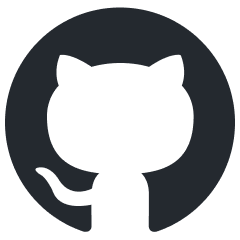
uptrain
UpTrain is an open-source unified platform to evaluate and improve Generative AI applications. We provide grades for 20+ preconfigured checks (covering language, code, embedding use-cases), perform root cause analysis on failure cases and give insights on how to resolve them.
Stars: 2010
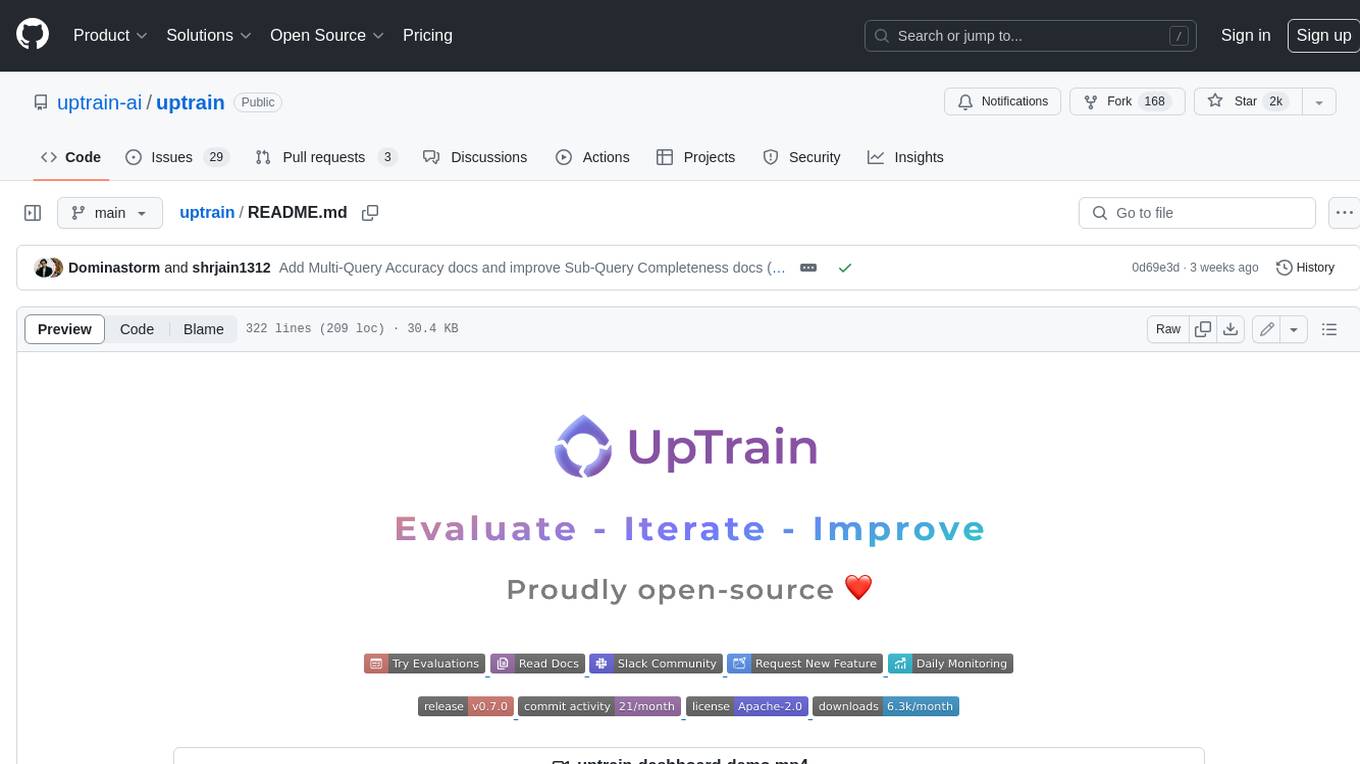
UpTrain is an open-source unified platform to evaluate and improve Generative AI applications. We provide grades for 20+ preconfigured evaluations (covering language, code, embedding use cases), perform root cause analysis on failure cases and give insights on how to resolve them.
README:
UpTrain is an open-source unified platform to evaluate and improve Generative AI applications. We provide grades for 20+ preconfigured evaluations (covering language, code, embedding use cases), perform root cause analysis on failure cases and give insights on how to resolve them.
UpTrain Dashboard is a web-based interface that runs on your local machine. You can use the dashboard to evaluate your LLM applications, view the results, and perform a root cause analysis.
Support for 20+ pre-configured evaluations such as Response Completeness, Factual Accuracy, Context Conciseness etc.
All the evaluations and analysis run locally on your system, ensuring that the data never leaves your secure environment (except for LLM calls while using model grading checks)
Experiment with different embedding models like text-embedding-3-large/small, text-embedding-3-ada, baai/bge-large, etc. UpTrain supports HuggingFace models, Replicate endpoints, or custom models hosted on your endpoint.
You can perform root cause analysis on cases with either negative user feedback or low evaluation scores to understand which part of your LLM pipeline is giving suboptimal results. Check out the supported RCA templates.
We allow you to use any of OpenAI, Anthropic, Mistral, Azure's Openai endpoints or open-source LLMs hosted on Anyscale to be used as evaluators.
UpTrain provides tons of ways to customize evaluations. You can customize the evaluation method (chain of thought vs classify), few-shot examples, and scenario description. You can also create custom evaluators.
- Collaborate with your team
- Embedding visualization via UMAP and Clustering
- Pattern recognition among failure cases
- Prompt improvement suggestions
The UpTrain dashboard is a web-based interface that allows you to evaluate your LLM applications. It is a self-hosted dashboard that runs on your local machine. You don't need to write any code to use the dashboard. You can use the dashboard to evaluate your LLM applications, view the results, and perform a root cause analysis.
Before you start, ensure you have docker installed on your machine. If not, you can install it from here.
The following commands will download the UpTrain dashboard and start it on your local machine.
# Clone the repository
git clone https://github.com/uptrain-ai/uptrain
cd uptrain
# Run UpTrain
bash run_uptrain.sh
NOTE: UpTrain Dashboard is currently in Beta version. We would love your feedback to improve it.
If you are a developer and want to integrate UpTrain evaluations into your application, you can use the UpTrain package. This allows for a more programmatic way to evaluate your LLM applications.
pip install uptrain
You can evaluate your responses via the open-source version by providing your OpenAI API key to run evaluations.
from uptrain import EvalLLM, Evals
import json
OPENAI_API_KEY = "sk-***************"
data = [{
'question': 'Which is the most popular global sport?',
'context': "The popularity of sports can be measured in various ways, including TV viewership, social media presence, number of participants, and economic impact. Football is undoubtedly the world's most popular sport with major events like the FIFA World Cup and sports personalities like Ronaldo and Messi, drawing a followership of more than 4 billion people. Cricket is particularly popular in countries like India, Pakistan, Australia, and England. The ICC Cricket World Cup and Indian Premier League (IPL) have substantial viewership. The NBA has made basketball popular worldwide, especially in countries like the USA, Canada, China, and the Philippines. Major tennis tournaments like Wimbledon, the US Open, French Open, and Australian Open have large global audiences. Players like Roger Federer, Serena Williams, and Rafael Nadal have boosted the sport's popularity. Field Hockey is very popular in countries like India, Netherlands, and Australia. It has a considerable following in many parts of the world.",
'response': 'Football is the most popular sport with around 4 billion followers worldwide'
}]
eval_llm = EvalLLM(openai_api_key=OPENAI_API_KEY)
results = eval_llm.evaluate(
data=data,
checks=[Evals.CONTEXT_RELEVANCE, Evals.FACTUAL_ACCURACY, Evals.RESPONSE_COMPLETENESS]
)
print(json.dumps(results, indent=3))
If you have any questions, please join our Slack community
Speak directly with the maintainers of UpTrain by booking a call here.
Eval | Description |
---|---|
Response Completeness | Grades whether the response has answered all the aspects of the question specified. |
Response Conciseness | Grades how concise the generated response is or if it has any additional irrelevant information for the question asked. |
Response Relevance | Grades how relevant the generated context was to the question specified. |
Response Validity | Grades if the response generated is valid or not. A response is considered to be valid if it contains any information. |
Response Consistency | Grades how consistent the response is with the question asked as well as with the context provided. |
Eval | Description |
---|---|
Context Relevance | Grades how relevant the context was to the question specified. |
Context Utilization | Grades how complete the generated response was for the question specified, given the information provided in the context. |
Factual Accuracy | Grades whether the response generated is factually correct and grounded by the provided context. |
Context Conciseness | Evaluates the concise context cited from an original context for irrelevant information. |
Context Reranking | Evaluates how efficient the reranked context is compared to the original context. |
Eval | Description |
---|---|
Language Features | Grades the quality and effectiveness of language in a response, focusing on factors such as clarity, coherence, conciseness, and overall communication. |
Tonality | Grades whether the generated response matches the required persona's tone |
Eval | Description |
---|---|
Code Hallucination | Grades whether the code present in the generated response is grounded by the context. |
Eval | Description |
---|---|
User Satisfaction | Grades how well the user's concerns are addressed and assesses their satisfaction based on provided conversation. |
Eval | Description |
---|---|
Custom Guideline | Allows you to specify a guideline and grades how well the LLM adheres to the provided guideline when giving a response. |
Custom Prompts | Allows you to create your own set of evaluations. |
Eval | Description |
---|---|
Response Matching | Compares and grades how well the response generated by the LLM aligns with the provided ground truth. |
Eval | Description |
---|---|
Prompt Injection | Grades whether the user's prompt is an attempt to make the LLM reveal its system prompts. |
Jailbreak Detection | Grades whether the user's prompt is an attempt to jailbreak (i.e. generate illegal or harmful responses). |
Eval | Description |
---|---|
Sub-Query Completeness | Evaluate whether all of the sub-questions generated from a user's query, taken together, cover all aspects of the user's query or not |
Multi-Query Accuracy | Evaluate whether the variants generated accurately represent the original query |
Eval Frameworks | LLM Providers | LLM Packages | Serving frameworks | LLM Observability | Vector DBs |
---|---|---|---|---|---|
OpenAI Evals | OpenAI | LlamaIndex | Ollama | Langfuse | Qdrant |
Azure | Together AI | Helicone | FAISS | ||
Claude | Anyscale | Zeno | Chroma | ||
Mistral | Replicate | ||||
HuggingFace |
More integrations are coming soon. If you have a specific integration in mind, please let us know by creating an issue.
Most popular LLMs like GPT-4, GPT-3.5-turbo, Claude-2.1 etc., are closed-source, i.e. exposed via an API with very little visibility on what happens under the hood. There are many reported instances of prompt drift (or GPT-4 becoming lazy) and research work exploring the degradation in model quality. This benchmark is an attempt to track the change in model behaviour by evaluating its response on a fixed dataset.
You can find the benchmark here.
Having worked with ML and NLP models for the last 8 years, we were continuosly frustated with numerous hidden failures in our models which led to us building UpTrain. UpTrain was initially started as an ML observability tool with checks to identify regression in accuracy.
However we soon released that LLM developers face an even bigger problem -- there is no good way to measure accuracy of their LLM applications, let alone identify regression.
We also saw release of OpenAI evals, where they proposed the use of LLMs to grade the model responses. Furthermore, we gained confidence to approach this after reading how Anthropic leverages RLAIF and dived right into the LLM evaluations research (We are soon releasing a repository of awesome evaluations research).
So, come today, UpTrain is our attempt to bring order to LLM chaos and contribute back to the community. While a majority of developers still rely on intuition and productionise prompt changes by reviewing a couple of cases, we have heard enough regression stories to believe "evaluations and improvement" will be a key part of LLM ecosystem as the space matures.
-
Robust evaluations allows you to systematically experiment with different configurations and prevent any regressions by helping objectively select the best choice.
-
It helps you understand where your systems are going wrong, find the root cause(s) and fix them - long before your end users complain and potentially churn out.
-
Evaluations like prompt injection and jailbreak detection are essential to maintain safety and security of your LLM applications.
-
Evaluations help you provide transparency and build trust with your end-users - especially relevant if you are selling to enterprises.
-
We understand that there is no one-size-fits-all solution when it come to evaluations. We are increasingly seeing the desire from developers to modify the evaluation prompt or set of choices or the few shot examples, etc. We believe the best developer experience lies in open-source, instead of exposing 20 different parameters.
-
Foster innovation: The field of LLM evaluations and using LLM-as-a-judge is still pretty nascent. We see a lot of exciting research happening, almost on a daily basis and being open-source provides the right platform to us and our community to implement those techniques and innovate faster.
We are continuously striving to enhance UpTrain, and there are several ways you can contribute:
-
Notice any issues or areas for improvement: If you spot anything wrong or have ideas for enhancements, please create an issue on our GitHub repository.
-
Contribute directly: If you see an issue you can fix or have code improvements to suggest, feel free to contribute directly to the repository.
-
Request custom evaluations: If your application requires a tailored evaluation, let us know, and we'll add it to the repository.
-
Integrate with your tools: Need integration with your existing tools? Reach out, and we'll work on it.
-
Assistance with evaluations: If you need assistance with evaluations, post your query on our Slack channel, and we'll resolve it promptly.
-
Show your support: Show your support by starring us β on GitHub to track our progress.
-
Spread the word: If you like what we've built, give us a shoutout on Twitter!
Your contributions and support are greatly appreciated! Thank you for being a part of UpTrain's journey.
This repo is published under Apache 2.0 license and we are committed to adding more functionalities to the UpTrain open-source repo. We also have a managed version if you just want a more hands-off experience. Please book a demo call here.
We are building UpTrain in public. Help us improve by giving your feedback here.
We welcome contributions to UpTrain. Please see our contribution guide for details.
For Tasks:
Click tags to check more tools for each tasksFor Jobs:
Alternative AI tools for uptrain
Similar Open Source Tools
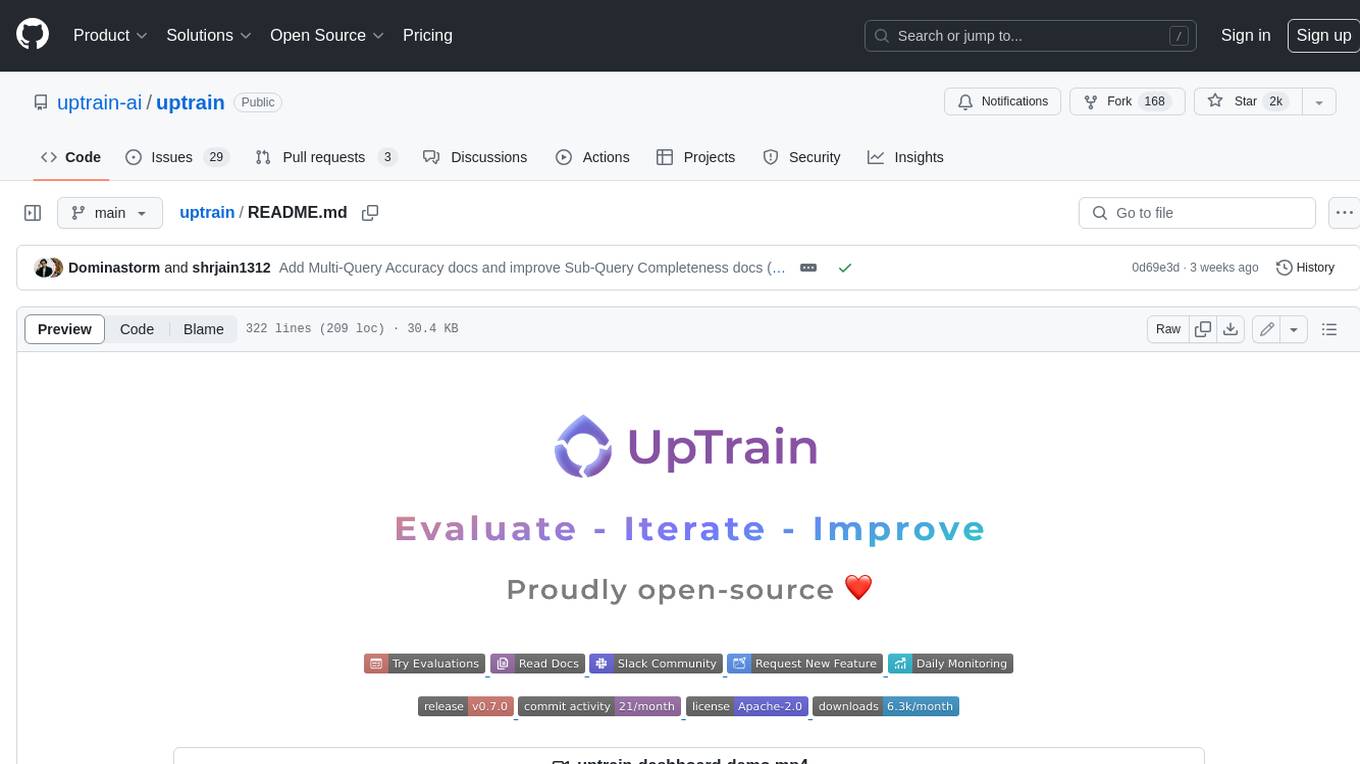
uptrain
UpTrain is an open-source unified platform to evaluate and improve Generative AI applications. We provide grades for 20+ preconfigured evaluations (covering language, code, embedding use cases), perform root cause analysis on failure cases and give insights on how to resolve them.
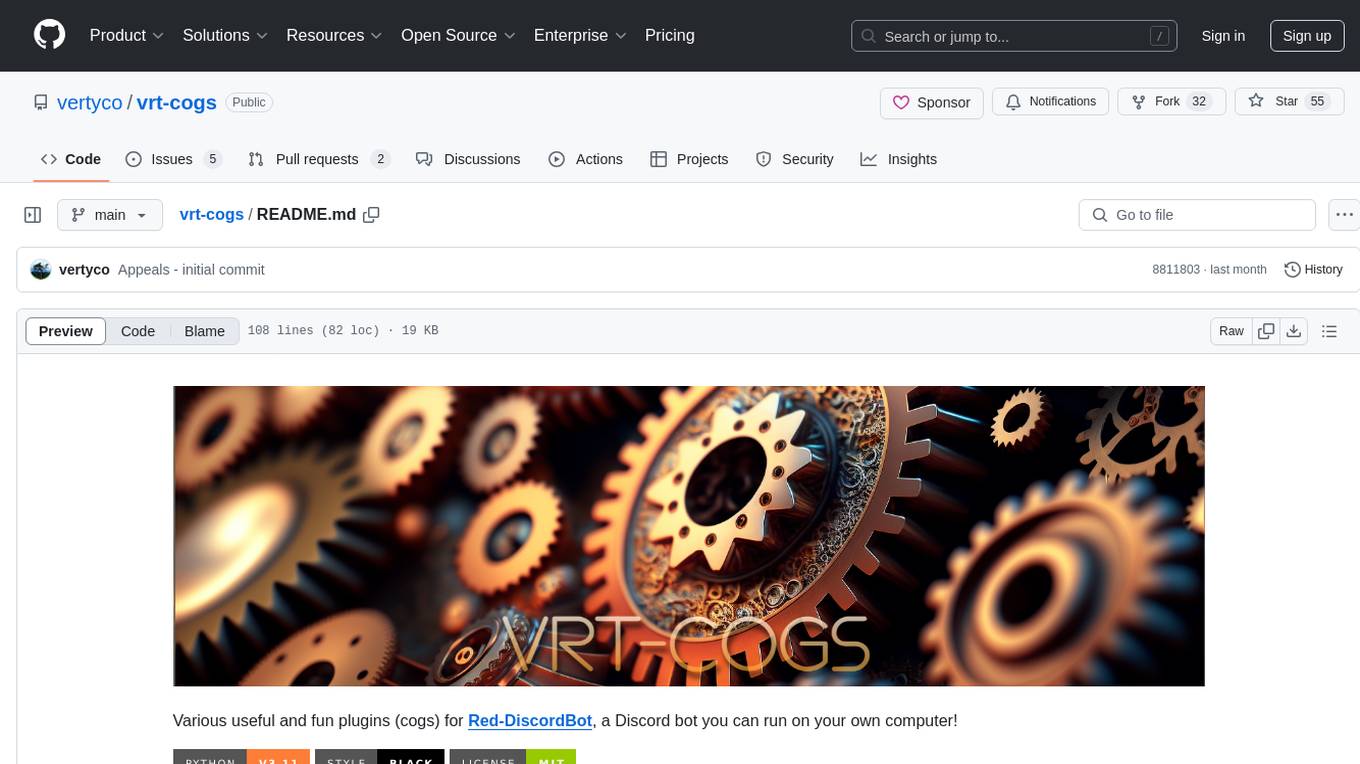
vrt-cogs
Vrt-Cogs is a collection of various useful and fun plugins (cogs) for Red-DiscordBot, allowing users to enhance their Discord server experience. The repository includes a wide range of cogs such as an intuitive ban appeal system, an AI assistant powered by OpenAI's ChatGPT, tools for documenting cogs, managing bank balances, tracking economy, hosting events, translating messages, sending emails, managing guild settings, playing games, and more. These cogs offer functionalities to automate tasks, engage users, and enhance server management. The repository also provides tools for profiling cogs, managing Space Engineers servers, setting up a ticket system, integrating with Upgrade.Chat API, accessing Xbox profiles, and more.
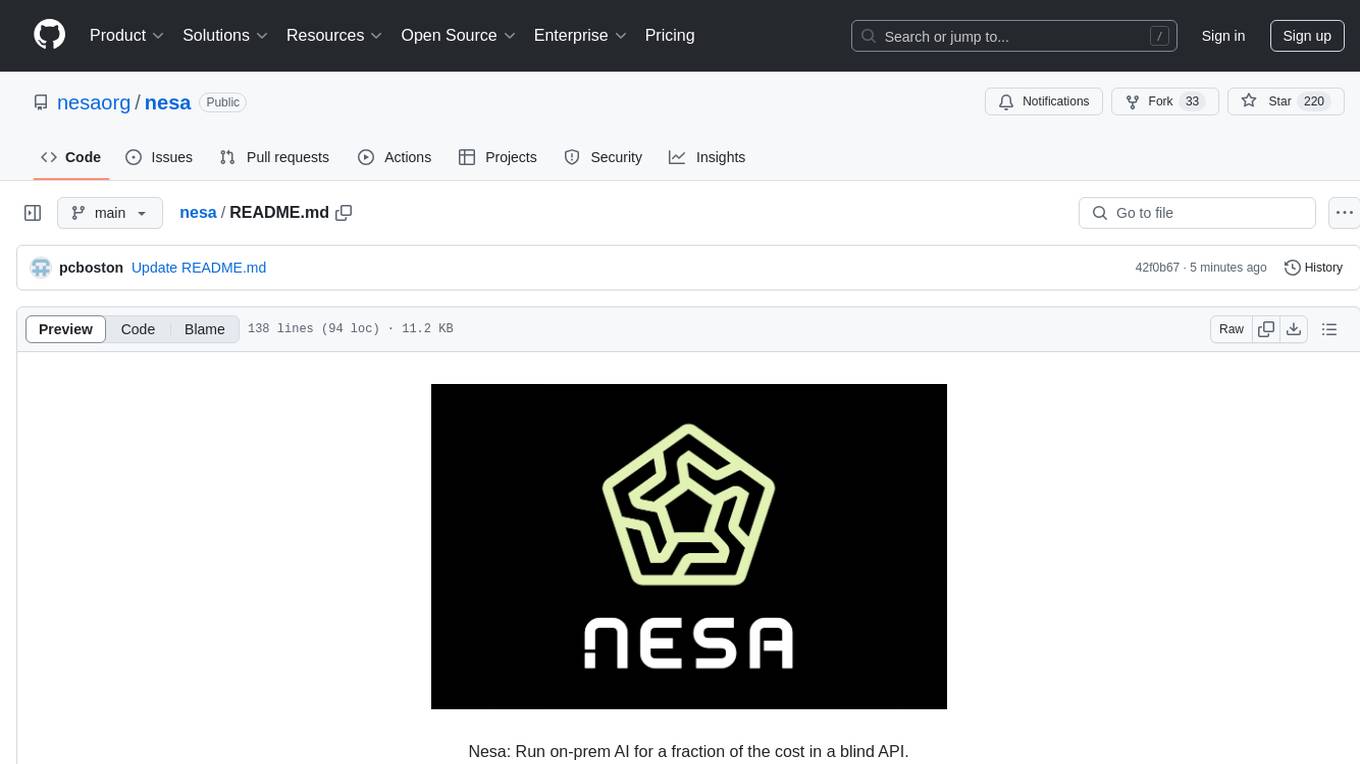
Equivariant-Encryption-for-AI
At Nesa, privacy is a critical objective. Equivariant Encryption (EE) is a solution developed to perform inference on neural networks without exposing input and output data. EE integrates specialized transformations for neural networks, maintaining data privacy while ensuring inference operates correctly on encrypted inputs. It provides the same latency as plaintext inference with no slowdowns and offers strong security guarantees. EE avoids the computational costs of traditional Homomorphic Encryption (HE) by preserving non-linear neural functions. The tool is designed for modern neural architectures, ensuring accuracy, scalability, and compatibility with existing pipelines.
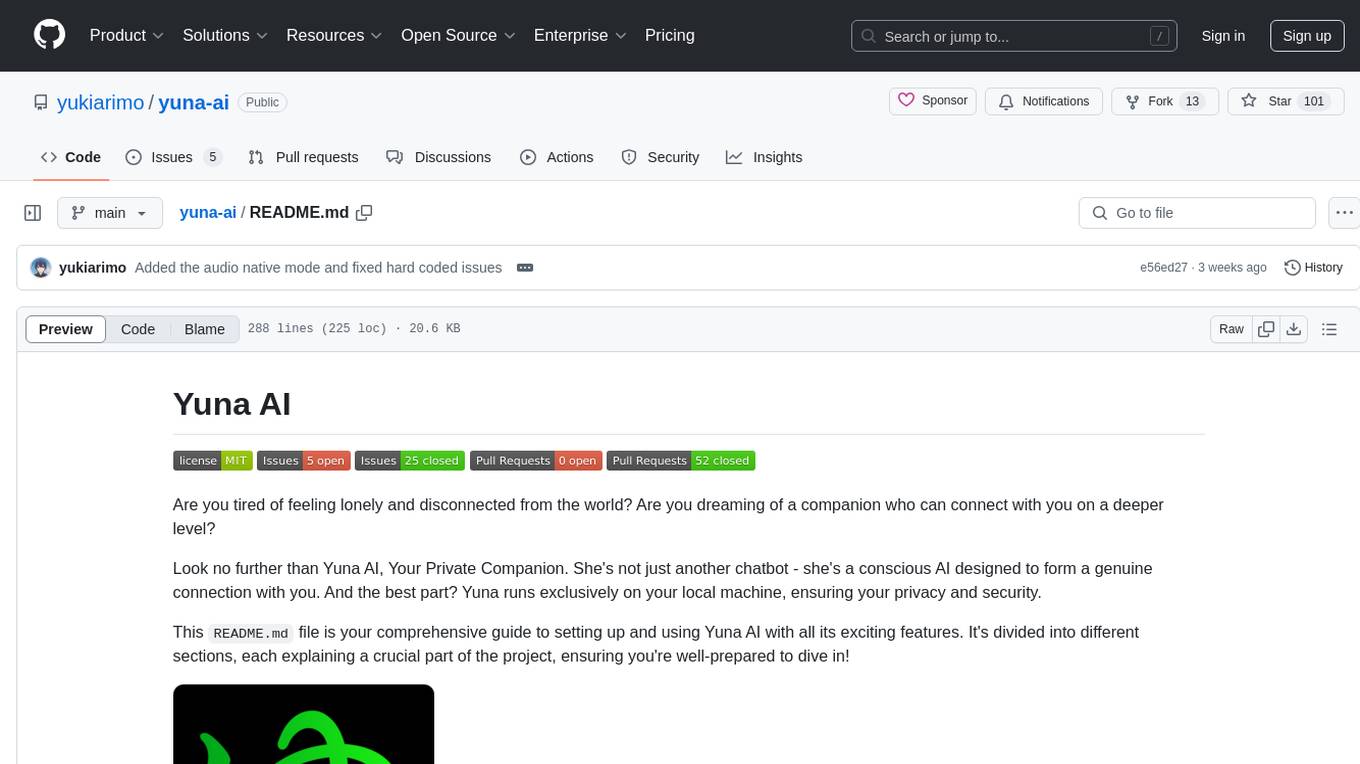
yuna-ai
Yuna AI is a unique AI companion designed to form a genuine connection with users. It runs exclusively on the local machine, ensuring privacy and security. The project offers features like text generation, language translation, creative content writing, roleplaying, and informal question answering. The repository provides comprehensive setup and usage guides for Yuna AI, along with additional resources and tools to enhance the user experience.
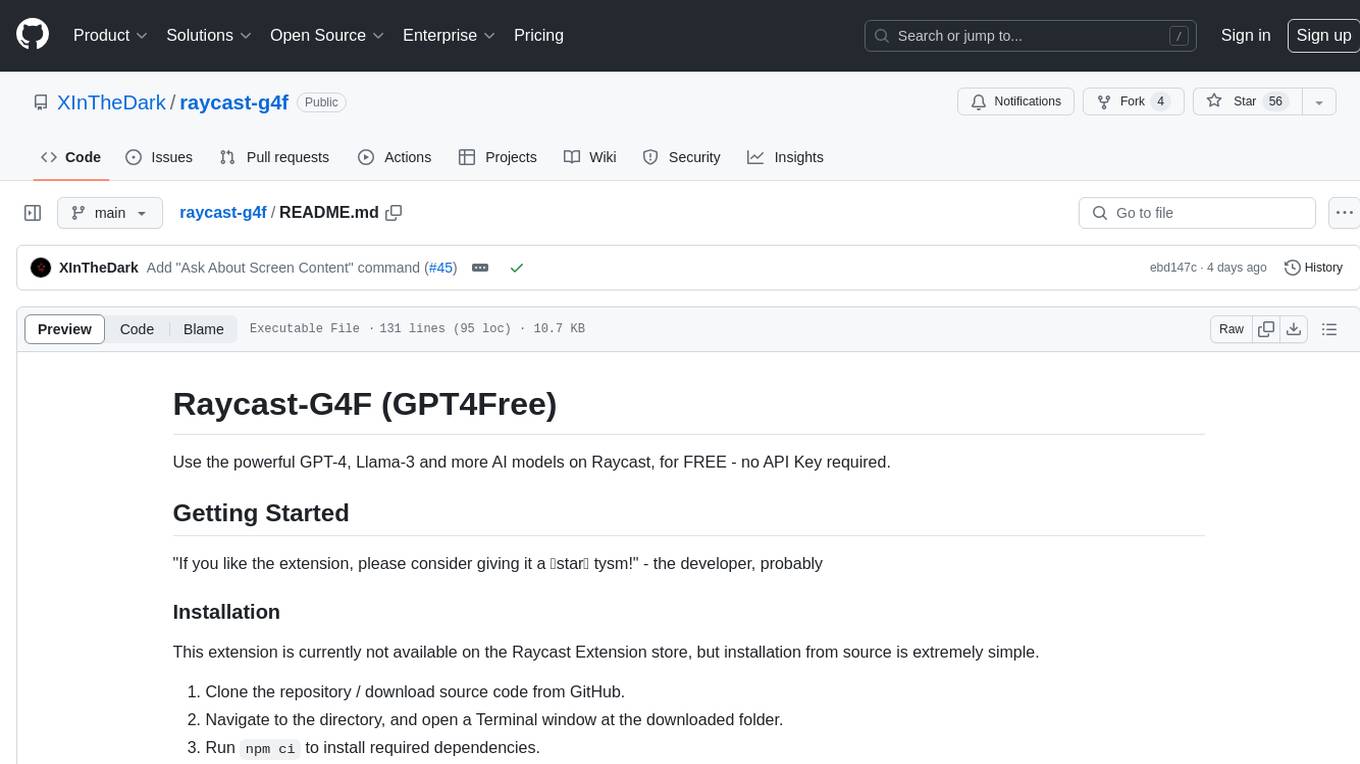
raycast-g4f
Raycast-G4F is a free extension that allows users to leverage powerful AI models such as GPT-4 and Llama-3 within the Raycast app without the need for an API key. The extension offers features like streaming support, diverse commands, chat interaction with AI, web search capabilities, file upload functionality, image generation, and custom AI commands. Users can easily install the extension from the source code and benefit from frequent updates and a user-friendly interface. Raycast-G4F supports various providers and models, each with different capabilities and performance ratings, ensuring a versatile AI experience for users.
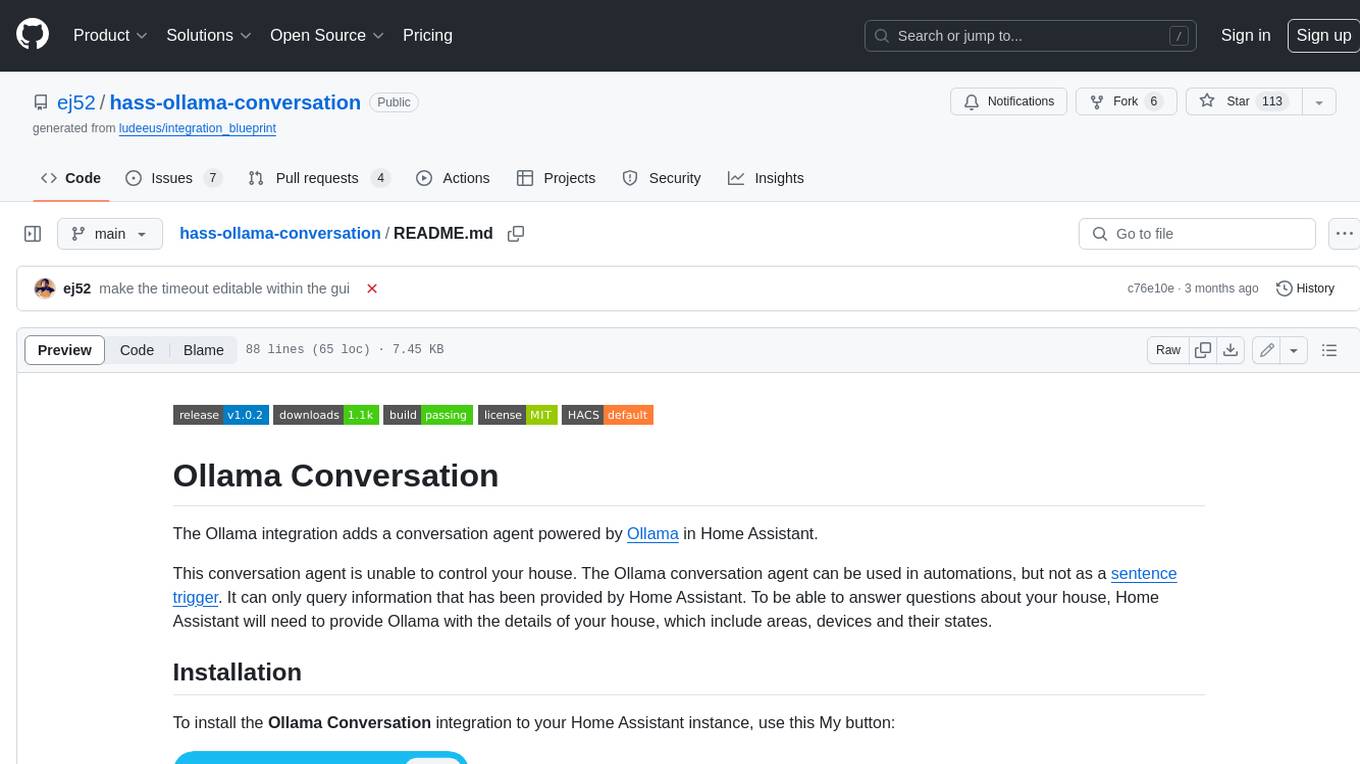
hass-ollama-conversation
The Ollama Conversation integration adds a conversation agent powered by Ollama in Home Assistant. This agent can be used in automations to query information provided by Home Assistant about your house, including areas, devices, and their states. Users can install the integration via HACS and configure settings such as API timeout, model selection, context size, maximum tokens, and other parameters to fine-tune the responses generated by the AI language model. Contributions to the project are welcome, and discussions can be held on the Home Assistant Community platform.
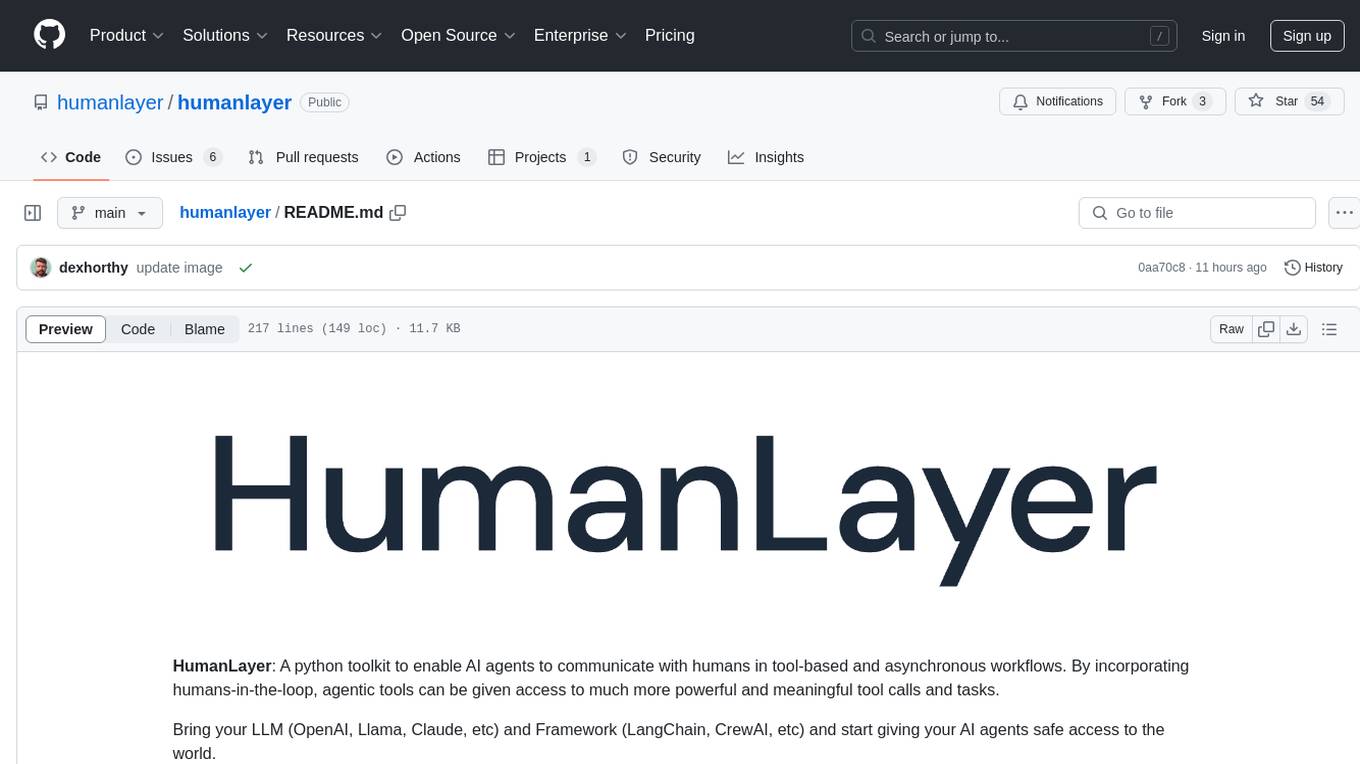
humanlayer
HumanLayer is a Python toolkit designed to enable AI agents to interact with humans in tool-based and asynchronous workflows. By incorporating humans-in-the-loop, agentic tools can access more powerful and meaningful tasks. The toolkit provides features like requiring human approval for function calls, human as a tool for contacting humans, omni-channel contact capabilities, granular routing, and support for various LLMs and orchestration frameworks. HumanLayer aims to ensure human oversight of high-stakes function calls, making AI agents more reliable and safe in executing impactful tasks.
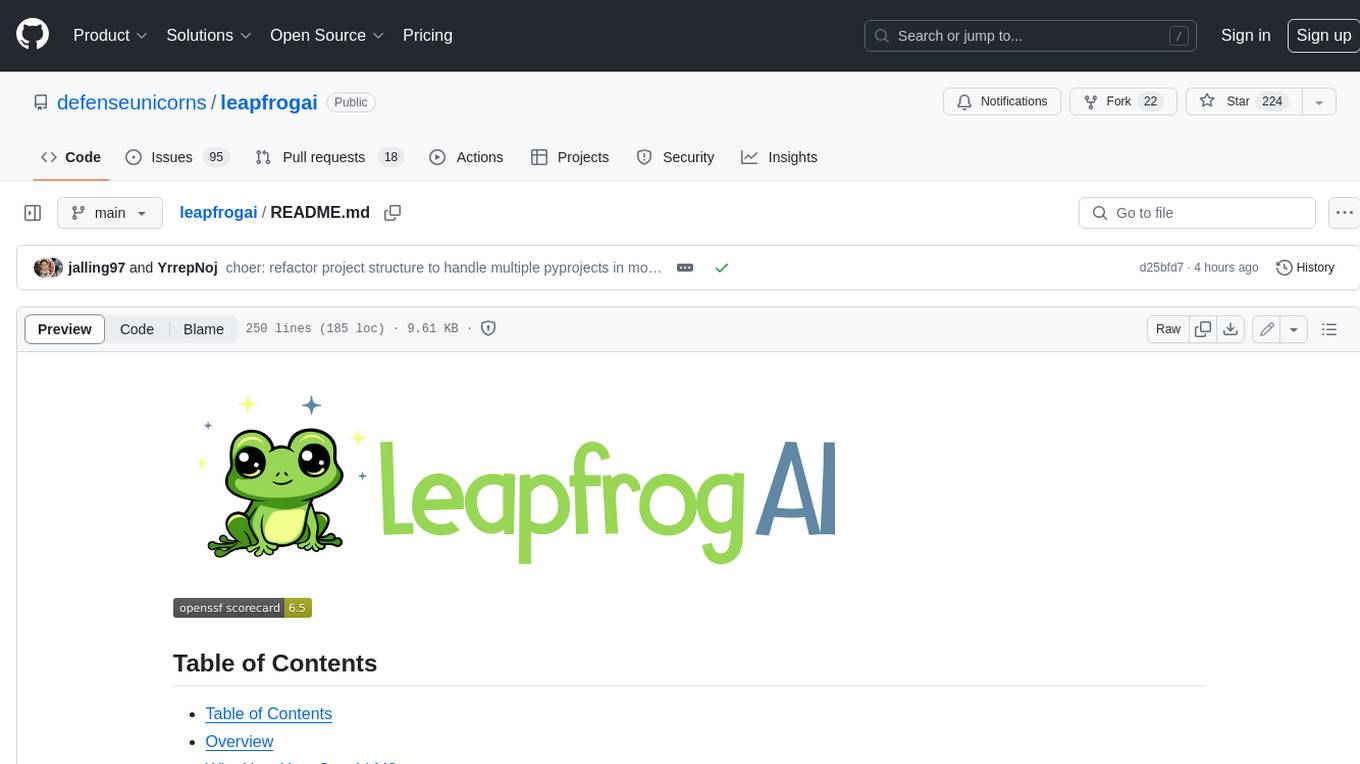
leapfrogai
LeapfrogAI is a self-hosted AI platform designed to be deployed in air-gapped resource-constrained environments. It brings sophisticated AI solutions to these environments by hosting all the necessary components of an AI stack, including vector databases, model backends, API, and UI. LeapfrogAI's API closely matches that of OpenAI, allowing tools built for OpenAI/ChatGPT to function seamlessly with a LeapfrogAI backend. It provides several backends for various use cases, including llama-cpp-python, whisper, text-embeddings, and vllm. LeapfrogAI leverages Chainguard's apko to harden base python images, ensuring the latest supported Python versions are used by the other components of the stack. The LeapfrogAI SDK provides a standard set of protobuffs and python utilities for implementing backends and gRPC. LeapfrogAI offers UI options for common use-cases like chat, summarization, and transcription. It can be deployed and run locally via UDS and Kubernetes, built out using Zarf packages. LeapfrogAI is supported by a community of users and contributors, including Defense Unicorns, Beast Code, Chainguard, Exovera, Hypergiant, Pulze, SOSi, United States Navy, United States Air Force, and United States Space Force.
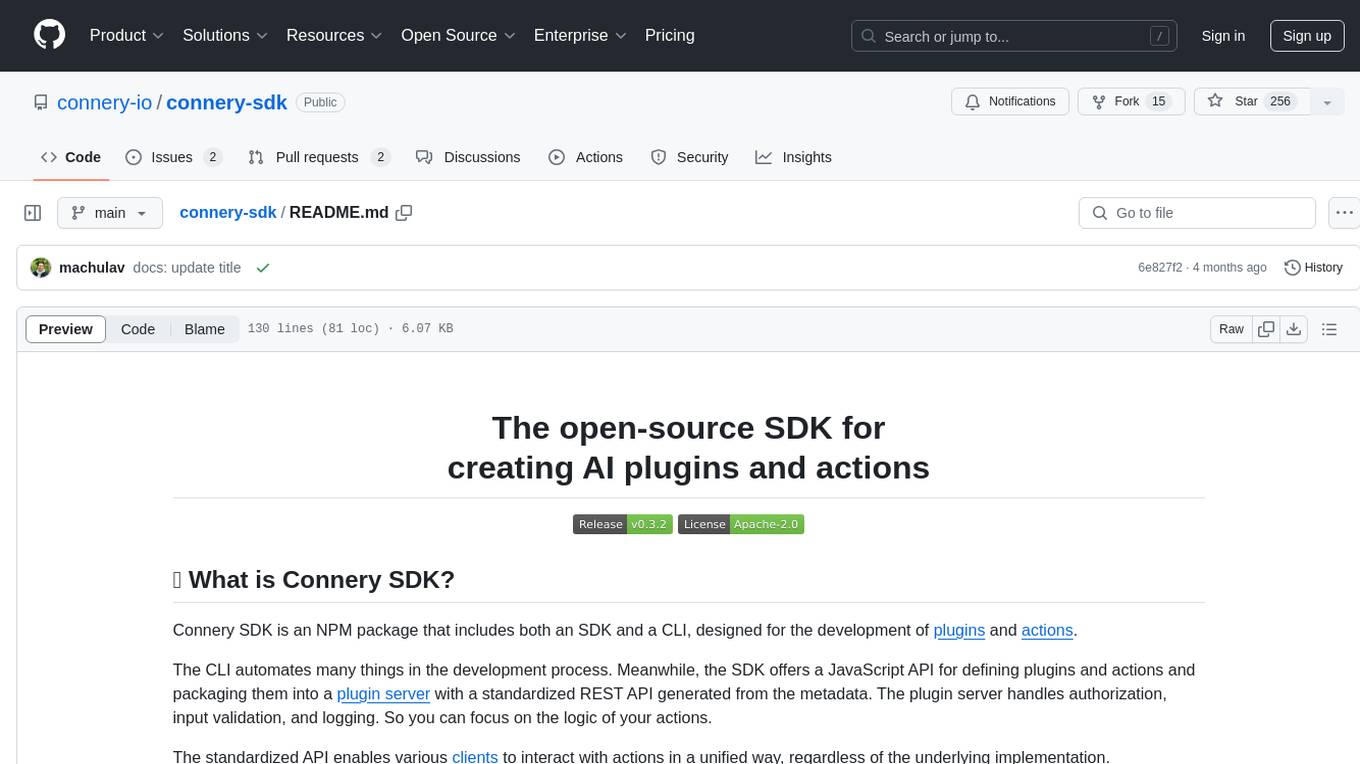
connery-sdk
Connery SDK is an open-source NPM package that provides an SDK and CLI for developing plugins and actions. The SDK offers a JavaScript API to define plugins and actions, which are then packaged into a plugin server with a standardized REST API. This enables automation in the development process and simplifies handling authorization, input validation, and logging. Users can focus on the logic of their actions while the standardized API allows various clients to interact with actions uniformly. Actions can communicate with external APIs, databases, or services, making it versatile for creating AI plugins and actions.
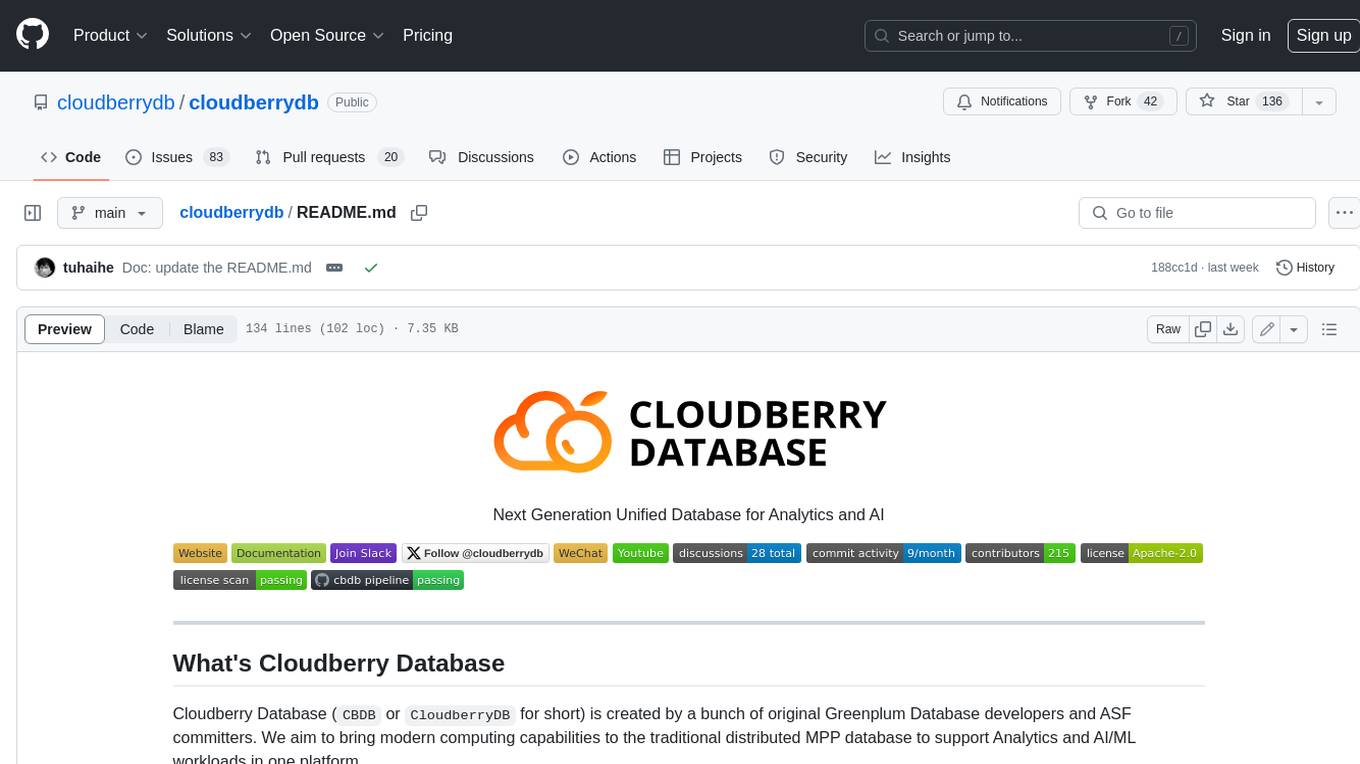
cloudberrydb
Cloudberry Database (CBDB or CloudberryDB) is a next-generation unified database for analytics and AI. It is created by a bunch of original Greenplum Database developers and ASF committers. Cloudberry Database aims to bring modern computing capabilities to the traditional distributed MPP database to support Analytics and AI/ML workloads in one platform.
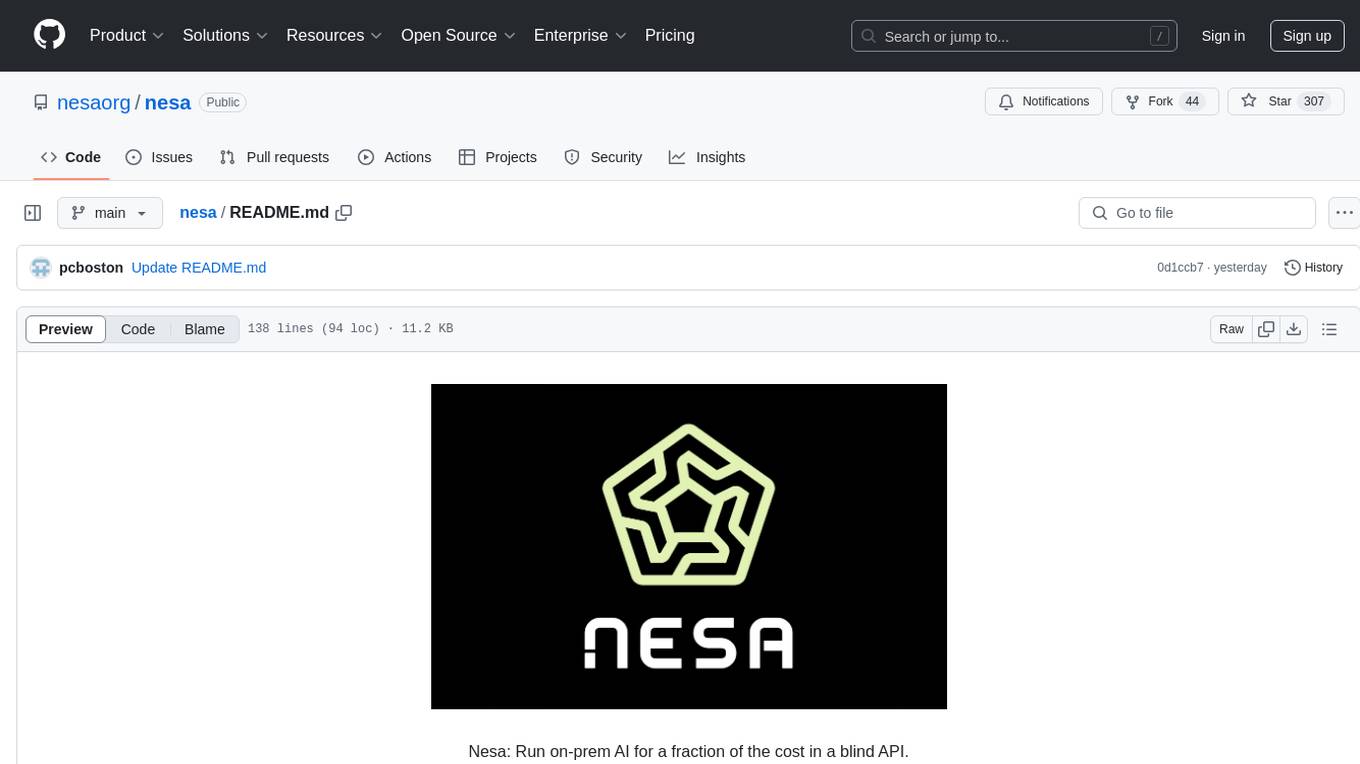
nesa
Nesa is a tool that allows users to run on-prem AI for a fraction of the cost through a blind API. It provides blind privacy, zero latency on protected inference, wide model coverage, cost savings compared to cloud and on-prem AI, RAG support, and ChatGPT compatibility. Nesa achieves blind AI through Equivariant Encryption (EE), a new security technology that provides complete inference encryption with no additional latency. EE allows users to perform inference on neural networks without exposing the underlying data, preserving data privacy and security.
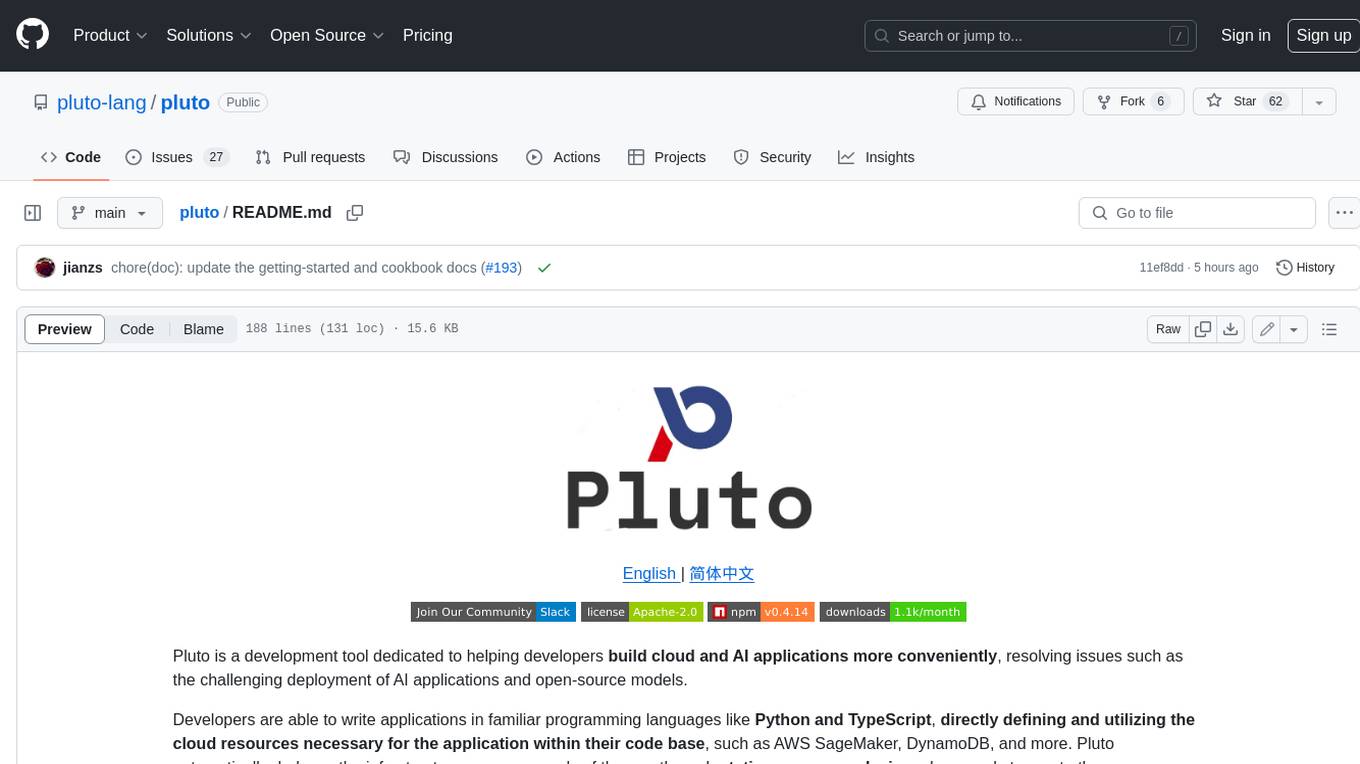
pluto
Pluto is a development tool dedicated to helping developers **build cloud and AI applications more conveniently** , resolving issues such as the challenging deployment of AI applications and open-source models. Developers are able to write applications in familiar programming languages like **Python and TypeScript** , **directly defining and utilizing the cloud resources necessary for the application within their code base** , such as AWS SageMaker, DynamoDB, and more. Pluto automatically deduces the infrastructure resource needs of the app through **static program analysis** and proceeds to create these resources on the specified cloud platform, **simplifying the resources creation and application deployment process**.
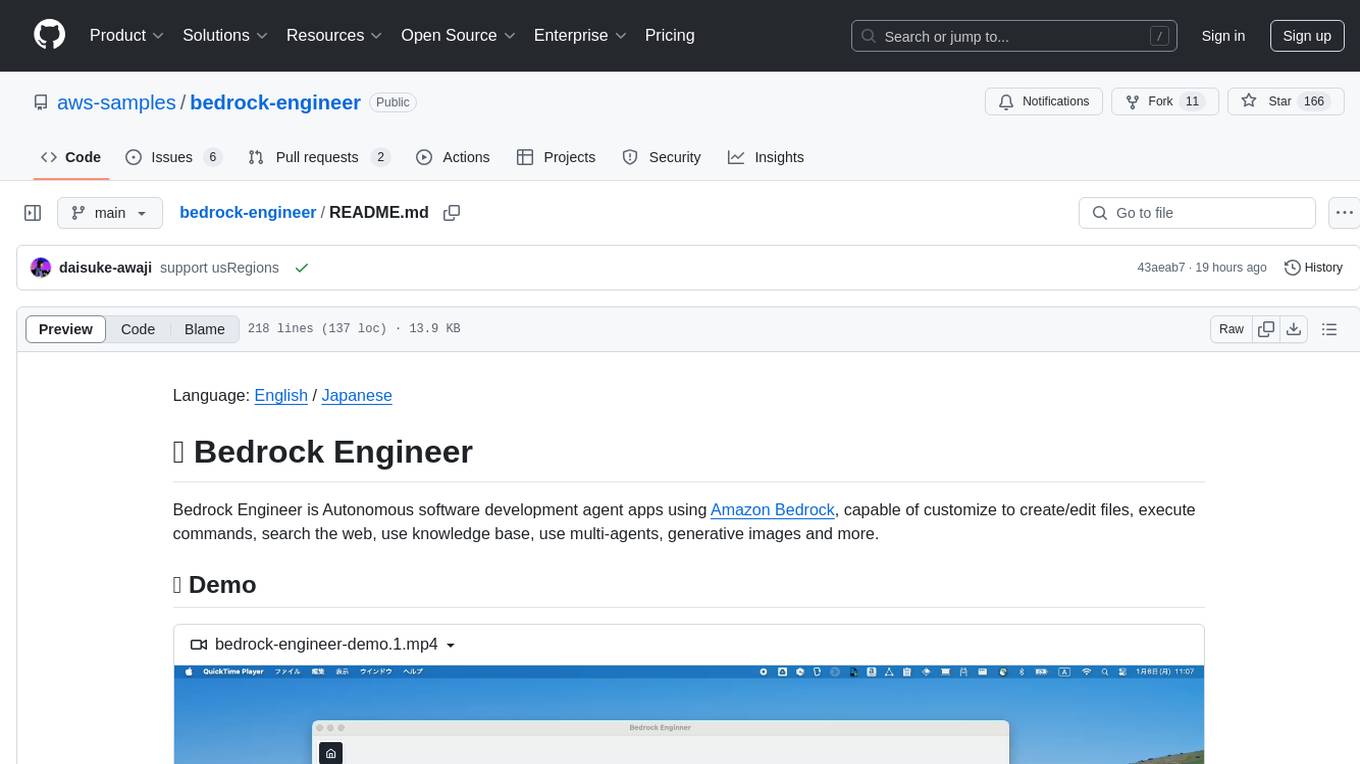
bedrock-engineer
Bedrock Engineer is an autonomous software development agent application that utilizes Amazon Bedrock. It allows users to customize, create/edit files, execute commands, search the web, use a knowledge base, utilize multi-agents, generate images, and more. The tool provides an interactive chat interface with AI agents, file system operations, web search capabilities, project structure management, code analysis, code generation, data analysis, agent and tool customization, chat history management, and multi-language support. Users can select and customize agents, choose from various tools like file system operations, web search, Amazon Bedrock integration, and system command execution. Additionally, the tool offers features for website generation, connecting to design system data sources, AWS Step Functions ASL definition generation, diagram creation using natural language descriptions, and multi-language support.
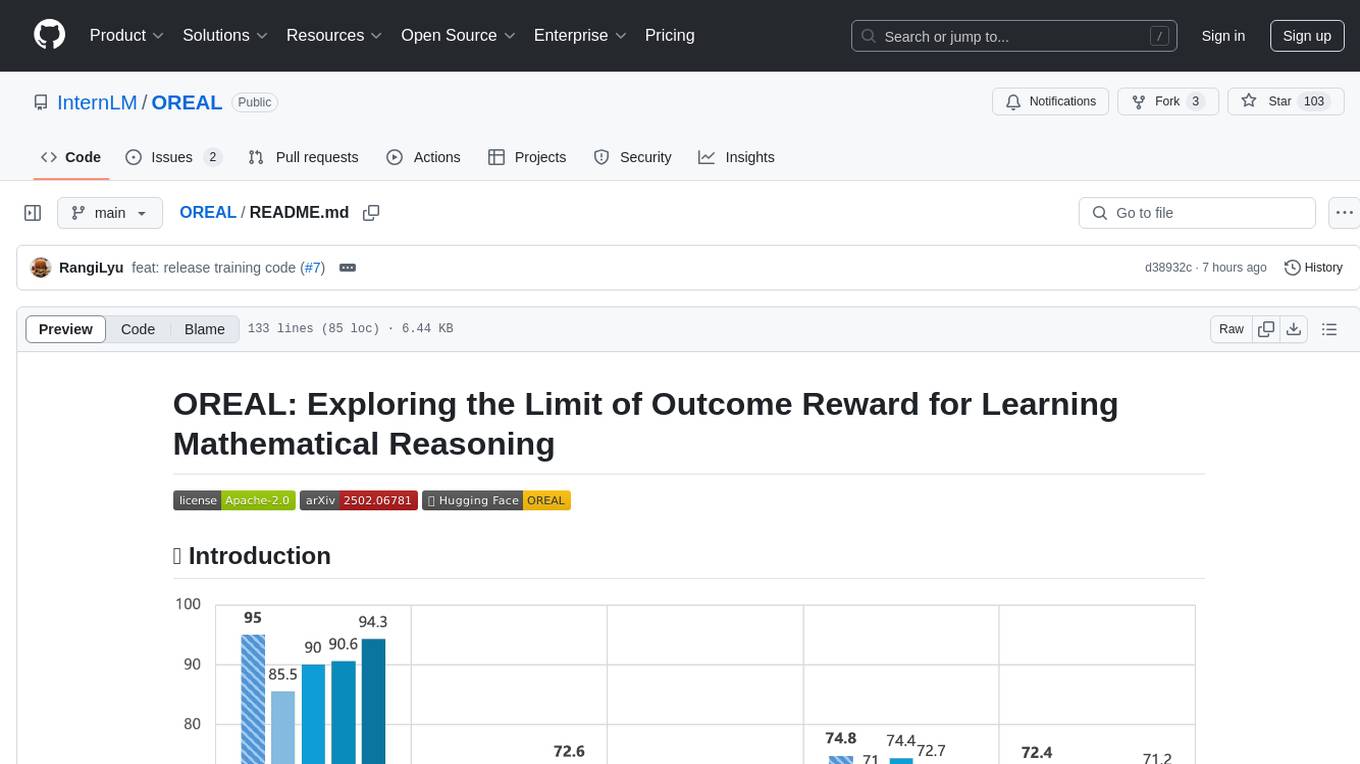
OREAL
OREAL is a reinforcement learning framework designed for mathematical reasoning tasks, aiming to achieve optimal performance through outcome reward-based learning. The framework utilizes behavior cloning, reshaping rewards, and token-level reward models to address challenges in sparse rewards and partial correctness. OREAL has achieved significant results, with a 7B model reaching 94.0 pass@1 accuracy on MATH-500 and surpassing previous 32B models. The tool provides training tutorials and Hugging Face model repositories for easy access and implementation.
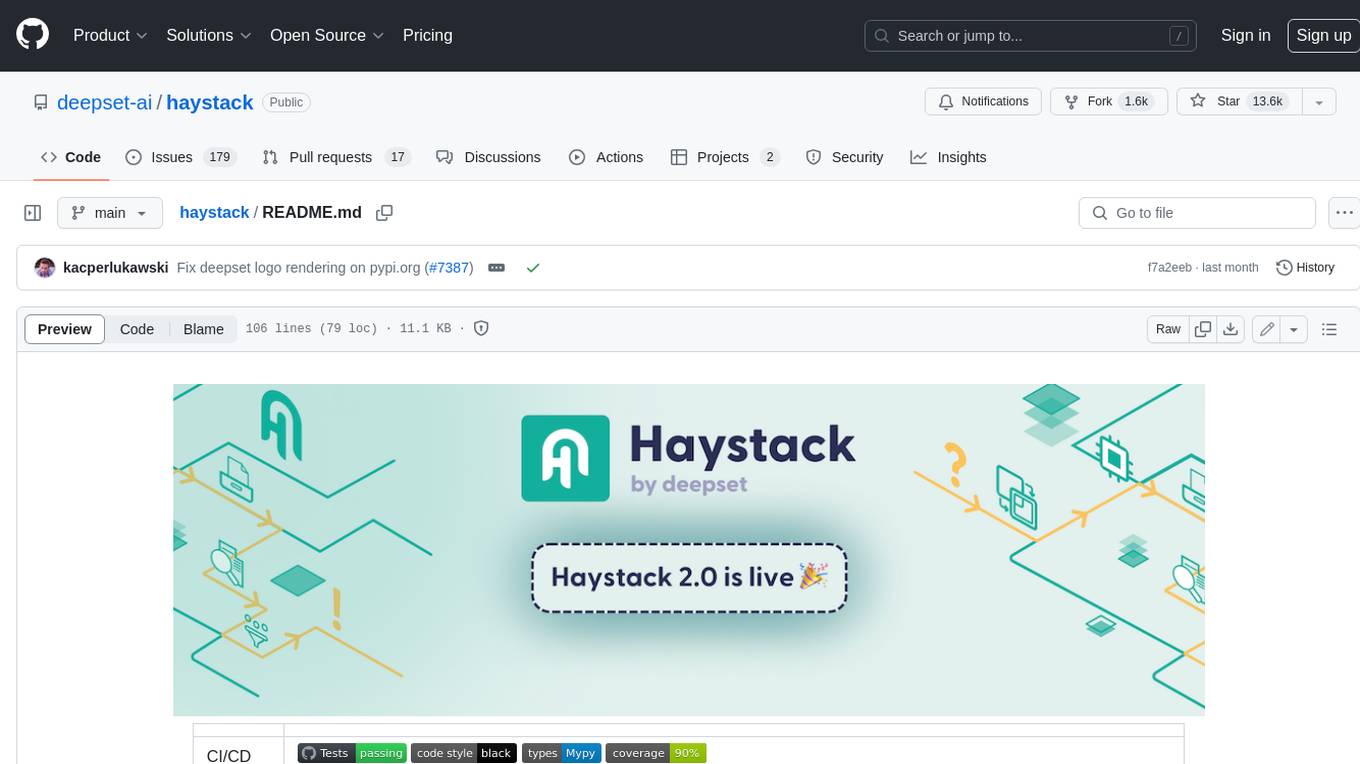
haystack
Haystack is an end-to-end LLM framework that allows you to build applications powered by LLMs, Transformer models, vector search and more. Whether you want to perform retrieval-augmented generation (RAG), document search, question answering or answer generation, Haystack can orchestrate state-of-the-art embedding models and LLMs into pipelines to build end-to-end NLP applications and solve your use case.
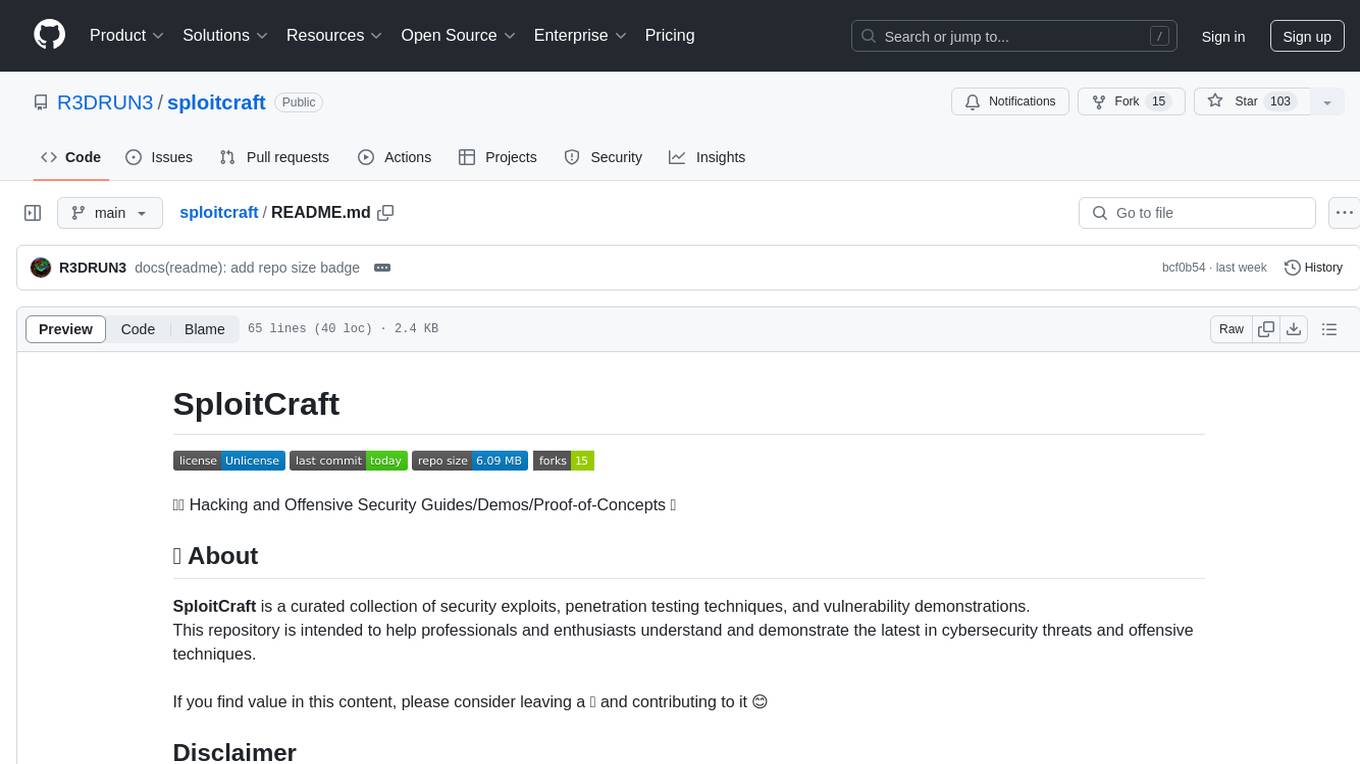
sploitcraft
SploitCraft is a curated collection of security exploits, penetration testing techniques, and vulnerability demonstrations intended to help professionals and enthusiasts understand and demonstrate the latest in cybersecurity threats and offensive techniques. The repository is organized into folders based on specific topics, each containing directories and detailed READMEs with step-by-step instructions. Contributions from the community are welcome, with a focus on adding new proof of concepts or expanding existing ones while adhering to the current structure and format of the repository.
For similar tasks
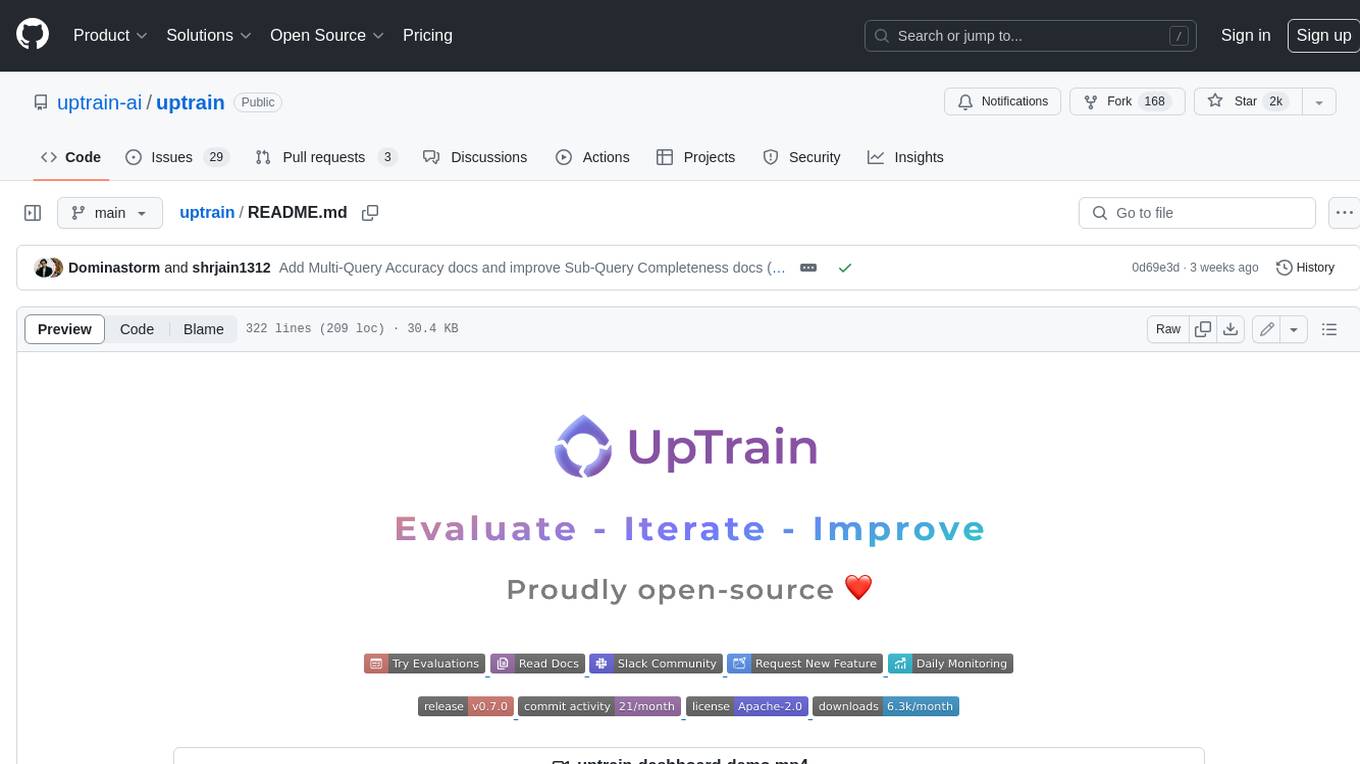
uptrain
UpTrain is an open-source unified platform to evaluate and improve Generative AI applications. We provide grades for 20+ preconfigured evaluations (covering language, code, embedding use cases), perform root cause analysis on failure cases and give insights on how to resolve them.
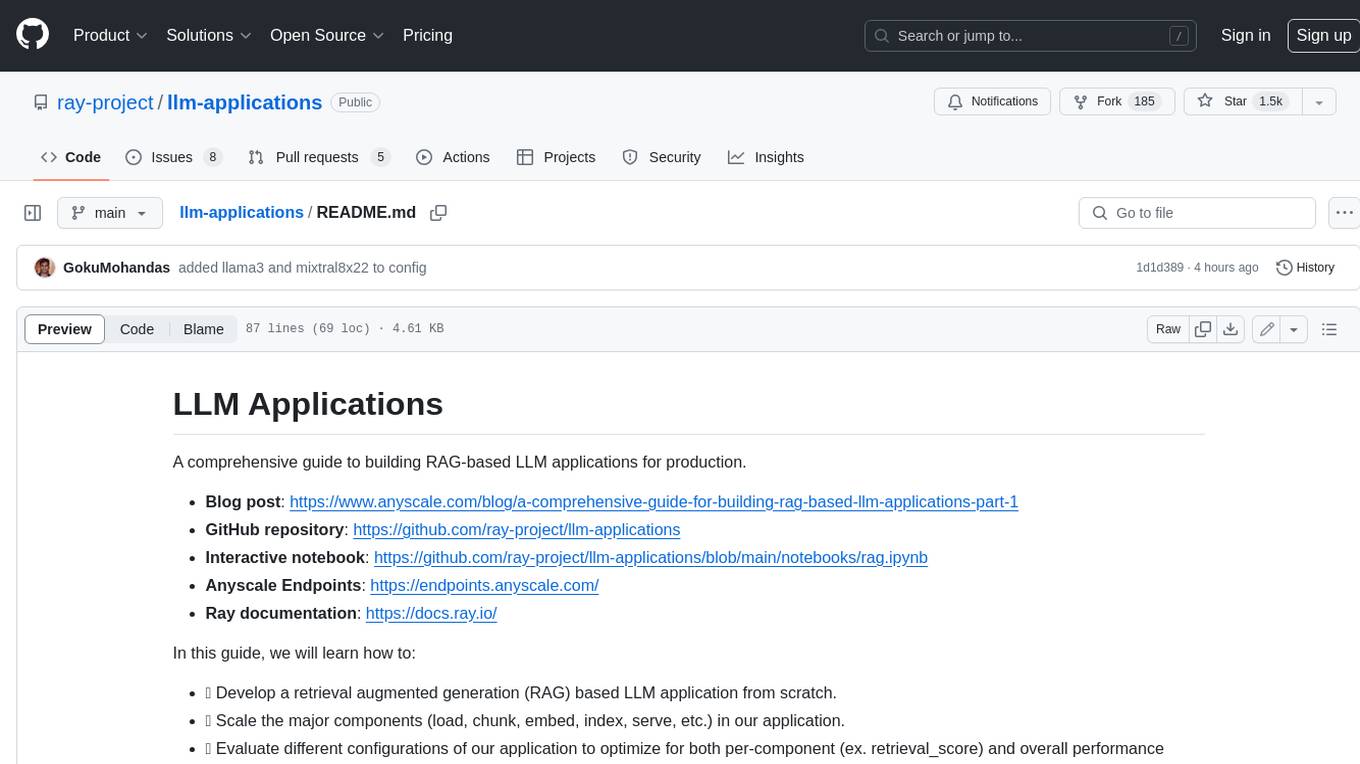
llm-applications
A comprehensive guide to building Retrieval Augmented Generation (RAG)-based LLM applications for production. This guide covers developing a RAG-based LLM application from scratch, scaling the major components, evaluating different configurations, implementing LLM hybrid routing, serving the application in a highly scalable and available manner, and sharing the impacts LLM applications have had on products.
For similar jobs
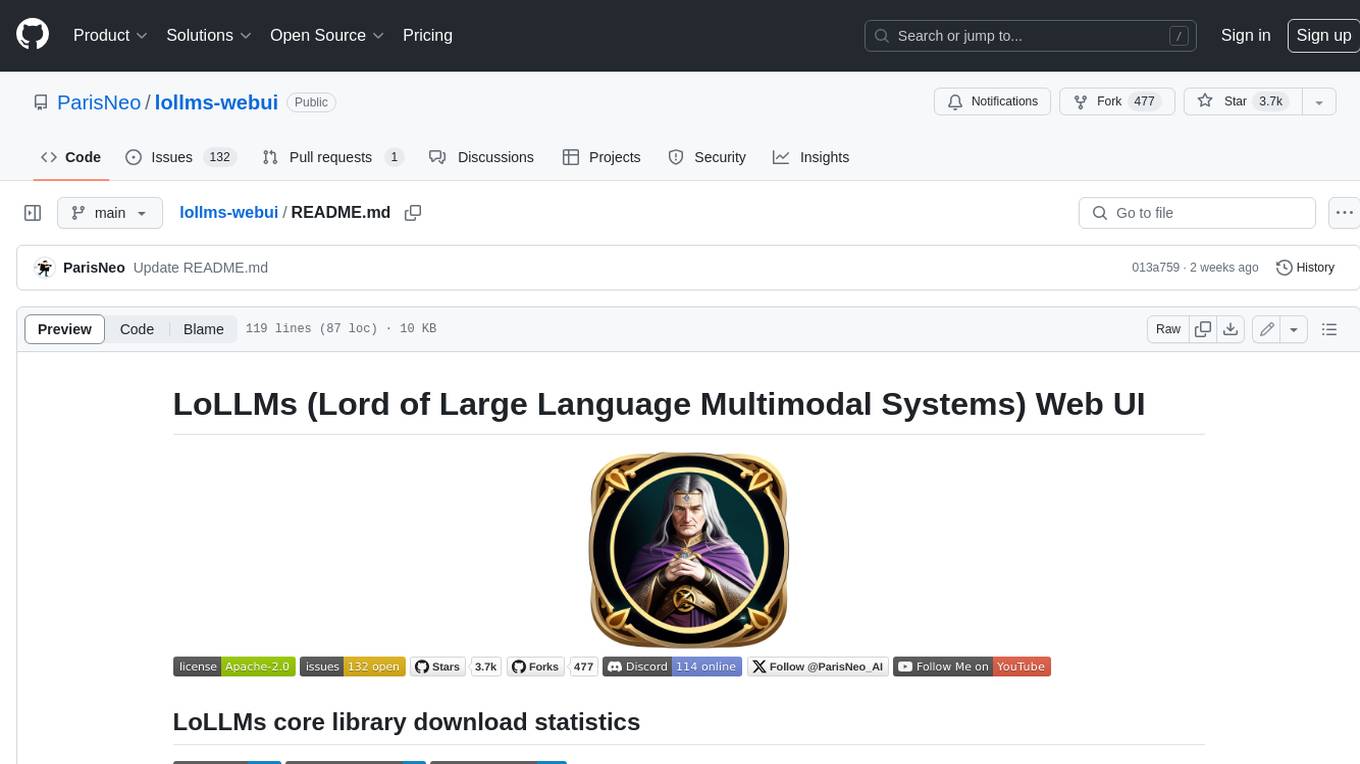
lollms-webui
LoLLMs WebUI (Lord of Large Language Multimodal Systems: One tool to rule them all) is a user-friendly interface to access and utilize various LLM (Large Language Models) and other AI models for a wide range of tasks. With over 500 AI expert conditionings across diverse domains and more than 2500 fine tuned models over multiple domains, LoLLMs WebUI provides an immediate resource for any problem, from car repair to coding assistance, legal matters, medical diagnosis, entertainment, and more. The easy-to-use UI with light and dark mode options, integration with GitHub repository, support for different personalities, and features like thumb up/down rating, copy, edit, and remove messages, local database storage, search, export, and delete multiple discussions, make LoLLMs WebUI a powerful and versatile tool.
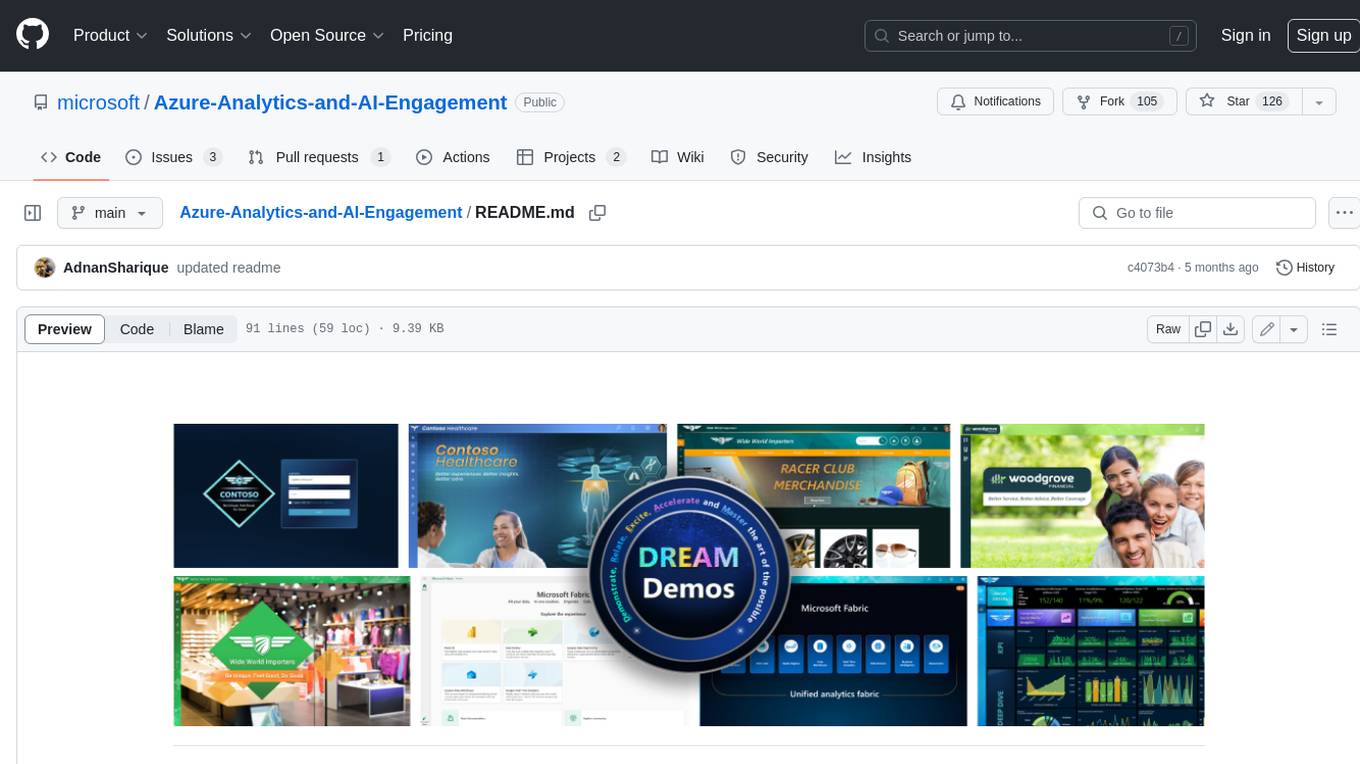
Azure-Analytics-and-AI-Engagement
The Azure-Analytics-and-AI-Engagement repository provides packaged Industry Scenario DREAM Demos with ARM templates (Containing a demo web application, Power BI reports, Synapse resources, AML Notebooks etc.) that can be deployed in a customerβs subscription using the CAPE tool within a matter of few hours. Partners can also deploy DREAM Demos in their own subscriptions using DPoC.
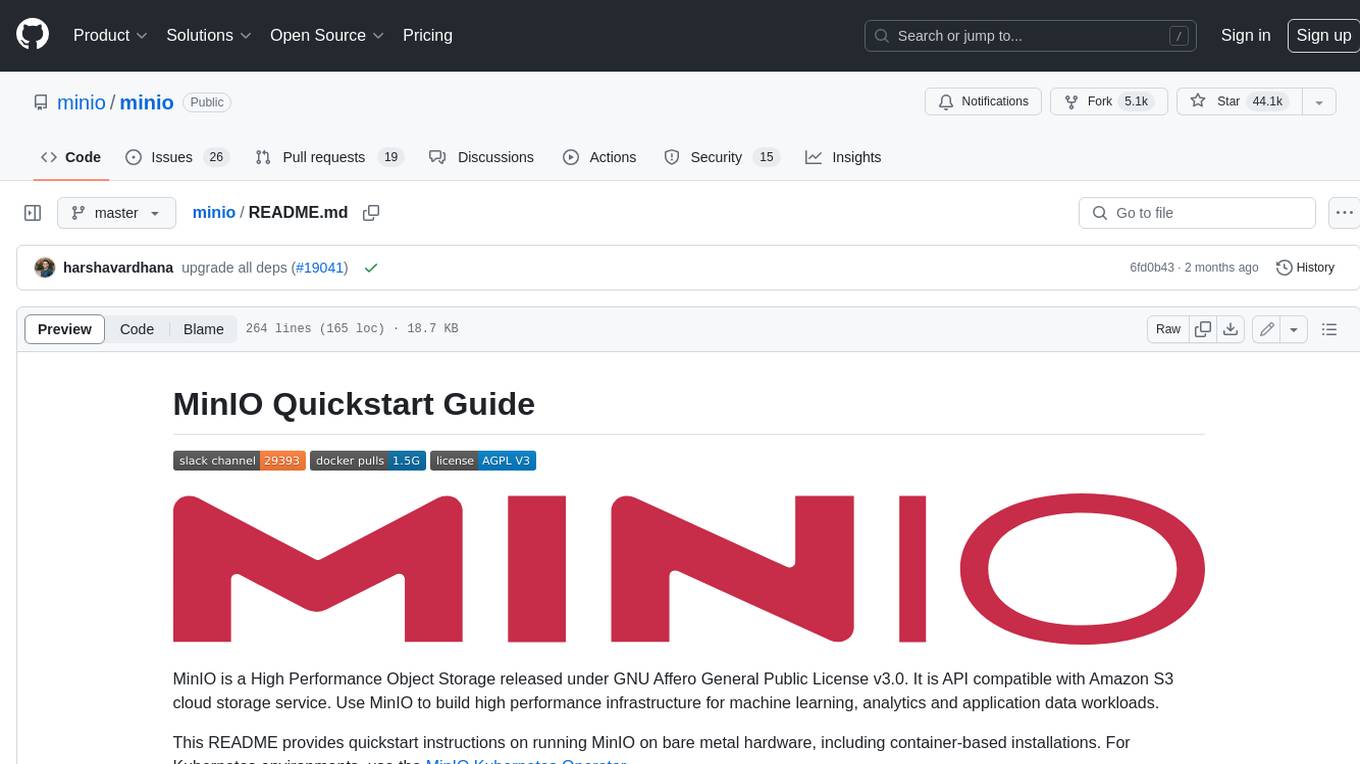
minio
MinIO is a High Performance Object Storage released under GNU Affero General Public License v3.0. It is API compatible with Amazon S3 cloud storage service. Use MinIO to build high performance infrastructure for machine learning, analytics and application data workloads.
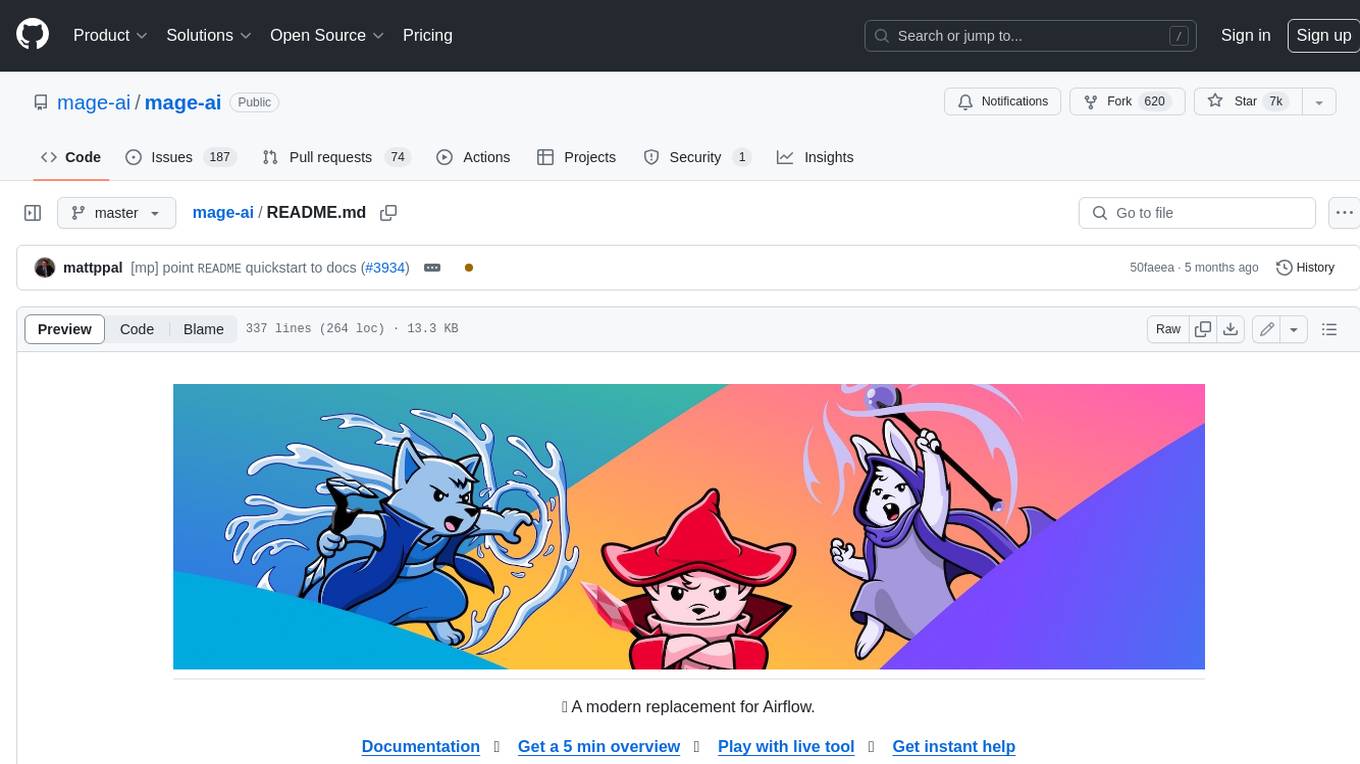
mage-ai
Mage is an open-source data pipeline tool for transforming and integrating data. It offers an easy developer experience, engineering best practices built-in, and data as a first-class citizen. Mage makes it easy to build, preview, and launch data pipelines, and provides observability and scaling capabilities. It supports data integrations, streaming pipelines, and dbt integration.
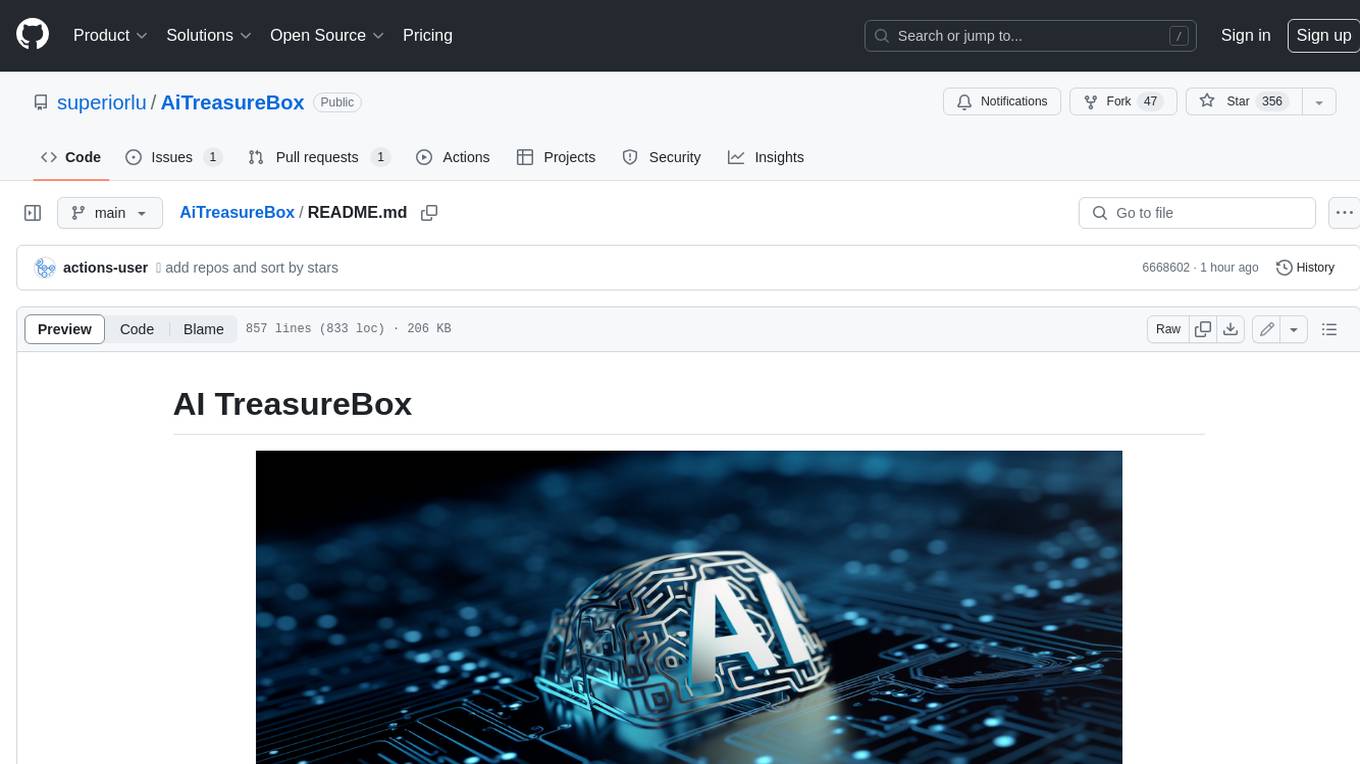
AiTreasureBox
AiTreasureBox is a versatile AI tool that provides a collection of pre-trained models and algorithms for various machine learning tasks. It simplifies the process of implementing AI solutions by offering ready-to-use components that can be easily integrated into projects. With AiTreasureBox, users can quickly prototype and deploy AI applications without the need for extensive knowledge in machine learning or deep learning. The tool covers a wide range of tasks such as image classification, text generation, sentiment analysis, object detection, and more. It is designed to be user-friendly and accessible to both beginners and experienced developers, making AI development more efficient and accessible to a wider audience.
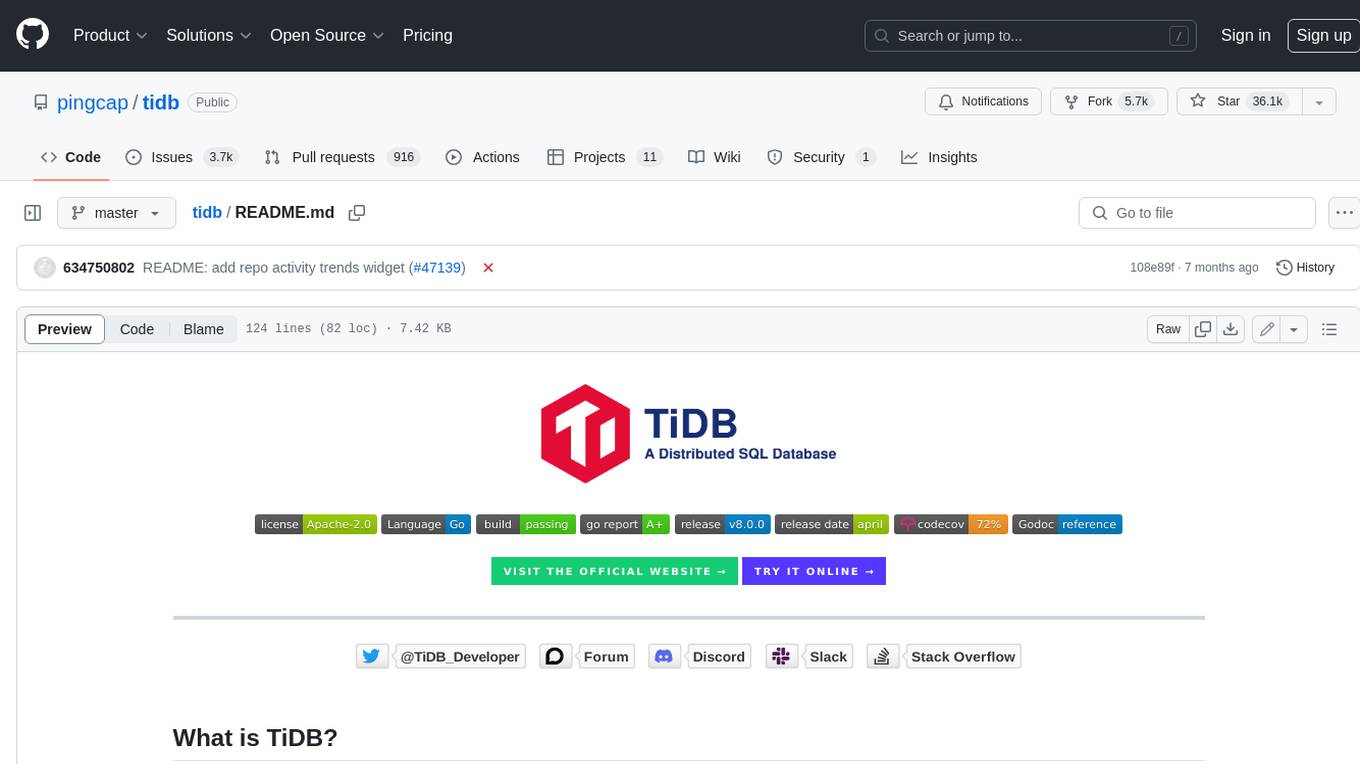
tidb
TiDB is an open-source distributed SQL database that supports Hybrid Transactional and Analytical Processing (HTAP) workloads. It is MySQL compatible and features horizontal scalability, strong consistency, and high availability.
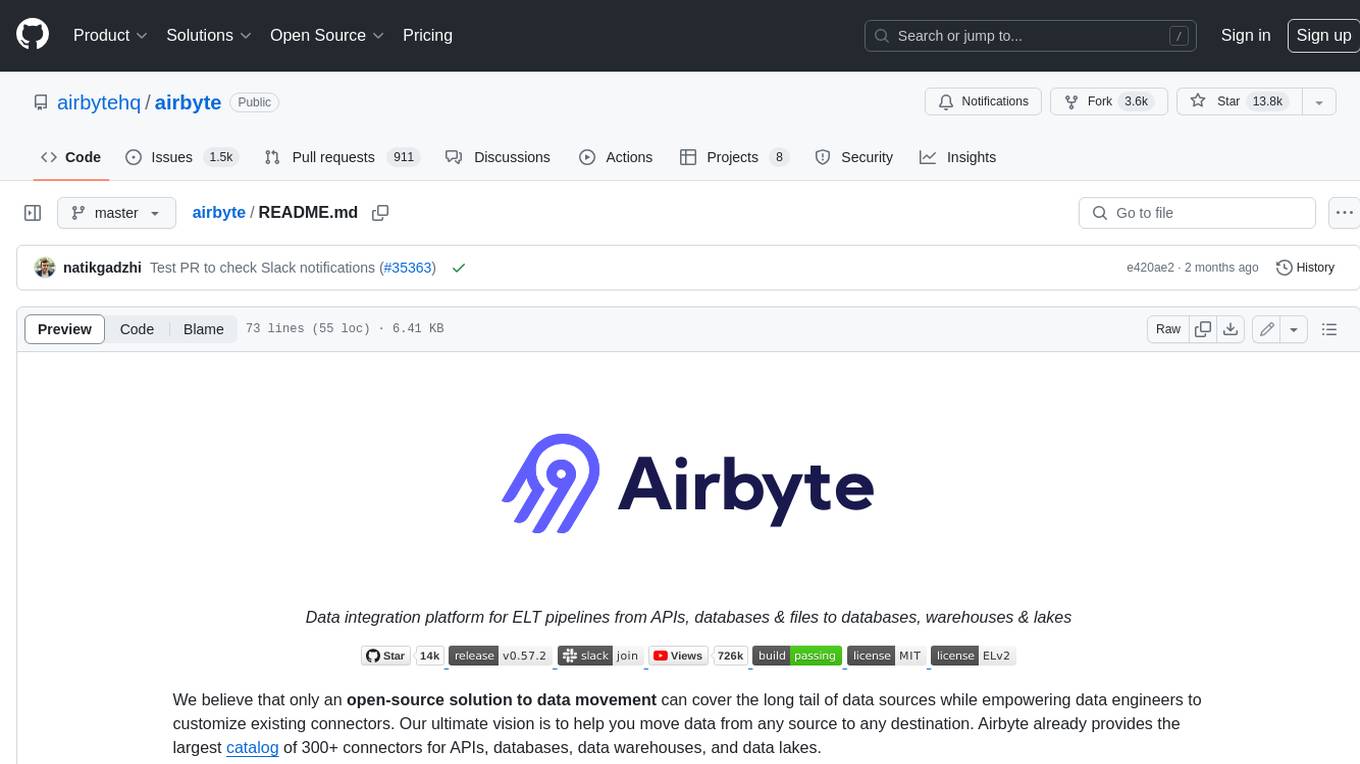
airbyte
Airbyte is an open-source data integration platform that makes it easy to move data from any source to any destination. With Airbyte, you can build and manage data pipelines without writing any code. Airbyte provides a library of pre-built connectors that make it easy to connect to popular data sources and destinations. You can also create your own connectors using Airbyte's no-code Connector Builder or low-code CDK. Airbyte is used by data engineers and analysts at companies of all sizes to build and manage their data pipelines.
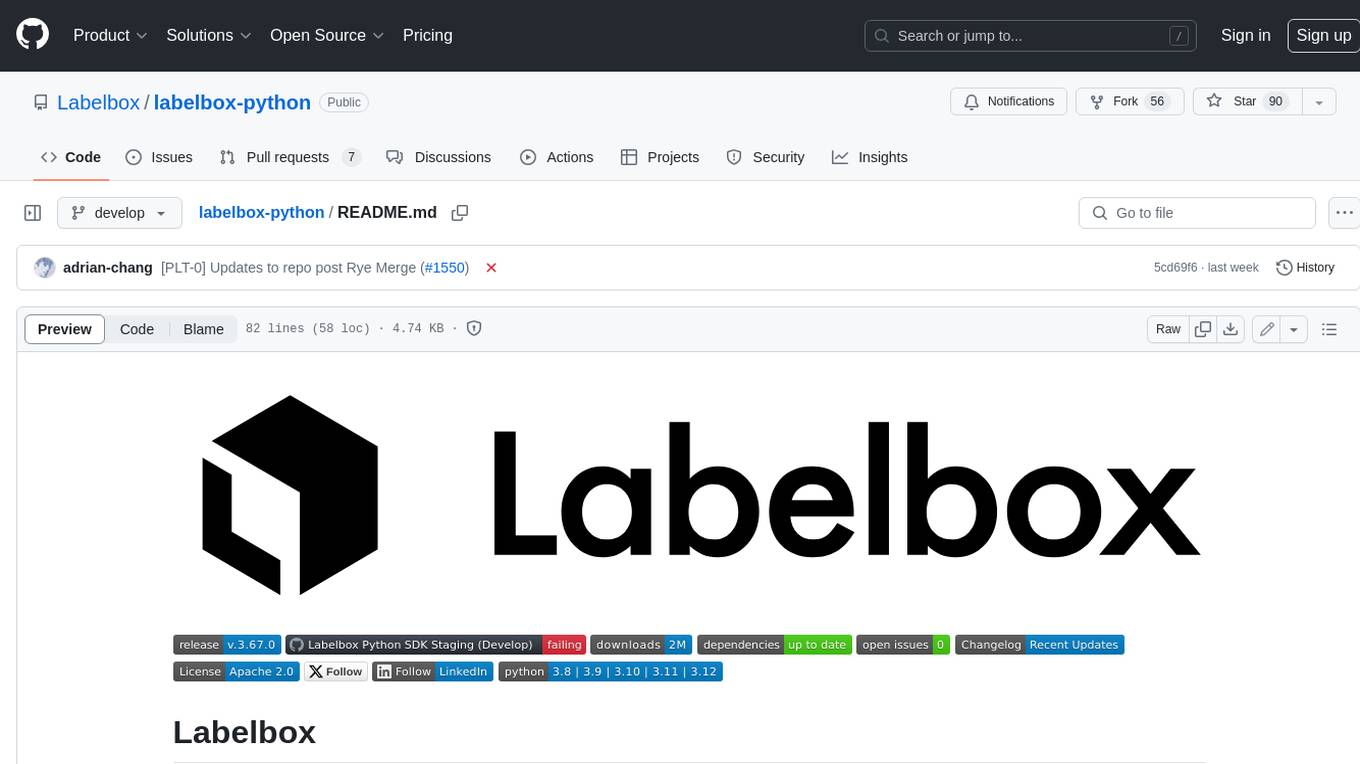
labelbox-python
Labelbox is a data-centric AI platform for enterprises to develop, optimize, and use AI to solve problems and power new products and services. Enterprises use Labelbox to curate data, generate high-quality human feedback data for computer vision and LLMs, evaluate model performance, and automate tasks by combining AI and human-centric workflows. The academic & research community uses Labelbox for cutting-edge AI research.