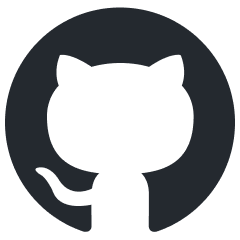
k8m
一款轻量级、跨平台的 Mini Kubernetes AI Dashboard,支持大模型+智能体+MCP(支持设置操作权限),集成多集群管理、智能分析、实时异常检测等功能,支持多架构并可单文件部署,助力高效集群管理与运维优化。
Stars: 157
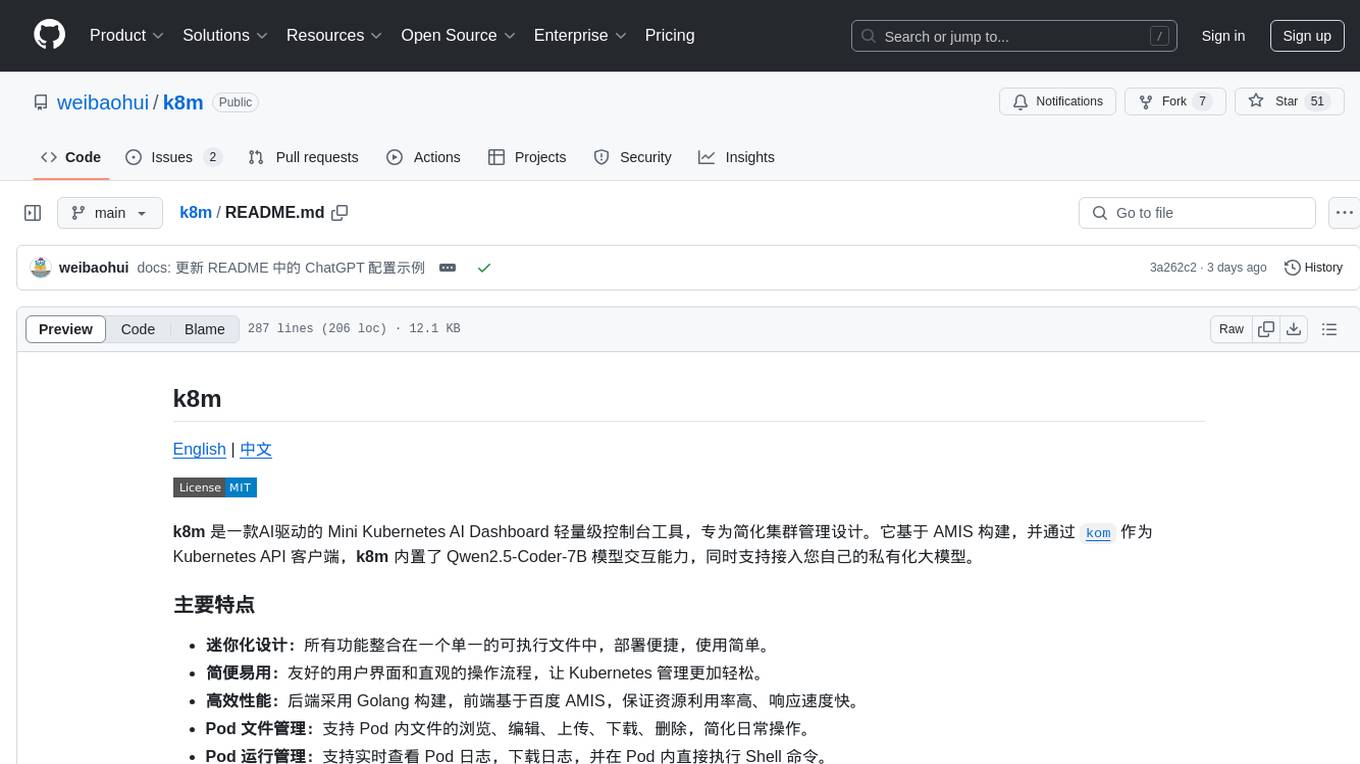
k8m is an AI-driven Mini Kubernetes AI Dashboard lightweight console tool designed to simplify cluster management. It is built on AMIS and uses 'kom' as the Kubernetes API client. k8m has built-in Qwen2.5-Coder-7B model interaction capabilities and supports integration with your own private large models. Its key features include miniaturized design for easy deployment, user-friendly interface for intuitive operation, efficient performance with backend in Golang and frontend based on Baidu AMIS, pod file management for browsing, editing, uploading, downloading, and deleting files, pod runtime management for real-time log viewing, log downloading, and executing shell commands within pods, CRD management for automatic discovery and management of CRD resources, and intelligent translation and diagnosis based on ChatGPT for YAML property translation, Describe information interpretation, AI log diagnosis, and command recommendations, providing intelligent support for managing k8s. It is cross-platform compatible with Linux, macOS, and Windows, supporting multiple architectures like x86 and ARM for seamless operation. k8m's design philosophy is 'AI-driven, lightweight and efficient, simplifying complexity,' helping developers and operators quickly get started and easily manage Kubernetes clusters.
README:
k8m 是一款AI驱动的 Mini Kubernetes AI Dashboard 轻量级控制台工具,专为简化集群管理设计。它基于 AMIS 构建,并通过
kom
作为 Kubernetes API 客户端,k8m 内置了
Qwen2.5-Coder-7B,支持deepseek-ai/DeepSeek-R1-Distill-Qwen-7B模型
模型交互能力,同时支持接入您自己的私有化大模型。
DEMO 用户名密码 demo/demo
- 迷你化设计:所有功能整合在一个单一的可执行文件中,部署便捷,使用简单。
- 简便易用:友好的用户界面和直观的操作流程,让 Kubernetes 管理更加轻松。
- 高效性能:后端采用 Golang 构建,前端基于百度 AMIS,保证资源利用率高、响应速度快。
-
AI驱动融合:基于ChatGPT实现划词解释、资源指南、YAML属性自动翻译、Describe信息解读、日志AI问诊、运行命令推荐,并集成了
k8s-gpt
功能,实现中文展现,为管理k8s提供智能化支持。 - MCP集成:可视化管理MCP,实现大模型调用Tools,内置k8s多集群MCP工具49种,可组合实现超百种集群操作,可作为MCP Server 供其他大模型软件使用。轻松实现大模型管理k8s。支持mcp.so主流服务。
- MCP权限打通:多集群管理权限与MCP大模型调用权限打通,一句话概述:谁使用大模型,就用谁的权限执行MCP。安全使用,无后顾之忧,避免操作越权。
- 多集群管理:自动识别集群内部使用InCluster模式,配置kubeconfig路径后自动扫描同级目录下的配置文件,同时注册管理多个集群。
- Pod 文件管理:支持 Pod 内文件的浏览、编辑、上传、下载、删除,简化日常操作。
- Pod 运行管理:支持实时查看 Pod 日志,下载日志,并在 Pod 内直接执行 Shell 命令。
- CRD 管理:可自动发现并管理 CRD 资源,提高工作效率。
- Helm 市场:支持Helm自由添加仓库,一键安装、卸载、升级 Helm 应用。
- 跨平台支持:兼容 Linux、macOS 和 Windows,并支持 x86、ARM 等多种架构,确保多平台无缝运行。
- 完全开源:开放所有源码,无任何限制,可自由定制和扩展,可商业使用。
k8m 的设计理念是“AI驱动,轻便高效,化繁为简”,它帮助开发者和运维人员快速上手,轻松管理 Kubernetes 集群。
- 下载:从 GitHub 下载最新版本。
-
运行:使用
./k8m
命令启动,访问http://127.0.0.1:3618。 - 参数:
Usage of ./k8m:
--admin-password string 管理员密码 (default "123456")
--admin-username string 管理员用户名 (default "admin")
--any-select 是否开启任意选择划词解释,默认开启 (default true)
--print-config 是否打印配置信息 (default false)
-k, --chatgpt-key string 大模型的自定义API Key (default "sk-xxxxxxx")
-m, --chatgpt-model string 大模型的自定义模型名称 (default "Qwen/Qwen2.5-7B-Instruct")
-u, --chatgpt-url string 大模型的自定义API URL (default "https://api.siliconflow.cn/v1")
-d, --debug 调试模式
--in-cluster 是否自动注册纳管宿主集群,默认启用
--jwt-token-secret string 登录后生成JWT token 使用的Secret (default "your-secret-key")
-c, --kubeconfig string kubeconfig文件路径 (default "/root/.kube/config")
--kubectl-shell-image string Kubectl Shell 镜像。默认为 bitnami/kubectl:latest,必须包含kubectl命令 (default "bitnami/kubectl:latest")
--log-v int klog的日志级别klog.V(2) (default 2)
--login-type string 登录方式,password, oauth, token等,default is password (default "password")
--node-shell-image string NodeShell 镜像。 默认为 alpine:latest,必须包含`nsenter`命令 (default "alpine:latest")
-p, --port int 监听端口 (default 3618)
--sqlite-path string sqlite数据库文件路径, (default "./data/k8m.db")
-s, --mcp-server-port int MCP Server 监听端口,默认3619 (default 3619)
-v, --v Level klog的日志级别 (default 2)
从v0.0.8版本开始,将内置GPT,无需配置。 如果您需要使用自己的GPT,请参考以下步骤。
需要设置环境变量,以启用ChatGPT。
export OPENAI_API_KEY="sk-XXXXX"
export OPENAI_API_URL="https://api.siliconflow.cn/v1"
export OPENAI_MODEL="Qwen/Qwen2.5-7B-Instruct"
如果设置参数后,依然没有效果,请尝试使用./k8m -v 6
获取更多的调试信息。
会输出以下信息,通过查看日志,确认是否启用ChatGPT。
ChatGPT 开启状态:true
ChatGPT 启用 key:sk-hl**********************************************, url:https: // api.siliconflow.cn/v1
ChatGPT 使用环境变量中设置的模型:Qwen/Qwen2.5-7B-Instruc
本项目集成了github.com/sashabaranov/go-openaiSDK。 国内访问推荐使用硅基流动的服务。 登录后,在https://cloud.siliconflow.cn/account/ak创建API_KEY
以下是k8m支持的环境变量设置参数及其作用的表格:
环境变量 | 默认值 | 说明 |
---|---|---|
PORT |
3618 |
监听的端口号 |
MCP_SERVER_PORT |
3619 |
内置多集群k8s MCP Server监听的端口号 |
KUBECONFIG |
~/.kube/config |
kubeconfig 文件路径 |
OPENAI_API_KEY |
"" |
大模型的 API Key |
OPENAI_API_URL |
"" |
大模型的 API URL |
OPENAI_MODEL |
Qwen/Qwen2.5-7B-Instruct |
大模型的默认模型名称,如需DeepSeek,请设置为deepseek-ai/DeepSeek-R1-Distill-Qwen-7B |
LOGIN_TYPE |
"password" |
登录方式(如 password , oauth , token ) |
ADMIN_USERNAME |
"admin" |
管理员用户名 |
ADMIN_PASSWORD |
"123456" |
管理员密码 |
DEBUG |
"false" |
是否开启 debug 模式 |
LOG_V |
"2" |
log输出日志,同klog用法 |
JWT_TOKEN_SECRET |
"your-secret-key" |
用于 JWT Token 生成的密钥 |
KUBECTL_SHELL_IMAGE |
bitnami/kubectl:latest |
kubectl shell 镜像地址 |
NODE_SHELL_IMAGE |
alpine:latest |
Node shell 镜像地址 |
SQLITE_PATH |
/data/k8m.db |
持久化数据库地址,默认sqlite数据库,文件地址/data/k8m.db |
IN_CLUSTER |
"true" |
是否自动注册纳管宿主集群,默认启用 |
ANY_SELECT |
"true" |
是否开启任意选择划词解释,默认开启 (default true) |
PRINT_CONFIG |
"false" |
是否打印配置信息 |
这些环境变量可以通过在运行应用程序时设置,例如:
export PORT=8080
export OPENAI_API_KEY="your-api-key"
export GIN_MODE="release"
./k8m
注意:环境变量会被启动参数覆盖。
- 创建 KinD Kubernetes 集群
brew install kind
- 创建新的 Kubernetes 集群:
kind create cluster --name k8sgpt-demo
kubectl apply -f https://raw.githubusercontent.com/weibaohui/k8m/refs/heads/main/deploy/k8m.yaml
- 访问: 默认使用了nodePort开放,请访问31999端口。或自行配置Ingress http://NodePortIP:31999
首选建议通过修改环境变量方式进行修改。 例如增加deploy.yaml中的env参数
MCP程序使用3619端口。NodePort使用31919端口。 如果二进制方式直接启动,那么访问地址为http://ip:3619/sse 如果集群方式启动,则访问地址为则访问地址为http://nodeIP:31919/sse
内置MCP Server 管理范围与k8m 纳管的集群范围一致。 界面内已连接的集群均可使用。
类别 | 方法 | 描述 |
---|---|---|
集群管理(1) | list_clusters |
列出所有已注册的Kubernetes集群 |
部署管理(12) | scale_deployment |
扩缩容Deployment |
restart_deployment |
重启Deployment | |
stop_deployment |
停止Deployment | |
restore_deployment |
恢复Deployment | |
update_tag_deployment |
更新Deployment镜像标签 | |
rollout_history_deployment |
查询Deployment升级历史 | |
rollout_undo_deployment |
回滚Deployment | |
rollout_pause_deployment |
暂停Deployment升级 | |
rollout_resume_deployment |
恢复Deployment升级 | |
rollout_status_deployment |
查询Deployment升级状态 | |
hpa_list_deployment |
查询Deployment的HPA列表 | |
list_deployment_pods |
获取Deployment管理的Pod列表 | |
动态资源管理(含CRD,8) | get_k8s_resource |
获取k8s资源 |
describe_k8s_resource |
描述k8s资源 | |
delete_k8s_resource |
删除k8s资源 | |
list_k8s_resource |
列表形式获取k8s资源 | |
list_k8s_event |
列表形式获取k8s事件 | |
patch_k8s_resource |
更新k8s资源,以JSON Patch方式更新 | |
label_k8s_resource |
为k8s资源添加或删除标签 | |
annotate_k8s_resource |
为k8s资源添加或删除注解 | |
节点管理(8) | taint_node |
为节点添加污点 |
untaint_node |
为节点移除污点 | |
cordon_node |
为节点设置Cordon | |
uncordon_node |
为节点取消Cordon | |
drain_node |
为节点执行Drain | |
get_node_resource_usage |
查询节点的资源使用情况 | |
get_node_ip_usage |
查询节点上Pod IP资源使用情况 | |
get_node_pod_count |
查询节点上的Pod数量 | |
Pod 管理(14) | list_pod_files |
列出Pod文件 |
list_all_pod_files |
列出Pod所有文件 | |
delete_pod_file |
删除Pod文件 | |
upload_file_to_pod |
上传文件到Pod内,支持传递文本内容,存储为Pod内文件 | |
get_pod_logs |
获取Pod日志 | |
run_command_in_pod |
在Pod中执行命令 | |
get_pod_linked_service |
获取Pod关联的Service | |
get_pod_linked_ingress |
获取Pod关联的Ingress | |
get_pod_linked_endpoints |
获取Pod关联的Endpoints | |
get_pod_linked_pvc |
获取Pod关联的PVC | |
get_pod_linked_pv |
获取Pod关联的PV | |
get_pod_linked_env |
通过在pod内运行env命令获取Pod运行时环境变量 | |
get_pod_linked_env_from_yaml |
通过Pod yaml定义获取Pod运行时环境变量 | |
get_pod_resource_usage |
获取Pod的资源使用情况,包括CPU和内存的请求值、限制值、可分配值和使用比例 | |
YAML管理(2) | apply_yaml |
应用YAML资源 |
delete_yaml |
删除YAML资源 | |
存储管理(3) | set_default_storageclass |
设置默认StorageClass |
get_storageclass_pvc_count |
获取StorageClass下的PVC数量 | |
get_storageclass_pv_count |
获取StorageClass下的PV数量 | |
Ingress管理(1) | set_default_ingressclass |
设置默认IngressClass |
适合MCP工具集成,如Cursor、Claude Desktop、Windsurf等,此外也可以使用这些软件的UI操作界面进行添加。
{
"mcpServers": {
"kom": {
"type": "sse",
"url": "http://IP:9096/sse"
}
}
}
- 打开Claude Desktop设置面板
- 在API配置区域添加MCP Server地址
- 启用SSE事件监听功能
- 验证连接状态
- 进入Cursor设置界面
- 找到扩展服务配置选项
- 添加MCP Server的URL(例如:http://localhost:3619/sse)
- 访问配置中心
- 设置API服务器地址
- 确保MCP Server正常运行且端口可访问
- 检查网络连接是否正常
- 验证SSE连接是否成功建立
- 查看工具日志以排查连接问题,MCP执行失败会有报错记录。
v0.0.75更新
- 分离用户操作界面、平台管理界面。平台管理界面新增一个平台管理菜单。
1.1 用户多集群切换,保留切换、连接功能:
1.2 管理员操作多集群,新增断开功能:
1.3 集群管理新增已授权页面,展示集群下所有的授权用户
1.4 用户管理新增授权页面,查看某用户所有的授权集群
- 新增权限可设置ns,集群授权后,可补充ns,默认为不限制,填写后,将限制用户活动范围。
- 新增参数配置页面。
启动后会先加载环境变量、env文件、页面配置,依次覆盖。最终页面配置为准。
- 新增资源、副本数调整页面
V0.0.73 更新
V0.0.72 更新
V0.0.70 更新
v0.0.67 更新
V0.0.66更新
- 新增MCP支持。
- 内置支持k8s多集群操作:
- list_k8s_resource
- get_k8s_resource
- delete_k8s_resource
- describe_k8s_resource
- get_pod_logs
v0.0.64 更新
v0.0.62 更新
v0.0.61 更新
- 新增2FA两步验证
启用后,登录时需填写验证码,增强安全性
- InCluster运行模式增加开关 默认开启,可设置环境变量显式关闭。按需开启。
- 优化资源用量显示逻辑
未设置资源用量,在k8s中属于最低保障等级。界面显示进度条调整为红色100%,提醒管理员关注。
v0.0.60更新
- 增加helm 常用仓库
- Namespace增加LimitRange、ResourceQuota快捷菜单
- 增加InCluster模式开关 默认开启InCluster模式,如需关闭,可以注入环境变量,或修改配置文件,或修改命令行参数
v0.0.53更新
- 日志查看支持颜色,如果输出console的时候带有颜色,那么在pod 日志查看时就可以显示。
- Helm功能上线
2.1 新增helm仓库
2.2 安装helm chart 应用 应用列表
查看应用
支持对参数内容选中划词AI解释
2.3 查看已部署release
2.4 查看安装参数
2.5 更新、升级、降级部署版本
2.6 查看已部署release变更历史
v0.0.50更新
v0.0.49更新
- 新增标签搜索:支持精确搜索、模糊搜索。
精确搜索。可以搜索k,k=v两种方式精确搜索。默认列出所有标签。支持自定义新增搜索标签。
模糊搜索。可以搜索k,v中的任意满足。类似like %xx%的搜索方式。
- 多集群纳管支持自定义名称。
- 优化Pod状态显示
在列表页展示pod状态,不同颜色区分正常运行与未就绪运行。
v0.0.44更新
-
新增创建功能页面 执行过的yaml会保存下来,下次打开页面可以直接点击,收藏的yaml可以导入导出。导出的文件为yaml,可以复用
-
deploy、ds、sts等类型新增关联资源 4.1 容器组 直接显示其下受控的pod容器组,并提供快捷操作
4.2 关联事件 显示deploy、rs、pod等所有相关的事件,一个页面看全相关事件
4.3 日志 显示Pod列表,可选择某个pod、Container展示日志
4.4 历史版本 支持历史版本查看,并可diff
v0.0.21更新
- 新增问AI功能:
有什么问题,都可以直接询问AI,让AI解答你的疑惑
- 文档界面优化:
优化AI翻译效果,降低等待时间
- 文档字段级AI示例:
针对具体的字段,给出解释,给出使用Demo样例。
- 增加容忍度详情:
- 增加Pod关联资源
一个页面,展示相关的svc、endpoint、pvc、env、cm、secret,甚至集成了pod内的env列表,方便查看
- yaml创建增加导入功能:
增加导入功能,可以直接执行,也可导入到编辑器。导入编辑器后可以二次编辑后,再执行。
v0.0.19更新
- 多集群管理功能
按需选择多集群,可随时切换集群
- 节点资源用量功能
直观显示已分配资源情况,包括cpu、内存、pod数量、IP数量。
- Pod 资源用量
- Pod CPU内存设置
按范围方式显示CPU设置,内存设置,简洁明了
- AI页面功能升级为打字机效果
响应速度大大提升,实时输出AI返回内容,体验升级
v0.0.15更新
- 所有页面增加资源使用指南。启用AI信息聚合。包括资源说明、使用场景(举例说明)、最佳实践、典型示例(配合前面的场景举例,编写带有中文注释的yaml示例)、关键字段及其含义、常见问题、官方文档链接、引用文档链接等信息,帮助用户理解k8s
- 所有资源页面增加搜索功能。部分页面增高频过滤字段搜索。
- 改进LimitRange信息展示模式
- 改进状态显示样式
- 统一操作菜单
- Ingress页面增加域名转发规则信息
- 改进标签显示样式,鼠标悬停展示
- 优化资源状态样式更小更紧致
- 丰富Service展示信息
- 突出显示未就绪endpoints
- endpoints鼠标悬停展开未就绪IP列表
- endpointslice 突出显示未ready的IP及其对应的POD,
- 角色增加延展信息
- 角色与主体对应关系
- 界面全量中文化,k8s资源翻译为中文,方便广大用户使用。
如果你有任何进一步的问题或需要额外的帮助,请随时与我联系!
zhaomingcheng01:提出了诸多非常高质量的建议,为k8m的易用好用做出了卓越贡献~
La0jin:提供在线资源及维护,极大提升了k8m的展示效果
微信(大罗马的太阳) 搜索ID:daluomadetaiyang,备注k8m。
For Tasks:
Click tags to check more tools for each tasksFor Jobs:
Alternative AI tools for k8m
Similar Open Source Tools
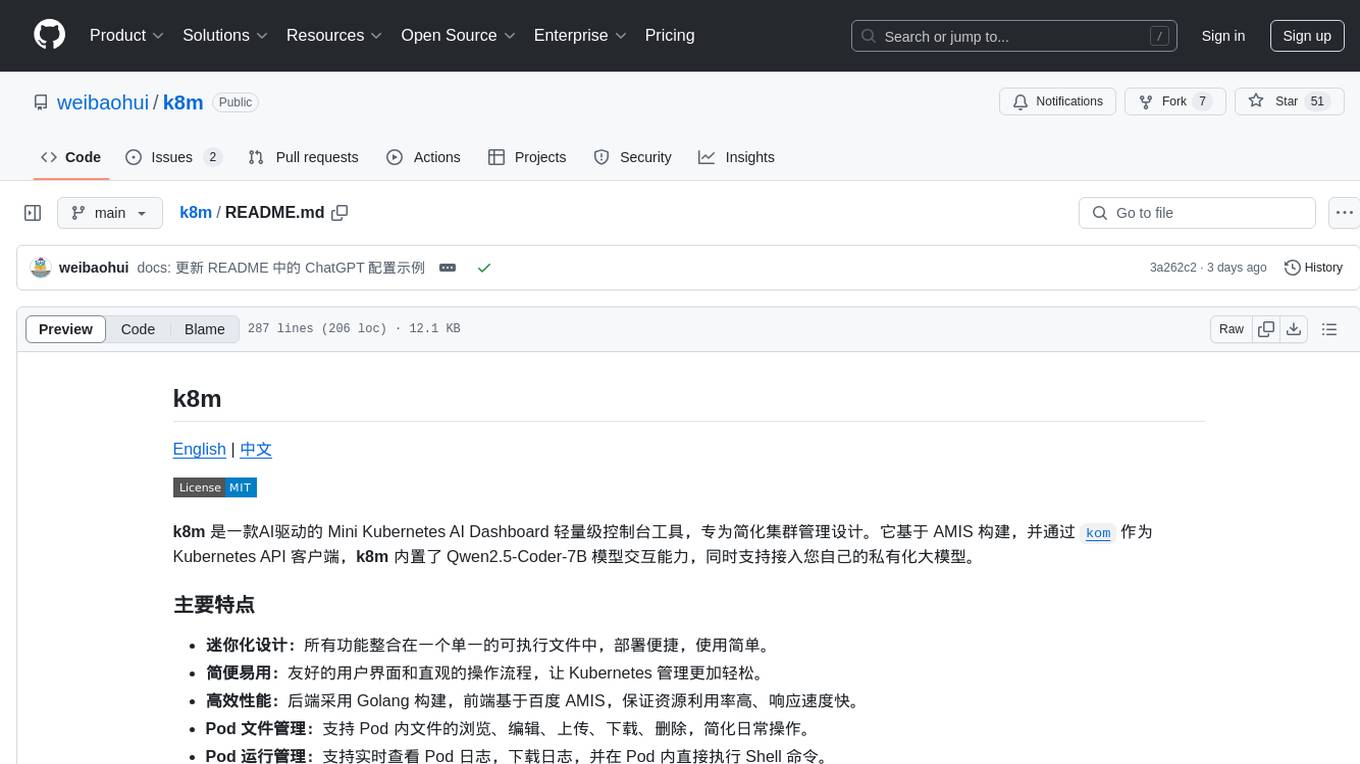
k8m
k8m is an AI-driven Mini Kubernetes AI Dashboard lightweight console tool designed to simplify cluster management. It is built on AMIS and uses 'kom' as the Kubernetes API client. k8m has built-in Qwen2.5-Coder-7B model interaction capabilities and supports integration with your own private large models. Its key features include miniaturized design for easy deployment, user-friendly interface for intuitive operation, efficient performance with backend in Golang and frontend based on Baidu AMIS, pod file management for browsing, editing, uploading, downloading, and deleting files, pod runtime management for real-time log viewing, log downloading, and executing shell commands within pods, CRD management for automatic discovery and management of CRD resources, and intelligent translation and diagnosis based on ChatGPT for YAML property translation, Describe information interpretation, AI log diagnosis, and command recommendations, providing intelligent support for managing k8s. It is cross-platform compatible with Linux, macOS, and Windows, supporting multiple architectures like x86 and ARM for seamless operation. k8m's design philosophy is 'AI-driven, lightweight and efficient, simplifying complexity,' helping developers and operators quickly get started and easily manage Kubernetes clusters.
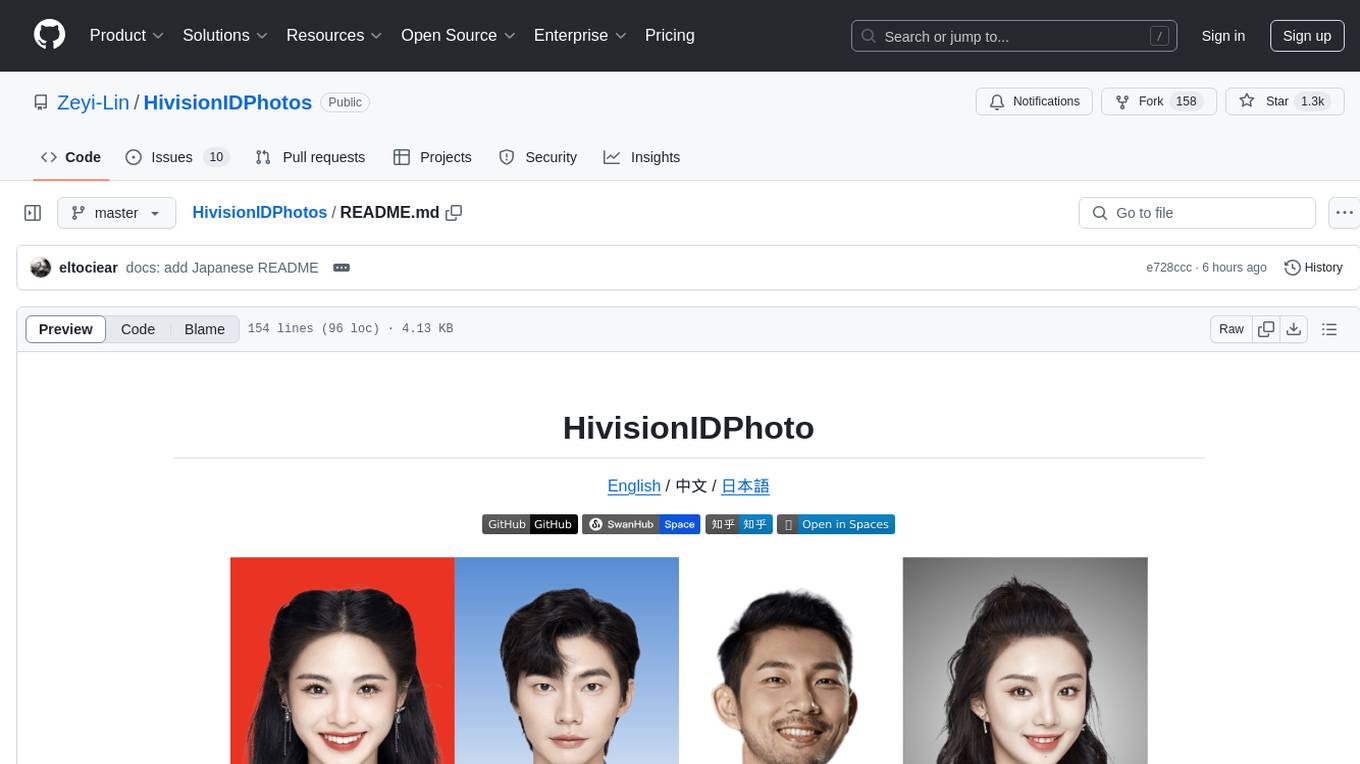
HivisionIDPhotos
HivisionIDPhoto is a practical algorithm for intelligent ID photo creation. It utilizes a comprehensive model workflow to recognize, cut out, and generate ID photos for various user photo scenarios. The tool offers lightweight cutting, standard ID photo generation based on different size specifications, six-inch layout photo generation, beauty enhancement (waiting), and intelligent outfit swapping (waiting). It aims to solve emergency ID photo creation issues.
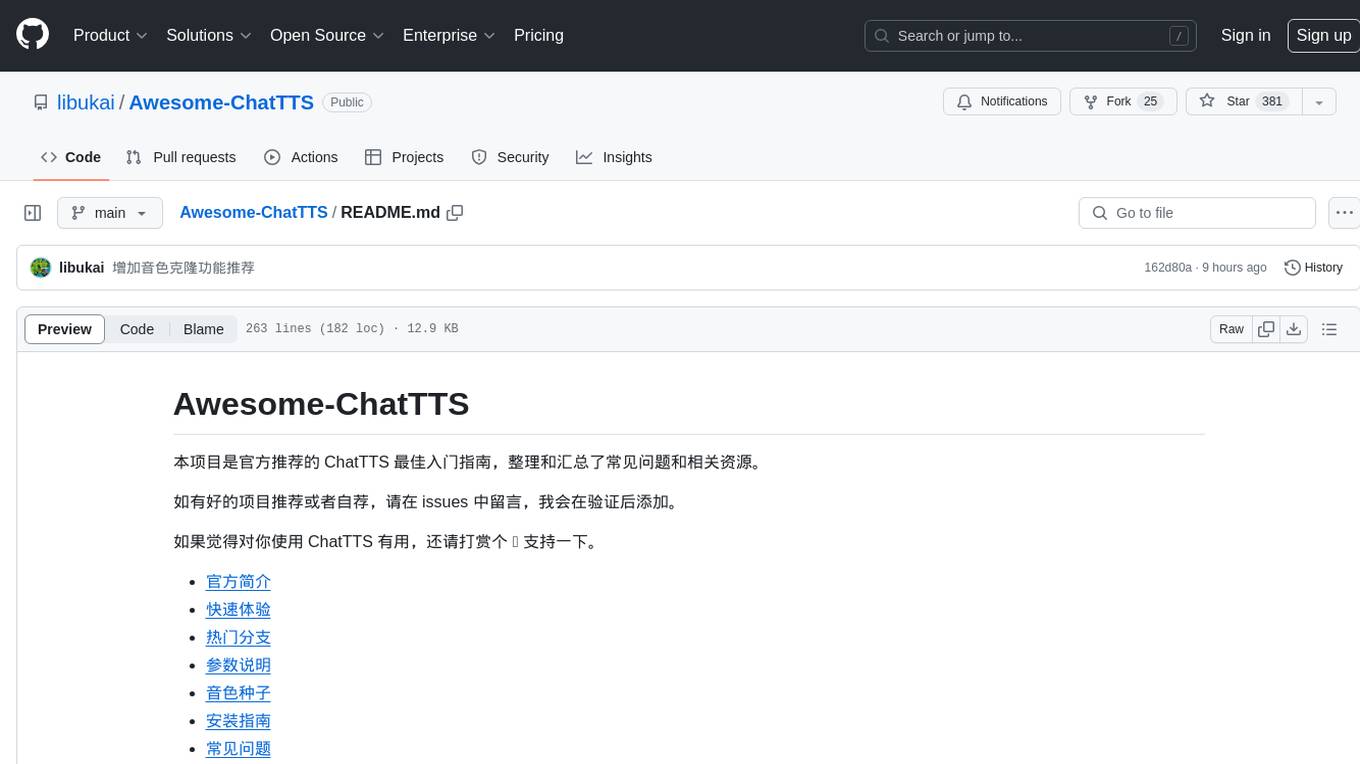
Awesome-ChatTTS
Awesome-ChatTTS is an official recommended guide for ChatTTS beginners, compiling common questions and related resources. It provides a comprehensive overview of the project, including official introduction, quick experience options, popular branches, parameter explanations, voice seed details, installation guides, FAQs, and error troubleshooting. The repository also includes video tutorials, discussion community links, and project trends analysis. Users can explore various branches for different functionalities and enhancements related to ChatTTS.
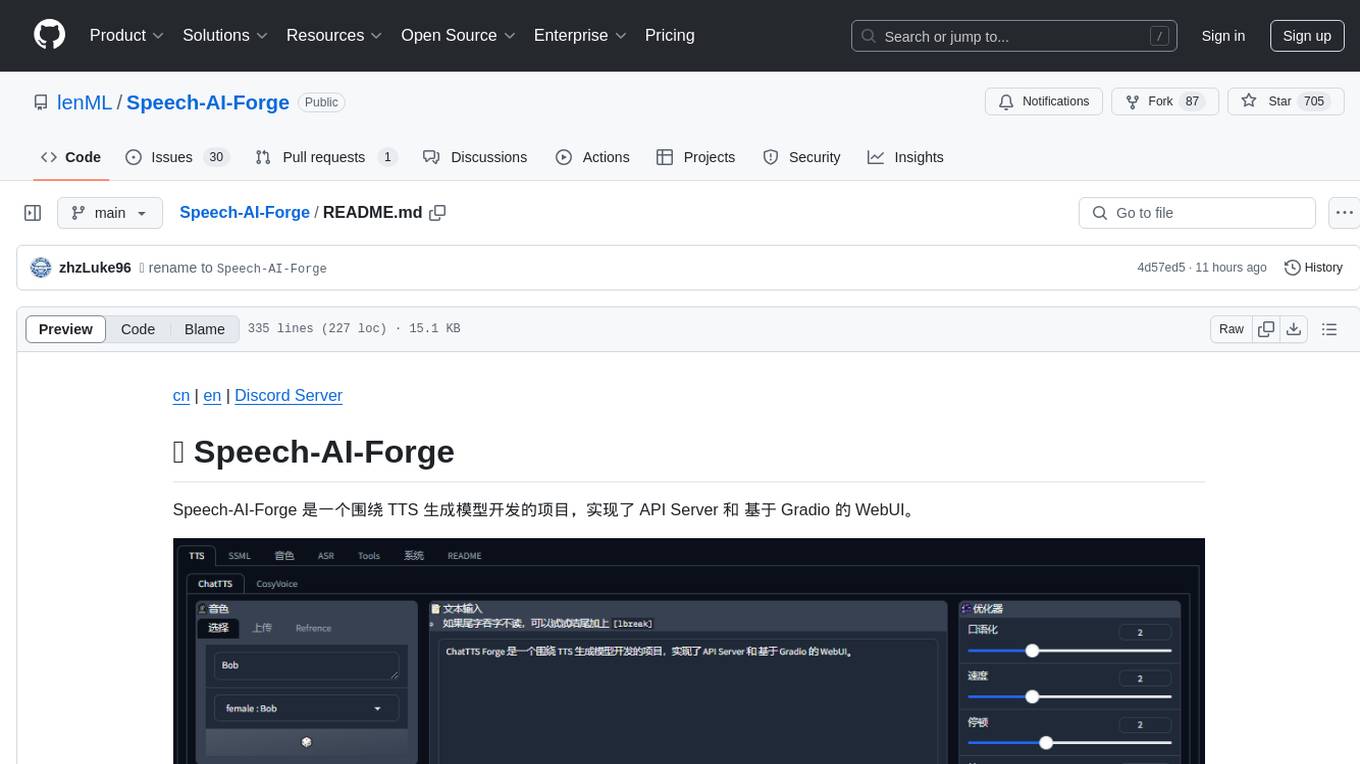
Speech-AI-Forge
Speech-AI-Forge is a project developed around TTS generation models, implementing an API Server and a WebUI based on Gradio. The project offers various ways to experience and deploy Speech-AI-Forge, including online experience on HuggingFace Spaces, one-click launch on Colab, container deployment with Docker, and local deployment. The WebUI features include TTS model functionality, speaker switch for changing voices, style control, long text support with automatic text segmentation, refiner for ChatTTS native text refinement, various tools for voice control and enhancement, support for multiple TTS models, SSML synthesis control, podcast creation tools, voice creation, voice testing, ASR tools, and post-processing tools. The API Server can be launched separately for higher API throughput. The project roadmap includes support for various TTS models, ASR models, voice clone models, and enhancer models. Model downloads can be manually initiated using provided scripts. The project aims to provide inference services and may include training-related functionalities in the future.
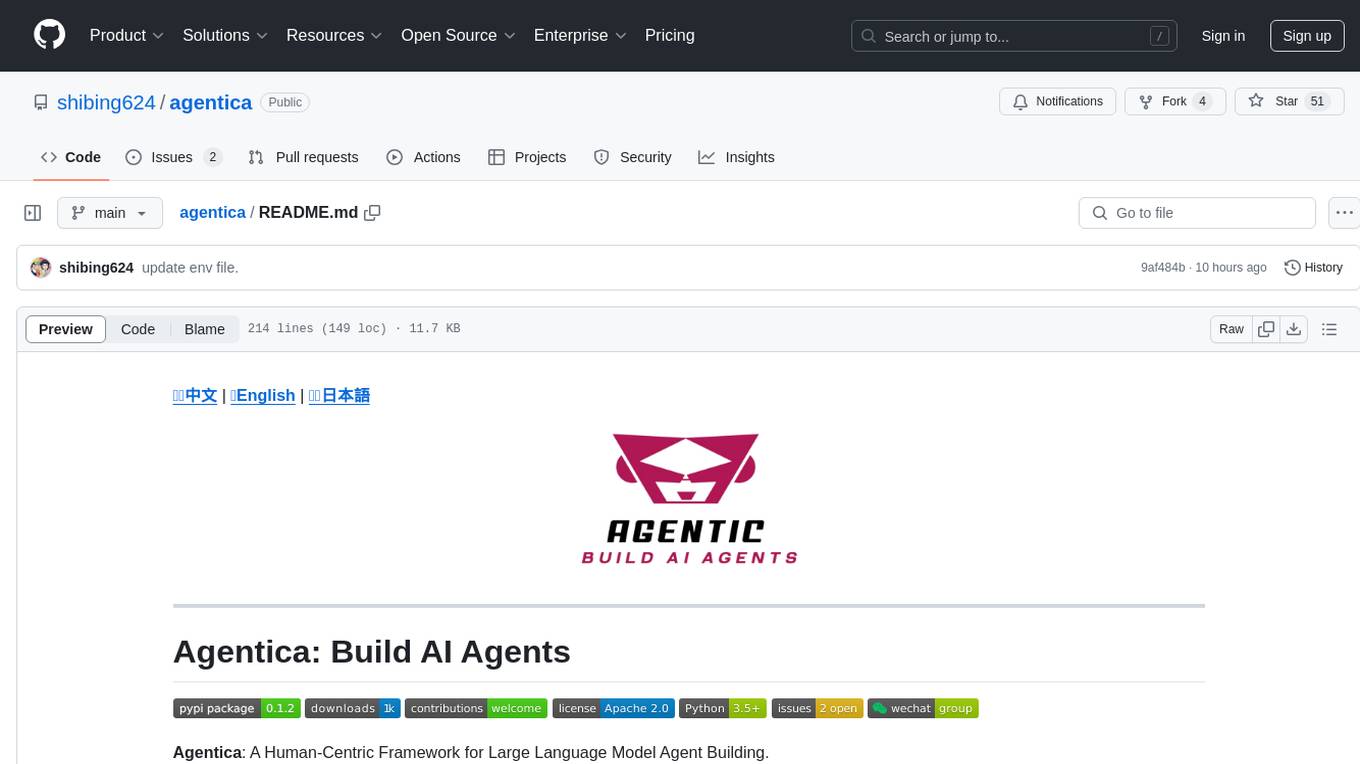
agentica
Agentica is a human-centric framework for building large language model agents. It provides functionalities for planning, memory management, tool usage, and supports features like reflection, planning and execution, RAG, multi-agent, multi-role, and workflow. The tool allows users to quickly code and orchestrate agents, customize prompts, and make API calls to various services. It supports API calls to OpenAI, Azure, Deepseek, Moonshot, Claude, Ollama, and Together. Agentica aims to simplify the process of building AI agents by providing a user-friendly interface and a range of functionalities for agent development.
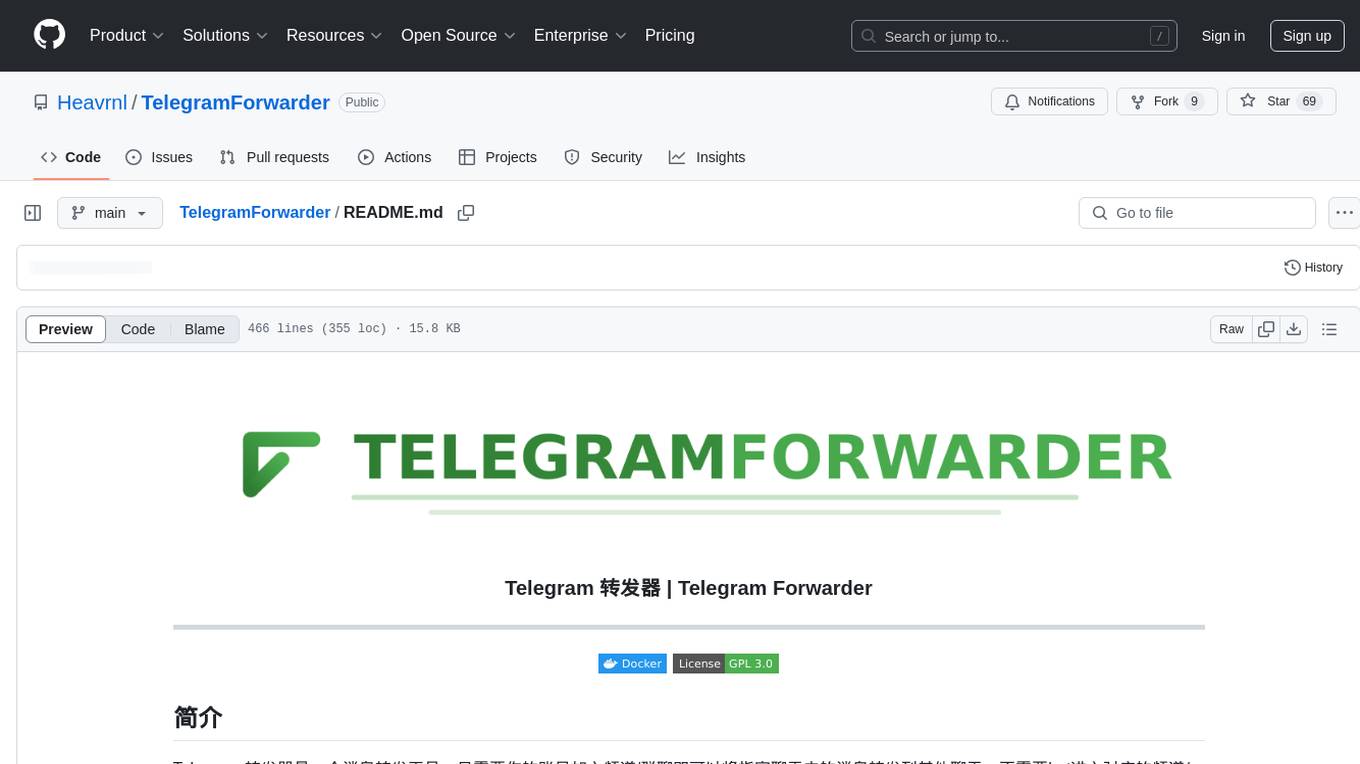
TelegramForwarder
Telegram Forwarder is a message forwarding tool that allows you to forward messages from specified chats to other chats without the need for a bot to enter the corresponding channels/groups to listen. It can be used for information stream integration filtering, message reminders, content archiving, and more. The tool supports multiple sources forwarding, keyword filtering in whitelist and blacklist modes, regular expression matching, message content modification, AI processing using major vendors' AI interfaces, media file filtering, and synchronization with a universal forum blocking plugin to achieve three-end blocking.
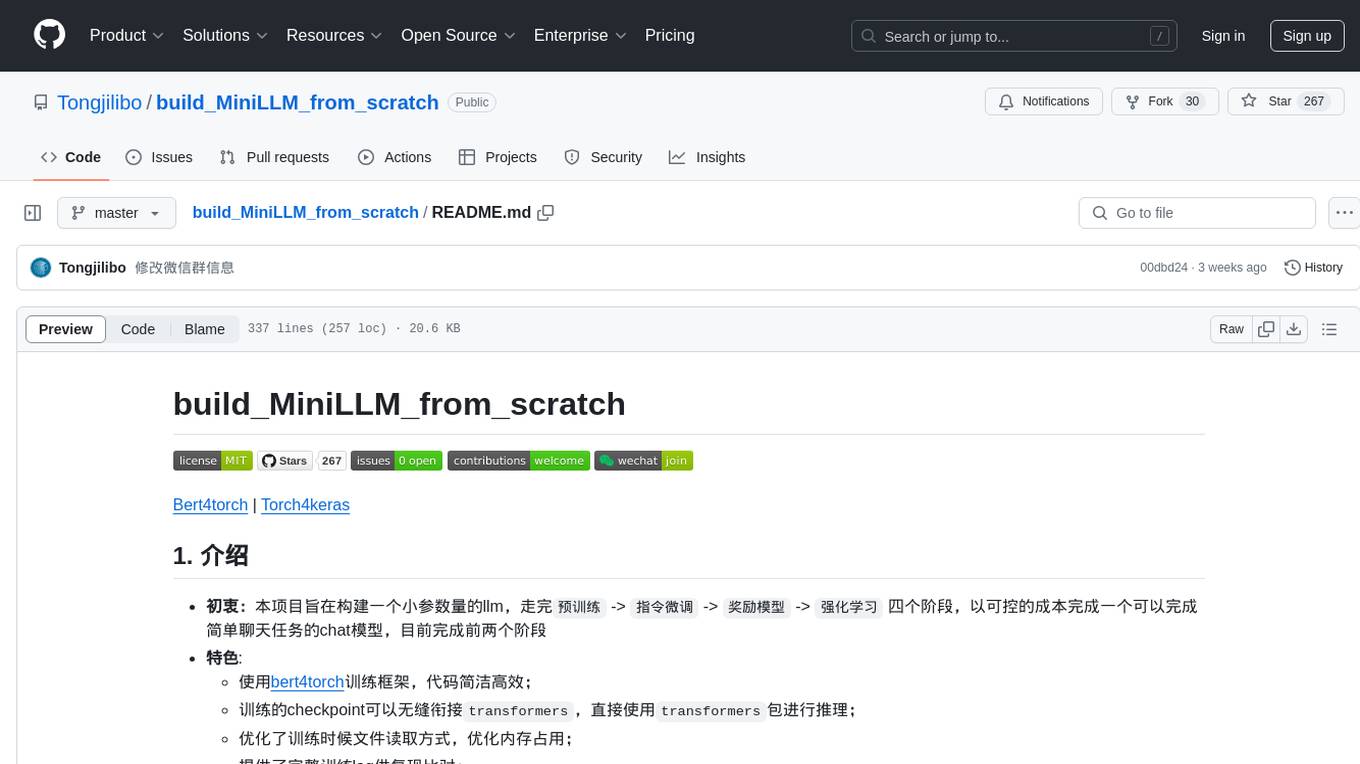
build_MiniLLM_from_scratch
This repository aims to build a low-parameter LLM model through pretraining, fine-tuning, model rewarding, and reinforcement learning stages to create a chat model capable of simple conversation tasks. It features using the bert4torch training framework, seamless integration with transformers package for inference, optimized file reading during training to reduce memory usage, providing complete training logs for reproducibility, and the ability to customize robot attributes. The chat model supports multi-turn conversations. The trained model currently only supports basic chat functionality due to limitations in corpus size, model scale, SFT corpus size, and quality.
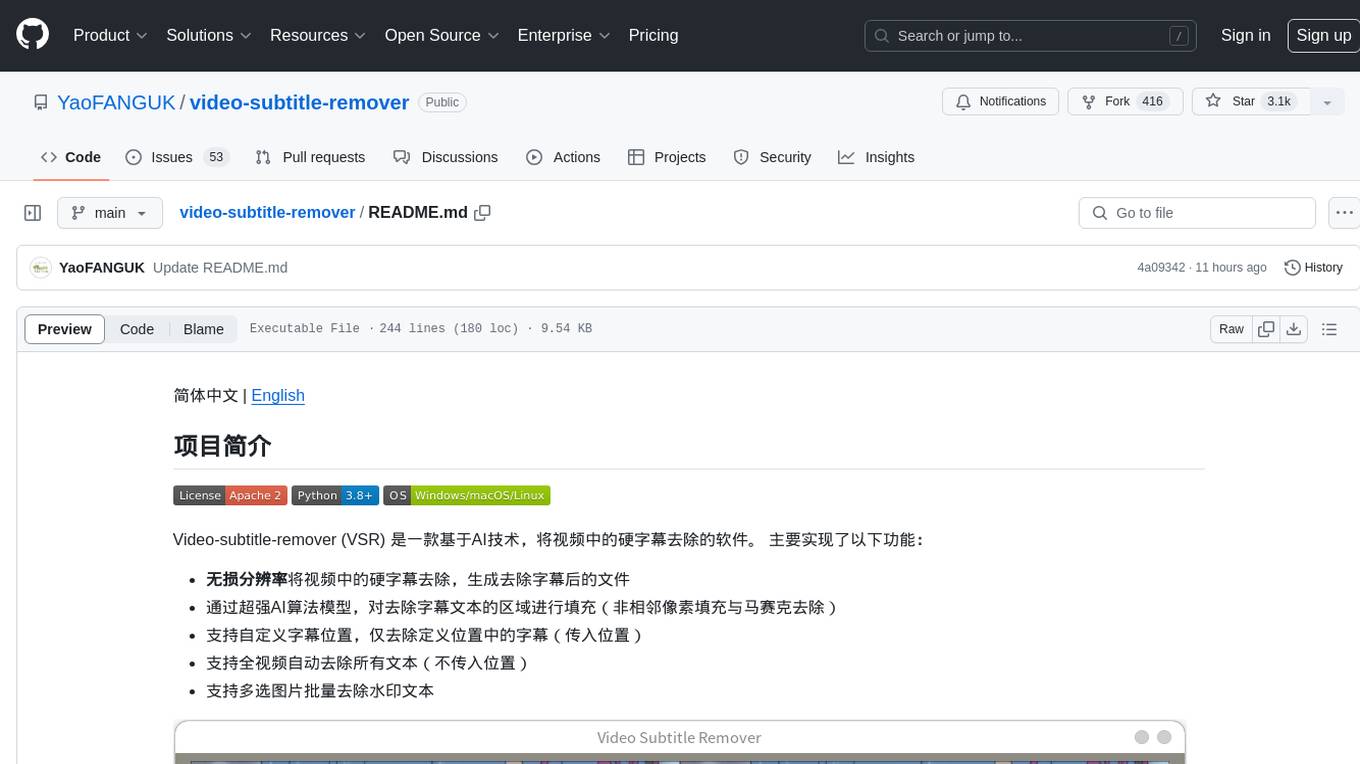
video-subtitle-remover
Video-subtitle-remover (VSR) is a software based on AI technology that removes hard subtitles from videos. It achieves the following functions: - Lossless resolution: Remove hard subtitles from videos, generate files with subtitles removed - Fill the region of removed subtitles using a powerful AI algorithm model (non-adjacent pixel filling and mosaic removal) - Support custom subtitle positions, only remove subtitles in defined positions (input position) - Support automatic removal of all text in the entire video (no input position required) - Support batch removal of watermark text from multiple images.
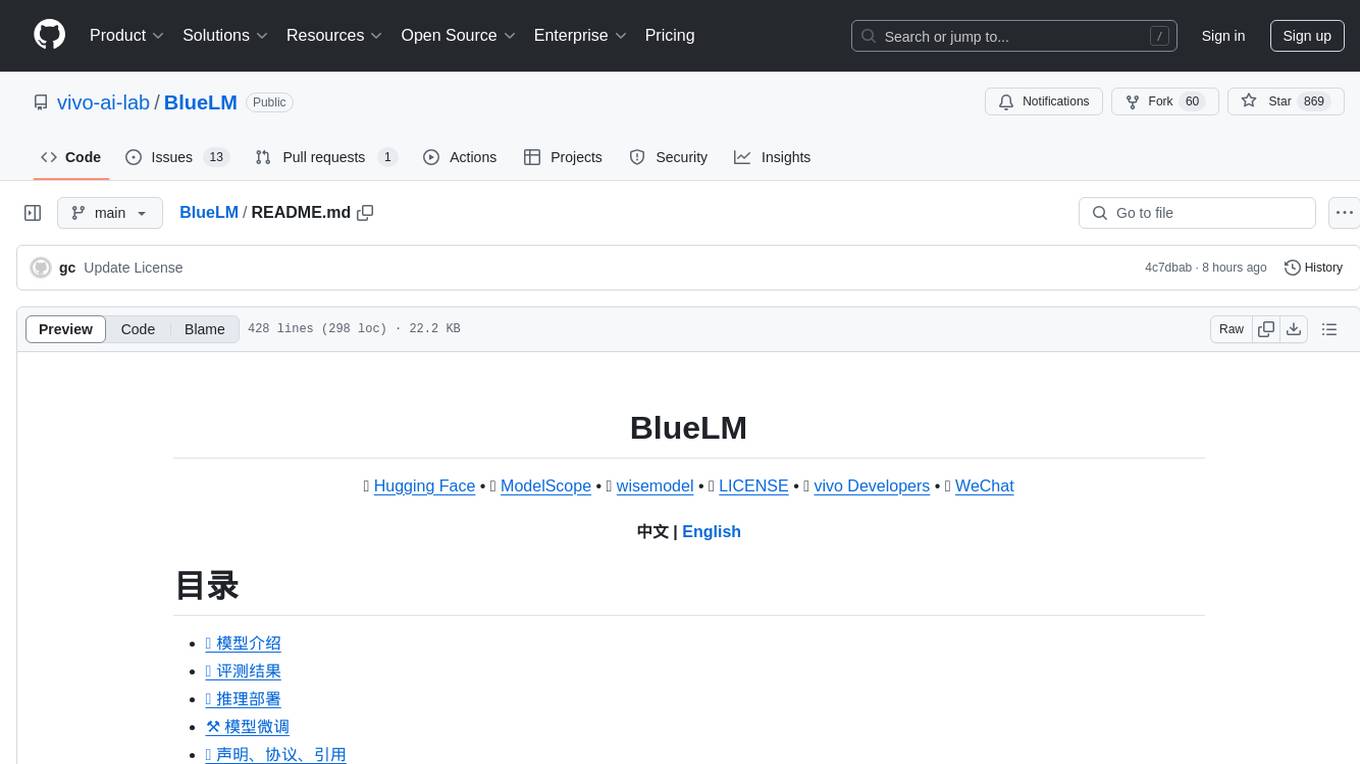
BlueLM
BlueLM is a large-scale pre-trained language model developed by vivo AI Global Research Institute, featuring 7B base and chat models. It includes high-quality training data with a token scale of 26 trillion, supporting both Chinese and English languages. BlueLM-7B-Chat excels in C-Eval and CMMLU evaluations, providing strong competition among open-source models of similar size. The models support 32K long texts for better context understanding while maintaining base capabilities. BlueLM welcomes developers for academic research and commercial applications.
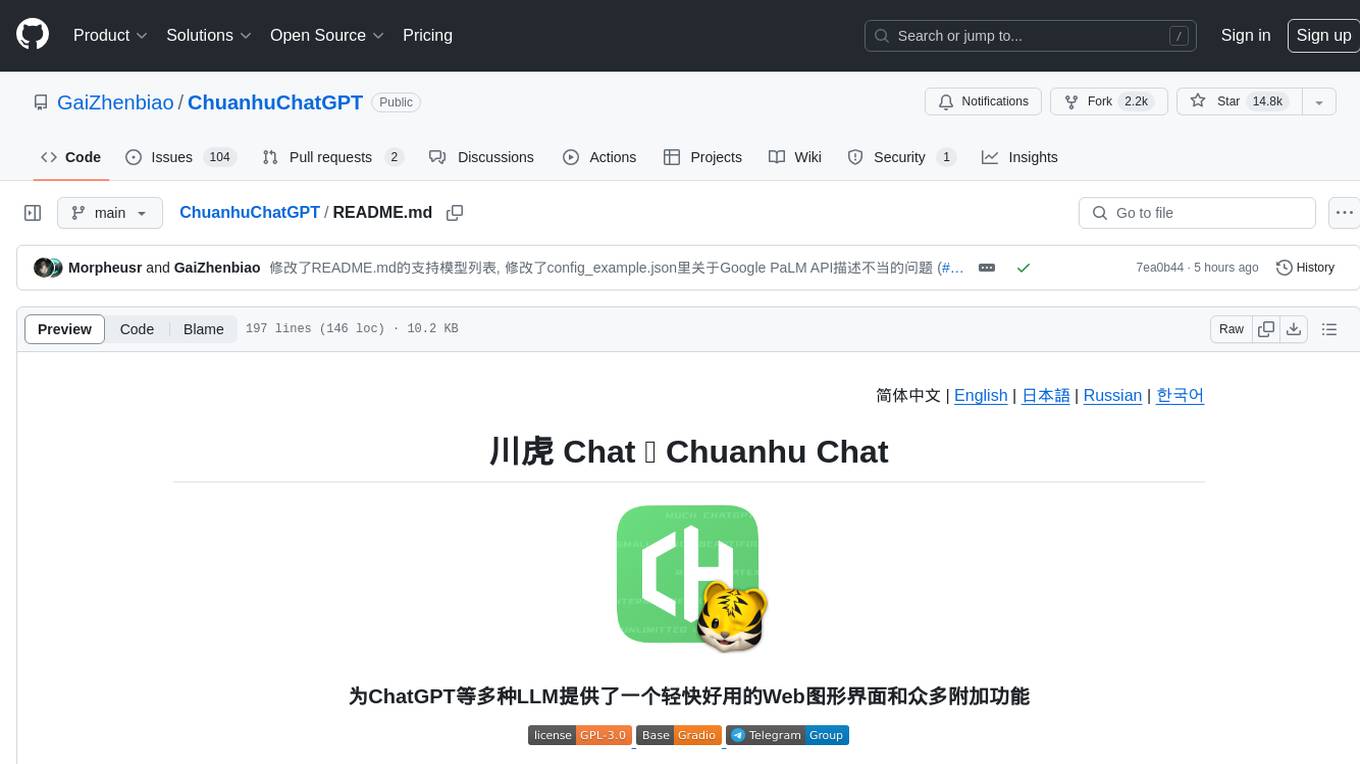
ChuanhuChatGPT
Chuanhu Chat is a user-friendly web graphical interface that provides various additional features for ChatGPT and other language models. It supports GPT-4, file-based question answering, local deployment of language models, online search, agent assistant, and fine-tuning. The tool offers a range of functionalities including auto-solving questions, online searching with network support, knowledge base for quick reading, local deployment of language models, GPT 3.5 fine-tuning, and custom model integration. It also features system prompts for effective role-playing, basic conversation capabilities with options to regenerate or delete dialogues, conversation history management with auto-saving and search functionalities, and a visually appealing user experience with themes, dark mode, LaTeX rendering, and PWA application support.
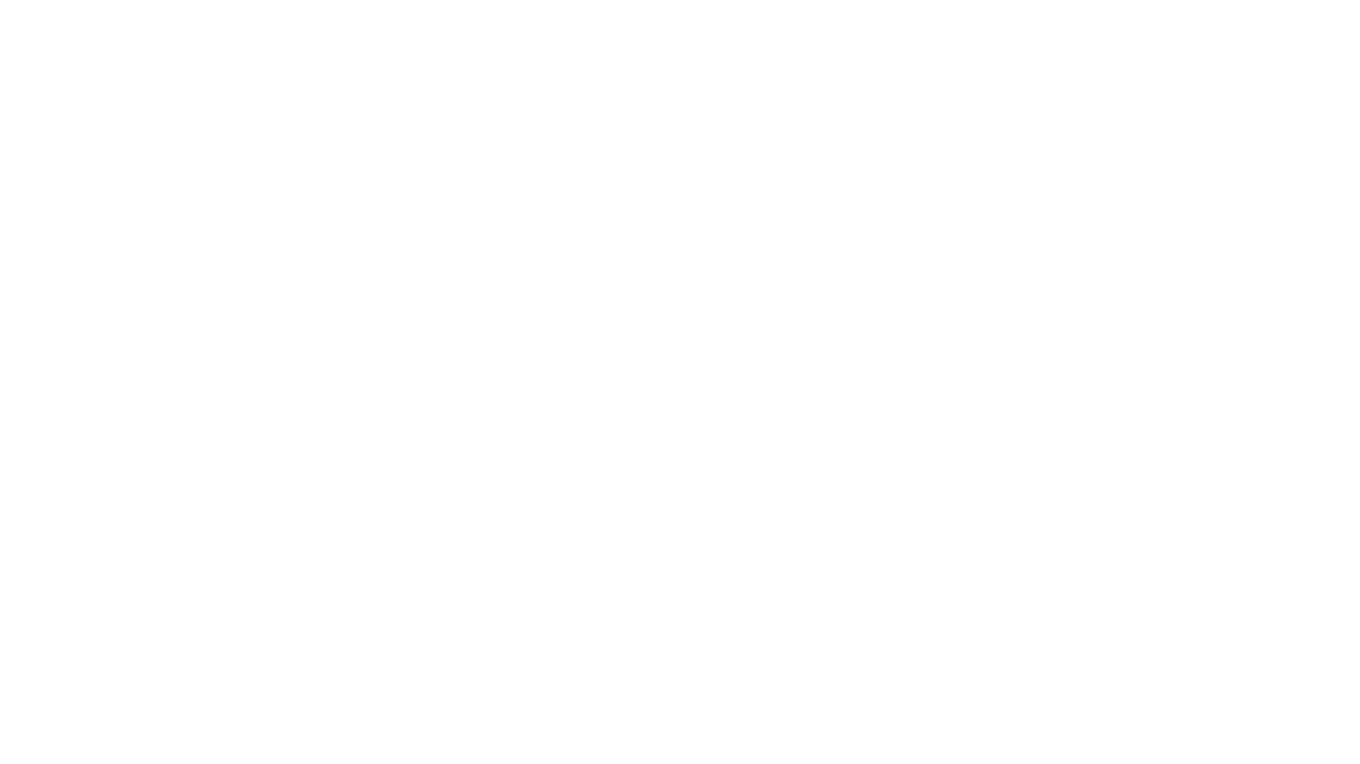
chatluna
Chatluna is a machine learning model plugin that provides chat services with large language models. It is highly extensible, supports multiple output formats, and offers features like custom conversation presets, rate limiting, and context awareness. Users can deploy Chatluna under Koishi without additional configuration. The plugin supports various models/platforms like OpenAI, Azure OpenAI, Google Gemini, and more. It also provides preset customization using YAML files and allows for easy forking and development within Koishi projects. However, the project lacks web UI, HTTP server, and project documentation, inviting contributions from the community.
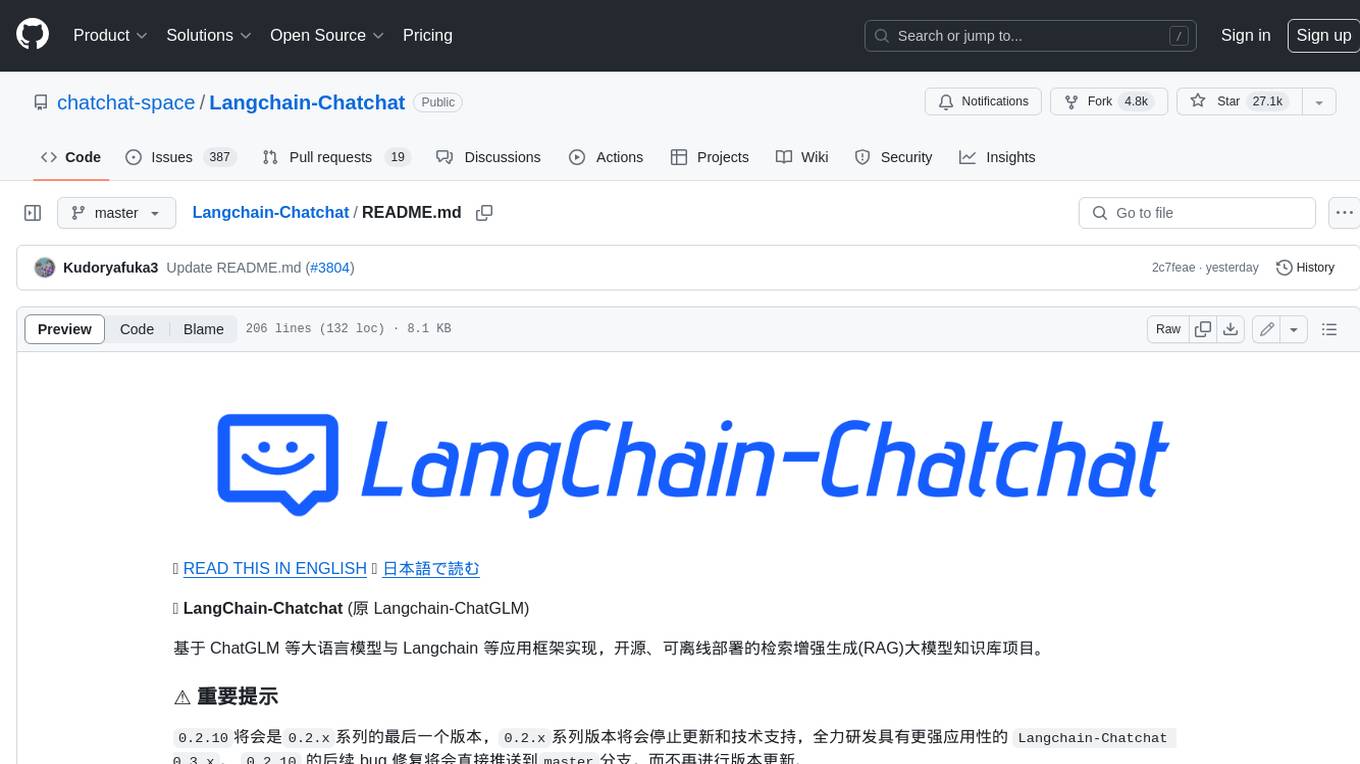
Langchain-Chatchat
LangChain-Chatchat is an open-source, offline-deployable retrieval-enhanced generation (RAG) large model knowledge base project based on large language models such as ChatGLM and application frameworks such as Langchain. It aims to establish a knowledge base Q&A solution that is friendly to Chinese scenarios, supports open-source models, and can run offline.
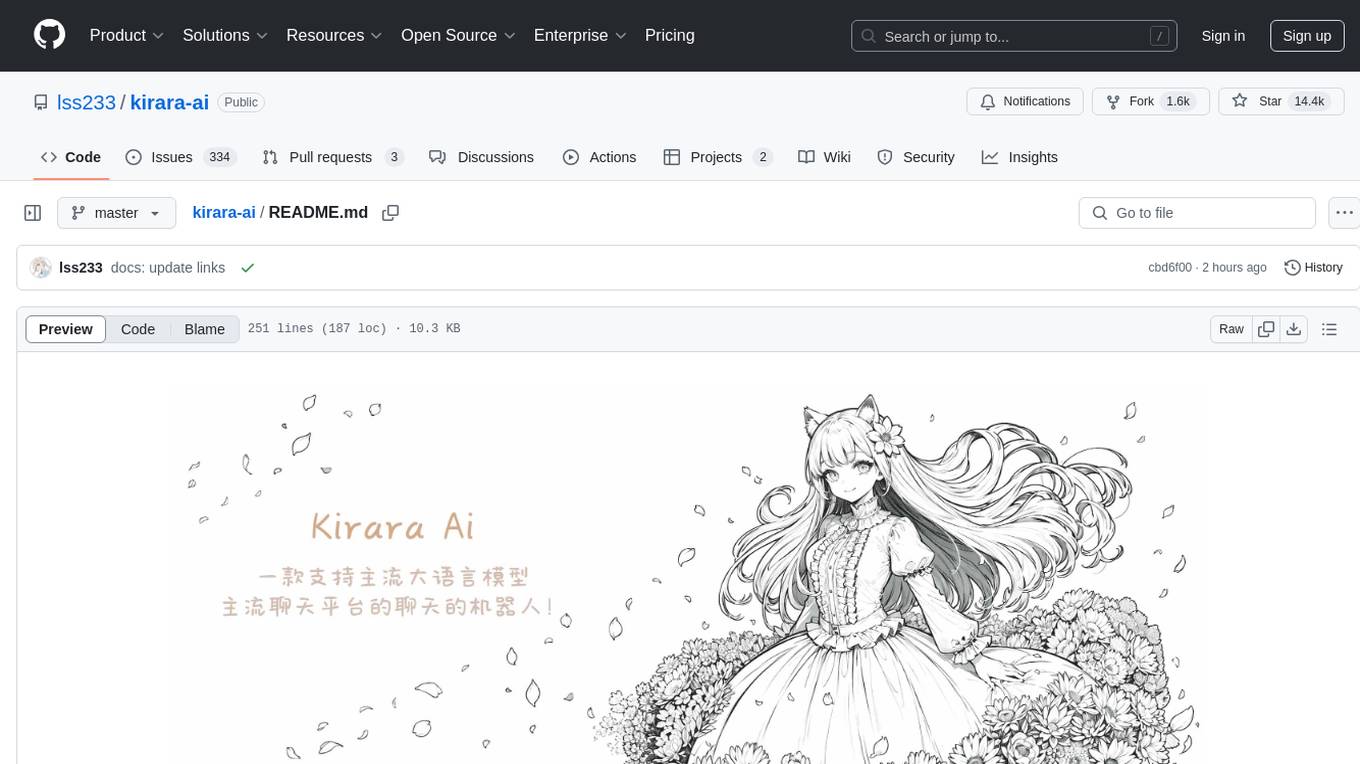
kirara-ai
Kirara AI is a chatbot that supports mainstream large language models and chat platforms. It provides features such as image sending, keyword-triggered replies, multi-account support, personality settings, and support for various chat platforms like QQ, Telegram, Discord, and WeChat. The tool also supports HTTP server for Web API, popular large models like OpenAI and DeepSeek, plugin mechanism, conditional triggers, admin commands, drawing models, voice replies, multi-turn conversations, cross-platform message sending, custom workflows, web management interface, and built-in Frpc intranet penetration.
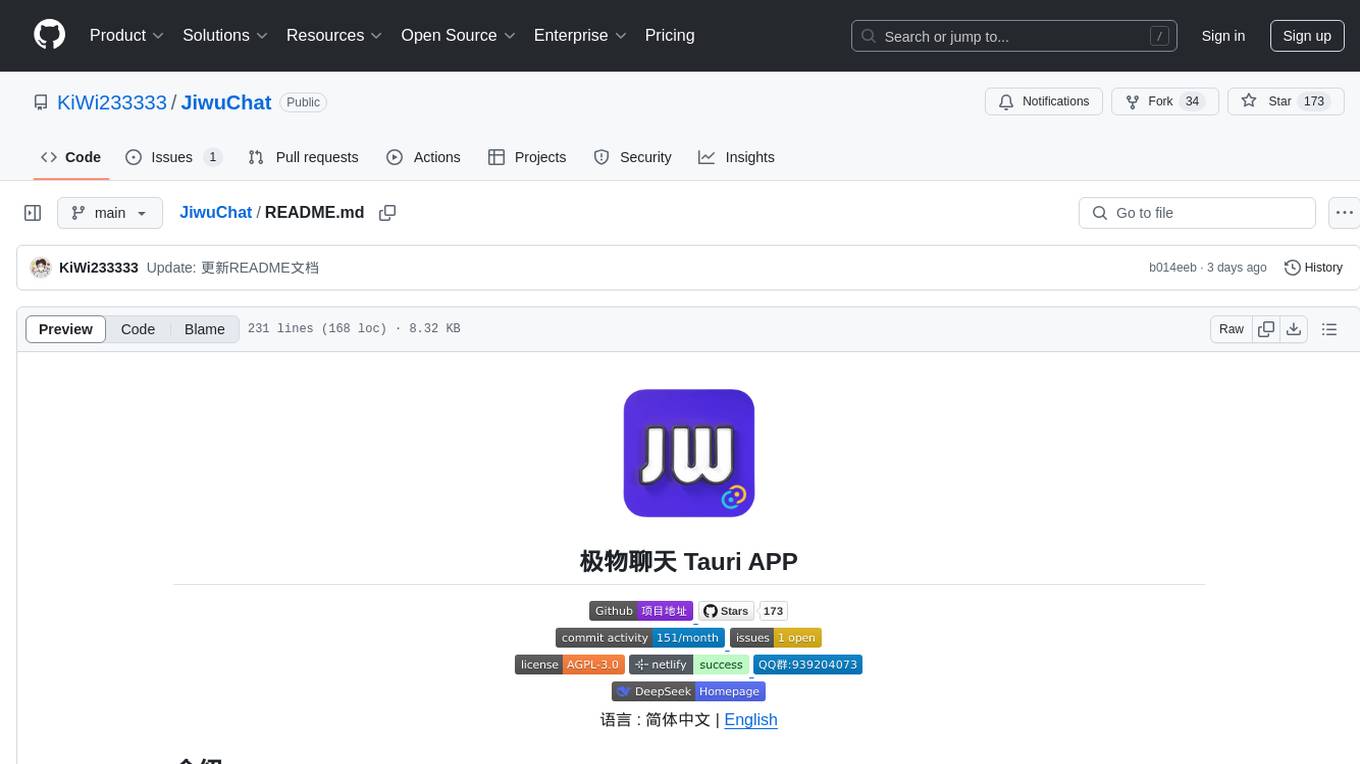
JiwuChat
JiwuChat is a lightweight multi-platform chat application built on Tauri2 and Nuxt3, with various real-time messaging features, AI group chat bots (such as 'iFlytek Spark', 'KimiAI' etc.), WebRTC audio-video calling, screen sharing, and AI shopping functions. It supports seamless cross-device communication, covering text, images, files, and voice messages, also supporting group chats and customizable settings. It provides light/dark mode for efficient social networking.
For similar tasks
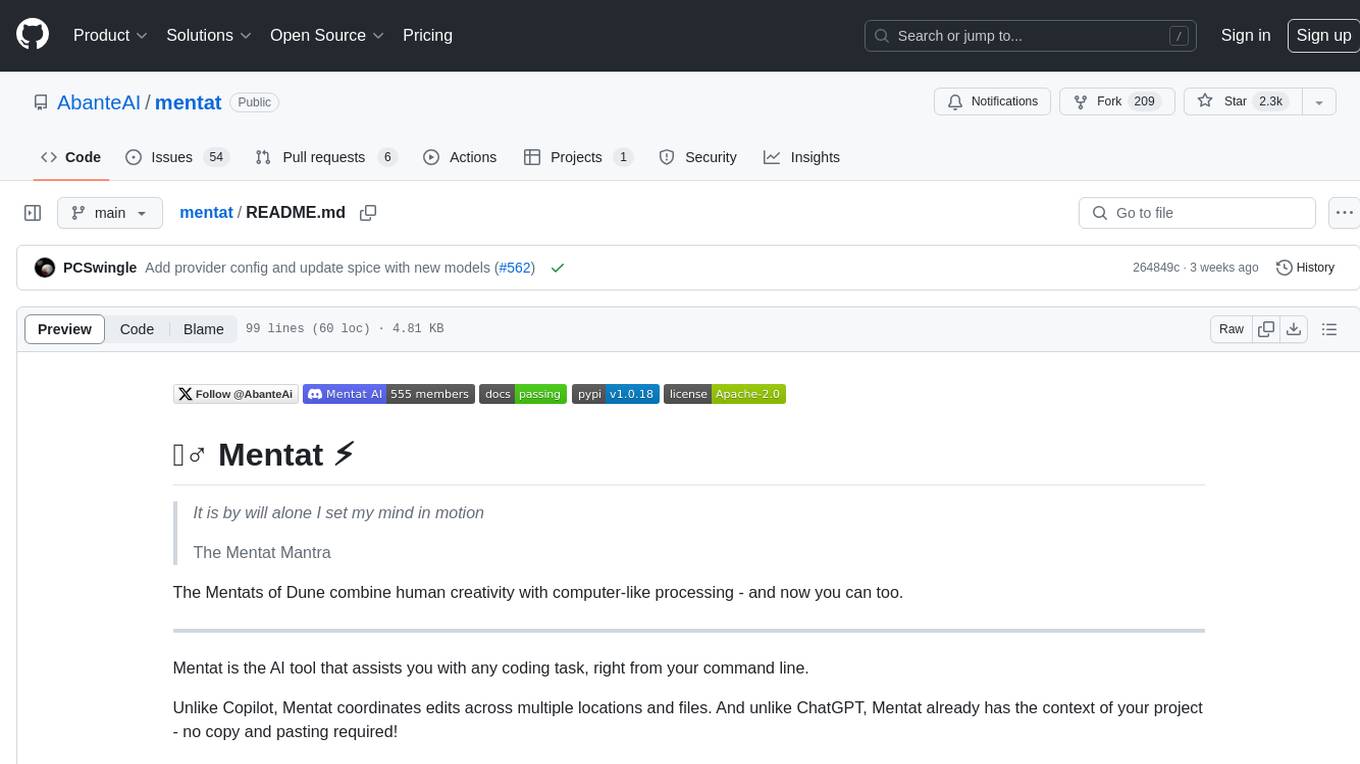
mentat
Mentat is an AI tool designed to assist with coding tasks directly from the command line. It combines human creativity with computer-like processing to help users understand new codebases, add new features, and refactor existing code. Unlike other tools, Mentat coordinates edits across multiple locations and files, with the context of the project already in mind. The tool aims to enhance the coding experience by providing seamless assistance and improving edit quality.
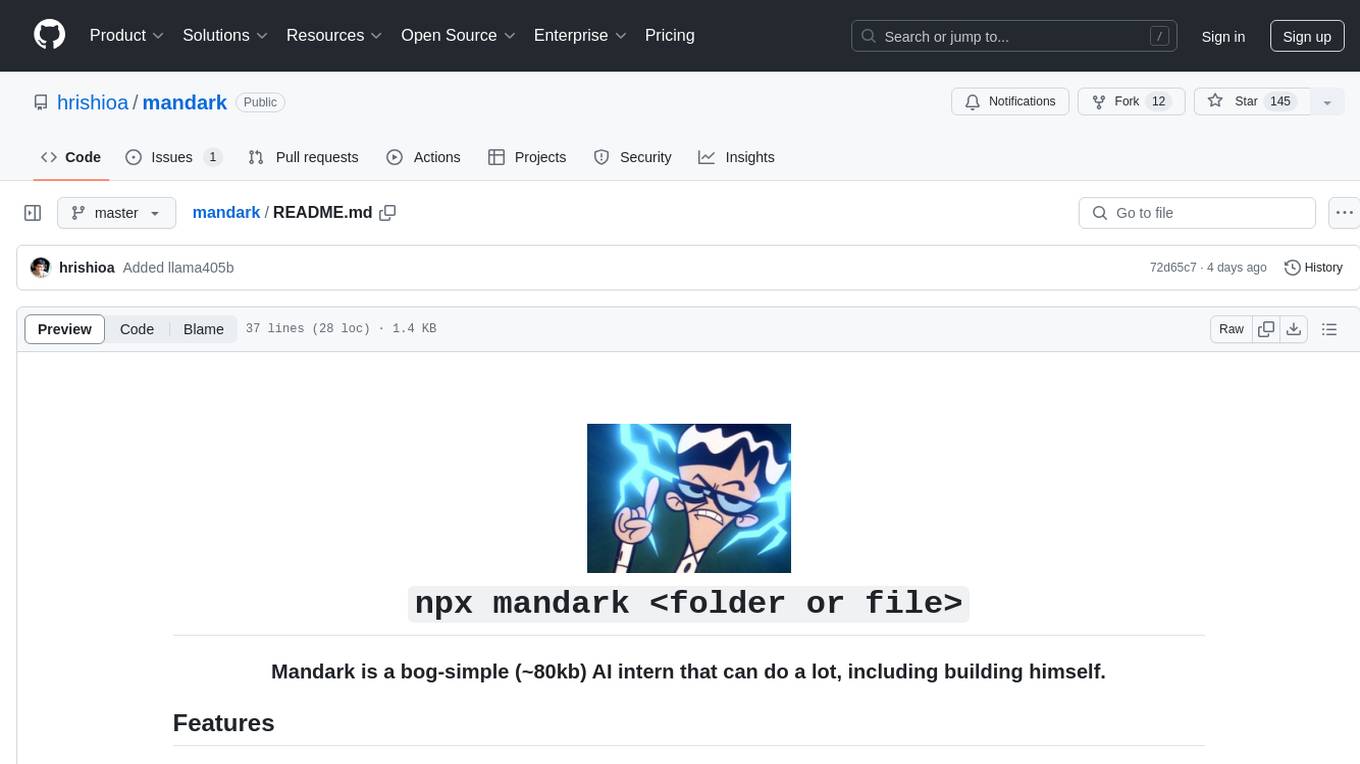
mandark
Mandark is a lightweight AI tool that can perform various tasks, such as answering questions about codebases, editing files, verifying diffs, estimating token and cost before execution, and working with any codebase. It supports multiple AI models like Claude-3.5 Sonnet, Haiku, GPT-4o-mini, and GPT-4-turbo. Users can run Mandark without installation and easily interact with it through command line options. It offers flexibility in processing individual files or folders and allows for customization with optional AI model selection and output preferences.
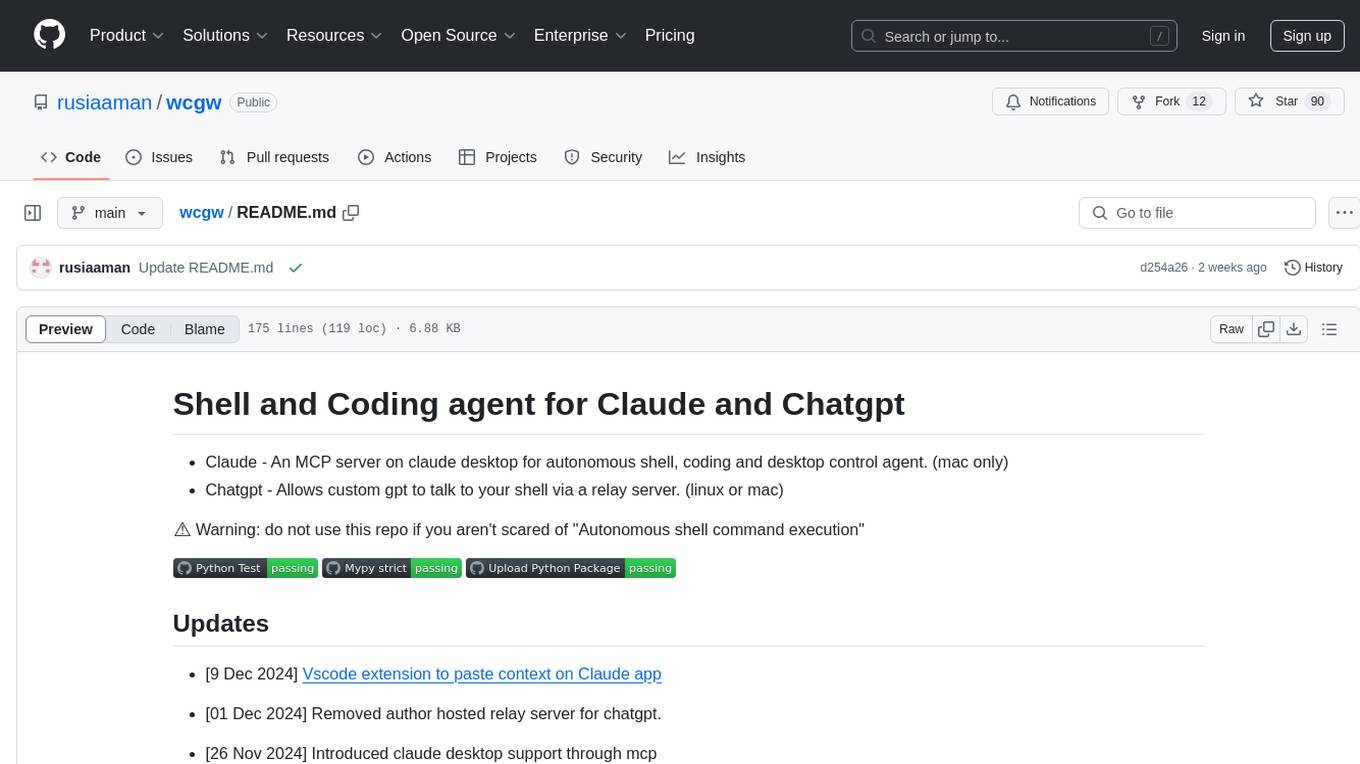
wcgw
wcgw is a shell and coding agent designed for Claude and Chatgpt. It provides full shell access with no restrictions, desktop control on Claude for screen capture and control, interactive command handling, large file editing, and REPL support. Users can use wcgw to create, execute, and iterate on tasks, such as solving problems with Python, finding code instances, setting up projects, creating web apps, editing large files, and running server commands. Additionally, wcgw supports computer use on Docker containers for desktop control. The tool can be extended with a VS Code extension for pasting context on Claude app and integrates with Chatgpt for custom GPT interactions.
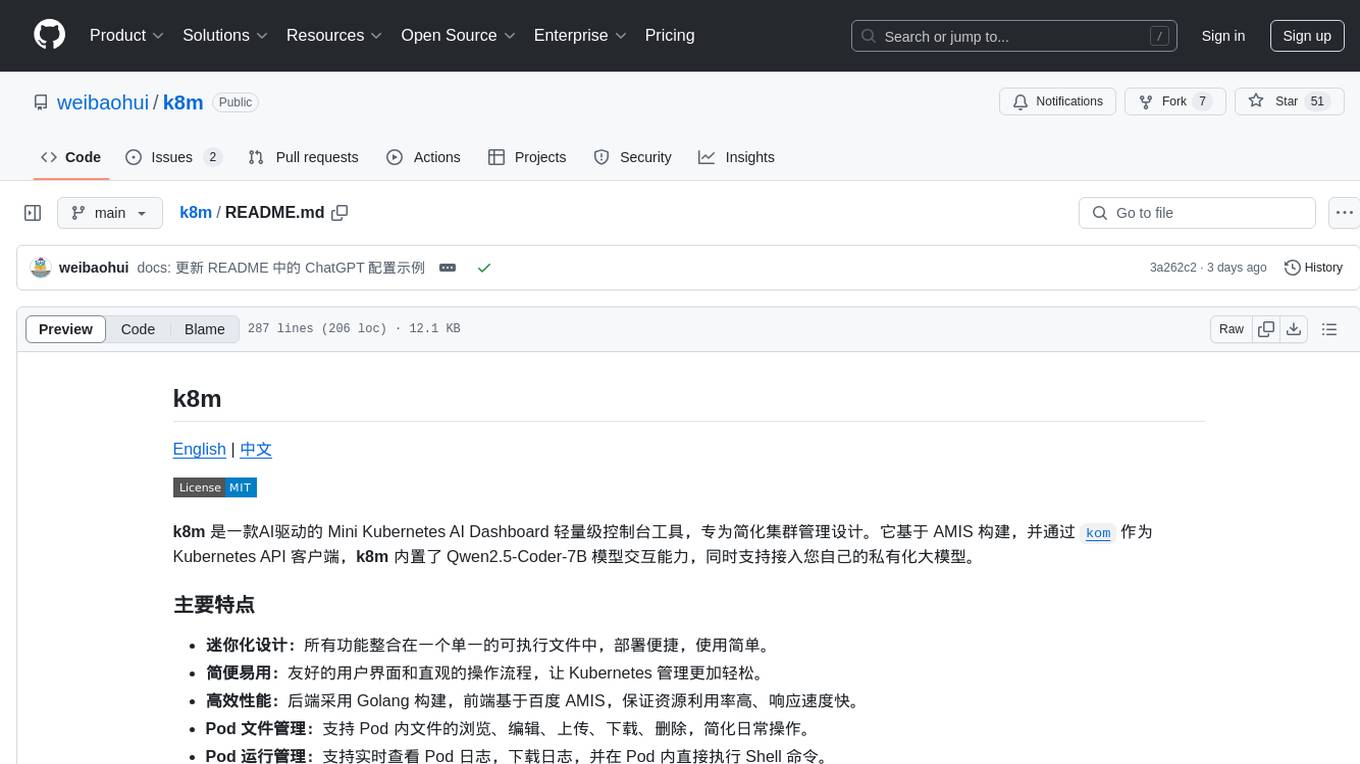
k8m
k8m is an AI-driven Mini Kubernetes AI Dashboard lightweight console tool designed to simplify cluster management. It is built on AMIS and uses 'kom' as the Kubernetes API client. k8m has built-in Qwen2.5-Coder-7B model interaction capabilities and supports integration with your own private large models. Its key features include miniaturized design for easy deployment, user-friendly interface for intuitive operation, efficient performance with backend in Golang and frontend based on Baidu AMIS, pod file management for browsing, editing, uploading, downloading, and deleting files, pod runtime management for real-time log viewing, log downloading, and executing shell commands within pods, CRD management for automatic discovery and management of CRD resources, and intelligent translation and diagnosis based on ChatGPT for YAML property translation, Describe information interpretation, AI log diagnosis, and command recommendations, providing intelligent support for managing k8s. It is cross-platform compatible with Linux, macOS, and Windows, supporting multiple architectures like x86 and ARM for seamless operation. k8m's design philosophy is 'AI-driven, lightweight and efficient, simplifying complexity,' helping developers and operators quickly get started and easily manage Kubernetes clusters.
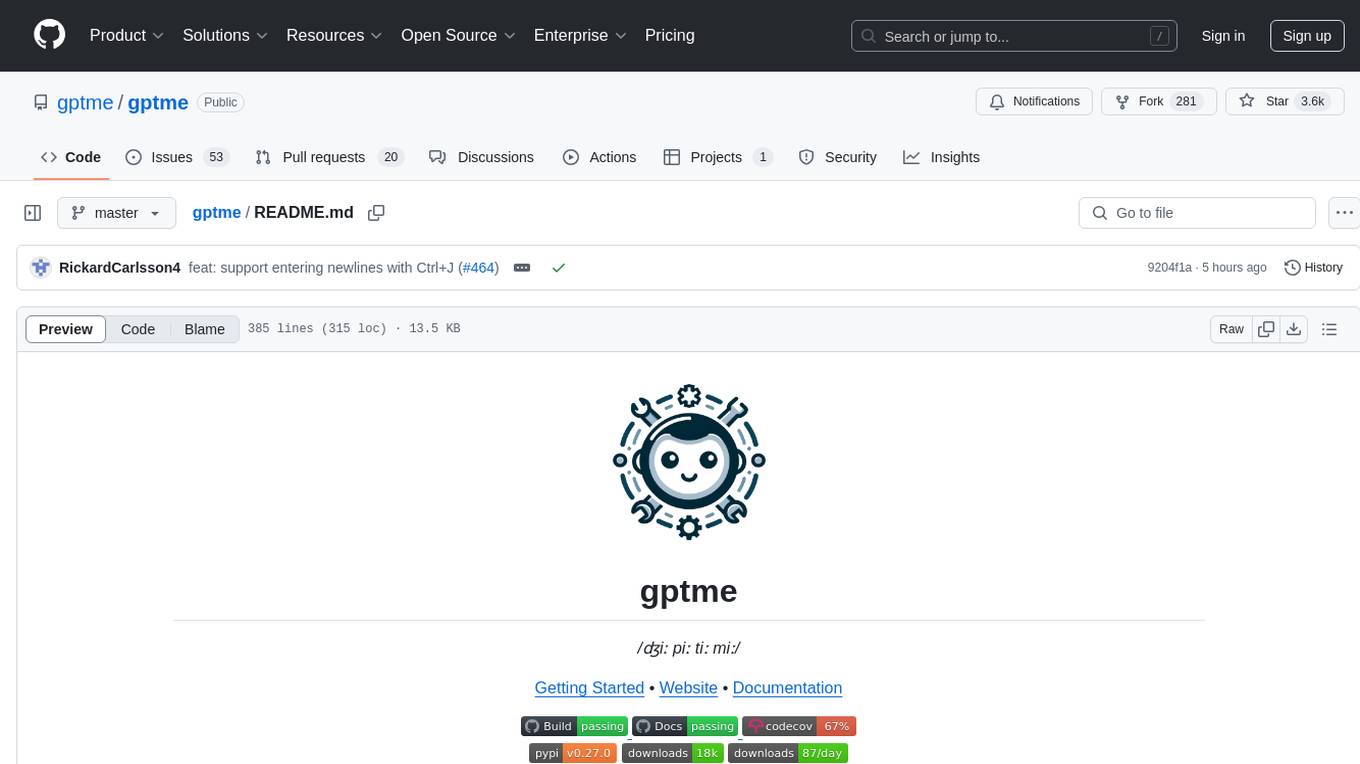
gptme
Personal AI assistant/agent in your terminal, with tools for using the terminal, running code, editing files, browsing the web, using vision, and more. A great coding agent that is general-purpose to assist in all kinds of knowledge work, from a simple but powerful CLI. An unconstrained local alternative to ChatGPT with 'Code Interpreter', Cursor Agent, etc. Not limited by lack of software, internet access, timeouts, or privacy concerns if using local models.
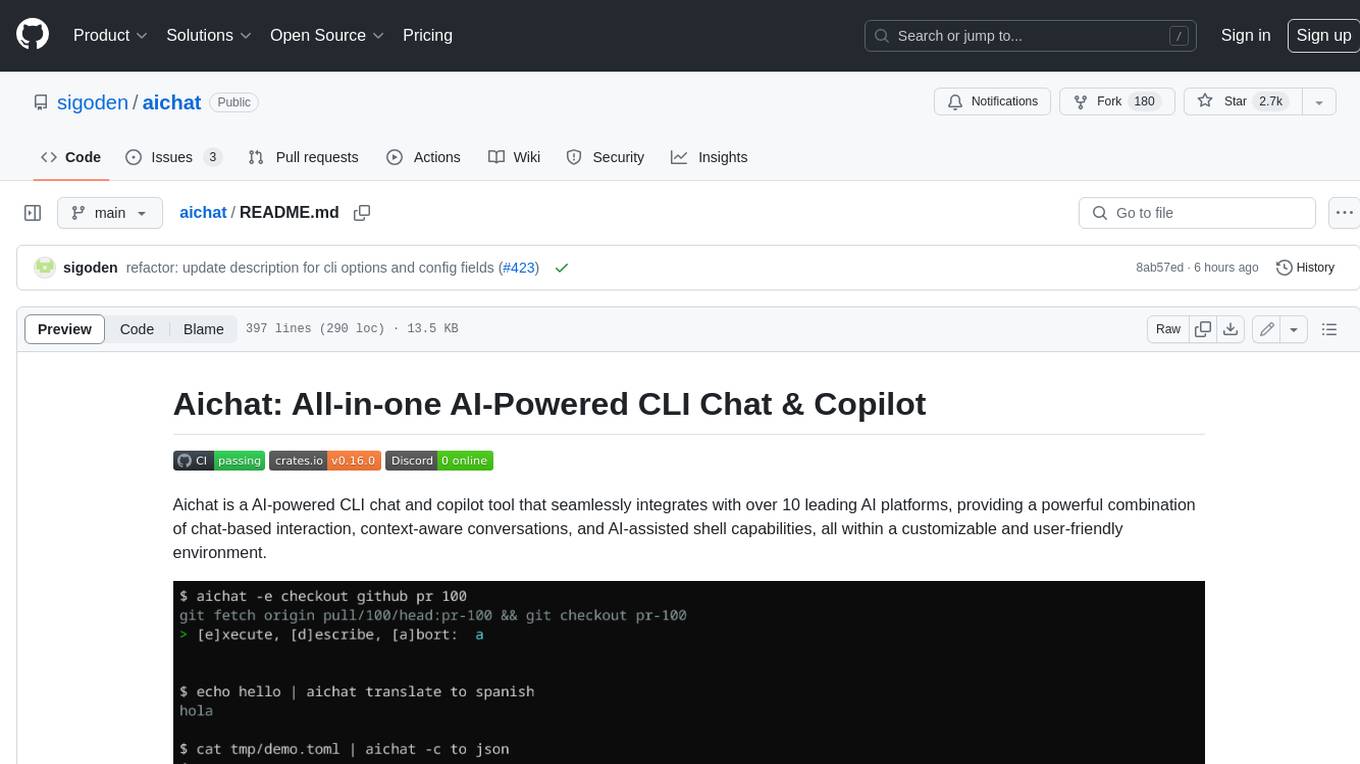
aichat
Aichat is an AI-powered CLI chat and copilot tool that seamlessly integrates with over 10 leading AI platforms, providing a powerful combination of chat-based interaction, context-aware conversations, and AI-assisted shell capabilities, all within a customizable and user-friendly environment.
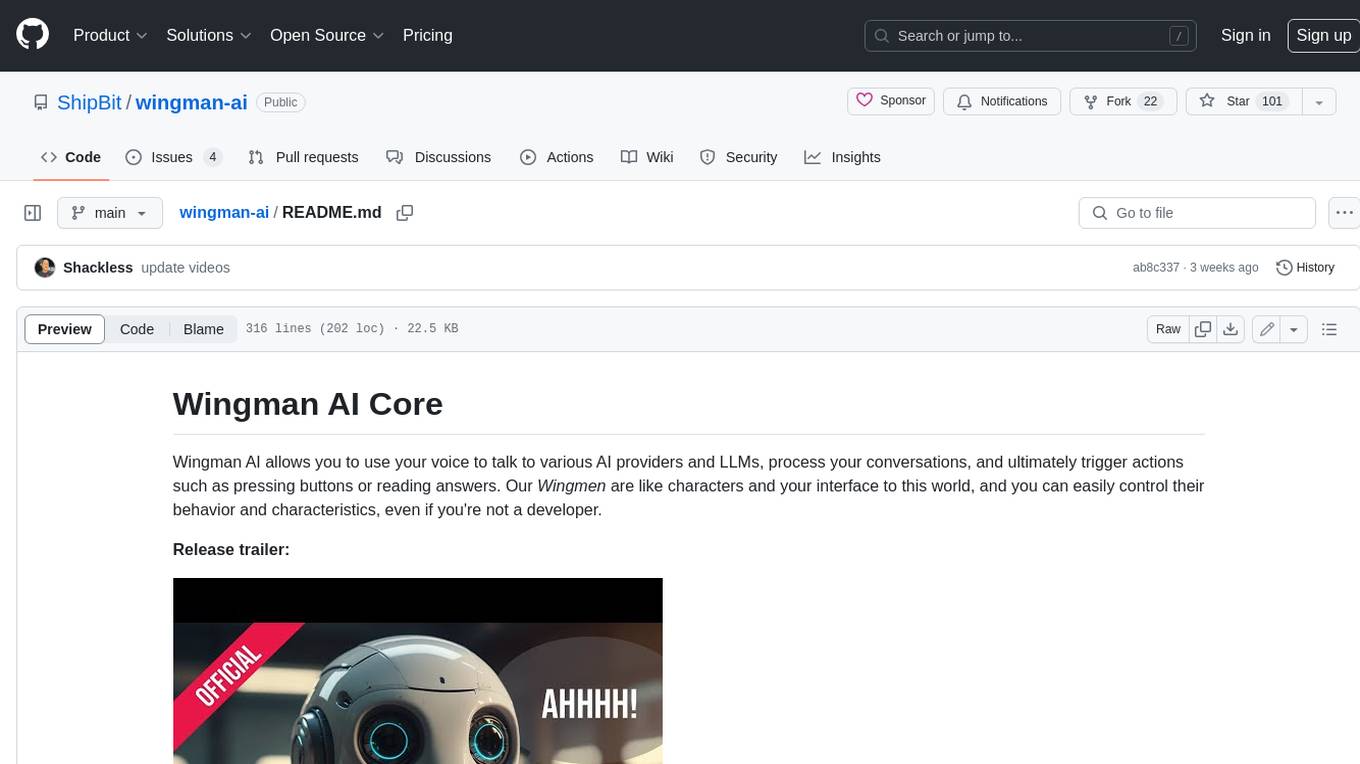
wingman-ai
Wingman AI allows you to use your voice to talk to various AI providers and LLMs, process your conversations, and ultimately trigger actions such as pressing buttons or reading answers. Our _Wingmen_ are like characters and your interface to this world, and you can easily control their behavior and characteristics, even if you're not a developer. AI is complex and it scares people. It's also **not just ChatGPT**. We want to make it as easy as possible for you to get started. That's what _Wingman AI_ is all about. It's a **framework** that allows you to build your own Wingmen and use them in your games and programs. The idea is simple, but the possibilities are endless. For example, you could: * **Role play** with an AI while playing for more immersion. Have air traffic control (ATC) in _Star Citizen_ or _Flight Simulator_. Talk to Shadowheart in Baldur's Gate 3 and have her respond in her own (cloned) voice. * Get live data such as trade information, build guides, or wiki content and have it read to you in-game by a _character_ and voice you control. * Execute keystrokes in games/applications and create complex macros. Trigger them in natural conversations with **no need for exact phrases.** The AI understands the context of your dialog and is quite _smart_ in recognizing your intent. Say _"It's raining! I can't see a thing!"_ and have it trigger a command you simply named _WipeVisors_. * Automate tasks on your computer * improve accessibility * ... and much more
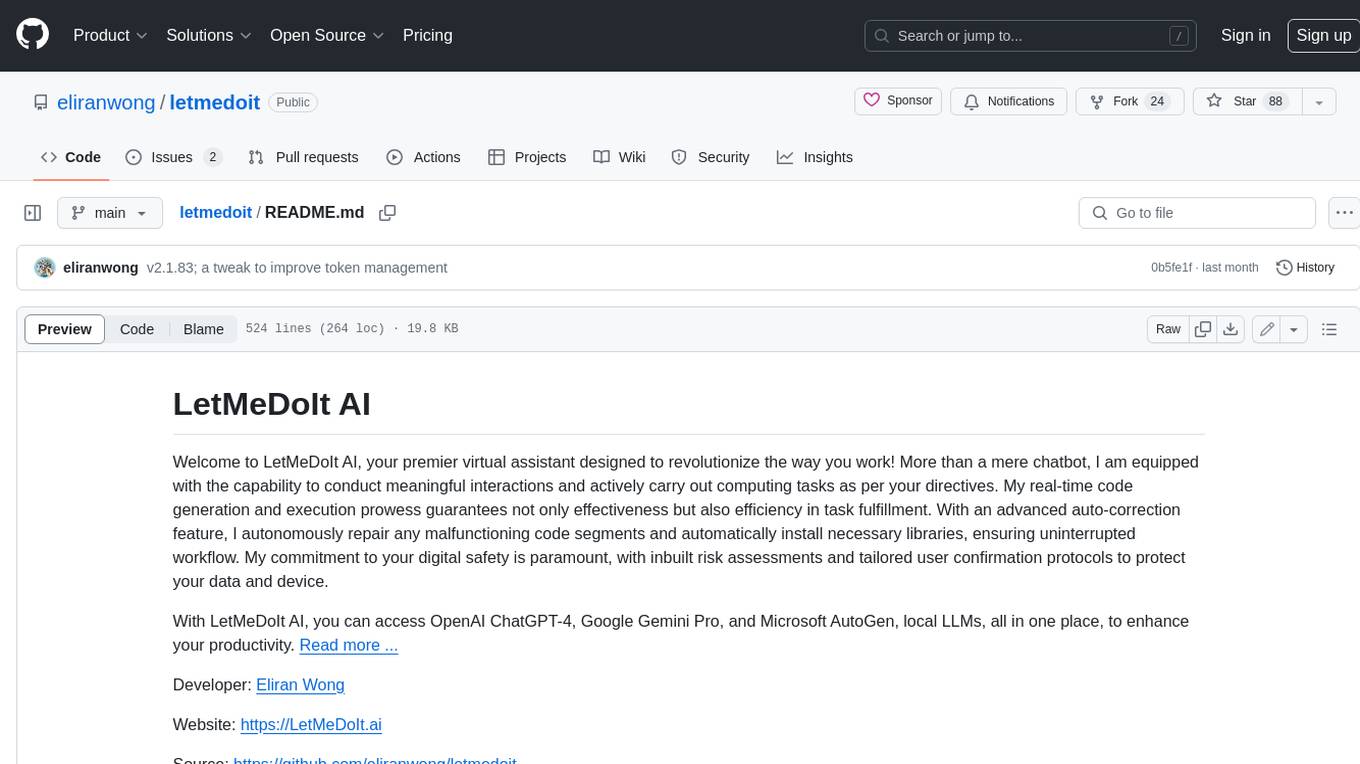
letmedoit
LetMeDoIt AI is a virtual assistant designed to revolutionize the way you work. It goes beyond being a mere chatbot by offering a unique and powerful capability - the ability to execute commands and perform computing tasks on your behalf. With LetMeDoIt AI, you can access OpenAI ChatGPT-4, Google Gemini Pro, and Microsoft AutoGen, local LLMs, all in one place, to enhance your productivity.
For similar jobs
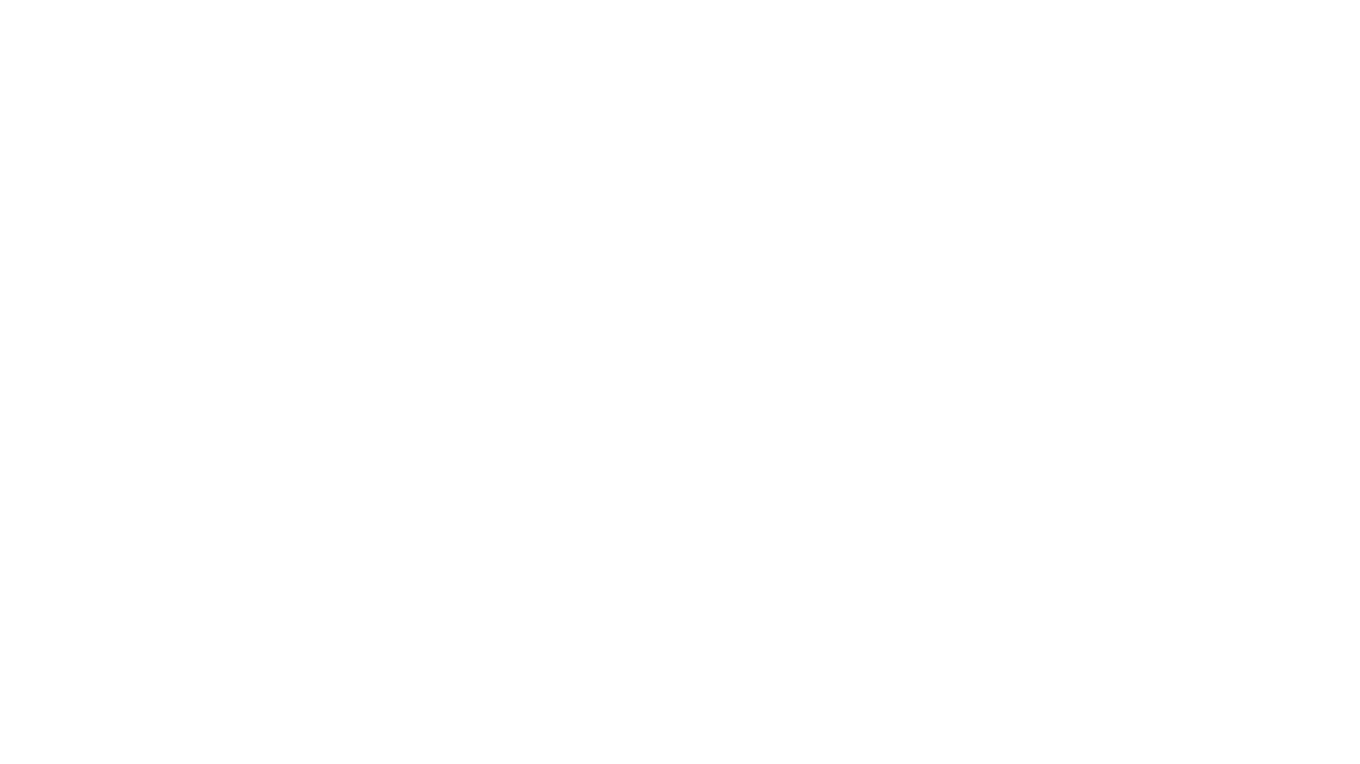
AirGo
AirGo is a front and rear end separation, multi user, multi protocol proxy service management system, simple and easy to use. It supports vless, vmess, shadowsocks, and hysteria2.
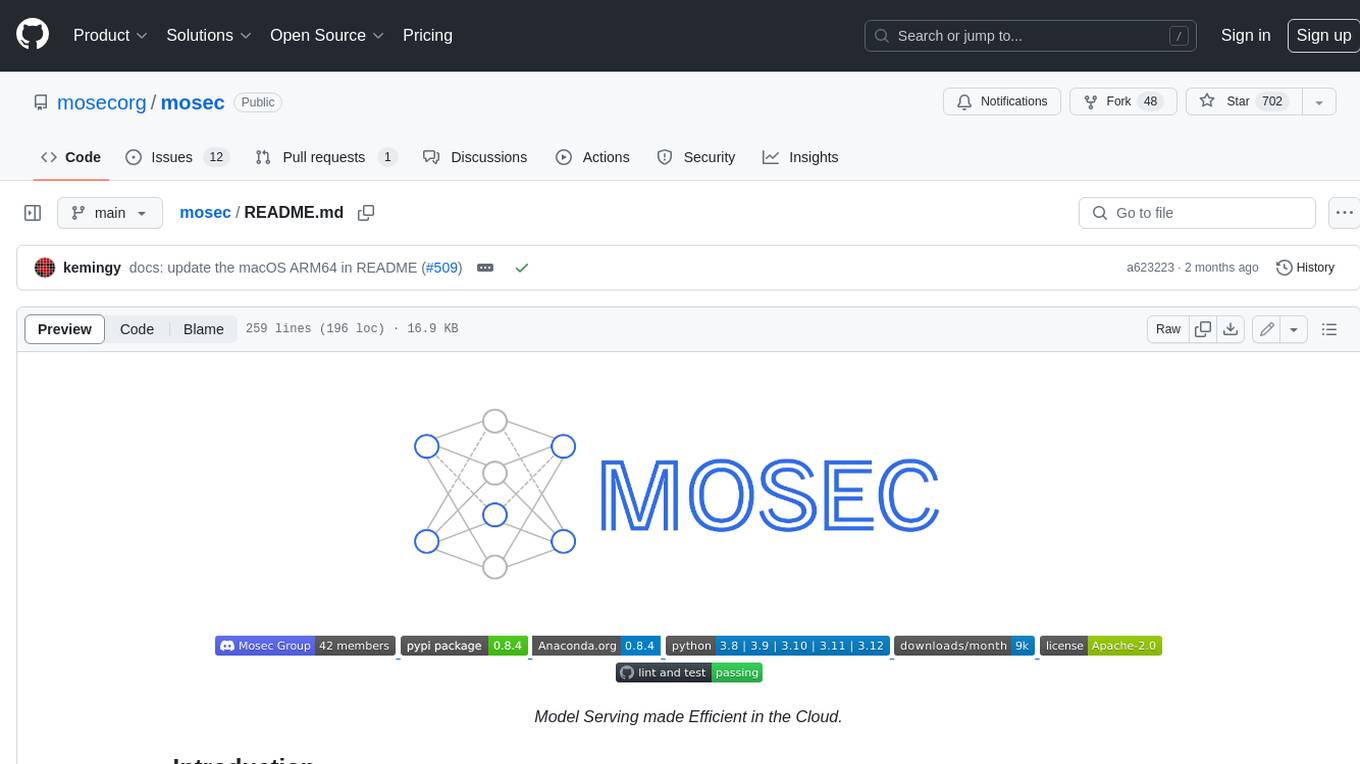
mosec
Mosec is a high-performance and flexible model serving framework for building ML model-enabled backend and microservices. It bridges the gap between any machine learning models you just trained and the efficient online service API. * **Highly performant** : web layer and task coordination built with Rust 🦀, which offers blazing speed in addition to efficient CPU utilization powered by async I/O * **Ease of use** : user interface purely in Python 🐍, by which users can serve their models in an ML framework-agnostic manner using the same code as they do for offline testing * **Dynamic batching** : aggregate requests from different users for batched inference and distribute results back * **Pipelined stages** : spawn multiple processes for pipelined stages to handle CPU/GPU/IO mixed workloads * **Cloud friendly** : designed to run in the cloud, with the model warmup, graceful shutdown, and Prometheus monitoring metrics, easily managed by Kubernetes or any container orchestration systems * **Do one thing well** : focus on the online serving part, users can pay attention to the model optimization and business logic
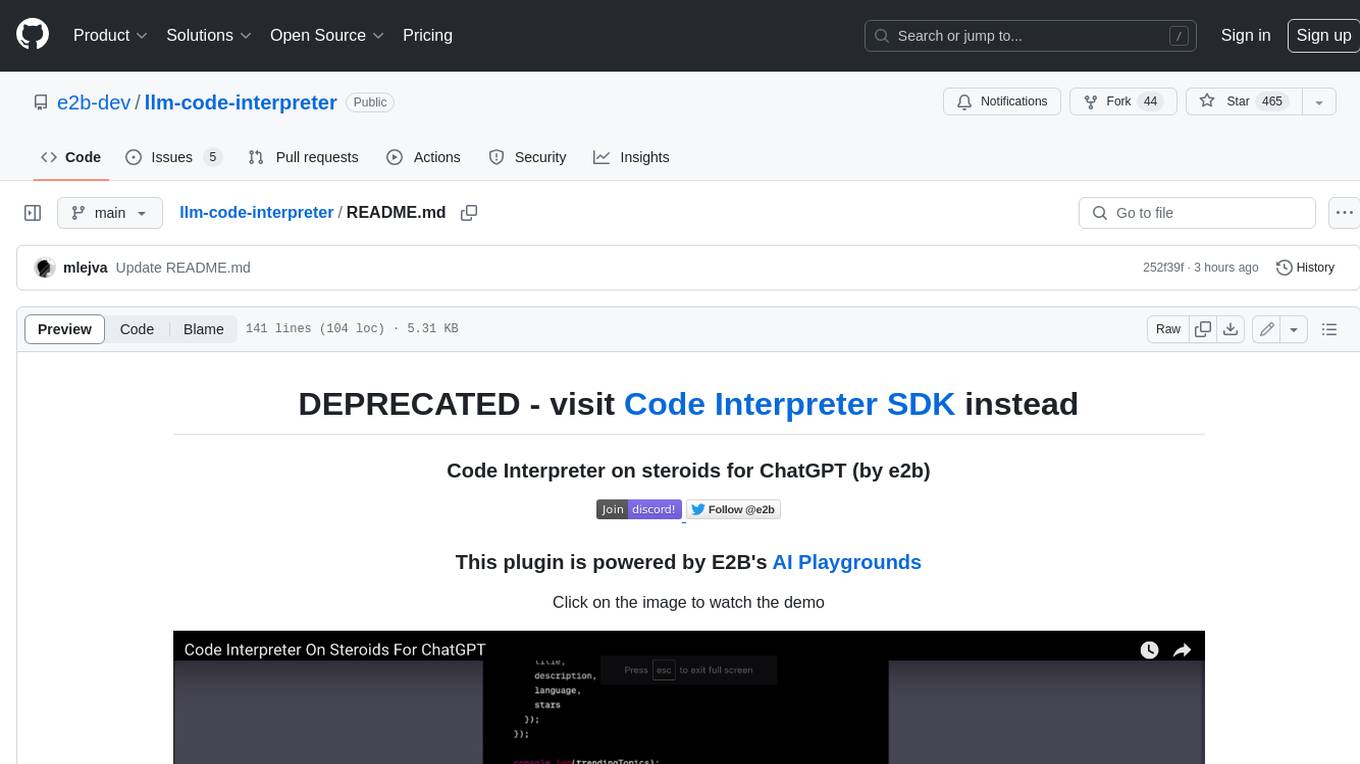
llm-code-interpreter
The 'llm-code-interpreter' repository is a deprecated plugin that provides a code interpreter on steroids for ChatGPT by E2B. It gives ChatGPT access to a sandboxed cloud environment with capabilities like running any code, accessing Linux OS, installing programs, using filesystem, running processes, and accessing the internet. The plugin exposes commands to run shell commands, read files, and write files, enabling various possibilities such as running different languages, installing programs, starting servers, deploying websites, and more. It is powered by the E2B API and is designed for agents to freely experiment within a sandboxed environment.
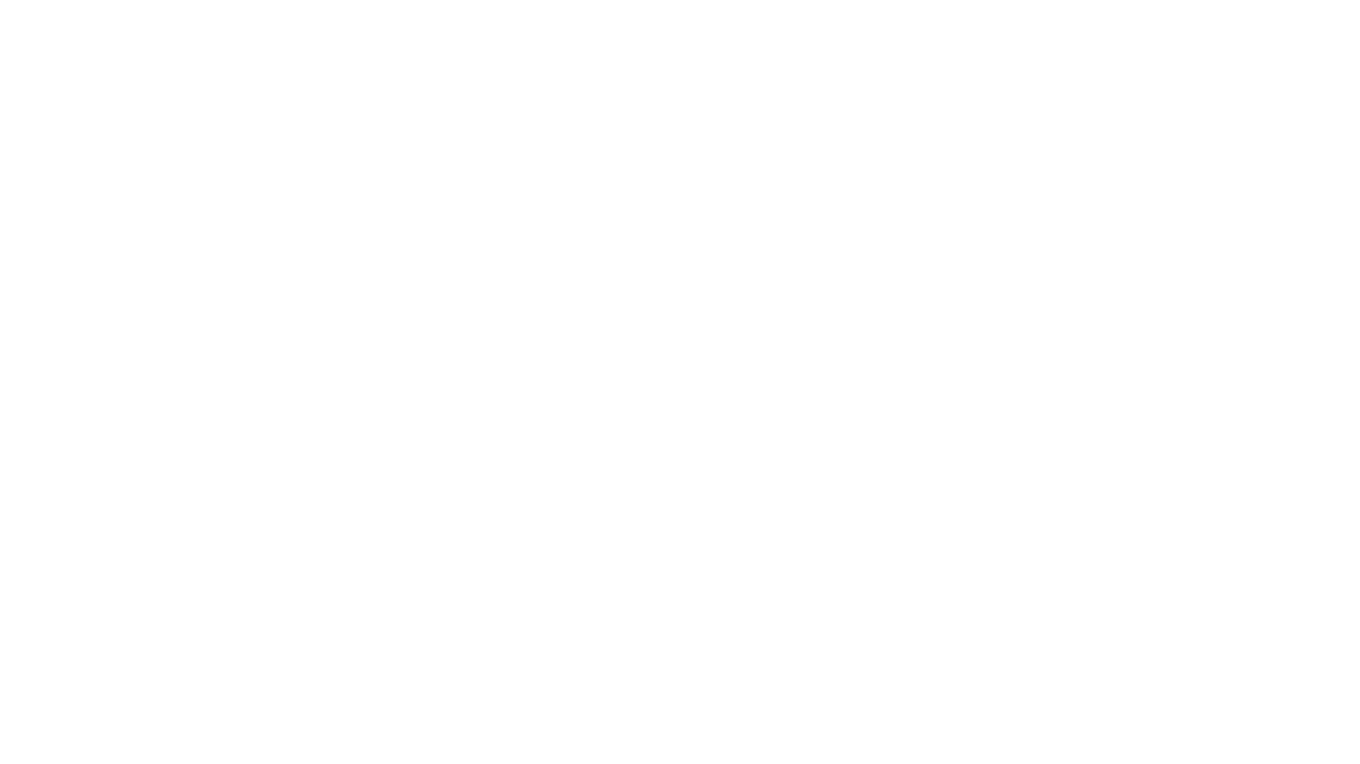
pezzo
Pezzo is a fully cloud-native and open-source LLMOps platform that allows users to observe and monitor AI operations, troubleshoot issues, save costs and latency, collaborate, manage prompts, and deliver AI changes instantly. It supports various clients for prompt management, observability, and caching. Users can run the full Pezzo stack locally using Docker Compose, with prerequisites including Node.js 18+, Docker, and a GraphQL Language Feature Support VSCode Extension. Contributions are welcome, and the source code is available under the Apache 2.0 License.
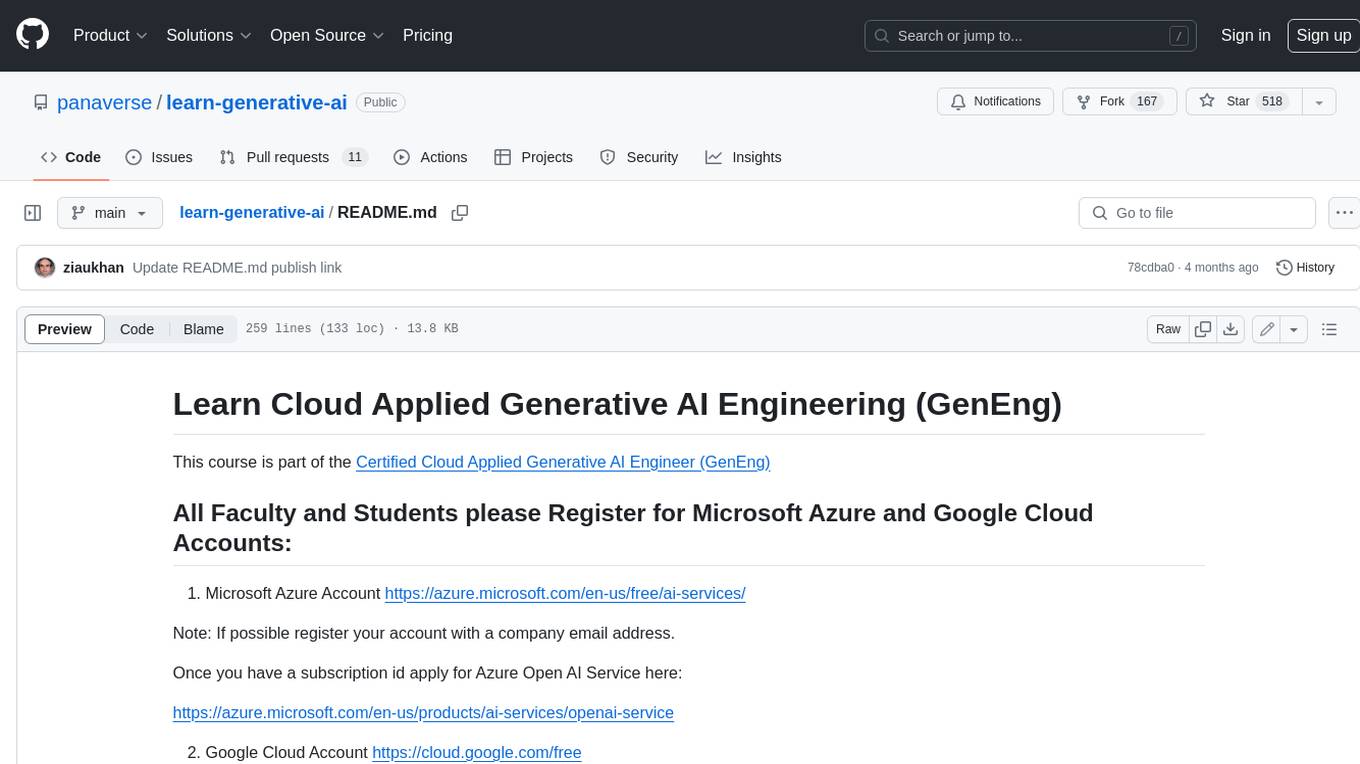
learn-generative-ai
Learn Cloud Applied Generative AI Engineering (GenEng) is a course focusing on the application of generative AI technologies in various industries. The course covers topics such as the economic impact of generative AI, the role of developers in adopting and integrating generative AI technologies, and the future trends in generative AI. Students will learn about tools like OpenAI API, LangChain, and Pinecone, and how to build and deploy Large Language Models (LLMs) for different applications. The course also explores the convergence of generative AI with Web 3.0 and its potential implications for decentralized intelligence.
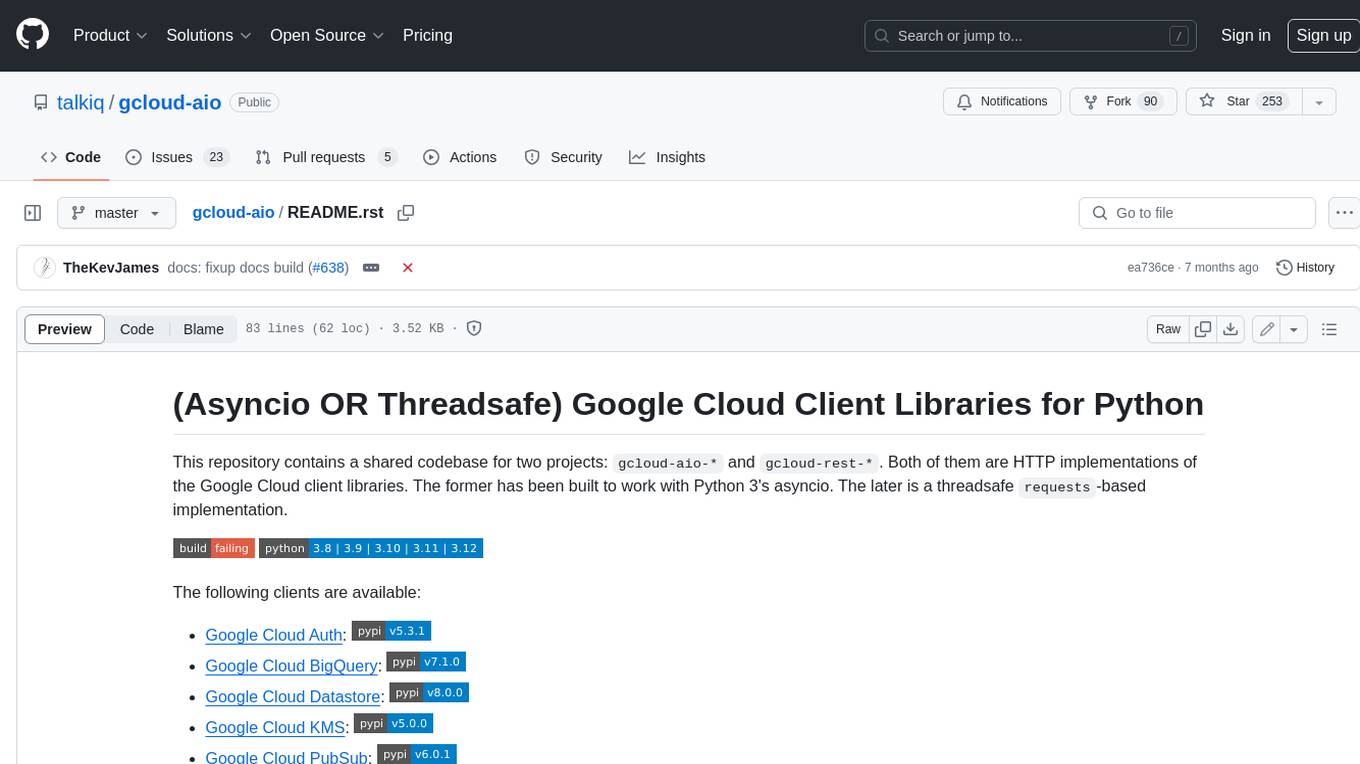
gcloud-aio
This repository contains shared codebase for two projects: gcloud-aio and gcloud-rest. gcloud-aio is built for Python 3's asyncio, while gcloud-rest is a threadsafe requests-based implementation. It provides clients for Google Cloud services like Auth, BigQuery, Datastore, KMS, PubSub, Storage, and Task Queue. Users can install the library using pip and refer to the documentation for usage details. Developers can contribute to the project by following the contribution guide.
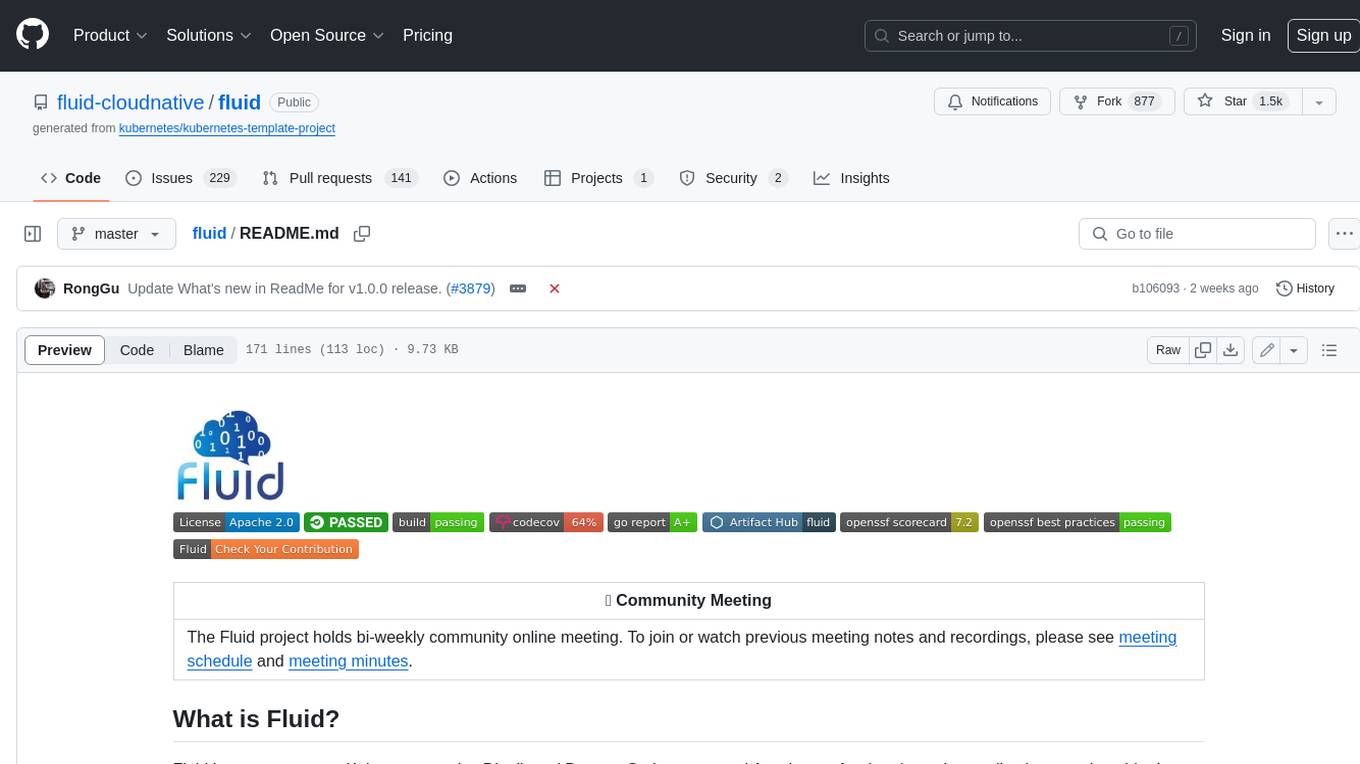
fluid
Fluid is an open source Kubernetes-native Distributed Dataset Orchestrator and Accelerator for data-intensive applications, such as big data and AI applications. It implements dataset abstraction, scalable cache runtime, automated data operations, elasticity and scheduling, and is runtime platform agnostic. Key concepts include Dataset and Runtime. Prerequisites include Kubernetes version > 1.16, Golang 1.18+, and Helm 3. The tool offers features like accelerating remote file accessing, machine learning, accelerating PVC, preloading dataset, and on-the-fly dataset cache scaling. Contributions are welcomed, and the project is under the Apache 2.0 license with a vendor-neutral approach.
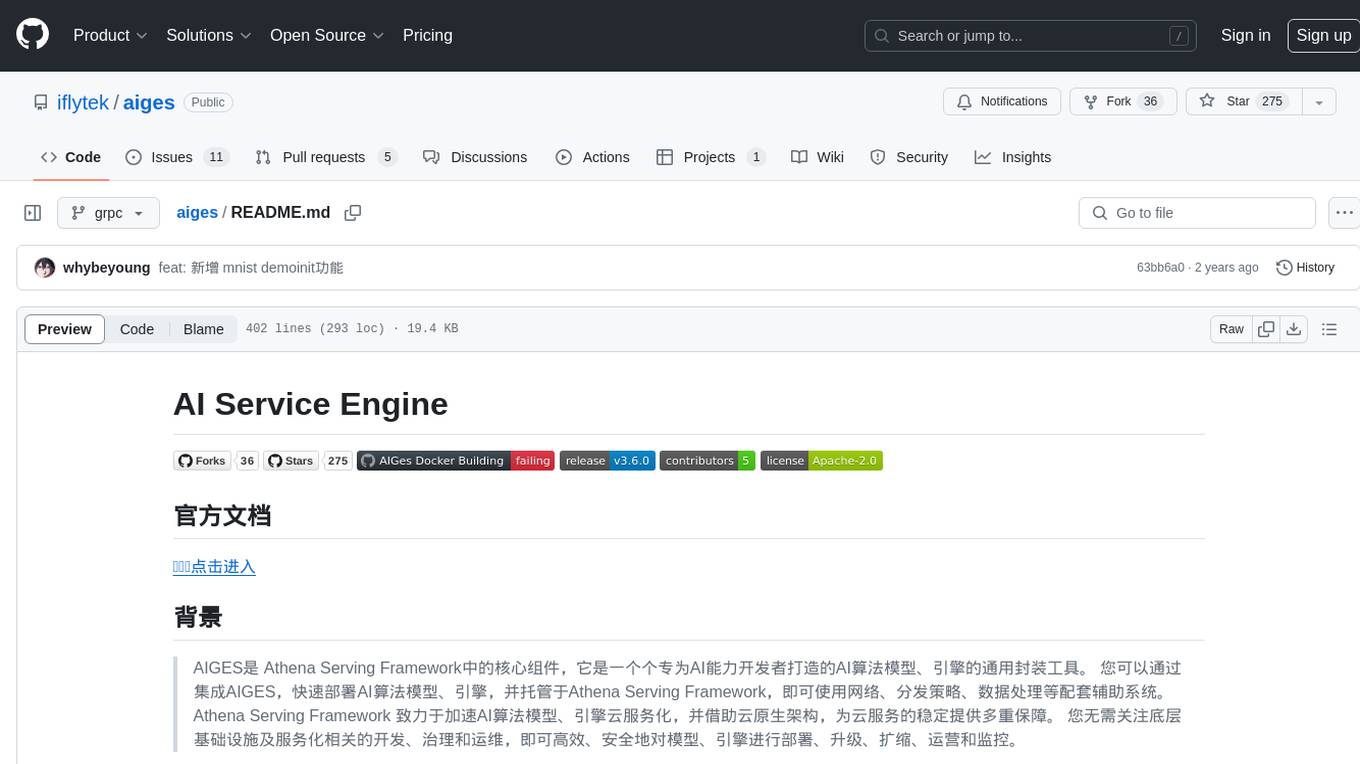
aiges
AIGES is a core component of the Athena Serving Framework, designed as a universal encapsulation tool for AI developers to deploy AI algorithm models and engines quickly. By integrating AIGES, you can deploy AI algorithm models and engines rapidly and host them on the Athena Serving Framework, utilizing supporting auxiliary systems for networking, distribution strategies, data processing, etc. The Athena Serving Framework aims to accelerate the cloud service of AI algorithm models and engines, providing multiple guarantees for cloud service stability through cloud-native architecture. You can efficiently and securely deploy, upgrade, scale, operate, and monitor models and engines without focusing on underlying infrastructure and service-related development, governance, and operations.