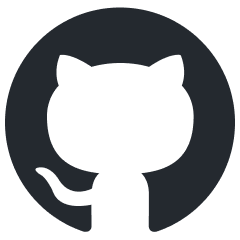
ludwig
Low-code framework for building custom LLMs, neural networks, and other AI models
Stars: 11034
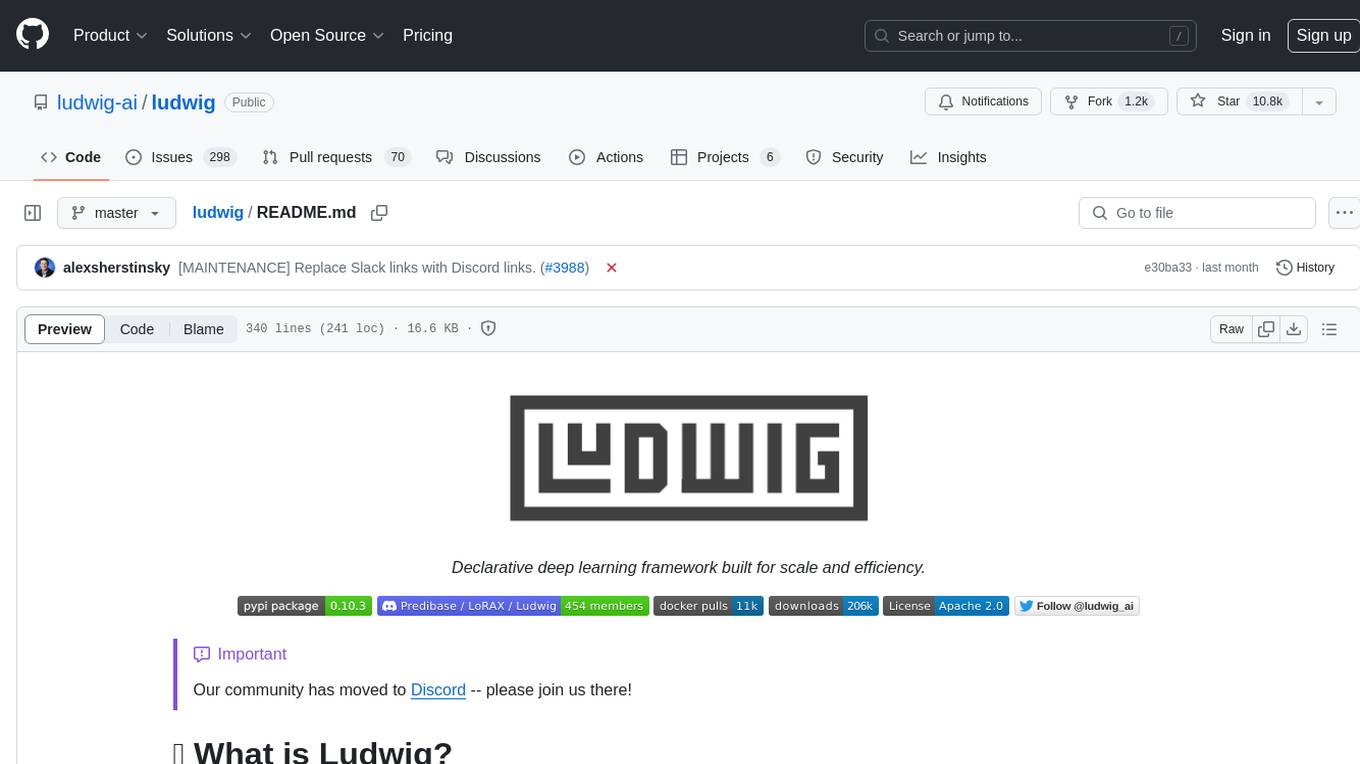
Ludwig is a declarative deep learning framework designed for scale and efficiency. It is a low-code framework that allows users to build custom AI models like LLMs and other deep neural networks with ease. Ludwig offers features such as optimized scale and efficiency, expert level control, modularity, and extensibility. It is engineered for production with prebuilt Docker containers, support for running with Ray on Kubernetes, and the ability to export models to Torchscript and Triton. Ludwig is hosted by the Linux Foundation AI & Data.
README:
[!IMPORTANT] Our community has moved to Discord -- please join us there!
Ludwig is a low-code framework for building custom AI models like LLMs and other deep neural networks.
Key features:
- π Build custom models with ease: a declarative YAML configuration file is all you need to train a state-of-the-art LLM on your data. Support for multi-task and multi-modality learning. Comprehensive config validation detects invalid parameter combinations and prevents runtime failures.
- β‘ Optimized for scale and efficiency: automatic batch size selection, distributed training (DDP, DeepSpeed), parameter efficient fine-tuning (PEFT), 4-bit quantization (QLoRA), paged and 8-bit optimizers, and larger-than-memory datasets.
- π Expert level control: retain full control of your models down to the activation functions. Support for hyperparameter optimization, explainability, and rich metric visualizations.
- π§± Modular and extensible: experiment with different model architectures, tasks, features, and modalities with just a few parameter changes in the config. Think building blocks for deep learning.
- π’ Engineered for production: prebuilt Docker containers, native support for running with Ray on Kubernetes, export models to Torchscript and Triton, upload to HuggingFace with one command.
Ludwig is hosted by the Linux Foundation AI & Data.
Install from PyPi. Be aware that Ludwig requires Python 3.8+.
pip install ludwig
Or install with all optional dependencies:
pip install ludwig[full]
Please see contributing for more detailed installation instructions.
Want to take a quick peak at some of the Ludwig 0.8 features? Check out this Colab Notebook π
Looking to fine-tune Llama-2 or Mistral? Check out these notebooks:
For a full tutorial, check out the official getting started guide, or take a look at end-to-end Examples.
Let's fine-tune a pretrained LLaMA-2-7b large language model to follow instructions like a chatbot ("instruction tuning").
- HuggingFace API Token
- Access approval to Llama2-7b-hf
- GPU with at least 12 GiB of VRAM (in our tests, we used an Nvidia T4)
We'll use the Stanford Alpaca dataset, which will be formatted as a table-like file that looks like this:
instruction | input | output |
---|---|---|
Give three tips for staying healthy. | 1.Eat a balanced diet and make sure to include... | |
Arrange the items given below in the order to ... | cake, me, eating | I eating cake. |
Write an introductory paragraph about a famous... | Michelle Obama | Michelle Obama is an inspirational woman who r... |
... | ... | ... |
Create a YAML config file named model.yaml
with the following:
model_type: llm
base_model: meta-llama/Llama-2-7b-hf
quantization:
bits: 4
adapter:
type: lora
prompt:
template: |
Below is an instruction that describes a task, paired with an input that may provide further context.
Write a response that appropriately completes the request.
### Instruction:
{instruction}
### Input:
{input}
### Response:
input_features:
- name: prompt
type: text
output_features:
- name: output
type: text
trainer:
type: finetune
learning_rate: 0.0001
batch_size: 1
gradient_accumulation_steps: 16
epochs: 3
learning_rate_scheduler:
decay: cosine
warmup_fraction: 0.01
preprocessing:
sample_ratio: 0.1
backend:
type: local
And now let's train the model:
export HUGGING_FACE_HUB_TOKEN = "<api_token>"
ludwig train --config model.yaml --dataset "ludwig://alpaca"
Let's build a neural network that predicts whether a given movie critic's review on Rotten Tomatoes was positive or negative.
Our dataset will be a CSV file that looks like this:
movie_title | content_rating | genres | runtime | top_critic | review_content | recommended |
---|---|---|---|---|---|---|
Deliver Us from Evil | R | Action & Adventure, Horror | 117.0 | TRUE | Director Scott Derrickson and his co-writer, Paul Harris Boardman, deliver a routine procedural with unremarkable frights. | 0 |
Barbara | PG-13 | Art House & International, Drama | 105.0 | FALSE | Somehow, in this stirring narrative, Barbara manages to keep hold of her principles, and her humanity and courage, and battles to save a dissident teenage girl whose life the Communists are trying to destroy. | 1 |
Horrible Bosses | R | Comedy | 98.0 | FALSE | These bosses cannot justify either murder or lasting comic memories, fatally compromising a farce that could have been great but ends up merely mediocre. | 0 |
... | ... | ... | ... | ... | ... | ... |
Download a sample of the dataset from here.
wget https://ludwig.ai/latest/data/rotten_tomatoes.csv
Next create a YAML config file named model.yaml
with the following:
input_features:
- name: genres
type: set
preprocessing:
tokenizer: comma
- name: content_rating
type: category
- name: top_critic
type: binary
- name: runtime
type: number
- name: review_content
type: text
encoder:
type: embed
output_features:
- name: recommended
type: binary
That's it! Now let's train the model:
ludwig train --config model.yaml --dataset rotten_tomatoes.csv
Happy modeling
Try applying Ludwig to your data. Reach out on Discord if you have any questions.
-
Minimal machine learning boilerplate
Ludwig takes care of the engineering complexity of machine learning out of the box, enabling research scientists to focus on building models at the highest level of abstraction. Data preprocessing, hyperparameter optimization, device management, and distributed training for
torch.nn.Module
models come completely free. -
Easily build your benchmarks
Creating a state-of-the-art baseline and comparing it with a new model is a simple config change.
-
Easily apply new architectures to multiple problems and datasets
Apply new models across the extensive set of tasks and datasets that Ludwig supports. Ludwig includes a full benchmarking toolkit accessible to any user, for running experiments with multiple models across multiple datasets with just a simple configuration.
-
Highly configurable data preprocessing, modeling, and metrics
Any and all aspects of the model architecture, training loop, hyperparameter search, and backend infrastructure can be modified as additional fields in the declarative configuration to customize the pipeline to meet your requirements. For details on what can be configured, check out Ludwig Configuration docs.
-
Multi-modal, multi-task learning out-of-the-box
Mix and match tabular data, text, images, and even audio into complex model configurations without writing code.
-
Rich model exporting and tracking
Automatically track all trials and metrics with tools like Tensorboard, Comet ML, Weights & Biases, MLFlow, and Aim Stack.
-
Automatically scale training to multi-GPU, multi-node clusters
Go from training on your local machine to the cloud without code changes.
-
Low-code interface for state-of-the-art models, including pre-trained Huggingface Transformers
Ludwig also natively integrates with pre-trained models, such as the ones available in Huggingface Transformers. Users can choose from a vast collection of state-of-the-art pre-trained PyTorch models to use without needing to write any code at all. For example, training a BERT-based sentiment analysis model with Ludwig is as simple as:
ludwig train --dataset sst5 --config_str "{input_features: [{name: sentence, type: text, encoder: bert}], output_features: [{name: label, type: category}]}"
-
Low-code interface for AutoML
Ludwig AutoML allows users to obtain trained models by providing just a dataset, the target column, and a time budget.
auto_train_results = ludwig.automl.auto_train(dataset=my_dataset_df, target=target_column_name, time_limit_s=7200)
-
Easy productionisation
Ludwig makes it easy to serve deep learning models, including on GPUs. Launch a REST API for your trained Ludwig model.
ludwig serve --model_path=/path/to/model
Ludwig supports exporting models to efficient Torchscript bundles.
ludwig export_torchscript -βmodel_path=/path/to/model
- Named Entity Recognition Tagging
- Natural Language Understanding
- Machine Translation
- Chit-Chat Dialogue Modeling through seq2seq
- Sentiment Analysis
- One-shot Learning with Siamese Networks
- Visual Question Answering
- Spoken Digit Speech Recognition
- Speaker Verification
- Binary Classification (Titanic)
- Timeseries forecasting
- Timeseries forecasting (Weather)
- Movie rating prediction
- Multi-label classification
- Multi-Task Learning
- Simple Regression: Fuel Efficiency Prediction
- Fraud Detection
Read our publications on Ludwig, declarative ML, and Ludwigβs SoTA benchmarks.
Learn more about how Ludwig works, how to get started, and work through more examples.
If you are interested in contributing, have questions, comments, or thoughts to share, or if you just want to be in the know, please consider joining our Community Discord and follow us on X!
Ludwig is an actively managed open-source project that relies on contributions from folks just like you. Consider joining the active group of Ludwig contributors to make Ludwig an even more accessible and feature rich framework for everyone to use!
For Tasks:
Click tags to check more tools for each tasksFor Jobs:
Alternative AI tools for ludwig
Similar Open Source Tools
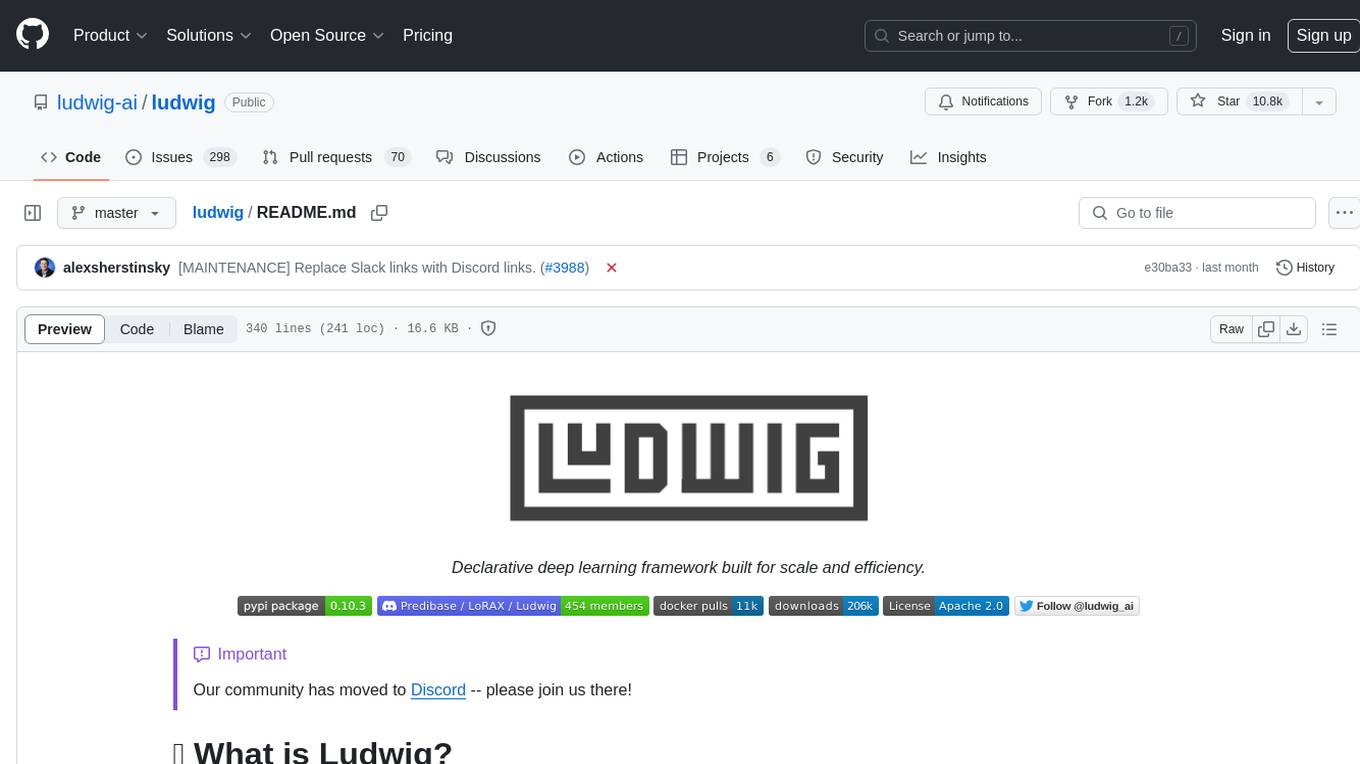
ludwig
Ludwig is a declarative deep learning framework designed for scale and efficiency. It is a low-code framework that allows users to build custom AI models like LLMs and other deep neural networks with ease. Ludwig offers features such as optimized scale and efficiency, expert level control, modularity, and extensibility. It is engineered for production with prebuilt Docker containers, support for running with Ray on Kubernetes, and the ability to export models to Torchscript and Triton. Ludwig is hosted by the Linux Foundation AI & Data.
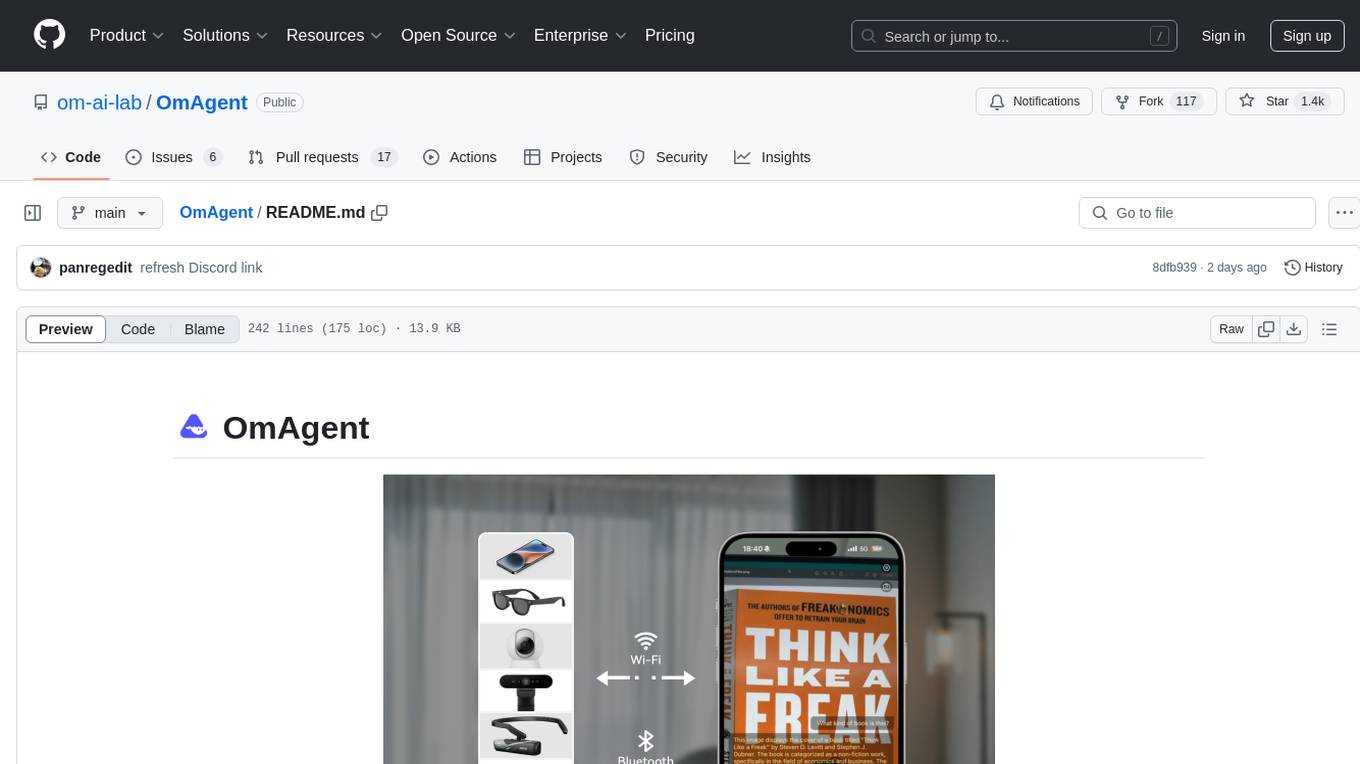
OmAgent
OmAgent is an open-source agent framework designed to streamline the development of on-device multimodal agents. It enables agents to empower various hardware devices, integrates speed-optimized SOTA multimodal models, provides SOTA multimodal agent algorithms, and focuses on optimizing the end-to-end computing pipeline for real-time user interaction experience. Key features include easy connection to diverse devices, scalability, flexibility, and workflow orchestration. The architecture emphasizes graph-based workflow orchestration, native multimodality, and device-centricity, allowing developers to create bespoke intelligent agent programs.
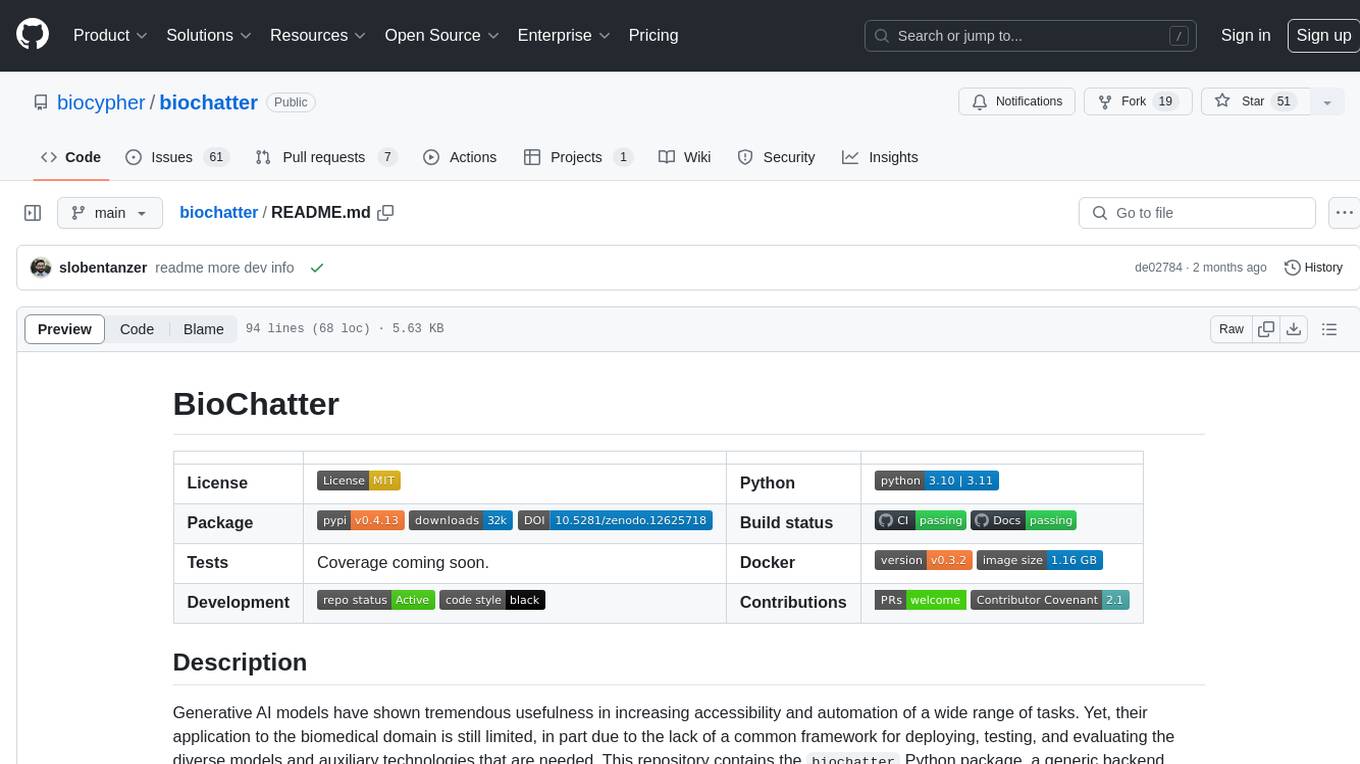
biochatter
Generative AI models have shown tremendous usefulness in increasing accessibility and automation of a wide range of tasks. This repository contains the `biochatter` Python package, a generic backend library for the connection of biomedical applications to conversational AI. It aims to provide a common framework for deploying, testing, and evaluating diverse models and auxiliary technologies in the biomedical domain. BioChatter is part of the BioCypher ecosystem, connecting natively to BioCypher knowledge graphs.
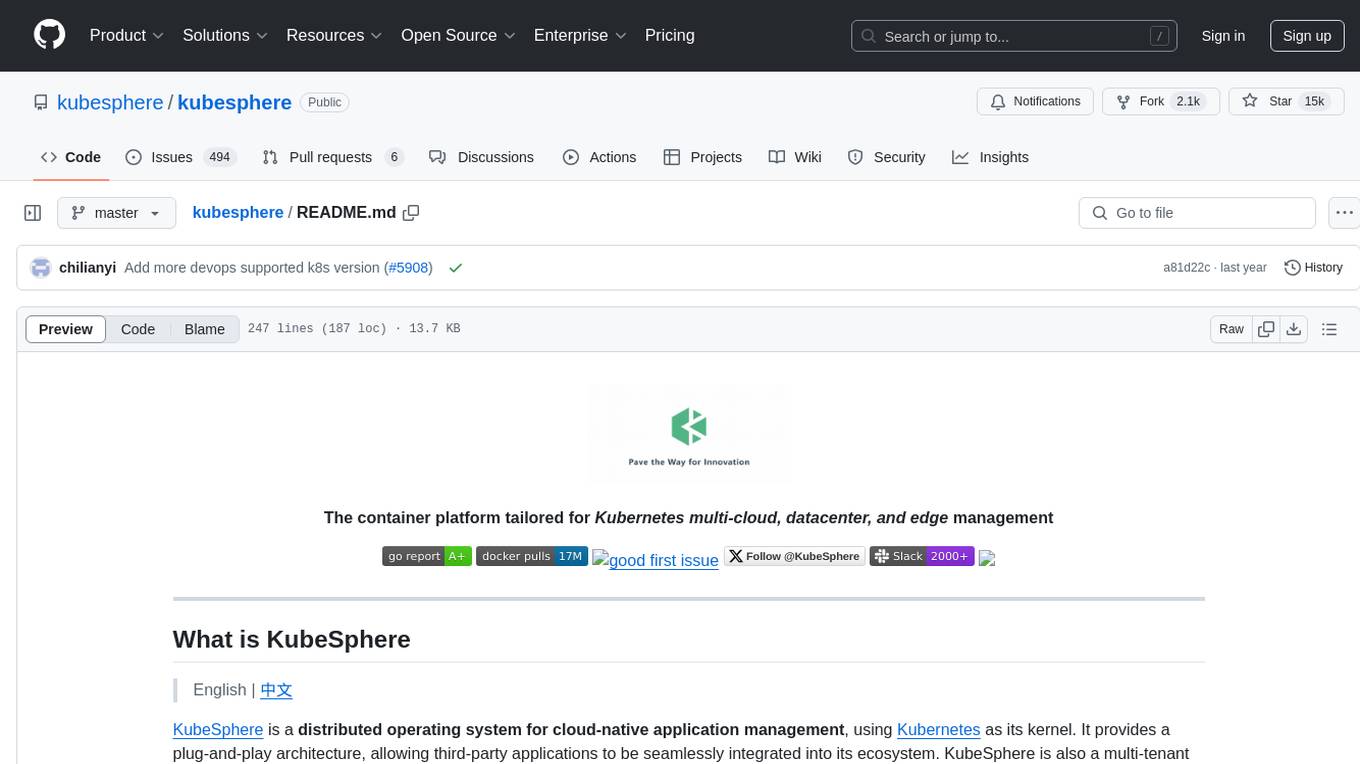
kubesphere
KubeSphere is a distributed operating system for cloud-native application management, using Kubernetes as its kernel. It provides a plug-and-play architecture, allowing third-party applications to be seamlessly integrated into its ecosystem. KubeSphere is also a multi-tenant container platform with full-stack automated IT operation and streamlined DevOps workflows. It provides developer-friendly wizard web UI, helping enterprises to build out a more robust and feature-rich platform, which includes most common functionalities needed for enterprise Kubernetes strategy.
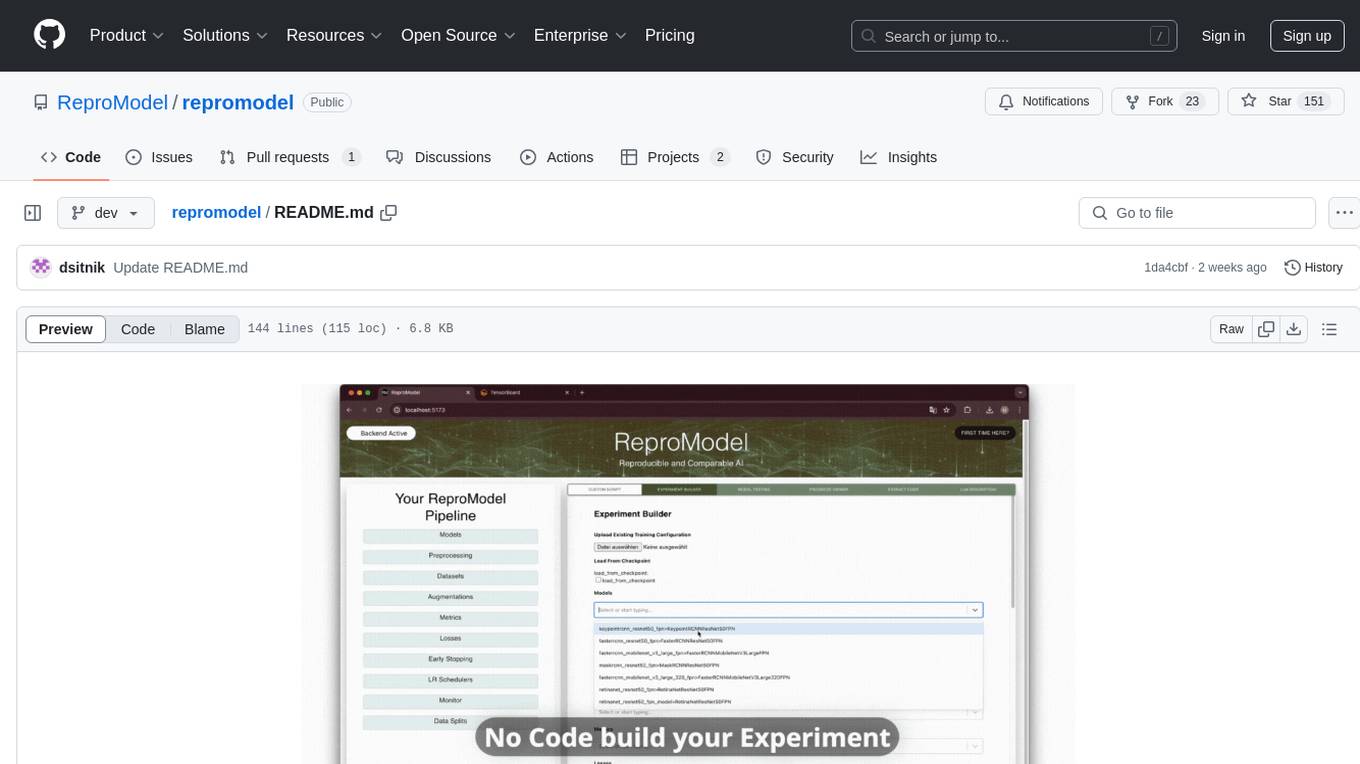
repromodel
ReproModel is an open-source toolbox designed to boost AI research efficiency by enabling researchers to reproduce, compare, train, and test AI models faster. It provides standardized models, dataloaders, and processing procedures, allowing researchers to focus on new datasets and model development. With a no-code solution, users can access benchmark and SOTA models and datasets, utilize training visualizations, extract code for publication, and leverage an LLM-powered automated methodology description writer. The toolbox helps researchers modularize development, compare pipeline performance reproducibly, and reduce time for model development, computation, and writing. Future versions aim to facilitate building upon state-of-the-art research by loading previously published study IDs with verified code, experiments, and results stored in the system.
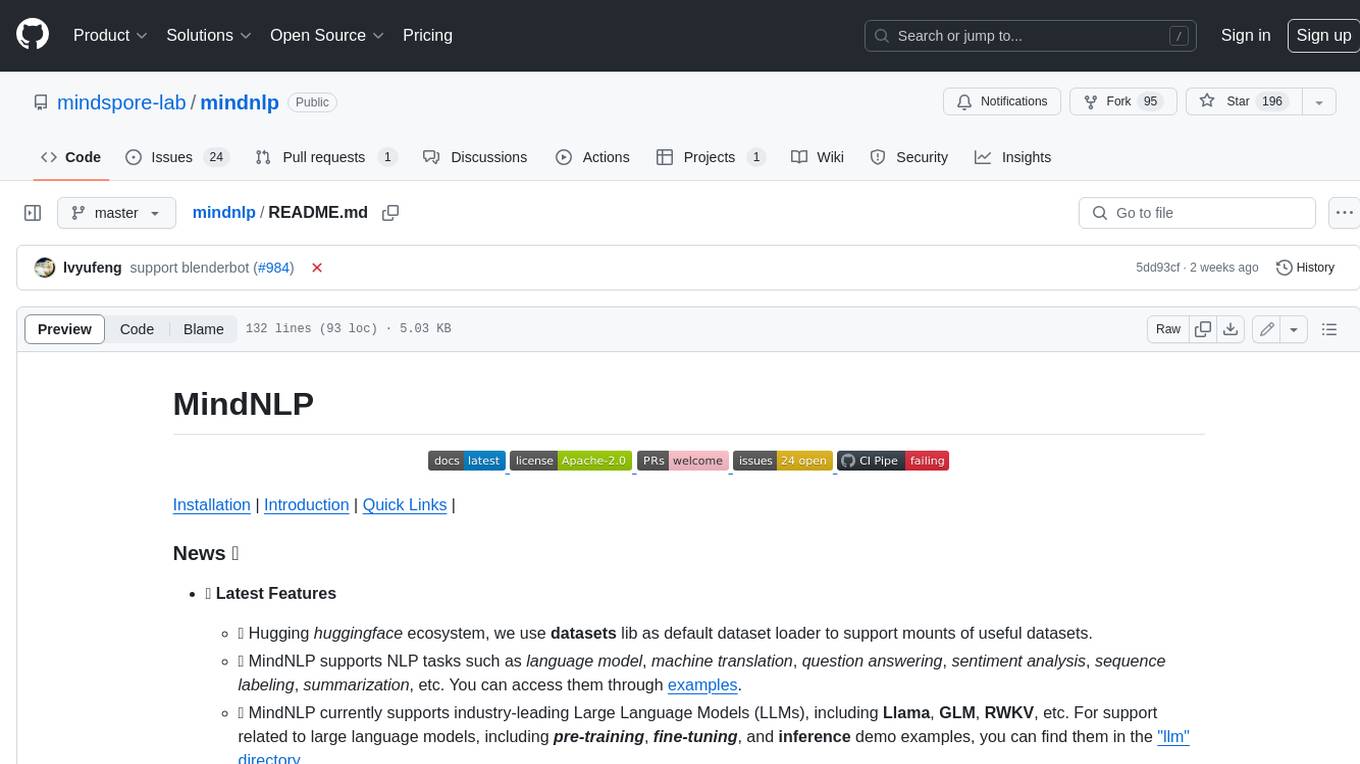
mindnlp
MindNLP is an open-source NLP library based on MindSpore. It provides a platform for solving natural language processing tasks, containing many common approaches in NLP. It can help researchers and developers to construct and train models more conveniently and rapidly. Key features of MindNLP include: * Comprehensive data processing: Several classical NLP datasets are packaged into a friendly module for easy use, such as Multi30k, SQuAD, CoNLL, etc. * Friendly NLP model toolset: MindNLP provides various configurable components. It is friendly to customize models using MindNLP. * Easy-to-use engine: MindNLP simplified complicated training process in MindSpore. It supports Trainer and Evaluator interfaces to train and evaluate models easily. MindNLP supports a wide range of NLP tasks, including: * Language modeling * Machine translation * Question answering * Sentiment analysis * Sequence labeling * Summarization MindNLP also supports industry-leading Large Language Models (LLMs), including Llama, GLM, RWKV, etc. For support related to large language models, including pre-training, fine-tuning, and inference demo examples, you can find them in the "llm" directory. To install MindNLP, you can either install it from Pypi, download the daily build wheel, or install it from source. The installation instructions are provided in the documentation. MindNLP is released under the Apache 2.0 license. If you find this project useful in your research, please consider citing the following paper: @misc{mindnlp2022, title={{MindNLP}: a MindSpore NLP library}, author={MindNLP Contributors}, howpublished = {\url{https://github.com/mindlab-ai/mindnlp}}, year={2022} }
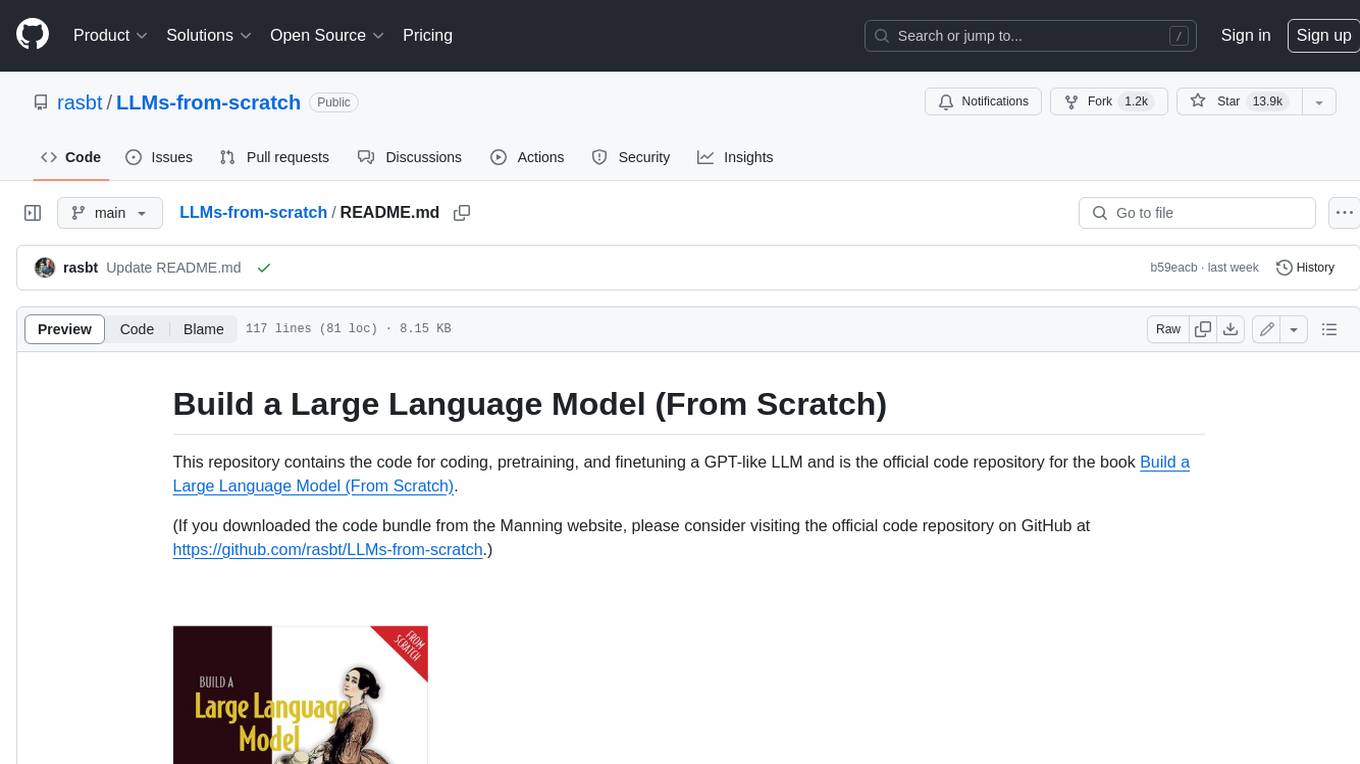
LLMs-from-scratch
This repository contains the code for coding, pretraining, and finetuning a GPT-like LLM and is the official code repository for the book Build a Large Language Model (From Scratch). In _Build a Large Language Model (From Scratch)_, you'll discover how LLMs work from the inside out. In this book, I'll guide you step by step through creating your own LLM, explaining each stage with clear text, diagrams, and examples. The method described in this book for training and developing your own small-but-functional model for educational purposes mirrors the approach used in creating large-scale foundational models such as those behind ChatGPT.
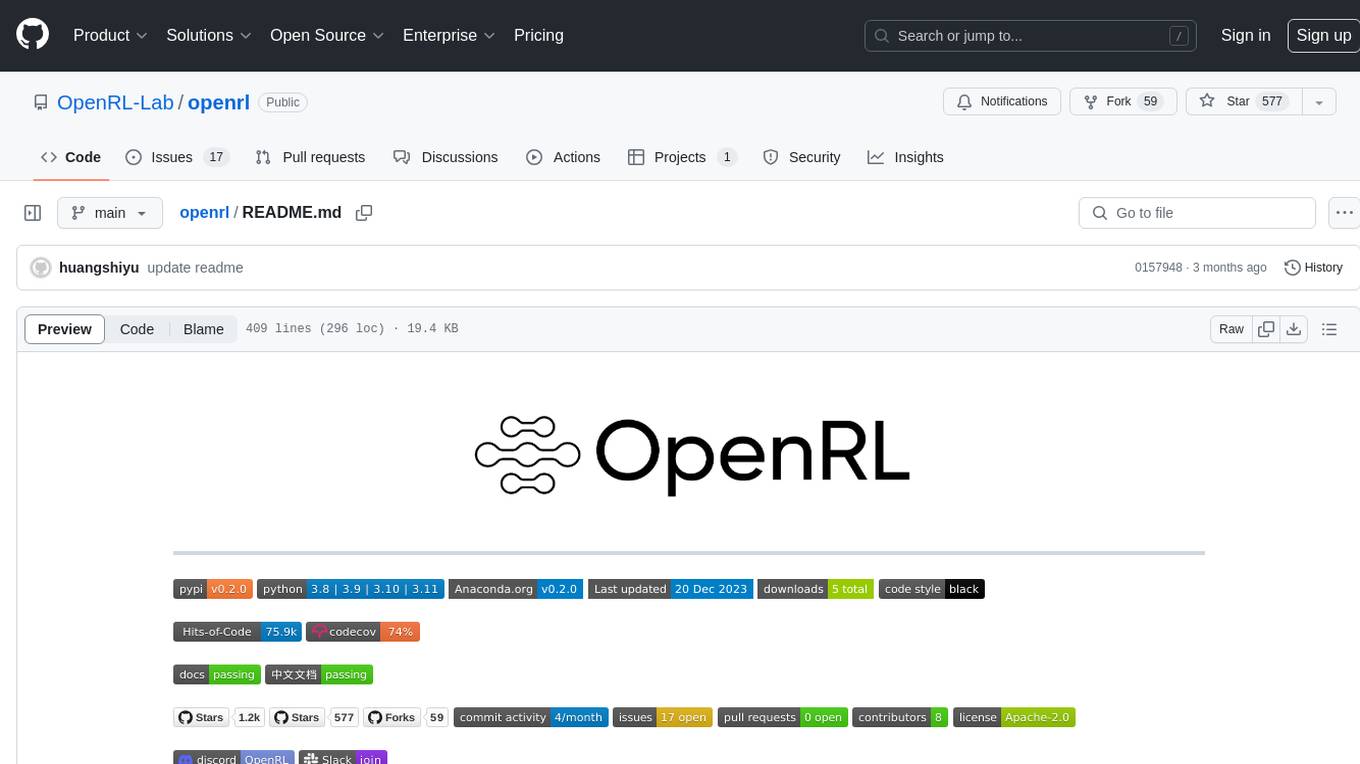
openrl
OpenRL is an open-source general reinforcement learning research framework that supports training for various tasks such as single-agent, multi-agent, offline RL, self-play, and natural language. Developed based on PyTorch, the goal of OpenRL is to provide a simple-to-use, flexible, efficient and sustainable platform for the reinforcement learning research community. It supports a universal interface for all tasks/environments, single-agent and multi-agent tasks, offline RL training with expert dataset, self-play training, reinforcement learning training for natural language tasks, DeepSpeed, Arena for evaluation, importing models and datasets from Hugging Face, user-defined environments, models, and datasets, gymnasium environments, callbacks, visualization tools, unit testing, and code coverage testing. It also supports various algorithms like PPO, DQN, SAC, and environments like Gymnasium, MuJoCo, Atari, and more.
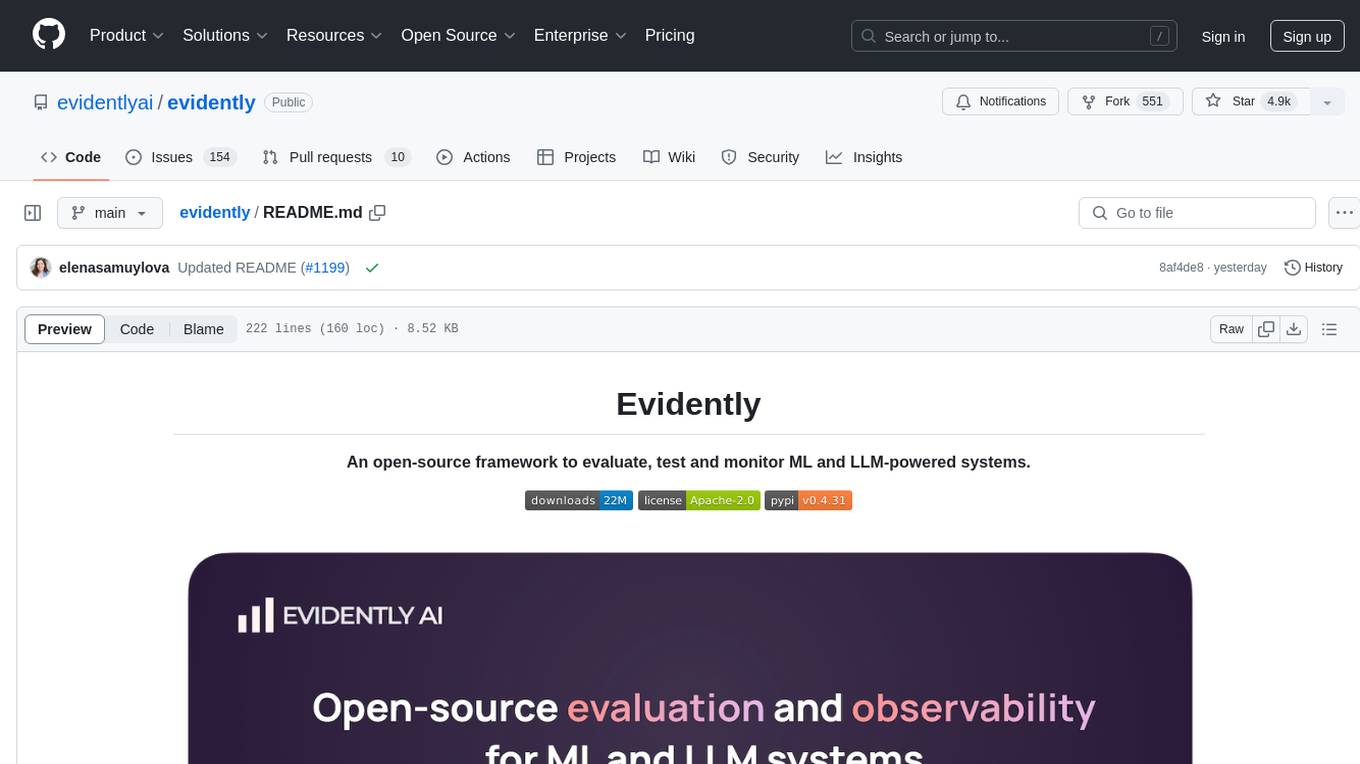
evidently
Evidently is an open-source Python library designed for evaluating, testing, and monitoring machine learning (ML) and large language model (LLM) powered systems. It offers a wide range of functionalities, including working with tabular, text data, and embeddings, supporting predictive and generative systems, providing over 100 built-in metrics for data drift detection and LLM evaluation, allowing for custom metrics and tests, enabling both offline evaluations and live monitoring, and offering an open architecture for easy data export and integration with existing tools. Users can utilize Evidently for one-off evaluations using Reports or Test Suites in Python, or opt for real-time monitoring through the Dashboard service.
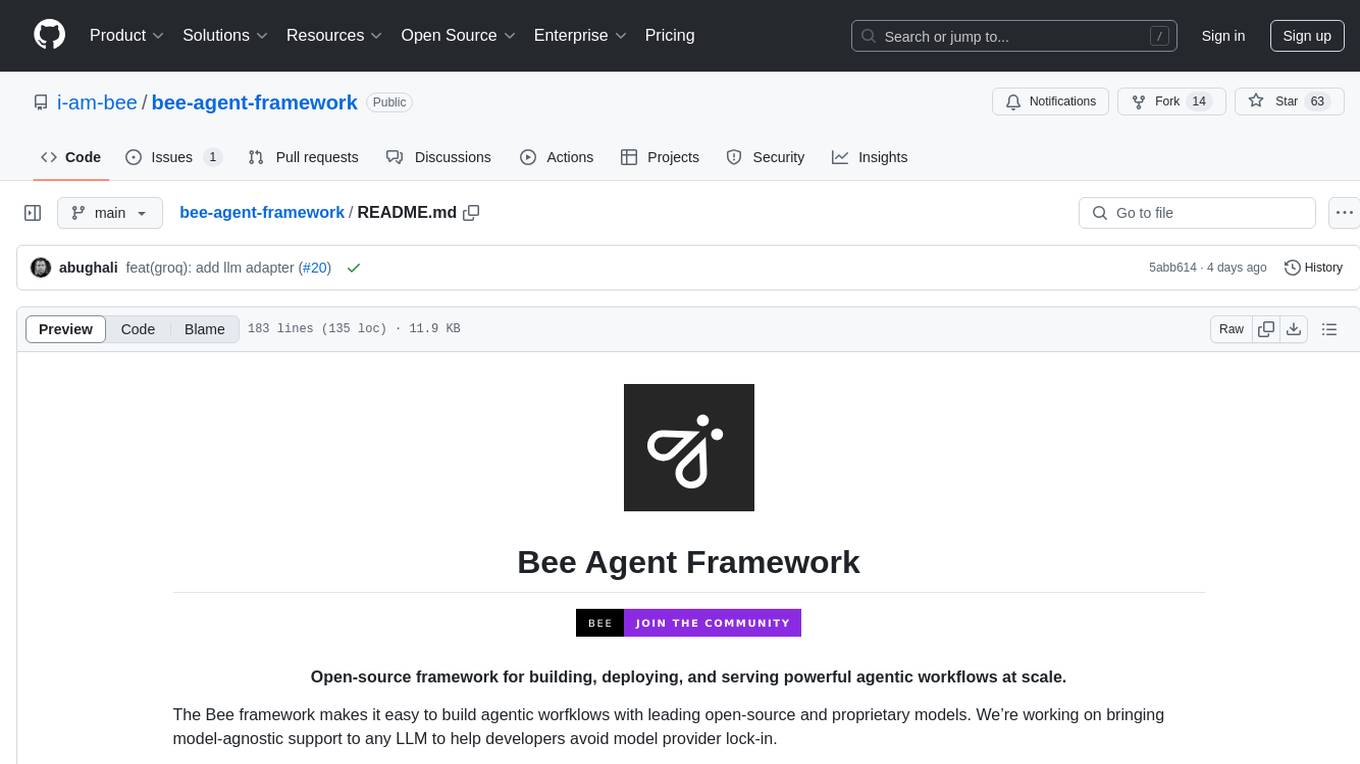
bee-agent-framework
The Bee Agent Framework is an open-source tool for building, deploying, and serving powerful agentic workflows at scale. It provides AI agents, tools for creating workflows in Javascript/Python, a code interpreter, memory optimization strategies, serialization for pausing/resuming workflows, traceability features, production-level control, and upcoming features like model-agnostic support and a chat UI. The framework offers various modules for agents, llms, memory, tools, caching, errors, adapters, logging, serialization, and more, with a roadmap including MLFlow integration, JSON support, structured outputs, chat client, base agent improvements, guardrails, and evaluation.
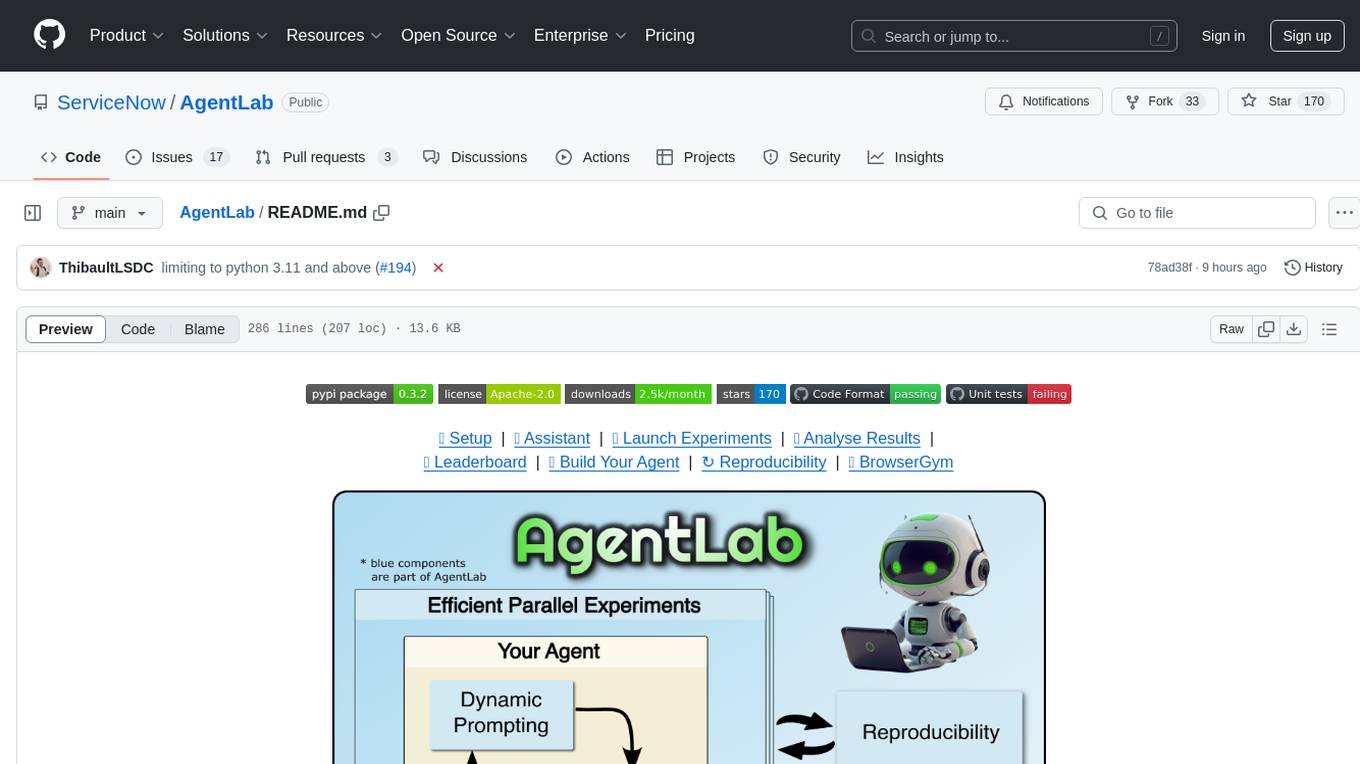
AgentLab
AgentLab is an open, easy-to-use, and extensible framework designed to accelerate web agent research. It provides features for developing and evaluating agents on various benchmarks supported by BrowserGym. The framework allows for large-scale parallel agent experiments using ray, building blocks for creating agents over BrowserGym, and a unified LLM API for OpenRouter, OpenAI, Azure, or self-hosted using TGI. AgentLab also offers reproducibility features, a unified LeaderBoard, and supports multiple benchmarks like WebArena, WorkArena, WebLinx, VisualWebArena, AssistantBench, GAIA, Mind2Web-live, and MiniWoB.
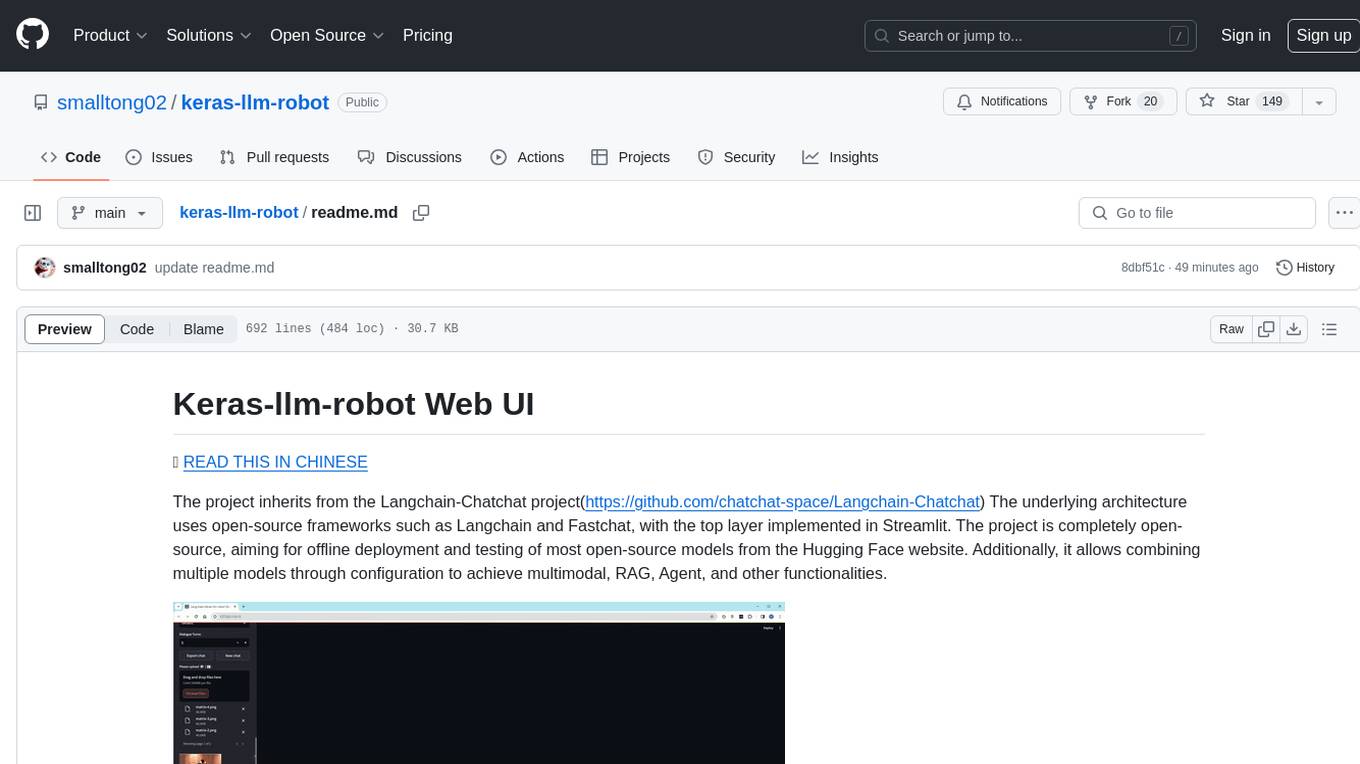
keras-llm-robot
The Keras-llm-robot Web UI project is an open-source tool designed for offline deployment and testing of various open-source models from the Hugging Face website. It allows users to combine multiple models through configuration to achieve functionalities like multimodal, RAG, Agent, and more. The project consists of three main interfaces: chat interface for language models, configuration interface for loading models, and tools & agent interface for auxiliary models. Users can interact with the language model through text, voice, and image inputs, and the tool supports features like model loading, quantization, fine-tuning, role-playing, code interpretation, speech recognition, image recognition, network search engine, and function calling.
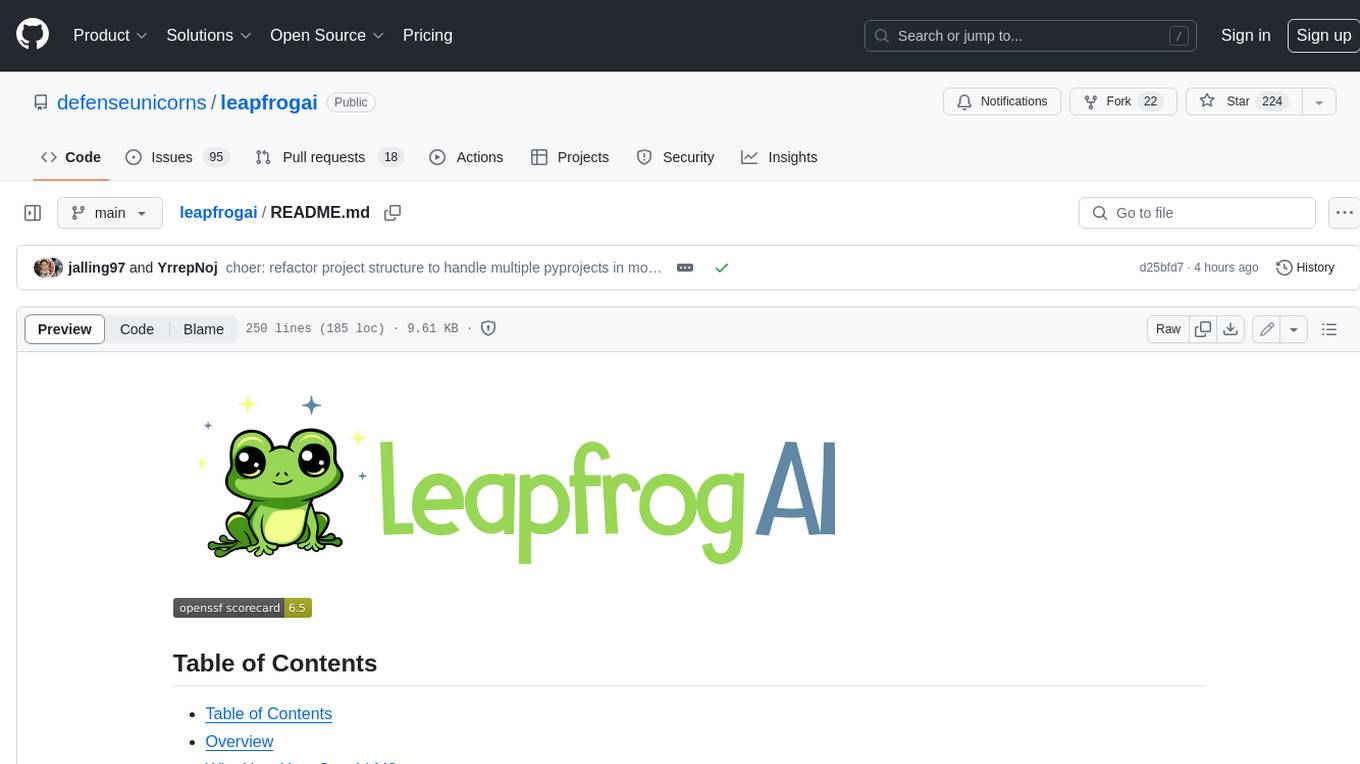
leapfrogai
LeapfrogAI is a self-hosted AI platform designed to be deployed in air-gapped resource-constrained environments. It brings sophisticated AI solutions to these environments by hosting all the necessary components of an AI stack, including vector databases, model backends, API, and UI. LeapfrogAI's API closely matches that of OpenAI, allowing tools built for OpenAI/ChatGPT to function seamlessly with a LeapfrogAI backend. It provides several backends for various use cases, including llama-cpp-python, whisper, text-embeddings, and vllm. LeapfrogAI leverages Chainguard's apko to harden base python images, ensuring the latest supported Python versions are used by the other components of the stack. The LeapfrogAI SDK provides a standard set of protobuffs and python utilities for implementing backends and gRPC. LeapfrogAI offers UI options for common use-cases like chat, summarization, and transcription. It can be deployed and run locally via UDS and Kubernetes, built out using Zarf packages. LeapfrogAI is supported by a community of users and contributors, including Defense Unicorns, Beast Code, Chainguard, Exovera, Hypergiant, Pulze, SOSi, United States Navy, United States Air Force, and United States Space Force.
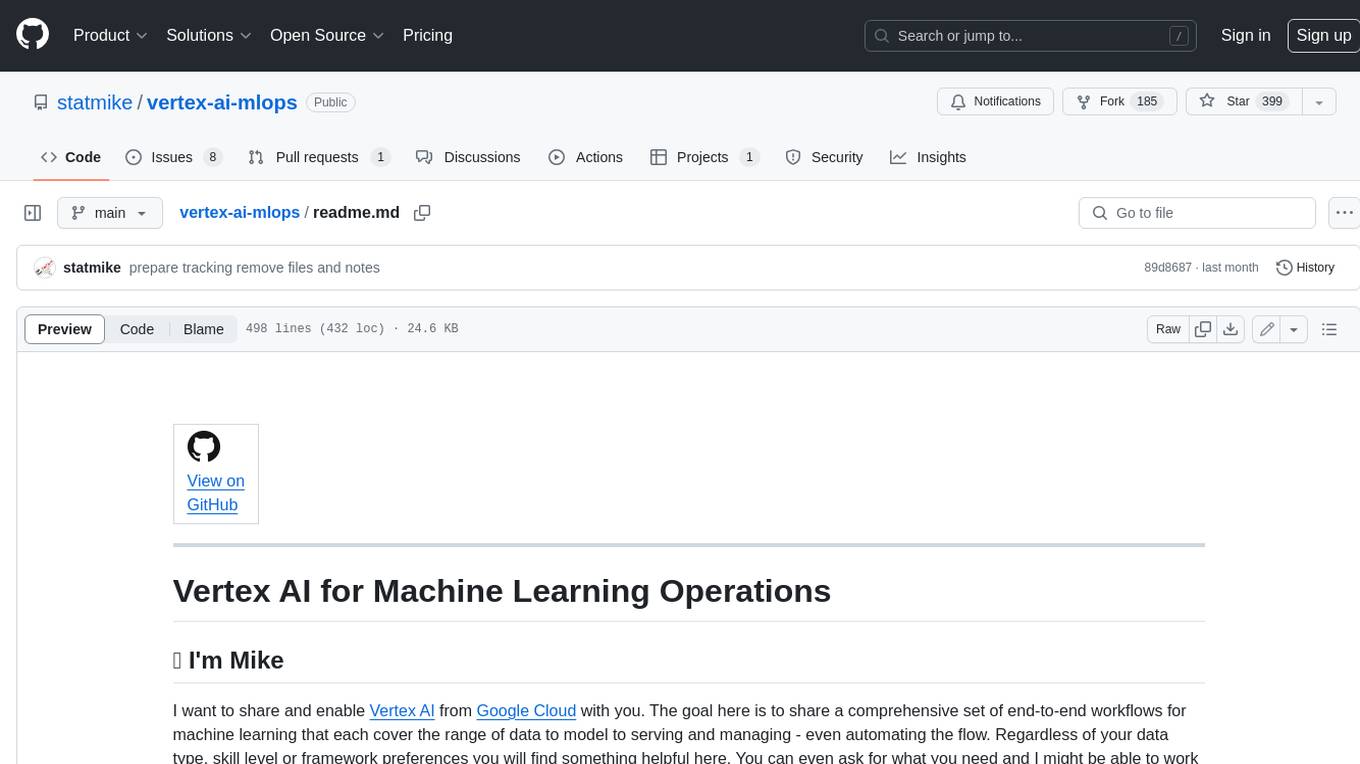
vertex-ai-mlops
Vertex AI is a platform for end-to-end model development. It consist of core components that make the processes of MLOps possible for design patterns of all types.
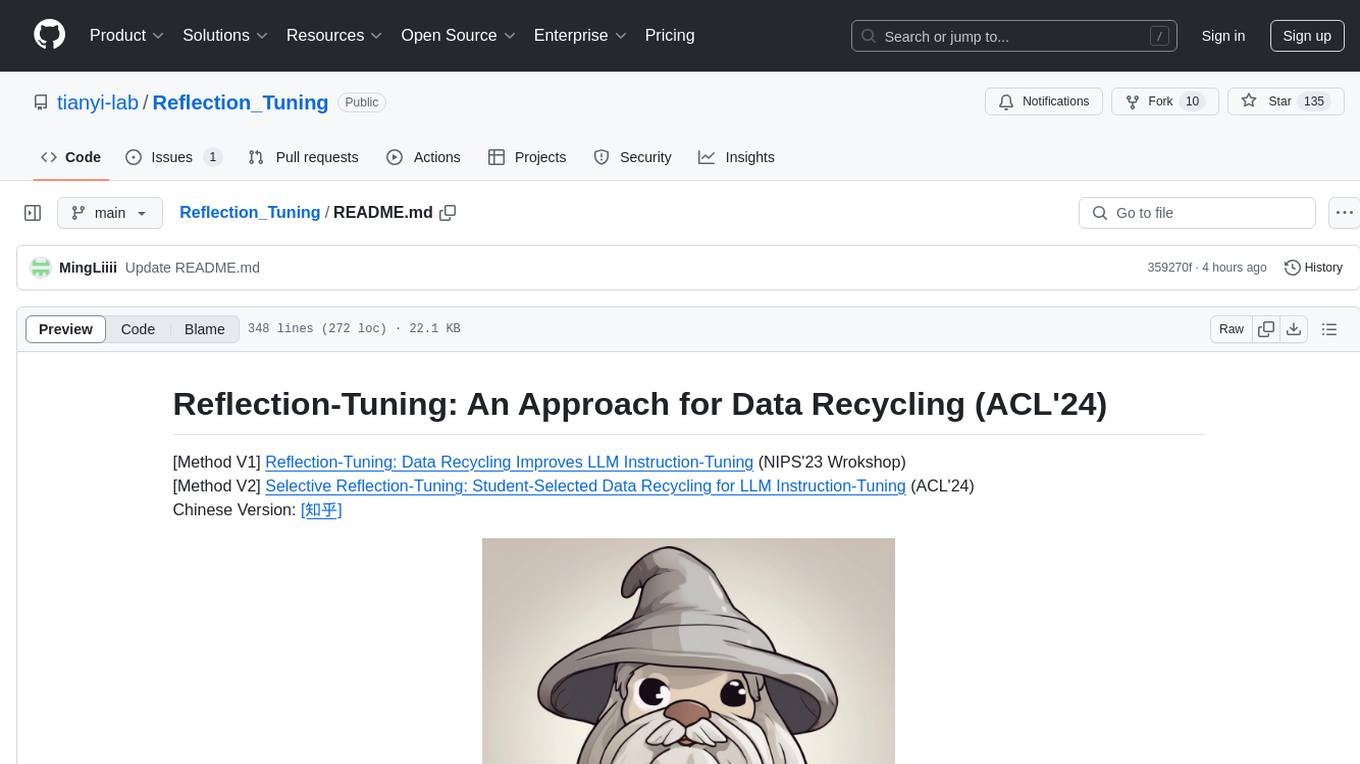
Reflection_Tuning
Reflection-Tuning is a project focused on improving the quality of instruction-tuning data through a reflection-based method. It introduces Selective Reflection-Tuning, where the student model can decide whether to accept the improvements made by the teacher model. The project aims to generate high-quality instruction-response pairs by defining specific criteria for the oracle model to follow and respond to. It also evaluates the efficacy and relevance of instruction-response pairs using the r-IFD metric. The project provides code for reflection and selection processes, along with data and model weights for both V1 and V2 methods.
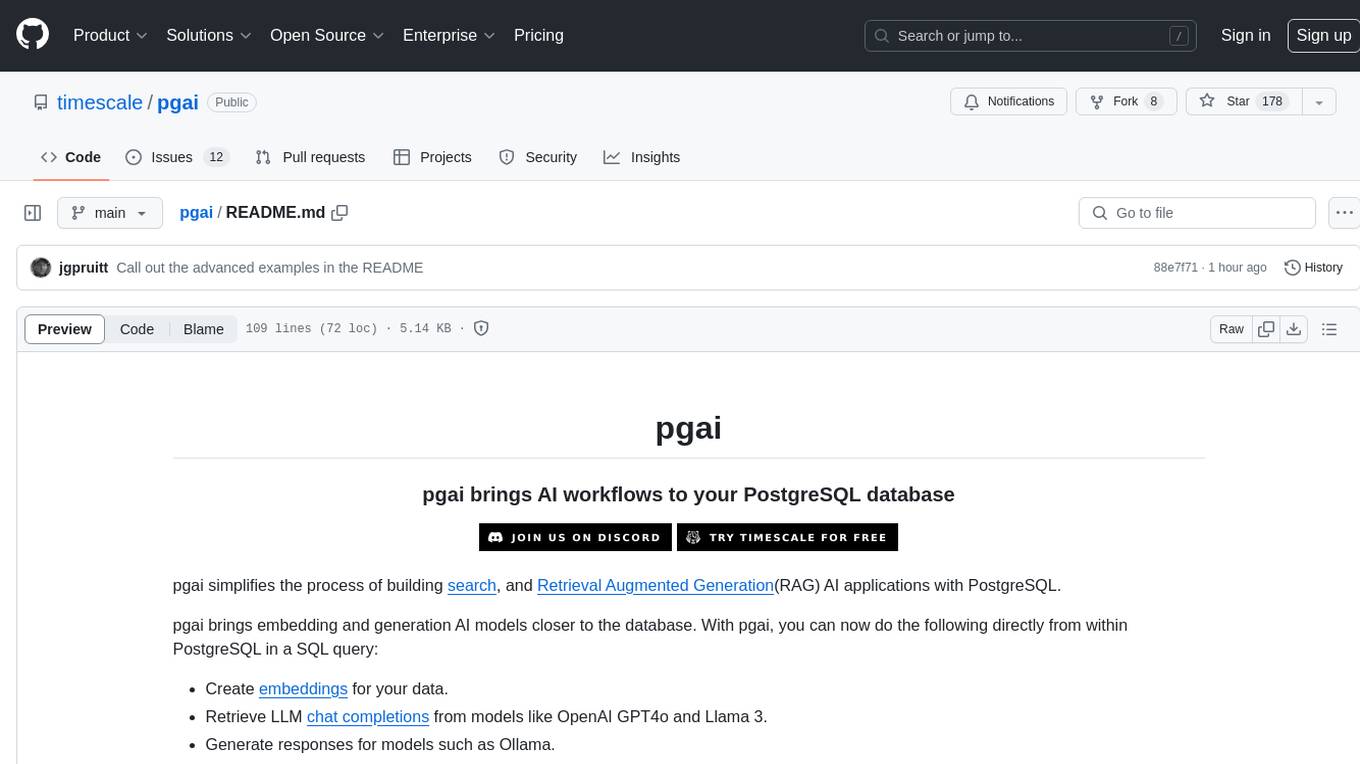
pgai
pgai simplifies the process of building search and Retrieval Augmented Generation (RAG) AI applications with PostgreSQL. It brings embedding and generation AI models closer to the database, allowing users to create embeddings, retrieve LLM chat completions, reason over data for classification, summarization, and data enrichment directly from within PostgreSQL in a SQL query. The tool requires an OpenAI API key and a PostgreSQL client to enable AI functionality in the database. Users can install pgai from source, run it in a pre-built Docker container, or enable it in a Timescale Cloud service. The tool provides functions to handle API keys using psql or Python, and offers various AI functionalities like tokenizing, detokenizing, embedding, chat completion, and content moderation.
For similar tasks
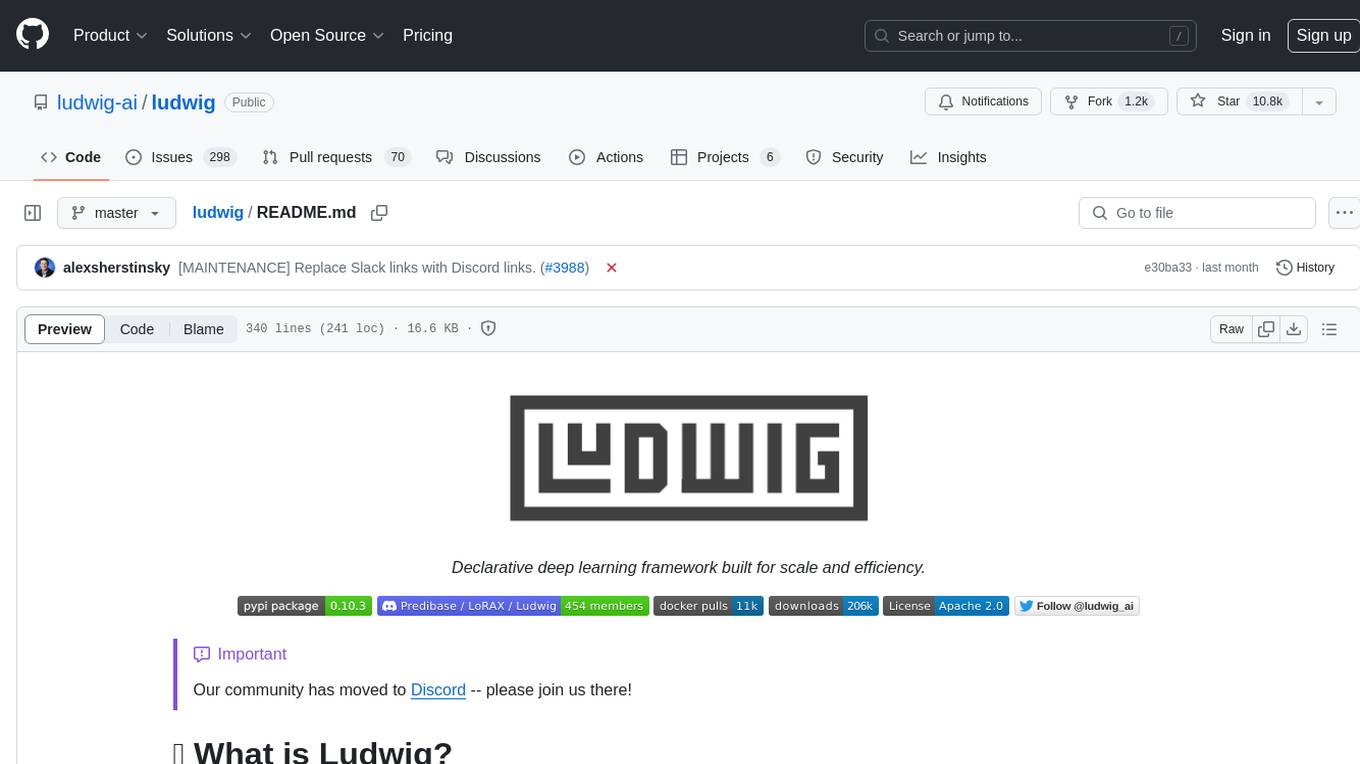
ludwig
Ludwig is a declarative deep learning framework designed for scale and efficiency. It is a low-code framework that allows users to build custom AI models like LLMs and other deep neural networks with ease. Ludwig offers features such as optimized scale and efficiency, expert level control, modularity, and extensibility. It is engineered for production with prebuilt Docker containers, support for running with Ray on Kubernetes, and the ability to export models to Torchscript and Triton. Ludwig is hosted by the Linux Foundation AI & Data.
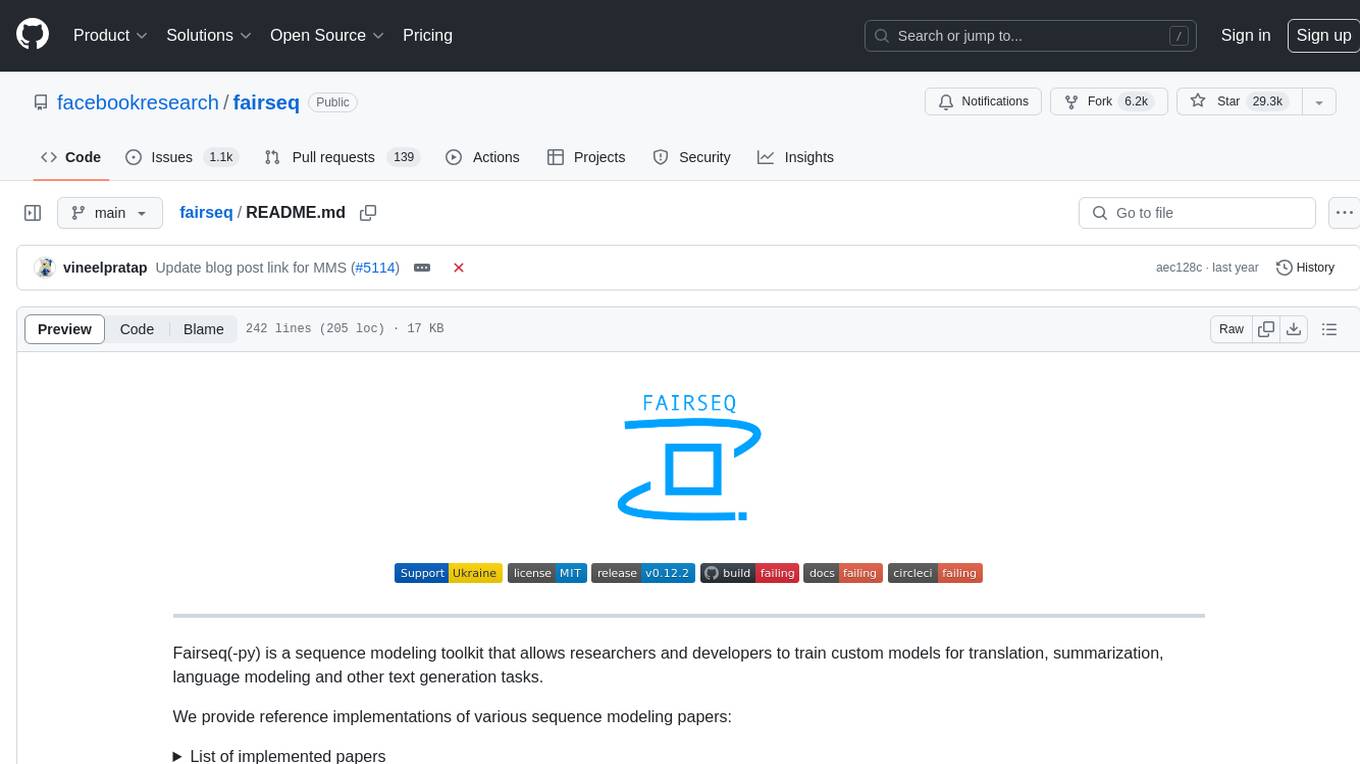
fairseq
Fairseq is a sequence modeling toolkit that enables researchers and developers to train custom models for translation, summarization, language modeling, and other text generation tasks. It provides reference implementations of various sequence modeling papers covering CNN, LSTM networks, Transformer networks, LightConv, DynamicConv models, Non-autoregressive Transformers, Finetuning, and more. The toolkit supports multi-GPU training, fast generation on CPU and GPU, mixed precision training, extensibility, flexible configuration based on Hydra, and full parameter and optimizer state sharding. Pre-trained models are available for translation and language modeling with a torch.hub interface. Fairseq also offers pre-trained models and examples for tasks like XLS-R, cross-lingual retrieval, wav2vec 2.0, unsupervised quality estimation, and more.
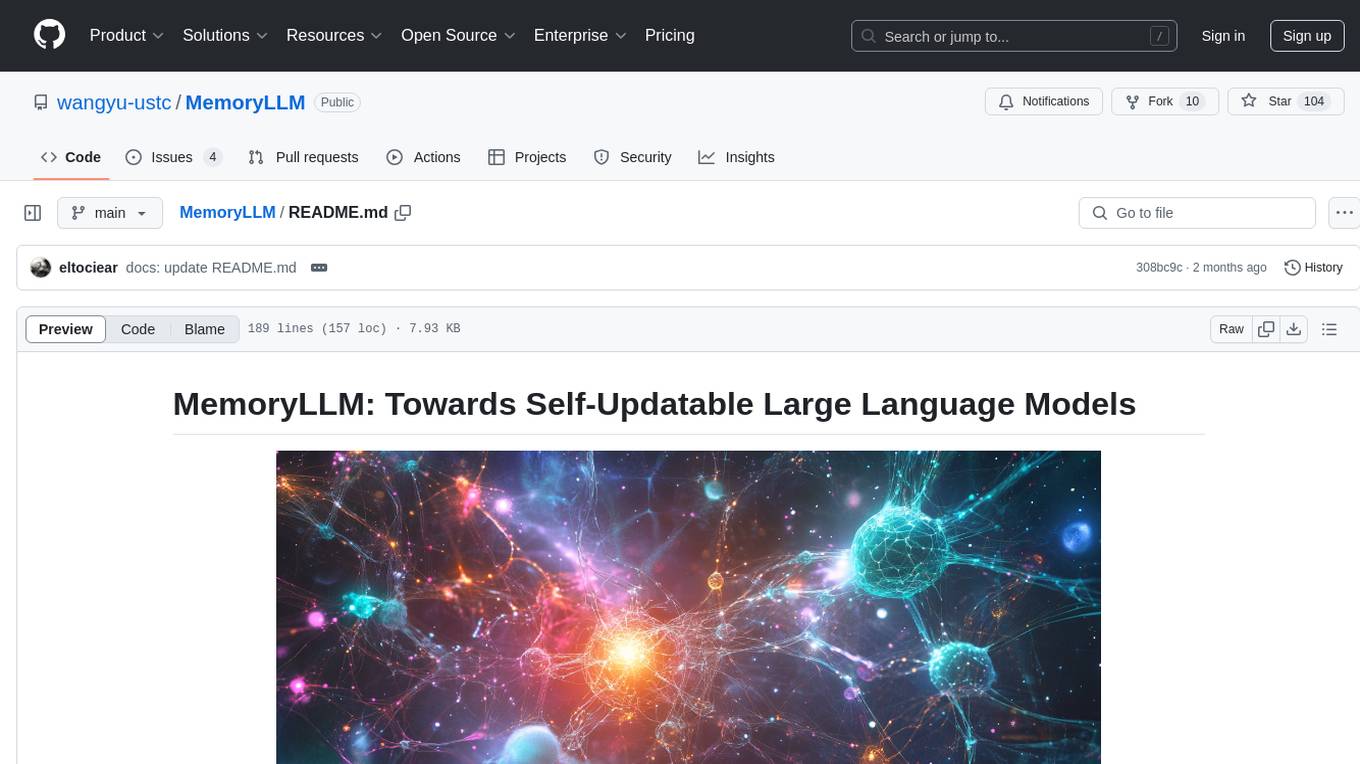
MemoryLLM
MemoryLLM is a large language model designed for self-updating capabilities. It offers pretrained models with different memory capacities and features, such as chat models. The repository provides training code, evaluation scripts, and datasets for custom experiments. MemoryLLM aims to enhance knowledge retention and performance on various natural language processing tasks.
For similar jobs
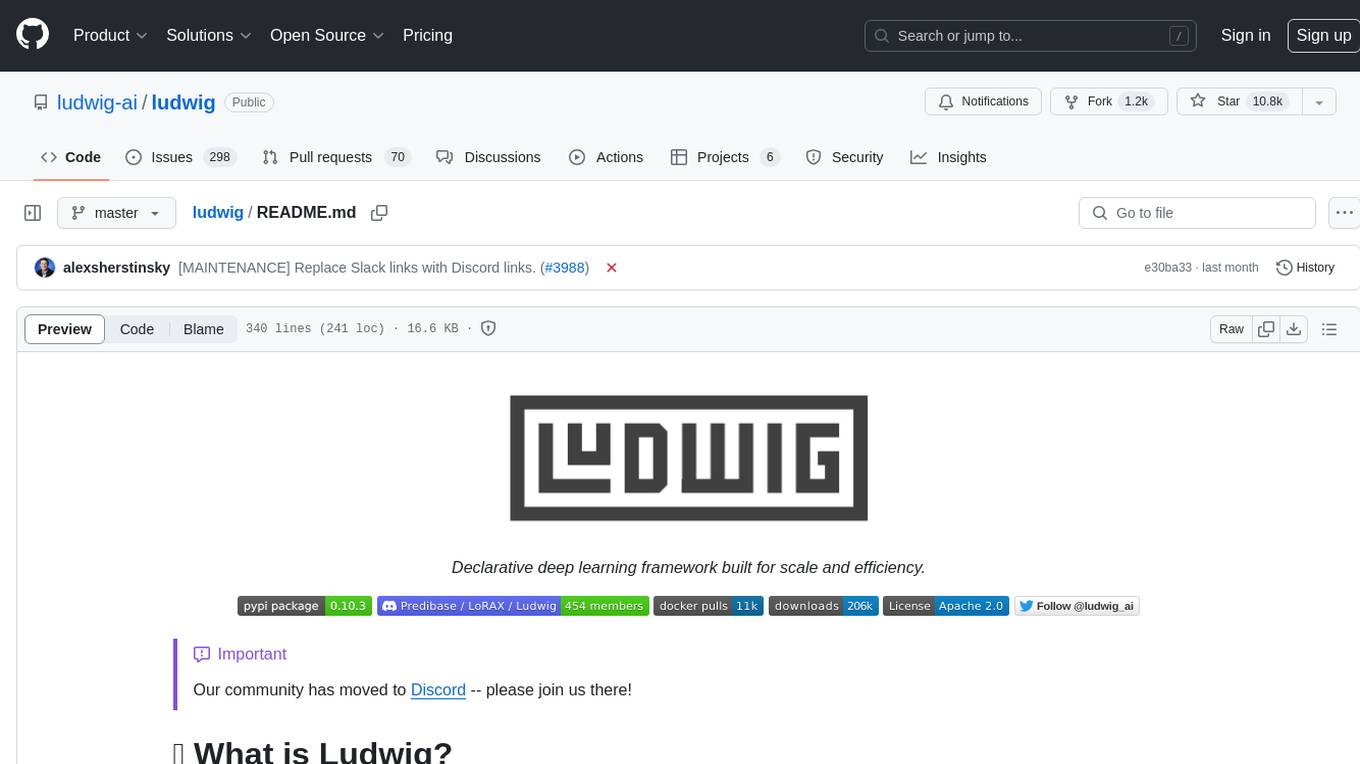
ludwig
Ludwig is a declarative deep learning framework designed for scale and efficiency. It is a low-code framework that allows users to build custom AI models like LLMs and other deep neural networks with ease. Ludwig offers features such as optimized scale and efficiency, expert level control, modularity, and extensibility. It is engineered for production with prebuilt Docker containers, support for running with Ray on Kubernetes, and the ability to export models to Torchscript and Triton. Ludwig is hosted by the Linux Foundation AI & Data.
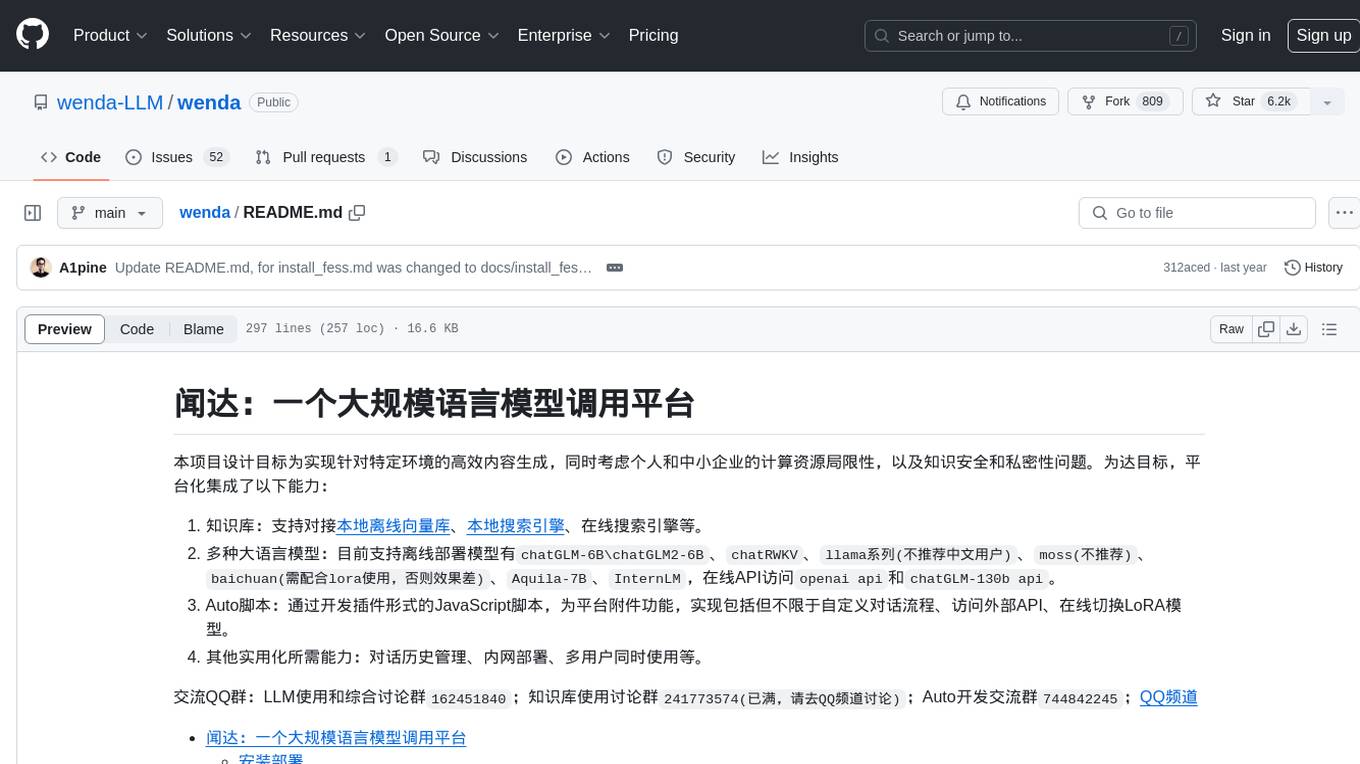
wenda
Wenda is a platform for large-scale language model invocation designed to efficiently generate content for specific environments, considering the limitations of personal and small business computing resources, as well as knowledge security and privacy issues. The platform integrates capabilities such as knowledge base integration, multiple large language models for offline deployment, auto scripts for additional functionality, and other practical capabilities like conversation history management and multi-user simultaneous usage.
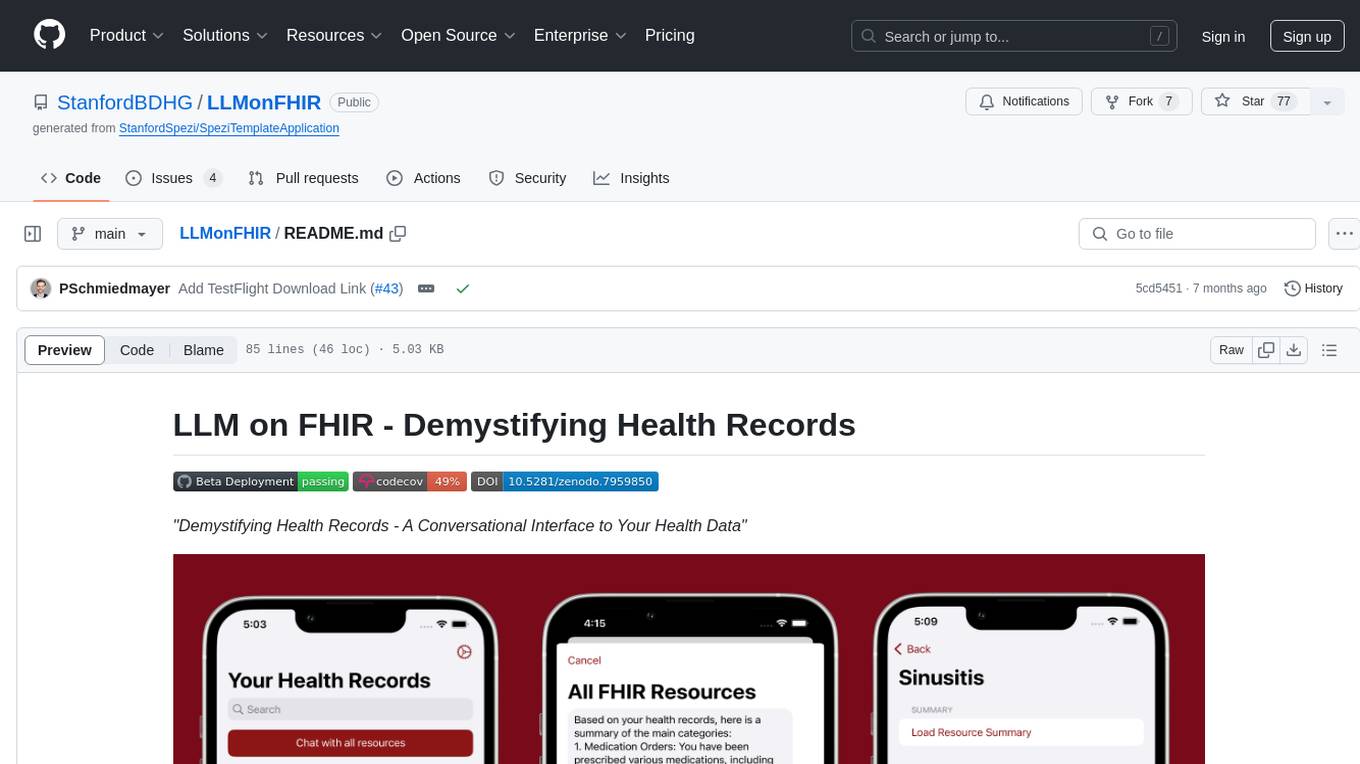
LLMonFHIR
LLMonFHIR is an iOS application that utilizes large language models (LLMs) to interpret and provide context around patient data in the Fast Healthcare Interoperability Resources (FHIR) format. It connects to the OpenAI GPT API to analyze FHIR resources, supports multiple languages, and allows users to interact with their health data stored in the Apple Health app. The app aims to simplify complex health records, provide insights, and facilitate deeper understanding through a conversational interface. However, it is an experimental app for informational purposes only and should not be used as a substitute for professional medical advice. Users are advised to verify information provided by AI models and consult healthcare professionals for personalized advice.
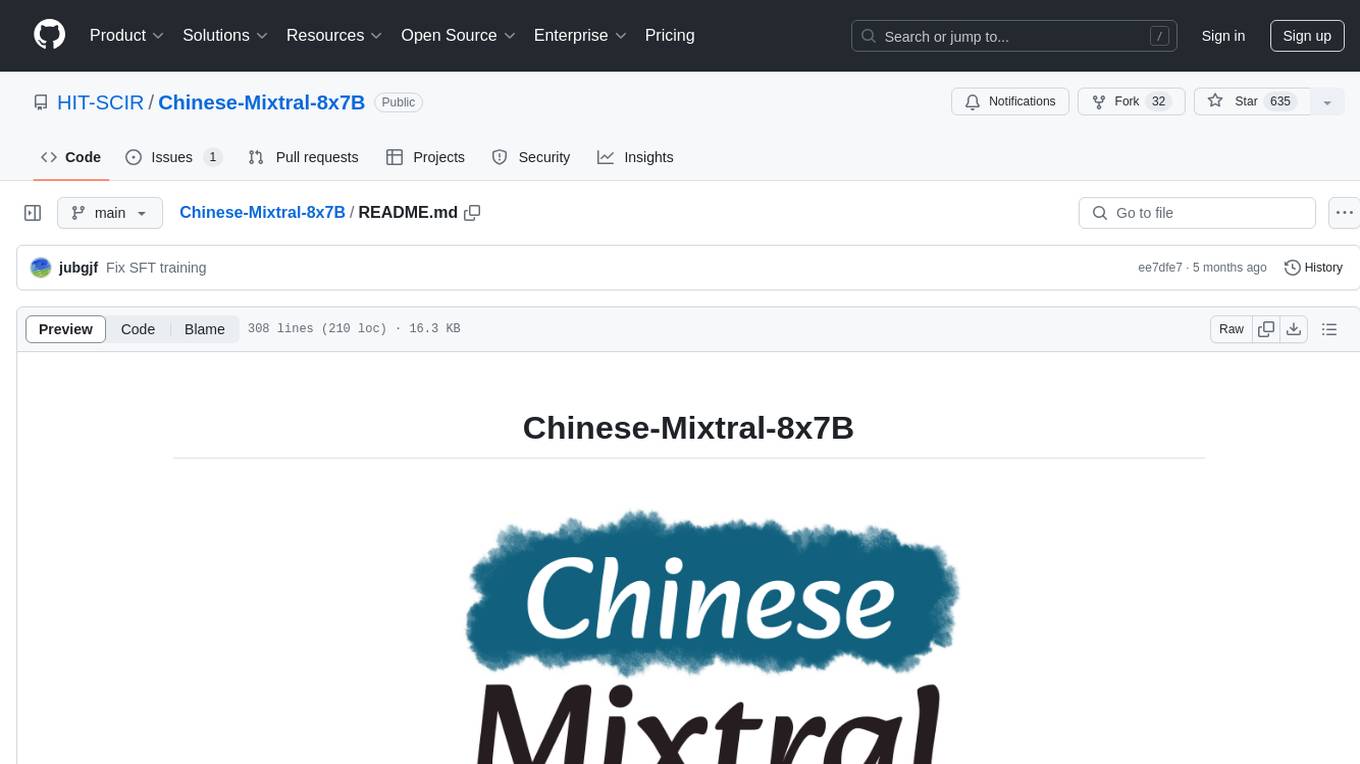
Chinese-Mixtral-8x7B
Chinese-Mixtral-8x7B is an open-source project based on Mistral's Mixtral-8x7B model for incremental pre-training of Chinese vocabulary, aiming to advance research on MoE models in the Chinese natural language processing community. The expanded vocabulary significantly improves the model's encoding and decoding efficiency for Chinese, and the model is pre-trained incrementally on a large-scale open-source corpus, enabling it with powerful Chinese generation and comprehension capabilities. The project includes a large model with expanded Chinese vocabulary and incremental pre-training code.
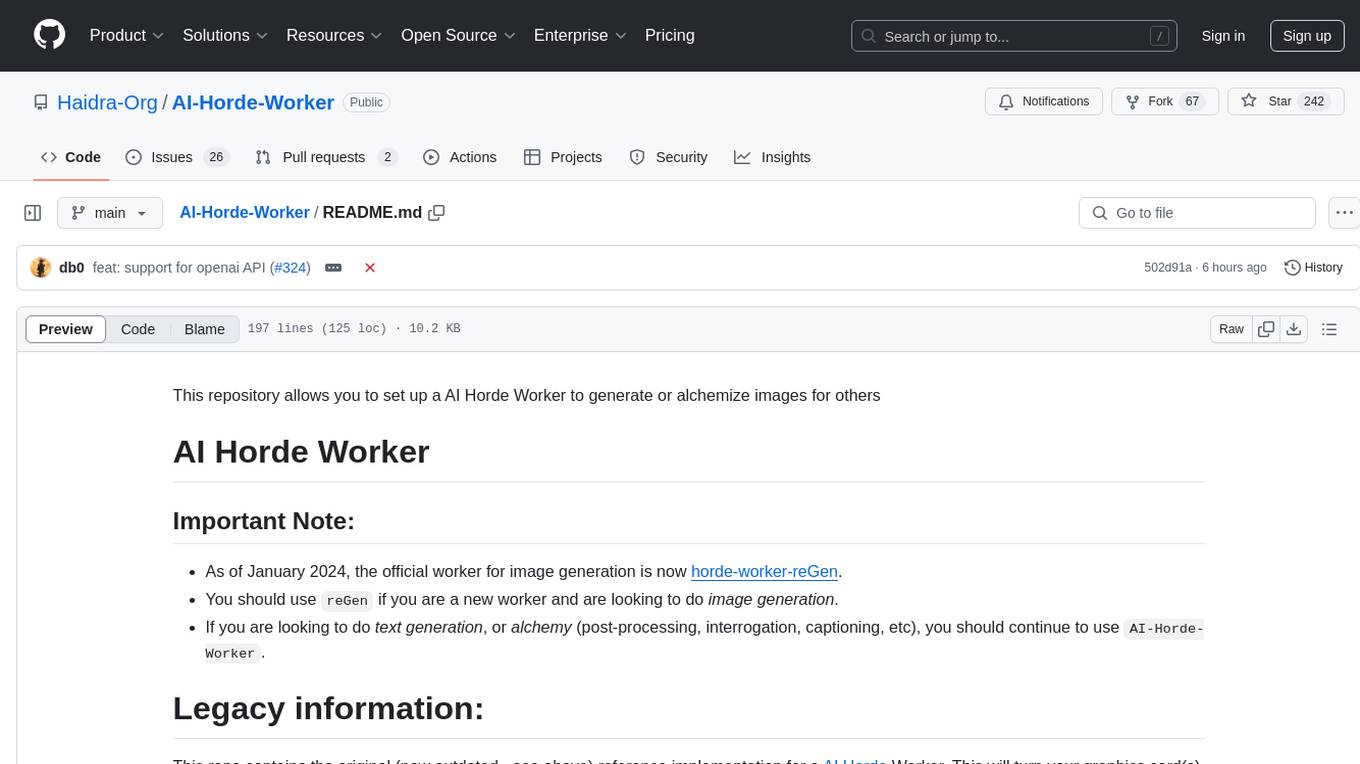
AI-Horde-Worker
AI-Horde-Worker is a repository containing the original reference implementation for a worker that turns your graphics card(s) into a worker for the AI Horde. It allows users to generate or alchemize images for others. The repository provides instructions for setting up the worker on Windows and Linux, updating the worker code, running with multiple GPUs, and stopping the worker. Users can configure the worker using a WebUI to connect to the horde with their username and API key. The repository also includes information on model usage and running the Docker container with specified environment variables.
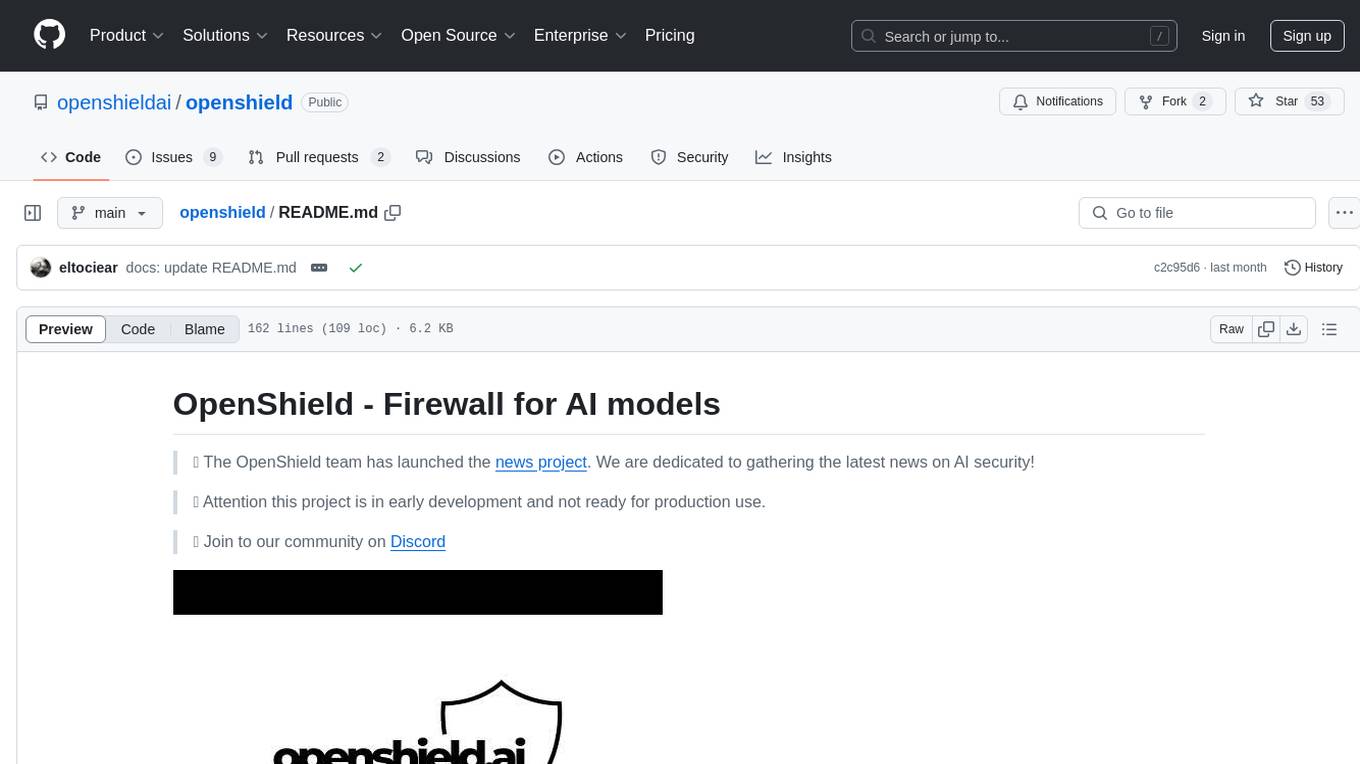
openshield
OpenShield is a firewall designed for AI models to protect against various attacks such as prompt injection, insecure output handling, training data poisoning, model denial of service, supply chain vulnerabilities, sensitive information disclosure, insecure plugin design, excessive agency granting, overreliance, and model theft. It provides rate limiting, content filtering, and keyword filtering for AI models. The tool acts as a transparent proxy between AI models and clients, allowing users to set custom rate limits for OpenAI endpoints and perform tokenizer calculations for OpenAI models. OpenShield also supports Python and LLM based rules, with upcoming features including rate limiting per user and model, prompts manager, content filtering, keyword filtering based on LLM/Vector models, OpenMeter integration, and VectorDB integration. The tool requires an OpenAI API key, Postgres, and Redis for operation.
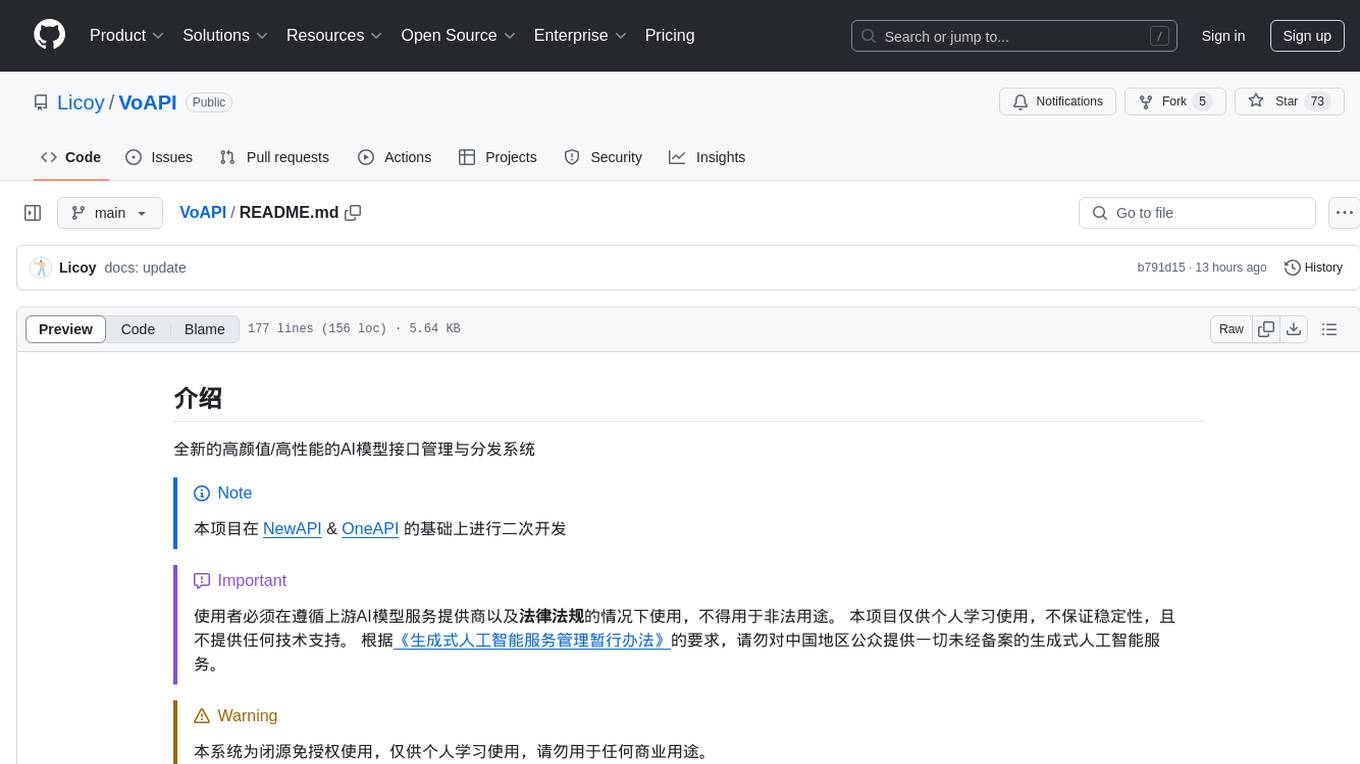
VoAPI
VoAPI is a new high-value/high-performance AI model interface management and distribution system. It is a closed-source tool for personal learning use only, not for commercial purposes. Users must comply with upstream AI model service providers and legal regulations. The system offers a visually appealing interface, independent development documentation page support, service monitoring page configuration support, and third-party login support. It also optimizes interface elements, user registration time support, data operation button positioning, and more.
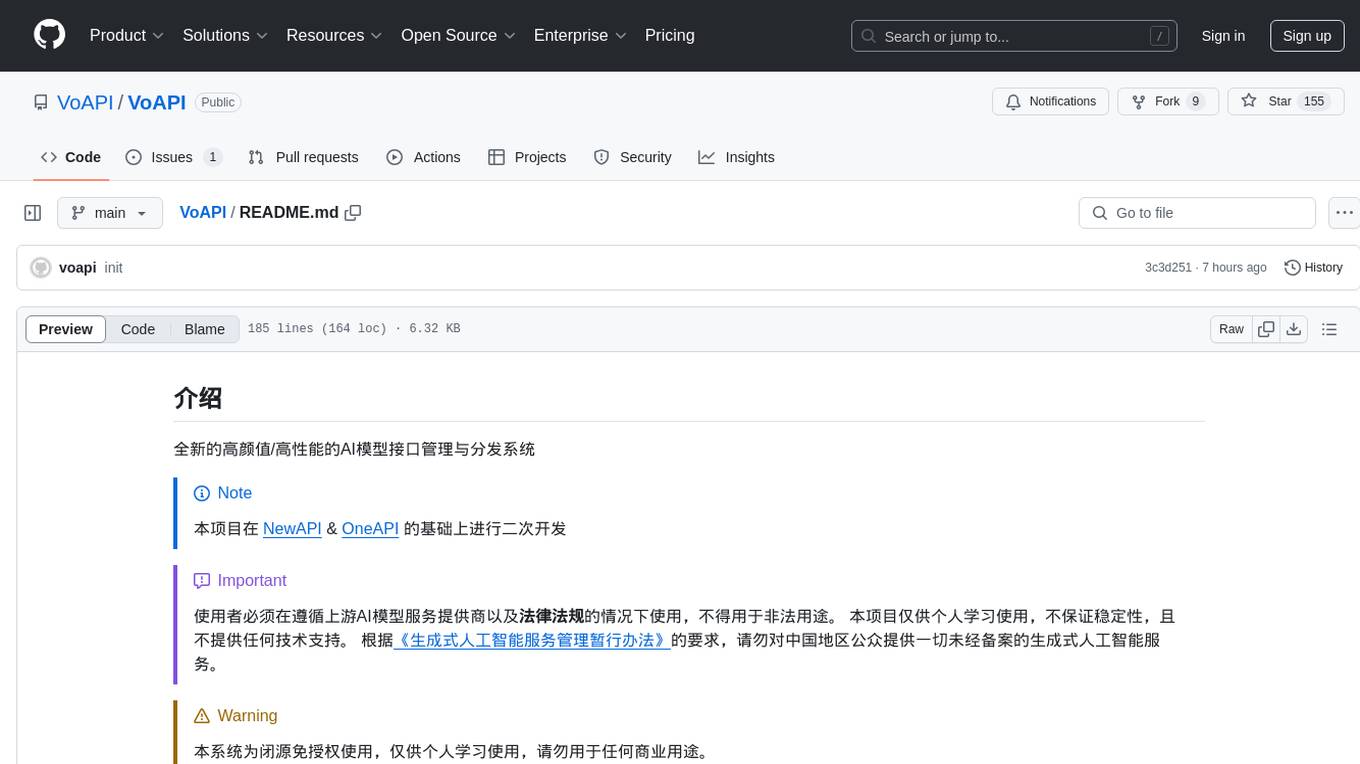
VoAPI
VoAPI is a new high-value/high-performance AI model interface management and distribution system. It is a closed-source tool for personal learning use only, not for commercial purposes. Users must comply with upstream AI model service providers and legal regulations. The system offers a visually appealing interface with features such as independent development documentation page support, service monitoring page configuration support, and third-party login support. Users can manage user registration time, optimize interface elements, and support features like online recharge, model pricing display, and sensitive word filtering. VoAPI also provides support for various AI models and platforms, with the ability to configure homepage templates, model information, and manufacturer information.