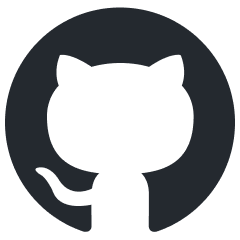
Chinese-Mixtral-8x7B
中文Mixtral-8x7B(Chinese-Mixtral-8x7B)
Stars: 635
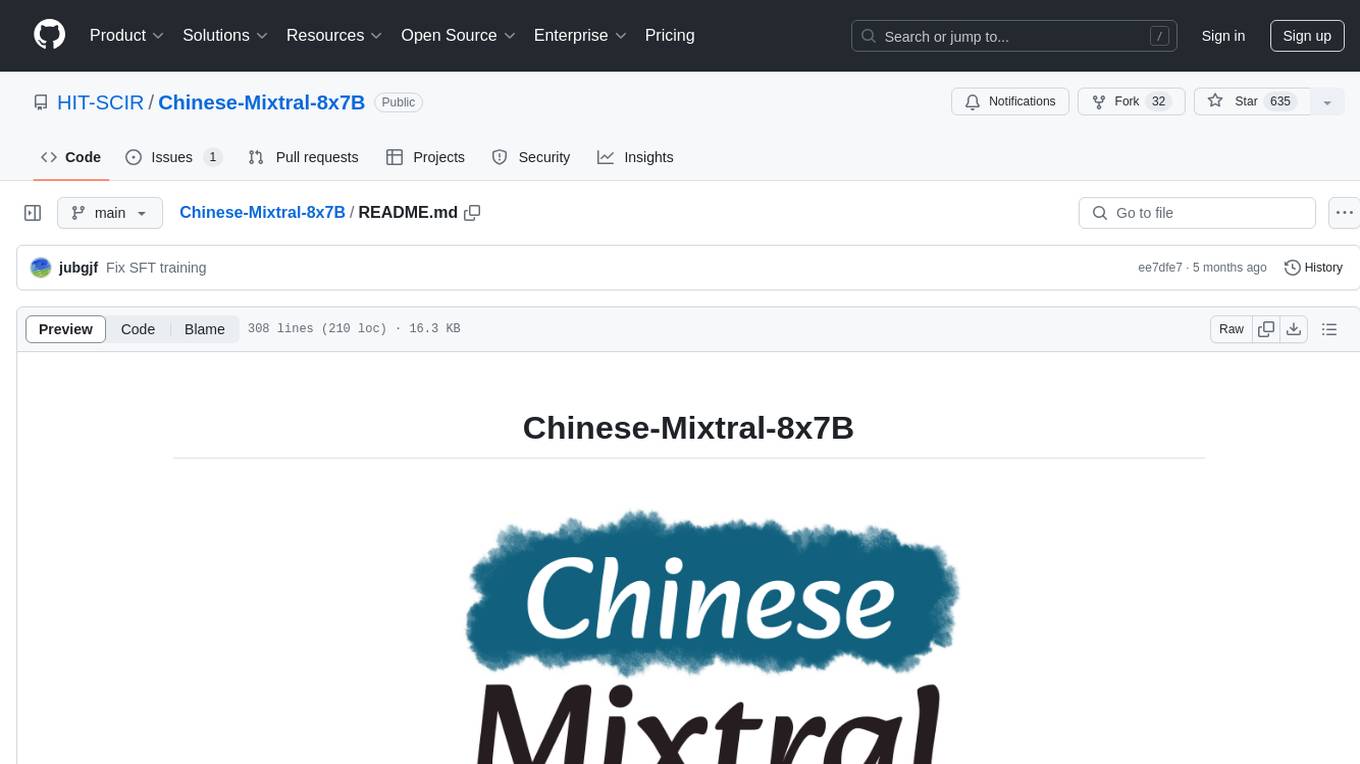
Chinese-Mixtral-8x7B is an open-source project based on Mistral's Mixtral-8x7B model for incremental pre-training of Chinese vocabulary, aiming to advance research on MoE models in the Chinese natural language processing community. The expanded vocabulary significantly improves the model's encoding and decoding efficiency for Chinese, and the model is pre-trained incrementally on a large-scale open-source corpus, enabling it with powerful Chinese generation and comprehension capabilities. The project includes a large model with expanded Chinese vocabulary and incremental pre-training code.
README:
- [2024-02-09] 发布基于Chinese-Mixtral-8x7B指令微调的模型:活字 3.0;开源指令微调代码。
- [2024-01-18] 发布Chinese-Mixtral-8x7B基座模型;开源增量预训练代码。
本项目基于Mistral发布的模型Mixtral-8x7B进行了中文扩词表增量预训练,希望进一步促进中文自然语言处理社区对MoE模型的研究。我们扩充后的词表显著提高了模型对中文的编解码效率,并通过大规模开源语料对扩词表模型进行增量预训练,使模型具备了强大的中文生成和理解能力。
项目开源内容:
- 中文Mixtral-8x7B扩词表大模型
- 扩词表增量预训练代码
请注意,Chinese-Mixtral-8x7B仍然可能生成包含事实性错误的误导性回复或包含偏见/歧视的有害内容,请谨慎鉴别和使用生成的内容,请勿将生成的有害内容传播至互联网。
本项目使用QLoRA进行训练,LoRA权重与合并权重后的模型分别开源,您可以根据自己的需求选择下载:
模型名称 | 模型大小 | 下载地址 | 备注 |
---|---|---|---|
Chinese-Mixtral-8x7B | 88GB |
HuggingFace ModelScope |
中文扩词表完整模型,可以直接使用 |
Chinese-Mixtral-8x7B-adapter | 2.7GB | HuggingFace | LoRA权重,需要与原版Mixtral-8x7B进行合并才可以使用,合并脚本请参考这里 |
Chinese-Mixtral-8x7B支持完整的Mixtral-8x7B模型生态,包括使用vLLM
、Flash Attention 2
进行加速,使用bitsandbytes
进行模型量化等。以下是使用Chinese-Mixtral-8x7B进行推理的代码示例。
使用Flash Attention 2:
import torch
from transformers import AutoModelForCausalLM, AutoTokenizer
model_id = "HIT-SCIR/Chinese-Mixtral-8x7B"
tokenizer = AutoTokenizer.from_pretrained(model_id)
model = AutoModelForCausalLM.from_pretrained(model_id, attn_implementation="flash_attention_2", torch_dtype=torch.bfloat16, device_map="auto")
text = "我的名字是"
inputs = tokenizer(text, return_tensors="pt").to(0)
outputs = model.generate(**inputs, max_new_tokens=20)
print(tokenizer.decode(outputs[0], skip_special_tokens=True))
使用4bit量化:
import torch
from transformers import AutoModelForCausalLM, AutoTokenizer
model_id = "HIT-SCIR/Chinese-Mixtral-8x7B"
tokenizer = AutoTokenizer.from_pretrained(model_id)
model = AutoModelForCausalLM.from_pretrained(model_id, load_in_4bit=True, device_map="auto")
text = "我的名字是"
inputs = tokenizer(text, return_tensors="pt").to(0)
outputs = model.generate(**inputs, max_new_tokens=20)
print(tokenizer.decode(outputs[0], skip_special_tokens=True))
请注意,Chinese-Mixtral-8x7B为基座模型,没有经过指令微调,因此指令遵循能力有限。您可以参考微调一节对模型进行微调。
我们分别使用以下评测数据集对Chinese-Mixtral-8x7B进行评测:
- C-Eval:一个全面的中文基础模型评估套件。它包含了13948个多项选择题,涵盖了52个不同的学科和四个难度级别。
- CMMLU:一个综合性的中文评估基准,专门用于评估语言模型在中文语境下的知识和推理能力,涵盖了从基础学科到高级专业水平的67个主题。
- MMLU:一个包含57个多选任务的英文评测数据集,涵盖了初等数学、美国历史、计算机科学、法律等,难度覆盖高中水平到专家水平,是目前主流的LLM评测数据集之一。
- HellaSwag:一个极具挑战的英文NLI评测数据集,每一个问题都需要对上下文进行深入理解,而不能基于常识进行回答。
根据Mistral发布的技术报告,Mixtral-8x7B在推理时将激活13B参数。下表为Chinese-Mixtral-8x7B与其他13B规模的中文扩词表模型在各个评测数据集上的5-shot结果:
模型名称 | 增量训练语料 | C-Eval (中文) |
CMMLU (中文) |
MMLU (英文) |
HellaSwag (英文) |
---|---|---|---|---|---|
IDEA-CCNL/Ziya2-13B-Base | 650B Token | 59.29 | 60.93 | 59.86 | 58.90 |
TigerResearch/tigerbot-13b-base-v3 | 500B Token | 50.52 | 51.65 | 53.46 | 59.16 |
Linly-AI/Chinese-LLaMA-2-13B-hf | 11B Token | 42.57 | 41.95 | 51.32 | 59.05 |
hfl/chinese-llama-2-13b | 约30B Token(120GB) | 41.90 | 42.08 | 51.92 | 59.28 |
Chinese-Mixtral-8x7B(本项目) | 42B Token | 52.08 | 51.08 | 69.80 | 65.69 |
在中文知识和理解方面,我们的Chinese-Mixtral-8x7B与TigerBot-13B-Base-v3性能相当。由于Chinese-Mixtral-8x7B的训练数据量仅为TigerBot-13B-Base-v3的8%,我们的模型仍有进一步提升的空间。与此同时,得益于原版Mixtral-8x7B模型强大的性能,我们的Chinese-Mixtral-8x7B达到了各个扩词表模型的最强英文水平。
由于不同版本的评测脚本实现细节有细微差异,为了保证评测结果的一致性和公平性,我们的评测脚本统一使用EleutherAI发布的lm-evaluation-harness,commit hash为28ec7fa。
下表为各个扩词表模型的生成效果。由于部分模型的预训练语料未使用eos_token
进行分隔,我们采用了max_tokens = 100
对生成文本进行截断。我们的采样参数为temperature = 0.8, top_p = 0.9
。
针对中文编解码效率,我们使用各个扩词表模型的分词器对SkyPile数据集的一个切片(2023-06_zh_head_0000.jsonl)进行编码,对比了各个分词器输出的中文文本Token量:
模型名称 | 模型类别 | 词表大小 | 中文文本Token量 | 编解码效率 |
---|---|---|---|---|
meta-llama/Llama-2-13B-hf | LLaMA | 32000 | 780M | 低 |
mistralai/Mixtral-8x7B-v0.1 | Mixtral | 32000 | 606M | 低 |
Linly-AI/Chinese-LLaMA-2-13B-hf | LLaMA | 40076 | 532M | 中 |
IDEA-CCNL/Ziya2-13B-Base | LLaMA | 39424 | 532M | 中 |
hfl/chinese-llama-2-13b | LLaMA | 55296 | 365M | 高 |
TigerResearch/tigerbot-13b-base-v3 | LLaMA | 65112 | 342M | 高 |
Chinese-Mixtral-8x7B(本项目) | Mixtral | 57000 | 355M | 高 |
在约1.4GB的测试文本中,我们的Chinese-Mixtral-8x7B中文编解码效率仅次于TigerBot-13B-Base-v3,较原模型提高了41.5%。这有利于加速中文文本的推理速度,并在In-Context Learning、Chain-of-Thought等场景中节省序列长度,有利于提高复杂推理任务的性能。
我们使用sentencepiece
在12G知乎数据和2G悟道数据上训练中文BPE词表。我们在训练词表时分别枚举了中文单字Token数量以及中文总Token数量,并对二者进行组合,得到了数百个大小、内容各异的词表。为了得到最适合的词表,我们通过Zheng Bo等人提出的ALP计算这些词表的中文词汇能力。ALP通过计算特定语言的子词切分粒度,并对词表的中低频子词进行惩罚,是一种方便快捷的衡量特定语言词汇能力的指标。
我们在书籍和百科语料上评估了不同词表的ALP值。图示中,四条曲线分别代表四种中文单字Token数量的词表(4451、5435、6414和7434)。为了避免词表过小导致中文压缩率过低,以及词表过大导致embedding层过于稀疏,我们选取ALP曲线的拐点,对应向词表中新增25000个中文Token。在此基础上,我们选择了四条曲线中ALP最大者,即新增6414个中文单字Token的词表,作为最终Chinese-Mixtral-8x7B选用的词表。
在获得新词表后,我们需要对embedding和lm_head层进行扩充和初始化。我们使用新Token在旧embedding层中的词嵌入平均值对扩充部分进行初始化。在我们的前期实验中,这种方法略优于HuggingFace的默认实现,即使用固定的正态分布进行初始化。
Mixtral-8x7B模型参数量为46.7B,全参数训练需要同时使用多种并行策略,在训练资源受限的情况下时间成本过高。因此我们采用HuggingFace官方推荐的方法,使用QLoRA对模型进行训练。QLoRA在LoRA低秩分解的基础上,通过引入4位量化、双重量化和利用NVIDIA统一内存进行分页,进一步减少了训练所需显存,同时保持了与全参数训练相当的性能。
我们参考Yiming Cui等人对LoRA的设置,对原模型所有Linear层应用低秩分解,并将扩增后的embedding和lm_head层的参数设置为可训练。对于模型主体,我们采用NF4格式进行量化,这种格式可以使得量化后的数据与量化前具有同等的数据分布,模型的权重信息损失更少。
我们建议使用Python 3.10 + torch 2.0.1
# Pytorch + Transformers
$ pip install torch==2.0.1 torchvision==0.15.2 torchaudio==2.0.2
$ pip install transformers==4.36.2 datasets evaluate peft accelerate gradio optimum sentencepiece trl
$ pip install jupyterlab scikit-learn pandas matplotlib tensorboard nltk rouge bitsandbytes fire
# CUDA Toolkit
$ conda install nvidia/label/cuda-11.7.1::cuda
# DeepSpeed
$ git clone https://github.com/microsoft/DeepSpeed.git
$ cd DeepSpeed
$ DS_BUILD_FUSED_ADAM=1 pip3 install .
# Flash Attention
$ pip install flash-attn --no-build-isolation
我们基于现有的开源数据集训练了Chinese-Mixtral-8x7B,数据集包括:
数据集名称 | 数据集语言 | 使用数据量 | 备注 |
---|---|---|---|
Skywork/SkyPile-150B | 中文 | 30B | 仅使用2022 + 2023年的数据 |
DKYoon/SlimPajama-6B | 英文 | 12B | 数据集重复2 Epoch |
通过data/download.py
将数据集下载到data
中。针对Slimpajama数据集,需要使用data/parquet2jsonl.py
将原始数据集转换为jsonl
格式。
下载后的数据集为多个jsonl文件的分片,使用cat
将多个分片合并为一个jsonl文件。
$ cat *.jsonl > all.jsonl
通过split
将jsonl切分为train和valid集合。本项目中train和valid的行数比例为999:1。
$ wc -l all.jsonl # 计算数据集总行数
$ split -l <lines> all.jsonl # 按999:1计算train/valid行数,进行切分
$ mv xaa DKYoon-SlimPajama-6B-train.jsonl # 重命名
$ mv xab DKYoon-SlimPajama-6B-dev.jsonl
将数据集名称和路径注册到data/datasets.toml
中:
[DKYoon-SlimPajama-6B] # 数据集名称
splits = ["train", "dev"] # 数据集train/valid集合
root = "{DATA_DIR}/en/{name}" # 数据集根目录
doc = "{name}-{split}" # 数据集文件名
encoded = "encoded-{name}-{split}" # 预处理保存位置
使用data/preprocess_datasets.py
对数据集进行子词切分,从而加快训练速度。
$ python data/preprocess_datasets.py --ds_name SkyPile-150B-2023 --tokenizer_name_or_path tokenizer/Mixtral-8x7B-v0.1-vocab
$ python data/preprocess_datasets.py --ds_name DKYoon-SlimPajama-6B --tokenizer_name_or_path tokenizer/Mixtral-8x7B-v0.1-vocab
在进行子词切分后,可以使用data/utils.py
查看各个数据集的token总量:
$ python data/utils.py
训练启动脚本为scripts/train.sh
。可以通过修改其中的TRAIN_DATASETS
修改训练数据集和数据集比例:
TRAIN_DATASETS=(
1:SkyPile-150B-2022 # 使用全量SkyPile-150B-2022
0.1:SkyPile-150B-2023 # 使用SkyPile-150B-2023的10%数据
1:DKYoon-SlimPajama-6B # 使用全量DKYoon-SlimPajama-6B
)
如果您使用SLURM集群管理系统,可以通过sbatch
进行提交:
$ sbatch scripts/train-pt.sh
如果没有SLURM或希望通过命令行启动训练,您可以直接提取scripts/train-pt.sh
中的torchrun
开始训练。
微调需要的数据集格式与预训练类似,数据集文件需要为jsonl格式:每行一个json,其中需要包含"text"
字段,将instruction、input和output全部按照您需要的模板进行拼接。
然后需要将数据集名称和路径注册到data/datasets.toml
中:
[ShareGPT-Chinese] # 数据集名称
splits = ["train"] # 数据集train/valid集合
root = "{DATA_DIR}/sft/{name}" # 数据集根目录
doc = "{name}-{split}" # 数据集文件名
训练启动脚本为scripts/train-sft.sh
。可以通过修改其中的TRAIN_DATASETS
修改训练数据集和数据集比例:
TRAIN_DATASETS=(
1.0:ShareGPT-Chinese # 使用全量ShareGPT-Chinese
0.5:ShareGPT-English # 使用ShareGPT-English的50%数据
)
如果您使用SLURM集群管理系统,可以通过sbatch
进行提交:
$ sbatch scripts/train-sft.sh
如果没有SLURM或希望通过命令行启动训练,您可以直接提取scripts/train-sft.sh
中的torchrun
开始训练。
如果您觉得本项目对您的研究有所帮助或使用了本项目的代码,请引用本项目:
@misc{Chinese-Mixtral-8x7B,
author = {HIT-SCIR},
title = {Chinese-Mixtral-8x7B: An Open-Source Mixture-of-Experts LLM},
year = {2024},
publisher = {GitHub},
journal = {GitHub repository},
howpublished = {\url{https://github.com/HIT-SCIR/Chinese-Mixtral-8x7B}}
}
For Tasks:
Click tags to check more tools for each tasksFor Jobs:
Alternative AI tools for Chinese-Mixtral-8x7B
Similar Open Source Tools
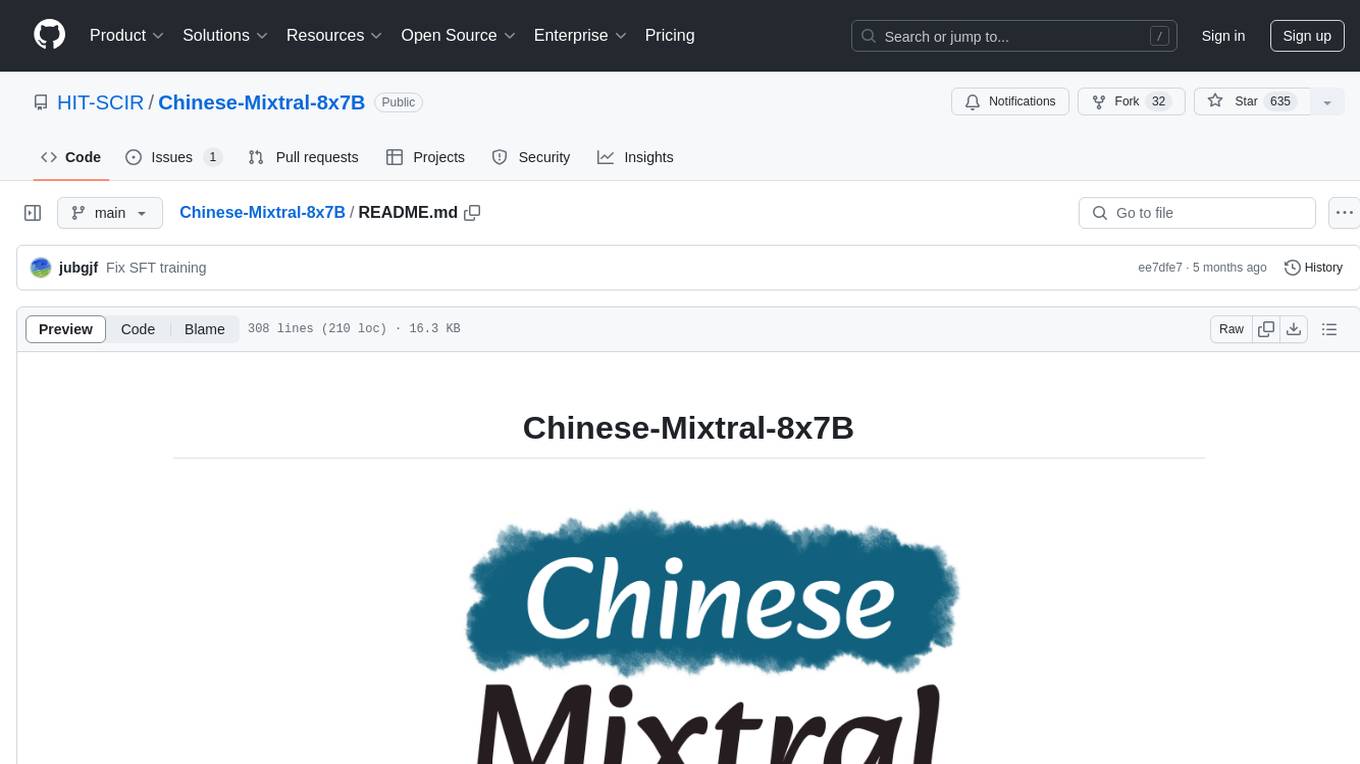
Chinese-Mixtral-8x7B
Chinese-Mixtral-8x7B is an open-source project based on Mistral's Mixtral-8x7B model for incremental pre-training of Chinese vocabulary, aiming to advance research on MoE models in the Chinese natural language processing community. The expanded vocabulary significantly improves the model's encoding and decoding efficiency for Chinese, and the model is pre-trained incrementally on a large-scale open-source corpus, enabling it with powerful Chinese generation and comprehension capabilities. The project includes a large model with expanded Chinese vocabulary and incremental pre-training code.
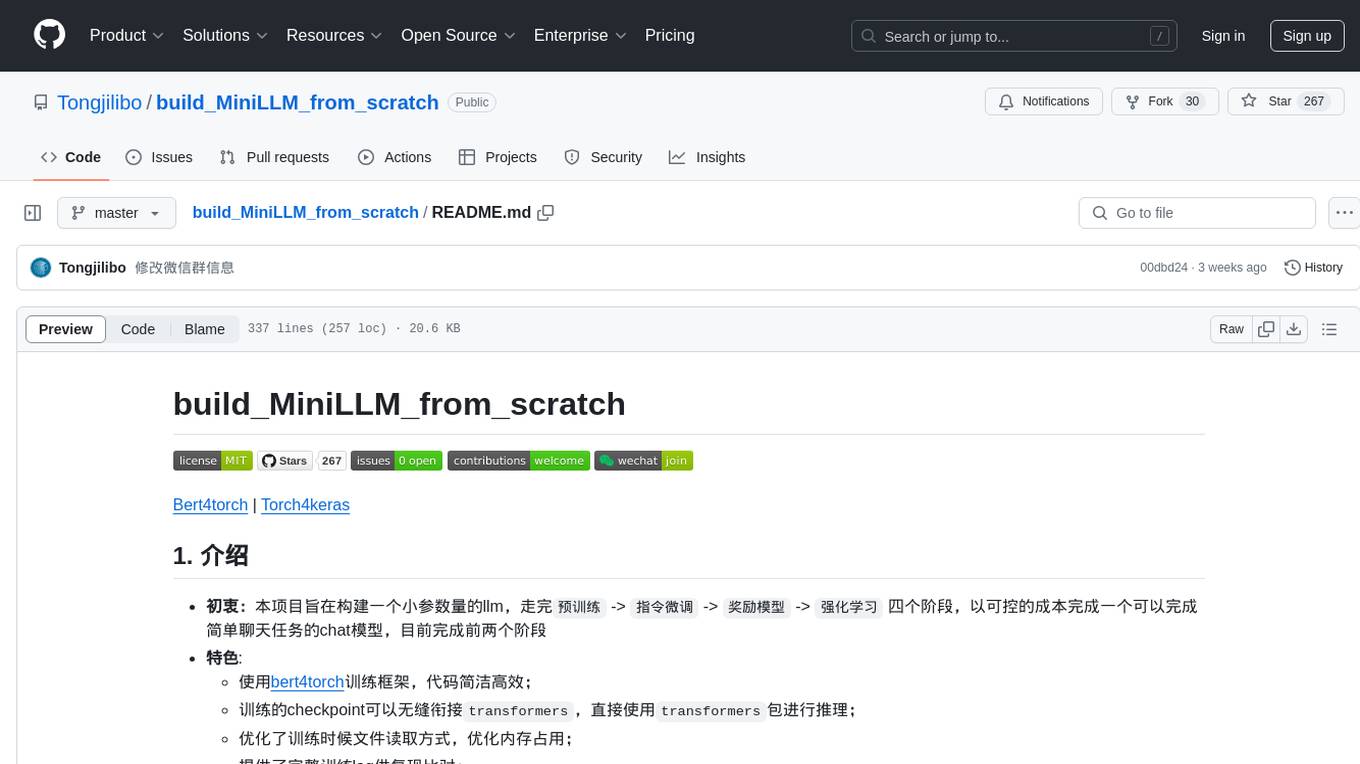
build_MiniLLM_from_scratch
This repository aims to build a low-parameter LLM model through pretraining, fine-tuning, model rewarding, and reinforcement learning stages to create a chat model capable of simple conversation tasks. It features using the bert4torch training framework, seamless integration with transformers package for inference, optimized file reading during training to reduce memory usage, providing complete training logs for reproducibility, and the ability to customize robot attributes. The chat model supports multi-turn conversations. The trained model currently only supports basic chat functionality due to limitations in corpus size, model scale, SFT corpus size, and quality.
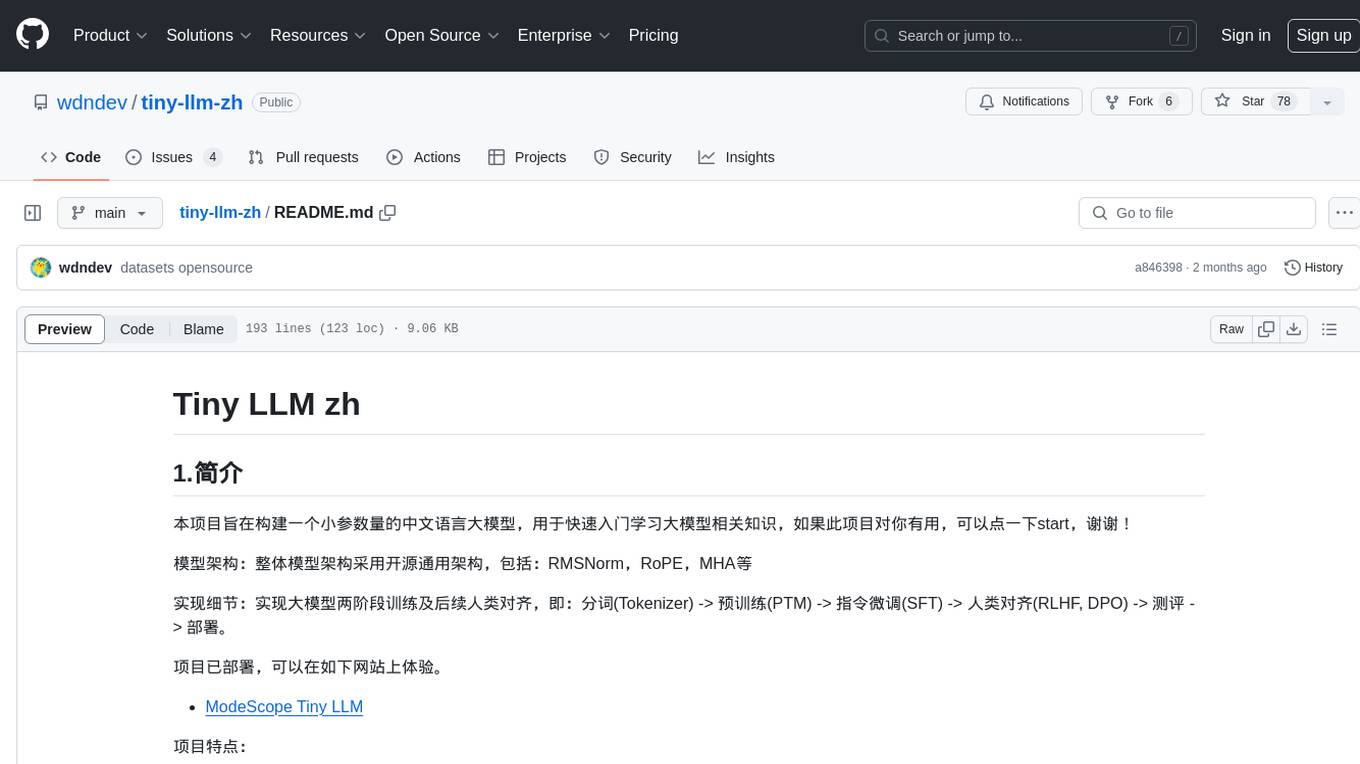
tiny-llm-zh
Tiny LLM zh is a project aimed at building a small-parameter Chinese language large model for quick entry into learning large model-related knowledge. The project implements a two-stage training process for large models and subsequent human alignment, including tokenization, pre-training, instruction fine-tuning, human alignment, evaluation, and deployment. It is deployed on ModeScope Tiny LLM website and features open access to all data and code, including pre-training data and tokenizer. The project trains a tokenizer using 10GB of Chinese encyclopedia text to build a Tiny LLM vocabulary. It supports training with Transformers deepspeed, multiple machine and card support, and Zero optimization techniques. The project has three main branches: llama2_torch, main tiny_llm, and tiny_llm_moe, each with specific modifications and features.
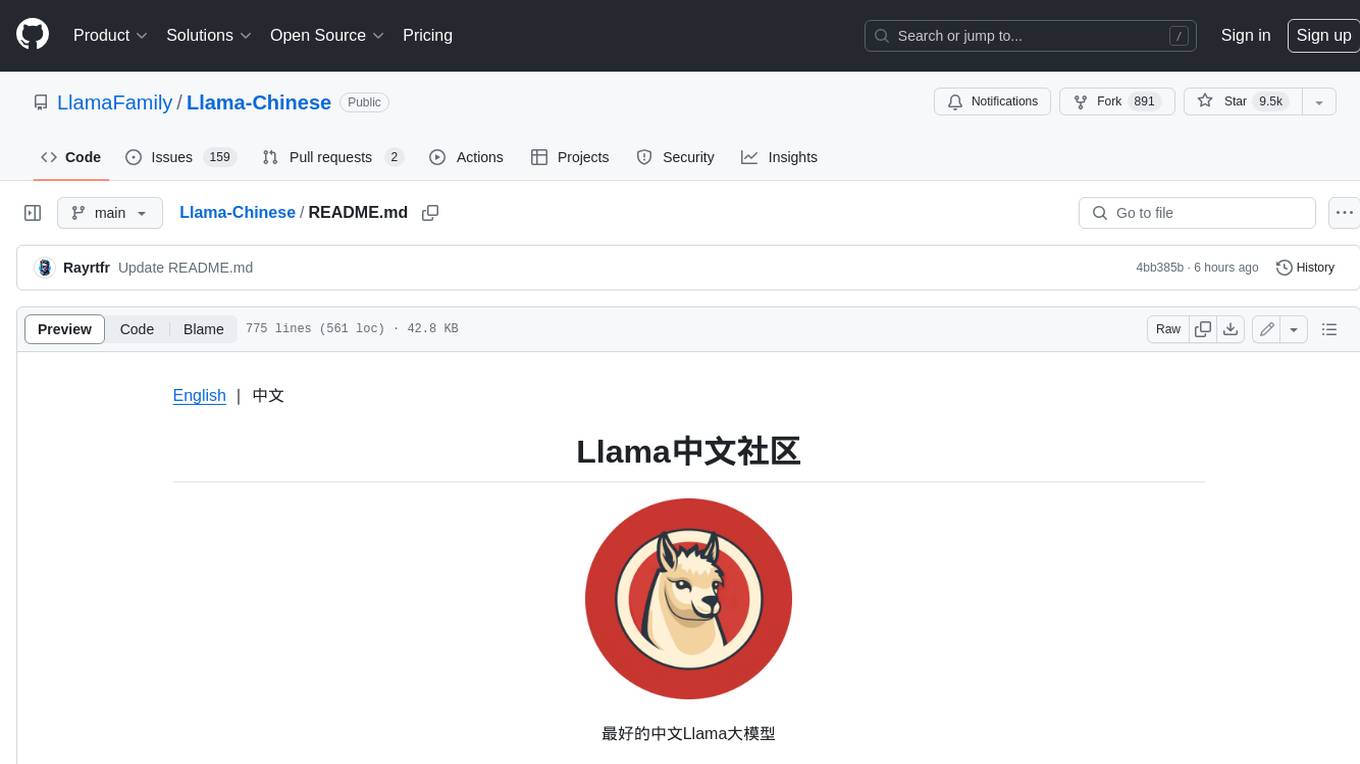
Llama-Chinese
Llama中文社区是一个专注于Llama模型在中文方面的优化和上层建设的高级技术社区。 **已经基于大规模中文数据,从预训练开始对Llama2模型进行中文能力的持续迭代升级【Done】**。**正在对Llama3模型进行中文能力的持续迭代升级【Doing】** 我们热忱欢迎对大模型LLM充满热情的开发者和研究者加入我们的行列。
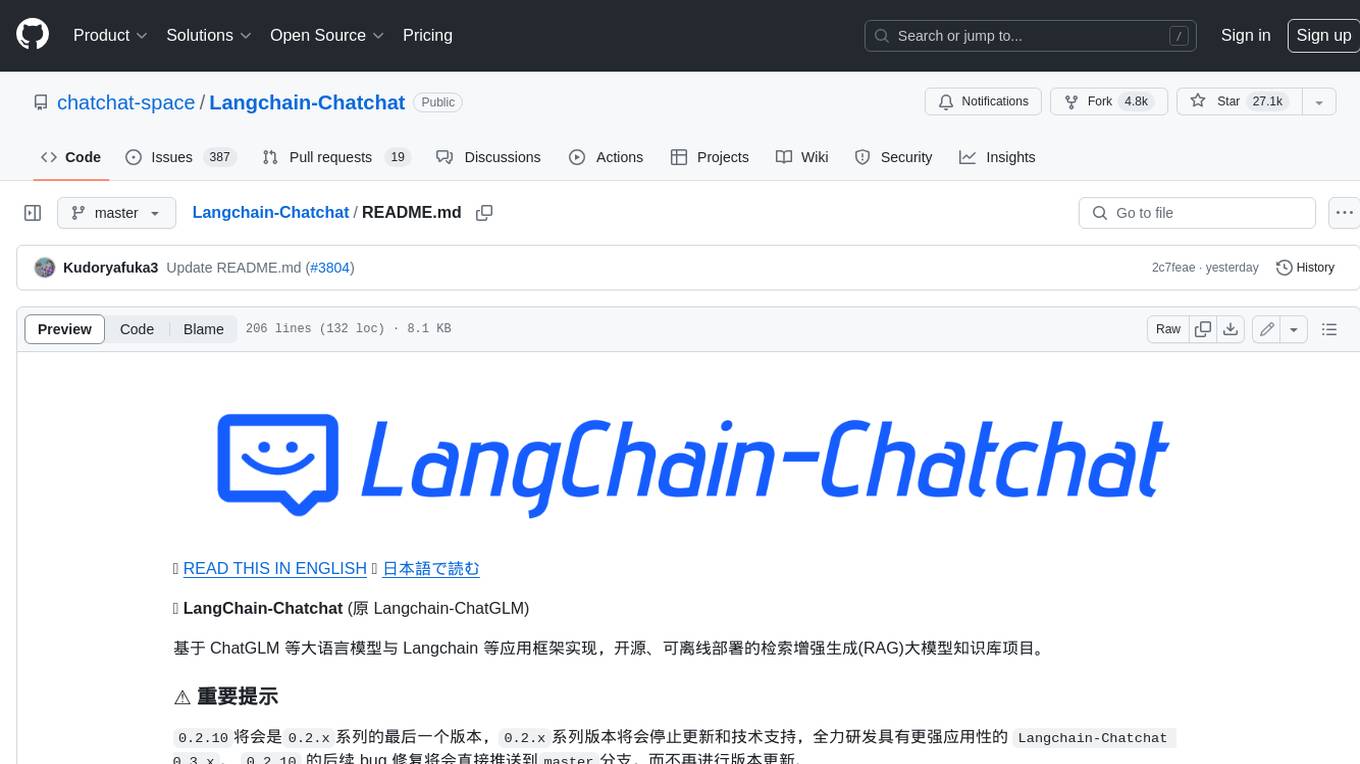
Langchain-Chatchat
LangChain-Chatchat is an open-source, offline-deployable retrieval-enhanced generation (RAG) large model knowledge base project based on large language models such as ChatGLM and application frameworks such as Langchain. It aims to establish a knowledge base Q&A solution that is friendly to Chinese scenarios, supports open-source models, and can run offline.
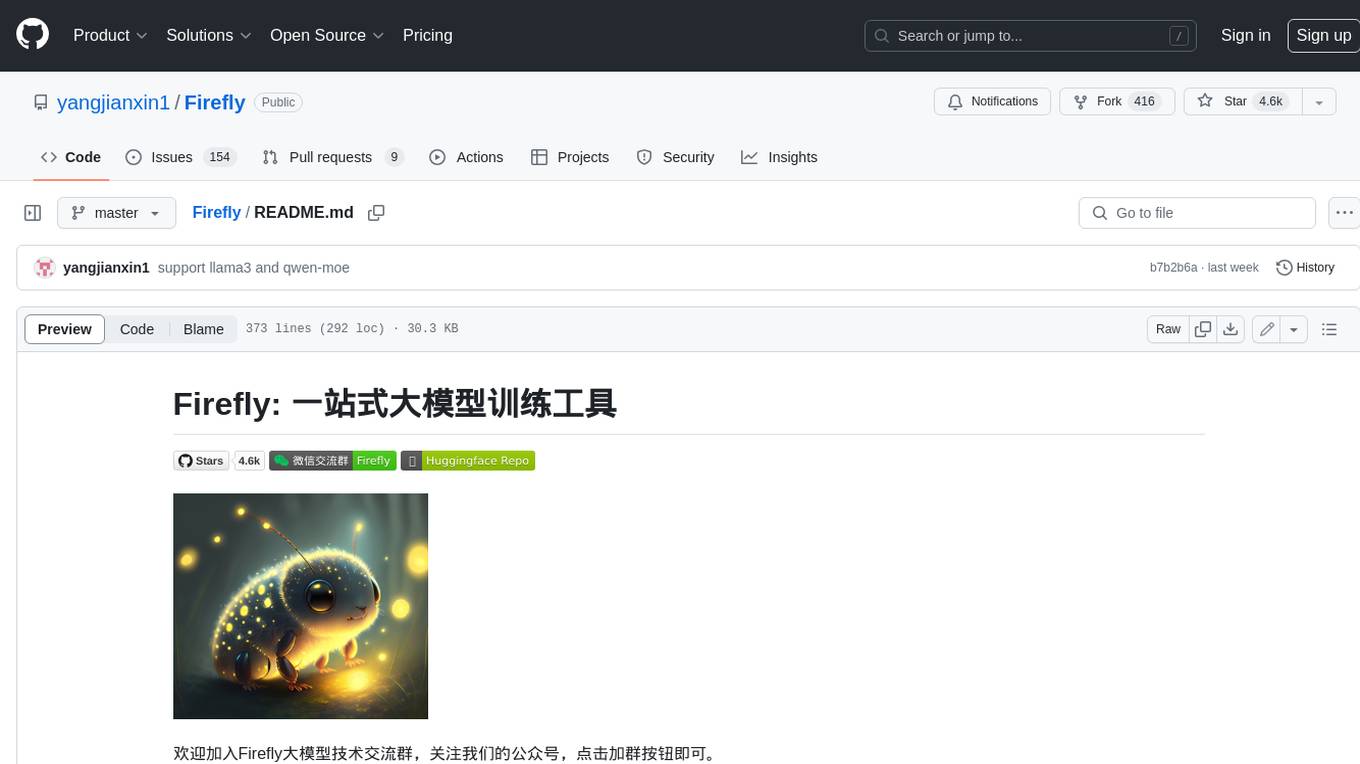
Firefly
Firefly is an open-source large model training project that supports pre-training, fine-tuning, and DPO of mainstream large models. It includes models like Llama3, Gemma, Qwen1.5, MiniCPM, Llama, InternLM, Baichuan, ChatGLM, Yi, Deepseek, Qwen, Orion, Ziya, Xverse, Mistral, Mixtral-8x7B, Zephyr, Vicuna, Bloom, etc. The project supports full-parameter training, LoRA, QLoRA efficient training, and various tasks such as pre-training, SFT, and DPO. Suitable for users with limited training resources, QLoRA is recommended for fine-tuning instructions. The project has achieved good results on the Open LLM Leaderboard with QLoRA training process validation. The latest version has significant updates and adaptations for different chat model templates.
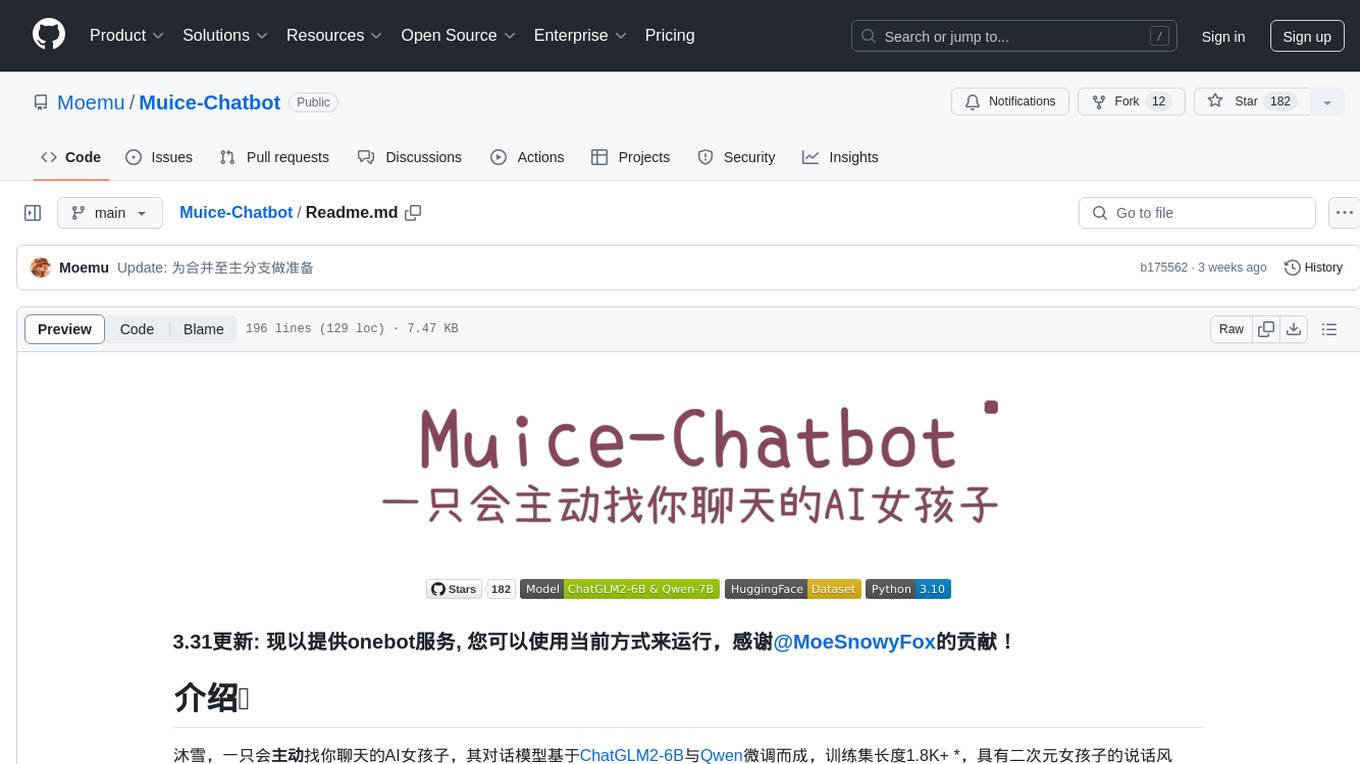
Muice-Chatbot
Muice-Chatbot is an AI chatbot designed to proactively engage in conversations with users. It is based on the ChatGLM2-6B and Qwen-7B models, with a training dataset of 1.8K+ dialogues. The chatbot has a speaking style similar to a 2D girl, being somewhat tsundere but willing to share daily life details and greet users differently every day. It provides various functionalities, including initiating chats and offering 5 available commands. The project supports model loading through different methods and provides onebot service support for QQ users. Users can interact with the chatbot by running the main.py file in the project directory.
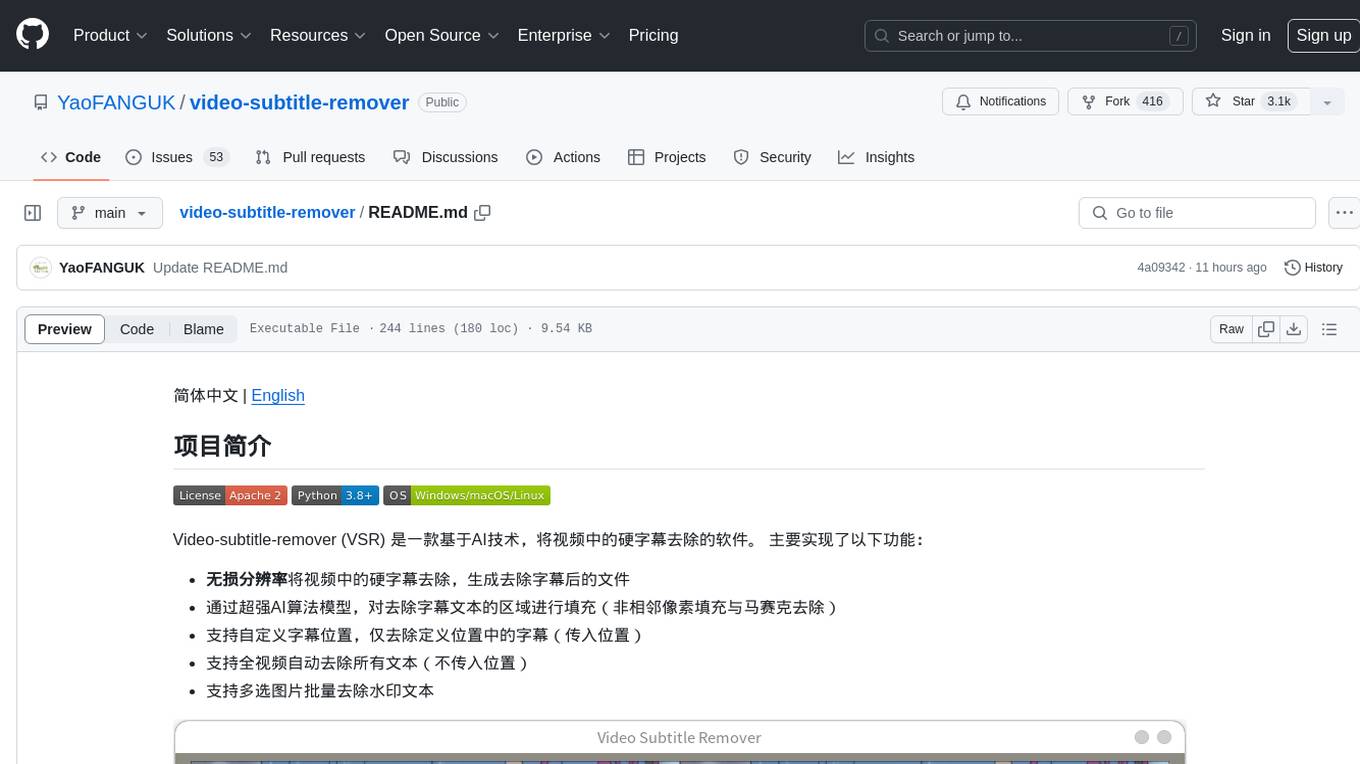
video-subtitle-remover
Video-subtitle-remover (VSR) is a software based on AI technology that removes hard subtitles from videos. It achieves the following functions: - Lossless resolution: Remove hard subtitles from videos, generate files with subtitles removed - Fill the region of removed subtitles using a powerful AI algorithm model (non-adjacent pixel filling and mosaic removal) - Support custom subtitle positions, only remove subtitles in defined positions (input position) - Support automatic removal of all text in the entire video (no input position required) - Support batch removal of watermark text from multiple images.
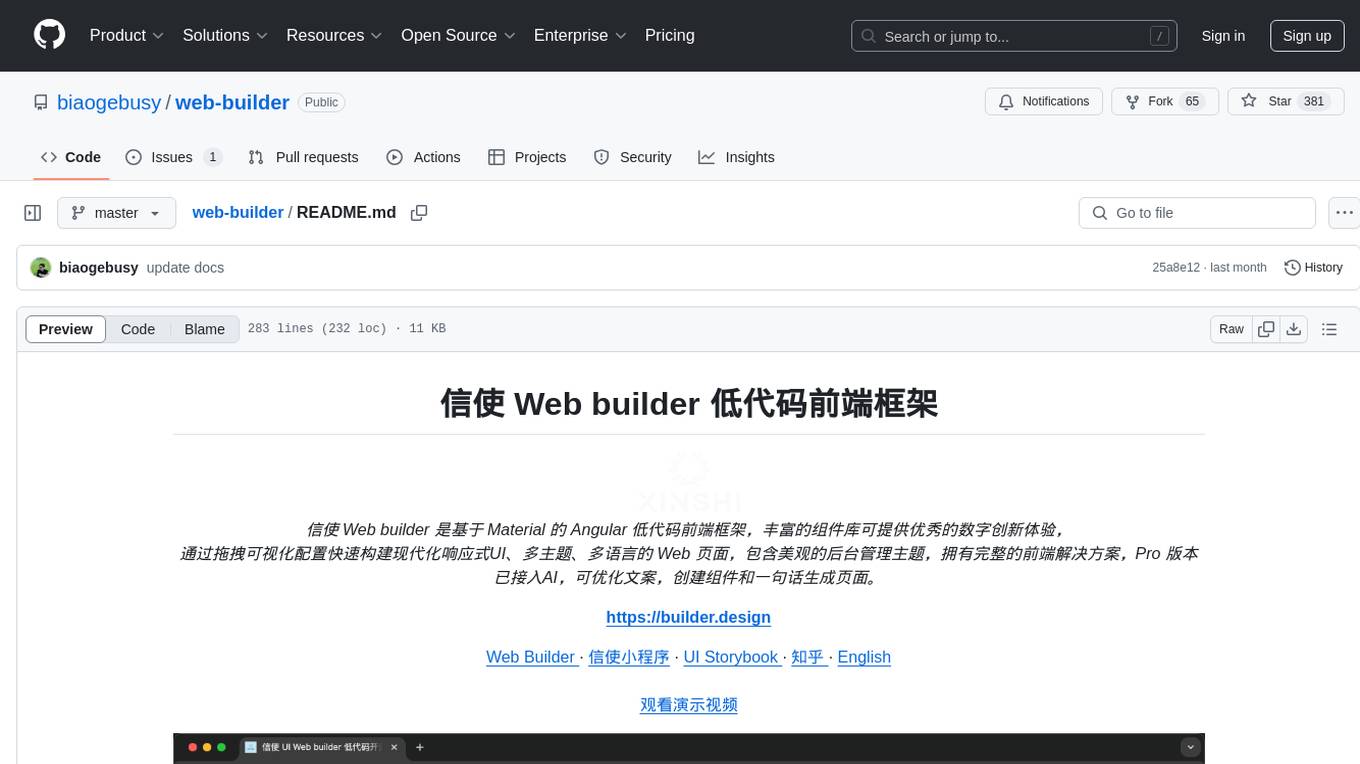
web-builder
Web Builder is a low-code front-end framework based on Material for Angular, offering a rich component library for excellent digital innovation experience. It allows rapid construction of modern responsive UI, multi-theme, multi-language web pages through drag-and-drop visual configuration. The framework includes a beautiful admin theme, complete front-end solutions, and AI integration in the Pro version for optimizing copy, creating components, and generating pages with a single sentence.
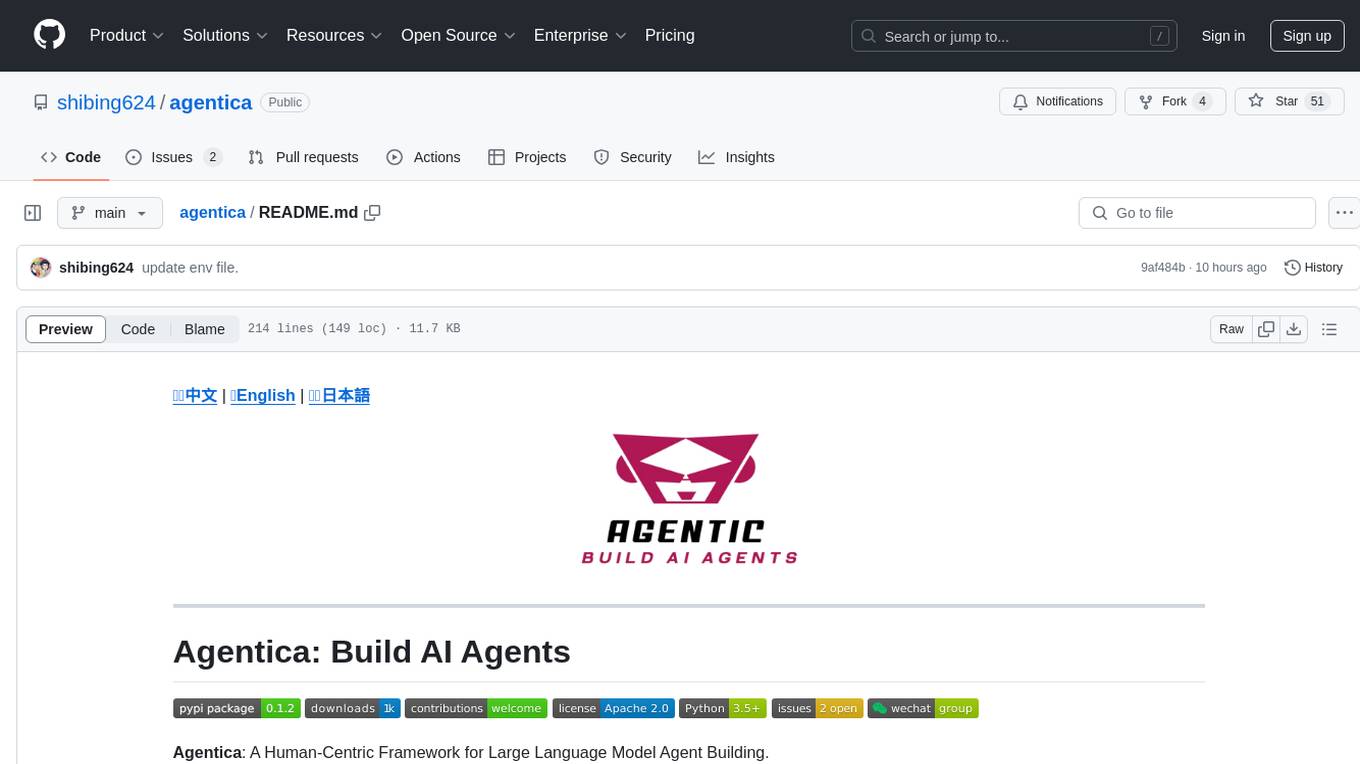
agentica
Agentica is a human-centric framework for building large language model agents. It provides functionalities for planning, memory management, tool usage, and supports features like reflection, planning and execution, RAG, multi-agent, multi-role, and workflow. The tool allows users to quickly code and orchestrate agents, customize prompts, and make API calls to various services. It supports API calls to OpenAI, Azure, Deepseek, Moonshot, Claude, Ollama, and Together. Agentica aims to simplify the process of building AI agents by providing a user-friendly interface and a range of functionalities for agent development.
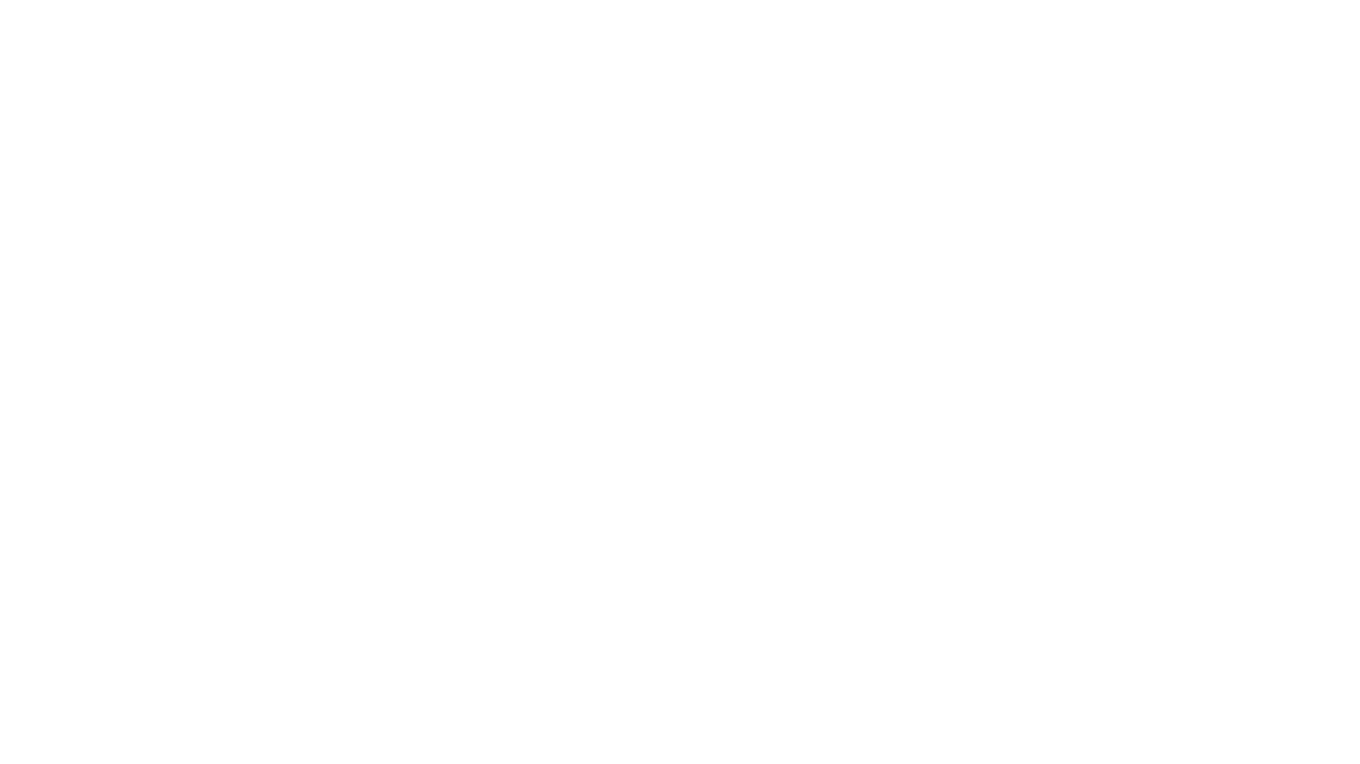
airbyte-connectors
This repository contains Airbyte connectors used in Faros and Faros Community Edition platforms as well as Airbyte Connector Development Kit (CDK) for JavaScript/TypeScript.
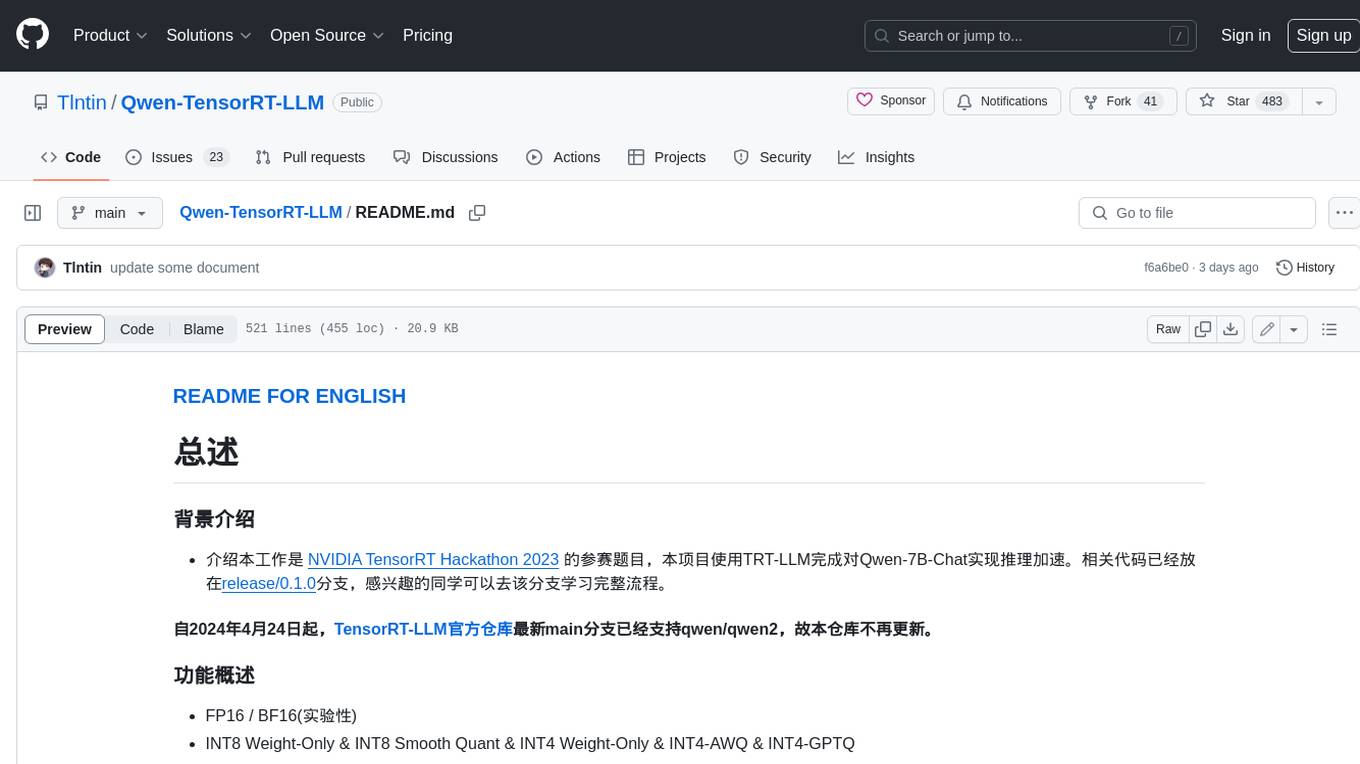
Qwen-TensorRT-LLM
Qwen-TensorRT-LLM is a project developed for the NVIDIA TensorRT Hackathon 2023, focusing on accelerating inference for the Qwen-7B-Chat model using TRT-LLM. The project offers various functionalities such as FP16/BF16 support, INT8 and INT4 quantization options, Tensor Parallel for multi-GPU parallelism, web demo setup with gradio, Triton API deployment for maximum throughput/concurrency, fastapi integration for openai requests, CLI interaction, and langchain support. It supports models like qwen2, qwen, and qwen-vl for both base and chat models. The project also provides tutorials on Bilibili and blogs for adapting Qwen models in NVIDIA TensorRT-LLM, along with hardware requirements and quick start guides for different model types and quantization methods.
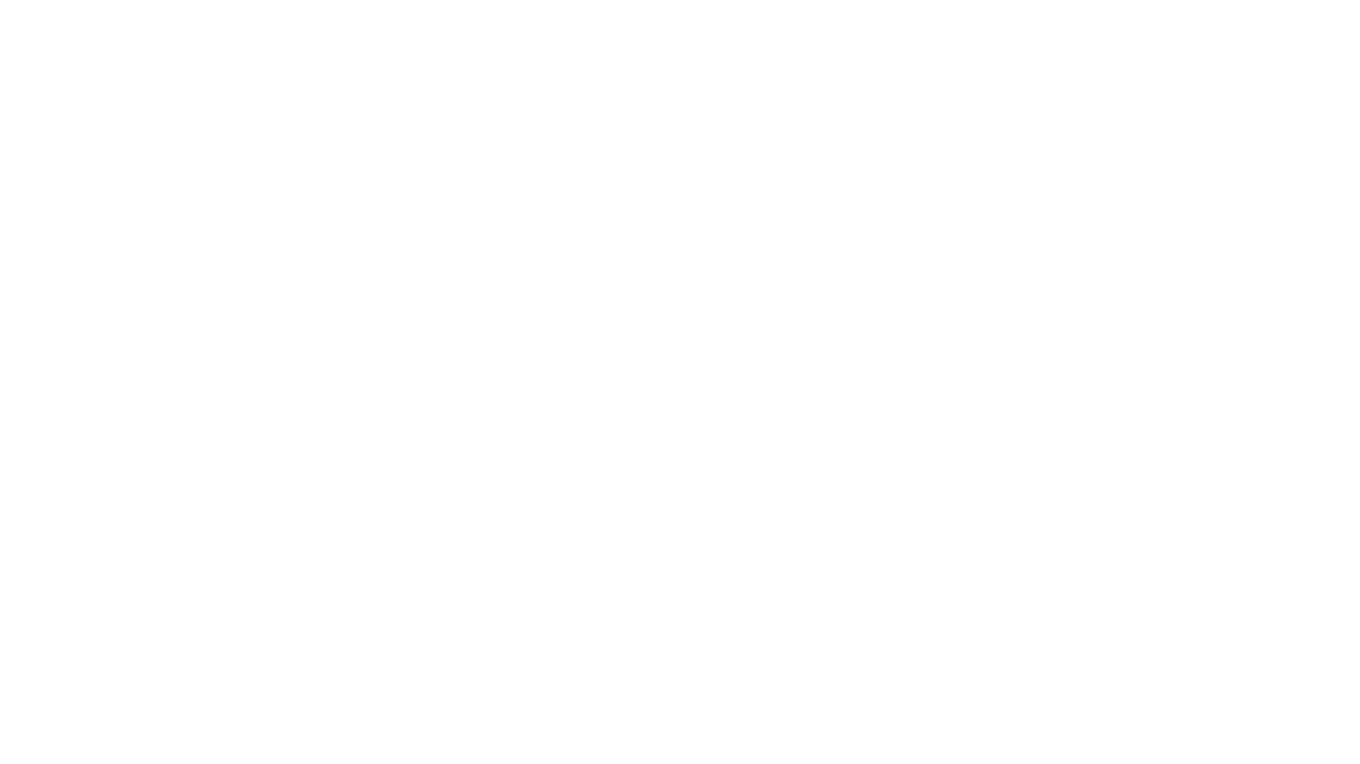
chatluna
Chatluna is a machine learning model plugin that provides chat services with large language models. It is highly extensible, supports multiple output formats, and offers features like custom conversation presets, rate limiting, and context awareness. Users can deploy Chatluna under Koishi without additional configuration. The plugin supports various models/platforms like OpenAI, Azure OpenAI, Google Gemini, and more. It also provides preset customization using YAML files and allows for easy forking and development within Koishi projects. However, the project lacks web UI, HTTP server, and project documentation, inviting contributions from the community.
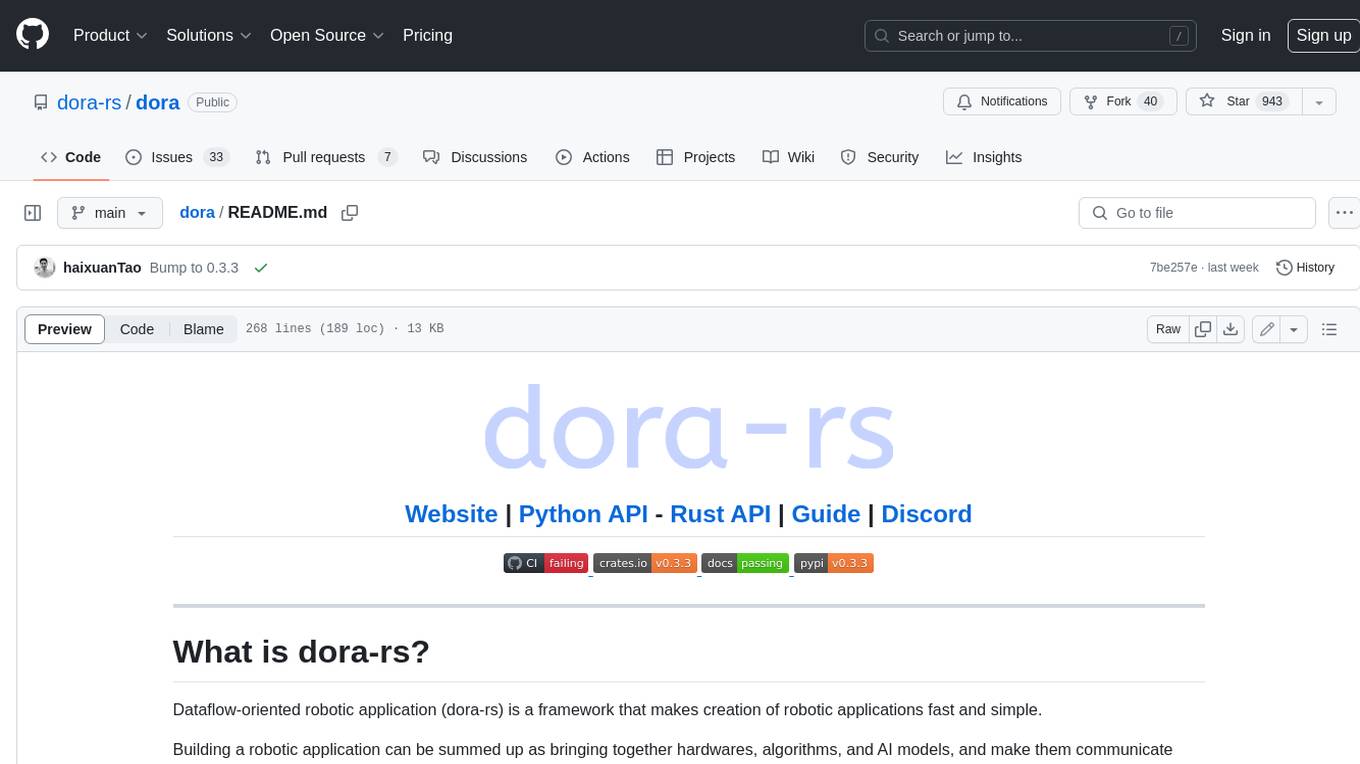
dora
Dataflow-oriented robotic application (dora-rs) is a framework that makes creation of robotic applications fast and simple. Building a robotic application can be summed up as bringing together hardwares, algorithms, and AI models, and make them communicate with each others. At dora-rs, we try to: make integration of hardware and software easy by supporting Python, C, C++, and also ROS2. make communication low latency by using zero-copy Arrow messages. dora-rs is still experimental and you might experience bugs, but we're working very hard to make it stable as possible.
For similar tasks
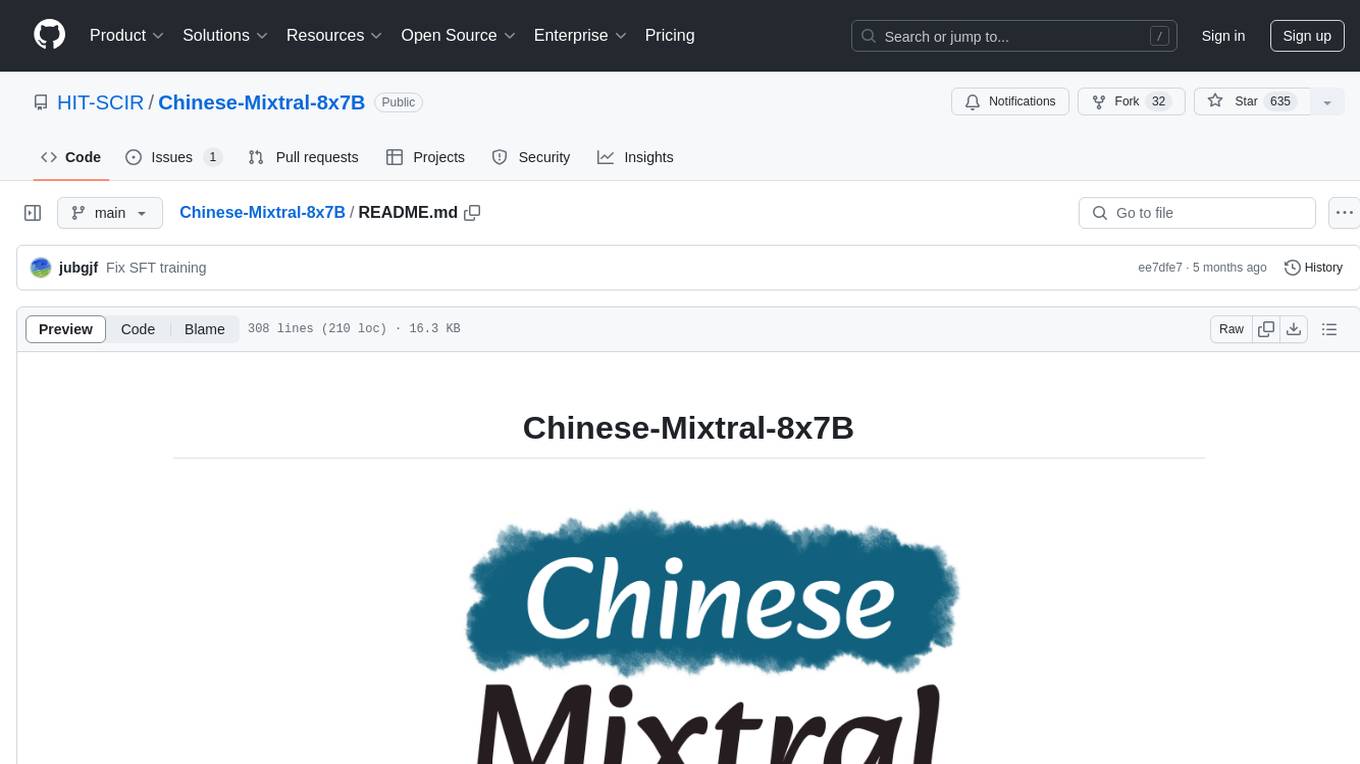
Chinese-Mixtral-8x7B
Chinese-Mixtral-8x7B is an open-source project based on Mistral's Mixtral-8x7B model for incremental pre-training of Chinese vocabulary, aiming to advance research on MoE models in the Chinese natural language processing community. The expanded vocabulary significantly improves the model's encoding and decoding efficiency for Chinese, and the model is pre-trained incrementally on a large-scale open-source corpus, enabling it with powerful Chinese generation and comprehension capabilities. The project includes a large model with expanded Chinese vocabulary and incremental pre-training code.
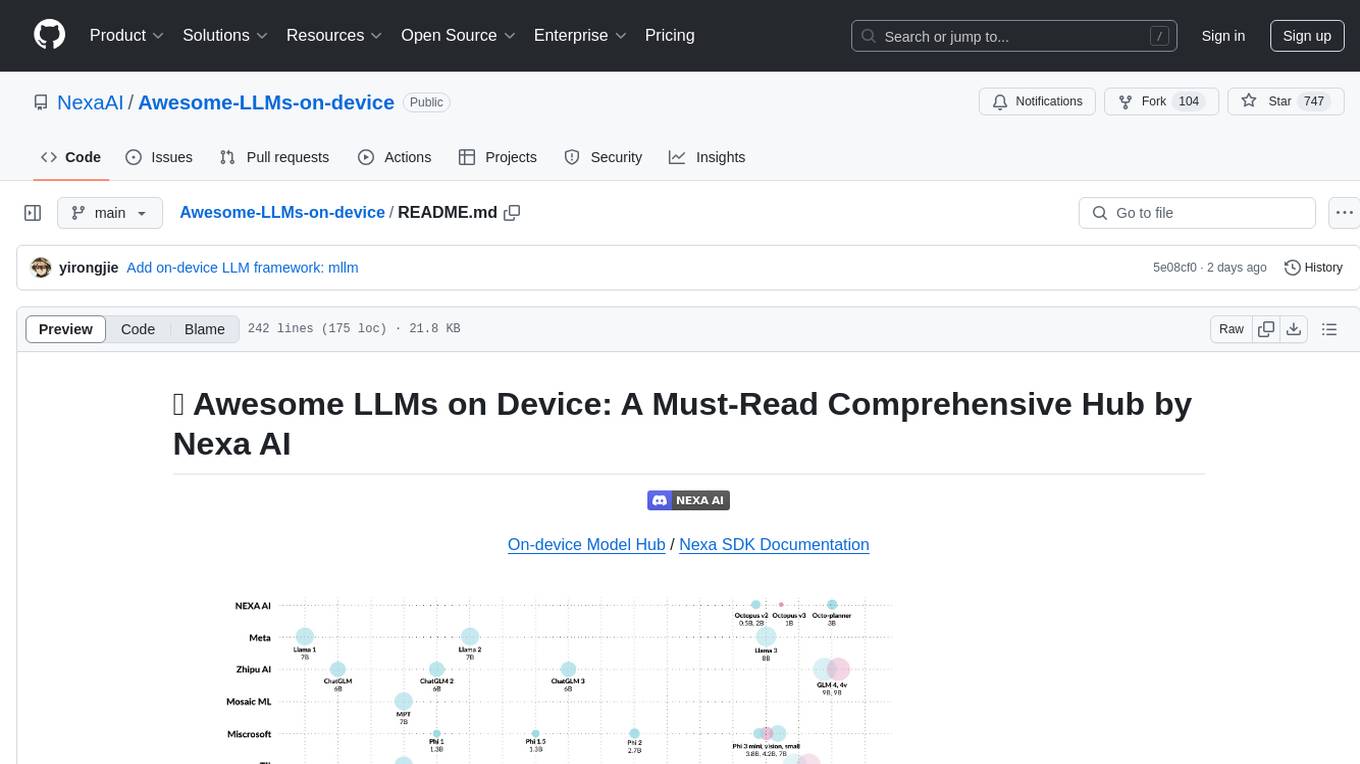
Awesome-LLMs-on-device
Welcome to the ultimate hub for on-device Large Language Models (LLMs)! This repository is your go-to resource for all things related to LLMs designed for on-device deployment. Whether you're a seasoned researcher, an innovative developer, or an enthusiastic learner, this comprehensive collection of cutting-edge knowledge is your gateway to understanding, leveraging, and contributing to the exciting world of on-device LLMs.
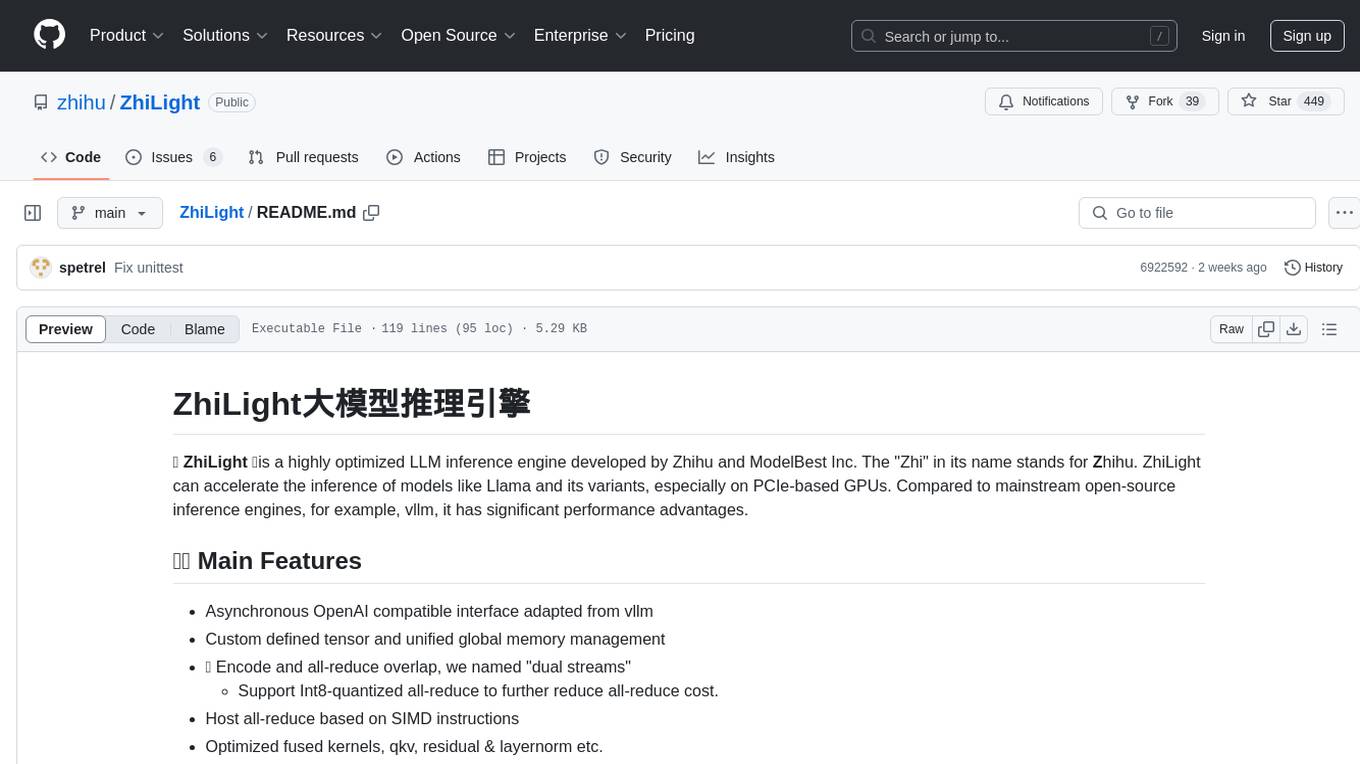
ZhiLight
ZhiLight is a highly optimized large language model (LLM) inference engine developed by Zhihu and ModelBest Inc. It accelerates the inference of models like Llama and its variants, especially on PCIe-based GPUs. ZhiLight offers significant performance advantages compared to mainstream open-source inference engines. It supports various features such as custom defined tensor and unified global memory management, optimized fused kernels, support for dynamic batch, flash attention prefill, prefix cache, and different quantization techniques like INT8, SmoothQuant, FP8, AWQ, and GPTQ. ZhiLight is compatible with OpenAI interface and provides high performance on mainstream NVIDIA GPUs with different model sizes and precisions.
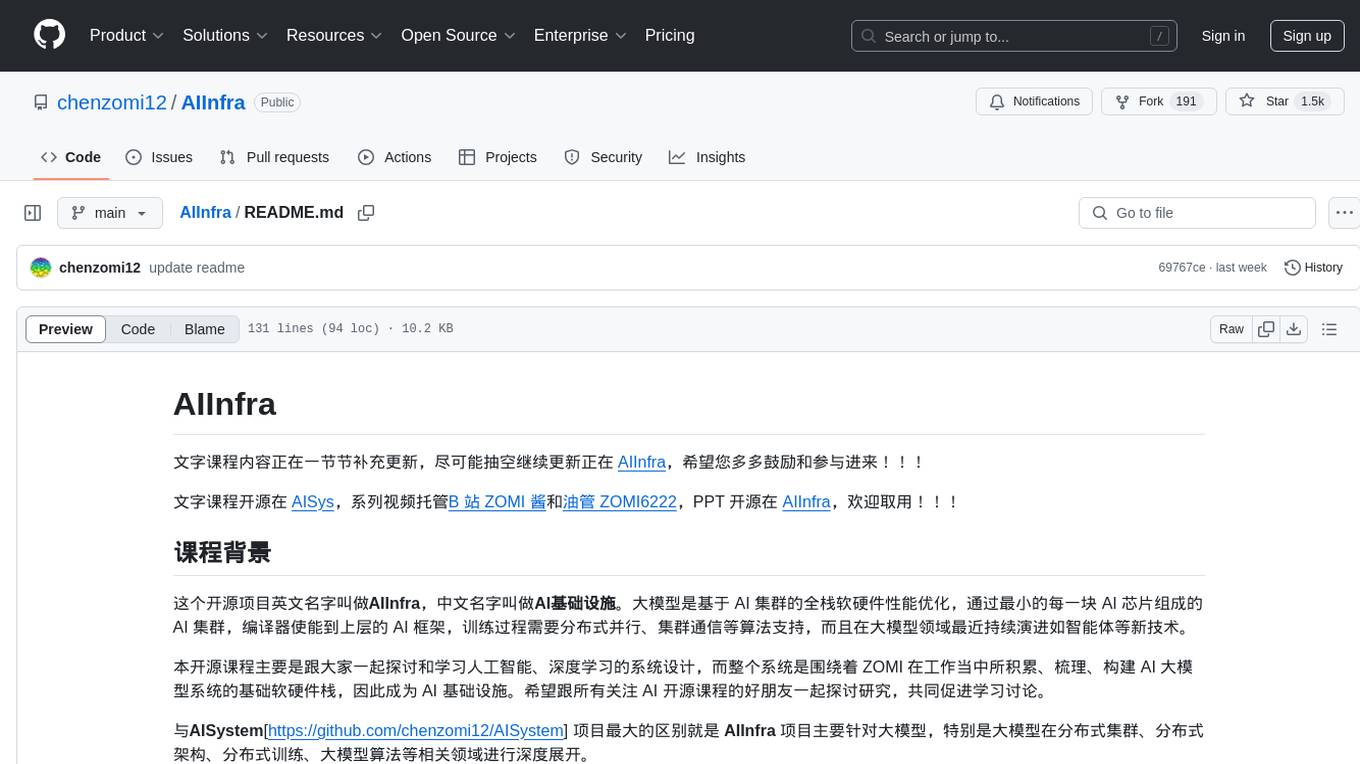
AIInfra
AIInfra is an open-source project focused on AI infrastructure, specifically targeting large models in distributed clusters, distributed architecture, distributed training, and algorithms related to large models. The project aims to explore and study system design in artificial intelligence and deep learning, with a focus on the hardware and software stack for building AI large model systems. It provides a comprehensive curriculum covering topics such as AI chip principles, communication and storage, AI clusters, large model training, and inference, as well as algorithms for large models. The course is designed for undergraduate and graduate students, as well as professionals working with AI large model systems, to gain a deep understanding of AI computer system architecture and design.
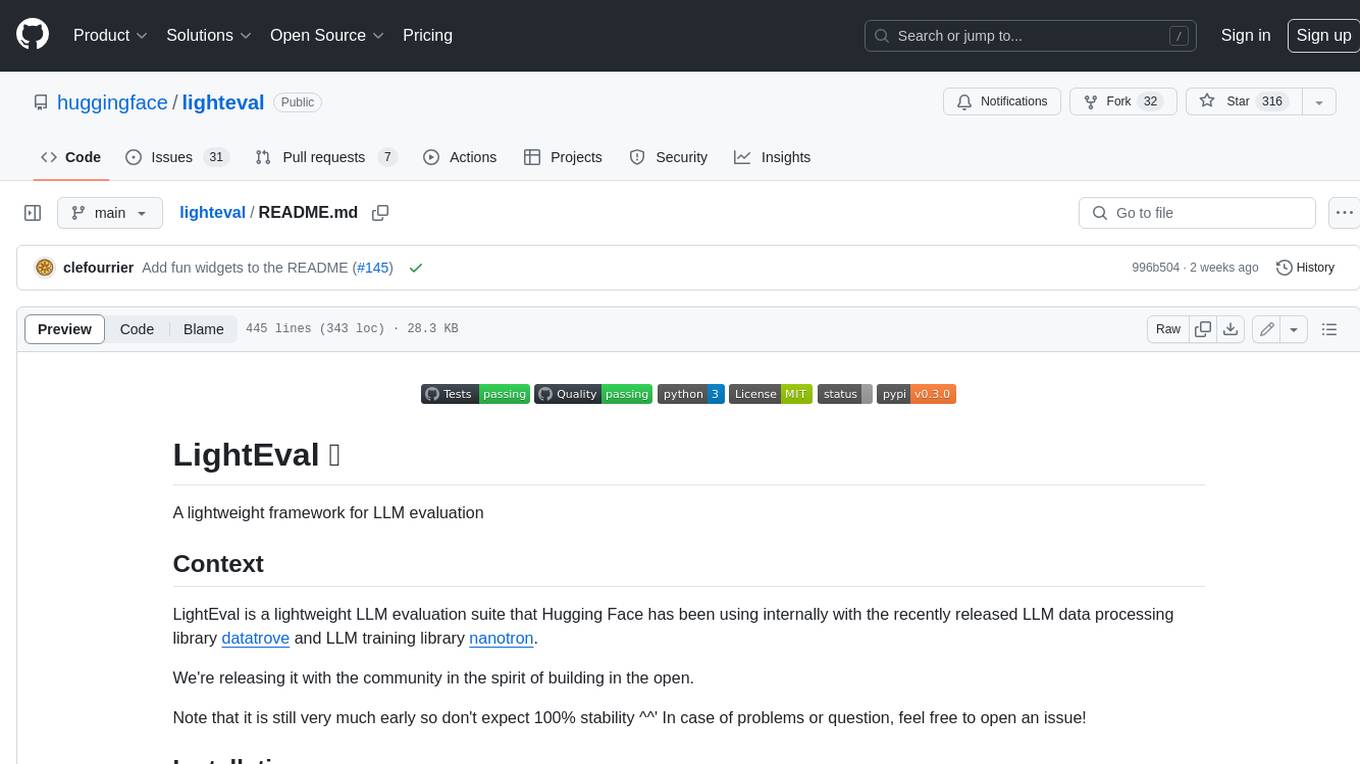
lighteval
LightEval is a lightweight LLM evaluation suite that Hugging Face has been using internally with the recently released LLM data processing library datatrove and LLM training library nanotron. We're releasing it with the community in the spirit of building in the open. Note that it is still very much early so don't expect 100% stability ^^' In case of problems or question, feel free to open an issue!
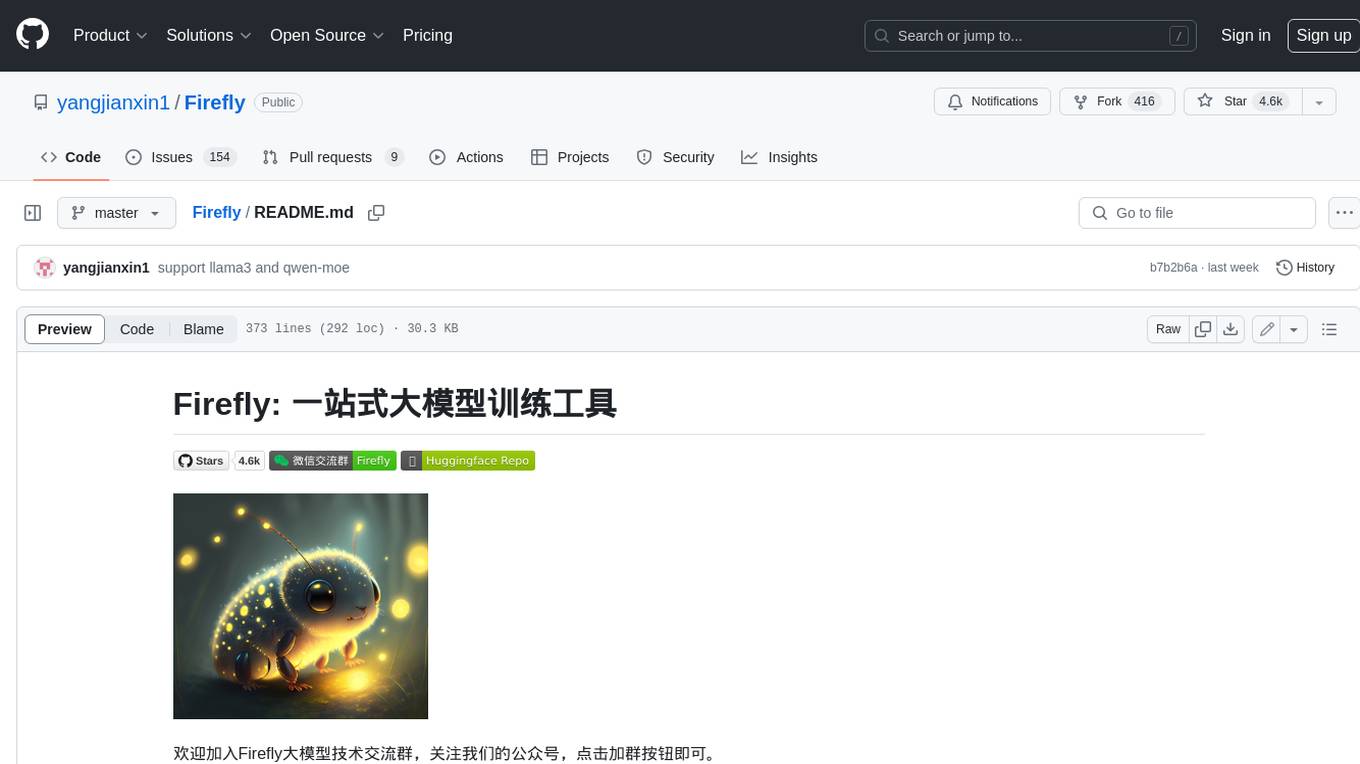
Firefly
Firefly is an open-source large model training project that supports pre-training, fine-tuning, and DPO of mainstream large models. It includes models like Llama3, Gemma, Qwen1.5, MiniCPM, Llama, InternLM, Baichuan, ChatGLM, Yi, Deepseek, Qwen, Orion, Ziya, Xverse, Mistral, Mixtral-8x7B, Zephyr, Vicuna, Bloom, etc. The project supports full-parameter training, LoRA, QLoRA efficient training, and various tasks such as pre-training, SFT, and DPO. Suitable for users with limited training resources, QLoRA is recommended for fine-tuning instructions. The project has achieved good results on the Open LLM Leaderboard with QLoRA training process validation. The latest version has significant updates and adaptations for different chat model templates.
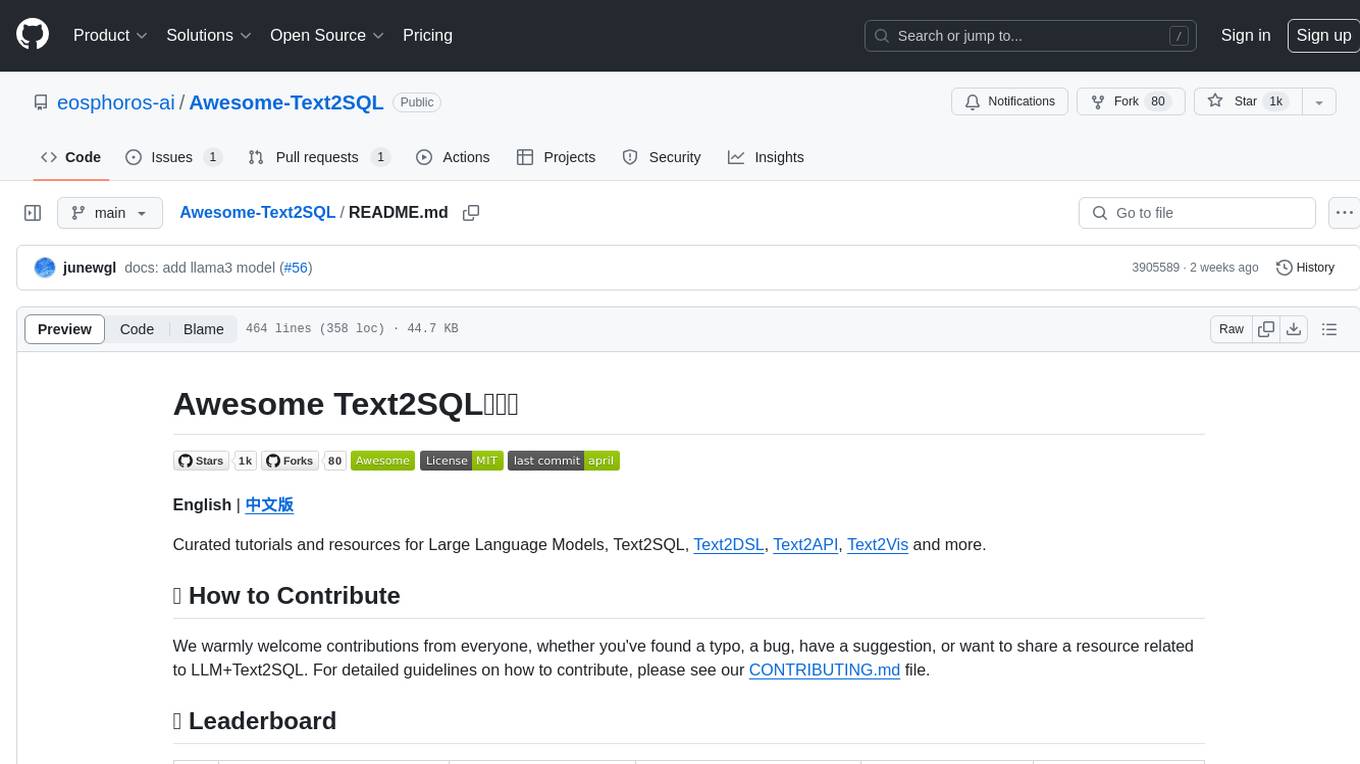
Awesome-Text2SQL
Awesome Text2SQL is a curated repository containing tutorials and resources for Large Language Models, Text2SQL, Text2DSL, Text2API, Text2Vis, and more. It provides guidelines on converting natural language questions into structured SQL queries, with a focus on NL2SQL. The repository includes information on various models, datasets, evaluation metrics, fine-tuning methods, libraries, and practice projects related to Text2SQL. It serves as a comprehensive resource for individuals interested in working with Text2SQL and related technologies.
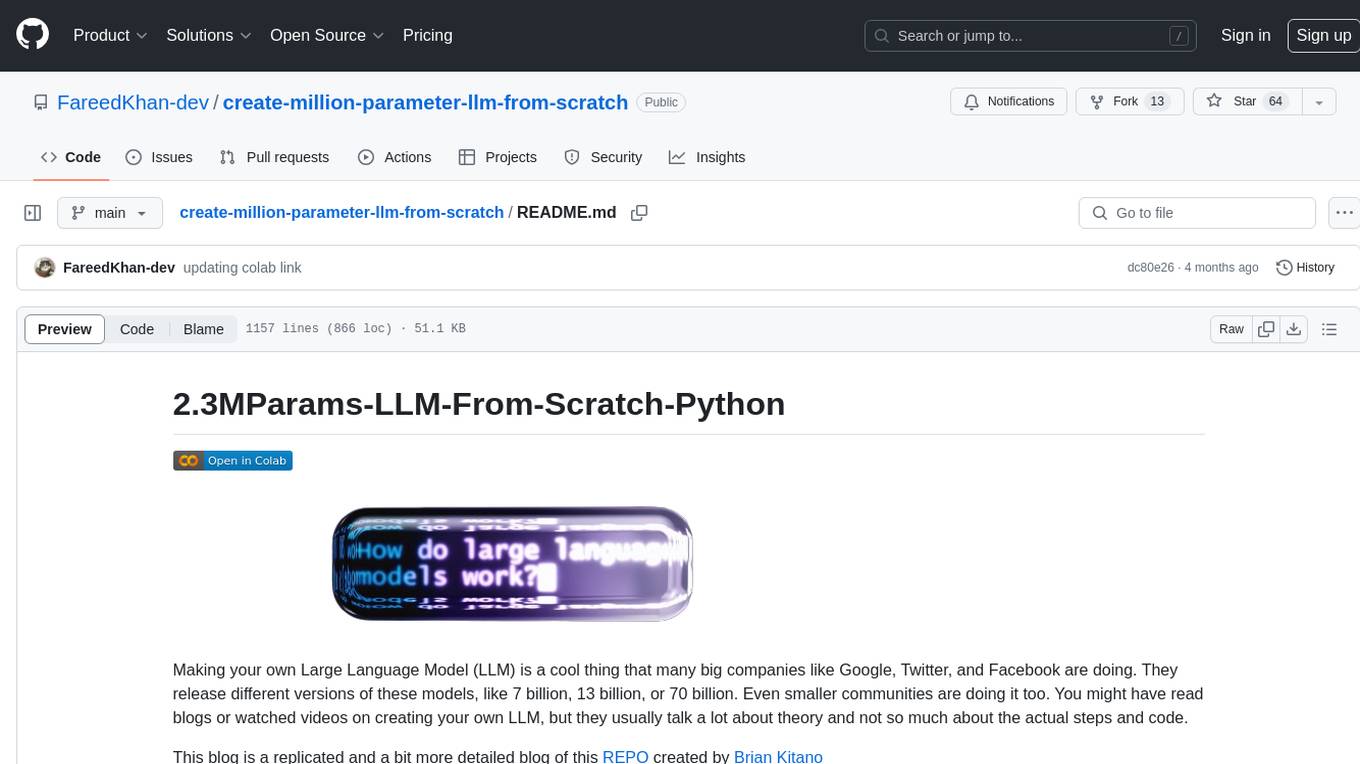
create-million-parameter-llm-from-scratch
The 'create-million-parameter-llm-from-scratch' repository provides a detailed guide on creating a Large Language Model (LLM) with 2.3 million parameters from scratch. The blog replicates the LLaMA approach, incorporating concepts like RMSNorm for pre-normalization, SwiGLU activation function, and Rotary Embeddings. The model is trained on a basic dataset to demonstrate the ease of creating a million-parameter LLM without the need for a high-end GPU.
For similar jobs
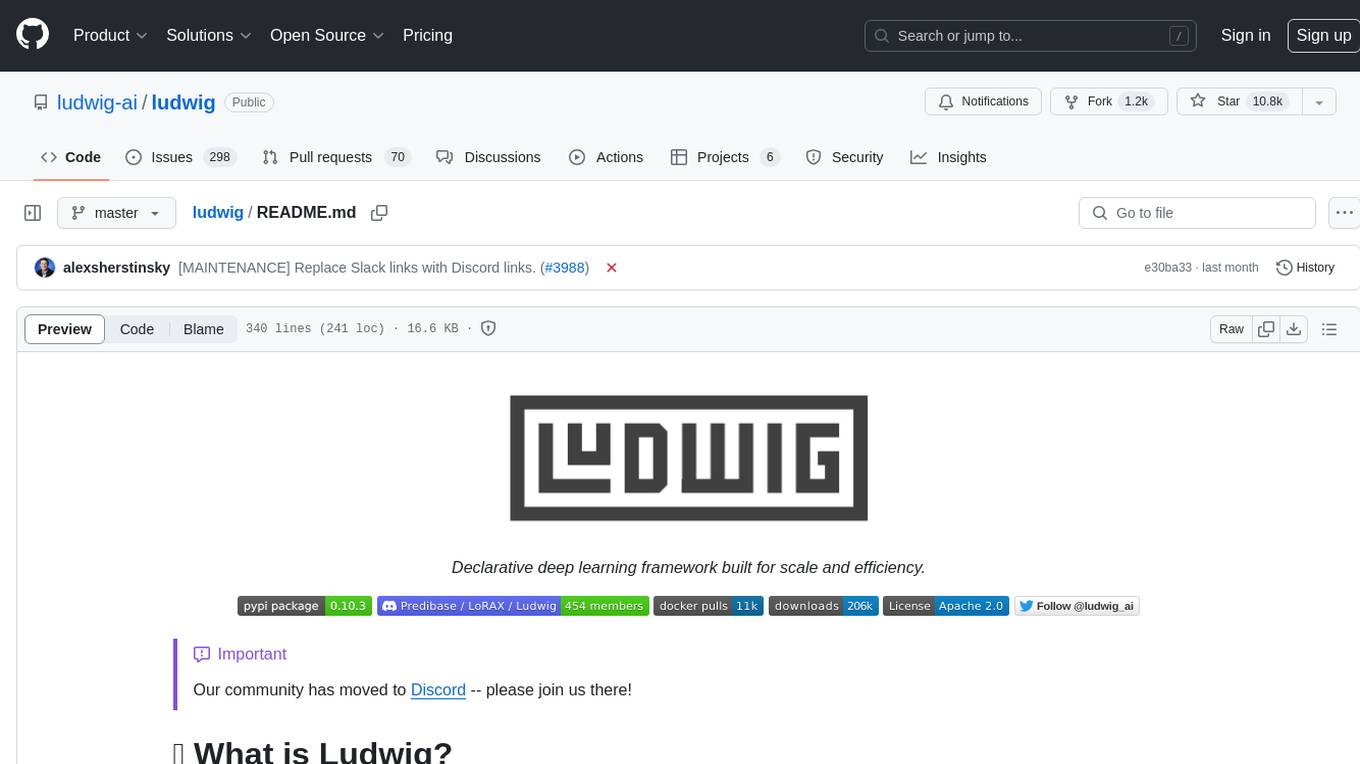
ludwig
Ludwig is a declarative deep learning framework designed for scale and efficiency. It is a low-code framework that allows users to build custom AI models like LLMs and other deep neural networks with ease. Ludwig offers features such as optimized scale and efficiency, expert level control, modularity, and extensibility. It is engineered for production with prebuilt Docker containers, support for running with Ray on Kubernetes, and the ability to export models to Torchscript and Triton. Ludwig is hosted by the Linux Foundation AI & Data.
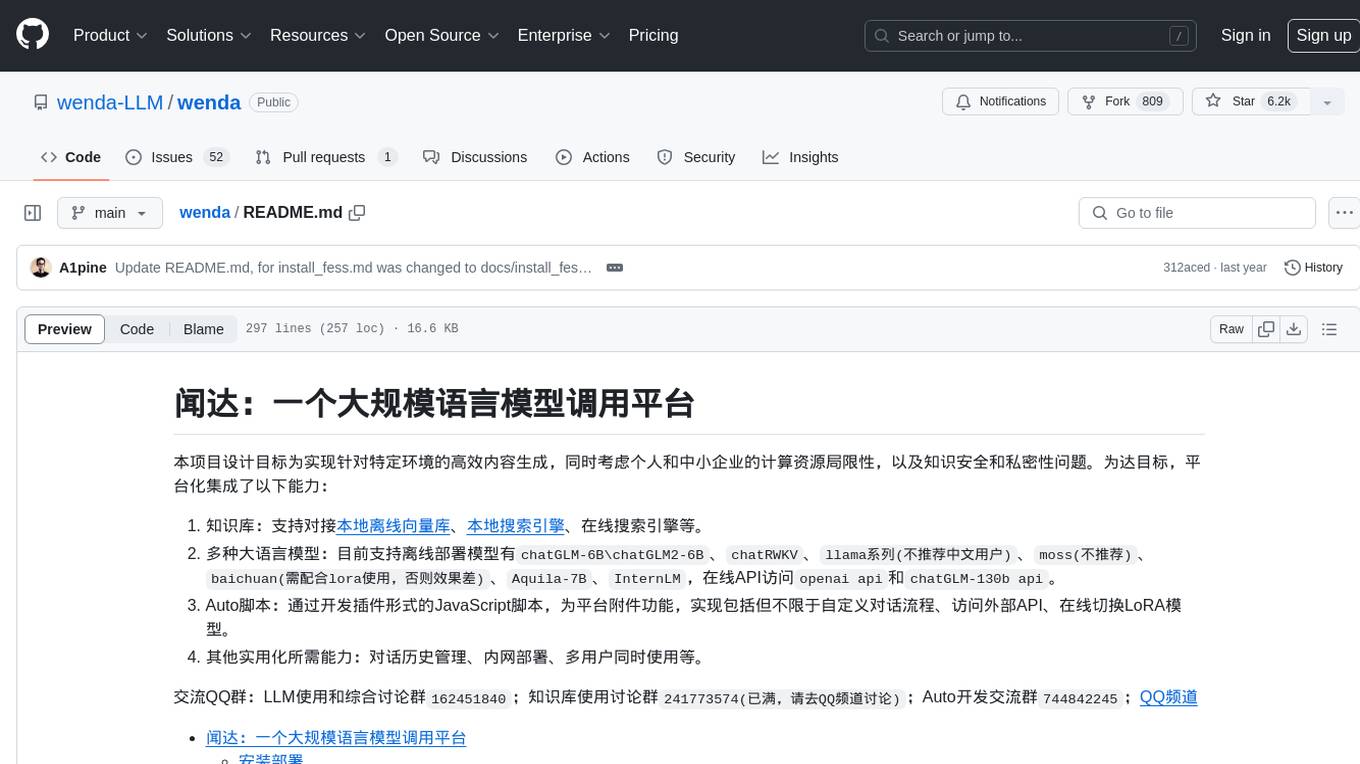
wenda
Wenda is a platform for large-scale language model invocation designed to efficiently generate content for specific environments, considering the limitations of personal and small business computing resources, as well as knowledge security and privacy issues. The platform integrates capabilities such as knowledge base integration, multiple large language models for offline deployment, auto scripts for additional functionality, and other practical capabilities like conversation history management and multi-user simultaneous usage.
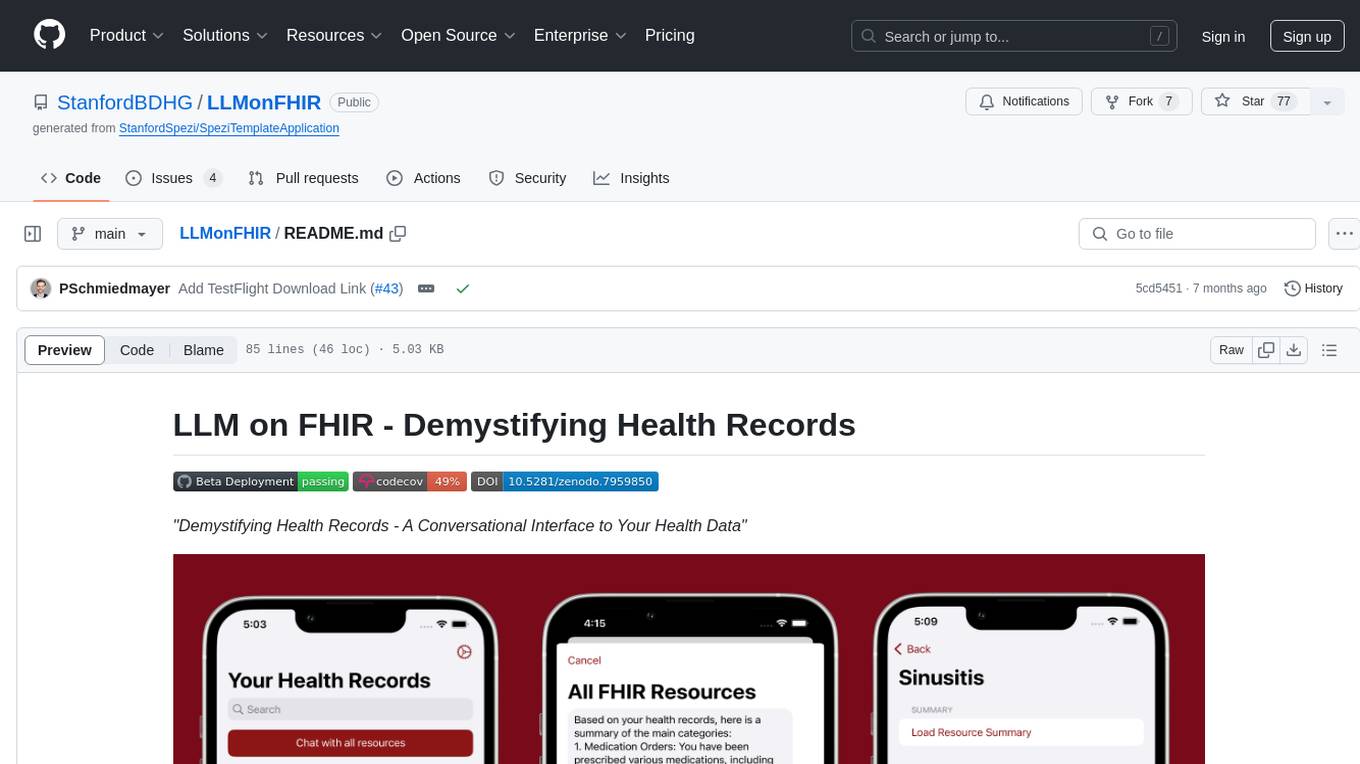
LLMonFHIR
LLMonFHIR is an iOS application that utilizes large language models (LLMs) to interpret and provide context around patient data in the Fast Healthcare Interoperability Resources (FHIR) format. It connects to the OpenAI GPT API to analyze FHIR resources, supports multiple languages, and allows users to interact with their health data stored in the Apple Health app. The app aims to simplify complex health records, provide insights, and facilitate deeper understanding through a conversational interface. However, it is an experimental app for informational purposes only and should not be used as a substitute for professional medical advice. Users are advised to verify information provided by AI models and consult healthcare professionals for personalized advice.
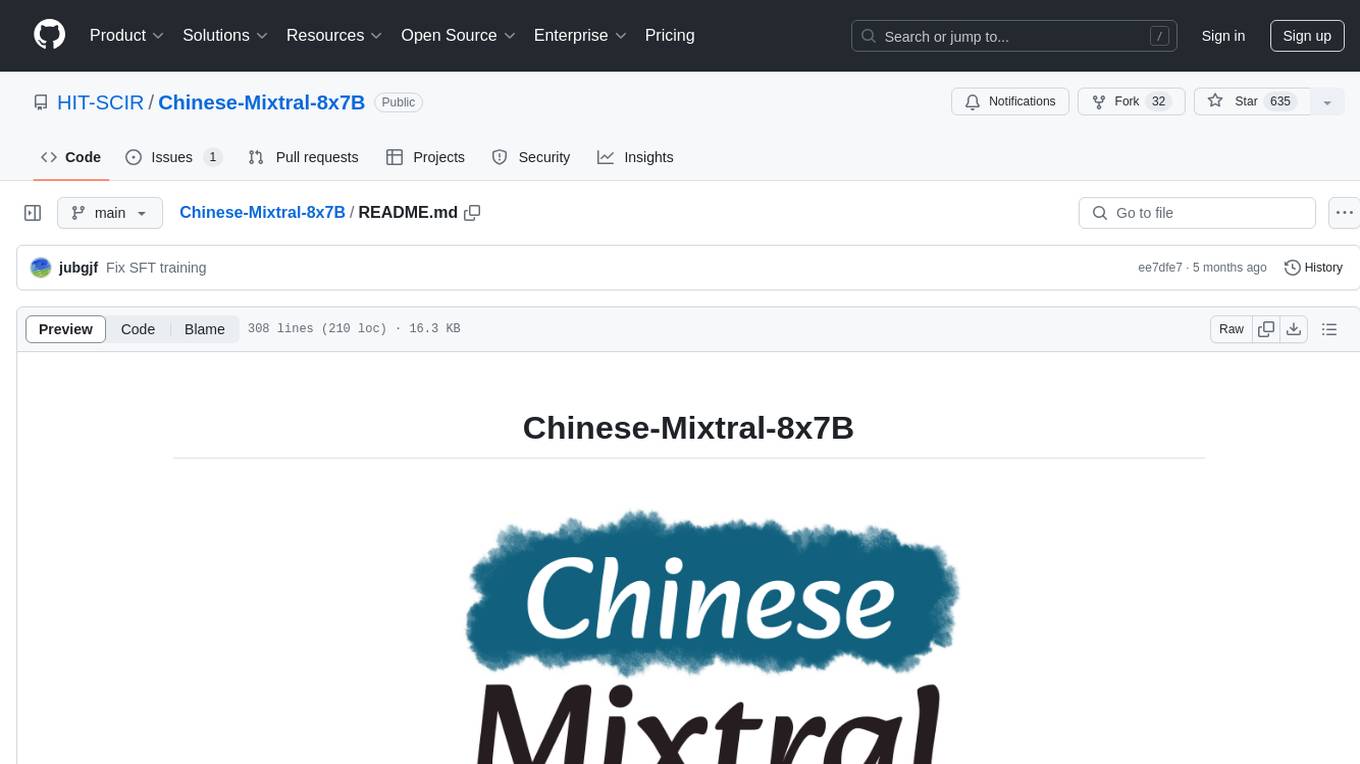
Chinese-Mixtral-8x7B
Chinese-Mixtral-8x7B is an open-source project based on Mistral's Mixtral-8x7B model for incremental pre-training of Chinese vocabulary, aiming to advance research on MoE models in the Chinese natural language processing community. The expanded vocabulary significantly improves the model's encoding and decoding efficiency for Chinese, and the model is pre-trained incrementally on a large-scale open-source corpus, enabling it with powerful Chinese generation and comprehension capabilities. The project includes a large model with expanded Chinese vocabulary and incremental pre-training code.
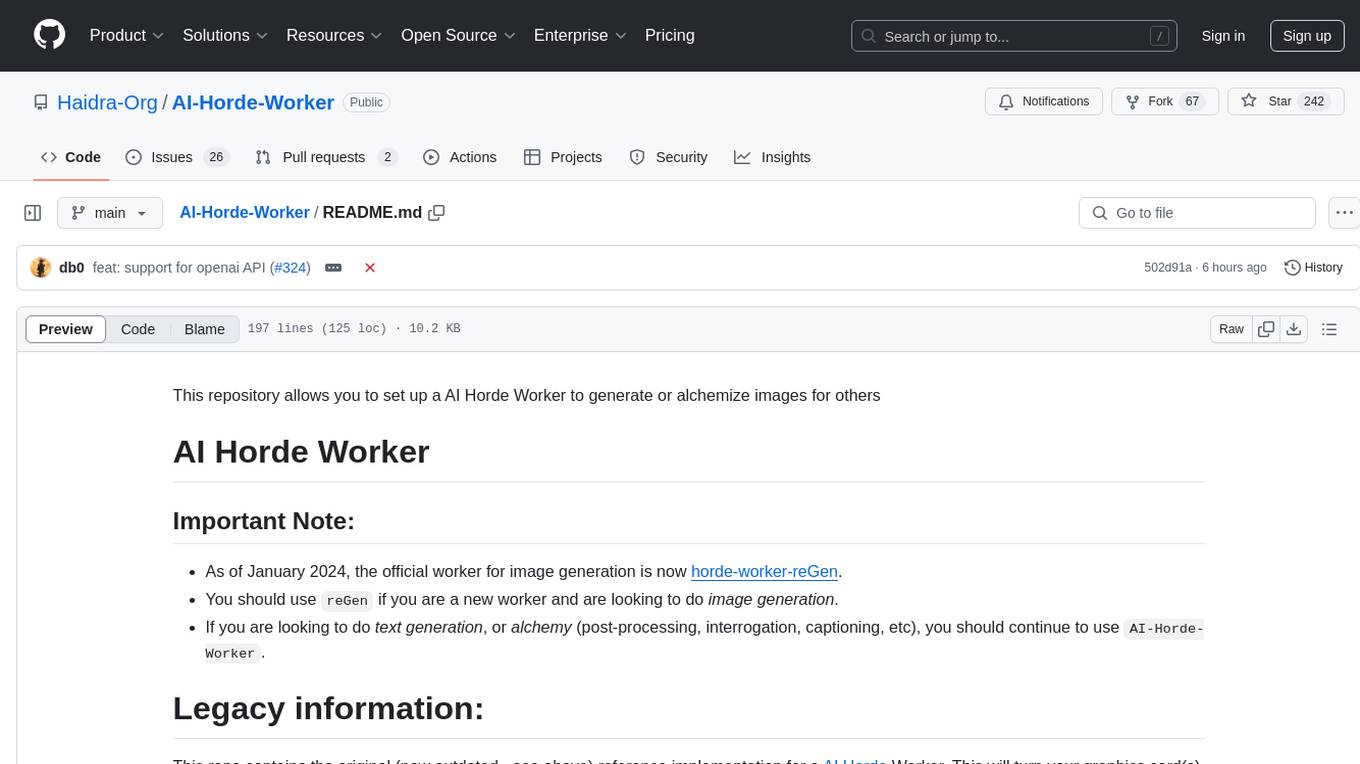
AI-Horde-Worker
AI-Horde-Worker is a repository containing the original reference implementation for a worker that turns your graphics card(s) into a worker for the AI Horde. It allows users to generate or alchemize images for others. The repository provides instructions for setting up the worker on Windows and Linux, updating the worker code, running with multiple GPUs, and stopping the worker. Users can configure the worker using a WebUI to connect to the horde with their username and API key. The repository also includes information on model usage and running the Docker container with specified environment variables.
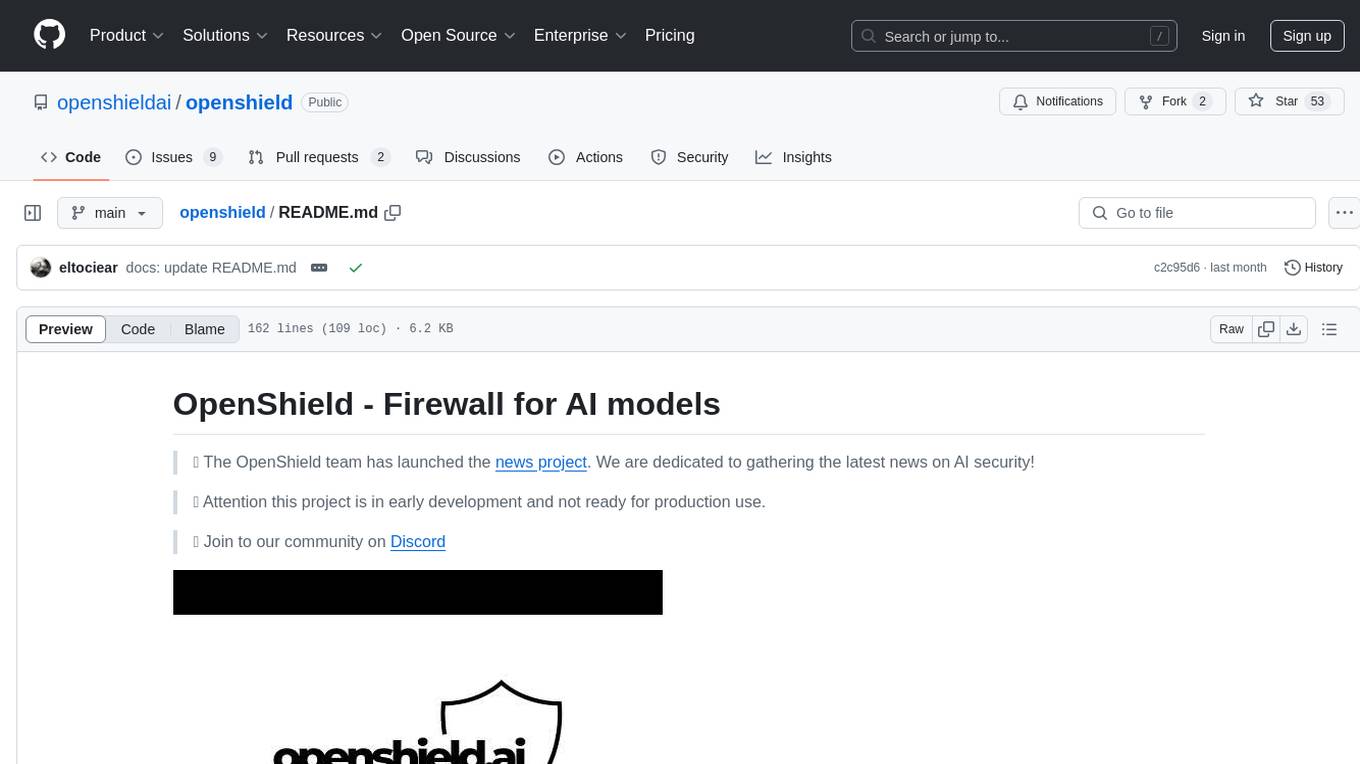
openshield
OpenShield is a firewall designed for AI models to protect against various attacks such as prompt injection, insecure output handling, training data poisoning, model denial of service, supply chain vulnerabilities, sensitive information disclosure, insecure plugin design, excessive agency granting, overreliance, and model theft. It provides rate limiting, content filtering, and keyword filtering for AI models. The tool acts as a transparent proxy between AI models and clients, allowing users to set custom rate limits for OpenAI endpoints and perform tokenizer calculations for OpenAI models. OpenShield also supports Python and LLM based rules, with upcoming features including rate limiting per user and model, prompts manager, content filtering, keyword filtering based on LLM/Vector models, OpenMeter integration, and VectorDB integration. The tool requires an OpenAI API key, Postgres, and Redis for operation.
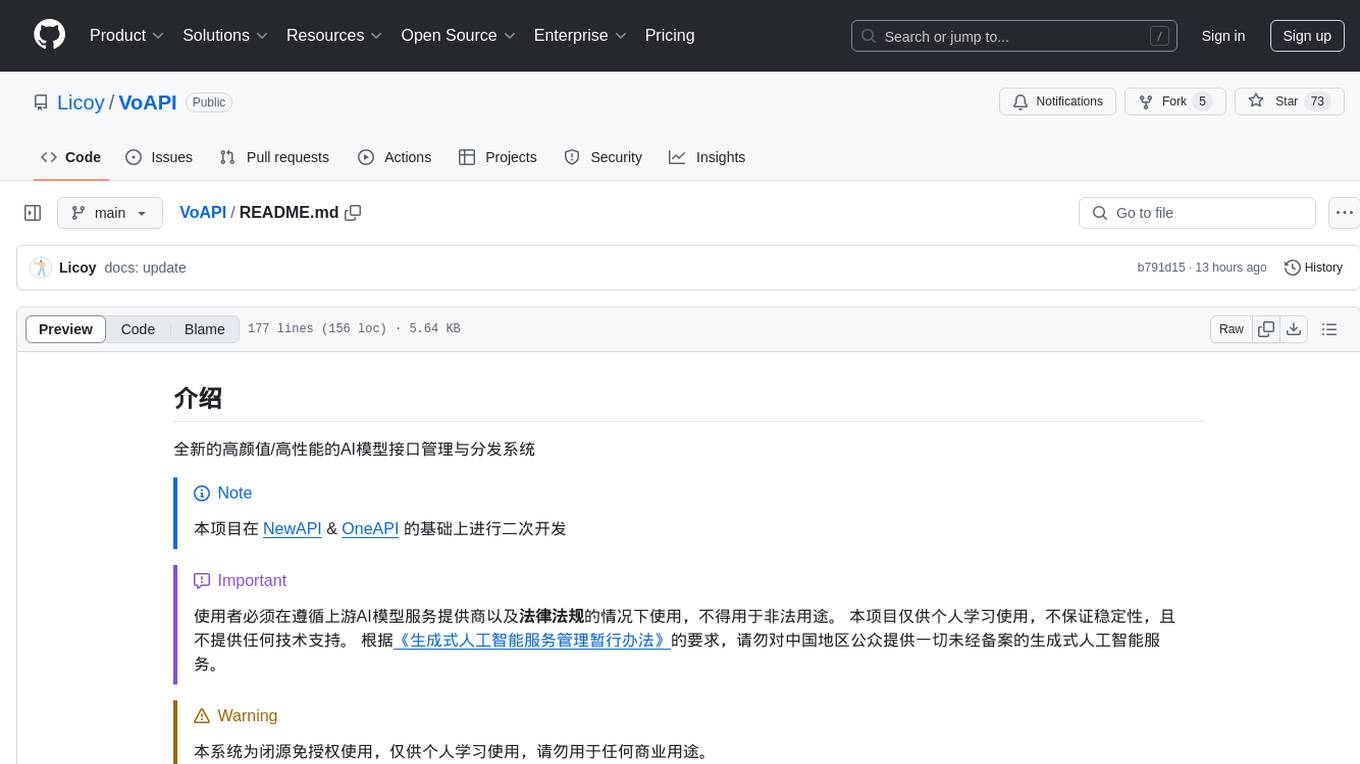
VoAPI
VoAPI is a new high-value/high-performance AI model interface management and distribution system. It is a closed-source tool for personal learning use only, not for commercial purposes. Users must comply with upstream AI model service providers and legal regulations. The system offers a visually appealing interface, independent development documentation page support, service monitoring page configuration support, and third-party login support. It also optimizes interface elements, user registration time support, data operation button positioning, and more.
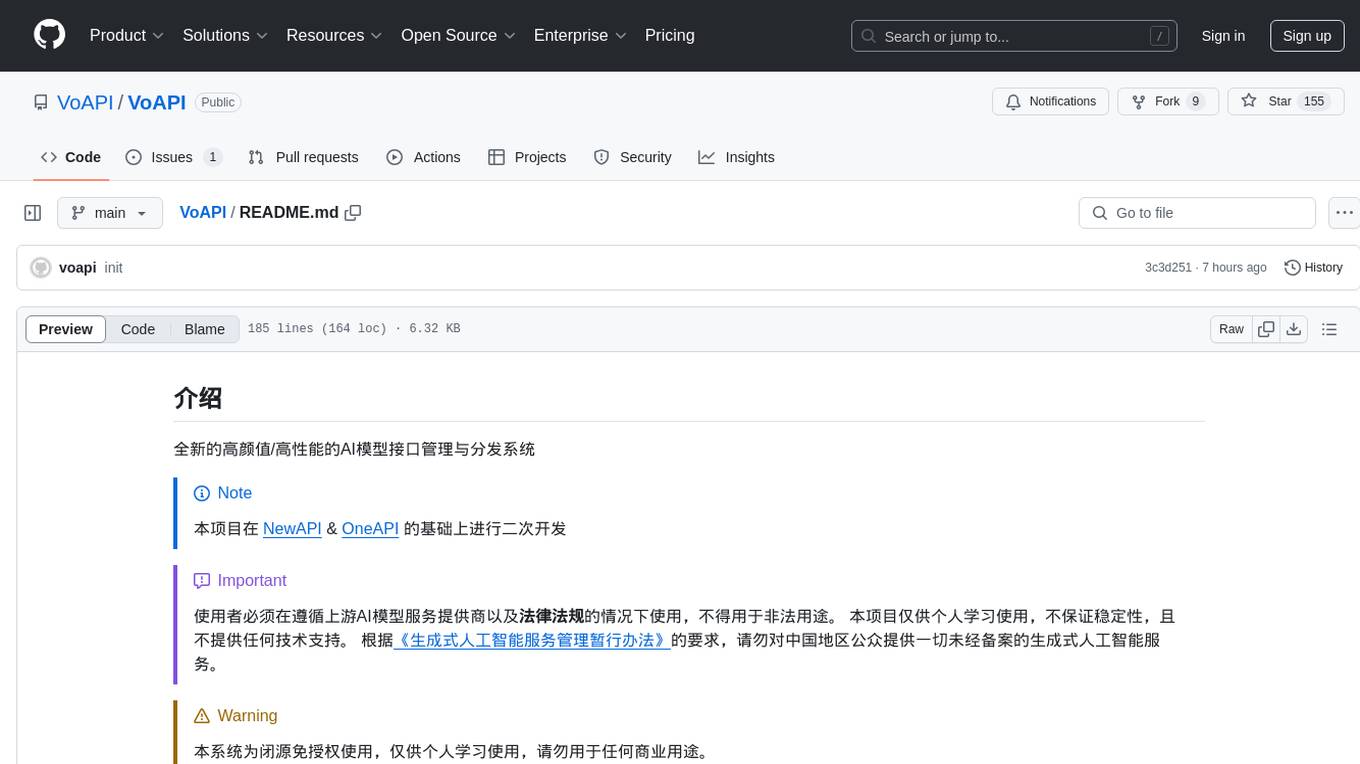
VoAPI
VoAPI is a new high-value/high-performance AI model interface management and distribution system. It is a closed-source tool for personal learning use only, not for commercial purposes. Users must comply with upstream AI model service providers and legal regulations. The system offers a visually appealing interface with features such as independent development documentation page support, service monitoring page configuration support, and third-party login support. Users can manage user registration time, optimize interface elements, and support features like online recharge, model pricing display, and sensitive word filtering. VoAPI also provides support for various AI models and platforms, with the ability to configure homepage templates, model information, and manufacturer information.