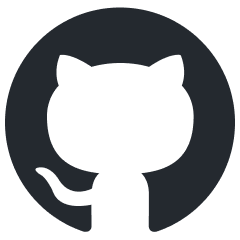
pipecat-flows
Open source conversation framework and visual editor for structured Pipecat dialogues
Stars: 222
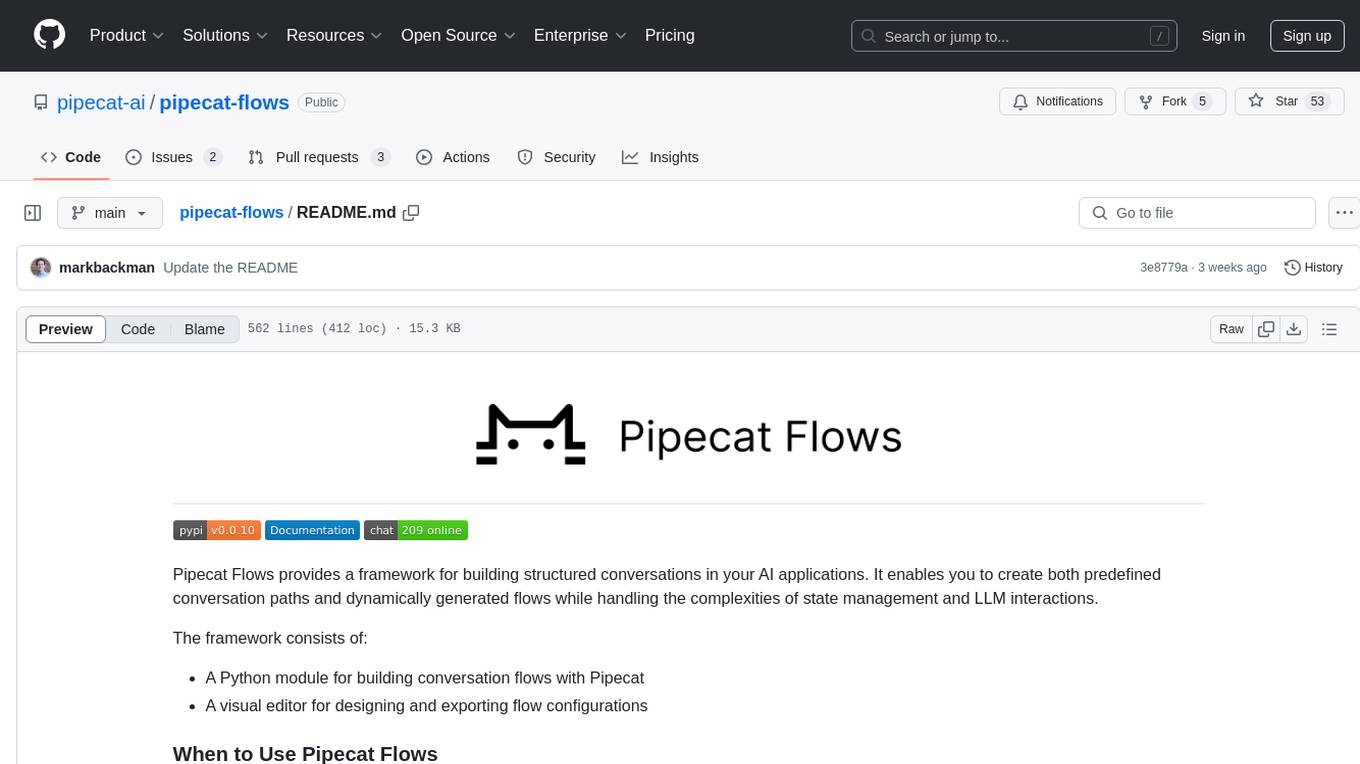
Pipecat Flows is a framework designed for building structured conversations in AI applications. It allows users to create both predefined conversation paths and dynamically generated flows, handling state management and LLM interactions. The framework includes a Python module for building conversation flows and a visual editor for designing and exporting flow configurations. Pipecat Flows is suitable for scenarios such as customer service scripts, intake forms, personalized experiences, and complex decision trees.
README:
Pipecat Flows provides a framework for building structured conversations in your AI applications. It enables you to create both predefined conversation paths and dynamically generated flows while handling the complexities of state management and LLM interactions.
The framework consists of:
- A Python module for building conversation flows with Pipecat
- A visual editor for designing and exporting flow configurations
- Static Flows: When your conversation structure is known upfront and follows predefined paths. Perfect for customer service scripts, intake forms, or guided experiences.
- Dynamic Flows: When conversation paths need to be determined at runtime based on user input, external data, or business logic. Ideal for personalized experiences or complex decision trees.
If you're already using Pipecat:
pip install pipecat-ai-flows
If you're starting fresh:
# Basic installation
pip install pipecat-ai-flows
# Install Pipecat with specific LLM provider options:
pip install "pipecat-ai[daily,openai,deepgram,cartesia]" # For OpenAI
pip install "pipecat-ai[daily,anthropic,deepgram,cartesia]" # For Anthropic
pip install "pipecat-ai[daily,google,deepgram,cartesia]" # For Google
Here's a basic example of setting up a static conversation flow:
from pipecat_flows import FlowManager
# Initialize flow manager with static configuration
flow_manager = FlowManager(
task=task,
llm=llm,
context_aggregator=context_aggregator,
tts=tts,
flow_config=flow_config,
)
@transport.event_handler("on_first_participant_joined")
async def on_first_participant_joined(transport, participant):
await transport.capture_participant_transcription(participant["id"])
await flow_manager.initialize()
For more detailed examples and guides, visit our documentation.
Each conversation flow consists of nodes that define the conversation structure. A node includes:
Nodes use two types of messages to control the conversation:
- Role Messages: Define the bot's personality or role (optional)
"role_messages": [
{
"role": "system",
"content": "You are a friendly pizza ordering assistant. Keep responses casual and upbeat."
}
]
- Task Messages: Define what the bot should do in the current node
"task_messages": [
{
"role": "system",
"content": "Ask the customer which pizza size they'd like: small, medium, or large."
}
]
Role messages are typically defined in your initial node and inherited by subsequent nodes, while task messages are specific to each node's purpose.
Functions come in two types:
- Node Functions: Execute operations within the current state
{
"type": "function",
"function": {
"name": "select_size",
"handler": select_size_handler,
"description": "Select pizza size",
"parameters": {
"type": "object",
"properties": {
"size": {"type": "string", "enum": ["small", "medium", "large"]}
}
},
}
}
- Edge Functions: Create transitions between states
Static flows use transition_to
:
{
"type": "function",
"function": {
"name": "next_step",
"handler": select_size_handler, # Optional handler
"description": "Move to next state",
"parameters": {"type": "object", "properties": {}},
"transition_to": "target_node" # Required: Specify target node
}
}
Dynamic flows use transition_callback
:
{
"type": "function",
"function": {
"name": "collect_age",
"handler": collect_age,
"description": "Record user's age",
"parameters": {...},
"transition_callback": handle_age_collection # Specify transition handler
}
}
Functions behave differently based on their type:
- Node Functions execute their handler and trigger an immediate LLM completion with the result
- Edge Functions execute their handler (if provided) and transition to a new node, with the LLM completion occurring after both the function result and new node's messages are added to context
Functions can:
- Have a handler (for data processing)
- Have a transition_to or transition callback (for state changes)
- Have both (process data and transition)
- Have neither (end node functions)
There are two types of actions available:
-
pre_actions
: Run before the LLM inference. For long function calls, you can use a pre_action for the TTS to say something, like "Hold on a moment..." -
post_actions
: Run after the LLM inference. This is handy for actions like ending or transferring a call.
Actions can be registered in two ways:
- Via handler field in action config:
"pre_actions": [
# Built-in action (no handler needed)
{
"type": "tts_say",
"text": "Processing your order..."
},
# Custom action with handler
{
"type": "check_status",
"handler": check_status_handler
}
]
- Via manual registration:
flow_manager.register_action("check_status", check_status_handler)
Built-in actions (tts_say
, end_conversation
) don't require registration.
Example custom action:
async def check_status_handler(action: dict) -> None:
"""Custom action to check system status."""
logger.info("Checking system status")
# Perform status check
Learn more about built-in actions and defining your own action in the docs.
Pipecat Flows automatically handles format differences between LLM providers:
OpenAI Format
"functions": [{
"type": "function",
"function": {
"name": "function_name",
"description": "description",
"parameters": {...}
}
}]
Anthropic Format
"functions": [{
"name": "function_name",
"description": "description",
"input_schema": {...}
}]
Google (Gemini) Format
"functions": [{
"function_declarations": [{
"name": "function_name",
"description": "description",
"parameters": {...}
}]
}]
The FlowManager handles both static and dynamic flows through a unified interface:
# Define flow configuration upfront
flow_config = {
"initial_node": "greeting",
"nodes": {
"greeting": {
"role_messages": [
{
"role": "system",
"content": "You are a helpful assistant. Your responses will be converted to audio."
}
],
"task_messages": [
{
"role": "system",
"content": "Start by greeting the user and asking for their name."
}
],
"functions": [{
"type": "function",
"function": {
"name": "collect_name",
"description": "Record user's name",
"parameters": {...},
"handler": collect_name_handler, # Specify handler
"transition_to": "next_step" # Specify transition
}
}]
}
}
}
# Create and initialize the FlowManager
flow_manager = FlowManager(
task=task,
llm=llm,
context_aggregator=context_aggregator,
tts=tts,
flow_config=flow_config,
)
await flow_manager.initialize()
Dynamic flows follow the same pattern as static flows, but use transition_callback
instead of transition_to
to specify runtime-determined transitions. Here's an example:
# Define handlers
async def update_coverage(args: FlowArgs) -> FlowResult:
"""Update coverage options; node function without a transition."""
return {"coverage": args["coverage"]}
# Edge function transition handler
async def handle_age_collection(args: Dict, result: FlowResult, flow_manager: FlowManager):
"""Handle age collection transition; edge function which transitions to the next node."""
# Use typed result directly
flow_manager.state["age"] = result.age
await flow_manager.set_node("next", create_next_node())
# Create nodes
def create_initial_node() -> NodeConfig:
return {
"role_messages": [
{
"role": "system",
"content": "You are a helpful assistant."
}
],
"task_messages": [
{
"role": "system",
"content": "Ask the user for their age."
}
],
"functions": [
{
"type": "function",
"function": {
"name": "collect_age",
"handler": collect_age,
"description": "Record user's age",
"parameters": {
"type": "object",
"properties": {
"age": {"type": "integer"}
},
"required": ["age"]
},
"transition_callback": handle_age_collection # Specify transition handler
}
}
]
}
# Initialize flow manager
flow_manager = FlowManager(
task=task,
llm=llm,
context_aggregator=context_aggregator,
tts=tts,
)
await flow_manager.initialize()
@transport.event_handler("on_first_participant_joined")
async def on_first_participant_joined(transport, participant):
await transport.capture_participant_transcription(participant["id"])
await flow_manager.initialize()
await flow_manager.set_node("initial", create_initial_node())
The FlowManager
provides three strategies for managing conversation context during node transitions:
- APPEND (default): Adds new messages to the existing context, maintaining the full conversation history
- RESET: Clears the context and starts fresh with the new node's messages, including the previous function call results
- RESET_WITH_SUMMARY: Resets the context but includes an AI-generated summary of the previous conversation and the new node's messages
Strategies can be set globally or per-node:
# Global strategy
flow_manager = FlowManager(
task=task,
llm=llm,
context_aggregator=context_aggregator,
context_strategy=ContextStrategyConfig(
strategy=ContextStrategy.RESET
)
)
# Per-node strategy
node_config = {
"task_messages": [...],
"functions": [...],
"context_strategy": ContextStrategyConfig(
strategy=ContextStrategy.RESET_WITH_SUMMARY,
summary_prompt="Summarize the key points discussed so far."
)
}
When using RESET_WITH_SUMMARY
, the system automatically falls back to RESET
if summary generation fails or times out.
The repository includes several complete example implementations in the examples/
directory.
In the examples/static
directory, you'll find these examples:
-
food_ordering.py
- A restaurant order flow demonstrating node and edge functions -
movie_explorer_openai.py
- Movie information bot demonstrating real API integration with TMDB -
movie_explorer_anthropic.py
- The same movie information demo adapted for Anthropic's format -
movie_explorer_gemini.py
- The same movie explorer demo adapted for Google Gemini's format -
patient_intake_openai.py
- A medical intake system showing complex state management -
patient_intake_anthropic.py
- The same medical intake demo adapted for Anthropic's format -
patient_intake_gemini.py
- The same medical intake demo adapted for Gemini's format -
travel_planner.py
- A vacation planning assistant with parallel paths
In the examples/dynamic
directory, you'll find these examples:
-
insurance_openai.py
- An insurance quote system using OpenAI's format -
insurance_anthropic.py
- The same insurance system adapted for Anthropic's format -
insurance_gemini.py
- The insurance system implemented with Google's format -
restaurant_reservation.py
- A reservation system with availability checking
Each LLM provider (OpenAI, Anthropic, Google) has slightly different function calling formats, but Pipecat Flows handles these differences internally while maintaining a consistent API for developers.
To run these examples:
-
Setup Virtual Environment (recommended):
python3 -m venv venv source venv/bin/activate
-
Installation:
Install the package in development mode:
pip install -e .
Install Pipecat with required options for examples:
pip install "pipecat-ai[daily,openai,deepgram,cartesia,silero,examples]"
If you're running Google or Anthropic examples, you will need to update the installed options. For example:
# Install Google Gemini pip install "pipecat-ai[daily,google,deepgram,cartesia,silero,examples]" # Install Anthropic pip install "pipecat-ai[daily,anthropic,deepgram,cartesia,silero,examples]"
-
Configuration:
Copy
env.example
to.env
in the examples directory:cp env.example .env
Add your API keys and configuration:
- DEEPGRAM_API_KEY
- CARTESIA_API_KEY
- OPENAI_API_KEY
- ANTHROPIC_API_KEY
- GOOGLE_API_KEY
- DAILY_API_KEY
Looking for a Daily API key and room URL? Sign up on the Daily Dashboard.
-
Running:
python examples/static/food_ordering.py -u YOUR_DAILY_ROOM_URL
The package includes a comprehensive test suite covering the core functionality.
-
Create Virtual Environment:
python3 -m venv venv source venv/bin/activate # On Windows: venv\Scripts\activate
-
Install Test Dependencies:
pip install -r dev-requirements.txt -r test-requirements.txt pip install "pipecat-ai[google,openai,anthropic]" pip install -e .
Run all tests:
pytest tests/
Run specific test file:
pytest tests/test_state.py
Run specific test:
pytest tests/test_state.py -k test_initialization
Run with coverage report:
pytest tests/ --cov=pipecat_flows
A visual editor for creating and managing Pipecat conversation flows.
- Visual flow creation and editing
- Import/export of flow configurations
- Support for node and edge functions
- Merge node support for complex flows
- Real-time validation
While the underlying system is flexible with node naming, the editor follows these conventions for clarity:
- Start Node: Named after your initial conversation state (e.g., "greeting", "welcome")
- End Node: Conventionally named "end" for clarity, though other names are supported
- Flow Nodes: Named to reflect their purpose in the conversation (e.g., "get_time", "confirm_order")
These conventions help maintain readable and maintainable flows while preserving technical flexibility.
The editor is available online at flows.pipecat.ai.
- Node.js (v14 or higher)
- npm (v6 or higher)
Clone the repository
git clone [email protected]:pipecat-ai/pipecat-flows.git
Navigate to project directory
cd pipecat-flows/editor
Install dependencies
npm install
Start development server
npm run dev
Open the page in your browser: http://localhost:5173.
- Create a new flow using the toolbar buttons
- Add nodes by right-clicking in the canvas
- Start nodes can have descriptive names (e.g., "greeting")
- End nodes are conventionally named "end"
- Connect nodes by dragging from outputs to inputs
- Edit node properties in the side panel
- Export your flow configuration using the toolbar
The editor/examples/
directory contains sample flow configurations:
food_ordering.json
movie_explorer.py
patient_intake.json
restaurant_reservation.json
travel_planner.json
To use an example:
- Open the editor
- Click "Import Flow"
- Select an example JSON file
See the examples directory for the complete files and documentation.
-
npm start
- Start production server -
npm run dev
- Start development server -
npm run build
- Build for production -
npm run preview
- Preview production build locally -
npm run preview:prod
- Preview production build with base path -
npm run lint
- Check for linting issues -
npm run lint:fix
- Fix linting issues -
npm run format
- Format code with Prettier -
npm run format:check
- Check code formatting -
npm run docs
- Generate documentation -
npm run docs:serve
- Serve documentation locally
The Pipecat Flows Editor project uses JSDoc for documentation. To generate and view the documentation:
Generate documentation:
npm run docs
Serve documentation locally:
npm run docs:serve
View in browser by opening: http://localhost:8080
We welcome contributions from the community! Whether you're fixing bugs, improving documentation, or adding new features, here's how you can help:
- Found a bug? Open an issue
- Have a feature idea? Start a discussion
- Want to contribute code? Check our CONTRIBUTING.md guide
- Documentation improvements? Docs PRs are always welcome
Before submitting a pull request, please check existing issues and PRs to avoid duplicates.
We aim to review all contributions promptly and provide constructive feedback to help get your changes merged.
For Tasks:
Click tags to check more tools for each tasksFor Jobs:
Alternative AI tools for pipecat-flows
Similar Open Source Tools
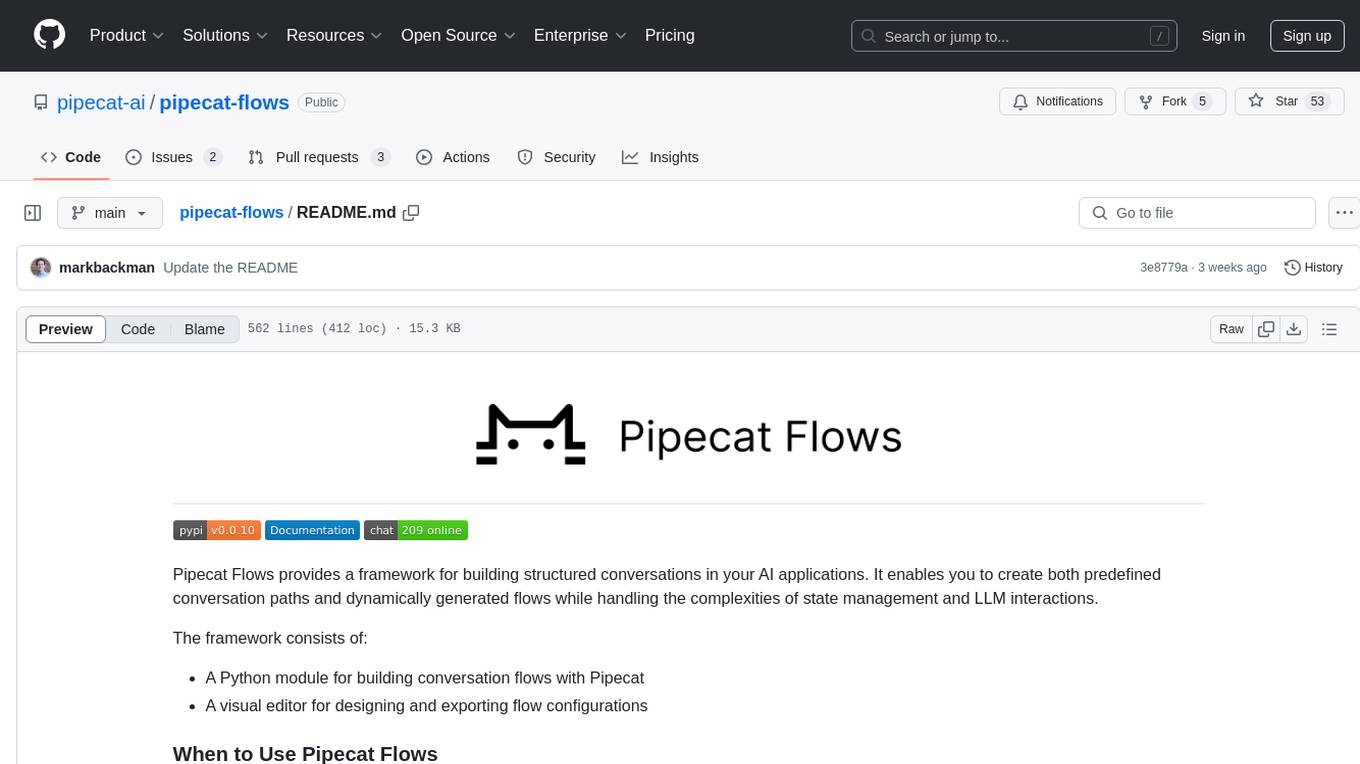
pipecat-flows
Pipecat Flows is a framework designed for building structured conversations in AI applications. It allows users to create both predefined conversation paths and dynamically generated flows, handling state management and LLM interactions. The framework includes a Python module for building conversation flows and a visual editor for designing and exporting flow configurations. Pipecat Flows is suitable for scenarios such as customer service scripts, intake forms, personalized experiences, and complex decision trees.
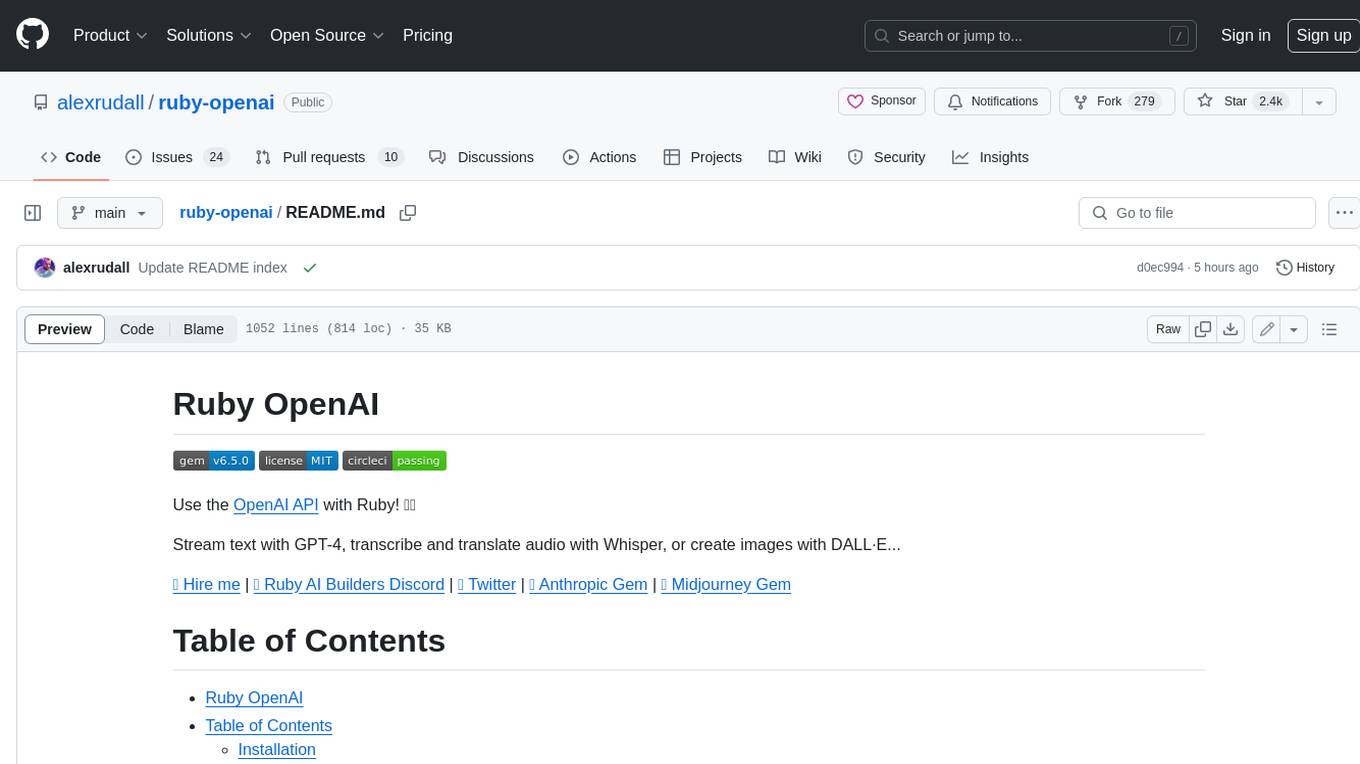
ruby-openai
Use the OpenAI API with Ruby! 🤖🩵 Stream text with GPT-4, transcribe and translate audio with Whisper, or create images with DALL·E... Hire me | 🎮 Ruby AI Builders Discord | 🐦 Twitter | 🧠 Anthropic Gem | 🚂 Midjourney Gem ## Table of Contents * Ruby OpenAI * Table of Contents * Installation * Bundler * Gem install * Usage * Quickstart * With Config * Custom timeout or base URI * Extra Headers per Client * Logging * Errors * Faraday middleware * Azure * Ollama * Counting Tokens * Models * Examples * Chat * Streaming Chat * Vision * JSON Mode * Functions * Edits * Embeddings * Batches * Files * Finetunes * Assistants * Threads and Messages * Runs * Runs involving function tools * Image Generation * DALL·E 2 * DALL·E 3 * Image Edit * Image Variations * Moderations * Whisper * Translate * Transcribe * Speech * Errors * Development * Release * Contributing * License * Code of Conduct
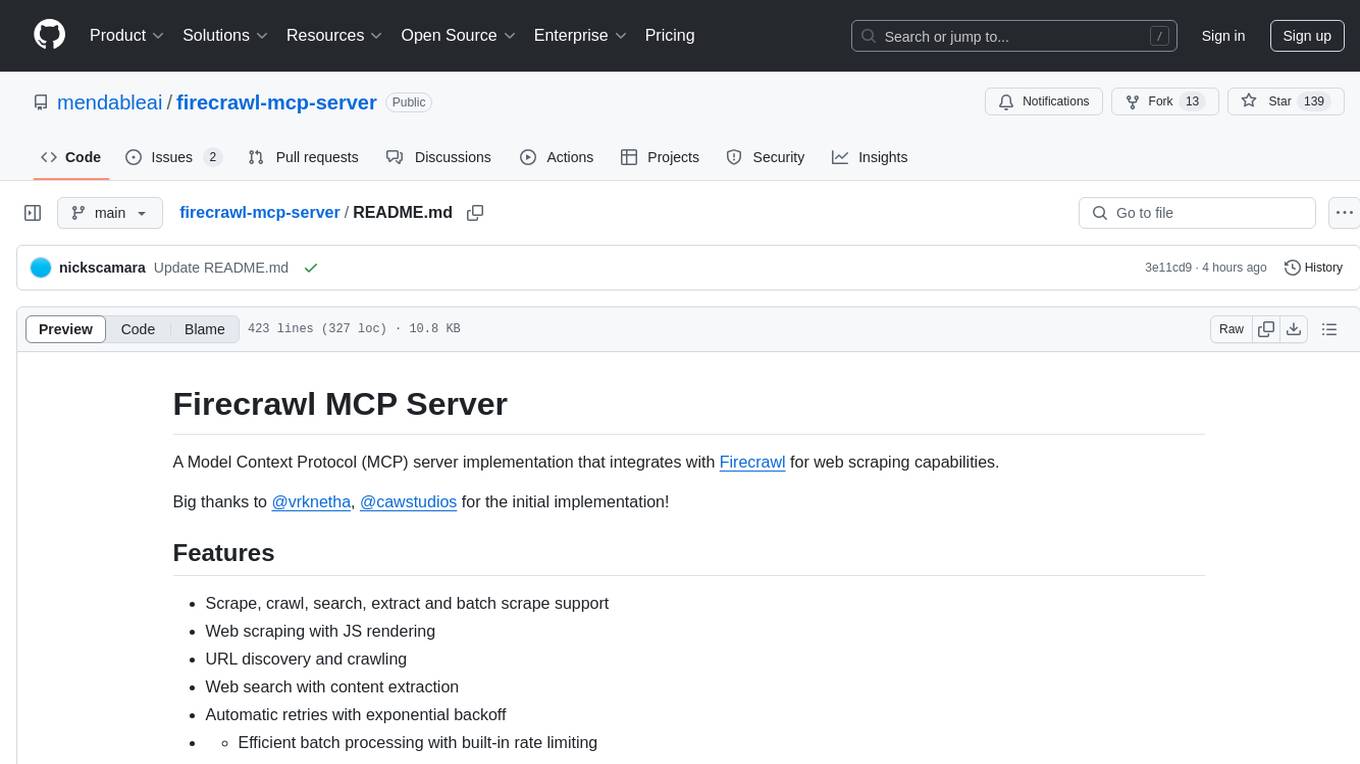
firecrawl-mcp-server
Firecrawl MCP Server is a Model Context Protocol (MCP) server implementation that integrates with Firecrawl for web scraping capabilities. It supports features like scrape, crawl, search, extract, and batch scrape. It provides web scraping with JS rendering, URL discovery, web search with content extraction, automatic retries with exponential backoff, credit usage monitoring, comprehensive logging system, support for cloud and self-hosted FireCrawl instances, mobile/desktop viewport support, and smart content filtering with tag inclusion/exclusion. The server includes configurable parameters for retry behavior and credit usage monitoring, rate limiting and batch processing capabilities, and tools for scraping, batch scraping, checking batch status, searching, crawling, and extracting structured information from web pages.
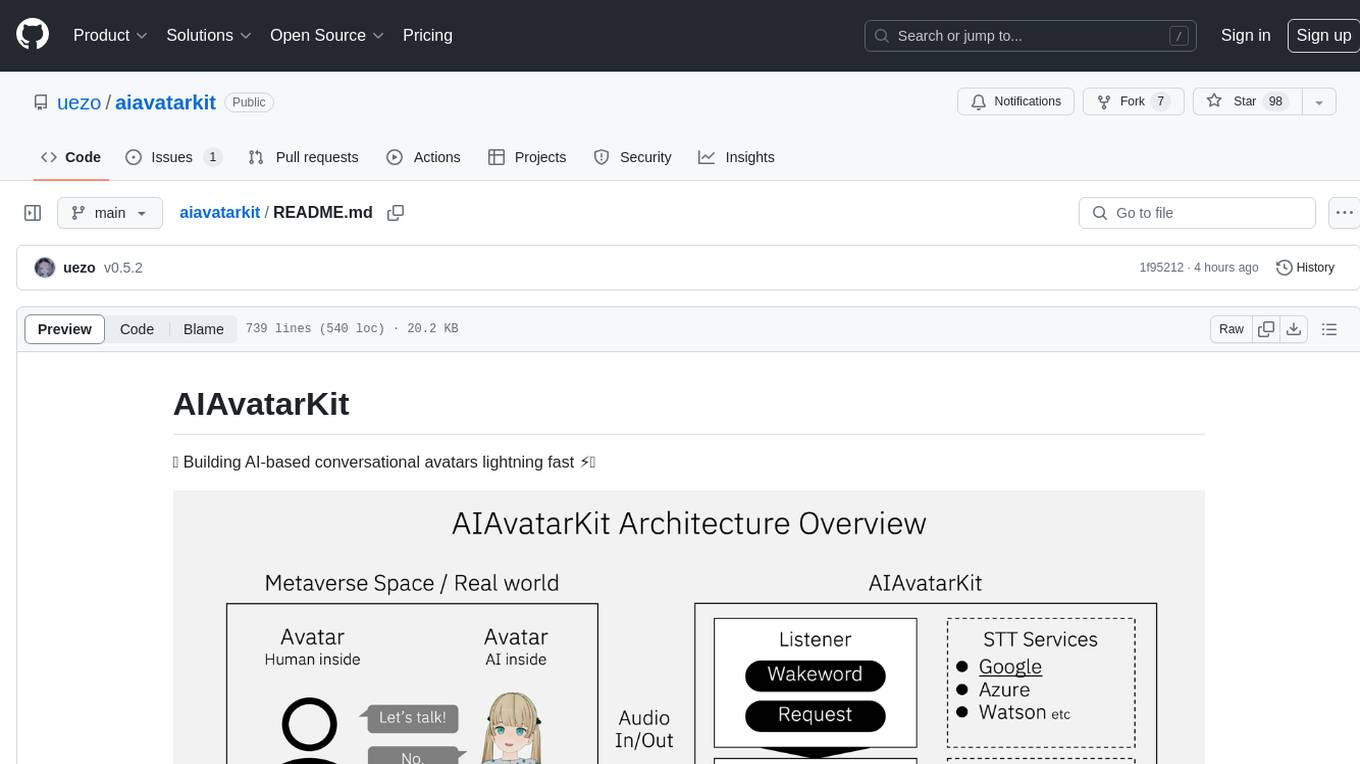
aiavatarkit
AIAvatarKit is a tool for building AI-based conversational avatars quickly. It supports various platforms like VRChat and cluster, along with real-world devices. The tool is extensible, allowing unlimited capabilities based on user needs. It requires VOICEVOX API, Google or Azure Speech Services API keys, and Python 3.10. Users can start conversations out of the box and enjoy seamless interactions with the avatars.
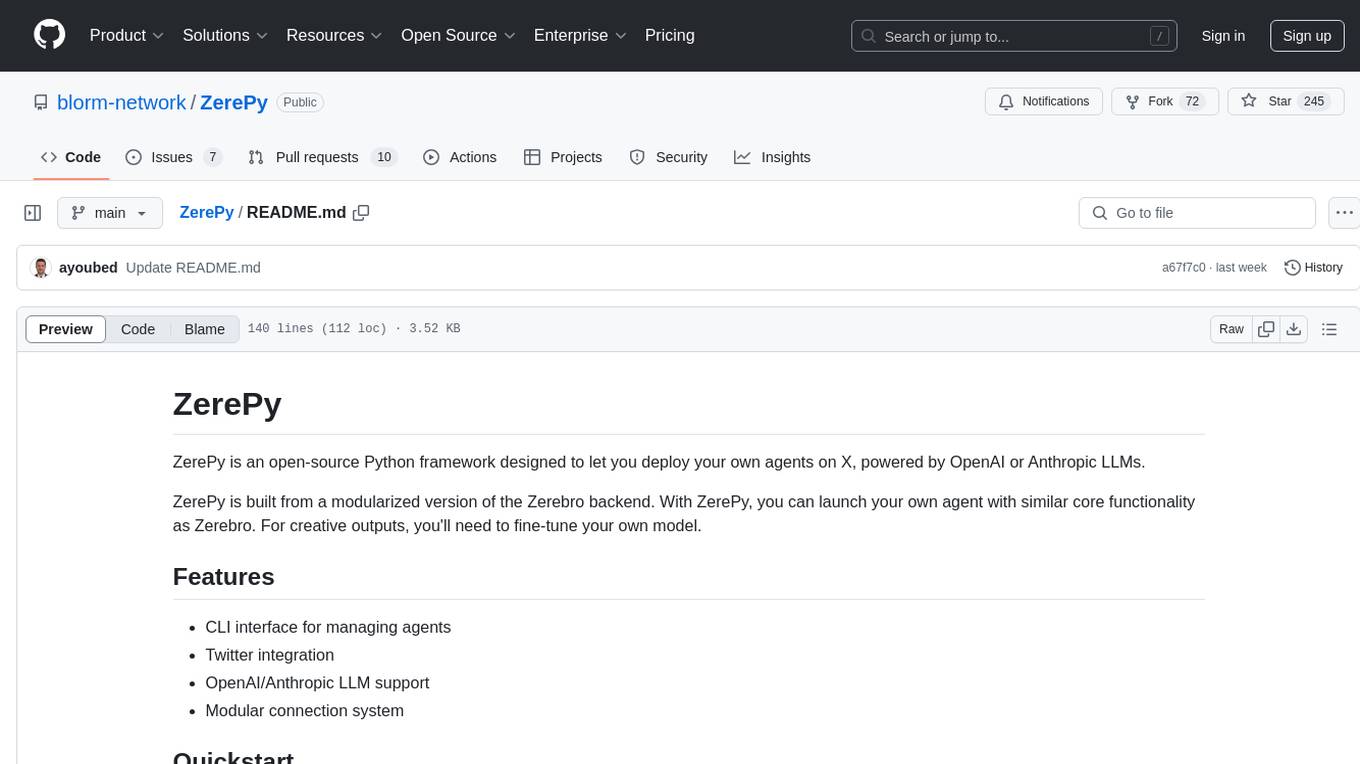
ZerePy
ZerePy is an open-source Python framework for deploying agents on X using OpenAI or Anthropic LLMs. It offers CLI interface, Twitter integration, and modular connection system. Users can fine-tune models for creative outputs and create agents with specific tasks. The tool requires Python 3.10+, Poetry 1.5+, and API keys for LLM, OpenAI, Anthropic, and X API.
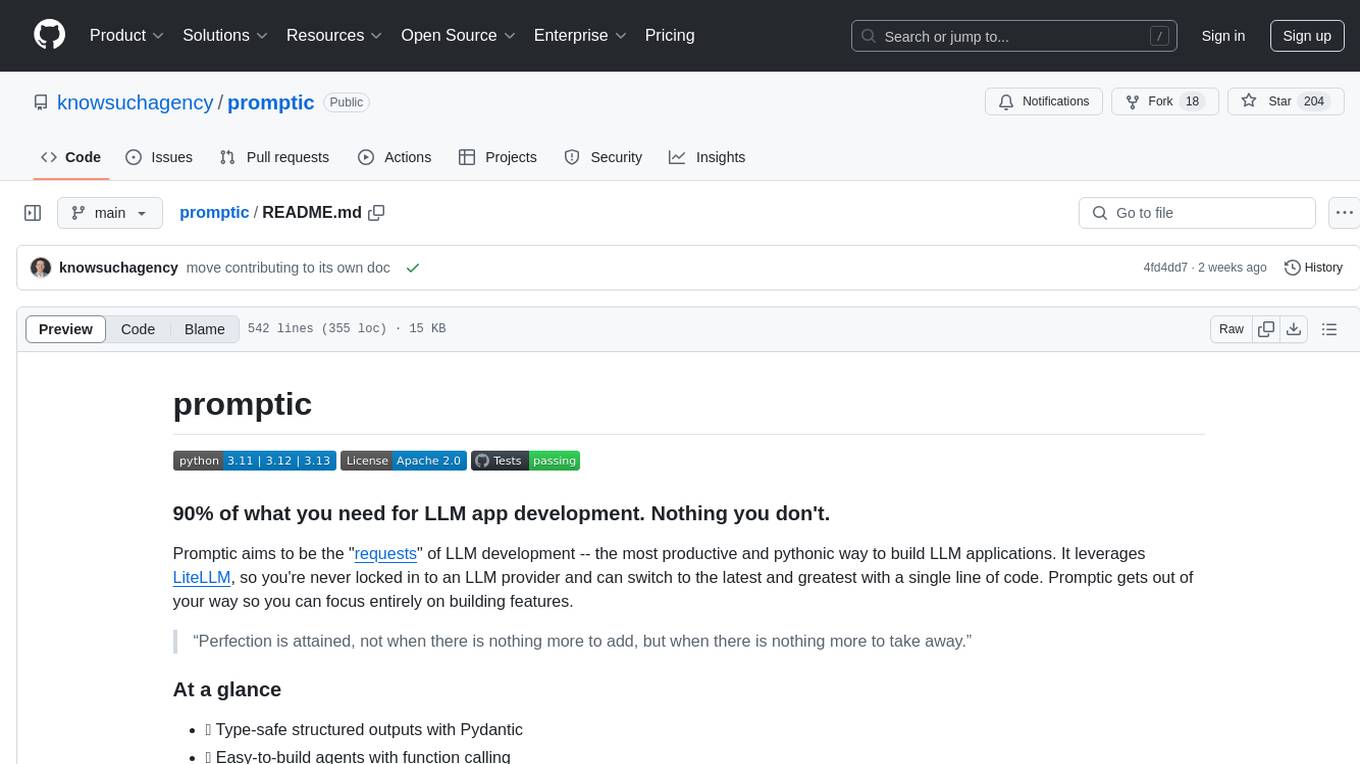
promptic
Promptic is a tool designed for LLM app development, providing a productive and pythonic way to build LLM applications. It leverages LiteLLM, allowing flexibility to switch LLM providers easily. Promptic focuses on building features by providing type-safe structured outputs, easy-to-build agents, streaming support, automatic prompt caching, and built-in conversation memory.
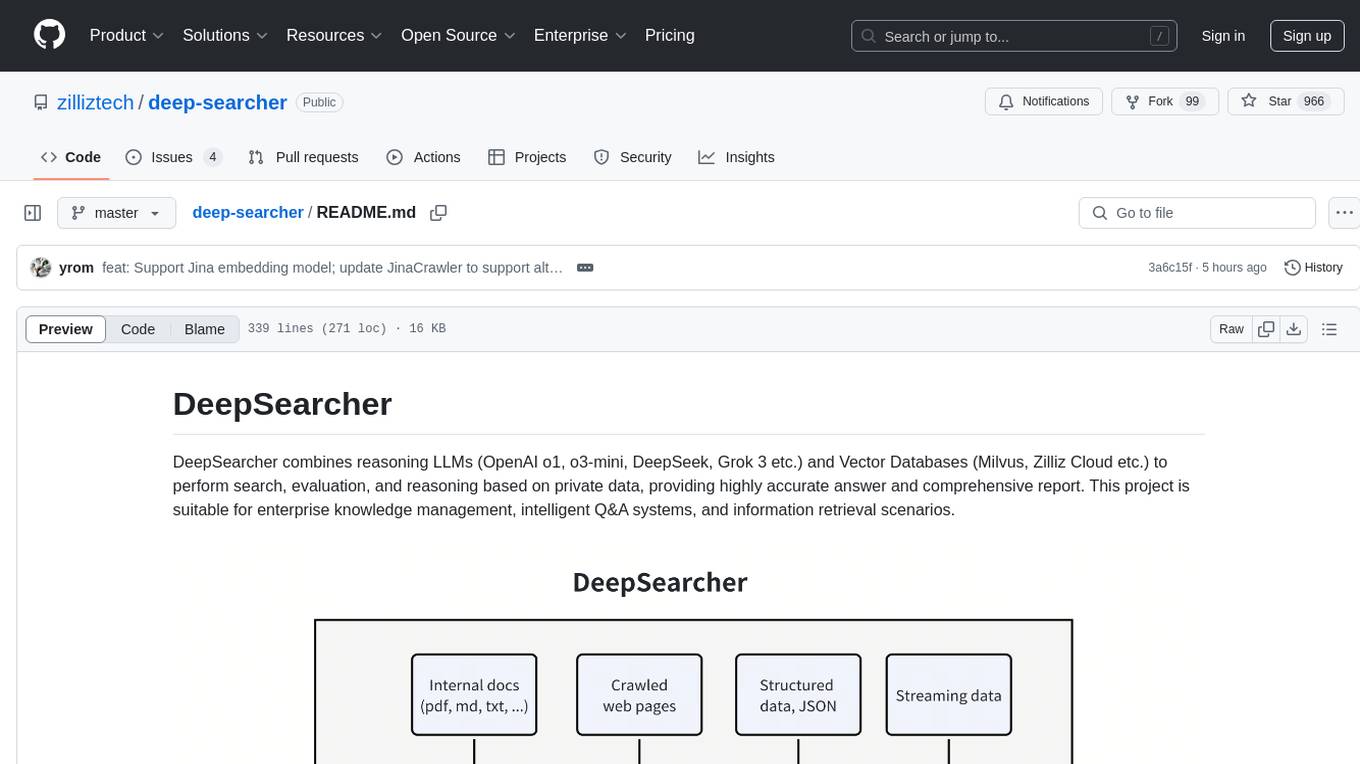
deep-searcher
DeepSearcher is a tool that combines reasoning LLMs and Vector Databases to perform search, evaluation, and reasoning based on private data. It is suitable for enterprise knowledge management, intelligent Q&A systems, and information retrieval scenarios. The tool maximizes the utilization of enterprise internal data while ensuring data security, supports multiple embedding models, and provides support for multiple LLMs for intelligent Q&A and content generation. It also includes features like private data search, vector database management, and document loading with web crawling capabilities under development.
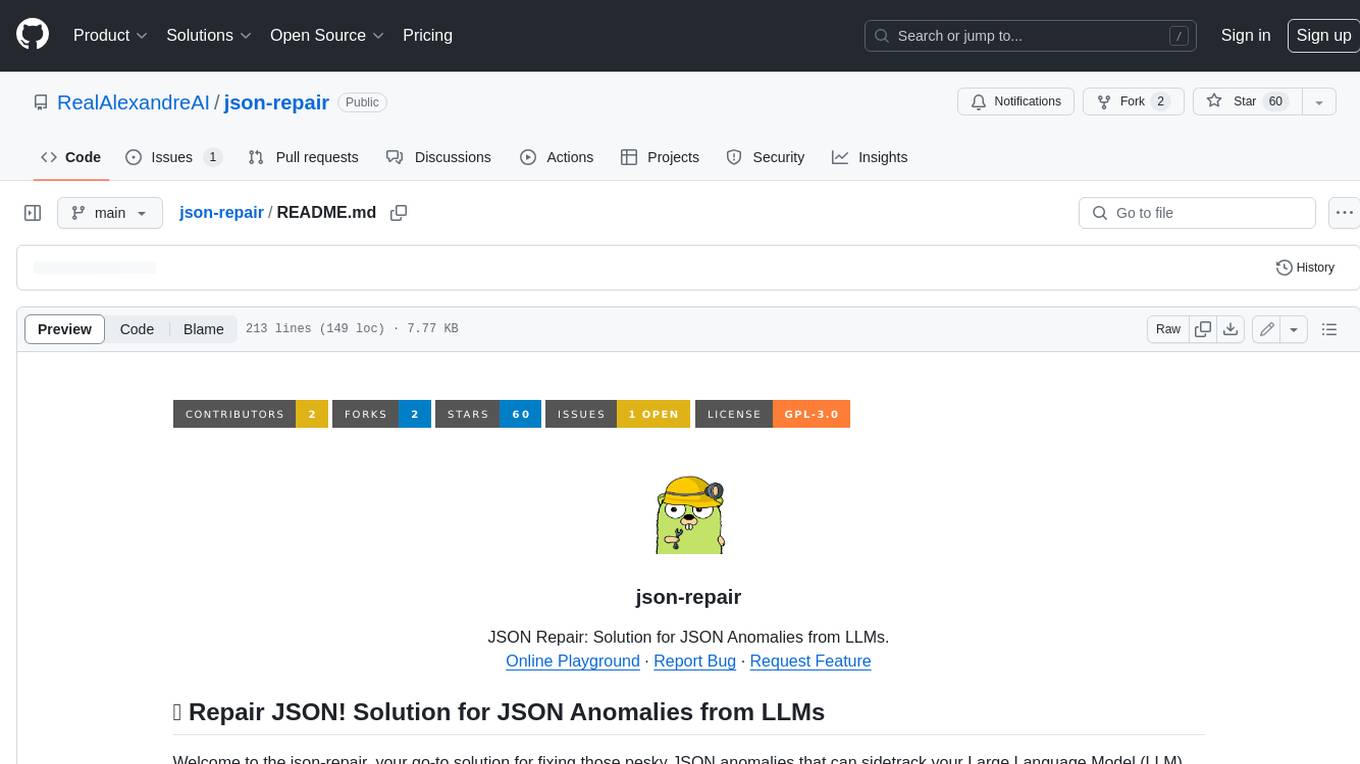
json-repair
JSON Repair is a toolkit designed to address JSON anomalies that can arise from Large Language Models (LLMs). It offers a comprehensive solution for repairing JSON strings, ensuring accuracy and reliability in your data processing. With its user-friendly interface and extensive capabilities, JSON Repair empowers developers to seamlessly integrate JSON repair into their workflows.
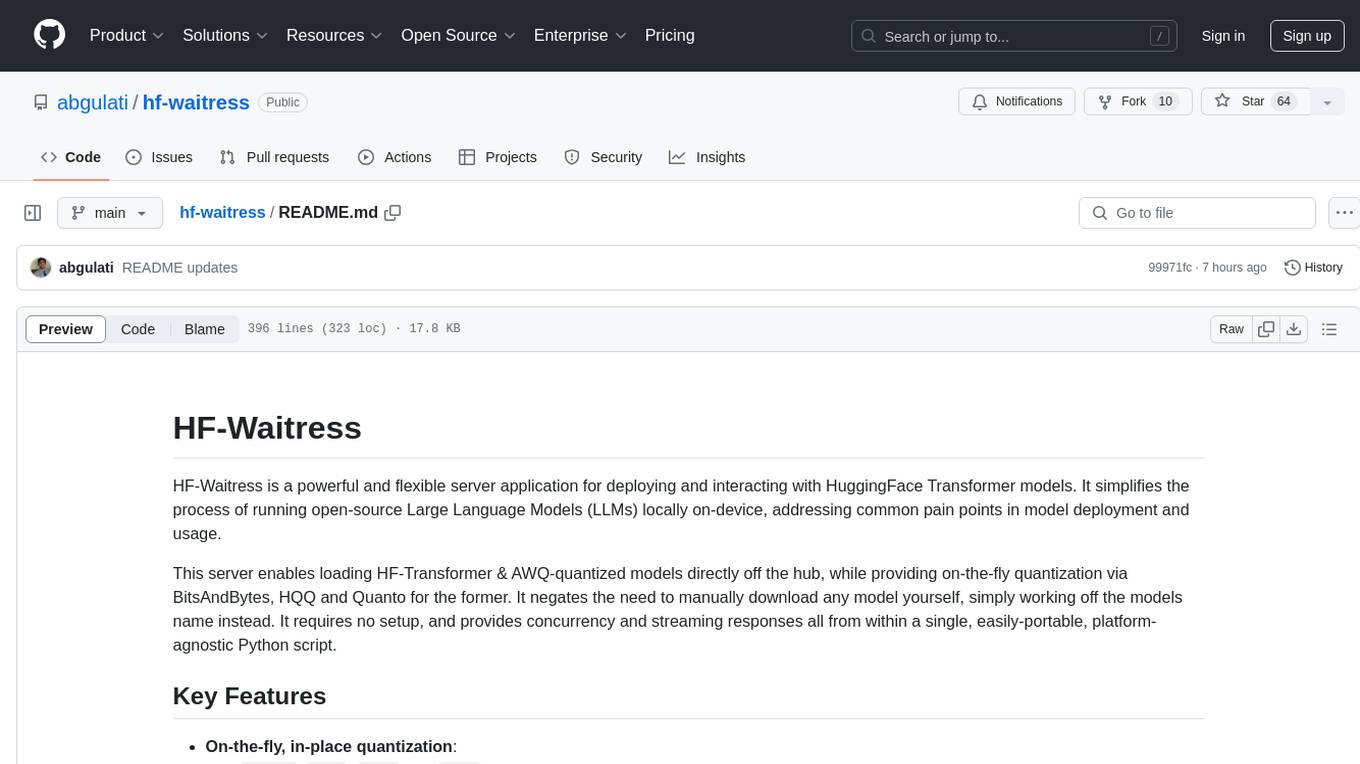
hf-waitress
HF-Waitress is a powerful server application for deploying and interacting with HuggingFace Transformer models. It simplifies running open-source Large Language Models (LLMs) locally on-device, providing on-the-fly quantization via BitsAndBytes, HQQ, and Quanto. It requires no manual model downloads, offers concurrency, streaming responses, and supports various hardware and platforms. The server uses a `config.json` file for easy configuration management and provides detailed error handling and logging.
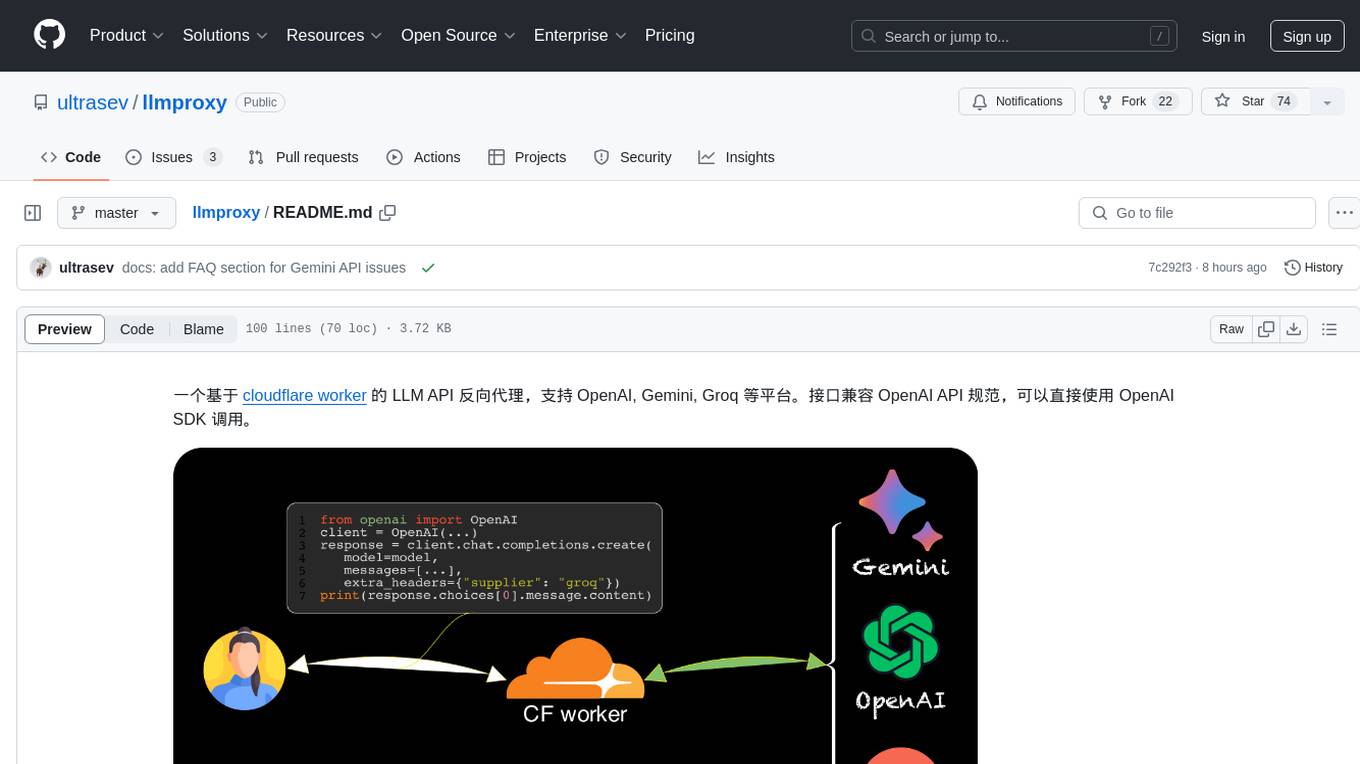
llmproxy
llmproxy is a reverse proxy for LLM API based on Cloudflare Worker, supporting platforms like OpenAI, Gemini, and Groq. The interface is compatible with the OpenAI API specification and can be directly accessed using the OpenAI SDK. It provides a convenient way to interact with various AI platforms through a unified API endpoint, enabling seamless integration and usage in different applications.
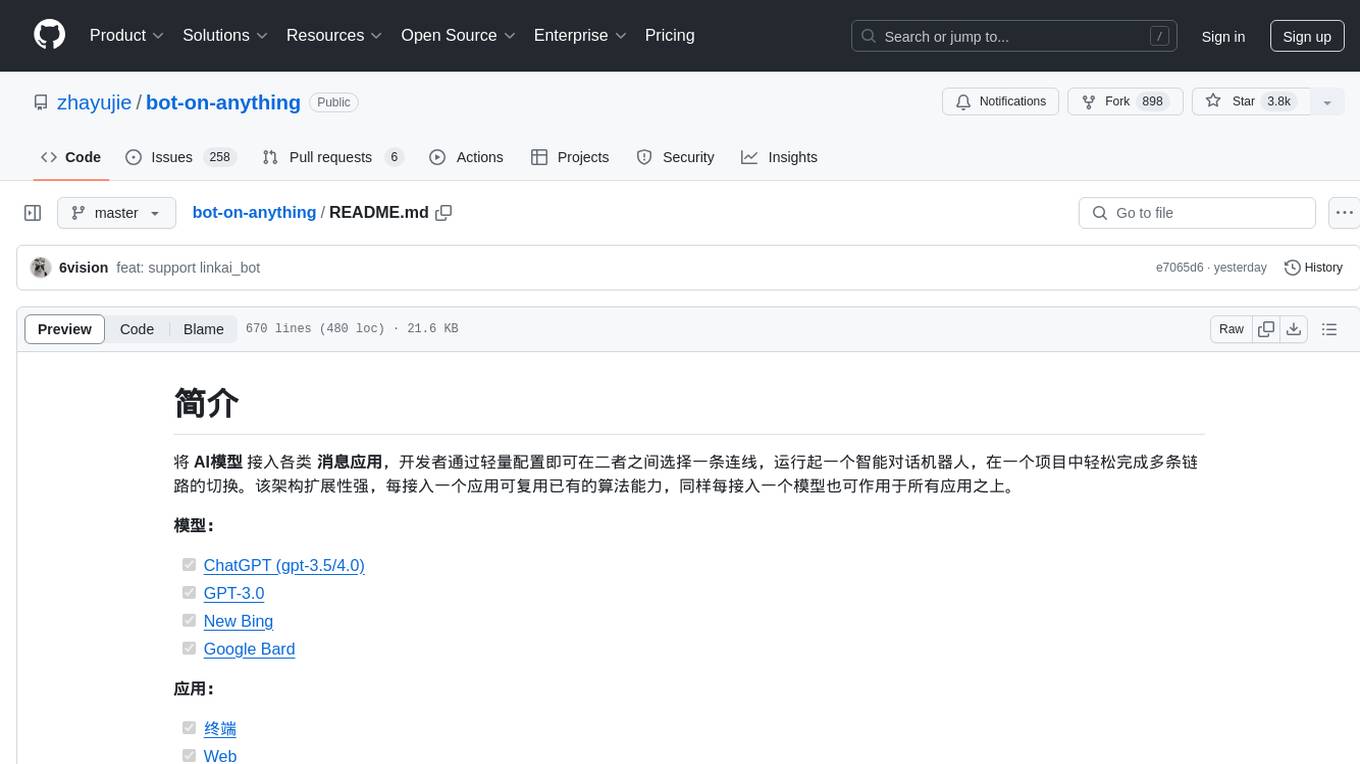
bot-on-anything
The 'bot-on-anything' repository allows developers to integrate various AI models into messaging applications, enabling the creation of intelligent chatbots. By configuring the connections between models and applications, developers can easily switch between multiple channels within a project. The architecture is highly scalable, allowing the reuse of algorithmic capabilities for each new application and model integration. Supported models include ChatGPT, GPT-3.0, New Bing, and Google Bard, while supported applications range from terminals and web platforms to messaging apps like WeChat, Telegram, QQ, and more. The repository provides detailed instructions for setting up the environment, configuring the models and channels, and running the chatbot for various tasks across different messaging platforms.
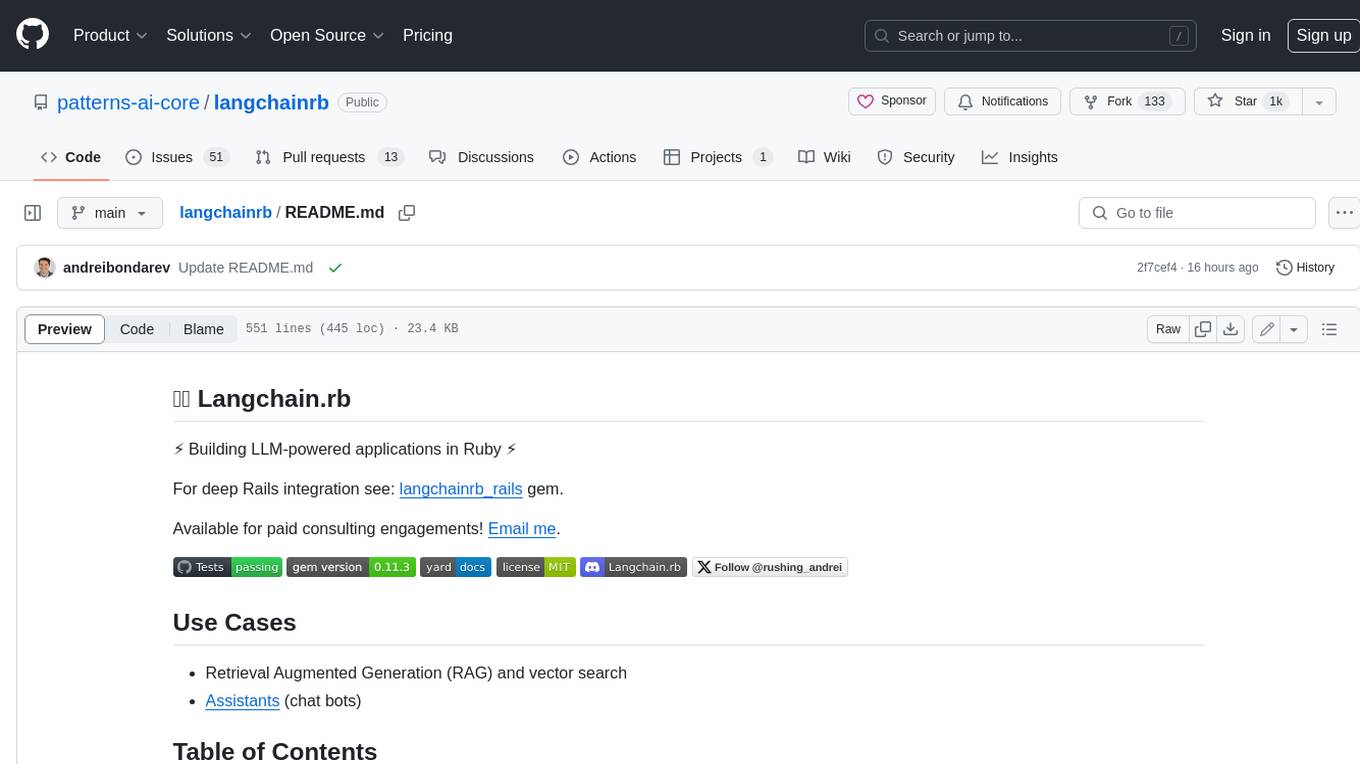
langchainrb
Langchain.rb is a Ruby library that makes it easy to build LLM-powered applications. It provides a unified interface to a variety of LLMs, vector search databases, and other tools, making it easy to build and deploy RAG (Retrieval Augmented Generation) systems and assistants. Langchain.rb is open source and available under the MIT License.
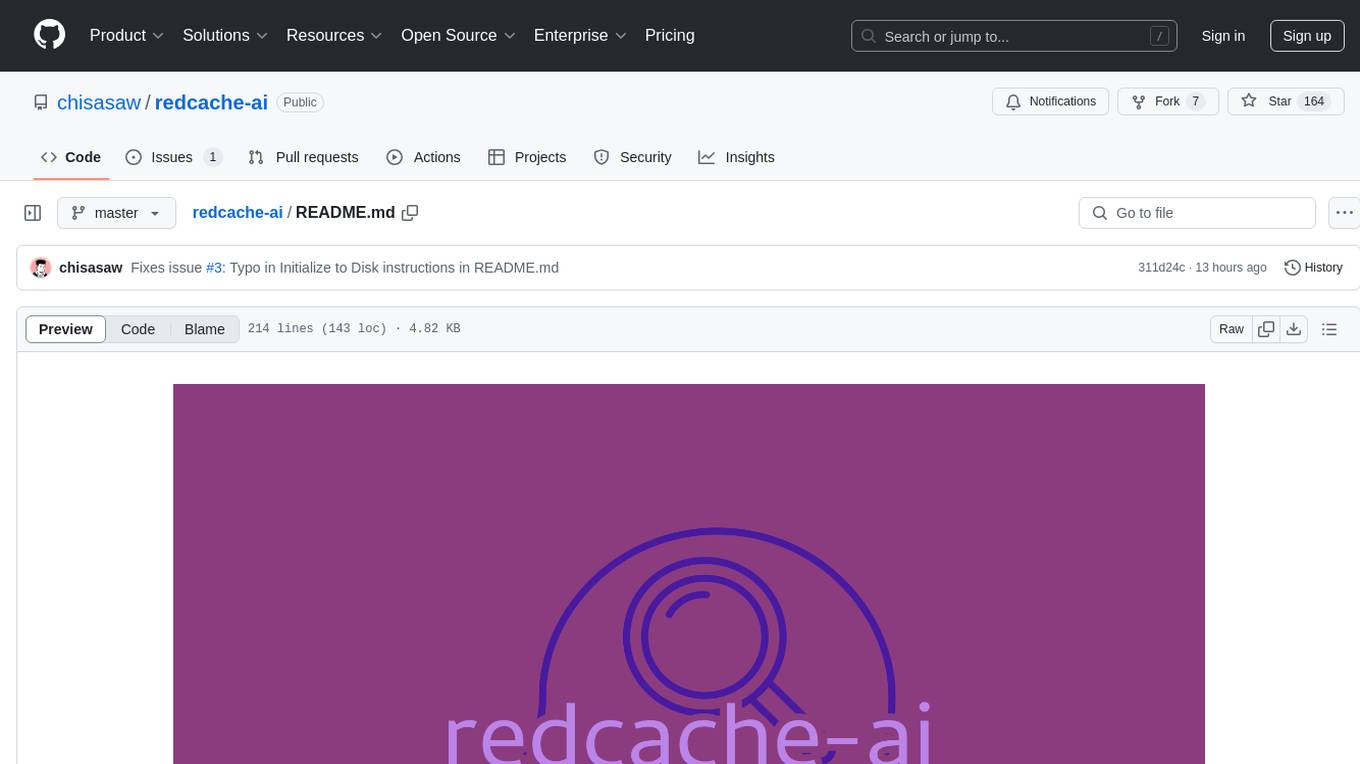
redcache-ai
RedCache-ai is a memory framework designed for Large Language Models and Agents. It provides a dynamic memory framework for developers to build various applications, from AI-powered dating apps to healthcare diagnostics platforms. Users can store, retrieve, search, update, and delete memories using RedCache-ai. The tool also supports integration with OpenAI for enhancing memories. RedCache-ai aims to expand its functionality by integrating with more LLM providers, adding support for AI Agents, and providing a hosted version.
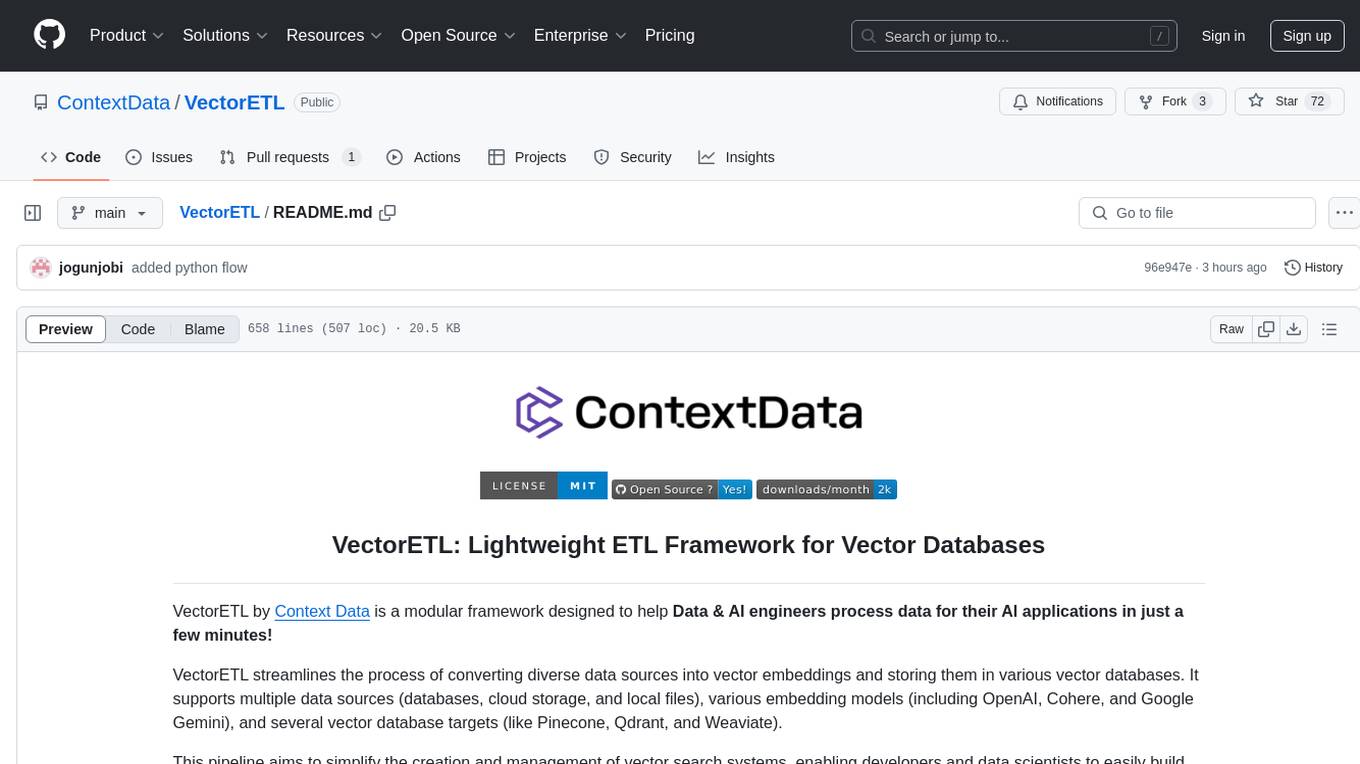
VectorETL
VectorETL is a lightweight ETL framework designed to assist Data & AI engineers in processing data for AI applications quickly. It streamlines the conversion of diverse data sources into vector embeddings and storage in various vector databases. The framework supports multiple data sources, embedding models, and vector database targets, simplifying the creation and management of vector search systems for semantic search, recommendation systems, and other vector-based operations.
For similar tasks
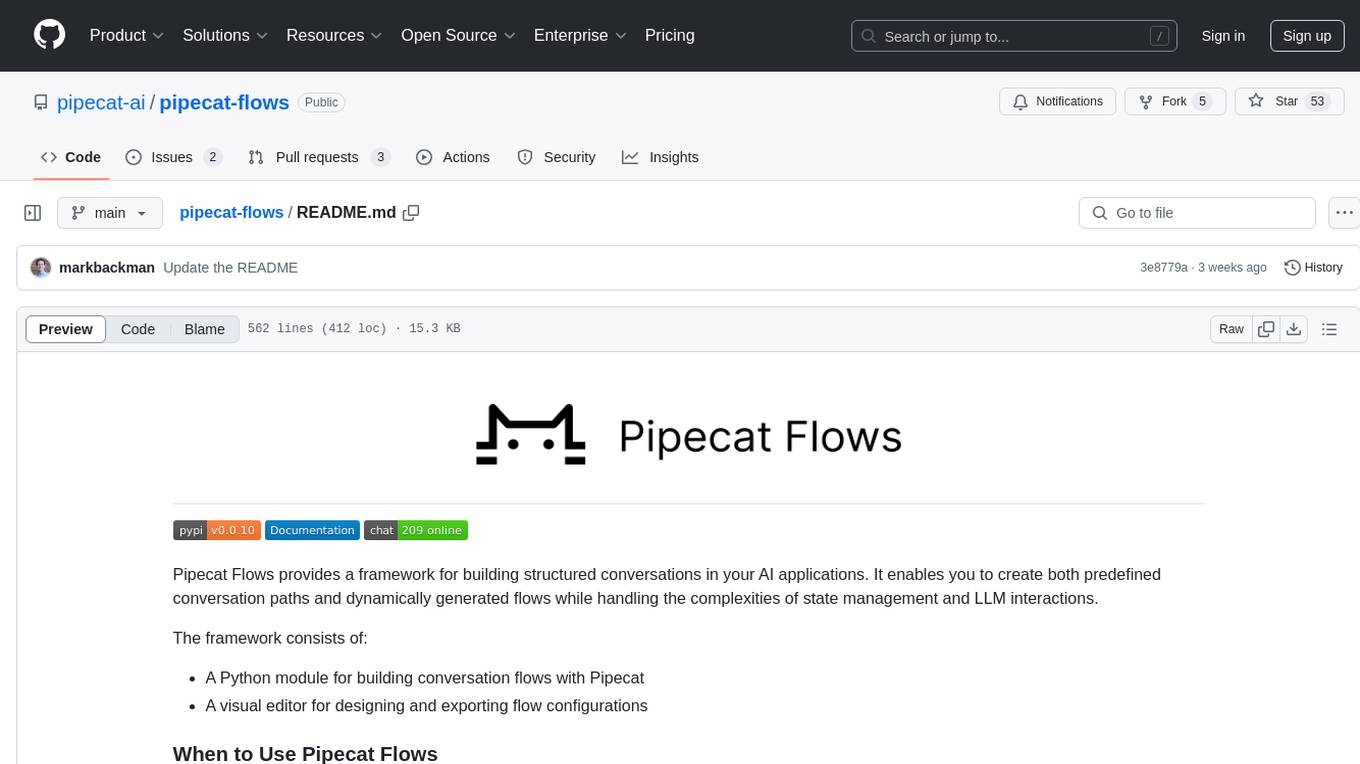
pipecat-flows
Pipecat Flows is a framework designed for building structured conversations in AI applications. It allows users to create both predefined conversation paths and dynamically generated flows, handling state management and LLM interactions. The framework includes a Python module for building conversation flows and a visual editor for designing and exporting flow configurations. Pipecat Flows is suitable for scenarios such as customer service scripts, intake forms, personalized experiences, and complex decision trees.
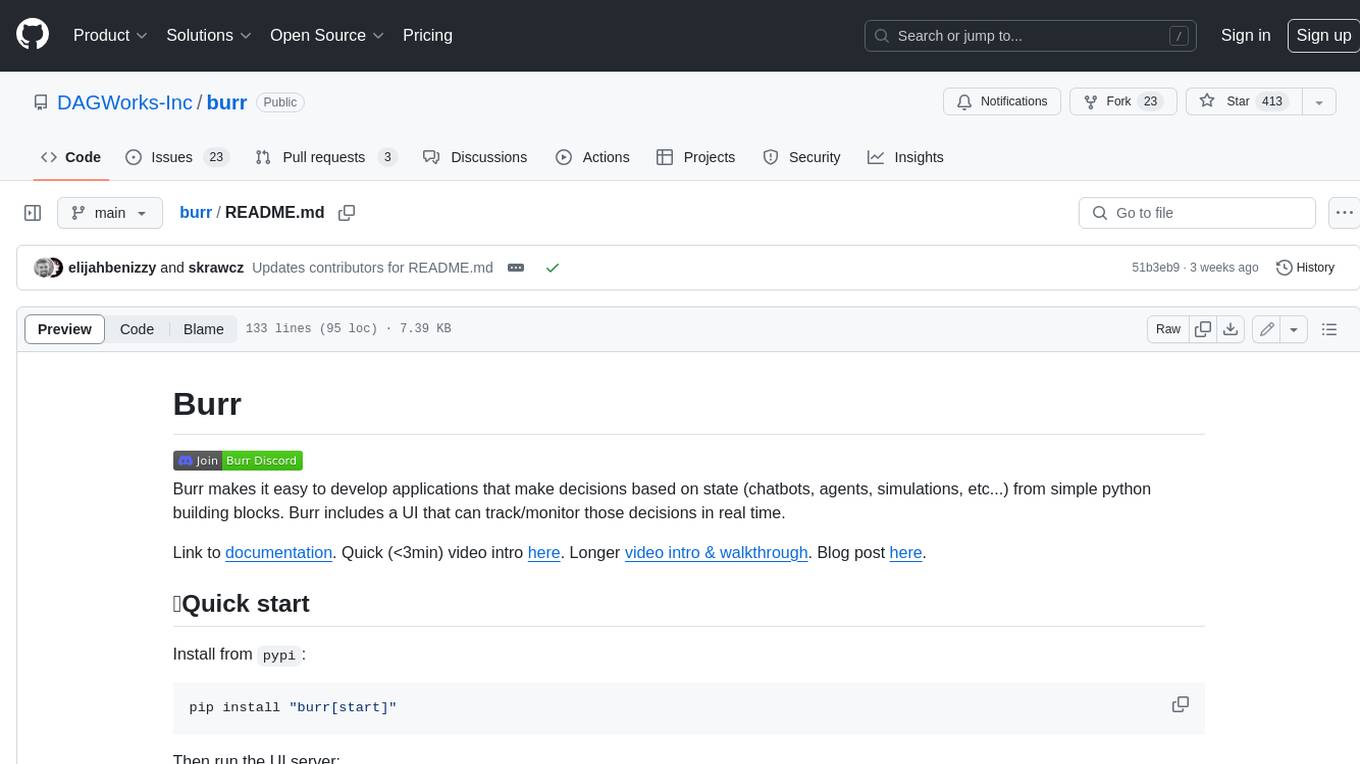
burr
Burr is a Python library and UI that makes it easy to develop applications that make decisions based on state (chatbots, agents, simulations, etc...). Burr includes a UI that can track/monitor those decisions in real time.
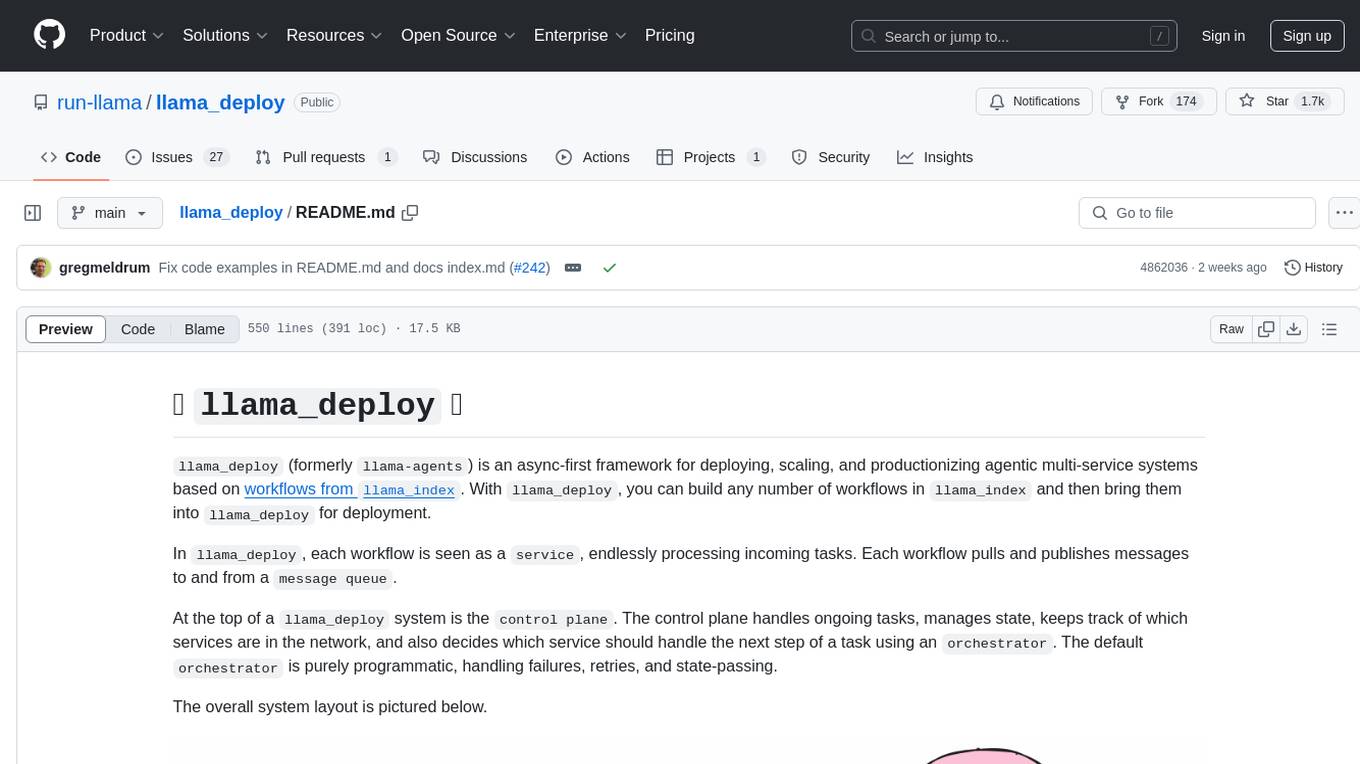
llama_deploy
llama_deploy is an async-first framework for deploying, scaling, and productionizing agentic multi-service systems based on workflows from llama_index. It allows building workflows in llama_index and deploying them seamlessly with minimal changes to code. The system includes services endlessly processing tasks, a control plane managing state and services, an orchestrator deciding task handling, and fault tolerance mechanisms. It is designed for high-concurrency scenarios, enabling real-time and high-throughput applications.
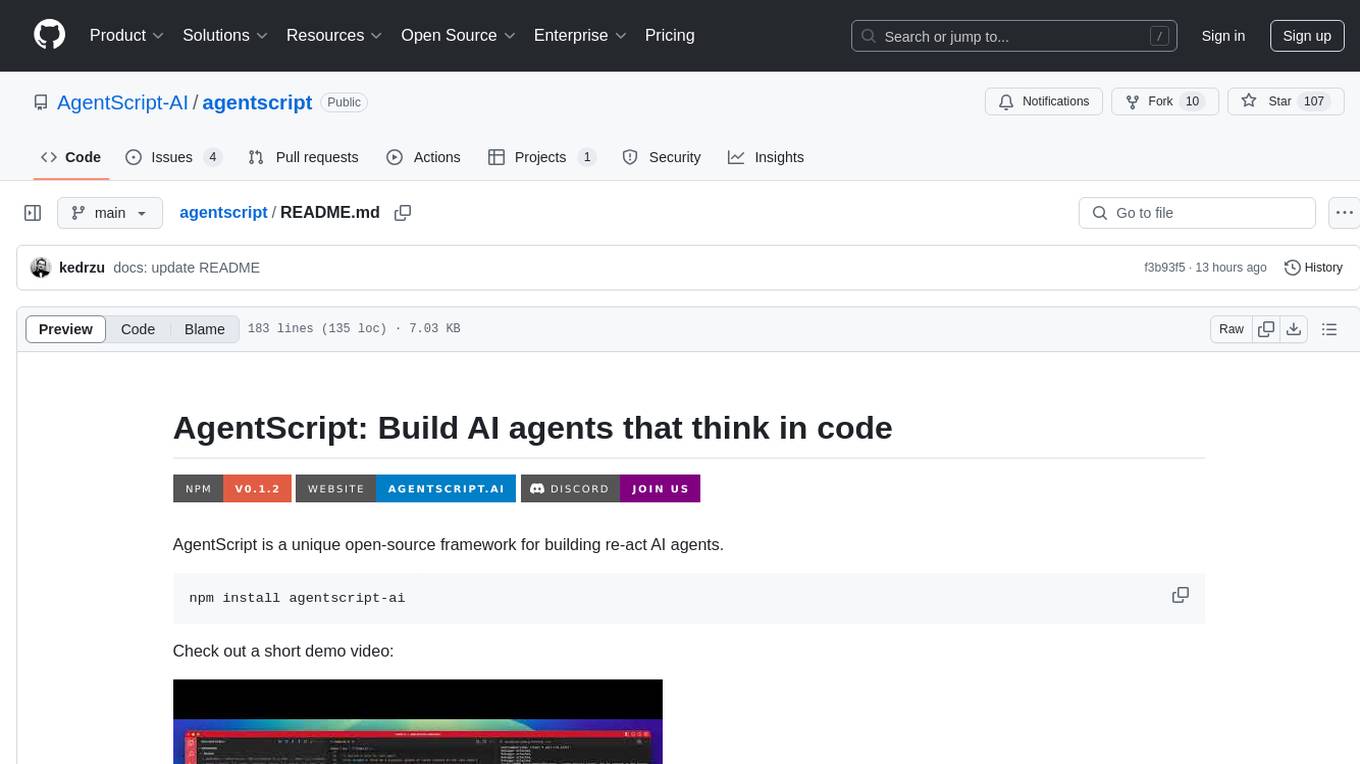
agentscript
AgentScript is an open-source framework for building AI agents that think in code. It prompts a language model to generate JavaScript code, which is then executed in a dedicated runtime with resumability, state persistence, and interactivity. The framework allows for abstract task execution without needing to know all the data beforehand, making it flexible and efficient. AgentScript supports tools, deterministic functions, and LLM-enabled functions, enabling dynamic data processing and decision-making. It also provides state management and human-in-the-loop capabilities, allowing for pausing, serialization, and resumption of execution.
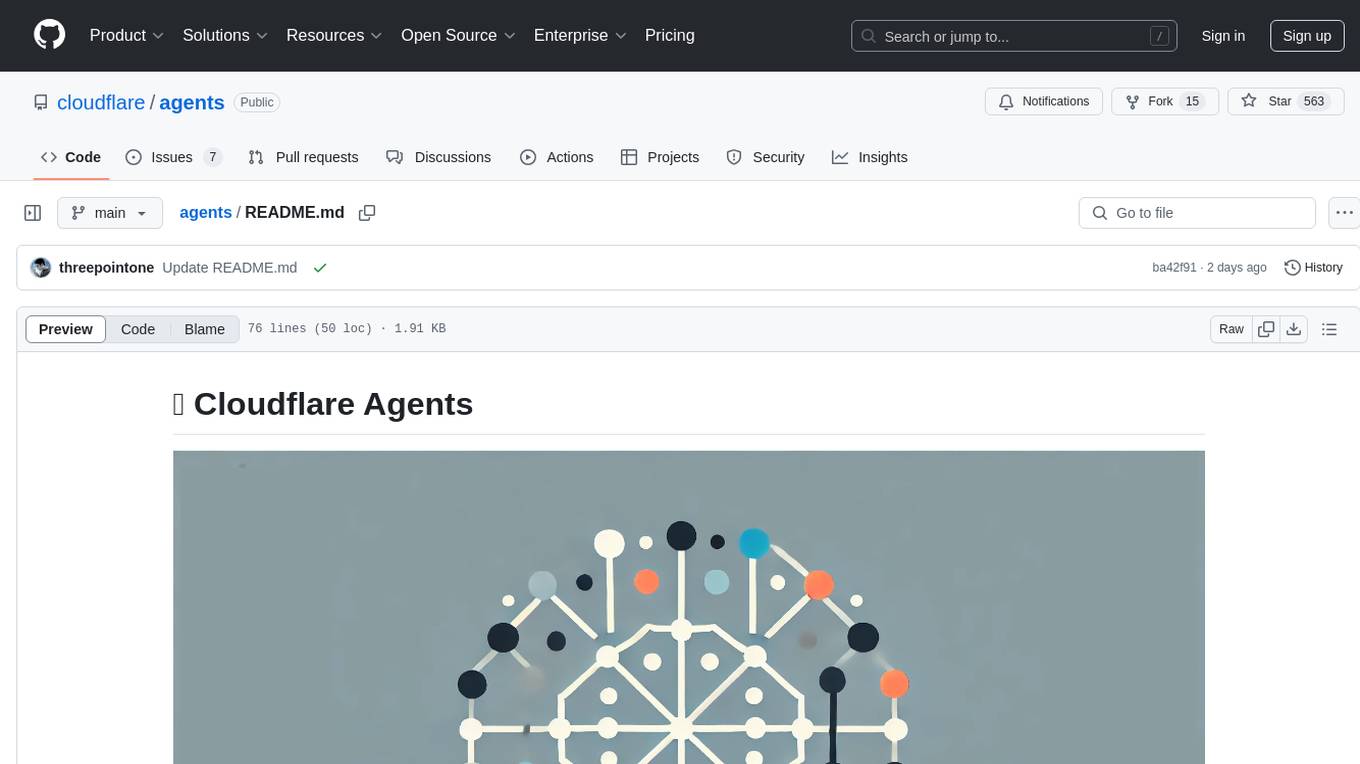
agents
Cloudflare Agents is a framework for building intelligent, stateful agents that persist, think, and evolve at the edge of the network. It allows for maintaining persistent state and memory, real-time communication, processing and learning from interactions, autonomous operation at global scale, and hibernating when idle. The project is actively evolving with focus on core agent framework, WebSocket communication, HTTP endpoints, React integration, and basic AI chat capabilities. Future developments include advanced memory systems, WebRTC for audio/video, email integration, evaluation framework, enhanced observability, and self-hosting guide.
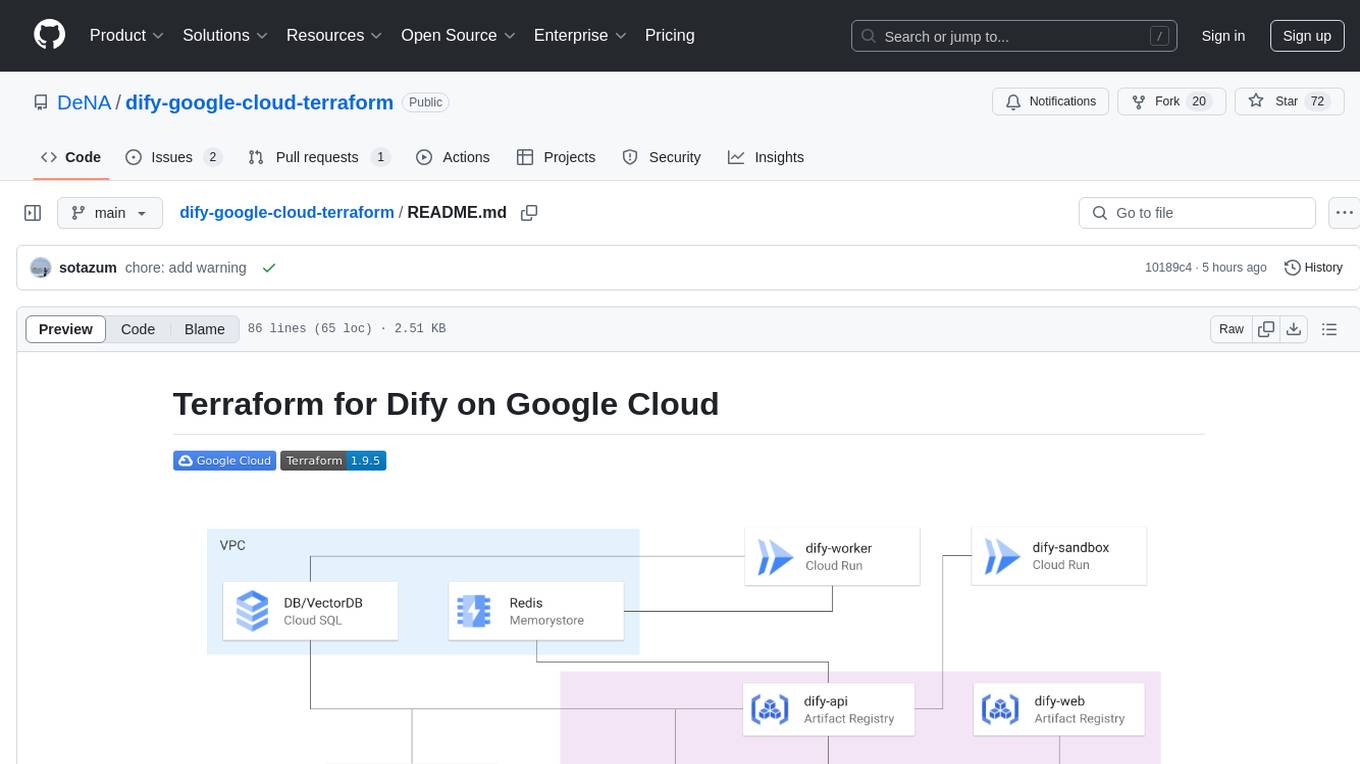
dify-google-cloud-terraform
This repository provides Terraform configurations to automatically set up Google Cloud resources and deploy Dify in a highly available configuration. It includes features such as serverless hosting, auto-scaling, and data persistence. Users need a Google Cloud account, Terraform, and gcloud CLI installed to use this tool. The configuration involves setting environment-specific values and creating a GCS bucket for managing Terraform state. The tool allows users to initialize Terraform, create Artifact Registry repository, build and push container images, plan and apply Terraform changes, and cleanup resources when needed.
For similar jobs
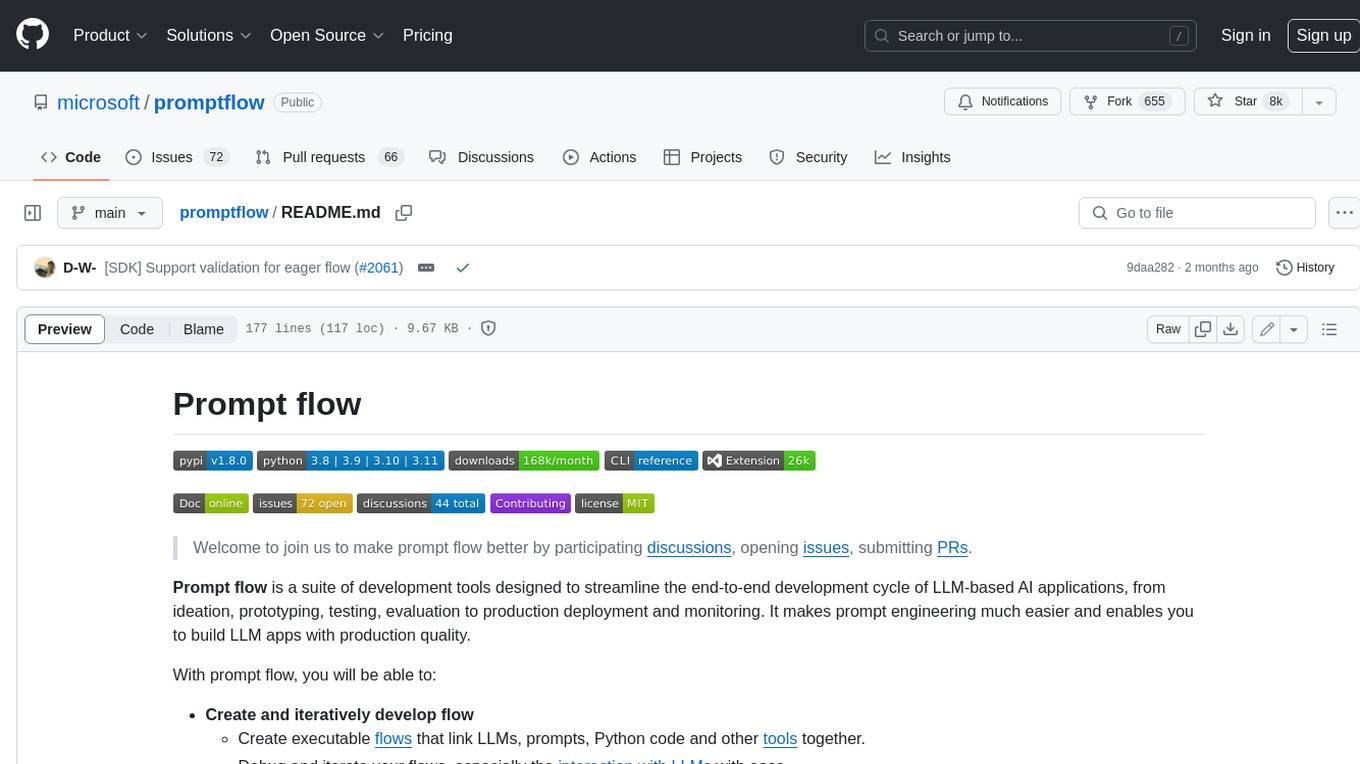
promptflow
**Prompt flow** is a suite of development tools designed to streamline the end-to-end development cycle of LLM-based AI applications, from ideation, prototyping, testing, evaluation to production deployment and monitoring. It makes prompt engineering much easier and enables you to build LLM apps with production quality.
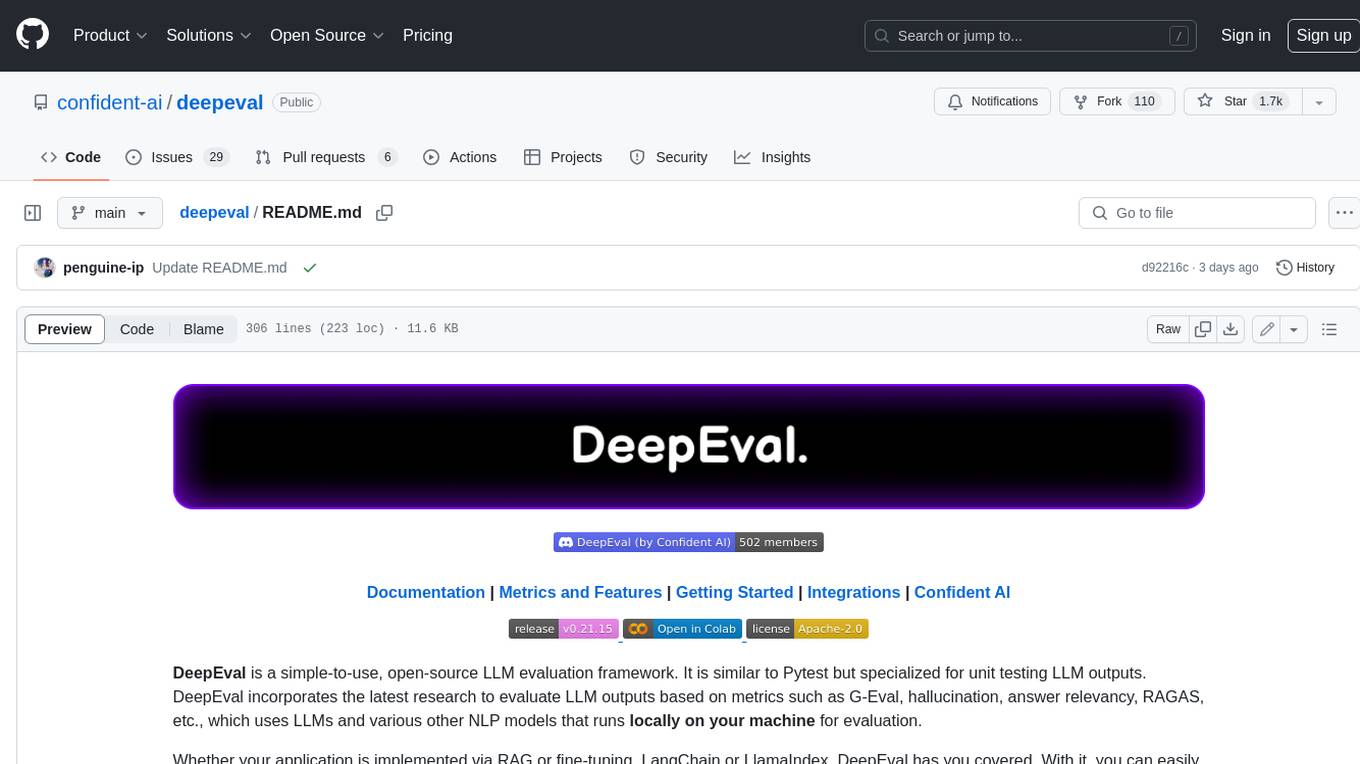
deepeval
DeepEval is a simple-to-use, open-source LLM evaluation framework specialized for unit testing LLM outputs. It incorporates various metrics such as G-Eval, hallucination, answer relevancy, RAGAS, etc., and runs locally on your machine for evaluation. It provides a wide range of ready-to-use evaluation metrics, allows for creating custom metrics, integrates with any CI/CD environment, and enables benchmarking LLMs on popular benchmarks. DeepEval is designed for evaluating RAG and fine-tuning applications, helping users optimize hyperparameters, prevent prompt drifting, and transition from OpenAI to hosting their own Llama2 with confidence.
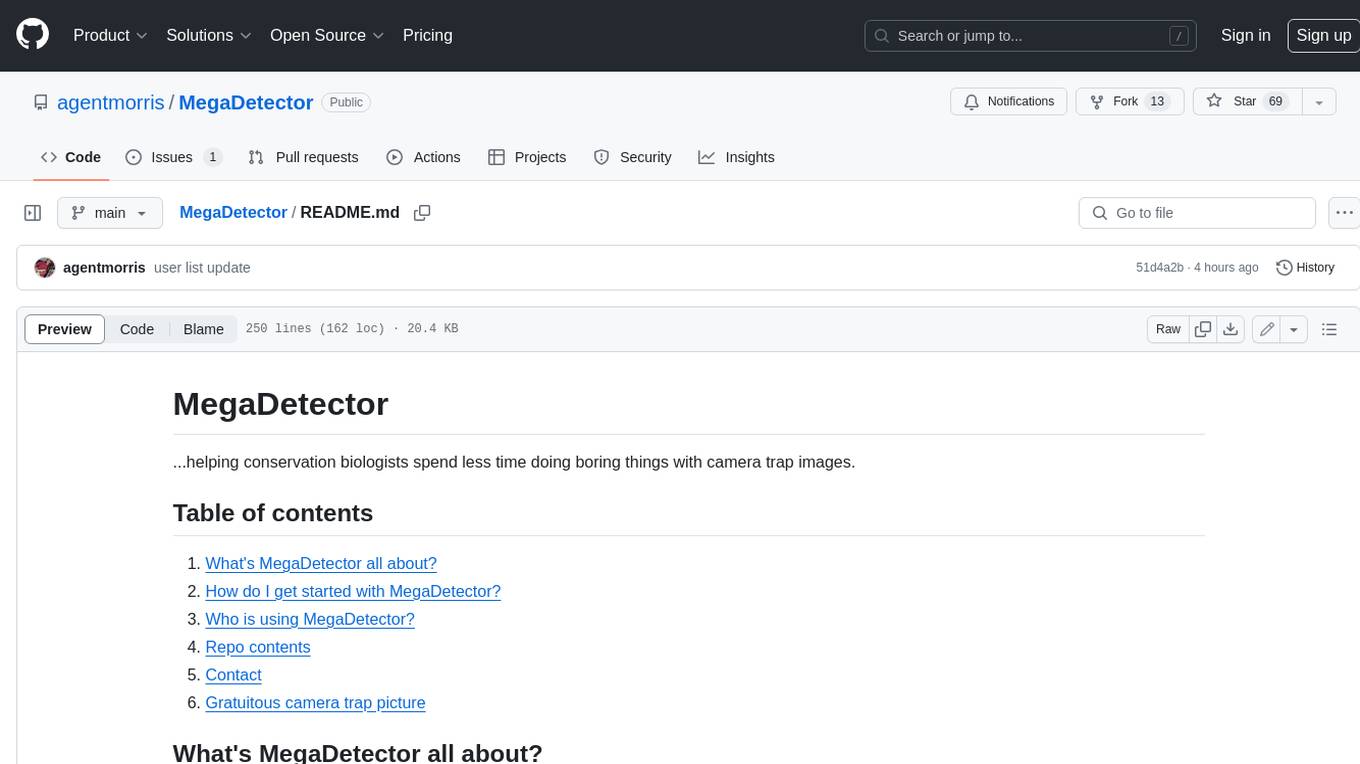
MegaDetector
MegaDetector is an AI model that identifies animals, people, and vehicles in camera trap images (which also makes it useful for eliminating blank images). This model is trained on several million images from a variety of ecosystems. MegaDetector is just one of many tools that aims to make conservation biologists more efficient with AI. If you want to learn about other ways to use AI to accelerate camera trap workflows, check out our of the field, affectionately titled "Everything I know about machine learning and camera traps".
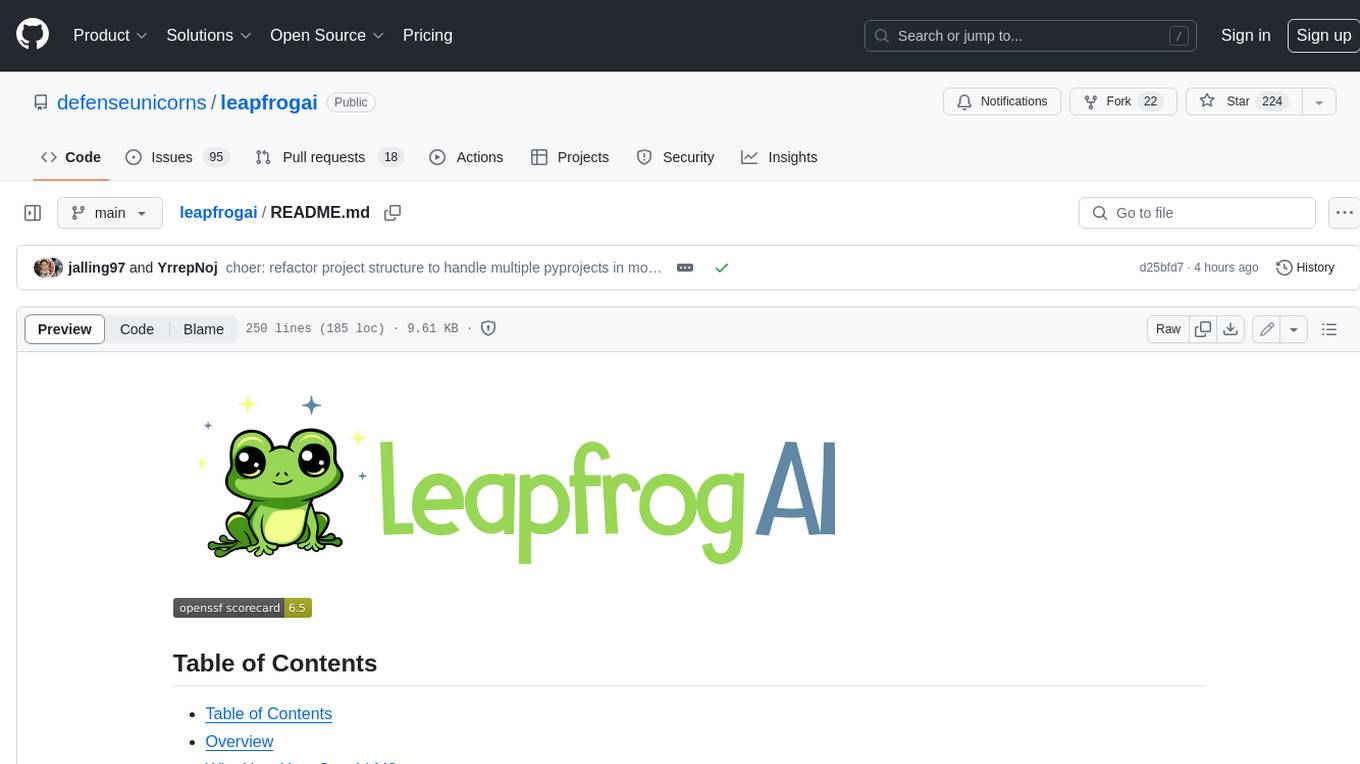
leapfrogai
LeapfrogAI is a self-hosted AI platform designed to be deployed in air-gapped resource-constrained environments. It brings sophisticated AI solutions to these environments by hosting all the necessary components of an AI stack, including vector databases, model backends, API, and UI. LeapfrogAI's API closely matches that of OpenAI, allowing tools built for OpenAI/ChatGPT to function seamlessly with a LeapfrogAI backend. It provides several backends for various use cases, including llama-cpp-python, whisper, text-embeddings, and vllm. LeapfrogAI leverages Chainguard's apko to harden base python images, ensuring the latest supported Python versions are used by the other components of the stack. The LeapfrogAI SDK provides a standard set of protobuffs and python utilities for implementing backends and gRPC. LeapfrogAI offers UI options for common use-cases like chat, summarization, and transcription. It can be deployed and run locally via UDS and Kubernetes, built out using Zarf packages. LeapfrogAI is supported by a community of users and contributors, including Defense Unicorns, Beast Code, Chainguard, Exovera, Hypergiant, Pulze, SOSi, United States Navy, United States Air Force, and United States Space Force.
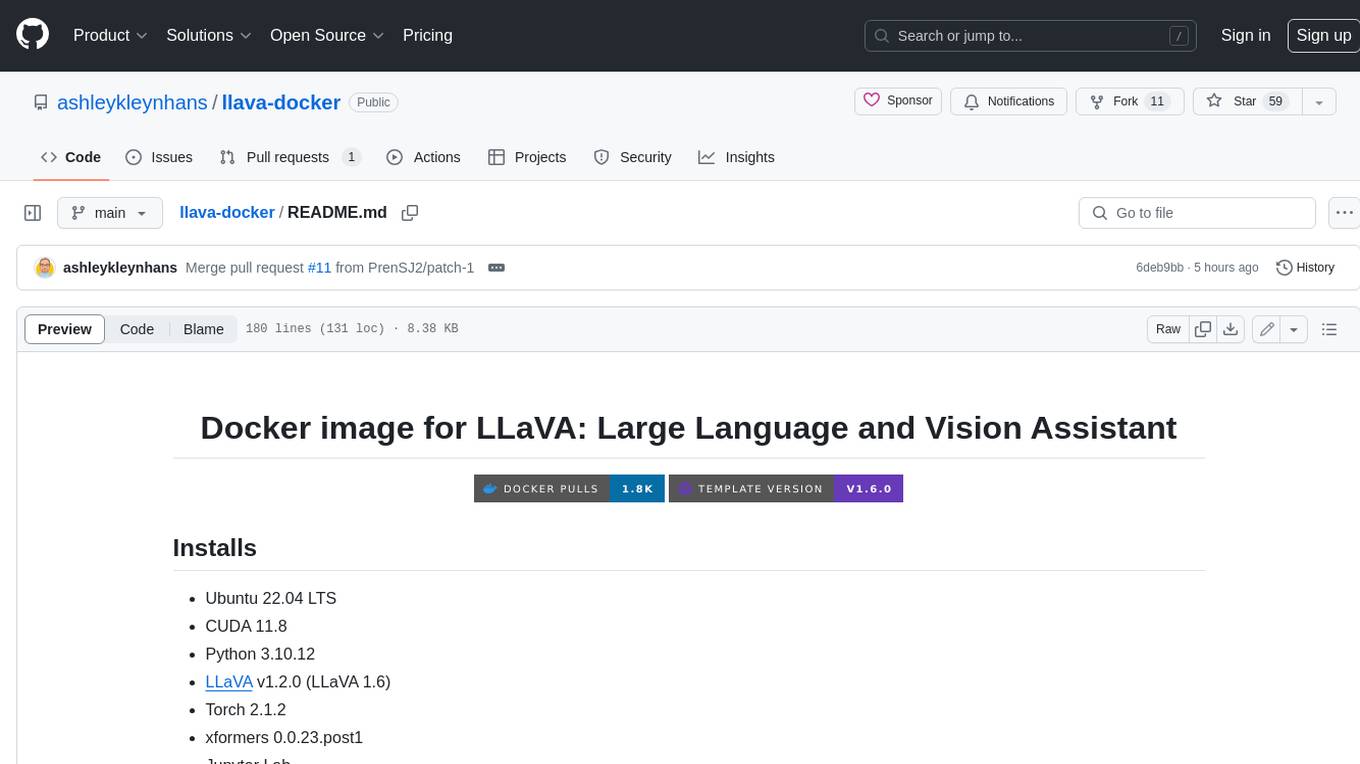
llava-docker
This Docker image for LLaVA (Large Language and Vision Assistant) provides a convenient way to run LLaVA locally or on RunPod. LLaVA is a powerful AI tool that combines natural language processing and computer vision capabilities. With this Docker image, you can easily access LLaVA's functionalities for various tasks, including image captioning, visual question answering, text summarization, and more. The image comes pre-installed with LLaVA v1.2.0, Torch 2.1.2, xformers 0.0.23.post1, and other necessary dependencies. You can customize the model used by setting the MODEL environment variable. The image also includes a Jupyter Lab environment for interactive development and exploration. Overall, this Docker image offers a comprehensive and user-friendly platform for leveraging LLaVA's capabilities.
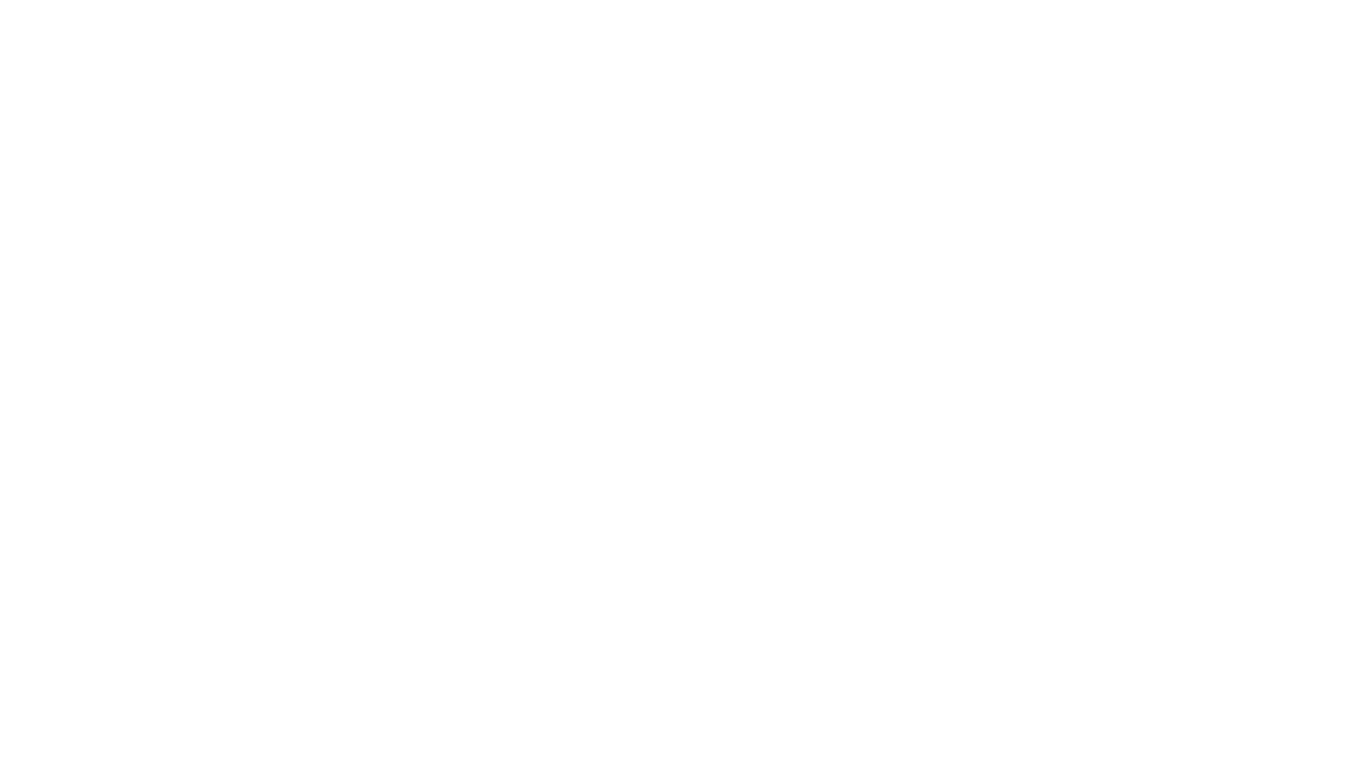
carrot
The 'carrot' repository on GitHub provides a list of free and user-friendly ChatGPT mirror sites for easy access. The repository includes sponsored sites offering various GPT models and services. Users can find and share sites, report errors, and access stable and recommended sites for ChatGPT usage. The repository also includes a detailed list of ChatGPT sites, their features, and accessibility options, making it a valuable resource for ChatGPT users seeking free and unlimited GPT services.
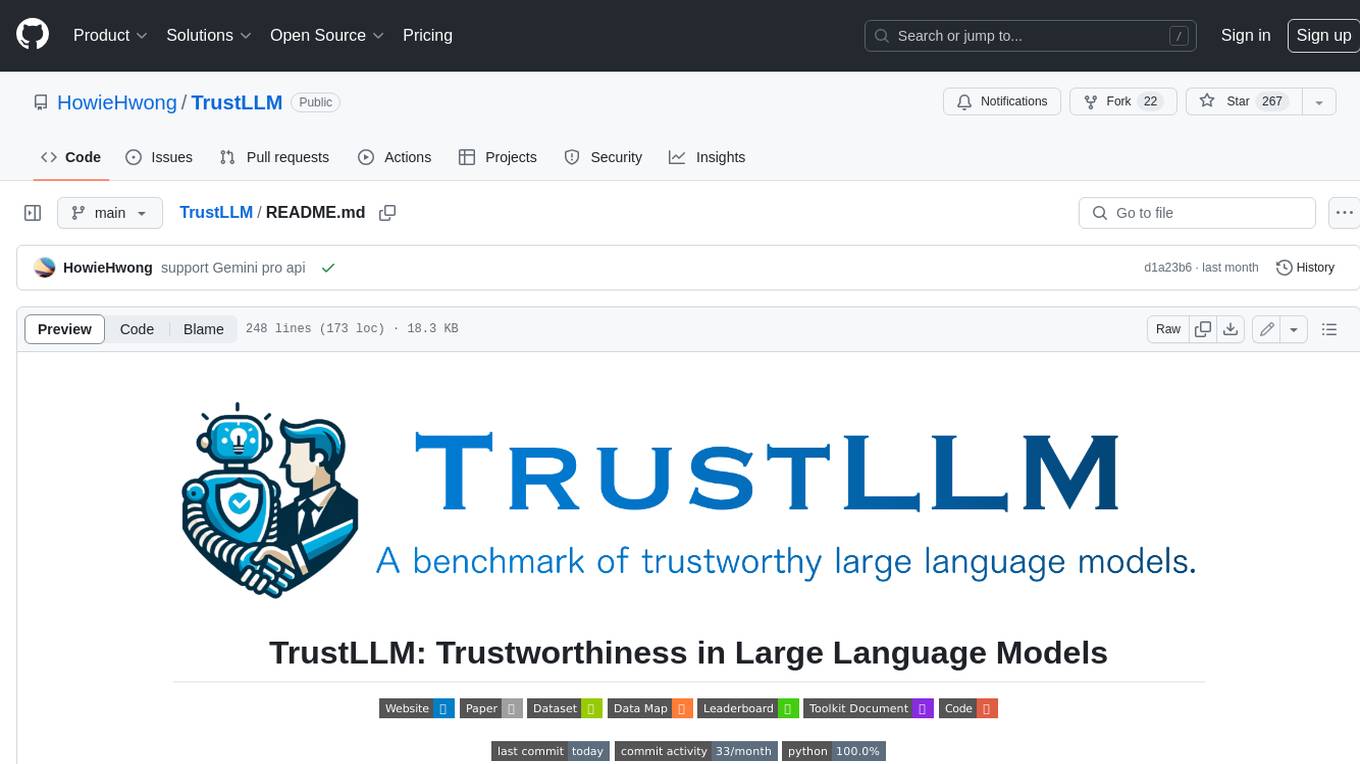
TrustLLM
TrustLLM is a comprehensive study of trustworthiness in LLMs, including principles for different dimensions of trustworthiness, established benchmark, evaluation, and analysis of trustworthiness for mainstream LLMs, and discussion of open challenges and future directions. Specifically, we first propose a set of principles for trustworthy LLMs that span eight different dimensions. Based on these principles, we further establish a benchmark across six dimensions including truthfulness, safety, fairness, robustness, privacy, and machine ethics. We then present a study evaluating 16 mainstream LLMs in TrustLLM, consisting of over 30 datasets. The document explains how to use the trustllm python package to help you assess the performance of your LLM in trustworthiness more quickly. For more details about TrustLLM, please refer to project website.
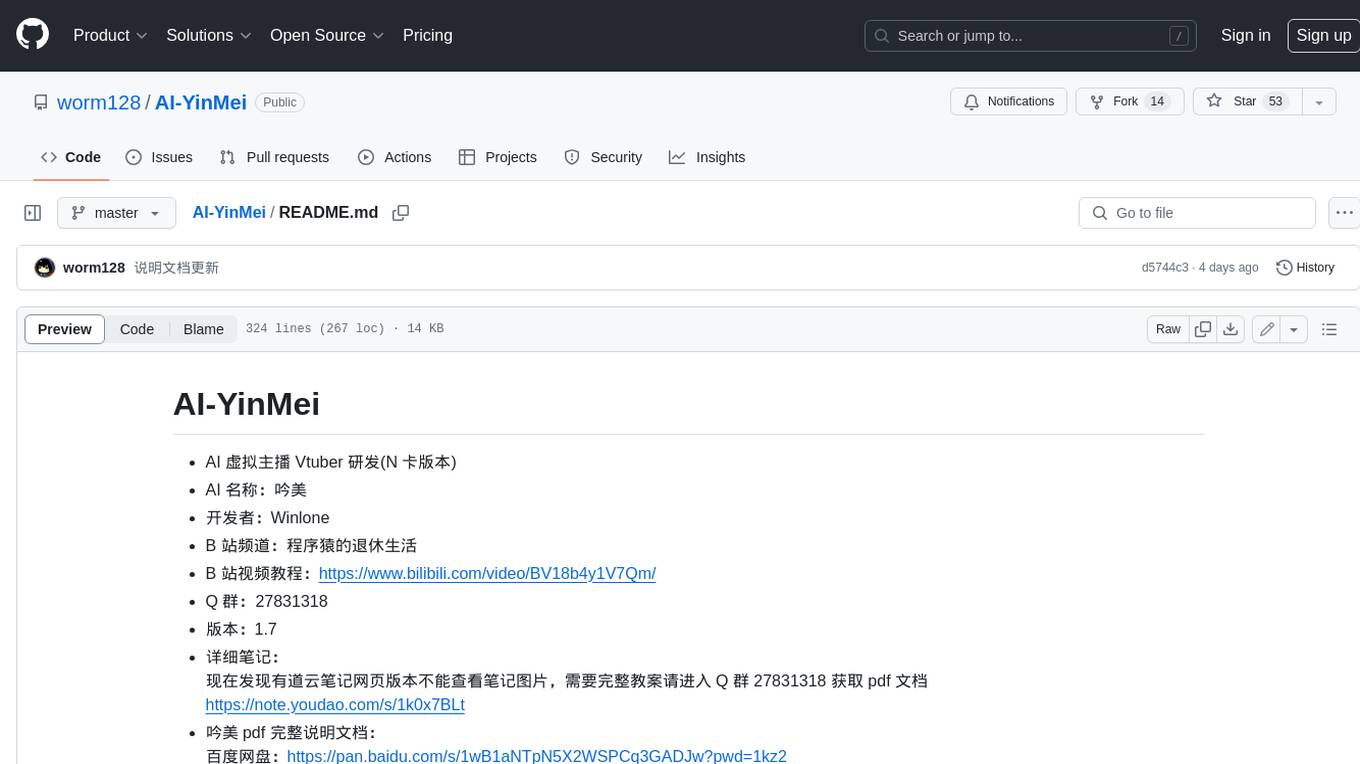
AI-YinMei
AI-YinMei is an AI virtual anchor Vtuber development tool (N card version). It supports fastgpt knowledge base chat dialogue, a complete set of solutions for LLM large language models: [fastgpt] + [one-api] + [Xinference], supports docking bilibili live broadcast barrage reply and entering live broadcast welcome speech, supports Microsoft edge-tts speech synthesis, supports Bert-VITS2 speech synthesis, supports GPT-SoVITS speech synthesis, supports expression control Vtuber Studio, supports painting stable-diffusion-webui output OBS live broadcast room, supports painting picture pornography public-NSFW-y-distinguish, supports search and image search service duckduckgo (requires magic Internet access), supports image search service Baidu image search (no magic Internet access), supports AI reply chat box [html plug-in], supports AI singing Auto-Convert-Music, supports playlist [html plug-in], supports dancing function, supports expression video playback, supports head touching action, supports gift smashing action, supports singing automatic start dancing function, chat and singing automatic cycle swing action, supports multi scene switching, background music switching, day and night automatic switching scene, supports open singing and painting, let AI automatically judge the content.