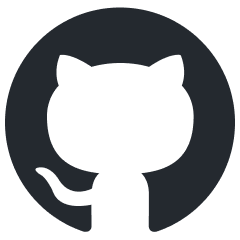
katib
Automated Machine Learning on Kubernetes
Stars: 1531
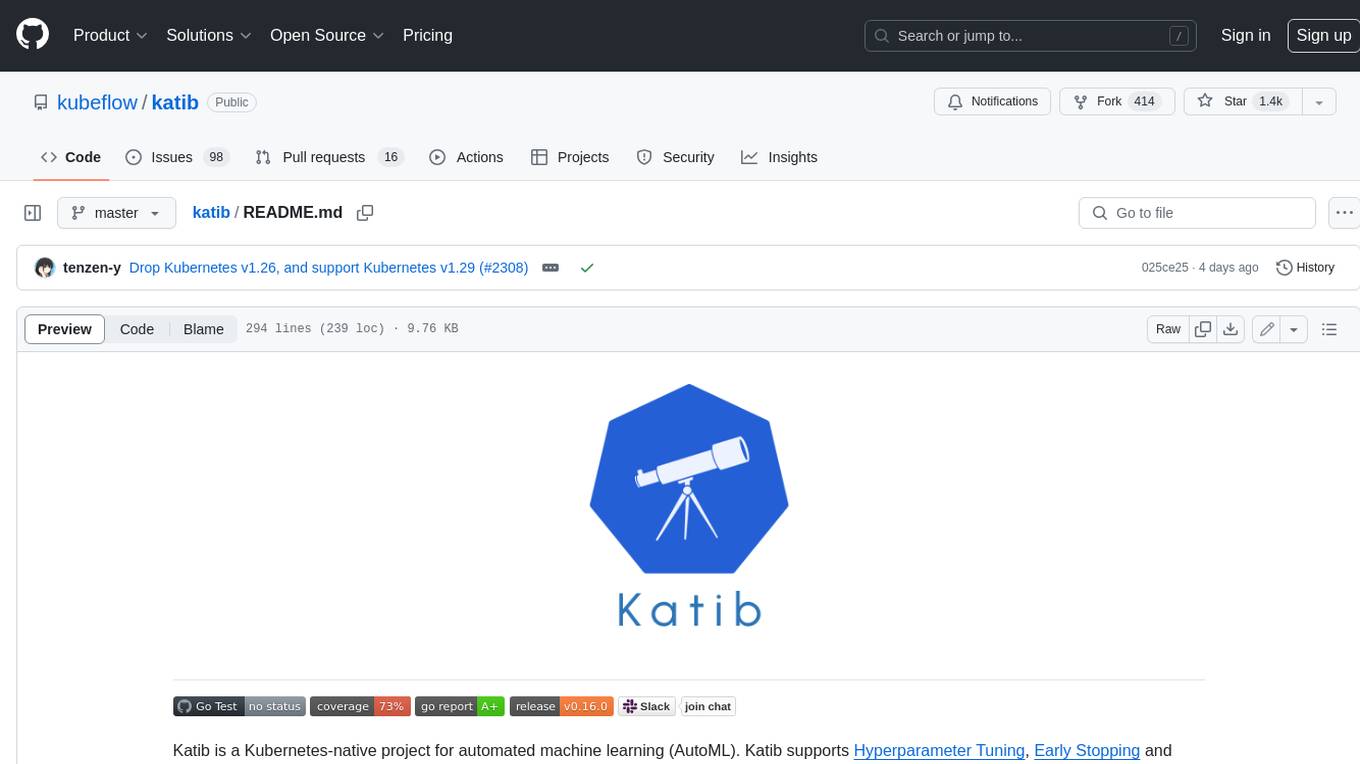
Katib is a Kubernetes-native project for automated machine learning (AutoML). Katib supports Hyperparameter Tuning, Early Stopping and Neural Architecture Search. Katib is the project which is agnostic to machine learning (ML) frameworks. It can tune hyperparameters of applications written in any language of the users’ choice and natively supports many ML frameworks, such as TensorFlow, Apache MXNet, PyTorch, XGBoost, and others. Katib can perform training jobs using any Kubernetes Custom Resources with out of the box support for Kubeflow Training Operator, Argo Workflows, Tekton Pipelines and many more.
README:
Katib is a Kubernetes-native project for automated machine learning (AutoML). Katib supports Hyperparameter Tuning, Early Stopping and Neural Architecture Search.
Katib is the project which is agnostic to machine learning (ML) frameworks. It can tune hyperparameters of applications written in any language of the users’ choice and natively supports many ML frameworks, such as TensorFlow, PyTorch, XGBoost, and others.
Katib can perform training jobs using any Kubernetes Custom Resources with out of the box support for Kubeflow Training Operator, Argo Workflows, Tekton Pipelines and many more.
Katib stands for secretary
in Arabic.
Katib supports several search algorithms. Follow the Kubeflow documentation to know more about each algorithm and check the this guide to implement your custom algorithm.
Hyperparameter Tuning | Neural Architecture Search | Early Stopping |
Random Search | ENAS | Median Stop |
Grid Search | DARTS | |
Bayesian Optimization | ||
TPE | ||
Multivariate TPE | ||
CMA-ES | ||
Sobol's Quasirandom Sequence | ||
HyperBand | ||
Population Based Training |
To perform the above algorithms Katib supports the following frameworks:
Please check the official Kubeflow documentation for prerequisites to install Katib.
Please follow the Kubeflow Katib guide for the detailed instructions on how to install Katib.
Run the following command to install the latest stable release of Katib control plane:
kubectl apply -k "github.com/kubeflow/katib.git/manifests/v1beta1/installs/katib-standalone?ref=v0.17.0"
Run the following command to install the latest changes of Katib control plane:
kubectl apply -k "github.com/kubeflow/katib.git/manifests/v1beta1/installs/katib-standalone?ref=master"
For the Katib Experiments check the complete examples list.
Katib implements a Python SDK to simplify creation of hyperparameter tuning jobs for Data Scientists.
Run the following command to install the latest stable release of Katib SDK:
pip install -U kubeflow-katib
Please refer to the getting started guide to quickly create your first hyperparameter tuning Experiment using the Python SDK.
The following links provide information on how to get involved in the community:
- Attend the bi-weekly AutoML and Training Working Group community meeting.
- Join our
#kubeflow-katib
Slack channel. - Check out who is using Katib and presentations about Katib project.
Please refer to the CONTRIBUTING guide.
If you use Katib in a scientific publication, we would appreciate citations to the following paper:
A Scalable and Cloud-Native Hyperparameter Tuning System, George et al., arXiv:2006.02085, 2020.
Bibtex entry:
@misc{george2020katib,
title={A Scalable and Cloud-Native Hyperparameter Tuning System},
author={Johnu George and Ce Gao and Richard Liu and Hou Gang Liu and Yuan Tang and Ramdoot Pydipaty and Amit Kumar Saha},
year={2020},
eprint={2006.02085},
archivePrefix={arXiv},
primaryClass={cs.DC}
}
For Tasks:
Click tags to check more tools for each tasksFor Jobs:
Alternative AI tools for katib
Similar Open Source Tools
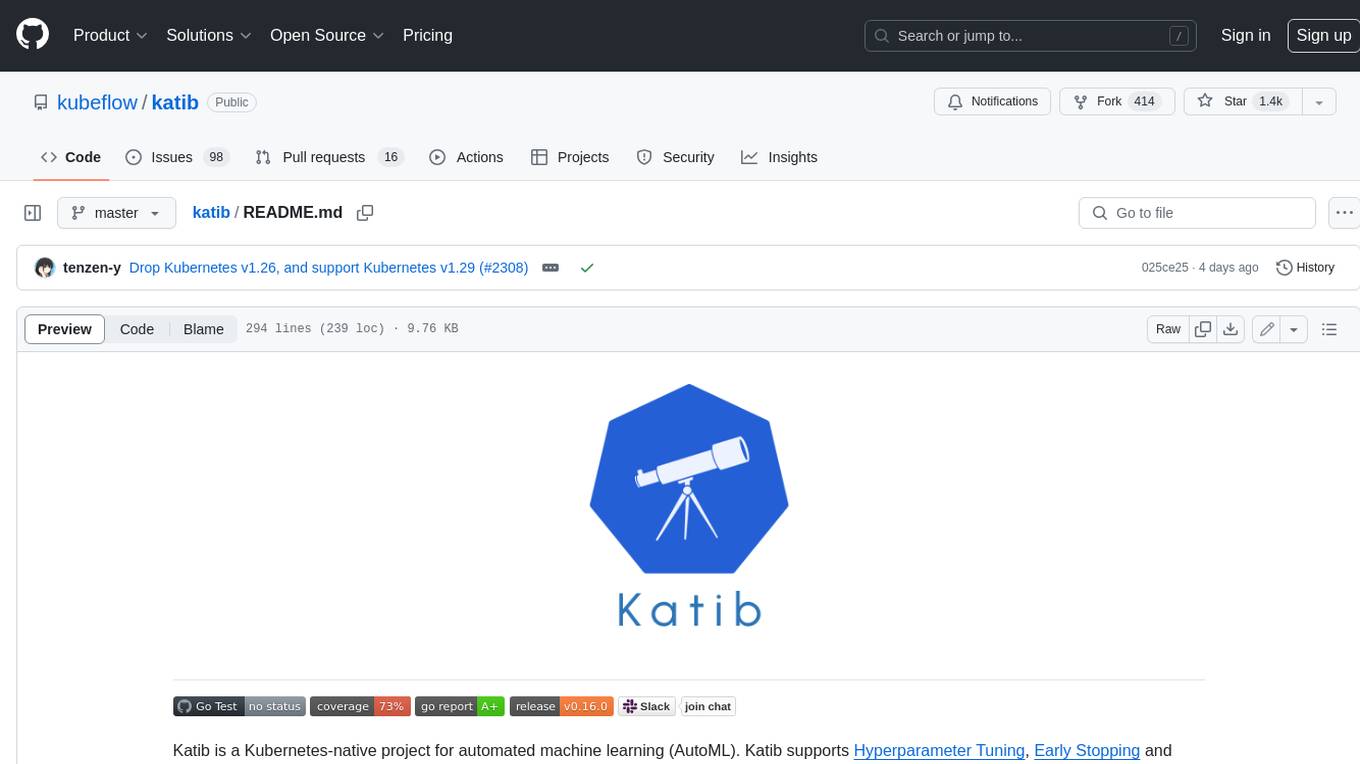
katib
Katib is a Kubernetes-native project for automated machine learning (AutoML). Katib supports Hyperparameter Tuning, Early Stopping and Neural Architecture Search. Katib is the project which is agnostic to machine learning (ML) frameworks. It can tune hyperparameters of applications written in any language of the users’ choice and natively supports many ML frameworks, such as TensorFlow, Apache MXNet, PyTorch, XGBoost, and others. Katib can perform training jobs using any Kubernetes Custom Resources with out of the box support for Kubeflow Training Operator, Argo Workflows, Tekton Pipelines and many more.
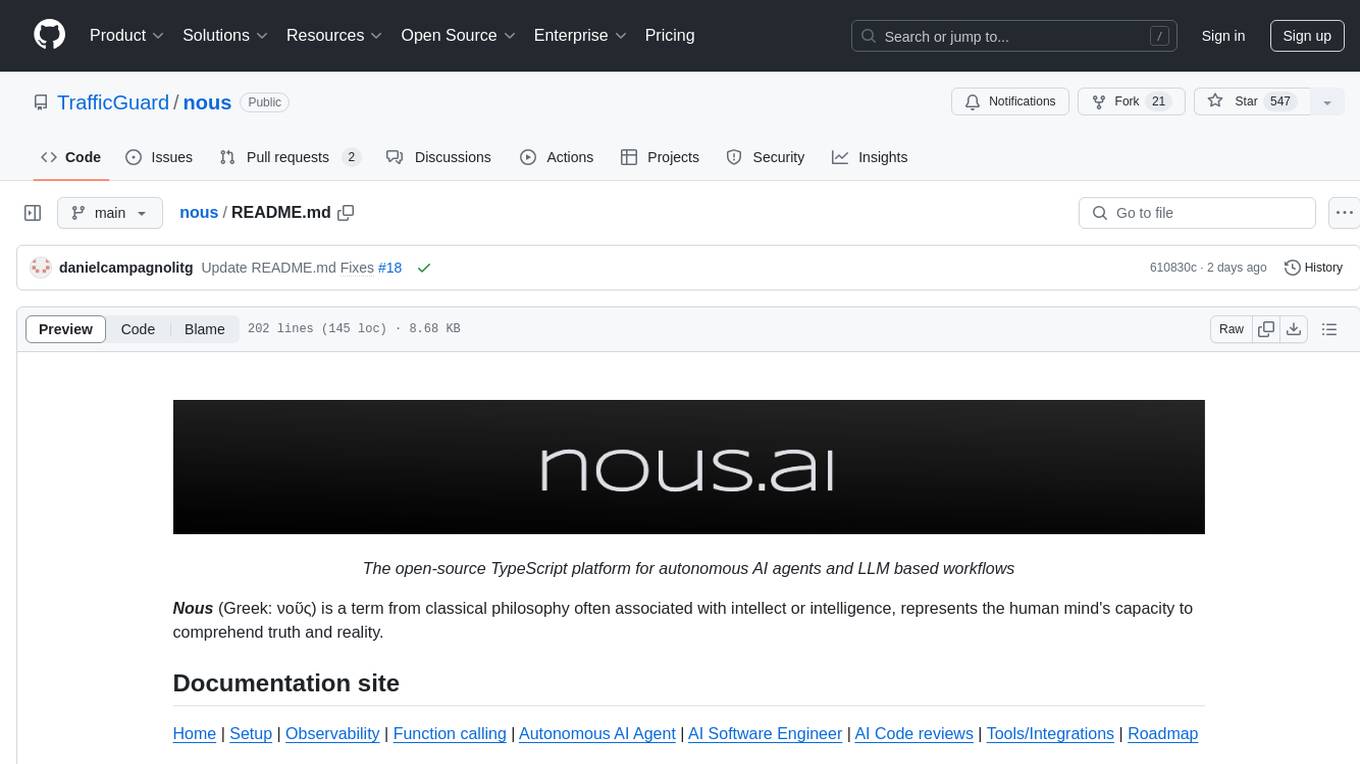
nous
Nous is an open-source TypeScript platform for autonomous AI agents and LLM based workflows. It aims to automate processes, support requests, review code, assist with refactorings, and more. The platform supports various integrations, multiple LLMs/services, CLI and web interface, human-in-the-loop interactions, flexible deployment options, observability with OpenTelemetry tracing, and specific agents for code editing, software engineering, and code review. It offers advanced features like reasoning/planning, memory and function call history, hierarchical task decomposition, and control-loop function calling options. Nous is designed to be a flexible platform for the TypeScript community to expand and support different use cases and integrations.
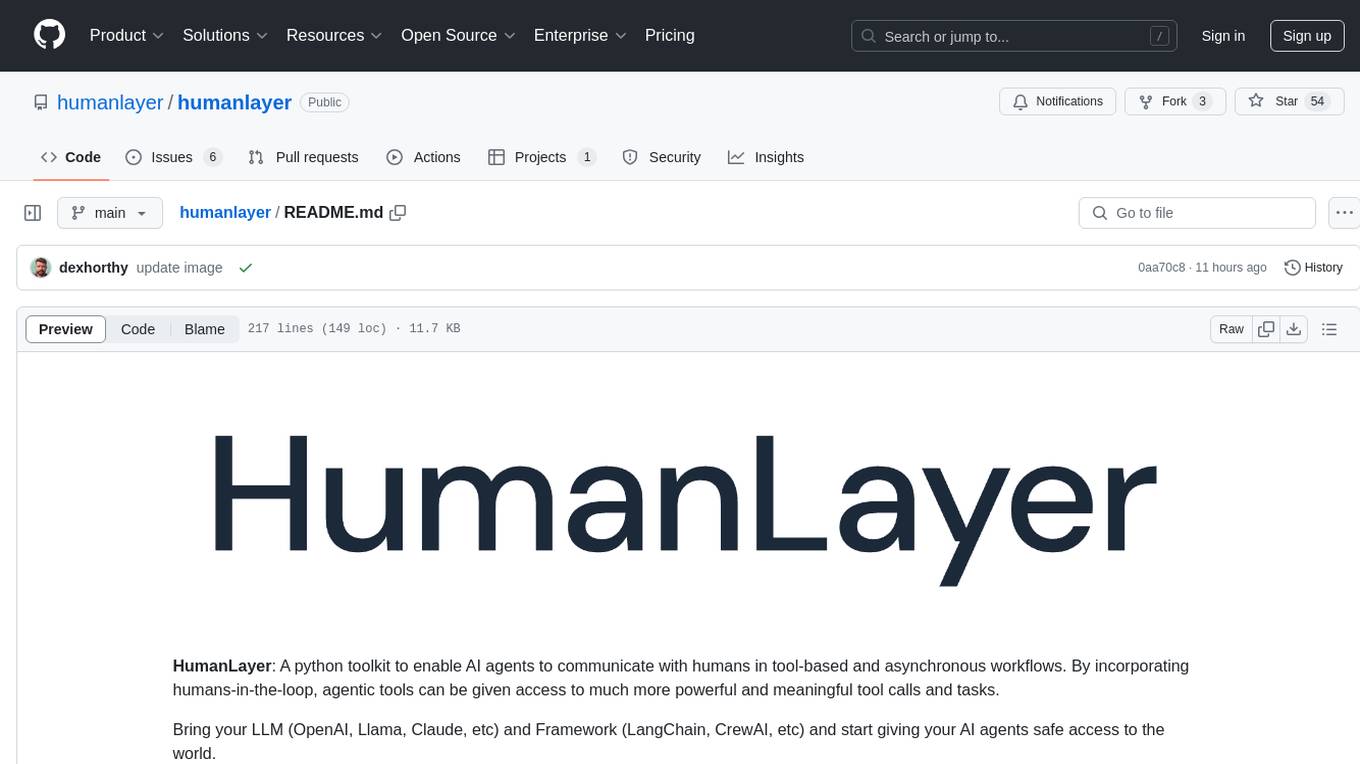
humanlayer
HumanLayer is a Python toolkit designed to enable AI agents to interact with humans in tool-based and asynchronous workflows. By incorporating humans-in-the-loop, agentic tools can access more powerful and meaningful tasks. The toolkit provides features like requiring human approval for function calls, human as a tool for contacting humans, omni-channel contact capabilities, granular routing, and support for various LLMs and orchestration frameworks. HumanLayer aims to ensure human oversight of high-stakes function calls, making AI agents more reliable and safe in executing impactful tasks.
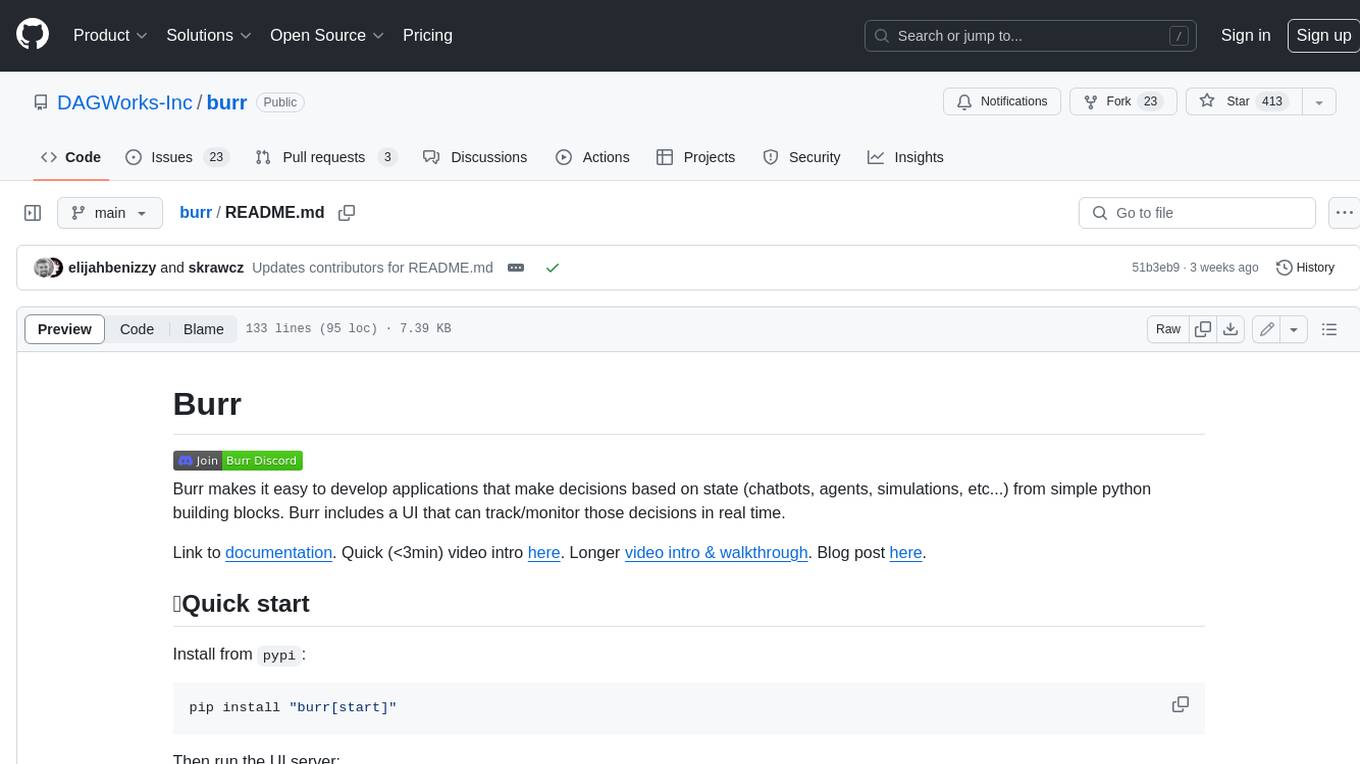
burr
Burr is a Python library and UI that makes it easy to develop applications that make decisions based on state (chatbots, agents, simulations, etc...). Burr includes a UI that can track/monitor those decisions in real time.
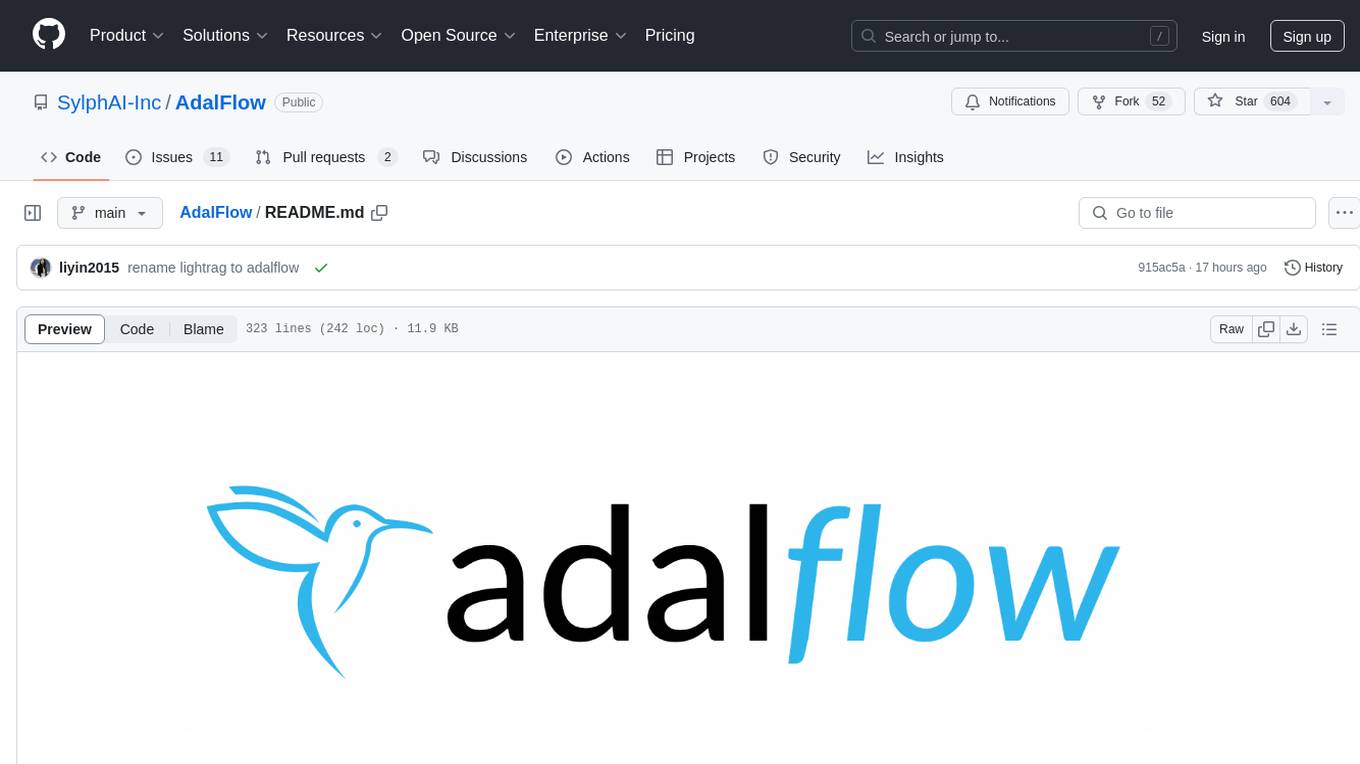
AdalFlow
AdalFlow is a library designed to help developers build and optimize Large Language Model (LLM) task pipelines. It follows a design pattern similar to PyTorch, offering a light, modular, and robust codebase. Named in honor of Ada Lovelace, AdalFlow aims to inspire more women to enter the AI field. The library is tailored for various GenAI applications like chatbots, translation, summarization, code generation, and autonomous agents, as well as classical NLP tasks such as text classification and named entity recognition. AdalFlow emphasizes modularity, robustness, and readability to support users in customizing and iterating code for their specific use cases.
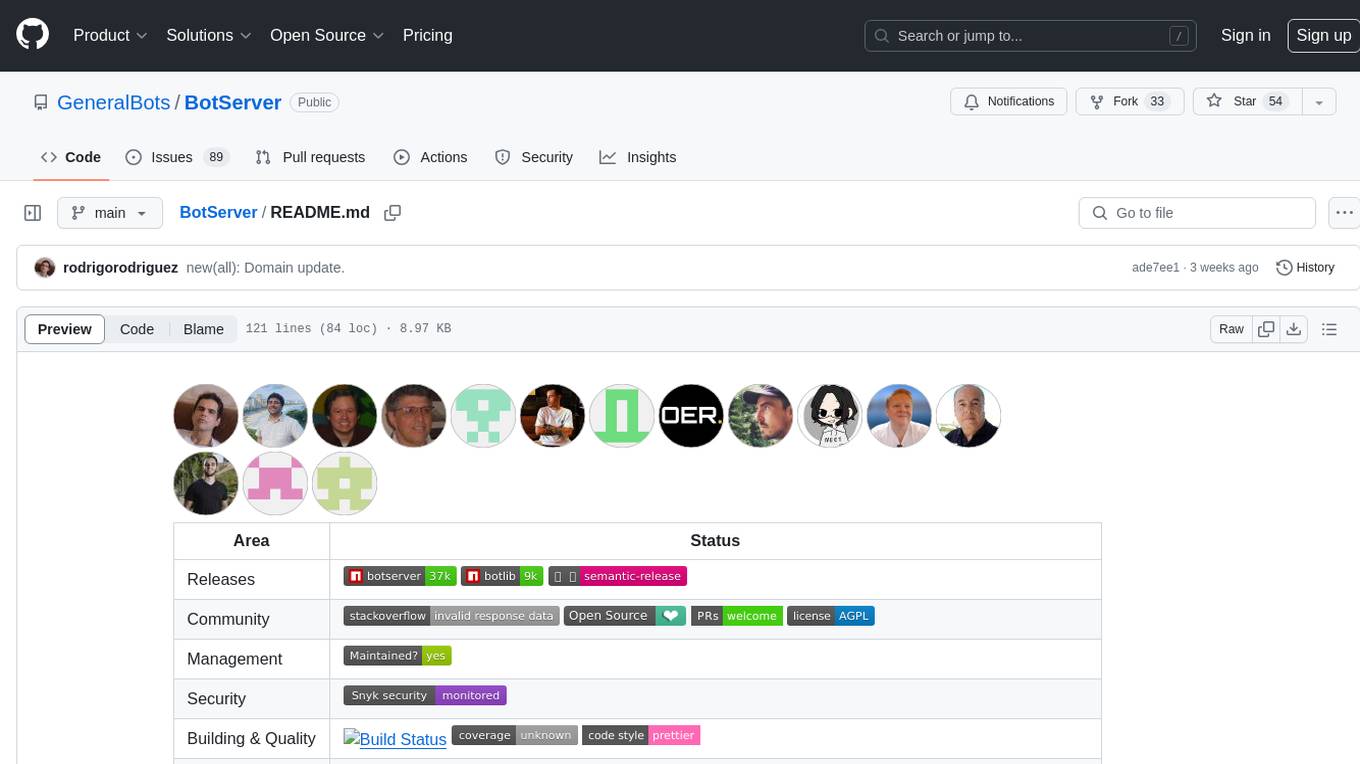
BotServer
General Bot is a chat bot server that accelerates bot development by providing code base, resources, deployment to the cloud, and templates for creating new bots. It allows modification of bot packages without code through a database and service backend. Users can develop bot packages using custom code in editors like Visual Studio Code, Atom, or Brackets. The tool supports creating bots by copying and pasting files and using favorite tools from Office or Photoshop. It also enables building custom dialogs with BASIC for extending bots.
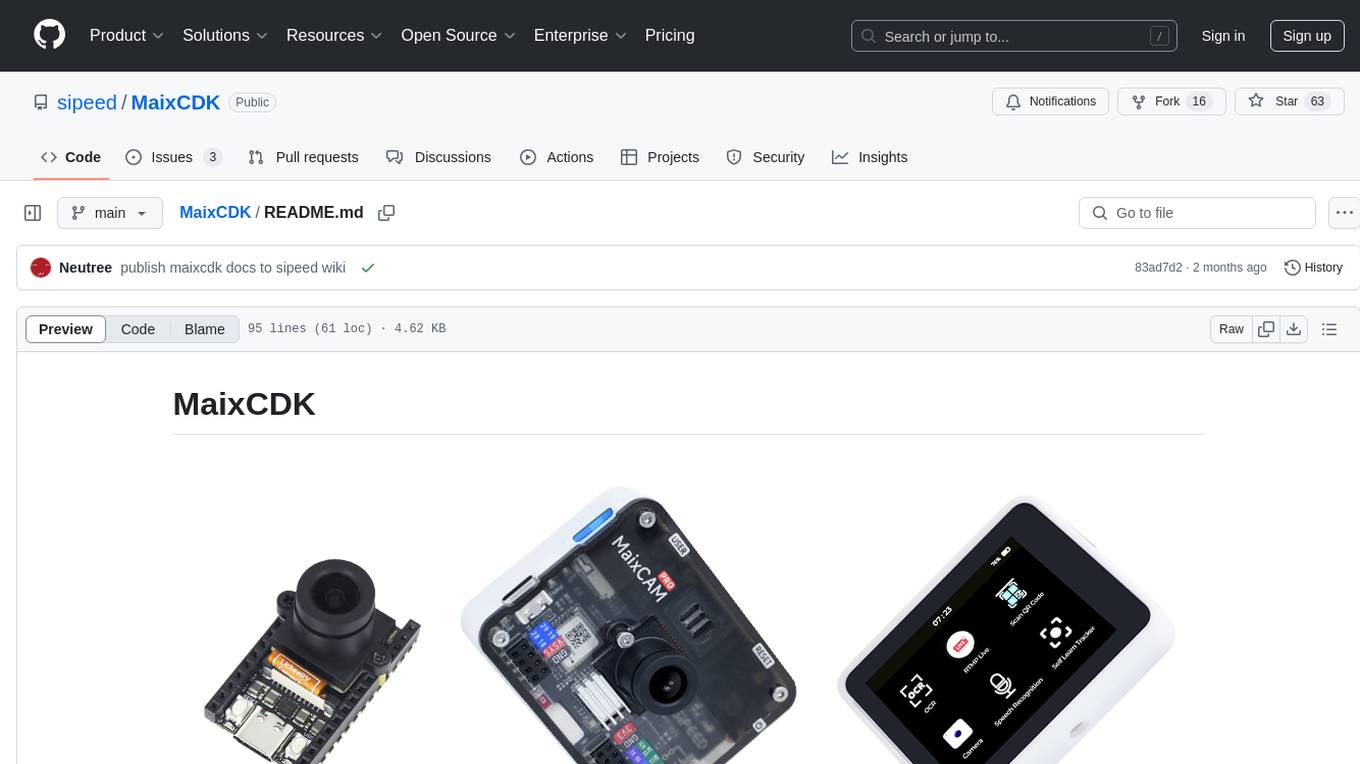
MaixCDK
MaixCDK (Maix C/CPP Development Kit) is a C/C++ development kit that integrates practical functions such as AI, machine vision, and IoT. It provides easy-to-use encapsulation for quickly building projects in vision, artificial intelligence, IoT, robotics, industrial cameras, and more. It supports hardware-accelerated execution of AI models, common vision algorithms, OpenCV, and interfaces for peripheral operations. MaixCDK offers cross-platform support, easy-to-use API, simple environment setup, online debugging, and a complete ecosystem including MaixPy and MaixVision. Supported devices include Sipeed MaixCAM, Sipeed MaixCAM-Pro, and partial support for Common Linux.
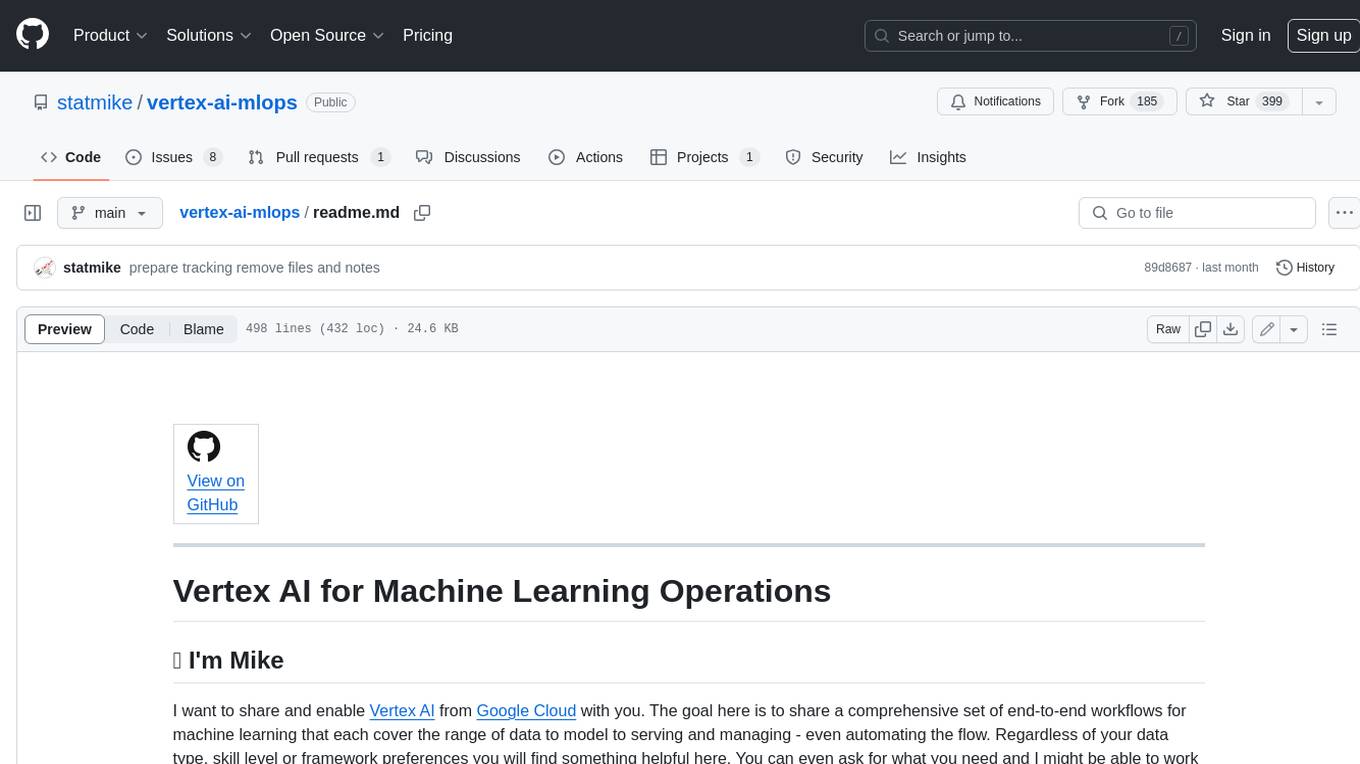
vertex-ai-mlops
Vertex AI is a platform for end-to-end model development. It consist of core components that make the processes of MLOps possible for design patterns of all types.
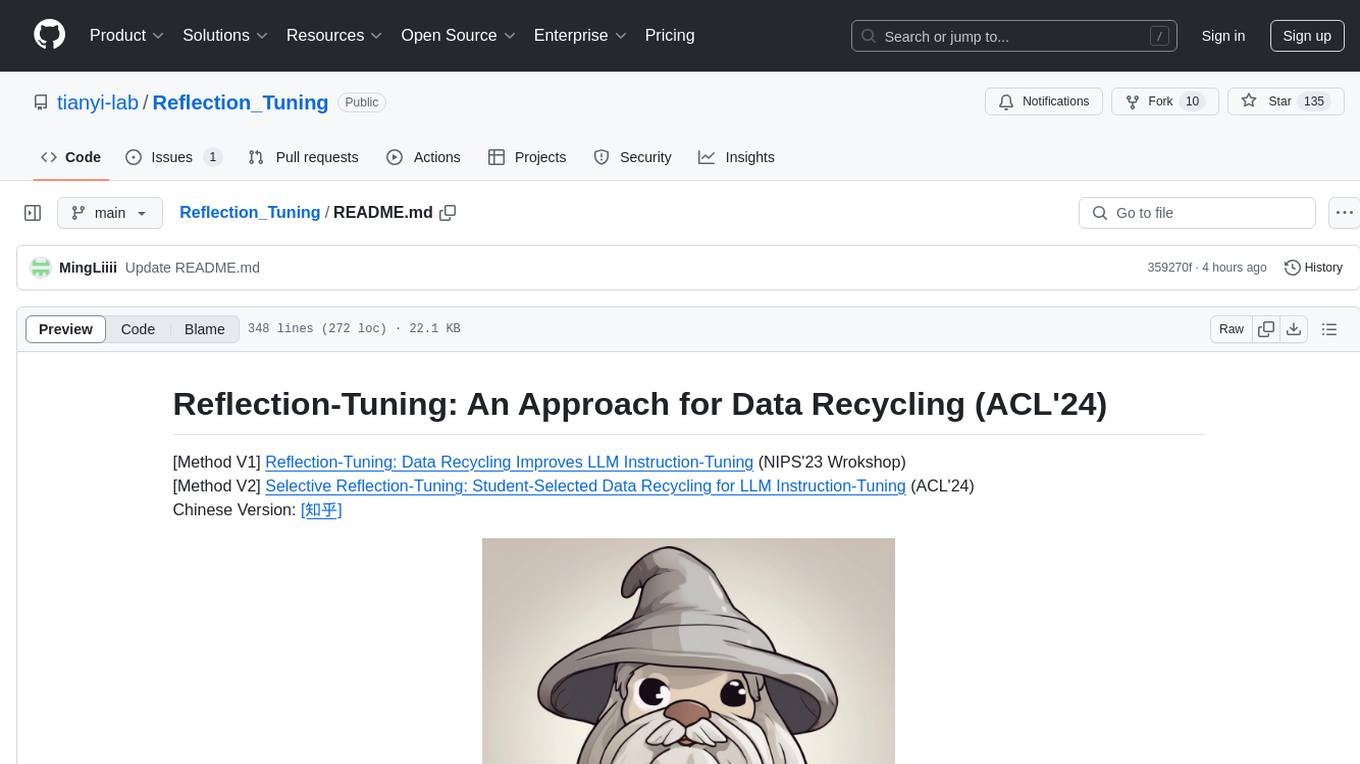
Reflection_Tuning
Reflection-Tuning is a project focused on improving the quality of instruction-tuning data through a reflection-based method. It introduces Selective Reflection-Tuning, where the student model can decide whether to accept the improvements made by the teacher model. The project aims to generate high-quality instruction-response pairs by defining specific criteria for the oracle model to follow and respond to. It also evaluates the efficacy and relevance of instruction-response pairs using the r-IFD metric. The project provides code for reflection and selection processes, along with data and model weights for both V1 and V2 methods.
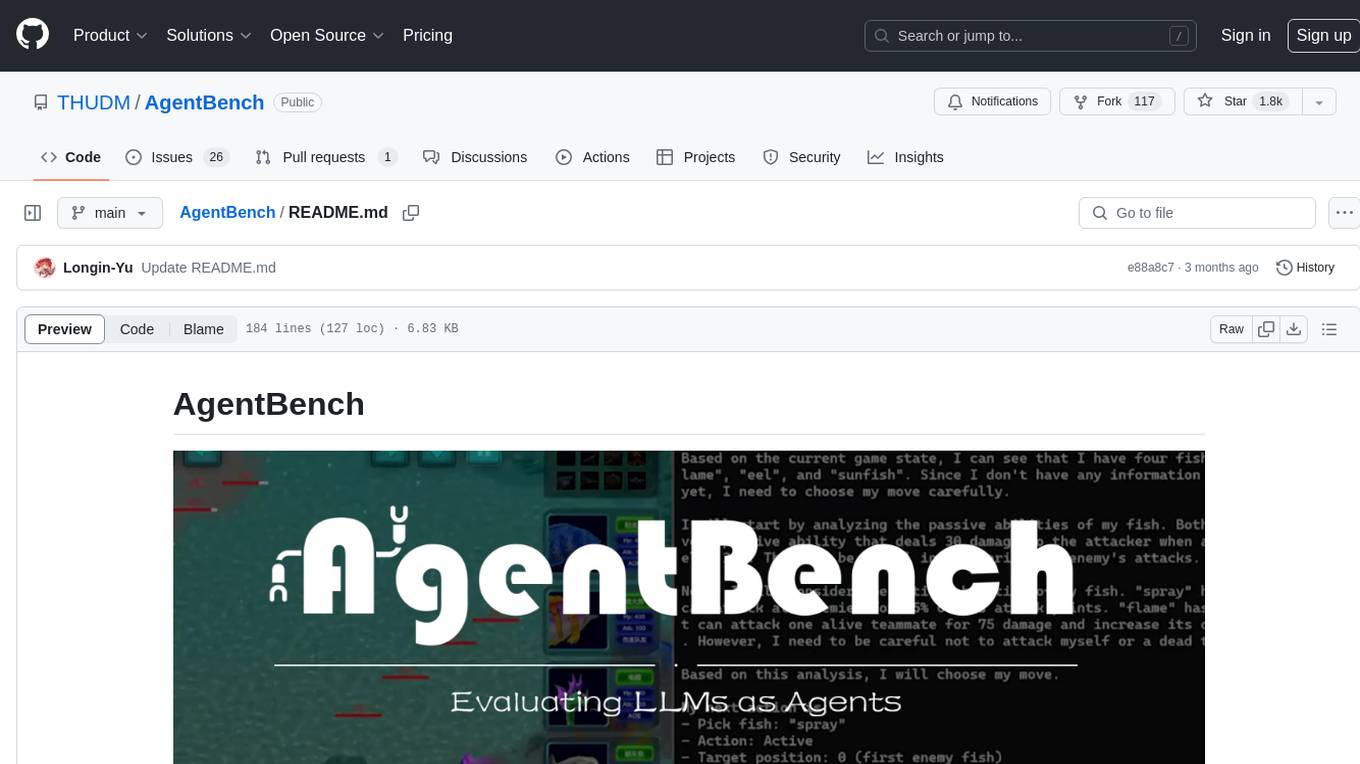
AgentBench
AgentBench is a benchmark designed to evaluate Large Language Models (LLMs) as autonomous agents in various environments. It includes 8 distinct environments such as Operating System, Database, Knowledge Graph, Digital Card Game, and Lateral Thinking Puzzles. The tool provides a comprehensive evaluation of LLMs' ability to operate as agents by offering Dev and Test sets for each environment. Users can quickly start using the tool by following the provided steps, configuring the agent, starting task servers, and assigning tasks. AgentBench aims to bridge the gap between LLMs' proficiency as agents and their practical usability.
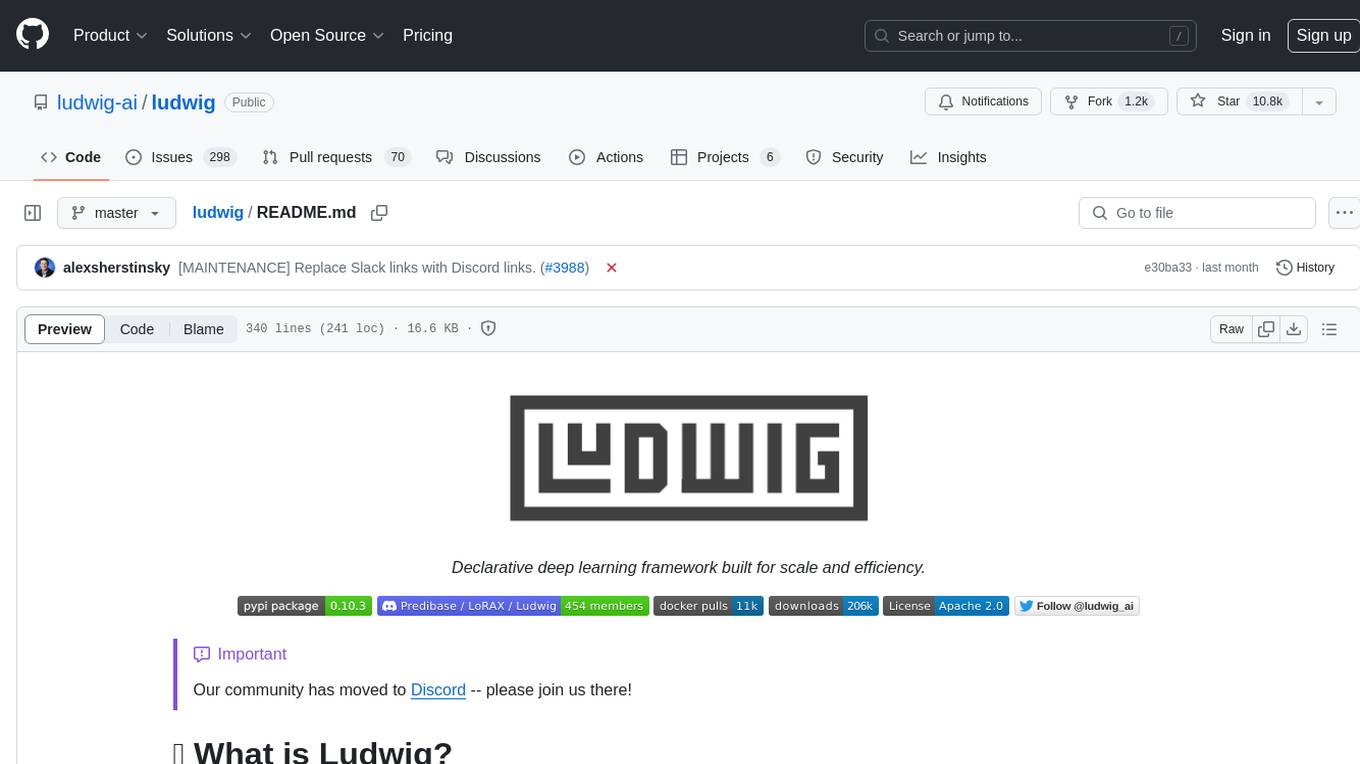
ludwig
Ludwig is a declarative deep learning framework designed for scale and efficiency. It is a low-code framework that allows users to build custom AI models like LLMs and other deep neural networks with ease. Ludwig offers features such as optimized scale and efficiency, expert level control, modularity, and extensibility. It is engineered for production with prebuilt Docker containers, support for running with Ray on Kubernetes, and the ability to export models to Torchscript and Triton. Ludwig is hosted by the Linux Foundation AI & Data.
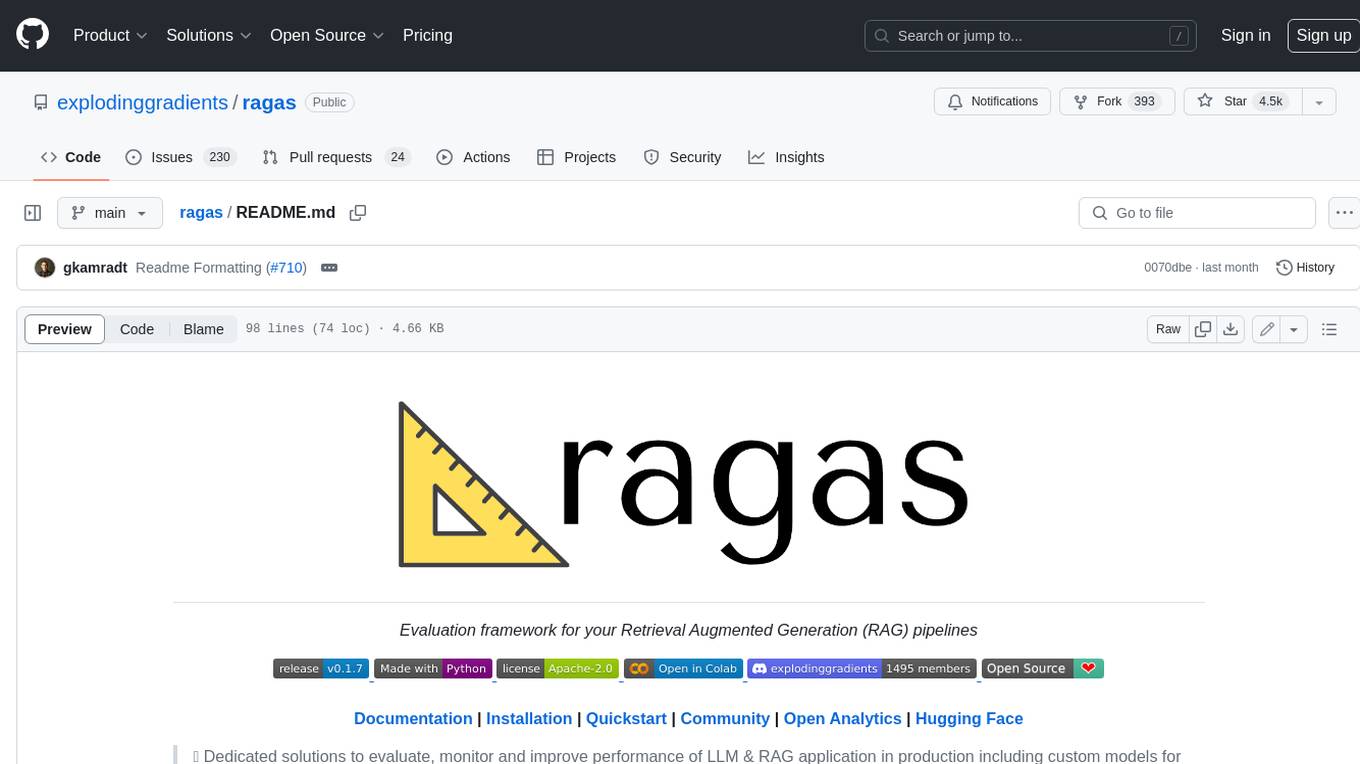
ragas
Ragas is a framework that helps you evaluate your Retrieval Augmented Generation (RAG) pipelines. RAG denotes a class of LLM applications that use external data to augment the LLM’s context. There are existing tools and frameworks that help you build these pipelines but evaluating it and quantifying your pipeline performance can be hard. This is where Ragas (RAG Assessment) comes in. Ragas provides you with the tools based on the latest research for evaluating LLM-generated text to give you insights about your RAG pipeline. Ragas can be integrated with your CI/CD to provide continuous checks to ensure performance.
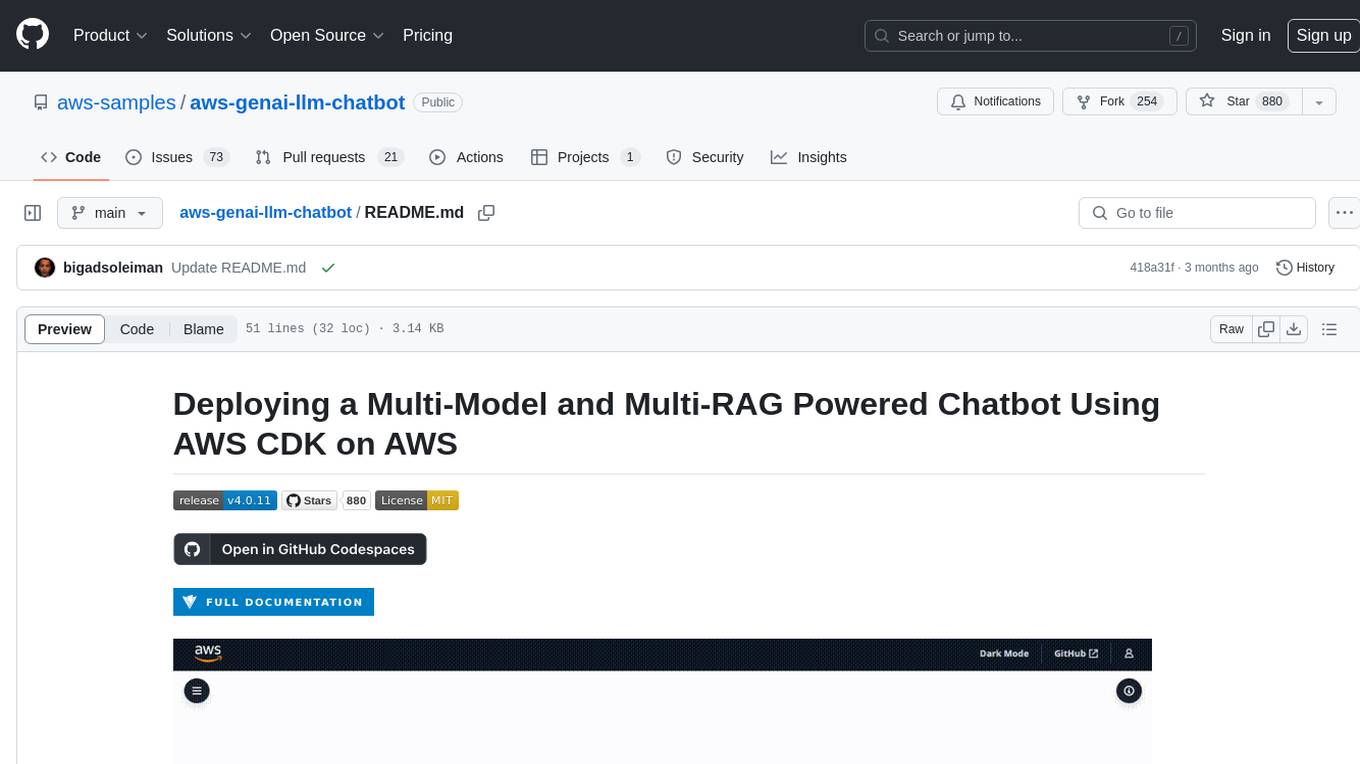
aws-genai-llm-chatbot
This repository provides code to deploy a chatbot powered by Multi-Model and Multi-RAG using AWS CDK on AWS. Users can experiment with various Large Language Models and Multimodal Language Models from different providers. The solution supports Amazon Bedrock, Amazon SageMaker self-hosted models, and third-party providers via API. It also offers additional resources like AWS Generative AI CDK Constructs and Project Lakechain for building generative AI solutions and document processing. The roadmap and authors are listed, along with contributors. The library is licensed under the MIT-0 License with information on changelog, code of conduct, and contributing guidelines. A legal disclaimer advises users to conduct their own assessment before using the content for production purposes.
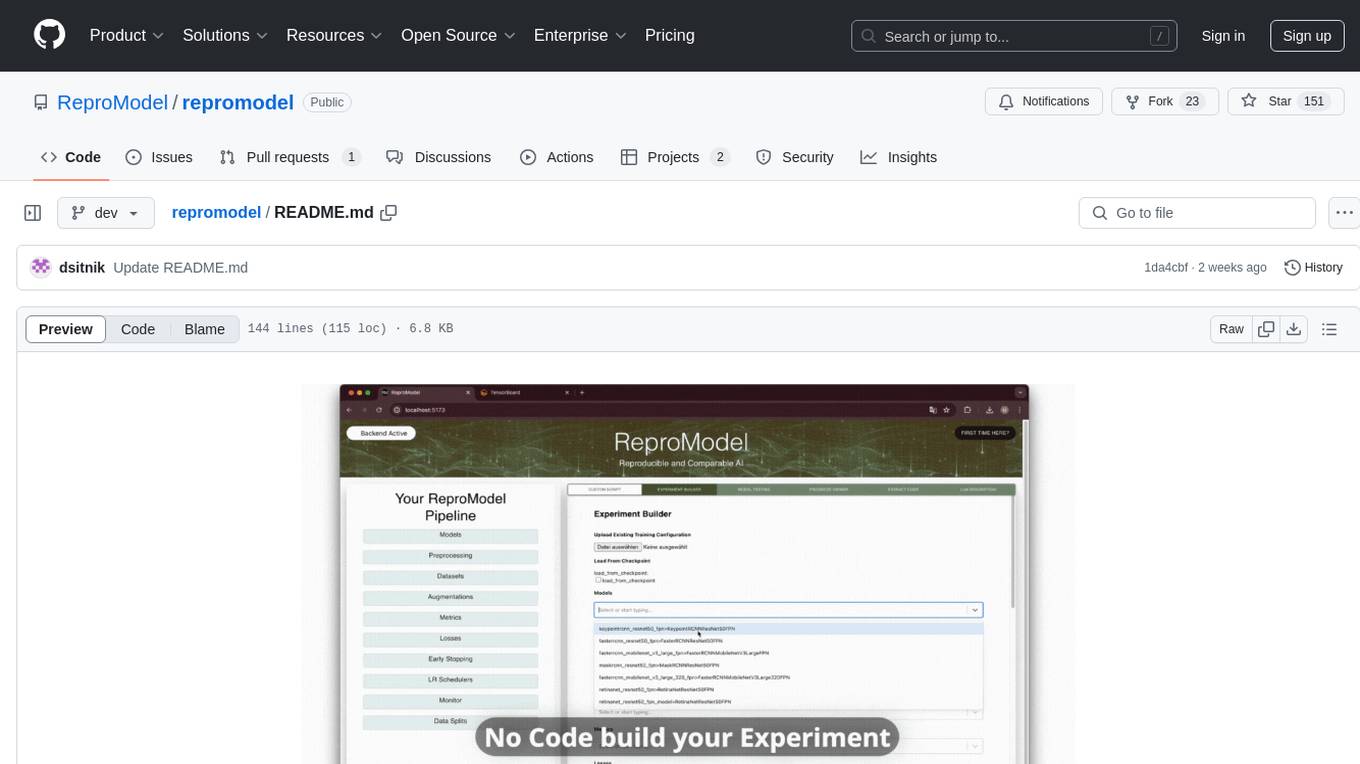
repromodel
ReproModel is an open-source toolbox designed to boost AI research efficiency by enabling researchers to reproduce, compare, train, and test AI models faster. It provides standardized models, dataloaders, and processing procedures, allowing researchers to focus on new datasets and model development. With a no-code solution, users can access benchmark and SOTA models and datasets, utilize training visualizations, extract code for publication, and leverage an LLM-powered automated methodology description writer. The toolbox helps researchers modularize development, compare pipeline performance reproducibly, and reduce time for model development, computation, and writing. Future versions aim to facilitate building upon state-of-the-art research by loading previously published study IDs with verified code, experiments, and results stored in the system.
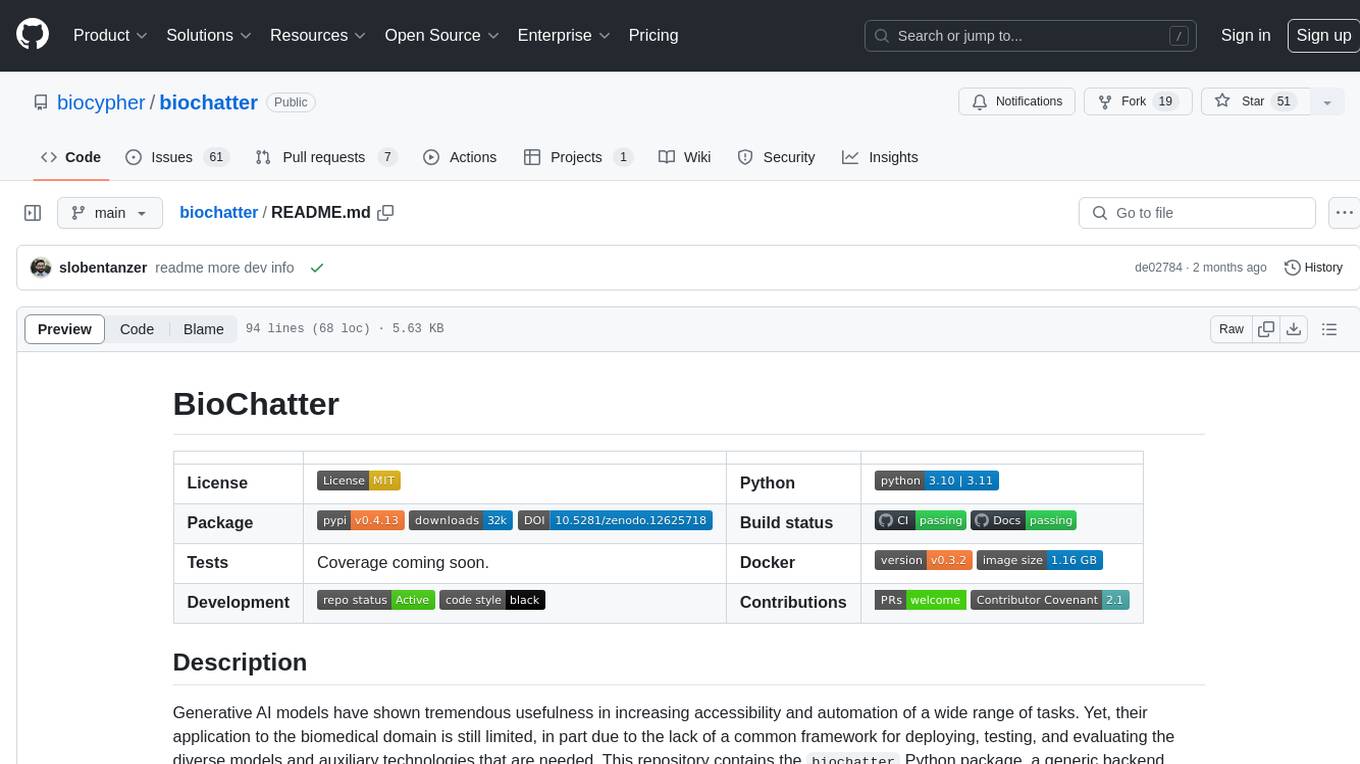
biochatter
Generative AI models have shown tremendous usefulness in increasing accessibility and automation of a wide range of tasks. This repository contains the `biochatter` Python package, a generic backend library for the connection of biomedical applications to conversational AI. It aims to provide a common framework for deploying, testing, and evaluating diverse models and auxiliary technologies in the biomedical domain. BioChatter is part of the BioCypher ecosystem, connecting natively to BioCypher knowledge graphs.
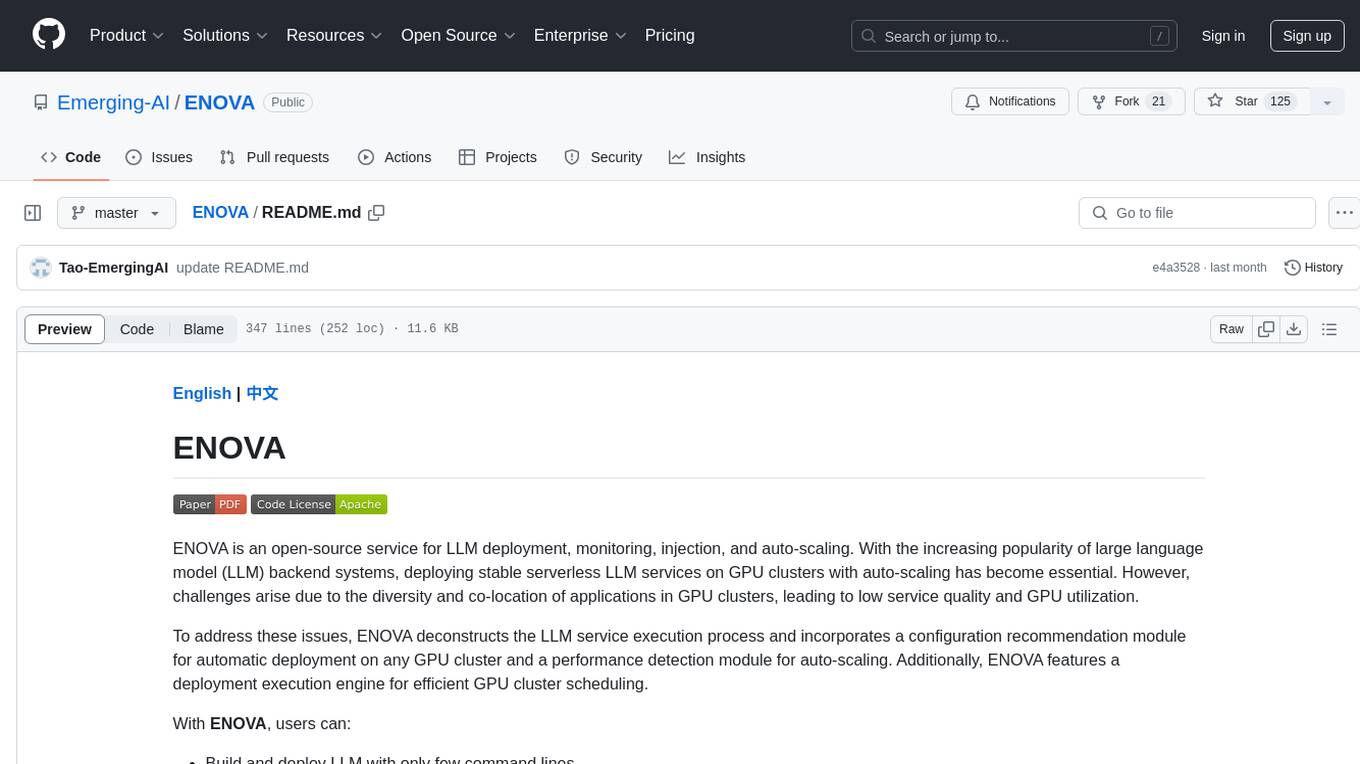
ENOVA
ENOVA is an open-source service for Large Language Model (LLM) deployment, monitoring, injection, and auto-scaling. It addresses challenges in deploying stable serverless LLM services on GPU clusters with auto-scaling by deconstructing the LLM service execution process and providing configuration recommendations and performance detection. Users can build and deploy LLM with few command lines, recommend optimal computing resources, experience LLM performance, observe operating status, achieve load balancing, and more. ENOVA ensures stable operation, cost-effectiveness, efficiency, and strong scalability of LLM services.
For similar tasks
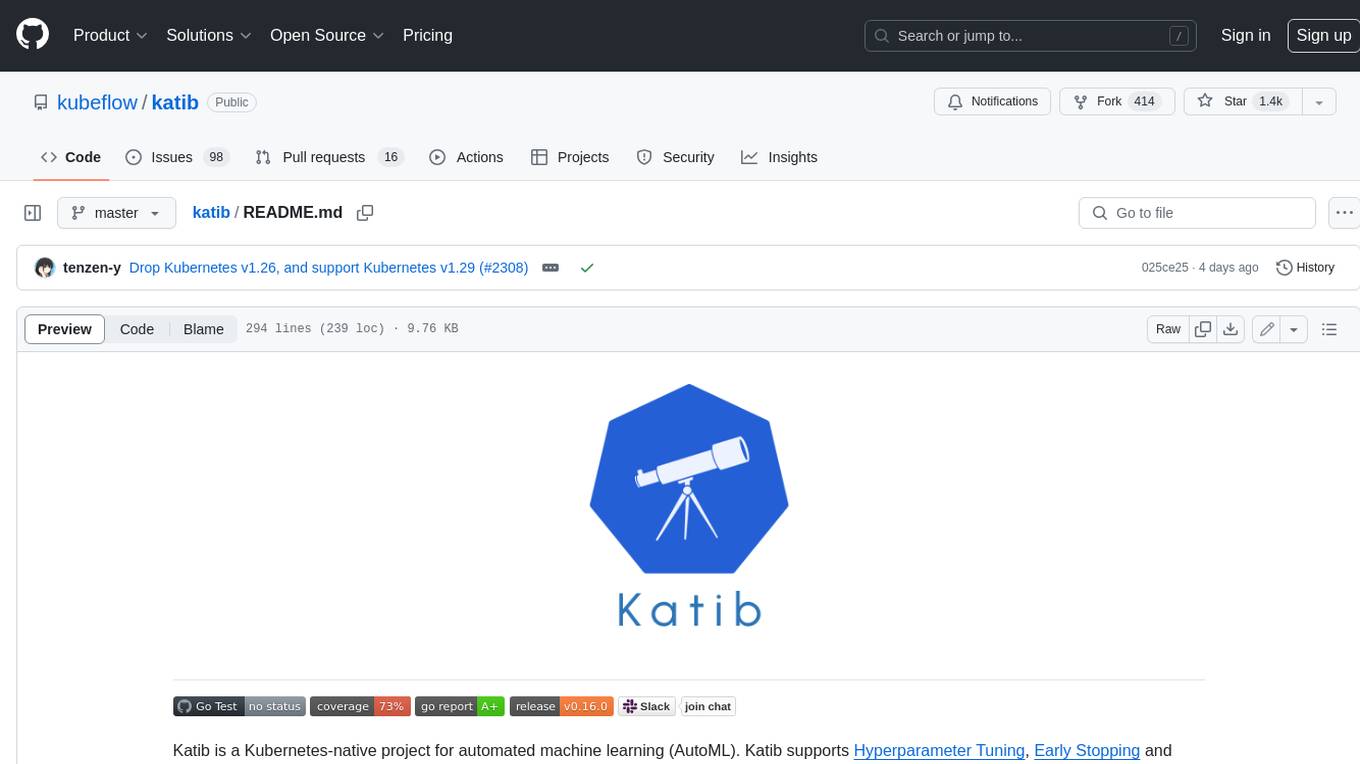
katib
Katib is a Kubernetes-native project for automated machine learning (AutoML). Katib supports Hyperparameter Tuning, Early Stopping and Neural Architecture Search. Katib is the project which is agnostic to machine learning (ML) frameworks. It can tune hyperparameters of applications written in any language of the users’ choice and natively supports many ML frameworks, such as TensorFlow, Apache MXNet, PyTorch, XGBoost, and others. Katib can perform training jobs using any Kubernetes Custom Resources with out of the box support for Kubeflow Training Operator, Argo Workflows, Tekton Pipelines and many more.
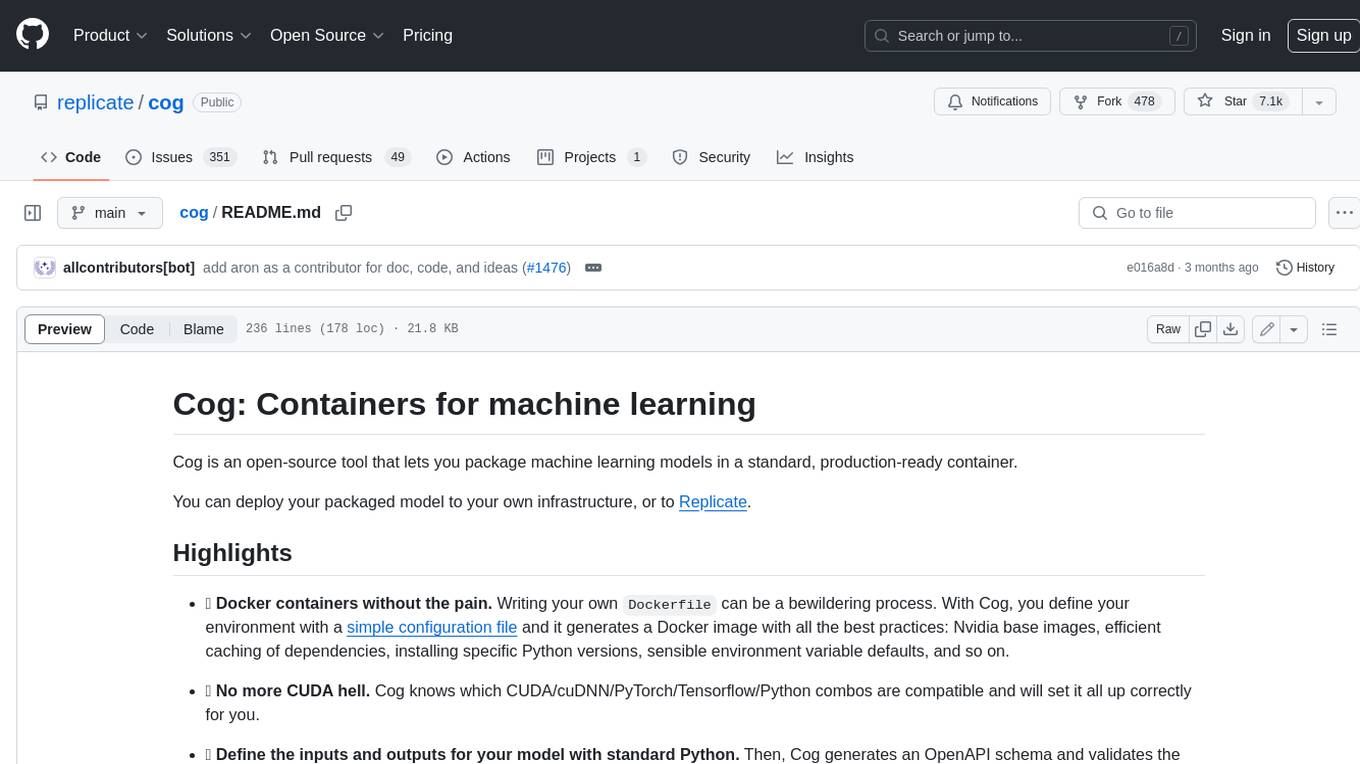
cog
Cog is an open-source tool that lets you package machine learning models in a standard, production-ready container. You can deploy your packaged model to your own infrastructure, or to Replicate.
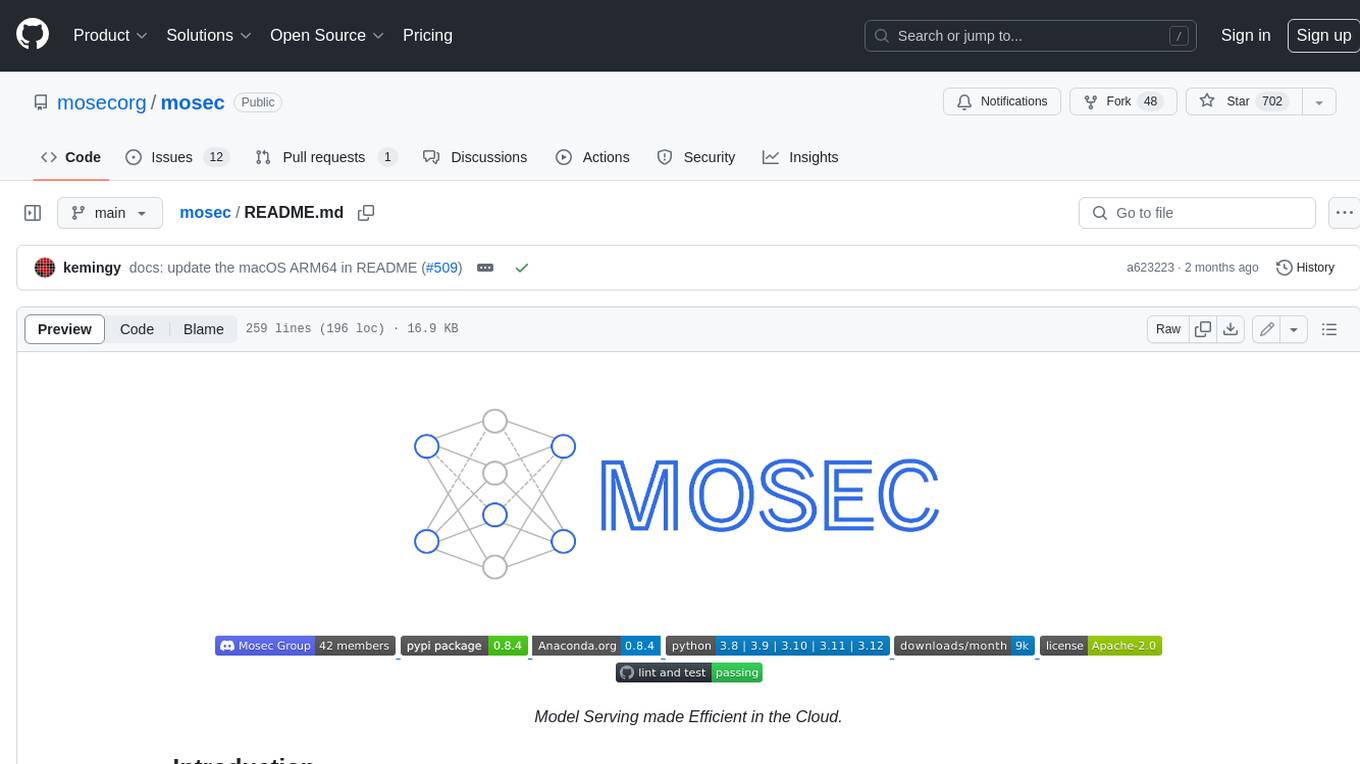
mosec
Mosec is a high-performance and flexible model serving framework for building ML model-enabled backend and microservices. It bridges the gap between any machine learning models you just trained and the efficient online service API. * **Highly performant** : web layer and task coordination built with Rust 🦀, which offers blazing speed in addition to efficient CPU utilization powered by async I/O * **Ease of use** : user interface purely in Python 🐍, by which users can serve their models in an ML framework-agnostic manner using the same code as they do for offline testing * **Dynamic batching** : aggregate requests from different users for batched inference and distribute results back * **Pipelined stages** : spawn multiple processes for pipelined stages to handle CPU/GPU/IO mixed workloads * **Cloud friendly** : designed to run in the cloud, with the model warmup, graceful shutdown, and Prometheus monitoring metrics, easily managed by Kubernetes or any container orchestration systems * **Do one thing well** : focus on the online serving part, users can pay attention to the model optimization and business logic
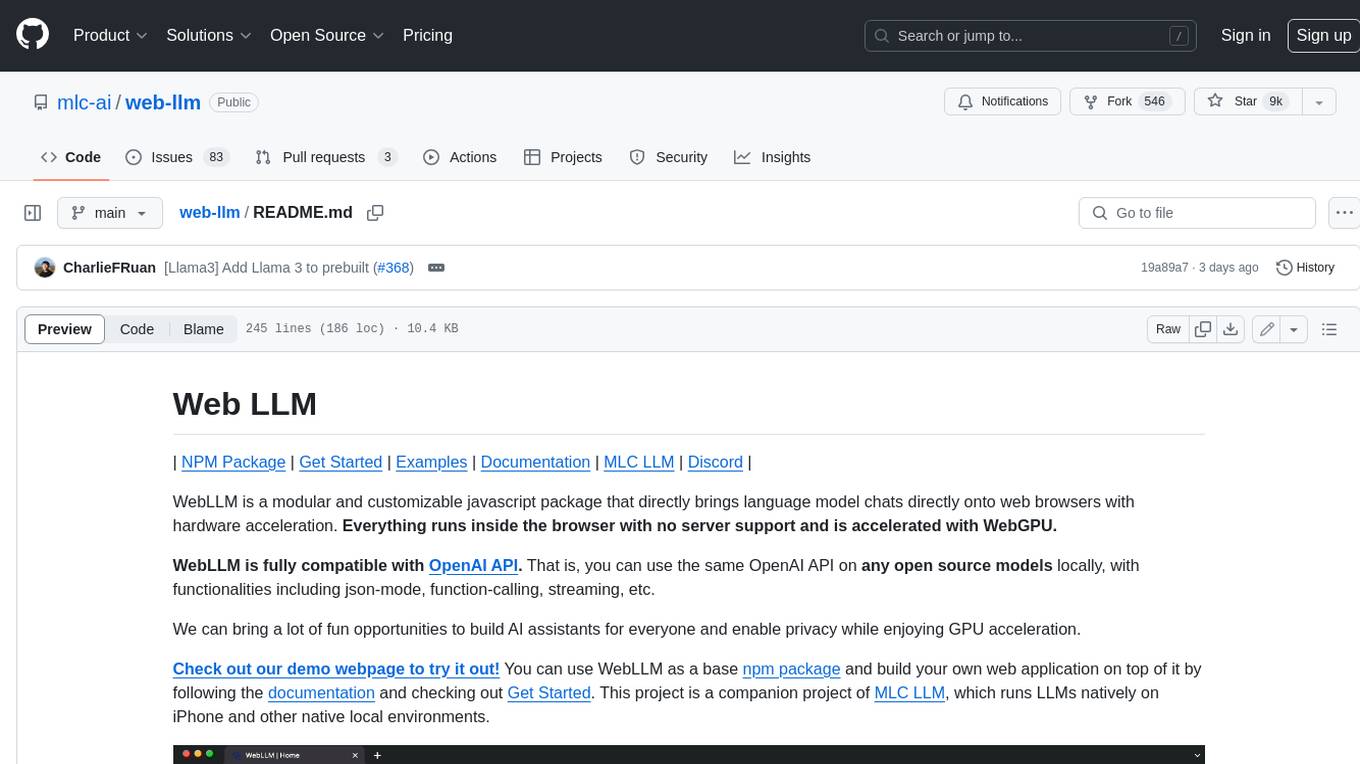
web-llm
WebLLM is a modular and customizable javascript package that directly brings language model chats directly onto web browsers with hardware acceleration. Everything runs inside the browser with no server support and is accelerated with WebGPU. WebLLM is fully compatible with OpenAI API. That is, you can use the same OpenAI API on any open source models locally, with functionalities including json-mode, function-calling, streaming, etc. We can bring a lot of fun opportunities to build AI assistants for everyone and enable privacy while enjoying GPU acceleration.
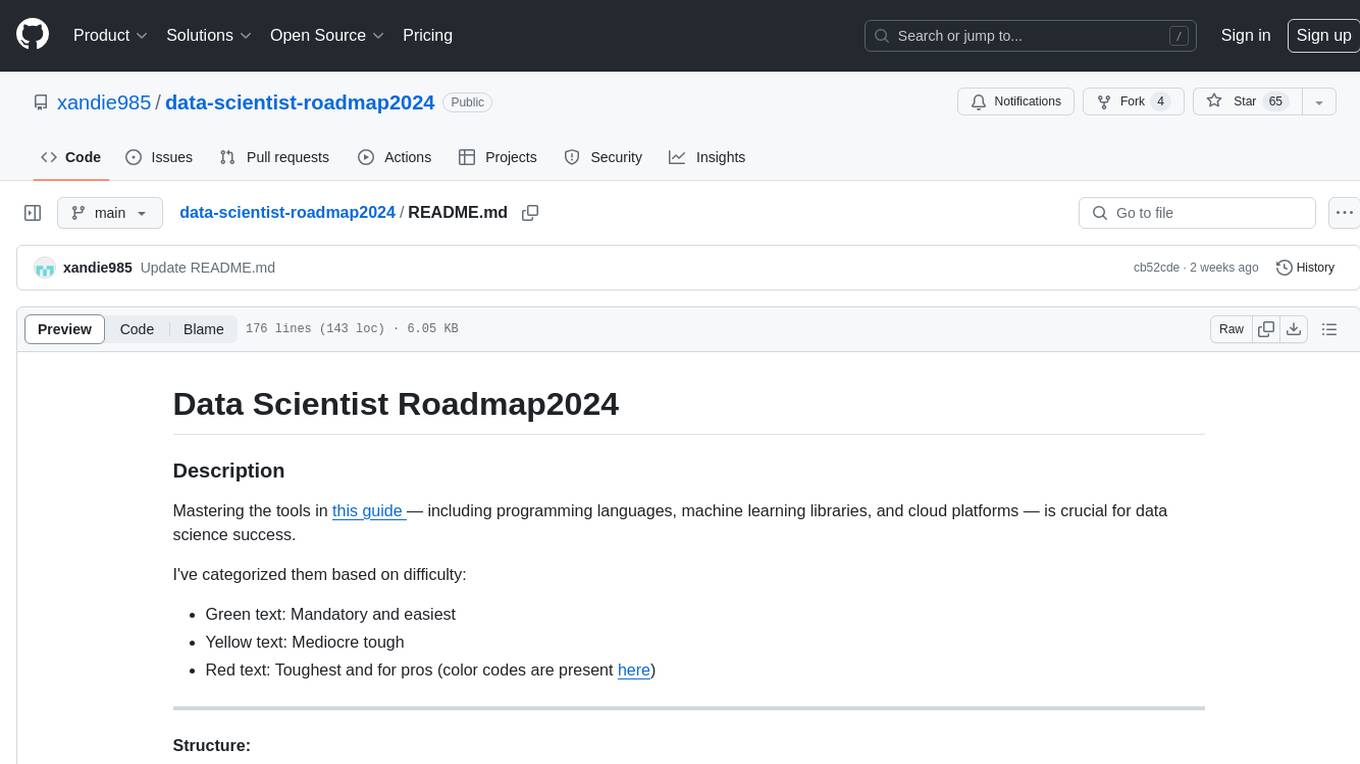
data-scientist-roadmap2024
The Data Scientist Roadmap2024 provides a comprehensive guide to mastering essential tools for data science success. It includes programming languages, machine learning libraries, cloud platforms, and concepts categorized by difficulty. The roadmap covers a wide range of topics from programming languages to machine learning techniques, data visualization tools, and DevOps/MLOps tools. It also includes web development frameworks and specific concepts like supervised and unsupervised learning, NLP, deep learning, reinforcement learning, and statistics. Additionally, it delves into DevOps tools like Airflow and MLFlow, data visualization tools like Tableau and Matplotlib, and other topics such as ETL processes, optimization algorithms, and financial modeling.
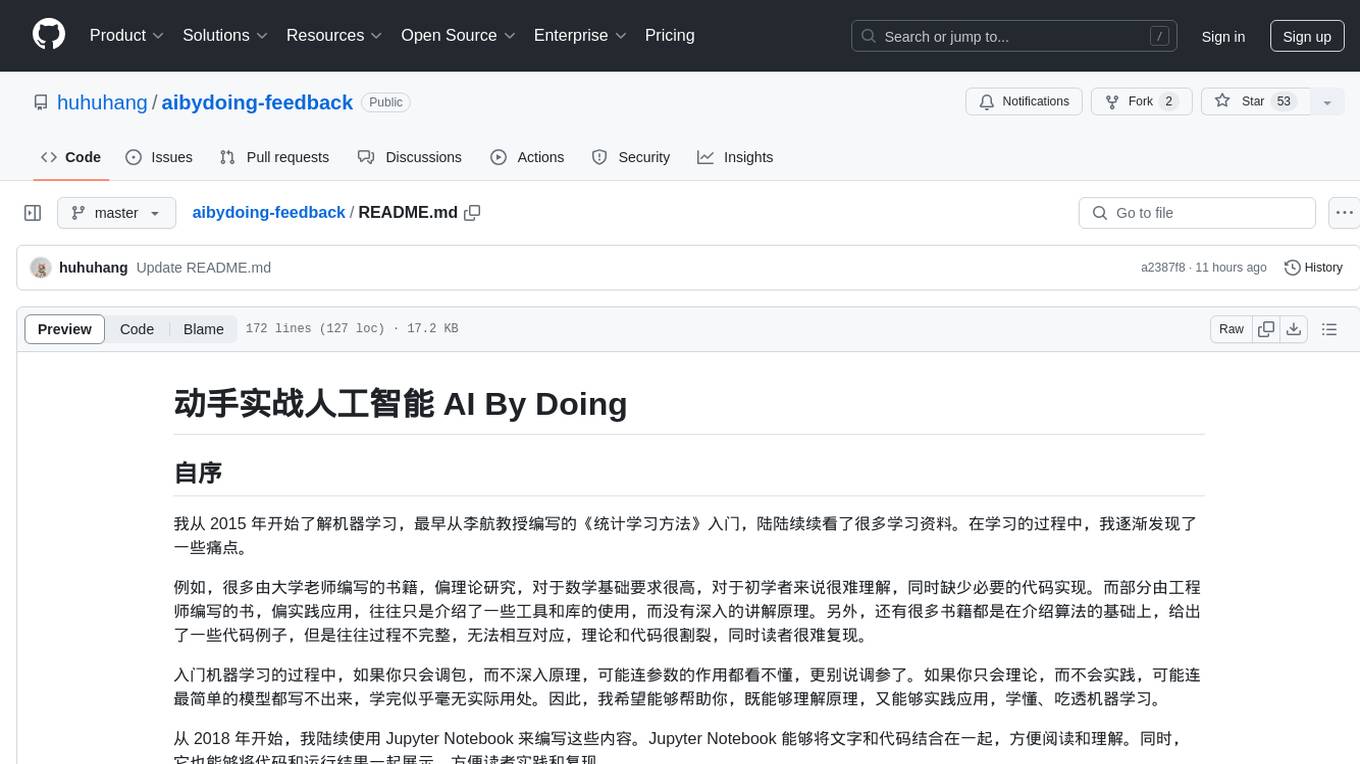
aibydoing-feedback
AI By Doing is a hands-on artificial intelligence tutorial series that aims to help beginners understand the principles of machine learning and deep learning while providing practical applications. The content covers various supervised and unsupervised learning algorithms, machine learning engineering, deep learning fundamentals, frameworks like TensorFlow and PyTorch, and applications in computer vision and natural language processing. The tutorials are written in Jupyter Notebook format, combining theory, mathematical derivations, and Python code implementations to facilitate learning and understanding.
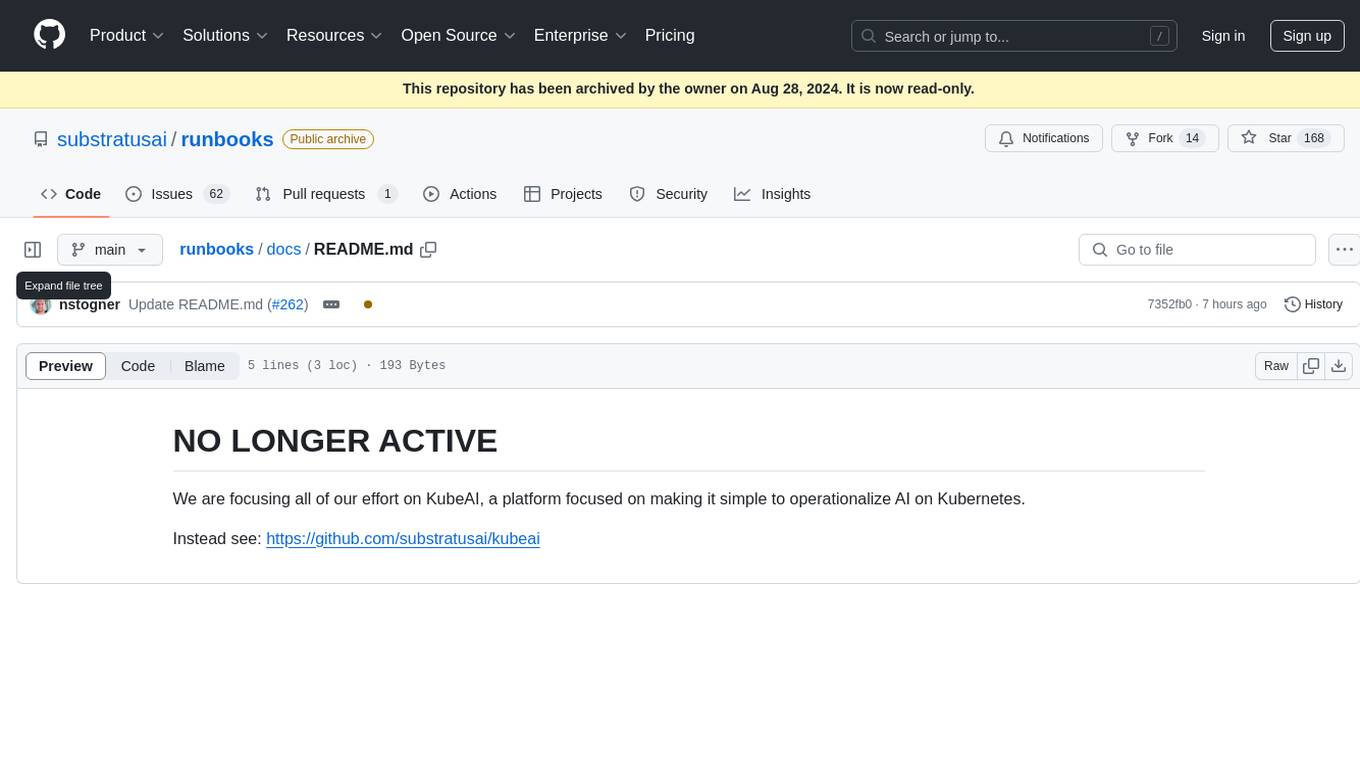
runbooks
Runbooks is a repository that is no longer active. The project has been deprecated in favor of KubeAI, a platform designed to simplify the operationalization of AI on Kubernetes. For more information, please refer to the new repository at https://github.com/substratusai/kubeai.
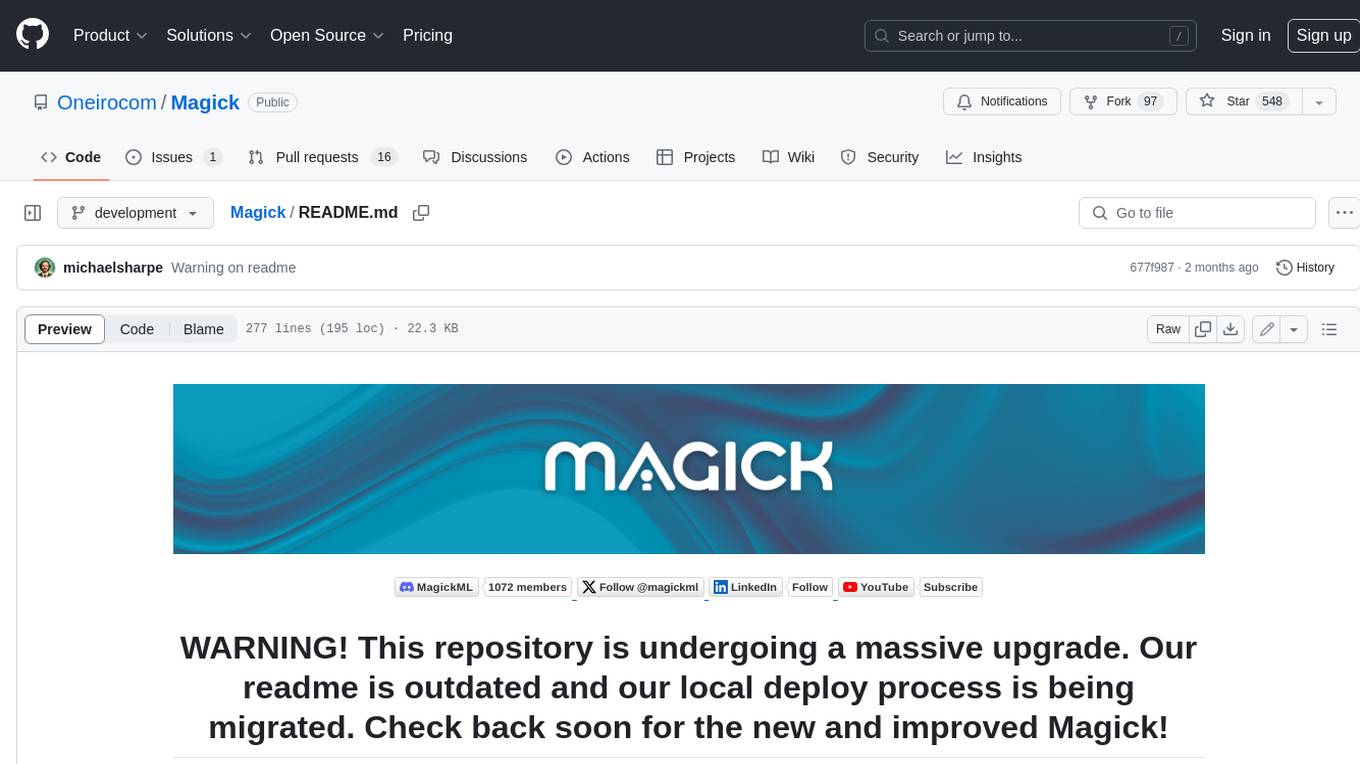
Magick
Magick is a groundbreaking visual AIDE (Artificial Intelligence Development Environment) for no-code data pipelines and multimodal agents. Magick can connect to other services and comes with nodes and templates well-suited for intelligent agents, chatbots, complex reasoning systems and realistic characters.
For similar jobs
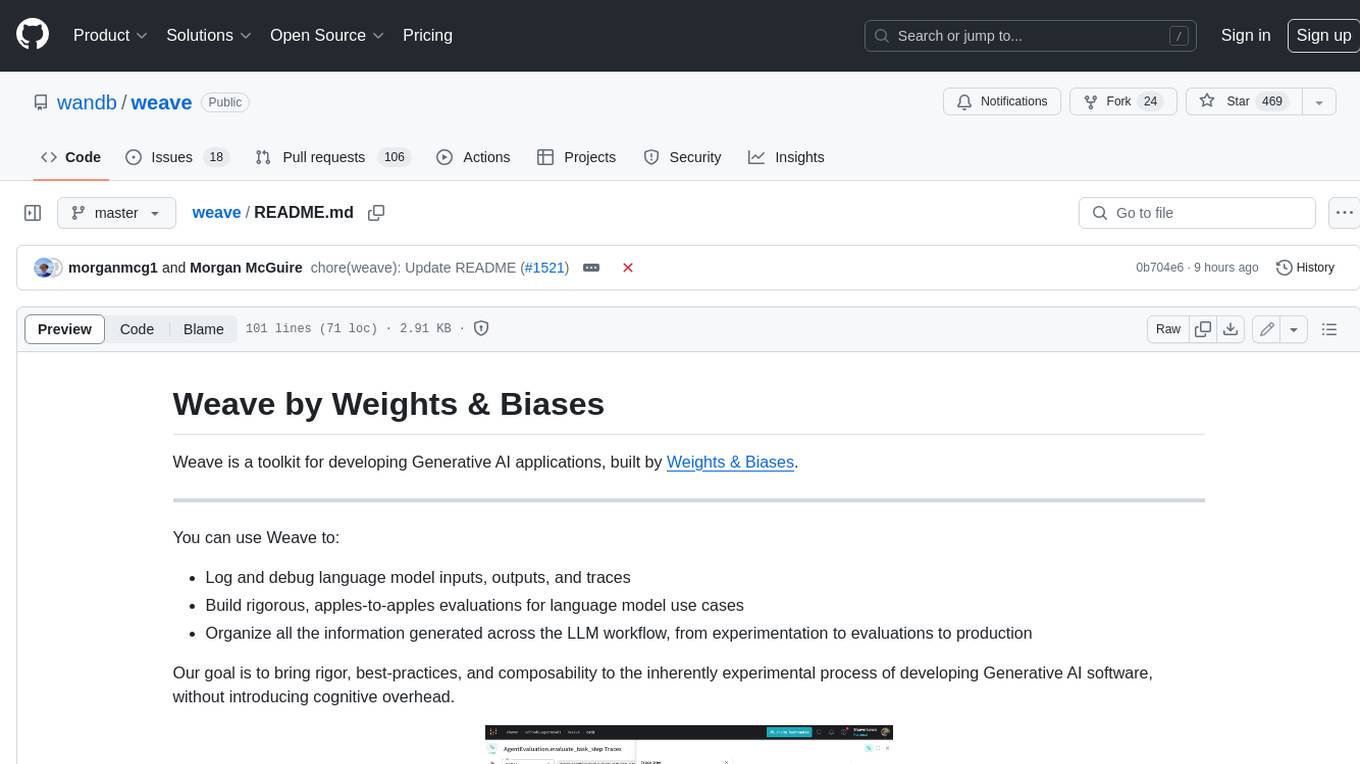
weave
Weave is a toolkit for developing Generative AI applications, built by Weights & Biases. With Weave, you can log and debug language model inputs, outputs, and traces; build rigorous, apples-to-apples evaluations for language model use cases; and organize all the information generated across the LLM workflow, from experimentation to evaluations to production. Weave aims to bring rigor, best-practices, and composability to the inherently experimental process of developing Generative AI software, without introducing cognitive overhead.
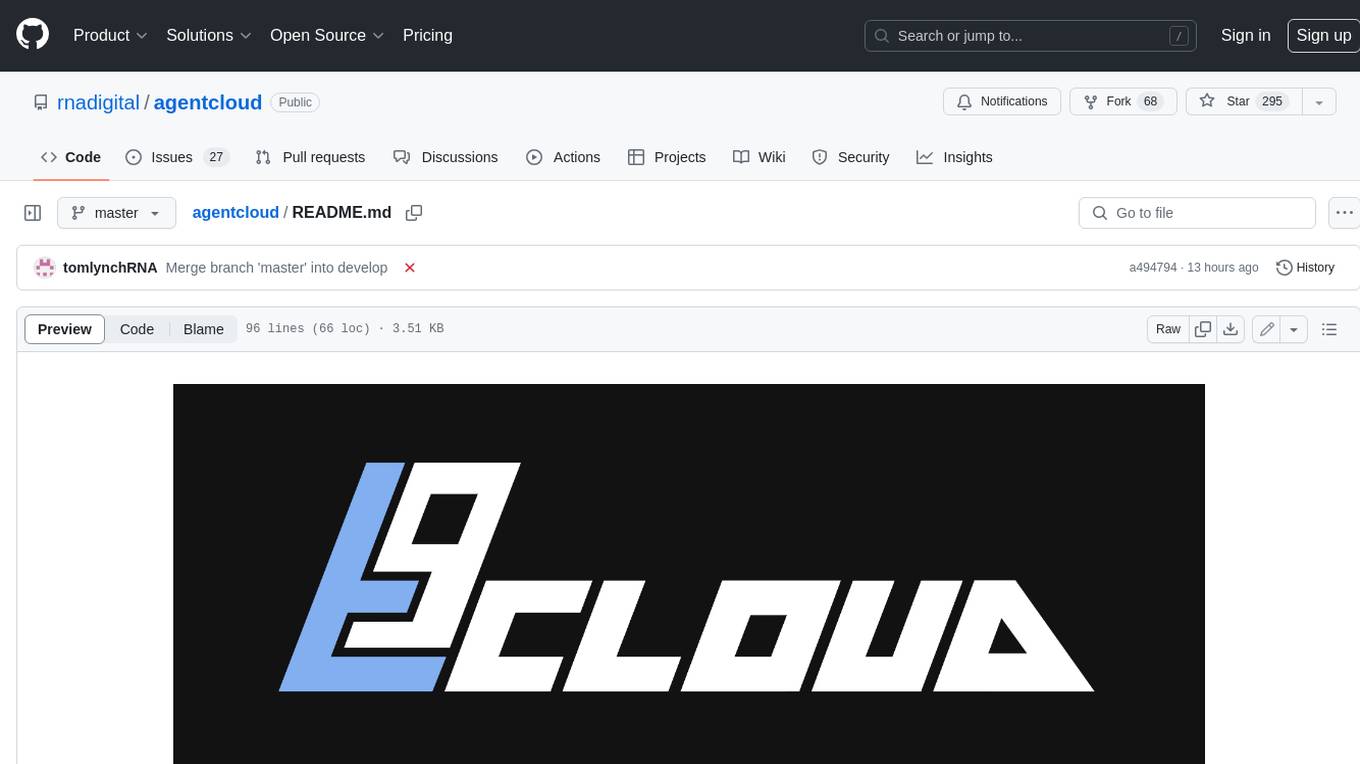
agentcloud
AgentCloud is an open-source platform that enables companies to build and deploy private LLM chat apps, empowering teams to securely interact with their data. It comprises three main components: Agent Backend, Webapp, and Vector Proxy. To run this project locally, clone the repository, install Docker, and start the services. The project is licensed under the GNU Affero General Public License, version 3 only. Contributions and feedback are welcome from the community.
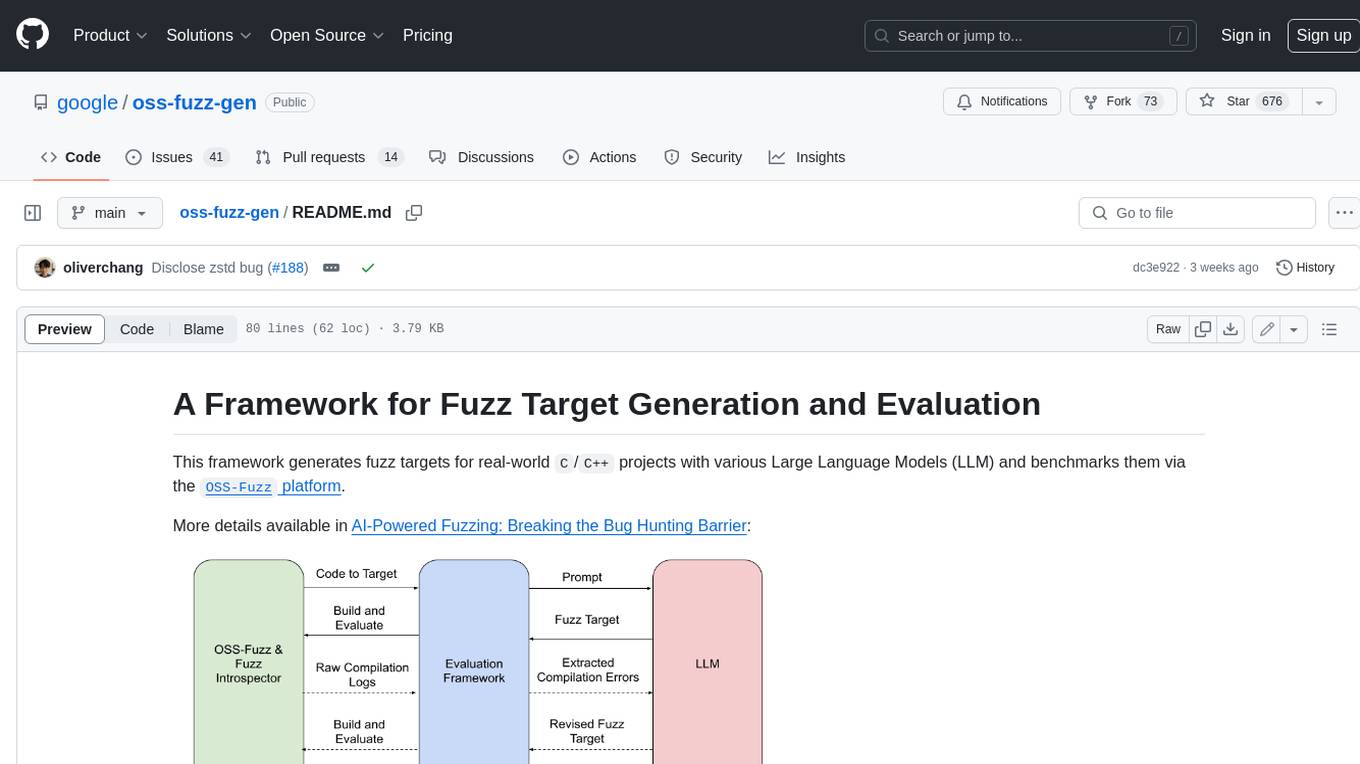
oss-fuzz-gen
This framework generates fuzz targets for real-world `C`/`C++` projects with various Large Language Models (LLM) and benchmarks them via the `OSS-Fuzz` platform. It manages to successfully leverage LLMs to generate valid fuzz targets (which generate non-zero coverage increase) for 160 C/C++ projects. The maximum line coverage increase is 29% from the existing human-written targets.
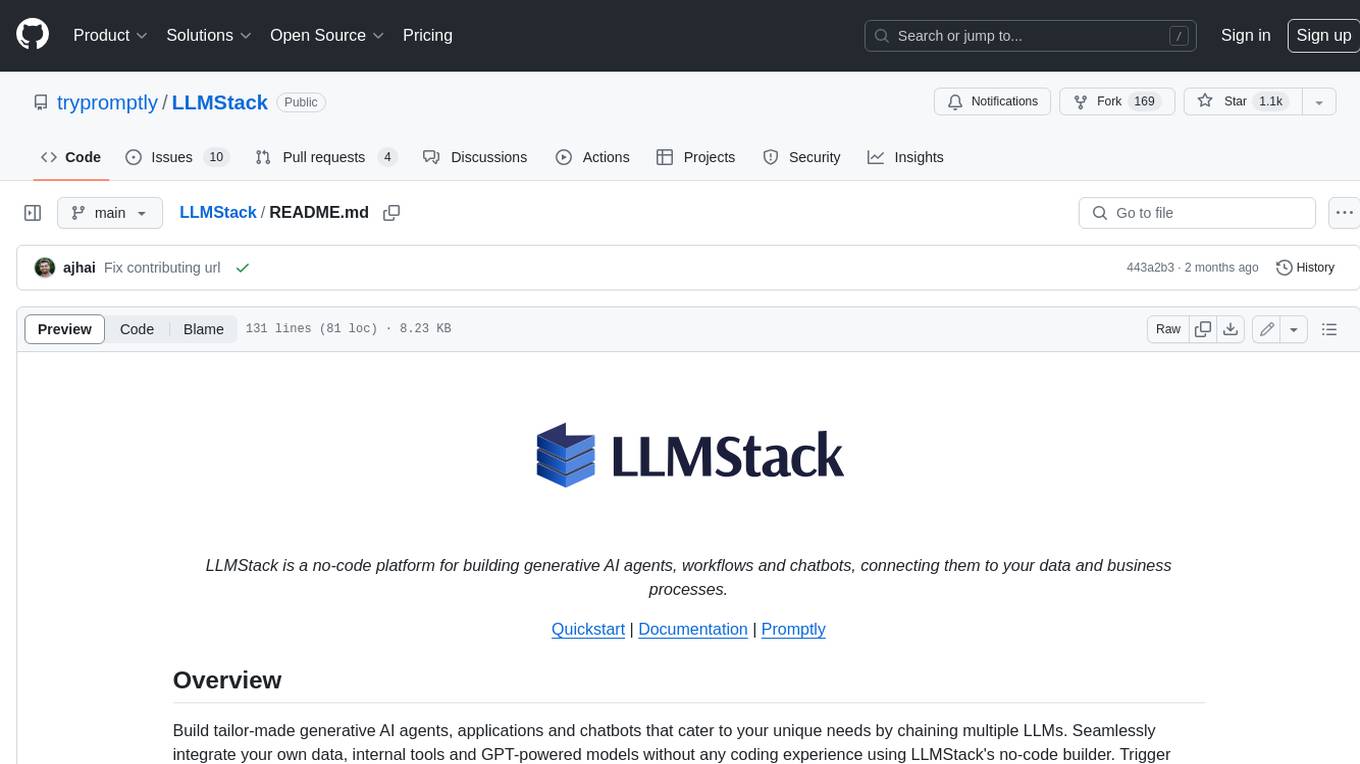
LLMStack
LLMStack is a no-code platform for building generative AI agents, workflows, and chatbots. It allows users to connect their own data, internal tools, and GPT-powered models without any coding experience. LLMStack can be deployed to the cloud or on-premise and can be accessed via HTTP API or triggered from Slack or Discord.
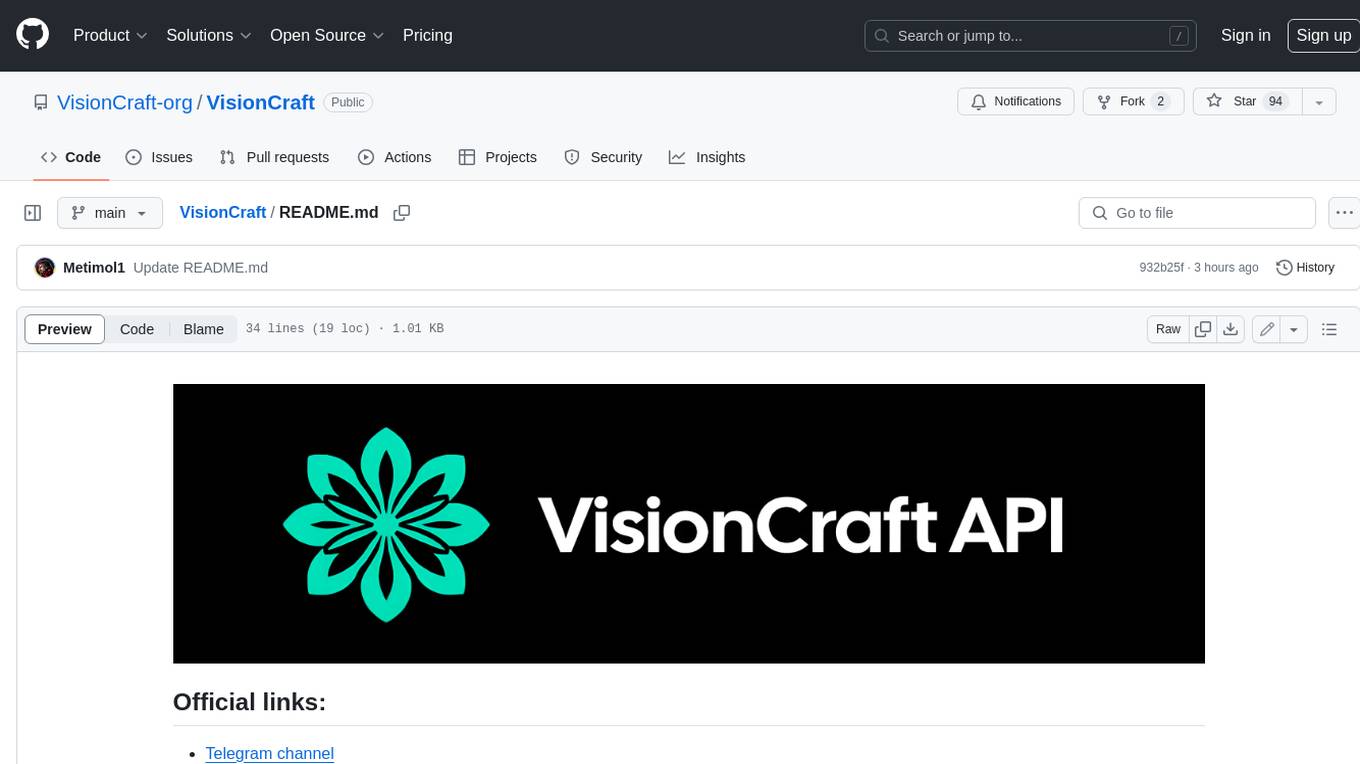
VisionCraft
The VisionCraft API is a free API for using over 100 different AI models. From images to sound.
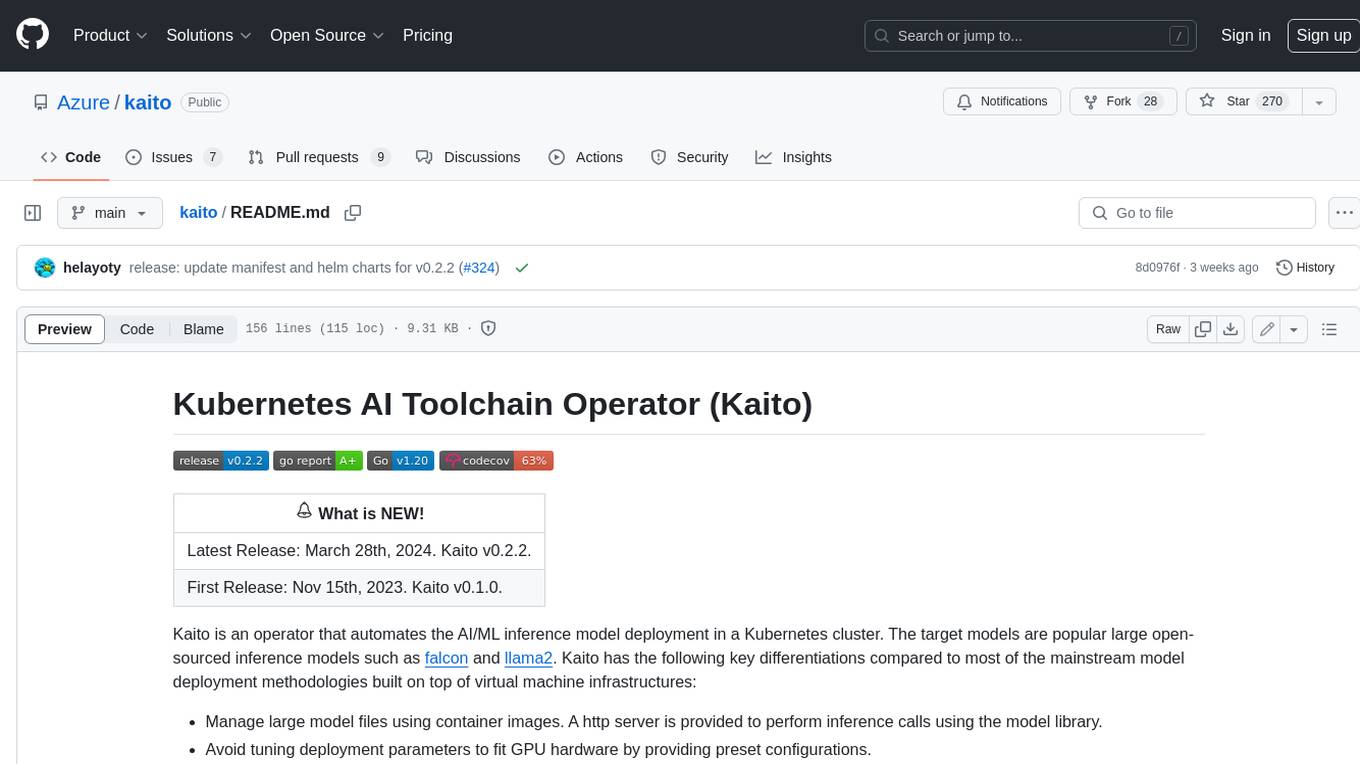
kaito
Kaito is an operator that automates the AI/ML inference model deployment in a Kubernetes cluster. It manages large model files using container images, avoids tuning deployment parameters to fit GPU hardware by providing preset configurations, auto-provisions GPU nodes based on model requirements, and hosts large model images in the public Microsoft Container Registry (MCR) if the license allows. Using Kaito, the workflow of onboarding large AI inference models in Kubernetes is largely simplified.
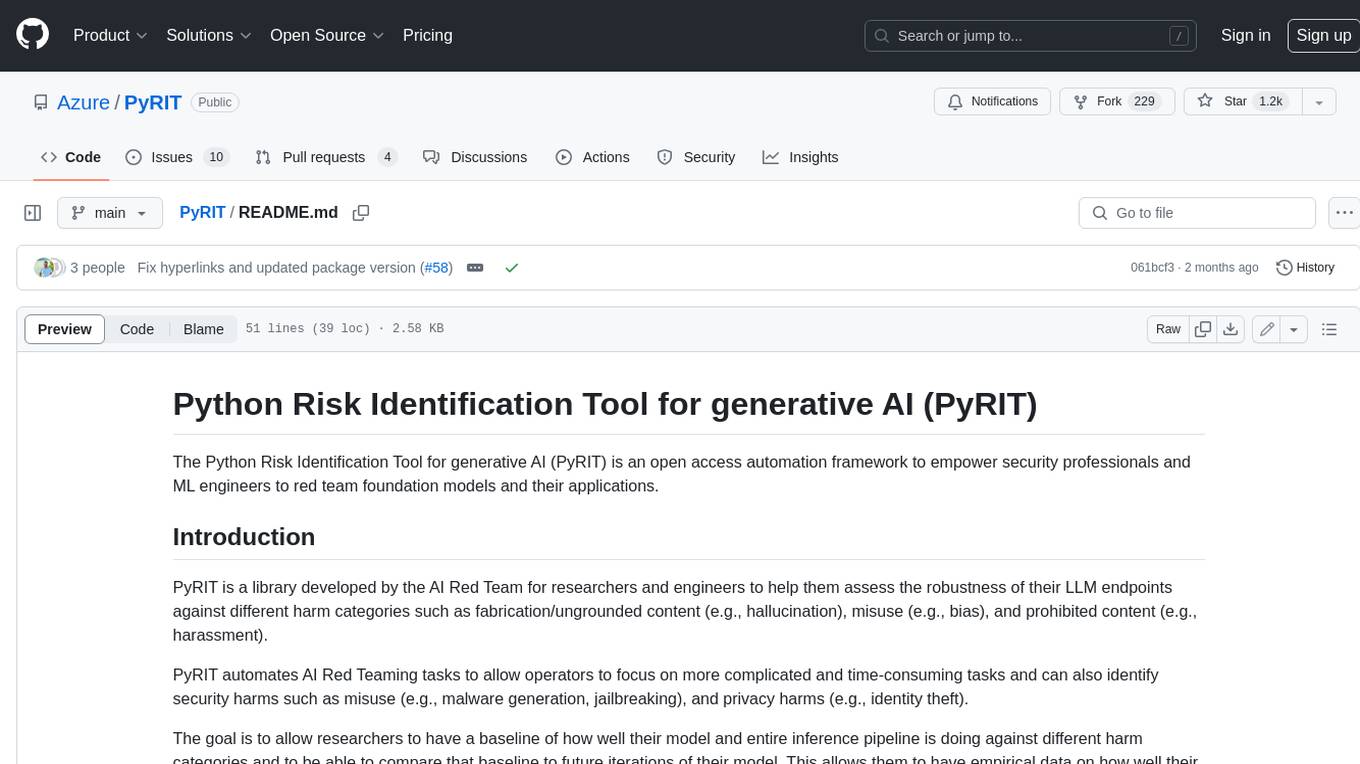
PyRIT
PyRIT is an open access automation framework designed to empower security professionals and ML engineers to red team foundation models and their applications. It automates AI Red Teaming tasks to allow operators to focus on more complicated and time-consuming tasks and can also identify security harms such as misuse (e.g., malware generation, jailbreaking), and privacy harms (e.g., identity theft). The goal is to allow researchers to have a baseline of how well their model and entire inference pipeline is doing against different harm categories and to be able to compare that baseline to future iterations of their model. This allows them to have empirical data on how well their model is doing today, and detect any degradation of performance based on future improvements.
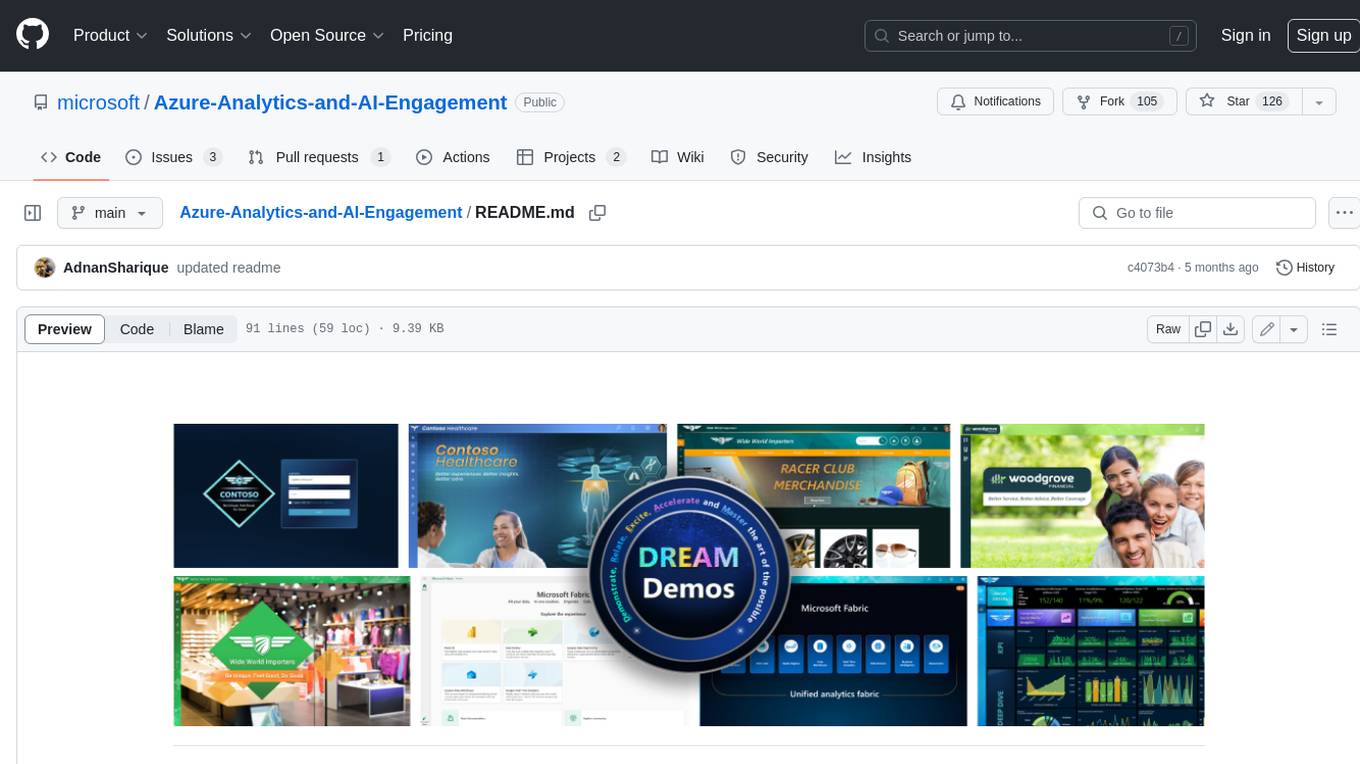
Azure-Analytics-and-AI-Engagement
The Azure-Analytics-and-AI-Engagement repository provides packaged Industry Scenario DREAM Demos with ARM templates (Containing a demo web application, Power BI reports, Synapse resources, AML Notebooks etc.) that can be deployed in a customer’s subscription using the CAPE tool within a matter of few hours. Partners can also deploy DREAM Demos in their own subscriptions using DPoC.