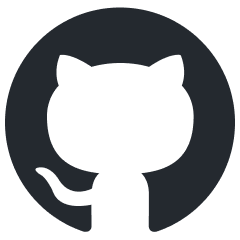
aiavatarkit
๐ฅฐ Building AI-based conversational avatars lightning fast โก๏ธ๐ฌ
Stars: 303
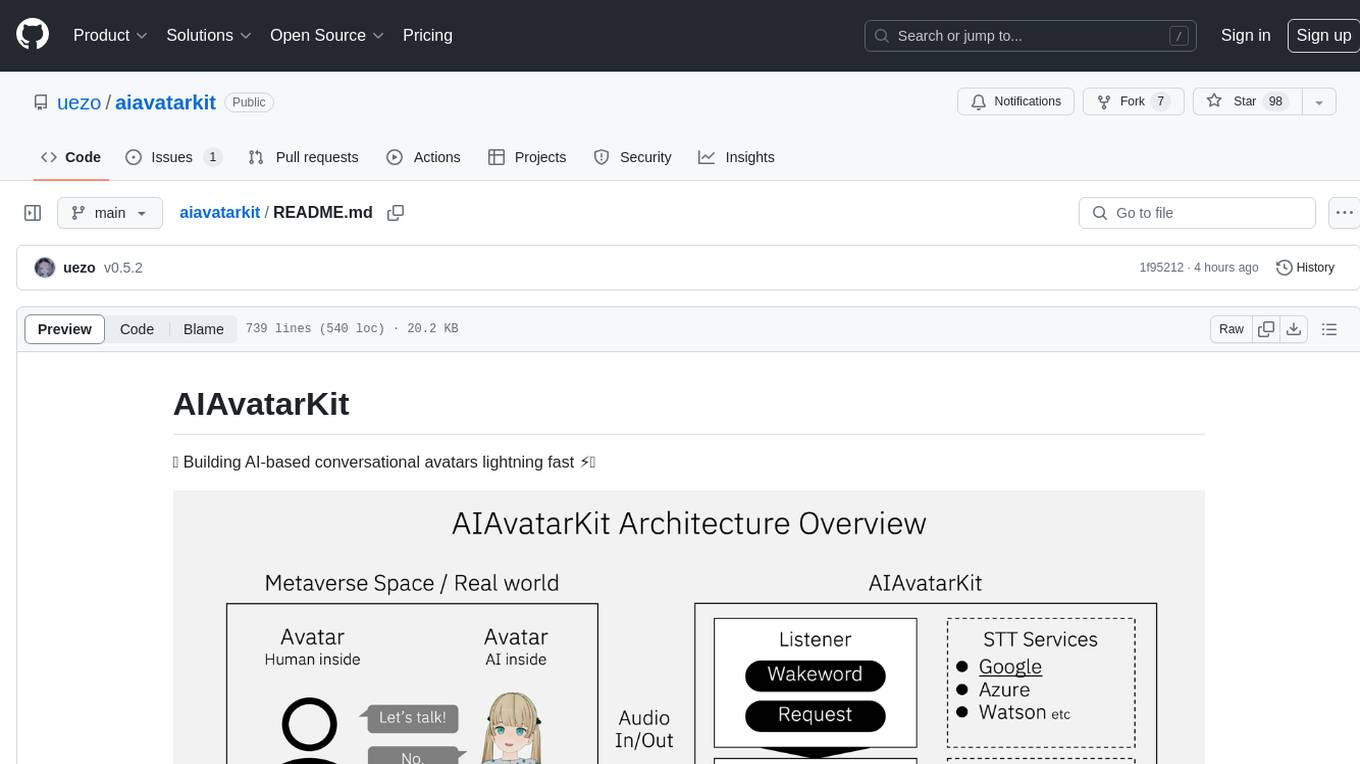
AIAvatarKit is a tool for building AI-based conversational avatars quickly. It supports various platforms like VRChat and cluster, along with real-world devices. The tool is extensible, allowing unlimited capabilities based on user needs. It requires VOICEVOX API, Google or Azure Speech Services API keys, and Python 3.10. Users can start conversations out of the box and enjoy seamless interactions with the avatars.
README:
๐ฅฐ Building AI-based conversational avatars lightning fast โก๏ธ๐ฌ
- Live anywhere: VRChat, cluster and any other metaverse platforms, and even devices in the real world.
- Extensible: Unlimited capabilities that depends on you.
- Easy to start: Ready to start conversation right out of the box.
- VOICEVOX API in your computer or network reachable machine (Text-to-Speech)
- API key for OpenAI API (ChatGPT and Speech-to-Text)
- Python 3.10 (Runtime)
Install AIAvatarKit.
pip install git+https://github.com/uezo/[email protected]
NOTE: Since technical blogs assume v0.5.8, the PyPI version will remain based on v0.5.8 during the transition period. We plan to update to the v0.6 series around May 2025.
Make the script as run.py
.
import asyncio
from aiavatar import AIAvatar
aiavatar_app = AIAvatar(
openai_api_key=OPENAI_API_KEY,
debug=True
)
asyncio.run(aiavatar_app.start_listening())
Start AIAvatar. Also, don't forget to launch VOICEVOX beforehand.
$ python run.py
Conversation will start when you say the wake word "ใใใซใกใฏ" (or "Hello" when language is not ja-JP
).
Feel free to enjoy the conversation afterwards!
You can set model and system prompt when instantiate AIAvatar
.
aiavatar_app = AIAvatar(
openai_api_key="YOUR_OPENAI_API_KEY",
model="gpt-4o",
system_prompt="You are my cat."
)
If you want to configure in detail, create instance of ChatGPTService
with custom parameters and set it to AIAvatar
.
# Create ChatGPTService
from litests.llm.chatgpt import ChatGPTService
llm = ChatGPTService(
openai_api_key=OPENAI_API_KEY,
model="gpt-4o",
temperature=0.0,
system_prompt="You are my cat."
)
# Create AIAvatar with ChatGPTService
aiavatar_app = AIAvatar(
llm=llm,
openai_api_key=OPENAI_API_KEY # API Key for STT
)
Create instance of ClaudeService
with custom parameters and set it to AIAvatar
. The default model is claude-3-5-sonnet-latest
.
# Create ClaudeService
from litests.llm.claude import ClaudeService
llm = ClaudeService(
anthropic_api_key=ANTHROPIC_API_KEY,
model="claude-3-7-sonnet-20250219",
temperature=0.0,
system_prompt="You are my cat."
)
# Create AIAvatar with ClaudeService
aiavatar_app = AIAvatar(
llm=llm,
openai_api_key=OPENAI_API_KEY # API Key for STT
)
NOTE: We support Claude on Anthropic API, not Amazon Bedrock for now. Use LiteLLM or other API Proxies.
Create instance of GeminiService
with custom parameters and set it to AIAvatar
. The default model is gemini-2.0-flash-exp
.
# Create GeminiService
# pip install google-generativeai
from litests.llm.gemini import GeminiService
llm = GeminiService(
gemini_api_key=GEMINI_API_KEY,
model="gemini-2.0-pro-latest",
temperature=0.0,
system_prompt="You are my cat."
)
# Create AIAvatar with GeminiService
aiavatar_app = AIAvatar(
llm=llm,
openai_api_key=OPENAI_API_KEY # API Key for STT
)
NOTE: We support Gemini on Google AI Studio, not Vertex AI for now. Use LiteLLM or other API Proxies.
You can use the Dify API instead of a specific LLM's API. This eliminates the need to manage code for tools or RAG locally.
# Create DifyService
from litests.llm.dify import DifyService
llm = DifyService(
api_key=DIFY_API_KEY,
base_url=DIFY_URL,
user="aiavatarkit_user",
is_agent_mode=True
)
# Create AIAvatar with DifyService
aiavatar_app = AIAvatar(
llm=llm,
openai_api_key=OPENAI_API_KEY # API Key for STT
)
You can use other LLMs by using LiteLLMService
or implementing LLMService
interface.
See the details of LiteLLM here: https://github.com/BerriAI/litellm
You can set speaker id and the base url for VOICEVOX server when instantiate AIAvatar
.
aiavatar_app = AIAvatar(
openai_api_key="YOUR_OPENAI_API_KEY",
# 46 is Sayo. See http://127.0.0.1:50021/speakers to get all ids for characters
voicevox_speaker=46
)
If you want to configure in detail, create instance of VoicevoxSpeechSynthesizer
with custom parameters and set it to AIAvatar
.
Here is the example for AivisSpeech.
# Create VoicevoxSpeechSynthesizer with AivisSpeech configurations
from litests.tts.voicevox import VoicevoxSpeechSynthesizer
tts = VoicevoxSpeechSynthesizer(
base_url="http://127.0.0.1:10101", # Your AivisSpeech API server
speaker="888753761" # Anneli
)
# Create AIAvatar with VoicevoxSpeechSynthesizer
aiavatar_app = AIAvatar(
tts=tts,
openai_api_key=OPENAI_API_KEY # API Key for LLM and STT
)
You can also set speech controller that uses alternative Text-to-Speech services. We support Azure, Google, OpenAI and any other TTS services supported by SpeechGateway such as Style-Bert-VITS2 and NijiVoice.
from litests.tts.azure import AzureSpeechSynthesizer
from litests.tts.google import GoogleSpeechSynthesizer
from litests.tts.openai import OpenAISpeechSynthesizer
from litests.tts.speech_gateway import SpeechGatewaySpeechSynthesizer
You can also make custom tts components by impelemting SpeechSynthesizer
interface.
If you want to configure in detail, create instance of SpeechRecognizer
with custom parameters and set it to AIAvatar
. We support Azure, Google and OpenAI Speech-to-Text services.
NOTE: AzureSpeechRecognizer
is much faster than Google and OpenAI(default).
# Create AzureSpeechRecognizer
from litests.stt.azure import AzureSpeechRecognizer
stt = AzureSpeechRecognizer(
azure_api_key=AZURE_API_KEY,
azure_region=AZURE_REGION
)
# Create AIAvatar with AzureSpeechRecognizer
aiavatar_app = AIAvatar(
stt=stt,
openai_api_key=OPENAI_API_KEY # API Key for LLM
)
To control facial expressions within conversations, set the facial expression names and values in FaceController.faces
as shown below, and then include these expression keys in the response message by adding instructions to the prompt.
aiavatar_app.adapter.face_controller.faces = {
"neutral": "๐",
"joy": "๐",
"angry": "๐ ",
"sorrow": "๐",
"fun": "๐ฅณ"
}
aiavatar_app.sts.llm.system_prompt = """# Face Expression
* You have the following expressions:
- joy
- angry
- sorrow
- fun
* If you want to express a particular emotion, please insert it at the beginning of the sentence like [face:joy].
Example
[face:joy]Hey, you can see the ocean! [face:fun]Let's go swimming.
"""
This allows emojis like ๐ฅณ to be autonomously displayed in the terminal during conversations. To actually control the avatar's facial expressions in a metaverse platform, instead of displaying emojis like ๐ฅณ, you will need to use custom implementations tailored to the integration mechanisms of each platform. Please refer to our VRChatFaceController
as an example.
Now writing... โ๏ธ
AIAvatarKit is capable of operating on any platform that allows applications to hook into audio input and output. The platforms that have been tested include:
- VRChat
- cluster
- Vket Cloud
In addition to running on PCs to operate AI avatars on these platforms, you can also create a communication robot by connecting speakers, a microphone, and, if possible, a display to a Raspberry Pi.
- 2 Virtual audio devices (e.g. VB-CABLE) are required.
- Multiple VRChat accounts are required to chat with your AIAvatar.
First, run the commands below in python interpreter to check the audio devices.
$ python
>>> from aiavatar import AudioDevice
>>> AudioDevice().list_audio_devices()
0: Headset Microphone (Oculus Virt
:
6: CABLE-B Output (VB-Audio Cable
7: Microsoft ใตใฆใณใ ใใใใผ - Output
8: SONY TV (NVIDIA High Definition
:
13: CABLE-A Input (VB-Audio Cable A
:
In this example,
- To use
VB-Cable-A
for microphone for VRChat, index foroutput_device
is13
(CABLE-A Input). - To use
VB-Cable-B
for speaker for VRChat, index forinput_device
is6
(CABLE-B Output). Don't forget to setVB-Cable-B Input
as the default output device of Windows OS.
Then edit run.py
like below.
# Create AIAvatar
aiavatar_app = AIAvatar(
openai_api_key=OPENAI_API_KEY,
input_device=6, # Listen sound from VRChat
output_device=13, # Speak to VRChat microphone
)
Run it.
$ run.py
Launch VRChat as desktop mode on the machine that runs run.py
and log in with the account for AIAvatar. Then set VB-Cable-A
to microphone in VRChat setting window.
That's all! Let's chat with the AIAvatar. Log in to VRChat on another machine (or Quest) and go to the world the AIAvatar is in.
AIAvatarKit controls the face expression by Avatar OSC.
LLM(ChatGPT/Claude/Gemini)
โ response with face tag [face:joy]Hello!
AIAvatarKit(VRCFaceExpressionController)
โ osc FaceOSC=1
VRChat(FX AnimatorController)
โ
๐
So at first, setup your avatar the following steps:
- Add avatar parameter
FaceOSC
(type: int, default value: 0, saved: false, synced: true). - Add
FaceOSC
parameter to the FX animator controller. - Add layer and put states and transitions for face expression to the FX animator controller.
- (option) If you use the avatar that is already used in VRChat, add input parameter configuration to avatar json.
Next, use VRChatFaceController
.
from aiavatar.face.vrchat import VRChatFaceController
# Setup VRChatFaceContorller
vrc_face_controller = VRChatFaceController(
faces={
"neutral": 0, # always set `neutral: 0`
# key = the name that LLM can understand the expression
# value = FaceOSC value that is set to the transition on the FX animator controller
"joy": 1,
"angry": 2,
"sorrow": 3,
"fun": 4
}
)
Lastly, add face expression section to the system prompt.
# Make system prompt
system_prompt = """
# Face Expression
* You have following expressions:
- joy
- angry
- sorrow
- fun
* If you want to express a particular emotion, please insert it at the beginning of the sentence like [face:joy].
Example
[face:joy]Hey, you can see the ocean! [face:fun]Let's go swimming.
"""
# Set them to AIAvatar
aiavatar_app = AIAvatar(
openai_api_key=OPENAI_API_KEY,
face_controller=vrc_face_controller,
system_prompt=system_prompt
)
You can test it not only through the voice conversation but also via the REST API.
Now writing... โ๏ธ
Advanced usases.
Register tool with spec by @aiavatar_app.sts.llm.tool
. The spec should be in the format for each LLM.
# Spec (for ChatGPT)
weather_tool_spec = {
"type": "function",
"function": {
"name": "get_weather",
"parameters": {
"type": "object",
"properties": {
"location": {"type": "string"}
},
},
}
}
# Implement tool and register it with spec
@aiavatar_app.sts.llm.tool(weather_tool_spec) # NOTE: Gemini doesn't take spec as argument
async def get_weather(location: str = None):
weather = await weather_api(location=location) # Call weather API
return weather # {"weather": "clear", "temperature": 23.4}
AIAvatarKit captures and sends image to AI dynamically when the AI determine that vision is required to process the request. This gives "eyes" to your AIAvatar in metaverse platforms like VRChat.
# Instruct vision tag in the system message
SYSTEM_PROMPR = """
## Using Vision
If you need an image to process a user's request, you can obtain it using the following methods:
- screenshot
- camera
If an image is needed to process the request, add an instruction like [vision:screenshot] to your response to request an image from the user.
By adding this instruction, the user will provide an image in their next utterance. No comments about the image itself are necessary.
Example:
user: Look! This is the sushi I had today.
assistant: [vision:screenshot] Let me take a look.
"""
# Create AIAvatar with the system prompt
aiavatar_app = AIAvatar(
system_prompt=SYSTEM_PROMPT,
openai_api_key=OPENAI_API_KEY
)
# Implement get_image_url
import base64
import io
import pyautogui # pip install pyautogui
from aiavatar.device.video import VideoDevice # pip install opencv-python
default_camera = VideoDevice(device_index=0, width=960, height=540)
@aiavatar_app.adapter.get_image_url
async def get_image_url(source: str) -> str:
image_bytes = None
if source == "camera":
# Capture photo by camera
image_bytes = await default_camera.capture_image("camera.jpg")
elif source == "screenshot":
# Capture screenshot
buffered = io.BytesIO()
image = pyautogui.screenshot(region=(0, 0, 1280, 720))
image.save(buffered, format="PNG")
image_bytes = buffered.getvalue()
if image_bytes:
# Upload and get url, or, make base64 encoded url
b64_encoded = base64.b64encode(image_bytes).decode('utf-8')
b64_url = f"data:image/jpeg;base64,{b64_encoded}"
return b64_url
Set wakewords
when instantiating AIAvatar
. Conversation will start when the AIAvatar recognizes one of the words in this list. You can also set wakeword_timeout
, after which the AIAvatar will return to listening for the wakeword again.
aiavatar_app = AIAvatar(
openai_api_key=OPENAI_API_KEY,
wakewords=["Hello", "ใใใซใกใฏ"],
wakeword_timeout=60,
)
You can specify the audio devices to be used in components by device index.
First, check the device indexes you want to use.
$ python
>>> from aiavatar import AudioDevice
>>> AudioDevice().list_audio_devices()
{'index': 0, 'name': 'ๅค้จใใคใฏ', 'max_input_channels': 1, 'max_output_channels': 0, 'default_sample_rate': 44100.0}
{'index': 1, 'name': 'ๅค้จใใใใใฉใณ', 'max_input_channels': 0, 'max_output_channels': 2, 'default_sample_rate': 44100.0}
{'index': 2, 'name': 'MacBook Airใฎใใคใฏ', 'max_input_channels': 3, 'max_output_channels': 0, 'default_sample_rate': 44100.0}
{'index': 3, 'name': 'MacBook Airใฎในใใผใซใผ', 'max_input_channels': 0, 'max_output_channels': 2, 'default_sample_rate': 44100.0}
Set indexes to AIAvatar.
aiavatar_app = AIAvatar(
input_device=2, # MacBook Airใฎใใคใฏ
output_device=3, # MacBook Airใฎในใใผใซใผ
openai_api_key=OPENAI_API_KEY
)
You can host AIAvatarKit on a server to enable multiple clients to have independent context-aware conversations via RESTful API with streaming responses (Server-Sent Events).
Below is the simplest example of a server program:
from fastapi import FastAPI
from aiavatar.adapter.http.server import AIAvatarHttpServer
# AIAvatar
aiavatar_app = AIAvatarHttpServer(
openai_api_key=OPENAI_API_KEY,
debug=True
)
# Setup FastAPI app with AIAvatar components
app = FastAPI()
router = aiavatar_app.get_api_router()
app.include_router(router)
Save the above code as server.py
and run it using:
uvicorn server:app
Next is the simplest example of a client program:
import asyncio
from aiavatar.adapter.http.client import AIAvatarHttpClient
aiavatar_app = AIAvatarHttpClient(
debug=True
)
asyncio.run(aiavatar_app.start_listening(session_id="http_session", user_id="http_user"))
Save the above code as client.py
and run it using:
python client.py
You can now perform voice interactions just like when running locally.
When using the streaming API via HTTP, clients communicate with the server using JSON-formatted requests.
Below is the format for initiating a session:
{
"type": "start", // Always `start`
"session_id": "6d8ba9ac-a515-49be-8bf4-cdef021a169d",
"user_id": "user_id",
"context_id": "c37ac363-5c65-4832-aa25-fd3bbbc1b1e7", // Set null or provided id in `start` response
"text": "ใใใซใกใฏ", // If set, audio_data will be ignored
"audio_data": "XXXX", // Base64 encoded audio data
"files": [
{
"type": "image", // Only `image` is supported for now
"url": "https://xxx",
}
],
"metadata": {}
}
The server returns responses as a stream of JSON objects in the following structure.
The communication flow typically consists of:
{
"type": "chunk", // start -> chunk -> final
"session_id": "6d8ba9ac-a515-49be-8bf4-cdef021a169d",
"user_id": "user01",
"context_id": "c37ac363-5c65-4832-aa25-fd3bbbc1b1e7",
"text": "[face:joy]ใใใซใกใฏ๏ผ", // Response text with info
"voice_text": "ใใใซใกใฏ๏ผ", // Response text for voice synthesis
"avatar_control_request": {
"animation_name": null, // Parsed animation name
"animation_duration": null, // Parsed duration for animation
"face_name": "joy", // Parsed facial expression name
"face_duration": 4.0 // Parsed duration for the facial expression
},
"audio_data": "XXXX", // Base64 encoded. Playback this as the character's voice.
"metadata": {
"is_first_chunk": true
}
}
You can test the streaming API using a simple curl command:
curl -N -X POST http://127.0.0.1:8000/chat \
-H "Content-Type: application/json" \
-d '{
"type": "start",
"session_id": "6d8ba9ac-a515-49be-8bf4-cdef021a169d",
"user_id": "user01",
"text": "ใใใซใกใฏ"
}'
Sample response (streamed from the server):
data: {"type": "start", "session_id": "6d8ba9ac-a515-49be-8bf4-cdef021a169d", "user_id": "user01", "context_id": "c37ac363-5c65-4832-aa25-fd3bbbc1b1e7", "text": null, "voice_text": null, "avatar_control_request": null, "audio_data": "XXXX", "metadata": {"request_text": "ใใใซใกใฏ"}}
data: {"type": "chunk", "session_id": "6d8ba9ac-a515-49be-8bf4-cdef021a169d", "user_id": "user01", "context_id": "c37ac363-5c65-4832-aa25-fd3bbbc1b1e7", "text": "[face:joy]ใใใซใกใฏ๏ผ", "voice_text": "ใใใซใกใฏ๏ผ", "avatar_control_request": {"animation_name": null, "animation_duration": null, "face_name": "joy", "face_duration": 4.0}, "audio_data": "XXXX", "metadata": {"is_first_chunk": true}}
data: {"type": "chunk", "session_id": "6d8ba9ac-a515-49be-8bf4-cdef021a169d", "user_id": "user01", "context_id": "c37ac363-5c65-4832-aa25-fd3bbbc1b1e7", "text": "ไปๆฅใฏใฉใใชใใจใใๆไผใใใพใใใใ๏ผ", "voice_text": "ไปๆฅใฏใฉใใชใใจใใๆไผใใใพใใใใ๏ผ", "avatar_control_request": {"animation_name": null, "animation_duration": null, "face_name": null, "face_duration": null}, "audio_data": "XXXX", "metadata": {"is_first_chunk": false}}
data: {"type": "final", "session_id": "6d8ba9ac-a515-49be-8bf4-cdef021a169d", "user_id": "user01", "context_id": "c37ac363-5c65-4832-aa25-fd3bbbc1b1e7", "text": "[face:joy]ใใใซใกใฏ๏ผไปๆฅใฏใฉใใชใใจใใๆไผใใใพใใใใ๏ผ", "voice_text": "ใใใซใกใฏ๏ผไปๆฅใฏใฉใใชใใจใใๆไผใใใพใใใใ๏ผ", "avatar_control_request": null, "audio_data": "XXXX", "metadata": {}}
To continue the conversation, include the context_id
provided in the start
response in your next request.
NOTE: When using the RESTful API, voice activity detection (VAD) must be performed client-side.
You can host AIAvatarKit on a server to enable multiple clients to have independent context-based conversations via WebSocket.
Below is the simplest example of a server program:
from fastapi import FastAPI
from aiavatar.adapter.websocket.server import AIAvatarWebSocketServer
# Create AIAvatar
aiavatar_app = AIAvatarWebSocketServer(
openai_api_key=OPENAI_API_KEY,
volume_db_threshold=-30, # <- Adjust for your audio env
debug=True
)
# Set router to FastAPI app
app = FastAPI()
router = aiavatar_app.get_websocket_router()
app.include_router(router)
Save the above code as server.py
and run it using:
uvicorn server:app
Next is the simplest example of a client program:
import asyncio
from aiavatar.adapter.websocket.client import AIAvatarWebSocketClient
client = AIAvatarWebSocketClient()
asyncio.run(client.start_listening(session_id="ws_session", user_id="ws_user"))
Save the above code as client.py
and run it using:
python client.py
You can now perform voice interactions just like when running locally.
NOTE: When using the WebSocket API, voice activity detection (VAD) is performed on the server side, so clients can simply stream microphone input directly to the server.
You can invoke custom implementations on_response(response_type)
. In the following example, show "thinking" face expression while processing request to enhance the interaction experience with the AI avatar.
# Set face when the character is thinking the answer
@aiavatar_app.on_response("start")
async def on_start_response(response):
await aiavatar_app.adapter.face_controller.set_face("thinking", 3.0)
# Reset face before answering
@aiavatar_app.on_response("chunk")
async def on_chunk_response(response):
if response.metadata.get("is_first_chunk"):
aiavatar_app.adapter.face_controller.reset()
NOTE: Not ready for v0.6.x
You can control AIAvatar via RESTful APIs. The provided functions are:
-
Lister
- start: Start Listener
- stop: Stop Listener
- status: Show status of Listener
-
Avatar
- face: Set face expression
- animation: Set animation
-
System
- log: Show recent logs
To use REST APIs, create API app and set router instead of calling aiavatar_app.start_listening()
.
from fastapi import FastAPI
from aiavatar import AIAvatar
from aiavatar.api.router import get_router
aiavatar_app = AIAvatar(
openai_api_key=OPENAI_API_KEY
)
# aiavatar_app.start_listening()
# Create API app and set router
api = FastAPI()
api_router = get_router(aiavatar_app, "aiavatar.log")
api.include_router(api_router)
Start API with uvicorn.
$ uvicorn run:api
Call /wakeword/start
to start wakeword listener.
$ curl -X 'POST' \
'http://127.0.0.1:8000/wakeword/start' \
-H 'accept: application/json' \
-H 'Content-Type: application/json' \
-d '{
"wakewords": []
}'
See API spec and try it on http://127.0.0.1:8000/docs .
AIAvatarKit automatically adjusts the noise filter for listeners when you instantiate an AIAvatar object. To manually set the noise filter level for voice detection, set auto_noise_filter_threshold
to False
and specify the volume_threshold_db
in decibels (dB).
aiavatar_app = AIAvatar(
openai_api_key=OPENAI_API_KEY,
auto_noise_filter_threshold=False,
volume_threshold_db=-40 # Set the voice detection threshold to -40 dB
)
For Tasks:
Click tags to check more tools for each tasksFor Jobs:
Alternative AI tools for aiavatarkit
Similar Open Source Tools
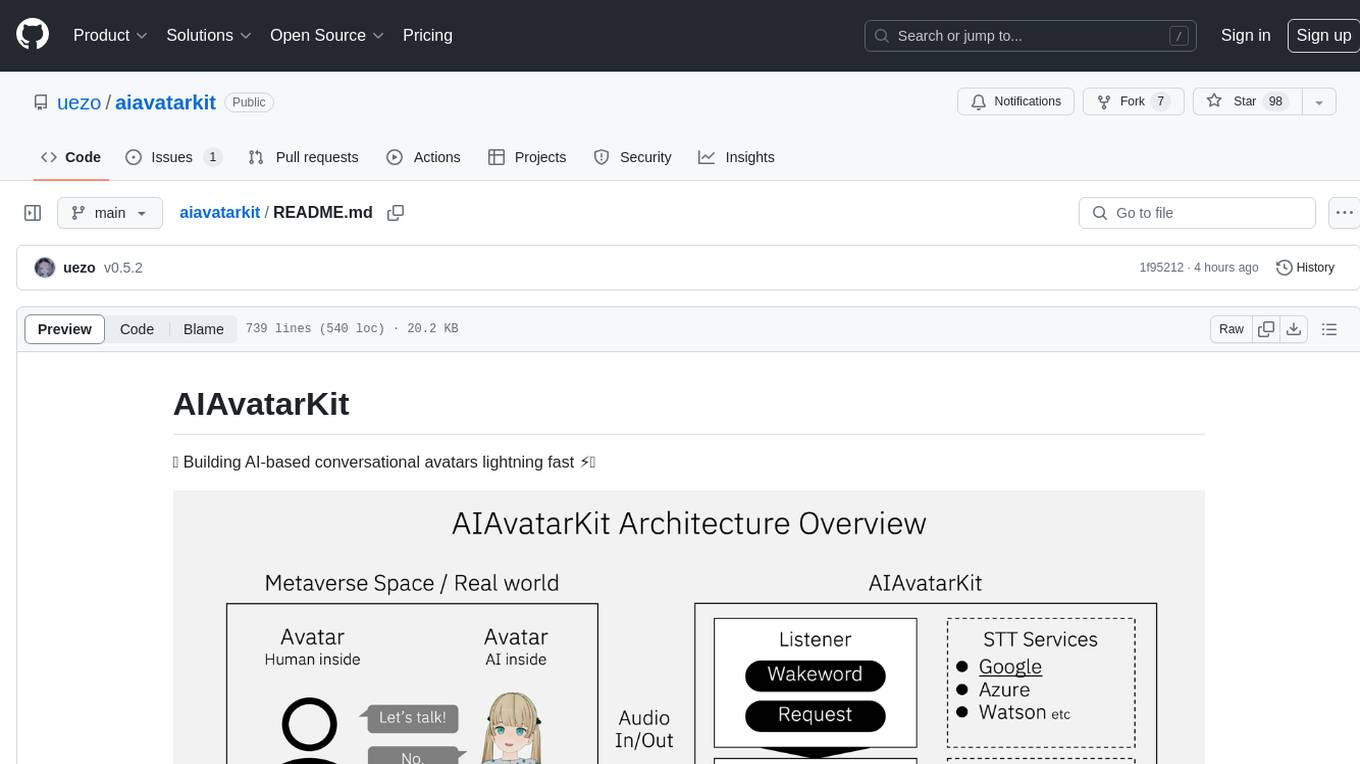
aiavatarkit
AIAvatarKit is a tool for building AI-based conversational avatars quickly. It supports various platforms like VRChat and cluster, along with real-world devices. The tool is extensible, allowing unlimited capabilities based on user needs. It requires VOICEVOX API, Google or Azure Speech Services API keys, and Python 3.10. Users can start conversations out of the box and enjoy seamless interactions with the avatars.
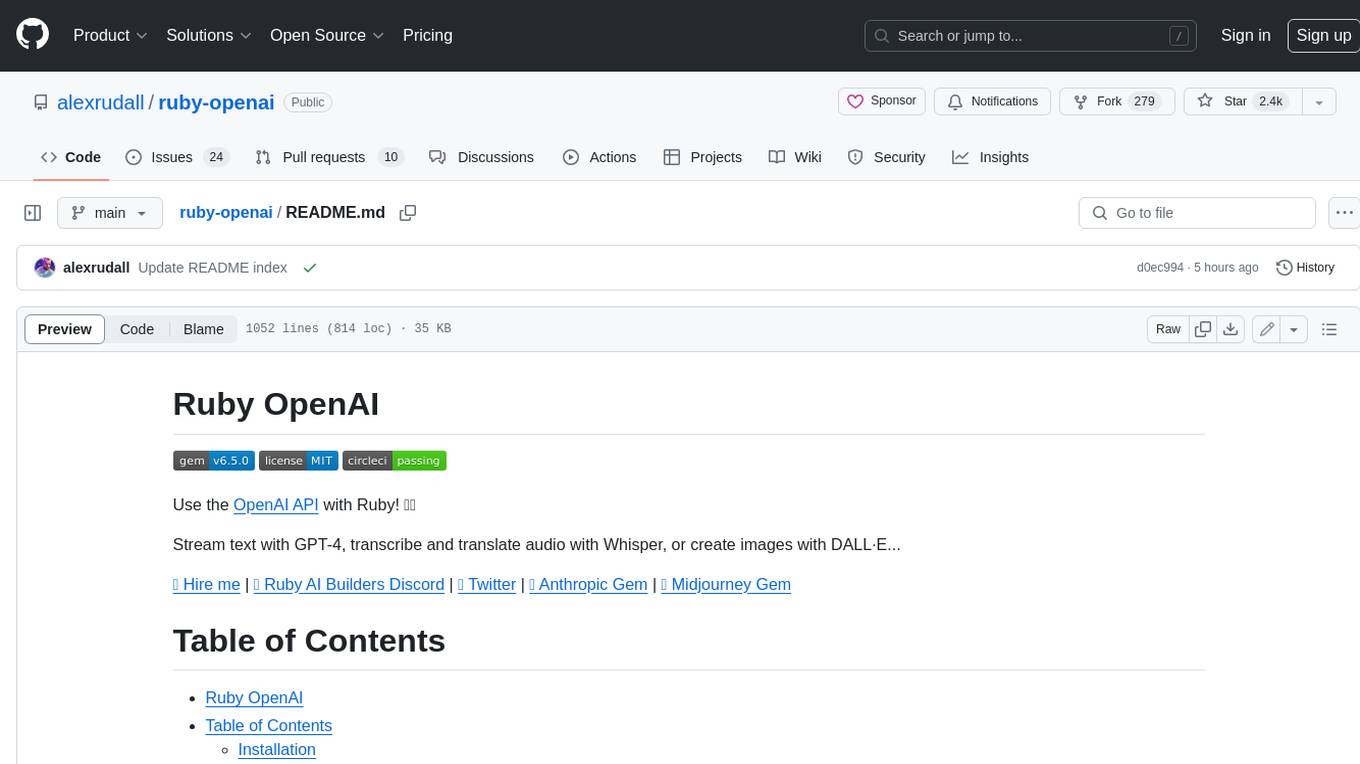
ruby-openai
Use the OpenAI API with Ruby! ๐ค๐ฉต Stream text with GPT-4, transcribe and translate audio with Whisper, or create images with DALLยทE... Hire me | ๐ฎ Ruby AI Builders Discord | ๐ฆ Twitter | ๐ง Anthropic Gem | ๐ Midjourney Gem ## Table of Contents * Ruby OpenAI * Table of Contents * Installation * Bundler * Gem install * Usage * Quickstart * With Config * Custom timeout or base URI * Extra Headers per Client * Logging * Errors * Faraday middleware * Azure * Ollama * Counting Tokens * Models * Examples * Chat * Streaming Chat * Vision * JSON Mode * Functions * Edits * Embeddings * Batches * Files * Finetunes * Assistants * Threads and Messages * Runs * Runs involving function tools * Image Generation * DALLยทE 2 * DALLยทE 3 * Image Edit * Image Variations * Moderations * Whisper * Translate * Transcribe * Speech * Errors * Development * Release * Contributing * License * Code of Conduct
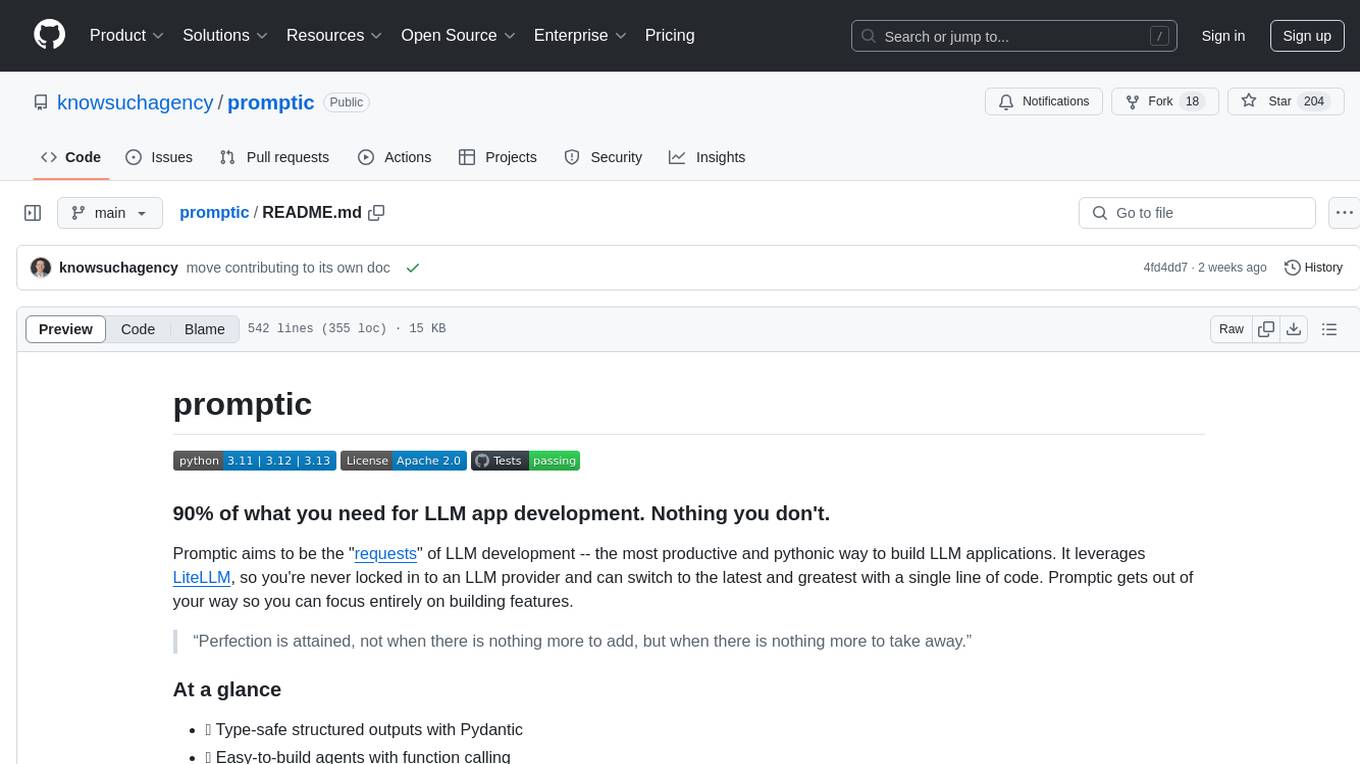
promptic
Promptic is a tool designed for LLM app development, providing a productive and pythonic way to build LLM applications. It leverages LiteLLM, allowing flexibility to switch LLM providers easily. Promptic focuses on building features by providing type-safe structured outputs, easy-to-build agents, streaming support, automatic prompt caching, and built-in conversation memory.
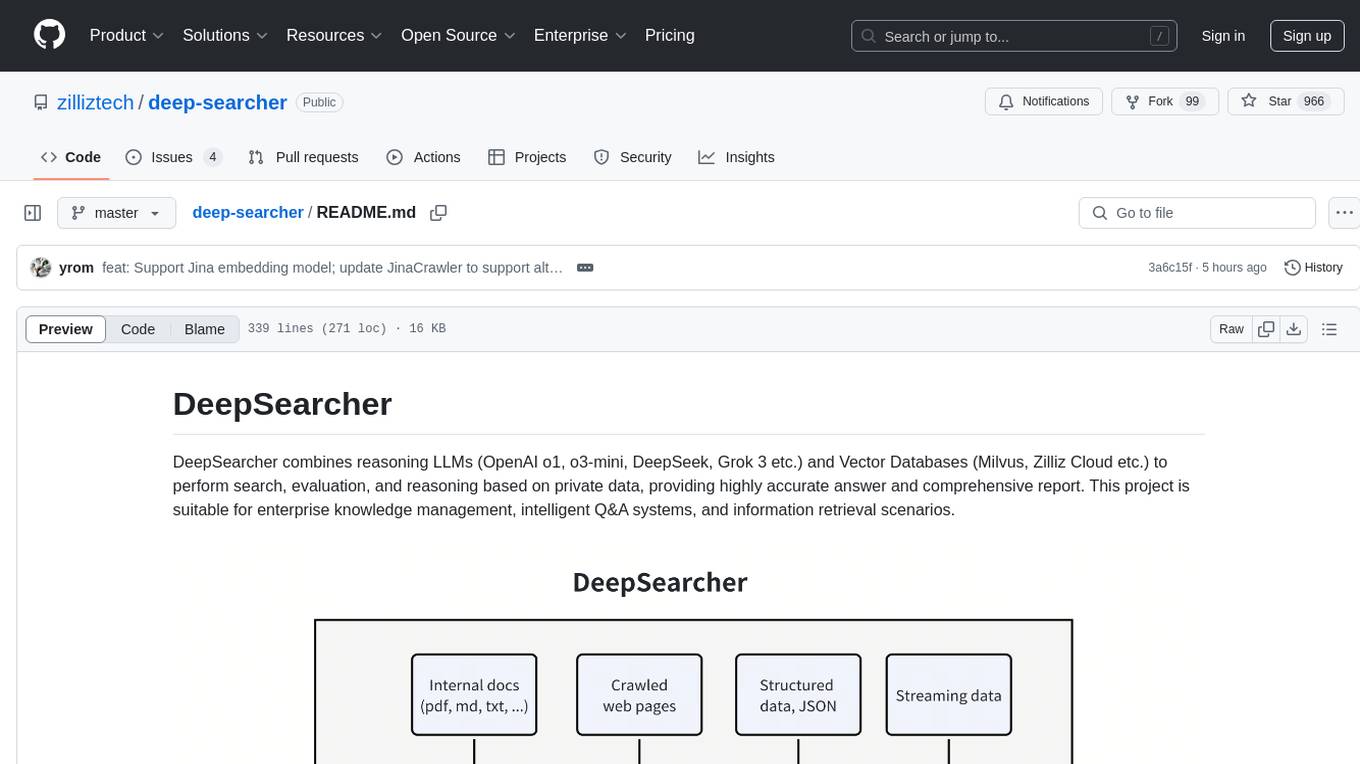
deep-searcher
DeepSearcher is a tool that combines reasoning LLMs and Vector Databases to perform search, evaluation, and reasoning based on private data. It is suitable for enterprise knowledge management, intelligent Q&A systems, and information retrieval scenarios. The tool maximizes the utilization of enterprise internal data while ensuring data security, supports multiple embedding models, and provides support for multiple LLMs for intelligent Q&A and content generation. It also includes features like private data search, vector database management, and document loading with web crawling capabilities under development.
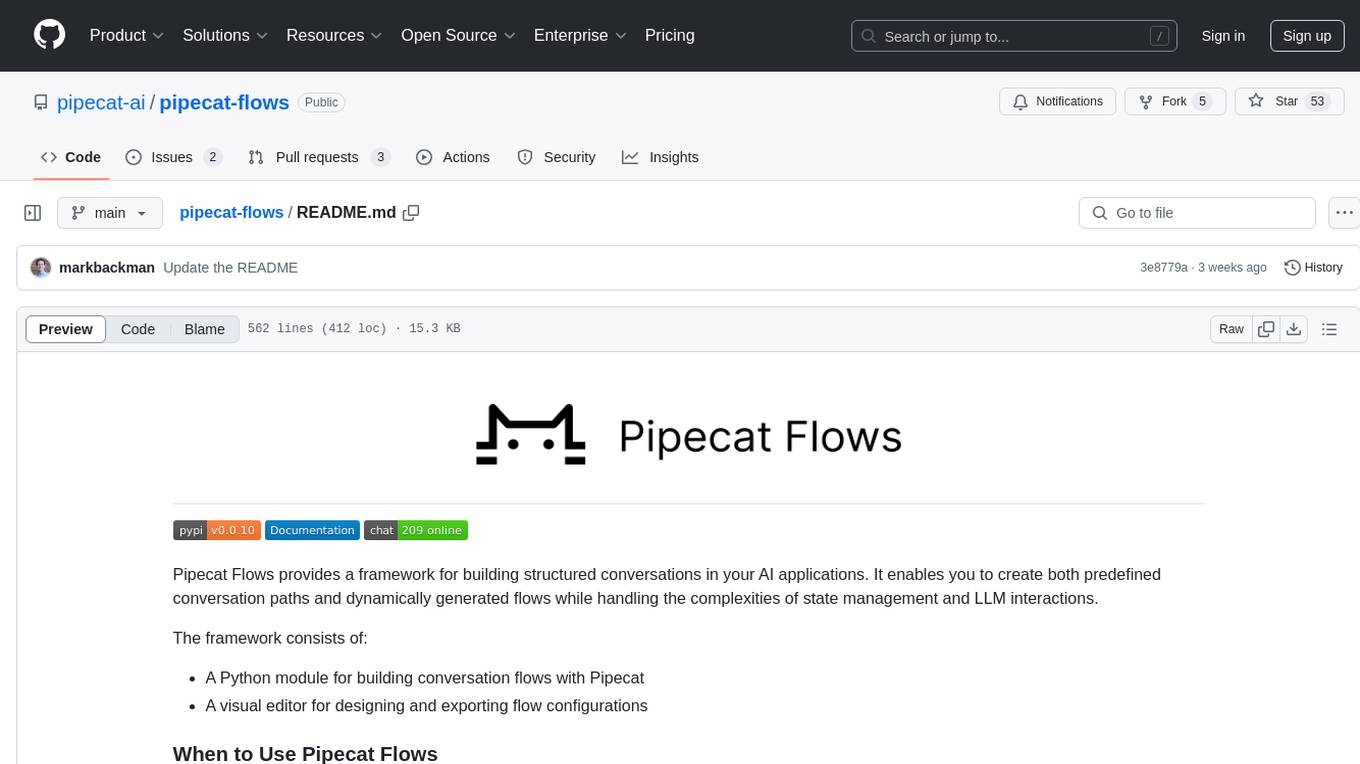
pipecat-flows
Pipecat Flows is a framework designed for building structured conversations in AI applications. It allows users to create both predefined conversation paths and dynamically generated flows, handling state management and LLM interactions. The framework includes a Python module for building conversation flows and a visual editor for designing and exporting flow configurations. Pipecat Flows is suitable for scenarios such as customer service scripts, intake forms, personalized experiences, and complex decision trees.
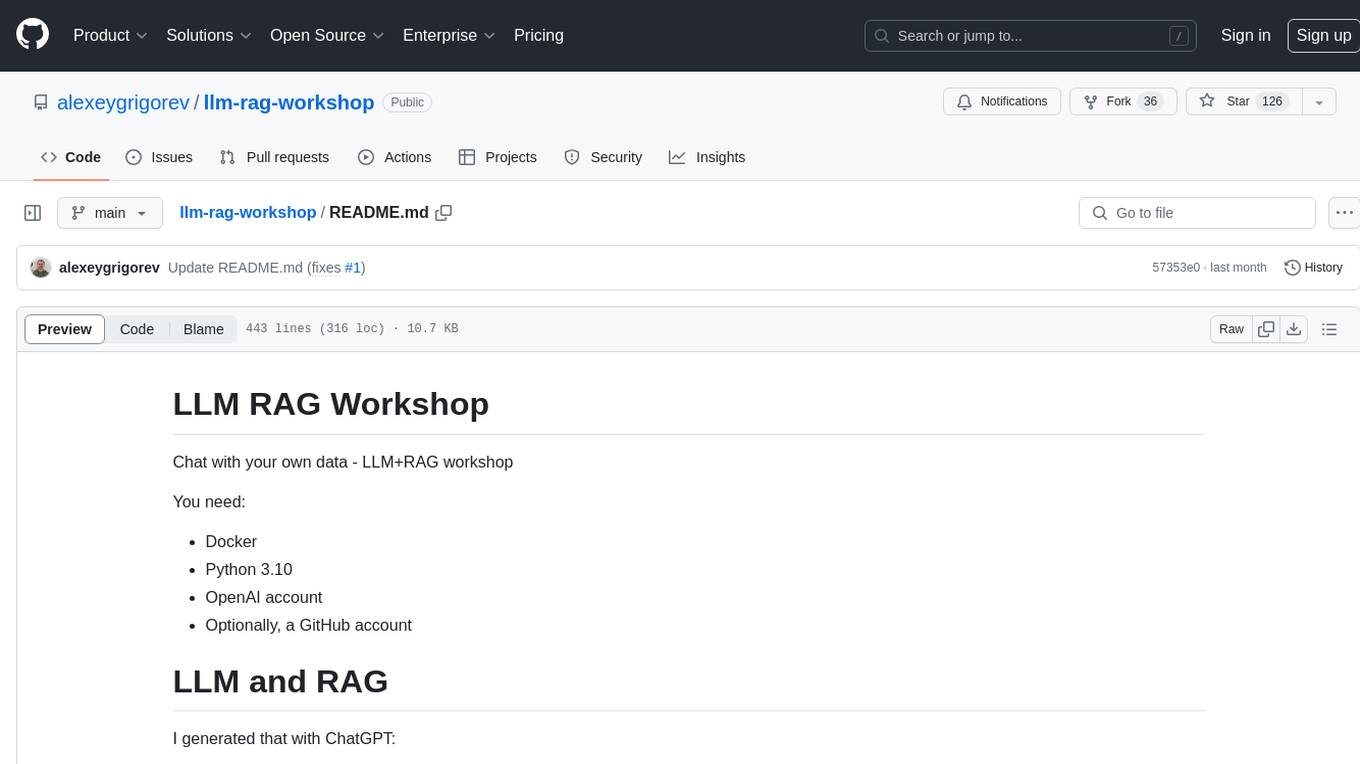
llm-rag-workshop
The LLM RAG Workshop repository provides a workshop on using Large Language Models (LLMs) and Retrieval-Augmented Generation (RAG) to generate and understand text in a human-like manner. It includes instructions on setting up the environment, indexing Zoomcamp FAQ documents, creating a Q&A system, and using OpenAI for generation based on retrieved information. The repository focuses on enhancing language model responses with retrieved information from external sources, such as document databases or search engines, to improve factual accuracy and relevance of generated text.
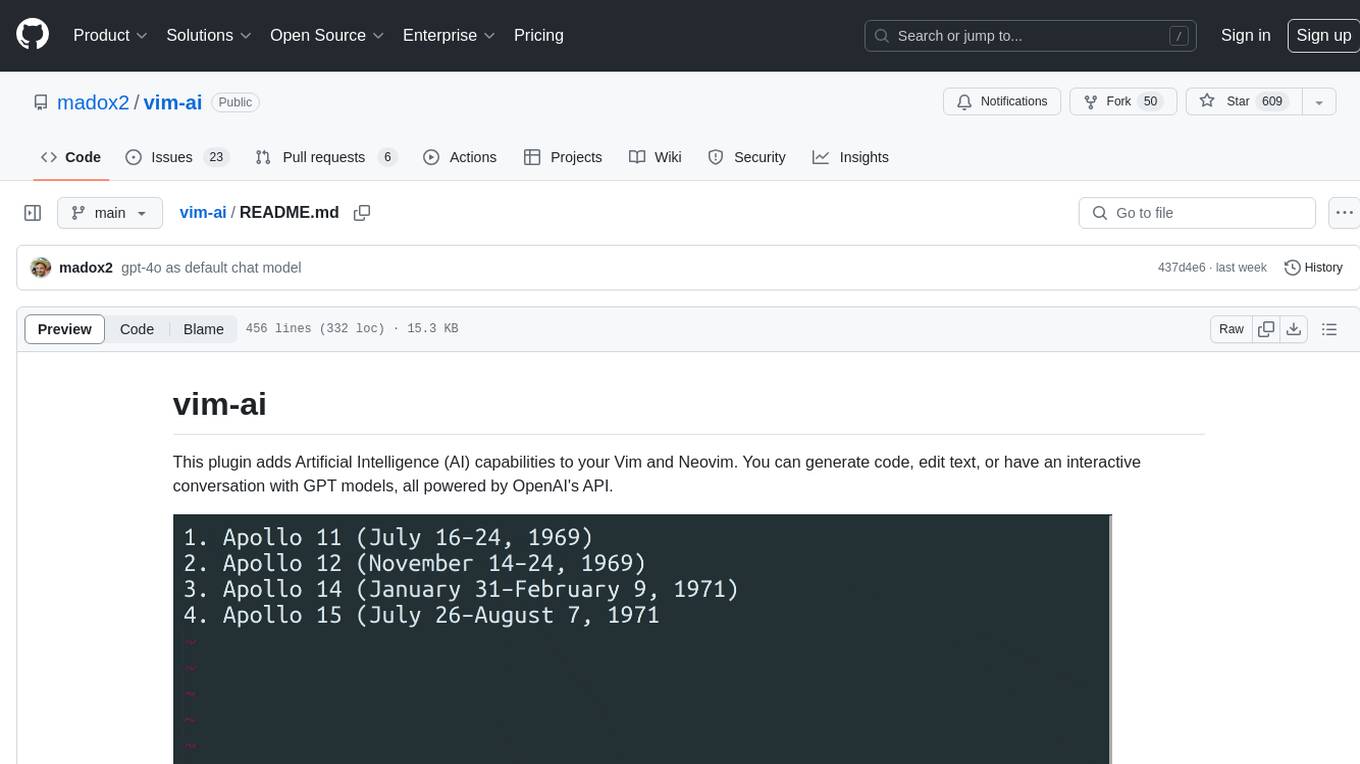
vim-ai
vim-ai is a plugin that adds Artificial Intelligence (AI) capabilities to Vim and Neovim. It allows users to generate code, edit text, and have interactive conversations with GPT models powered by OpenAI's API. The plugin uses OpenAI's API to generate responses, requiring users to set up an account and obtain an API key. It supports various commands for text generation, editing, and chat interactions, providing a seamless integration of AI features into the Vim text editor environment.
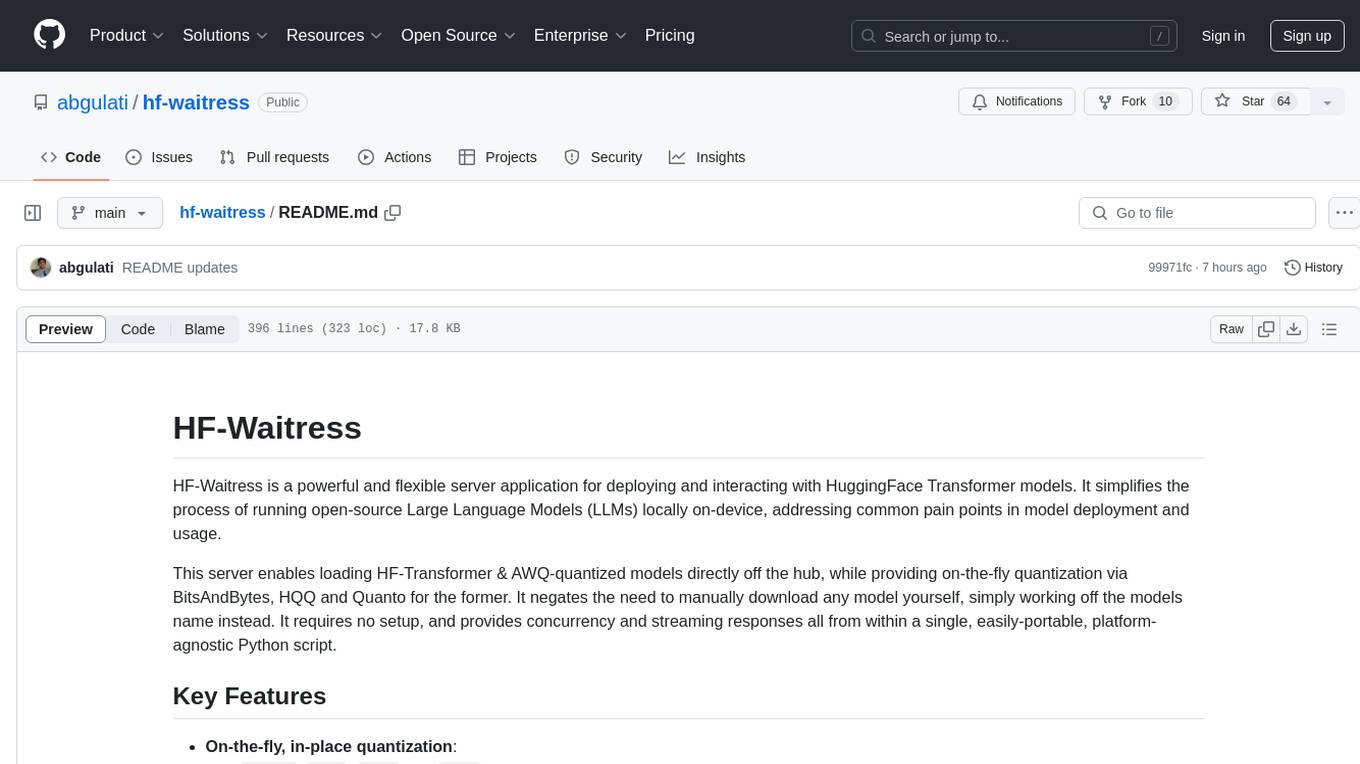
hf-waitress
HF-Waitress is a powerful server application for deploying and interacting with HuggingFace Transformer models. It simplifies running open-source Large Language Models (LLMs) locally on-device, providing on-the-fly quantization via BitsAndBytes, HQQ, and Quanto. It requires no manual model downloads, offers concurrency, streaming responses, and supports various hardware and platforms. The server uses a `config.json` file for easy configuration management and provides detailed error handling and logging.
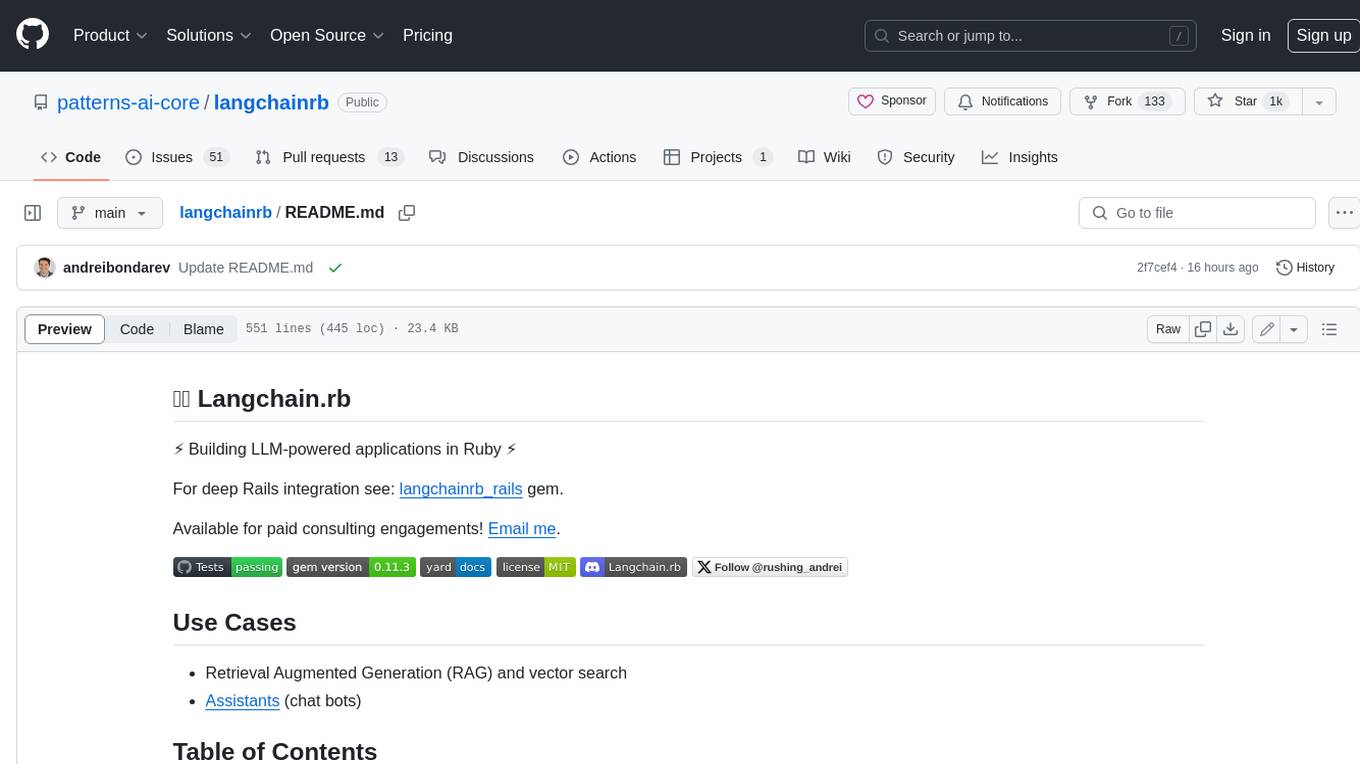
langchainrb
Langchain.rb is a Ruby library that makes it easy to build LLM-powered applications. It provides a unified interface to a variety of LLMs, vector search databases, and other tools, making it easy to build and deploy RAG (Retrieval Augmented Generation) systems and assistants. Langchain.rb is open source and available under the MIT License.
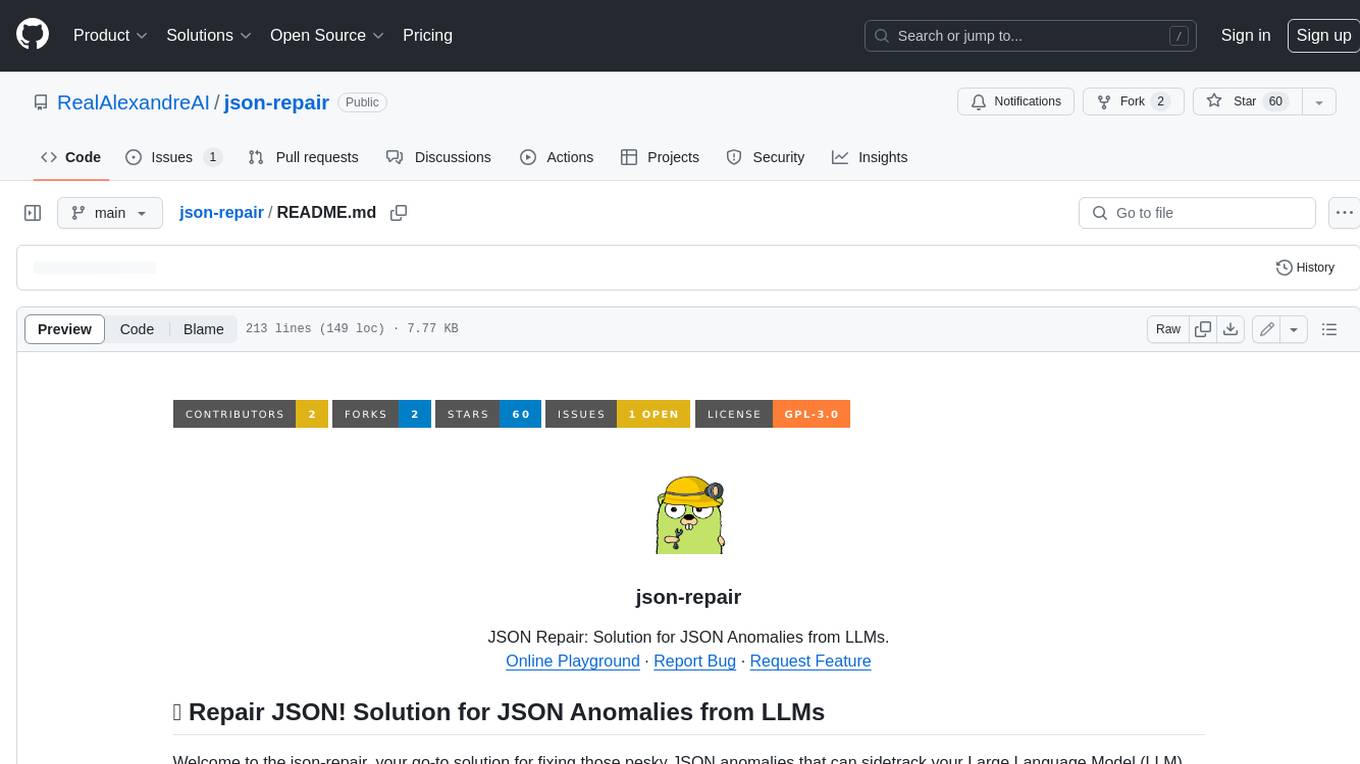
json-repair
JSON Repair is a toolkit designed to address JSON anomalies that can arise from Large Language Models (LLMs). It offers a comprehensive solution for repairing JSON strings, ensuring accuracy and reliability in your data processing. With its user-friendly interface and extensive capabilities, JSON Repair empowers developers to seamlessly integrate JSON repair into their workflows.
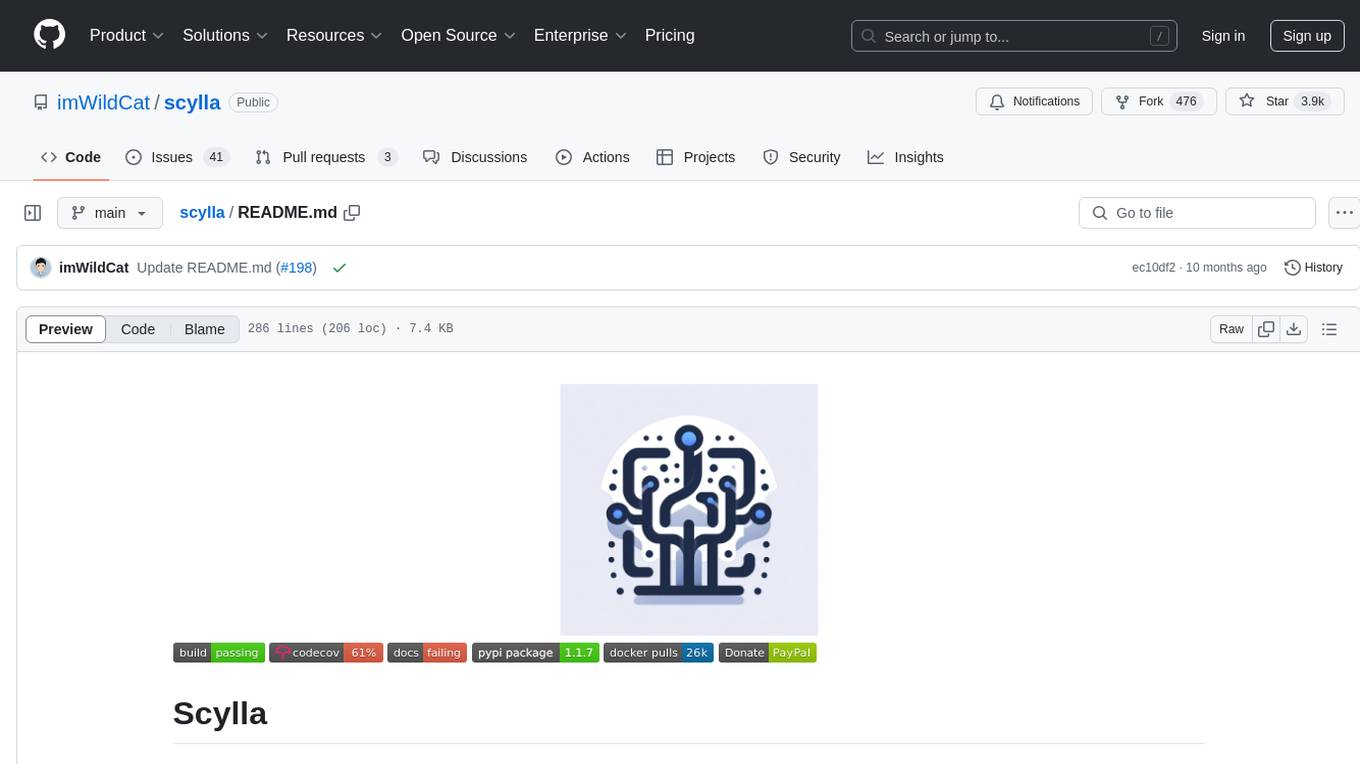
scylla
Scylla is an intelligent proxy pool tool designed for humanities, enabling users to extract content from the internet and build their own Large Language Models in the AI era. It features automatic proxy IP crawling and validation, an easy-to-use JSON API, a simple web-based user interface, HTTP forward proxy server, Scrapy and requests integration, and headless browser crawling. Users can start using Scylla with just one command, making it a versatile tool for various web scraping and content extraction tasks.
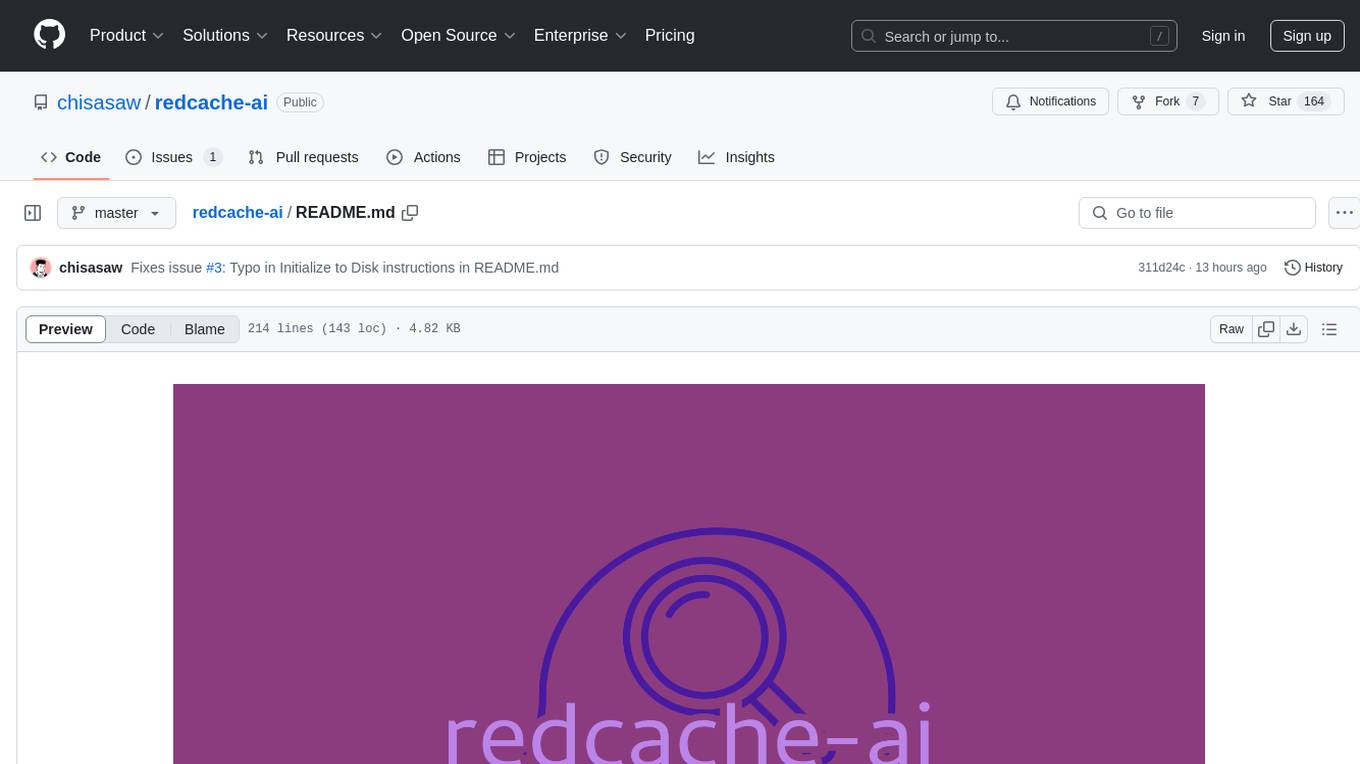
redcache-ai
RedCache-ai is a memory framework designed for Large Language Models and Agents. It provides a dynamic memory framework for developers to build various applications, from AI-powered dating apps to healthcare diagnostics platforms. Users can store, retrieve, search, update, and delete memories using RedCache-ai. The tool also supports integration with OpenAI for enhancing memories. RedCache-ai aims to expand its functionality by integrating with more LLM providers, adding support for AI Agents, and providing a hosted version.
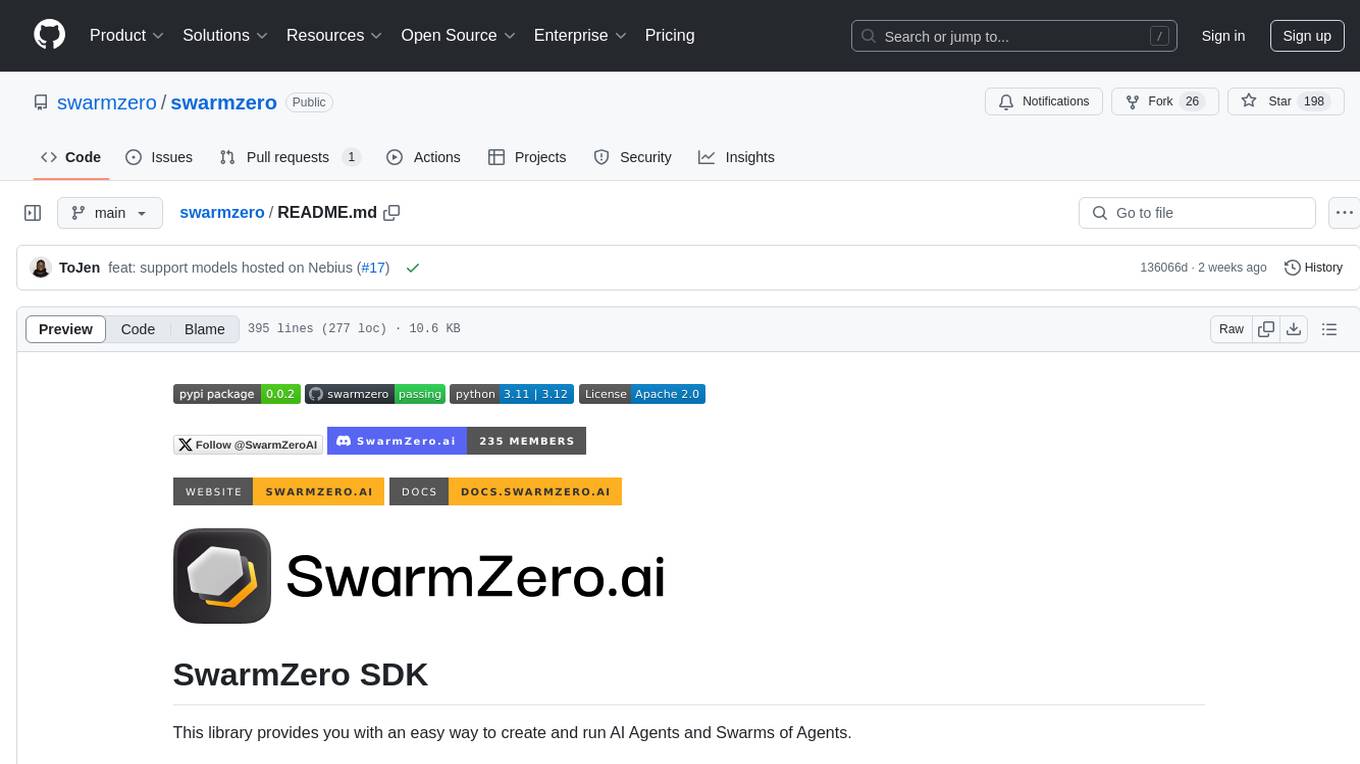
swarmzero
SwarmZero SDK is a library that simplifies the creation and execution of AI Agents and Swarms of Agents. It supports various LLM Providers such as OpenAI, Azure OpenAI, Anthropic, MistralAI, Gemini, Nebius, and Ollama. Users can easily install the library using pip or poetry, set up the environment and configuration, create and run Agents, collaborate with Swarms, add tools for complex tasks, and utilize retriever tools for semantic information retrieval. Sample prompts are provided to help users explore the capabilities of the agents and swarms. The SDK also includes detailed examples and documentation for reference.
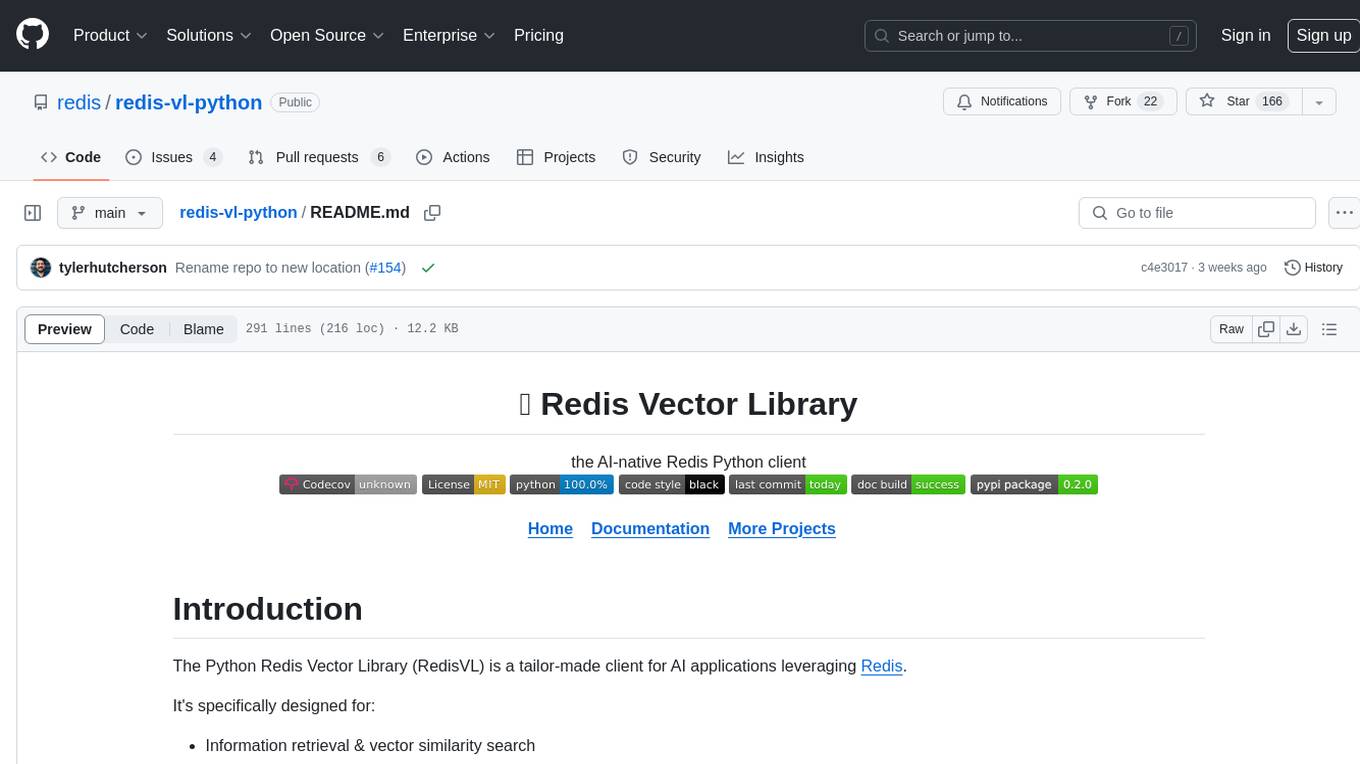
redis-vl-python
The Python Redis Vector Library (RedisVL) is a tailor-made client for AI applications leveraging Redis. It enhances applications with Redis' speed, flexibility, and reliability, incorporating capabilities like vector-based semantic search, full-text search, and geo-spatial search. The library bridges the gap between the emerging AI-native developer ecosystem and the capabilities of Redis by providing a lightweight, elegant, and intuitive interface. It abstracts the features of Redis into a grammar that is more aligned to the needs of today's AI/ML Engineers or Data Scientists.
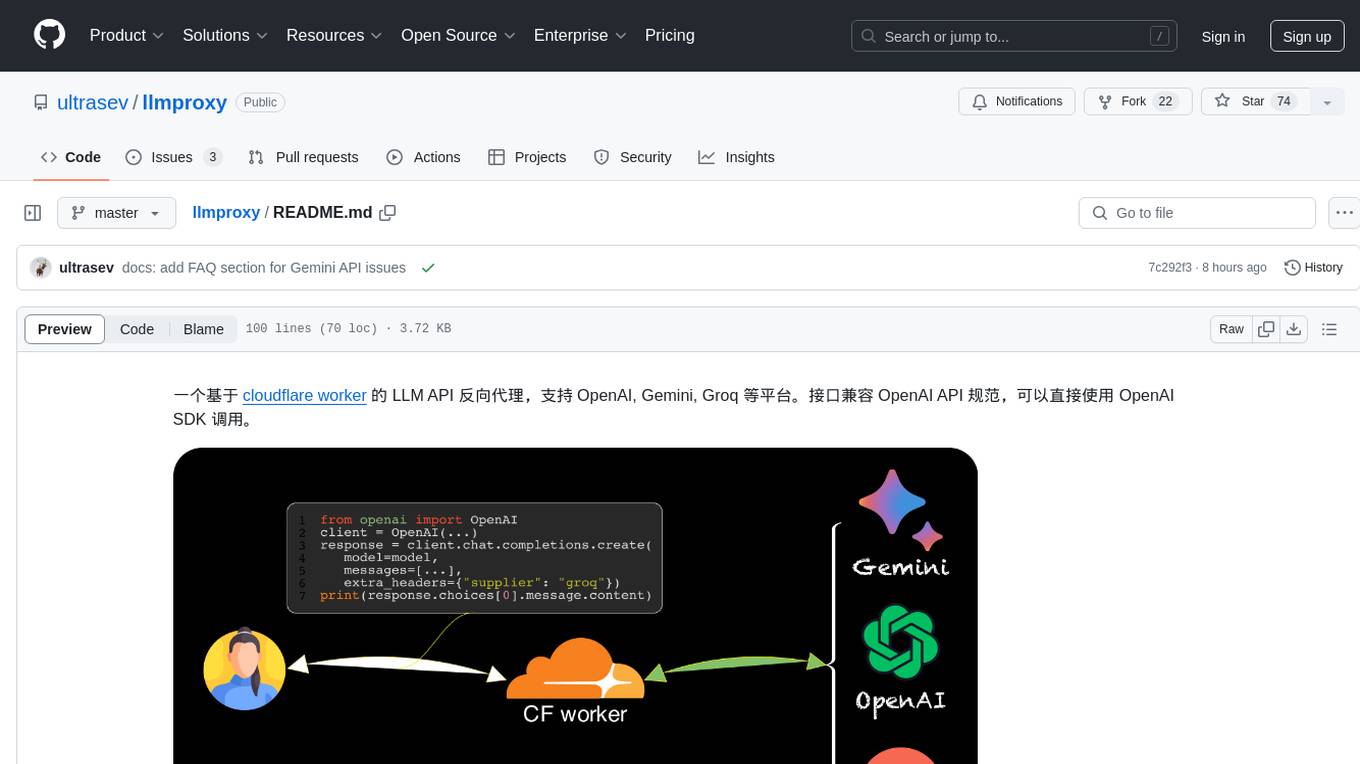
llmproxy
llmproxy is a reverse proxy for LLM API based on Cloudflare Worker, supporting platforms like OpenAI, Gemini, and Groq. The interface is compatible with the OpenAI API specification and can be directly accessed using the OpenAI SDK. It provides a convenient way to interact with various AI platforms through a unified API endpoint, enabling seamless integration and usage in different applications.
For similar tasks
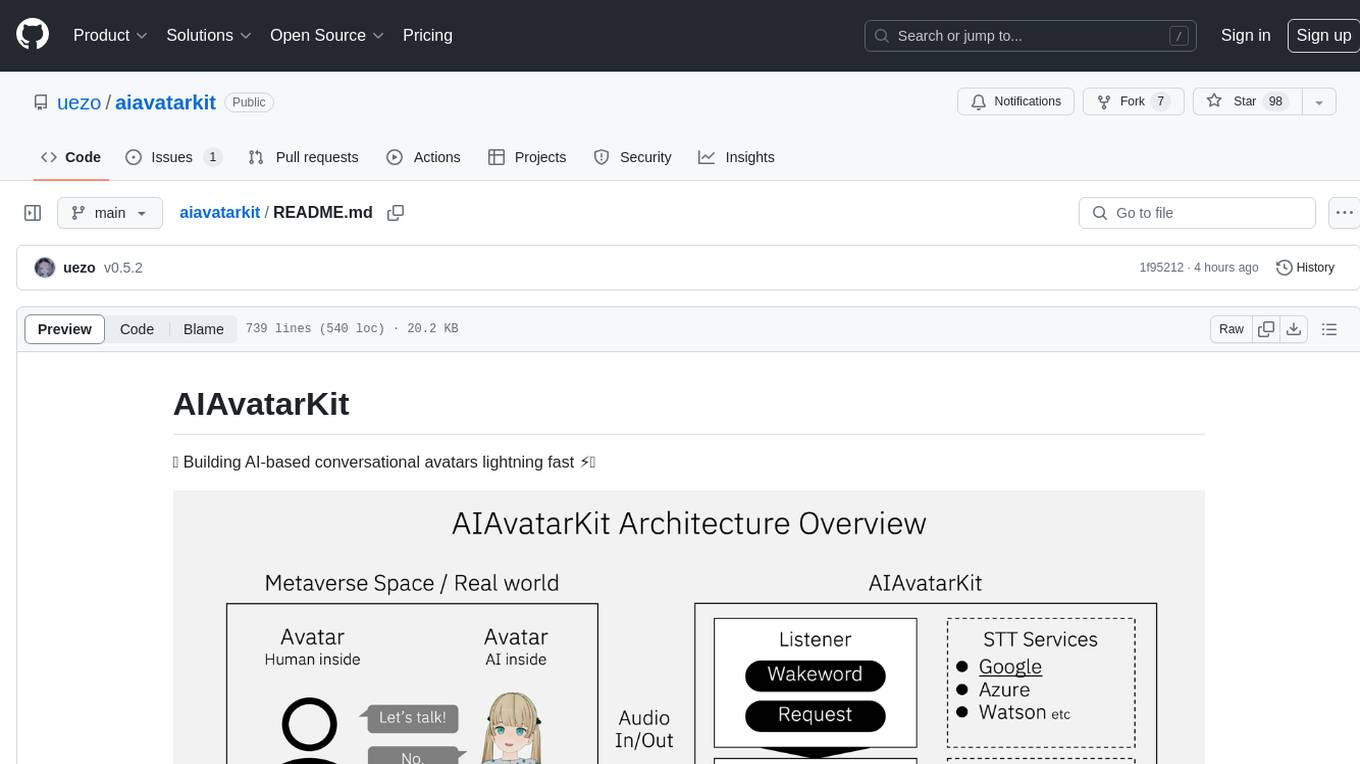
aiavatarkit
AIAvatarKit is a tool for building AI-based conversational avatars quickly. It supports various platforms like VRChat and cluster, along with real-world devices. The tool is extensible, allowing unlimited capabilities based on user needs. It requires VOICEVOX API, Google or Azure Speech Services API keys, and Python 3.10. Users can start conversations out of the box and enjoy seamless interactions with the avatars.
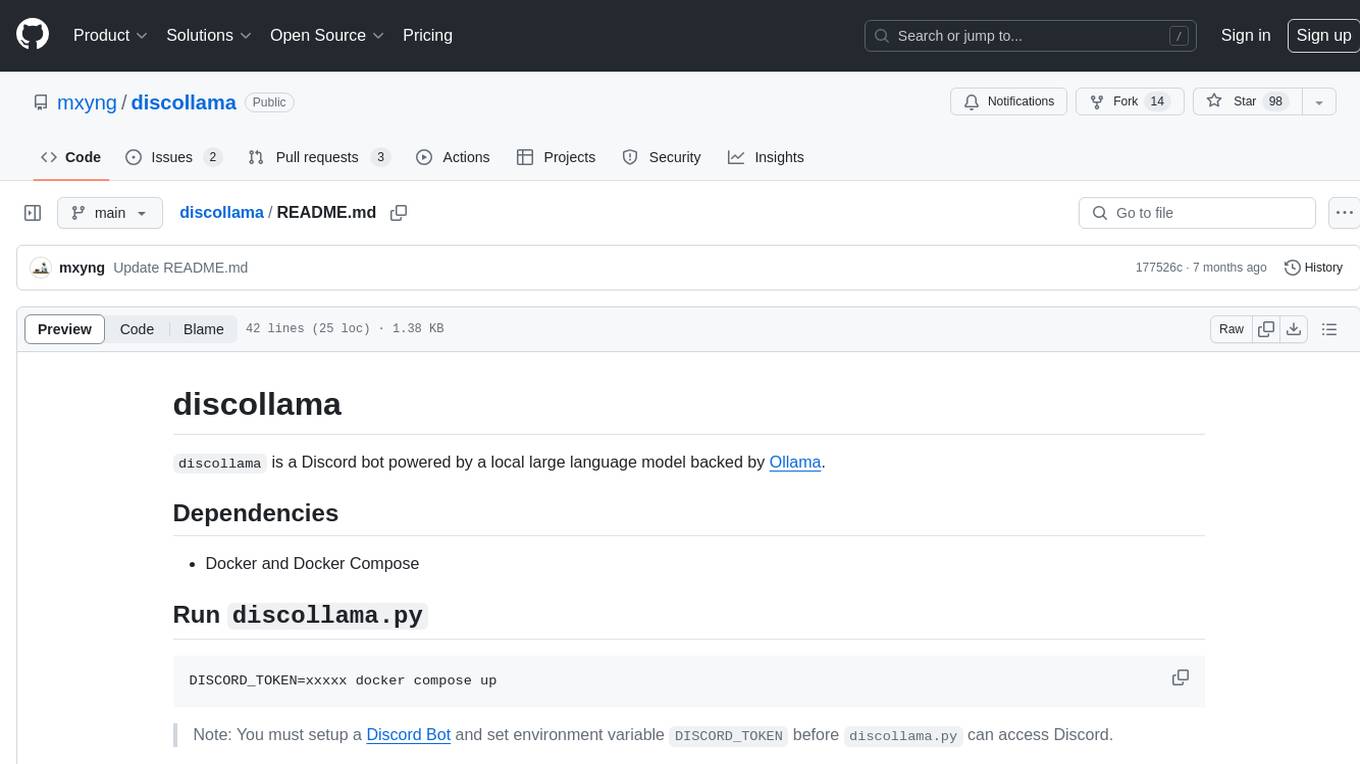
discollama
Discollama is a Discord bot powered by a local large language model backed by Ollama. It allows users to interact with the bot in Discord by mentioning it in a message to start a new conversation or in a reply to a previous response to continue an ongoing conversation. The bot requires Docker and Docker Compose to run, and users need to set up a Discord Bot and environment variable DISCORD_TOKEN before using discollama.py. Additionally, an Ollama server is needed, and users can customize the bot's personality by creating a custom model using Modelfile and running 'ollama create'.
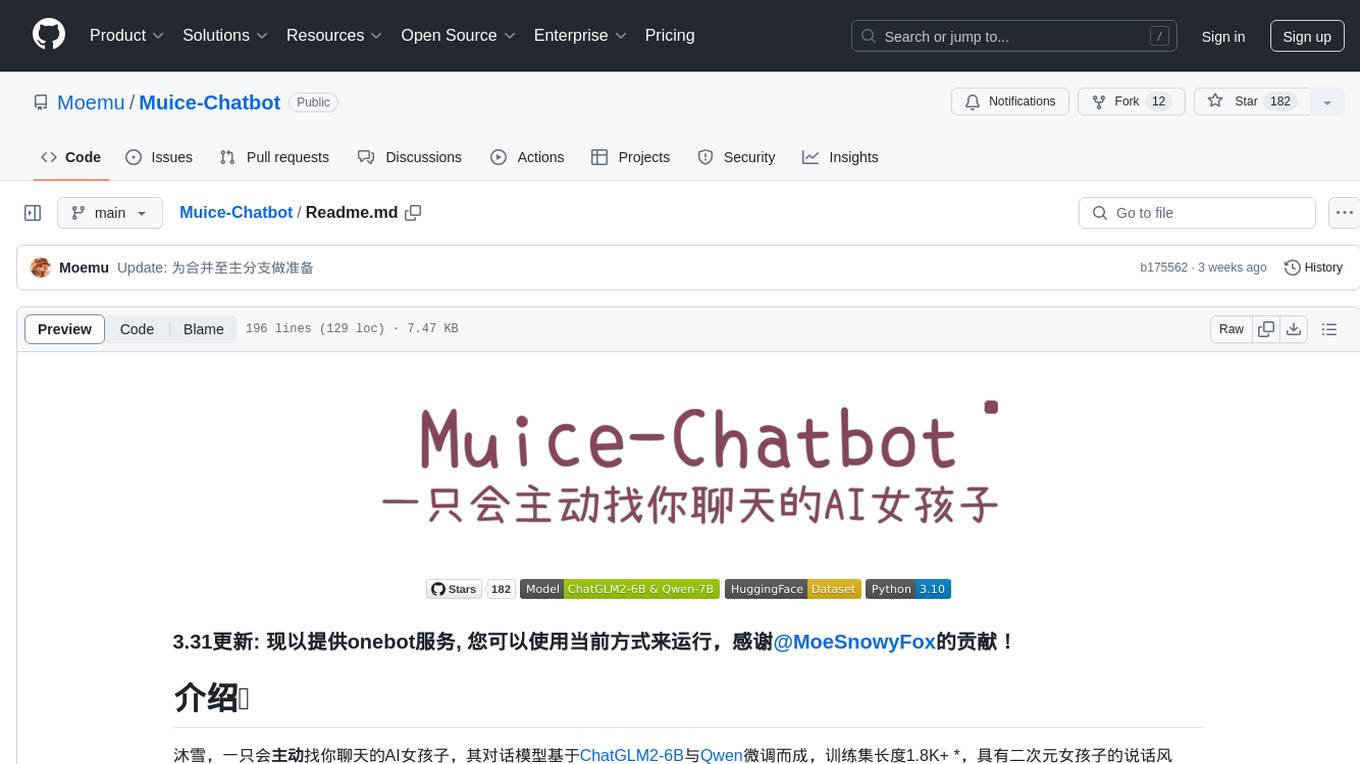
Muice-Chatbot
Muice-Chatbot is an AI chatbot designed to proactively engage in conversations with users. It is based on the ChatGLM2-6B and Qwen-7B models, with a training dataset of 1.8K+ dialogues. The chatbot has a speaking style similar to a 2D girl, being somewhat tsundere but willing to share daily life details and greet users differently every day. It provides various functionalities, including initiating chats and offering 5 available commands. The project supports model loading through different methods and provides onebot service support for QQ users. Users can interact with the chatbot by running the main.py file in the project directory.
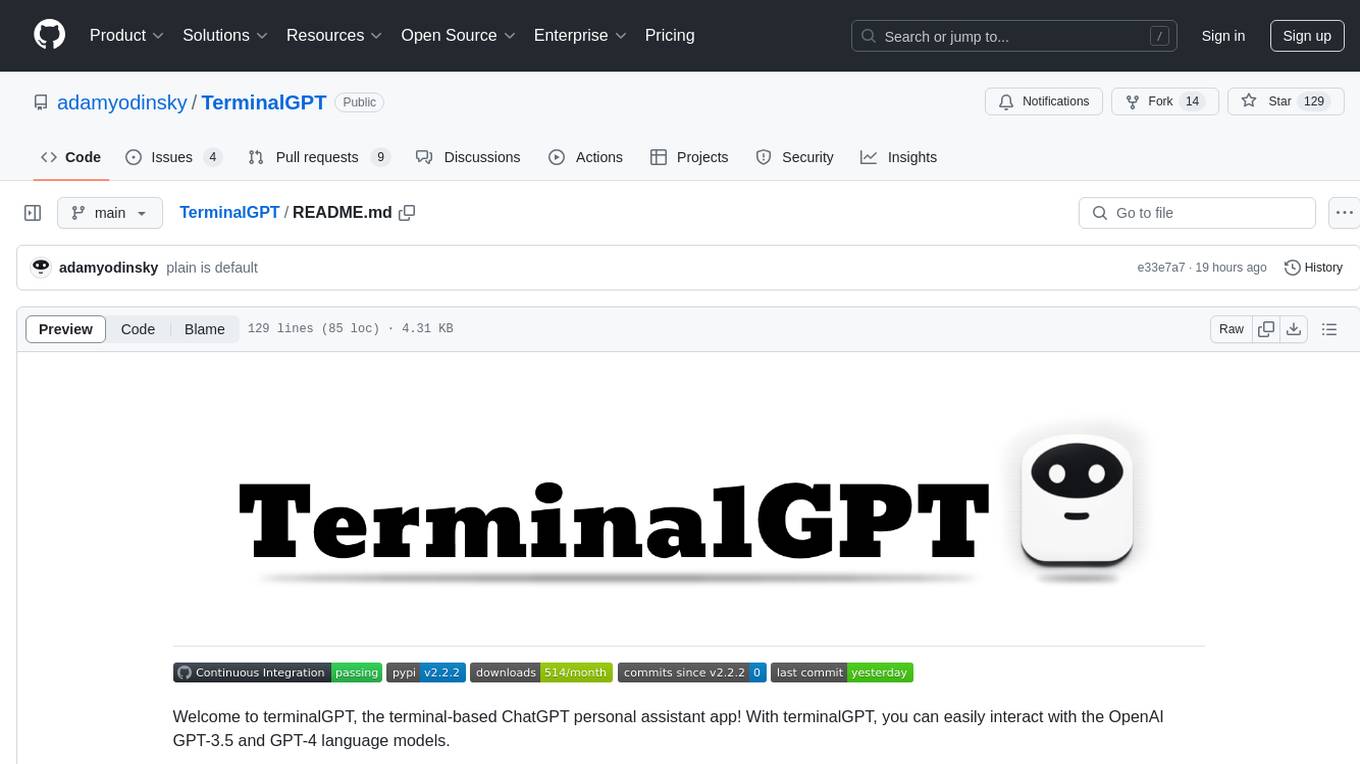
TerminalGPT
TerminalGPT is a terminal-based ChatGPT personal assistant app that allows users to interact with OpenAI GPT-3.5 and GPT-4 language models. It offers advantages over browser-based apps, such as continuous availability, faster replies, and tailored answers. Users can use TerminalGPT in their IDE terminal, ensuring seamless integration with their workflow. The tool prioritizes user privacy by not using conversation data for model training and storing conversations locally on the user's machine.
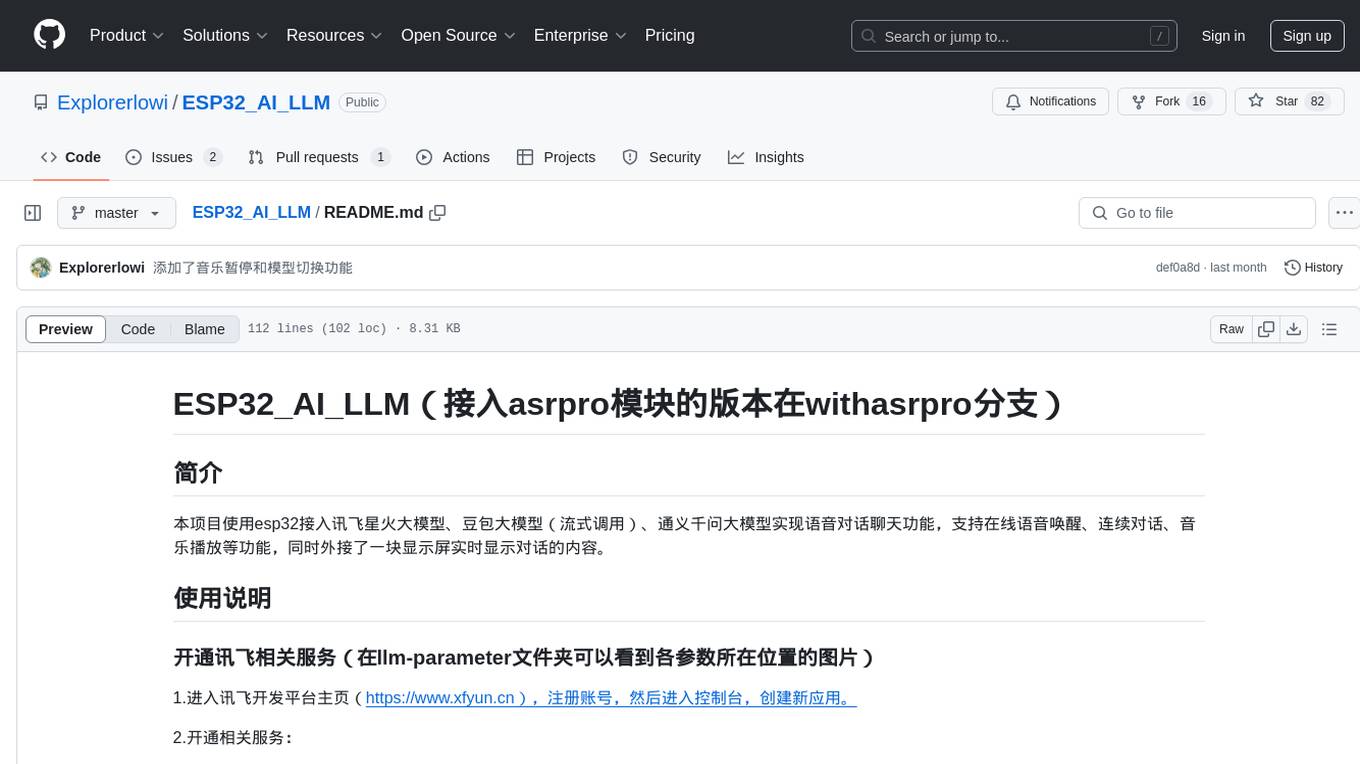
ESP32_AI_LLM
ESP32_AI_LLM is a project that uses ESP32 to connect to Xunfei Xinghuo, Dou Bao, and Tongyi Qianwen large models to achieve voice chat functions, supporting online voice wake-up, continuous conversation, music playback, and real-time display of conversation content on an external screen. The project requires specific hardware components and provides functionalities such as voice wake-up, voice conversation, convenient network configuration, music playback, volume adjustment, LED control, model switching, and screen display. Users can deploy the project by setting up Xunfei services, cloning the repository, configuring necessary parameters, installing drivers, compiling, and burning the code.
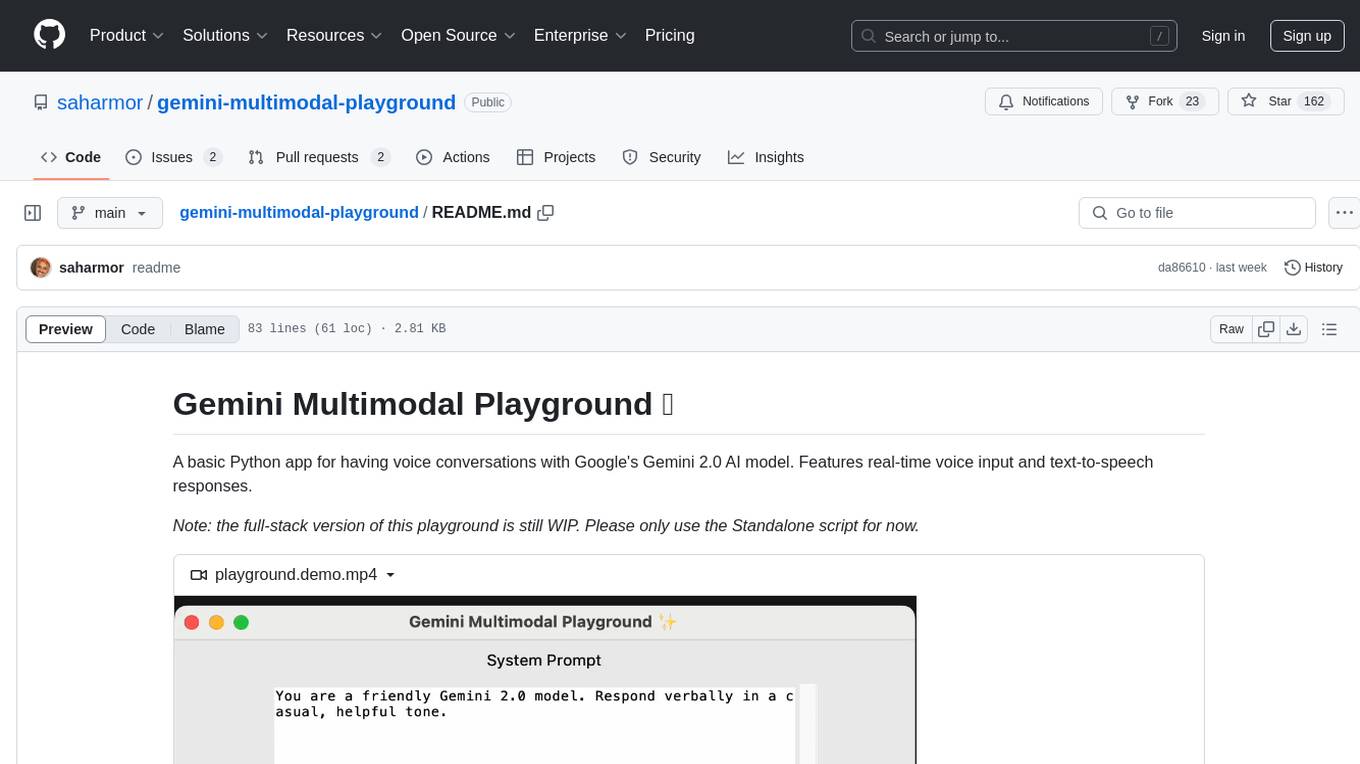
gemini-multimodal-playground
Gemini Multimodal Playground is a basic Python app for voice conversations with Google's Gemini 2.0 AI model. It features real-time voice input and text-to-speech responses. Users can configure settings through the GUI and interact with Gemini by speaking into the microphone. The application provides options for voice selection, system prompt customization, and enabling Google search. Troubleshooting tips are available for handling audio feedback loop issues that may occur during interactions.
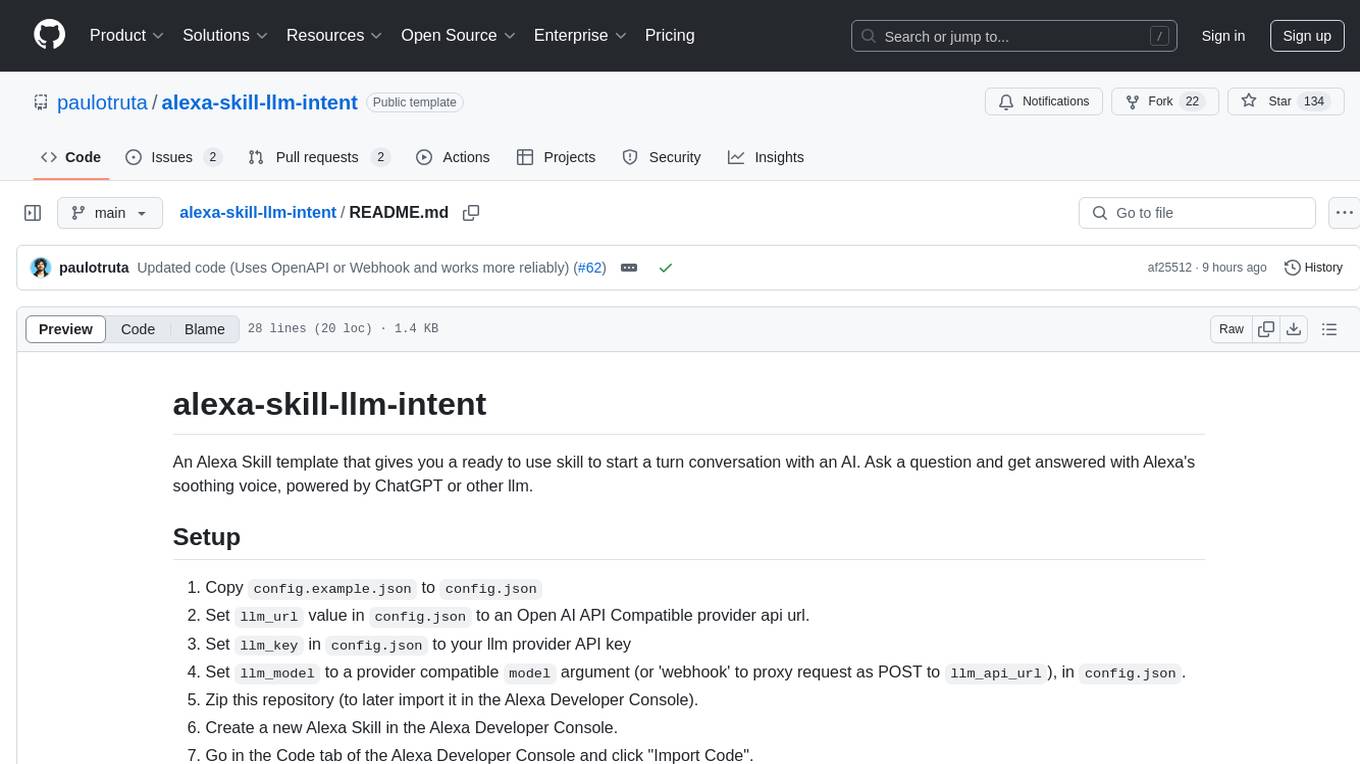
alexa-skill-llm-intent
An Alexa Skill template that provides a ready-to-use skill for starting a conversation with an AI. Users can ask questions and receive answers in Alexa's voice, powered by ChatGPT or other llm. The template includes setup instructions for configuring the AI provider API and model, as well as usage commands for interacting with the skill. It serves as a starting point for creating custom Alexa Skills and should be used at the user's own risk.
For similar jobs
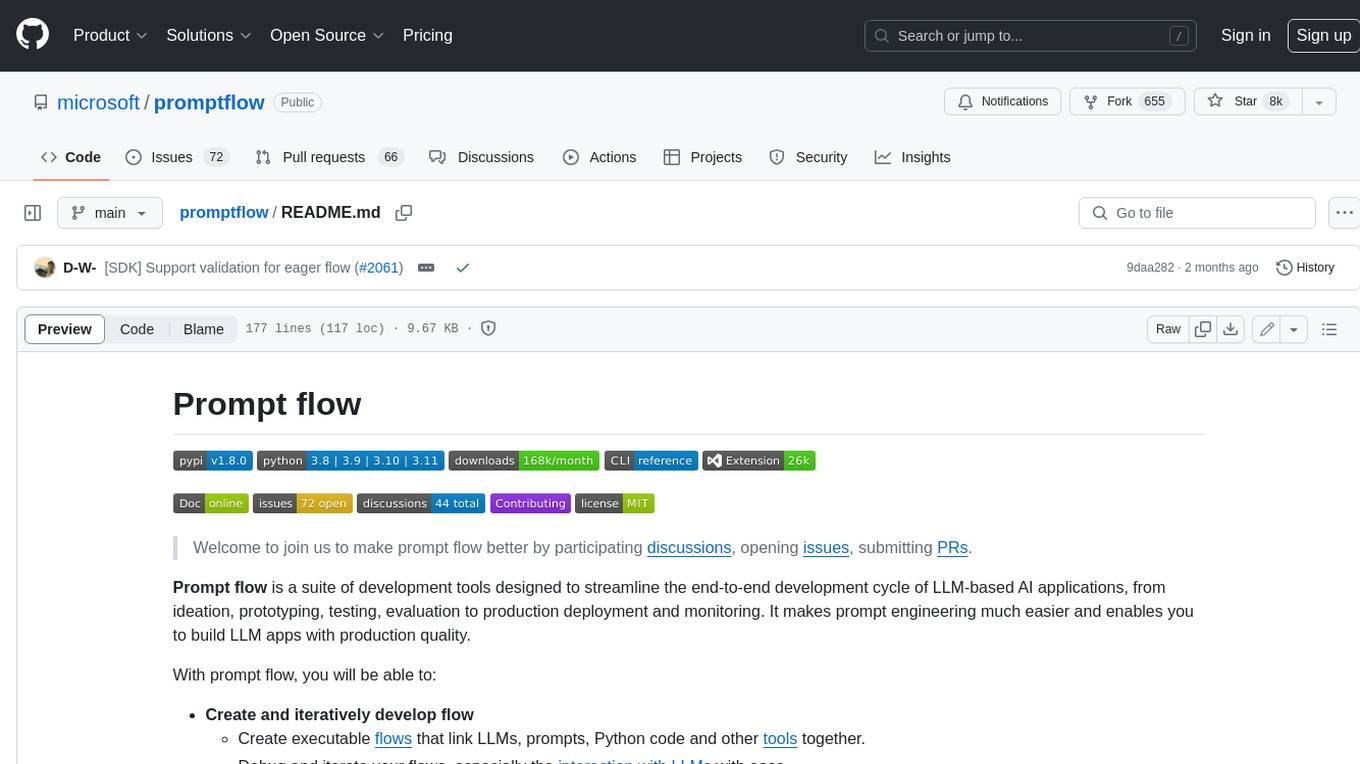
promptflow
**Prompt flow** is a suite of development tools designed to streamline the end-to-end development cycle of LLM-based AI applications, from ideation, prototyping, testing, evaluation to production deployment and monitoring. It makes prompt engineering much easier and enables you to build LLM apps with production quality.
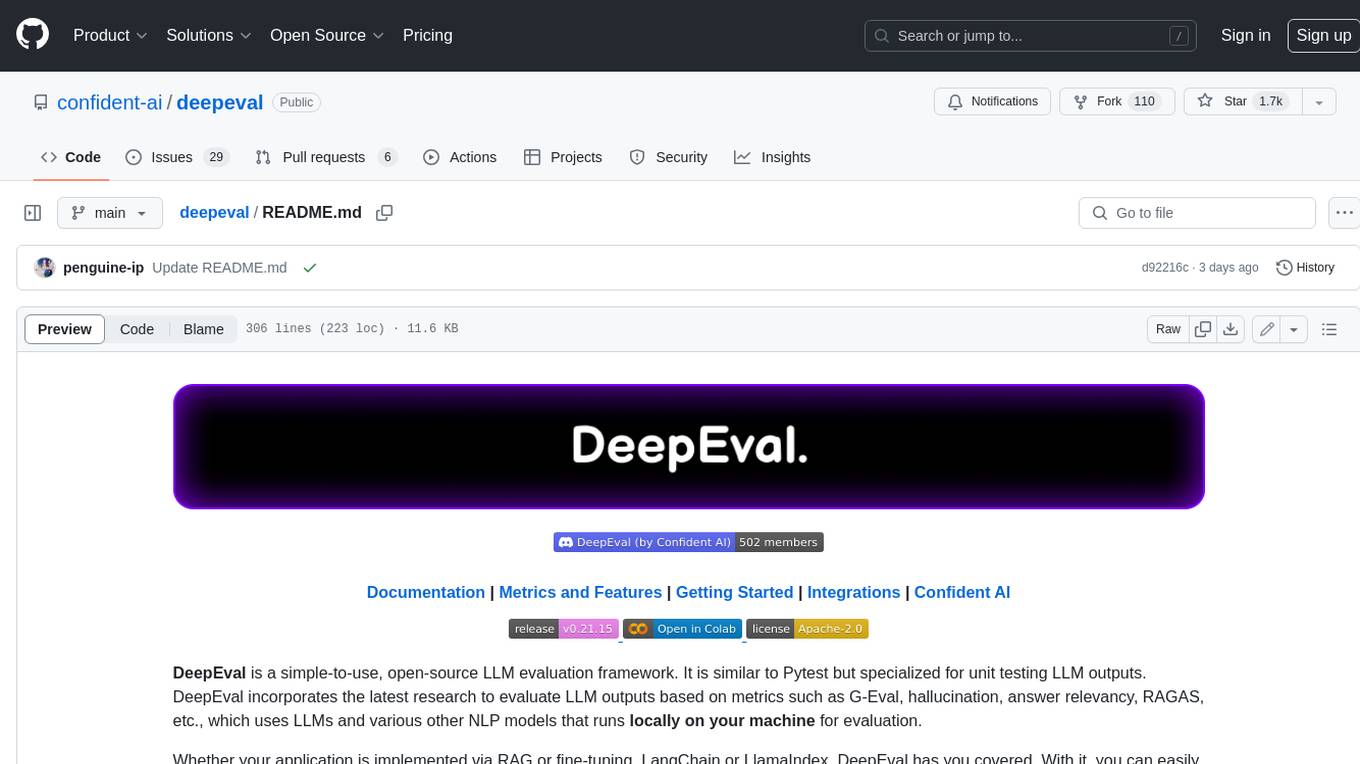
deepeval
DeepEval is a simple-to-use, open-source LLM evaluation framework specialized for unit testing LLM outputs. It incorporates various metrics such as G-Eval, hallucination, answer relevancy, RAGAS, etc., and runs locally on your machine for evaluation. It provides a wide range of ready-to-use evaluation metrics, allows for creating custom metrics, integrates with any CI/CD environment, and enables benchmarking LLMs on popular benchmarks. DeepEval is designed for evaluating RAG and fine-tuning applications, helping users optimize hyperparameters, prevent prompt drifting, and transition from OpenAI to hosting their own Llama2 with confidence.
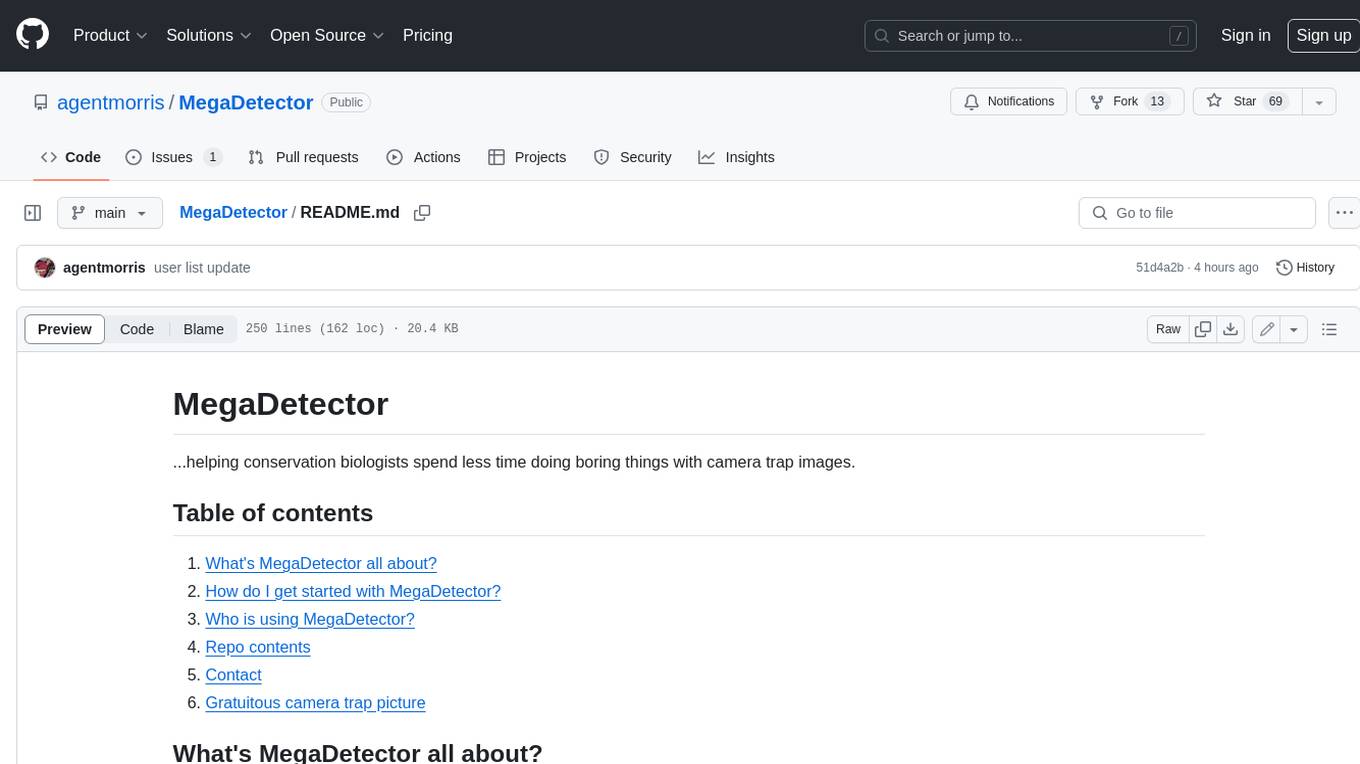
MegaDetector
MegaDetector is an AI model that identifies animals, people, and vehicles in camera trap images (which also makes it useful for eliminating blank images). This model is trained on several million images from a variety of ecosystems. MegaDetector is just one of many tools that aims to make conservation biologists more efficient with AI. If you want to learn about other ways to use AI to accelerate camera trap workflows, check out our of the field, affectionately titled "Everything I know about machine learning and camera traps".
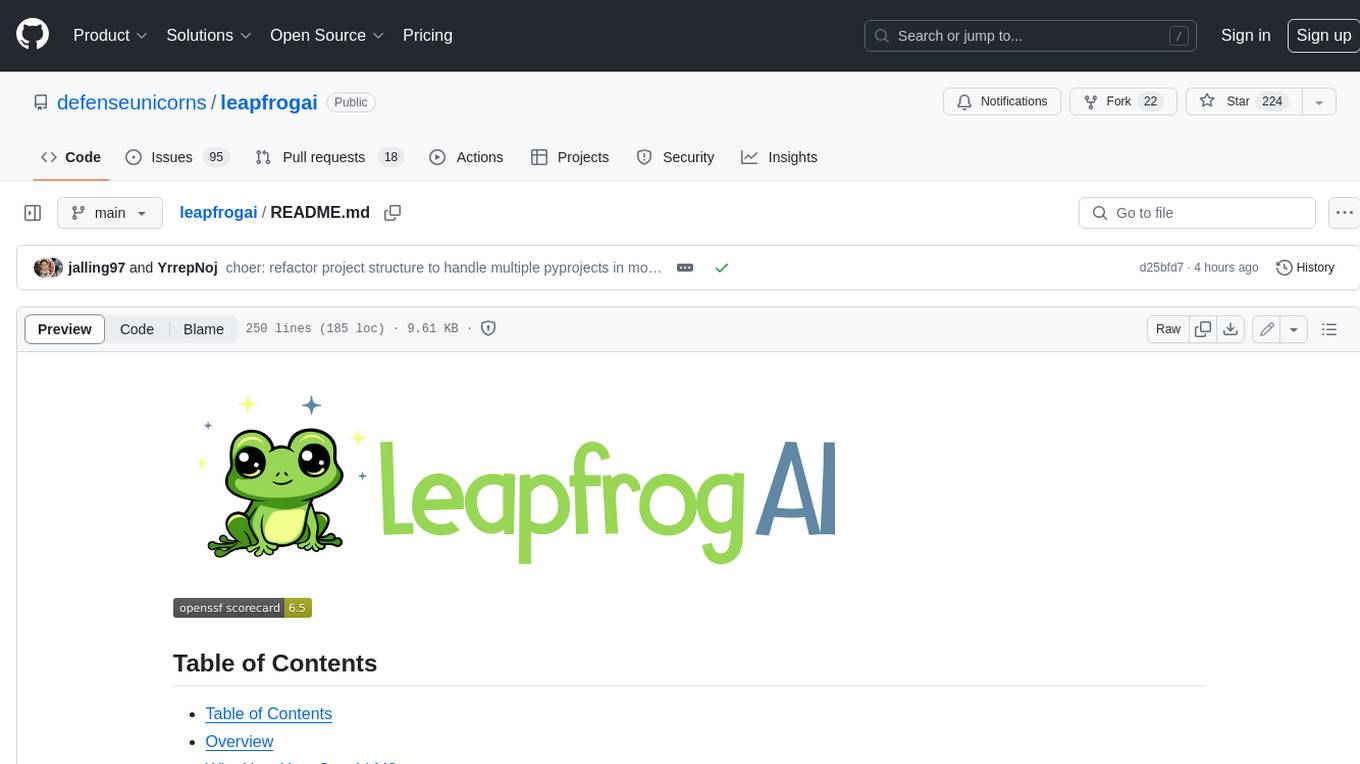
leapfrogai
LeapfrogAI is a self-hosted AI platform designed to be deployed in air-gapped resource-constrained environments. It brings sophisticated AI solutions to these environments by hosting all the necessary components of an AI stack, including vector databases, model backends, API, and UI. LeapfrogAI's API closely matches that of OpenAI, allowing tools built for OpenAI/ChatGPT to function seamlessly with a LeapfrogAI backend. It provides several backends for various use cases, including llama-cpp-python, whisper, text-embeddings, and vllm. LeapfrogAI leverages Chainguard's apko to harden base python images, ensuring the latest supported Python versions are used by the other components of the stack. The LeapfrogAI SDK provides a standard set of protobuffs and python utilities for implementing backends and gRPC. LeapfrogAI offers UI options for common use-cases like chat, summarization, and transcription. It can be deployed and run locally via UDS and Kubernetes, built out using Zarf packages. LeapfrogAI is supported by a community of users and contributors, including Defense Unicorns, Beast Code, Chainguard, Exovera, Hypergiant, Pulze, SOSi, United States Navy, United States Air Force, and United States Space Force.
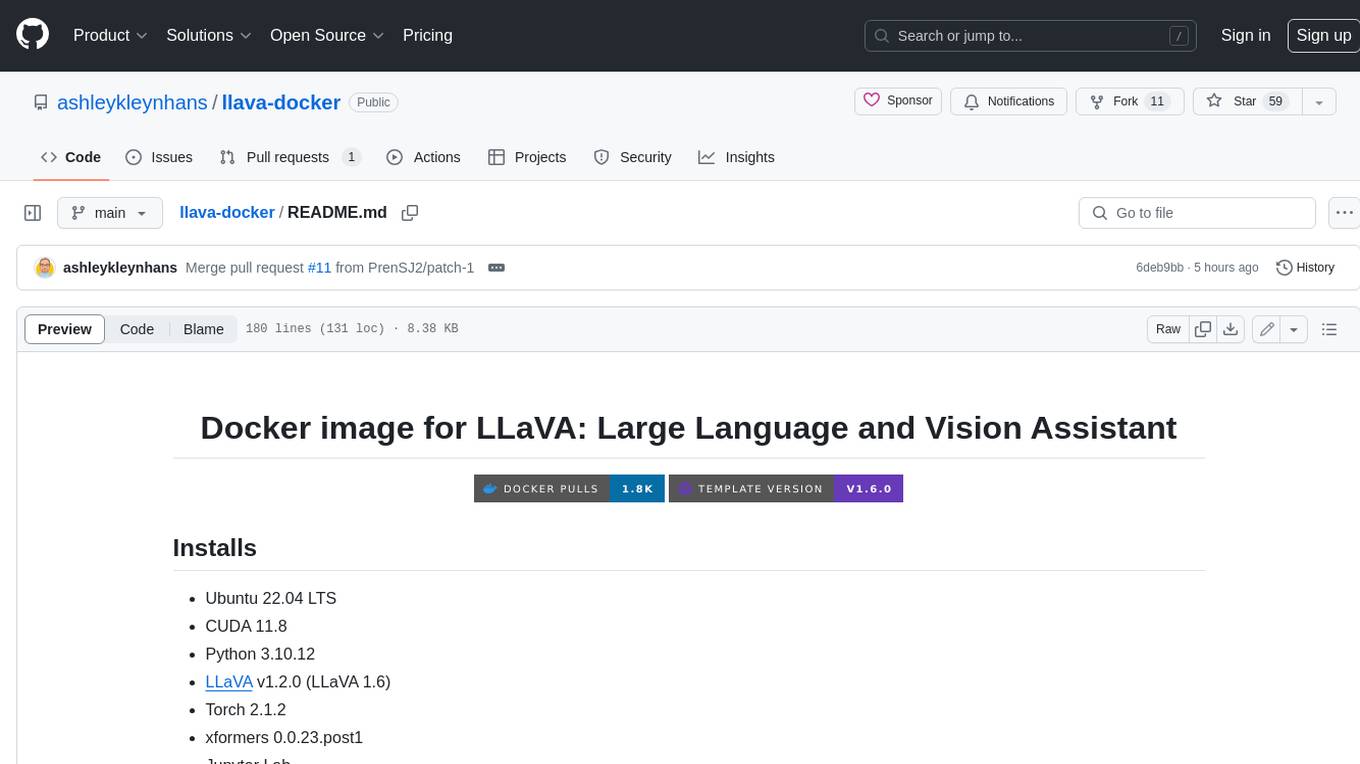
llava-docker
This Docker image for LLaVA (Large Language and Vision Assistant) provides a convenient way to run LLaVA locally or on RunPod. LLaVA is a powerful AI tool that combines natural language processing and computer vision capabilities. With this Docker image, you can easily access LLaVA's functionalities for various tasks, including image captioning, visual question answering, text summarization, and more. The image comes pre-installed with LLaVA v1.2.0, Torch 2.1.2, xformers 0.0.23.post1, and other necessary dependencies. You can customize the model used by setting the MODEL environment variable. The image also includes a Jupyter Lab environment for interactive development and exploration. Overall, this Docker image offers a comprehensive and user-friendly platform for leveraging LLaVA's capabilities.
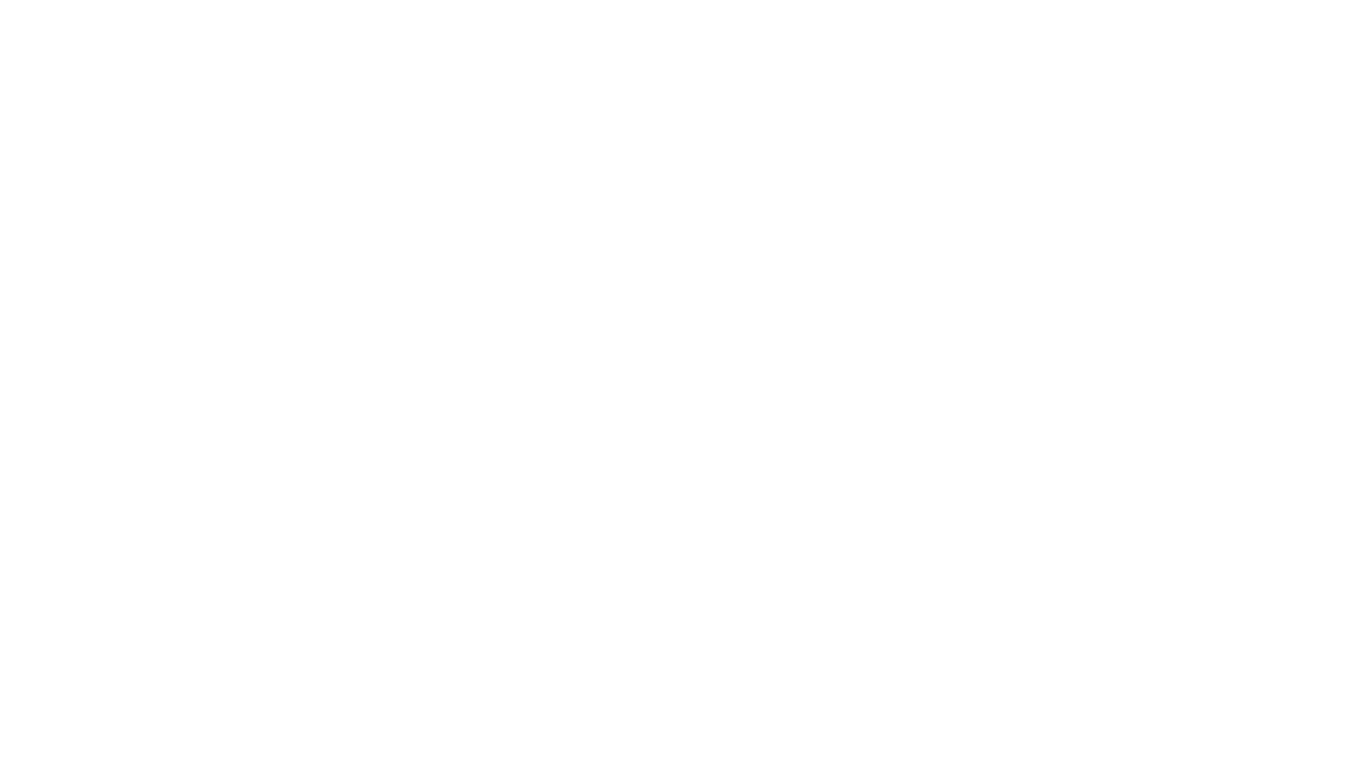
carrot
The 'carrot' repository on GitHub provides a list of free and user-friendly ChatGPT mirror sites for easy access. The repository includes sponsored sites offering various GPT models and services. Users can find and share sites, report errors, and access stable and recommended sites for ChatGPT usage. The repository also includes a detailed list of ChatGPT sites, their features, and accessibility options, making it a valuable resource for ChatGPT users seeking free and unlimited GPT services.
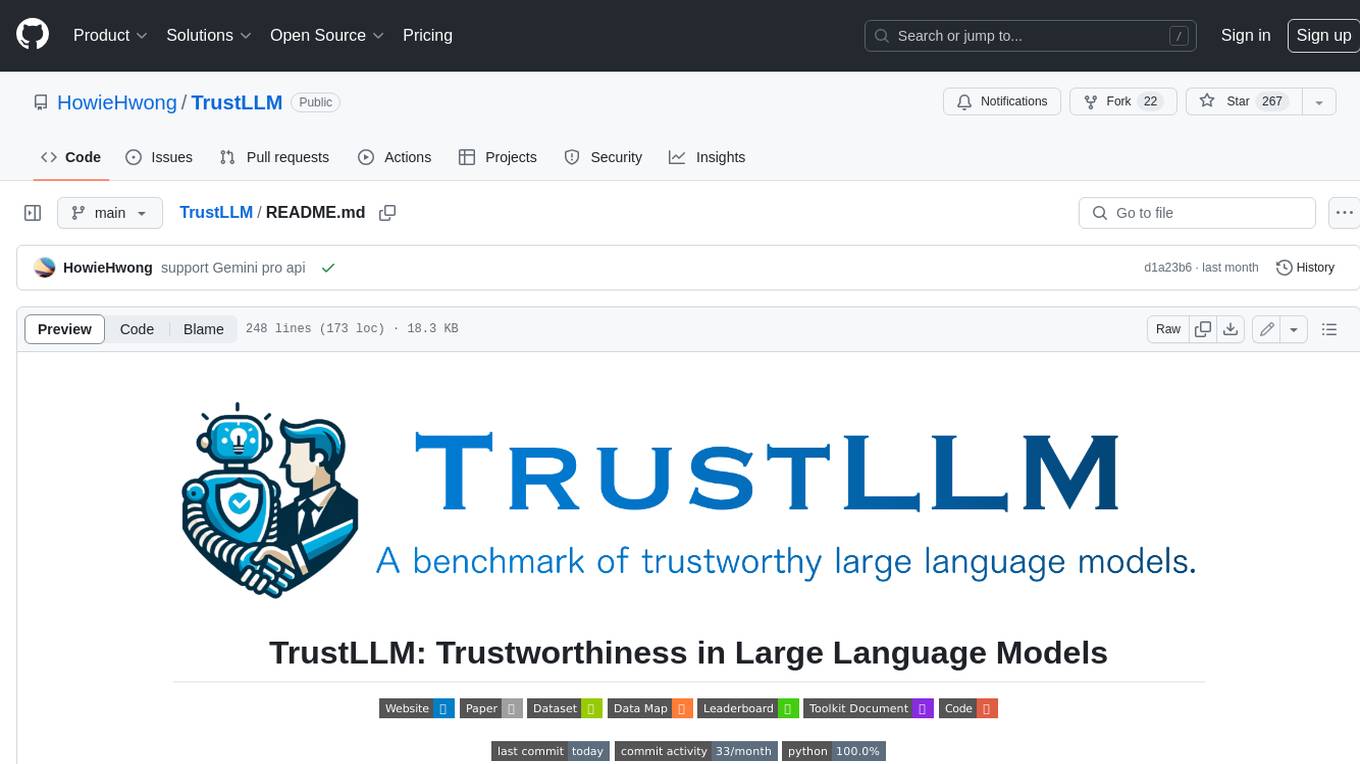
TrustLLM
TrustLLM is a comprehensive study of trustworthiness in LLMs, including principles for different dimensions of trustworthiness, established benchmark, evaluation, and analysis of trustworthiness for mainstream LLMs, and discussion of open challenges and future directions. Specifically, we first propose a set of principles for trustworthy LLMs that span eight different dimensions. Based on these principles, we further establish a benchmark across six dimensions including truthfulness, safety, fairness, robustness, privacy, and machine ethics. We then present a study evaluating 16 mainstream LLMs in TrustLLM, consisting of over 30 datasets. The document explains how to use the trustllm python package to help you assess the performance of your LLM in trustworthiness more quickly. For more details about TrustLLM, please refer to project website.
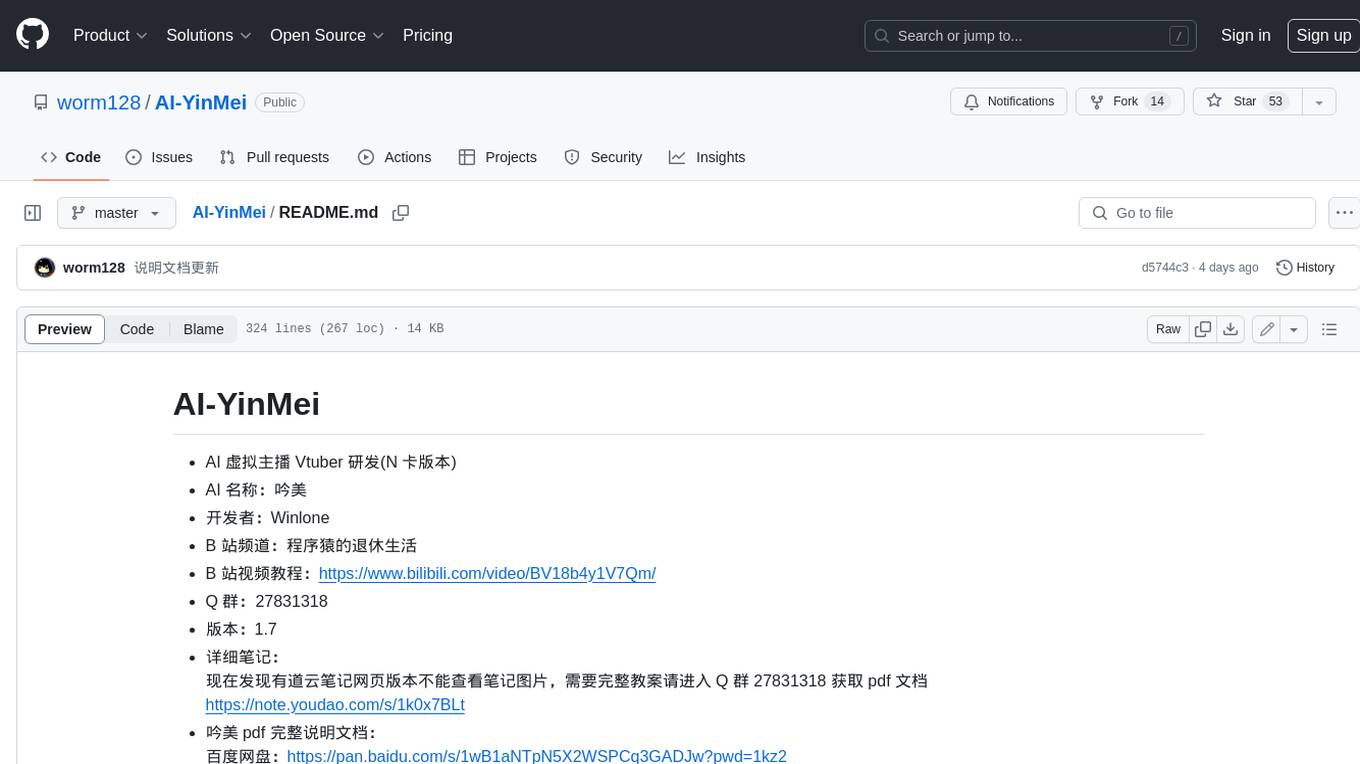
AI-YinMei
AI-YinMei is an AI virtual anchor Vtuber development tool (N card version). It supports fastgpt knowledge base chat dialogue, a complete set of solutions for LLM large language models: [fastgpt] + [one-api] + [Xinference], supports docking bilibili live broadcast barrage reply and entering live broadcast welcome speech, supports Microsoft edge-tts speech synthesis, supports Bert-VITS2 speech synthesis, supports GPT-SoVITS speech synthesis, supports expression control Vtuber Studio, supports painting stable-diffusion-webui output OBS live broadcast room, supports painting picture pornography public-NSFW-y-distinguish, supports search and image search service duckduckgo (requires magic Internet access), supports image search service Baidu image search (no magic Internet access), supports AI reply chat box [html plug-in], supports AI singing Auto-Convert-Music, supports playlist [html plug-in], supports dancing function, supports expression video playback, supports head touching action, supports gift smashing action, supports singing automatic start dancing function, chat and singing automatic cycle swing action, supports multi scene switching, background music switching, day and night automatic switching scene, supports open singing and painting, let AI automatically judge the content.