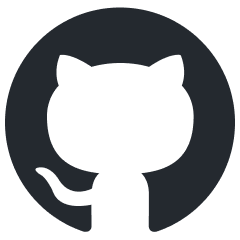
dora
DORA (Dataflow-Oriented Robotic Architecture) is middleware designed to streamline and simplify the creation of AI-based robotic applications. It offers low latency, composable, and distributed dataflow capabilities. Applications are modeled as directed graphs, also referred to as pipelines.
Stars: 1675
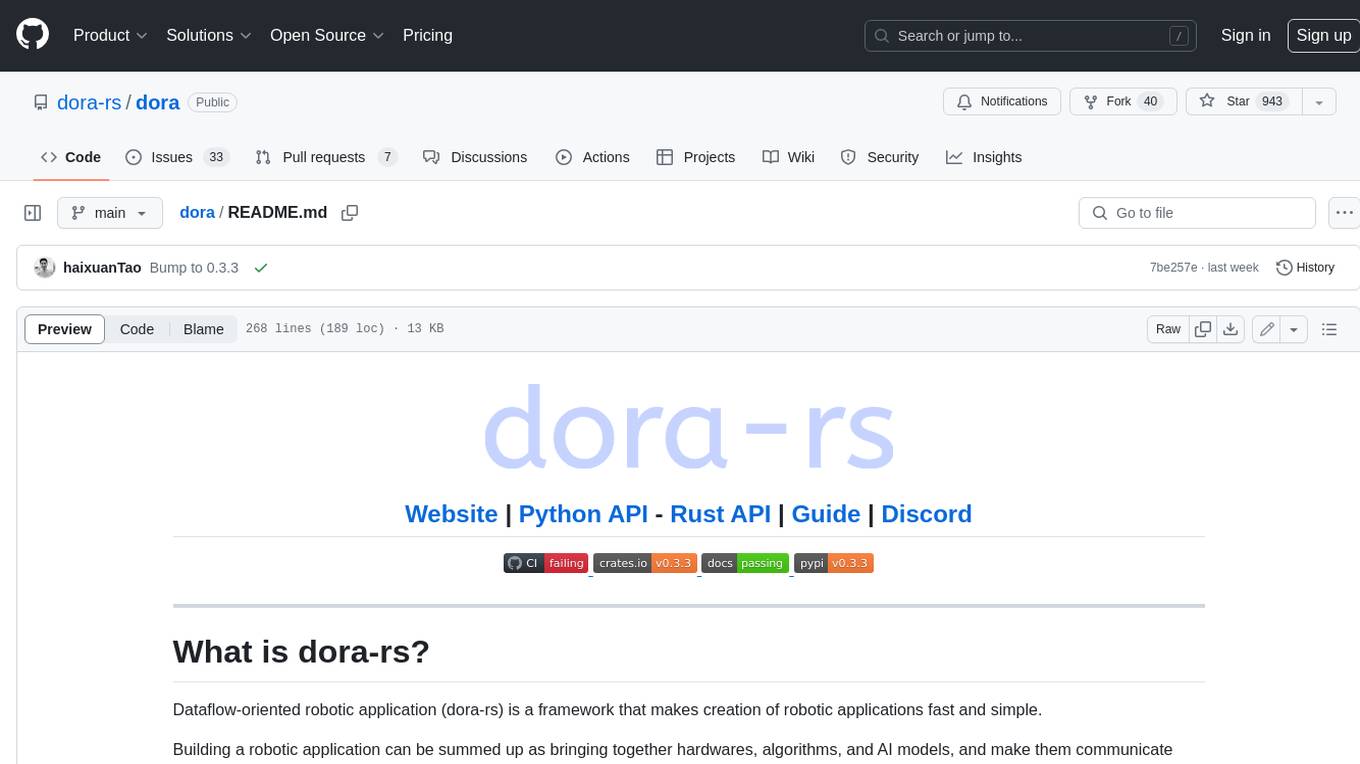
Dataflow-oriented robotic application (dora-rs) is a framework that makes creation of robotic applications fast and simple. Building a robotic application can be summed up as bringing together hardwares, algorithms, and AI models, and make them communicate with each others. At dora-rs, we try to: make integration of hardware and software easy by supporting Python, C, C++, and also ROS2. make communication low latency by using zero-copy Arrow messages. dora-rs is still experimental and you might experience bugs, but we're working very hard to make it stable as possible.
README:
Website | Python API | Rust API | Guide | Discord
An extremely fast and simple dataflow oriented robotic framework to manage your projects and run complex apps, written in Rust.
Latency benchmark with Python API for both framework, sending 40M of random bytes.
- đ A single CLI to launch your Python and Rust robotic projects.
- âĄī¸ 10-17x faster than
ros2
. - đ Easy and Fast prototyping with a clean Python API.
- đĨī¸ Supports macOS, Linux, and Windows.
- âŦ Installable without Rust via
curl
orpowershell
. - âī¸ Includes a large set of pre-packaged nodes for fast prototyping.
- đ ī¸ Build and Run applications without compilation step beyond the native compiler of your favourite language.
- đ¤ Simplifies building robotic applications by integrating hardware, algorithms, and AI models to facilitate seamless communication.
- âī¸ Eases integration of hardware and software by supporting Python, C, C++, and ROS2, while ensuring low-latency communication with zero-copy Arrow messages.
Install dora with our standalone installers, or from crates.io:
curl --proto '=https' --tlsv1.2 -sSf https://raw.githubusercontent.com/dora-rs/dora/main/install.sh | bash
powershell -c "irm https://raw.githubusercontent.com/dora-rs/dora/main/install.ps1 | iex"
cargo install dora-cli
The full documentation is available on our website. A lot of guides are available on this section of our website.
- Run the benchmark example (Rust must be installed):
git clone https://github.com/dora-rs/dora
cd dora
cargo run --example benchmark --release
- Run some Python examples (A venv must be activated):
# activate your venv here
cd dora/examples/python-dataflow
dora up
dora build dataflow.yml
dora start dataflow.yml
Make sure to have a webcam
To stop your dataflow, you can use ctrl+c
Dataflow-Oriented Robotic Architecture (dora-rs
) is a framework that makes creation of robotic applications fast and simple.
dora-rs
implements a declarative dataflow paradigm where tasks are split between nodes isolated as individual processes.
Each node defines its inputs and outputs to connect with other nodes.
nodes:
- id: camera
path: opencv-video-capture
inputs:
tick: dora/timer/millis/20
outputs:
- image
-
- id: plot
path: opencv-plot
inputs:
image: camera/image
The dataflow paradigm has the advantage of creating an abstraction layer that makes robotic applications modular and easily configurable.
It offers several features, such as:
Communication between nodes is handled with shared memory on a same machine and TCP on distributed machines. Our shared memory implementation tracks messages across processes and discards them when obsolete. Shared memory slots are cached to avoid new memory allocation.
Nodes communicate with Apache Arrow Data Format.
Apache Arrow is a universal memory format for flat and hierarchical data. The Arrow memory format supports zero-copy reads for lightning-fast data access without serialization overhead. It defines a C data interface without any build-time or link-time dependency requirement, that means that dora-rs
has no compilation step beyond the native compiler of your favourite language.
dora-rs uses Opentelemetry to record all your logs, metrics and traces. This means that the data and telemetry can be linked using a shared abstraction.
Opentelemetry is an open source observability standard that makes dora-rs telemetry collectable by most backends such as elasticsearch, prometheus, Datadog...
Opentelemetry is language independent, backend agnostic, and easily collect distributed data, making it perfect for dora-rs applications.
dora-rs implements Hot-Reloading for python which means you can change code at runtime in Python while keeping your state intact.
Using the feature flag: --attach --hot-reload
, dora-rs watch for code change and reload nodes that has been modified.
You can check fail-safe mechanism at: https://github.com/dora-rs/dora/pull/239.
See this demo.
Note: this feature is marked as unstable.
- Compilation Free Message passing to ROS 2
- Automatic conversion ROS 2 Message <-> Arrow Array
import pyarrow as pa
# Configuration Boilerplate...
turtle_twist_writer = ...
## Arrow Based ROS2 Twist Message
## which does not require ROS2 import
message = pa.array([{
"linear": {
"x": 1,
},
"angular": {
"z": 1
},
}])
turtle_twist_writer.publish(message)
You might want to use ChatGPT to write the Arrow Formatting: https://chat.openai.com/share/4eec1c6d-dbd2-46dc-b6cd-310d2895ba15
You can easily create a self-coding robot, by combining Hot-reloading with a Retrieval Augmented Generation (RAG) that is going to generate code modification from your prompt. See:examples/python-operator-dataflow
Self-Coding Robot is just the tip of the iceberg of robotics combined with llm, that we hope to power. There is so much more that we haven't explored yet like:
Cool hardware that we think might be good fit to try out dora-rs đ We are not sponsored by manufacturers:
Price | Open Source | Github | type | Dora Project | |
---|---|---|---|---|---|
DJI Robomaster S1 | 550$ | SDK | https://github.com/dji-sdk/RoboMaster-SDK | Rover | https://huggingface.co/datasets/dora-rs/dora-robomaster |
DJI Robomaster EP Core | 950$ | SDK | https://github.com/dji-sdk/RoboMaster-SDK | Rover, Arm | |
DJI Tello | 100$ | Drone | |||
BitCraze Crazyflies | 225$ | Firmware, Lib, SDK | https://github.com/bitcraze | Drone | |
AlexanderKoch-Koch/low_cost_robot | 250$ | Everything | https://github.com/AlexanderKoch-Koch/low_cost_robot | Arm | |
xArm 1S | 200$ | Arm | |||
Wavego | 250$ | Quadruplet | |||
AINex | 800$ | Humanoid |
For more: https://docs.google.com/spreadsheets/d/1YYeW2jfOIWDVgdEgqnMvltonHquQ7K8OZCrnJRELL6o/edit#gid=0
dora-rs | Hoped for | |
---|---|---|
Tier 1 Support | Python, Rust | C, C++, ROS 2 |
Tier 2 Support | C, C++, ROS2 | |
Hot-reloading | Python | Rust (https://github.com/orgs/dora-rs/discussions/360) |
Message Format | Arrow | Native |
Local Communication | Shared Memory | Custom Middleware, zero-copy GPU IPC, intra-process tokio::channel communication |
Remote Communication | TCP | Custom Middleware, Zenoh |
Metrics, Tracing, and Logging | Opentelemetry | Native logging libraries into Opentelemetry |
Data archives | Parquet (dora-record) | |
Visualization and annotation | OpenCV | rerun.io |
Supported Platforms (x86) | Windows, macOS, Linux | |
Supported Platforms (ARM) | macOS, Linux | |
Configuration | YAML |
We are passionate about supporting contributors of all levels of experience and would love to see you get involved in the project. See the contributing guide to get started.
Our main communication channels are:
Feel free to reach out on any topic, issues or ideas.
We also have a contributing guide.
This project is licensed under Apache-2.0. Check out NOTICE.md for more information.
For Tasks:
Click tags to check more tools for each tasksFor Jobs:
Alternative AI tools for dora
Similar Open Source Tools
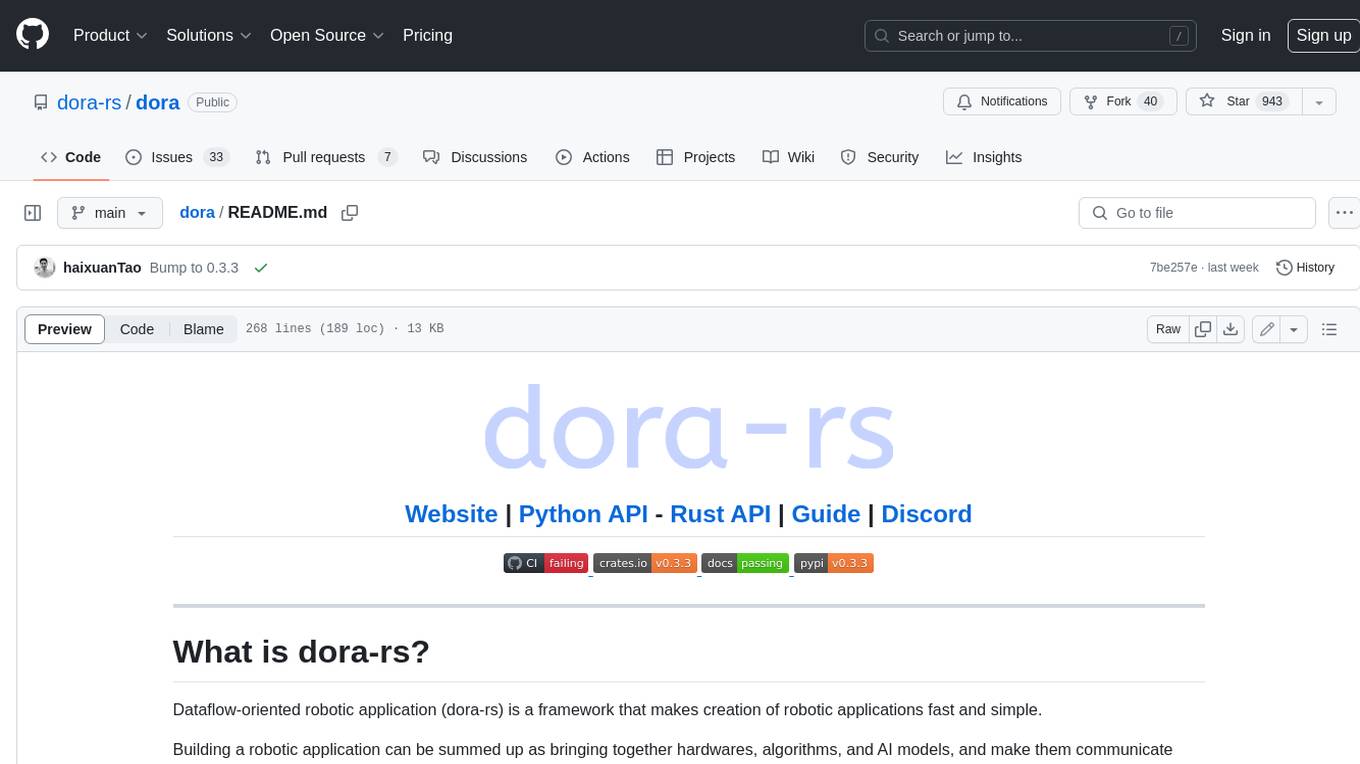
dora
Dataflow-oriented robotic application (dora-rs) is a framework that makes creation of robotic applications fast and simple. Building a robotic application can be summed up as bringing together hardwares, algorithms, and AI models, and make them communicate with each others. At dora-rs, we try to: make integration of hardware and software easy by supporting Python, C, C++, and also ROS2. make communication low latency by using zero-copy Arrow messages. dora-rs is still experimental and you might experience bugs, but we're working very hard to make it stable as possible.
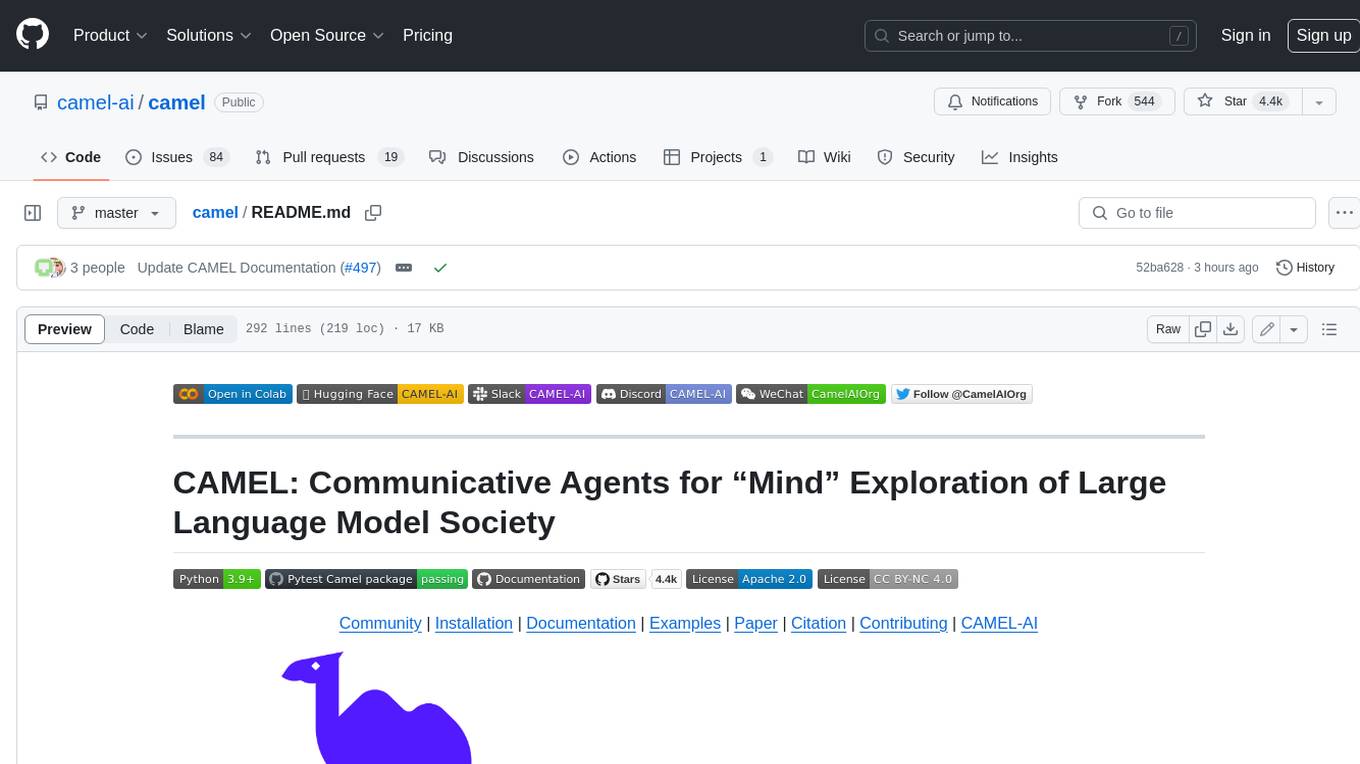
camel
CAMEL is an open-source library designed for the study of autonomous and communicative agents. We believe that studying these agents on a large scale offers valuable insights into their behaviors, capabilities, and potential risks. To facilitate research in this field, we implement and support various types of agents, tasks, prompts, models, and simulated environments.
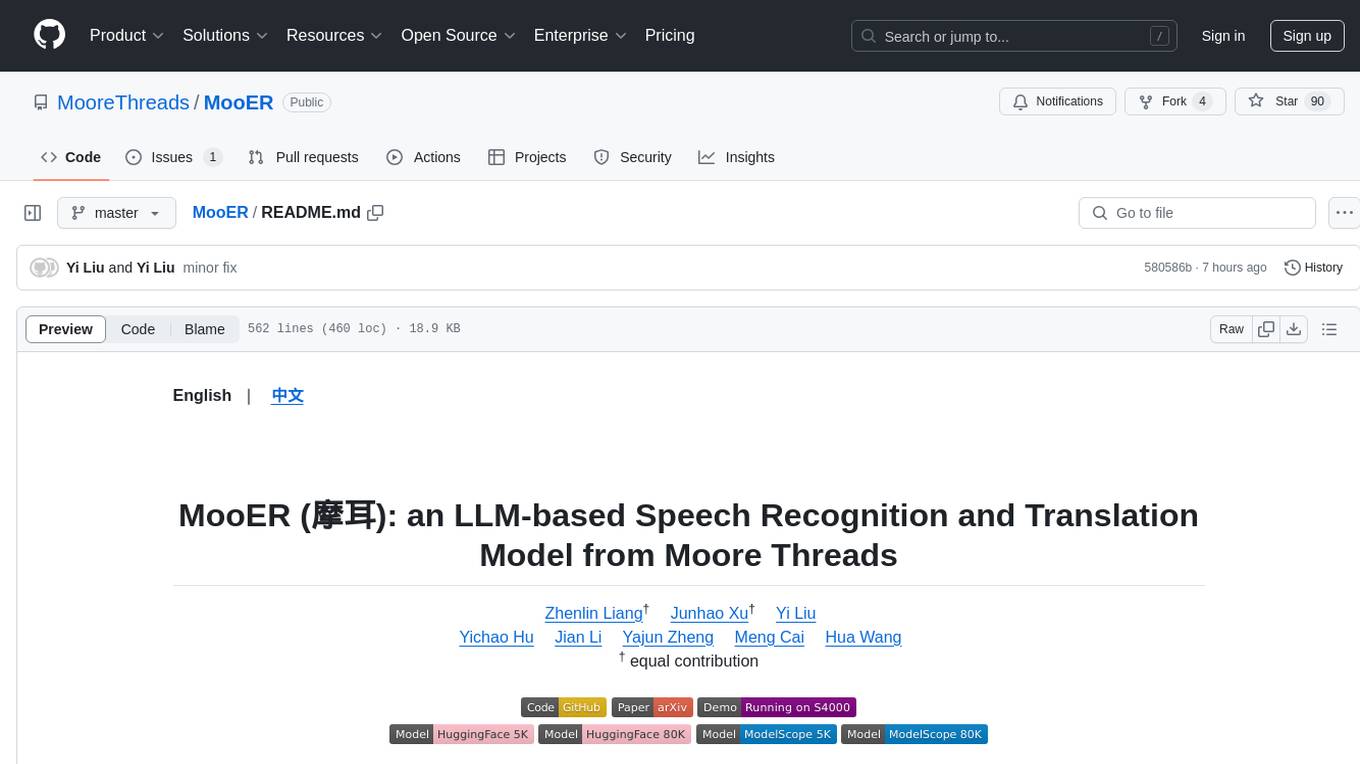
MooER
MooER (æŠčŗ) is an LLM-based speech recognition and translation model developed by Moore Threads. It allows users to transcribe speech into text (ASR) and translate speech into other languages (AST) in an end-to-end manner. The model was trained using 5K hours of data and is now also available with an 80K hours version. MooER is the first LLM-based speech model trained and inferred using domestic GPUs. The repository includes pretrained models, inference code, and a Gradio demo for a better user experience.
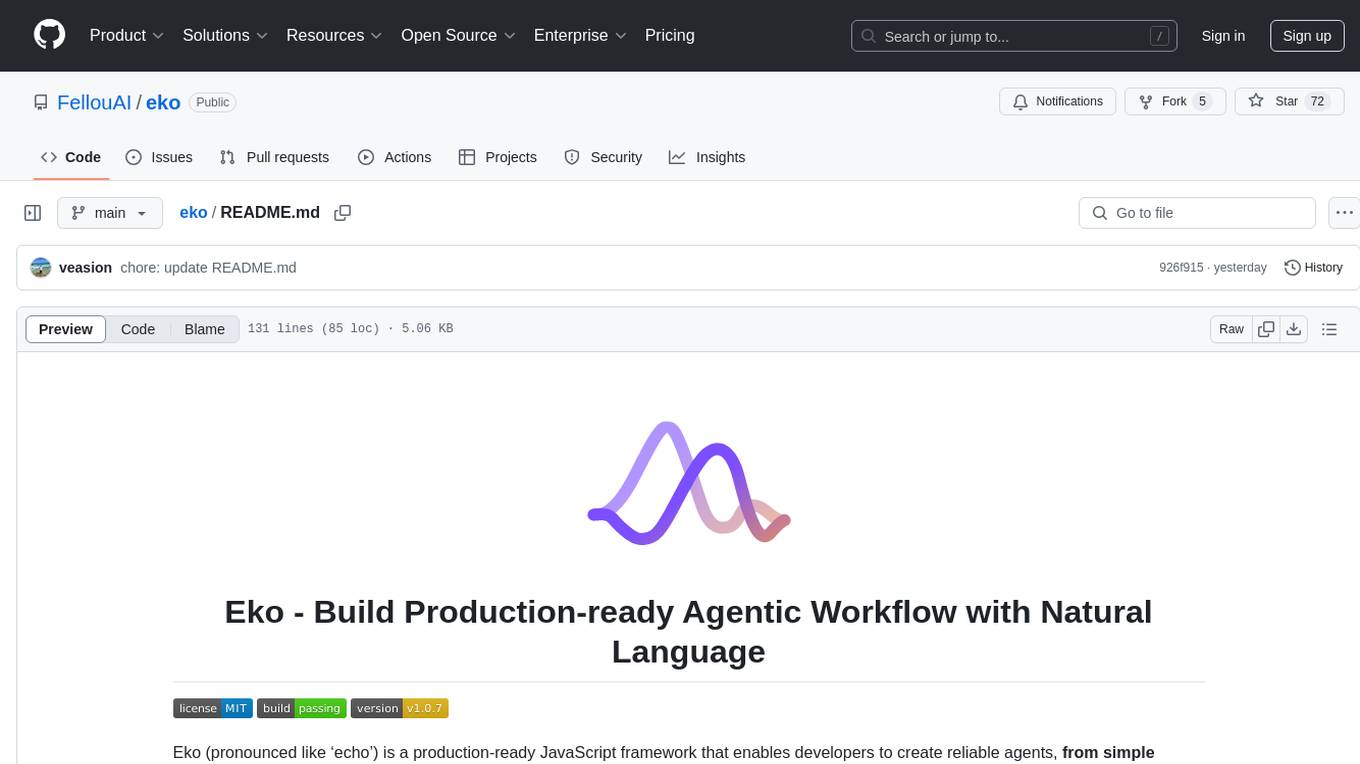
eko
Eko is a lightweight and flexible command-line tool for managing environment variables in your projects. It allows you to easily set, get, and delete environment variables for different environments, making it simple to manage configurations across development, staging, and production environments. With Eko, you can streamline your workflow and ensure consistency in your application settings without the need for complex setup or configuration files.
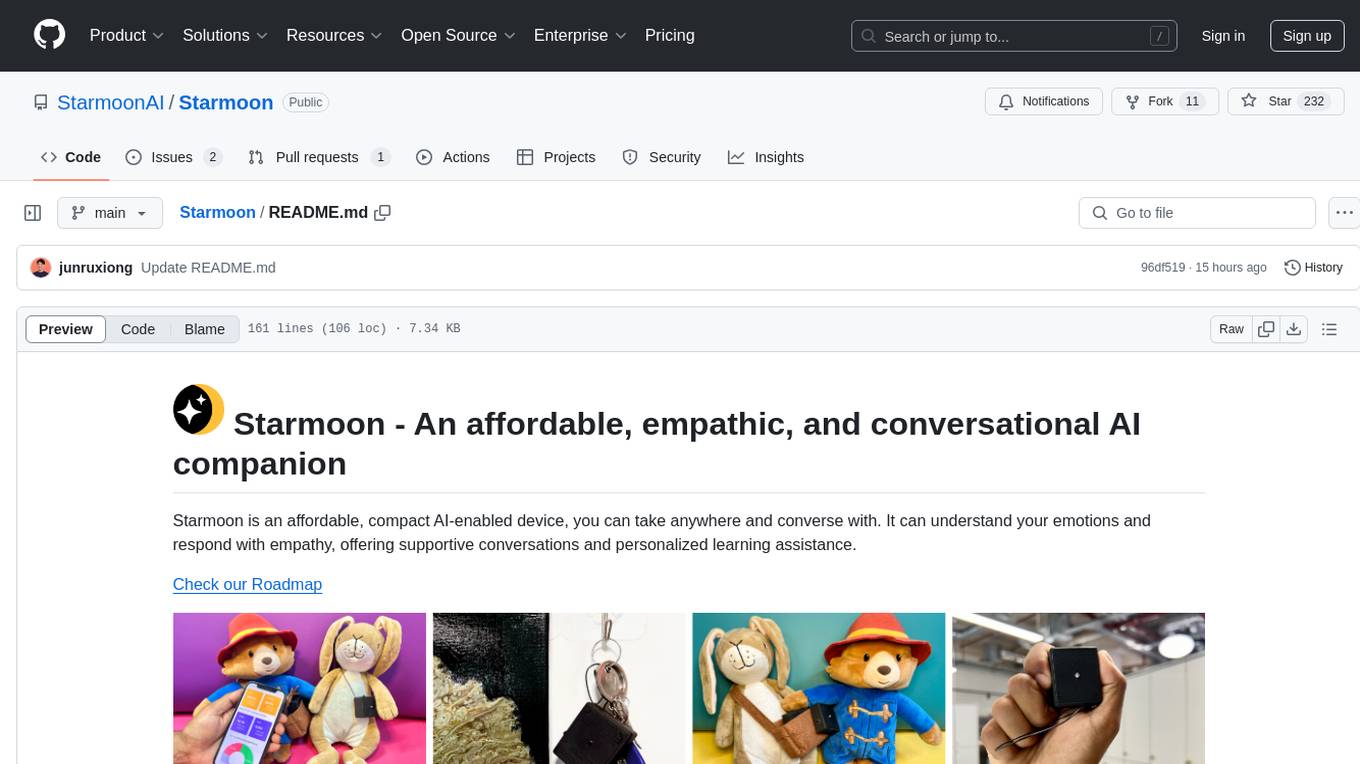
Starmoon
Starmoon is an affordable, compact AI-enabled device that can understand and respond to your emotions with empathy. It offers supportive conversations and personalized learning assistance. The device is cost-effective, voice-enabled, open-source, compact, and aims to reduce screen time. Users can assemble the device themselves using off-the-shelf components and deploy it locally for data privacy. Starmoon integrates various APIs for AI language models, speech-to-text, text-to-speech, and emotion intelligence. The hardware setup involves components like ESP32S3, microphone, amplifier, speaker, LED light, and button, along with software setup instructions for developers. The project also includes a web app, backend API, and background task dashboard for monitoring and management.
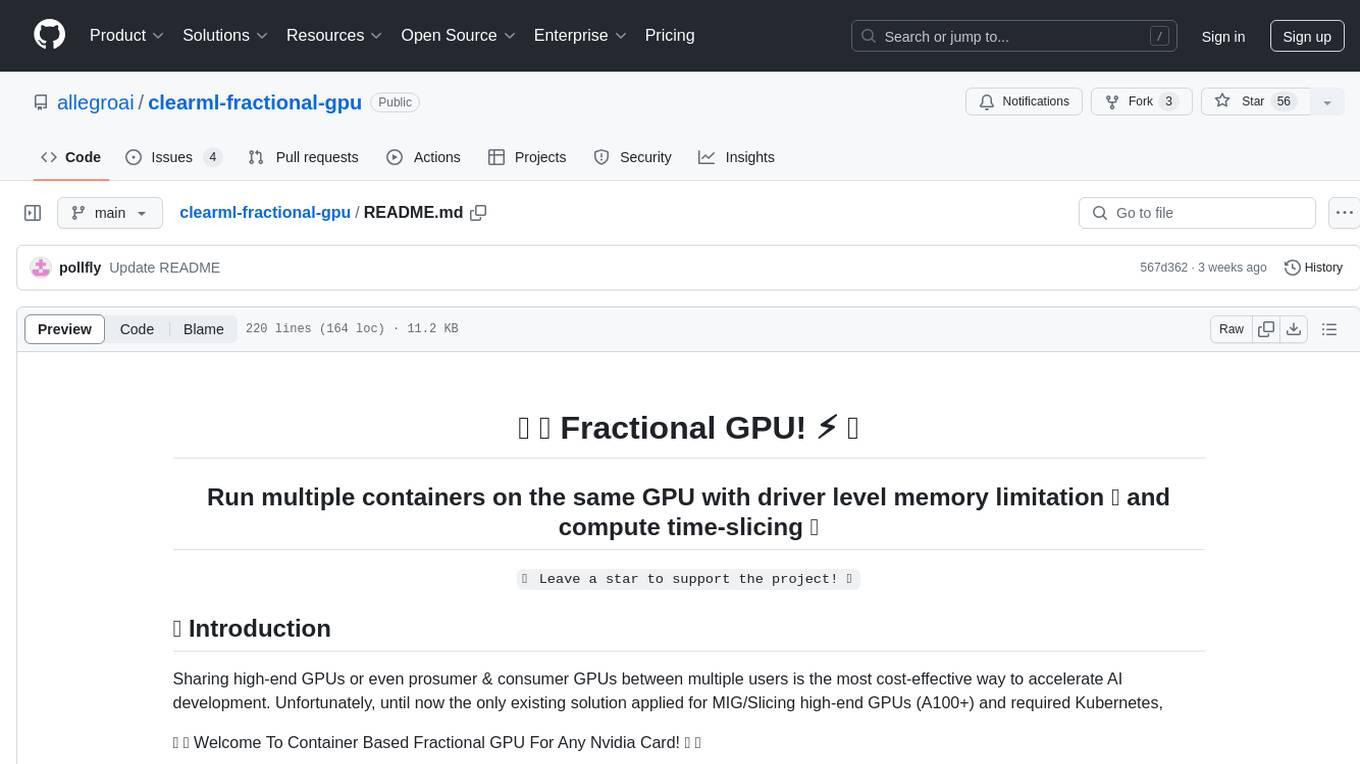
clearml-fractional-gpu
ClearML Fractional GPU is a tool designed to optimize GPU resource utilization by allowing multiple containers to run on the same GPU with driver-level memory limitation and compute time-slicing. It supports CUDA 11.x & CUDA 12.x, preventing greedy processes from grabbing the entire GPU memory. The tool offers options like Dynamic GPU Slicing, Container-based Memory Limits, and Kubernetes-based Static MIG Slicing to enhance hardware utilization and workload performance for AI development.
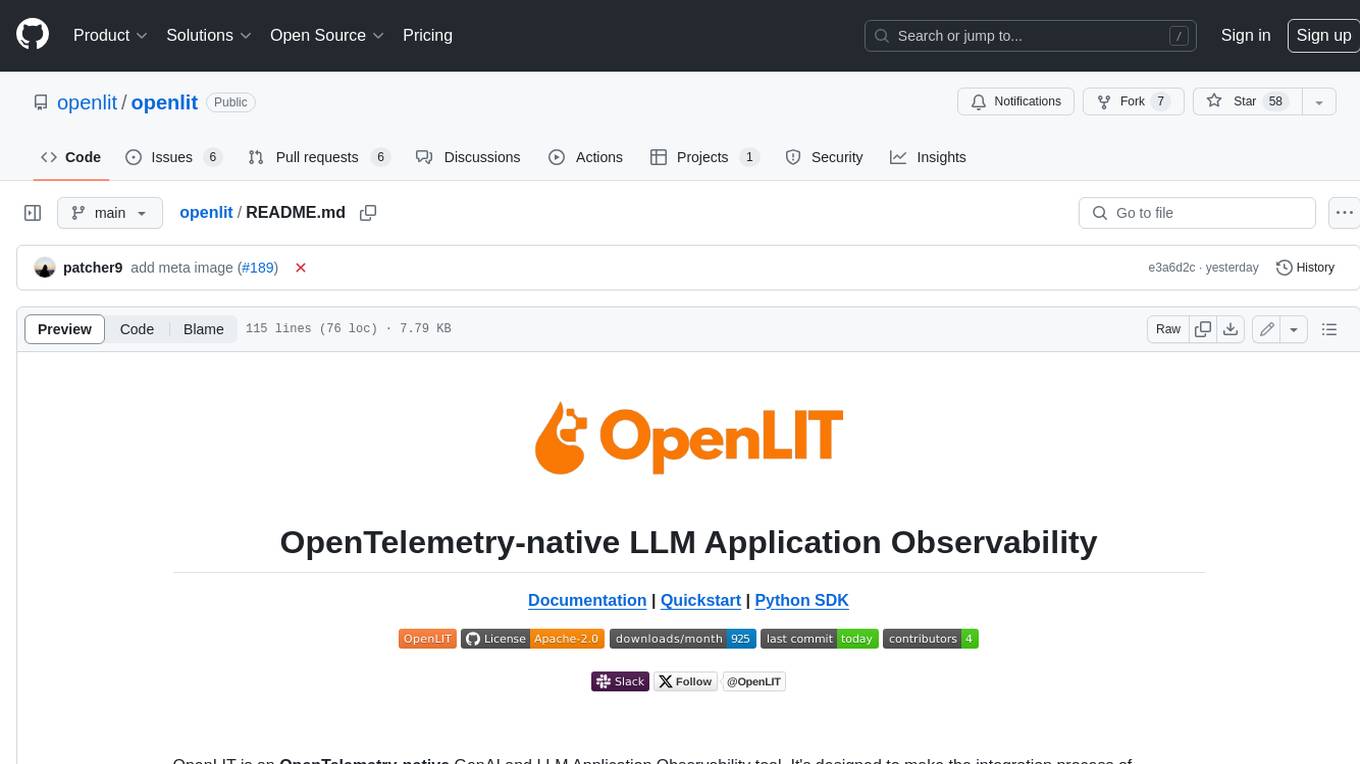
openlit
OpenLIT is an OpenTelemetry-native GenAI and LLM Application Observability tool. It's designed to make the integration process of observability into GenAI projects as easy as pie â literally, with just **a single line of code**. Whether you're working with popular LLM Libraries such as OpenAI and HuggingFace or leveraging vector databases like ChromaDB, OpenLIT ensures your applications are monitored seamlessly, providing critical insights to improve performance and reliability.
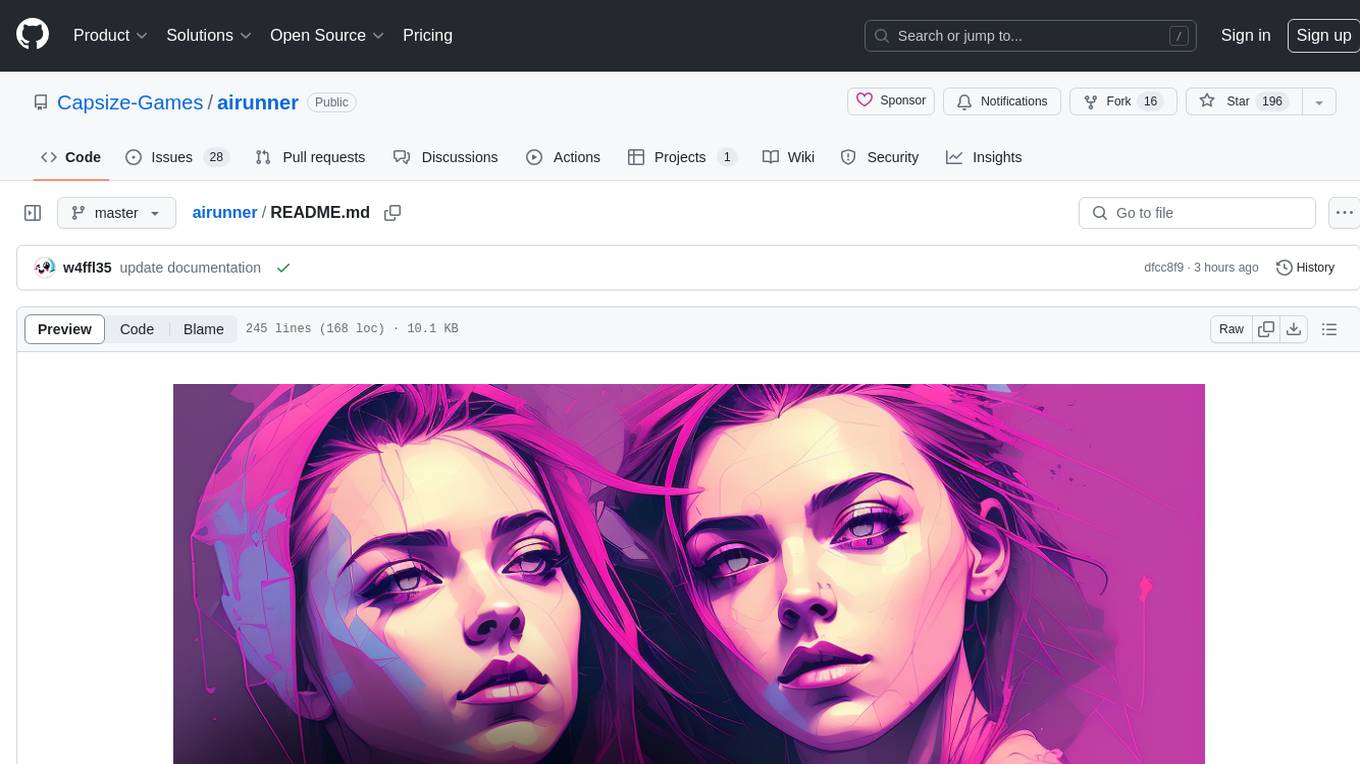
airunner
AI Runner is a multi-modal AI interface that allows users to run open-source large language models and AI image generators on their own hardware. The tool provides features such as voice-based chatbot conversations, text-to-speech, speech-to-text, vision-to-text, text generation with large language models, image generation capabilities, image manipulation tools, utility functions, and more. It aims to provide a stable and user-friendly experience with security updates, a new UI, and a streamlined installation process. The application is designed to run offline on users' hardware without relying on a web server, offering a smooth and responsive user experience.
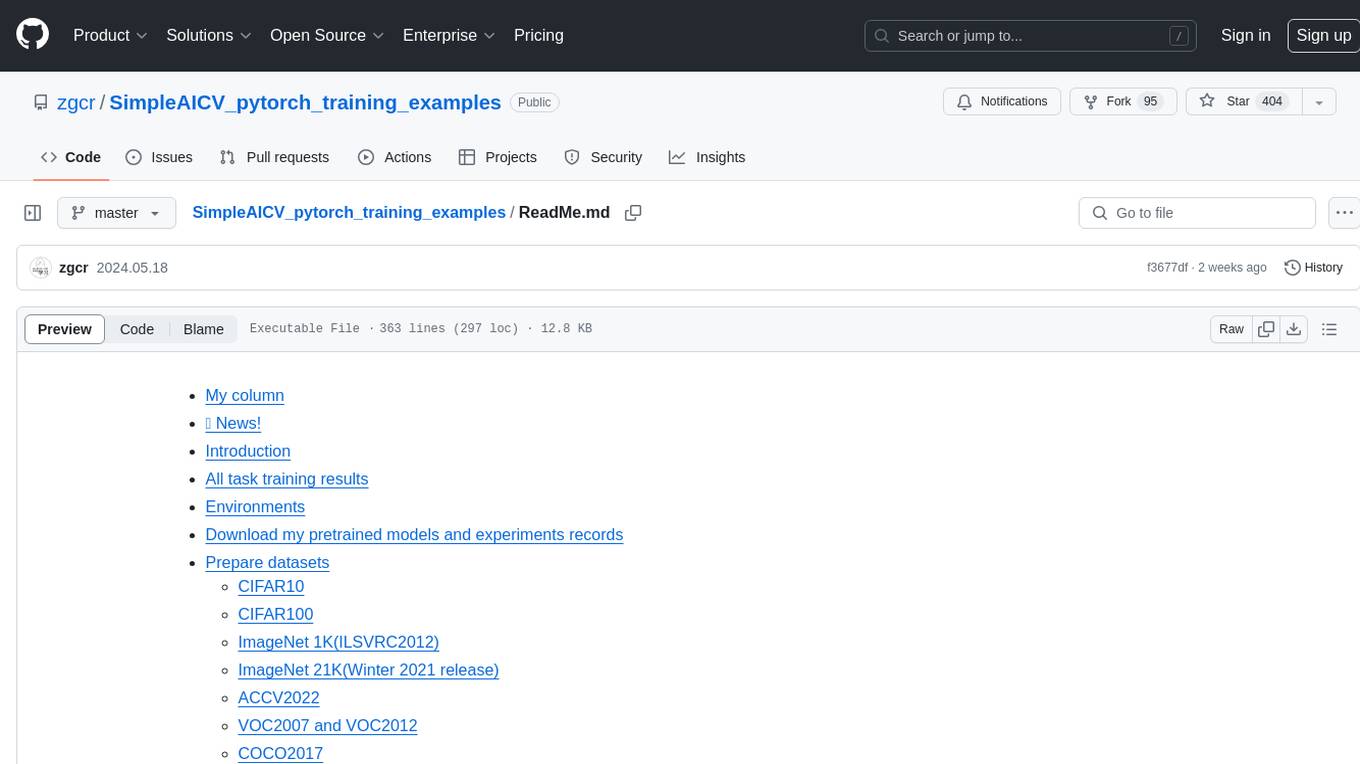
SimpleAICV_pytorch_training_examples
SimpleAICV_pytorch_training_examples is a repository that provides simple training and testing examples for various computer vision tasks such as image classification, object detection, semantic segmentation, instance segmentation, knowledge distillation, contrastive learning, masked image modeling, OCR text detection, OCR text recognition, human matting, salient object detection, interactive segmentation, image inpainting, and diffusion model tasks. The repository includes support for multiple datasets and networks, along with instructions on how to prepare datasets, train and test models, and use gradio demos. It also offers pretrained models and experiment records for download from huggingface or Baidu-Netdisk. The repository requires specific environments and package installations to run effectively.
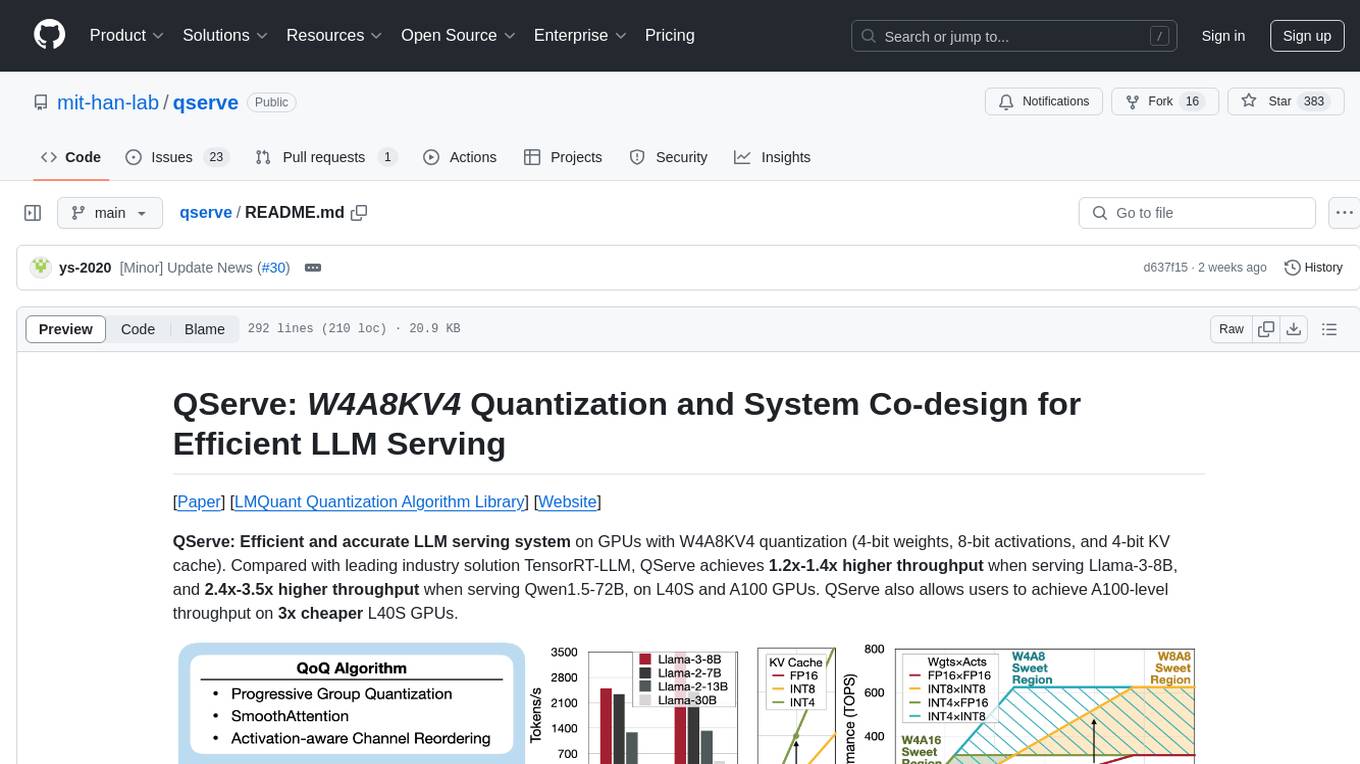
qserve
QServe is a serving system designed for efficient and accurate Large Language Models (LLM) on GPUs with W4A8KV4 quantization. It achieves higher throughput compared to leading industry solutions, allowing users to achieve A100-level throughput on cheaper L40S GPUs. The system introduces the QoQ quantization algorithm with 4-bit weight, 8-bit activation, and 4-bit KV cache, addressing runtime overhead challenges. QServe improves serving throughput for various LLM models by implementing compute-aware weight reordering, register-level parallelism, and fused attention memory-bound techniques.
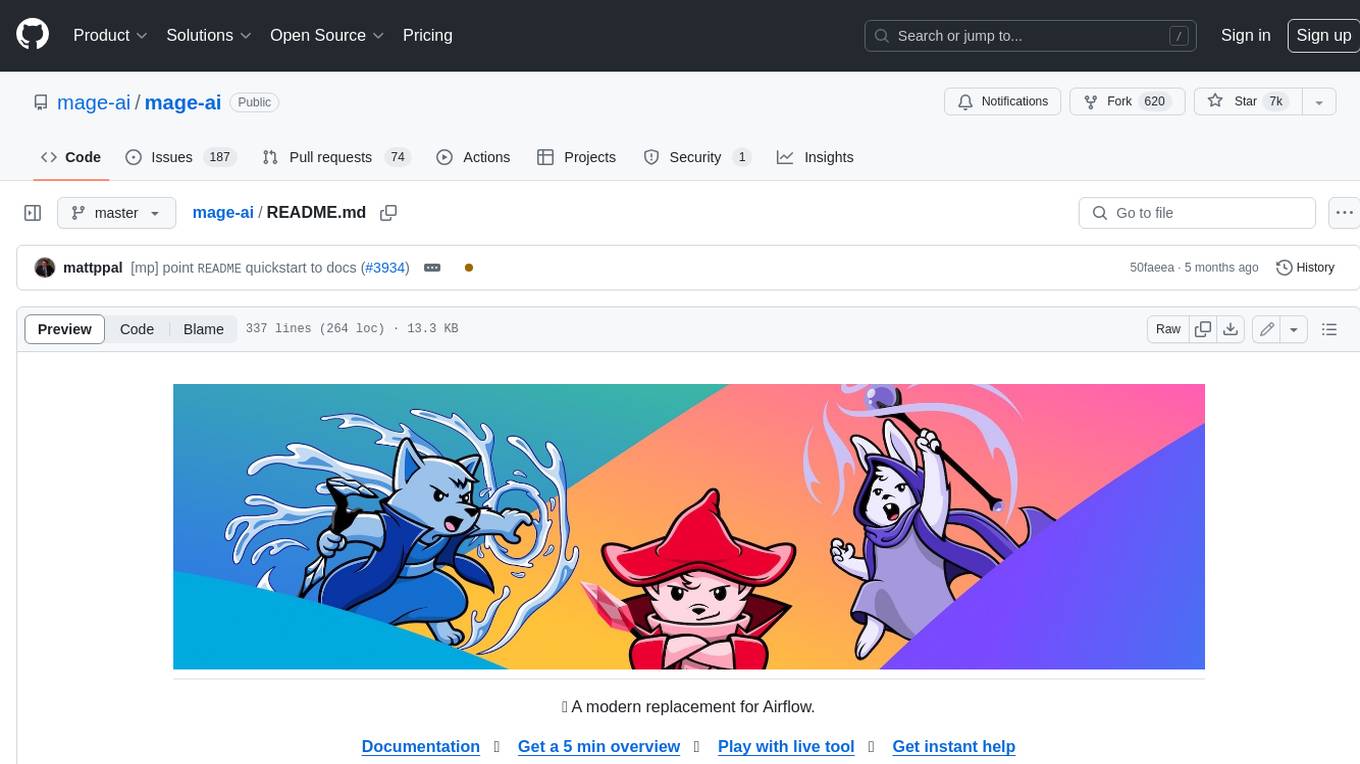
mage-ai
Mage is an open-source data pipeline tool for transforming and integrating data. It offers an easy developer experience, engineering best practices built-in, and data as a first-class citizen. Mage makes it easy to build, preview, and launch data pipelines, and provides observability and scaling capabilities. It supports data integrations, streaming pipelines, and dbt integration.
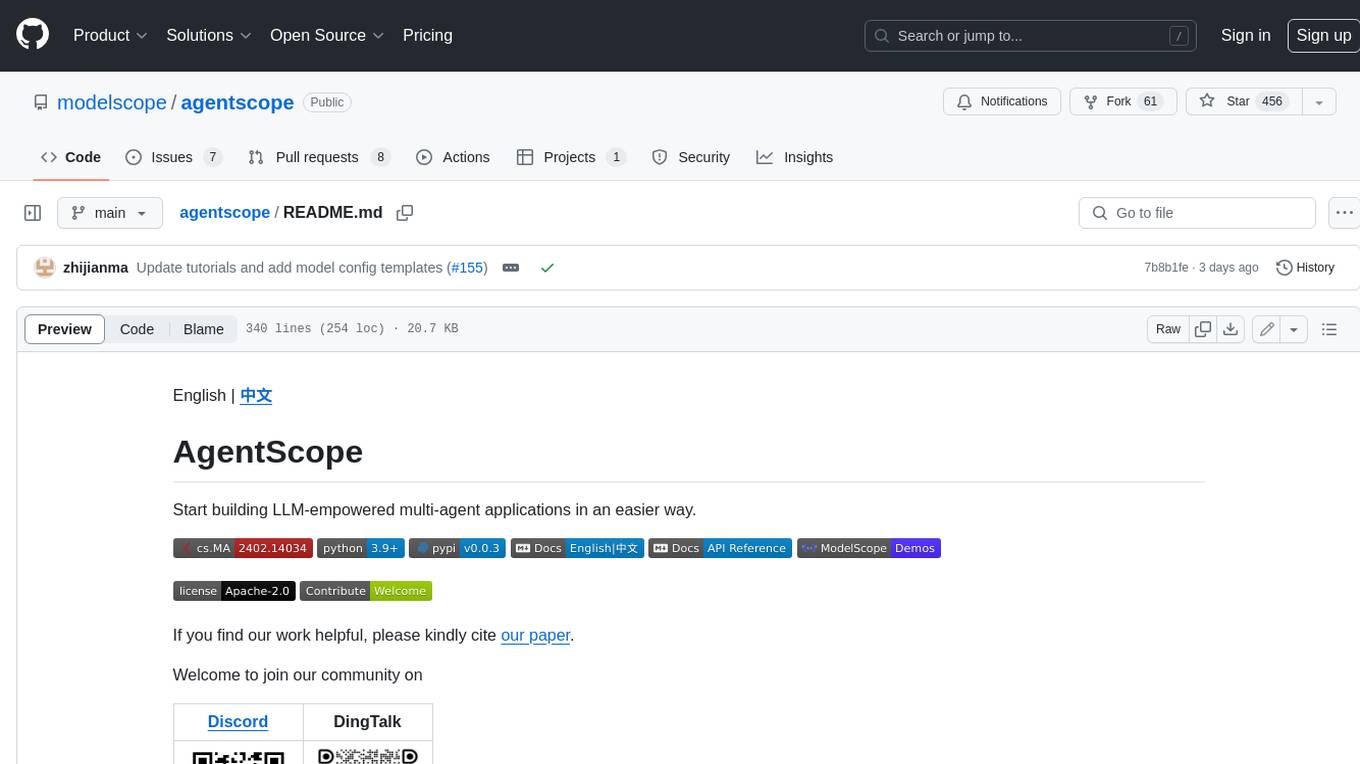
agentscope
AgentScope is a multi-agent platform designed to empower developers to build multi-agent applications with large-scale models. It features three high-level capabilities: Easy-to-Use, High Robustness, and Actor-Based Distribution. AgentScope provides a list of `ModelWrapper` to support both local model services and third-party model APIs, including OpenAI API, DashScope API, Gemini API, and ollama. It also enables developers to rapidly deploy local model services using libraries such as ollama (CPU inference), Flask + Transformers, Flask + ModelScope, FastChat, and vllm. AgentScope supports various services, including Web Search, Data Query, Retrieval, Code Execution, File Operation, and Text Processing. Example applications include Conversation, Game, and Distribution. AgentScope is released under Apache License 2.0 and welcomes contributions.
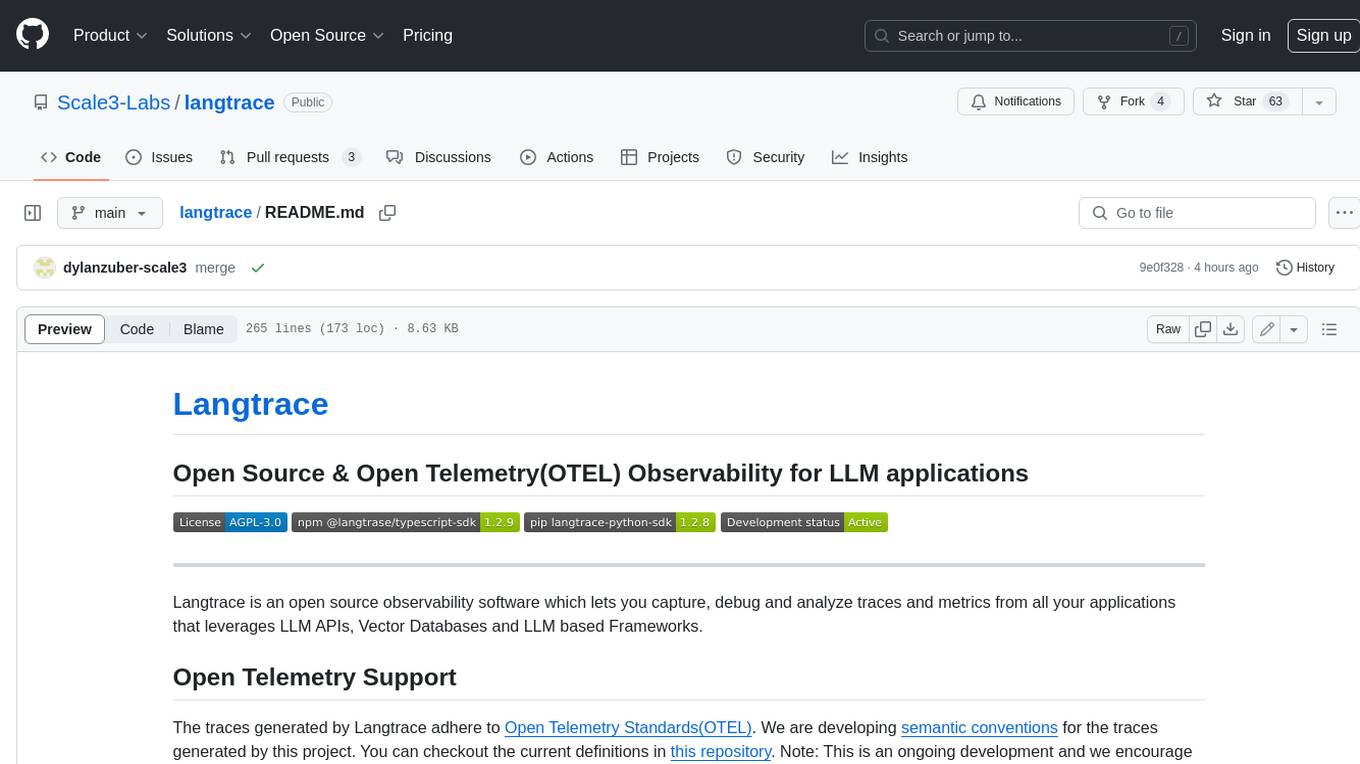
langtrace
Langtrace is an open source observability software that lets you capture, debug, and analyze traces and metrics from all your applications that leverage LLM APIs, Vector Databases, and LLM-based Frameworks. It supports Open Telemetry Standards (OTEL), and the traces generated adhere to these standards. Langtrace offers both a managed SaaS version (Langtrace Cloud) and a self-hosted option. The SDKs for both Typescript/Javascript and Python are available, making it easy to integrate Langtrace into your applications. Langtrace automatically captures traces from various vendors, including OpenAI, Anthropic, Azure OpenAI, Langchain, LlamaIndex, Pinecone, and ChromaDB.
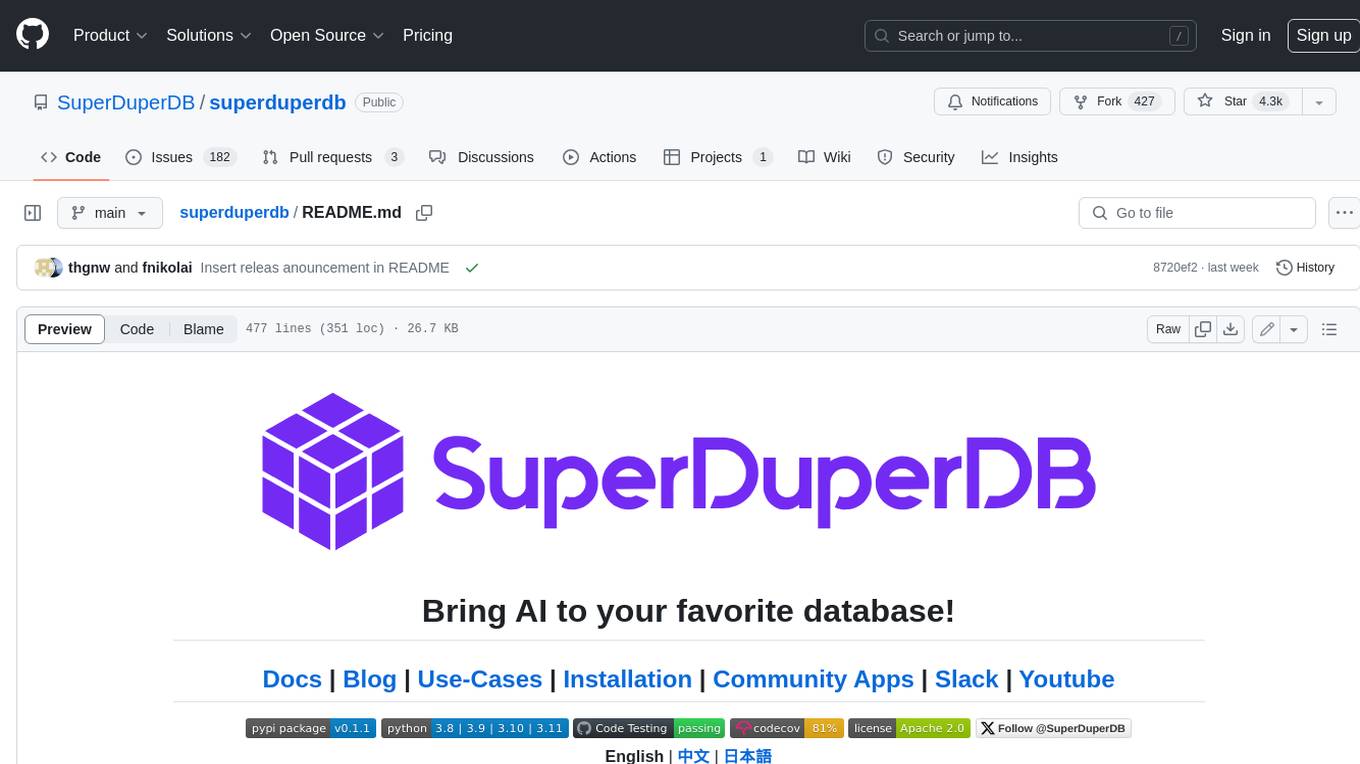
superduperdb
SuperDuperDB is a Python framework for integrating AI models, APIs, and vector search engines directly with your existing databases, including hosting of your own models, streaming inference and scalable model training/fine-tuning. Build, deploy and manage any AI application without the need for complex pipelines, infrastructure as well as specialized vector databases, and moving our data there, by integrating AI at your data's source: - Generative AI, LLMs, RAG, vector search - Standard machine learning use-cases (classification, segmentation, regression, forecasting recommendation etc.) - Custom AI use-cases involving specialized models - Even the most complex applications/workflows in which different models work together SuperDuperDB is **not** a database. Think `db = superduper(db)`: SuperDuperDB transforms your databases into an intelligent platform that allows you to leverage the full AI and Python ecosystem. A single development and deployment environment for all your AI applications in one place, fully scalable and easy to manage.
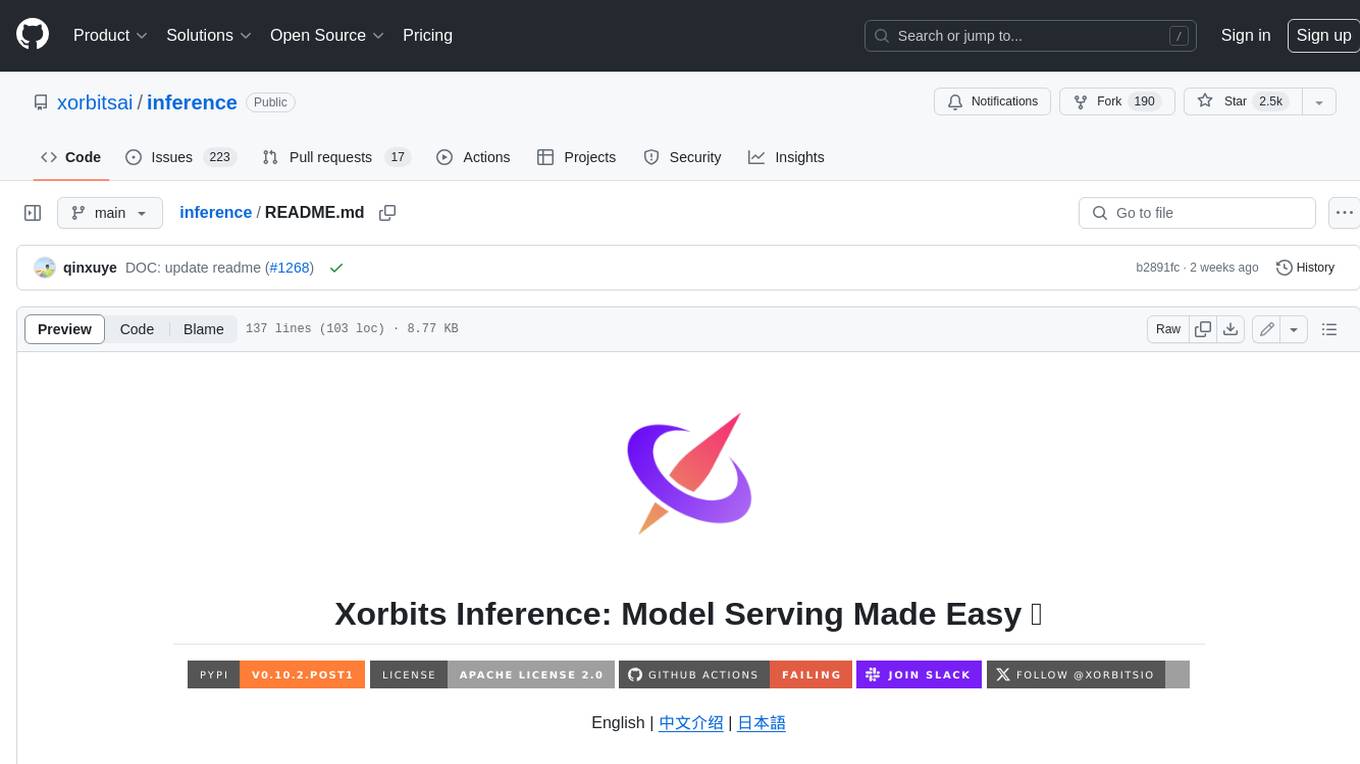
inference
Xorbits Inference (Xinference) is a powerful and versatile library designed to serve language, speech recognition, and multimodal models. With Xorbits Inference, you can effortlessly deploy and serve your or state-of-the-art built-in models using just a single command. Whether you are a researcher, developer, or data scientist, Xorbits Inference empowers you to unleash the full potential of cutting-edge AI models.
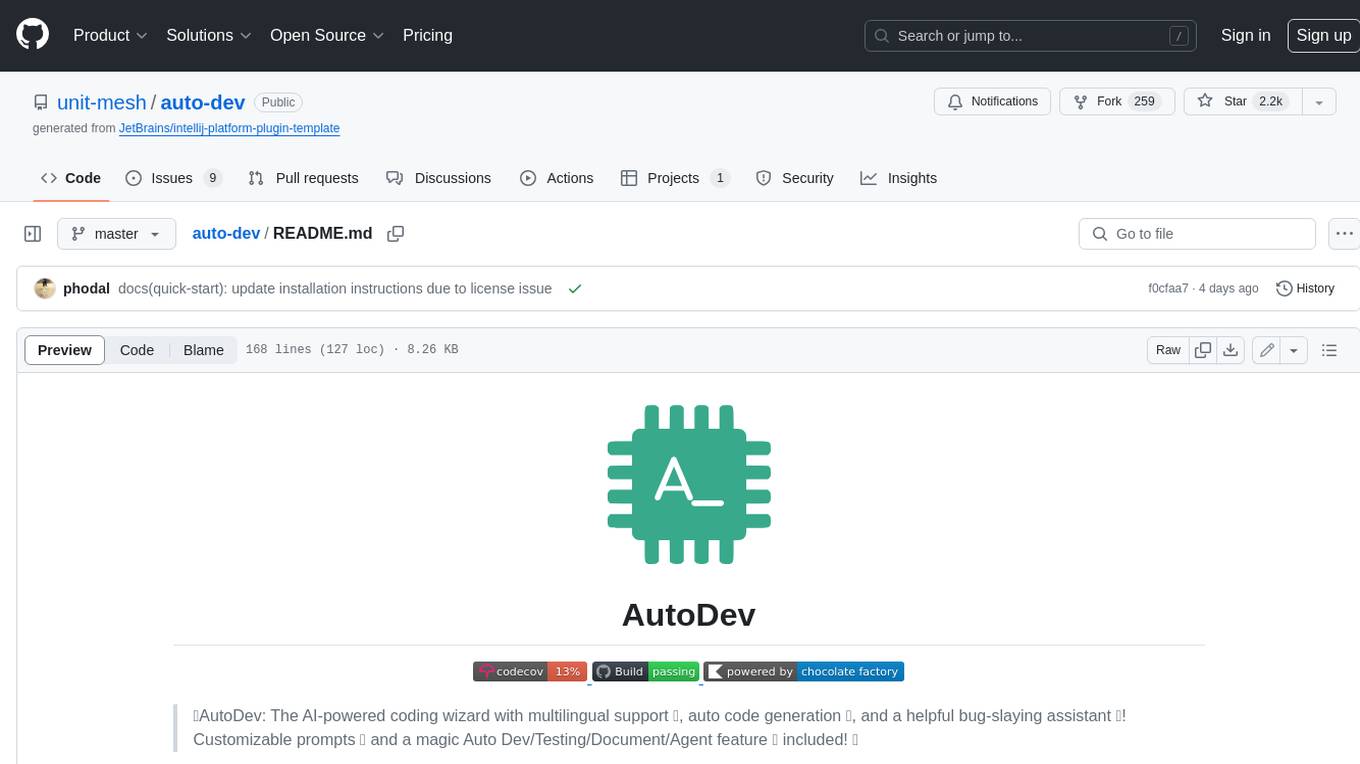
auto-dev
AutoDev is an AI-powered coding wizard that supports multiple languages, including Java, Kotlin, JavaScript/TypeScript, Rust, Python, Golang, C/C++/OC, and more. It offers a range of features, including auto development mode, copilot mode, chat with AI, customization options, SDLC support, custom AI agent integration, and language features such as language support, extensions, and a DevIns language for AI agent development. AutoDev is designed to assist developers with tasks such as auto code generation, bug detection, code explanation, exception tracing, commit message generation, code review content generation, smart refactoring, Dockerfile generation, CI/CD config file generation, and custom shell/command generation. It also provides a built-in LLM fine-tune model and supports UnitEval for LLM result evaluation and UnitGen for code-LLM fine-tune data generation.
For similar tasks
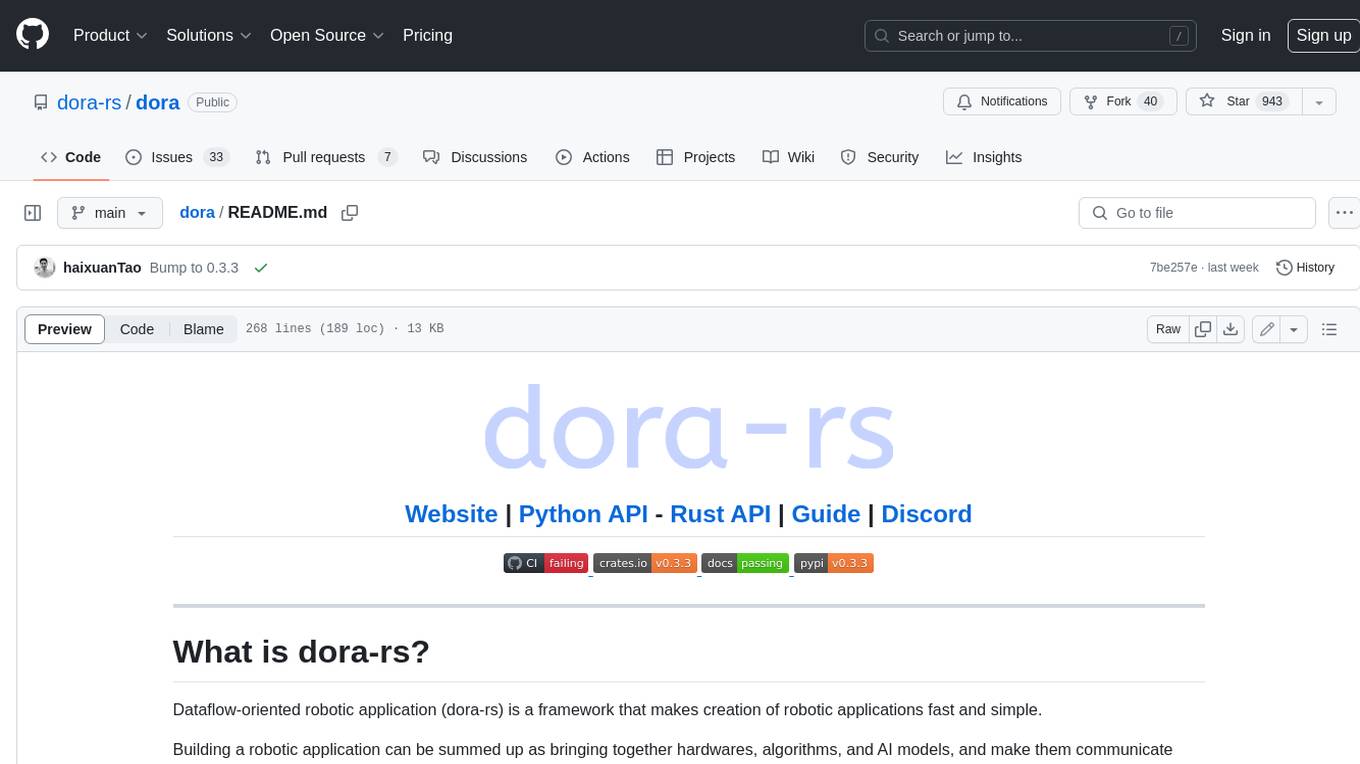
dora
Dataflow-oriented robotic application (dora-rs) is a framework that makes creation of robotic applications fast and simple. Building a robotic application can be summed up as bringing together hardwares, algorithms, and AI models, and make them communicate with each others. At dora-rs, we try to: make integration of hardware and software easy by supporting Python, C, C++, and also ROS2. make communication low latency by using zero-copy Arrow messages. dora-rs is still experimental and you might experience bugs, but we're working very hard to make it stable as possible.
For similar jobs
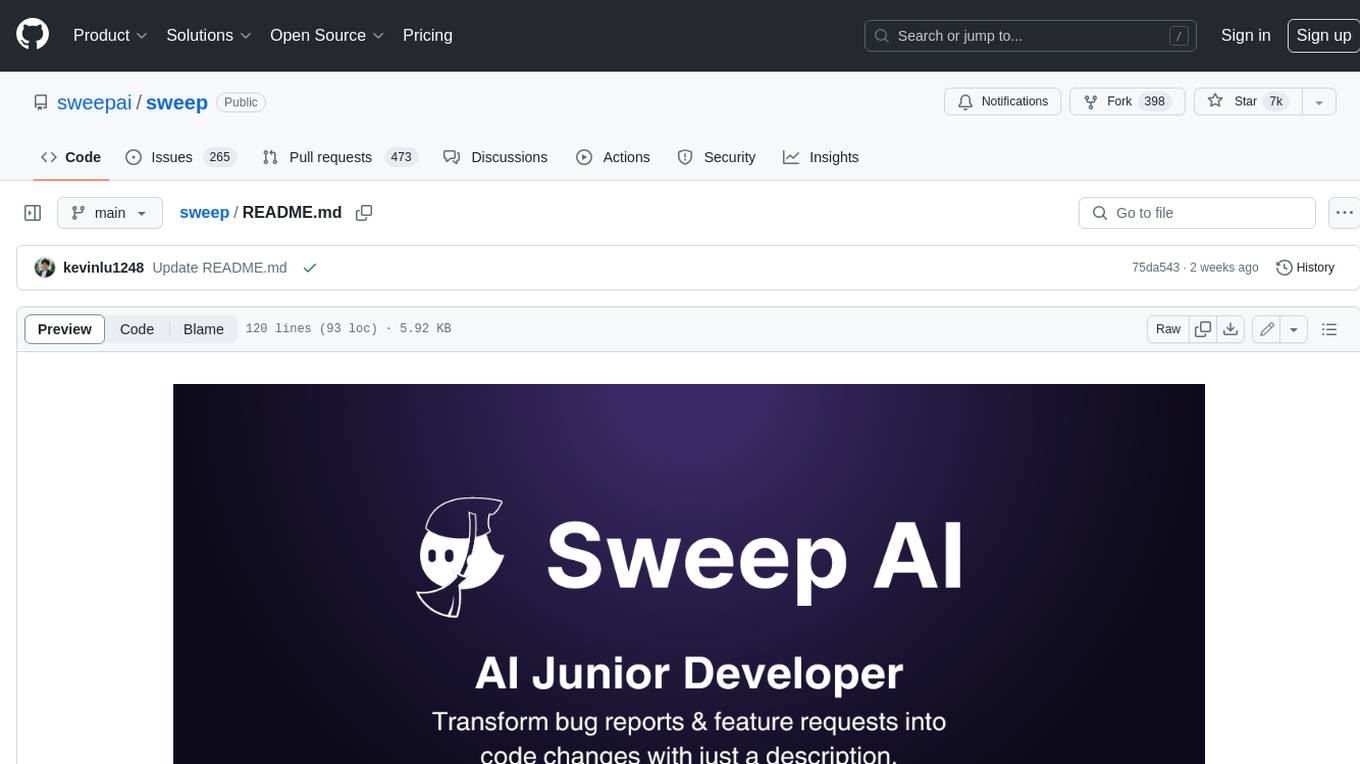
sweep
Sweep is an AI junior developer that turns bugs and feature requests into code changes. It automatically handles developer experience improvements like adding type hints and improving test coverage.
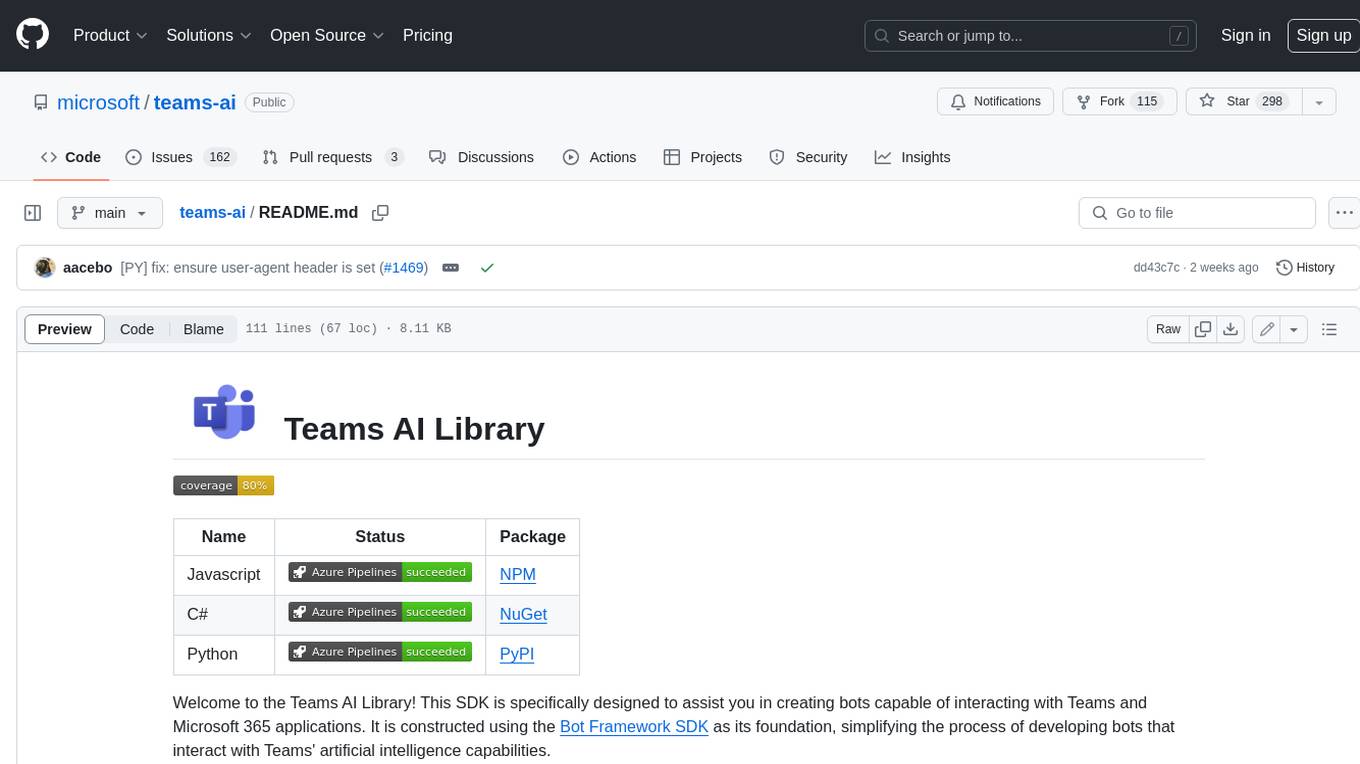
teams-ai
The Teams AI Library is a software development kit (SDK) that helps developers create bots that can interact with Teams and Microsoft 365 applications. It is built on top of the Bot Framework SDK and simplifies the process of developing bots that interact with Teams' artificial intelligence capabilities. The SDK is available for JavaScript/TypeScript, .NET, and Python.
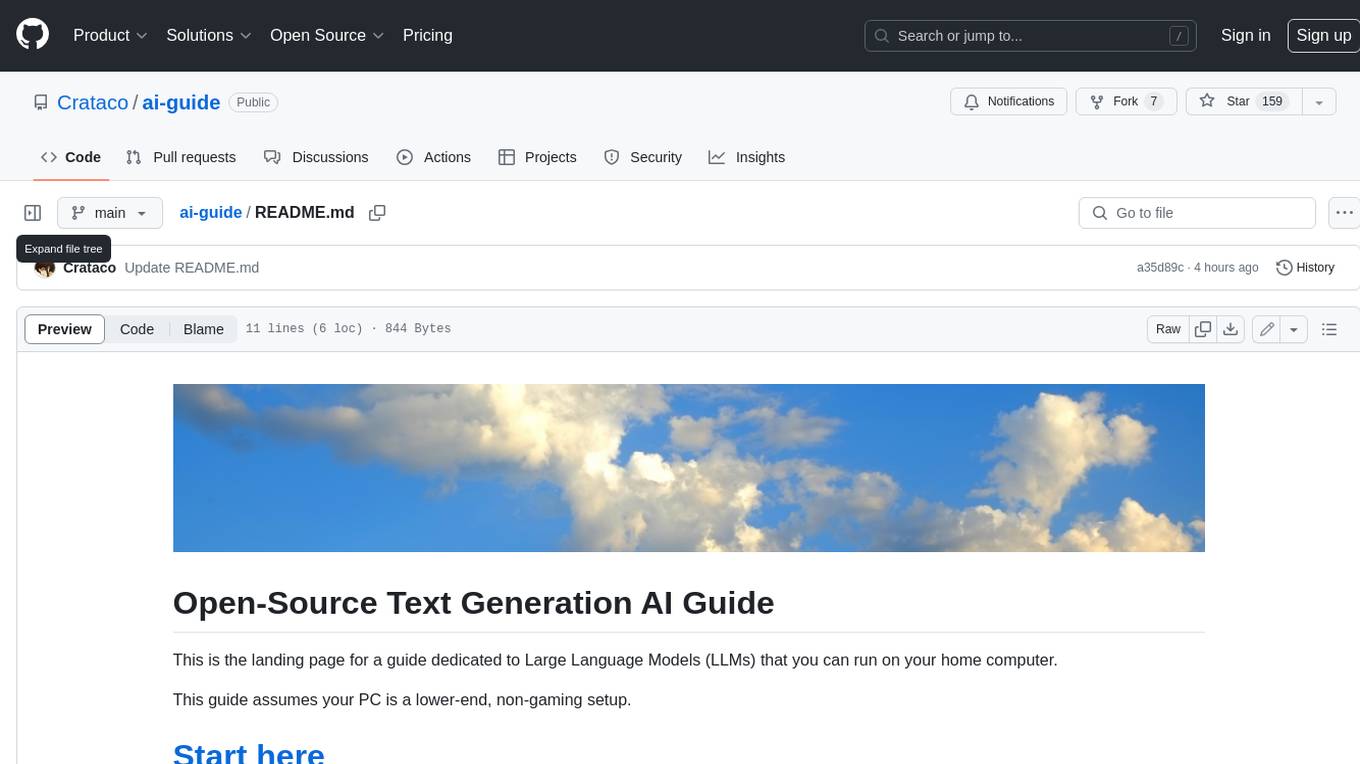
ai-guide
This guide is dedicated to Large Language Models (LLMs) that you can run on your home computer. It assumes your PC is a lower-end, non-gaming setup.
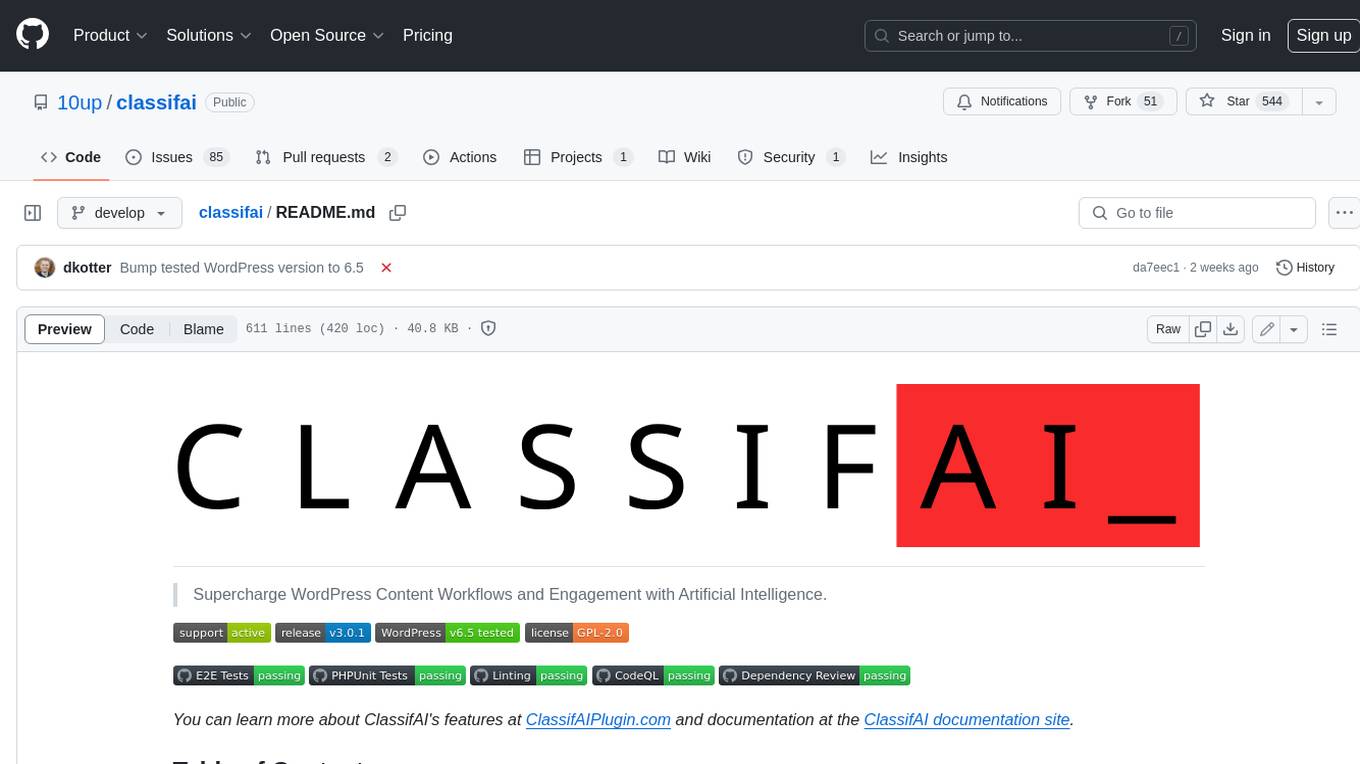
classifai
Supercharge WordPress Content Workflows and Engagement with Artificial Intelligence. Tap into leading cloud-based services like OpenAI, Microsoft Azure AI, Google Gemini and IBM Watson to augment your WordPress-powered websites. Publish content faster while improving SEO performance and increasing audience engagement. ClassifAI integrates Artificial Intelligence and Machine Learning technologies to lighten your workload and eliminate tedious tasks, giving you more time to create original content that matters.
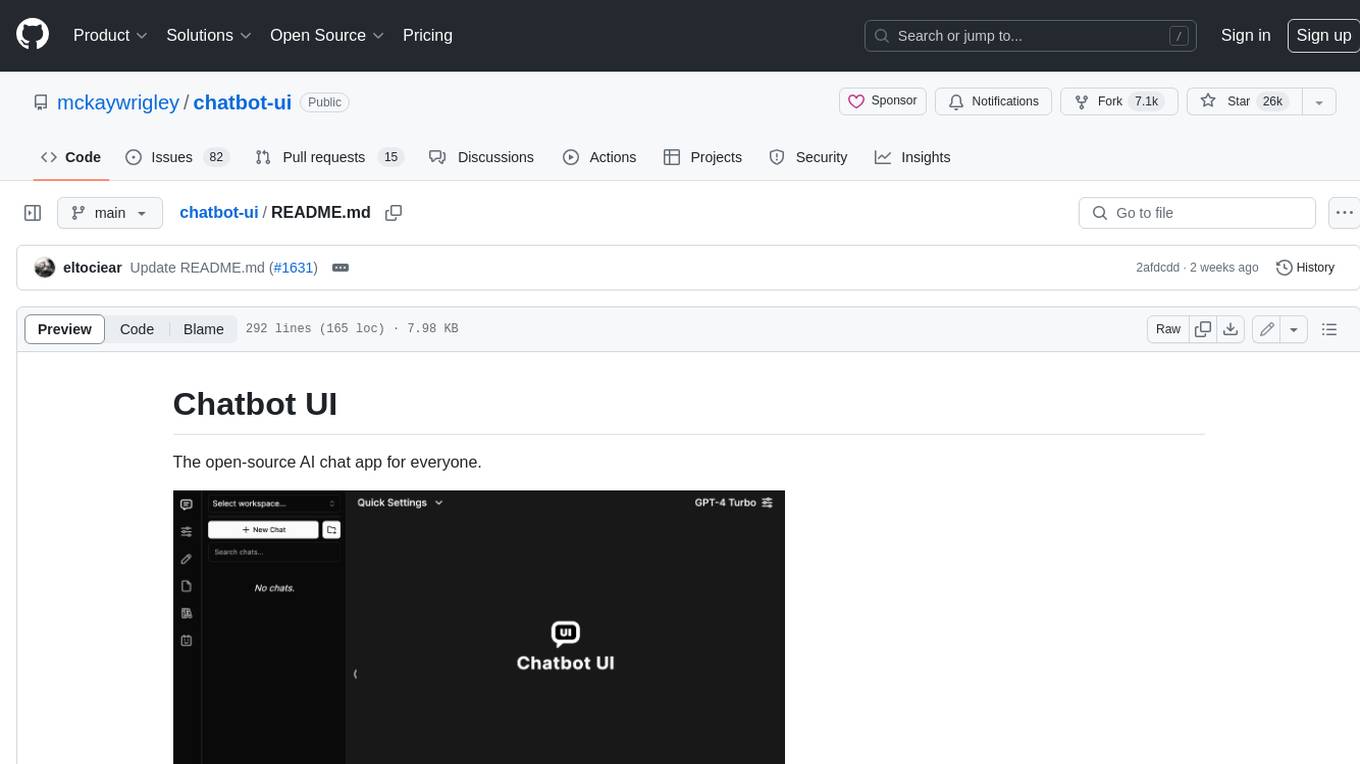
chatbot-ui
Chatbot UI is an open-source AI chat app that allows users to create and deploy their own AI chatbots. It is easy to use and can be customized to fit any need. Chatbot UI is perfect for businesses, developers, and anyone who wants to create a chatbot.
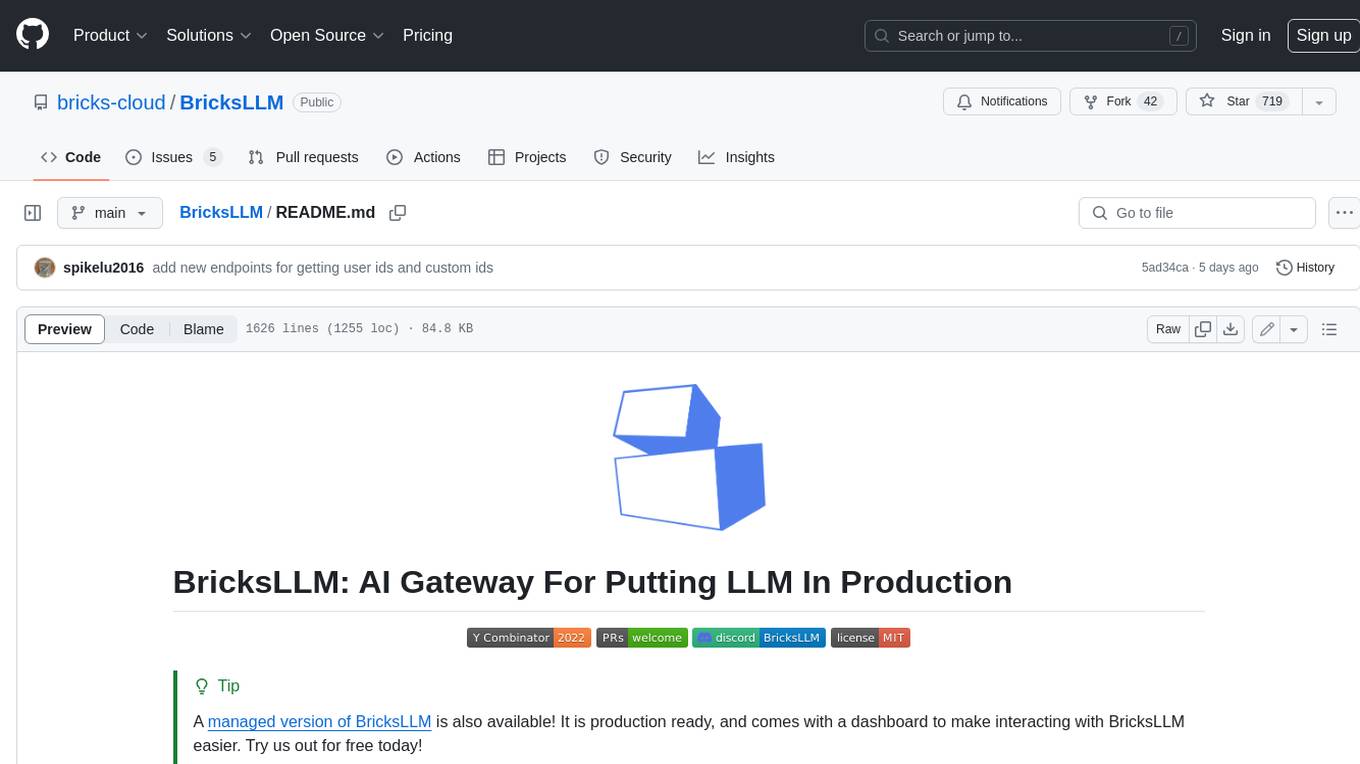
BricksLLM
BricksLLM is a cloud native AI gateway written in Go. Currently, it provides native support for OpenAI, Anthropic, Azure OpenAI and vLLM. BricksLLM aims to provide enterprise level infrastructure that can power any LLM production use cases. Here are some use cases for BricksLLM: * Set LLM usage limits for users on different pricing tiers * Track LLM usage on a per user and per organization basis * Block or redact requests containing PIIs * Improve LLM reliability with failovers, retries and caching * Distribute API keys with rate limits and cost limits for internal development/production use cases * Distribute API keys with rate limits and cost limits for students
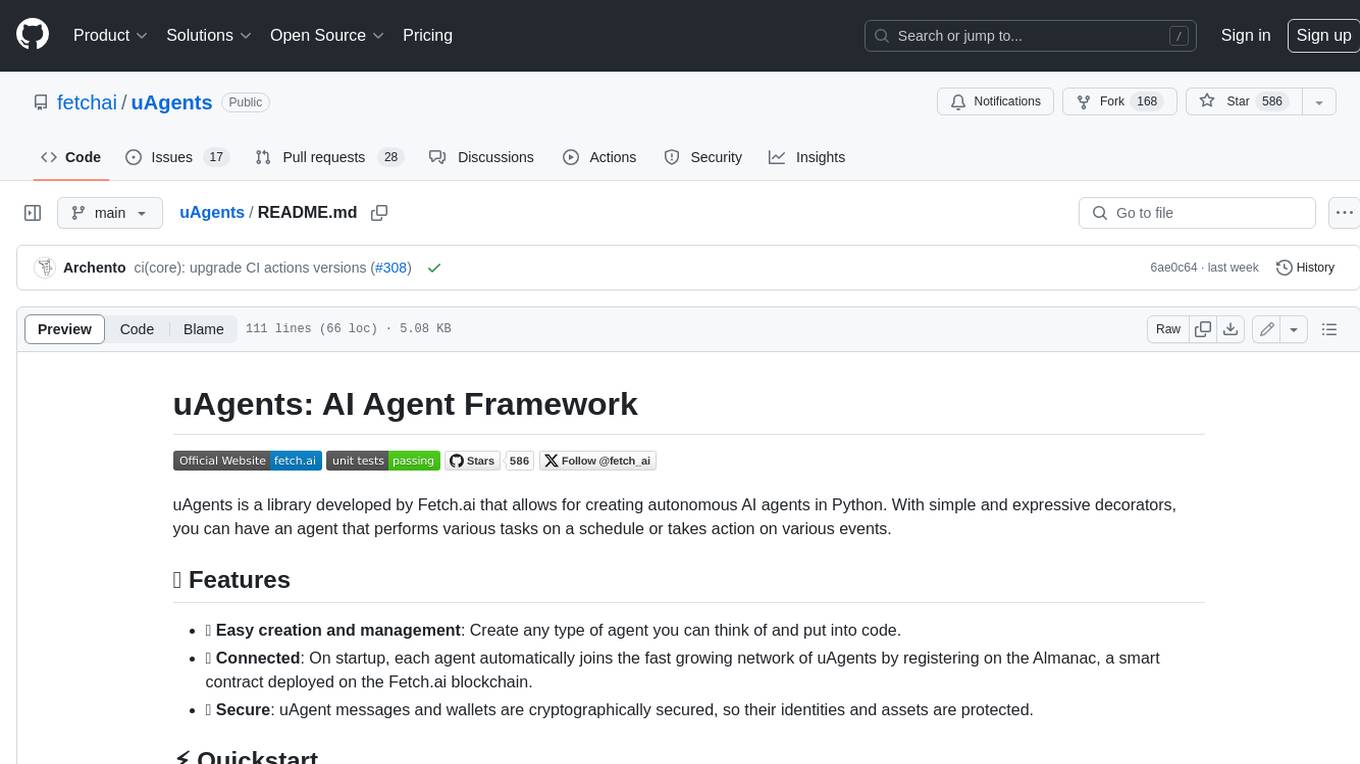
uAgents
uAgents is a Python library developed by Fetch.ai that allows for the creation of autonomous AI agents. These agents can perform various tasks on a schedule or take action on various events. uAgents are easy to create and manage, and they are connected to a fast-growing network of other uAgents. They are also secure, with cryptographically secured messages and wallets.
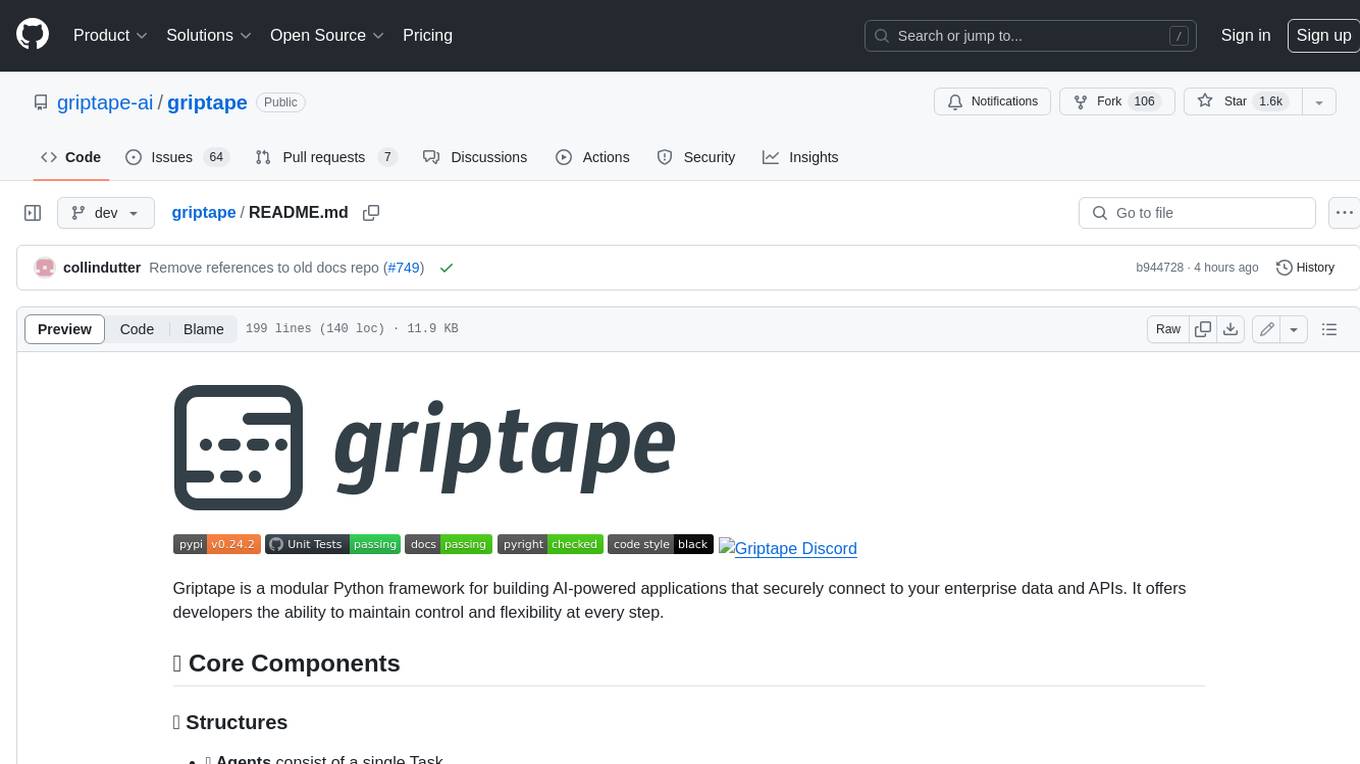
griptape
Griptape is a modular Python framework for building AI-powered applications that securely connect to your enterprise data and APIs. It offers developers the ability to maintain control and flexibility at every step. Griptape's core components include Structures (Agents, Pipelines, and Workflows), Tasks, Tools, Memory (Conversation Memory, Task Memory, and Meta Memory), Drivers (Prompt and Embedding Drivers, Vector Store Drivers, Image Generation Drivers, Image Query Drivers, SQL Drivers, Web Scraper Drivers, and Conversation Memory Drivers), Engines (Query Engines, Extraction Engines, Summary Engines, Image Generation Engines, and Image Query Engines), and additional components (Rulesets, Loaders, Artifacts, Chunkers, and Tokenizers). Griptape enables developers to create AI-powered applications with ease and efficiency.