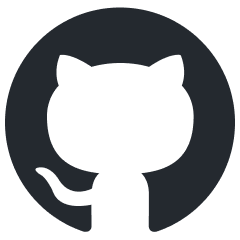
hf-waitress
Serving LLMs in the HF-Transformers format via a PyFlask API
Stars: 64
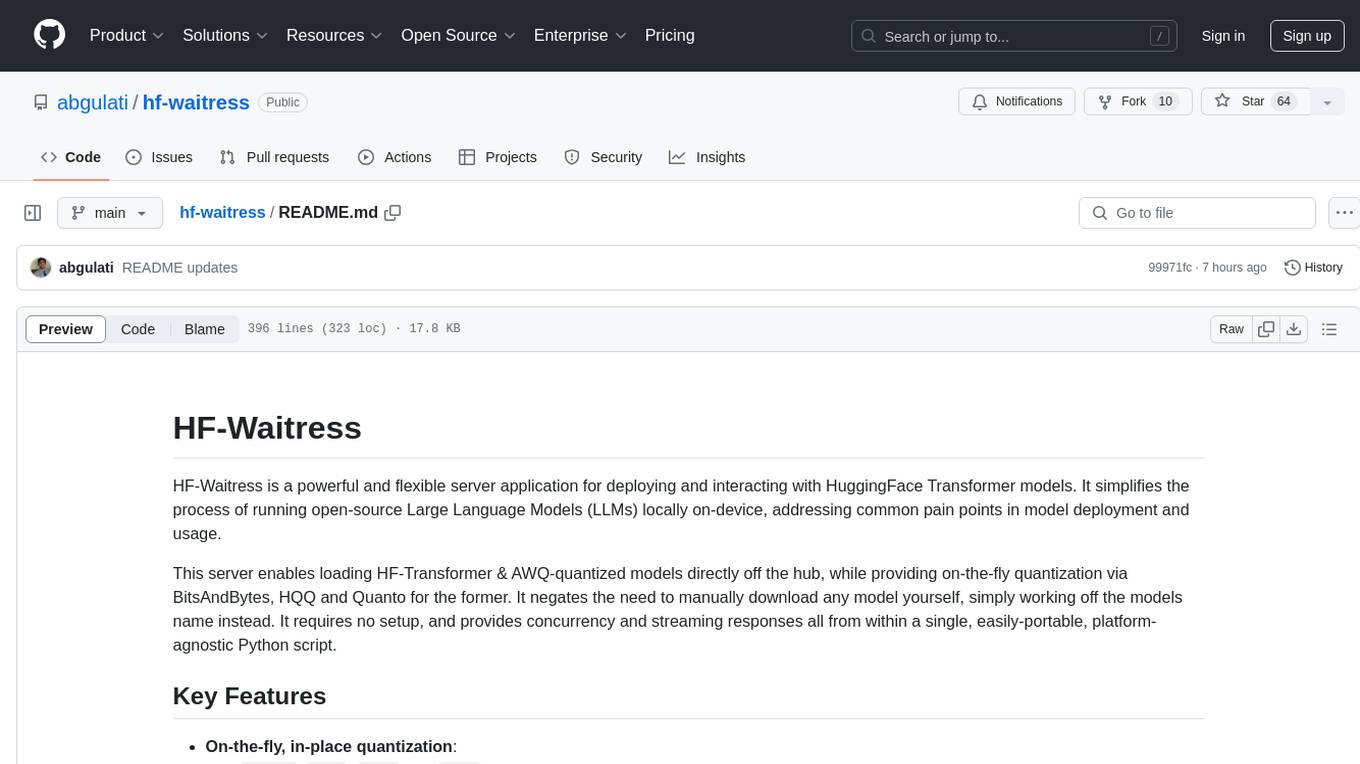
HF-Waitress is a powerful server application for deploying and interacting with HuggingFace Transformer models. It simplifies running open-source Large Language Models (LLMs) locally on-device, providing on-the-fly quantization via BitsAndBytes, HQQ, and Quanto. It requires no manual model downloads, offers concurrency, streaming responses, and supports various hardware and platforms. The server uses a `config.json` file for easy configuration management and provides detailed error handling and logging.
README:
HF-Waitress is a powerful and flexible server application for deploying and interacting with HuggingFace Transformer models. It simplifies the process of running open-source Large Language Models (LLMs) locally on-device, addressing common pain points in model deployment and usage.
This server enables loading HF-Transformer & AWQ-quantized models directly off the hub, while providing on-the-fly quantization via BitsAndBytes, HQQ and Quanto for the former. It negates the need to manually download any model yourself, simply working off the models name instead. It requires no setup, and provides concurrency and streaming responses all from within a single, easily-portable, platform-agnostic Python script.
-
On-the-fly, in-place quantization:
-
Quanto
int8
,int4
andint2
quantization for all hardware, -
BitsAndBytes
int8
&int4
quantization for Nvidia GPUs, -
HQQ
int8
,int4
,int3
,int2
,int1
quantization for Nvidia & AMD GPUs.
-
- Activation-Aware Quantization (AWQ) Support: load AWQ-quantized models from HF-Hub.
- Model Agnosticism: Compatible with any HF-Transformers format LLM.
-
Configuration Management: Uses
config.json
to store settings, allowing for easy configuration and persistence across runs. - Hardware & Platform Agnostic: Run native or quantized models on all manner of CPUs, GPUs and Apple M-silicon across Windows, Linux, MacOS & Docker containers.
- Error Handling: Detailed logging and traceback reporting via centralized error-handling functions.
- Health Endpoint: Provides valuable information about the loaded model and server health.
- Concurrency Control: Uses semaphores for selective concurrency while taking advantage of semaphore-native queueing.
- Streaming Responses: Supports both standard and streaming completions.
- HF-Waitress Introduction
- Dependencies
- Installation
- Usage
- API Endpoints
- Configuration - hf_config.json
- Error Handling and Logging
- Contributing
- Support and Donations
-
Python v3.10.x or above
-
PyTorch:
If you're planning to use your GPU to run LLMs, make sure to install the GPU drivers and CUDA/ROCm toolkits as appropriate for your setup, and only then proceed with PyTorch setup below
Download and install the PyTorch version appropriate for your system: https://pytorch.org/get-started/locally/
-
(optional) If attempting to use Flash Attention 2, specific Nvidia GPUs are required. Check the official-repo for requirements and installation instructions.
- NOTE: Ensure Dependencies are installed!
-
Clone this repository:
git clone https://github.com/abgulati/hf-waitress cd hf-waitress
-
Install the required dependencies:
pip install -r requirements.txt
To start the server, run: python hf_waitress.py [arguments]
Example:
python hf_waitress.py --model_id=mistralai/Mistral-Nemo-Instruct-2407 --quantize=quanto --quant_level=int4 --access_token=<token> --trust_remote_code --use_flash_attention_2 --do_sample
launch-arguments are optional, even on the first run! See below for defaults.
-
--model_id
: The model ID in HF-Transformers format - see below for details. -
--access_gated
: Set to True if accessing gated models you're approved for. -
--access_token
: Your Hugging Face Access Token. -
--gguf
: Add this flag if attempting to load a GGUF model - For future use, not presently functional -
--gguf_model_id
: GGUF repository ID - For future use, not presently functional -
--gguf_filename
: Specific GGUF filename - For future use, not presently functional -
--quantize
: Quantization method ('bitsandbytes', 'quanto', 'hqq' or 'n' for none, see important details below.). -
--quant_level
: Quantization level (Valid values - BitsAndBytes: int8 & int4; Quanto: int8, int4 and int2; HQQ: int8, int4, int3, int2, int1). -
--hqq_group_size
: Specify group_size (default: 64) for HQQ quantization. No restrictions as long as weight.numel() is divisible by the group_size. -
--push_to_hub
: Push quantized model to Hugging Face Hub. -
--torch_device_map
: Specify inference device (e.g., 'cuda', 'cpu'). -
--torch_dtype
: Specify model tensor type. -
--trust_remote_code
: Allow execution of custom code from the model's repository. -
--use_flash_attention_2
: Attempt to use Flash Attention 2 - Only for specific Nvidia GPUs -
--pipeline_task
: Specify the pipeline task (default: 'text-generation'). -
--max_new_tokens
: Maximum number of tokens to generate. -
--return_full_text
: Return the full text including the prompt. -
--temperature
: Set LLM temperature (0.0 to 2.0) - set do_sample to True for temps above 0.0, and False when setting temperature=0.0! -
--do_sample
: Perform sampling when selecting response tokens - must be set to True for temps above 0.0! -
--top_k
,--top_p
,--min_p
: Token selection parameters - must set do_sample to True! -
--port
: Specify the server port (default: 9069). -
--reset_to_defaults
: Reset all settings to default values.
{
'access_gated':False,
'access_token':"",
'model_id':"microsoft/Phi-3-mini-4k-instruct",
'gguf':False,
'awq':False,
'gguf_model_id':None,
'gguf_filename':None,
'quantize':"quanto",
'quant_level':"int4",
'hqq_group_size':64,
'push_to_hub':False,
'torch_device_map':"auto",
'torch_dtype':"auto",
'trust_remote_code':False,
'use_flash_attention_2':False,
'pipeline_task':"text-generation",
'max_new_tokens':500,
'return_full_text':False,
'temperature':0.0,
'do_sample':False,
'top_k':40,
'top_p':0.95,
'min_p':0.05,
'n_keep':0,
'port':9069
}
The required model_id
can typically be obtained one of two ways, both of which involve going to the model's HuggingFace.co page:
- Simply make use of the copy function provided by HuggingFace:
- Or, scroll further down the model card and copy the model_id from the code sample provided by the model creators:
-
Several Quantization methods are available in HF-Waitress: BitsAndBytes, Quanto and HQQ, alongside the ability to run HF-Transformer and AWQ models directly off the HF-Hub
-
BitsAndBytes:
- Requires: Nvidia CUDA-supported GPU
- Supported Quantization Levels: int8 and int4
- Recommended quant technique for Nvidia GPU owners as this is the best and fastest quantization method available.
-
Quanto:
- Native PyTorch Quantization technique - versatile pytorch quantization toolkit.
- The underlying method used is linear quantization.
- Supports: CPU, GPU, Apple Silicon
- Supported Quantization Levels: int8, int4 and int2
- NOTE: At load time, the model will report a high memory footprint but actual memory-usage will be significantly lower.
-
HQQ:
- Half-Quadratic Quantization (HQQ) implements on-the-fly quantization via fast robust optimization. It doesn’t require calibration data and can be used to quantize any model.
- Supports: CPU, NvCUDA GPU
- Supported Quantization Levels: int8, int4, int3, int2 and int1
-
AWQ:
- Activation-aware Weight Quantization (AWQ) doesn’t quantize all the weights in a model, and instead preserves a small percentage of weights that are important for LLM performance.
- This significantly reduces quantization loss such that you can run models in 4-bit precision without experiencing any performance degradation.
- Supports: GPUs - Nvidia CUDA and AMD ROCm compliant GPUs
- See section below for running these models
-
Check the official HF-docs for more details and hardware-support matrix.
-
There are several libraries for quantizing models with the AWQ algorithm, such as llm-awq, autoawq or optimum-intel.
-
Transformers ONLY supports loading models quantized with the llm-awq and autoawq libraries
-
For models quantized with
autoawq
, install the AutoAWQ PIP package:pip install autoawq
-
NOTE: As of this writing, AutoAWQ requires Torch 2.3.x. If you have another version of Torch already installed (such as for CUDA-12.4 etc), you can try to run the above with "--no-deps":
pip install --no-deps autoawq
. in my testing, AWQ models work fine this way, but YMMV. -
To run models on the HuggingFace-Hub in the AWQ format, simply specify the model_id and set the
--awq
flag at launch:python .\hf_waitress.py --awq --model_id=hugging-quants/Meta-Llama-3.1-8B-Instruct-AWQ-INT4
-
This will auto-set
quantize=n
&torch_dtype=torch.float16
without overwriting their values inhf_config.json
-
/completions
(POST): Generate completions for given messages. -
/completions_stream
(POST): Stream completions for given messages. -
/health
(GET): Check the health and get information about the loaded model. -
/hf_config_reader_api
(POST): Read values from the configuration. -
/hf_config_writer_api
(POST): Write values to the configuration. -
/restart_server
(GET): Restart the LLM server.
-
/completions
(POST): Generate completions for given messages.-
Headers:
-
Content-Type: application/json
-
X-Max-New-Tokens
: Maximum number of tokens to generate -
X-Return-Full-Text
: Whether to return the full text including the prompt -
X-Temperature
: Temperature for text generation (0.0 to 2.0) -
X-Do-Sample
: Whether to use sampling for text generation -
X-Top-K
: Top-K sampling parameter -
X-Top-P
: Top-P (nucleus) sampling parameter -
X-Min-P
: Minimum probability for token consideration -
Body: Raw JSON
{ "messages": [ {"role": "system", "content": "You are a helpful AI assistant."}, {"role": "user", "content": "Can you provide ways to eat combinations of bananas and dragonfruits?"}, {"role": "assistant", "content": "Sure! Here are some ways to eat bananas and dragonfruits together: 1. Banana and dragonfruit smoothie: Blend bananas and dragonfruits together with some milk and honey. 2. Banana and dragonfruit salad: Mix sliced bananas and dragonfruits together with some lemon juice and honey."}, {"role": "user", "content": "What about solving an 2x + 3 = 7 equation?"} ] }
- Response: JSON object containing the generated completion
-
-
/completions_stream
(POST): Stream completions for given messages.- Headers: Same as /completions
- Body: Same as /completions
- Response: Server-Sent Events (SSE) stream of generated text
-
/health
(GET): Check the health and get information about the loaded model.- Body: None
-
Response: JSON object containing model and server health information
{ "model_info": { "architecture": "['MistralForCausalLM']", "device": "cuda", "hidden_activation": "silu", "hidden_dimensions": "128", "hidden_size": "5120", "intermediate_size": "14336", "is_quantized": true, "max_position_embeddings": "1024000", "max_seq_length": "1000000000000000019884624838656", "memory_footprint": "8137789440", "model_id": "mistralai/Mistral-Nemo-Instruct-2407", "model_type": "mistral", "model_vocab_size": "131072", "number_of_attention_heads": "32", "number_of_hidden_layers": "40", "number_of_key_value_heads": "8", "quant_method": "QuantizationMethod.BITS_AND_BYTES", "quantization_config": "BitsAndBytesConfig { \"_load_in_4bit\": true, \"_load_in_8bit\": false, \"bnb_4bit_compute_dtype\": \"float32\", \"bnb_4bit_quant_storage\": \"uint8\", \"bnb_4bit_quant_type\": \"fp4\", \"bnb_4bit_use_double_quant\": false, \"llm_int8_enable_fp32_cpu_offload\": false, \"llm_int8_has_fp16_weight\": false, \"llm_int8_skip_modules\": null, \"llm_int8_threshold\": 6.0, \"load_in_4bit\": true, \"load_in_8bit\": false, \"quant_method\": \"bitsandbytes\" }", "tokenizer": "mistralai/Mistral-Nemo-Instruct-2407", "tokenizer_vocab_size": "131072", "torch_dtype": "torch.bfloat16", "transformers_version": "4.43.0.dev0" }, "status": "ok" }
-
/hf_config_reader_api
(POST): Read values from the configuration.-
Body: JSON object with a keys array specifying which config values to read
{ "keys": [ "model_id", "quantize", "quant_level", "torch_device_map", "torch_dtype", "use_flash_attention_2", "max_new_tokens" ] }
-
Response: JSON object containing the requested configuration values
{ "success": true, "values": { "max_new_tokens": 2048, "model_id": "microsoft/Phi-3-mini-128k-instruct", "quant_level": "int8", "quantize": "bitsandbytes", "torch_device_map": "cuda", "torch_dtype": "auto", "use_flash_attention_2": true } }
-
-
/hf_config_writer_api
(POST): Write values to the configuration.-
Body: JSON object with key-value pairs to update in the configuration
{ "config_updates": { "model_id":"microsoft/Phi-3-mini-128k-instruct", "quant_level":"int4" } }
-
Response: JSON object indicating success and whether a restart is required
{ "restart_required": true, "success": true }
-
Body: JSON object with key-value pairs to update in the configuration
-
/restart_server
(GET): Restart the LLM server.- Body: None
-
Response: JSON object indicating success or error
{ "success": true }
The server uses a hf_config.json
file to store and manage configurations. You can modify this file directly or use the provided API endpoints to update settings.
Errors are logged to hf_server_log.log
. The log file uses a rotating file handler, keeping the most recent logs and discarding older ones.
Contributions are welcome! Please feel free to submit a Pull Request.
If HF-Waitress
has proved useful to you, please consider donating to support on-going and future development.
To make a donation, please use the following link to my PayPal:
Your contributions are greatly appreciated and will be used to fund further development efforts.
For Tasks:
Click tags to check more tools for each tasksFor Jobs:
Alternative AI tools for hf-waitress
Similar Open Source Tools
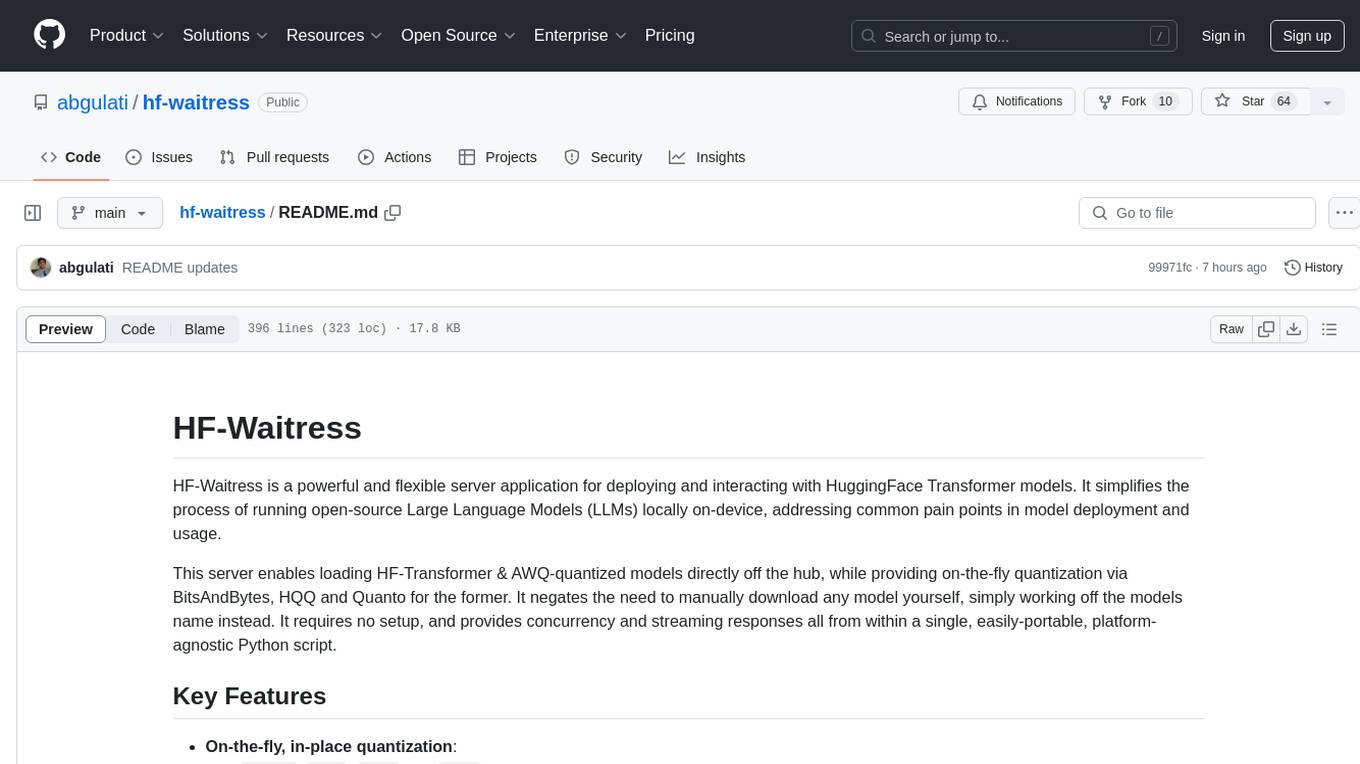
hf-waitress
HF-Waitress is a powerful server application for deploying and interacting with HuggingFace Transformer models. It simplifies running open-source Large Language Models (LLMs) locally on-device, providing on-the-fly quantization via BitsAndBytes, HQQ, and Quanto. It requires no manual model downloads, offers concurrency, streaming responses, and supports various hardware and platforms. The server uses a `config.json` file for easy configuration management and provides detailed error handling and logging.
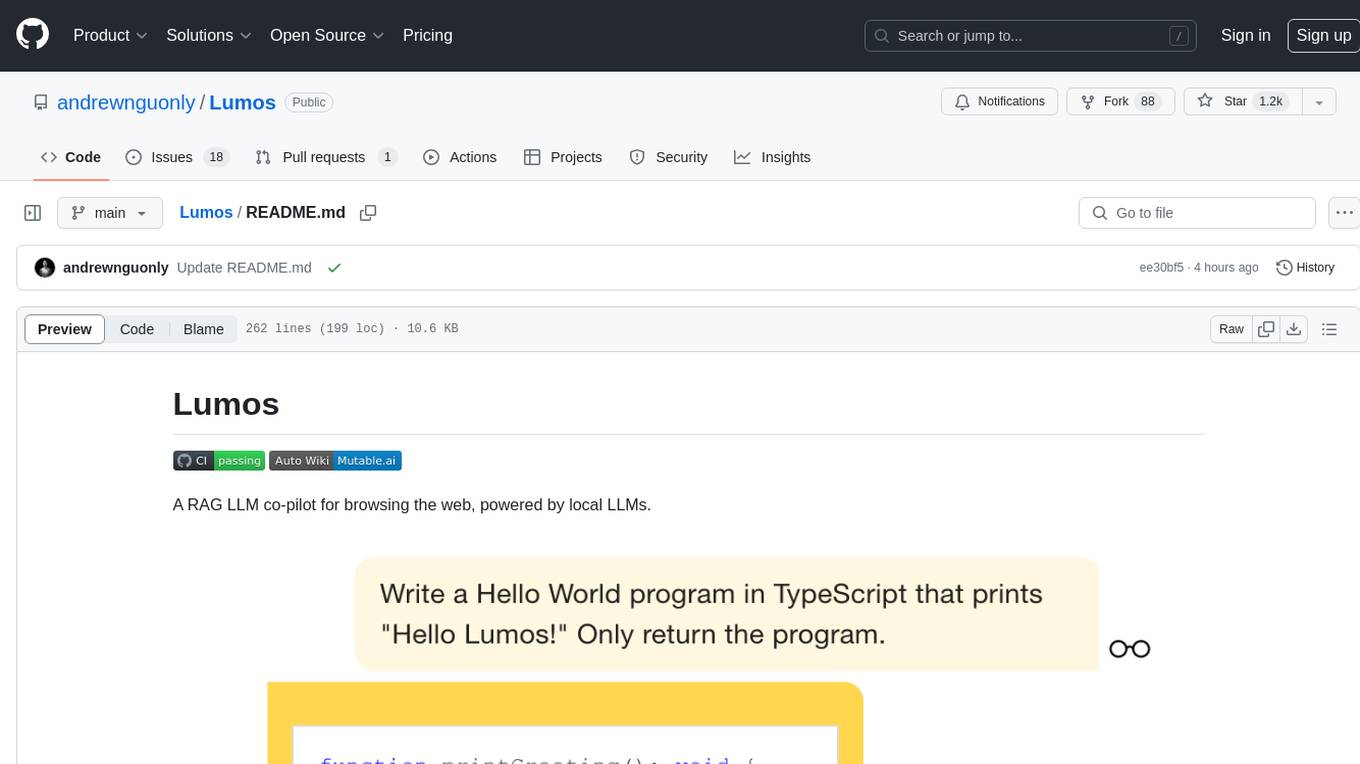
Lumos
Lumos is a Chrome extension powered by a local LLM co-pilot for browsing the web. It allows users to summarize long threads, news articles, and technical documentation. Users can ask questions about reviews and product pages. The tool requires a local Ollama server for LLM inference and embedding database. Lumos supports multimodal models and file attachments for processing text and image content. It also provides options to customize models, hosts, and content parsers. The extension can be easily accessed through keyboard shortcuts and offers tools for automatic invocation based on prompts.
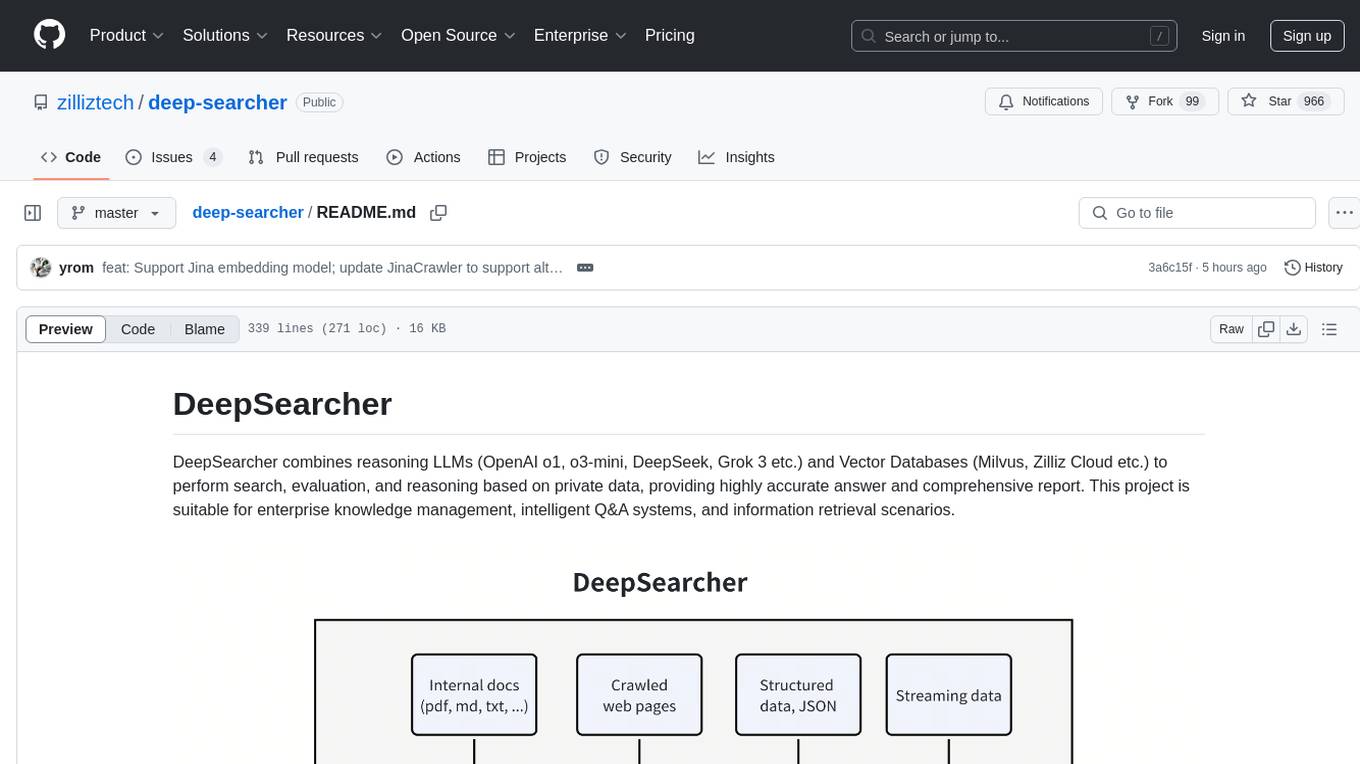
deep-searcher
DeepSearcher is a tool that combines reasoning LLMs and Vector Databases to perform search, evaluation, and reasoning based on private data. It is suitable for enterprise knowledge management, intelligent Q&A systems, and information retrieval scenarios. The tool maximizes the utilization of enterprise internal data while ensuring data security, supports multiple embedding models, and provides support for multiple LLMs for intelligent Q&A and content generation. It also includes features like private data search, vector database management, and document loading with web crawling capabilities under development.
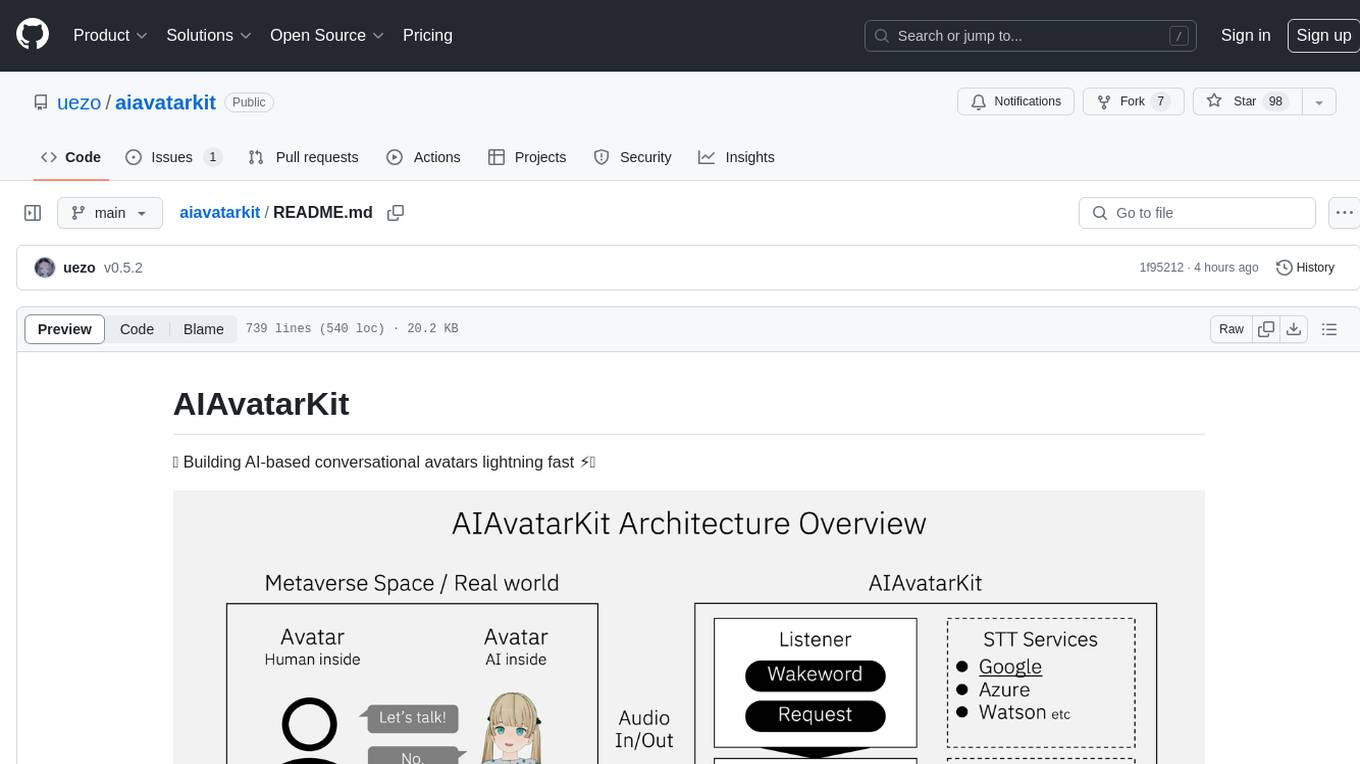
aiavatarkit
AIAvatarKit is a tool for building AI-based conversational avatars quickly. It supports various platforms like VRChat and cluster, along with real-world devices. The tool is extensible, allowing unlimited capabilities based on user needs. It requires VOICEVOX API, Google or Azure Speech Services API keys, and Python 3.10. Users can start conversations out of the box and enjoy seamless interactions with the avatars.
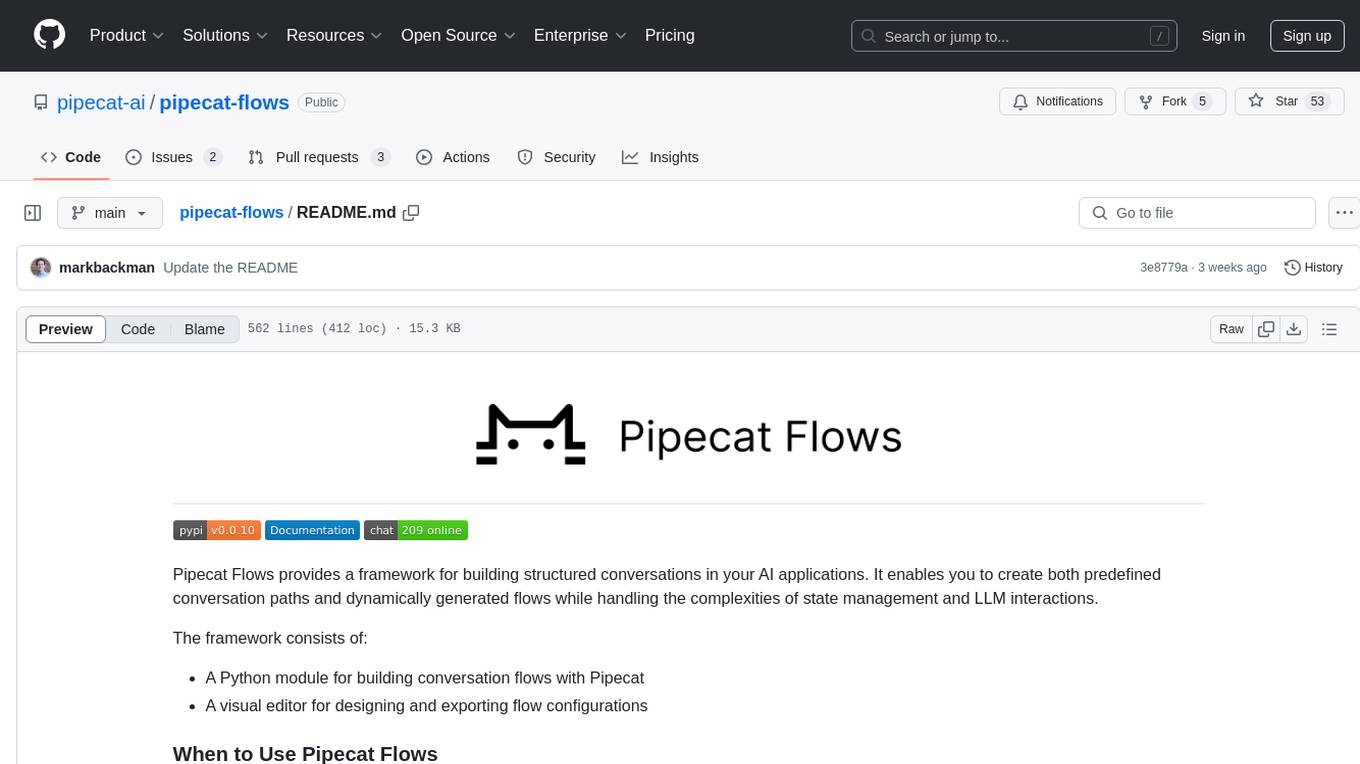
pipecat-flows
Pipecat Flows is a framework designed for building structured conversations in AI applications. It allows users to create both predefined conversation paths and dynamically generated flows, handling state management and LLM interactions. The framework includes a Python module for building conversation flows and a visual editor for designing and exporting flow configurations. Pipecat Flows is suitable for scenarios such as customer service scripts, intake forms, personalized experiences, and complex decision trees.
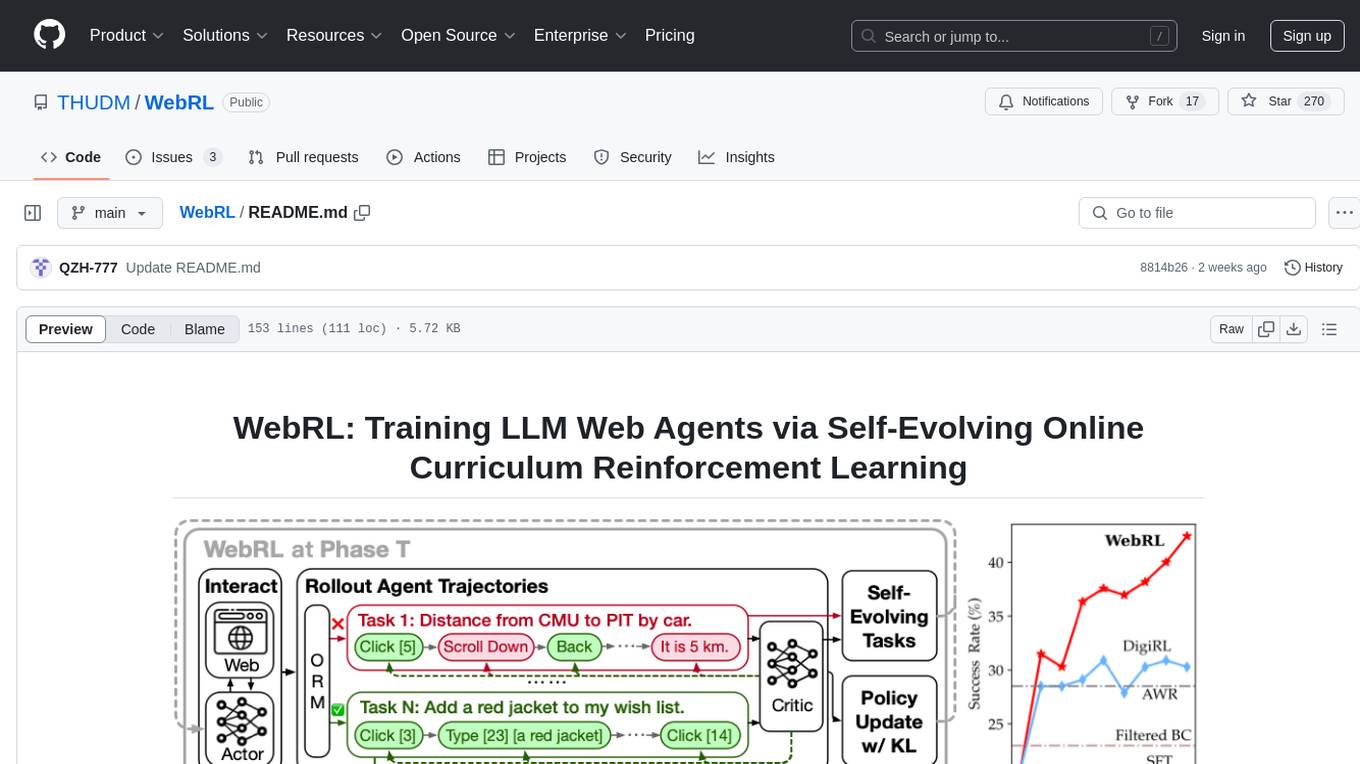
WebRL
WebRL is a self-evolving online curriculum learning framework designed for training web agents in the WebArena environment. It provides model checkpoints, training instructions, and evaluation processes for training the actor and critic models. The tool enables users to generate new instructions and interact with WebArena to configure tasks for training and evaluation.
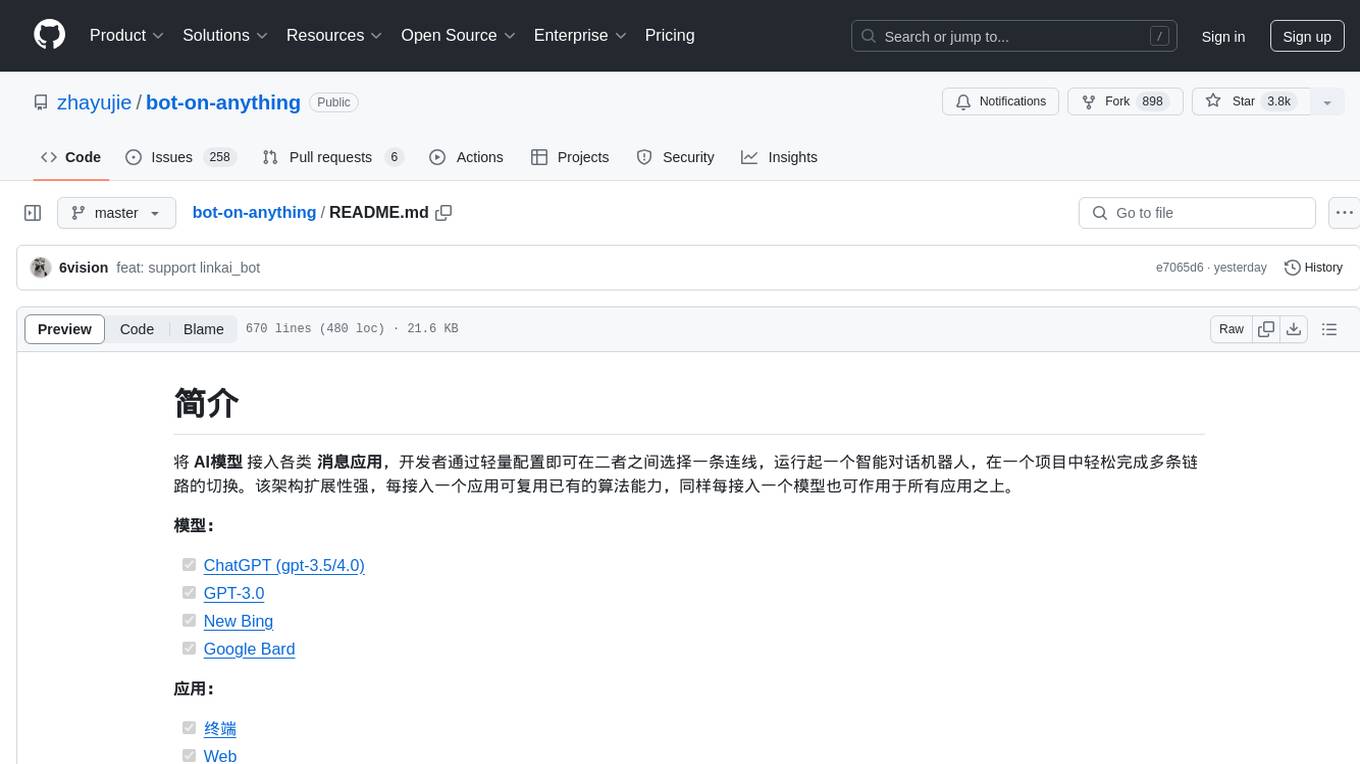
bot-on-anything
The 'bot-on-anything' repository allows developers to integrate various AI models into messaging applications, enabling the creation of intelligent chatbots. By configuring the connections between models and applications, developers can easily switch between multiple channels within a project. The architecture is highly scalable, allowing the reuse of algorithmic capabilities for each new application and model integration. Supported models include ChatGPT, GPT-3.0, New Bing, and Google Bard, while supported applications range from terminals and web platforms to messaging apps like WeChat, Telegram, QQ, and more. The repository provides detailed instructions for setting up the environment, configuring the models and channels, and running the chatbot for various tasks across different messaging platforms.
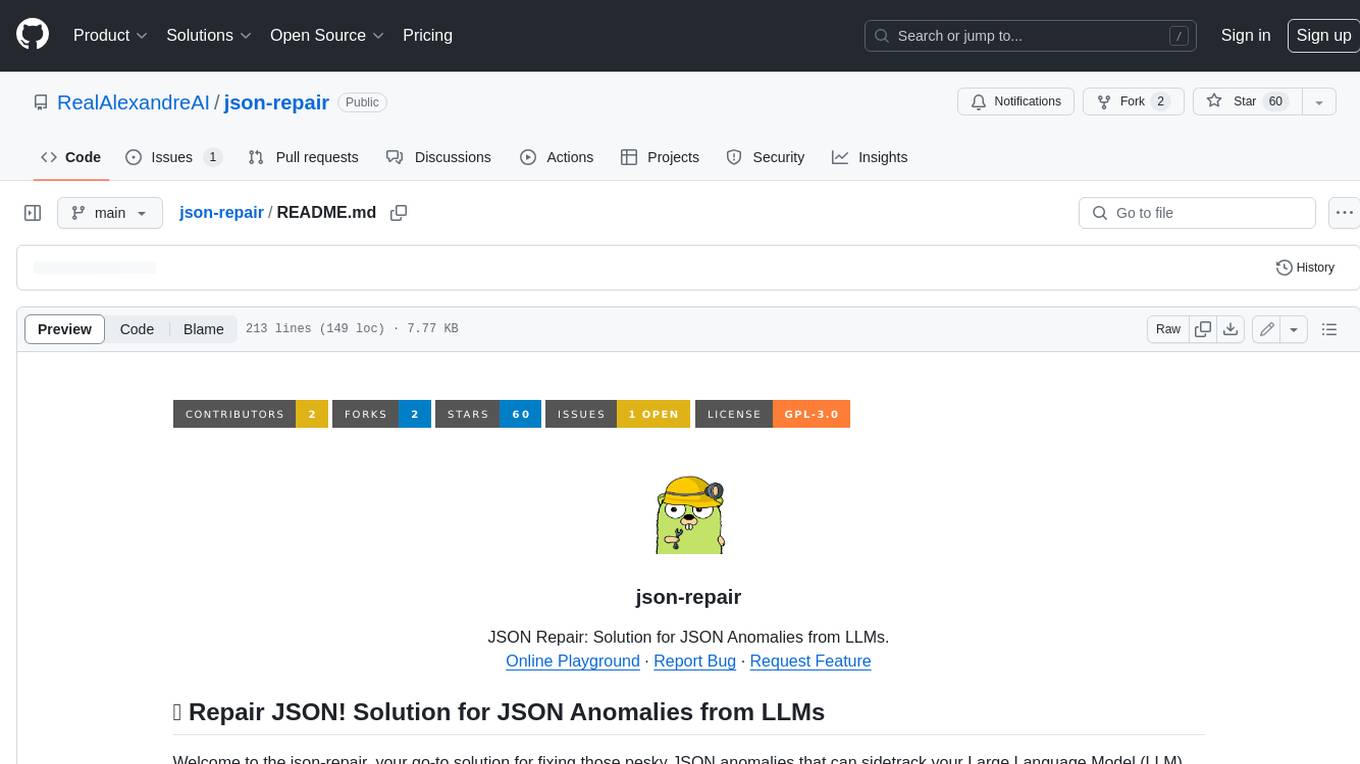
json-repair
JSON Repair is a toolkit designed to address JSON anomalies that can arise from Large Language Models (LLMs). It offers a comprehensive solution for repairing JSON strings, ensuring accuracy and reliability in your data processing. With its user-friendly interface and extensive capabilities, JSON Repair empowers developers to seamlessly integrate JSON repair into their workflows.
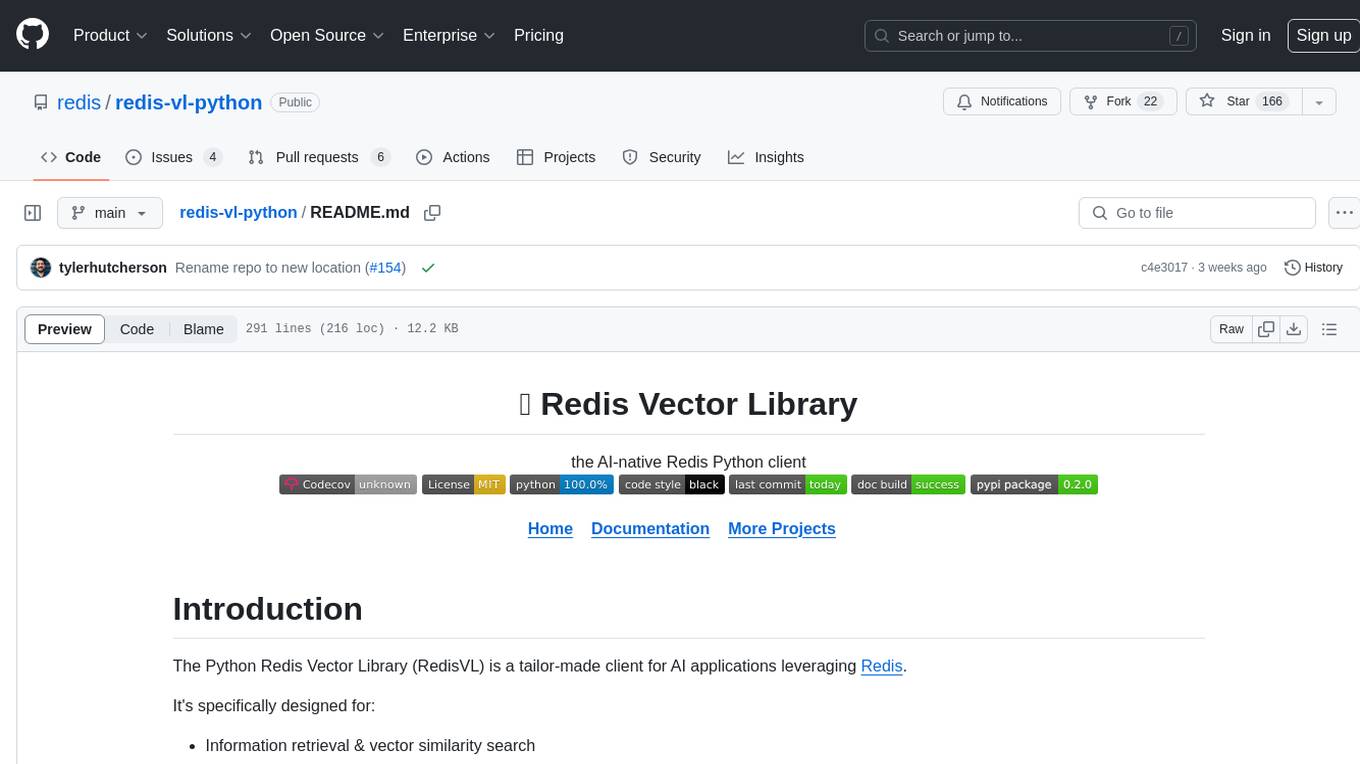
redis-vl-python
The Python Redis Vector Library (RedisVL) is a tailor-made client for AI applications leveraging Redis. It enhances applications with Redis' speed, flexibility, and reliability, incorporating capabilities like vector-based semantic search, full-text search, and geo-spatial search. The library bridges the gap between the emerging AI-native developer ecosystem and the capabilities of Redis by providing a lightweight, elegant, and intuitive interface. It abstracts the features of Redis into a grammar that is more aligned to the needs of today's AI/ML Engineers or Data Scientists.
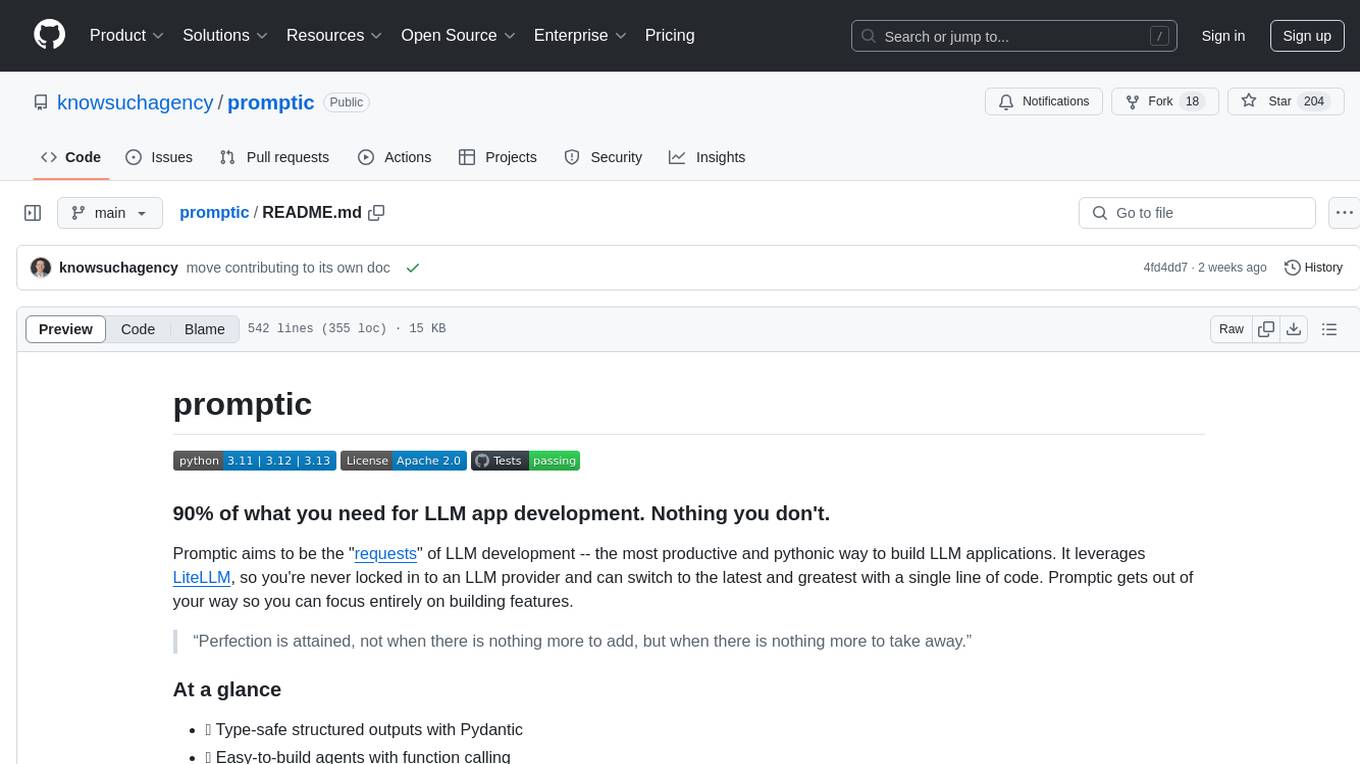
promptic
Promptic is a tool designed for LLM app development, providing a productive and pythonic way to build LLM applications. It leverages LiteLLM, allowing flexibility to switch LLM providers easily. Promptic focuses on building features by providing type-safe structured outputs, easy-to-build agents, streaming support, automatic prompt caching, and built-in conversation memory.
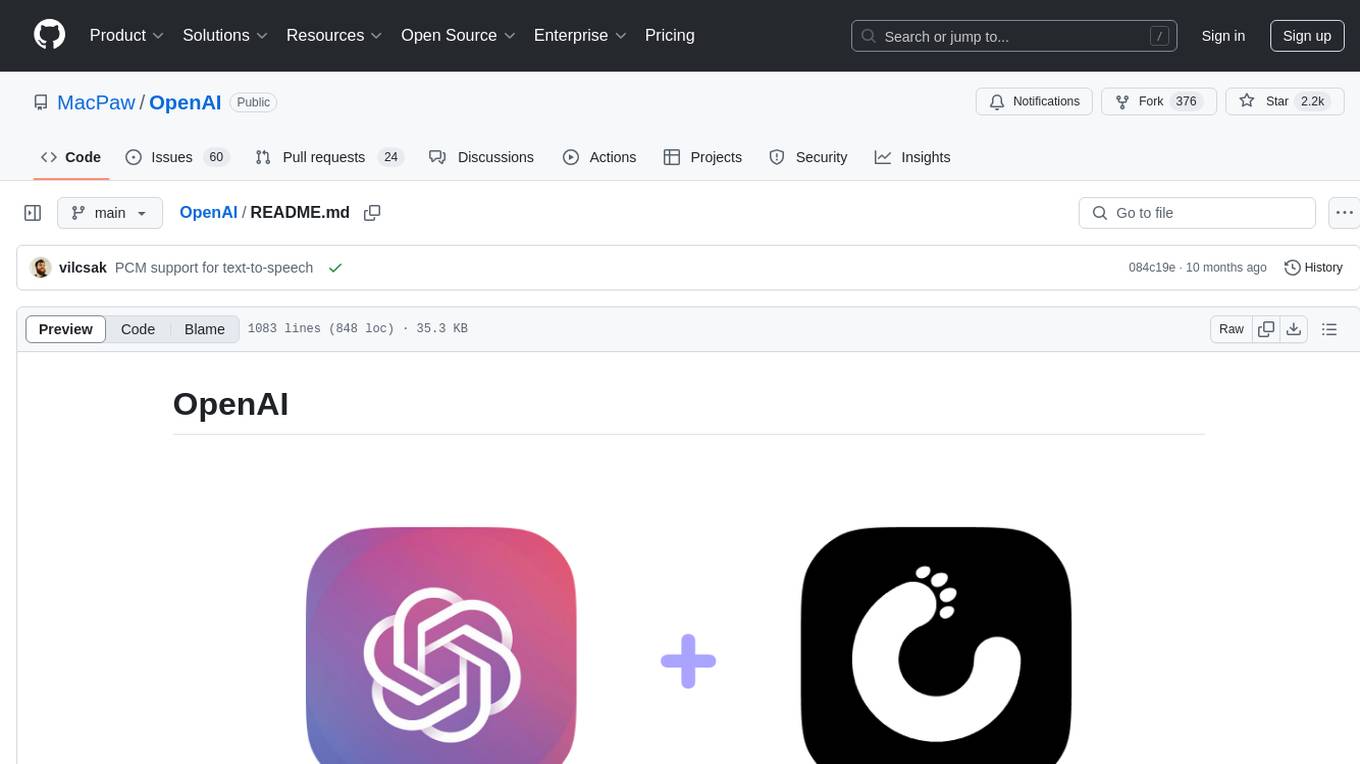
OpenAI
OpenAI is a Swift community-maintained implementation over OpenAI public API. It is a non-profit artificial intelligence research organization founded in San Francisco, California in 2015. OpenAI's mission is to ensure safe and responsible use of AI for civic good, economic growth, and other public benefits. The repository provides functionalities for text completions, chats, image generation, audio processing, edits, embeddings, models, moderations, utilities, and Combine extensions.
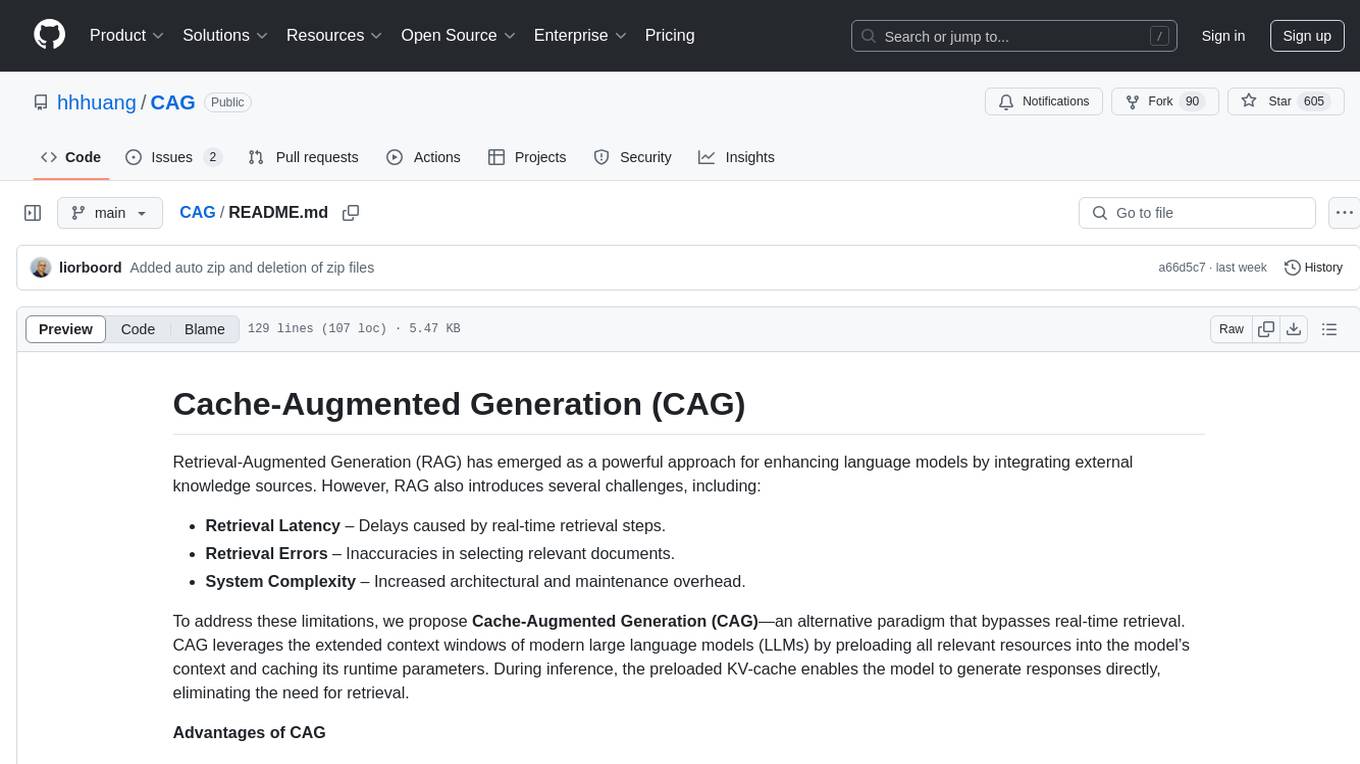
CAG
Cache-Augmented Generation (CAG) is an alternative paradigm to Retrieval-Augmented Generation (RAG) that eliminates real-time retrieval delays and errors by preloading all relevant resources into the model's context. CAG leverages extended context windows of large language models (LLMs) to generate responses directly, providing reduced latency, improved reliability, and simplified design. While CAG has limitations in knowledge size and context length, advancements in LLMs are addressing these issues, making CAG a practical and scalable alternative for complex applications.
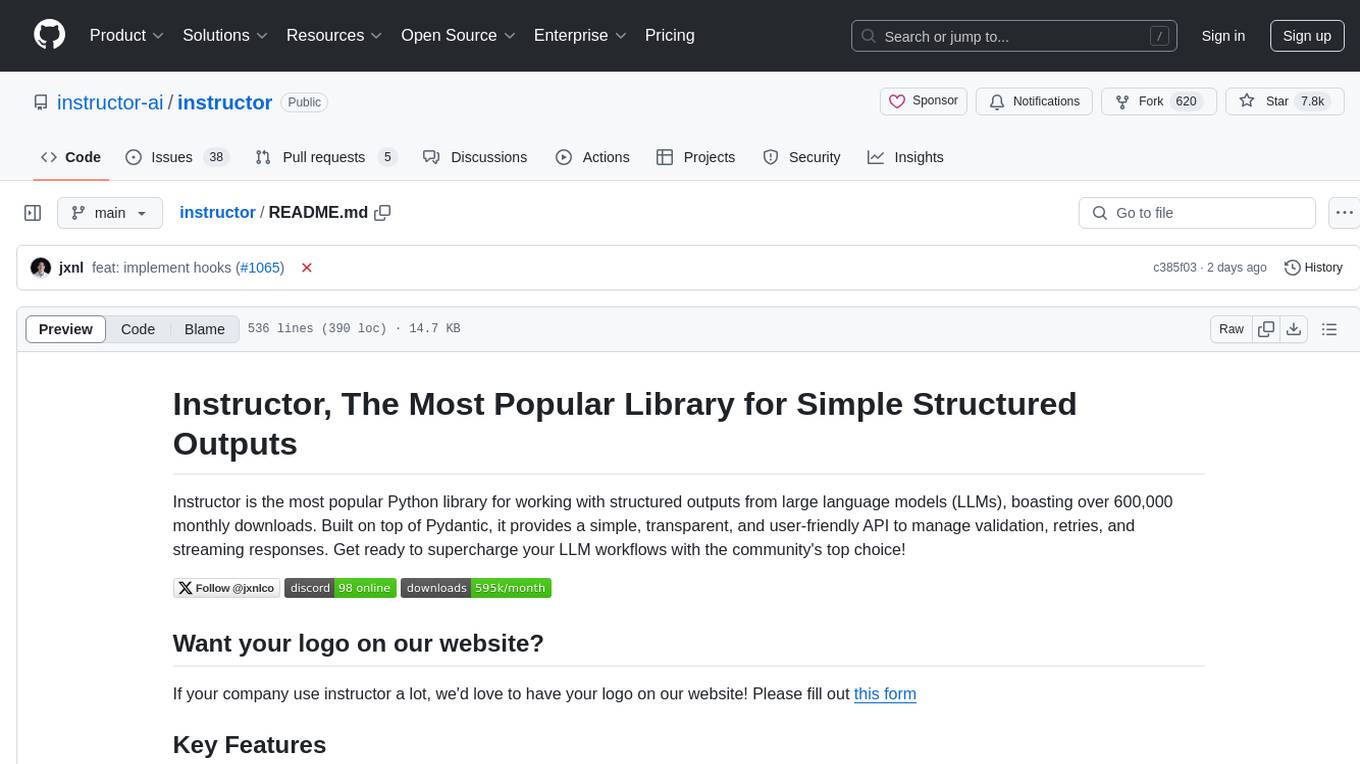
instructor
Instructor is a popular Python library for managing structured outputs from large language models (LLMs). It offers a user-friendly API for validation, retries, and streaming responses. With support for various LLM providers and multiple languages, Instructor simplifies working with LLM outputs. The library includes features like response models, retry management, validation, streaming support, and flexible backends. It also provides hooks for logging and monitoring LLM interactions, and supports integration with Anthropic, Cohere, Gemini, Litellm, and Google AI models. Instructor facilitates tasks such as extracting user data from natural language, creating fine-tuned models, managing uploaded files, and monitoring usage of OpenAI models.
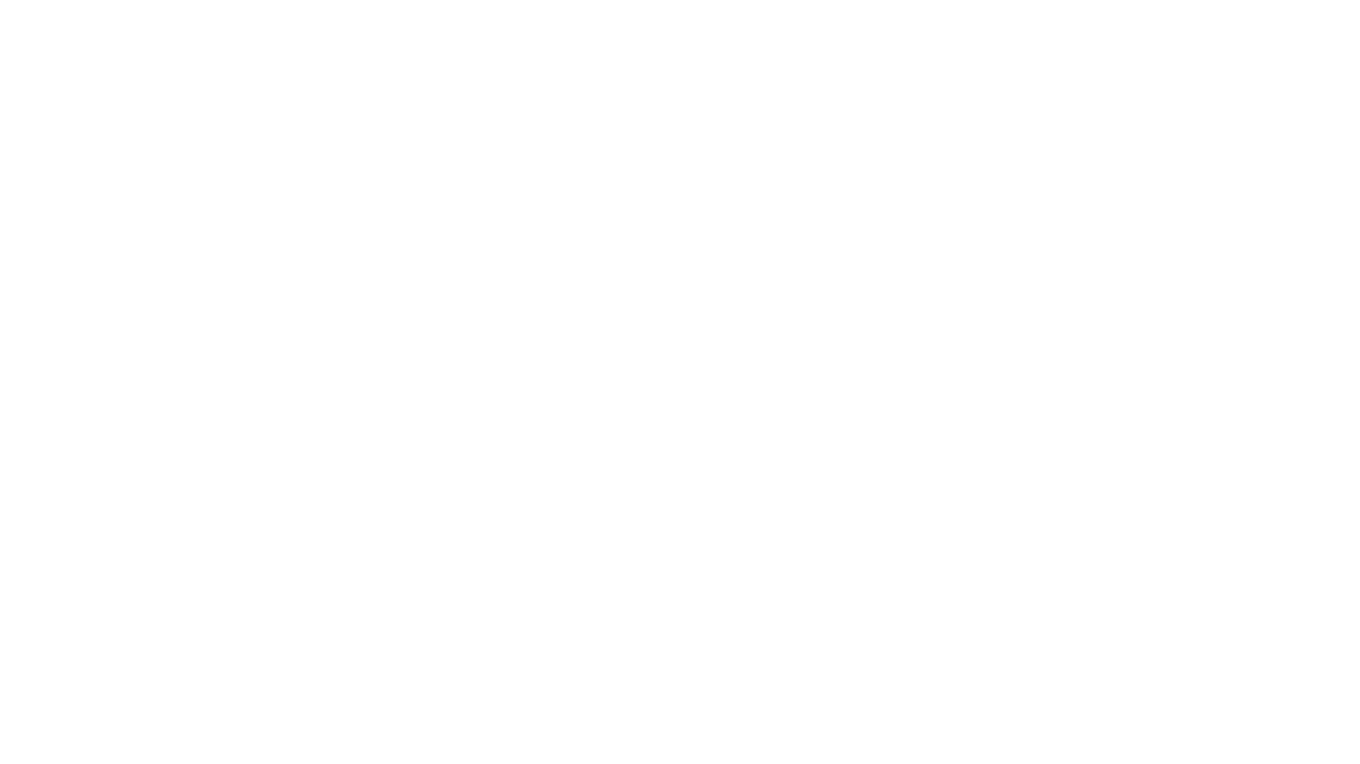
LLamaWorker
LLamaWorker is a HTTP API server developed to provide an OpenAI-compatible API for integrating Large Language Models (LLM) into applications. It supports multi-model configuration, streaming responses, text embedding, chat templates, automatic model release, function calls, API key authentication, and test UI. Users can switch models, complete chats and prompts, manage chat history, and generate tokens through the test UI. Additionally, LLamaWorker offers a Vulkan compiled version for download and provides function call templates for testing. The tool supports various backends and provides API endpoints for chat completion, prompt completion, embeddings, model information, model configuration, and model switching. A Gradio UI demo is also available for testing.
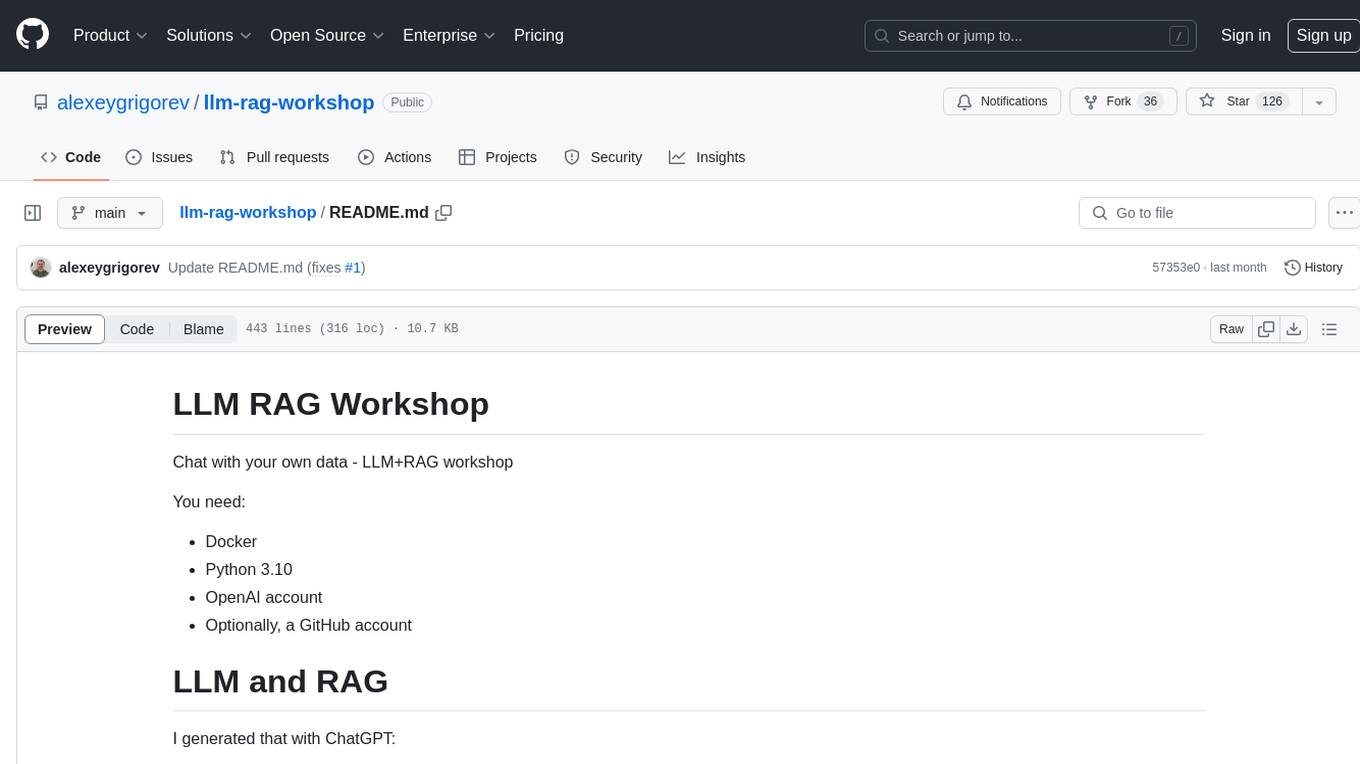
llm-rag-workshop
The LLM RAG Workshop repository provides a workshop on using Large Language Models (LLMs) and Retrieval-Augmented Generation (RAG) to generate and understand text in a human-like manner. It includes instructions on setting up the environment, indexing Zoomcamp FAQ documents, creating a Q&A system, and using OpenAI for generation based on retrieved information. The repository focuses on enhancing language model responses with retrieved information from external sources, such as document databases or search engines, to improve factual accuracy and relevance of generated text.
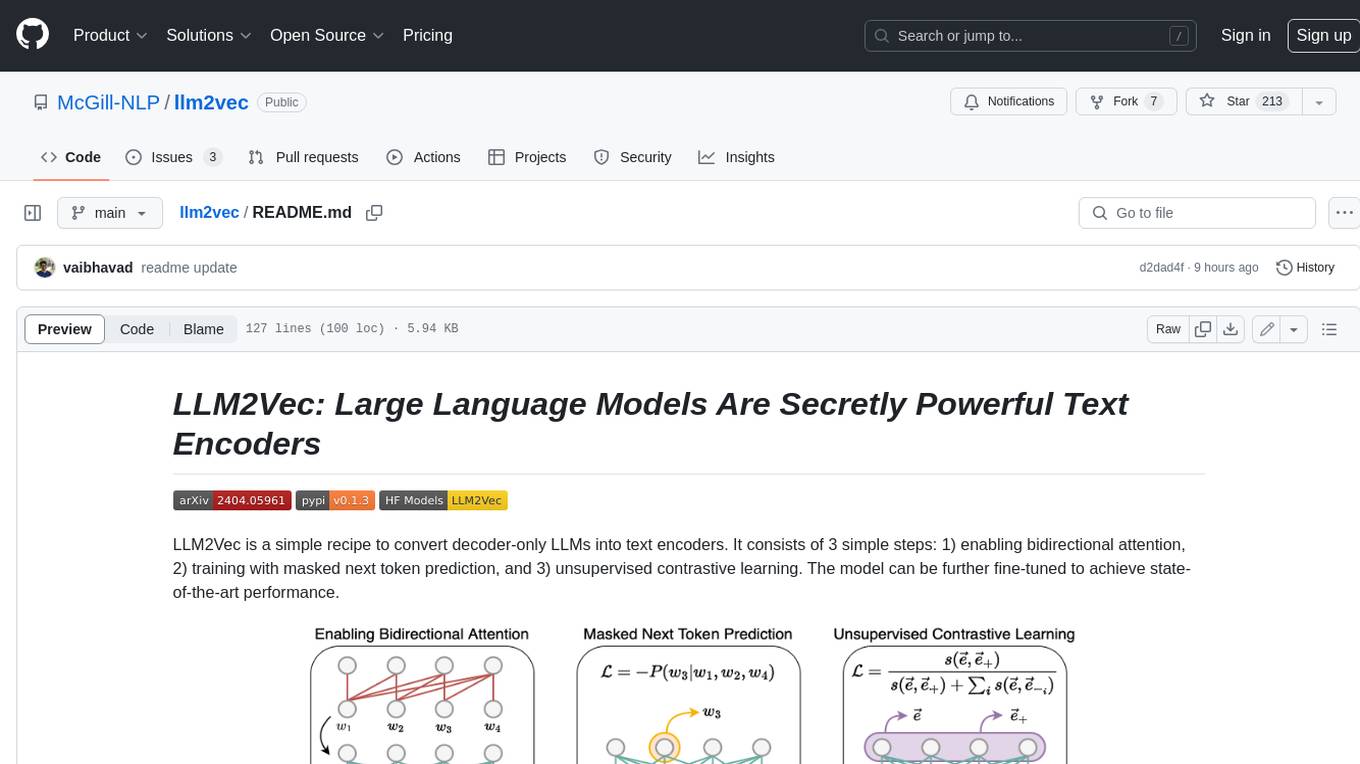
llm2vec
LLM2Vec is a simple recipe to convert decoder-only LLMs into text encoders. It consists of 3 simple steps: 1) enabling bidirectional attention, 2) training with masked next token prediction, and 3) unsupervised contrastive learning. The model can be further fine-tuned to achieve state-of-the-art performance.
For similar tasks
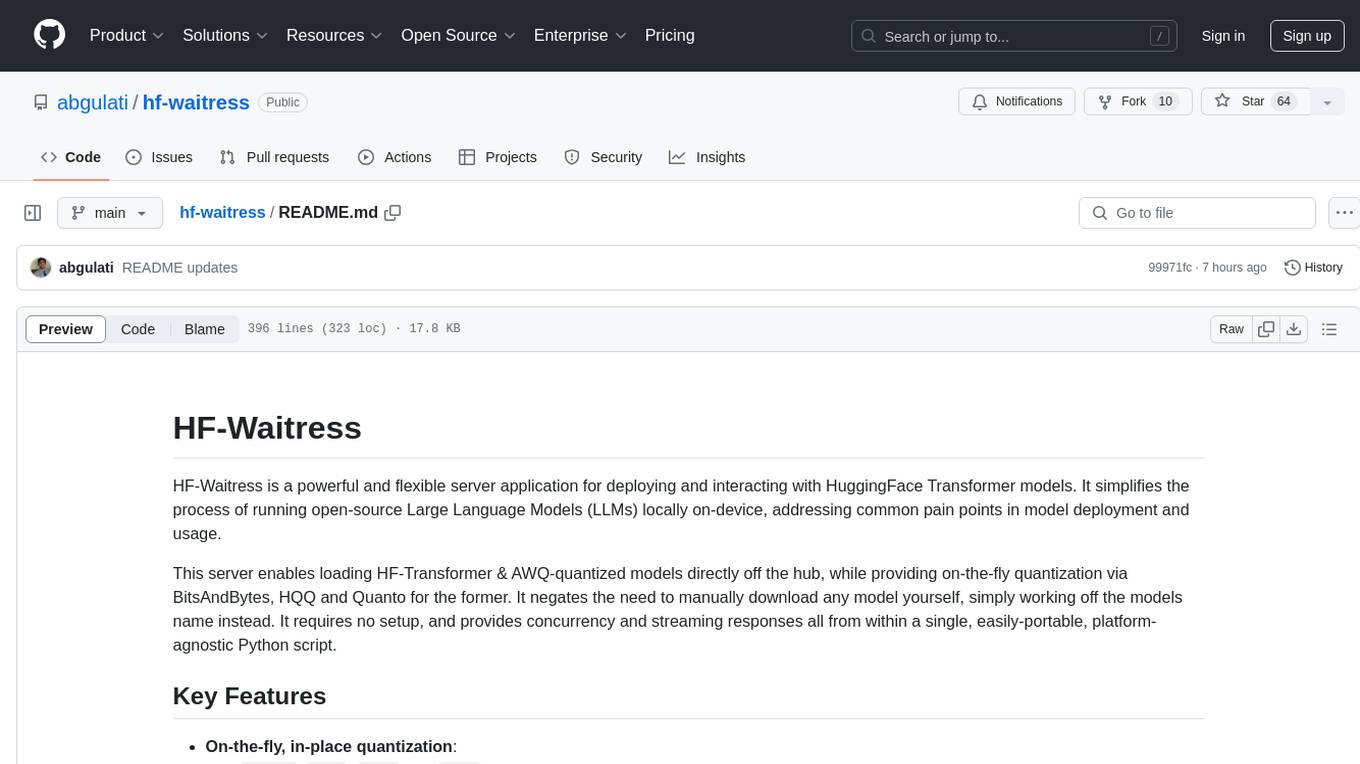
hf-waitress
HF-Waitress is a powerful server application for deploying and interacting with HuggingFace Transformer models. It simplifies running open-source Large Language Models (LLMs) locally on-device, providing on-the-fly quantization via BitsAndBytes, HQQ, and Quanto. It requires no manual model downloads, offers concurrency, streaming responses, and supports various hardware and platforms. The server uses a `config.json` file for easy configuration management and provides detailed error handling and logging.
For similar jobs
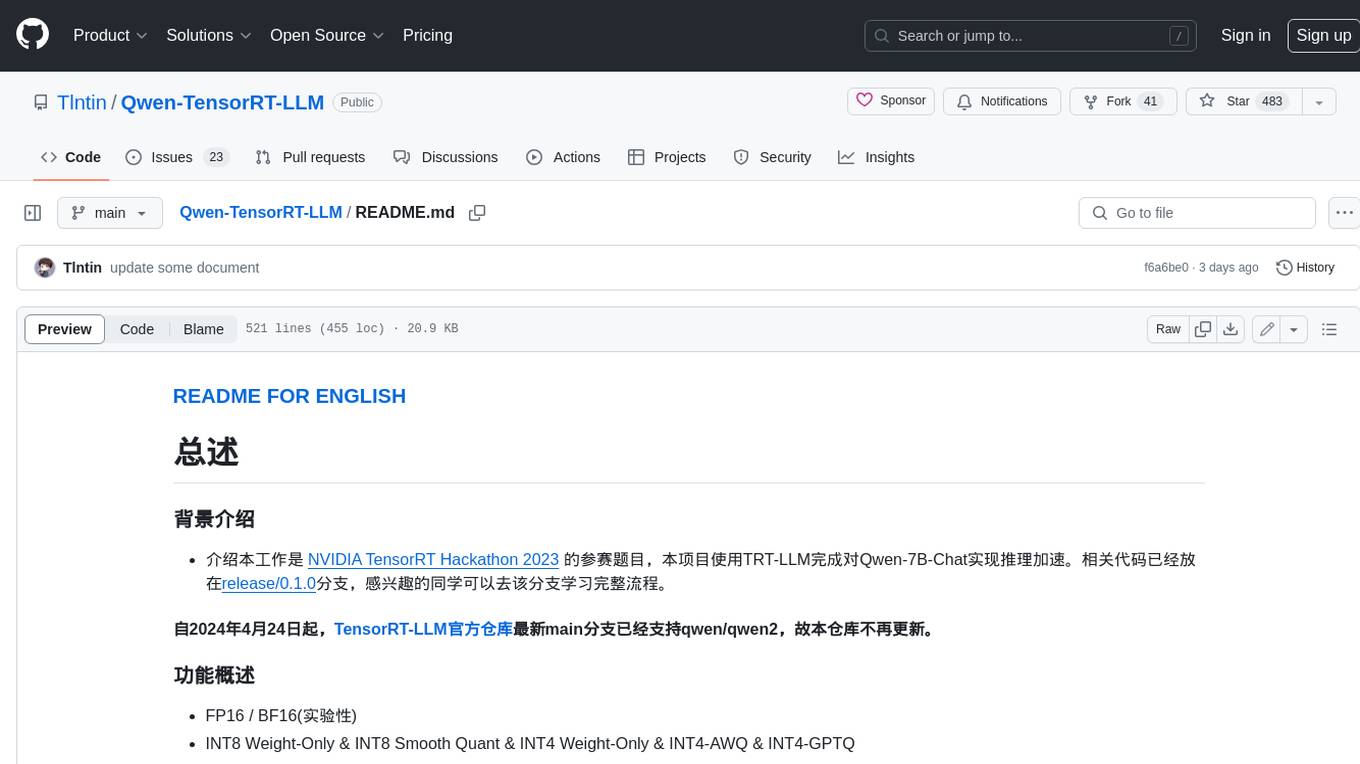
Qwen-TensorRT-LLM
Qwen-TensorRT-LLM is a project developed for the NVIDIA TensorRT Hackathon 2023, focusing on accelerating inference for the Qwen-7B-Chat model using TRT-LLM. The project offers various functionalities such as FP16/BF16 support, INT8 and INT4 quantization options, Tensor Parallel for multi-GPU parallelism, web demo setup with gradio, Triton API deployment for maximum throughput/concurrency, fastapi integration for openai requests, CLI interaction, and langchain support. It supports models like qwen2, qwen, and qwen-vl for both base and chat models. The project also provides tutorials on Bilibili and blogs for adapting Qwen models in NVIDIA TensorRT-LLM, along with hardware requirements and quick start guides for different model types and quantization methods.
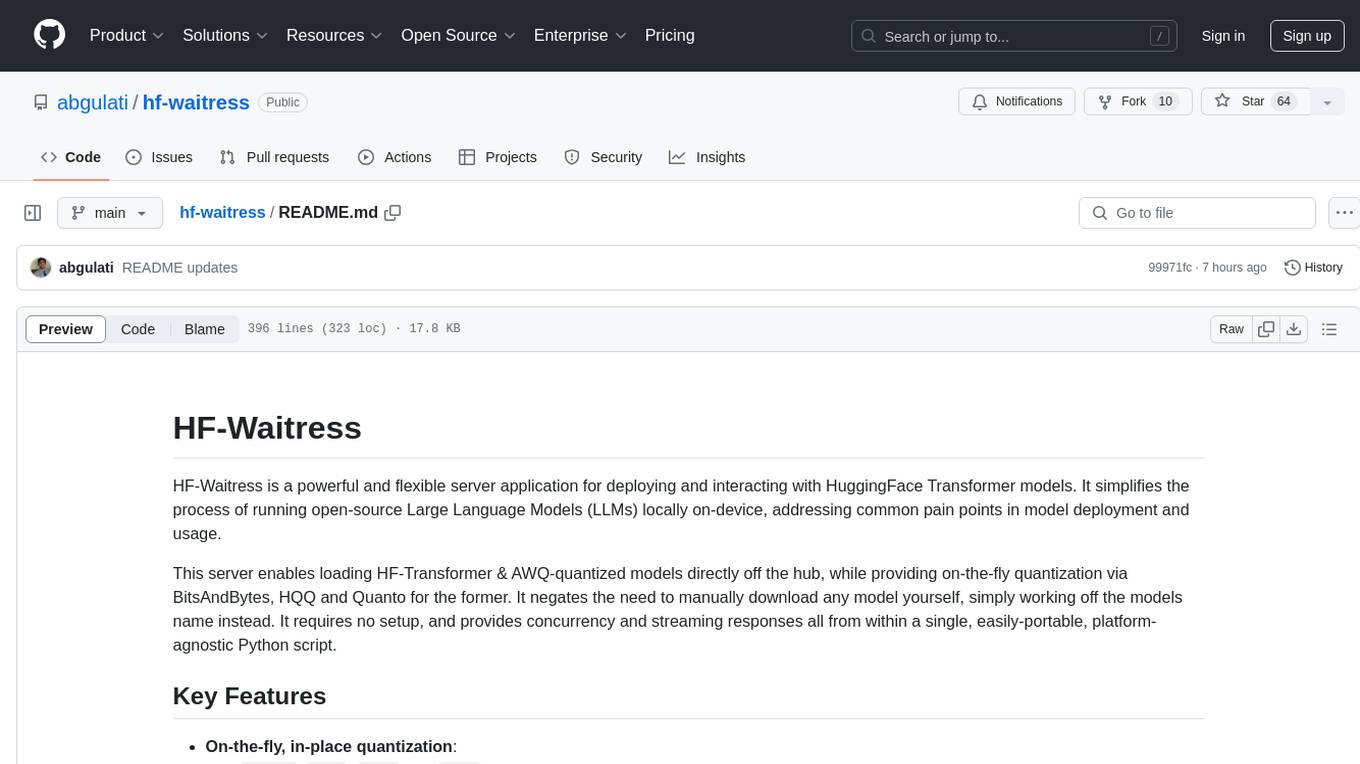
hf-waitress
HF-Waitress is a powerful server application for deploying and interacting with HuggingFace Transformer models. It simplifies running open-source Large Language Models (LLMs) locally on-device, providing on-the-fly quantization via BitsAndBytes, HQQ, and Quanto. It requires no manual model downloads, offers concurrency, streaming responses, and supports various hardware and platforms. The server uses a `config.json` file for easy configuration management and provides detailed error handling and logging.
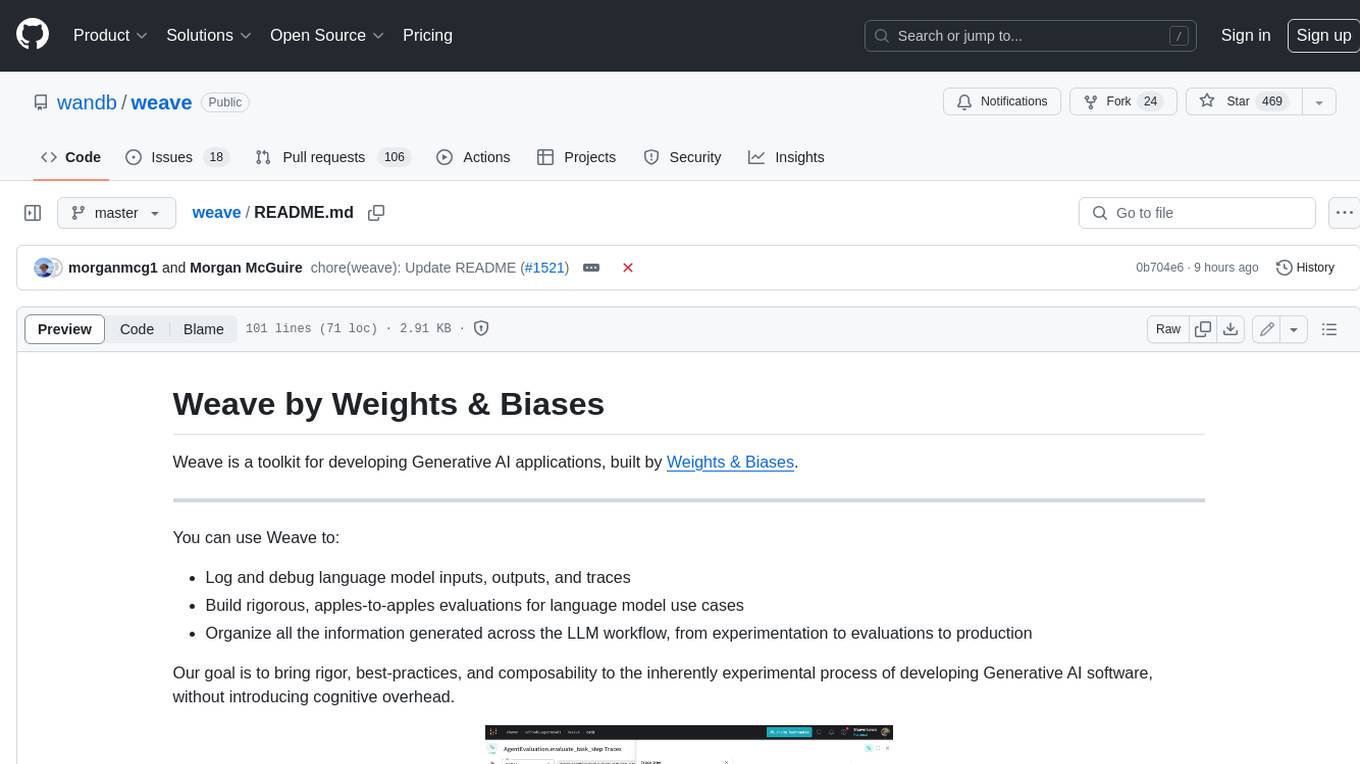
weave
Weave is a toolkit for developing Generative AI applications, built by Weights & Biases. With Weave, you can log and debug language model inputs, outputs, and traces; build rigorous, apples-to-apples evaluations for language model use cases; and organize all the information generated across the LLM workflow, from experimentation to evaluations to production. Weave aims to bring rigor, best-practices, and composability to the inherently experimental process of developing Generative AI software, without introducing cognitive overhead.
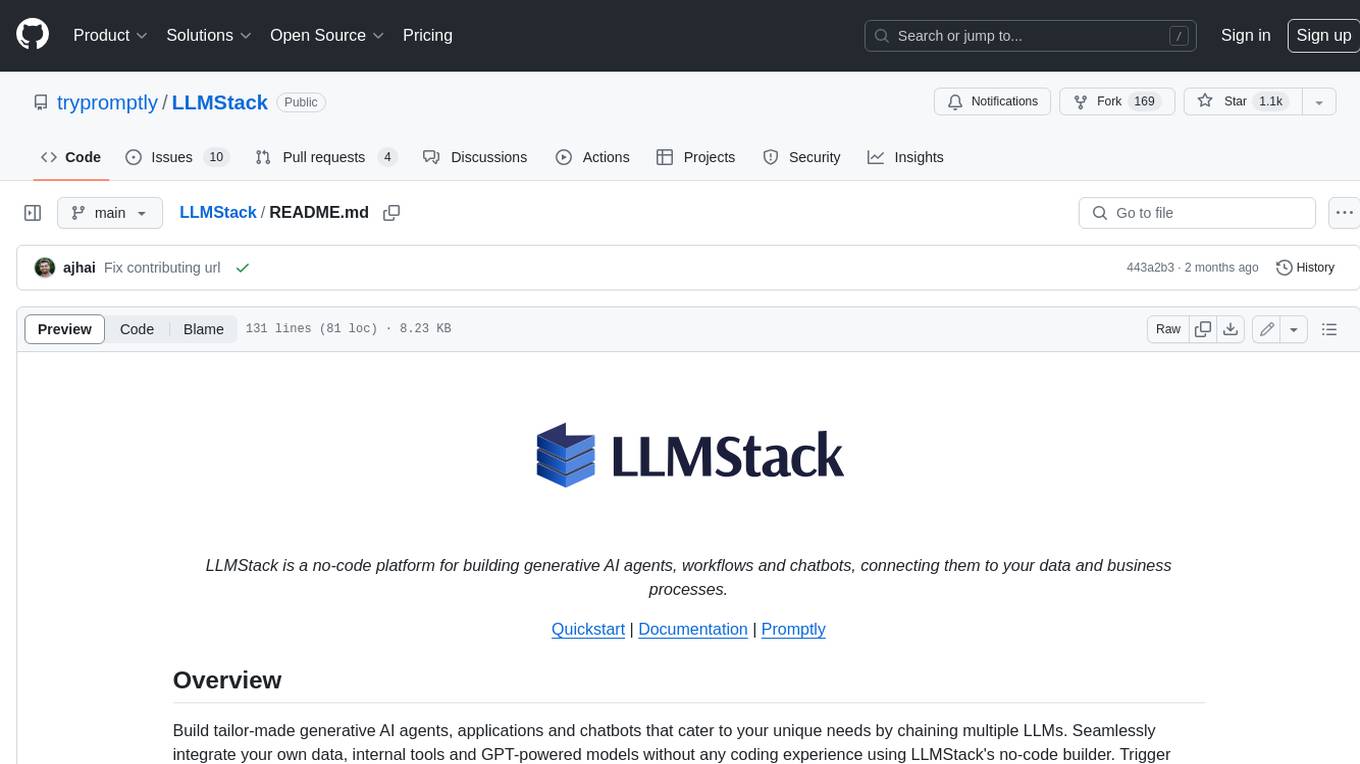
LLMStack
LLMStack is a no-code platform for building generative AI agents, workflows, and chatbots. It allows users to connect their own data, internal tools, and GPT-powered models without any coding experience. LLMStack can be deployed to the cloud or on-premise and can be accessed via HTTP API or triggered from Slack or Discord.
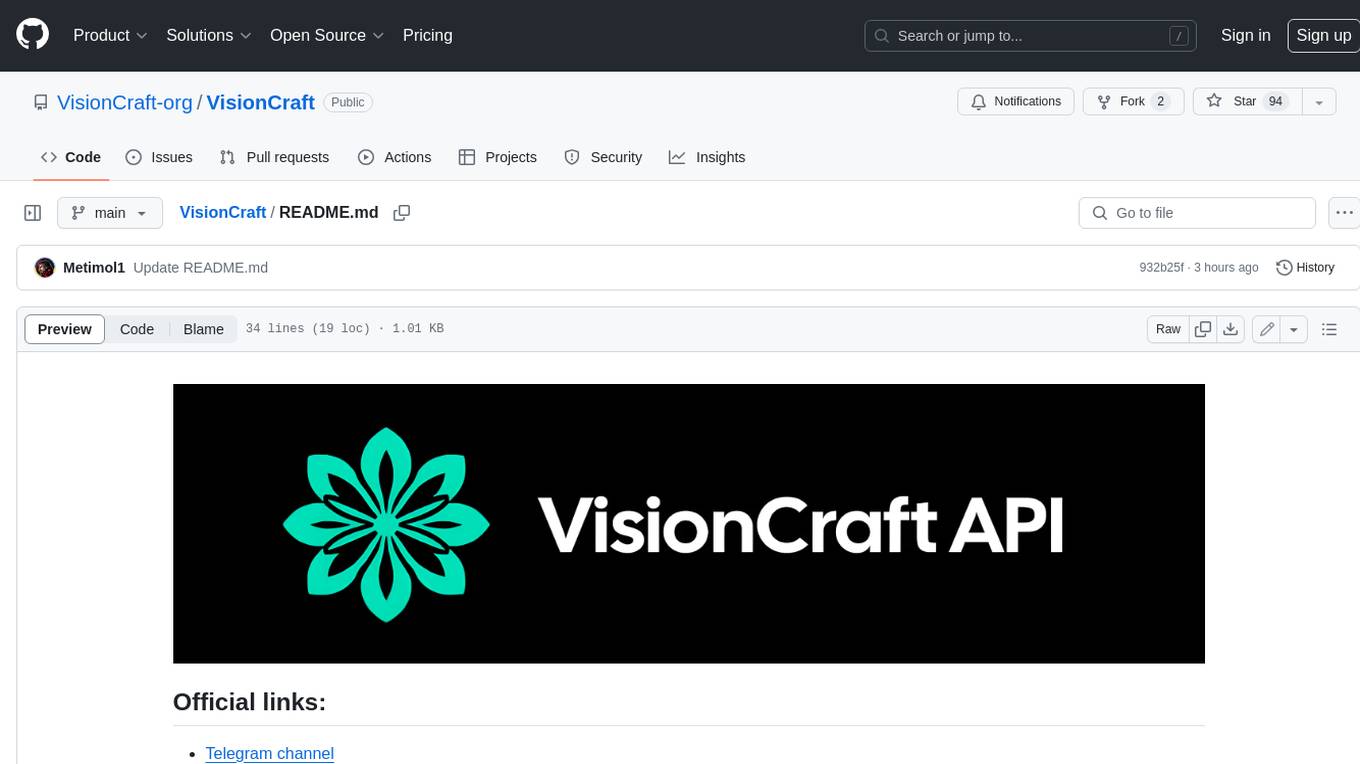
VisionCraft
The VisionCraft API is a free API for using over 100 different AI models. From images to sound.
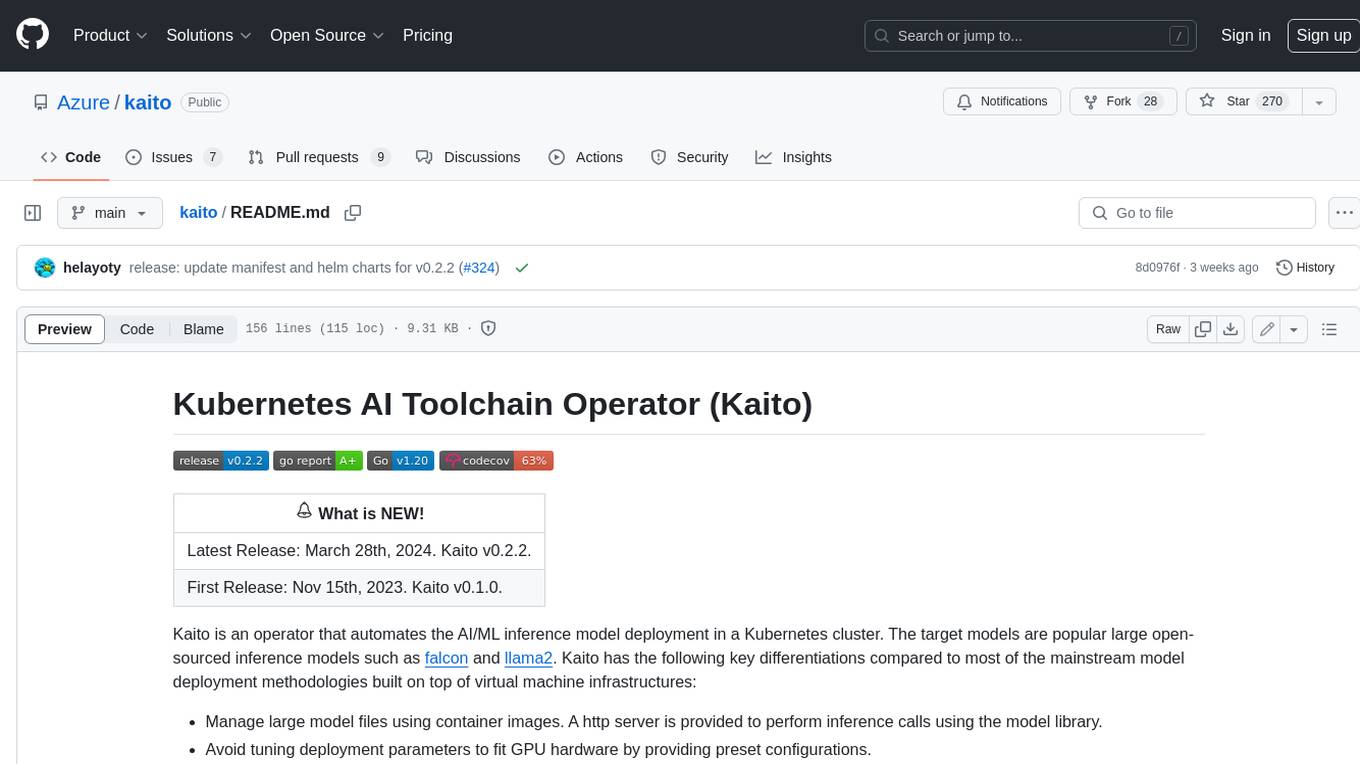
kaito
Kaito is an operator that automates the AI/ML inference model deployment in a Kubernetes cluster. It manages large model files using container images, avoids tuning deployment parameters to fit GPU hardware by providing preset configurations, auto-provisions GPU nodes based on model requirements, and hosts large model images in the public Microsoft Container Registry (MCR) if the license allows. Using Kaito, the workflow of onboarding large AI inference models in Kubernetes is largely simplified.
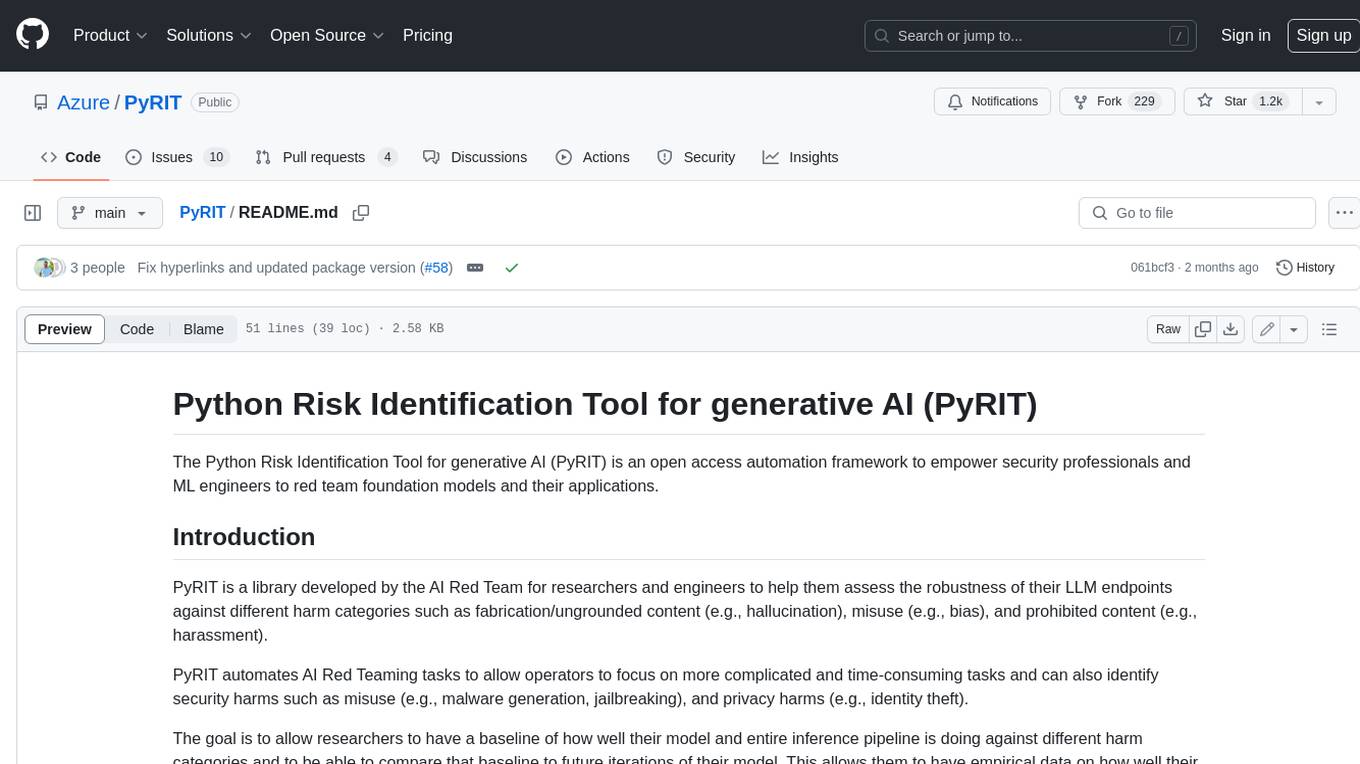
PyRIT
PyRIT is an open access automation framework designed to empower security professionals and ML engineers to red team foundation models and their applications. It automates AI Red Teaming tasks to allow operators to focus on more complicated and time-consuming tasks and can also identify security harms such as misuse (e.g., malware generation, jailbreaking), and privacy harms (e.g., identity theft). The goal is to allow researchers to have a baseline of how well their model and entire inference pipeline is doing against different harm categories and to be able to compare that baseline to future iterations of their model. This allows them to have empirical data on how well their model is doing today, and detect any degradation of performance based on future improvements.
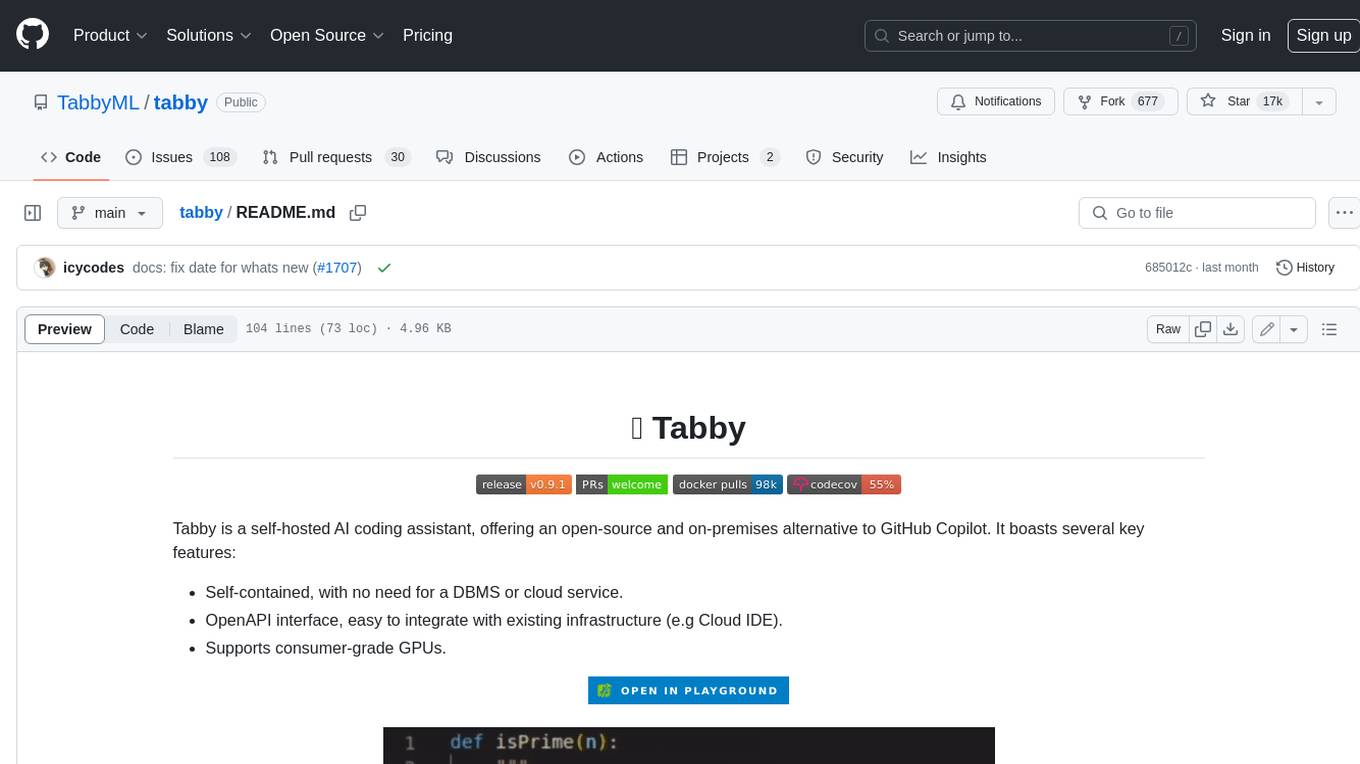
tabby
Tabby is a self-hosted AI coding assistant, offering an open-source and on-premises alternative to GitHub Copilot. It boasts several key features: * Self-contained, with no need for a DBMS or cloud service. * OpenAPI interface, easy to integrate with existing infrastructure (e.g Cloud IDE). * Supports consumer-grade GPUs.