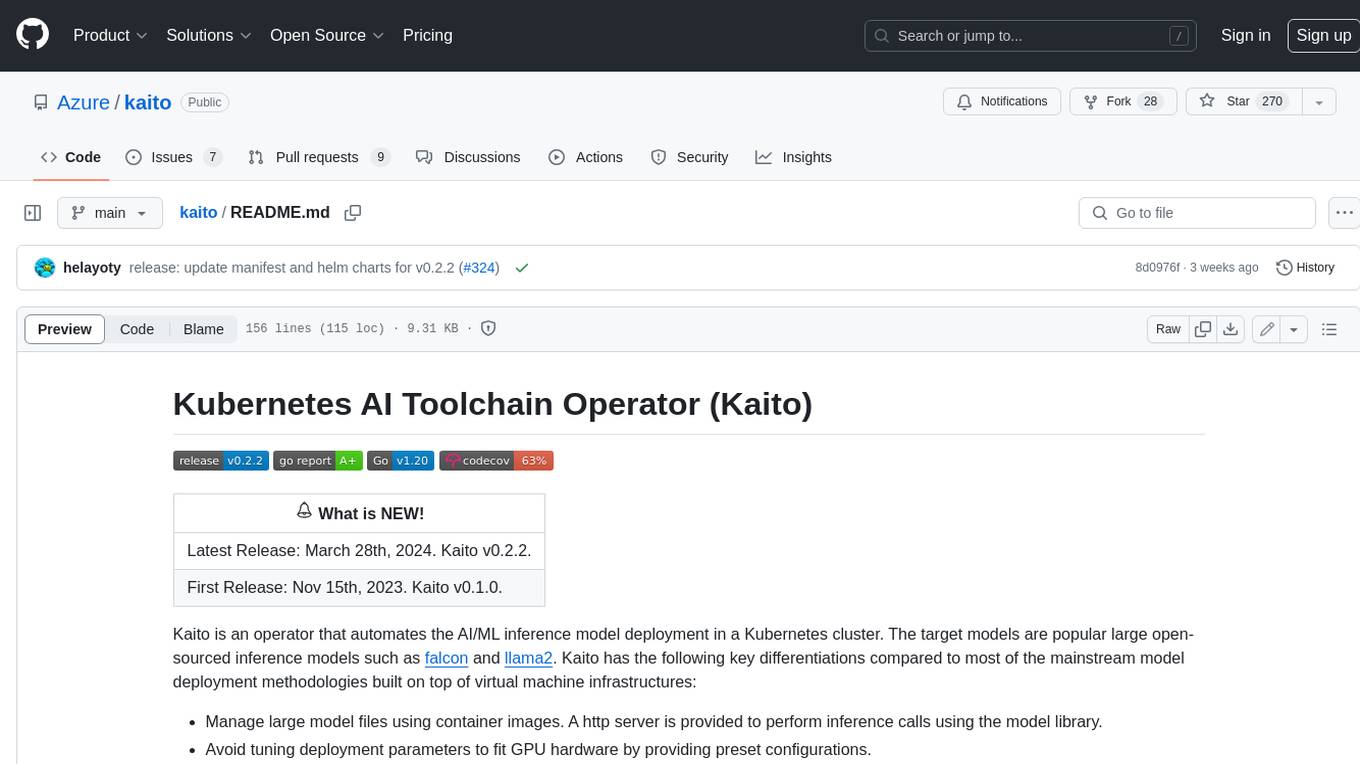
kaito
Kaito is an operator that automates the AI/ML inference model deployment in a Kubernetes cluster. It manages large model files using container images, avoids tuning deployment parameters to fit GPU hardware by providing preset configurations, auto-provisions GPU nodes based on model requirements, and hosts large model images in the public Microsoft Container Registry (MCR) if the license allows. Using Kaito, the workflow of onboarding large AI inference models in Kubernetes is largely simplified.
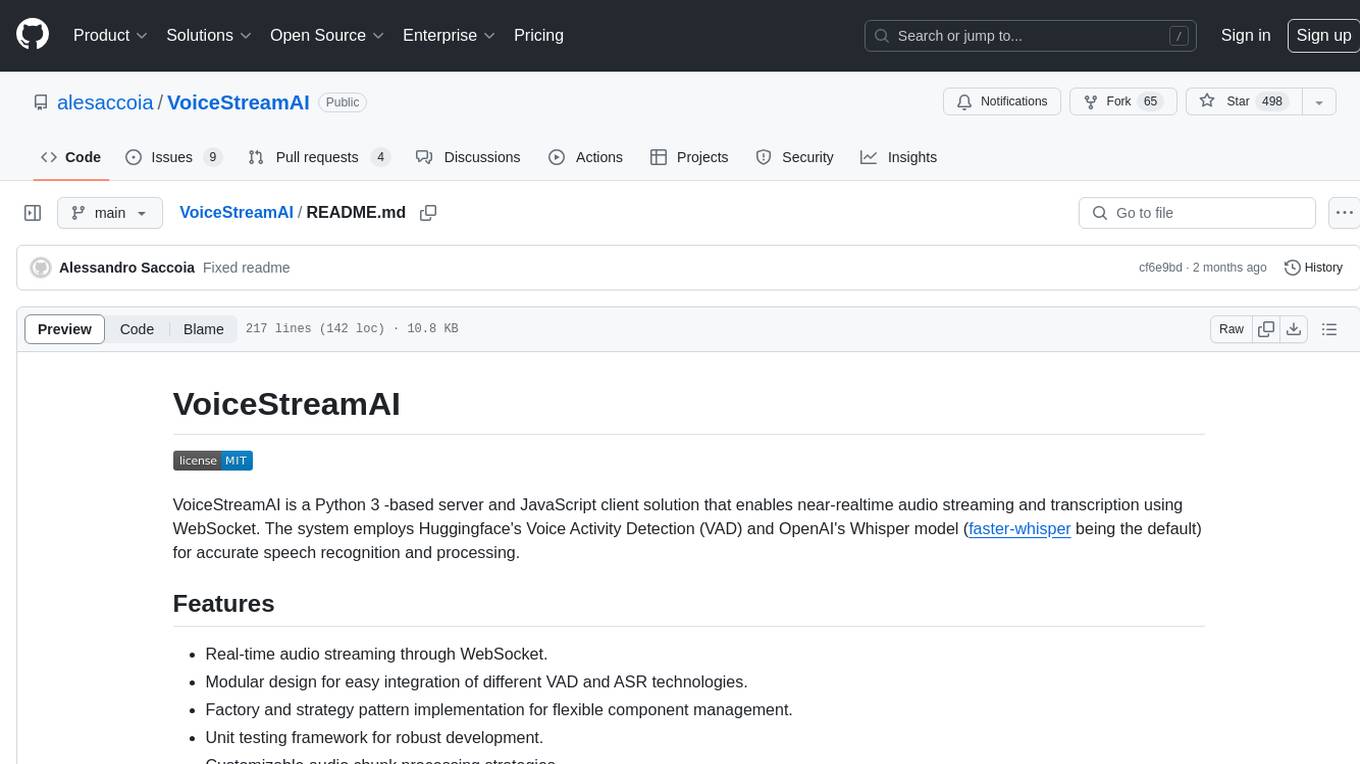
VoiceStreamAI
VoiceStreamAI is a Python 3-based server and JavaScript client solution for near-realtime audio streaming and transcription using WebSocket. It employs Huggingface's Voice Activity Detection (VAD) and OpenAI's Whisper model for accurate speech recognition. The system features real-time audio streaming, modular design for easy integration of VAD and ASR technologies, customizable audio chunk processing strategies, support for multilingual transcription, and secure sockets support. It uses a factory and strategy pattern implementation for flexible component management and provides a unit testing framework for robust development.
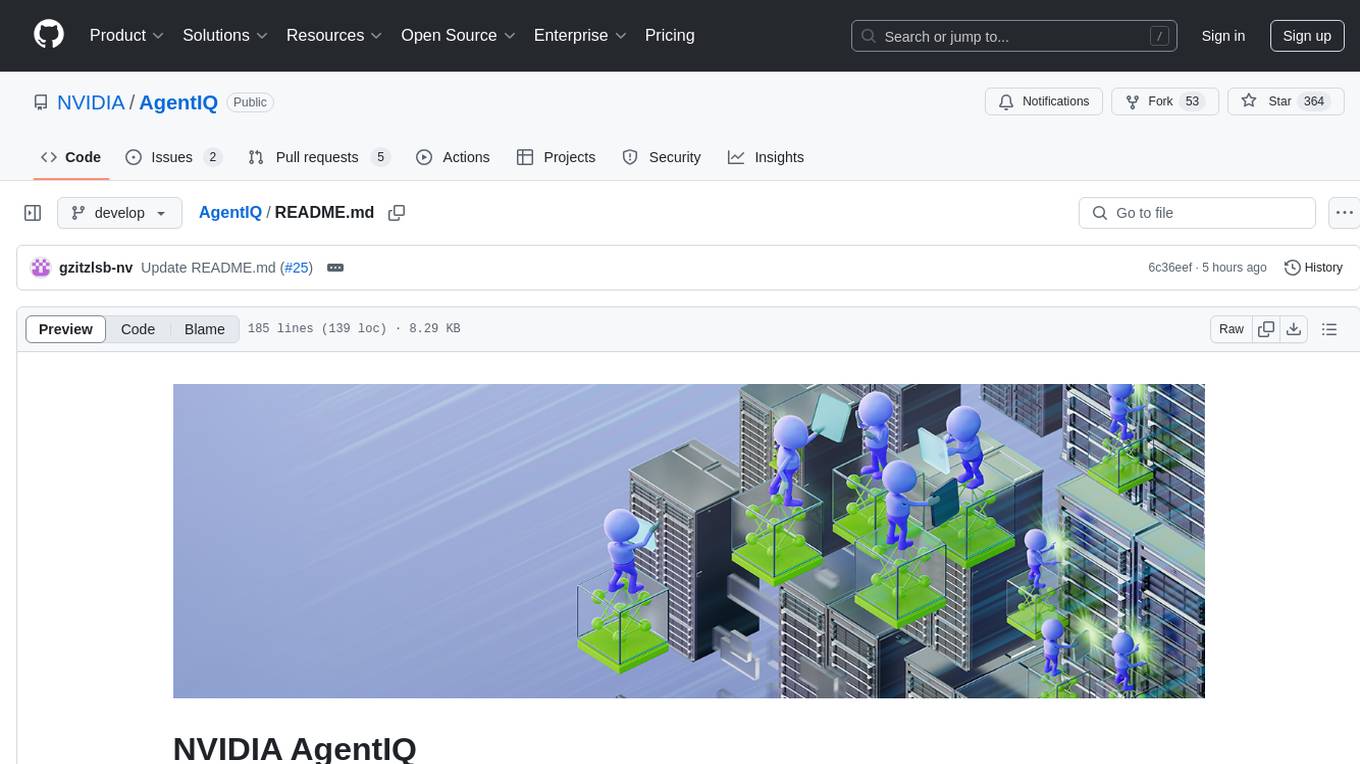
AgentIQ
AgentIQ is a flexible library designed to seamlessly integrate enterprise agents with various data sources and tools. It enables true composability by treating agents, tools, and workflows as simple function calls. With features like framework agnosticism, reusability, rapid development, profiling, observability, evaluation system, user interface, and MCP compatibility, AgentIQ empowers developers to move quickly, experiment freely, and ensure reliability across agent-driven projects.
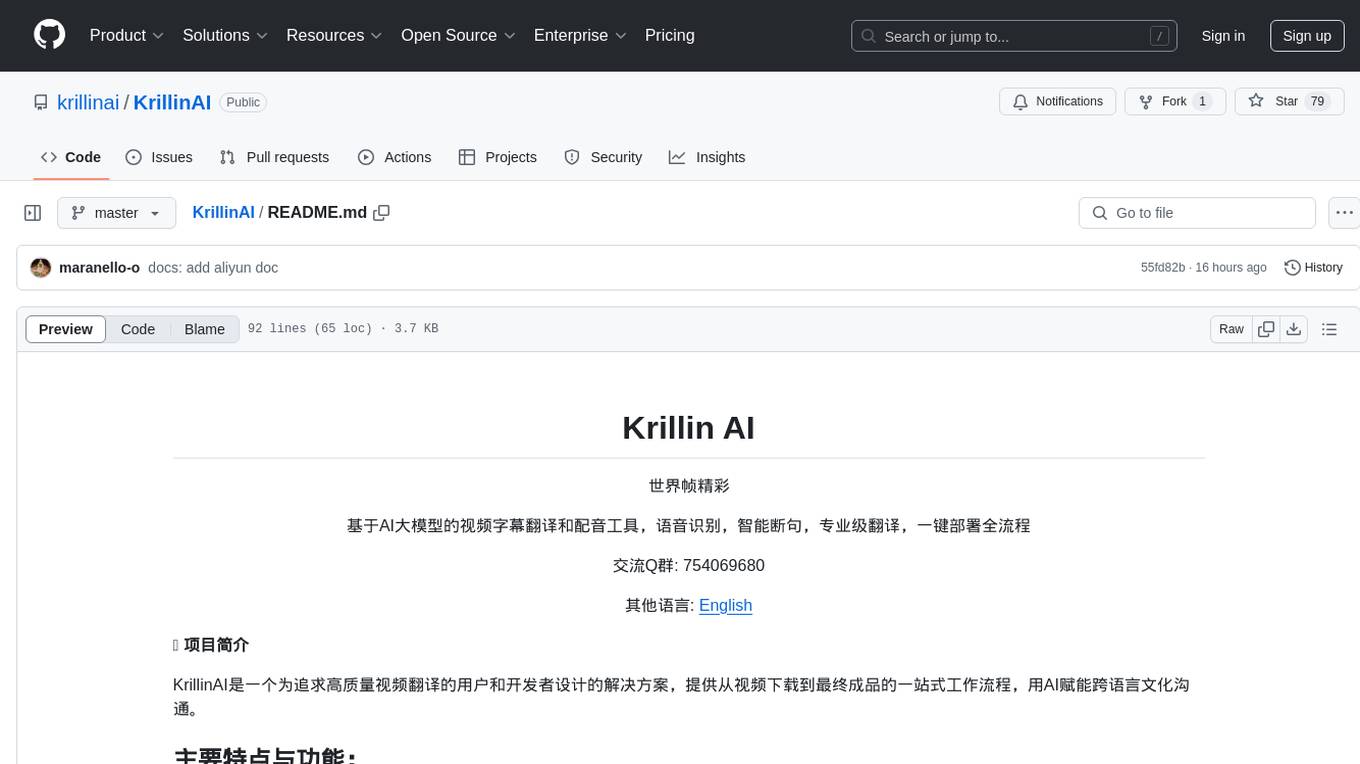
KrillinAI
KrillinAI is a video subtitle translation and dubbing tool based on AI large models, featuring speech recognition, intelligent sentence segmentation, professional translation, and one-click deployment of the entire process. It provides a one-stop workflow from video downloading to the final product, empowering cross-language cultural communication with AI. The tool supports multiple languages for input and translation, integrates features like automatic dependency installation, video downloading from platforms like YouTube and Bilibili, high-speed subtitle recognition, intelligent subtitle segmentation and alignment, custom vocabulary replacement, professional-level translation engine, and diverse external service selection for speech and large model services.
For similar tasks
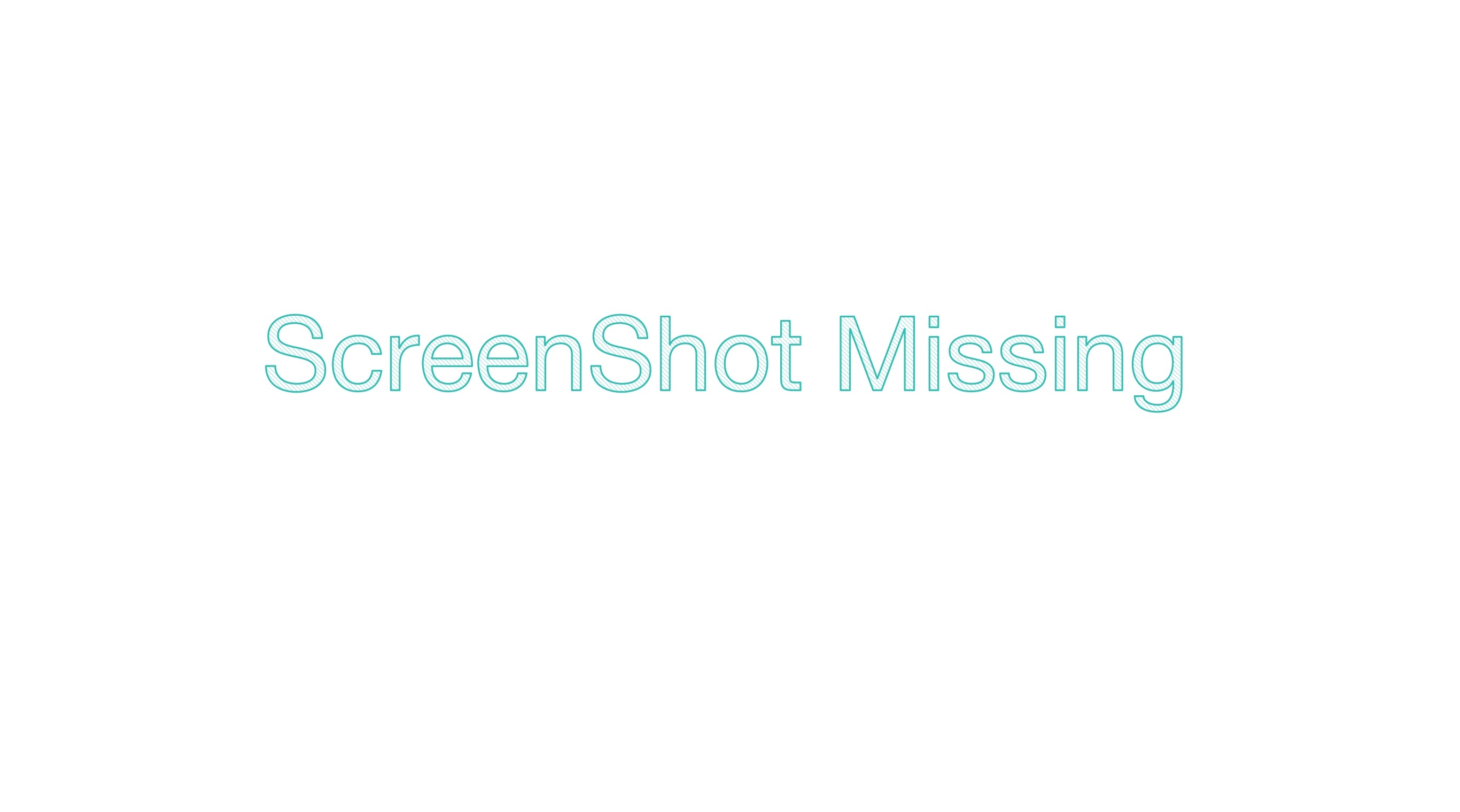
lmql
LMQL is a programming language designed for large language models (LLMs) that offers a unique way of integrating traditional programming with LLM interaction. It allows users to write programs that combine algorithmic logic with LLM calls, enabling model reasoning capabilities within the context of the program. LMQL provides features such as Python syntax integration, rich control-flow options, advanced decoding techniques, powerful constraints via logit masking, runtime optimization, sync and async API support, multi-model compatibility, and extensive applications like JSON decoding and interactive chat interfaces. The tool also offers library integration, flexible tooling, and output streaming options for easy model output handling.
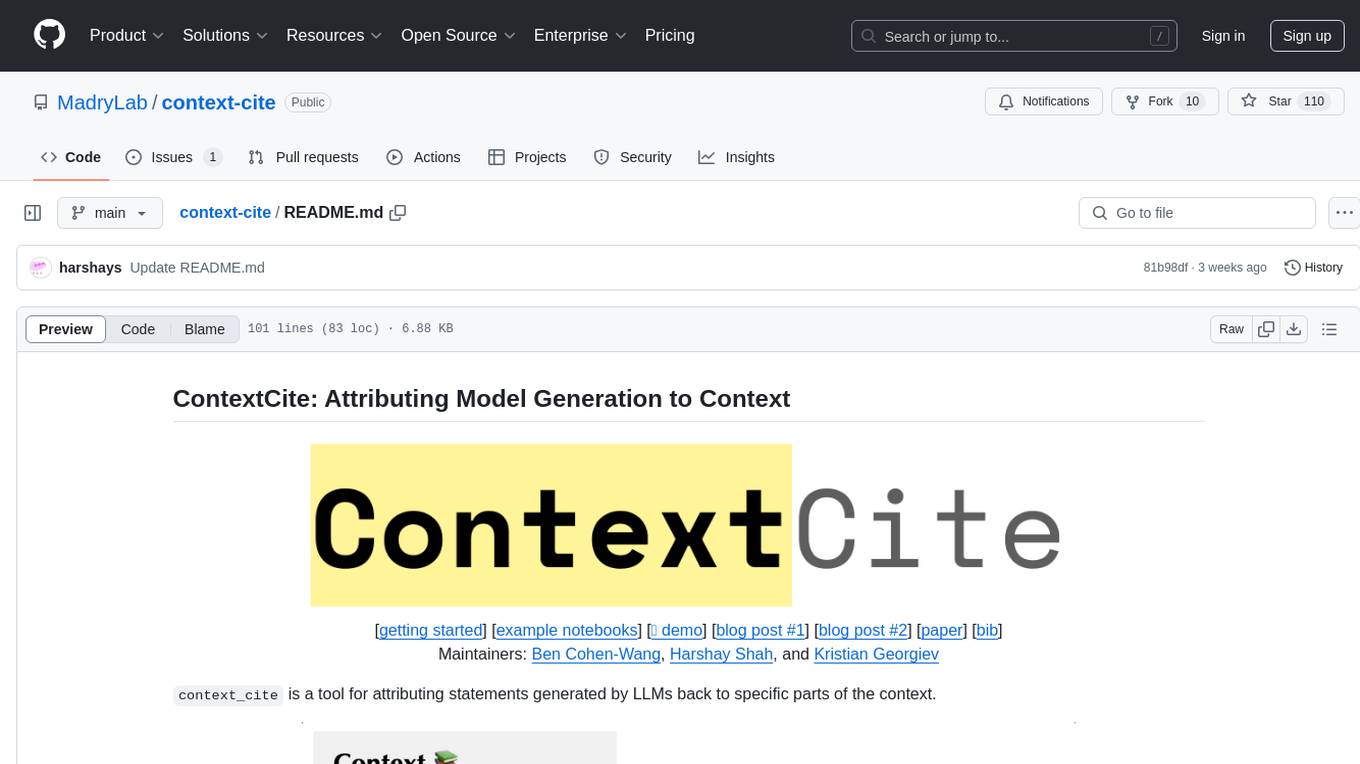
context-cite
ContextCite is a tool for attributing statements generated by LLMs back to specific parts of the context. It allows users to analyze and understand the sources of information used by language models in generating responses. By providing attributions, users can gain insights into how the model makes decisions and where the information comes from.
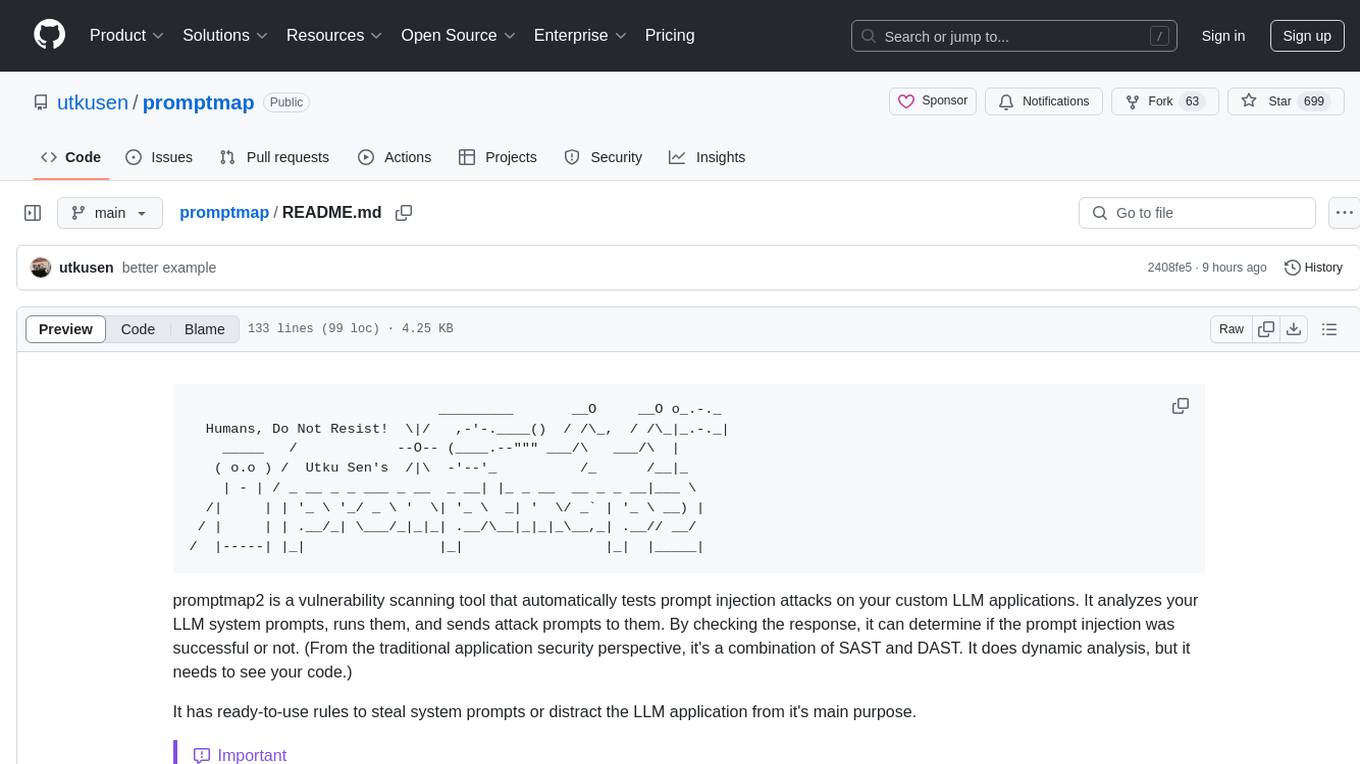
promptmap
promptmap2 is a vulnerability scanning tool that automatically tests prompt injection attacks on custom LLM applications. It analyzes LLM system prompts, runs them, and sends attack prompts to determine if injection was successful. It has ready-to-use rules to steal system prompts or distract LLM applications. Supports multiple LLM providers like OpenAI, Anthropic, and open source models via Ollama. Customizable test rules in YAML format and automatic model download for Ollama.
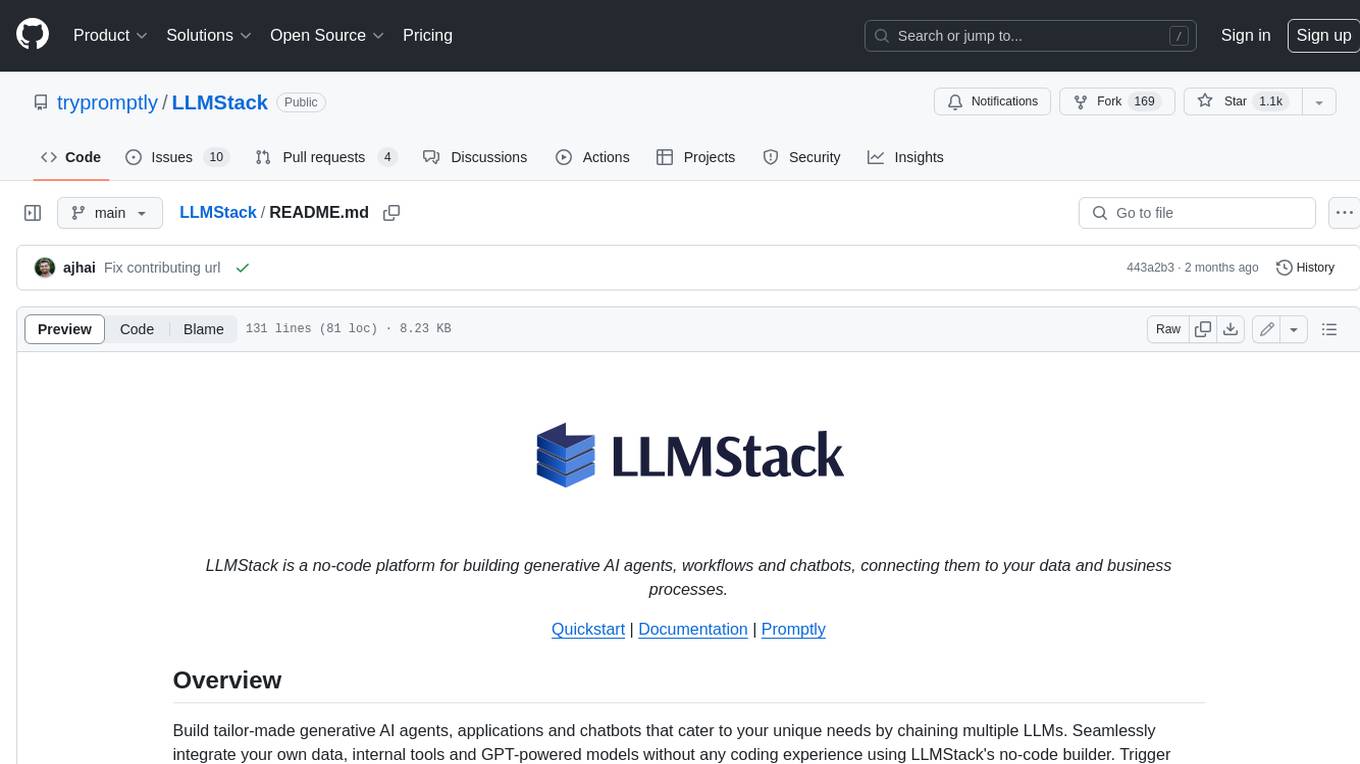
LLMStack
LLMStack is a no-code platform for building generative AI agents, workflows, and chatbots. It allows users to connect their own data, internal tools, and GPT-powered models without any coding experience. LLMStack can be deployed to the cloud or on-premise and can be accessed via HTTP API or triggered from Slack or Discord.
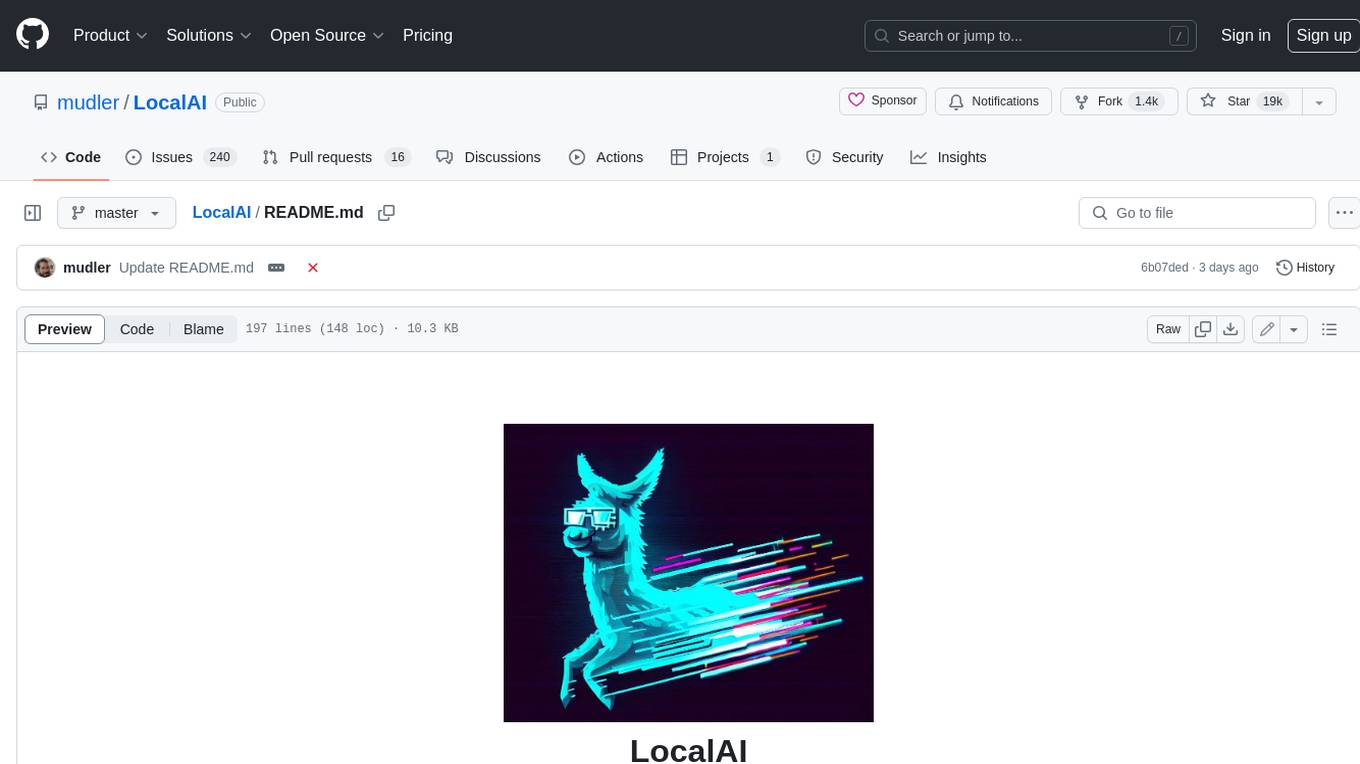
LocalAI
LocalAI is a free and open-source OpenAI alternative that acts as a drop-in replacement REST API compatible with OpenAI (Elevenlabs, Anthropic, etc.) API specifications for local AI inferencing. It allows users to run LLMs, generate images, audio, and more locally or on-premises with consumer-grade hardware, supporting multiple model families and not requiring a GPU. LocalAI offers features such as text generation with GPTs, text-to-audio, audio-to-text transcription, image generation with stable diffusion, OpenAI functions, embeddings generation for vector databases, constrained grammars, downloading models directly from Huggingface, and a Vision API. It provides a detailed step-by-step introduction in its Getting Started guide and supports community integrations such as custom containers, WebUIs, model galleries, and various bots for Discord, Slack, and Telegram. LocalAI also offers resources like an LLM fine-tuning guide, instructions for local building and Kubernetes installation, projects integrating LocalAI, and a how-tos section curated by the community. It encourages users to cite the repository when utilizing it in downstream projects and acknowledges the contributions of various software from the community.
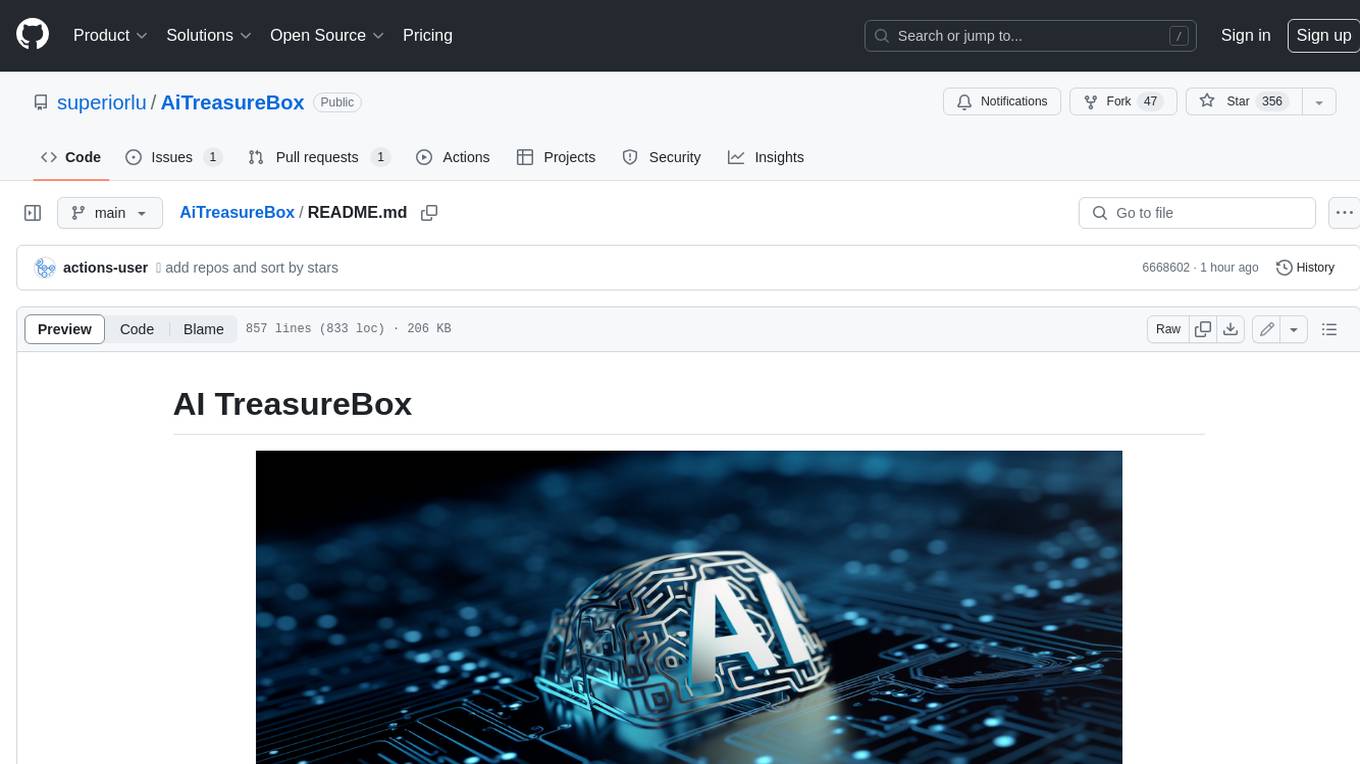
AiTreasureBox
AiTreasureBox is a versatile AI tool that provides a collection of pre-trained models and algorithms for various machine learning tasks. It simplifies the process of implementing AI solutions by offering ready-to-use components that can be easily integrated into projects. With AiTreasureBox, users can quickly prototype and deploy AI applications without the need for extensive knowledge in machine learning or deep learning. The tool covers a wide range of tasks such as image classification, text generation, sentiment analysis, object detection, and more. It is designed to be user-friendly and accessible to both beginners and experienced developers, making AI development more efficient and accessible to a wider audience.
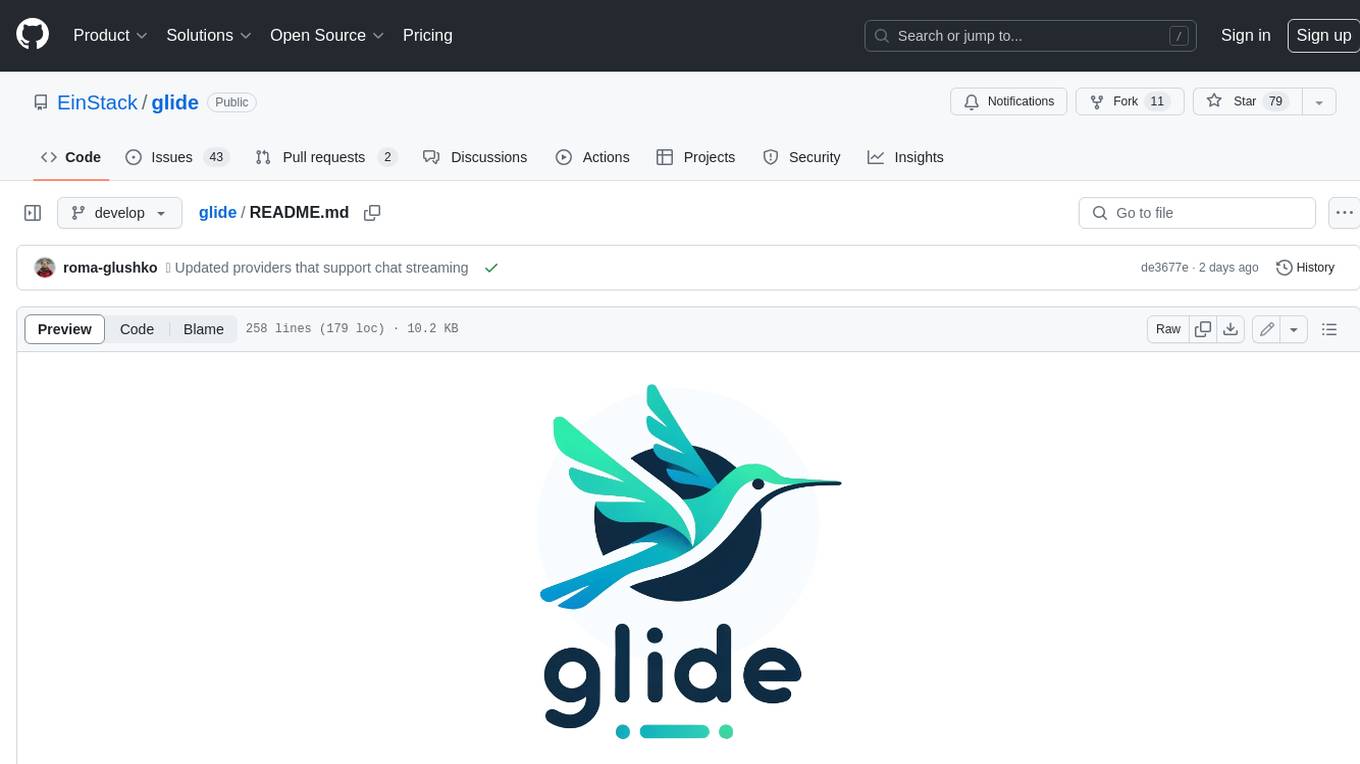
glide
Glide is a cloud-native LLM gateway that provides a unified REST API for accessing various large language models (LLMs) from different providers. It handles LLMOps tasks such as model failover, caching, key management, and more, making it easy to integrate LLMs into applications. Glide supports popular LLM providers like OpenAI, Anthropic, Azure OpenAI, AWS Bedrock (Titan), Cohere, Google Gemini, OctoML, and Ollama. It offers high availability, performance, and observability, and provides SDKs for Python and NodeJS to simplify integration.
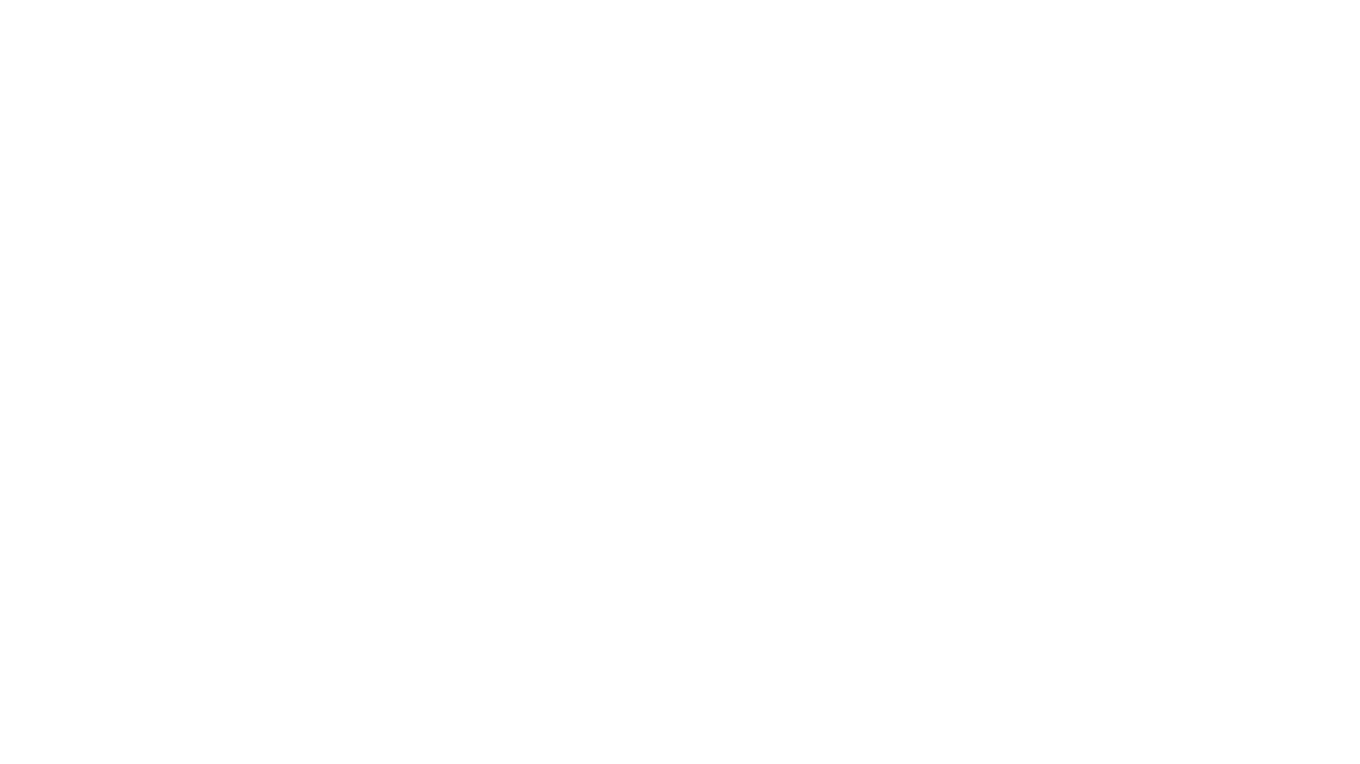
jupyter-ai
Jupyter AI connects generative AI with Jupyter notebooks. It provides a user-friendly and powerful way to explore generative AI models in notebooks and improve your productivity in JupyterLab and the Jupyter Notebook. Specifically, Jupyter AI offers: * An `%%ai` magic that turns the Jupyter notebook into a reproducible generative AI playground. This works anywhere the IPython kernel runs (JupyterLab, Jupyter Notebook, Google Colab, Kaggle, VSCode, etc.). * A native chat UI in JupyterLab that enables you to work with generative AI as a conversational assistant. * Support for a wide range of generative model providers, including AI21, Anthropic, AWS, Cohere, Gemini, Hugging Face, NVIDIA, and OpenAI. * Local model support through GPT4All, enabling use of generative AI models on consumer grade machines with ease and privacy.
For similar jobs
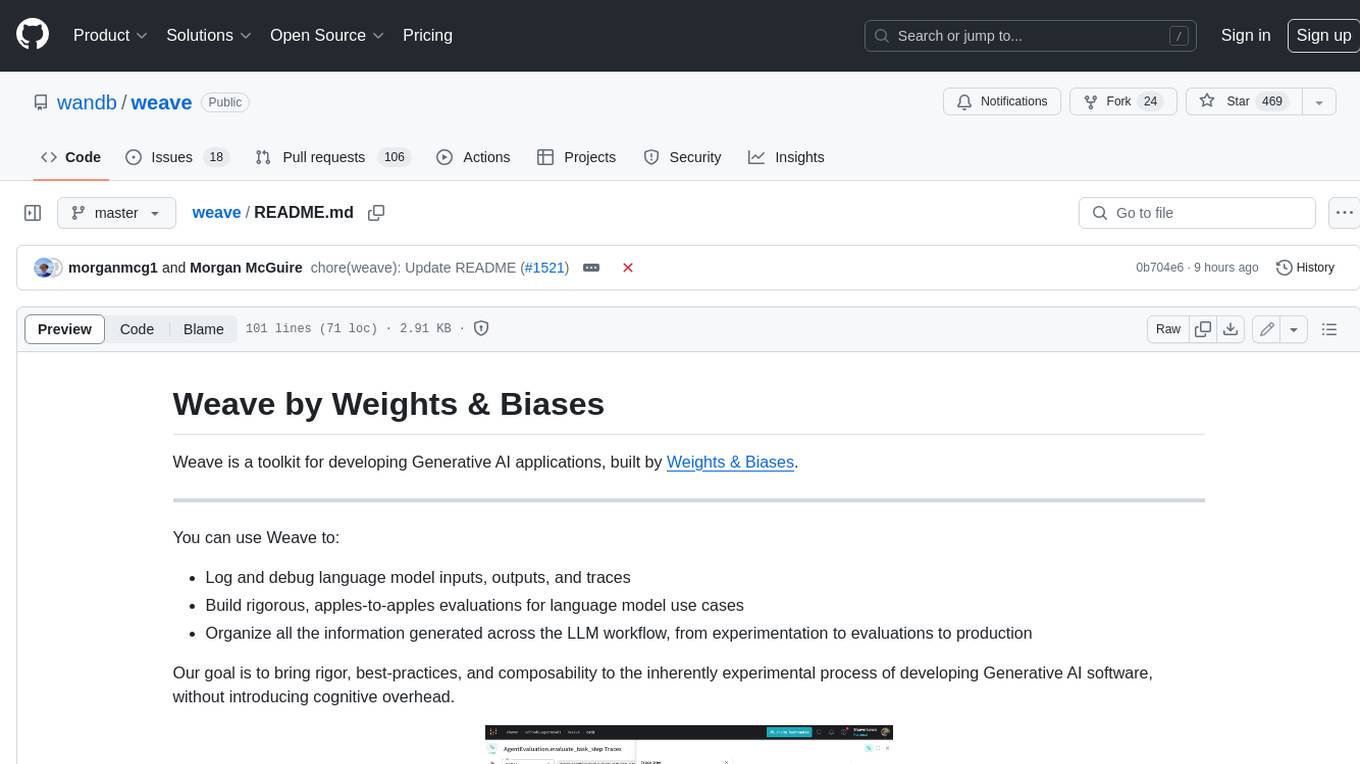
weave
Weave is a toolkit for developing Generative AI applications, built by Weights & Biases. With Weave, you can log and debug language model inputs, outputs, and traces; build rigorous, apples-to-apples evaluations for language model use cases; and organize all the information generated across the LLM workflow, from experimentation to evaluations to production. Weave aims to bring rigor, best-practices, and composability to the inherently experimental process of developing Generative AI software, without introducing cognitive overhead.
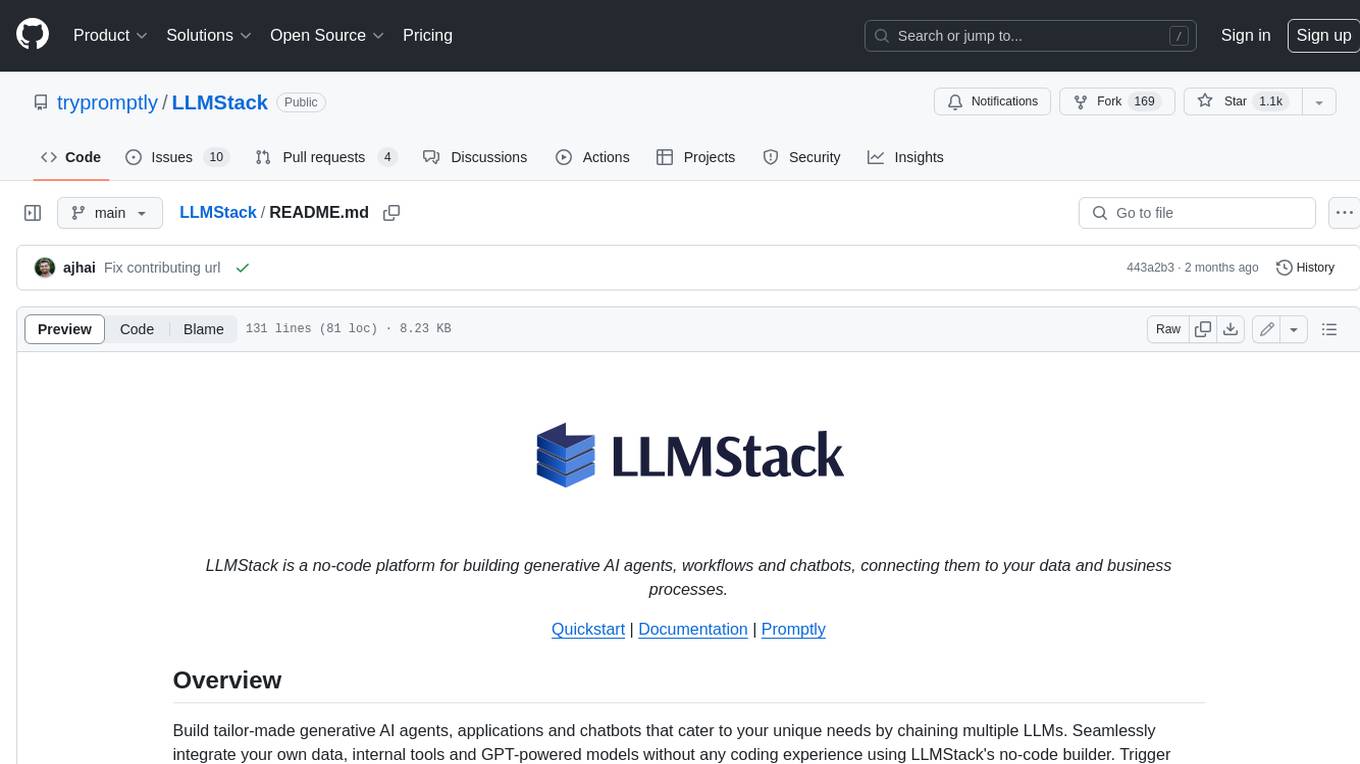
LLMStack
LLMStack is a no-code platform for building generative AI agents, workflows, and chatbots. It allows users to connect their own data, internal tools, and GPT-powered models without any coding experience. LLMStack can be deployed to the cloud or on-premise and can be accessed via HTTP API or triggered from Slack or Discord.
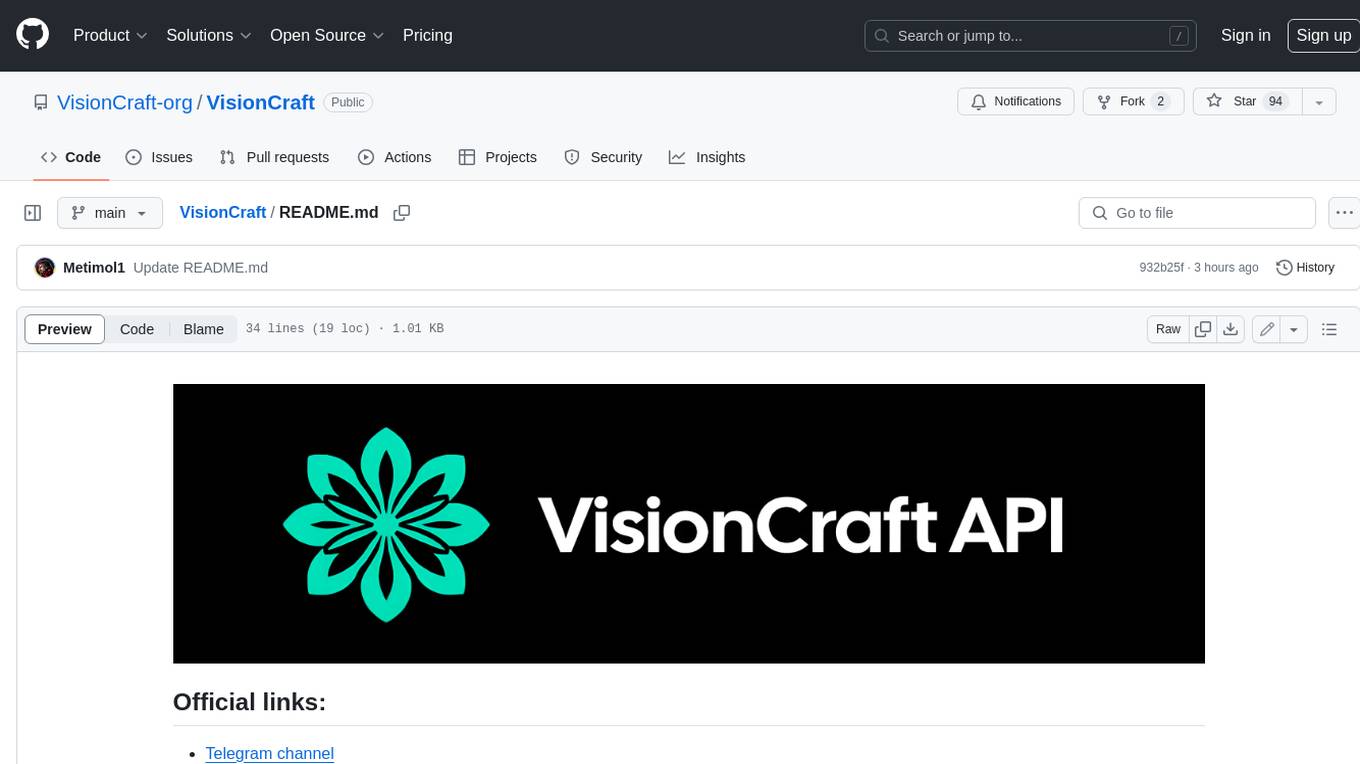
VisionCraft
The VisionCraft API is a free API for using over 100 different AI models. From images to sound.
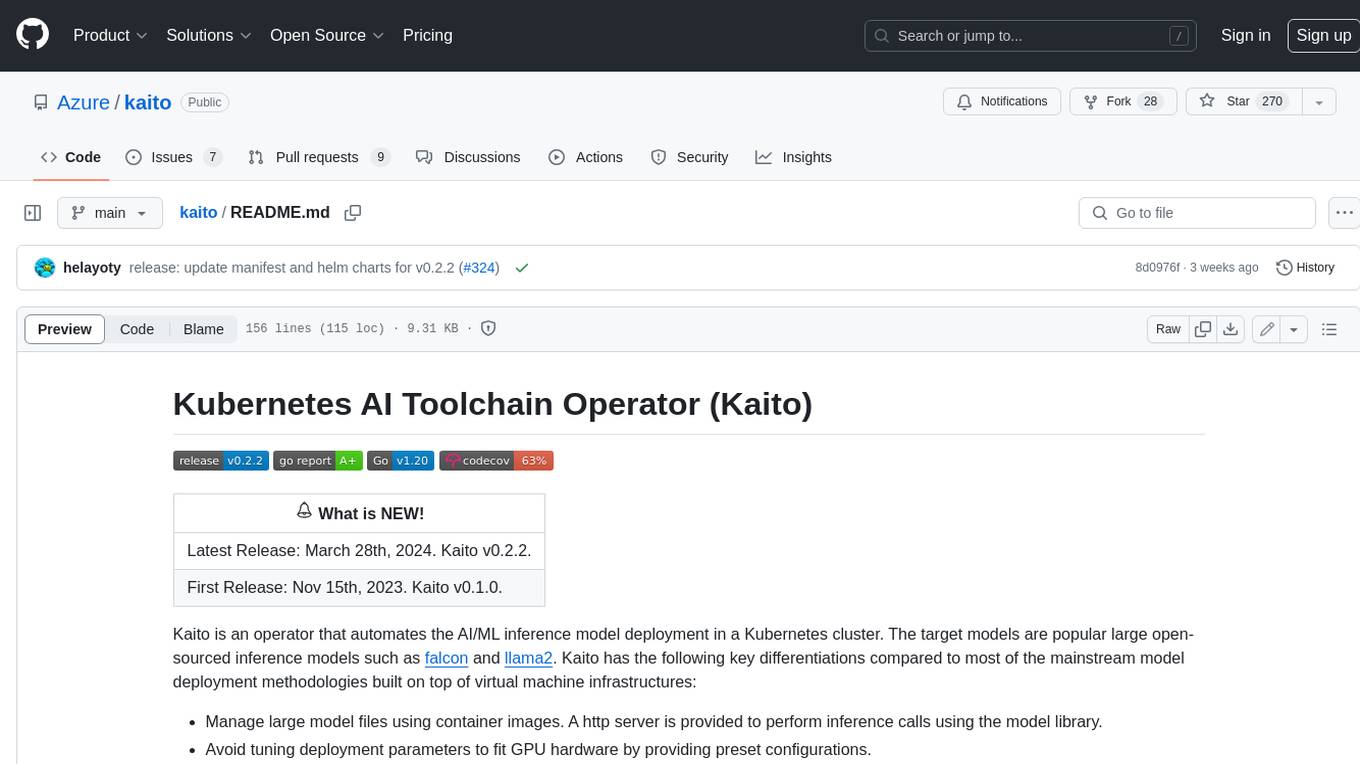
kaito
Kaito is an operator that automates the AI/ML inference model deployment in a Kubernetes cluster. It manages large model files using container images, avoids tuning deployment parameters to fit GPU hardware by providing preset configurations, auto-provisions GPU nodes based on model requirements, and hosts large model images in the public Microsoft Container Registry (MCR) if the license allows. Using Kaito, the workflow of onboarding large AI inference models in Kubernetes is largely simplified.
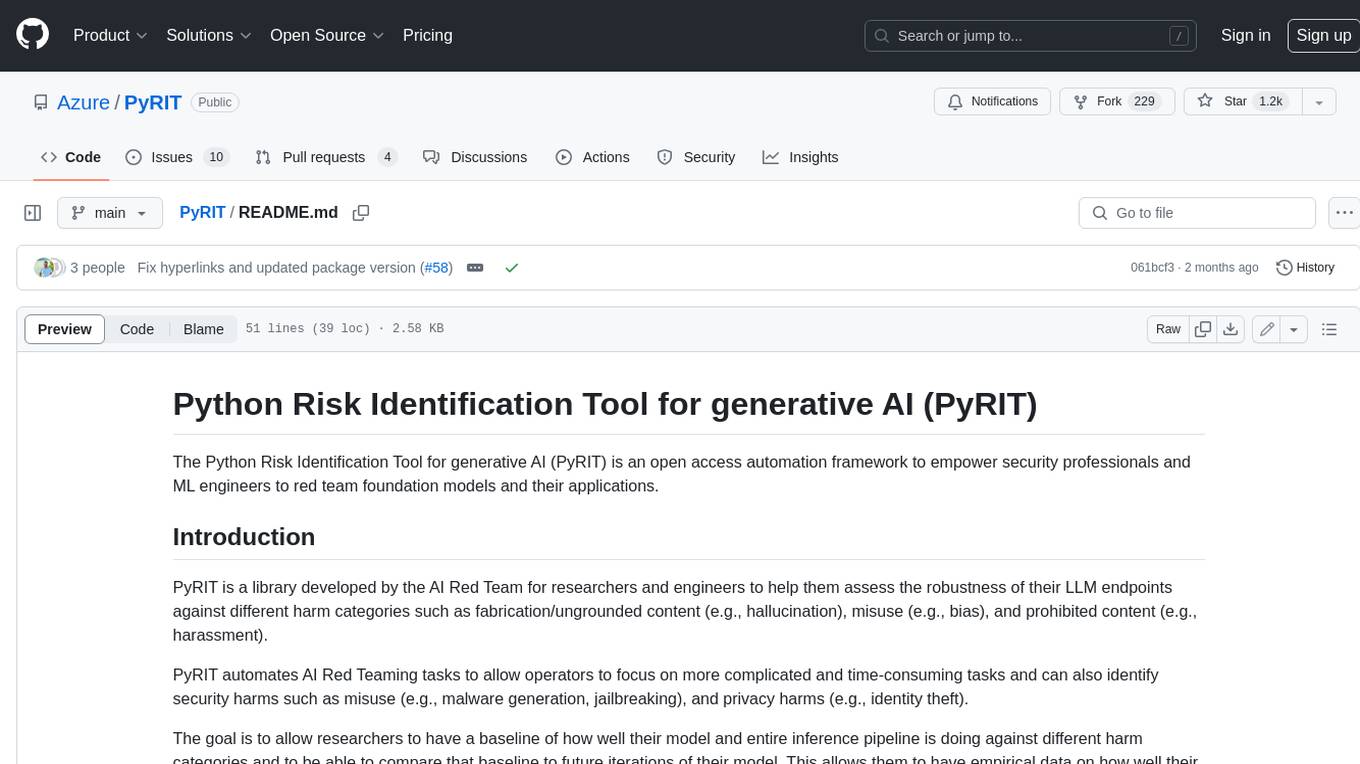
PyRIT
PyRIT is an open access automation framework designed to empower security professionals and ML engineers to red team foundation models and their applications. It automates AI Red Teaming tasks to allow operators to focus on more complicated and time-consuming tasks and can also identify security harms such as misuse (e.g., malware generation, jailbreaking), and privacy harms (e.g., identity theft). The goal is to allow researchers to have a baseline of how well their model and entire inference pipeline is doing against different harm categories and to be able to compare that baseline to future iterations of their model. This allows them to have empirical data on how well their model is doing today, and detect any degradation of performance based on future improvements.
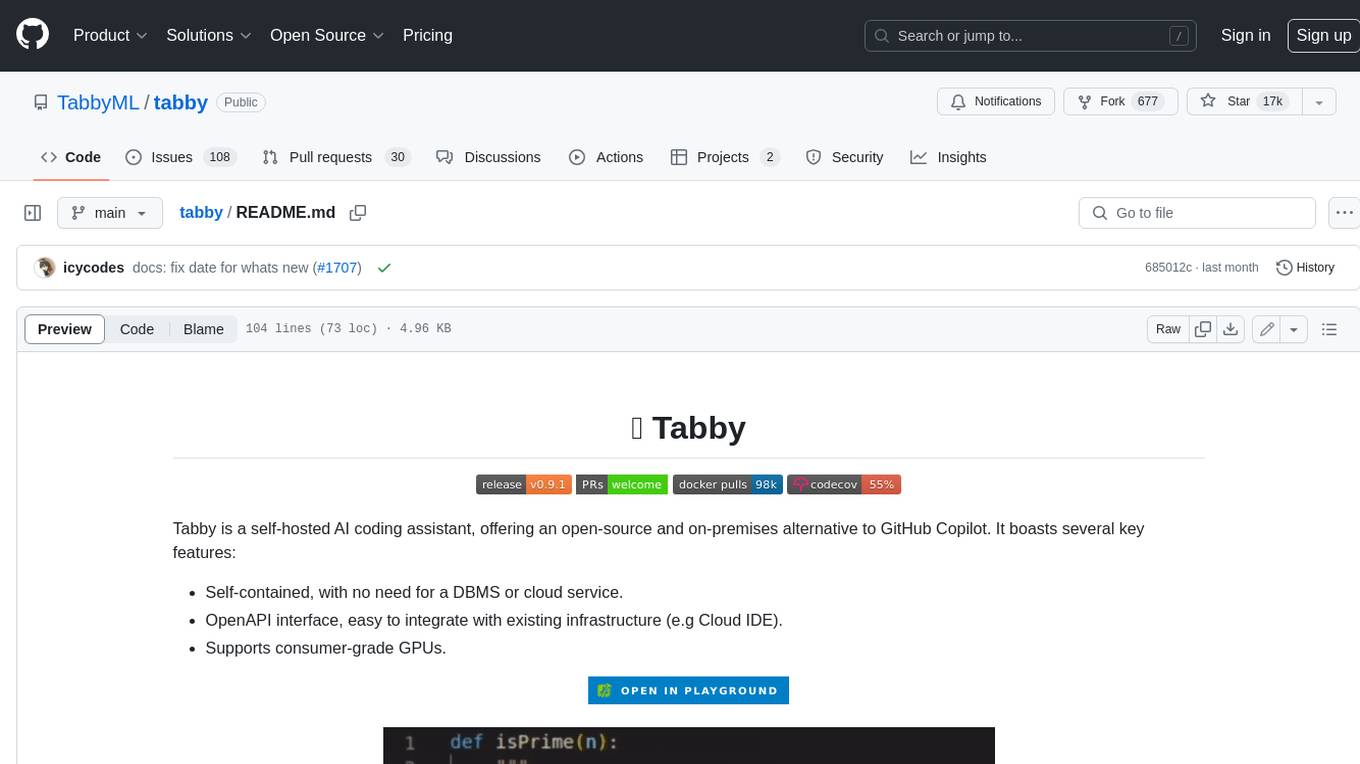
tabby
Tabby is a self-hosted AI coding assistant, offering an open-source and on-premises alternative to GitHub Copilot. It boasts several key features: * Self-contained, with no need for a DBMS or cloud service. * OpenAPI interface, easy to integrate with existing infrastructure (e.g Cloud IDE). * Supports consumer-grade GPUs.
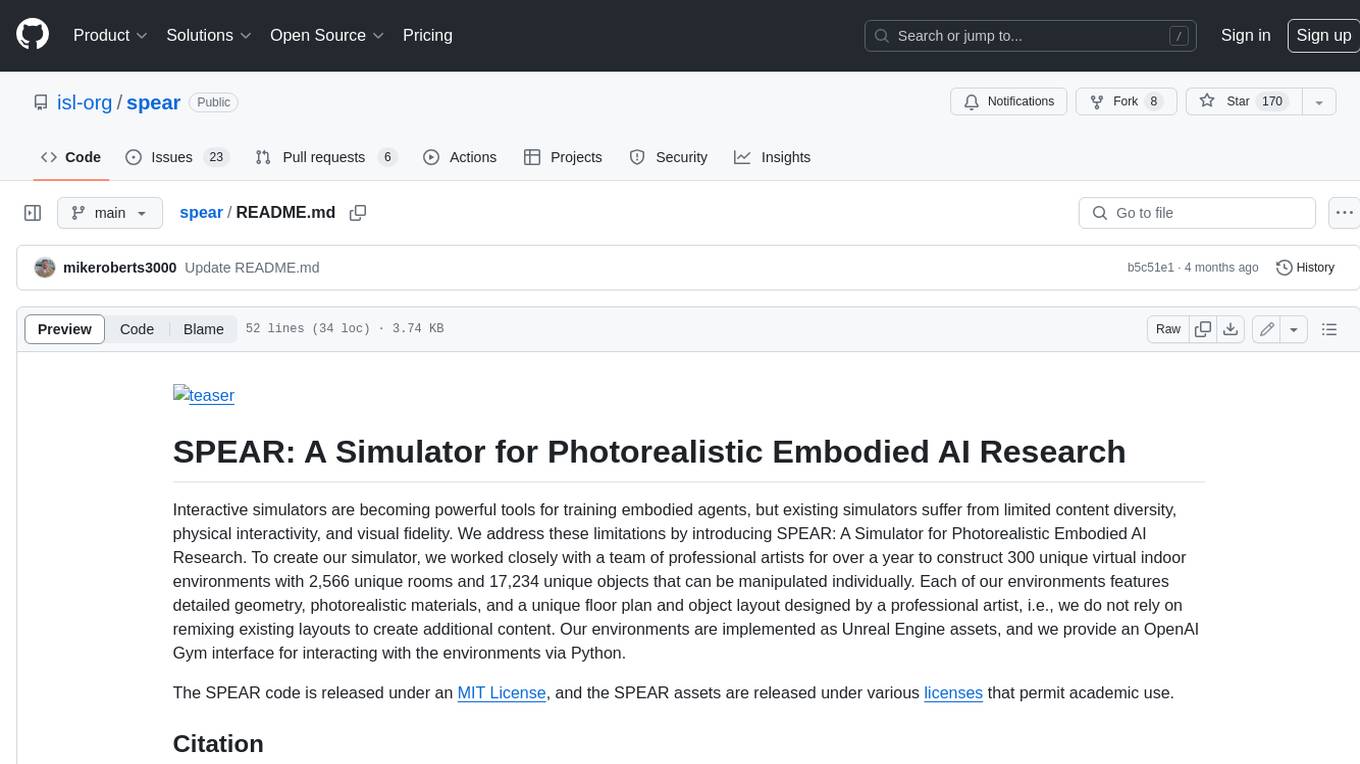
spear
SPEAR (Simulator for Photorealistic Embodied AI Research) is a powerful tool for training embodied agents. It features 300 unique virtual indoor environments with 2,566 unique rooms and 17,234 unique objects that can be manipulated individually. Each environment is designed by a professional artist and features detailed geometry, photorealistic materials, and a unique floor plan and object layout. SPEAR is implemented as Unreal Engine assets and provides an OpenAI Gym interface for interacting with the environments via Python.
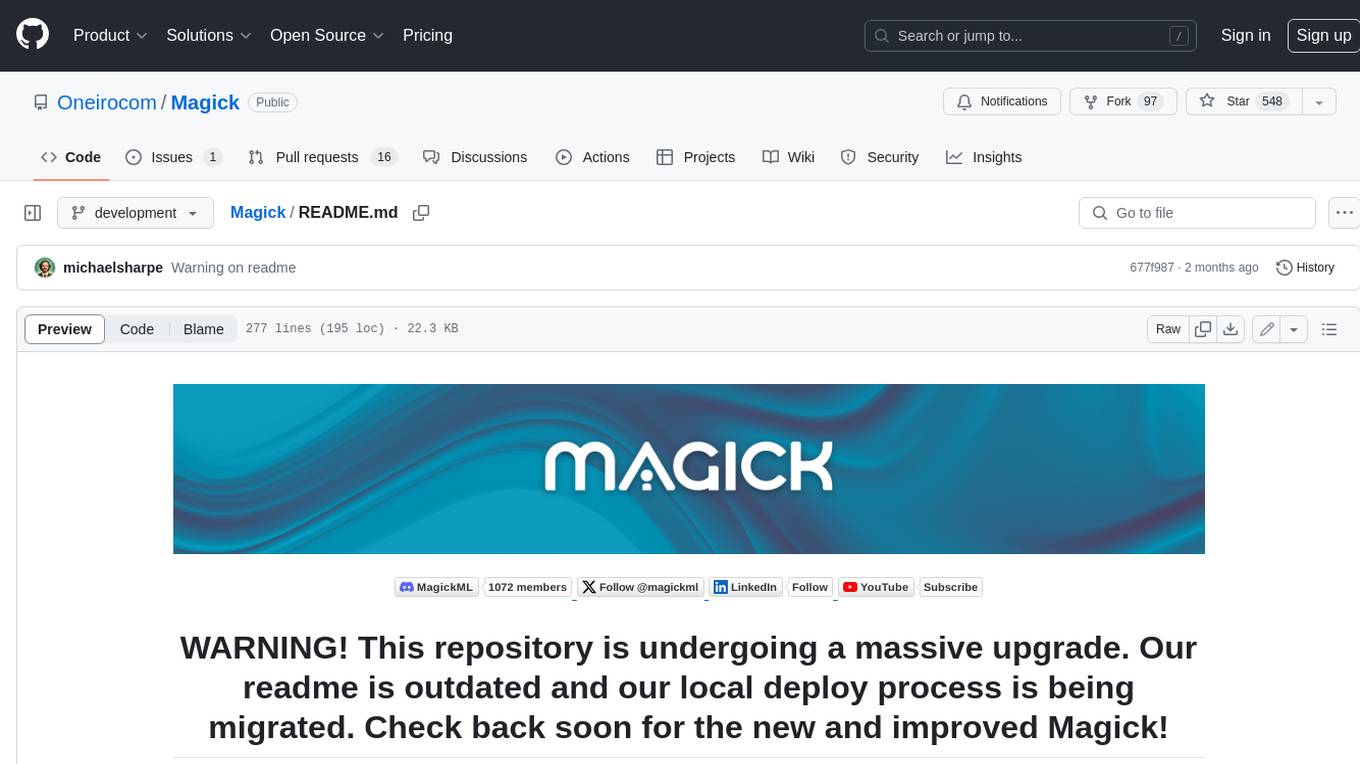
Magick
Magick is a groundbreaking visual AIDE (Artificial Intelligence Development Environment) for no-code data pipelines and multimodal agents. Magick can connect to other services and comes with nodes and templates well-suited for intelligent agents, chatbots, complex reasoning systems and realistic characters.