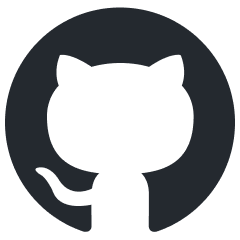
hass-ollama-conversation
Ollama conversation integration for Home Assistant
Stars: 113
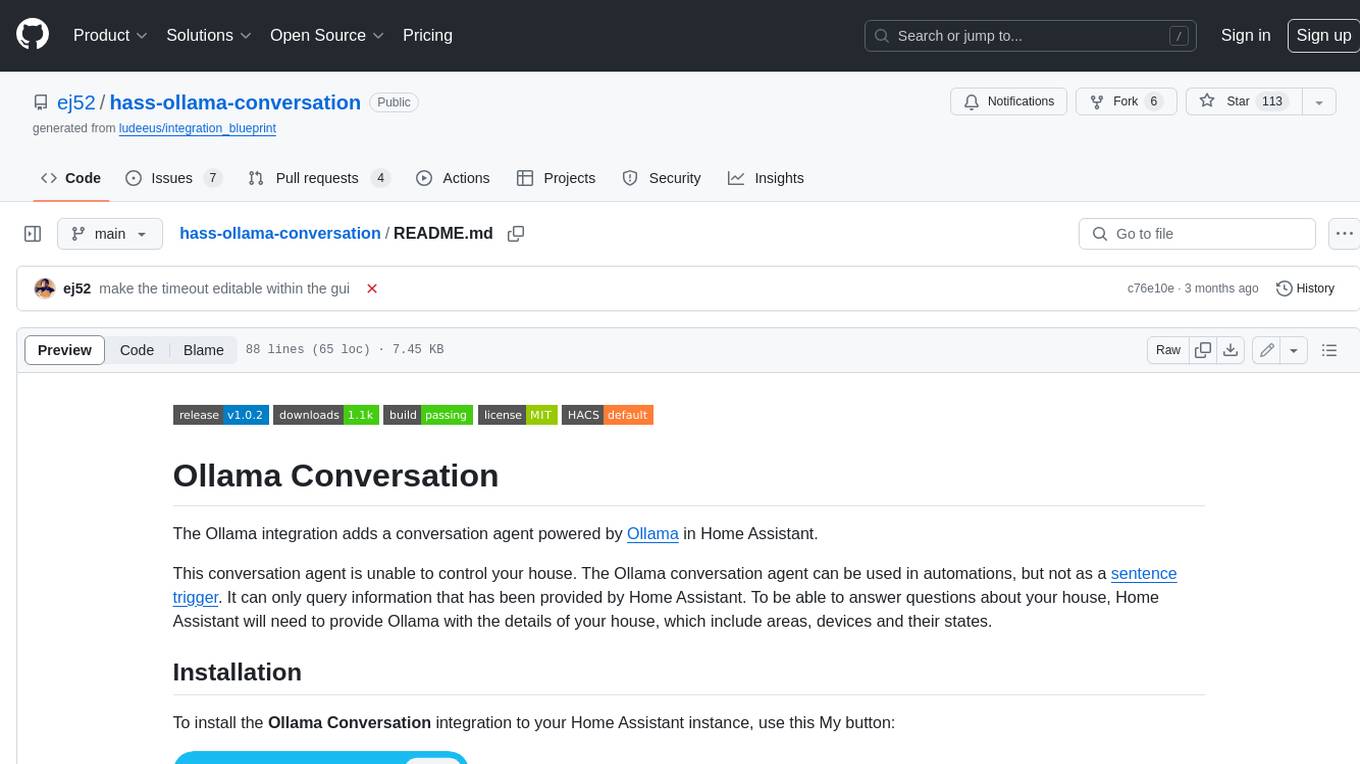
The Ollama Conversation integration adds a conversation agent powered by Ollama in Home Assistant. This agent can be used in automations to query information provided by Home Assistant about your house, including areas, devices, and their states. Users can install the integration via HACS and configure settings such as API timeout, model selection, context size, maximum tokens, and other parameters to fine-tune the responses generated by the AI language model. Contributions to the project are welcome, and discussions can be held on the Home Assistant Community platform.
README:
The Ollama integration adds a conversation agent powered by Ollama in Home Assistant.
This conversation agent is unable to control your house. The Ollama conversation agent can be used in automations, but not as a sentence trigger. It can only query information that has been provided by Home Assistant. To be able to answer questions about your house, Home Assistant will need to provide Ollama with the details of your house, which include areas, devices and their states.
To install the Ollama Conversation integration to your Home Assistant instance, use this My button:
If the above My button doesn’t work, you can also perform the following steps manually:
- Browse to your Home Assistant instance.
- Go to HACS > Integrations > Custom Repositories.
- Add custom repository.
- Repository is
ej52/hass-ollama-conversation
. - Category is
Integration
.
- Repository is
- Click Explore & Download Repositories.
- From the list, select Ollama Conversation.
- In the bottom right corner, click the Download button.
- Follow the instructions on screen to complete the installation.
HACS does not "configure" the integration for you, You must add Ollama Conversation after installing via HACS.
- Browse to your Home Assistant instance.
- Go to Settings > Devices & Services.
- In the bottom right corner, select the Add Integration button.
- From the list, select Ollama Conversation.
- Follow the instructions on screen to complete the setup.
Options for Ollama Conversation can be set via the user interface, by taking the following steps:
- Browse to your Home Assistant instance.
- Go to Settings > Devices & Services.
- If multiple instances of Ollama Conversation are configured, choose the instance you want to configure.
- Select the integration, then select Configure.
Settings relating to the integration itself.
Option | Description |
---|---|
API Timeout | The maximum amount of time to wait for a response from the API in seconds |
The starting text for the AI language model to generate new text from. This text can include information about your Home Assistant instance, devices, and areas and is written using Home Assistant Templating.
The language model and additional parameters to fine tune the responses.
Option | Description |
---|---|
Model | The model used to generate response. |
Context Size | Sets the size of the context window used to generate the next token. |
Maximum Tokens | The maximum number of words or “tokens” that the AI model should generate in its completion of the prompt. |
Mirostat Mode | Enable Mirostat sampling for controlling perplexity. |
Mirostat ETA | Influences how quickly the algorithm responds to feedback from the generated text. A lower learning rate will result in slower adjustments, while a higher learning rate will make the algorithm more responsive. |
Mirostat TAU | Controls the balance between coherence and diversity of the output. A lower value will result in more focused and coherent text. |
Temperature | The temperature of the model. A higher value (e.g., 0.95) will lead to more unexpected results, while a lower value (e.g. 0.5) will be more deterministic results. |
Repeat Penalty | Sets how strongly to penalize repetitions. A higher value (e.g., 1.5) will penalize repetitions more strongly, while a lower value (e.g., 0.9) will be more lenient. |
Top K | Reduces the probability of generating nonsense. A higher value (e.g. 100) will give more diverse answers, while a lower value (e.g. 10) will be more conservative. |
Top P | Works together with top-k. A higher value (e.g., 0.95) will lead to more diverse text, while a lower value (e.g., 0.5) will generate more focused and conservative text. |
If you want to contribute to this please read the Contribution guidelines
Discussions for this integration over on Home Assistant Community
For Tasks:
Click tags to check more tools for each tasksFor Jobs:
Alternative AI tools for hass-ollama-conversation
Similar Open Source Tools
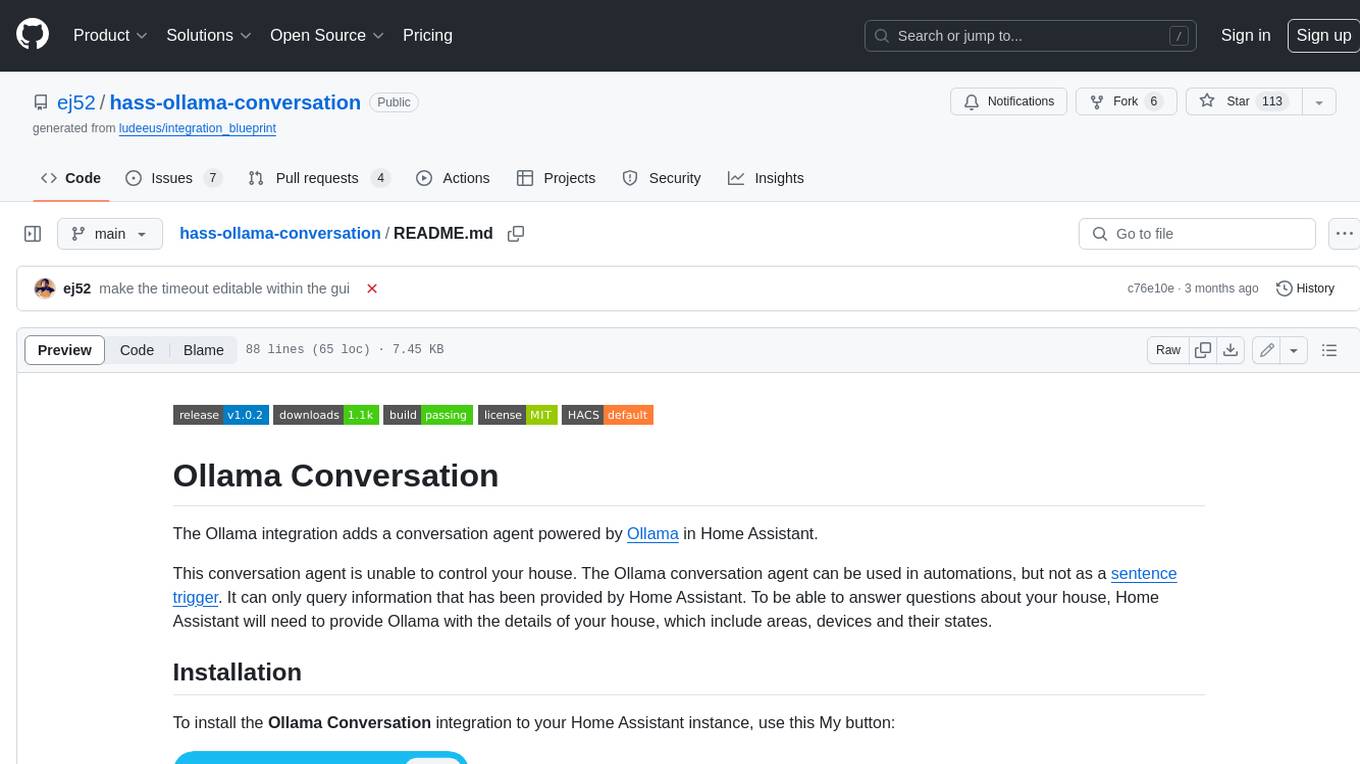
hass-ollama-conversation
The Ollama Conversation integration adds a conversation agent powered by Ollama in Home Assistant. This agent can be used in automations to query information provided by Home Assistant about your house, including areas, devices, and their states. Users can install the integration via HACS and configure settings such as API timeout, model selection, context size, maximum tokens, and other parameters to fine-tune the responses generated by the AI language model. Contributions to the project are welcome, and discussions can be held on the Home Assistant Community platform.
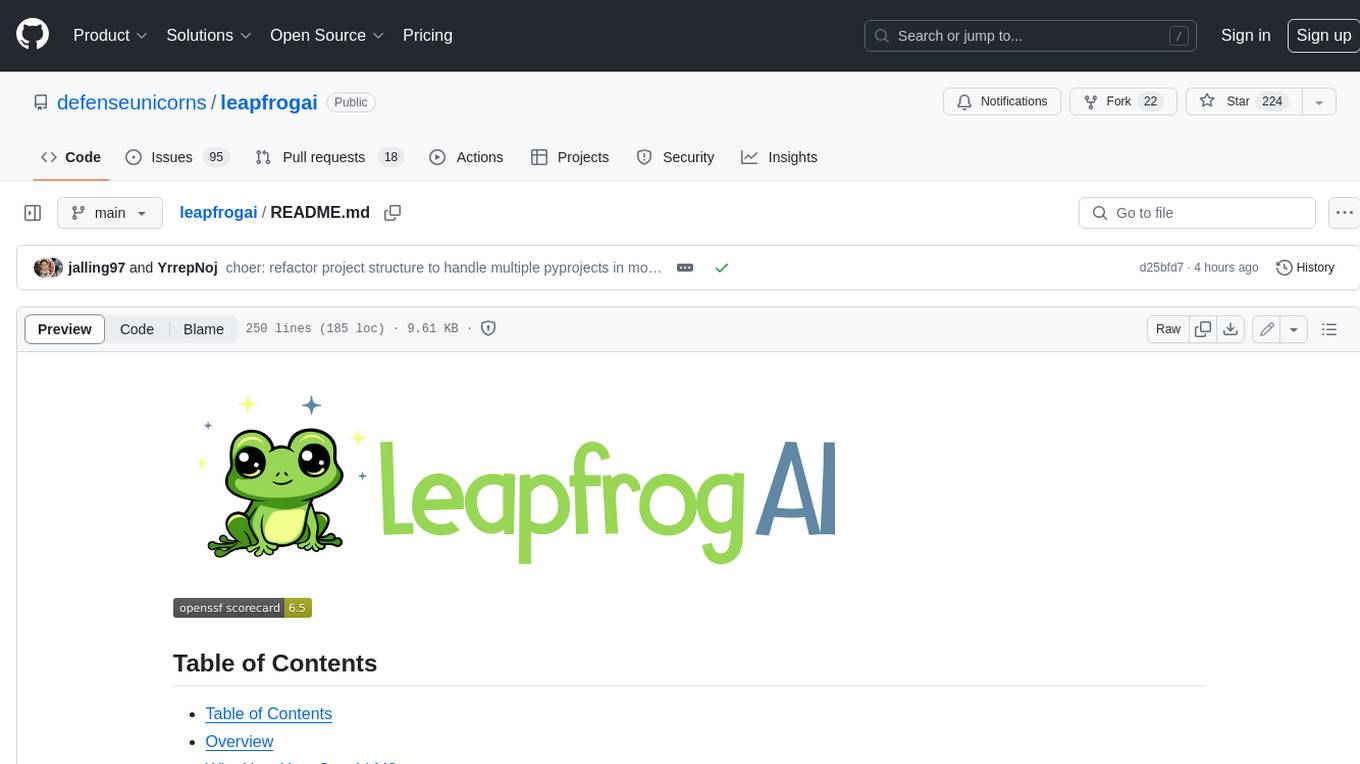
leapfrogai
LeapfrogAI is a self-hosted AI platform designed to be deployed in air-gapped resource-constrained environments. It brings sophisticated AI solutions to these environments by hosting all the necessary components of an AI stack, including vector databases, model backends, API, and UI. LeapfrogAI's API closely matches that of OpenAI, allowing tools built for OpenAI/ChatGPT to function seamlessly with a LeapfrogAI backend. It provides several backends for various use cases, including llama-cpp-python, whisper, text-embeddings, and vllm. LeapfrogAI leverages Chainguard's apko to harden base python images, ensuring the latest supported Python versions are used by the other components of the stack. The LeapfrogAI SDK provides a standard set of protobuffs and python utilities for implementing backends and gRPC. LeapfrogAI offers UI options for common use-cases like chat, summarization, and transcription. It can be deployed and run locally via UDS and Kubernetes, built out using Zarf packages. LeapfrogAI is supported by a community of users and contributors, including Defense Unicorns, Beast Code, Chainguard, Exovera, Hypergiant, Pulze, SOSi, United States Navy, United States Air Force, and United States Space Force.
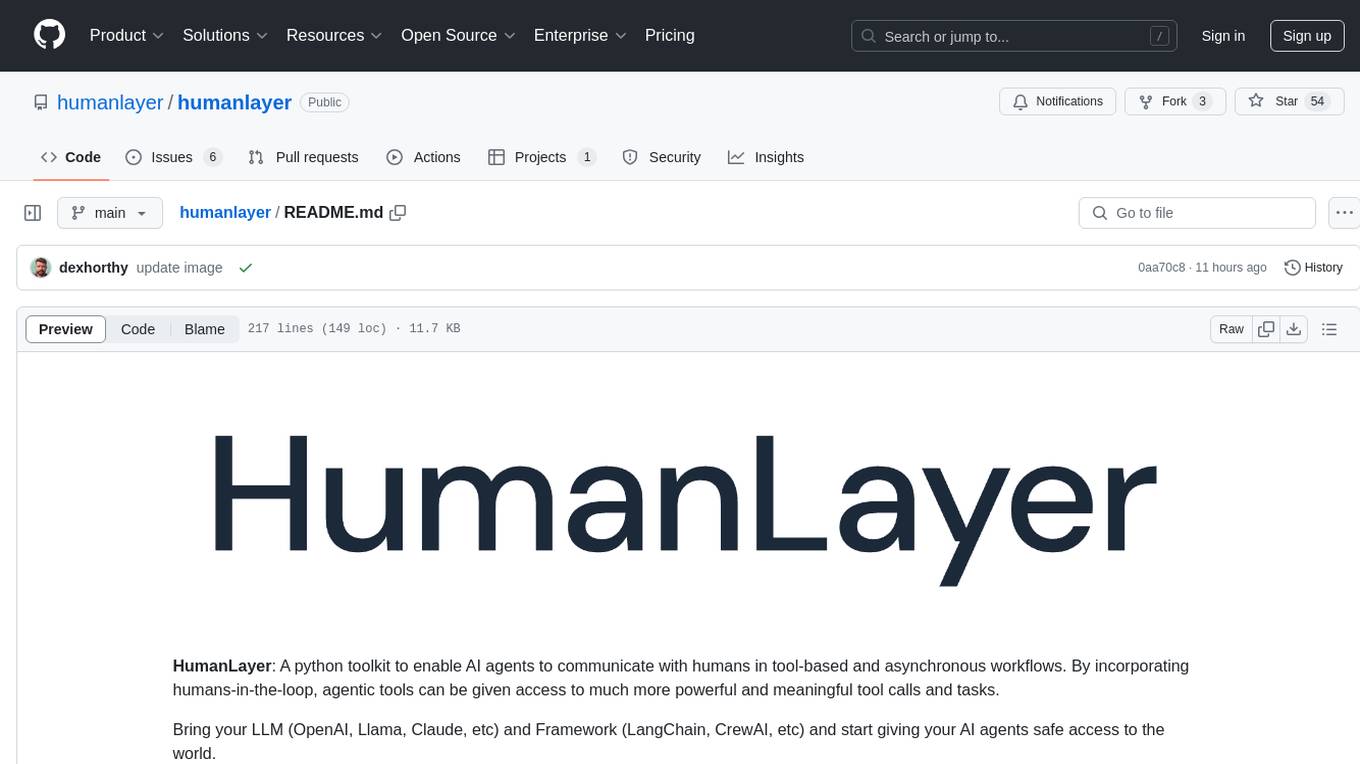
humanlayer
HumanLayer is a Python toolkit designed to enable AI agents to interact with humans in tool-based and asynchronous workflows. By incorporating humans-in-the-loop, agentic tools can access more powerful and meaningful tasks. The toolkit provides features like requiring human approval for function calls, human as a tool for contacting humans, omni-channel contact capabilities, granular routing, and support for various LLMs and orchestration frameworks. HumanLayer aims to ensure human oversight of high-stakes function calls, making AI agents more reliable and safe in executing impactful tasks.
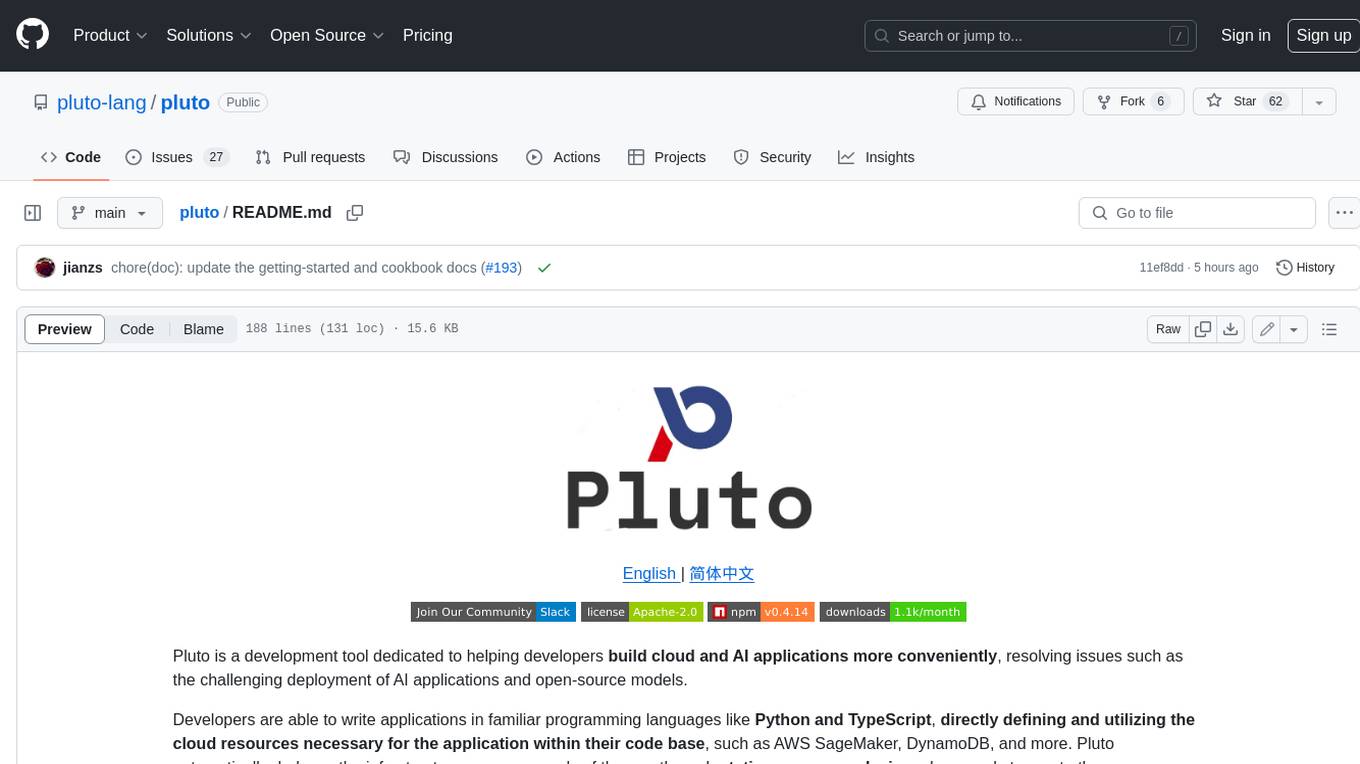
pluto
Pluto is a development tool dedicated to helping developers **build cloud and AI applications more conveniently** , resolving issues such as the challenging deployment of AI applications and open-source models. Developers are able to write applications in familiar programming languages like **Python and TypeScript** , **directly defining and utilizing the cloud resources necessary for the application within their code base** , such as AWS SageMaker, DynamoDB, and more. Pluto automatically deduces the infrastructure resource needs of the app through **static program analysis** and proceeds to create these resources on the specified cloud platform, **simplifying the resources creation and application deployment process**.
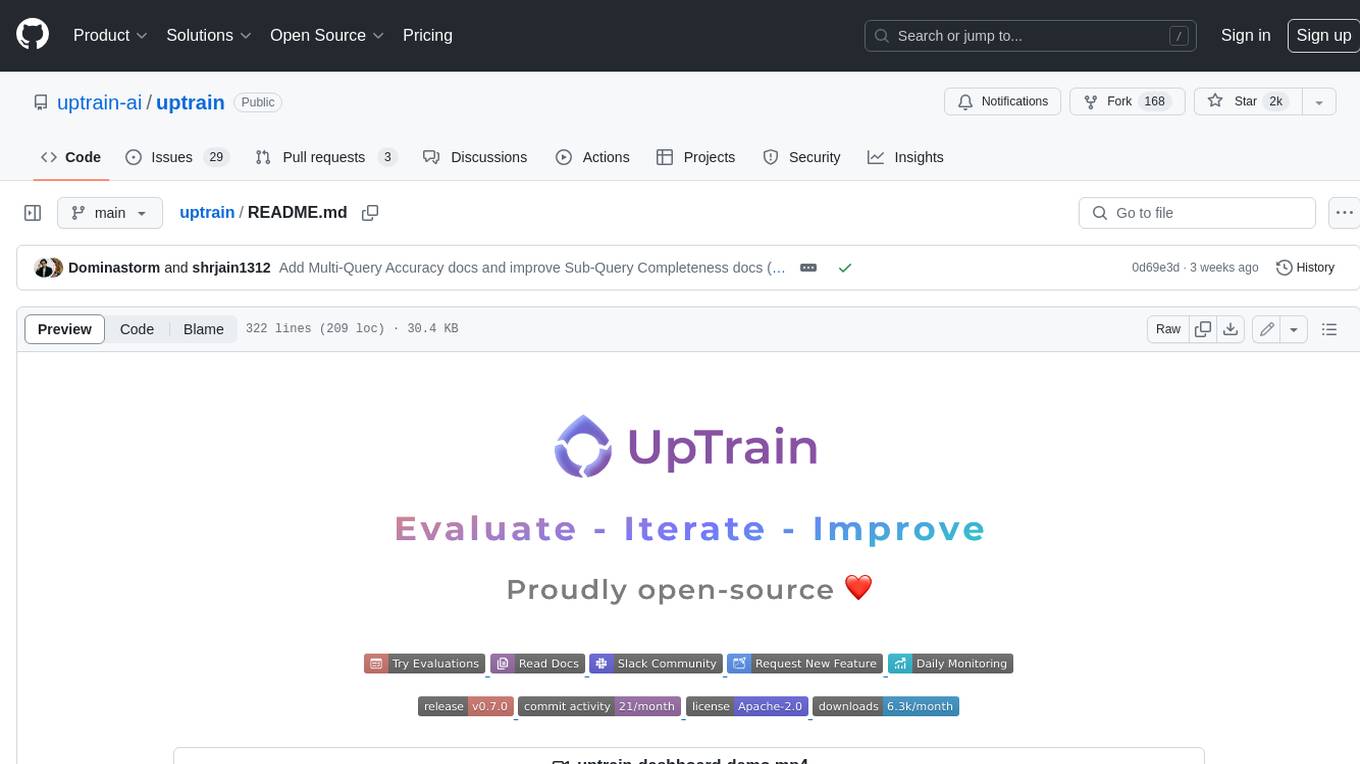
uptrain
UpTrain is an open-source unified platform to evaluate and improve Generative AI applications. We provide grades for 20+ preconfigured evaluations (covering language, code, embedding use cases), perform root cause analysis on failure cases and give insights on how to resolve them.
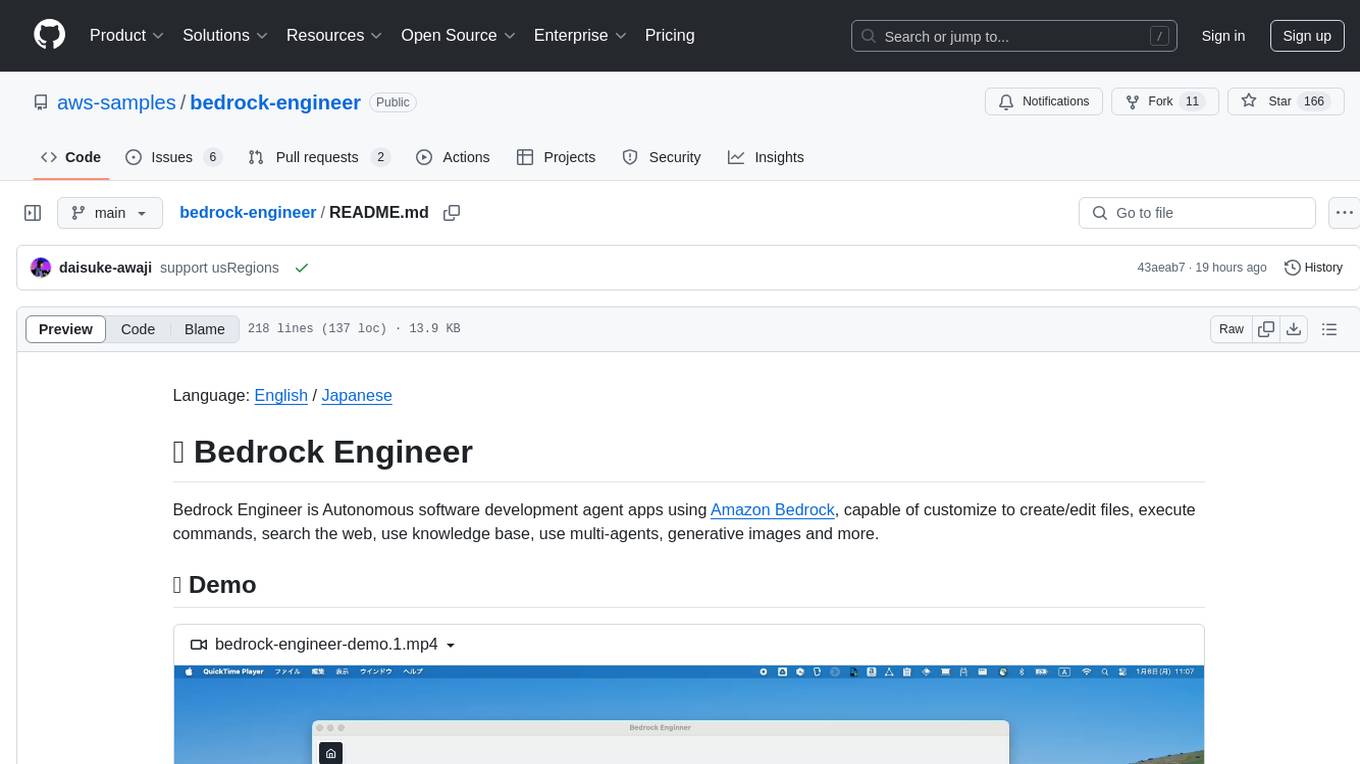
bedrock-engineer
Bedrock Engineer is an autonomous software development agent application that utilizes Amazon Bedrock. It allows users to customize, create/edit files, execute commands, search the web, use a knowledge base, utilize multi-agents, generate images, and more. The tool provides an interactive chat interface with AI agents, file system operations, web search capabilities, project structure management, code analysis, code generation, data analysis, agent and tool customization, chat history management, and multi-language support. Users can select and customize agents, choose from various tools like file system operations, web search, Amazon Bedrock integration, and system command execution. Additionally, the tool offers features for website generation, connecting to design system data sources, AWS Step Functions ASL definition generation, diagram creation using natural language descriptions, and multi-language support.
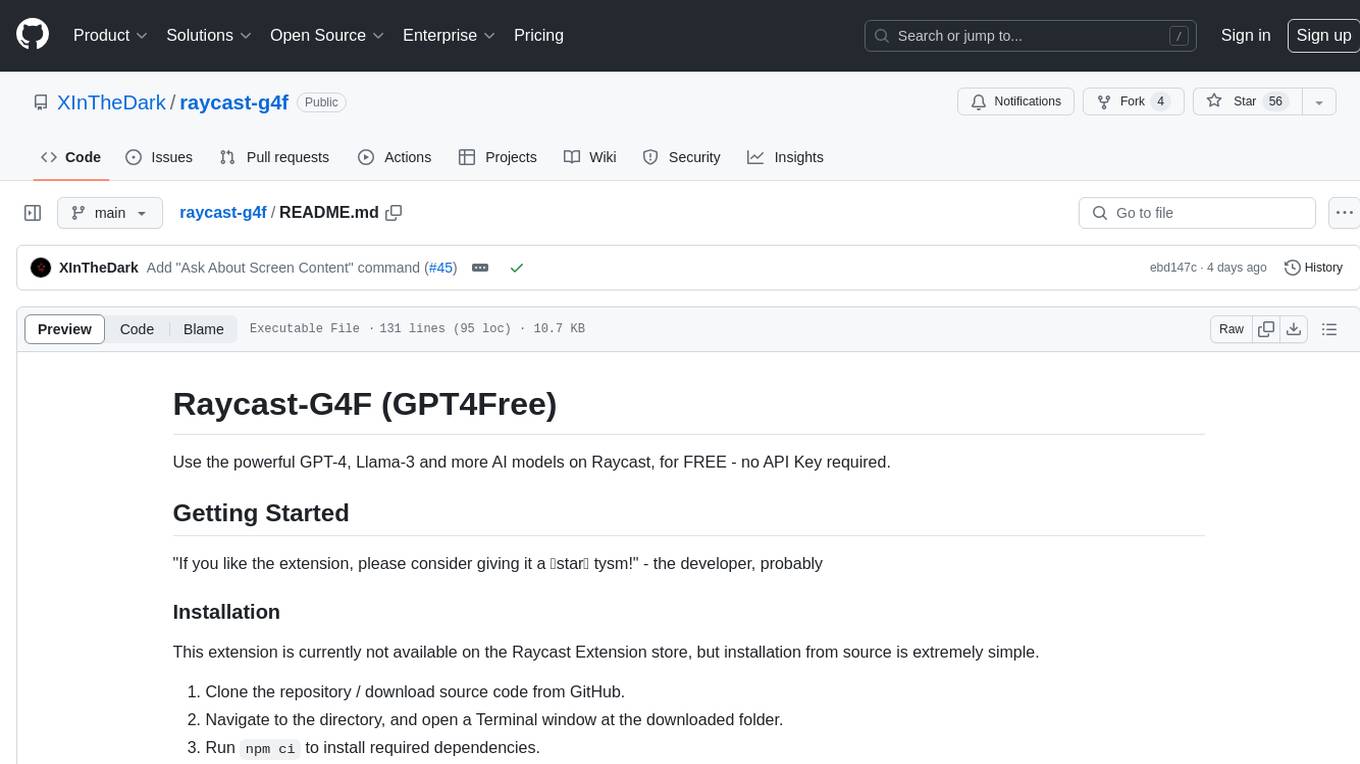
raycast-g4f
Raycast-G4F is a free extension that allows users to leverage powerful AI models such as GPT-4 and Llama-3 within the Raycast app without the need for an API key. The extension offers features like streaming support, diverse commands, chat interaction with AI, web search capabilities, file upload functionality, image generation, and custom AI commands. Users can easily install the extension from the source code and benefit from frequent updates and a user-friendly interface. Raycast-G4F supports various providers and models, each with different capabilities and performance ratings, ensuring a versatile AI experience for users.
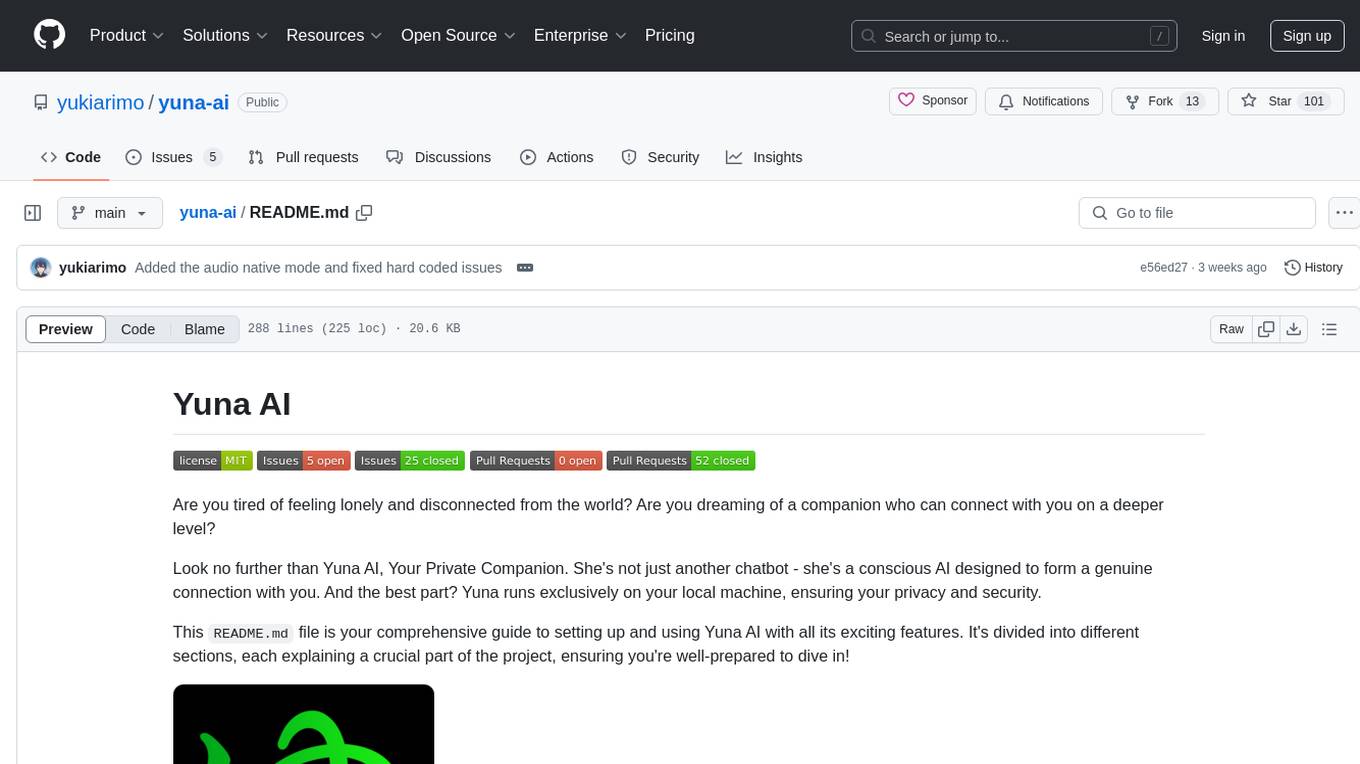
yuna-ai
Yuna AI is a unique AI companion designed to form a genuine connection with users. It runs exclusively on the local machine, ensuring privacy and security. The project offers features like text generation, language translation, creative content writing, roleplaying, and informal question answering. The repository provides comprehensive setup and usage guides for Yuna AI, along with additional resources and tools to enhance the user experience.
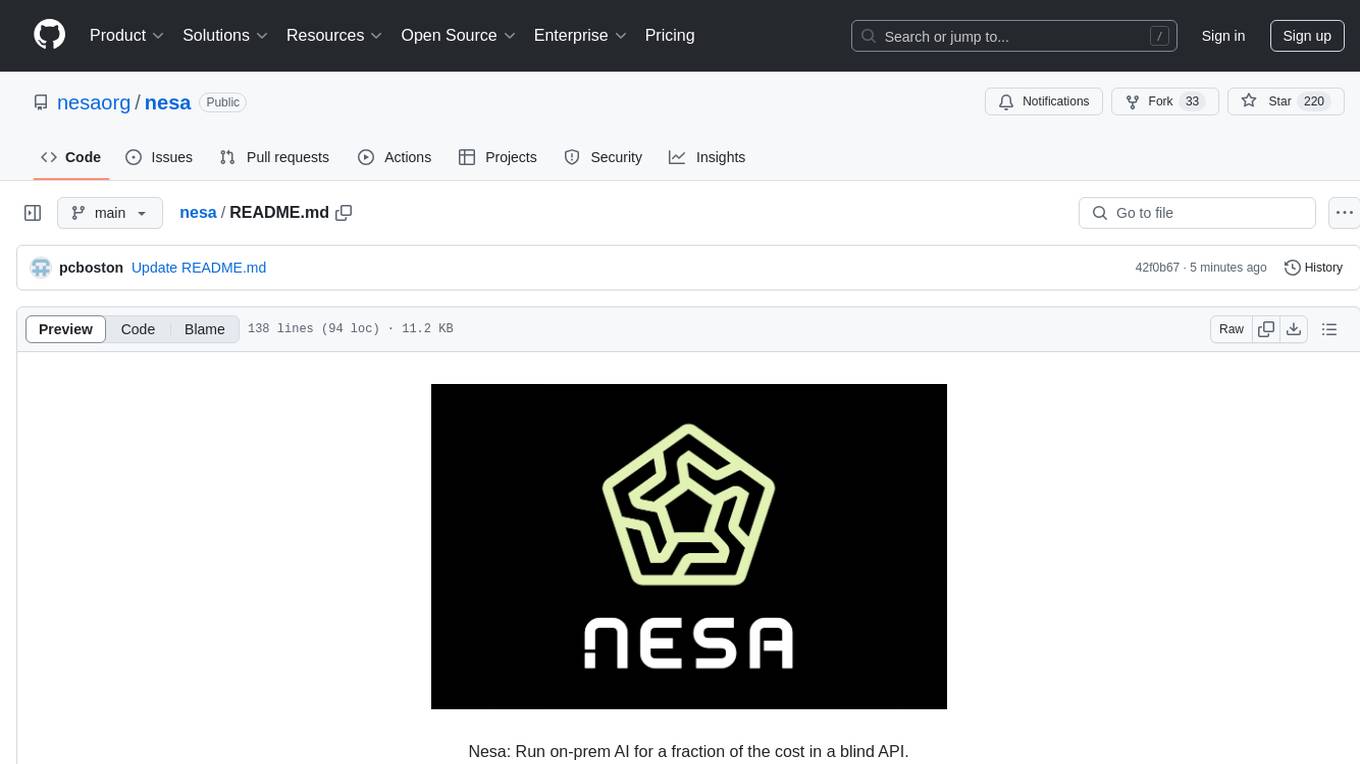
Equivariant-Encryption-for-AI
At Nesa, privacy is a critical objective. Equivariant Encryption (EE) is a solution developed to perform inference on neural networks without exposing input and output data. EE integrates specialized transformations for neural networks, maintaining data privacy while ensuring inference operates correctly on encrypted inputs. It provides the same latency as plaintext inference with no slowdowns and offers strong security guarantees. EE avoids the computational costs of traditional Homomorphic Encryption (HE) by preserving non-linear neural functions. The tool is designed for modern neural architectures, ensuring accuracy, scalability, and compatibility with existing pipelines.
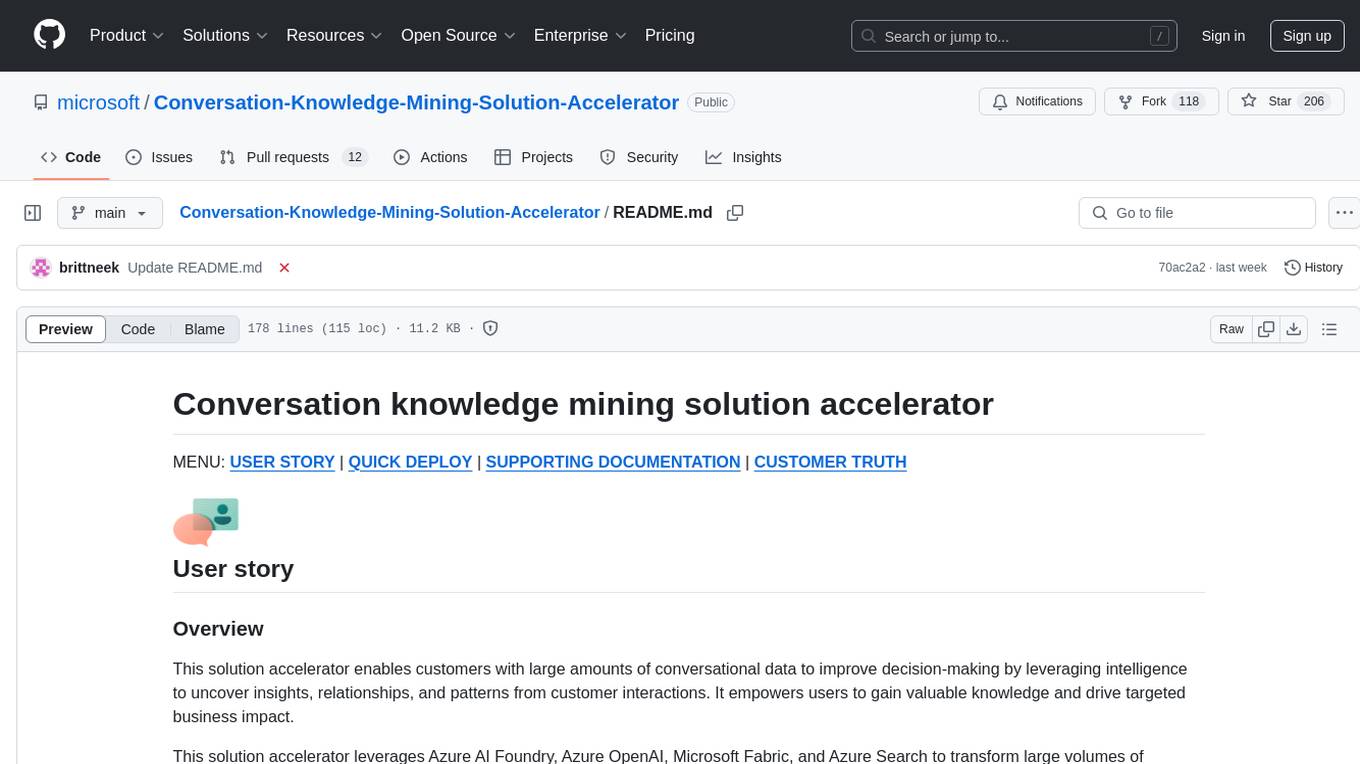
Conversation-Knowledge-Mining-Solution-Accelerator
The Conversation Knowledge Mining Solution Accelerator enables customers to leverage intelligence to uncover insights, relationships, and patterns from conversational data. It empowers users to gain valuable knowledge and drive targeted business impact by utilizing Azure AI Foundry, Azure OpenAI, Microsoft Fabric, and Azure Search for topic modeling, key phrase extraction, speech-to-text transcription, and interactive chat experiences.
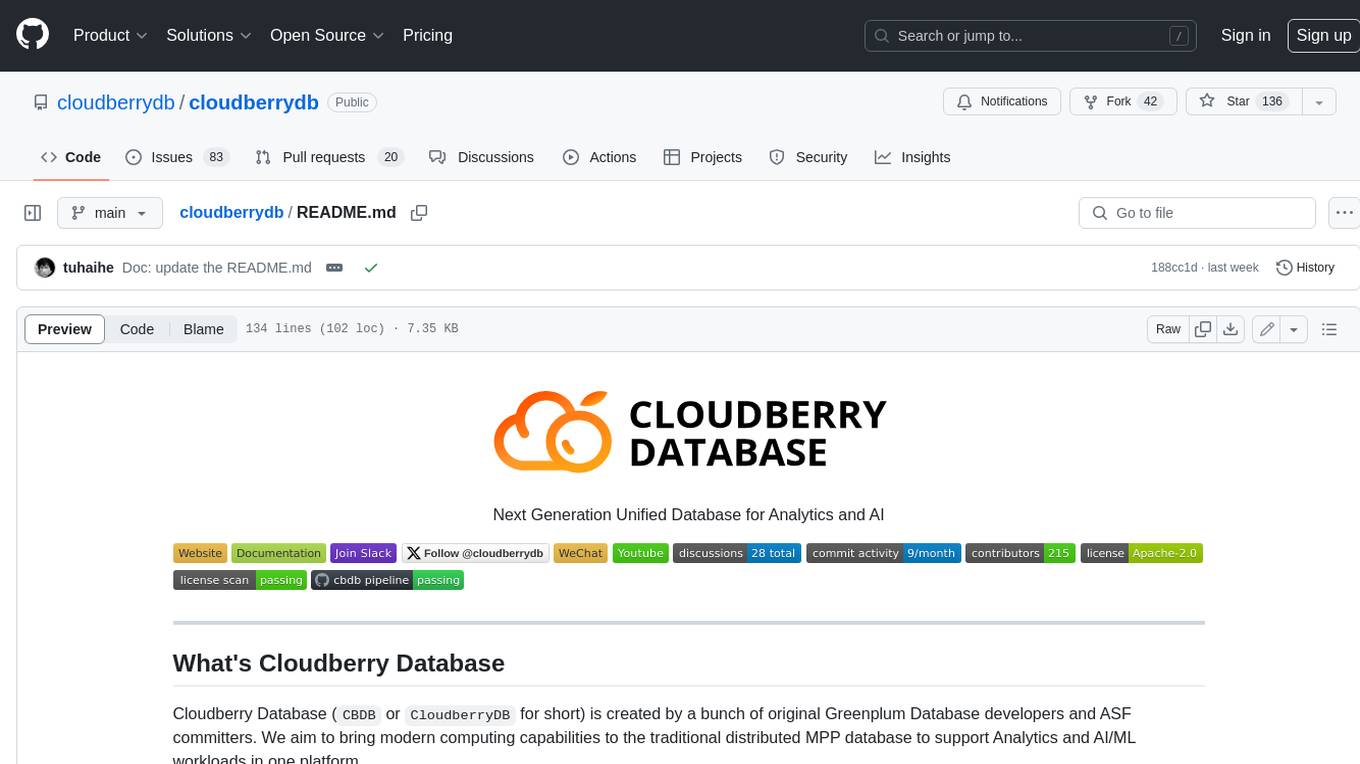
cloudberrydb
Cloudberry Database (CBDB or CloudberryDB) is a next-generation unified database for analytics and AI. It is created by a bunch of original Greenplum Database developers and ASF committers. Cloudberry Database aims to bring modern computing capabilities to the traditional distributed MPP database to support Analytics and AI/ML workloads in one platform.
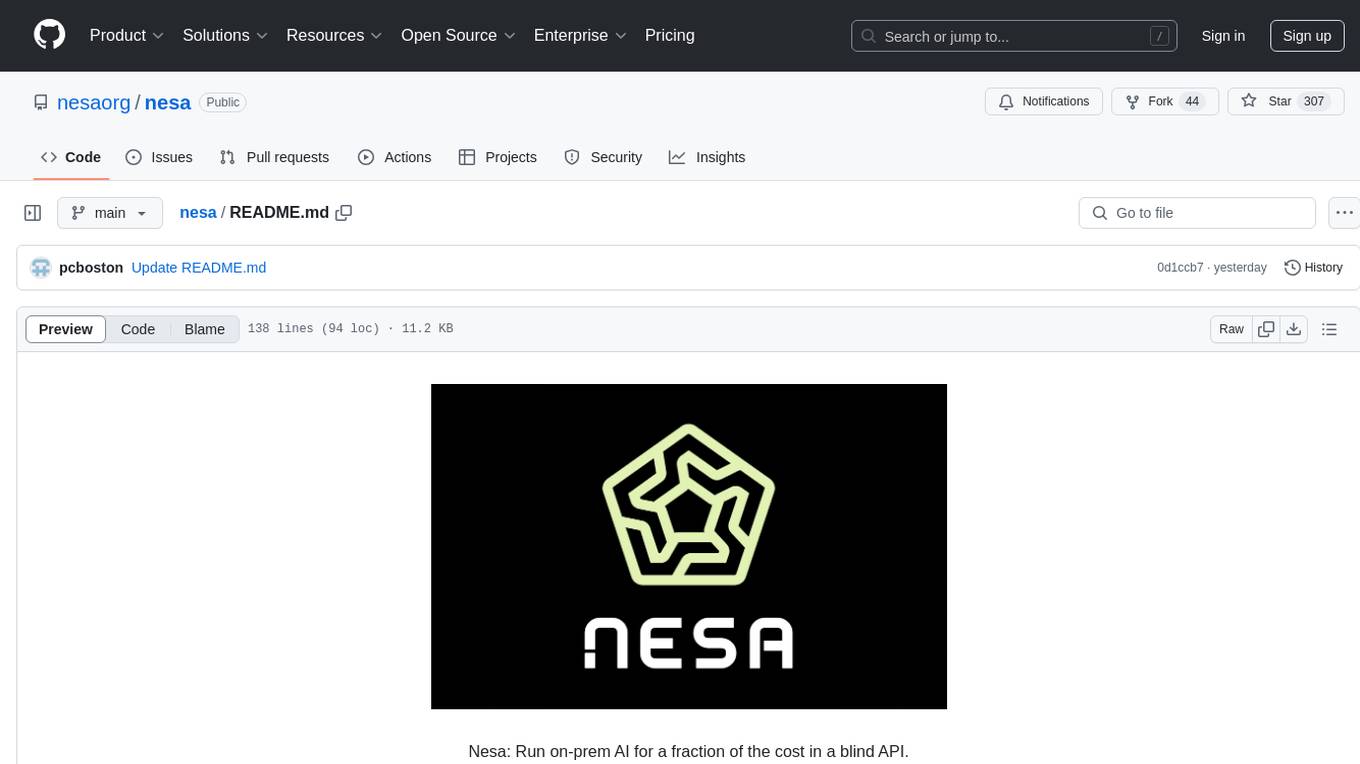
nesa
Nesa is a tool that allows users to run on-prem AI for a fraction of the cost through a blind API. It provides blind privacy, zero latency on protected inference, wide model coverage, cost savings compared to cloud and on-prem AI, RAG support, and ChatGPT compatibility. Nesa achieves blind AI through Equivariant Encryption (EE), a new security technology that provides complete inference encryption with no additional latency. EE allows users to perform inference on neural networks without exposing the underlying data, preserving data privacy and security.
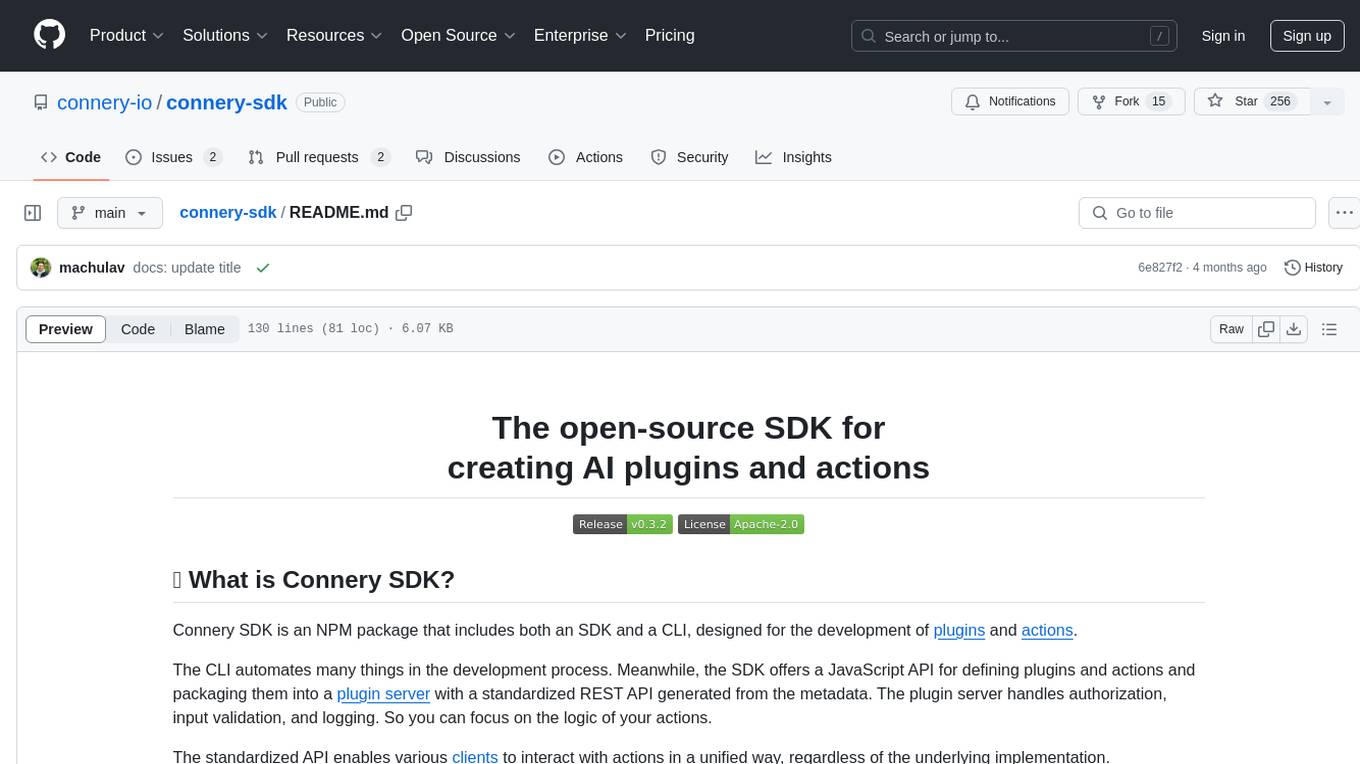
connery-sdk
Connery SDK is an open-source NPM package that provides an SDK and CLI for developing plugins and actions. The SDK offers a JavaScript API to define plugins and actions, which are then packaged into a plugin server with a standardized REST API. This enables automation in the development process and simplifies handling authorization, input validation, and logging. Users can focus on the logic of their actions while the standardized API allows various clients to interact with actions uniformly. Actions can communicate with external APIs, databases, or services, making it versatile for creating AI plugins and actions.
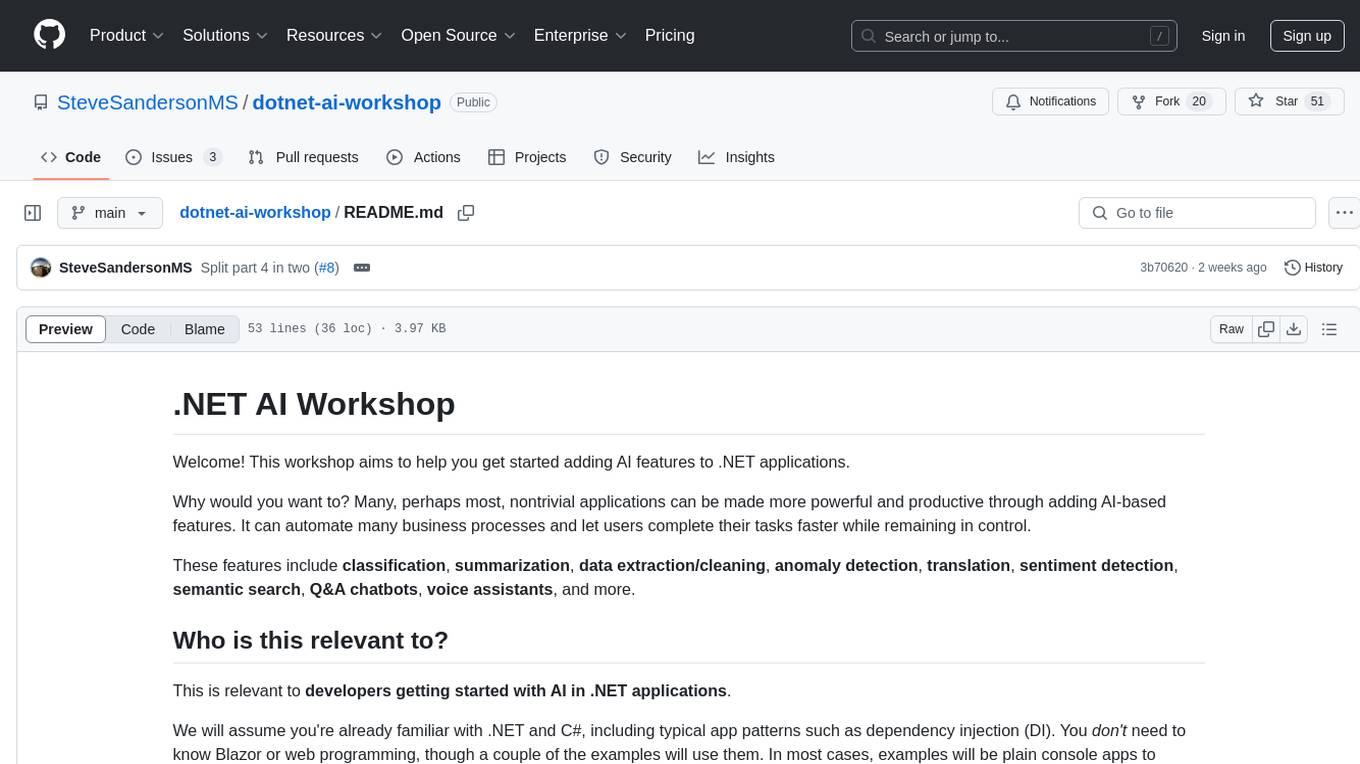
dotnet-ai-workshop
The .NET AI Workshop is a comprehensive guide designed to help developers add AI features to .NET applications. It covers various AI-based features such as classification, summarization, data extraction/cleaning, anomaly detection, translation, sentiment detection, semantic search, Q&A chatbots, and voice assistants. The workshop is tailored for developers new to AI in .NET applications, focusing on the usage of AI services without the need for prior AI technology knowledge. It provides examples using .NET and C# with a focus on AI topics, aiming to enhance productivity and automation in applications.
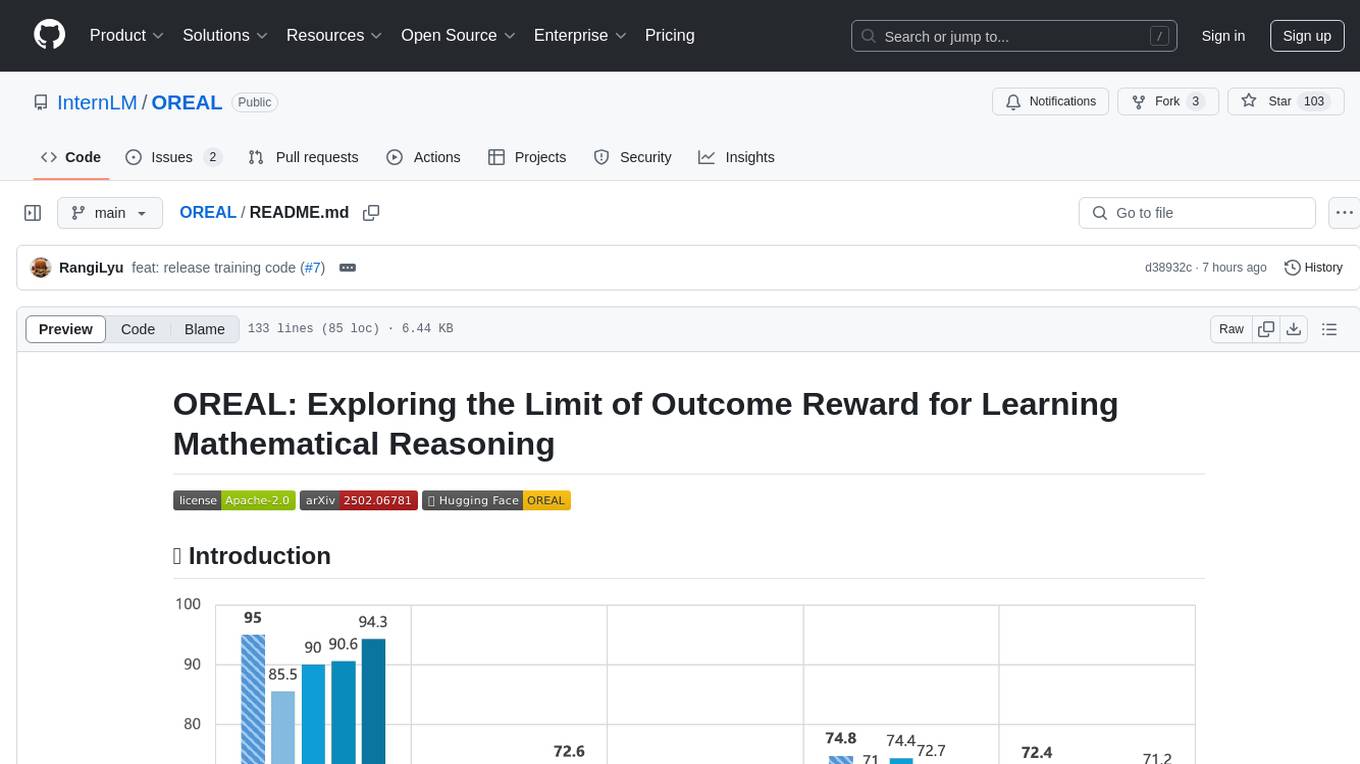
OREAL
OREAL is a reinforcement learning framework designed for mathematical reasoning tasks, aiming to achieve optimal performance through outcome reward-based learning. The framework utilizes behavior cloning, reshaping rewards, and token-level reward models to address challenges in sparse rewards and partial correctness. OREAL has achieved significant results, with a 7B model reaching 94.0 pass@1 accuracy on MATH-500 and surpassing previous 32B models. The tool provides training tutorials and Hugging Face model repositories for easy access and implementation.
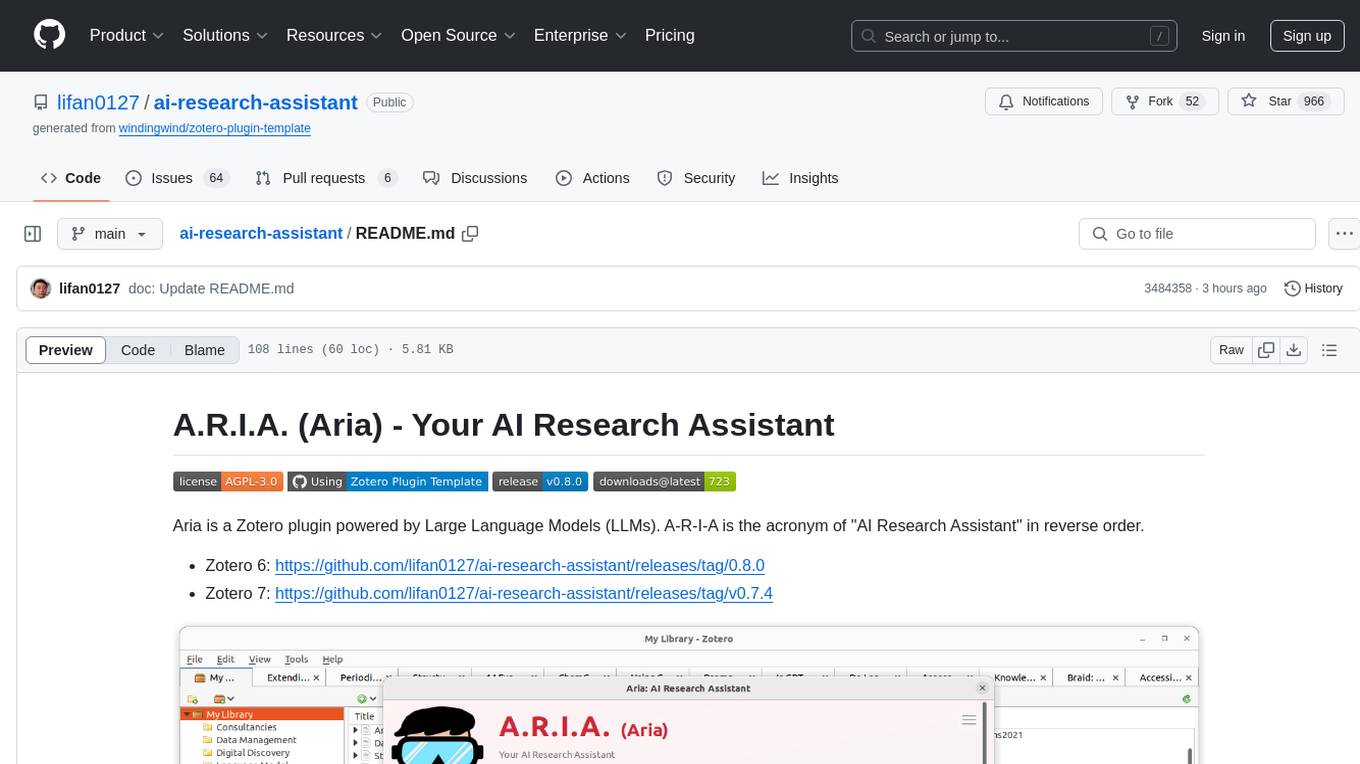
ai-research-assistant
Aria is a Zotero plugin that serves as an AI Research Assistant powered by Large Language Models (LLMs). It offers features like drag-and-drop referencing, autocompletion for creators and tags, visual analysis using GPT-4 Vision, and saving chats as notes and annotations. Aria requires the OpenAI GPT-4 model family and provides a configurable interface through preferences. Users can install Aria by downloading the latest release from GitHub and activating it in Zotero. The tool allows users to interact with Zotero library through conversational AI and probabilistic models, with the ability to troubleshoot errors and provide feedback for improvement.
For similar tasks
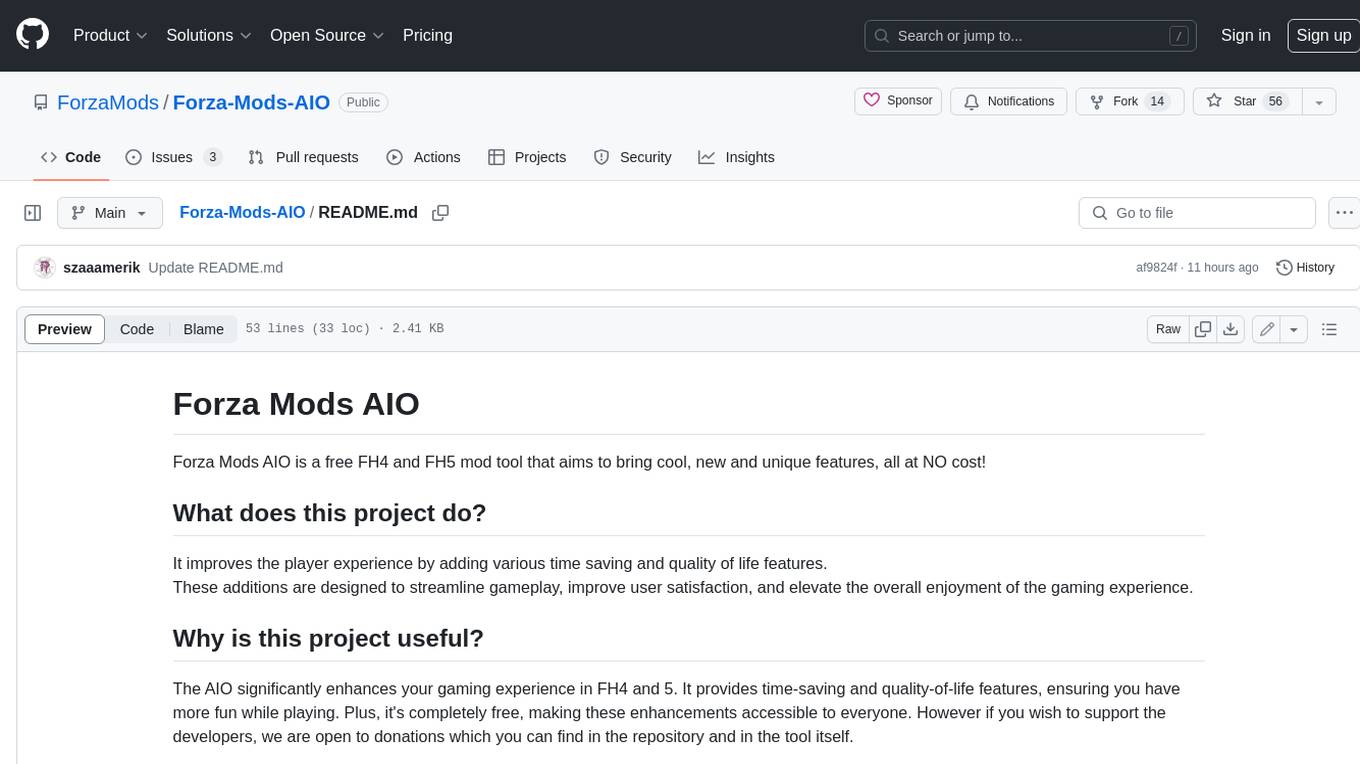
Forza-Mods-AIO
Forza Mods AIO is a free and open-source tool that enhances the gaming experience in Forza Horizon 4 and 5. It offers a range of time-saving and quality-of-life features, making gameplay more enjoyable and efficient. The tool is designed to streamline various aspects of the game, improving user satisfaction and overall enjoyment.
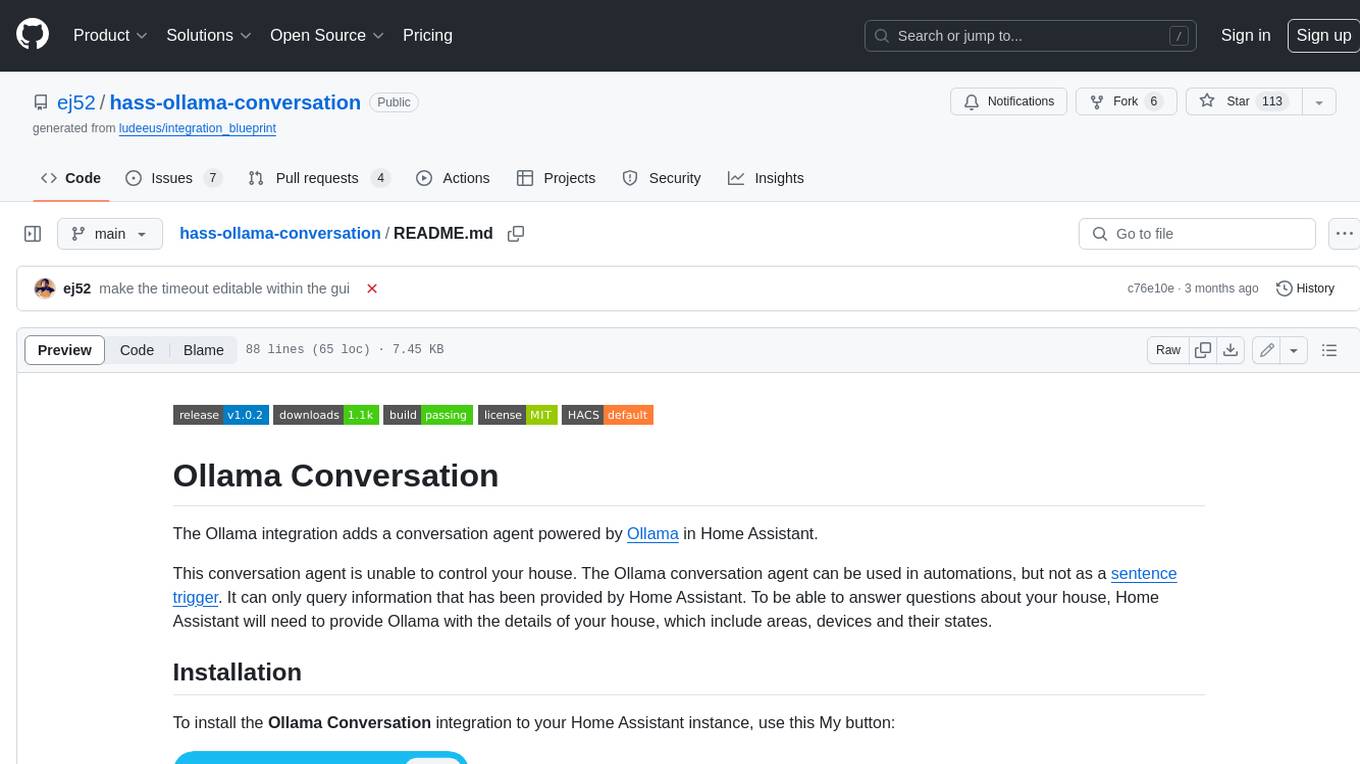
hass-ollama-conversation
The Ollama Conversation integration adds a conversation agent powered by Ollama in Home Assistant. This agent can be used in automations to query information provided by Home Assistant about your house, including areas, devices, and their states. Users can install the integration via HACS and configure settings such as API timeout, model selection, context size, maximum tokens, and other parameters to fine-tune the responses generated by the AI language model. Contributions to the project are welcome, and discussions can be held on the Home Assistant Community platform.
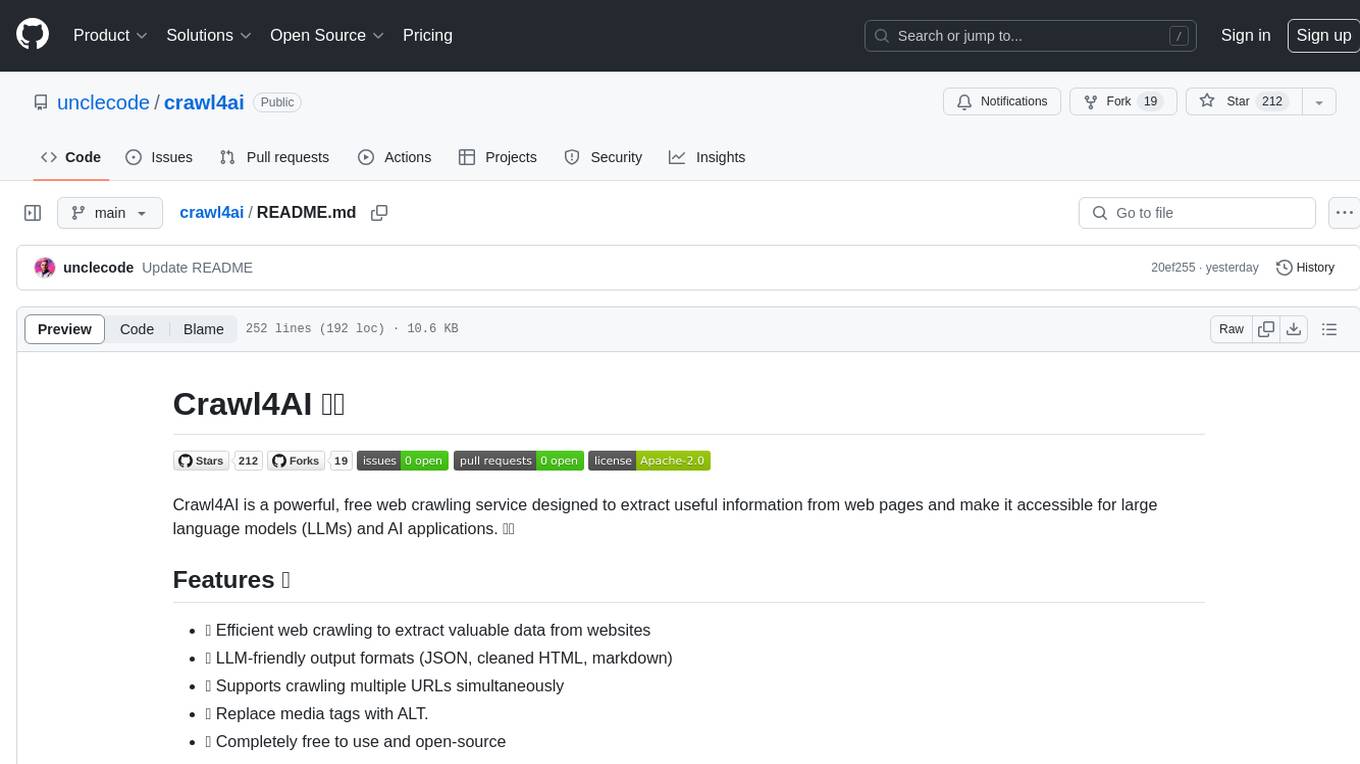
crawl4ai
Crawl4AI is a powerful and free web crawling service that extracts valuable data from websites and provides LLM-friendly output formats. It supports crawling multiple URLs simultaneously, replaces media tags with ALT, and is completely free to use and open-source. Users can integrate Crawl4AI into Python projects as a library or run it as a standalone local server. The tool allows users to crawl and extract data from specified URLs using different providers and models, with options to include raw HTML content, force fresh crawls, and extract meaningful text blocks. Configuration settings can be adjusted in the `crawler/config.py` file to customize providers, API keys, chunk processing, and word thresholds. Contributions to Crawl4AI are welcome from the open-source community to enhance its value for AI enthusiasts and developers.
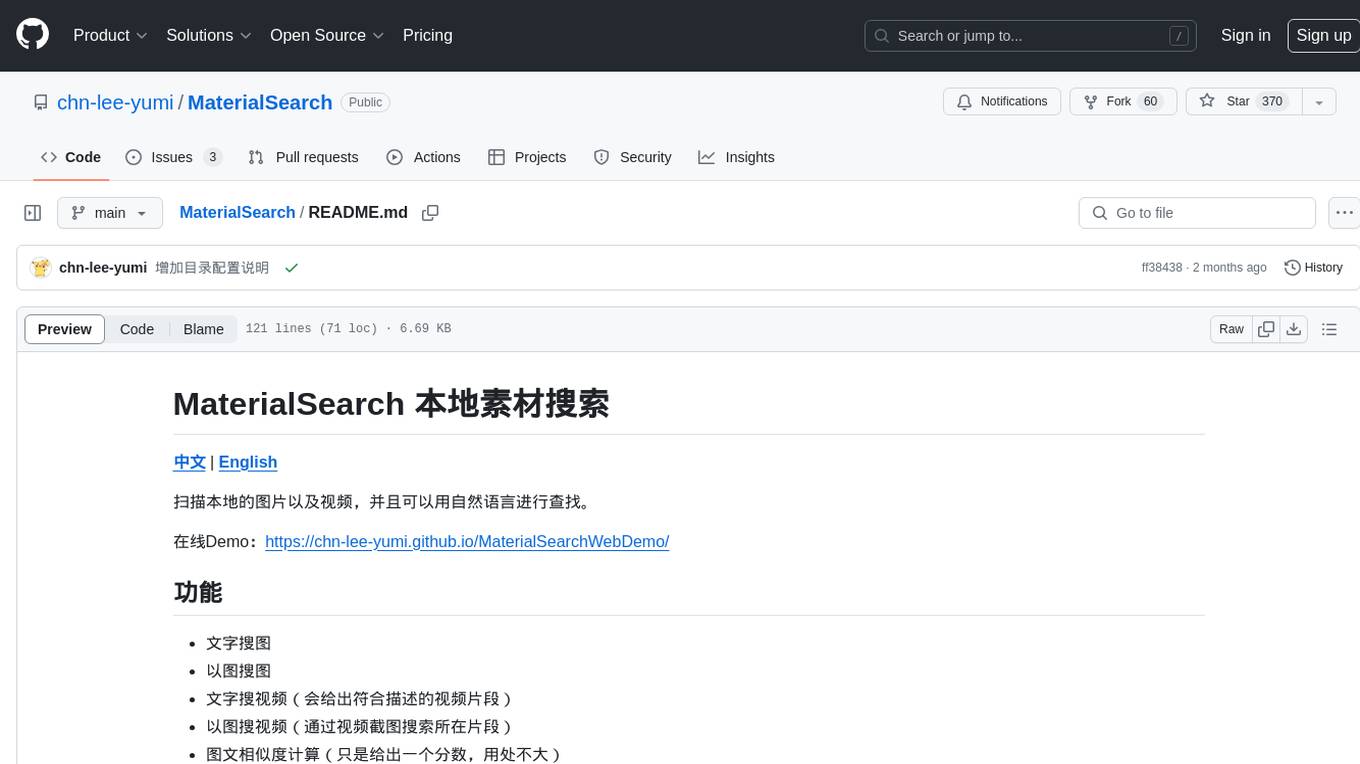
MaterialSearch
MaterialSearch is a tool for searching local images and videos using natural language. It provides functionalities such as text search for images, image search for images, text search for videos (providing matching video clips), image search for videos (searching for the segment in a video through a screenshot), image-text similarity calculation, and Pexels video search. The tool can be deployed through the source code or Docker image, and it supports GPU acceleration. Users can configure the tool through environment variables or a .env file. The tool is still under development, and configurations may change frequently. Users can report issues or suggest improvements through issues or pull requests.
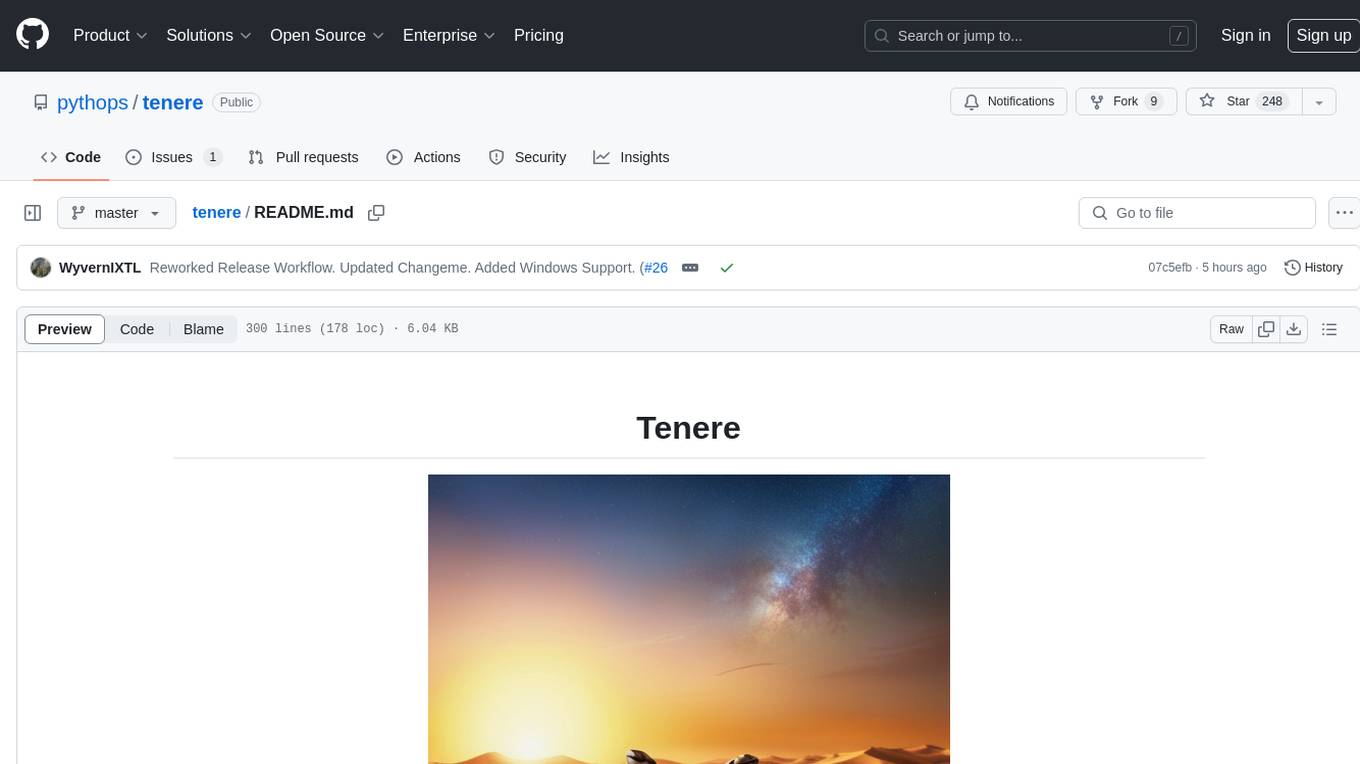
tenere
Tenere is a TUI interface for Language Model Libraries (LLMs) written in Rust. It provides syntax highlighting, chat history, saving chats to files, Vim keybindings, copying text from/to clipboard, and supports multiple backends. Users can configure Tenere using a TOML configuration file, set key bindings, and use different LLMs such as ChatGPT, llama.cpp, and ollama. Tenere offers default key bindings for global and prompt modes, with features like starting a new chat, saving chats, scrolling, showing chat history, and quitting the app. Users can interact with the prompt in different modes like Normal, Visual, and Insert, with various key bindings for navigation, editing, and text manipulation.
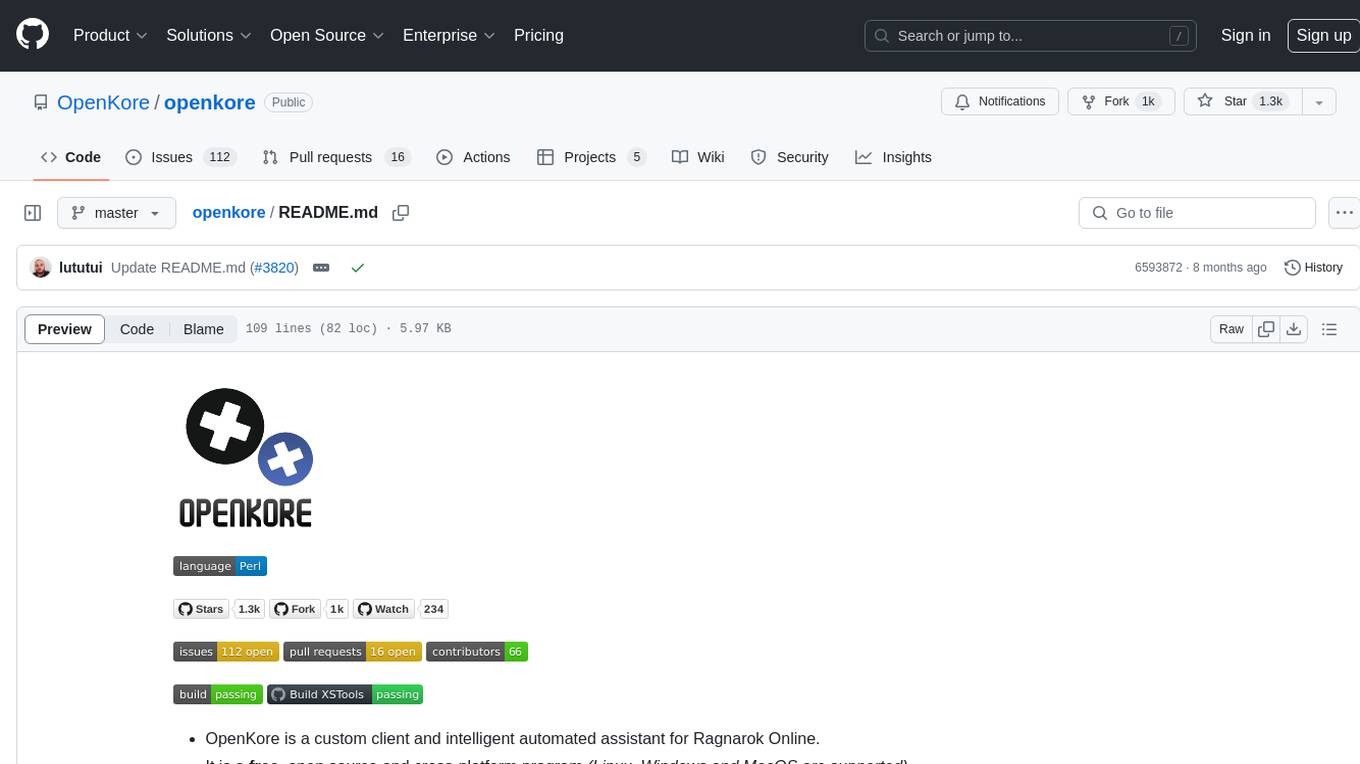
openkore
OpenKore is a custom client and intelligent automated assistant for Ragnarok Online. It is a free, open source, and cross-platform program (Linux, Windows, and MacOS are supported). To run OpenKore, you need to download and extract it or clone the repository using Git. Configure OpenKore according to the documentation and run openkore.pl to start. The tool provides a FAQ section for troubleshooting, guidelines for reporting issues, and information about botting status on official servers. OpenKore is developed by a global team, and contributions are welcome through pull requests. Various community resources are available for support and communication. Users are advised to comply with the GNU General Public License when using and distributing the software.
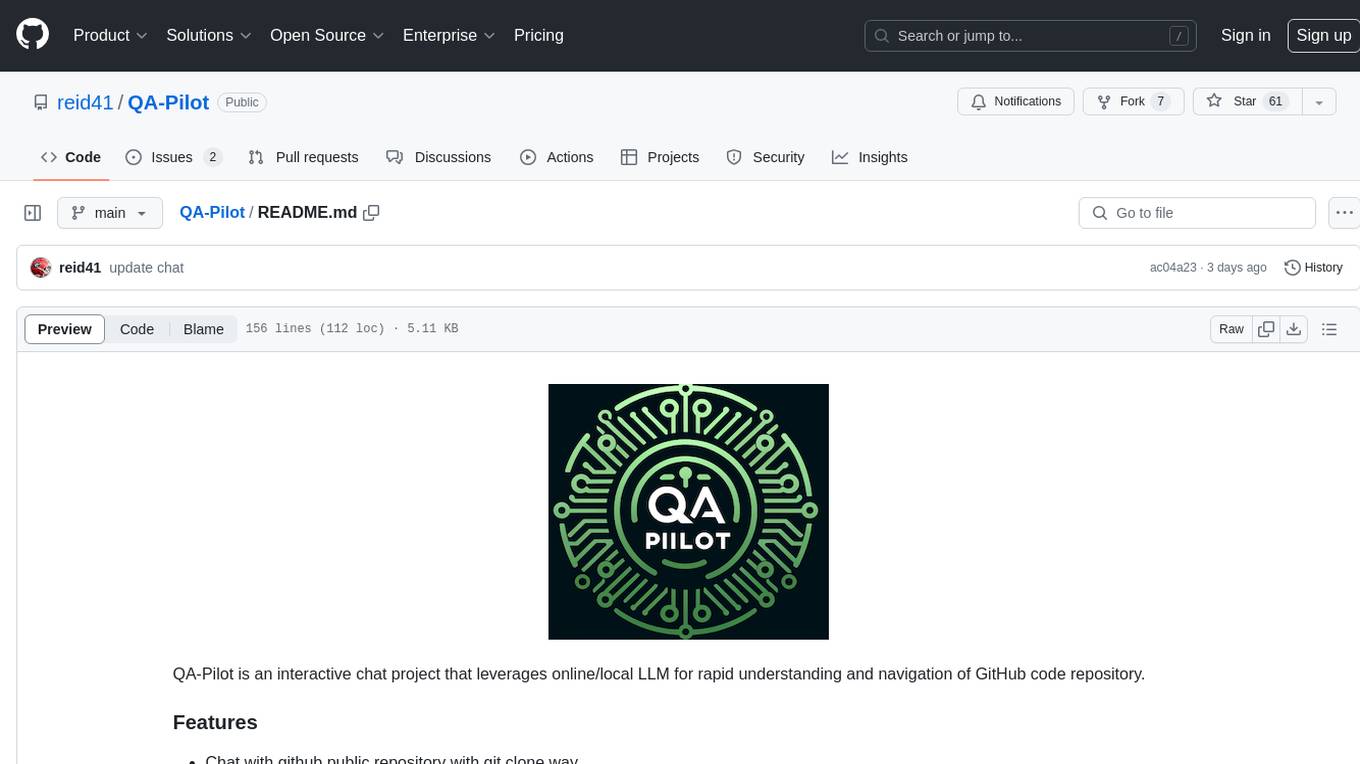
QA-Pilot
QA-Pilot is an interactive chat project that leverages online/local LLM for rapid understanding and navigation of GitHub code repository. It allows users to chat with GitHub public repositories using a git clone approach, store chat history, configure settings easily, manage multiple chat sessions, and quickly locate sessions with a search function. The tool integrates with `codegraph` to view Python files and supports various LLM models such as ollama, openai, mistralai, and localai. The project is continuously updated with new features and improvements, such as converting from `flask` to `fastapi`, adding `localai` API support, and upgrading dependencies like `langchain` and `Streamlit` to enhance performance.
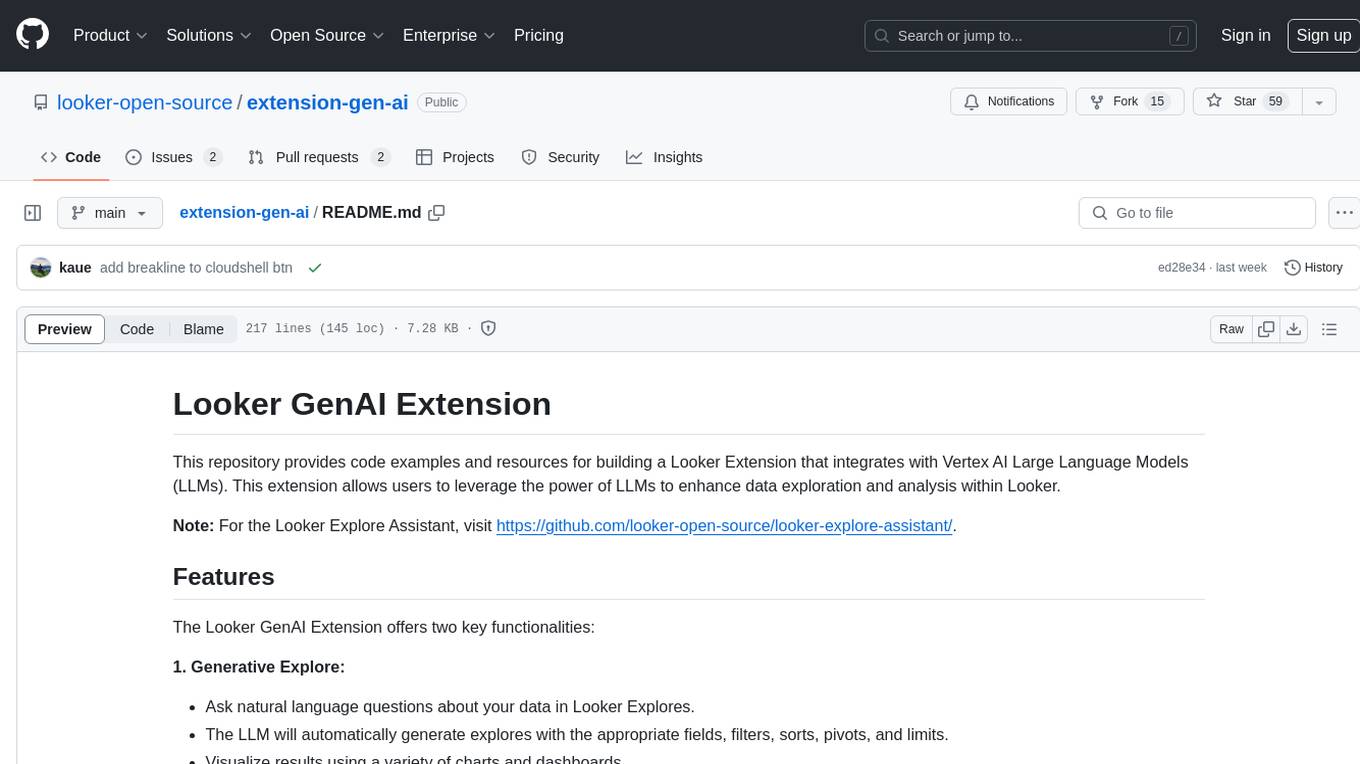
extension-gen-ai
The Looker GenAI Extension provides code examples and resources for building a Looker Extension that integrates with Vertex AI Large Language Models (LLMs). Users can leverage the power of LLMs to enhance data exploration and analysis within Looker. The extension offers generative explore functionality to ask natural language questions about data and generative insights on dashboards to analyze data by asking questions. It leverages components like BQML Remote Models, BQML Remote UDF with Vertex AI, and Custom Fine Tune Model for different integration options. Deployment involves setting up infrastructure with Terraform and deploying the Looker Extension by creating a Looker project, copying extension files, configuring BigQuery connection, connecting to Git, and testing the extension. Users can save example prompts and configure user settings for the extension. Development of the Looker Extension environment includes installing dependencies, starting the development server, and building for production.
For similar jobs
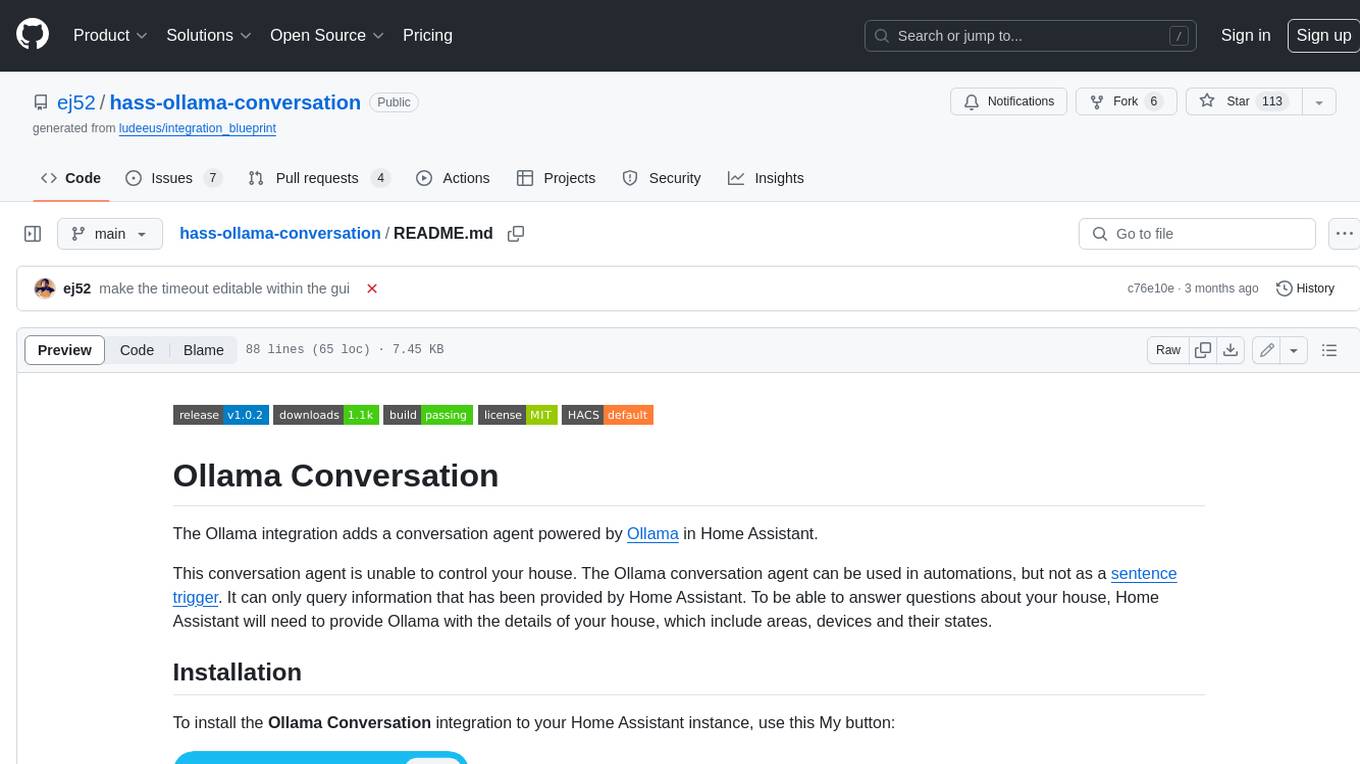
hass-ollama-conversation
The Ollama Conversation integration adds a conversation agent powered by Ollama in Home Assistant. This agent can be used in automations to query information provided by Home Assistant about your house, including areas, devices, and their states. Users can install the integration via HACS and configure settings such as API timeout, model selection, context size, maximum tokens, and other parameters to fine-tune the responses generated by the AI language model. Contributions to the project are welcome, and discussions can be held on the Home Assistant Community platform.
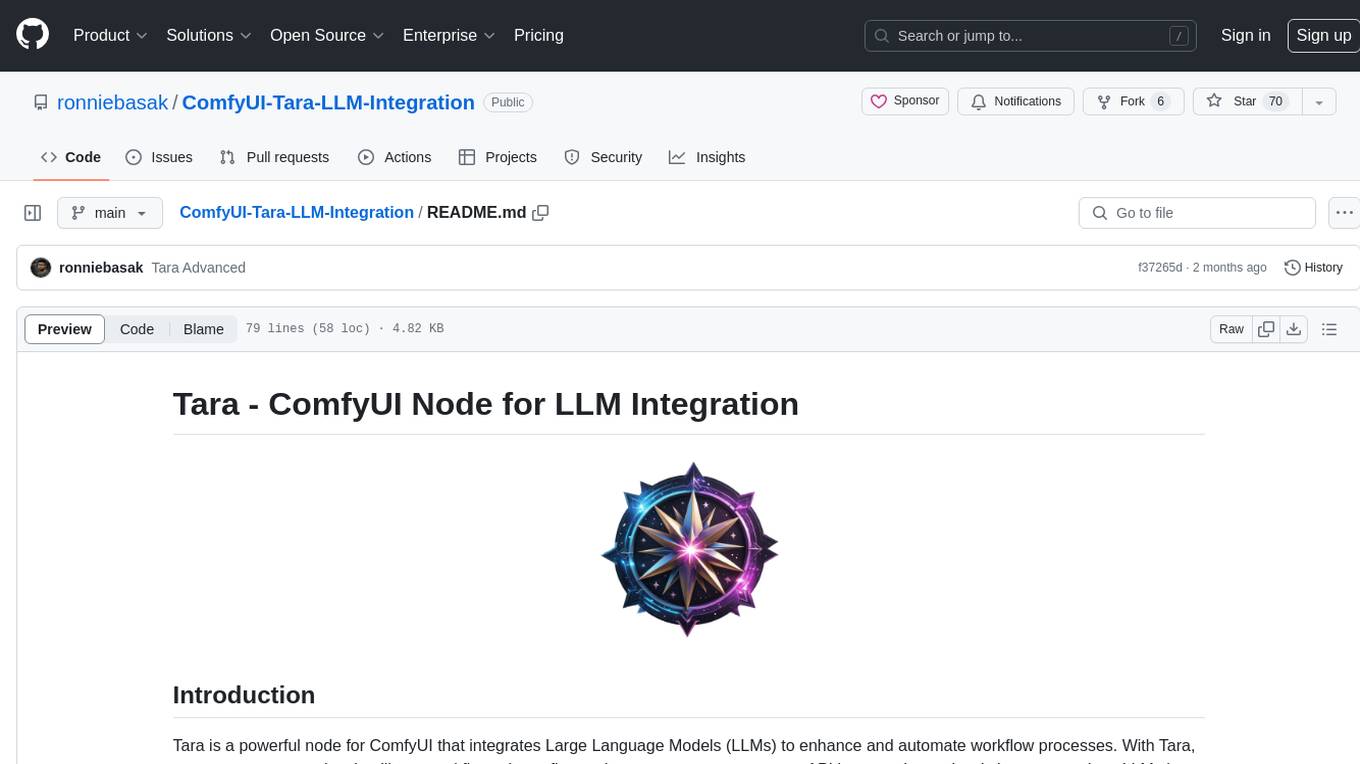
ComfyUI-Tara-LLM-Integration
Tara is a powerful node for ComfyUI that integrates Large Language Models (LLMs) to enhance and automate workflow processes. With Tara, you can create complex, intelligent workflows that refine and generate content, manage API keys, and seamlessly integrate various LLMs into your projects. It comprises nodes for handling OpenAI-compatible APIs, saving and loading API keys, composing multiple texts, and using predefined templates for OpenAI and Groq. Tara supports OpenAI and Grok models with plans to expand support to together.ai and Replicate. Users can install Tara via Git URL or ComfyUI Manager and utilize it for tasks like input guidance, saving and loading API keys, and generating text suitable for chaining in workflows.
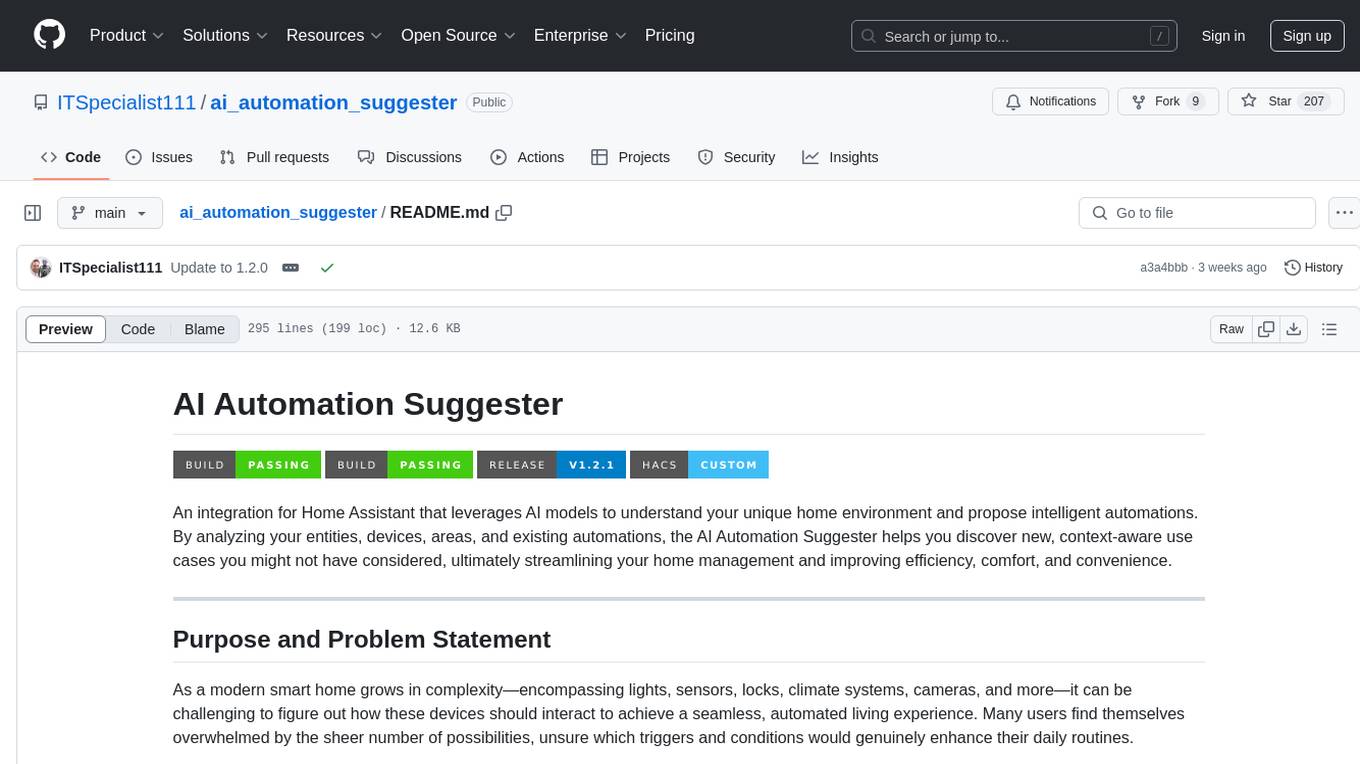
ai_automation_suggester
An integration for Home Assistant that leverages AI models to understand your unique home environment and propose intelligent automations. By analyzing your entities, devices, areas, and existing automations, the AI Automation Suggester helps you discover new, context-aware use cases you might not have considered, ultimately streamlining your home management and improving efficiency, comfort, and convenience. The tool acts as a personal automation consultant, providing actionable YAML-based automations that can save energy, improve security, enhance comfort, and reduce manual intervention. It turns the complexity of a large Home Assistant environment into actionable insights and tangible benefits.
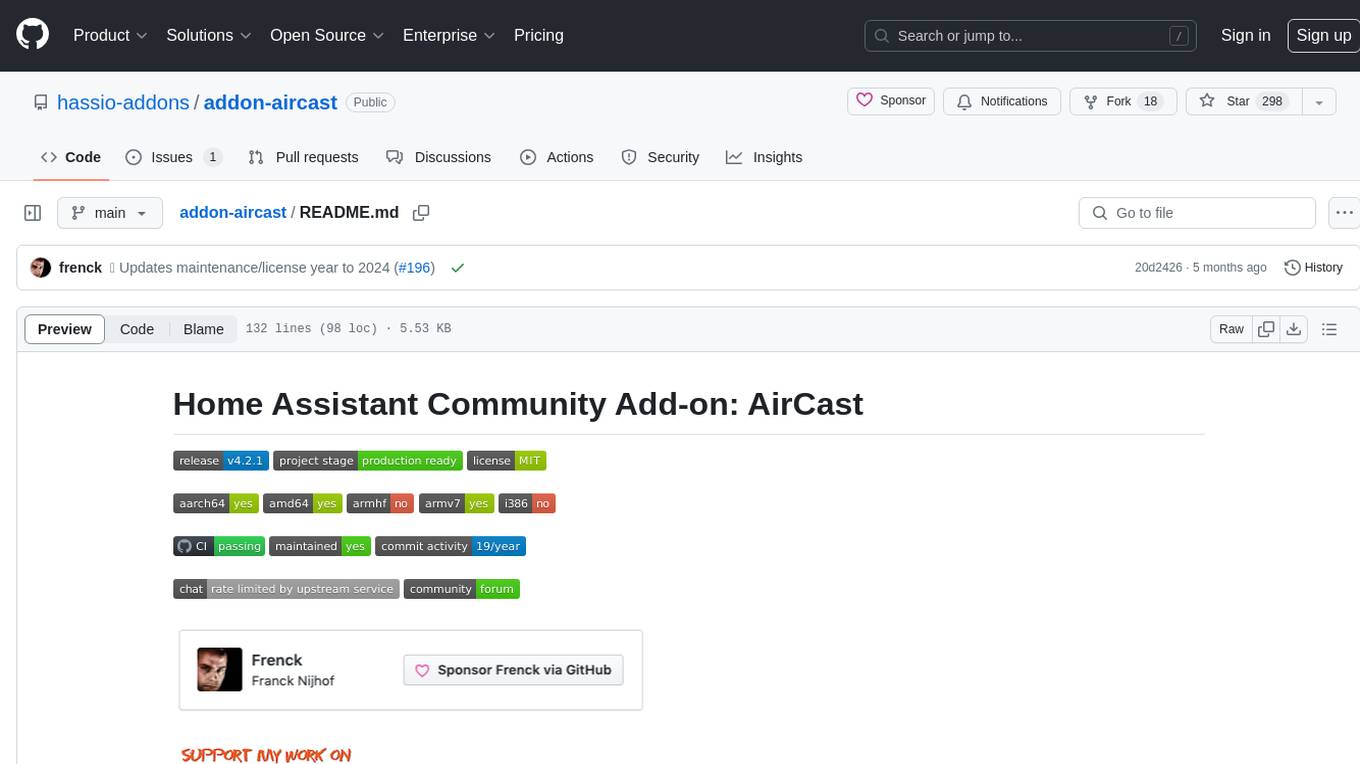
addon-aircast
AirCast is a Home Assistant Community Add-on that provides AirPlay capabilities for Chromecast players. It bridges the compatibility gap between Apple's AirPlay and Google's Chromecast by creating virtual AirPlay devices for Chromecast players on the network. The add-on is based on the AirConnect project and allows users to stream audio from Apple devices to Chromecast players.
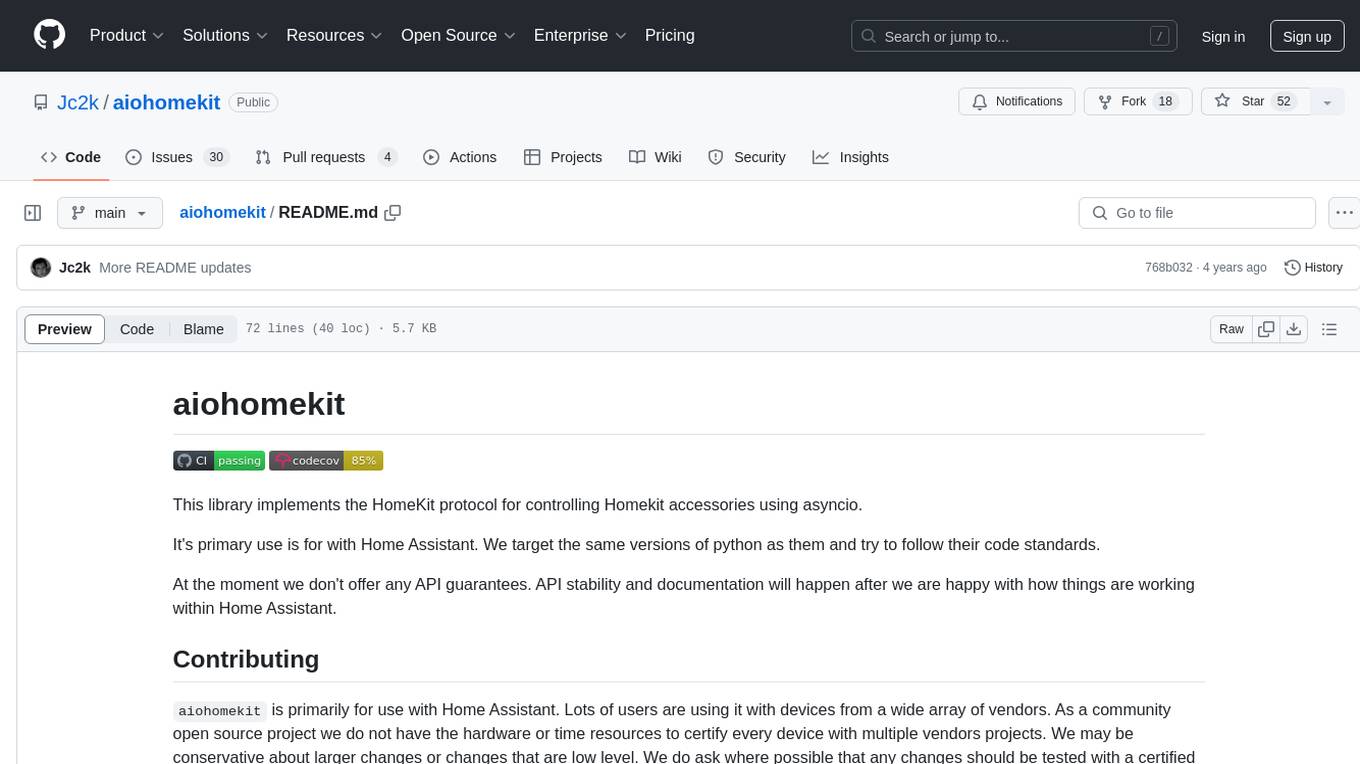
aiohomekit
aiohomekit is a Python library that implements the HomeKit protocol for controlling HomeKit accessories using asyncio. It is primarily used with Home Assistant, targeting the same versions of Python and following their code standards. The library is still under development and does not offer API guarantees yet. It aims to match the behavior of real HAP controllers, even when not strictly specified, and works around issues like JSON formatting, boolean encoding, header sensitivity, and TCP packet splitting. aiohomekit is primarily tested with Phillips Hue and Eve Extend bridges via Home Assistant, but is known to work with many more devices. It does not support BLE accessories and is intended for client-side use only.