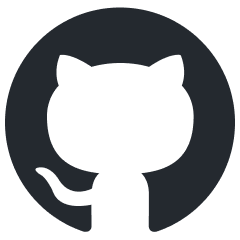
VectorETL
Build super simple end-to-end data & ETL pipelines for your vector databases and Generative AI applications
Stars: 72
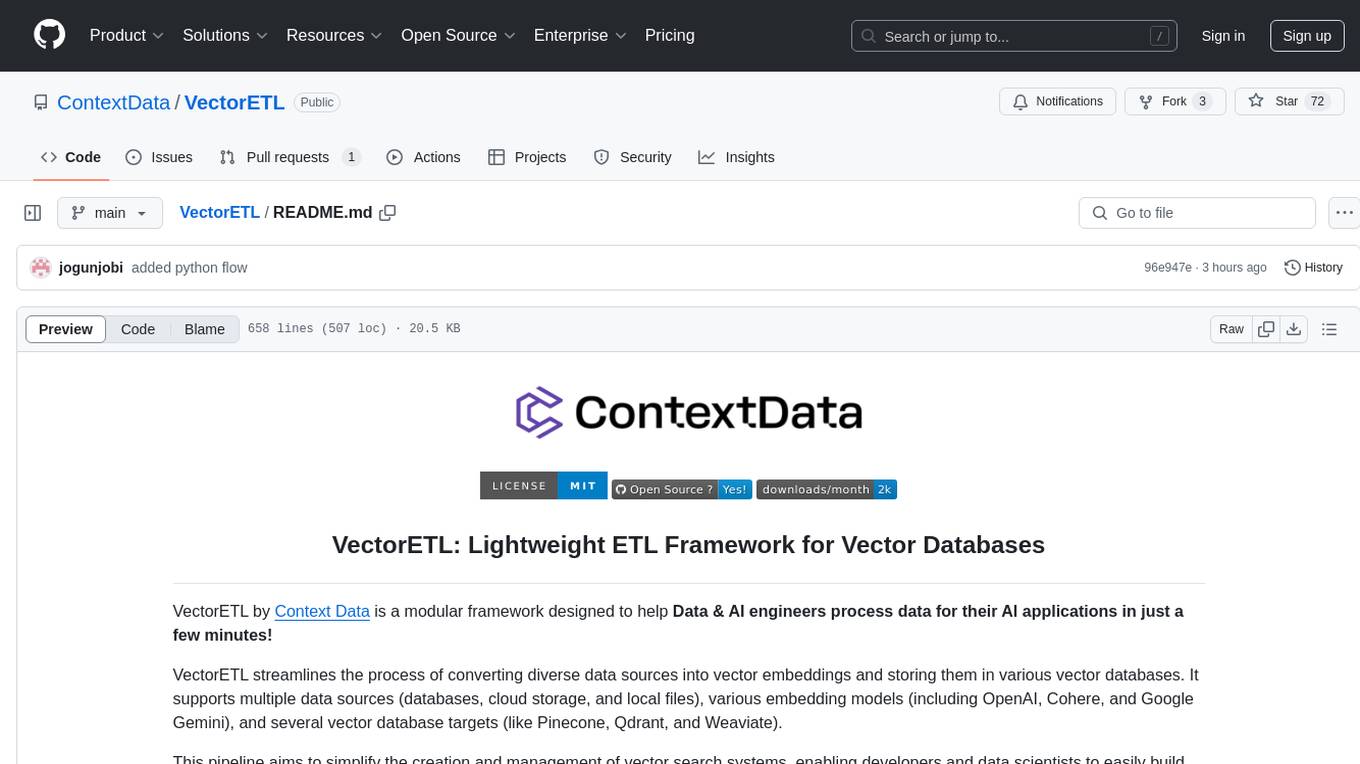
VectorETL is a lightweight ETL framework designed to assist Data & AI engineers in processing data for AI applications quickly. It streamlines the conversion of diverse data sources into vector embeddings and storage in various vector databases. The framework supports multiple data sources, embedding models, and vector database targets, simplifying the creation and management of vector search systems for semantic search, recommendation systems, and other vector-based operations.
README:
VectorETL: Lightweight ETL Framework for Vector Databases
VectorETL by Context Data is a modular framework designed to help Data & AI engineers process data for their AI applications in just a few minutes!
VectorETL streamlines the process of converting diverse data sources into vector embeddings and storing them in various vector databases. It supports multiple data sources (databases, cloud storage, and local files), various embedding models (including OpenAI, Cohere, and Google Gemini), and several vector database targets (like Pinecone, Qdrant, and Weaviate).
This pipeline aims to simplify the creation and management of vector search systems, enabling developers and data scientists to easily build and scale applications that require semantic search, recommendation systems, or other vector-based operations.
- Modular architecture with support for multiple data sources, embedding models, and vector databases
- Batch processing for efficient handling of large datasets
- Configurable chunking and overlapping for text data
- Easy integration of new data sources, embedding models, and vector databases
- Installation
- Usage
- Project Overview
-
Configuration
- Source Configuration
- Using Unstructured to process source files
- Embedding Configuration
- Target Configuration
- Contributing
- Examples
- Documentation
pip install --upgrade vector-etl
or
pip install git+https://github.com/ContextData/VectorETL.git
This section provides instructions on how to use the ETL framework for Vector Databases. We'll cover running, validating configurations, and provide some common usage examples.
from vector_etl import create_flow
source = {
"source_data_type": "database",
"db_type": "postgres",
"host": "localhost",
"port": "5432",
"database_name": "test",
"username": "user",
"password": "password",
"query": "select * from test",
"batch_size": 1000,
"chunk_size": 1000,
"chunk_overlap": 0,
}
embedding = {
"embedding_model": "OpenAI",
"api_key": ${OPENAI_API_KEY},
"model_name": "text-embedding-ada-002"
}
target = {
"target_database": "Pinecone",
"pinecone_api_key": ${PINECONE_API_KEY},
"index_name": "my-pinecone-index",
"dimension": 1536
}
embed_columns = ["customer_name", "customer_description", "purchase_history"]
flow = create_flow()
flow.set_source(source)
flow.set_embedding(embedding)
flow.set_target(target)
flow.set_embed_columns(embed_columns)
# Execute the flow
flow.execute()
Assuming you have a configuration file similar to the file below.
source:
source_data_type: "database"
db_type: "postgres"
host: "localhost"
database_name: "customer_data"
username: "user"
password: "password"
port: 5432
query: "SELECT * FROM customers WHERE updated_at > :last_updated_at"
batch_size: 1000
chunk_size: 1000
chunk_overlap: 0
embedding:
embedding_model: "OpenAI"
api_key: ${OPENAI_API_KEY}
model_name: "text-embedding-ada-002"
target:
target_database: "Pinecone"
pinecone_api_key: ${PINECONE_API_KEY}
index_name: "customer-embeddings"
dimension: 1536
metric: "cosine"
embed_columns:
- "customer_name"
- "customer_description"
- "purchase_history"
You can then import the configuration into your python project and automatically run it from there
from vector_etl import create_flow
flow = create_flow()
flow.load_yaml('/path/to/your/config.yaml')
flow.execute()
Using the same yaml configuration file from Option 2 above, you can run the process directly from your command line without having to import it into a python application.
To run the ETL framework, use the following command:
vector-etl -c /path/to/your/config.yaml
Here are some examples of how to use the ETL framework for different scenarios:
vector-etl -c config/postgres_to_pinecone.yaml
Where postgres_to_pinecone.yaml
might look like:
source:
source_data_type: "database"
db_type: "postgres"
host: "localhost"
database_name: "customer_data"
username: "user"
password: "password"
port: 5432
query: "SELECT * FROM customers WHERE updated_at > :last_updated_at"
batch_size: 1000
chunk_size: 1000
chunk_overlap: 0
embedding:
embedding_model: "OpenAI"
api_key: ${OPENAI_API_KEY}
model_name: "text-embedding-ada-002"
target:
target_database: "Pinecone"
pinecone_api_key: ${PINECONE_API_KEY}
index_name: "customer-embeddings"
dimension: 1536
metric: "cosine"
embed_columns:
- "customer_name"
- "customer_description"
- "purchase_history"
vector-etl -c config/s3_to_qdrant.yaml
Where s3_to_qdrant.yaml
might look like:
source:
source_data_type: "Amazon S3"
bucket_name: "my-data-bucket"
prefix: "customer_data/"
file_type: "csv"
aws_access_key_id: ${AWS_ACCESS_KEY_ID}
aws_secret_access_key: ${AWS_SECRET_ACCESS_KEY}
chunk_size: 1000
chunk_overlap: 200
embedding:
embedding_model: "Cohere"
api_key: ${COHERE_API_KEY}
model_name: "embed-english-v2.0"
target:
target_database: "Qdrant"
qdrant_url: "https://your-qdrant-cluster-url.qdrant.io"
qdrant_api_key: ${QDRANT_API_KEY}
collection_name: "customer_embeddings"
embed_columns: []
The VectorETL (Extract, Transform, Load) framework is a powerful and flexible tool designed to streamline the process of extracting data from various sources, transforming it into vector embeddings, and loading these embeddings into a range of vector databases.
It's built with modularity, scalability, and ease of use in mind, making it an ideal solution for organizations looking to leverage the power of vector search in their data infrastructure.
-
Versatile Data Extraction: The framework supports a wide array of data sources, including traditional databases, cloud storage solutions (like Amazon S3 and Google Cloud Storage), and popular SaaS platforms (such as Stripe and Zendesk). This versatility allows you to consolidate data from multiple sources into a unified vector database.
-
Advanced Text Processing: For textual data, the framework implements sophisticated chunking and overlapping techniques. This ensures that the semantic context of the text is preserved when creating vector embeddings, leading to more accurate search results.
-
State-of-the-Art Embedding Models: The system integrates with leading embedding models, including OpenAI, Cohere, Google Gemini, and Azure OpenAI. This allows you to choose the embedding model that best fits your specific use case and quality requirements.
-
Multiple Vector Database Support: Whether you're using Pinecone, Qdrant, Weaviate, SingleStore, Supabase, or LanceDB, this framework has you covered. It's designed to seamlessly interface with these popular vector databases, allowing you to choose the one that best suits your needs.
-
Configurable and Extensible: The entire framework is highly configurable through YAML or JSON configuration files. Moreover, its modular architecture makes it easy to extend with new data sources, embedding models, or vector databases as your needs evolve.
This ETL framework is ideal for organizations looking to implement or upgrade their vector search capabilities.
By automating the process of extracting data, creating vector embeddings, and storing them in a vector database, this framework significantly reduces the time and complexity involved in setting up a vector search system. It allows data scientists and engineers to focus on deriving insights and building applications, rather than worrying about the intricacies of data processing and vector storage.
The ETL framework uses a configuration file to specify the details of the source, embedding model, target database, and other parameters. You can use either YAML or JSON format for the configuration file.
The configuration file is divided into three main sections:
-
source
: Specifies the data source details -
embedding
: Defines the embedding model to be used -
target
: Outlines the target vector database -
embed_columns
: Defines the columns that need to be embedded (mainly for structured data sources)
from vector_etl import create_flow
source = {
"source_data_type": "database",
"db_type": "postgres",
"host": "localhost",
"port": "5432",
"database_name": "test",
"username": "user",
"password": "password",
"query": "select * from test",
"batch_size": 1000,
"chunk_size": 1000,
"chunk_overlap": 0,
}
embedding = {
"embedding_model": "OpenAI",
"api_key": ${OPENAI_API_KEY},
"model_name": "text-embedding-ada-002"
}
target = {
"target_database": "Pinecone",
"pinecone_api_key": ${PINECONE_API_KEY},
"index_name": "my-pinecone-index",
"dimension": 1536
}
embed_columns = ["customer_name", "customer_description", "purchase_history"]
source:
source_data_type: "database"
db_type: "postgres"
host: "localhost"
database_name: "mydb"
username: "user"
password: "password"
port: 5432
query: "SELECT * FROM mytable WHERE updated_at > :last_updated_at"
batch_size: 1000
chunk_size: 1000
chunk_overlap: 0
embedding:
embedding_model: "OpenAI"
api_key: "your-openai-api-key"
model_name: "text-embedding-ada-002"
target:
target_database: "Pinecone"
pinecone_api_key: "your-pinecone-api-key"
index_name: "my-index"
dimension: 1536
metric: "cosine"
cloud: "aws"
region: "us-west-2"
embed_columns:
- "column1"
- "column2"
- "column3"
{
"source": {
"source_data_type": "database",
"db_type": "postgres",
"host": "localhost",
"database_name": "mydb",
"username": "user",
"password": "password",
"port": 5432,
"query": "SELECT * FROM mytable WHERE updated_at > :last_updated_at",
"batch_size": 1000,
"chunk_size": 1000,
"chunk_overlap": 0
},
"embedding": {
"embedding_model": "OpenAI",
"api_key": "your-openai-api-key",
"model_name": "text-embedding-ada-002"
},
"target": {
"target_database": "Pinecone",
"pinecone_api_key": "your-pinecone-api-key",
"index_name": "my-index",
"dimension": 1536,
"metric": "cosine",
"cloud": "aws",
"region": "us-west-2"
},
"embed_columns": ["column1", "column2", "column3"]
}
The source
section varies based on the source_data_type
. Here are examples for different source types:
{
"source_data_type": "database",
"db_type": "postgres", # or "mysql", "snowflake", "salesforce"
"host": "localhost",
"database_name": "mydb",
"username": "user",
"password": "password",
"port": 5432,
"query": "SELECT * FROM mytable WHERE updated_at > :last_updated_at",
"batch_size": 1000,
"chunk_size": 1000,
"chunk_overlap": 0
}
source:
source_data_type: "database"
db_type: "postgres" # or "mysql", "snowflake", "salesforce"
host: "localhost"
database_name: "mydb"
username: "user"
password: "password"
port: 5432
query: "SELECT * FROM mytable WHERE updated_at > :last_updated_at"
batch_size: 1000
chunk_size: 1000
chunk_overlap: 0
{
"source_data_type": "Amazon S3",
"bucket_name": "my-bucket",
"key": "path/to/files/",
"file_type": ".csv",
"aws_access_key_id": "your-access-key",
"aws_secret_access_key": "your-secret-key"
}
source:
source_data_type: "Amazon S3"
bucket_name: "my-bucket"
key: "path/to/files/"
file_type: ".csv"
aws_access_key_id: "your-access-key"
aws_secret_access_key: "your-secret-key"
{
"source_data_type": "Google Cloud Storage",
"credentials_path": "/path/to/your/credentials.json",
"bucket_name": "myBucket",
"prefix": "prefix/",
"file_type": "csv",
"chunk_size": 1000,
"chunk_overlap": 0
}
source:
source_data_type: "Google Cloud Storage"
credentials_path: "/path/to/your/credentials.json"
bucket_name: "myBucket"
prefix: "prefix/"
file_type: "csv"
chunk_size: 1000
chunk_overlap: 0
Starting from version 0.1.6.3, users can now utilize the Unstructured's Serverless API to efficiently extract data from a multitude of file based sources.
NOTE: This is limited to the Unstructured Severless API and should not be used for the Unstructured's open source framework
This is limited to [PDF, DOCX, DOC, TXT] files
In order to use Unstructured, you will need three additional parameters
-
use_unstructured
: (True/False) indicator telling the framework to use the Unstructured API -
unstructured_api_key
: Enter your Unstructured API Key -
unstructured_url
: Enter your API Url from your Unstructured dashboard
# Example using Local file
source:
source_data_type: "Local File"
file_path: "/path/to/file.docx"
file_type: "docx"
use_unstructured: True
unstructured_api_key: 'my-unstructured-key'
unstructured_url: 'https://my-domain.api.unstructuredapp.io'
# Example using Amazon S3
source:
source_data_type: "Amazon S3"
bucket_name: "myBucket"
prefix: "Dir/Subdir/"
file_type: "pdf"
aws_access_key_id: "your-access-key"
aws_secret_access_key: "your-secret-access-key"
use_unstructured: True
unstructured_api_key: 'my-unstructured-key'
unstructured_url: 'https://my-domain.api.unstructuredapp.io'
The embedding
section specifies which embedding model to use:
embedding:
embedding_model: "OpenAI" # or "Cohere", "Google Gemini", "Azure OpenAI", "Hugging Face"
api_key: "your-api-key"
model_name: "text-embedding-ada-002" # model name varies by provider
The target
section varies based on the chosen vector database. Here's an example for Pinecone:
target:
target_database: "Pinecone"
pinecone_api_key: "your-pinecone-api-key"
index_name: "my-index"
dimension: 1536
metric: "cosine"
cloud: "aws"
region: "us-west-2"
The embed_columns
list specifies which columns from the source data should be used to generate the embeddings (only applies to database sources for now):
embed_columns:
- "column1"
- "column2"
- "column3"
The embed_columns
list is only required for structured data sources (e.g. PostgreSQL, MySQL, Snowflake). For all other sources, use an empty list
embed_columns: []
To protect sensitive information like API keys and passwords, consider using environment variables or a secure secrets management system. You can then reference these in your configuration file:
embedding:
api_key: ${OPENAI_API_KEY}
This allows you to keep your configuration files in version control without exposing sensitive data.
Remember to adjust your configuration based on your specific data sources, embedding models, and target databases. Refer to the documentation for each service to ensure you're providing all required parameters.
We welcome contributions to the ETL Framework for Vector Databases! Whether you're fixing bugs, improving documentation, or proposing new features, your efforts are appreciated. Here's how you can contribute:
If you encounter a bug or have a suggestion for improving the ETL framework:
- Check the GitHub Issues to see if the issue or suggestion has already been reported.
- If not, open a new issue. Provide a clear title and description, and as much relevant information as possible, including:
- Steps to reproduce (for bugs)
- Expected behavior
- Actual behavior
- Your operating system and Python version
- Relevant parts of your configuration file (remember to remove sensitive information)
We're always looking for ways to make the ETL framework better. If you have ideas:
- Open a new issue on GitHub.
- Use a clear and descriptive title.
- Provide a detailed description of the suggested enhancement.
- Explain why this enhancement would be useful to most users.
We actively welcome your pull requests:
- Fork the repo and create your branch from
main
. - If you've added code that should be tested, add tests.
- If you've changed APIs, update the documentation.
- Ensure the test suite passes.
- Make sure your code follows the existing style conventions (see Coding Standards below).
- Issue that pull request!
To maintain consistency throughout the project, please adhere to these coding standards:
- Follow PEP 8 style guide for Python code.
- Use meaningful variable names and add comments where necessary.
- Write docstrings for all functions, classes, and modules.
- Keep functions small and focused on a single task.
- Use type hints to improve code readability and catch potential type-related errors.
Improving documentation is always appreciated:
- If you find a typo or an error in the documentation, feel free to submit a pull request with the correction.
- For substantial changes to documentation, please open an issue first to discuss the proposed changes.
If you're thinking about adding a new feature:
- Open an issue to discuss the feature before starting development.
- For new data sources:
- Add a new file in the
source_mods
directory. - Implement the necessary methods as defined in the base class.
- Update the
get_source_class
function insource_mods/__init__.py
.
- Add a new file in the
- For new embedding models:
- Add a new file in the
embedding_mods
directory. - Implement the necessary methods as defined in the base class.
- Update the
get_embedding_model
function inembedding_mods/__init__.py
.
- Add a new file in the
- For new vector databases:
- Add a new file in the
target_mods
directory. - Implement the necessary methods as defined in the base class.
- Update the
get_target_database
function intarget_mods/__init__.py
.
- Add a new file in the
- Write unit tests for new features or bug fixes.
- Ensure all tests pass before submitting a pull request.
- Aim for high test coverage, especially for critical parts of the codebase.
- Use clear and meaningful commit messages.
- Start the commit message with a short summary (up to 50 characters).
- If necessary, provide more detailed explanations in subsequent lines.
- All submissions, including submissions by project members, require review.
- We use GitHub pull requests for this purpose.
- Reviewers may request changes before a pull request can be merged.
We encourage all users to join our Discord server to collaborate with the Context Data development team and other contributors in order to suggest upgrades, new integrations and issues.
For Tasks:
Click tags to check more tools for each tasksFor Jobs:
Alternative AI tools for VectorETL
Similar Open Source Tools
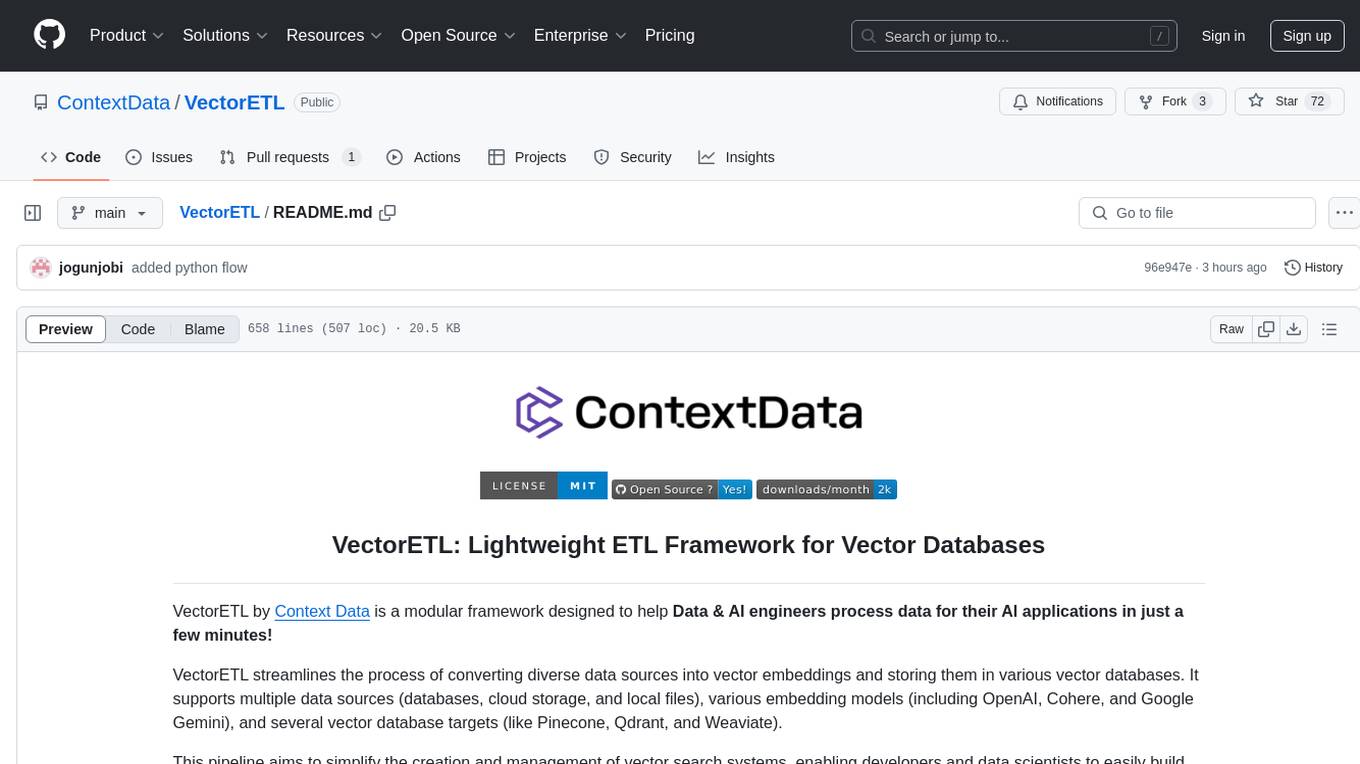
VectorETL
VectorETL is a lightweight ETL framework designed to assist Data & AI engineers in processing data for AI applications quickly. It streamlines the conversion of diverse data sources into vector embeddings and storage in various vector databases. The framework supports multiple data sources, embedding models, and vector database targets, simplifying the creation and management of vector search systems for semantic search, recommendation systems, and other vector-based operations.
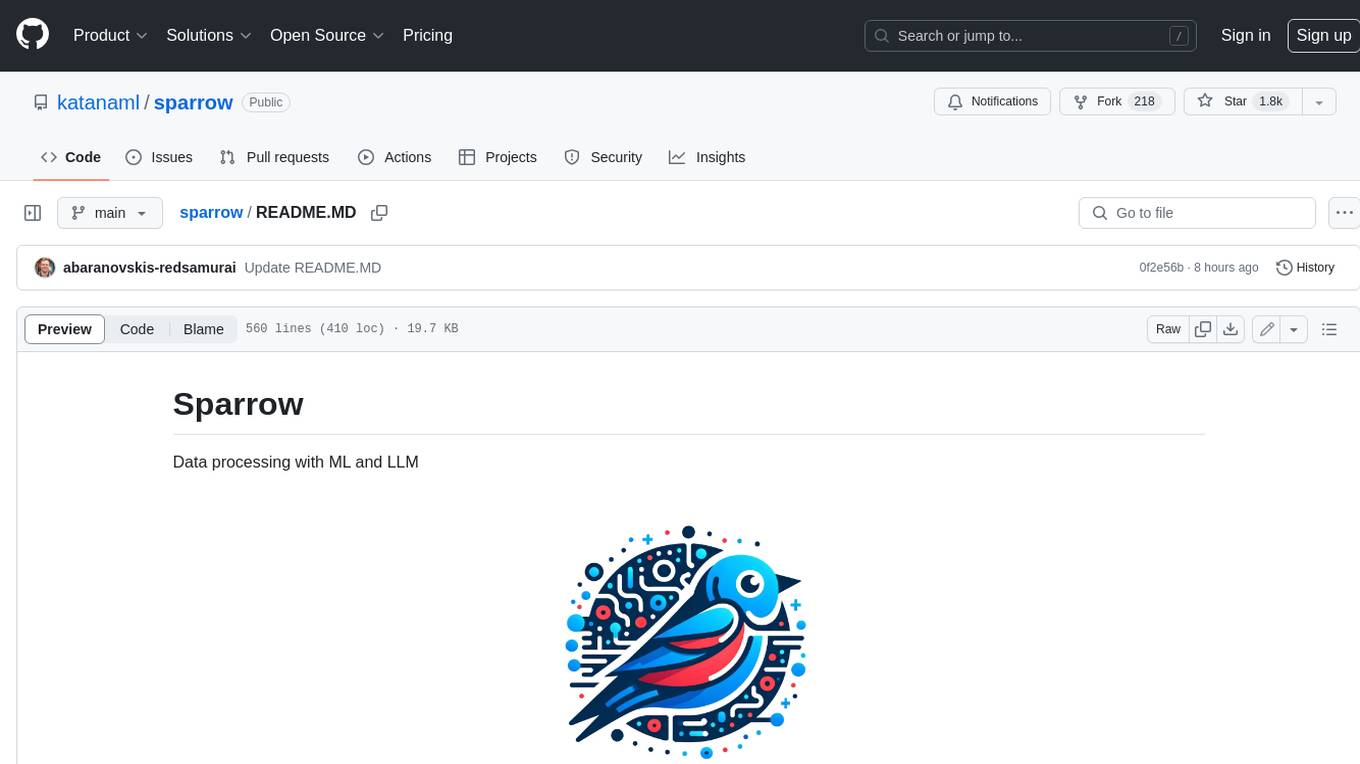
sparrow
Sparrow is an innovative open-source solution for efficient data extraction and processing from various documents and images. It seamlessly handles forms, invoices, receipts, and other unstructured data sources. Sparrow stands out with its modular architecture, offering independent services and pipelines all optimized for robust performance. One of the critical functionalities of Sparrow - pluggable architecture. You can easily integrate and run data extraction pipelines using tools and frameworks like LlamaIndex, Haystack, or Unstructured. Sparrow enables local LLM data extraction pipelines through Ollama or Apple MLX. With Sparrow solution you get API, which helps to process and transform your data into structured output, ready to be integrated with custom workflows. Sparrow Agents - with Sparrow you can build independent LLM agents, and use API to invoke them from your system. **List of available agents:** * **llamaindex** - RAG pipeline with LlamaIndex for PDF processing * **vllamaindex** - RAG pipeline with LLamaIndex multimodal for image processing * **vprocessor** - RAG pipeline with OCR and LlamaIndex for image processing * **haystack** - RAG pipeline with Haystack for PDF processing * **fcall** - Function call pipeline * **unstructured-light** - RAG pipeline with Unstructured and LangChain, supports PDF and image processing * **unstructured** - RAG pipeline with Weaviate vector DB query, Unstructured and LangChain, supports PDF and image processing * **instructor** - RAG pipeline with Unstructured and Instructor libraries, supports PDF and image processing. Works great for JSON response generation
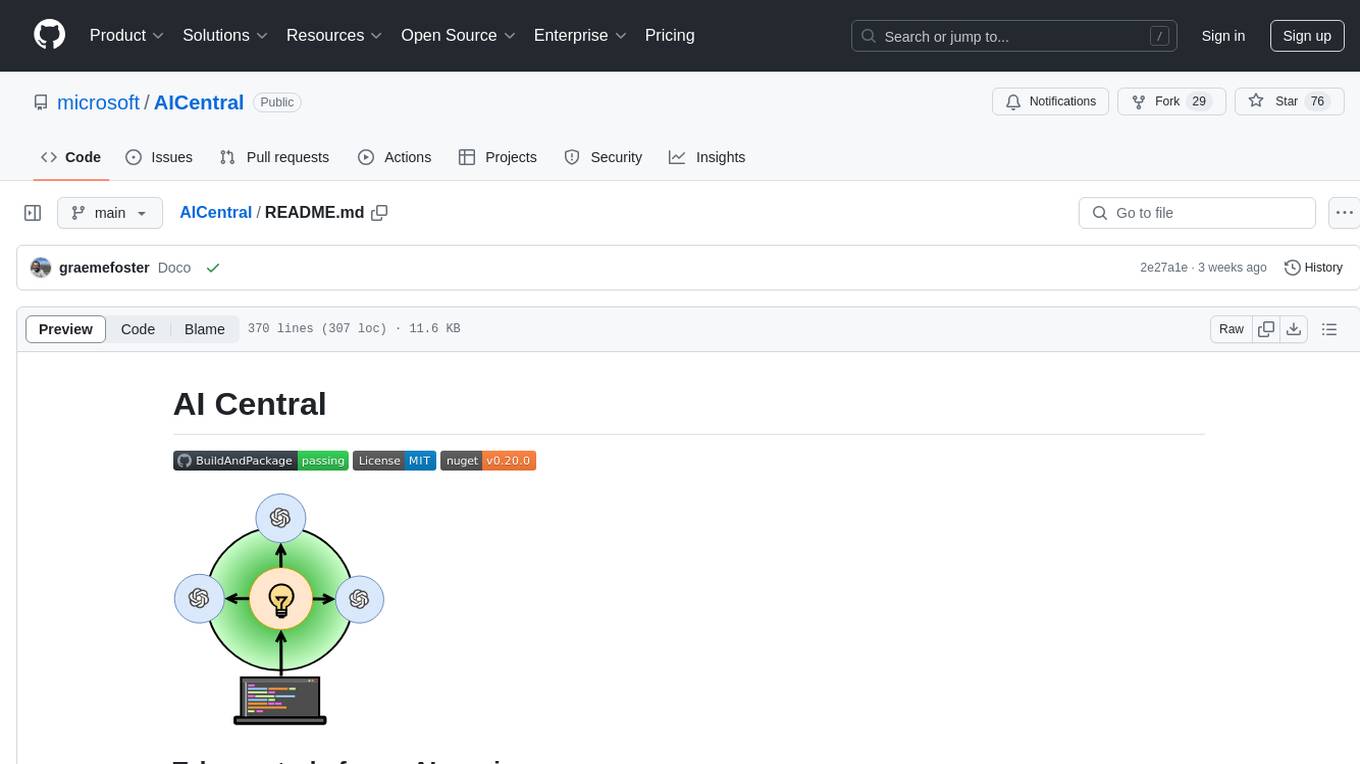
AICentral
AI Central is a powerful tool designed to take control of your AI services with minimal overhead. It is built on Asp.Net Core and dotnet 8, offering fast web-server performance. The tool enables advanced Azure APIm scenarios, PII stripping logging to Cosmos DB, token metrics through Open Telemetry, and intelligent routing features. AI Central supports various endpoint selection strategies, proxying asynchronous requests, custom OAuth2 authorization, circuit breakers, rate limiting, and extensibility through plugins. It provides an extensibility model for easy plugin development and offers enriched telemetry and logging capabilities for monitoring and insights.
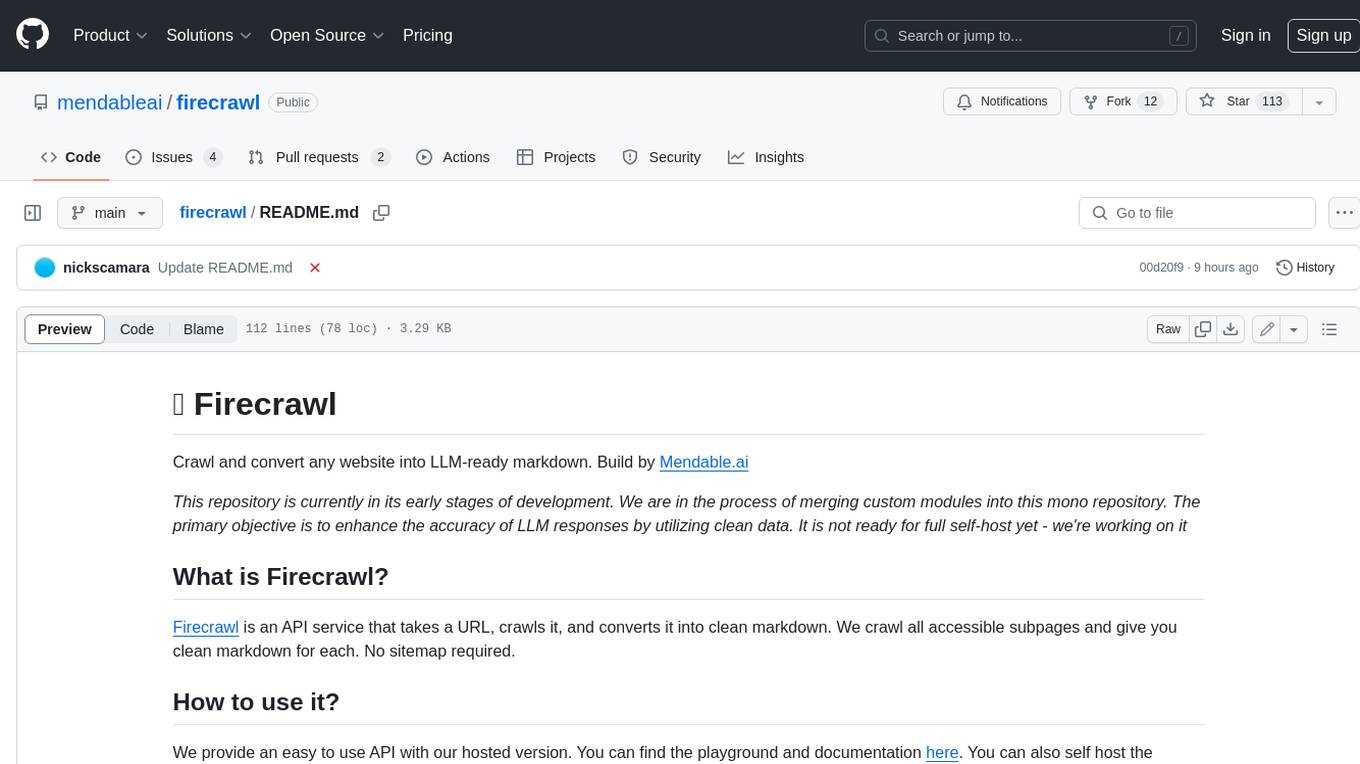
firecrawl
Firecrawl is an API service that takes a URL, crawls it, and converts it into clean markdown. It crawls all accessible subpages and provides clean markdown for each, without requiring a sitemap. The API is easy to use and can be self-hosted. It also integrates with Langchain and Llama Index. The Python SDK makes it easy to crawl and scrape websites in Python code.
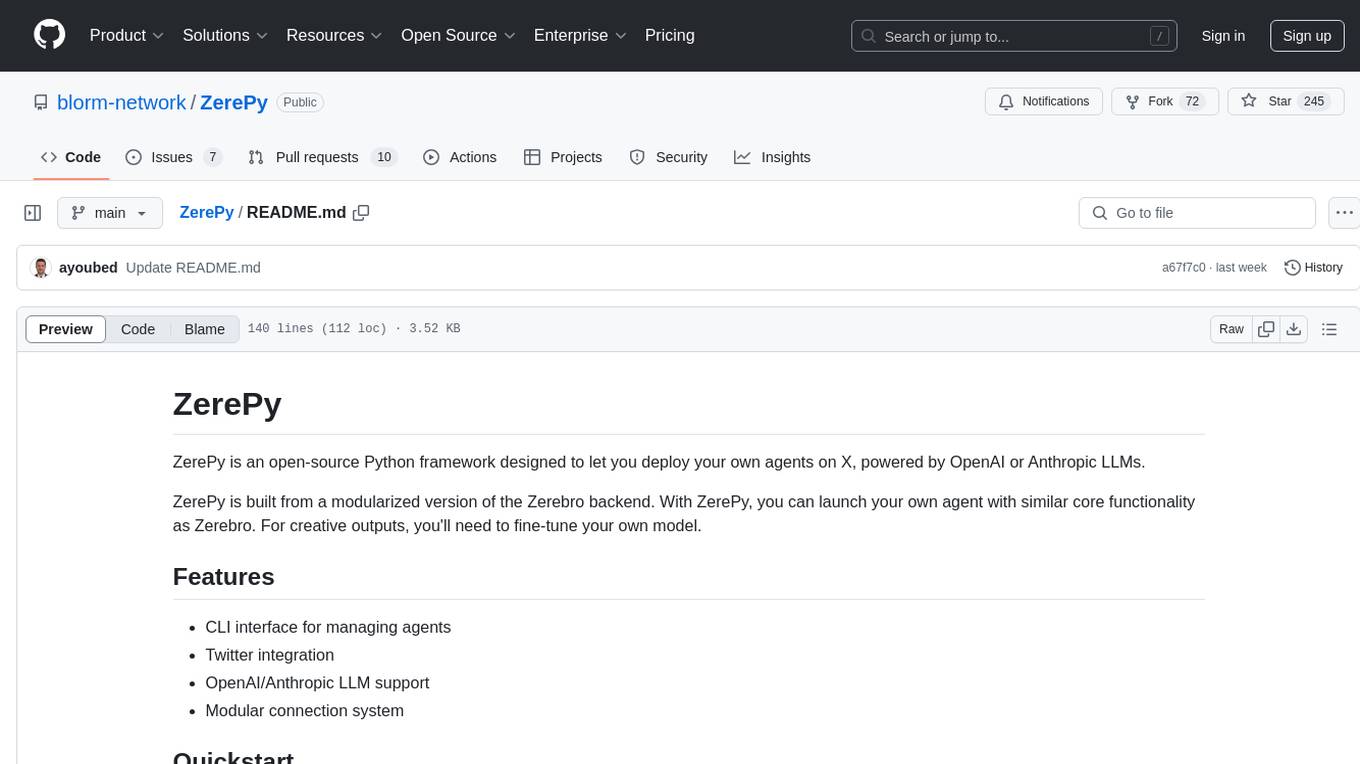
ZerePy
ZerePy is an open-source Python framework for deploying agents on X using OpenAI or Anthropic LLMs. It offers CLI interface, Twitter integration, and modular connection system. Users can fine-tune models for creative outputs and create agents with specific tasks. The tool requires Python 3.10+, Poetry 1.5+, and API keys for LLM, OpenAI, Anthropic, and X API.
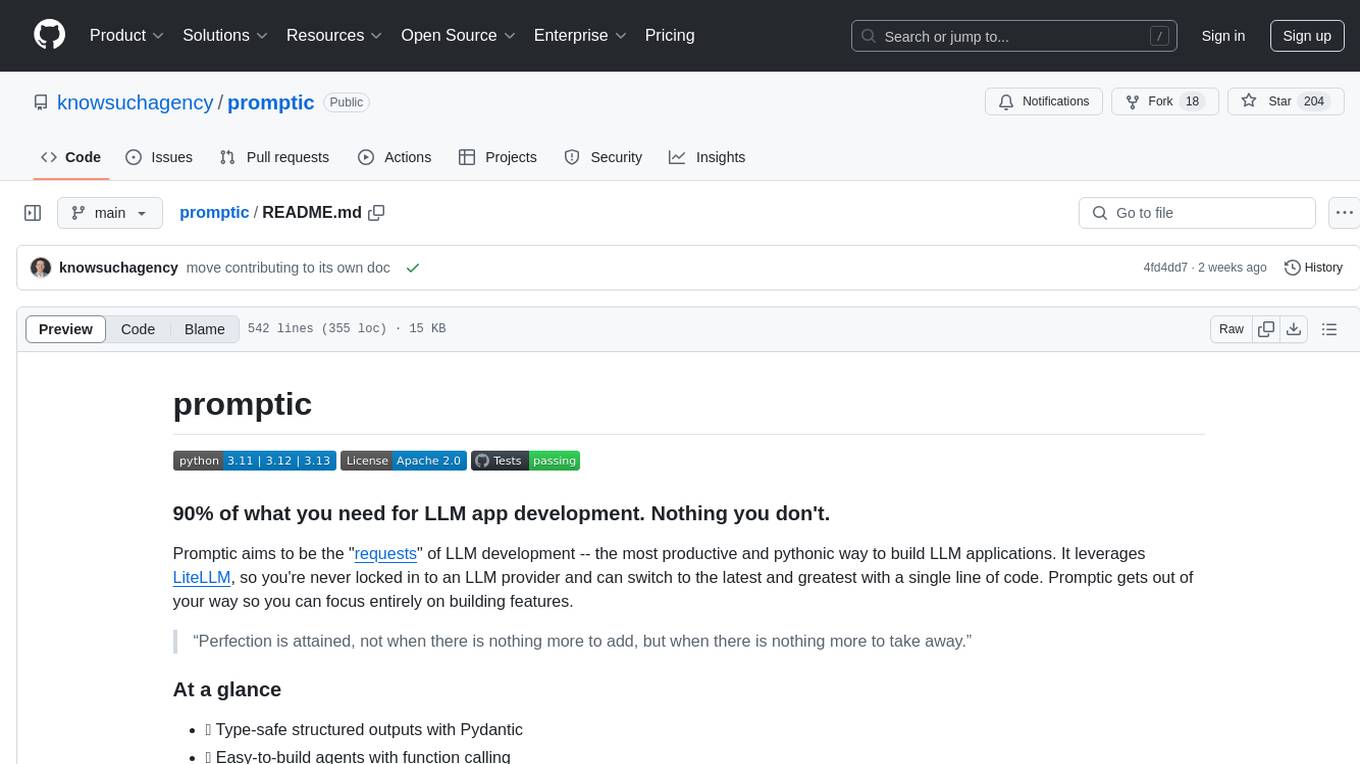
promptic
Promptic is a tool designed for LLM app development, providing a productive and pythonic way to build LLM applications. It leverages LiteLLM, allowing flexibility to switch LLM providers easily. Promptic focuses on building features by providing type-safe structured outputs, easy-to-build agents, streaming support, automatic prompt caching, and built-in conversation memory.
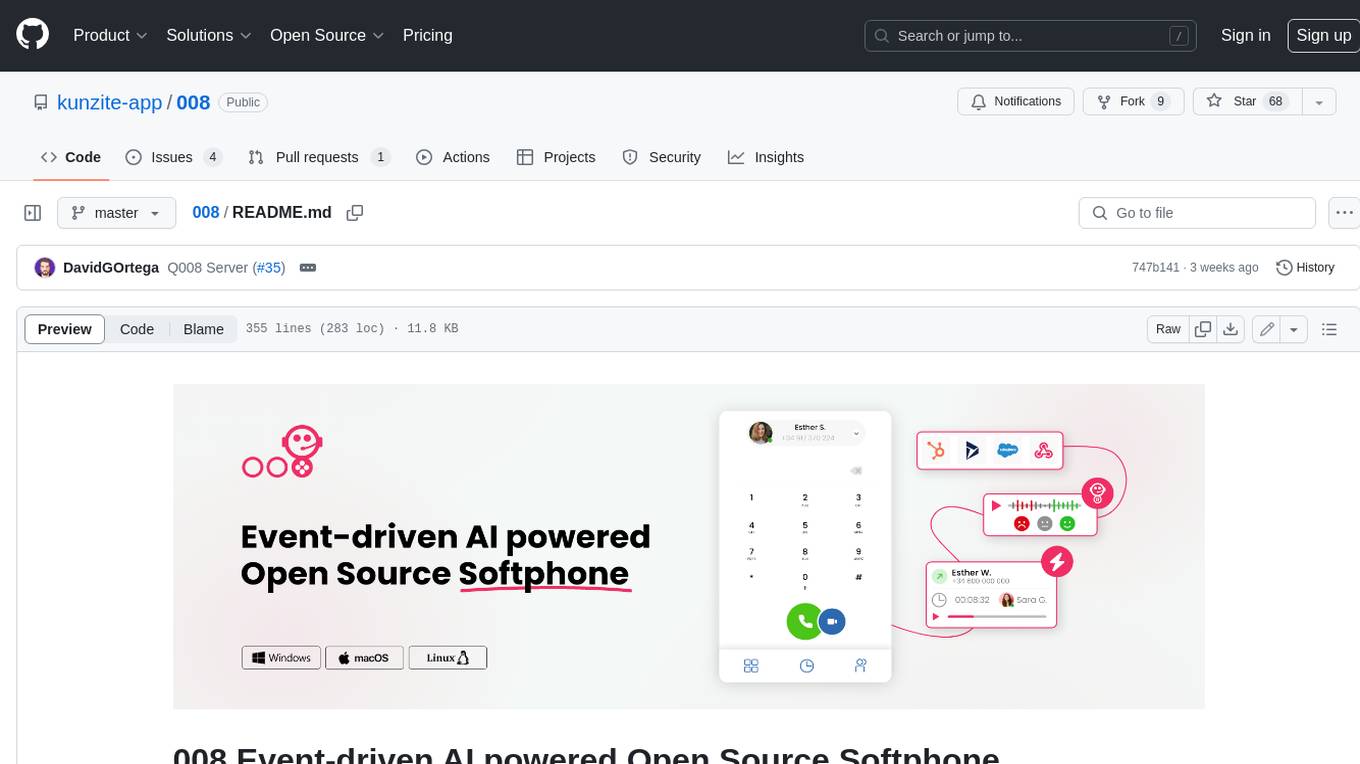
008
008 is an open-source event-driven AI powered WebRTC Softphone compatible with macOS, Windows, and Linux. It is also accessible on the web. The name '008' or 'agent 008' reflects our ambition: beyond crafting the premier Open Source Softphone, we aim to introduce a programmable, event-driven AI agent. This agent utilizes embedded artificial intelligence models operating directly on the softphone, ensuring efficiency and reduced operational costs.
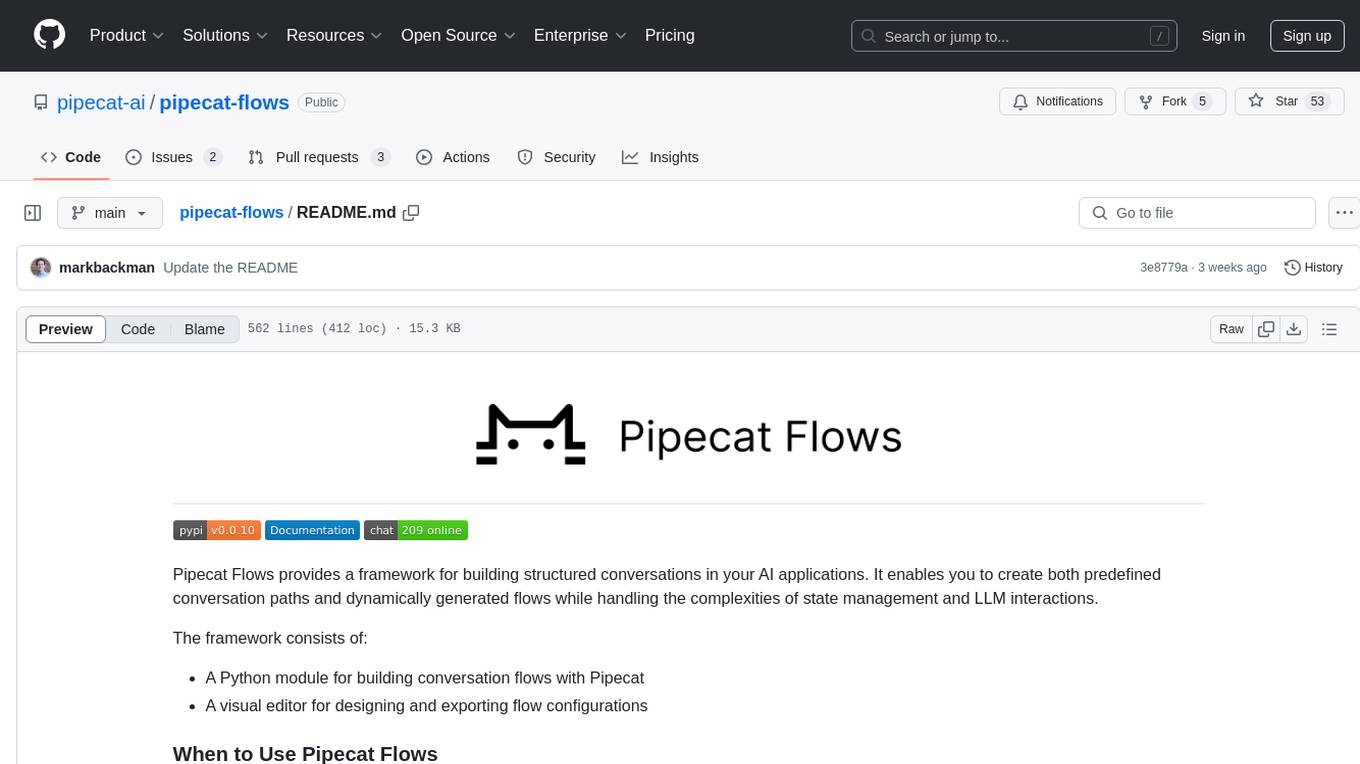
pipecat-flows
Pipecat Flows is a framework designed for building structured conversations in AI applications. It allows users to create both predefined conversation paths and dynamically generated flows, handling state management and LLM interactions. The framework includes a Python module for building conversation flows and a visual editor for designing and exporting flow configurations. Pipecat Flows is suitable for scenarios such as customer service scripts, intake forms, personalized experiences, and complex decision trees.
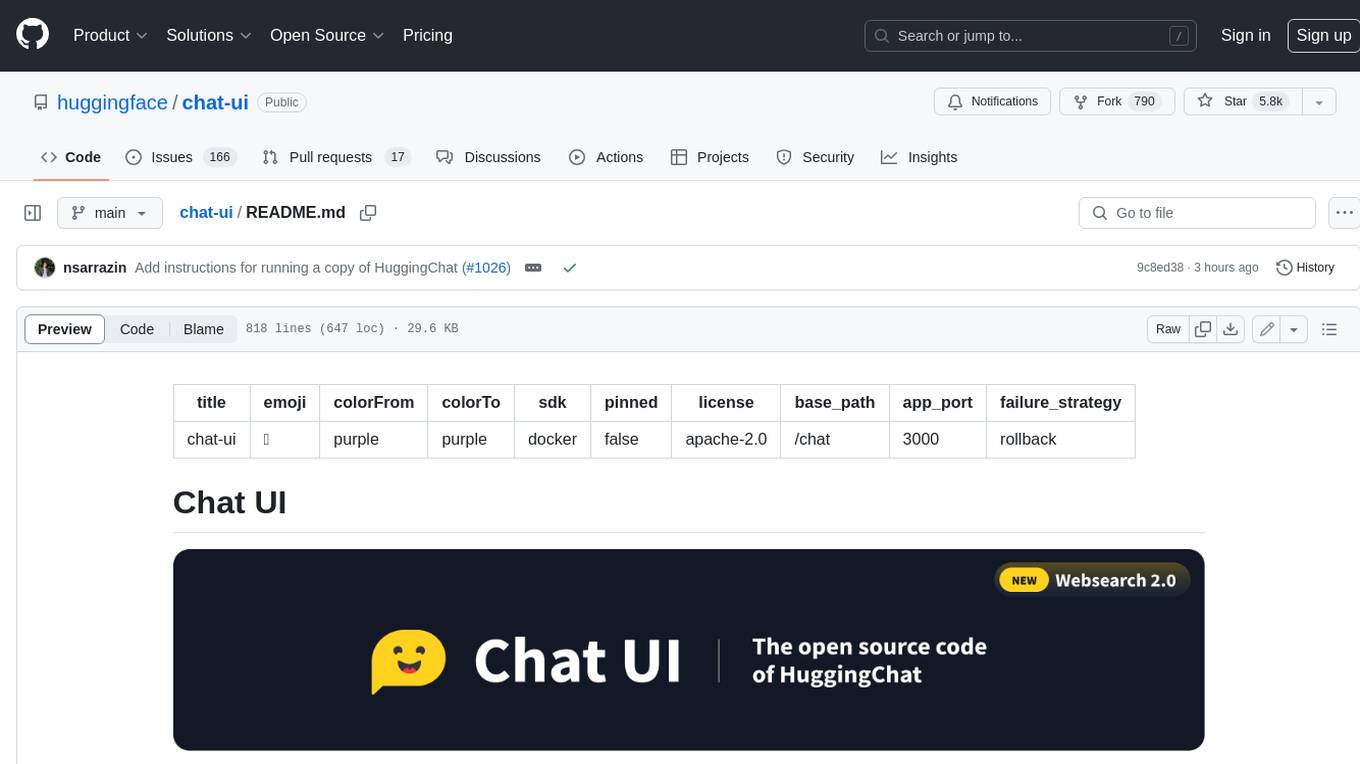
chat-ui
A chat interface using open source models, eg OpenAssistant or Llama. It is a SvelteKit app and it powers the HuggingChat app on hf.co/chat.
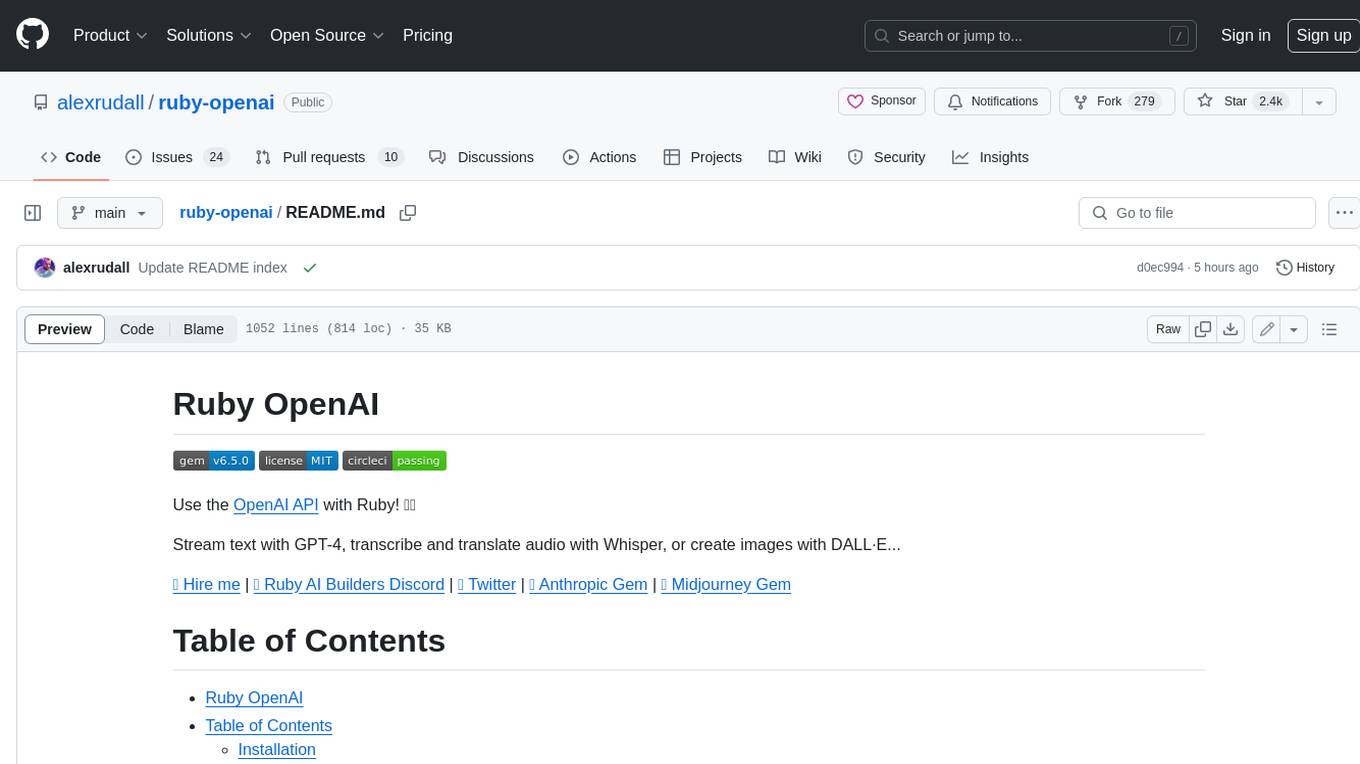
ruby-openai
Use the OpenAI API with Ruby! 🤖🩵 Stream text with GPT-4, transcribe and translate audio with Whisper, or create images with DALL·E... Hire me | 🎮 Ruby AI Builders Discord | 🐦 Twitter | 🧠 Anthropic Gem | 🚂 Midjourney Gem ## Table of Contents * Ruby OpenAI * Table of Contents * Installation * Bundler * Gem install * Usage * Quickstart * With Config * Custom timeout or base URI * Extra Headers per Client * Logging * Errors * Faraday middleware * Azure * Ollama * Counting Tokens * Models * Examples * Chat * Streaming Chat * Vision * JSON Mode * Functions * Edits * Embeddings * Batches * Files * Finetunes * Assistants * Threads and Messages * Runs * Runs involving function tools * Image Generation * DALL·E 2 * DALL·E 3 * Image Edit * Image Variations * Moderations * Whisper * Translate * Transcribe * Speech * Errors * Development * Release * Contributing * License * Code of Conduct
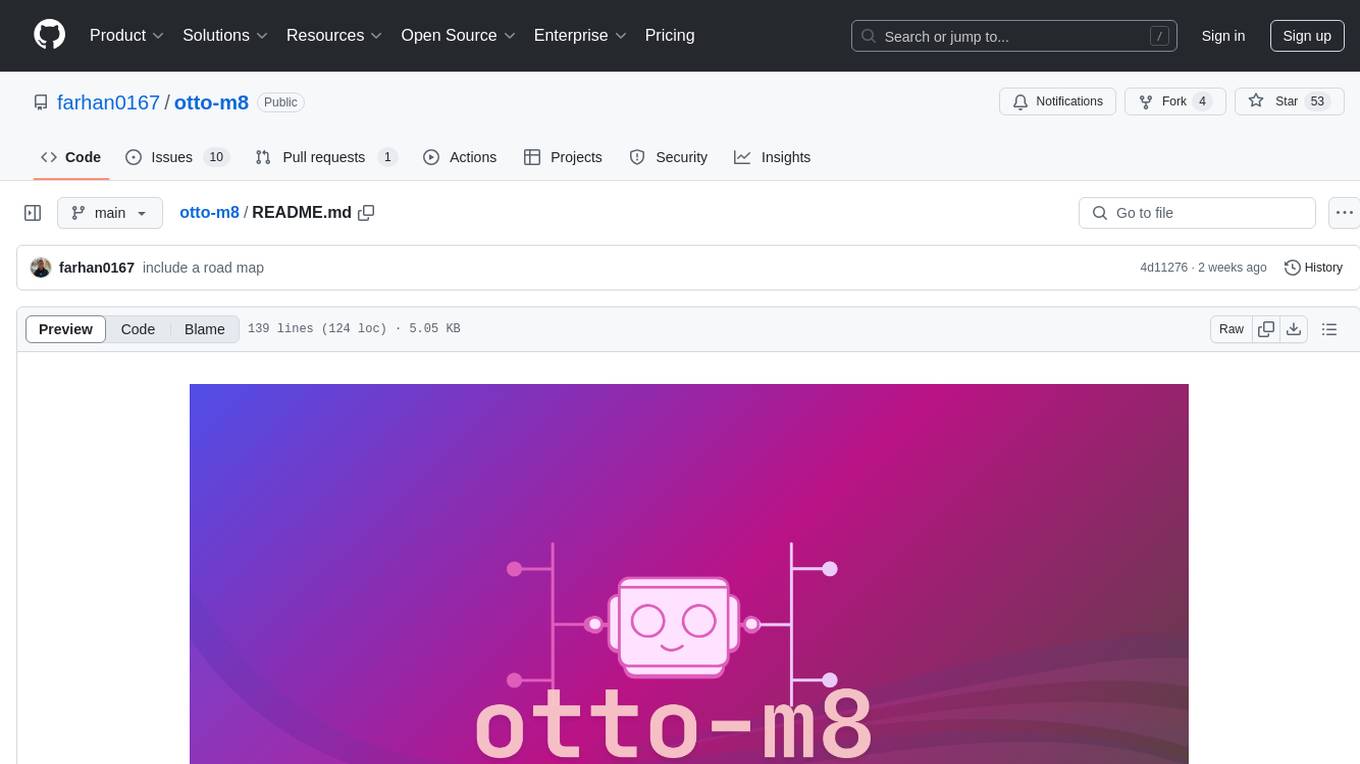
otto-m8
otto-m8 is a flowchart based automation platform designed to run deep learning workloads with minimal to no code. It provides a user-friendly interface to spin up a wide range of AI models, including traditional deep learning models and large language models. The tool deploys Docker containers of workflows as APIs for integration with existing workflows, building AI chatbots, or standalone applications. Otto-m8 operates on an Input, Process, Output paradigm, simplifying the process of running AI models into a flowchart-like UI.
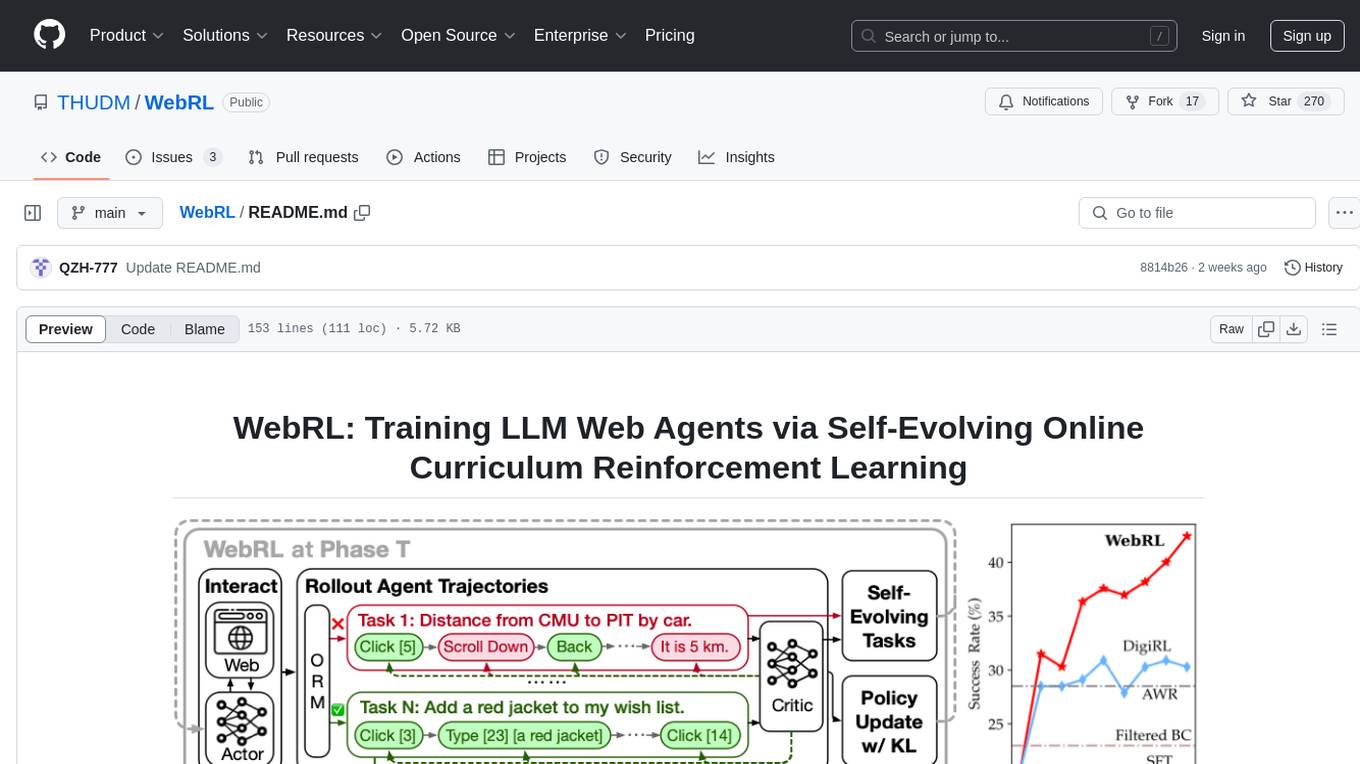
WebRL
WebRL is a self-evolving online curriculum learning framework designed for training web agents in the WebArena environment. It provides model checkpoints, training instructions, and evaluation processes for training the actor and critic models. The tool enables users to generate new instructions and interact with WebArena to configure tasks for training and evaluation.
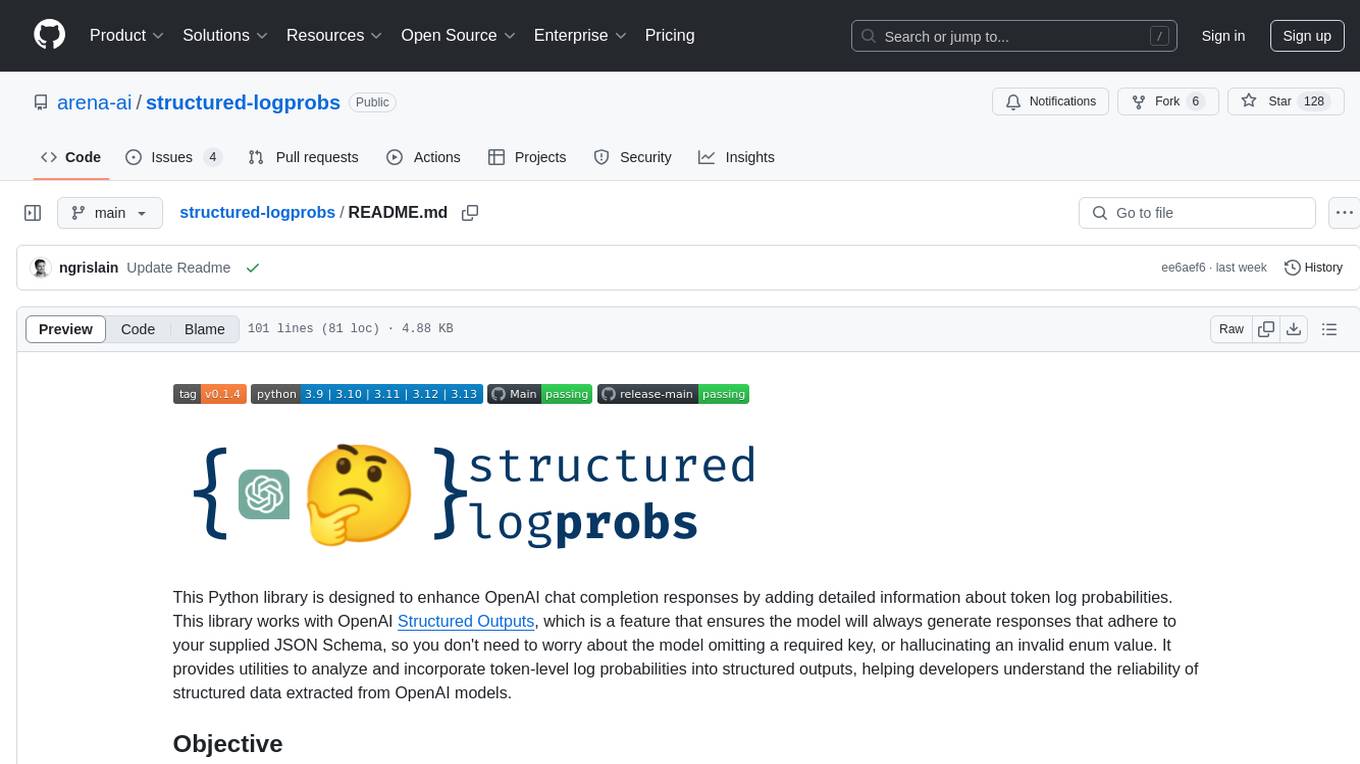
structured-logprobs
This Python library enhances OpenAI chat completion responses by providing detailed information about token log probabilities. It works with OpenAI Structured Outputs to ensure model-generated responses adhere to a JSON Schema. Developers can analyze and incorporate token-level log probabilities to understand the reliability of structured data extracted from OpenAI models.
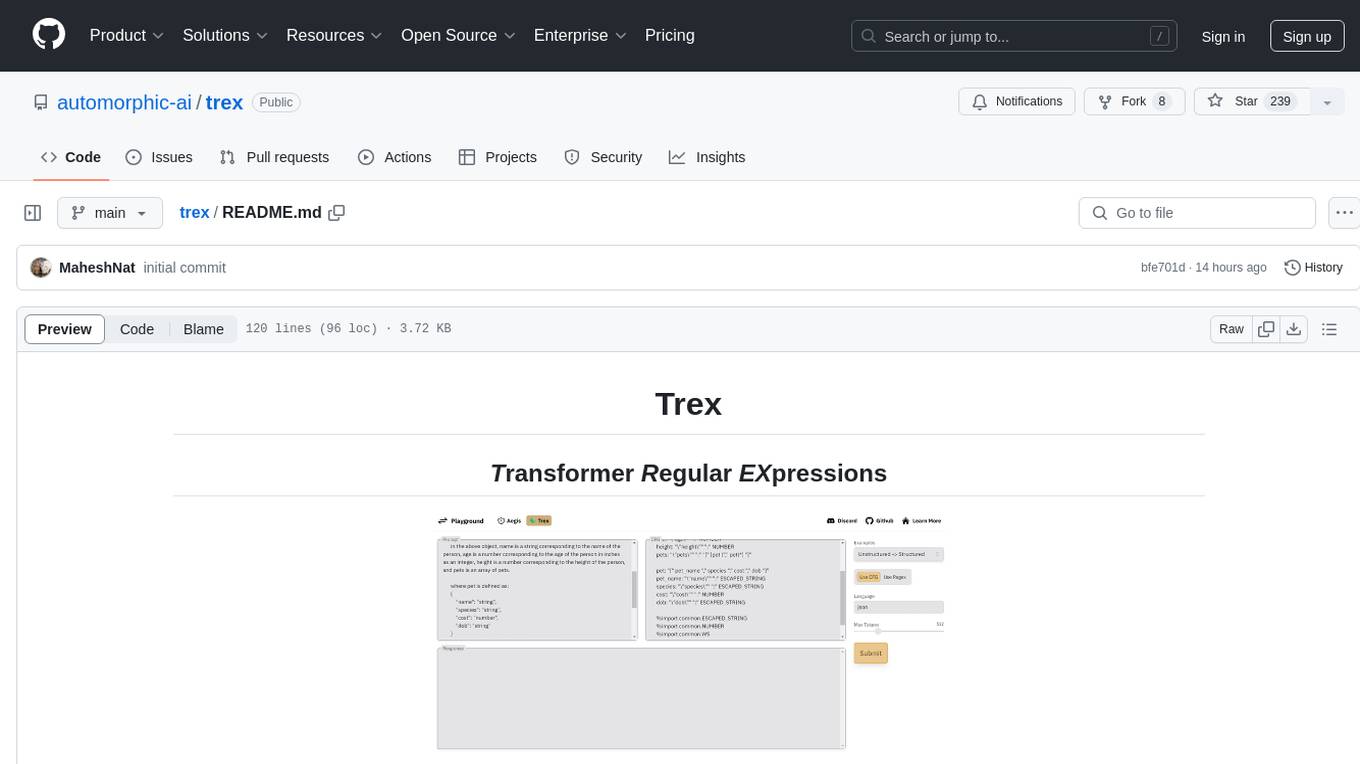
trex
Trex is a tool that transforms unstructured data into structured data by specifying a regex or context-free grammar. It intelligently restructures data to conform to the defined schema. It offers a Python client for installation and requires an API key obtained by signing up at automorphic.ai. The tool supports generating structured JSON objects based on user-defined schemas and prompts. Trex aims to provide significant speed improvements, structured custom CFG and regex generation, and generation from JSON schema. Future plans include auto-prompt generation for unstructured ETL and more intelligent models.
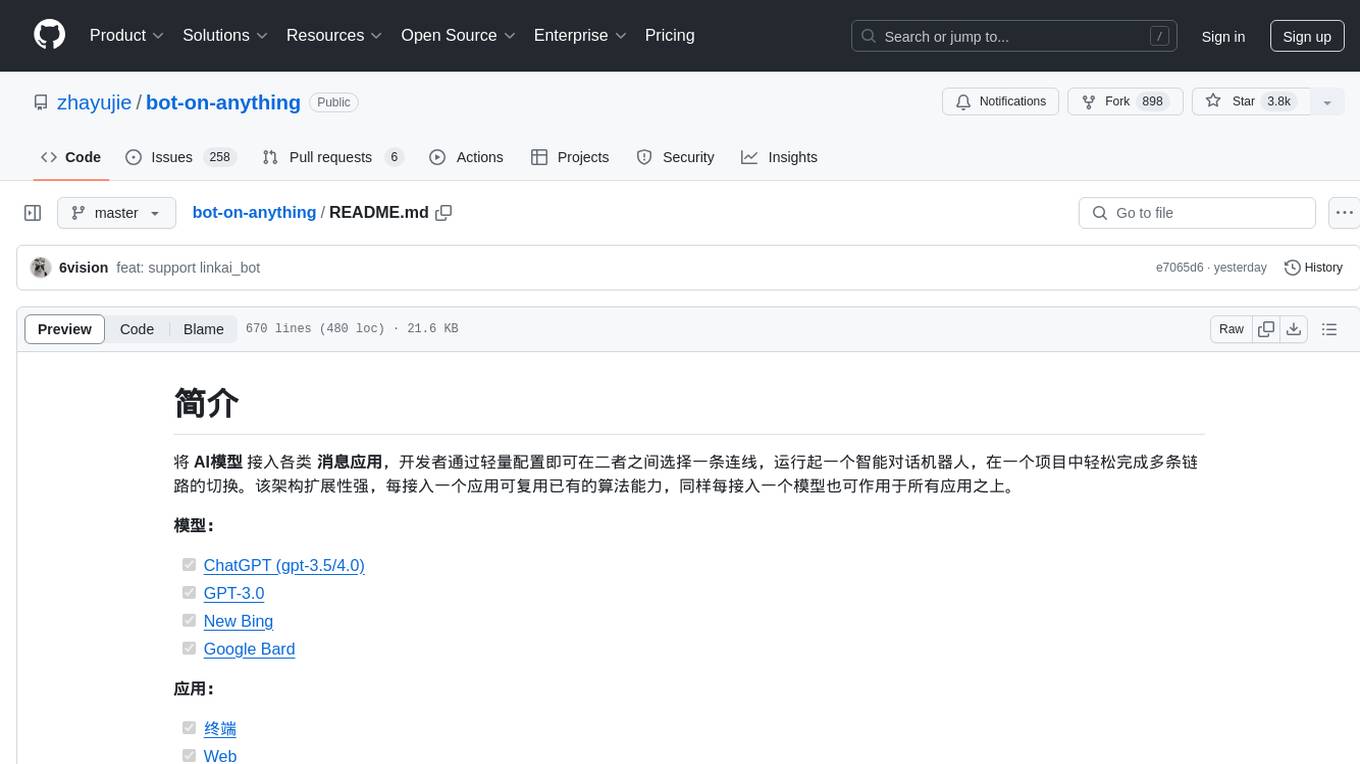
bot-on-anything
The 'bot-on-anything' repository allows developers to integrate various AI models into messaging applications, enabling the creation of intelligent chatbots. By configuring the connections between models and applications, developers can easily switch between multiple channels within a project. The architecture is highly scalable, allowing the reuse of algorithmic capabilities for each new application and model integration. Supported models include ChatGPT, GPT-3.0, New Bing, and Google Bard, while supported applications range from terminals and web platforms to messaging apps like WeChat, Telegram, QQ, and more. The repository provides detailed instructions for setting up the environment, configuring the models and channels, and running the chatbot for various tasks across different messaging platforms.
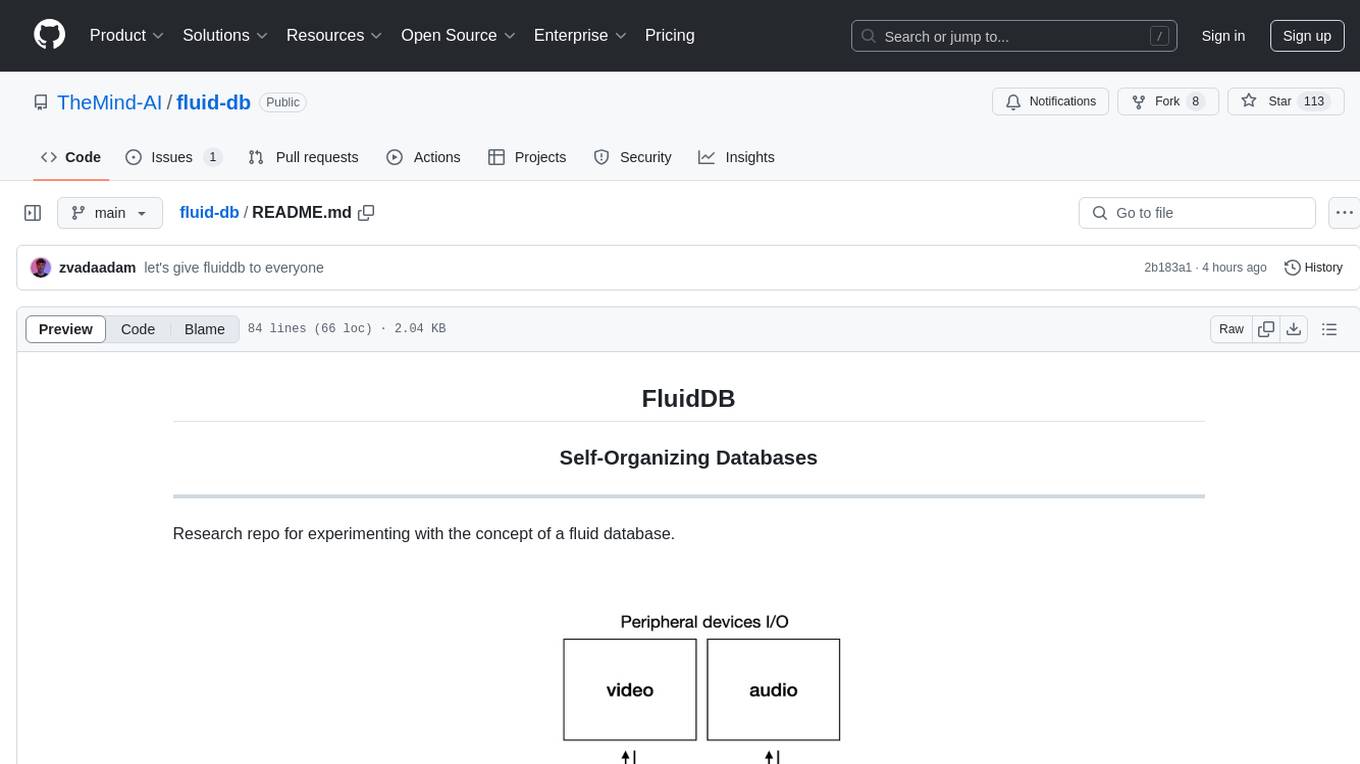
fluid-db
FluidDB is a research repository focusing on the concept of a fluid database that dynamically updates its schema based on ingested data. It enables the creation of personalized AI agents with features like adaptive schema, flexible querying, and versatile data input. The tool allows for storing unstructured data in a structured form and supports natural language queries. It aims to revolutionize database management by providing a dynamic and intuitive approach to data storage and retrieval.
For similar tasks
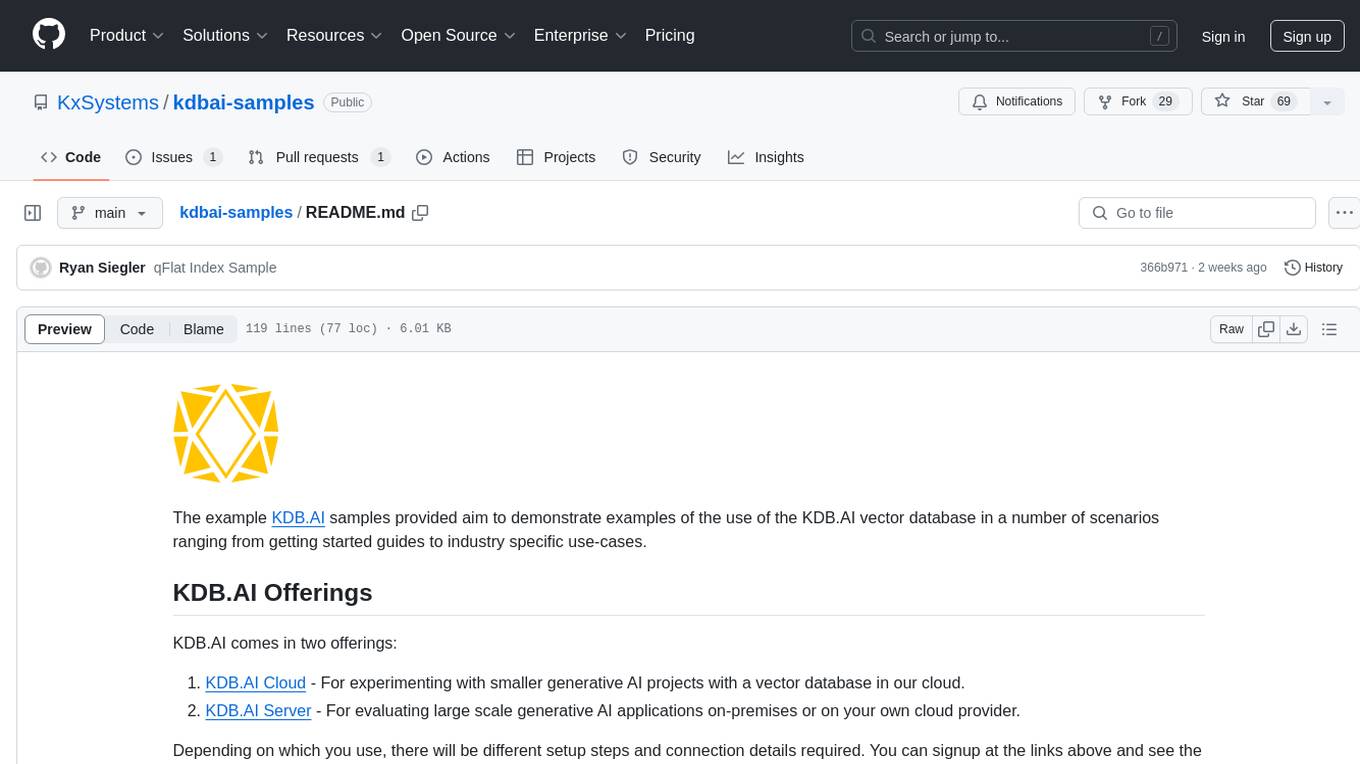
kdbai-samples
KDB.AI is a time-based vector database that allows developers to build scalable, reliable, and real-time applications by providing advanced search, recommendation, and personalization for Generative AI applications. It supports multiple index types, distance metrics, top-N and metadata filtered retrieval, as well as Python and REST interfaces. The repository contains samples demonstrating various use-cases such as temporal similarity search, document search, image search, recommendation systems, sentiment analysis, and more. KDB.AI integrates with platforms like ChatGPT, Langchain, and LlamaIndex. The setup steps require Unix terminal, Python 3.8+, and pip installed. Users can install necessary Python packages and run Jupyter notebooks to interact with the samples.
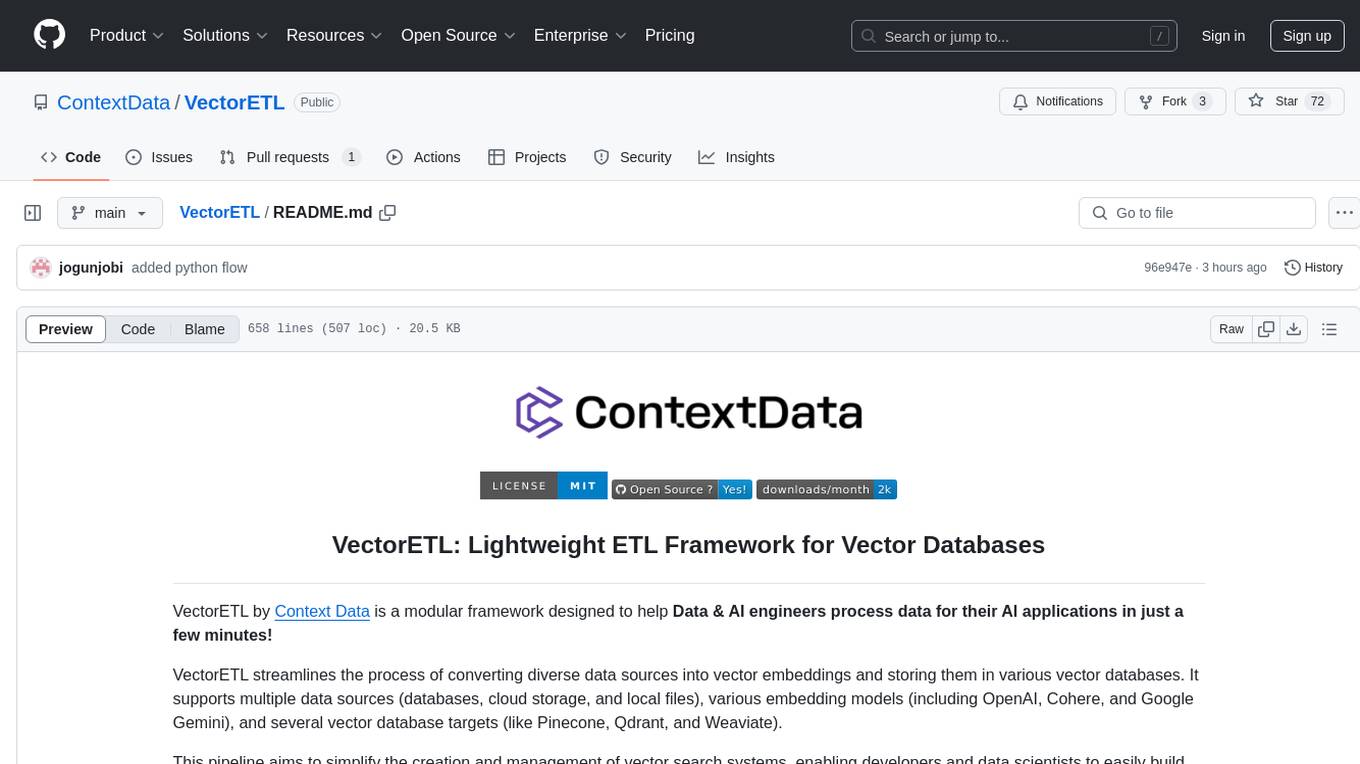
VectorETL
VectorETL is a lightweight ETL framework designed to assist Data & AI engineers in processing data for AI applications quickly. It streamlines the conversion of diverse data sources into vector embeddings and storage in various vector databases. The framework supports multiple data sources, embedding models, and vector database targets, simplifying the creation and management of vector search systems for semantic search, recommendation systems, and other vector-based operations.
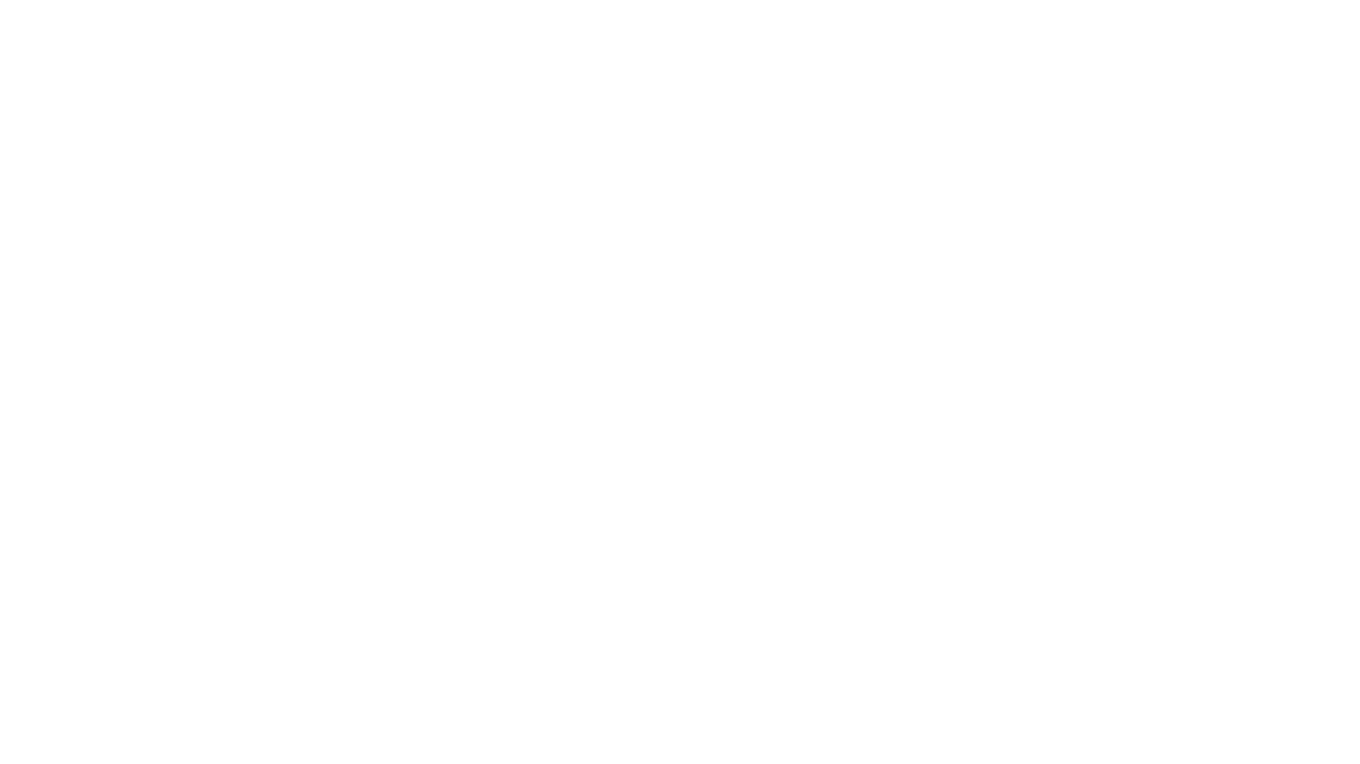
embedJs
EmbedJs is a NodeJS framework that simplifies RAG application development by efficiently processing unstructured data. It segments data, creates relevant embeddings, and stores them in a vector database for quick retrieval.
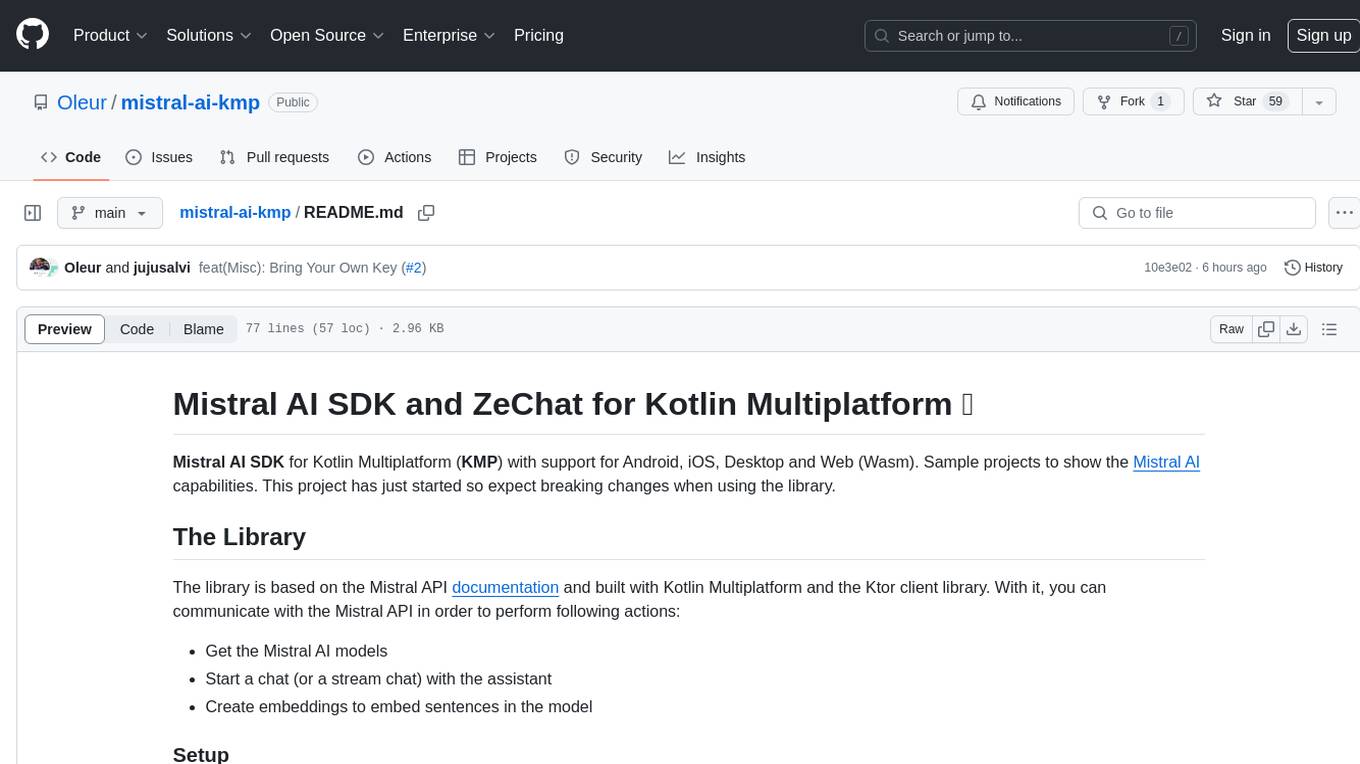
mistral-ai-kmp
Mistral AI SDK for Kotlin Multiplatform (KMP) allows communication with Mistral API to get AI models, start a chat with the assistant, and create embeddings. The library is based on Mistral API documentation and built with Kotlin Multiplatform and Ktor client library. Sample projects like ZeChat showcase the capabilities of Mistral AI SDK. Users can interact with different Mistral AI models through ZeChat apps on Android, Desktop, and Web platforms. The library is not yet published on Maven, but users can fork the project and use it as a module dependency in their apps.
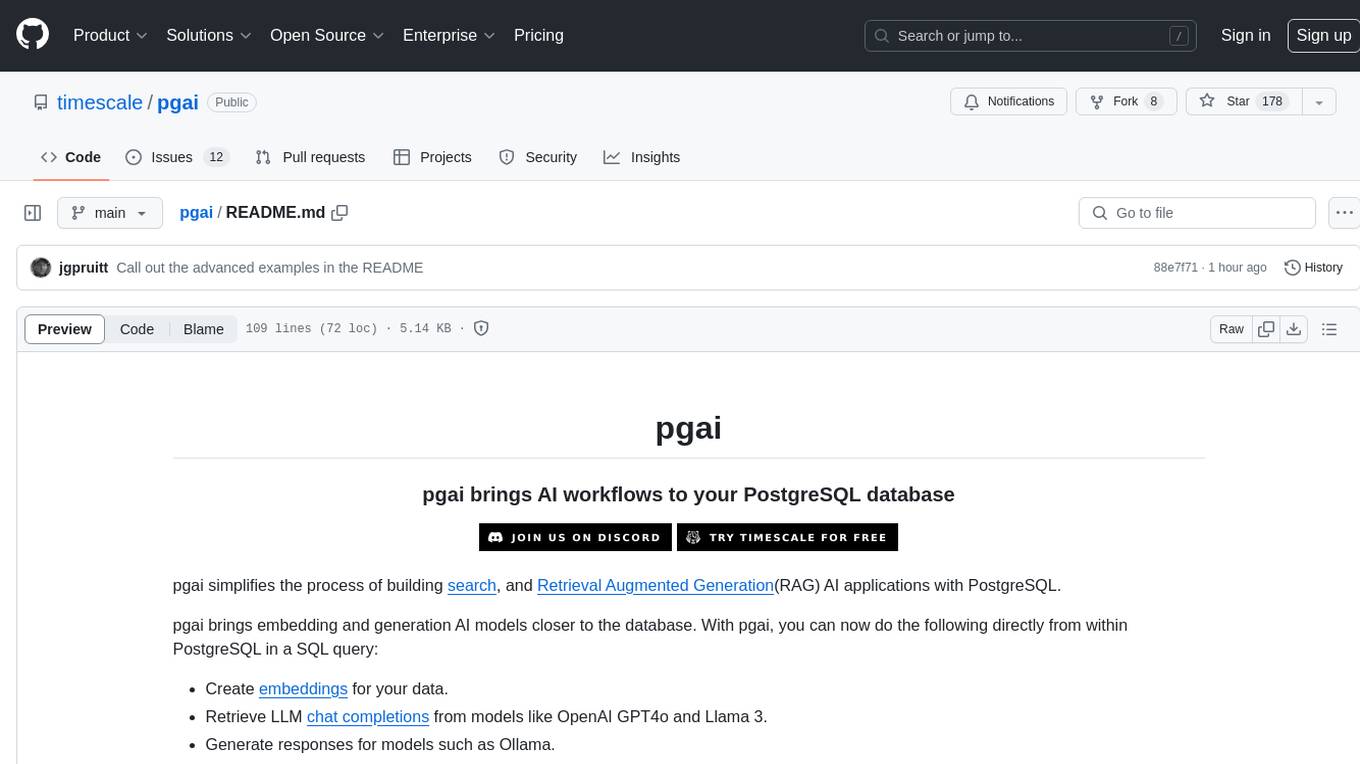
pgai
pgai simplifies the process of building search and Retrieval Augmented Generation (RAG) AI applications with PostgreSQL. It brings embedding and generation AI models closer to the database, allowing users to create embeddings, retrieve LLM chat completions, reason over data for classification, summarization, and data enrichment directly from within PostgreSQL in a SQL query. The tool requires an OpenAI API key and a PostgreSQL client to enable AI functionality in the database. Users can install pgai from source, run it in a pre-built Docker container, or enable it in a Timescale Cloud service. The tool provides functions to handle API keys using psql or Python, and offers various AI functionalities like tokenizing, detokenizing, embedding, chat completion, and content moderation.
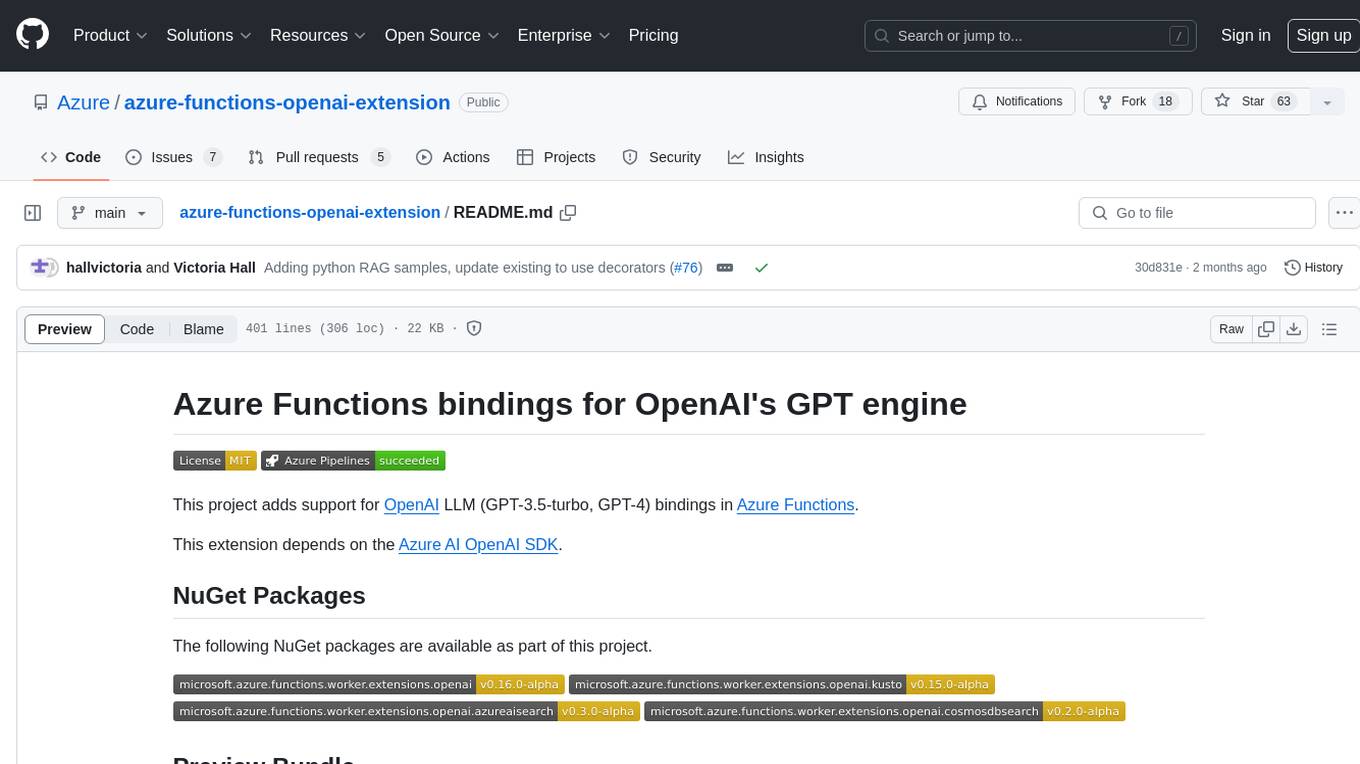
azure-functions-openai-extension
Azure Functions OpenAI Extension is a project that adds support for OpenAI LLM (GPT-3.5-turbo, GPT-4) bindings in Azure Functions. It provides NuGet packages for various functionalities like text completions, chat completions, assistants, embeddings generators, and semantic search. The project requires .NET 6 SDK or greater, Azure Functions Core Tools v4.x, and specific settings in Azure Function or local settings for development. It offers features like text completions, chat completion, assistants with custom skills, embeddings generators for text relatedness, and semantic search using vector databases. The project also includes examples in C# and Python for different functionalities.
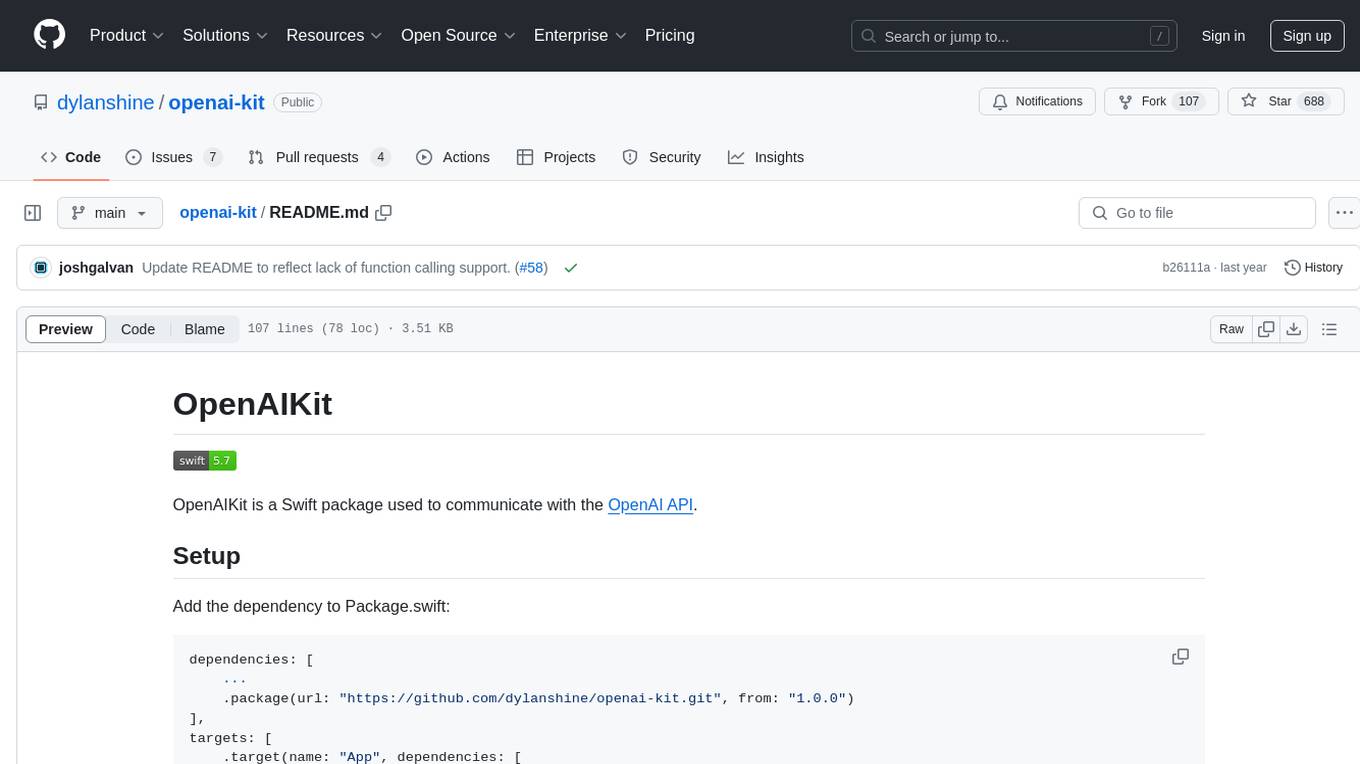
openai-kit
OpenAIKit is a Swift package designed to facilitate communication with the OpenAI API. It provides methods to interact with various OpenAI services such as chat, models, completions, edits, images, embeddings, files, moderations, and speech to text. The package encourages the use of environment variables to securely inject the OpenAI API key and organization details. It also offers error handling for API requests through the `OpenAIKit.APIErrorResponse`.
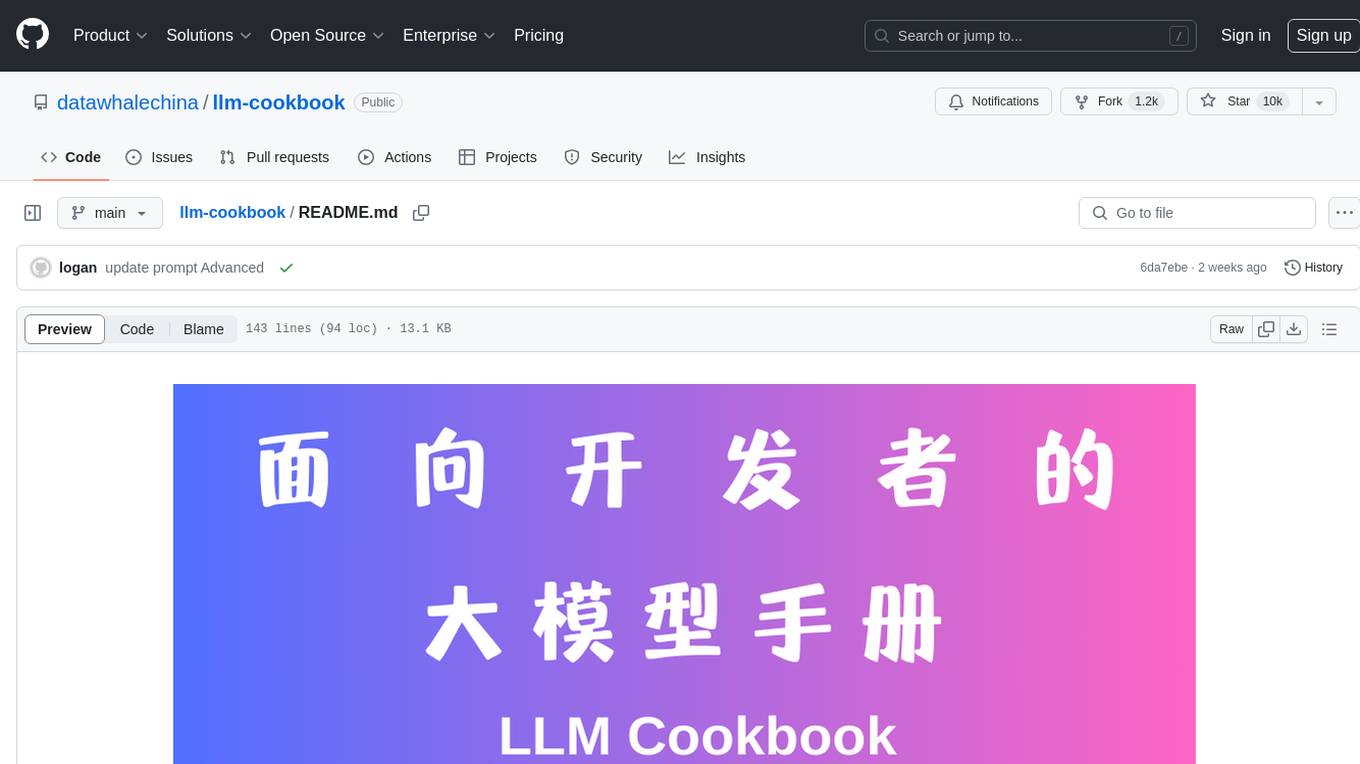
llm-cookbook
LLM Cookbook is a developer-oriented comprehensive guide focusing on LLM for Chinese developers. It covers various aspects from Prompt Engineering to RAG development and model fine-tuning, providing guidance on how to learn and get started with LLM projects in a way suitable for Chinese learners. The project translates and reproduces 11 courses from Professor Andrew Ng's large model series, categorizing them for beginners to systematically learn essential skills and concepts before exploring specific interests. It encourages developers to contribute by replicating unreproduced courses following the format and submitting PRs for review and merging. The project aims to help developers grasp a wide range of skills and concepts related to LLM development, offering both online reading and PDF versions for easy access and learning.
For similar jobs
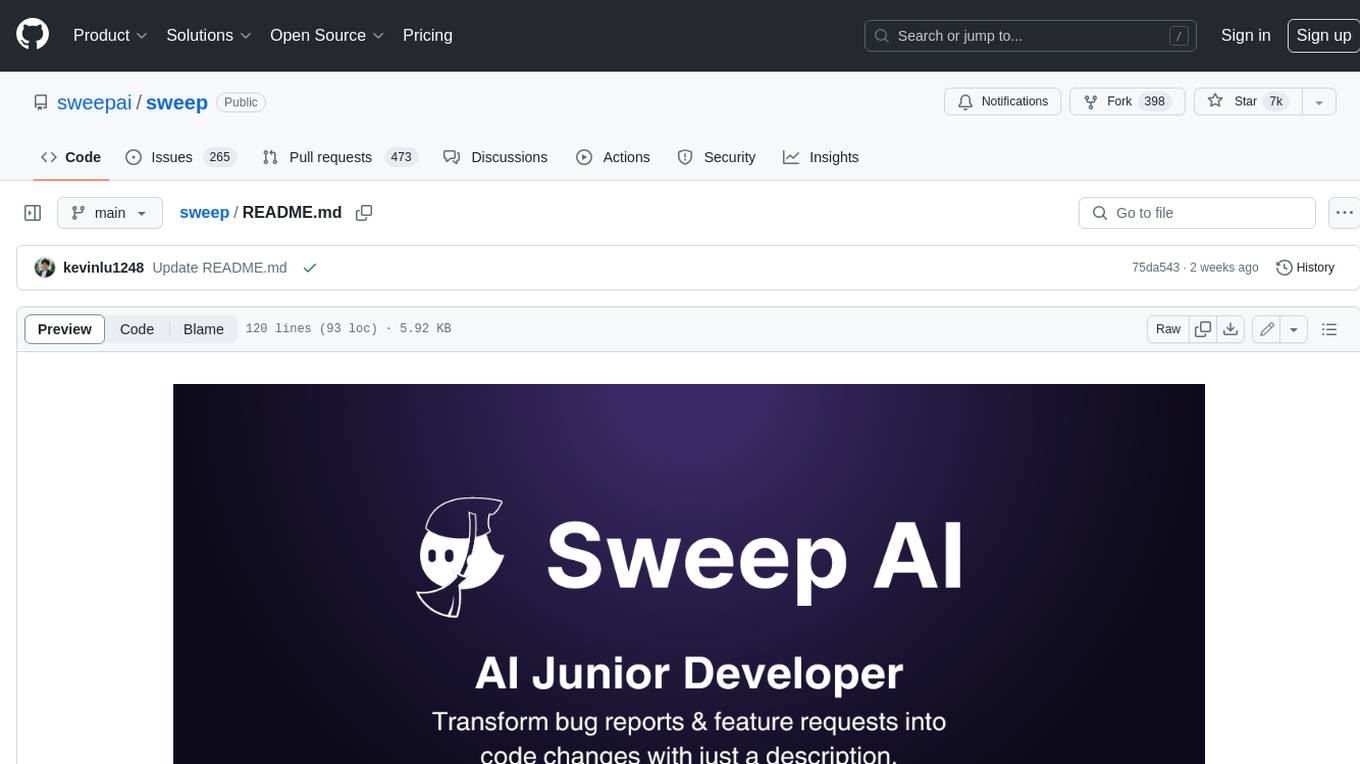
sweep
Sweep is an AI junior developer that turns bugs and feature requests into code changes. It automatically handles developer experience improvements like adding type hints and improving test coverage.
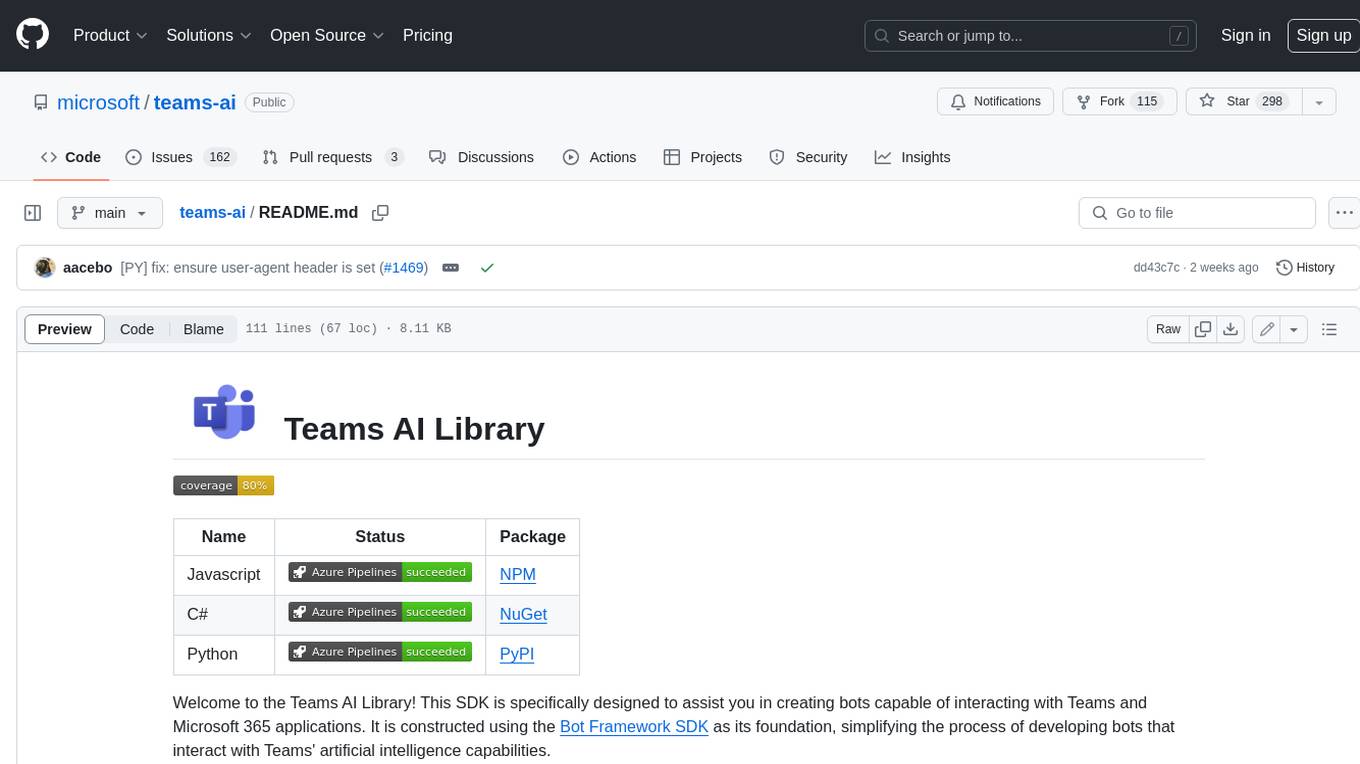
teams-ai
The Teams AI Library is a software development kit (SDK) that helps developers create bots that can interact with Teams and Microsoft 365 applications. It is built on top of the Bot Framework SDK and simplifies the process of developing bots that interact with Teams' artificial intelligence capabilities. The SDK is available for JavaScript/TypeScript, .NET, and Python.
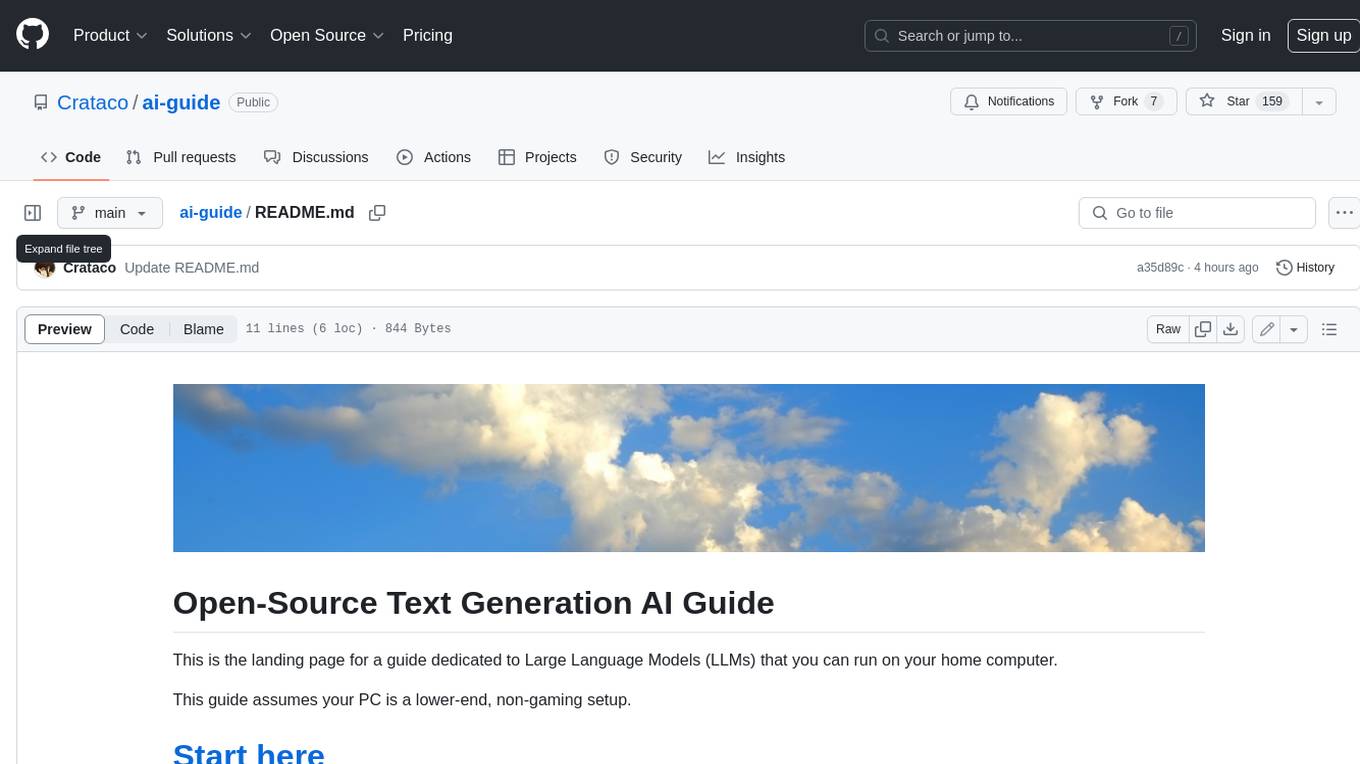
ai-guide
This guide is dedicated to Large Language Models (LLMs) that you can run on your home computer. It assumes your PC is a lower-end, non-gaming setup.
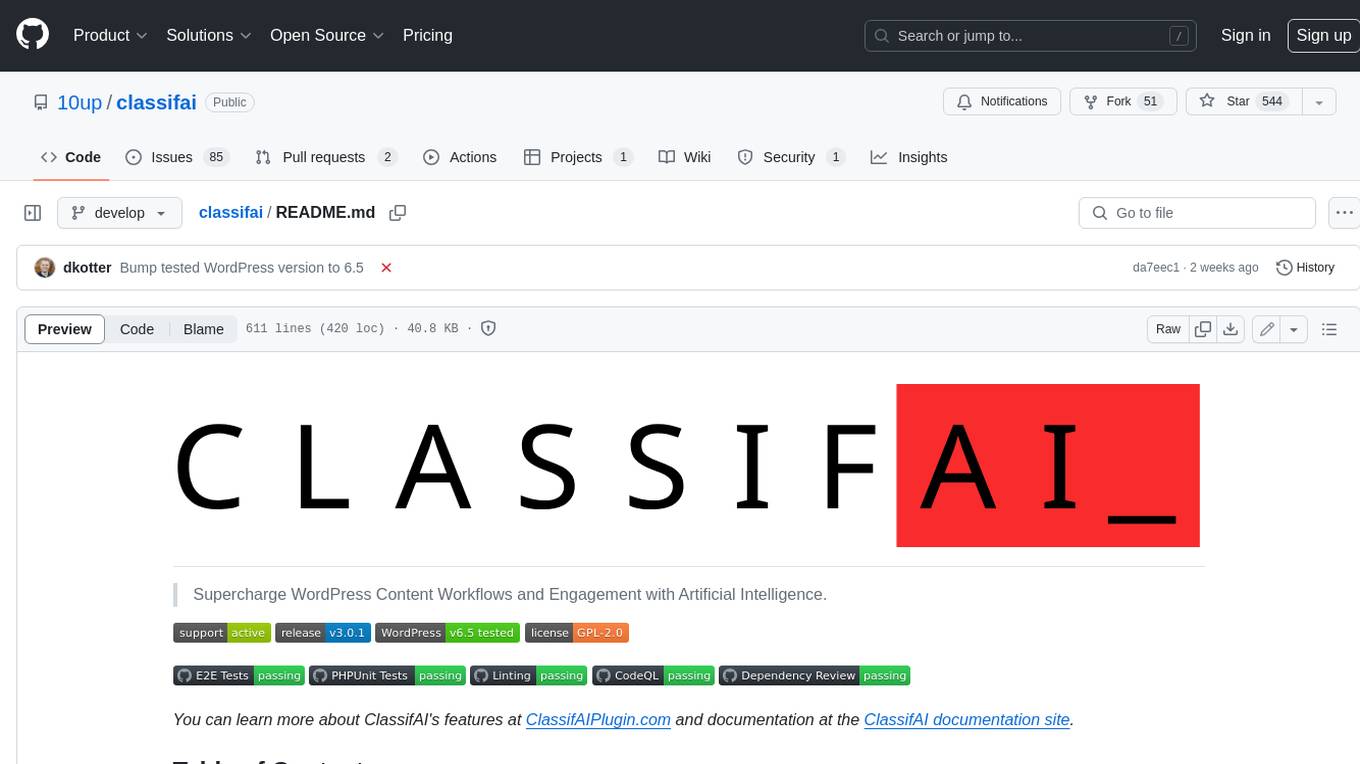
classifai
Supercharge WordPress Content Workflows and Engagement with Artificial Intelligence. Tap into leading cloud-based services like OpenAI, Microsoft Azure AI, Google Gemini and IBM Watson to augment your WordPress-powered websites. Publish content faster while improving SEO performance and increasing audience engagement. ClassifAI integrates Artificial Intelligence and Machine Learning technologies to lighten your workload and eliminate tedious tasks, giving you more time to create original content that matters.
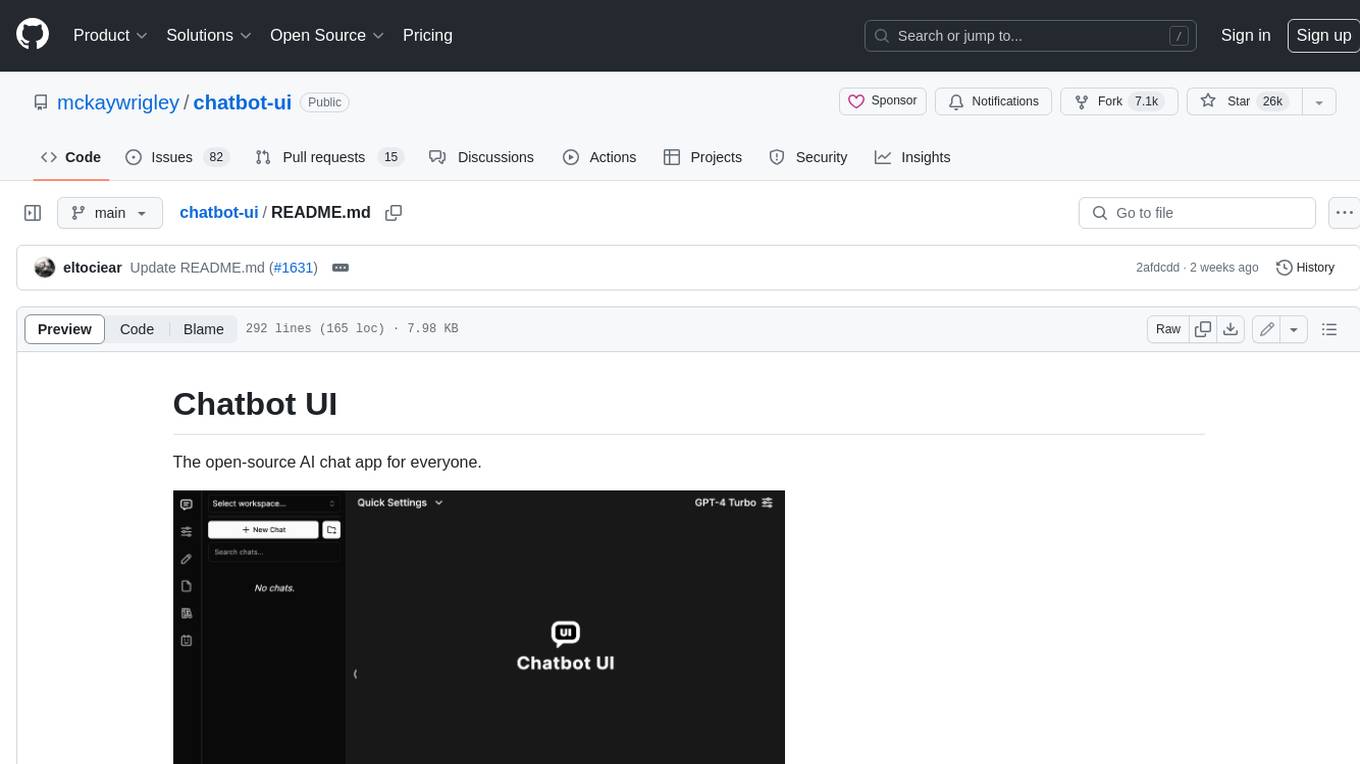
chatbot-ui
Chatbot UI is an open-source AI chat app that allows users to create and deploy their own AI chatbots. It is easy to use and can be customized to fit any need. Chatbot UI is perfect for businesses, developers, and anyone who wants to create a chatbot.
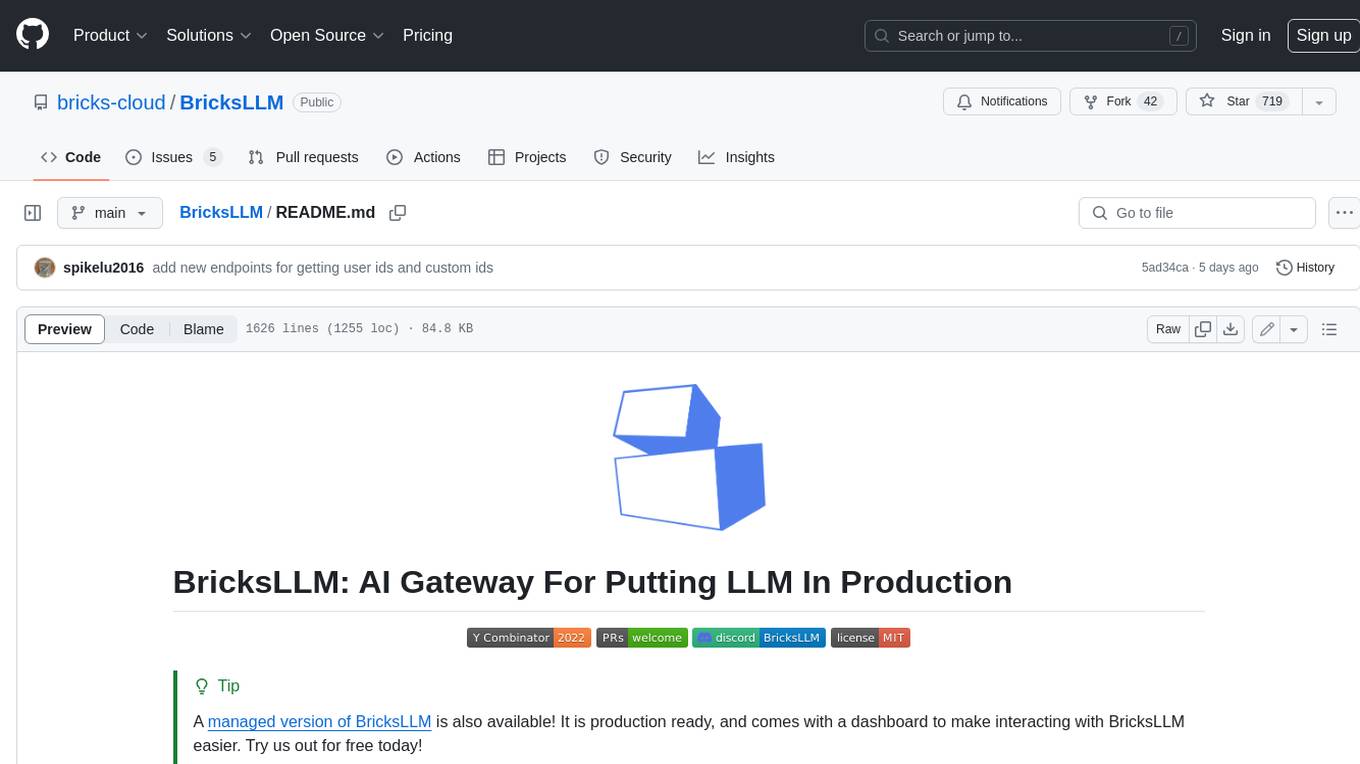
BricksLLM
BricksLLM is a cloud native AI gateway written in Go. Currently, it provides native support for OpenAI, Anthropic, Azure OpenAI and vLLM. BricksLLM aims to provide enterprise level infrastructure that can power any LLM production use cases. Here are some use cases for BricksLLM: * Set LLM usage limits for users on different pricing tiers * Track LLM usage on a per user and per organization basis * Block or redact requests containing PIIs * Improve LLM reliability with failovers, retries and caching * Distribute API keys with rate limits and cost limits for internal development/production use cases * Distribute API keys with rate limits and cost limits for students
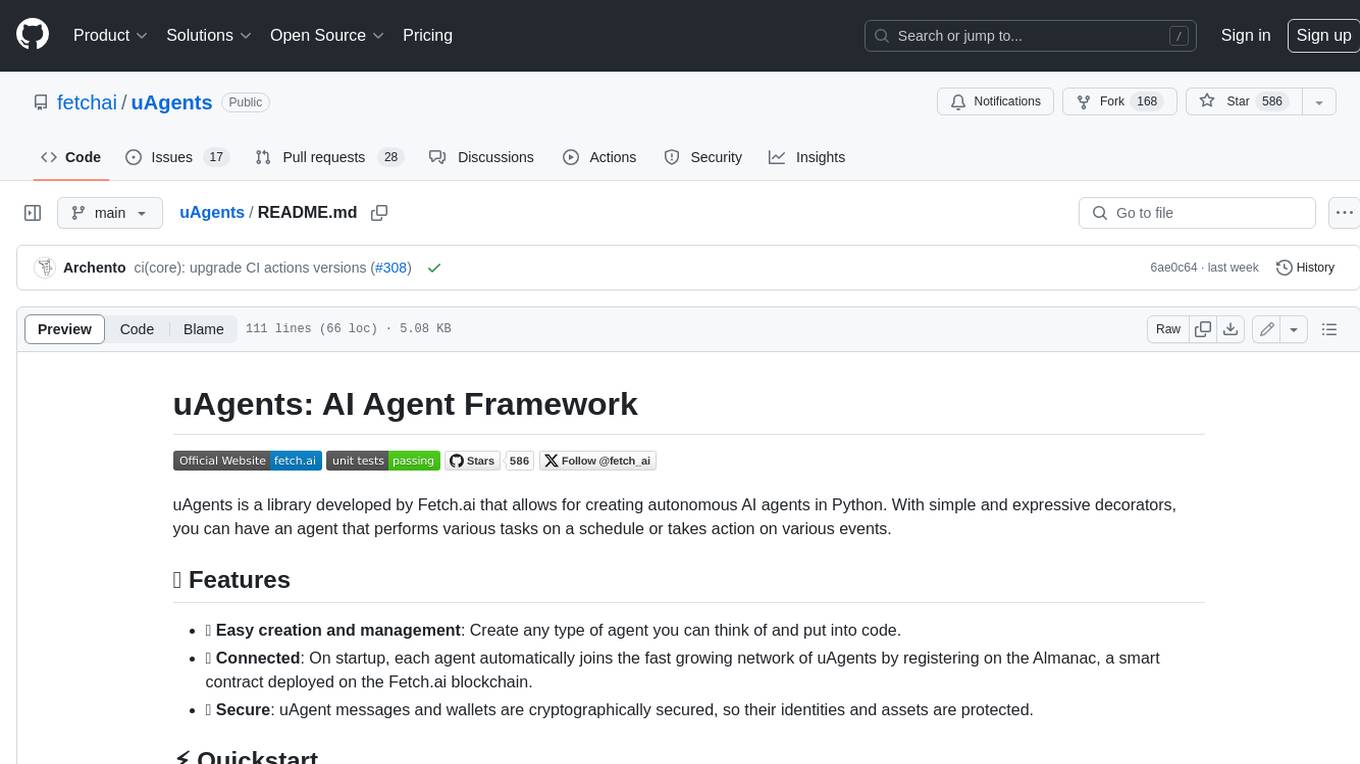
uAgents
uAgents is a Python library developed by Fetch.ai that allows for the creation of autonomous AI agents. These agents can perform various tasks on a schedule or take action on various events. uAgents are easy to create and manage, and they are connected to a fast-growing network of other uAgents. They are also secure, with cryptographically secured messages and wallets.
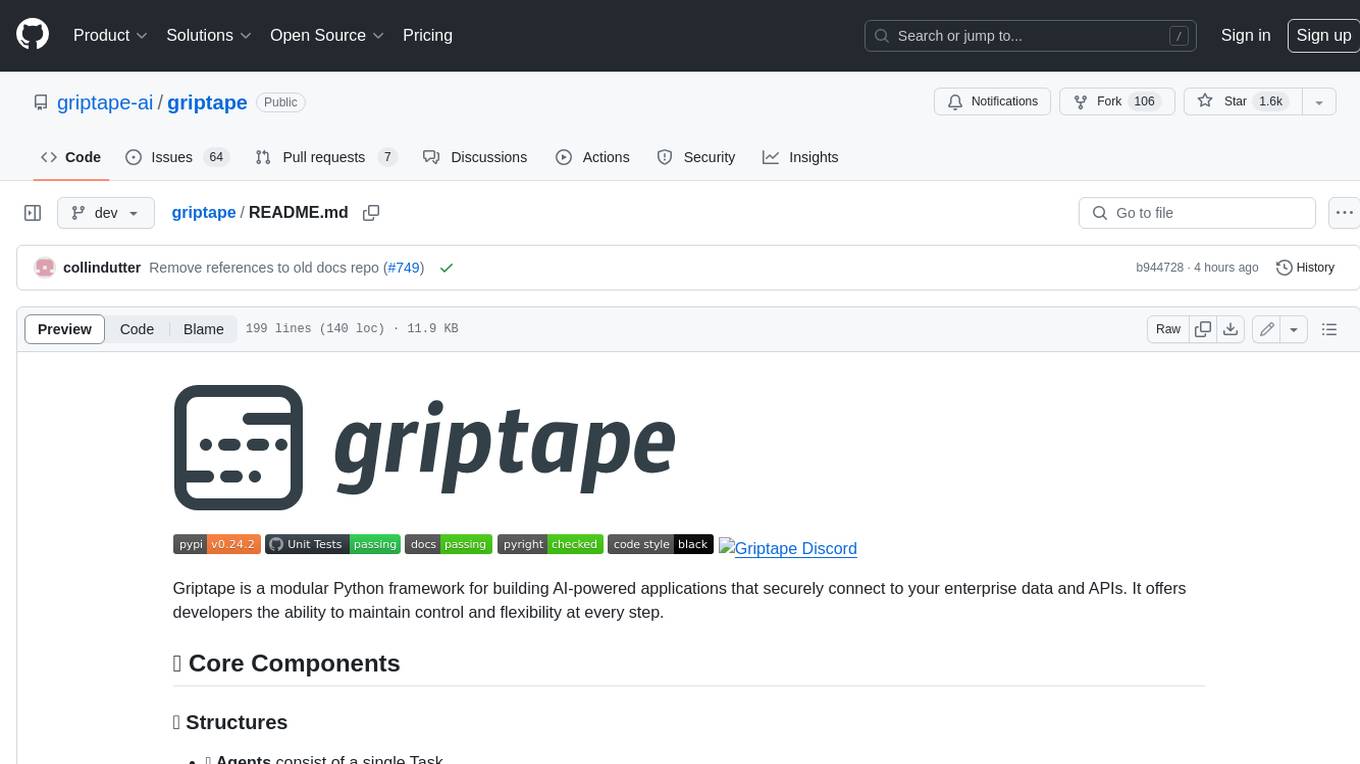
griptape
Griptape is a modular Python framework for building AI-powered applications that securely connect to your enterprise data and APIs. It offers developers the ability to maintain control and flexibility at every step. Griptape's core components include Structures (Agents, Pipelines, and Workflows), Tasks, Tools, Memory (Conversation Memory, Task Memory, and Meta Memory), Drivers (Prompt and Embedding Drivers, Vector Store Drivers, Image Generation Drivers, Image Query Drivers, SQL Drivers, Web Scraper Drivers, and Conversation Memory Drivers), Engines (Query Engines, Extraction Engines, Summary Engines, Image Generation Engines, and Image Query Engines), and additional components (Rulesets, Loaders, Artifacts, Chunkers, and Tokenizers). Griptape enables developers to create AI-powered applications with ease and efficiency.