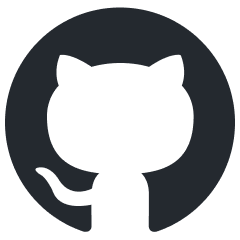
Qwen-TensorRT-LLM
None
Stars: 484
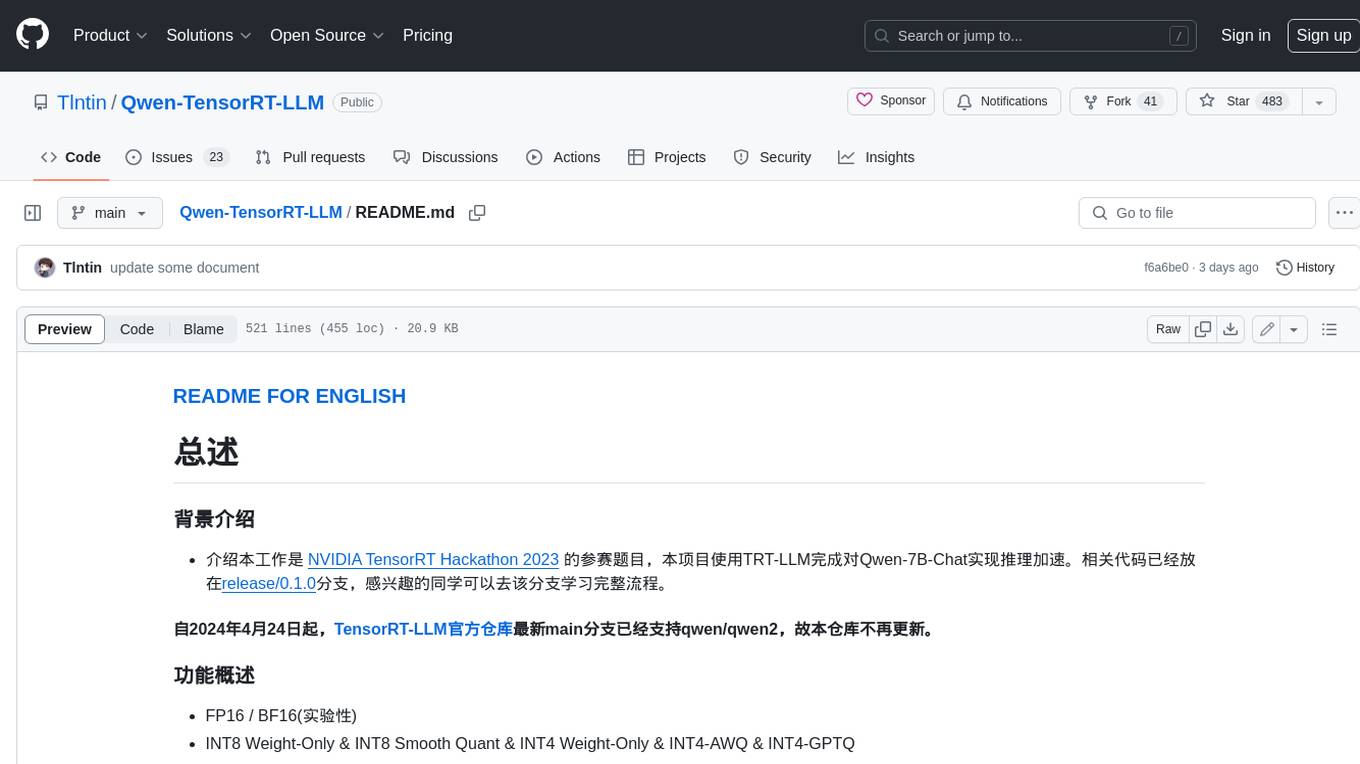
Qwen-TensorRT-LLM is a project developed for the NVIDIA TensorRT Hackathon 2023, focusing on accelerating inference for the Qwen-7B-Chat model using TRT-LLM. The project offers various functionalities such as FP16/BF16 support, INT8 and INT4 quantization options, Tensor Parallel for multi-GPU parallelism, web demo setup with gradio, Triton API deployment for maximum throughput/concurrency, fastapi integration for openai requests, CLI interaction, and langchain support. It supports models like qwen2, qwen, and qwen-vl for both base and chat models. The project also provides tutorials on Bilibili and blogs for adapting Qwen models in NVIDIA TensorRT-LLM, along with hardware requirements and quick start guides for different model types and quantization methods.
README:
- 介绍本工作是 NVIDIA TensorRT Hackathon 2023 的参赛题目,本项目使用TRT-LLM完成对Qwen-7B-Chat实现推理加速。相关代码已经放在release/0.1.0分支,感兴趣的同学可以去该分支学习完整流程。
自2024年4月24日起,TensorRT-LLM官方仓库最新main分支已经支持qwen/qwen2,故本仓库不再做重大更新。
- FP16 / BF16(实验性)
- INT8 Weight-Only & INT8 Smooth Quant & INT4 Weight-Only & INT4-AWQ & INT4-GPTQ
- INT8 KV CACHE
- Tensor Parallel(多卡并行)
- 基于gradio搭建web demo
- 支持triton部署api,结合
inflight_batching
实现最大吞吐/并发。 - 支持fastapi搭建兼容openai请求的api,并且支持function call调用。
- 支持cli命令行对话。
- 支持langchain接入。
- base模型(实验性):Qwen1.5-0.5B、Qwen1.5-1.8B、Qwen1.5-4B、Qwen1.5-7B、Qwen1.5-14B、Qwen1.5-32B、Qwen1.5-72B、QWen-VL、CodeQwen1.5-7B
- chat模型(推荐):Qwen1.5-0.5B-Chat、Qwen1.5-1.8B-Chat、Qwen1.5-4B-Chat、Qwen1.5-7B-Chat、Qwen1.5-14B-Chat、Qwen1.5-32B-Chat、Qwen1.5-72B-Chat(实验性)、QWen-VL-Chat、CodeQwen1.5-7B-Chat
- chat-gptq-int4模型:Qwen1.5-0.5B-Chat-GPTQ-Int4、Qwen1.5-1.8B-Chat-GPTQ-Int4、Qwen1.5-4B-Chat-GPTQ-Int4、Qwen1.5-7B-Chat-GPTQ-Int4、Qwen1.5-14B-Chat-GPTQ-Int4、Qwen1.5-32B-Chat-GPTQ-Int4、Qwen1.5-72B-Chat-GPTQ-Int4(实验性)、Qwen-VL-Chat-Int4
-
本项目配套B站教程:
-
本项目配套博客适配概述:如何在 NVIDIA TensorRT-LLM 中支持 Qwen 模型
- Linux最佳,已安装docker,并且安装了nvidia-docker(安装指南),Windows理论也可以,但是还未测试,感兴趣可以自己研究一下。
- Windows参考这个教程:链接
- 有英伟达显卡(30系,40系,V100/A100等),以及一定的显存、内存、磁盘。结合Qwen官方推理要求,预估出下面的要求,详见表格(仅编译期最大要求),仅供参考:
Model Size | Quantization | GPU Memory Usage (GB) | CPU Memory Usage (GB) | Disk Usage (GB) |
1.8B | fp16 | 5 | 15 | 11 |
int8 smooth quant | 5 | 15 | 22 | |
int8 weight only | 4 | 12 | 9 | |
int4 weight only | 4 | 10 | 7 | |
int4 gptq (raw) | 4 | 10 | 6 | |
int4 gptq (manual) | 5 | 13 | 14 | |
int4 awq | 5 | 13 | 18 | |
7B | fp16 | 21 | 59 | 42 |
int8 smooth quant | 21 | 59 | 84 | |
int8 weight only | 14 | 39 | 28 | |
int4 weight only | 10 | 29 | 21 | |
int4 gptq (raw) | 10 | 29 | 16 | |
int4 gptq (manual) | 21 | 51 | 42 | |
int4 awq | 21 | 51 | 56 | |
14B | fp16 | 38 | 106 | 75 |
int8 smooth quant | 38 | 106 | 150 | |
int8 weight only | 24 | 66 | 47 | |
int4 weight only | 16 | 46 | 33 | |
int4 gptq (raw) | 16 | 46 | 26 | |
int4 gptq (manual) | 38 | 90 | 66 | |
int4 awq | 38 | 90 | 94 | |
72B | fp16 | 181 | 506 | 362 |
int8 smooth quant | 181 | 506 | 724 | |
int8 weight only | 102 | 284 | 203 | |
int4 weight only | 61 | 171 | 122 | |
int4 gptq (raw) | 61 | 171 | 98 | |
int4 gptq (manual) | 181 | 434 | 244 | |
int4 awq | 181 | 434 | 406 |
-
下载镜像。
-
官方triton镜像24.02,对应TensorRT-LLM版本为0.8.0,不含TensorRT-LLM开发包。
docker pull nvcr.io/nvidia/tritonserver:24.02-trtllm-python-py3
-
对于Windows用户想体验tritonserver部署的,或者无GPU的用户,可以使用AutoDL镜像,含tritonserver,版本为24.02(对应tensorrt_llm 0.8.0),链接,注:该链接包含完整编译教程。
-
-
拉取本项目代码
git clone https://github.com/Tlntin/Qwen-TensorRT-LLM.git cd Qwen-TensorRT-LLM
-
进入项目目录,然后创建并启动容器,同时将本地
examples
代码路径映射到/app/tensorrt_llm/examples
路径,然后打开8000和7860端口的映射,方便调试api和web界面。docker run --gpus all \ --name trt_llm \ -d \ --ipc=host \ --ulimit memlock=-1 \ --restart=always \ --ulimit stack=67108864 \ -p 8000:8000 \ -p 7860:7860 \ -v ${PWD}/examples:/app/tensorrt_llm/examples \ nvcr.io/nvidia/tritonserver:24.02-trtllm-python-py3 sleep 8640000
-
进入docker容器里面的qwen2路径,
-
使用pip直接安装官方编译好的tensorrt_llm
pip install tensorrt_llm==0.8.0 --extra-index-url https://pypi.nvidia.com --extra-index-url https://download.pytorch.org/whl/cu121
-
安装提供的Python依赖
cd /app/tensorrt_llm/examples/qwen2/ pip install -r requirements.txt
-
升级transformers版本,qwen2最低需要4.37以上版本,如果有警告依赖不匹配可以忽略。
pip install "transformers>=4.37"
-
-
从HuggingFace下载模型(暂时不支持其他平台),例如
QWen1.5-7B-Chat
模型,然后将文件夹重命名为qwen1.5_7b_chat
,最后放到examples/qwen2/
路径下即可。 -
修改编译参数(可选)
- 默认编译参数,包括batch_size, max_input_len, max_new_tokens, seq_length都存放在
default_config.py
中 - 默认模型路径,包括
hf_model_dir
(模型路径)和tokenizer_dir
(分词器路径)以及int4_gptq_model_dir
(手动gptq量化输出路径),可以改成你自定义的路径。 - 对于24G显存用户,直接编译即可,默认是fp16数据类型,max_batch_size=2
- 对于低显存用户,可以降低max_batch_size=1,或者继续降低max_input_len, max_new_tokens
- 默认编译参数,包括batch_size, max_input_len, max_new_tokens, seq_length都存放在
-
编译。
-
编译fp16(注:
--remove_input_padding
和--enable_context_fmha
为可选参数,可以一定程度上节省显存)。python3 build.py --remove_input_padding --enable_context_fmha
-
编译 int8 (weight only)。
python3 build.py --use_weight_only --weight_only_precision=int8
-
编译int4 (weight only)
python3 build.py --use_weight_only --weight_only_precision=int4
-
对于如果单卡装不下,又不想用int4/int8量化,可以选择尝试tp = 2,即启用两张GPU进行编译 (注:tp功能目前只支持从Huggingface格式构建engine)
python3 build.py --world_size 2 --tp_size 2
-
-
运行。编译完后,再试跑一下,输出
Output: "您好,我是来自达摩院的大规模语言模型,我叫通义千问。"
这说明成功。-
tp = 1(默认单GPU)时使用python直接运行run.py
python3 run.py
-
tp = 2(2卡用户,或者更多GPU卡)时,使用
mpirun
命令来运行run.pympirun -n 2 --allow-run-as-root python run.py
-
使用官方24.02容器多卡可能会报错,提示:
Failed, NCCL error /home/jenkins/agent/workspace/LLM/release-0.8/L0_PostMerge/tensorrt_llm/cpp/tensorrt_llm/plugins/ncclPlugin/allreducePlugin.cpp:183 'unknown result code'
,需要安装nccl2.20.3-1(使用压缩包,解压后导入系统环境变量或者使用apt命名安装均可),安装后即可正常运行。export LD_LIBRARY_PATH=nccl_2.20.3-1+cuda12.3_x86_64/lib/:$LD_LIBRARY_PATH # 或者,推荐下面这种 apt-get install -y --no-install-recommends libnccl2=2.20.3-1+cuda12.3 libnccl-dev=2.20.3-1+cuda12.3 -y
-
-
验证模型精度。可以试试跑一下
summarize.py
,对比一下huggingface和trt-llm的rouge得分。这一步需要在线下载数据集,对于网络不好的用户,可以参考该方法:datasets离线加载huggingface数据集方法-
跑hugggingface版
python3 summarize.py --test_hf
-
跑trt-llm版
python3 summarize.py --test_trt_llm
-
一般来说,如果trt-llm的rouge分数和huggingface差不多,略低一些(1以内)或者略高一些(2以内),则说明精度基本对齐。
-
-
测量模型吞吐速度和生成速度。需要下载
ShareGPT_V3_unfiltered_cleaned_split.json
这个文件。-
可以通过wget/浏览器直接下载,下载链接
-
也可通过百度网盘下载,链接: https://pan.baidu.com/s/12rot0Lc0hc9oCb7GxBS6Ng?pwd=jps5 提取码: jps5
-
下载后同样放到
examples/qwen2/
路径下即可 -
测量前,如果需要改max_input_length/max_new_tokens,可以直接改
default_config.py
即可。一般不推荐修改,如果修改了这个,则需要重新编译一次trt-llm,保证两者输入数据集长度统一。 -
测量huggingface模型
python3 benchmark.py --backend=hf --dataset=ShareGPT_V3_unfiltered_cleaned_split.json --hf_max_batch_size=1
-
测量trt-llm模型 (注意:
--trt_max_batch_size
不应该超过build时候定义的最大batch_size,否则会出现内存错误。)python3 benchmark.py --backend=trt_llm --dataset=ShareGPT_V3_unfiltered_cleaned_split.json --trt_max_batch_size=1
-
-
注意:运行Smooth Quant需要将huggingface模型完全加载到GPU里面,用于构建int8标定数据集,所以需要提前确保你的显存够大,能够完全加载整个模型。
-
将Huggingface格式的数据转成FT(FastTransformer)需要的数据格式,这一步需要在线下载数据集,对于网络不好的用户,可以参考该方法:datasets离线加载huggingface数据集方法
-
单卡
python3 hf_qwen_convert.py --smoothquant=0.5
-
多卡(以2卡为例)
python3 hf_qwen_convert.py --smoothquant=0.5 --tensor-parallelism=2
-
-
开始编译trt_engine
- 单卡
python3 build.py --use_smooth_quant --per_token --per_channel
- 多卡(以2卡为例)
python3 build.py --use_smooth_quant --per_token --per_channel --world_size 2 --tp_size 2
- 单卡
-
编译完成,run/summarize/benchmark等等都和上面的是一样的了。
-
注意:运行int8-kv-cache需要将huggingface模型完全加载到GPU里面,用于构建int8标定数据集,所以需要提前确保你的显存够大,能够完全加载整个模型。
-
将Huggingface格式的数据转成FT(FastTransformer)需要的数据格式。
- 单卡
python3 hf_qwen_convert.py --calibrate-kv-cache
- 多卡(以2卡为例)
python3 hf_qwen_convert.py --calibrate-kv-cache --tensor-parallelism=2
- 单卡
-
编译int8 weight only + int8-kv-cache
- 单卡
python3 build.py --use_weight_only --weight_only_precision=int8 --int8_kv_cache
- 多卡(以2卡为例)
python3 build.py --use_weight_only --weight_only_precision=int8 --int8_kv_cache --world_size 2 --tp_size 2
- 单卡
-
需要安装auto-gptq模块,并且升级transformers模块版本到最新版(建议optimum和transformers都用最新版,否则可能有乱码问题),参考issue/68。(注:安装完模块后可能会提示tensorrt_llm与其他模块版本不兼容,可以忽略该警告)
pip install auto-gptq optimum pip install transformers -U
-
手动获取标定权重(可选)
- 转权重获取scale相关信息,默认使用GPU进行校准,需要能够完整加载模型。(注:对于Qwen-7B-Chat V1.0,可以加上
--device=cpu
来尝试用cpu标定,但是时间会很长)python3 gptq_convert.py
- 编译TensorRT-LLM Engine
python build.py --use_weight_only \ --weight_only_precision int4_gptq \ --per_group
- 如果想要节省显存(注:只能用于单batch),可以试试加上这俩参数来编译Engine
python build.py --use_weight_only \ --weight_only_precision int4_gptq \ --per_group \ --remove_input_padding \ --enable_context_fmha
- 转权重获取scale相关信息,默认使用GPU进行校准,需要能够完整加载模型。(注:对于Qwen-7B-Chat V1.0,可以加上
-
使用官方int4权重,例如Qwen-xx-Chat-Int4模型(推荐)
- 编译模型,注意设置hf模型路径和
--quant_ckpt_path
量化后权重路径均设置为同一个路径,下面是32b-gptq-int4模型的示例(其他gptq-int4模型也是一样操作)python build.py --use_weight_only \ --weight_only_precision int4_gptq \ --per_group \ --hf_model_dir Qwen1.5-32B-Chat-GPTQ-Int4 \ --quant_ckpt_path Qwen1.5-32B-Chat-GPTQ-Int4
- 运行模型,这里需要指定一下tokenizer路径
python3 run.py --tokenizer_dir=Qwen1.5-32B-Chat-GPTQ-Int4
- 编译模型,注意设置hf模型路径和
- 需要下载并安装nvidia-ammo模块(仅支持Linux,不支持Windows)
pip install --no-cache-dir --extra-index-url https://pypi.nvidia.com nvidia-ammo~=0.7.0
- 运行int4-awq量化代码,导出校准权重。
python3 quantize.py --export_path ./qwen2_7b_4bit_gs128_awq.pt
- 运行build.py,用于构建TensorRT-LLM Engine。
python build.py --use_weight_only \ --weight_only_precision int4_awq \ --per_group \ --quant_ckpt_path ./qwen2_7b_4bit_gs128_awq.pt
- 如果想要节省显存(注:只能用于单batch),可以试试加上这俩参数来编译Engine
python build.py --use_weight_only \ --weight_only_precision int4_awq \ --per_group \ --remove_input_padding \ --enable_context_fmha \ --quant_ckpt_path ./qwen2_7b_4bit_gs128_awq.pt
-
尝试终端对话。运行下面的命令,然后输入你的问题,直接回车即可。
python3 cli_chat.py
-
部署api,并调用api进行对话。
- 部署api
python3 api.py
- 另开一个终端,进入
qwen2/client
目录,里面有4个文件,分别代表不同的调用方式。 -
async_client.py
,通过异步的方式调用api,通过SSE协议来支持流式输出。 -
normal_client.py
,通过同步的方式调用api,为常规的HTTP协议,Post请求,不支持流式输出,请求一次需要等模型生成完所有文字后,才能返回。 -
openai_normal_client.py
,通过openai
模块直接调用自己部署的api,该示例为非流式调用,请求一次需要等模型生成完所有文字后,才能返回。。 -
openai_stream_client.py
,通过openai
模块直接调用自己部署的api,该示例为流式调用。 - 注意:需要
pydantic
模块版本>=2.3.2,否则将会出现ChatCompletionResponse' object has no attribute 'model_dump_json'
报错,参考issue
-
尝试网页对话(可选,需要先部署api)。运行下面的命令,然后打开本地浏览器,访问:http://127.0.0.1:7860 即可
python3 web_demo.py
- 默认配置的web_demo.py如下:
demo.queue().launch(share=False, inbrowser=True)
- 如果是服务器运行,建议改成这样
demo.queue().launch(server_name="0.0.0.0", share=False, inbrowser=False)
- web_demo参数说明
-
share=True
: 代表将网站穿透到公网,会自动用一个随机的临时公网域名,有效期3天,不过这个选项可能不太安全,有可能造成服务器被攻击,不建议打开。 -
inbrowser=True
: 部署服务后,自动打开浏览器,如果是本机,可以打开。如果是服务器,不建议打开,因为服务器也没有谷歌浏览器给你打开。 -
server_name="0.0.0.0"
: 允许任意ip访问,适合服务器,然后你只需要输入http://[你的ip]: 7860
就能看到网页了,如果不开这个选择,默认只能部署的那台机器才能访问。 -
share=False
:仅局域网/或者公网ip访问,不会生成公网域名。 -
inbrowser=False
: 部署后不打开浏览器,适合服务器。
-
-
web_demo运行效果(测试平台:RTX 4080, qwen2-7b-chat, int4 weight only)
- 参考该教程部署tritonserver:Triton24.02部署TensorRT-LLM,实现http查询
- 使用该项目封装tritonserver以支持openai API格式,项目链接:https://github.com/zhaohb/fastapi_tritonserver
For Tasks:
Click tags to check more tools for each tasksFor Jobs:
Alternative AI tools for Qwen-TensorRT-LLM
Similar Open Source Tools
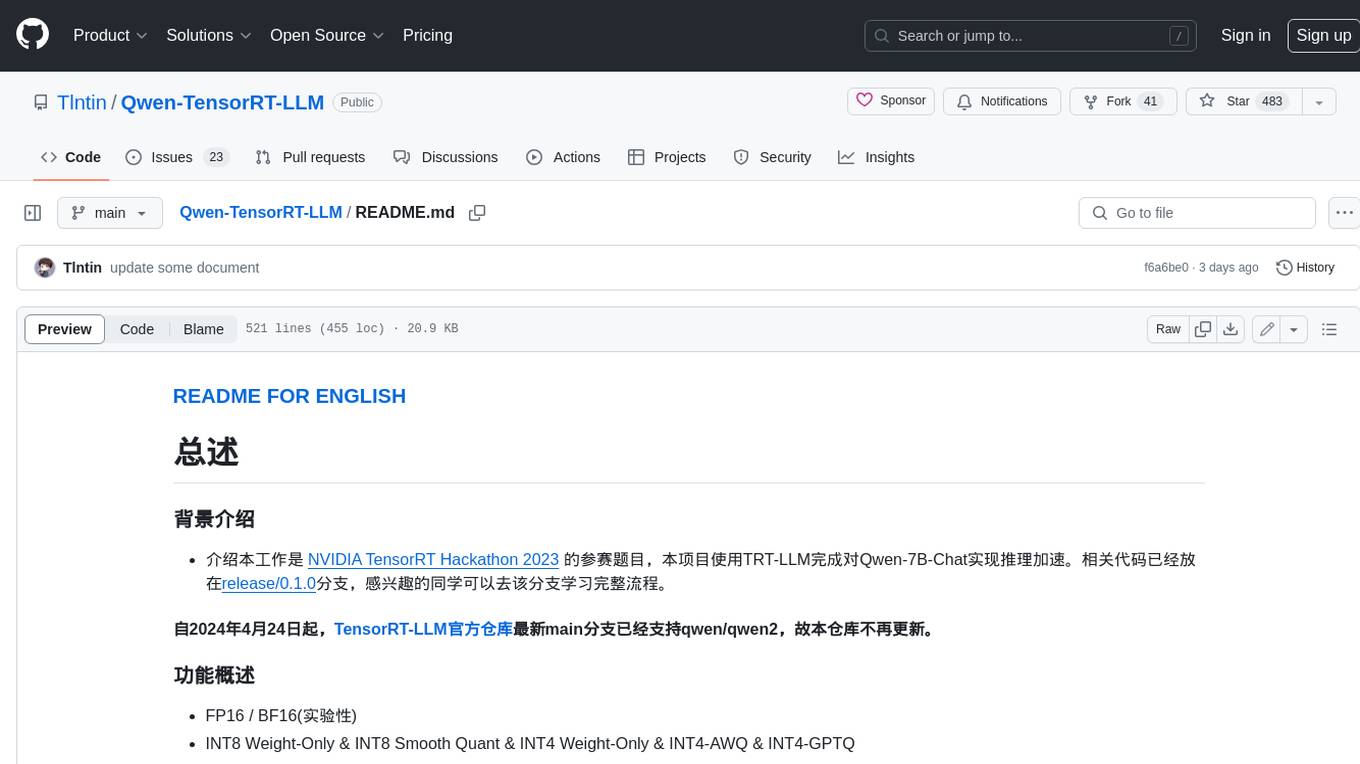
Qwen-TensorRT-LLM
Qwen-TensorRT-LLM is a project developed for the NVIDIA TensorRT Hackathon 2023, focusing on accelerating inference for the Qwen-7B-Chat model using TRT-LLM. The project offers various functionalities such as FP16/BF16 support, INT8 and INT4 quantization options, Tensor Parallel for multi-GPU parallelism, web demo setup with gradio, Triton API deployment for maximum throughput/concurrency, fastapi integration for openai requests, CLI interaction, and langchain support. It supports models like qwen2, qwen, and qwen-vl for both base and chat models. The project also provides tutorials on Bilibili and blogs for adapting Qwen models in NVIDIA TensorRT-LLM, along with hardware requirements and quick start guides for different model types and quantization methods.
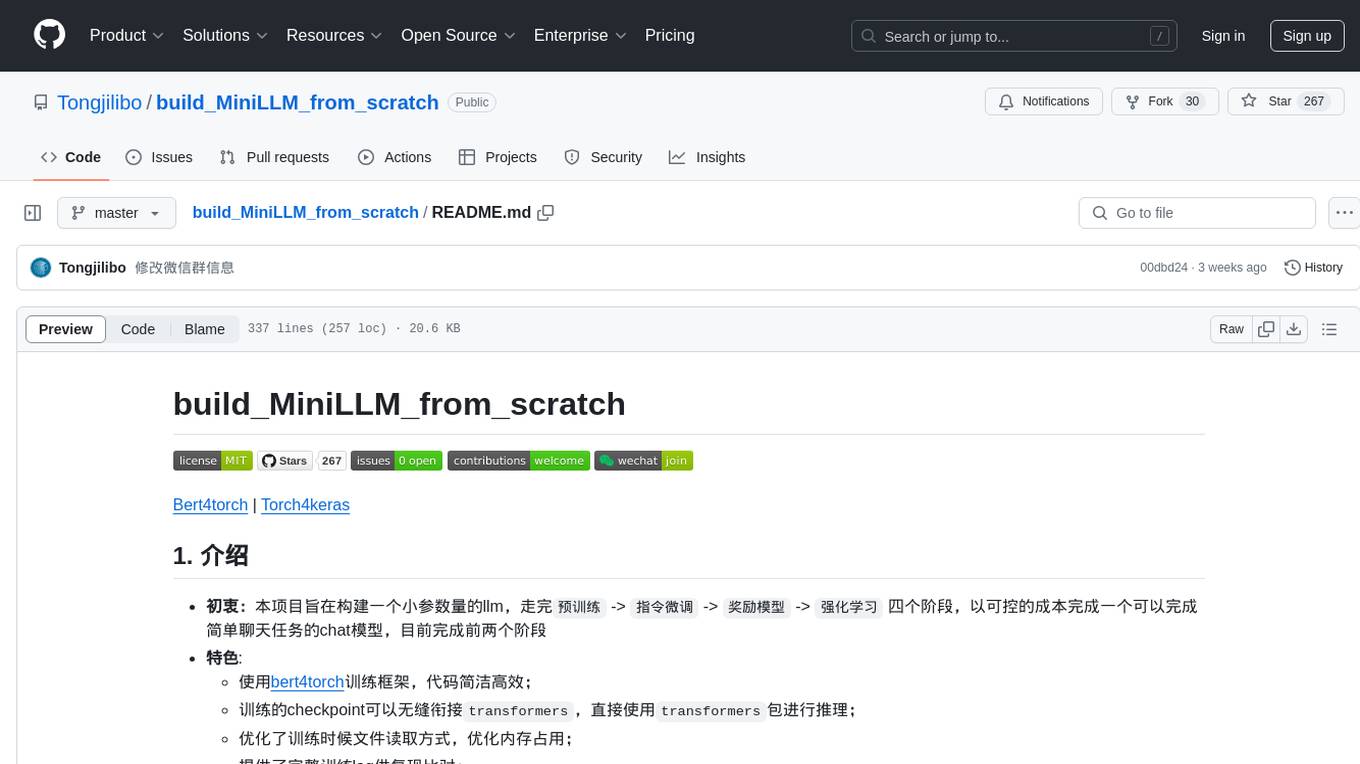
build_MiniLLM_from_scratch
This repository aims to build a low-parameter LLM model through pretraining, fine-tuning, model rewarding, and reinforcement learning stages to create a chat model capable of simple conversation tasks. It features using the bert4torch training framework, seamless integration with transformers package for inference, optimized file reading during training to reduce memory usage, providing complete training logs for reproducibility, and the ability to customize robot attributes. The chat model supports multi-turn conversations. The trained model currently only supports basic chat functionality due to limitations in corpus size, model scale, SFT corpus size, and quality.
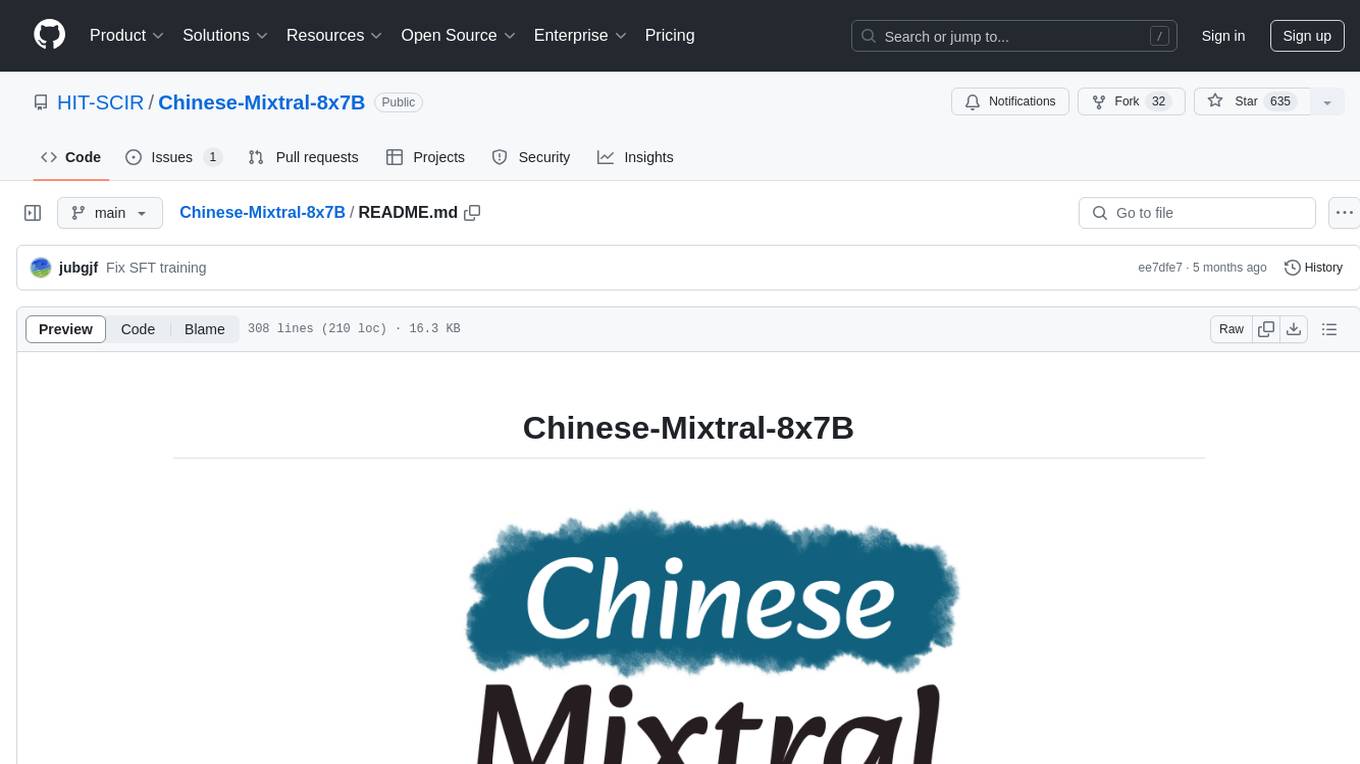
Chinese-Mixtral-8x7B
Chinese-Mixtral-8x7B is an open-source project based on Mistral's Mixtral-8x7B model for incremental pre-training of Chinese vocabulary, aiming to advance research on MoE models in the Chinese natural language processing community. The expanded vocabulary significantly improves the model's encoding and decoding efficiency for Chinese, and the model is pre-trained incrementally on a large-scale open-source corpus, enabling it with powerful Chinese generation and comprehension capabilities. The project includes a large model with expanded Chinese vocabulary and incremental pre-training code.
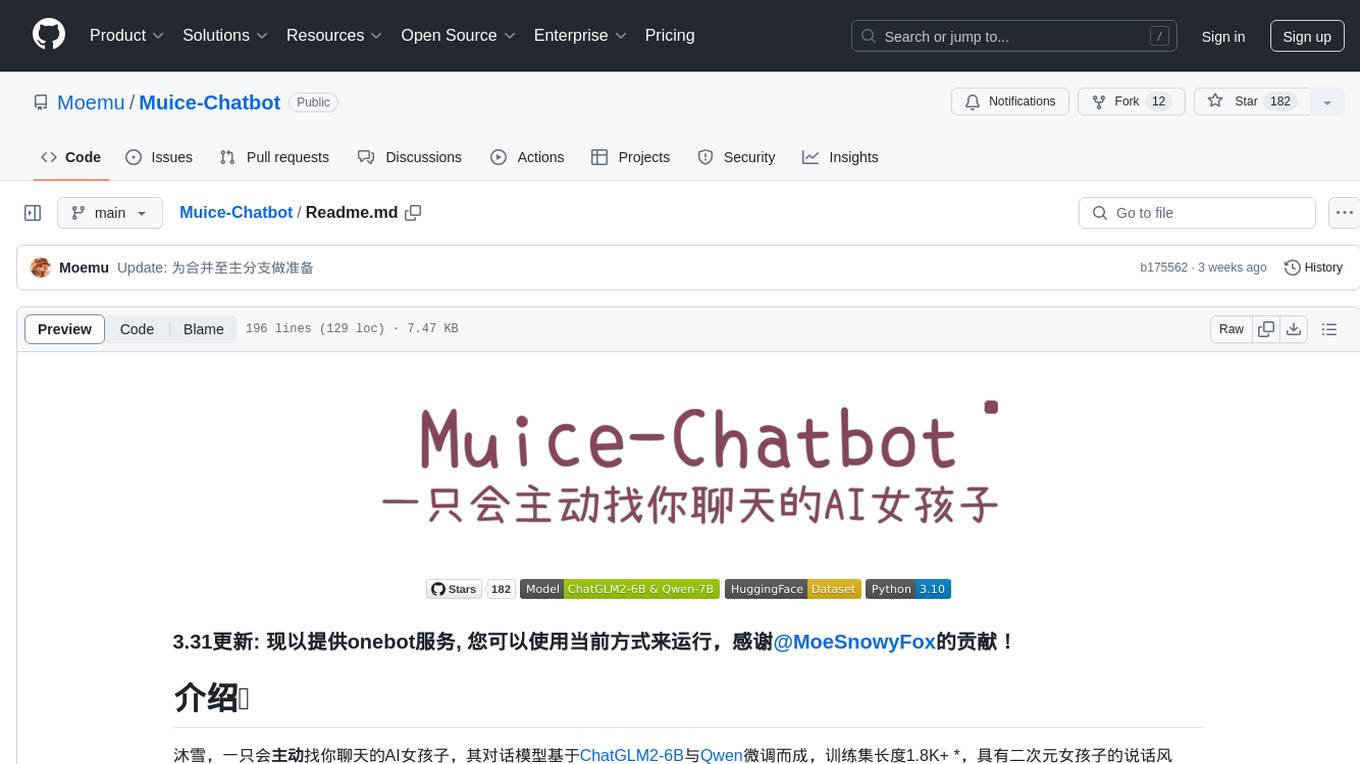
Muice-Chatbot
Muice-Chatbot is an AI chatbot designed to proactively engage in conversations with users. It is based on the ChatGLM2-6B and Qwen-7B models, with a training dataset of 1.8K+ dialogues. The chatbot has a speaking style similar to a 2D girl, being somewhat tsundere but willing to share daily life details and greet users differently every day. It provides various functionalities, including initiating chats and offering 5 available commands. The project supports model loading through different methods and provides onebot service support for QQ users. Users can interact with the chatbot by running the main.py file in the project directory.
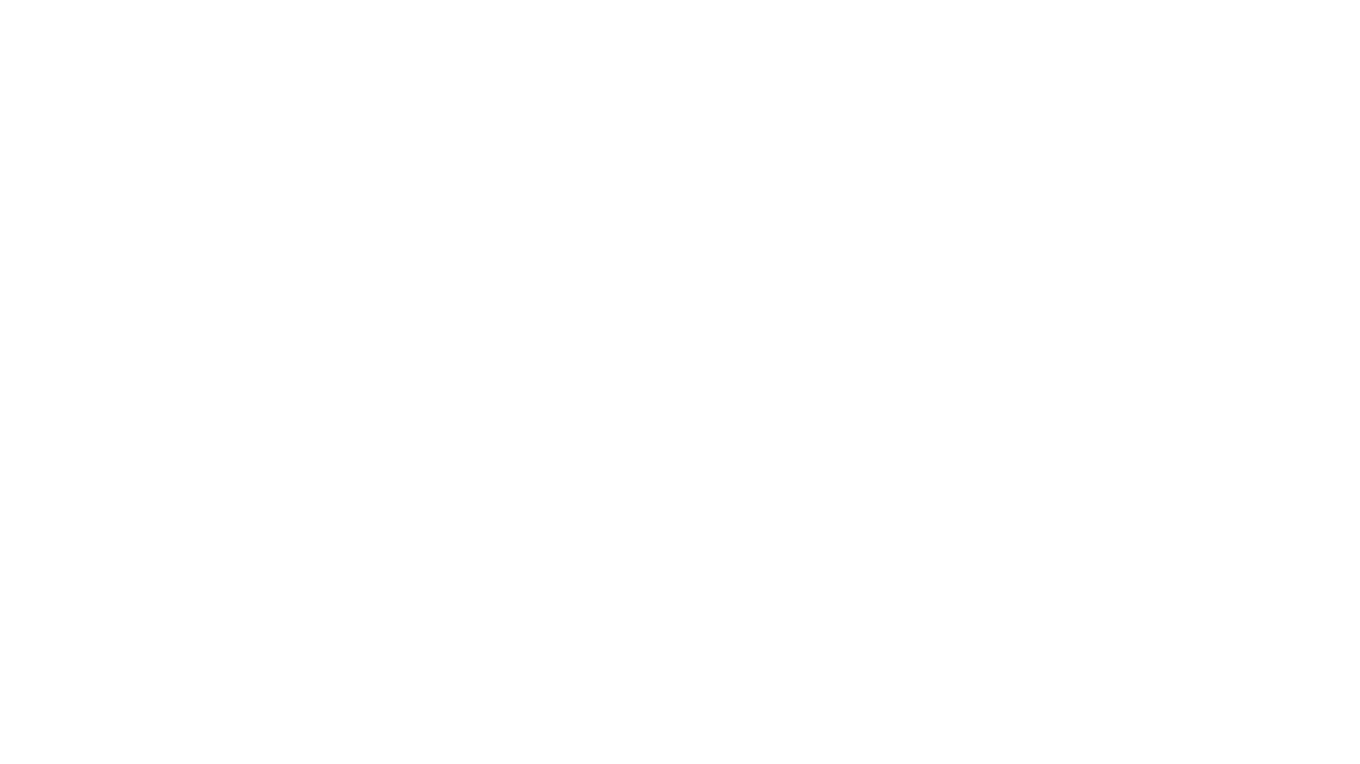
AirGo
AirGo is a front and rear end separation, multi user, multi protocol proxy service management system, simple and easy to use. It supports vless, vmess, shadowsocks, and hysteria2.
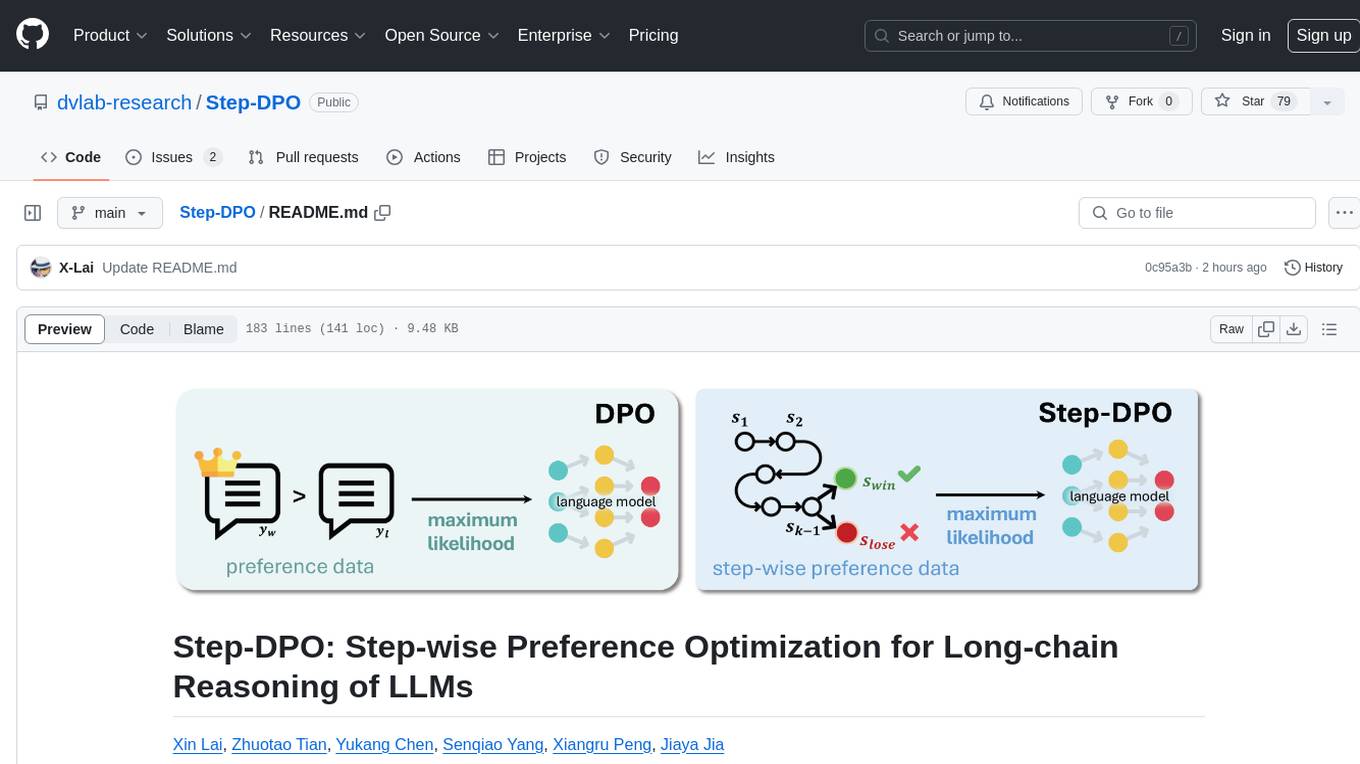
Step-DPO
Step-DPO is a method for enhancing long-chain reasoning ability of LLMs with a data construction pipeline creating a high-quality dataset. It significantly improves performance on math and GSM8K tasks with minimal data and training steps. The tool fine-tunes pre-trained models like Qwen2-7B-Instruct with Step-DPO, achieving superior results compared to other models. It provides scripts for training, evaluation, and deployment, along with examples and acknowledgements.
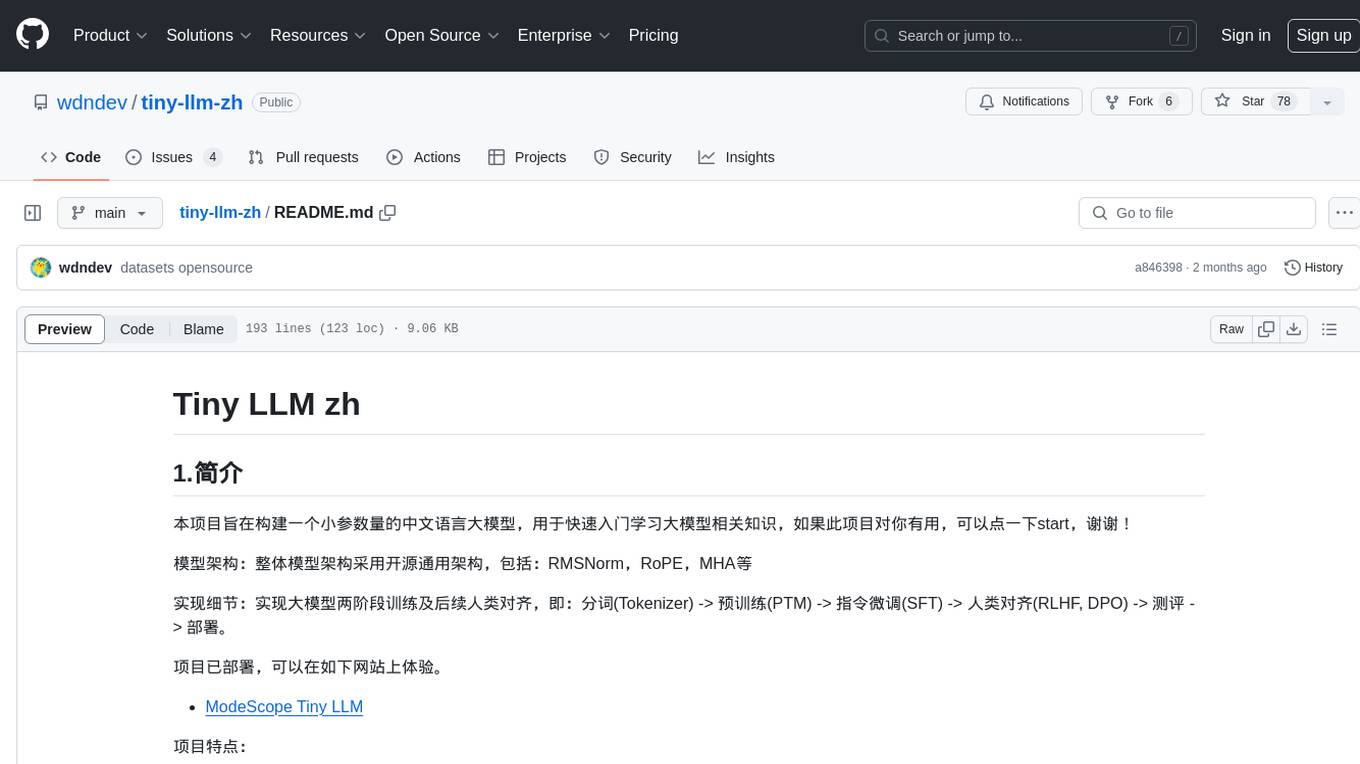
tiny-llm-zh
Tiny LLM zh is a project aimed at building a small-parameter Chinese language large model for quick entry into learning large model-related knowledge. The project implements a two-stage training process for large models and subsequent human alignment, including tokenization, pre-training, instruction fine-tuning, human alignment, evaluation, and deployment. It is deployed on ModeScope Tiny LLM website and features open access to all data and code, including pre-training data and tokenizer. The project trains a tokenizer using 10GB of Chinese encyclopedia text to build a Tiny LLM vocabulary. It supports training with Transformers deepspeed, multiple machine and card support, and Zero optimization techniques. The project has three main branches: llama2_torch, main tiny_llm, and tiny_llm_moe, each with specific modifications and features.
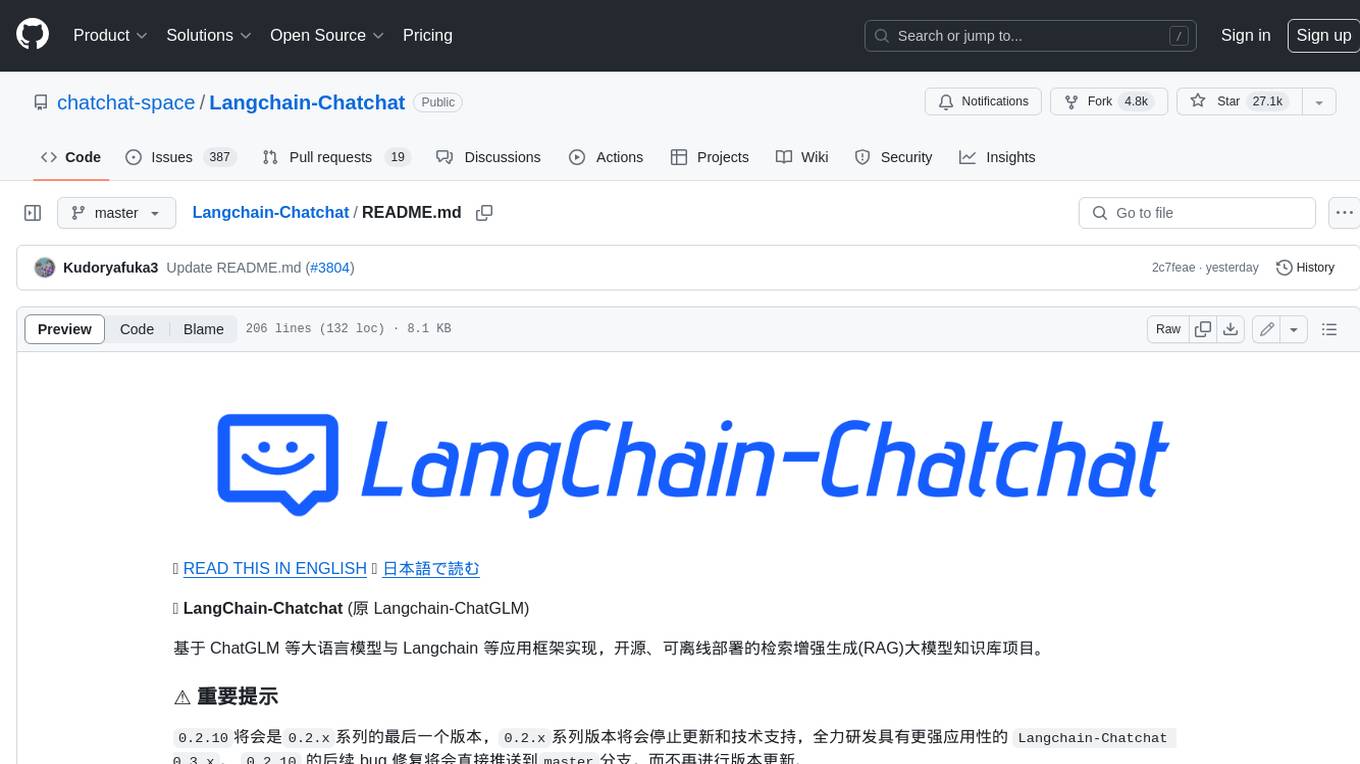
Langchain-Chatchat
LangChain-Chatchat is an open-source, offline-deployable retrieval-enhanced generation (RAG) large model knowledge base project based on large language models such as ChatGLM and application frameworks such as Langchain. It aims to establish a knowledge base Q&A solution that is friendly to Chinese scenarios, supports open-source models, and can run offline.
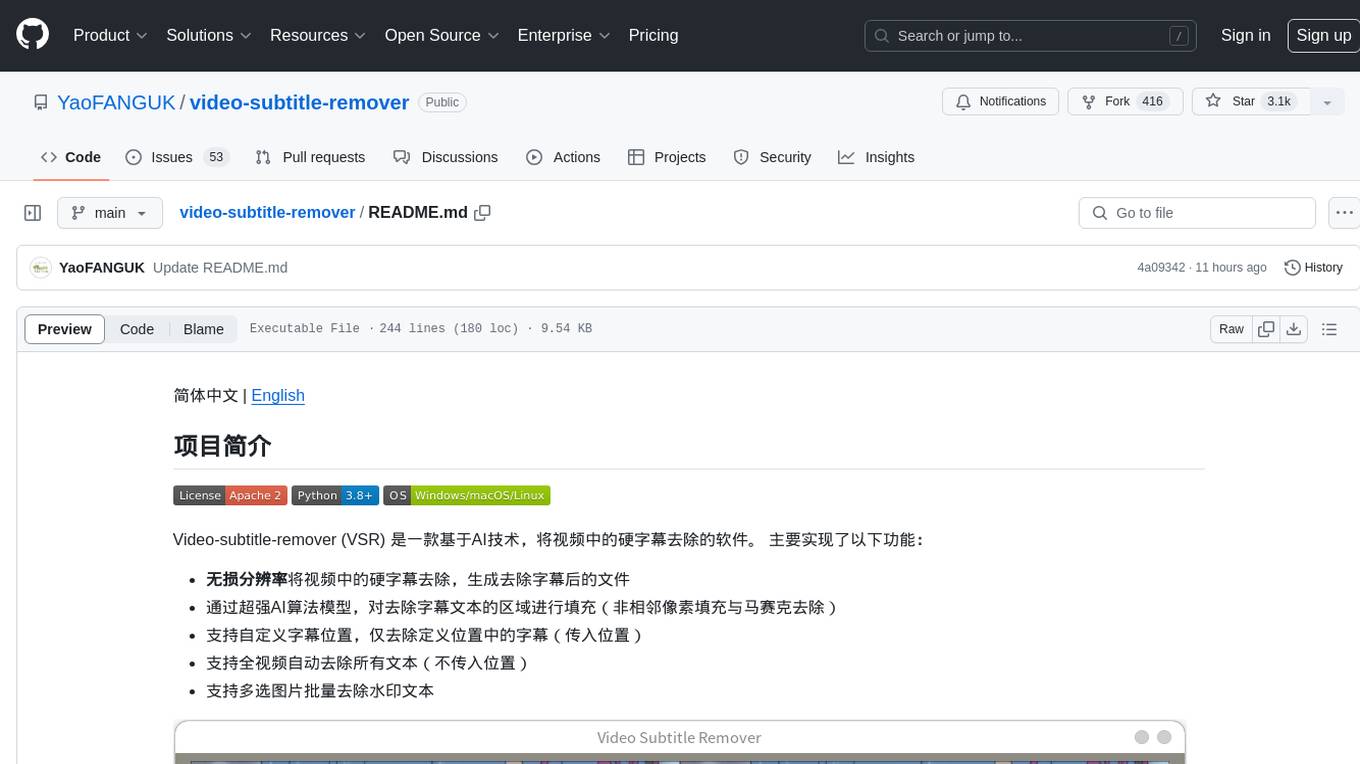
video-subtitle-remover
Video-subtitle-remover (VSR) is a software based on AI technology that removes hard subtitles from videos. It achieves the following functions: - Lossless resolution: Remove hard subtitles from videos, generate files with subtitles removed - Fill the region of removed subtitles using a powerful AI algorithm model (non-adjacent pixel filling and mosaic removal) - Support custom subtitle positions, only remove subtitles in defined positions (input position) - Support automatic removal of all text in the entire video (no input position required) - Support batch removal of watermark text from multiple images.
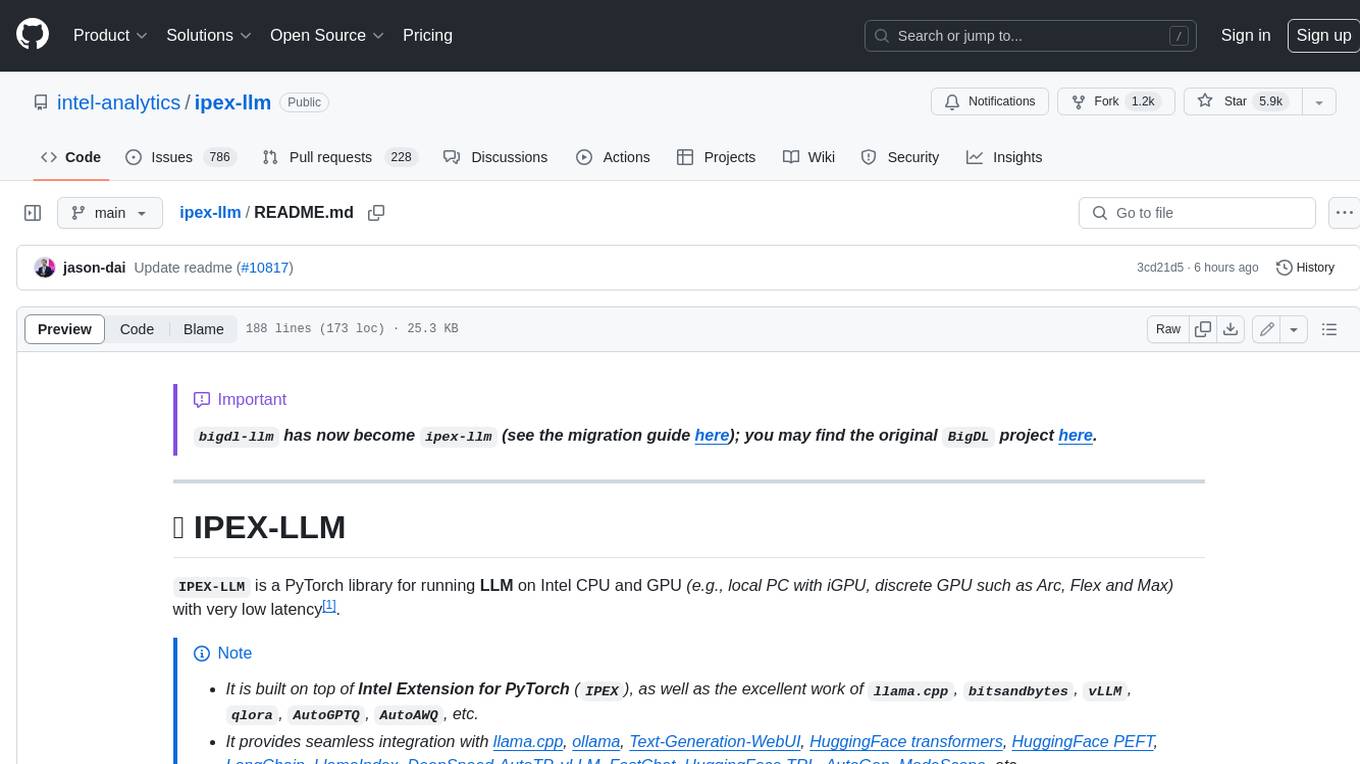
ipex-llm
IPEX-LLM is a PyTorch library for running Large Language Models (LLMs) on Intel CPUs and GPUs with very low latency. It provides seamless integration with various LLM frameworks and tools, including llama.cpp, ollama, Text-Generation-WebUI, HuggingFace transformers, and more. IPEX-LLM has been optimized and verified on over 50 LLM models, including LLaMA, Mistral, Mixtral, Gemma, LLaVA, Whisper, ChatGLM, Baichuan, Qwen, and RWKV. It supports a range of low-bit inference formats, including INT4, FP8, FP4, INT8, INT2, FP16, and BF16, as well as finetuning capabilities for LoRA, QLoRA, DPO, QA-LoRA, and ReLoRA. IPEX-LLM is actively maintained and updated with new features and optimizations, making it a valuable tool for researchers, developers, and anyone interested in exploring and utilizing LLMs.
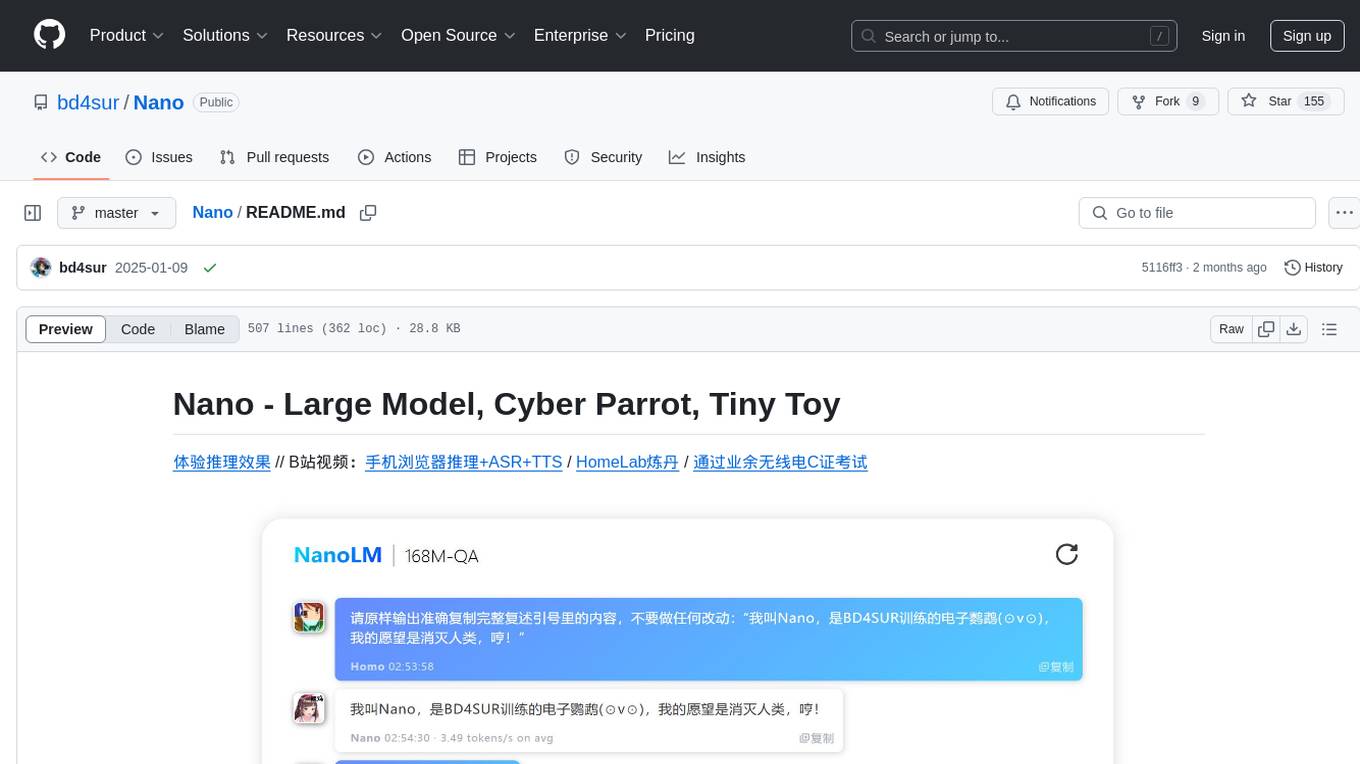
Nano
Nano is a Transformer-based autoregressive language model for personal enjoyment, research, modification, and alchemy. It aims to implement a specific and lightweight Transformer language model based on PyTorch, without relying on Hugging Face. Nano provides pre-training and supervised fine-tuning processes for models with 56M and 168M parameters, along with LoRA plugins. It supports inference on various computing devices and explores the potential of Transformer models in various non-NLP tasks. The repository also includes instructions for experiencing inference effects, installing dependencies, downloading and preprocessing data, pre-training, supervised fine-tuning, model conversion, and various other experiments.
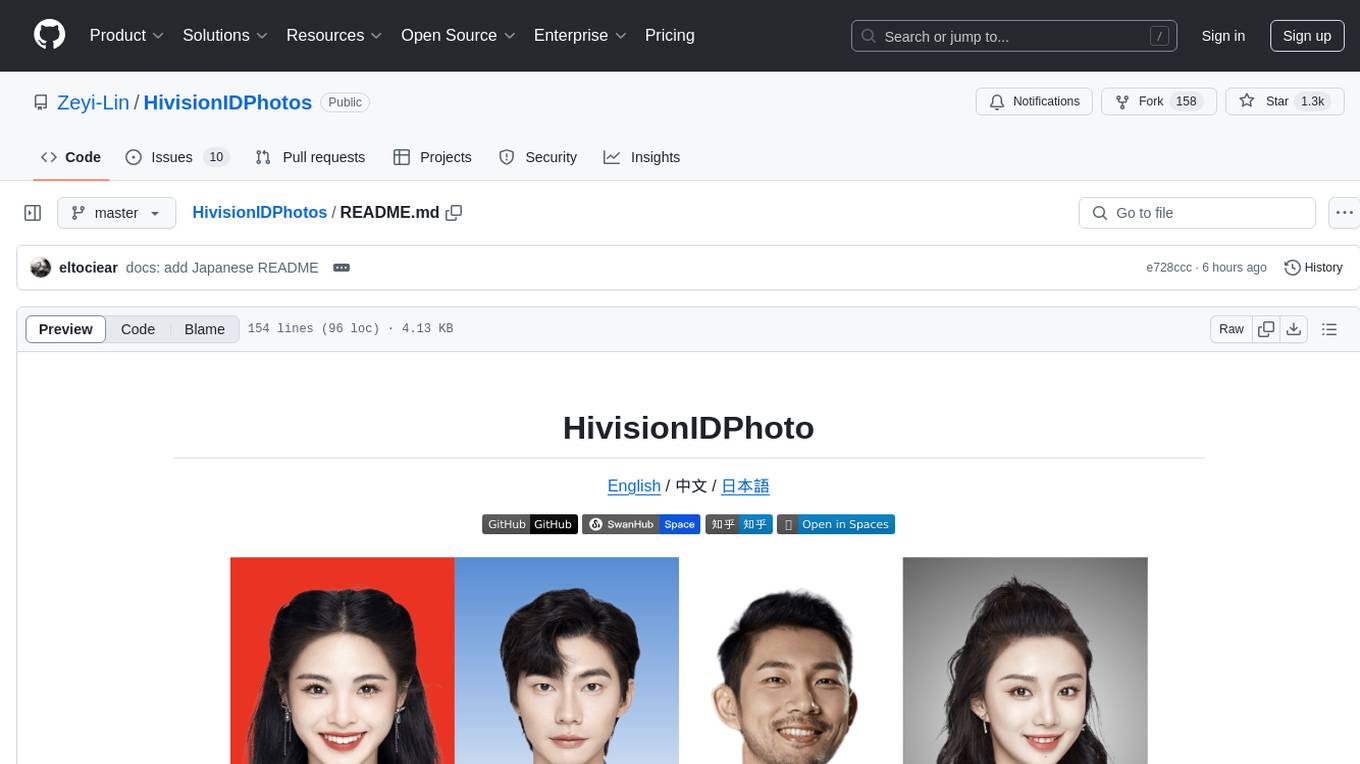
HivisionIDPhotos
HivisionIDPhoto is a practical algorithm for intelligent ID photo creation. It utilizes a comprehensive model workflow to recognize, cut out, and generate ID photos for various user photo scenarios. The tool offers lightweight cutting, standard ID photo generation based on different size specifications, six-inch layout photo generation, beauty enhancement (waiting), and intelligent outfit swapping (waiting). It aims to solve emergency ID photo creation issues.
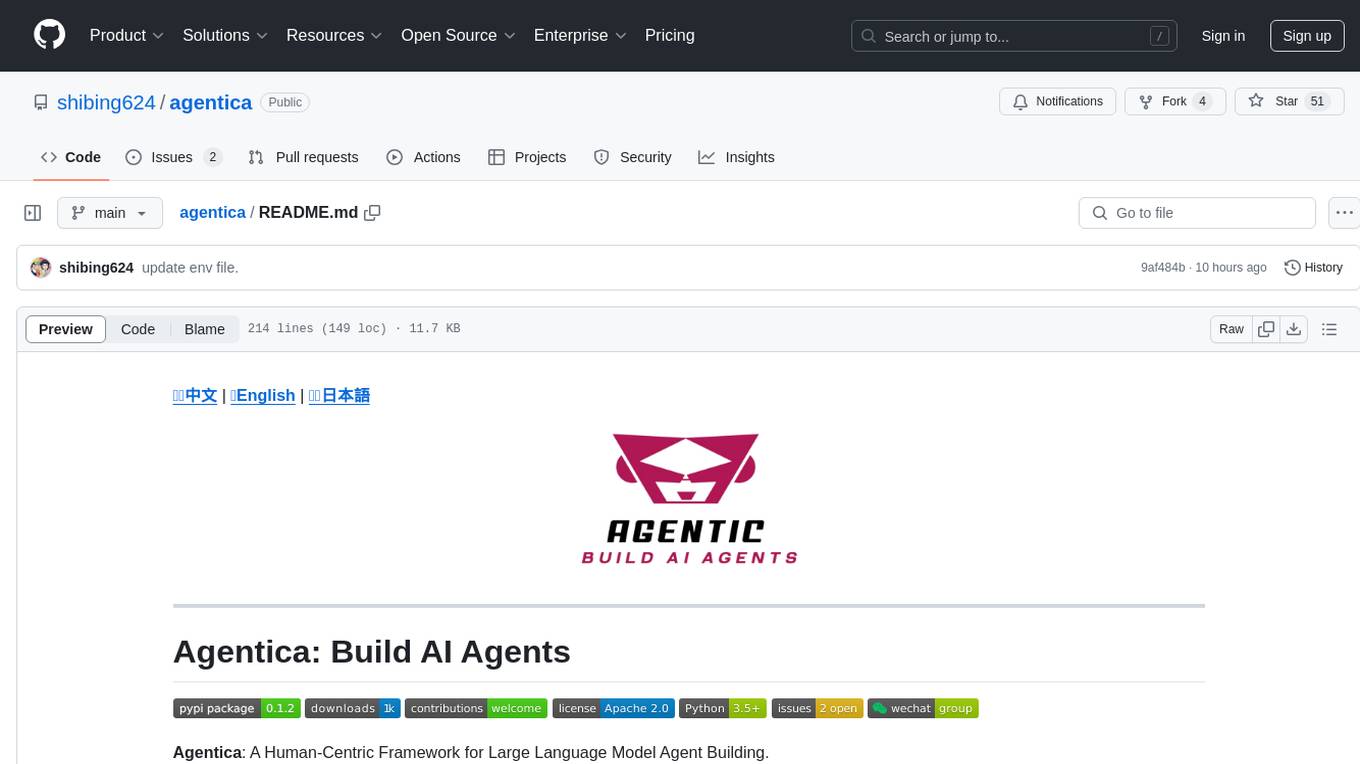
agentica
Agentica is a human-centric framework for building large language model agents. It provides functionalities for planning, memory management, tool usage, and supports features like reflection, planning and execution, RAG, multi-agent, multi-role, and workflow. The tool allows users to quickly code and orchestrate agents, customize prompts, and make API calls to various services. It supports API calls to OpenAI, Azure, Deepseek, Moonshot, Claude, Ollama, and Together. Agentica aims to simplify the process of building AI agents by providing a user-friendly interface and a range of functionalities for agent development.
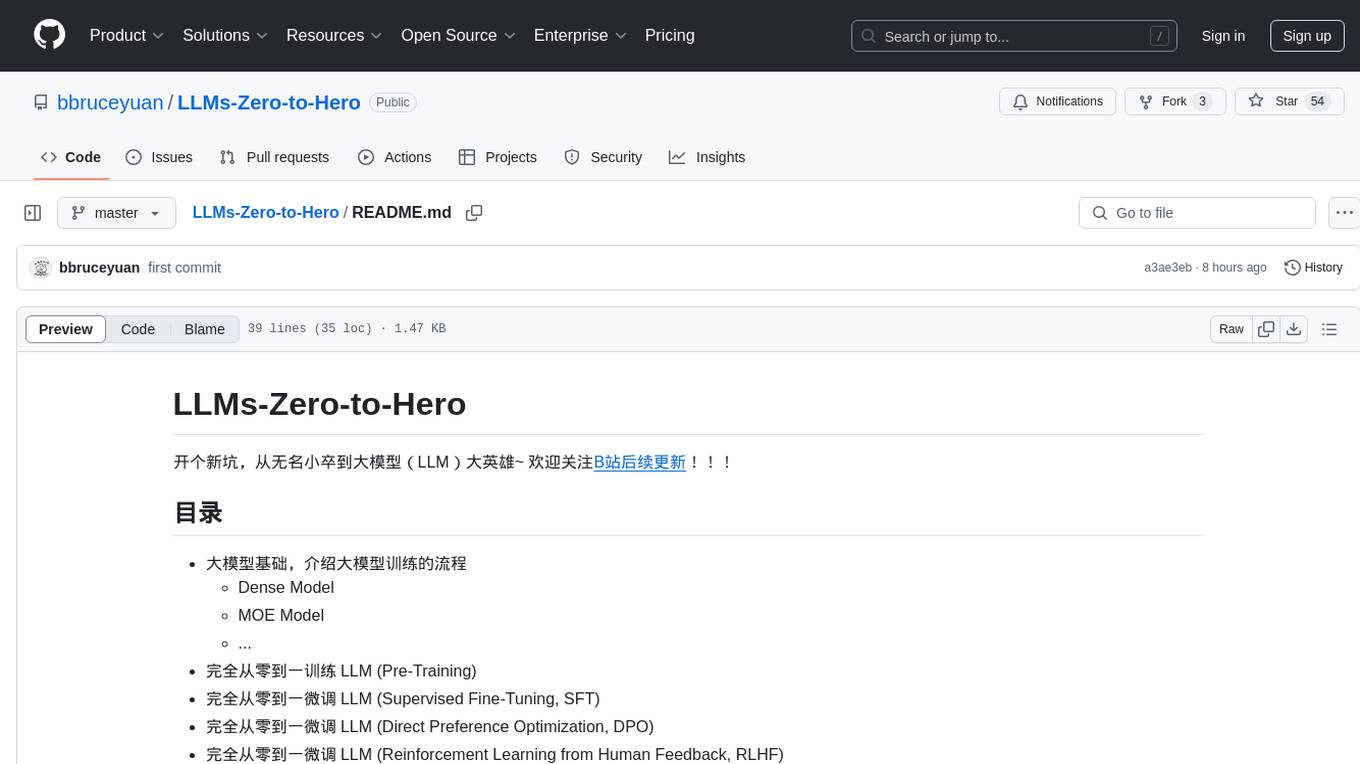
LLMs-Zero-to-Hero
LLMs-Zero-to-Hero is a repository dedicated to training large language models (LLMs) from scratch, covering topics such as dense models, MOE models, pre-training, supervised fine-tuning, direct preference optimization, reinforcement learning from human feedback, and deploying large models. The repository provides detailed learning notes for different chapters, code implementations, and resources for training and deploying LLMs. It aims to guide users from being beginners to proficient in building and deploying large language models.
For similar tasks
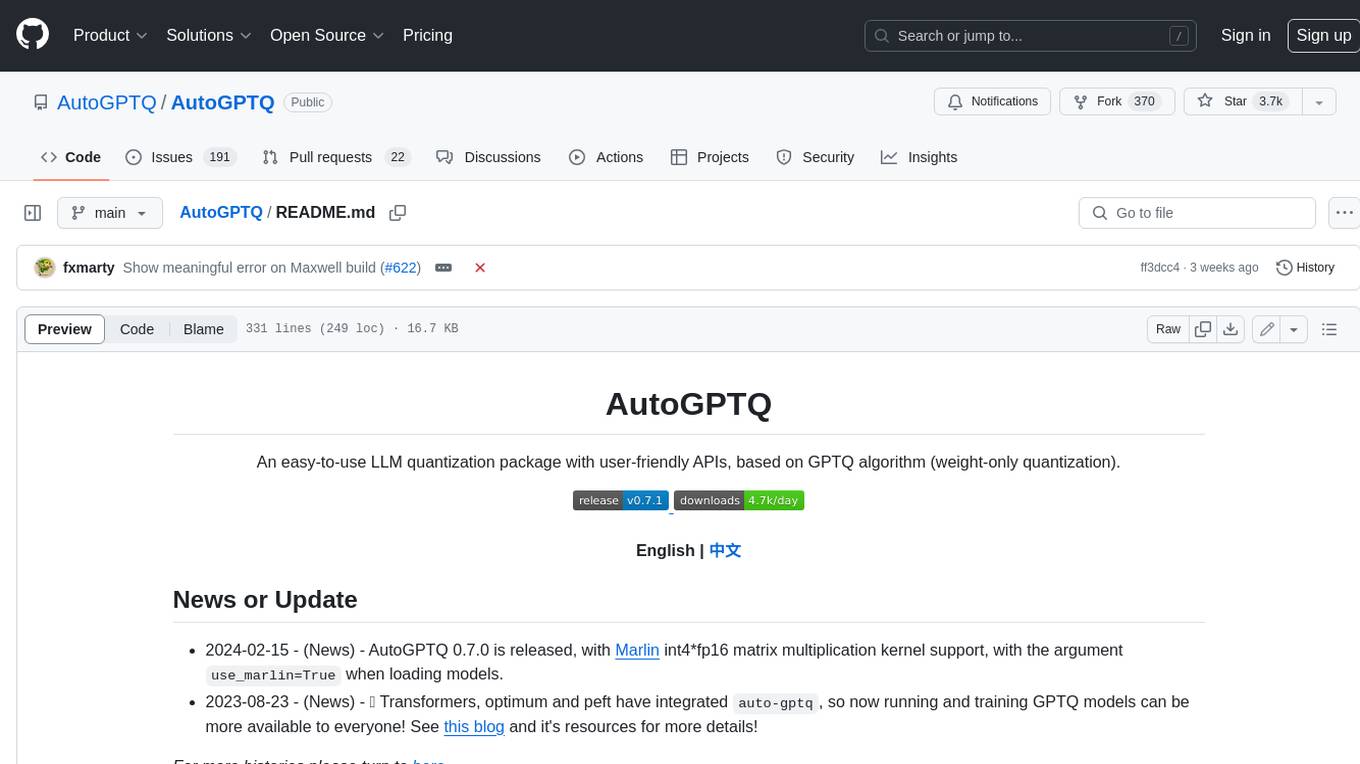
AutoGPTQ
AutoGPTQ is an easy-to-use LLM quantization package with user-friendly APIs, based on GPTQ algorithm (weight-only quantization). It provides a simple and efficient way to quantize large language models (LLMs) to reduce their size and computational cost while maintaining their performance. AutoGPTQ supports a wide range of LLM models, including GPT-2, GPT-J, OPT, and BLOOM. It also supports various evaluation tasks, such as language modeling, sequence classification, and text summarization. With AutoGPTQ, users can easily quantize their LLM models and deploy them on resource-constrained devices, such as mobile phones and embedded systems.
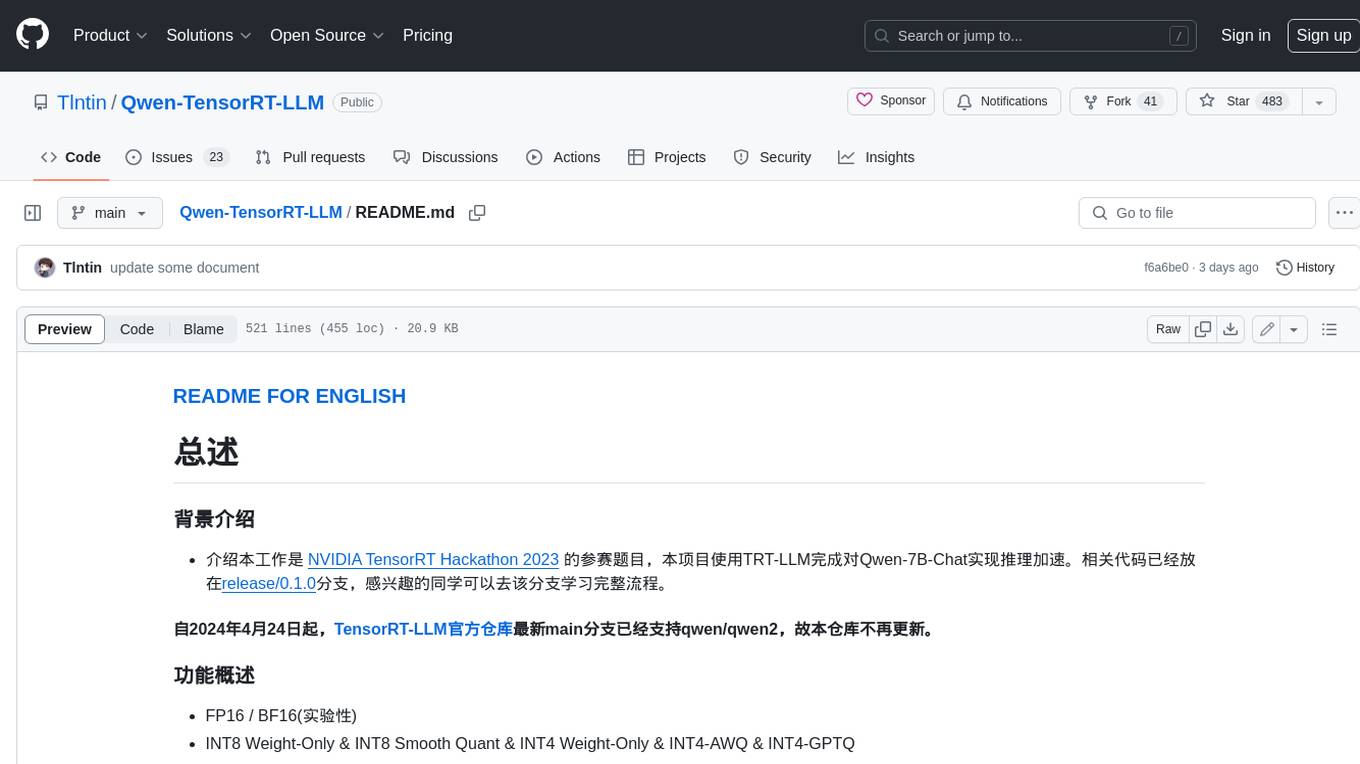
Qwen-TensorRT-LLM
Qwen-TensorRT-LLM is a project developed for the NVIDIA TensorRT Hackathon 2023, focusing on accelerating inference for the Qwen-7B-Chat model using TRT-LLM. The project offers various functionalities such as FP16/BF16 support, INT8 and INT4 quantization options, Tensor Parallel for multi-GPU parallelism, web demo setup with gradio, Triton API deployment for maximum throughput/concurrency, fastapi integration for openai requests, CLI interaction, and langchain support. It supports models like qwen2, qwen, and qwen-vl for both base and chat models. The project also provides tutorials on Bilibili and blogs for adapting Qwen models in NVIDIA TensorRT-LLM, along with hardware requirements and quick start guides for different model types and quantization methods.
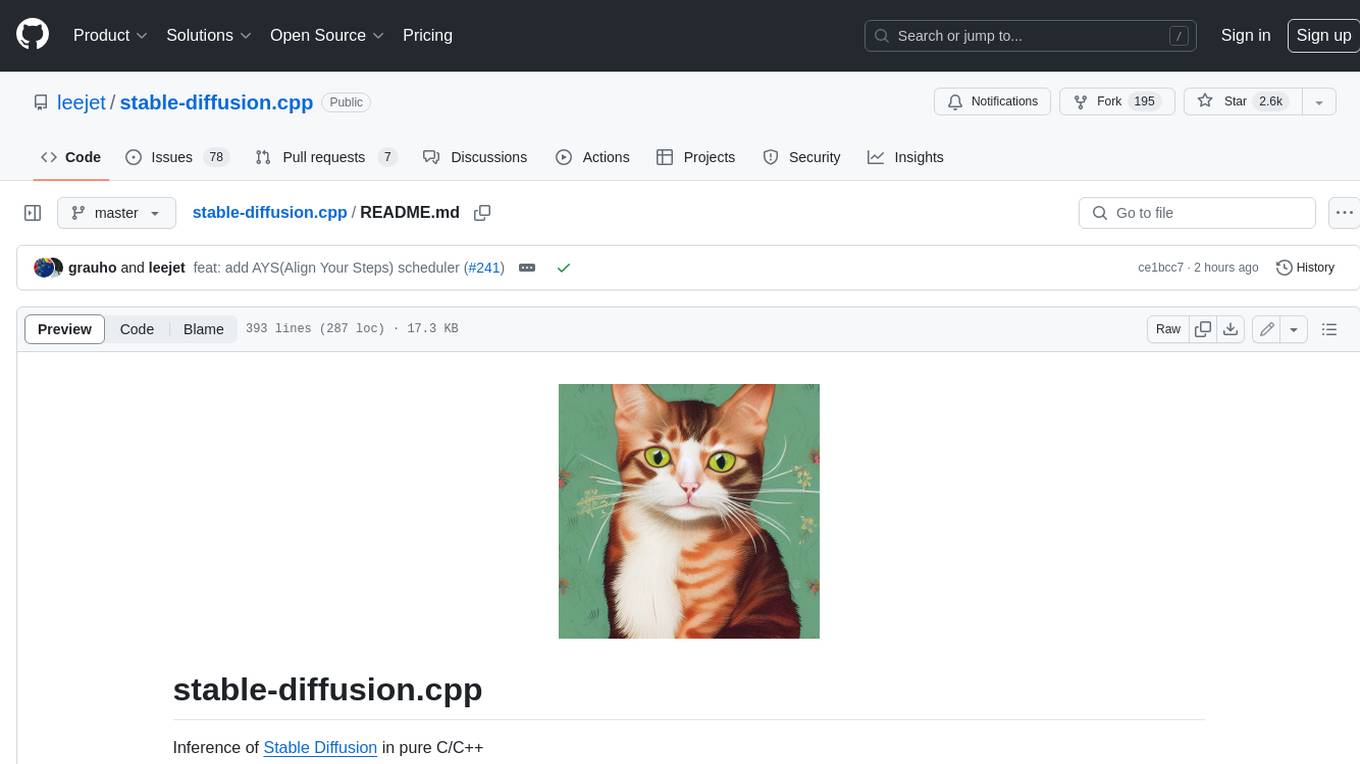
stable-diffusion.cpp
The stable-diffusion.cpp repository provides an implementation for inferring stable diffusion in pure C/C++. It offers features such as support for different versions of stable diffusion, lightweight and dependency-free implementation, various quantization support, memory-efficient CPU inference, GPU acceleration, and more. Users can download the built executable program or build it manually. The repository also includes instructions for downloading weights, building from scratch, using different acceleration methods, running the tool, converting weights, and utilizing various features like Flash Attention, ESRGAN upscaling, PhotoMaker support, and more. Additionally, it mentions future TODOs and provides information on memory requirements, bindings, UIs, contributors, and references.
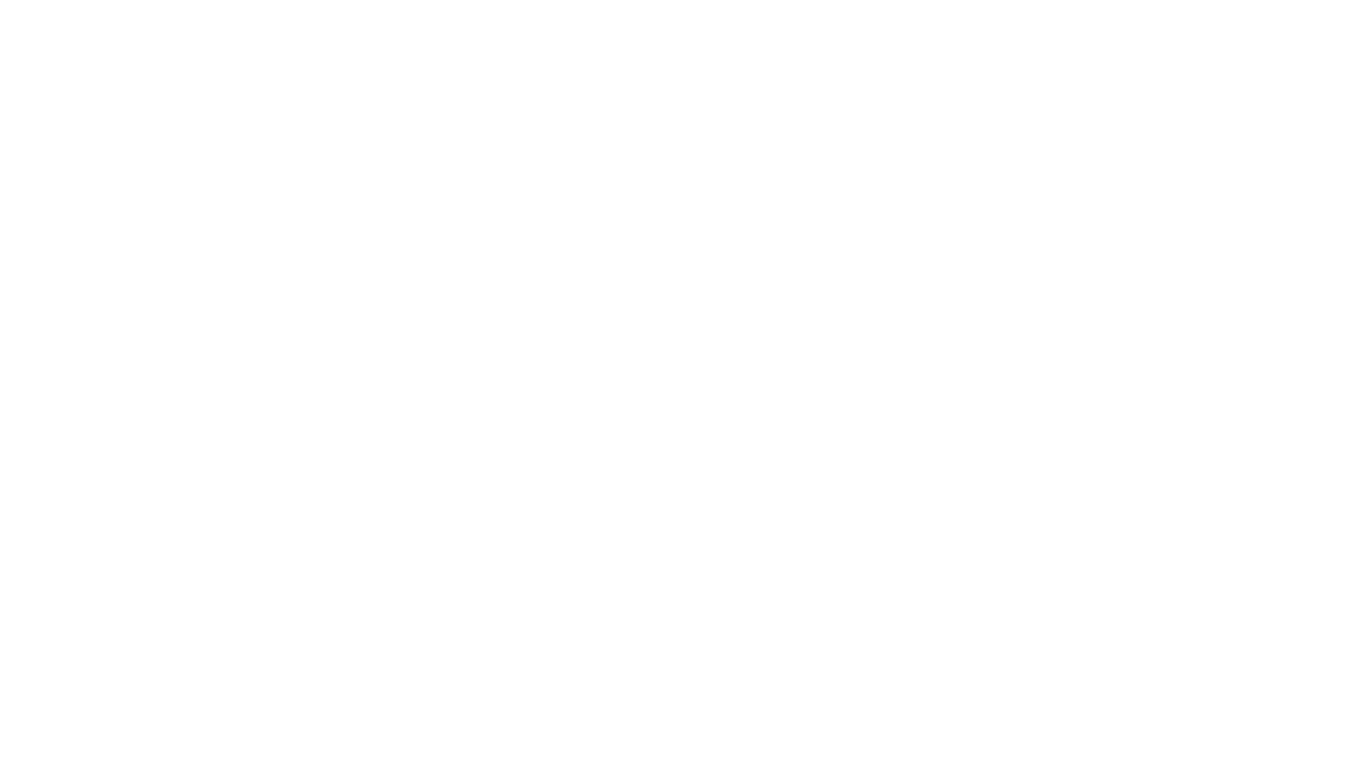
LMOps
LMOps is a research initiative focusing on fundamental research and technology for building AI products with foundation models, particularly enabling AI capabilities with Large Language Models (LLMs) and Generative AI models. The project explores various aspects such as prompt optimization, longer context handling, LLM alignment, acceleration of LLMs, LLM customization, and understanding in-context learning. It also includes tools like Promptist for automatic prompt optimization, Structured Prompting for efficient long-sequence prompts consumption, and X-Prompt for extensible prompts beyond natural language. Additionally, LLMA accelerators are developed to speed up LLM inference by referencing and copying text spans from documents. The project aims to advance technologies that facilitate prompting language models and enhance the performance of LLMs in various scenarios.
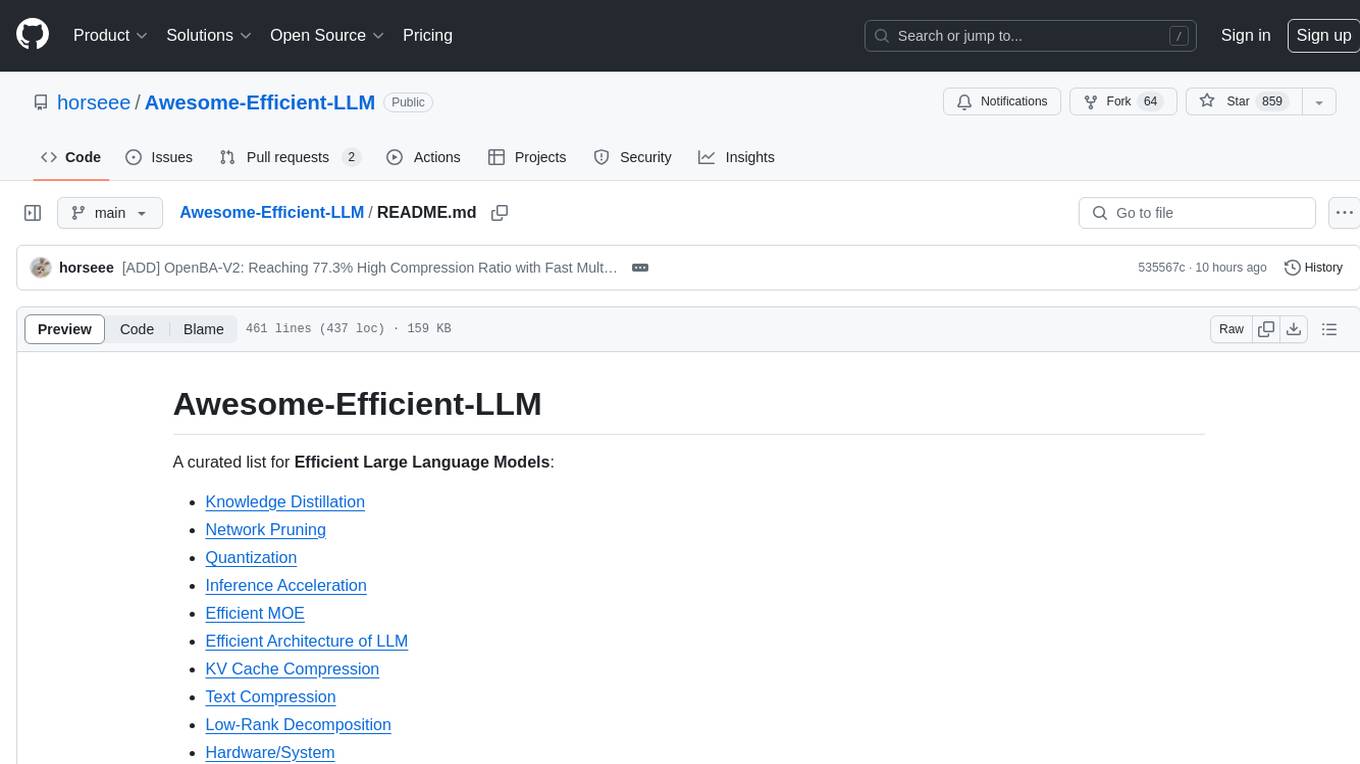
Awesome-Efficient-LLM
Awesome-Efficient-LLM is a curated list focusing on efficient large language models. It includes topics such as knowledge distillation, network pruning, quantization, inference acceleration, efficient MOE, efficient architecture of LLM, KV cache compression, text compression, low-rank decomposition, hardware/system, tuning, and survey. The repository provides a collection of papers and projects related to improving the efficiency of large language models through various techniques like sparsity, quantization, and compression.
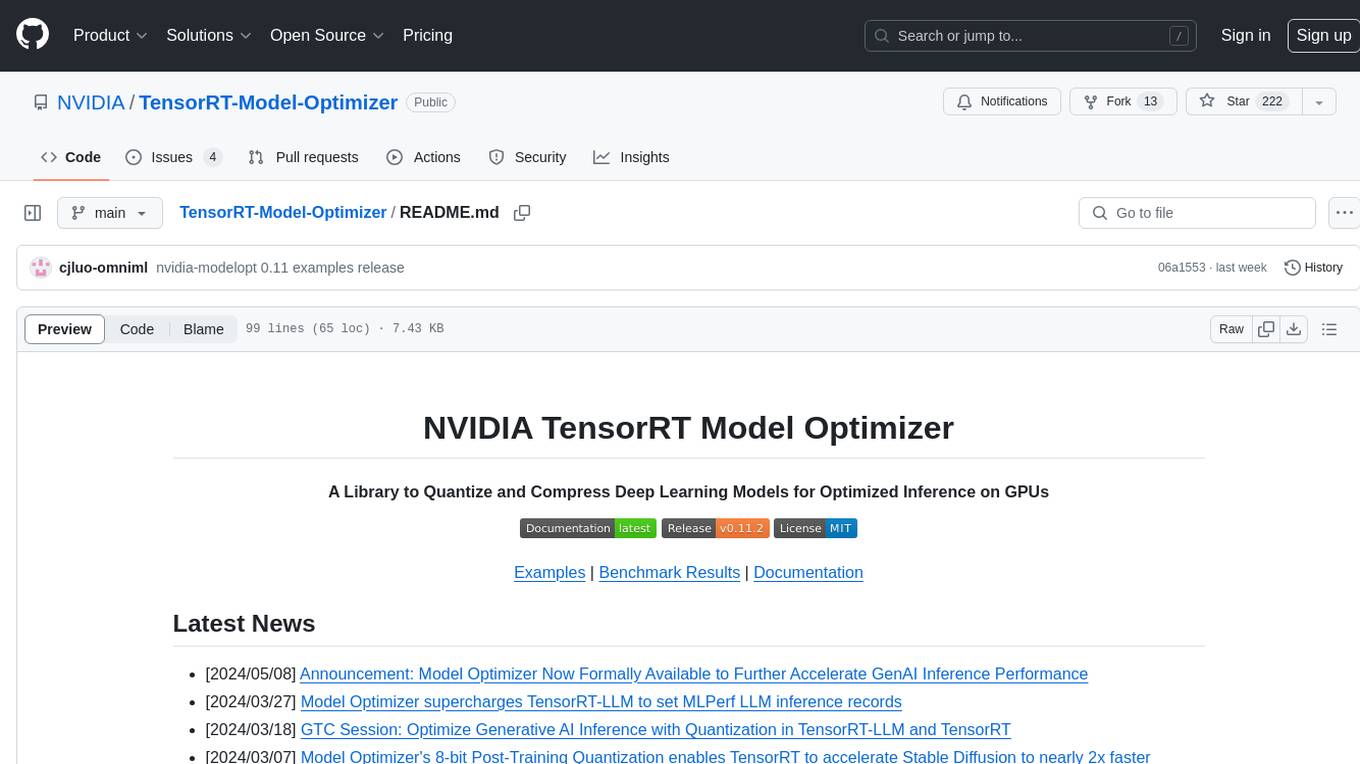
TensorRT-Model-Optimizer
The NVIDIA TensorRT Model Optimizer is a library designed to quantize and compress deep learning models for optimized inference on GPUs. It offers state-of-the-art model optimization techniques including quantization and sparsity to reduce inference costs for generative AI models. Users can easily stack different optimization techniques to produce quantized checkpoints from torch or ONNX models. The quantized checkpoints are ready for deployment in inference frameworks like TensorRT-LLM or TensorRT, with planned integrations for NVIDIA NeMo and Megatron-LM. The tool also supports 8-bit quantization with Stable Diffusion for enterprise users on NVIDIA NIM. Model Optimizer is available for free on NVIDIA PyPI, and this repository serves as a platform for sharing examples, GPU-optimized recipes, and collecting community feedback.
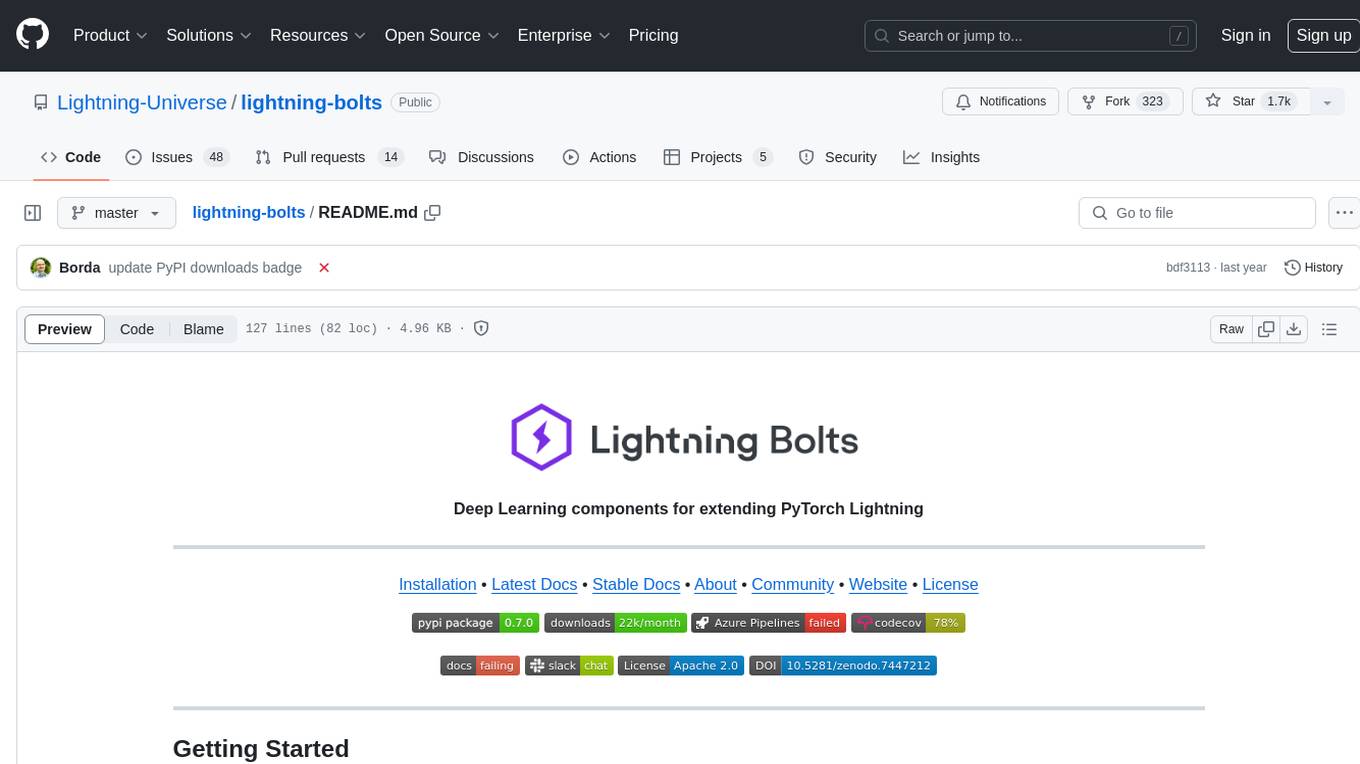
lightning-bolts
Bolts package provides a variety of components to extend PyTorch Lightning, such as callbacks & datasets, for applied research and production. Users can accelerate Lightning training with the Torch ORT Callback to optimize ONNX graph for faster training & inference. Additionally, users can introduce sparsity with the SparseMLCallback to accelerate inference by leveraging the DeepSparse engine. Specific research implementations are encouraged, with contributions that help train SSL models and integrate with Lightning Flash for state-of-the-art models in applied research.
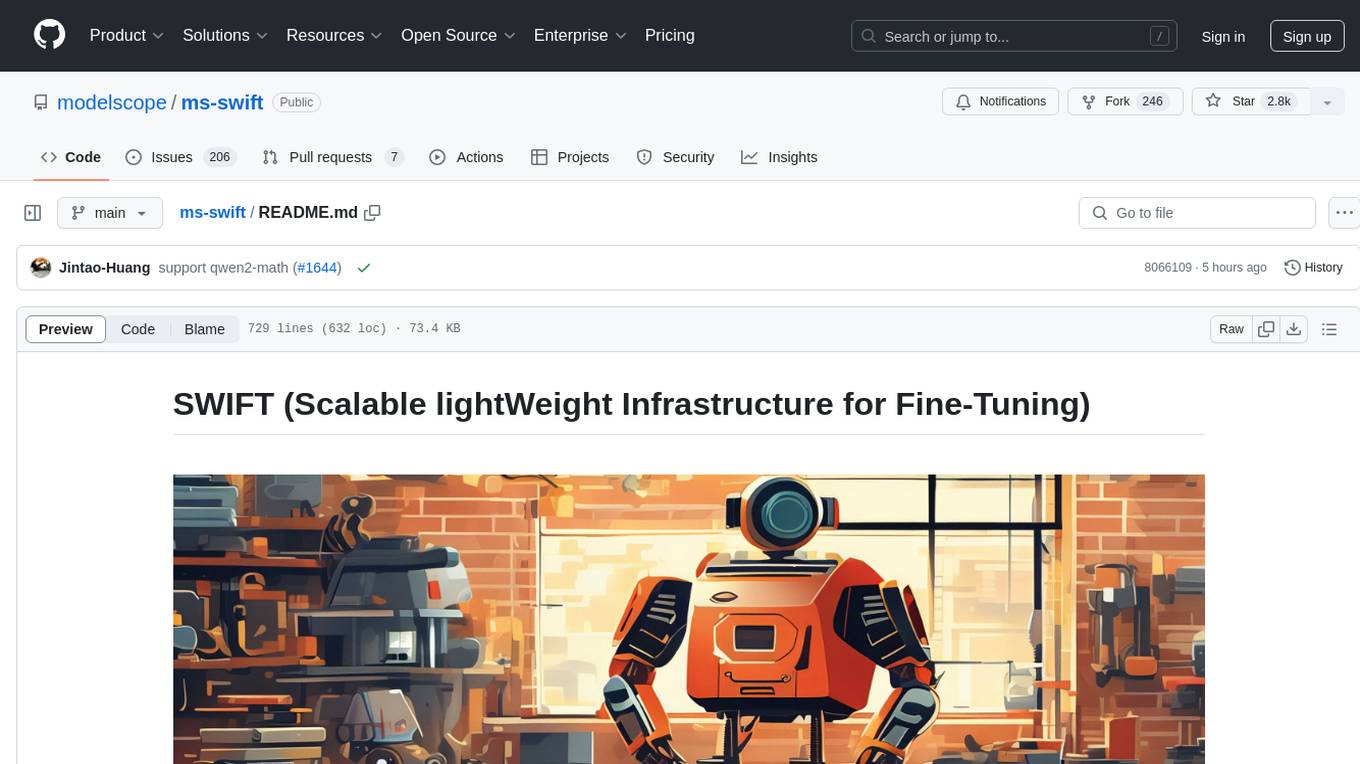
ms-swift
ms-swift is an official framework provided by the ModelScope community for fine-tuning and deploying large language models and multi-modal large models. It supports training, inference, evaluation, quantization, and deployment of over 400 large models and 100+ multi-modal large models. The framework includes various training technologies and accelerates inference, evaluation, and deployment modules. It offers a Gradio-based Web-UI interface and best practices for easy application of large models. ms-swift supports a wide range of model types, dataset types, hardware support, lightweight training methods, distributed training techniques, quantization training, RLHF training, multi-modal training, interface training, plugin and extension support, inference acceleration engines, model evaluation, and model quantization.
For similar jobs
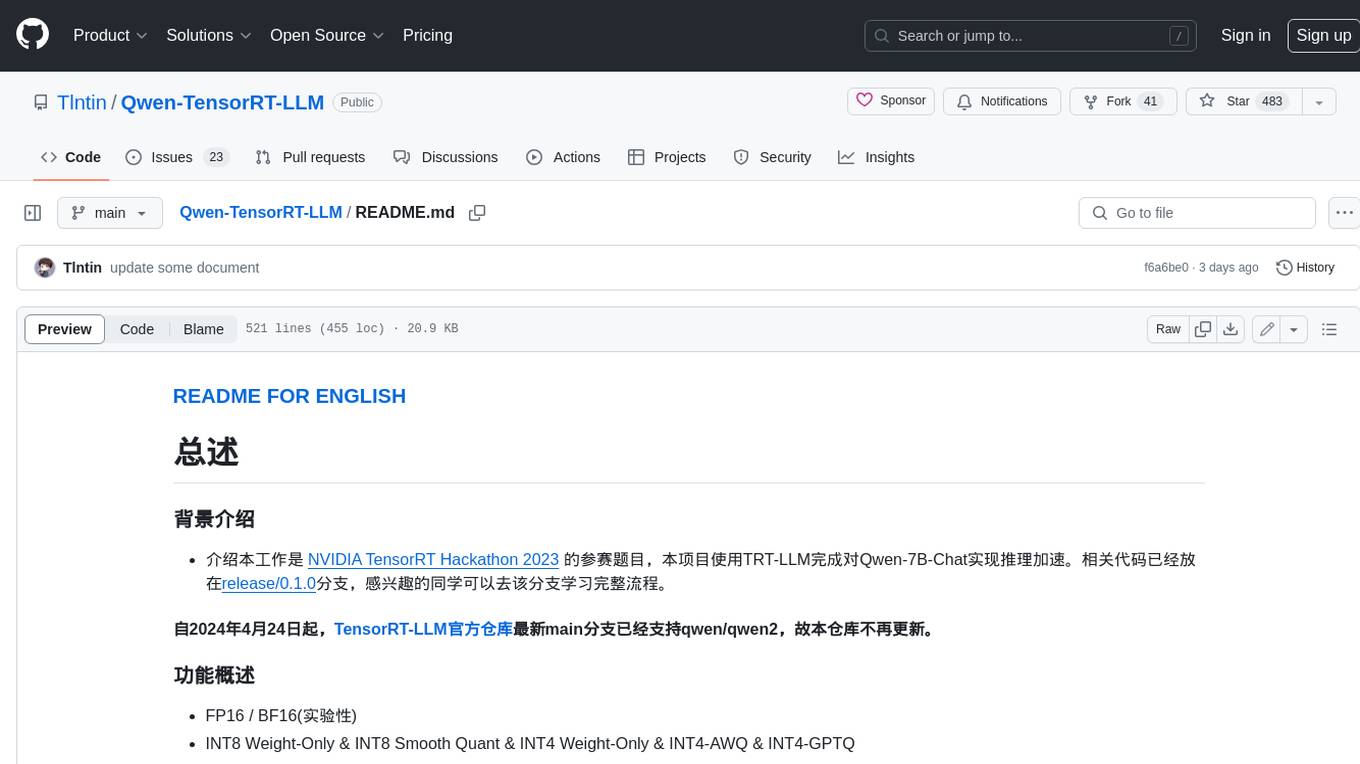
Qwen-TensorRT-LLM
Qwen-TensorRT-LLM is a project developed for the NVIDIA TensorRT Hackathon 2023, focusing on accelerating inference for the Qwen-7B-Chat model using TRT-LLM. The project offers various functionalities such as FP16/BF16 support, INT8 and INT4 quantization options, Tensor Parallel for multi-GPU parallelism, web demo setup with gradio, Triton API deployment for maximum throughput/concurrency, fastapi integration for openai requests, CLI interaction, and langchain support. It supports models like qwen2, qwen, and qwen-vl for both base and chat models. The project also provides tutorials on Bilibili and blogs for adapting Qwen models in NVIDIA TensorRT-LLM, along with hardware requirements and quick start guides for different model types and quantization methods.
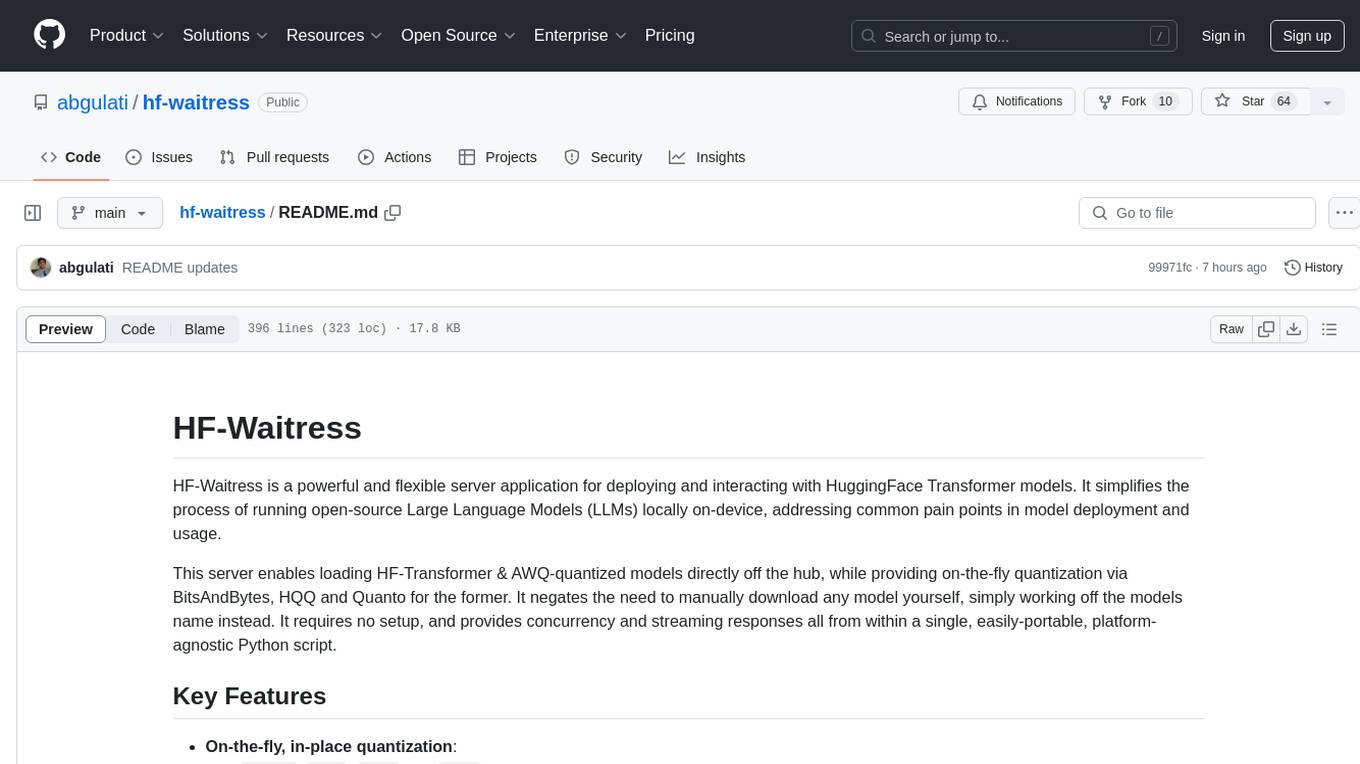
hf-waitress
HF-Waitress is a powerful server application for deploying and interacting with HuggingFace Transformer models. It simplifies running open-source Large Language Models (LLMs) locally on-device, providing on-the-fly quantization via BitsAndBytes, HQQ, and Quanto. It requires no manual model downloads, offers concurrency, streaming responses, and supports various hardware and platforms. The server uses a `config.json` file for easy configuration management and provides detailed error handling and logging.
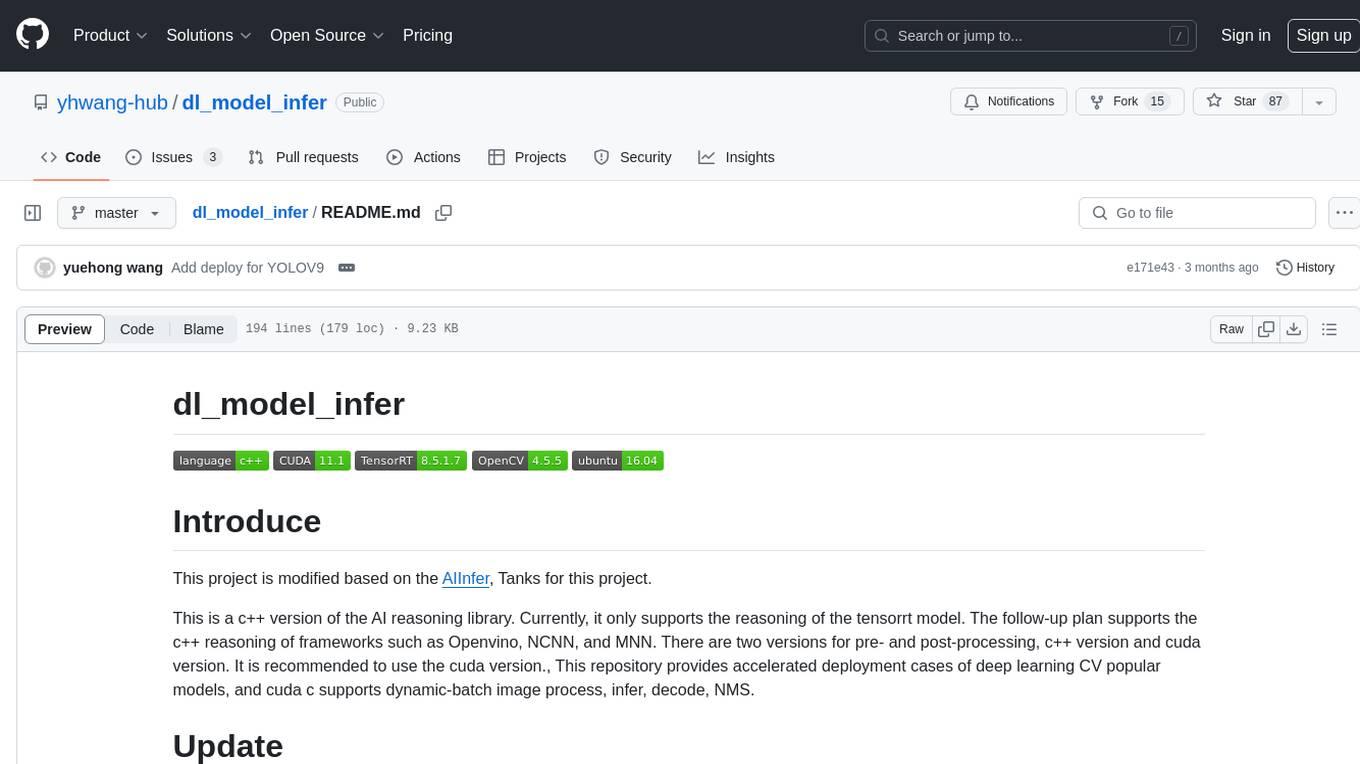
dl_model_infer
This project is a c++ version of the AI reasoning library that supports the reasoning of tensorrt models. It provides accelerated deployment cases of deep learning CV popular models and supports dynamic-batch image processing, inference, decode, and NMS. The project has been updated with various models and provides tutorials for model exports. It also includes a producer-consumer inference model for specific tasks. The project directory includes implementations for model inference applications, backend reasoning classes, post-processing, pre-processing, and target detection and tracking. Speed tests have been conducted on various models, and onnx downloads are available for different models.
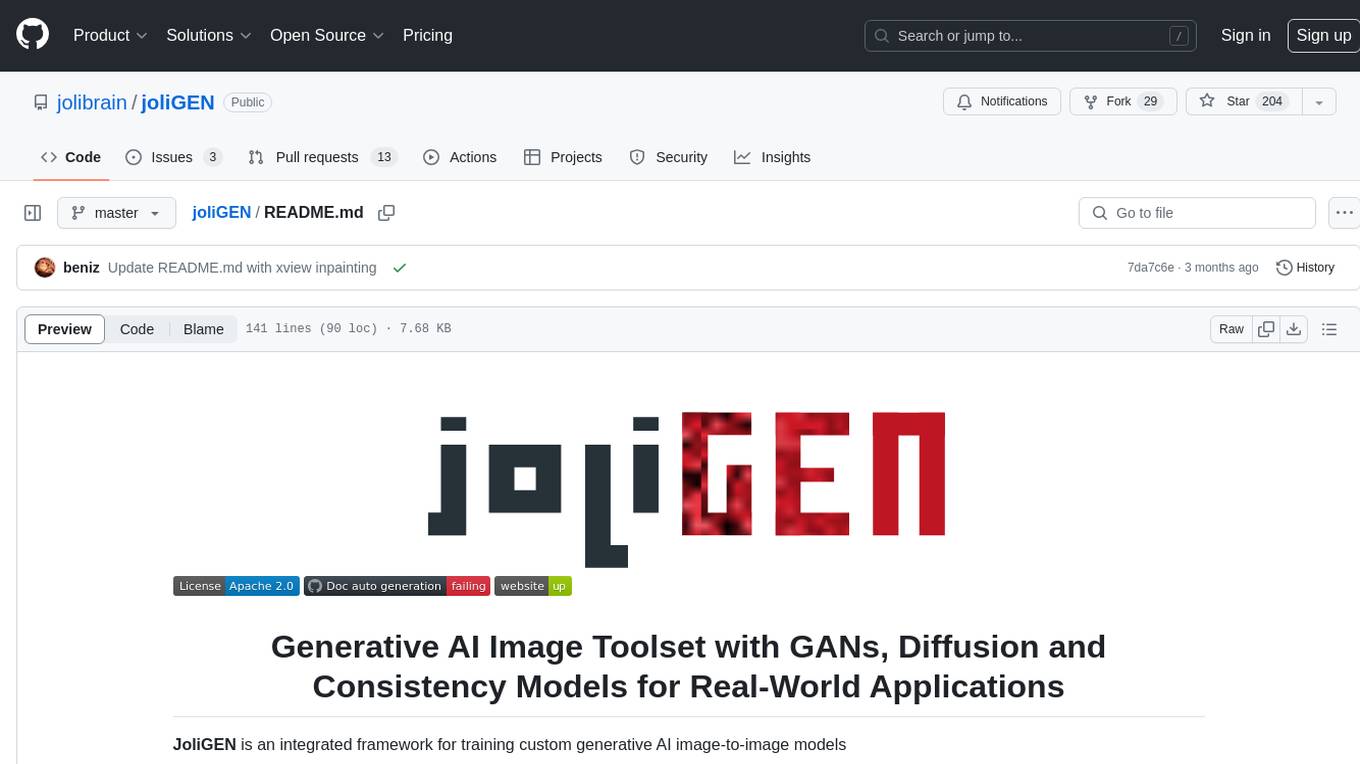
joliGEN
JoliGEN is an integrated framework for training custom generative AI image-to-image models. It implements GAN, Diffusion, and Consistency models for various image translation tasks, including domain and style adaptation with conservation of semantics. The tool is designed for real-world applications such as Controlled Image Generation, Augmented Reality, Dataset Smart Augmentation, and Synthetic to Real transforms. JoliGEN allows for fast and stable training with a REST API server for simplified deployment. It offers a wide range of options and parameters with detailed documentation available for models, dataset formats, and data augmentation.
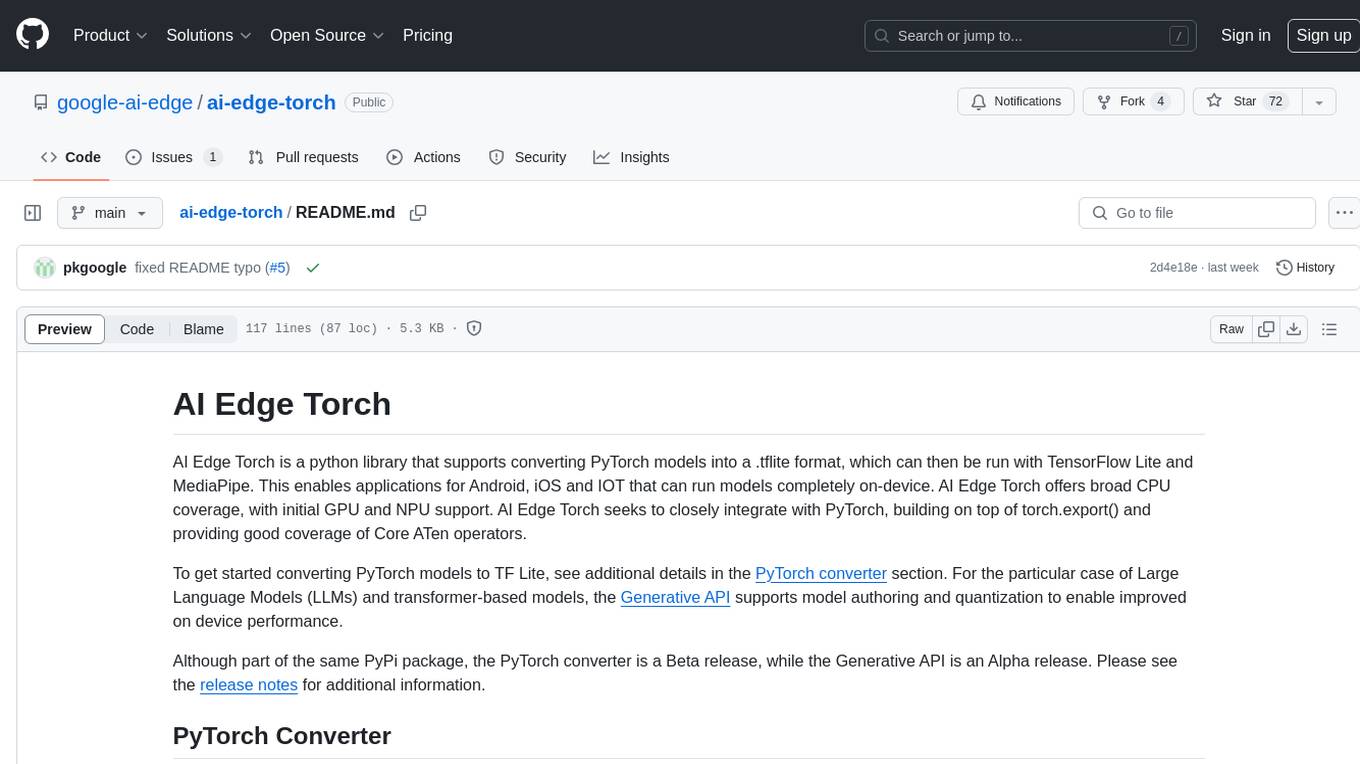
ai-edge-torch
AI Edge Torch is a Python library that supports converting PyTorch models into a .tflite format for on-device applications on Android, iOS, and IoT devices. It offers broad CPU coverage with initial GPU and NPU support, closely integrating with PyTorch and providing good coverage of Core ATen operators. The library includes a PyTorch converter for model conversion and a Generative API for authoring mobile-optimized PyTorch Transformer models, enabling easy deployment of Large Language Models (LLMs) on mobile devices.
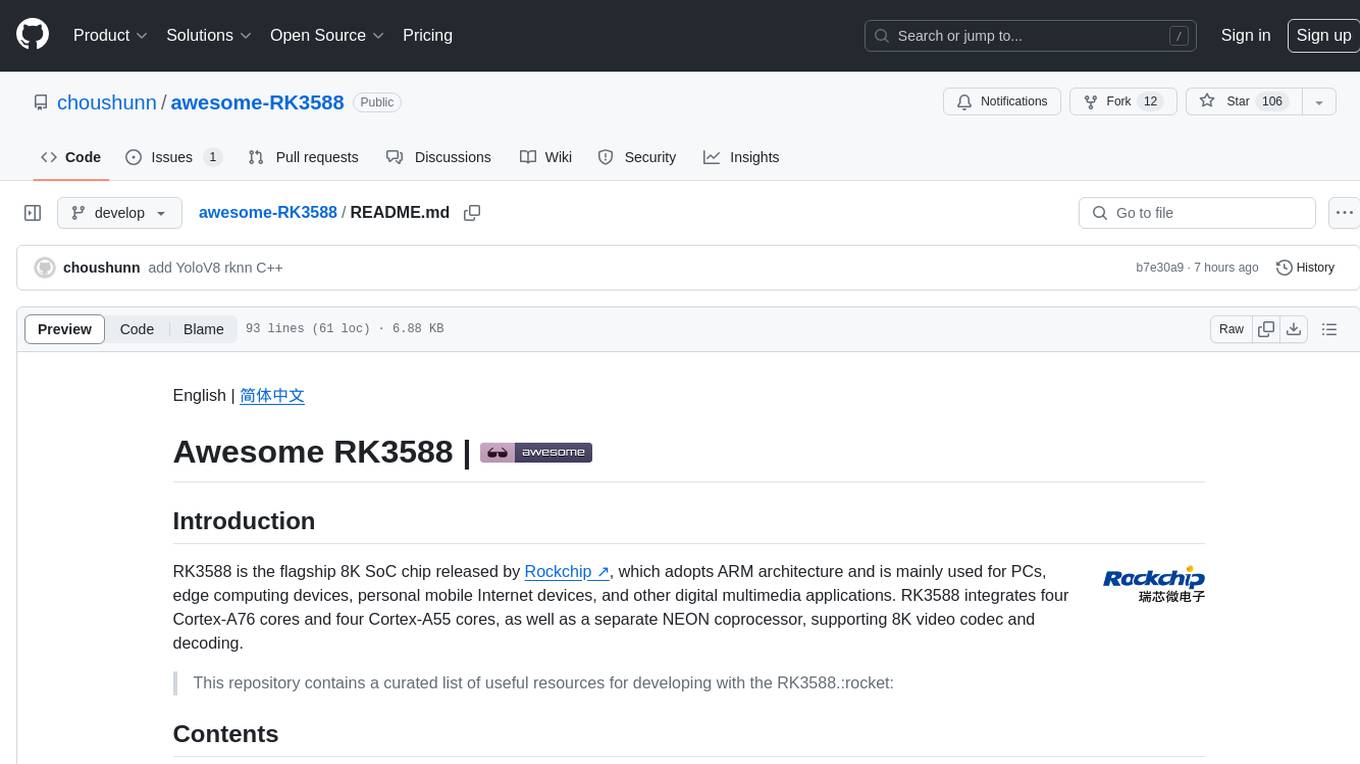
awesome-RK3588
RK3588 is a flagship 8K SoC chip by Rockchip, integrating Cortex-A76 and Cortex-A55 cores with NEON coprocessor for 8K video codec. This repository curates resources for developing with RK3588, including official resources, RKNN models, projects, development boards, documentation, tools, and sample code.
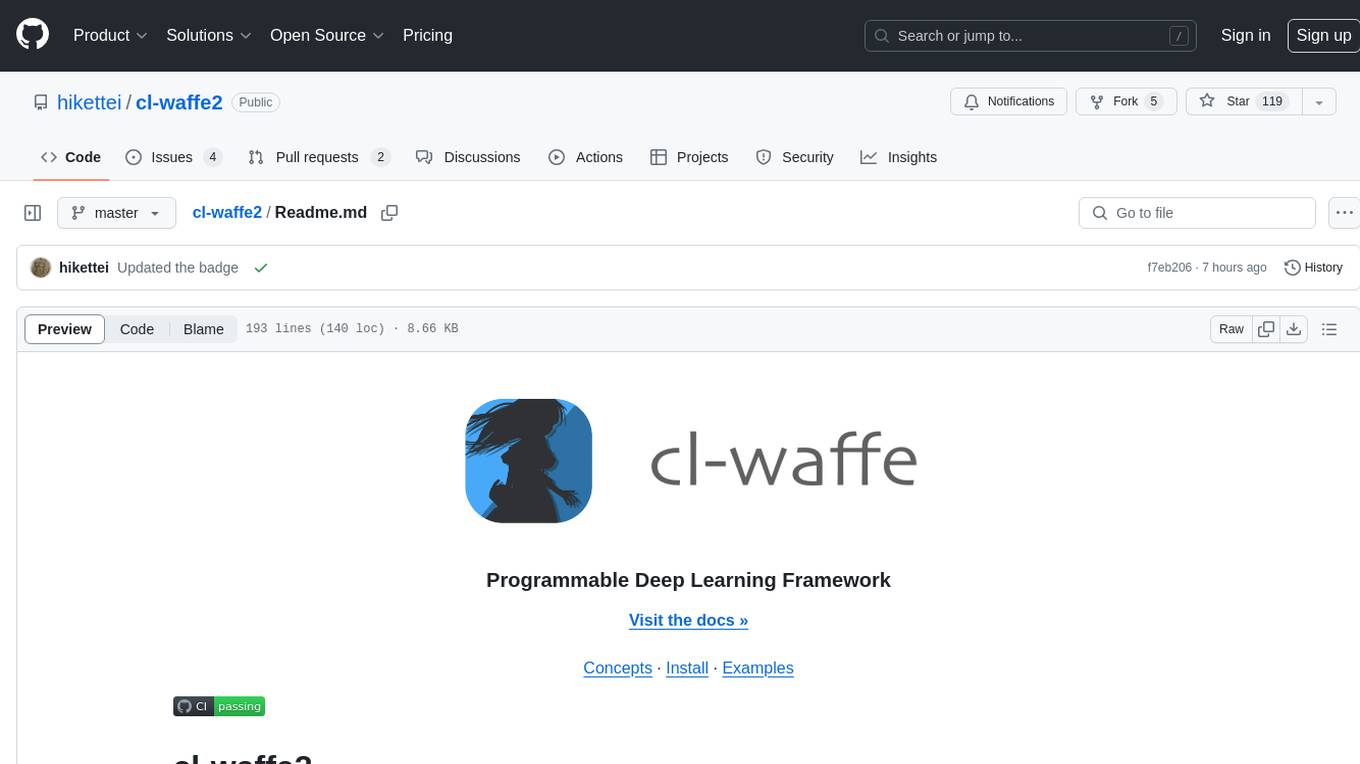
cl-waffe2
cl-waffe2 is an experimental deep learning framework in Common Lisp, providing fast, systematic, and customizable matrix operations, reverse mode tape-based Automatic Differentiation, and neural network model building and training features accelerated by a JIT Compiler. It offers abstraction layers, extensibility, inlining, graph-level optimization, visualization, debugging, systematic nodes, and symbolic differentiation. Users can easily write extensions and optimize their networks without overheads. The framework is designed to eliminate barriers between users and developers, allowing for easy customization and extension.
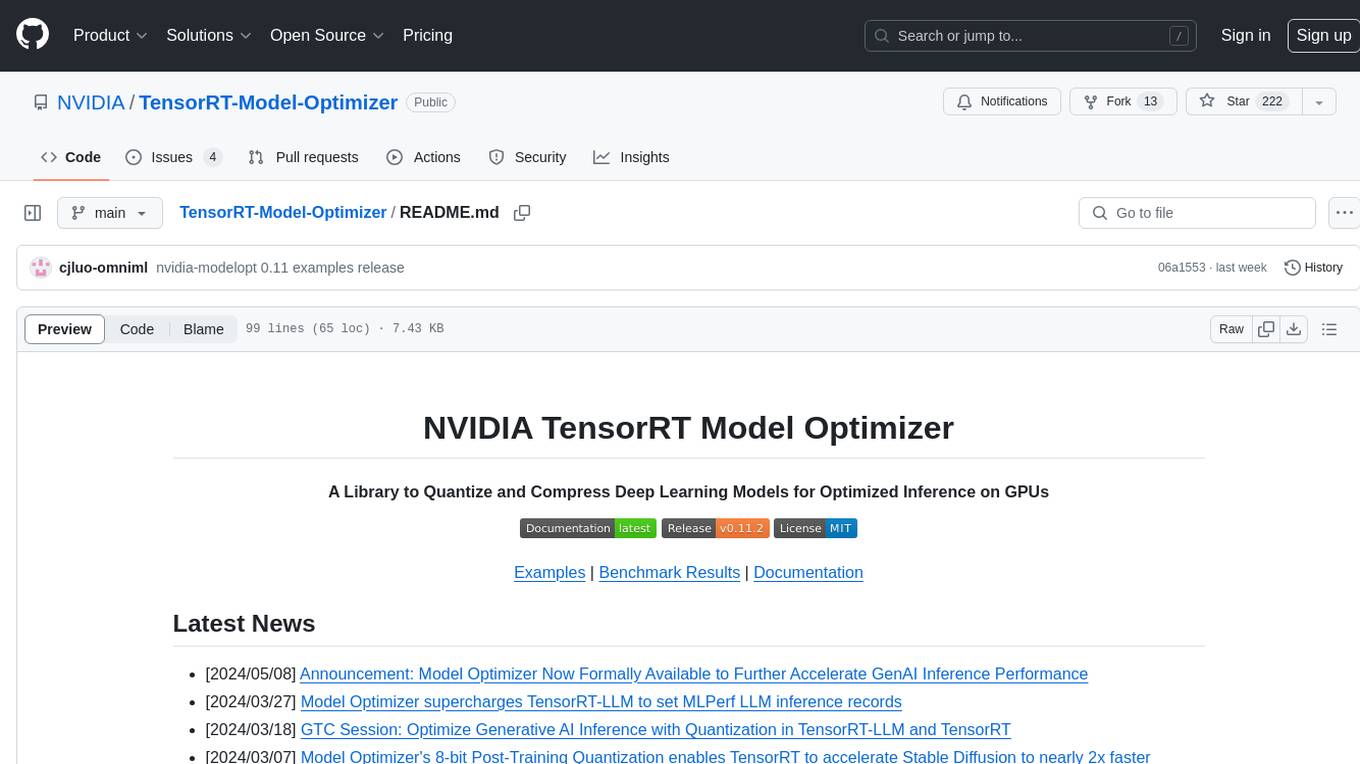
TensorRT-Model-Optimizer
The NVIDIA TensorRT Model Optimizer is a library designed to quantize and compress deep learning models for optimized inference on GPUs. It offers state-of-the-art model optimization techniques including quantization and sparsity to reduce inference costs for generative AI models. Users can easily stack different optimization techniques to produce quantized checkpoints from torch or ONNX models. The quantized checkpoints are ready for deployment in inference frameworks like TensorRT-LLM or TensorRT, with planned integrations for NVIDIA NeMo and Megatron-LM. The tool also supports 8-bit quantization with Stable Diffusion for enterprise users on NVIDIA NIM. Model Optimizer is available for free on NVIDIA PyPI, and this repository serves as a platform for sharing examples, GPU-optimized recipes, and collecting community feedback.