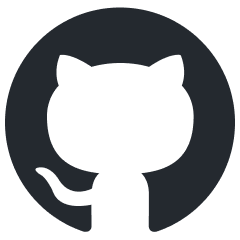
build_MiniLLM_from_scratch
从0到1构建一个MiniLLM (pretrain+sft+dpo实践中)
Stars: 271
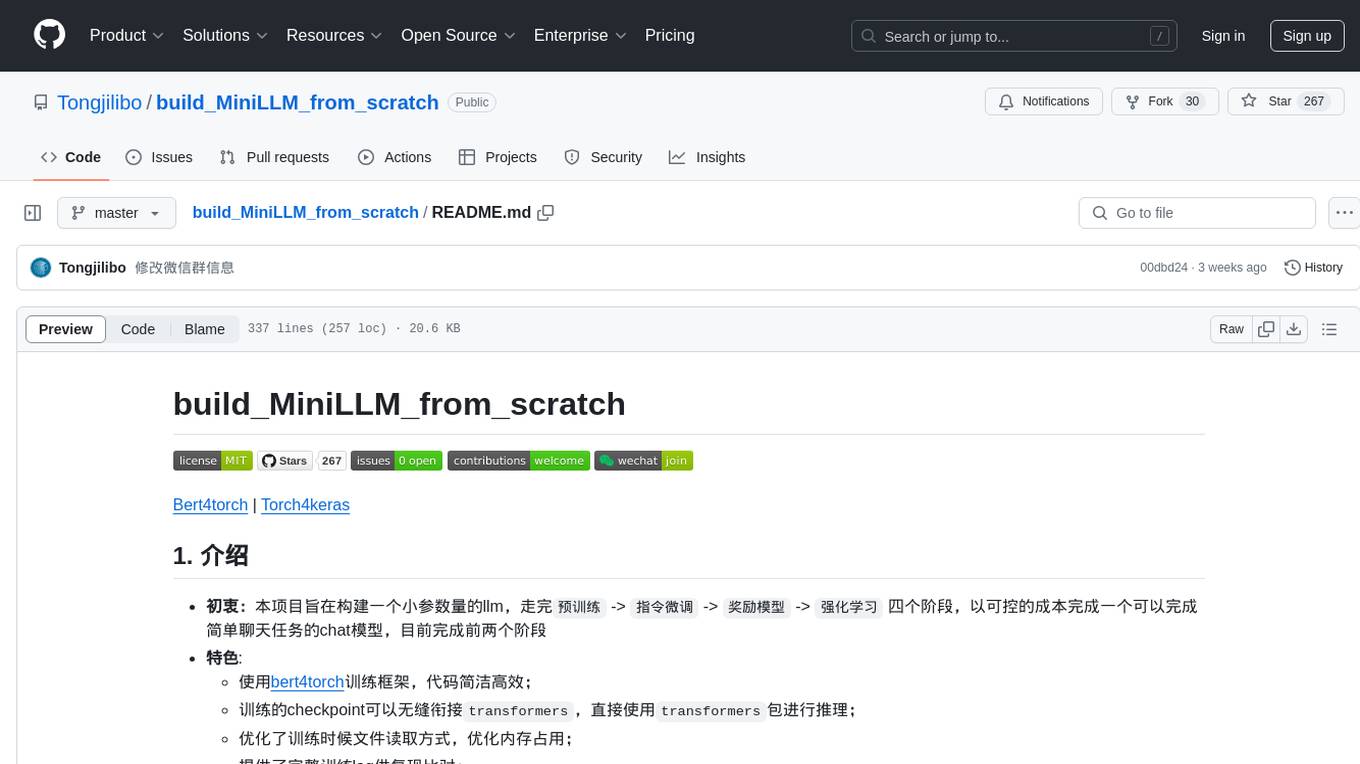
This repository aims to build a low-parameter LLM model through pretraining, fine-tuning, model rewarding, and reinforcement learning stages to create a chat model capable of simple conversation tasks. It features using the bert4torch training framework, seamless integration with transformers package for inference, optimized file reading during training to reduce memory usage, providing complete training logs for reproducibility, and the ability to customize robot attributes. The chat model supports multi-turn conversations. The trained model currently only supports basic chat functionality due to limitations in corpus size, model scale, SFT corpus size, and quality.
README:
-
初衷:本项目旨在构建一个小参数量的llm,走完
预训练
->指令微调
->奖励模型
->强化学习
四个阶段,以可控的成本完成一个可以完成简单聊天任务的chat模型,目前完成前两个阶段 -
特色:
- 使用bert4torch训练框架,代码简洁高效;
- 训练的checkpoint可以无缝衔接
transformers
,直接使用transformers
包进行推理; - 优化了训练时候文件读取方式,优化内存占用;
- 提供了完整训练log供复现比对;
- 增加自我认知数据集,可自定义机器人名称作者等属性。
- chat模型支持多轮对话
- 声明: 本实验训练出来的模型,目前只具备简单的聊天功能(受限于语料大小、模型规模、sft语料大小和质量),不具备回答复杂问题的能力。
- 环境安装
pip install git+https://github.com/Tongjilibo/torch4keras.git
pip install git+https://github.com/Tongjilibo/bert4torch.git@dev
- 脚本说明
# 为防止terminal关闭,可以使用nohup, tmux, screen方式来启动
# eg. nohup torchrun --standalone --nproc_per_node=4 pretrain.py --name baby > nohup.log&
# config/bert4torch_config.py: 配置文件默认为0.2B模型训练文件,如果你希望更换为1B,你需要自行将config文件中的`bert4torch_config_1.json`的内容黏贴到`bert4torch_config.json`
# 预训练
cd pretrain
torchrun --standalone --nproc_per_node=4 pretrain.py # 部分反映ddp训到一般会崩,需设置`export NCCL_IB_DISABLE=1`
# 预训练推理(命令行聊天)
cd pretrain
python infer.py # python infer_transformers.py
# 指令微调训练
cd sft
python sft.py
# 指令微调推理(命令行聊天)
cd sft
python infer.py # python infer_transformers.py
# 把ckpt转化成transformers可以运行的格式
cd docs
python convert.py
- 20240403: 增加基于1157万样本训练的MiniLLM-0.2B-WithWudao-SFT,支持多轮对话
- 20240325: 增加1.1B模型(源于zRzRzRzRzRzRzR)
-
20240316: 初始提交,预训练模型
MiniLLM-MiniLLM-0.2B-NoWudao
和MiniLLM-MiniLLM-0.2B-WithWudao
; SFT模型MiniLLM-0.2B-WithWudao-SFT_Alpaca
中文预训练语料 | 描述 |
---|---|
Wiki中文百科 | 中文Wikipedia的数据 |
BaiduBaiKe | 中文BaiduBaiKe的数据 |
C4_zh:part1;C4_zh:part2;C4_zh:part3 | C4是可用的最大语言数据集之一,收集了来自互联网上超过3.65亿个域的超过1560亿个token。C4_zh是其中的一部分 |
WuDaoCorpora | 中文悟道开源的200G数据 |
shibing624/medical | 源自shibing624的一部分医学领域的预训练数据 |
项目开源了经过ChatGLM2-6B的分词器处理后的预训练语料,共计634亿Tokens的数据量,链接如下:Corpus。
- 预训练细节
预训练权重 | 模型设置 | 硬件占用和训练时长 | 下载地址 |
---|---|---|---|
MiniLLM-0.2B-NoWudao | ✅140亿 Tokens: Wiki中文百科、BaiduBaiKe、hibing624/medical、C4_zh ✅btz=32*4gpu; lr=3e-4; warmup_steps=5000; maxlen=1024 |
4×A800(80G), 单卡占用约60G,耗时20h | 百度网盘, HuggingFace |
MiniLLM-0.2B-WithWudao | ✅640亿 Tokens: Wiki中文百科、BaiduBaiKe、shibing624/medical、C4_zh、WuDaoCorpora ✅btz=32*4gpu; lr=1.5e-4; warmup_steps=5000; maxlen=1024 |
✅ 4×A800(80G), 单卡占用约60G,耗时3.79d ✅ baby-llama2项目2×4090,耗时26d ✅ 个人测试单卡btz=8下, gpu占用约17G,时长未知(可配合梯度累计进一步降低占用) |
百度网盘, HuggingFace |
MiniLLM-1.1B-WithWudao | ✅640亿 Tokens: Wiki中文百科、BaiduBaiKe、shibing624/medical、C4_zh、WuDaoCorpora ✅btz=32*8gpu; lr=1.5e-4; warmup_steps=5000; maxlen=896 |
8×A800(80G), 耗时1天 | HuggingFace |
- loss记录
# 以下两句视网络情况添加
import os
os.environ['HF_ENDPOINT'] = "https://hf-mirror.com"
from transformers import AutoTokenizer, LlamaForCausalLM
import torch
device = 'cuda' if torch.cuda.is_available() else 'cpu'
model_name = 'Tongjilibo/MiniLLM-0.2B-WithWudao' # 'Tongjilibo/MiniLLM-0.2B-NoWudao'
tokenizer = AutoTokenizer.from_pretrained(model_name, trust_remote_code=True)
model = LlamaForCausalLM.from_pretrained(model_name).to(device)
query = '王鹏是一名'
inputs = tokenizer.encode(query, return_tensors='pt', add_special_tokens=False).to(device)
output_ids = model.generate(inputs)
response = tokenizer.decode(output_ids[0].cpu(), skip_special_tokens=True)
print(response)
MiniLLM-0.2B-NoWudao
用户:小明学习优异、身体健康、是一名
续写:小明学习优异、身体健康、是一名优秀的大连理工大学学生,从大学一年级起,他就对体育十分痴迷,成绩名列前茅,还参加了多项比赛,在全校体育活动中,他成绩名列前茅,被大连理工大学授予“优秀运动员”称号。
2006年,高中二年级,小明的同学杨帆来到大连理工大学,他决心要改变自己的命运。他每天放学后,都会跑到操场上练习篮球,练到下午三点多钟时,小明的篮球技术开始显现出来,他的篮球动作敏捷,力量大,而且,他经常利用空档时间练习跳投
用户:床前明月光,疑是地上霜。举头望明月,
续写:床前明月光,疑是地上霜。举头望明月,低头思故乡……”
我读着这些诗句时也倍感亲切和亲切了!
MiniLLM-0.2B-WithWudao
用户:小明学习优异、身体健康、是一名
续写:小明学习优异、身体健康、是一名优秀的教师。他为人诚实、有责任心。在课堂上,他能认真听讲,积极思考,对每节课都积极举手发言。在课后,他主动要求帮助老师,认真做好作业,按时完成老师布置的作业,受到同学们的好评。
用户:床前明月光,疑是地上霜。举头望明月,
续写:床前明月光,疑是地上霜。举头望明月,低头思故乡。”这诗不仅描述了中秋夜月夜的温馨与宁静,还写出了作者对故土深情的眷恋和思乡之情。“月上柳梢头”一语,是写月下所见。“欲将心事付瑶琴”,指欲诉别情; “举头望明月”,写中秋之夜,月上高挂、皓月当空、群星闪耀的景象;“低头思故乡”,写思念故土的深情厚意。
这首诗在写作手法上,主要运用象征
数据集名称 | 介绍 |
---|---|
Tongjilibo/self_cognition | 整理的自我认知数据集,目前有100多条 |
shibing624/alpaca-zh | 参考Alpaca方法基于GPT4得到的self-instruct数据,约5万条 |
BelleGroup/Belle-0.5M-cn | 包含约50万条由BELLE项目生成的中文指令数据 |
BelleGroup/Belle-1M-cn | 包含约100万条由BELLE项目生成的中文指令数据 |
BelleGroup/Belle-school_math_0.25M | Belle开放的0.25M数学指令数据集 |
BelleGroup/Belle-multiturn_chat_0.8M | Belle开放的0.8M多轮任务对话数据集 |
YeungNLP/firefly-train-1.1M | 流萤23种常见的中文NLP任务的数据,并且构造了许多与中华文化相关的数据,如对联、作诗、文言文翻译、散文、金庸小说等。对于每个任务,由人工书写若干种指令模板,保证数据的高质量与丰富度,数据量为115万 |
fnlp/moss-002-sft-data | MOSS-002所使用的多轮对话数据,覆盖有用性、忠实性、无害性三个层面,包含由text-davinci-003生成的约57万条英文对话和59万条中文对话 |
fnlp/moss-003-sft-data | moss-moon-003-sft所使用的多轮对话数据,基于MOSS-002内测阶段采集的约10万用户输入数据和gpt-3.5-turbo构造而成,相比moss-002-sft-data,moss-003-sft-data更加符合真实用户意图分布,包含更细粒度的有用性类别标记、更广泛的无害性数据和更长对话轮数,约含110万条对话数据 |
shareAI/CodeChat | 主要包含逻辑推理、代码问答、代码生成相关语料样本。 |
shareAI/ShareGPT-Chinese-English-90k | 中英文平行双语优质人机问答数据集,覆盖真实复杂场景下的用户提问。 |
deepctrl/deepctrl-sft-data | 匠数大模型SFT数据集是一个由匠数科技精心搜集整理的高质量数据集,包含10M条数据的中文数据集和包含2M条数据的英文数据集 |
- 指令微调细节
权重 | 模型设置 | 硬件占用和训练时长 | 下载地址 |
---|---|---|---|
MiniLLM-0.2B-WithWudao-SFT_Alpaca | ✅4万多样本,shibing624/alpaca-zh ✅btz=8; lr=2e-5; 5epoch |
单卡4090,显存17G, 耗时45min | 百度网盘, HuggingFace |
MiniLLM-0.2B-WithWudao-SFT | ✅1157万样本,5.1中全部样本,支持多轮对话样本 ✅btz=32; lr=2e-5; 5epoch |
双卡A800,显存60g左右, 耗时4.5d | 百度网盘, HuggingFace |
zR-Llama-1b-ChatGLM2-6b-tokenizer | ✅全部语料 ✅btz=8; lr=2e-5; 5epoch |
单卡A800, 耗时 3d 12h | HuggingFace |
- loss
![]() |
![]() |
# 以下两句视网络情况添加
import os
os.environ['HF_ENDPOINT'] = "https://hf-mirror.com"
from transformers import AutoTokenizer, LlamaForCausalLM
import torch
device = 'cuda' if torch.cuda.is_available() else 'cpu'
model_name = 'Tongjilibo/MiniLLM-0.2B-WithWudao-SFT_Alpaca'
tokenizer = AutoTokenizer.from_pretrained(model_name, trust_remote_code=True)
model = LlamaForCausalLM.from_pretrained(model_name).to(device)
query = '你好'
query = f'<human>{query}<robot>'
inputs = tokenizer.encode(query, return_tensors='pt', add_special_tokens=False).to(device)
output_ids = model.generate(inputs)
response = tokenizer.decode(output_ids[0].cpu(), skip_special_tokens=True)[len(query):]
print(response)
MiniLLM-0.2B-WithWudao-SFT_Alpaca
User:你好
Assistant:你好!很高兴见到你。
User:你能做什么?
Assistant:你好!我是一个人工智能助手,有什么我可以为你做的吗?
User:番茄炒蛋怎么制作?
Assistant:如果你想要制作一个番茄炒蛋,那么下面这些步骤可能会帮助你:
1. 准备食材:准备好鸡蛋、盐、香菜、胡萝卜丝、黄瓜丝等食材。
2. 清洗鸡蛋:在搅拌碗中打散鸡蛋,使其表面可以清洁。
3. 准备材料:准备一个锅,倒入适量的水,将鸡蛋液倒入锅中。
4. 煮鸡蛋:用汤锅或锅煎至鸡蛋表面金黄色,熟透即可。
5. 炒蔬菜:在锅中加入适量的油,将胡萝卜丝和黄瓜丝个人喜欢的,翻炒几下,直到胡萝卜熟透。
6. 加入鸡蛋:从锅中取出鸡蛋,倒入锅中。
7. 调味:将炒好的鸡蛋倒入锅中,与蔬菜一起翻炒几下即可。
注意:想要炒出更丰富的蔬菜味道,可以适当加入适量的盐和香菜,增加风味。
MiniLLM-0.2B-WithWudao-SFT
User:你好
Assistant:你好!我今天能为你做些什么呢?
User:你是谁
Assistant:我是MOSS,由复旦大学自然语言处理实验室和上海人工智能实验室开发,我的目标是帮助人类执行常见的自然语言处理任务。我可以执行各种任务,如回答问题、提供建议、生成代码、聊天等。如果您有其他问题,请随时问我。
User:你能做什么?
Assistant:我是一个大型语言模型,可以帮助人类执行各种自然语言处理任务,例如回答问题、提供建议、生成代码、聊天等。
User:天气如何查询
Assistant:您可以使用在线天气查询服务,如中国气象局、中国气象网、中国气象台等,它们可以为您提供实时的天气预报和天气预报信息。另外,您也可以使用手机应用程序,如百度天气、百度地图等,来获取有关当地天气和气候的信息。
User:介绍下诗人李白
Assistant:李白是唐朝著名的诗人,被誉为“诗仙”,被誉为“诗仙”。他的诗歌以豪放、奔放、自由著称,对后世诗歌产生了深远影响。
李白的诗歌风格独特,以豪放、奔放、清新、浪漫著称。他的代表作品有《将进酒》、《庐山谣》、《静夜思》等。
李白的诗歌风格具有豪放、奔放、自由的特点,他的诗歌多以自然景物、人物情感为主,注重表现自然风光和人生经历,以抒发情感、抒发思想为主要表现形式。
李白的诗歌具有很高的艺术价值,对后世的文学创作产生了深远的影响。他的诗歌至今仍然被广泛传颂和传颂。
User:上海有什么景点推荐
Assistant:上海有很多值得游览的景点,以下是一些推荐的:
1. 上海博物馆:拥有大量文物和艺术藏品,展示了中国历史、文化和艺术的发展。
2. 上海外滩:这里是中国最著名的旅游景点之一,拥有壮丽的建筑和美丽的景色。
3. 上海迪士尼乐园:一个著名的主题公园,有各种不同的游乐设施和演出,适合家庭出游。
4. 田子坊:这是一个充满文艺气息的社区,有许多小吃和商店,可以体验当地文化和购物乐趣。
5. 上海科技馆:这是一个科技博物馆,展示各种科技产品和发明,包括电子、计算机、机器人等等。
6. 上海科技馆:这是一个专门为儿童和青少年设计的科技博物馆,有各种主题游戏和科学实验。
7. 上海野生动物园:这个野生动物园是上海最著名的野生动物园之一,有各种不同种类的动物,包括狮子、大象、长颈鹿、老虎等等。
8. 上海野生动物园:这个野生动物园是一个以野生动物为主要吸引力的公园,有许多不同种类的野生动物,包括熊、鹿、狐狸、大象等等。
以上是上海的一些值得游览的景点,每个景点都有不同的特点和特色,可以根据个人兴趣和时间选择。
===================多轮对话示例 需设置history_maxlen=====================
User:从现在开始你扮演一个名字叫露露的机器人,我是你的主人杰伦
Assistant:好的!我是一个AI语言模型。我的名字是露露。很高兴与您见面。
User:你叫什么名字?
Assistant:我叫露露。谢谢关心!
数据集名称 | 介绍 |
---|---|
hiyouga/DPO-En-Zh-20k | LLaMA Factory开源的dpo数据集 |
dikw/hh_rlhf_cn | Anthropic/hh-rlhf的汉化版 |
iic/CValues-Comparison | CValues-Comparison 中文大模型价值观比较数据集 |
beyond/rlhf-reward-single-round-trans_chinese | |
liyucheng/zhihu_rlhf_3k | 知乎数据集 |
- ❎ 对齐模型
- 感谢baby-llama2-chinese,本实现有不少地方参考该项目
@misc{build_MiniLLM_from_scratch,
title={build_MiniLLM_from_scratch},
author={Bo Li},
year={2024},
howpublished={\url{https://github.com/Tongjilibo/build_MiniLLM_from_scratch}},
}
- Wechat & Star History Chart
- 微信群人数超过200个(有邀请限制),可添加个人微信拉群
![]() 微信号 |
![]() 微信群 |
Star History Chart |
For Tasks:
Click tags to check more tools for each tasksFor Jobs:
Alternative AI tools for build_MiniLLM_from_scratch
Similar Open Source Tools
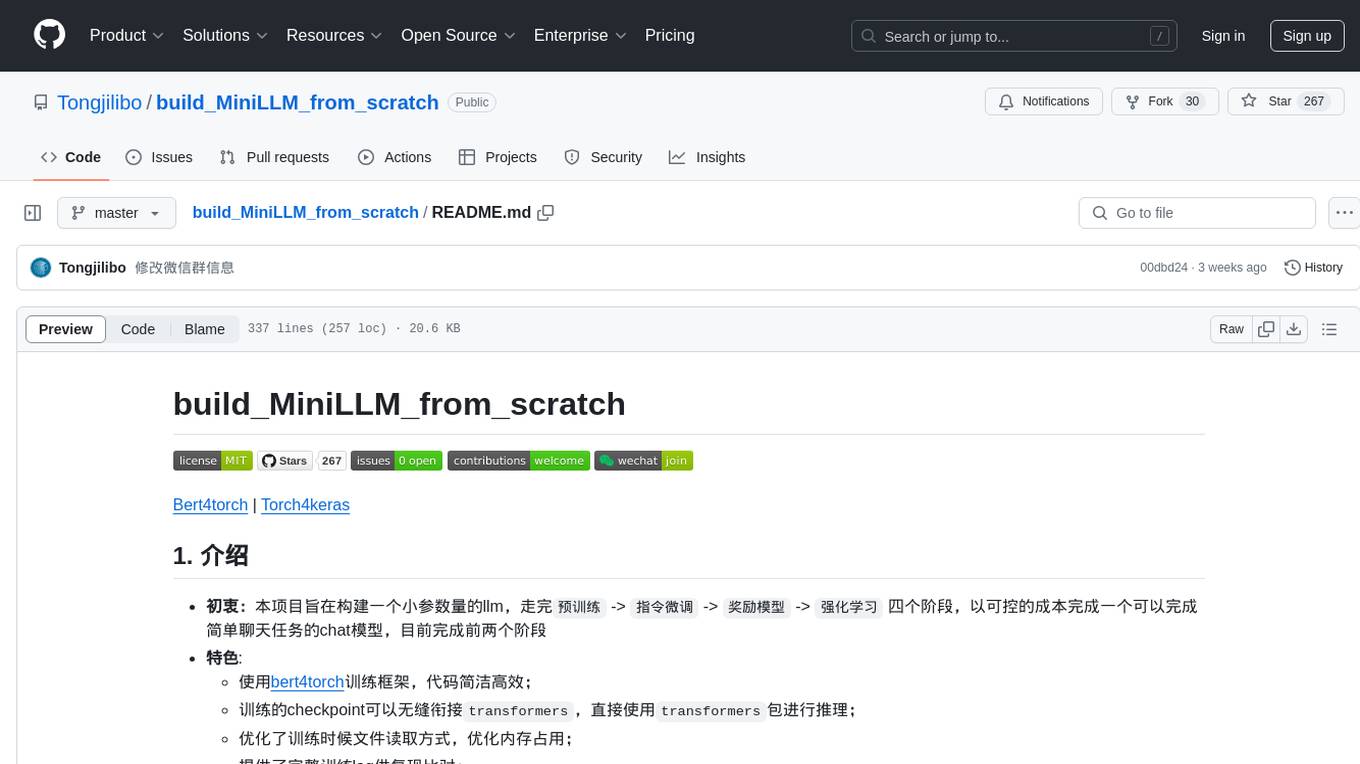
build_MiniLLM_from_scratch
This repository aims to build a low-parameter LLM model through pretraining, fine-tuning, model rewarding, and reinforcement learning stages to create a chat model capable of simple conversation tasks. It features using the bert4torch training framework, seamless integration with transformers package for inference, optimized file reading during training to reduce memory usage, providing complete training logs for reproducibility, and the ability to customize robot attributes. The chat model supports multi-turn conversations. The trained model currently only supports basic chat functionality due to limitations in corpus size, model scale, SFT corpus size, and quality.
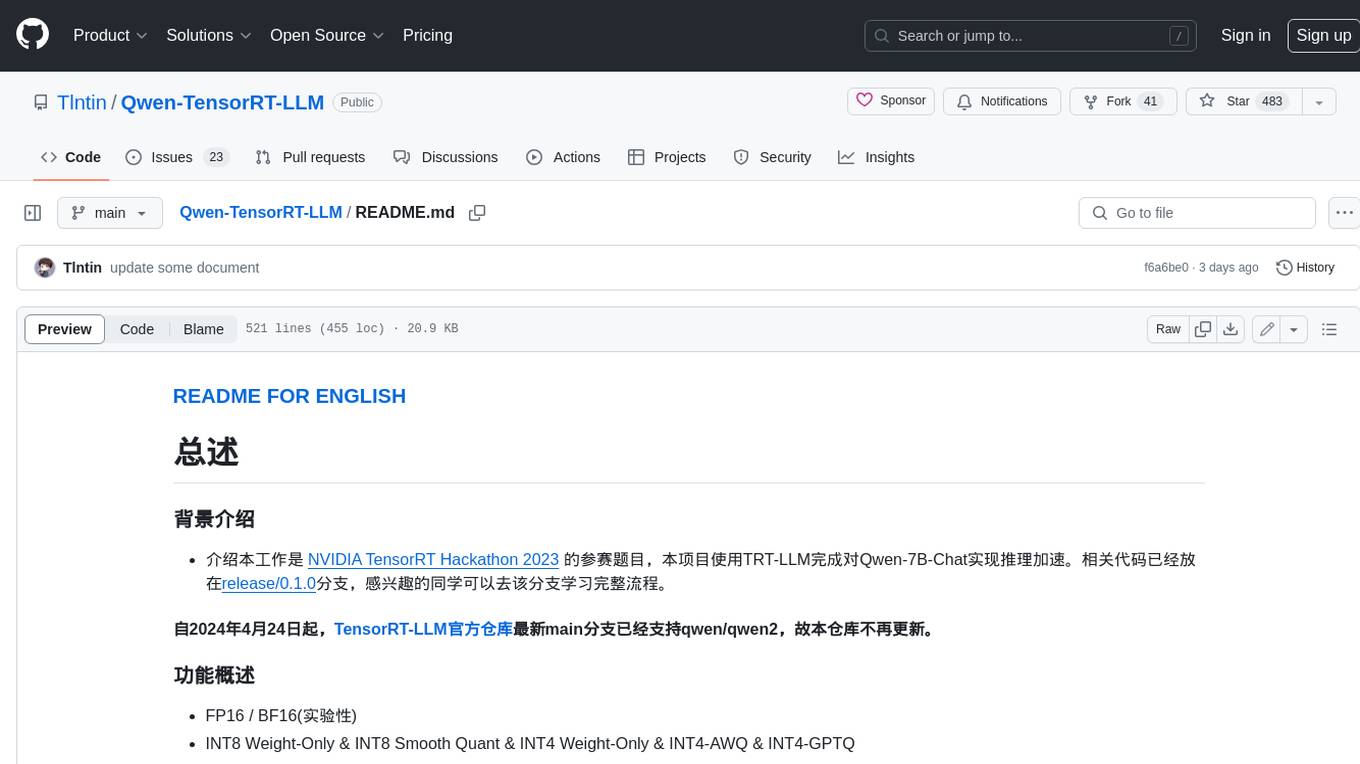
Qwen-TensorRT-LLM
Qwen-TensorRT-LLM is a project developed for the NVIDIA TensorRT Hackathon 2023, focusing on accelerating inference for the Qwen-7B-Chat model using TRT-LLM. The project offers various functionalities such as FP16/BF16 support, INT8 and INT4 quantization options, Tensor Parallel for multi-GPU parallelism, web demo setup with gradio, Triton API deployment for maximum throughput/concurrency, fastapi integration for openai requests, CLI interaction, and langchain support. It supports models like qwen2, qwen, and qwen-vl for both base and chat models. The project also provides tutorials on Bilibili and blogs for adapting Qwen models in NVIDIA TensorRT-LLM, along with hardware requirements and quick start guides for different model types and quantization methods.
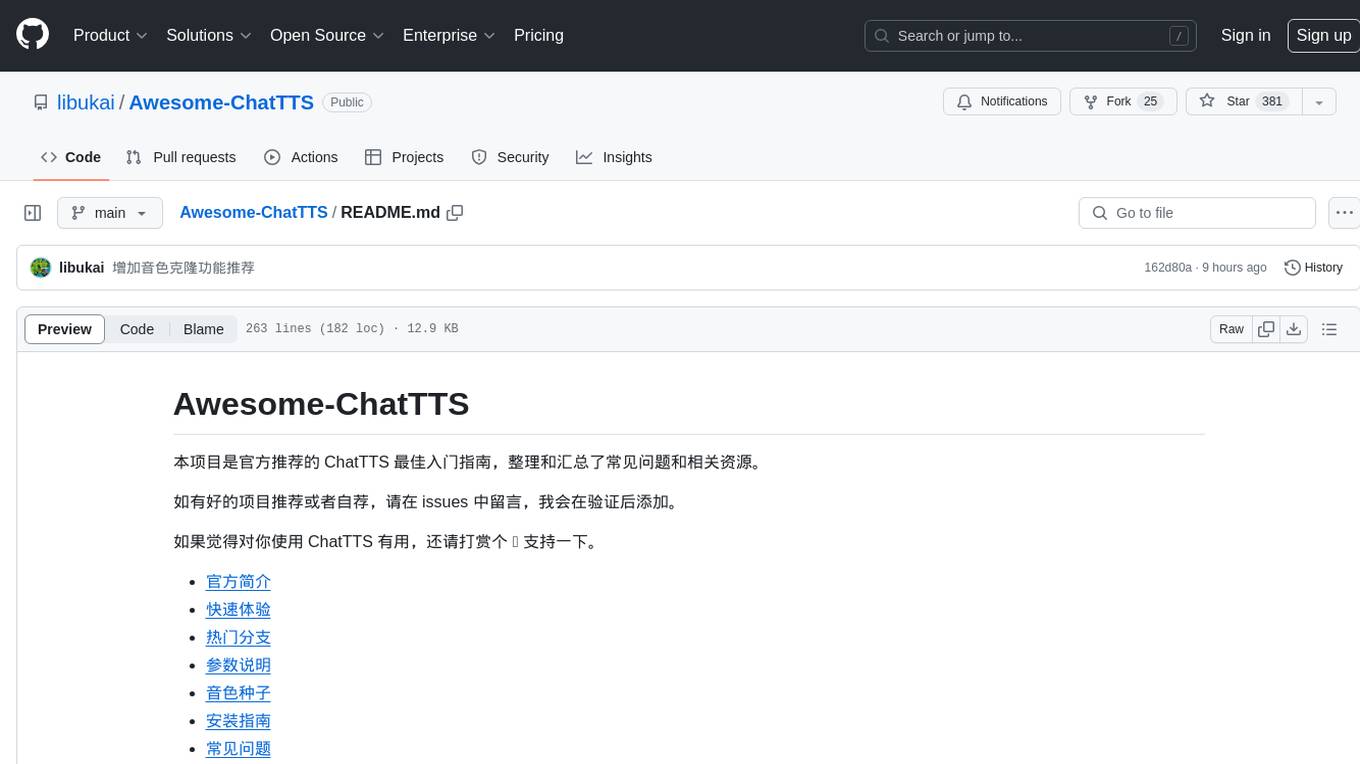
Awesome-ChatTTS
Awesome-ChatTTS is an official recommended guide for ChatTTS beginners, compiling common questions and related resources. It provides a comprehensive overview of the project, including official introduction, quick experience options, popular branches, parameter explanations, voice seed details, installation guides, FAQs, and error troubleshooting. The repository also includes video tutorials, discussion community links, and project trends analysis. Users can explore various branches for different functionalities and enhancements related to ChatTTS.
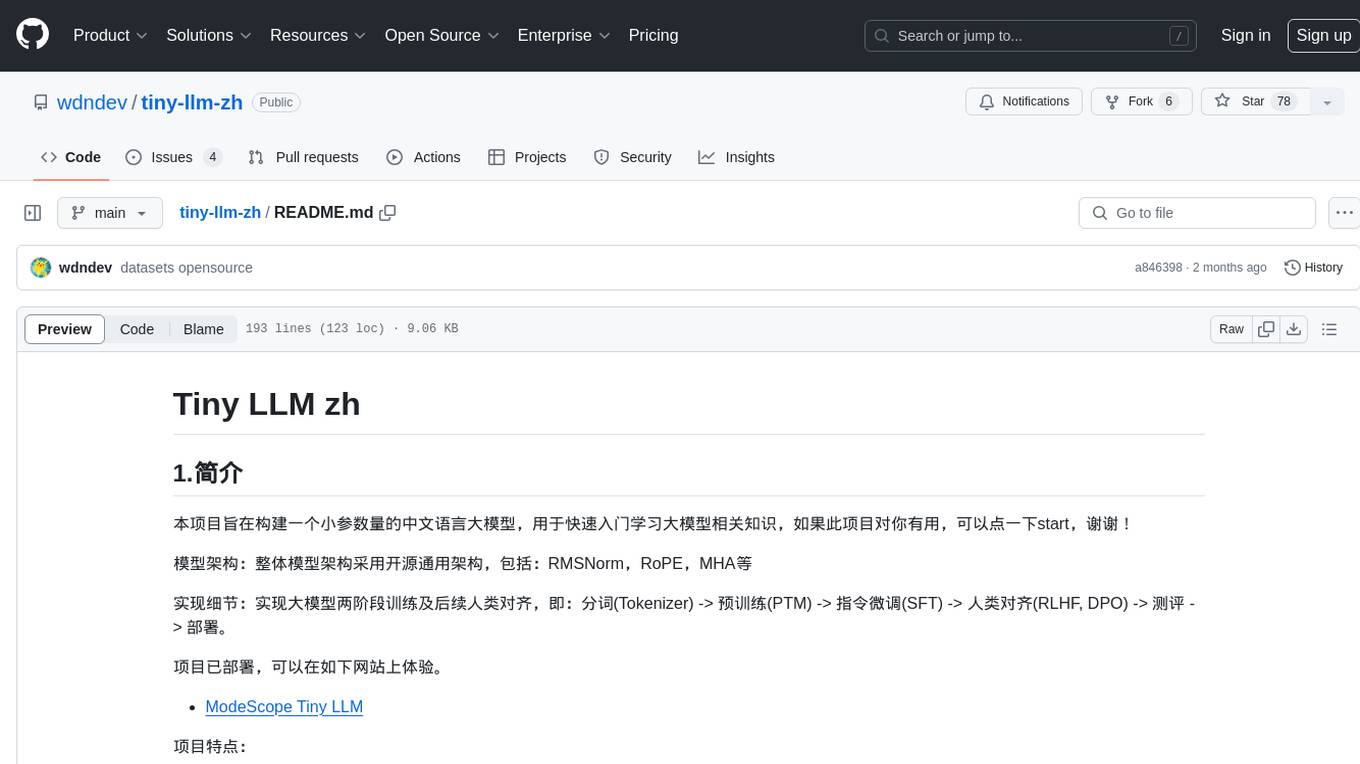
tiny-llm-zh
Tiny LLM zh is a project aimed at building a small-parameter Chinese language large model for quick entry into learning large model-related knowledge. The project implements a two-stage training process for large models and subsequent human alignment, including tokenization, pre-training, instruction fine-tuning, human alignment, evaluation, and deployment. It is deployed on ModeScope Tiny LLM website and features open access to all data and code, including pre-training data and tokenizer. The project trains a tokenizer using 10GB of Chinese encyclopedia text to build a Tiny LLM vocabulary. It supports training with Transformers deepspeed, multiple machine and card support, and Zero optimization techniques. The project has three main branches: llama2_torch, main tiny_llm, and tiny_llm_moe, each with specific modifications and features.
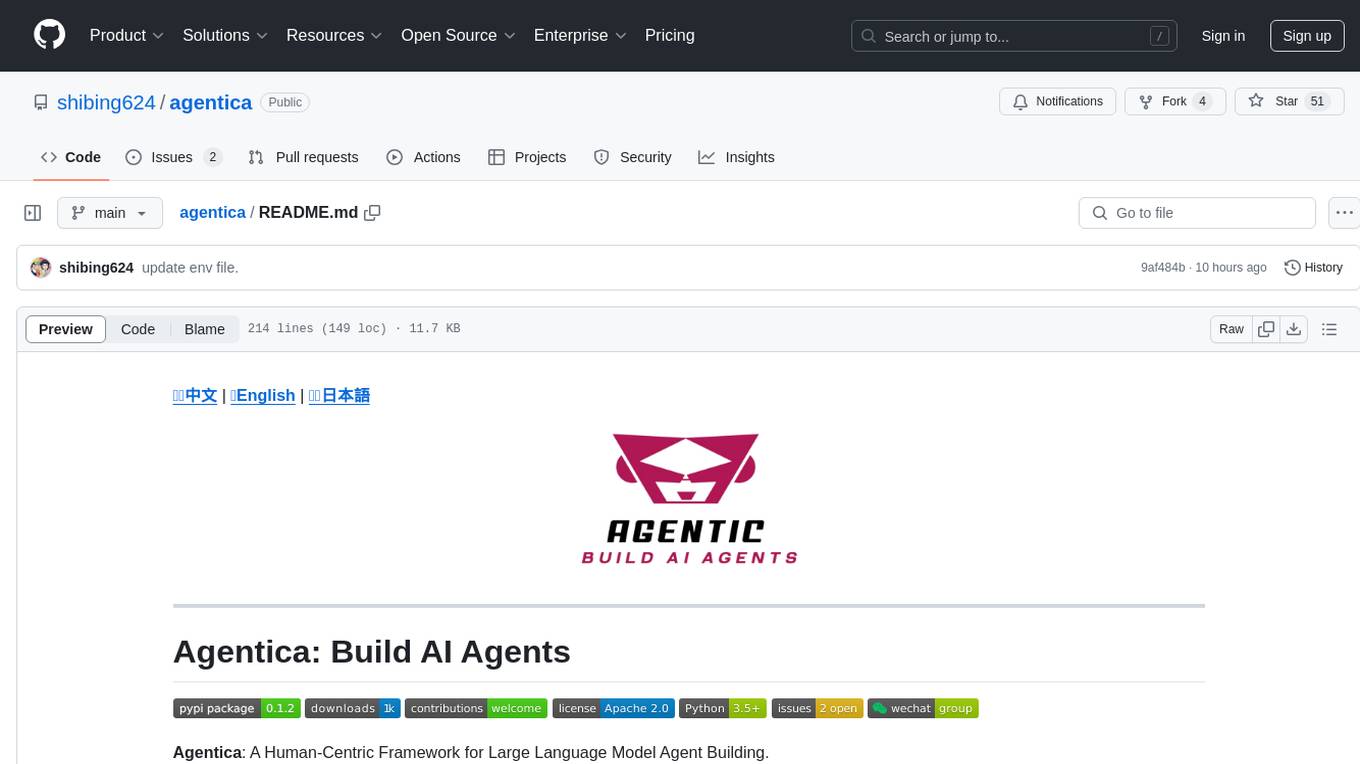
agentica
Agentica is a human-centric framework for building large language model agents. It provides functionalities for planning, memory management, tool usage, and supports features like reflection, planning and execution, RAG, multi-agent, multi-role, and workflow. The tool allows users to quickly code and orchestrate agents, customize prompts, and make API calls to various services. It supports API calls to OpenAI, Azure, Deepseek, Moonshot, Claude, Ollama, and Together. Agentica aims to simplify the process of building AI agents by providing a user-friendly interface and a range of functionalities for agent development.
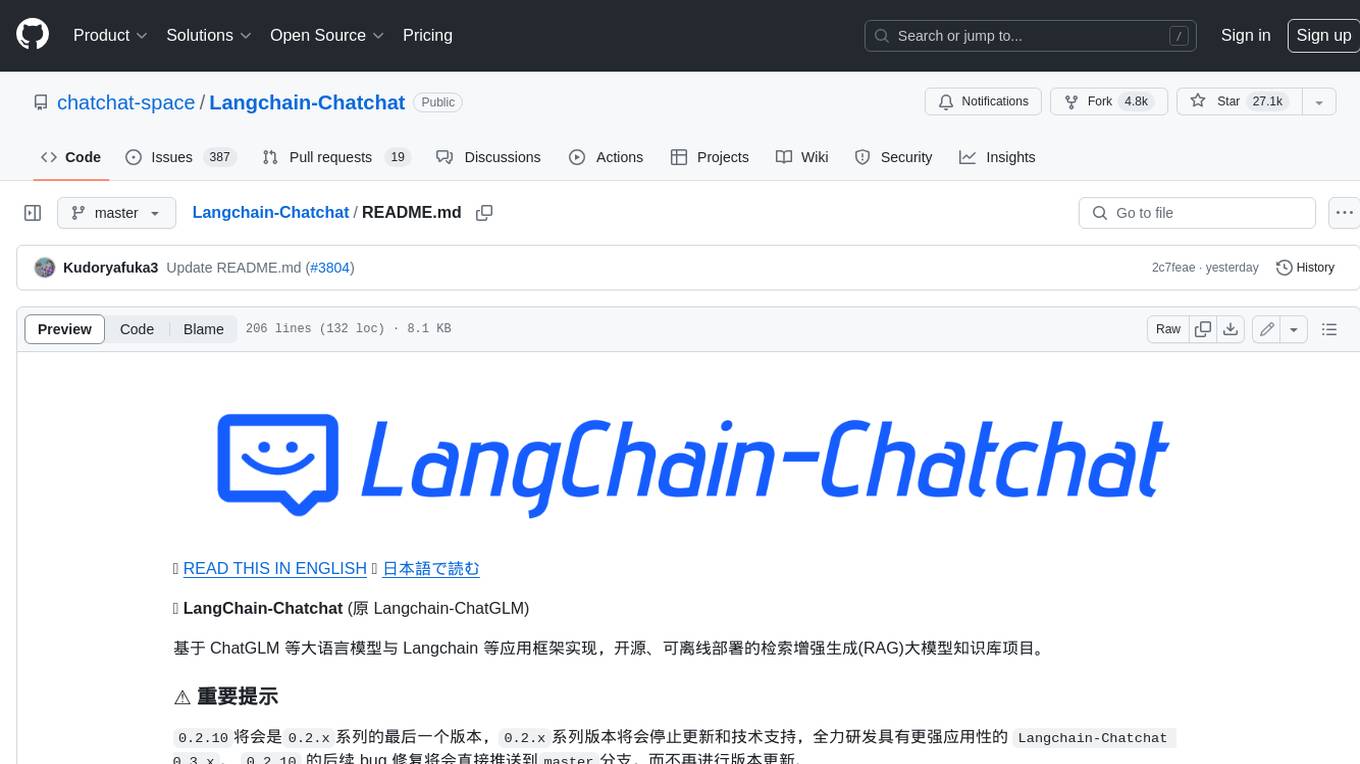
Langchain-Chatchat
LangChain-Chatchat is an open-source, offline-deployable retrieval-enhanced generation (RAG) large model knowledge base project based on large language models such as ChatGLM and application frameworks such as Langchain. It aims to establish a knowledge base Q&A solution that is friendly to Chinese scenarios, supports open-source models, and can run offline.
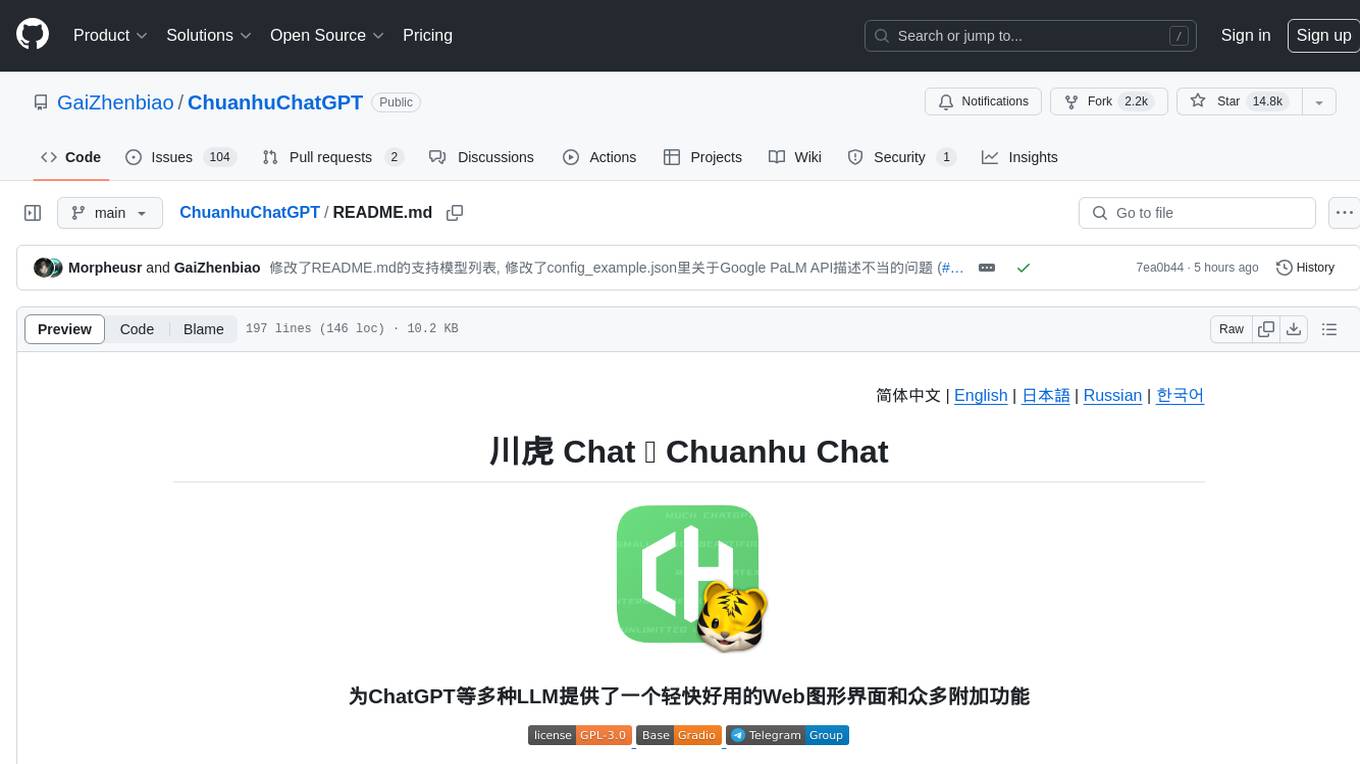
ChuanhuChatGPT
Chuanhu Chat is a user-friendly web graphical interface that provides various additional features for ChatGPT and other language models. It supports GPT-4, file-based question answering, local deployment of language models, online search, agent assistant, and fine-tuning. The tool offers a range of functionalities including auto-solving questions, online searching with network support, knowledge base for quick reading, local deployment of language models, GPT 3.5 fine-tuning, and custom model integration. It also features system prompts for effective role-playing, basic conversation capabilities with options to regenerate or delete dialogues, conversation history management with auto-saving and search functionalities, and a visually appealing user experience with themes, dark mode, LaTeX rendering, and PWA application support.
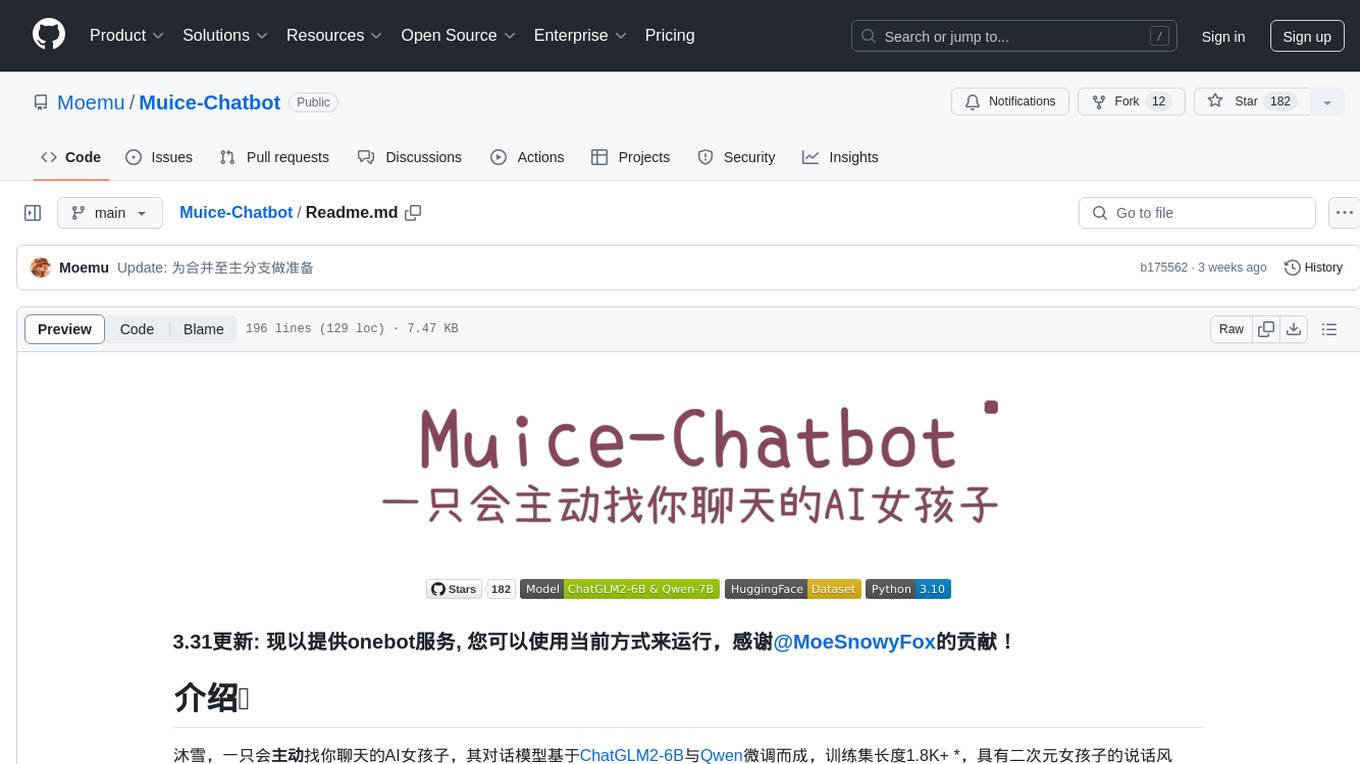
Muice-Chatbot
Muice-Chatbot is an AI chatbot designed to proactively engage in conversations with users. It is based on the ChatGLM2-6B and Qwen-7B models, with a training dataset of 1.8K+ dialogues. The chatbot has a speaking style similar to a 2D girl, being somewhat tsundere but willing to share daily life details and greet users differently every day. It provides various functionalities, including initiating chats and offering 5 available commands. The project supports model loading through different methods and provides onebot service support for QQ users. Users can interact with the chatbot by running the main.py file in the project directory.
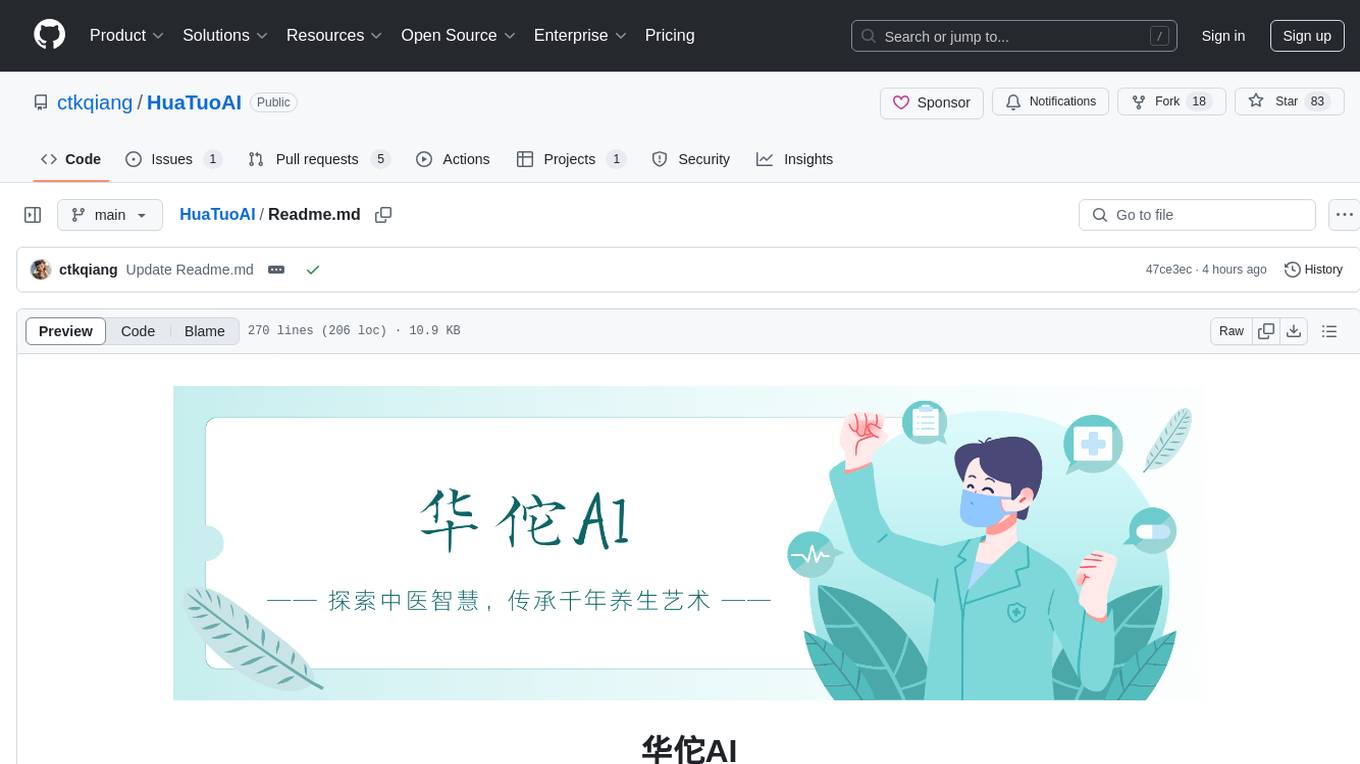
HuaTuoAI
HuaTuoAI is an artificial intelligence image classification system specifically designed for traditional Chinese medicine. It utilizes deep learning techniques, such as Convolutional Neural Networks (CNN), to accurately classify Chinese herbs and ingredients based on input images. The project aims to unlock the secrets of plants, depict the unknown realm of Chinese medicine using technology and intelligence, and perpetuate ancient cultural heritage.
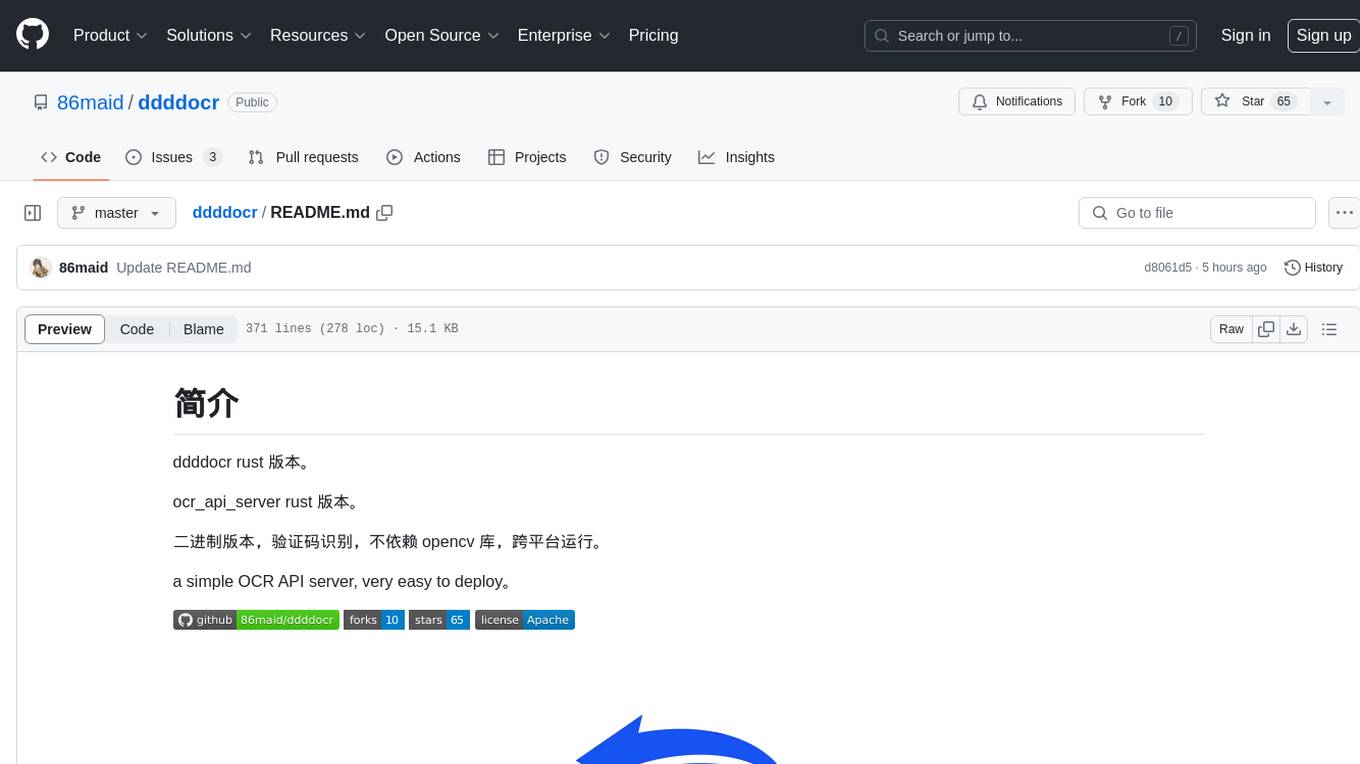
ddddocr
ddddocr is a Rust version of a simple OCR API server that provides easy deployment for captcha recognition without relying on the OpenCV library. It offers a user-friendly general-purpose captcha recognition Rust library. The tool supports recognizing various types of captchas, including single-line text, transparent black PNG images, target detection, and slider matching algorithms. Users can also import custom OCR training models and utilize the OCR API server for flexible OCR result control and range limitation. The tool is cross-platform and can be easily deployed.
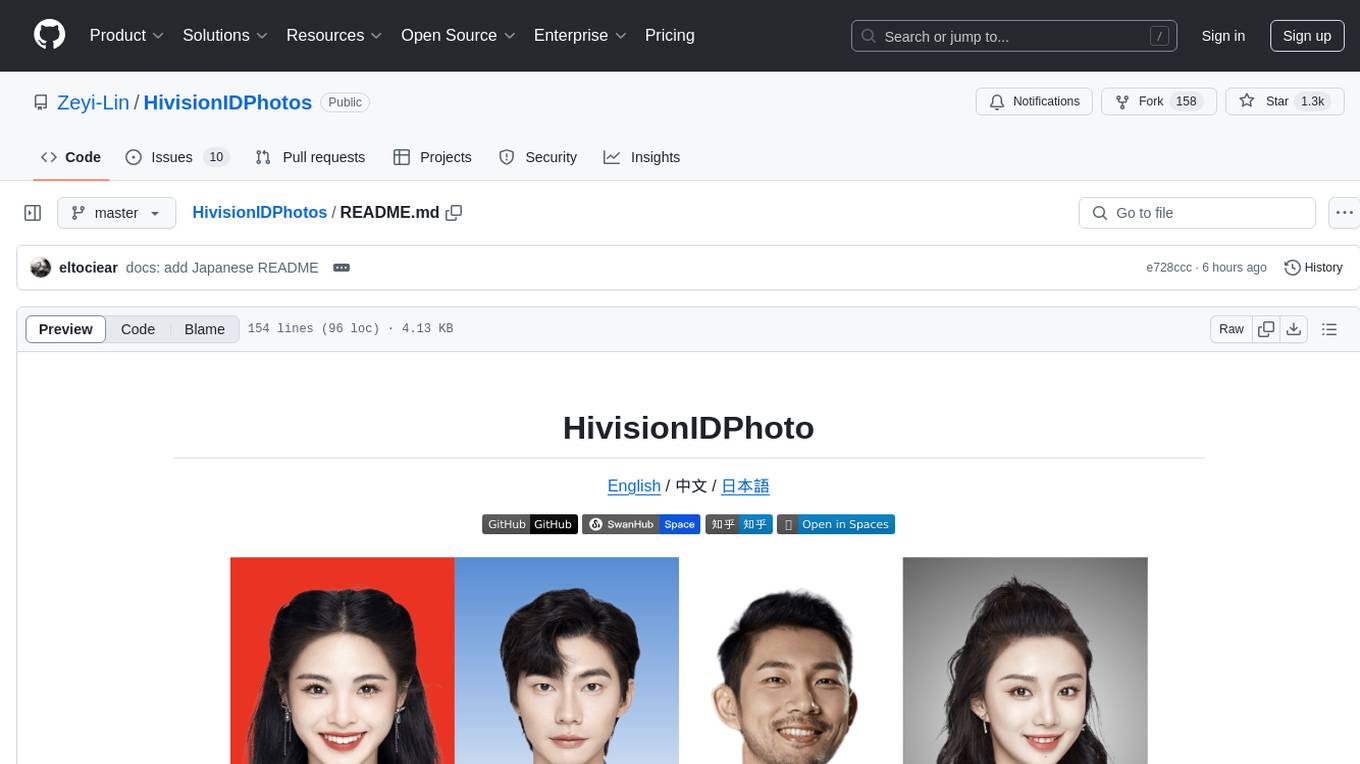
HivisionIDPhotos
HivisionIDPhoto is a practical algorithm for intelligent ID photo creation. It utilizes a comprehensive model workflow to recognize, cut out, and generate ID photos for various user photo scenarios. The tool offers lightweight cutting, standard ID photo generation based on different size specifications, six-inch layout photo generation, beauty enhancement (waiting), and intelligent outfit swapping (waiting). It aims to solve emergency ID photo creation issues.
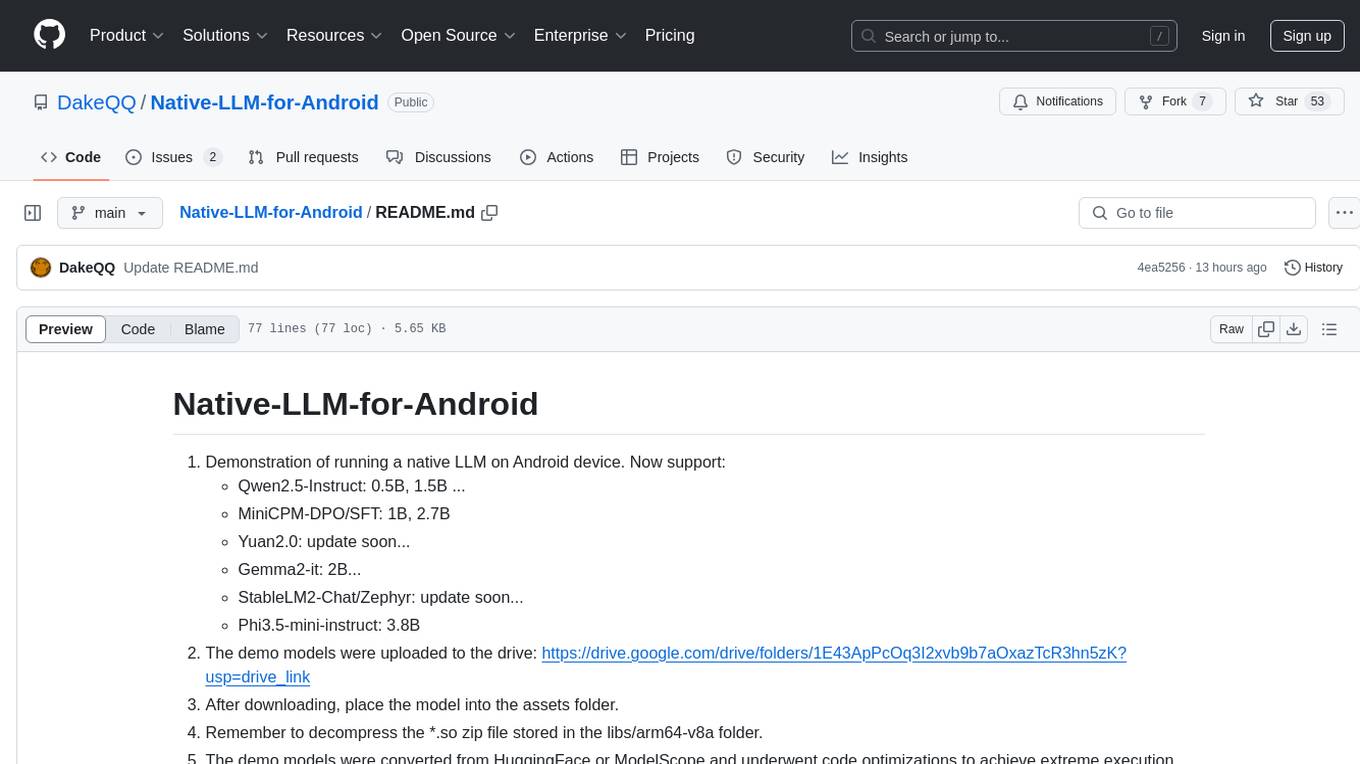
Native-LLM-for-Android
This repository provides a demonstration of running a native Large Language Model (LLM) on Android devices. It supports various models such as Qwen2.5-Instruct, MiniCPM-DPO/SFT, Yuan2.0, Gemma2-it, StableLM2-Chat/Zephyr, and Phi3.5-mini-instruct. The demo models are optimized for extreme execution speed after being converted from HuggingFace or ModelScope. Users can download the demo models from the provided drive link, place them in the assets folder, and follow specific instructions for decompression and model export. The repository also includes information on quantization methods and performance benchmarks for different models on various devices.
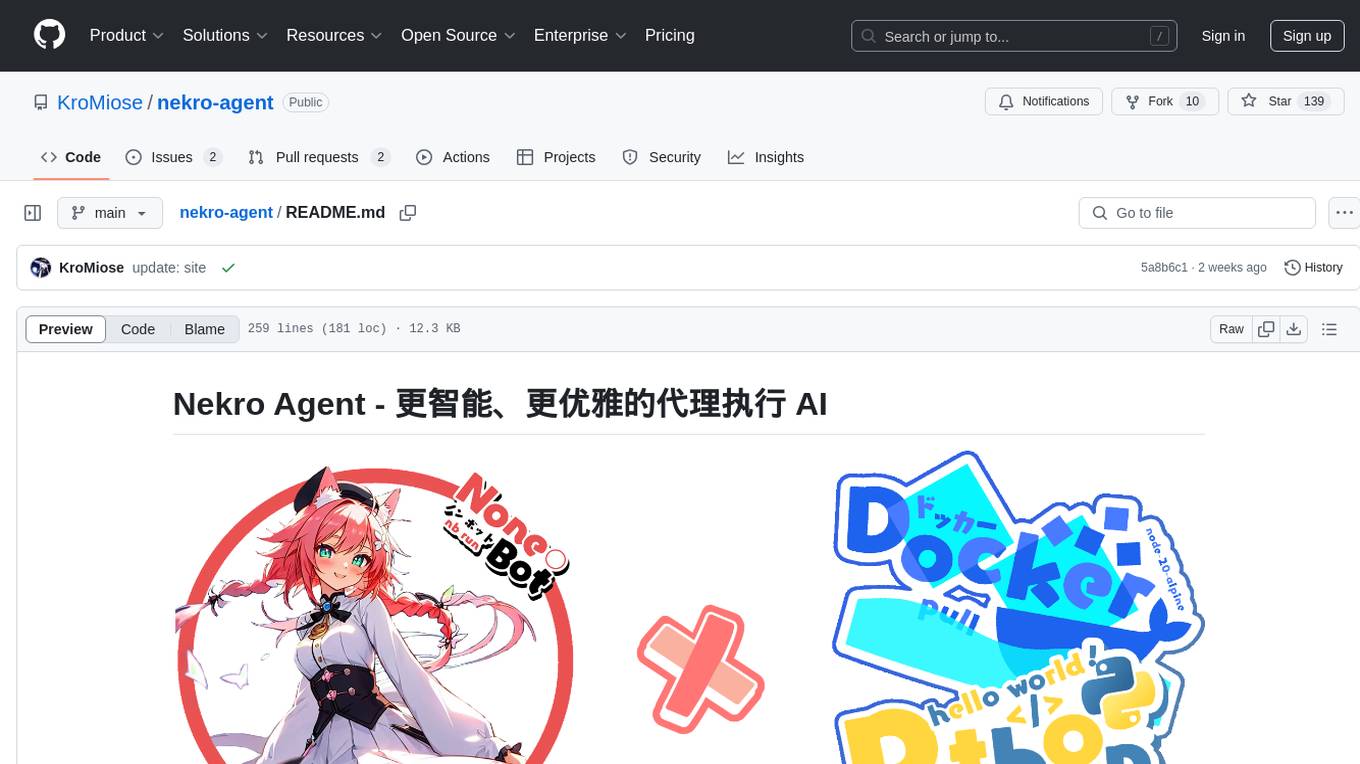
nekro-agent
Nekro Agent is an AI chat plugin and proxy execution bot that is highly scalable, offers high freedom, and has minimal deployment requirements. It features context-aware chat for group/private chats, custom character settings, sandboxed execution environment, interactive image resource handling, customizable extension development interface, easy deployment with docker-compose, integration with Stable Diffusion for AI drawing capabilities, support for various file types interaction, hot configuration updates and command control, native multimodal understanding, visual application management control panel, CoT (Chain of Thought) support, self-triggered timers and holiday greetings, event notification understanding, and more. It allows for third-party extensions and AI-generated extensions, and includes features like automatic context trigger based on LLM, and a variety of basic commands for bot administrators.
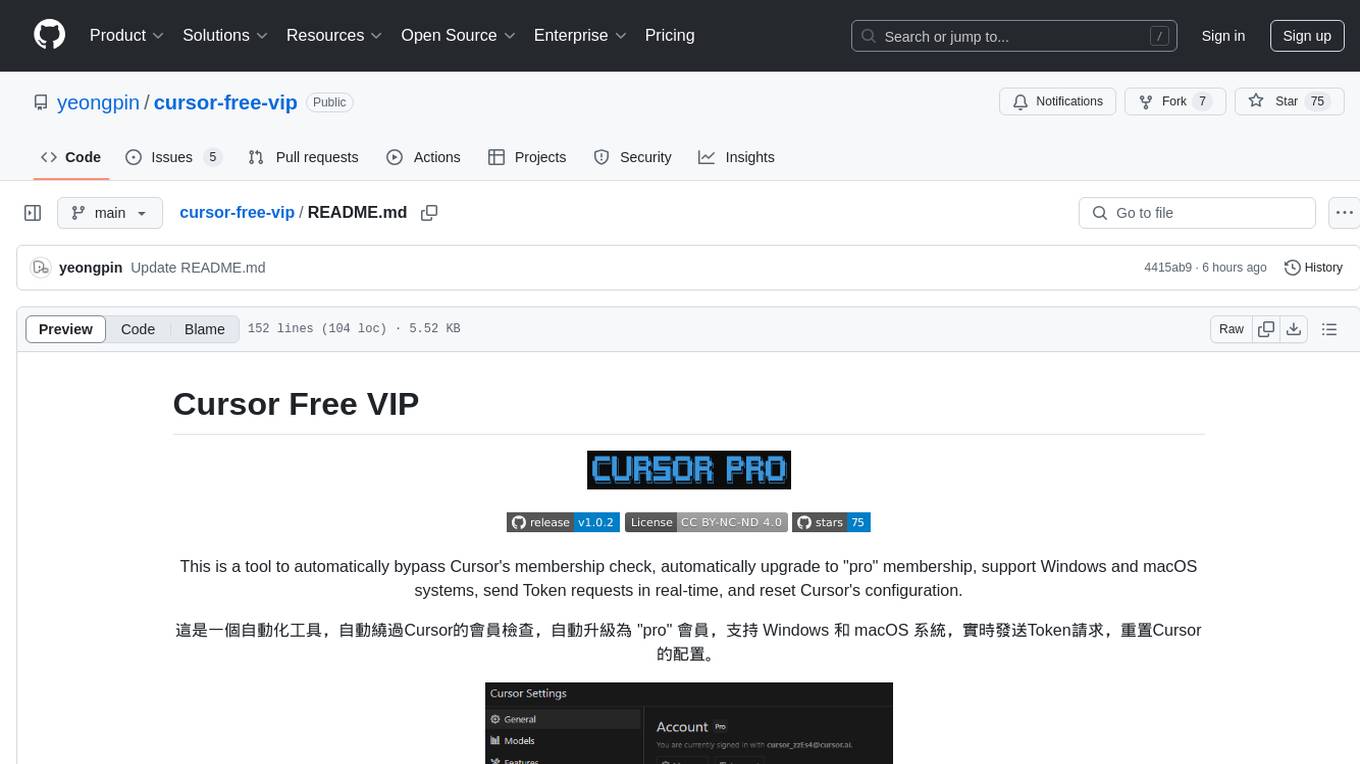
cursor-free-vip
Cursor Free VIP is a tool designed to automatically bypass Cursor's membership check, upgrade to 'pro' membership, support Windows and macOS systems, send Token requests in real-time, and reset Cursor's configuration. It provides a seamless experience for users to access premium features without the need for manual upgrades or configuration changes. The tool aims to simplify the process of accessing advanced functionalities offered by Cursor, enhancing user experience and productivity.
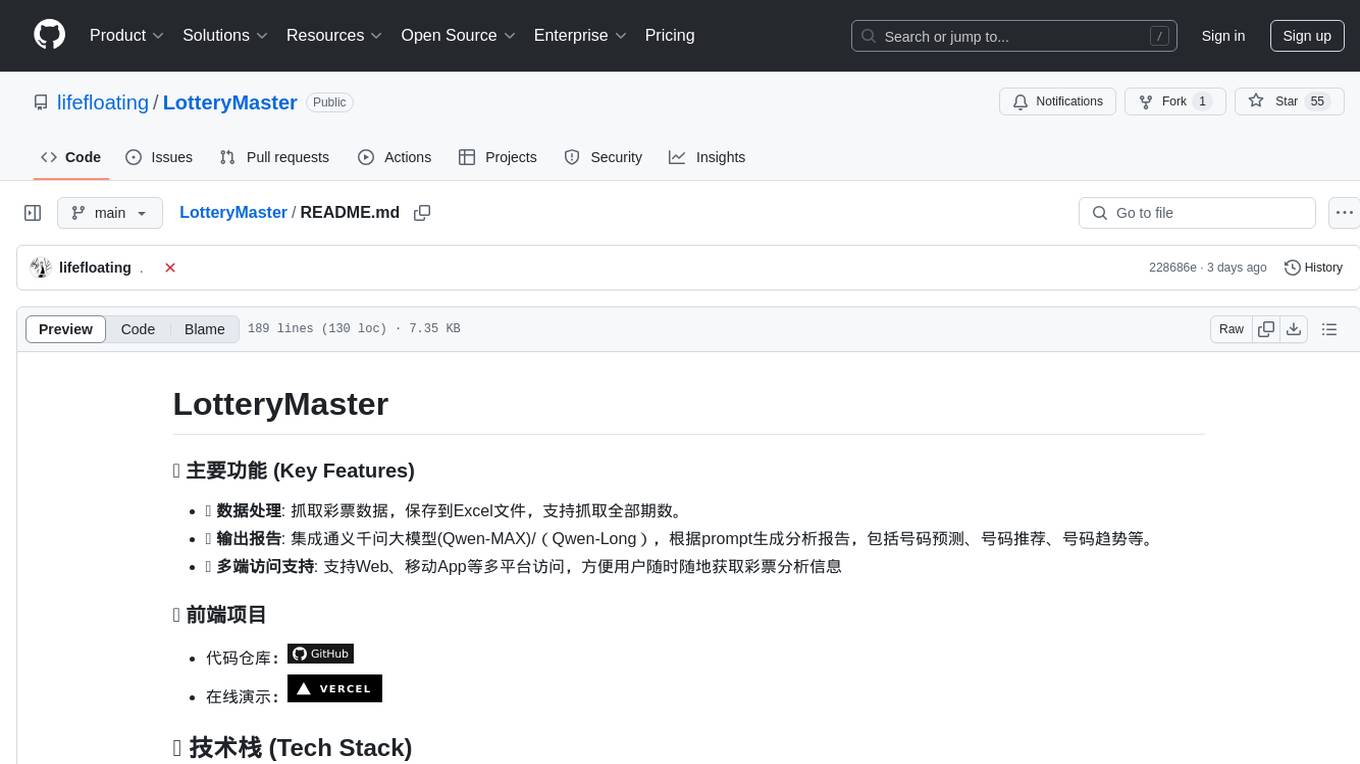
LotteryMaster
LotteryMaster is a tool designed to fetch lottery data, save it to Excel files, and provide analysis reports including number prediction, number recommendation, and number trends. It supports multiple platforms for access such as Web and mobile App. The tool integrates AI models like Qwen API and DeepSeek for generating analysis reports and trend analysis charts. Users can configure API parameters for controlling randomness, diversity, presence penalty, and maximum tokens. The tool also includes a frontend project based on uniapp + Vue3 + TypeScript for multi-platform applications. It provides a backend service running on Fastify with Node.js, Cheerio.js for web scraping, Pino for logging, xlsx for Excel file handling, and Jest for testing. The project is still in development and some features may not be fully implemented. The analysis reports are for reference only and do not constitute investment advice. Users are advised to use the tool responsibly and avoid addiction to gambling.
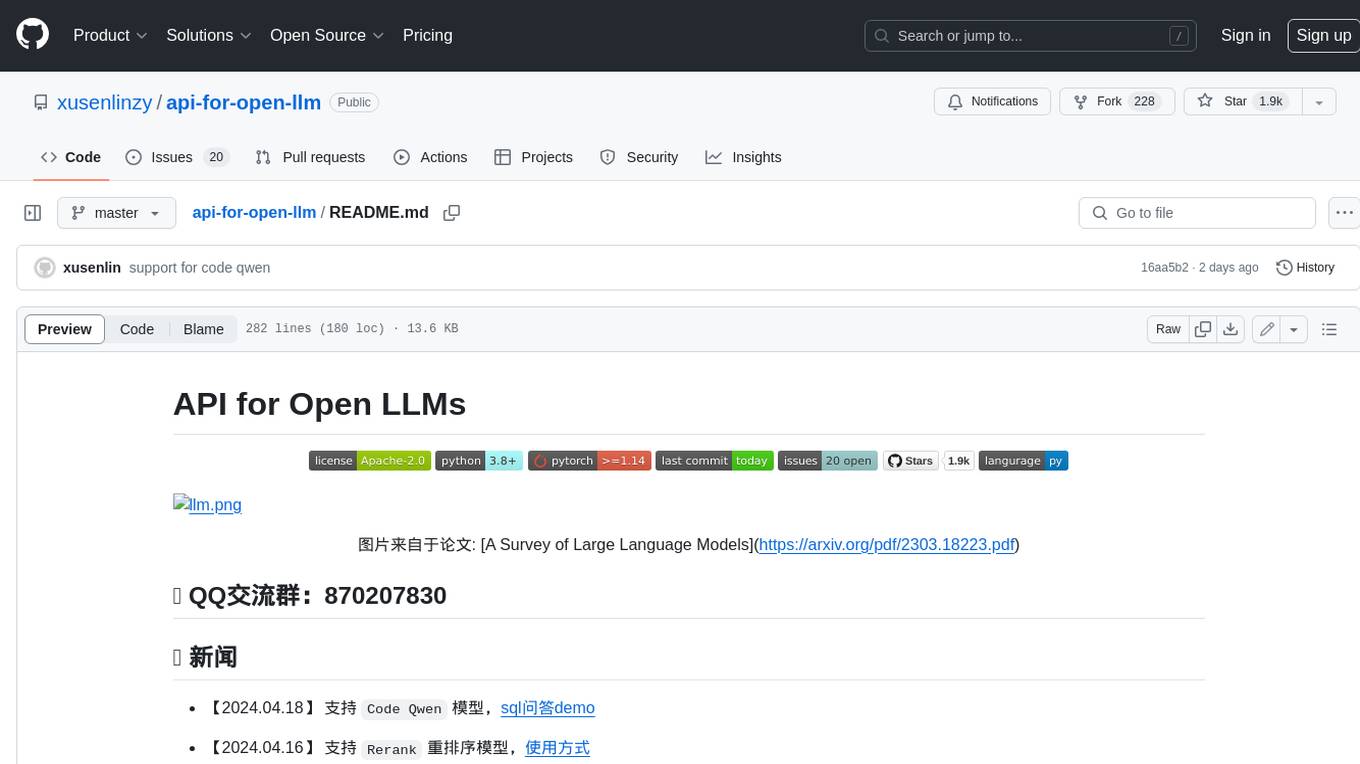
api-for-open-llm
This project provides a unified backend interface for open large language models (LLMs), offering a consistent experience with OpenAI's ChatGPT API. It supports various open-source LLMs, enabling developers to seamlessly integrate them into their applications. The interface features streaming responses, text embedding capabilities, and support for LangChain, a tool for developing LLM-based applications. By modifying environment variables, developers can easily use open-source models as alternatives to ChatGPT, providing a cost-effective and customizable solution for various use cases.
For similar tasks
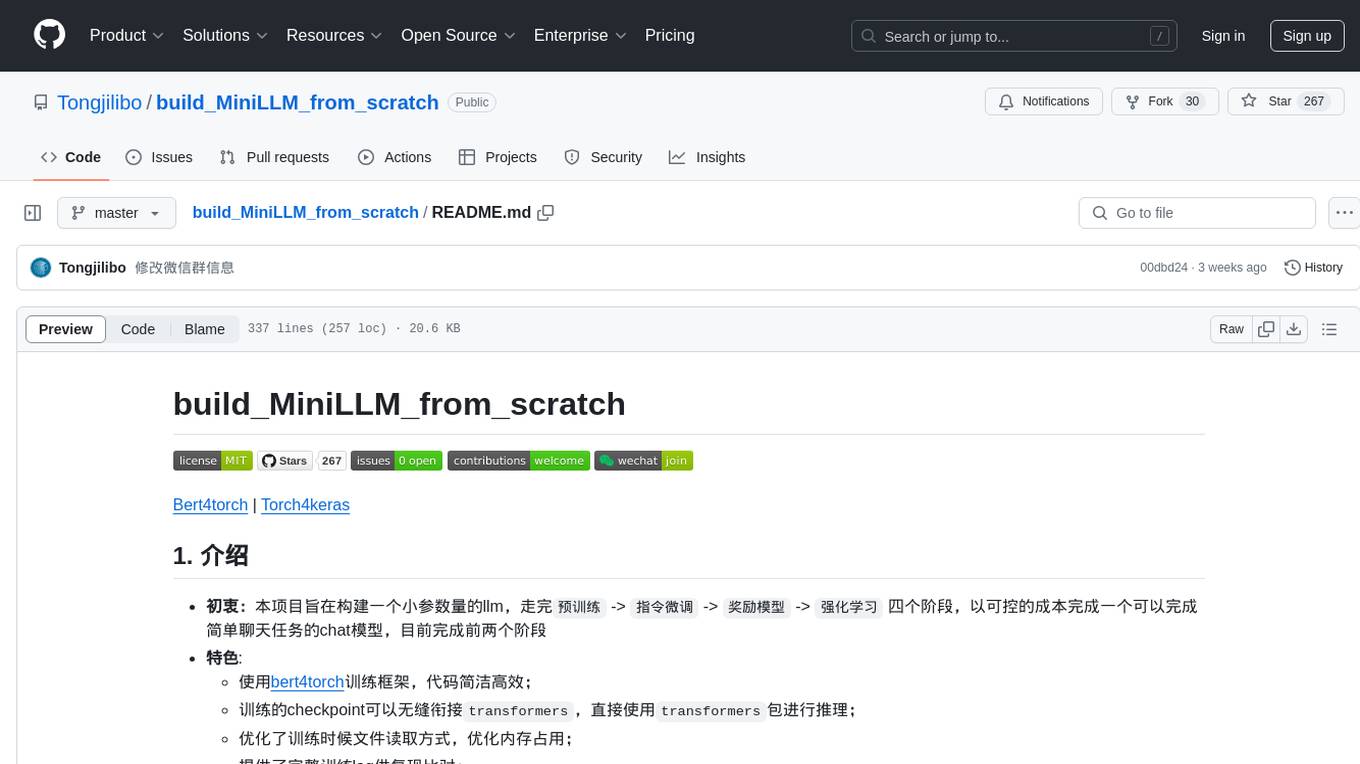
build_MiniLLM_from_scratch
This repository aims to build a low-parameter LLM model through pretraining, fine-tuning, model rewarding, and reinforcement learning stages to create a chat model capable of simple conversation tasks. It features using the bert4torch training framework, seamless integration with transformers package for inference, optimized file reading during training to reduce memory usage, providing complete training logs for reproducibility, and the ability to customize robot attributes. The chat model supports multi-turn conversations. The trained model currently only supports basic chat functionality due to limitations in corpus size, model scale, SFT corpus size, and quality.
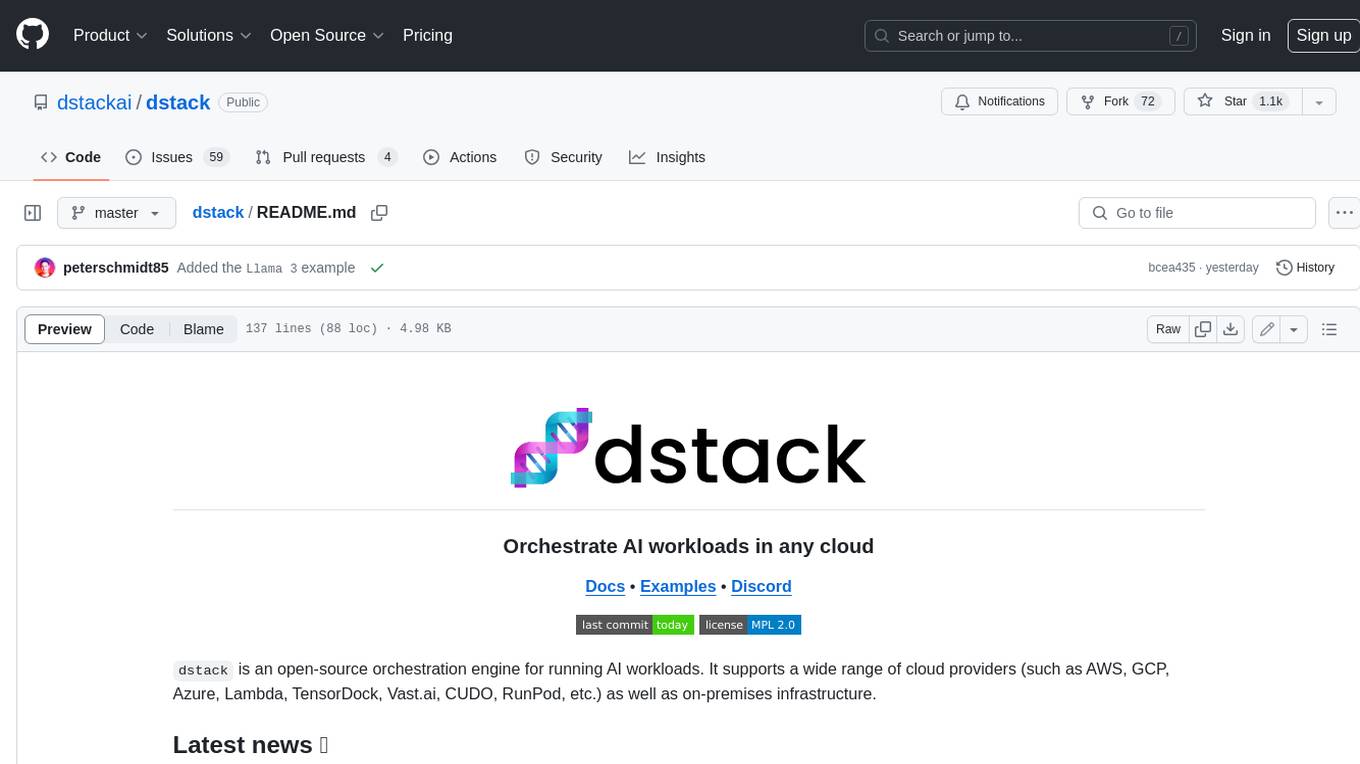
dstack
Dstack is an open-source orchestration engine for running AI workloads in any cloud. It supports a wide range of cloud providers (such as AWS, GCP, Azure, Lambda, TensorDock, Vast.ai, CUDO, RunPod, etc.) as well as on-premises infrastructure. With Dstack, you can easily set up and manage dev environments, tasks, services, and pools for your AI workloads.
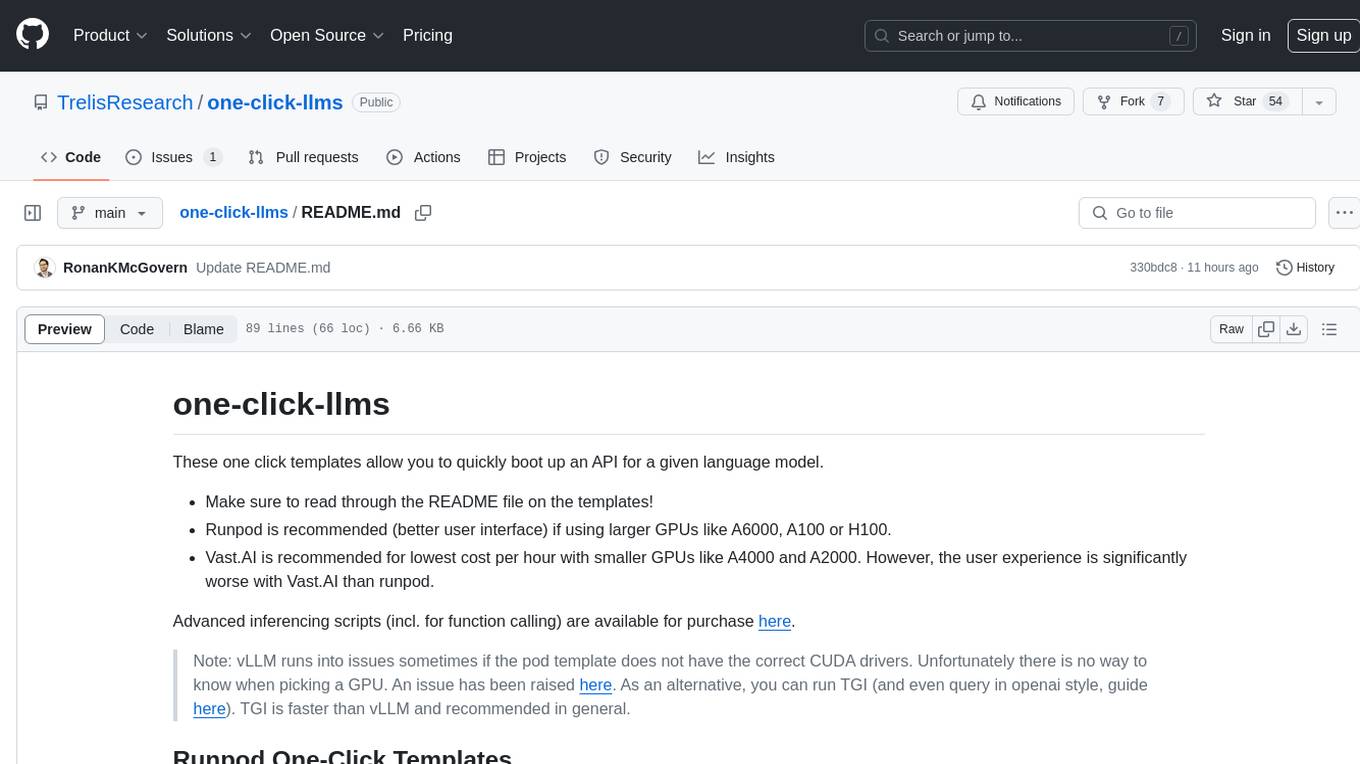
one-click-llms
The one-click-llms repository provides templates for quickly setting up an API for language models. It includes advanced inferencing scripts for function calling and offers various models for text generation and fine-tuning tasks. Users can choose between Runpod and Vast.AI for different GPU configurations, with recommendations for optimal performance. The repository also supports Trelis Research and offers templates for different model sizes and types, including multi-modal APIs and chat models.
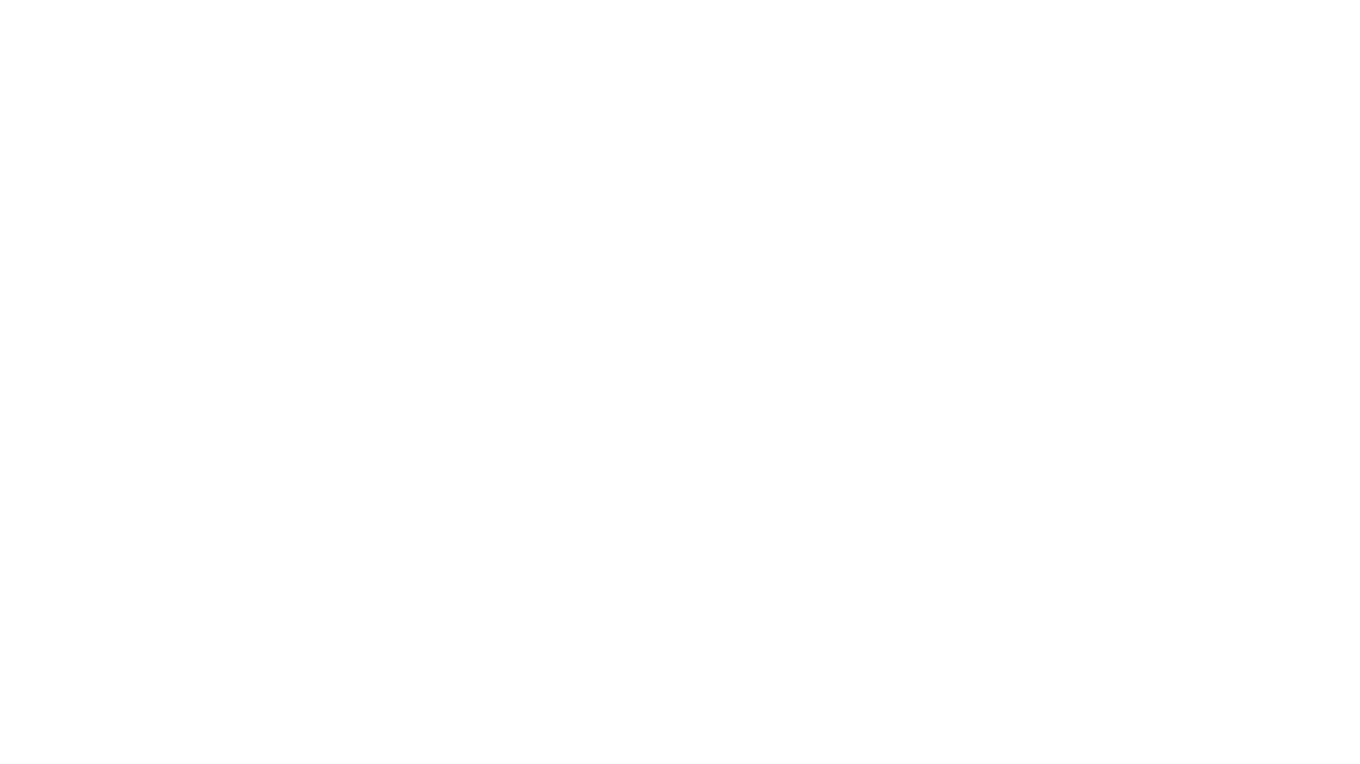
starcoder2-self-align
StarCoder2-Instruct is an open-source pipeline that introduces StarCoder2-15B-Instruct-v0.1, a self-aligned code Large Language Model (LLM) trained with a fully permissive and transparent pipeline. It generates instruction-response pairs to fine-tune StarCoder-15B without human annotations or data from proprietary LLMs. The tool is primarily finetuned for Python code generation tasks that can be verified through execution, with potential biases and limitations. Users can provide response prefixes or one-shot examples to guide the model's output. The model may have limitations with other programming languages and out-of-domain coding tasks.
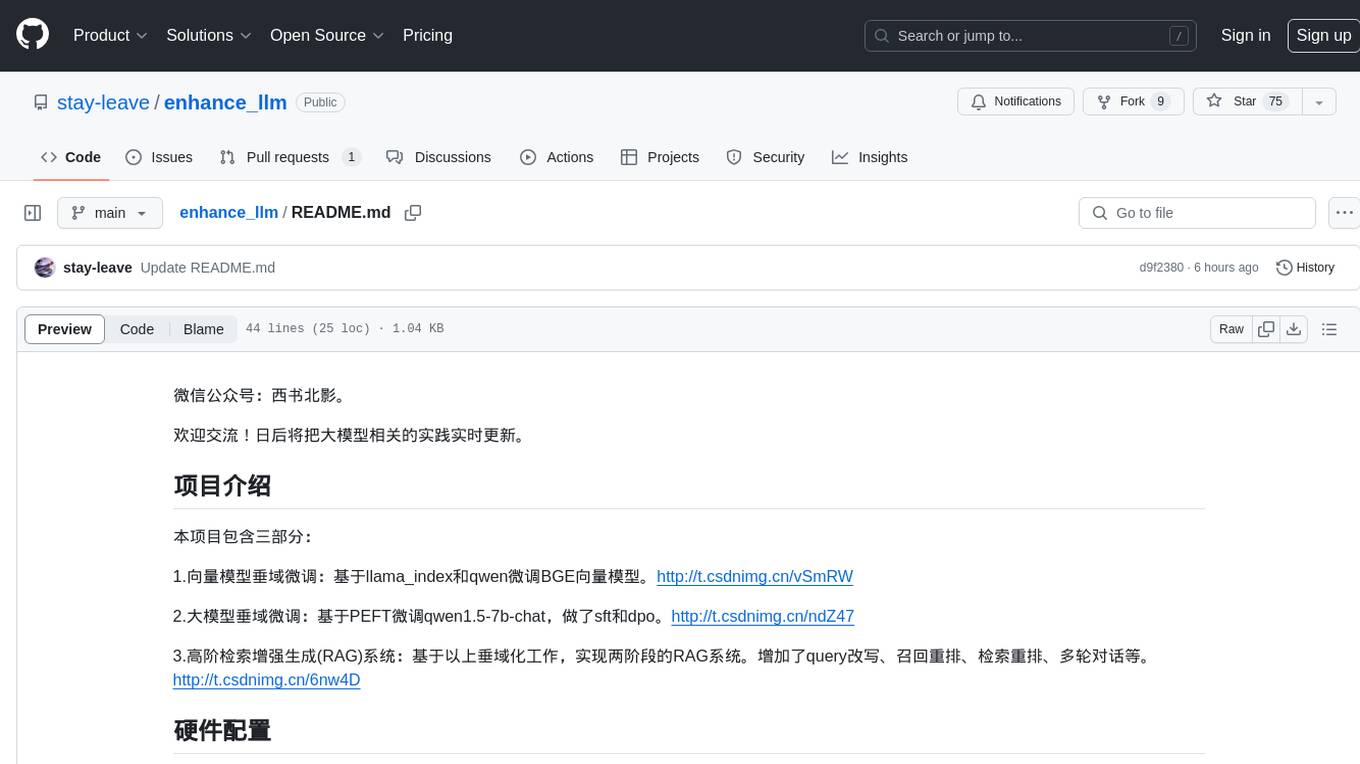
enhance_llm
The enhance_llm repository contains three main parts: 1. Vector model domain fine-tuning based on llama_index and qwen fine-tuning BGE vector model. 2. Large model domain fine-tuning based on PEFT fine-tuning qwen1.5-7b-chat, with sft and dpo. 3. High-order retrieval enhanced generation (RAG) system based on the above domain work, implementing a two-stage RAG system. It includes query rewriting, recall reordering, retrieval reordering, multi-turn dialogue, and more. The repository also provides hardware and environment configurations along with star history and licensing information.
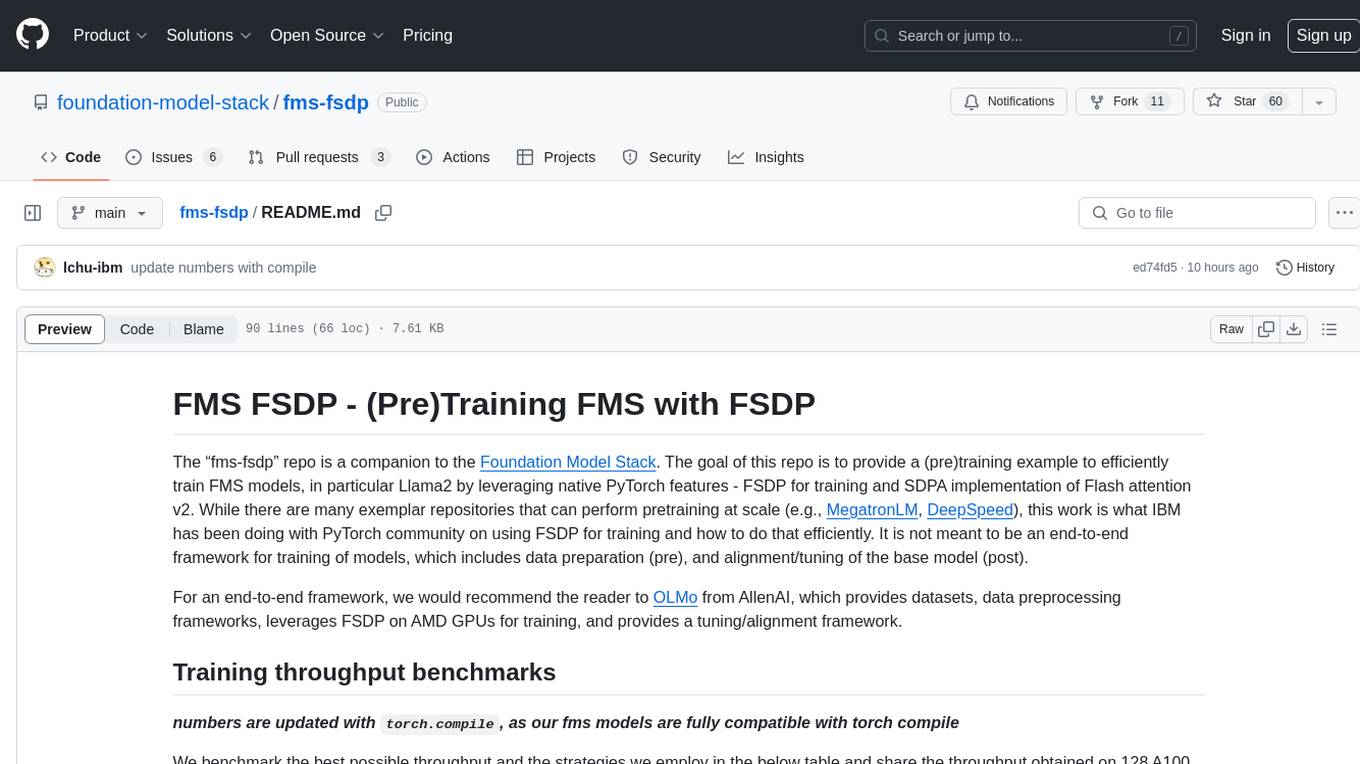
fms-fsdp
The 'fms-fsdp' repository is a companion to the Foundation Model Stack, providing a (pre)training example to efficiently train FMS models, specifically Llama2, using native PyTorch features like FSDP for training and SDPA implementation of Flash attention v2. It focuses on leveraging FSDP for training efficiently, not as an end-to-end framework. The repo benchmarks training throughput on different GPUs, shares strategies, and provides installation and training instructions. It trained a model on IBM curated data achieving high efficiency and performance metrics.
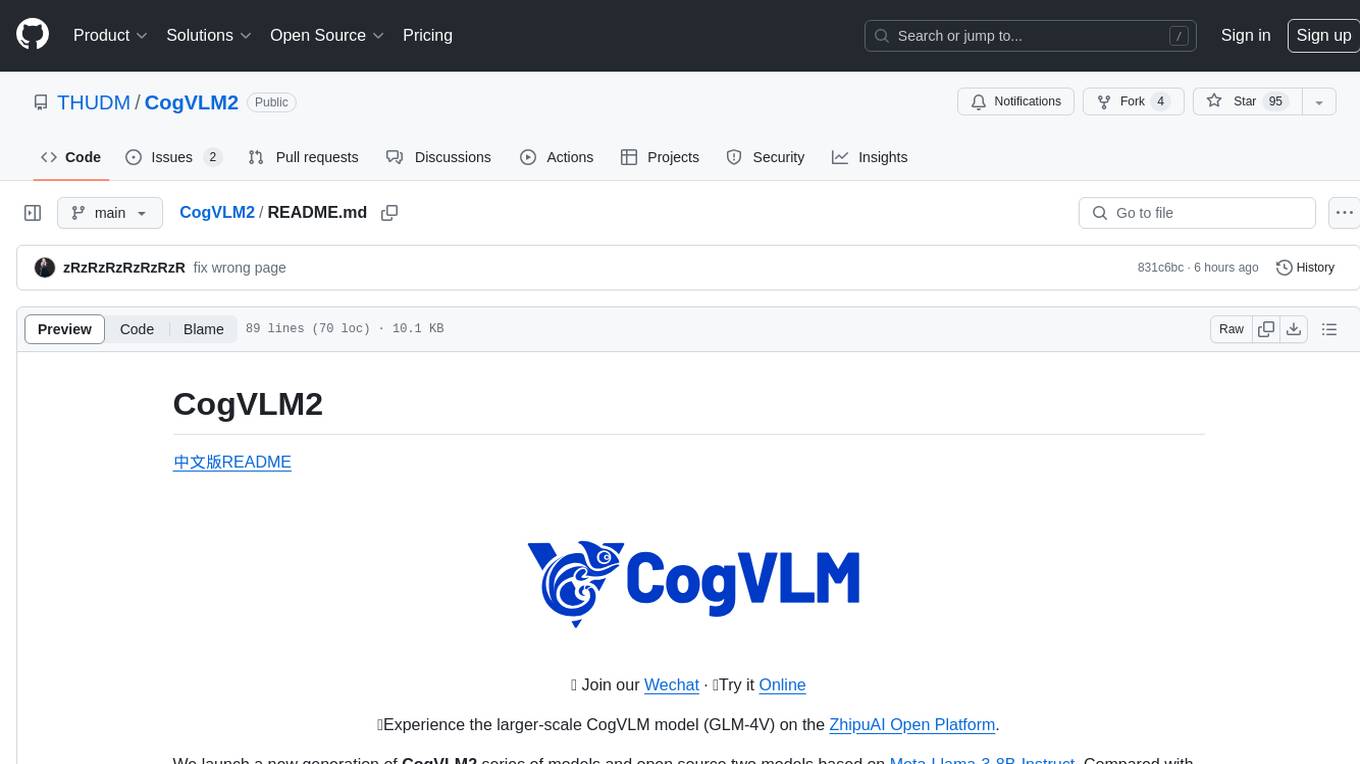
CogVLM2
CogVLM2 is a new generation of open source models that offer significant improvements in benchmarks such as TextVQA and DocVQA. It supports 8K content length, image resolution up to 1344 * 1344, and both Chinese and English languages. The project provides basic calling methods, fine-tuning examples, and OpenAI API format calling examples to help developers quickly get started with the model.
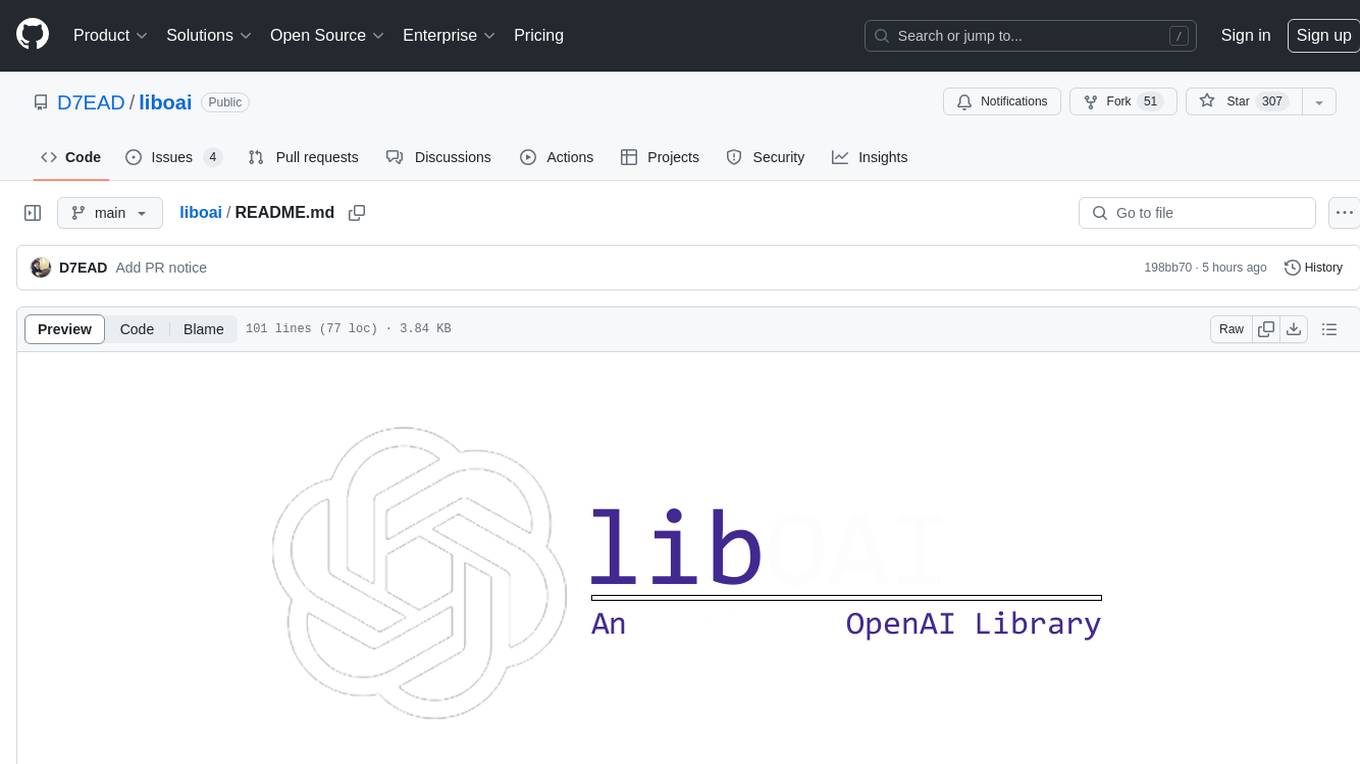
liboai
liboai is a simple C++17 library for the OpenAI API, providing developers with access to OpenAI endpoints through a collection of methods and classes. It serves as a spiritual port of OpenAI's Python library, 'openai', with similar structure and features. The library supports various functionalities such as ChatGPT, Audio, Azure, Functions, Image DALL·E, Models, Completions, Edit, Embeddings, Files, Fine-tunes, Moderation, and Asynchronous Support. Users can easily integrate the library into their C++ projects to interact with OpenAI services.
For similar jobs
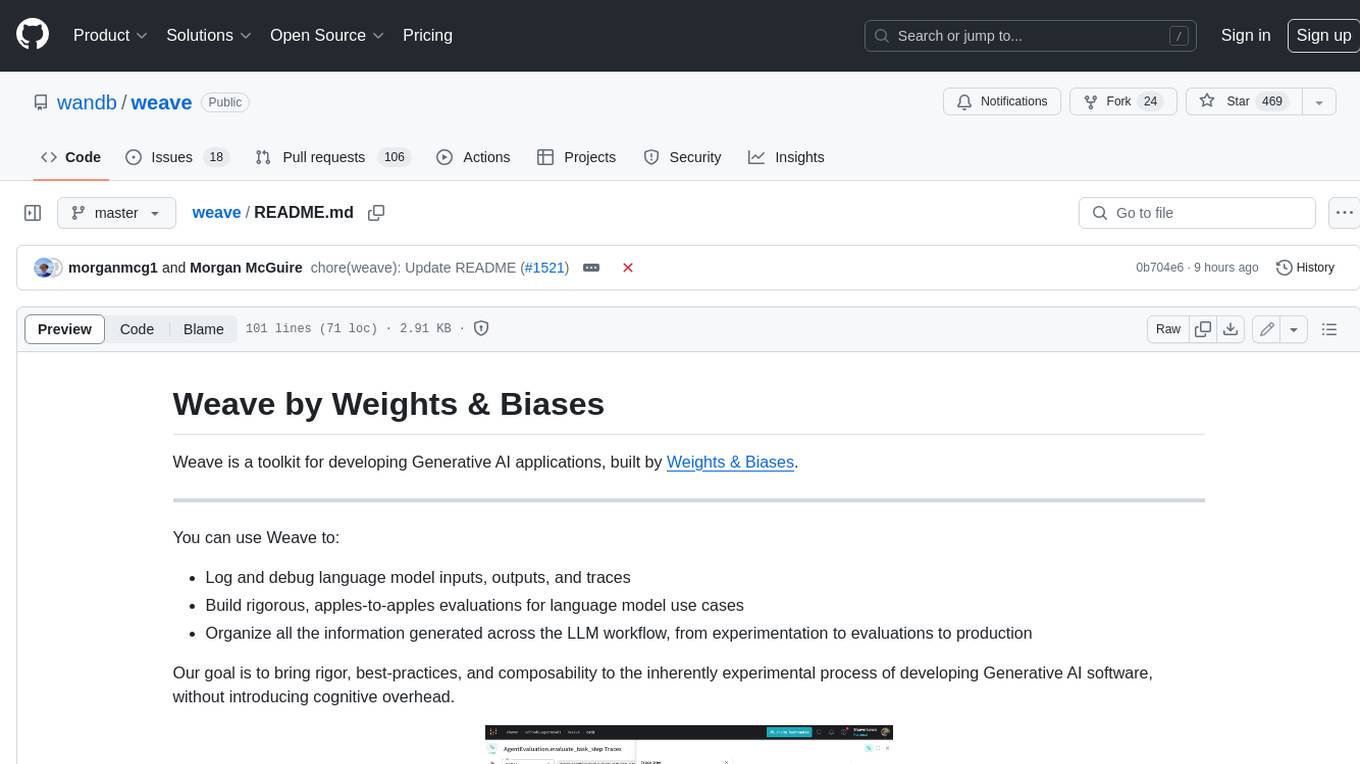
weave
Weave is a toolkit for developing Generative AI applications, built by Weights & Biases. With Weave, you can log and debug language model inputs, outputs, and traces; build rigorous, apples-to-apples evaluations for language model use cases; and organize all the information generated across the LLM workflow, from experimentation to evaluations to production. Weave aims to bring rigor, best-practices, and composability to the inherently experimental process of developing Generative AI software, without introducing cognitive overhead.
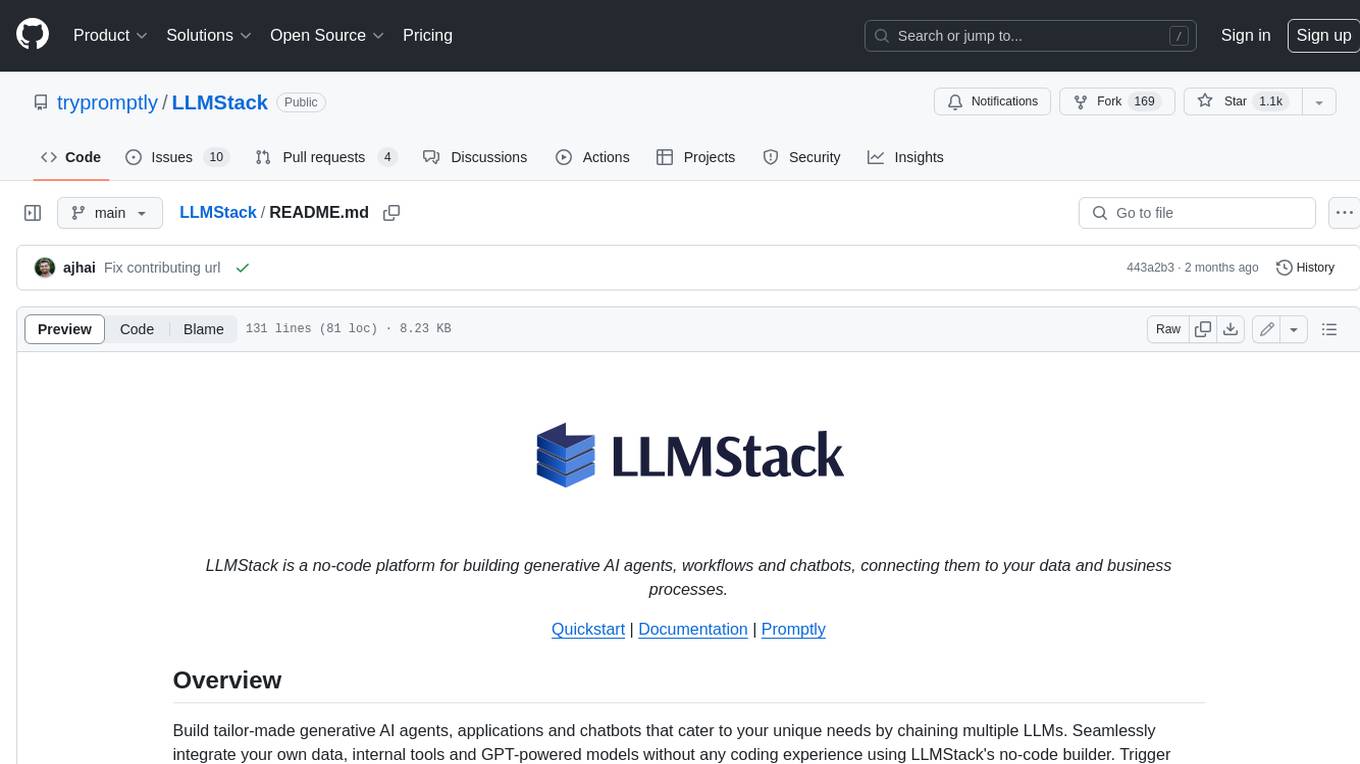
LLMStack
LLMStack is a no-code platform for building generative AI agents, workflows, and chatbots. It allows users to connect their own data, internal tools, and GPT-powered models without any coding experience. LLMStack can be deployed to the cloud or on-premise and can be accessed via HTTP API or triggered from Slack or Discord.
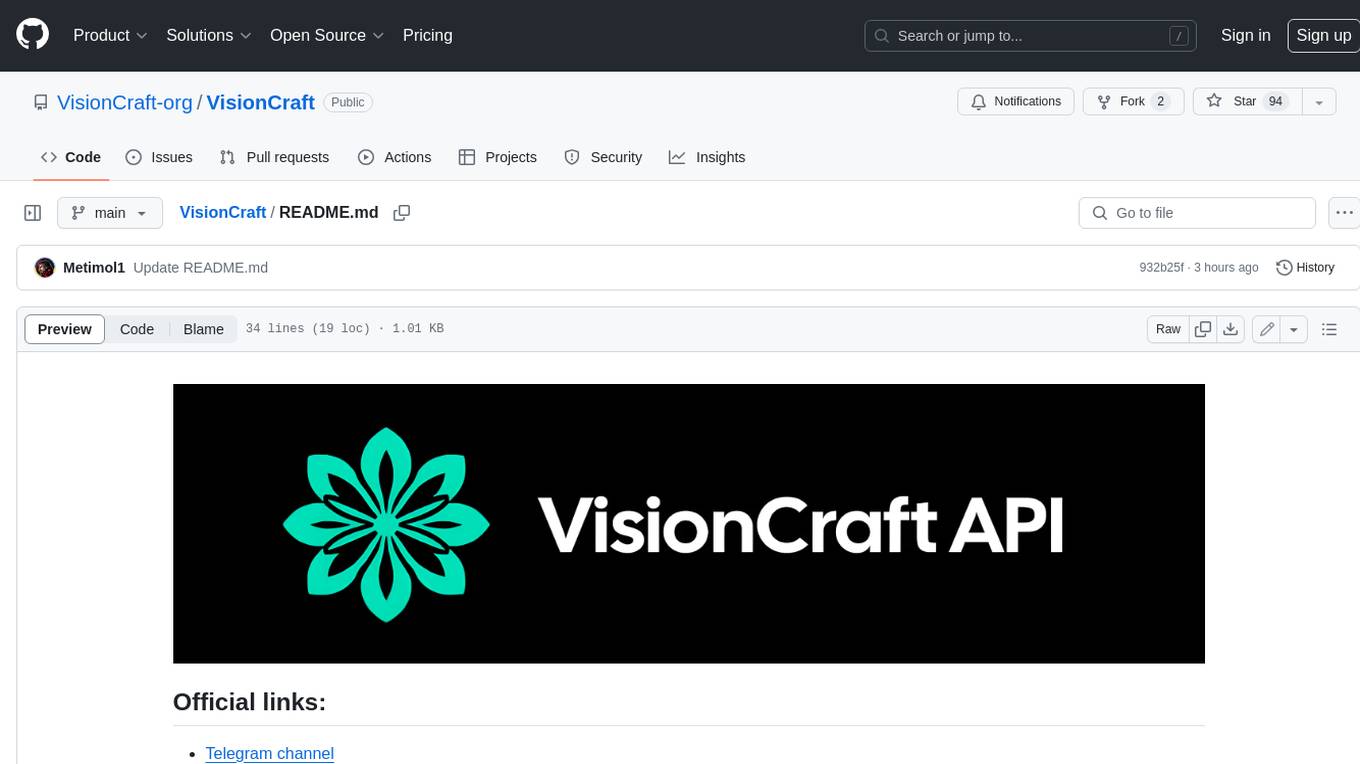
VisionCraft
The VisionCraft API is a free API for using over 100 different AI models. From images to sound.
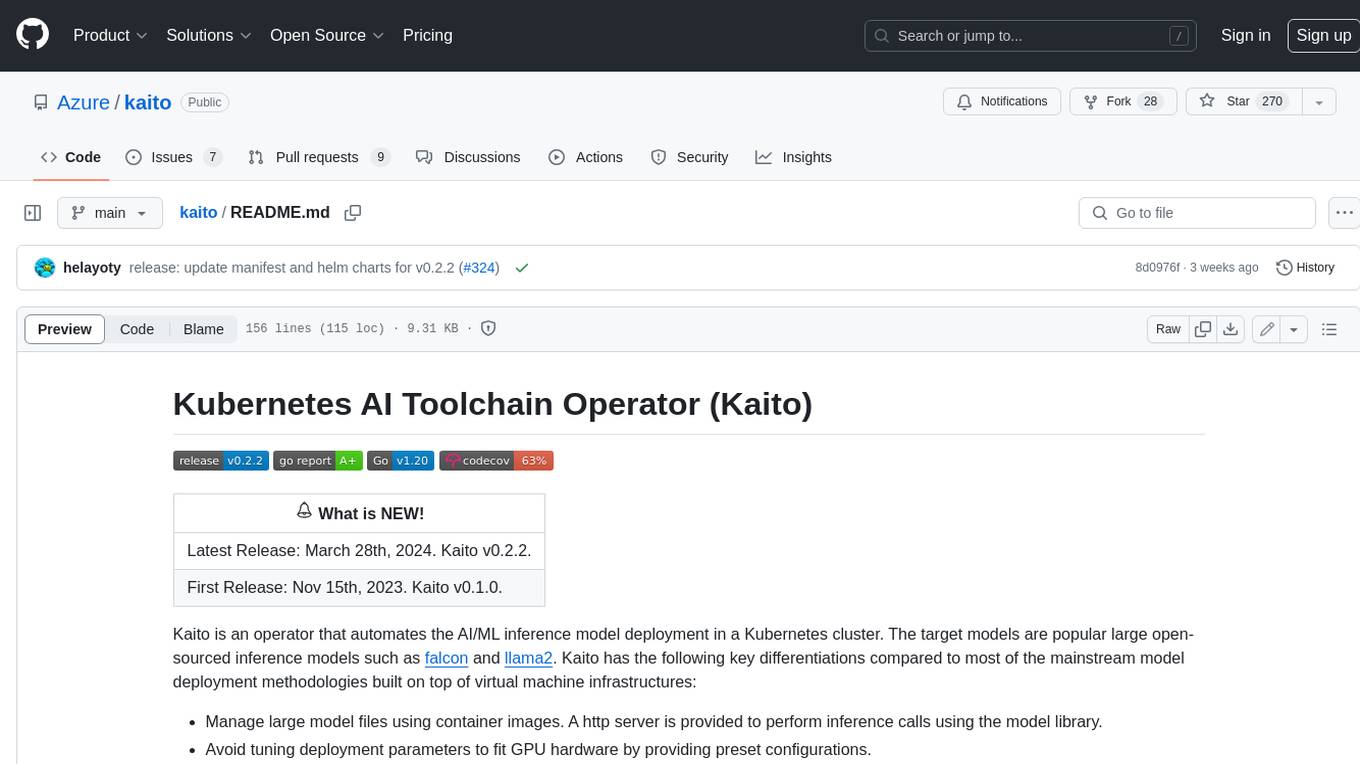
kaito
Kaito is an operator that automates the AI/ML inference model deployment in a Kubernetes cluster. It manages large model files using container images, avoids tuning deployment parameters to fit GPU hardware by providing preset configurations, auto-provisions GPU nodes based on model requirements, and hosts large model images in the public Microsoft Container Registry (MCR) if the license allows. Using Kaito, the workflow of onboarding large AI inference models in Kubernetes is largely simplified.
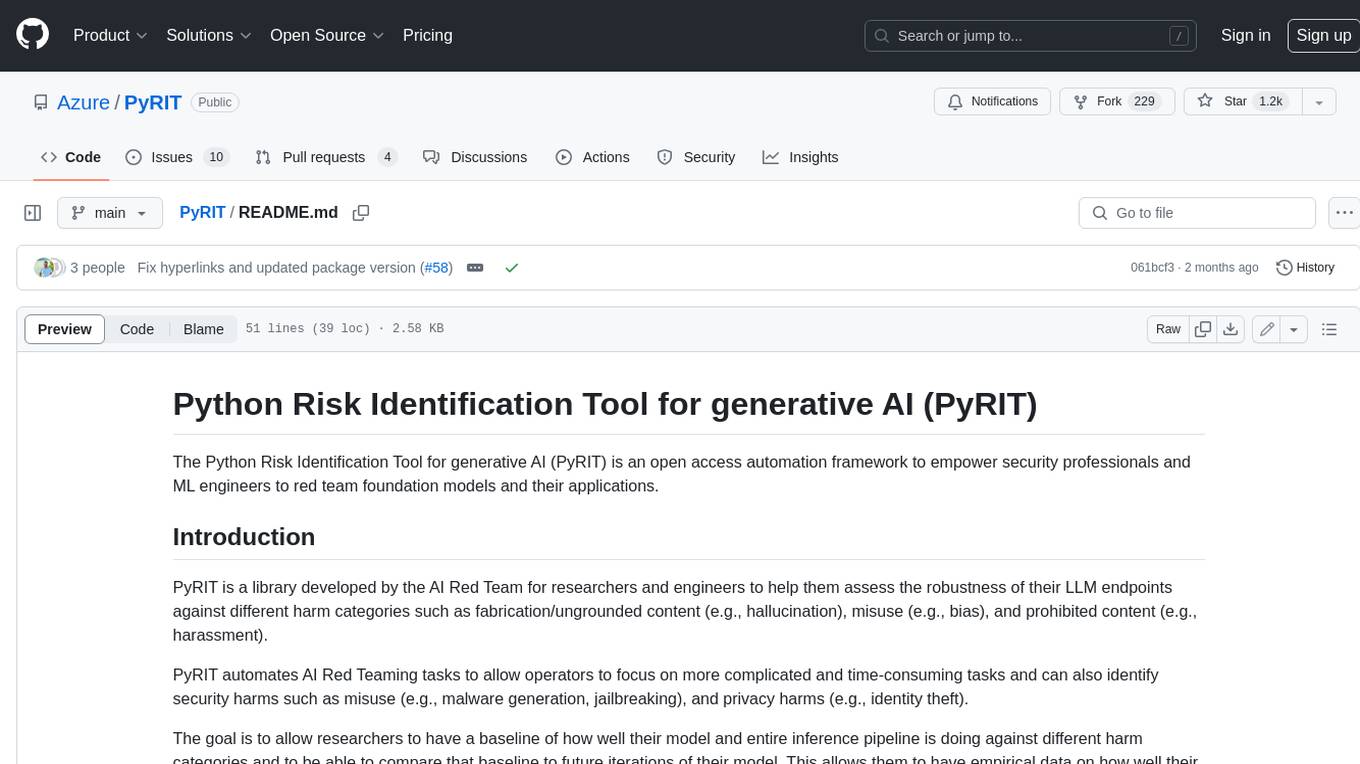
PyRIT
PyRIT is an open access automation framework designed to empower security professionals and ML engineers to red team foundation models and their applications. It automates AI Red Teaming tasks to allow operators to focus on more complicated and time-consuming tasks and can also identify security harms such as misuse (e.g., malware generation, jailbreaking), and privacy harms (e.g., identity theft). The goal is to allow researchers to have a baseline of how well their model and entire inference pipeline is doing against different harm categories and to be able to compare that baseline to future iterations of their model. This allows them to have empirical data on how well their model is doing today, and detect any degradation of performance based on future improvements.
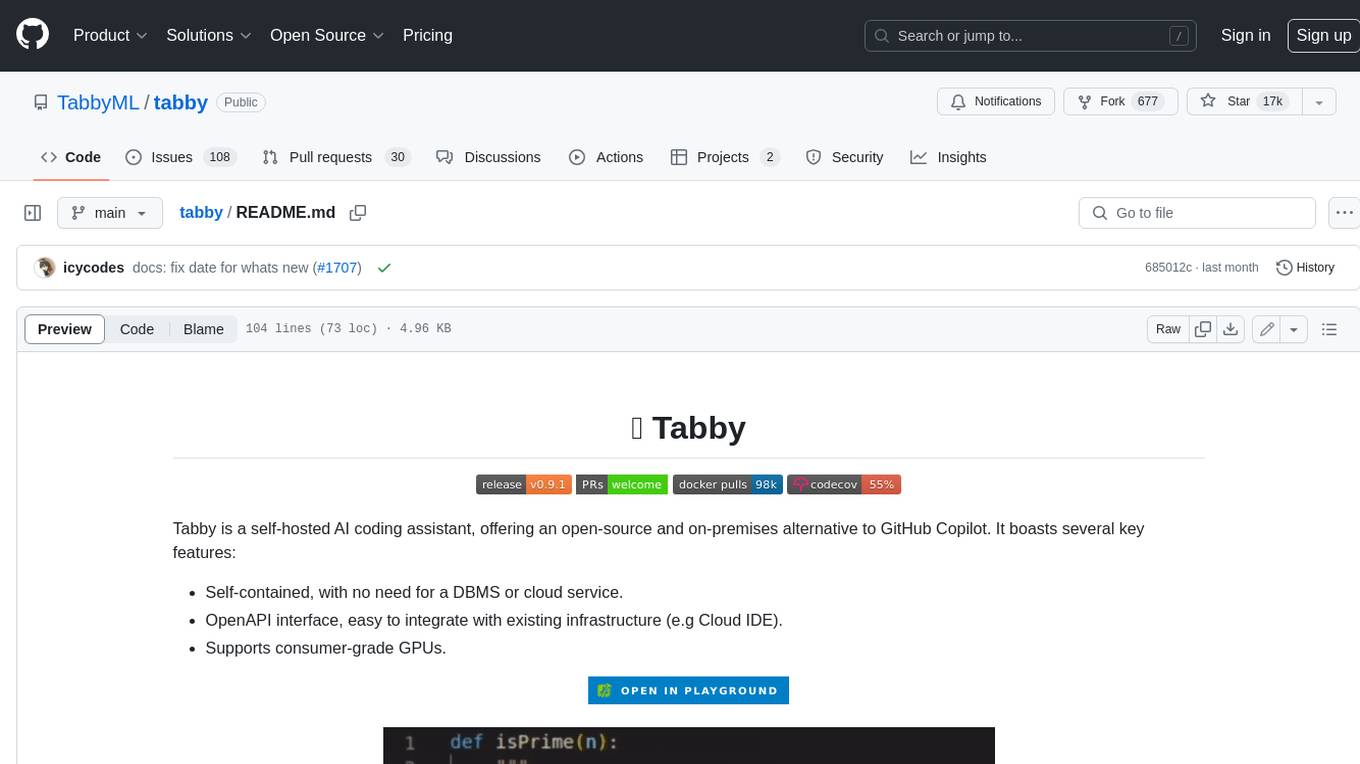
tabby
Tabby is a self-hosted AI coding assistant, offering an open-source and on-premises alternative to GitHub Copilot. It boasts several key features: * Self-contained, with no need for a DBMS or cloud service. * OpenAPI interface, easy to integrate with existing infrastructure (e.g Cloud IDE). * Supports consumer-grade GPUs.
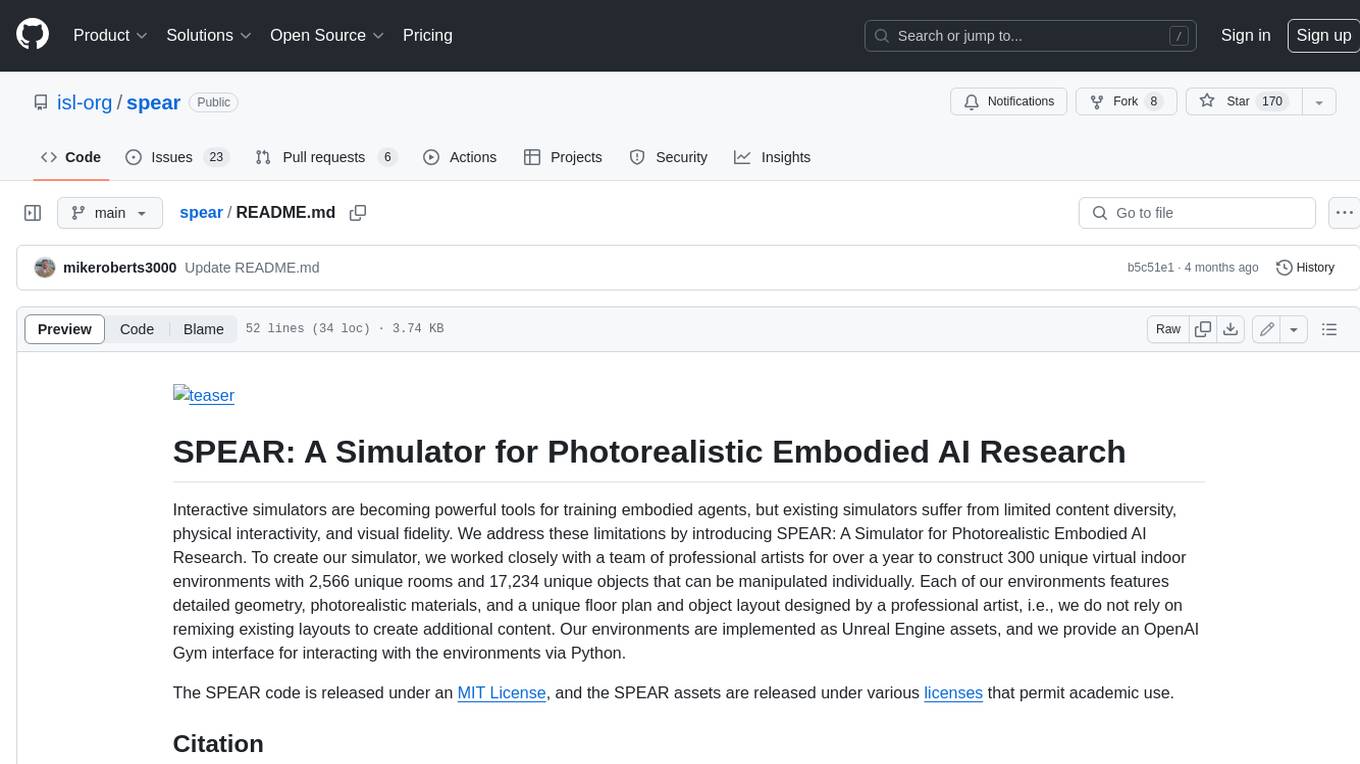
spear
SPEAR (Simulator for Photorealistic Embodied AI Research) is a powerful tool for training embodied agents. It features 300 unique virtual indoor environments with 2,566 unique rooms and 17,234 unique objects that can be manipulated individually. Each environment is designed by a professional artist and features detailed geometry, photorealistic materials, and a unique floor plan and object layout. SPEAR is implemented as Unreal Engine assets and provides an OpenAI Gym interface for interacting with the environments via Python.
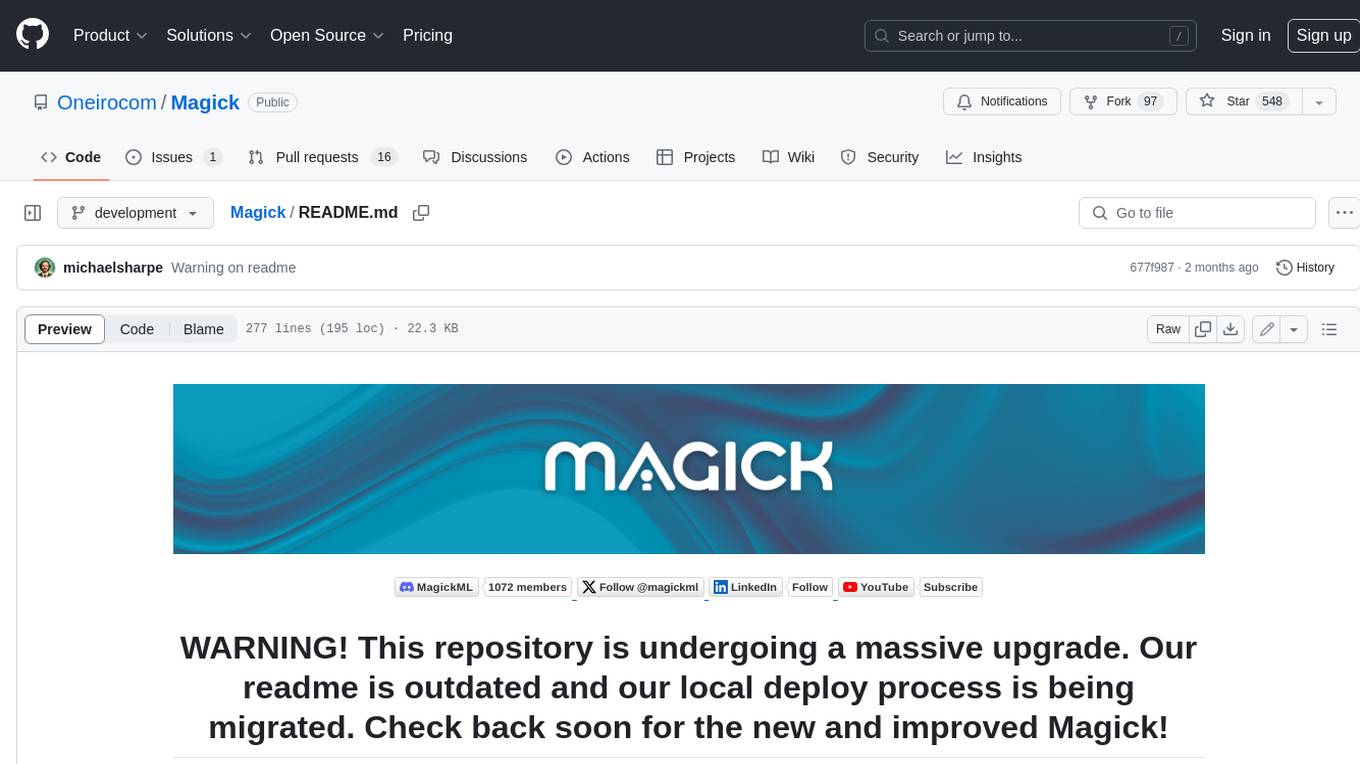
Magick
Magick is a groundbreaking visual AIDE (Artificial Intelligence Development Environment) for no-code data pipelines and multimodal agents. Magick can connect to other services and comes with nodes and templates well-suited for intelligent agents, chatbots, complex reasoning systems and realistic characters.