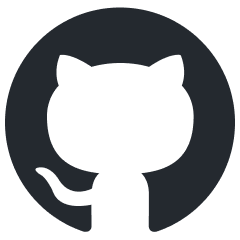
llm2vec
Code for 'LLM2Vec: Large Language Models Are Secretly Powerful Text Encoders'
Stars: 1152
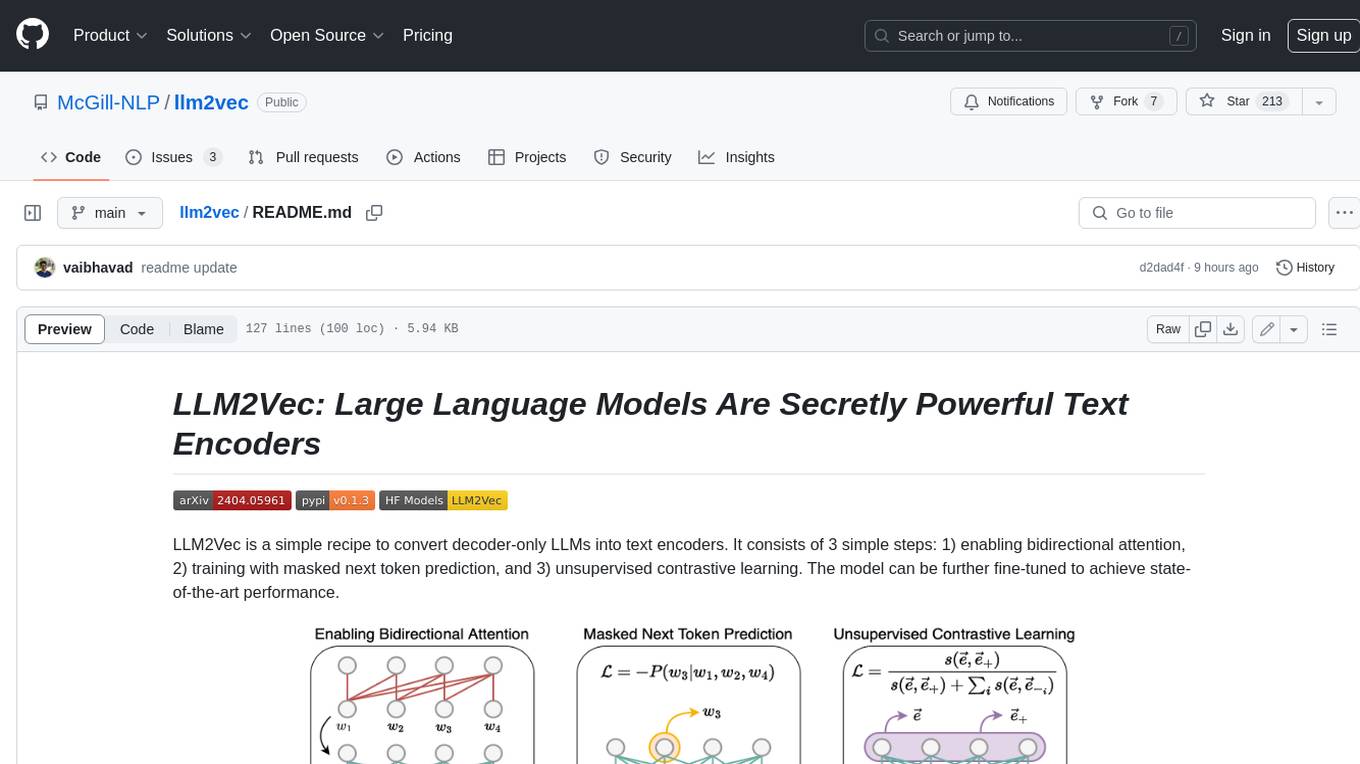
LLM2Vec is a simple recipe to convert decoder-only LLMs into text encoders. It consists of 3 simple steps: 1) enabling bidirectional attention, 2) training with masked next token prediction, and 3) unsupervised contrastive learning. The model can be further fine-tuned to achieve state-of-the-art performance.
README:
LLM2Vec is a simple recipe to convert decoder-only LLMs into text encoders. It consists of 3 simple steps: 1) enabling bidirectional attention, 2) training with masked next token prediction, and 3) unsupervised contrastive learning. The model can be further fine-tuned to achieve state-of-the-art performance.
**************************** Updates ****************************
-
04/07: Added support for Gemma and Qwen-2 models, huge thanks to @bzantium for the contribution.
-
30/04: We release LLM2Vec transformed Meta-Llama-3 checkpoints. See our HuggingFace collection for both supervised and unsupervised variants.
To use LLM2Vec, first install the llm2vec package from PyPI, followed by installing flash-attention:
pip install llm2vec
pip install flash-attn --no-build-isolation
You can also directly install the latest version of llm2vec by cloning the repository:
pip install -e .
pip install flash-attn --no-build-isolation
LLM2Vec class is a wrapper on top of HuggingFace models to support enabling bidirectionality in decoder-only LLMs, sequence encoding and pooling operations. The steps below showcase an example on how to use the library.
Initializing LLM2Vec model using pretrained LLMs is straightforward. The from_pretrained
method of LLM2Vec takes a base model identifier/path and an optional PEFT model identifier/path. All HuggingFace model loading arguments can be passed to from_pretrained
method. By default, the models are loaded with bidirectional connections enabled. This can be turned off by passing enable_bidirectional=False
to the from_pretrained
method.
Here, we first initialize the Llama-3 MNTP base model and load the unsupervised-trained LoRA weights (trained with SimCSE objective and wiki corpus).
import torch
from llm2vec import LLM2Vec
l2v = LLM2Vec.from_pretrained(
"McGill-NLP/LLM2Vec-Meta-Llama-3-8B-Instruct-mntp",
peft_model_name_or_path="McGill-NLP/LLM2Vec-Meta-Llama-3-8B-Instruct-mntp-unsup-simcse",
device_map="cuda" if torch.cuda.is_available() else "cpu",
torch_dtype=torch.bfloat16,
)
We can also load the model with supervised-trained LoRA weights (trained with contrastive learning and public E5 data) by changing the peft_model_name_or_path
.
import torch
from llm2vec import LLM2Vec
l2v = LLM2Vec.from_pretrained(
"McGill-NLP/LLM2Vec-Meta-Llama-3-8B-Instruct-mntp",
peft_model_name_or_path="McGill-NLP/LLM2Vec-Meta-Llama-3-8B-Instruct-mntp-supervised",
device_map="cuda" if torch.cuda.is_available() else "cpu",
torch_dtype=torch.bfloat16,
)
By default the LLM2Vec model uses the mean
pooling strategy. You can change the pooling strategy by passing the pooling_mode
argument to the from_pretrained
method. Similarly, you can change the maximum sequence length by passing the max_length
argument (default is 512).
This model now returns the text embedding for any input in the form of [[instruction1, text1], [instruction2, text2]]
or [text1, text2]
. While training, we provide instructions for both sentences in symmetric tasks, and only for for queries in asymmetric tasks.
# Encoding queries using instructions
instruction = (
"Given a web search query, retrieve relevant passages that answer the query:"
)
queries = [
[instruction, "how much protein should a female eat"],
[instruction, "summit define"],
]
q_reps = l2v.encode(queries)
# Encoding documents. Instruction are not required for documents
documents = [
"As a general guideline, the CDC's average requirement of protein for women ages 19 to 70 is 46 grams per day. But, as you can see from this chart, you'll need to increase that if you're expecting or training for a marathon. Check out the chart below to see how much protein you should be eating each day.",
"Definition of summit for English Language Learners. : 1 the highest point of a mountain : the top of a mountain. : 2 the highest level. : 3 a meeting or series of meetings between the leaders of two or more governments.",
]
d_reps = l2v.encode(documents)
# Compute cosine similarity
q_reps_norm = torch.nn.functional.normalize(q_reps, p=2, dim=1)
d_reps_norm = torch.nn.functional.normalize(d_reps, p=2, dim=1)
cos_sim = torch.mm(q_reps_norm, d_reps_norm.transpose(0, 1))
print(cos_sim)
"""
tensor([[0.6470, 0.1619],
[0.0786, 0.5844]])
"""
More examples of classification, clustering, sentence similarity etc are present in examples directory.
Meta-Llama-3-8B | Mistral-7B | Llama-2-7B | Sheared-Llama-1.3B | |
---|---|---|---|---|
Bi + MNTP | HF Link | HF Link | HF Link | HF Link |
Bi + MNTP + SimCSE | HF Link | HF Link** | HF Link | HF Link |
Bi + MNTP + Supervised | HF Link* | HF Link | HF Link | HF Link |
* State-of-the-art on MTEB among models trained on public data
** Unsupervised state-of-the-art on MTEB
To train the model with Masked Next Token Prediction (MNTP), you can use the experiments/run_mntp.py
script. It is adapted from HuggingFace Masked Language Modeling (MLM) script. To train the Meta-Llama-3-8B model with MNTP, run the following command:
python experiments/run_mntp.py train_configs/mntp/MetaLlama3.json
The Meta-Llama-3-8B training configuration file contains all the training hyperparameters and configurations used in our paper.
{
"model_name_or_path": "meta-llama/Meta-Llama-3-8B-Instruct",
"dataset_name": "wikitext",
"dataset_config_name": "wikitext-103-raw-v1",
"mask_token_type": "blank",
"data_collator_type": "default",
"mlm_probability": 0.2,
"lora_r": 16,
"gradient_checkpointing": true,
"torch_dtype": "bfloat16",
"attn_implementation": "flash_attention_2"
// ....
}
Similar configurations are also available forMistral-7B, Llama-2-7B, and Sheared-Llama-1.3B models.
For SimCSE training, we replicated the training procedure from SimCSE paper. For training, we use the dataset 1 million sentences from English Wikipedia released by the authors. It can be downloaded using the following command:
wget https://huggingface.co/datasets/princeton-nlp/datasets-for-simcse/resolve/main/wiki1m_for_simcse.txt
To use the training script with pre-set configurations, the downloaded file should be placed in the cache
directory. The directory layout should be as follows:
cache
└── wiki1m_for_simcse.txt
If the dataset is placed in a different directory, please change the dataset_file_path in the training configuration accordingly.
To train the Meta-Llama-3-8B model with SimCSE, run the following command:
python experiments/run_simcse.py train_configs/simcse/MetaLlama3.json
The Meta-Llama-3-8B training configuration file contains all the training hyperparameters and configurations used in our paper.
{
"model_name_or_path": "meta-llama/Meta-Llama-3-8B-Instruct",
"peft_model_name_or_path": "McGill-NLP/LLM2Vec-Meta-Llama-3-8B-Instruct-mntp",
"simcse_dropout": 0.3,
"bidirectional": true,
"pooling_mode": "mean",
"dataset_name": "Wiki1M",
"dataset_file_path": "cache/wiki1m_for_simcse.txt",
"learning_rate": 3e-5,
"loss_scale": 20,
"per_device_train_batch_size": 128,
"max_seq_length": 128,
"stop_after_n_steps": 1000,
"lora_r": 16,
"gradient_checkpointing": true,
"torch_dtype": "bfloat16",
"attn_implementation": "flash_attention_2",
// ....
}
Similar configurations are also available for Mistral, Llama-2-7B, and Sheared-Llama-1.3B models.
For supervised contrastive training, we use the public portion of dataset used in Improving Text Embeddings with Large Language Models, curated by authors of Repetition Improves Language Model Embeddings. The dataset can be downloaded from the GitHub page of Echo embeddings repository. To use the training script, the downloaded dataset should be placed in the cache
directory. The directory layout should be as follows:
cache
|── wiki1m_for_simcse.txt
└── echo-data
├── allnli_split1.jsonl
├── allnli_split2.jsonl
├── allnli.jsonl
├── dureader.jsonl
...
If the dataset is placed in a different directory, please change the dataset_file_path
in the training configuration accordingly.
To train the Meta-Llama-3-8B model with supervised contrastive learning, run the following command:
torchrun --nproc_per_node=8 experiments/run_supervised.py train_configs/supervised/MetaLlama3.json
The number of GPUs can be changed by modifying the --nproc_per_node
argument.
The Meta-Llama-3-8B training configuration file contains all the training hyperparameters and configurations used in our paper.
{
"model_name_or_path": "meta-llama/Meta-Llama-3-8B-Instruct",
"peft_model_name_or_path": "McGill-NLP/LLM2Vec-Meta-Llama-3-8B-Instruct-mntp",
"bidirectional": true,
"pooling_mode": "mean",
"dataset_name": "E5",
"dataset_file_path": "cache/echo-data",
"learning_rate": 2e-4,
"num_train_epochs": 3,
"warmup_steps": 300,
"per_device_train_batch_size": 64,
"lora_r": 16,
"gradient_checkpointing": true,
"torch_dtype": "bfloat16",
"attn_implementation": "flash_attention_2"
// ....
}
Similar configurations are also available for Mistral, Llama-2-7B, and Sheared-Llama-1.3B models.
To tune the model for word-level tasks, we define a classifier on top of the models, and only train the classifier weights. The code is adapted from HuggingFace token classification example. To train and test the classifier for Llama-2-7B MNTP model on pos_tags
task, run the following command:
python experiments/run_word_task.py train_configs/word-task/Llama2-bi-mntp.json
python experiments/test_word_task.py --config_file test_configs/word-task/Llama2-bi-mntp.json
The config files contain all the parameters and configurations used in our paper. For instance, Llama2-bi-mntp.json
includes:
{
"model_name_or_path": "meta-llama/Llama-2-7b-chat-hf",
"peft_addr": "McGill-NLP/LLM2Vec-Llama-2-7b-chat-hf-mntp", // or any local directory containing `adapter_model` files.
"model_class": "custom",
"bidirectional": true,
"classifier_dropout": 0.1,
"merge_subwords": true,
"retroactive_labels": "next_token",
"output_dir": "output/word-task/pos_tags/Llama2/bi-mntp",
"dataset_name": "conll2003",
"task": "pos_tags", // or ner_tags, or chunk_tags
// ....
}
train_configs/word-task and test_configs/word-task contain similar configurations for Llama-2-7B, Mistral-7B, and Sheared-Llama-1.3B for all Uni, Bi, Bi-MNTP, and Bi-MNTP-SimCSE (LLM2Vec) variants.
To evaluate the model on the MTEB benchmark, we use the experiments/mteb_eval.py
script. The script requires mteb>=1.12.60
, amongst other dependencies, which can be installed with the following command.
pip install llm2vec[evaluation]
The evaluation utilizes instructions for each task which are provided in the test_configs/mteb/task_to_instructions.json
file.
To evaluate the supervised trained Meta-Llama-3-8B model on the STS16
task, run the following command:
python experiments/mteb_eval.py --model_name McGill-NLP/LLM2Vec-Meta-Llama-3-8B-Instruct-mntp-supervised \
--task_name STS16 \
--task_to_instructions_fp test_configs/mteb/task_to_instructions.json \
--output_dir results
The evaluation script supports all the models available in the HuggingFace collection.
If you find our work helpful, please cite us:
@inproceedings{
llm2vec,
title={{LLM2V}ec: Large Language Models Are Secretly Powerful Text Encoders},
author={Parishad BehnamGhader and Vaibhav Adlakha and Marius Mosbach and Dzmitry Bahdanau and Nicolas Chapados and Siva Reddy},
booktitle={First Conference on Language Modeling},
year={2024},
url={https://openreview.net/forum?id=IW1PR7vEBf}
}
If you have any questions about the code, feel free to open an issue on the GitHub repository.
For Tasks:
Click tags to check more tools for each tasksFor Jobs:
Alternative AI tools for llm2vec
Similar Open Source Tools
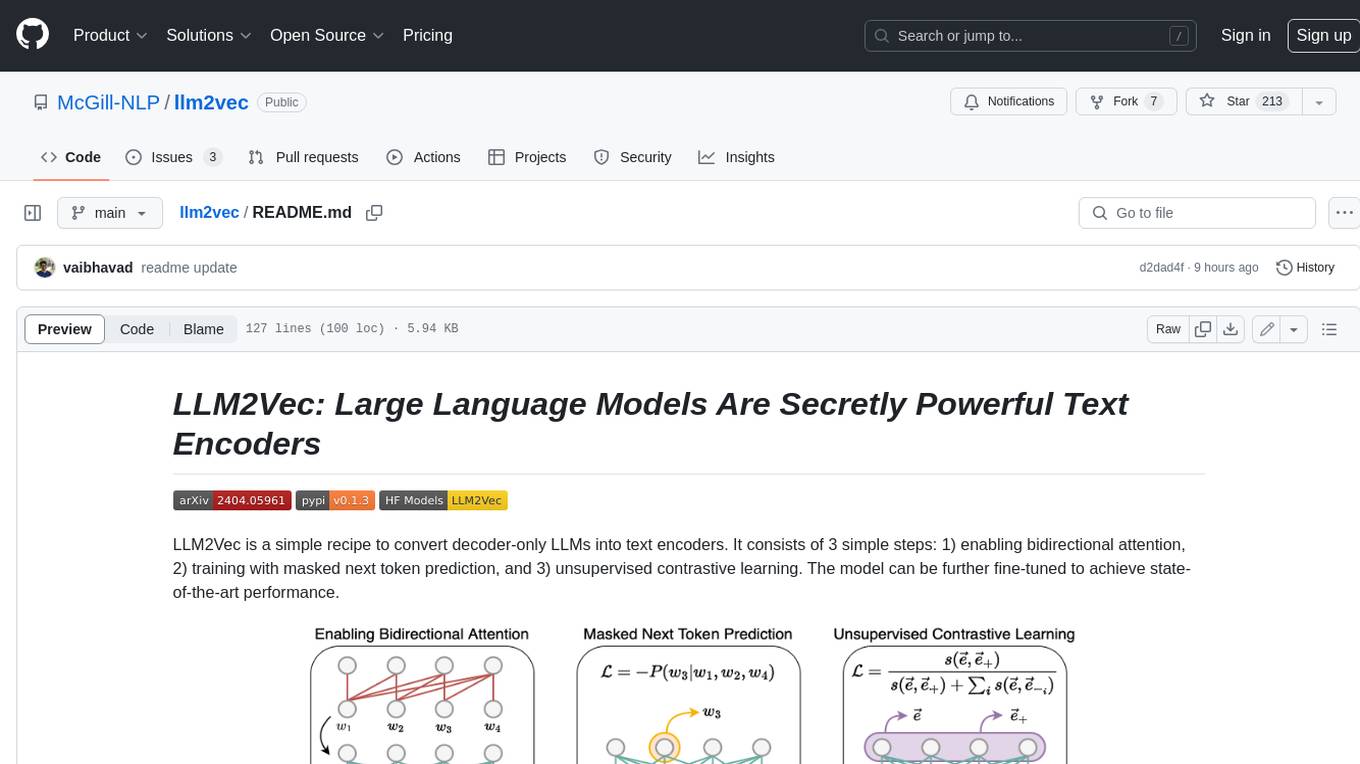
llm2vec
LLM2Vec is a simple recipe to convert decoder-only LLMs into text encoders. It consists of 3 simple steps: 1) enabling bidirectional attention, 2) training with masked next token prediction, and 3) unsupervised contrastive learning. The model can be further fine-tuned to achieve state-of-the-art performance.
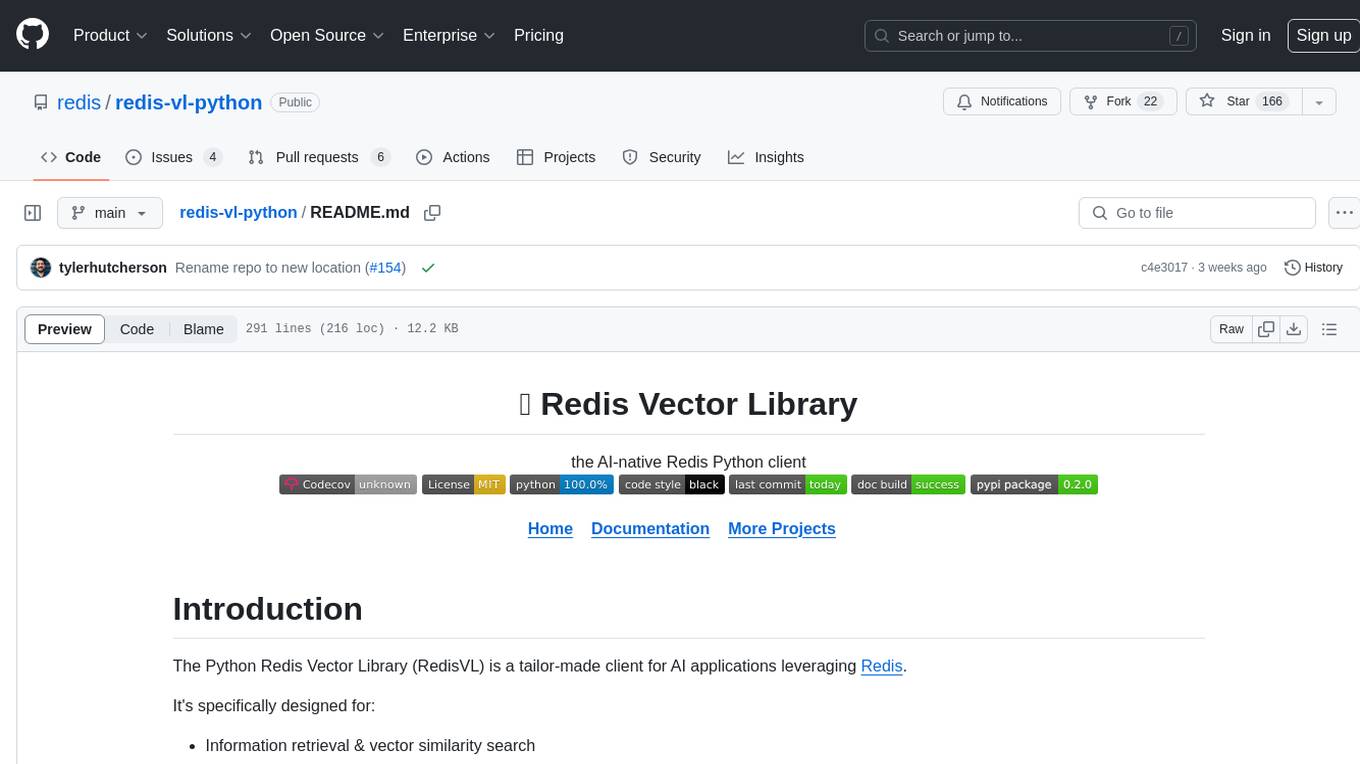
redis-vl-python
The Python Redis Vector Library (RedisVL) is a tailor-made client for AI applications leveraging Redis. It enhances applications with Redis' speed, flexibility, and reliability, incorporating capabilities like vector-based semantic search, full-text search, and geo-spatial search. The library bridges the gap between the emerging AI-native developer ecosystem and the capabilities of Redis by providing a lightweight, elegant, and intuitive interface. It abstracts the features of Redis into a grammar that is more aligned to the needs of today's AI/ML Engineers or Data Scientists.
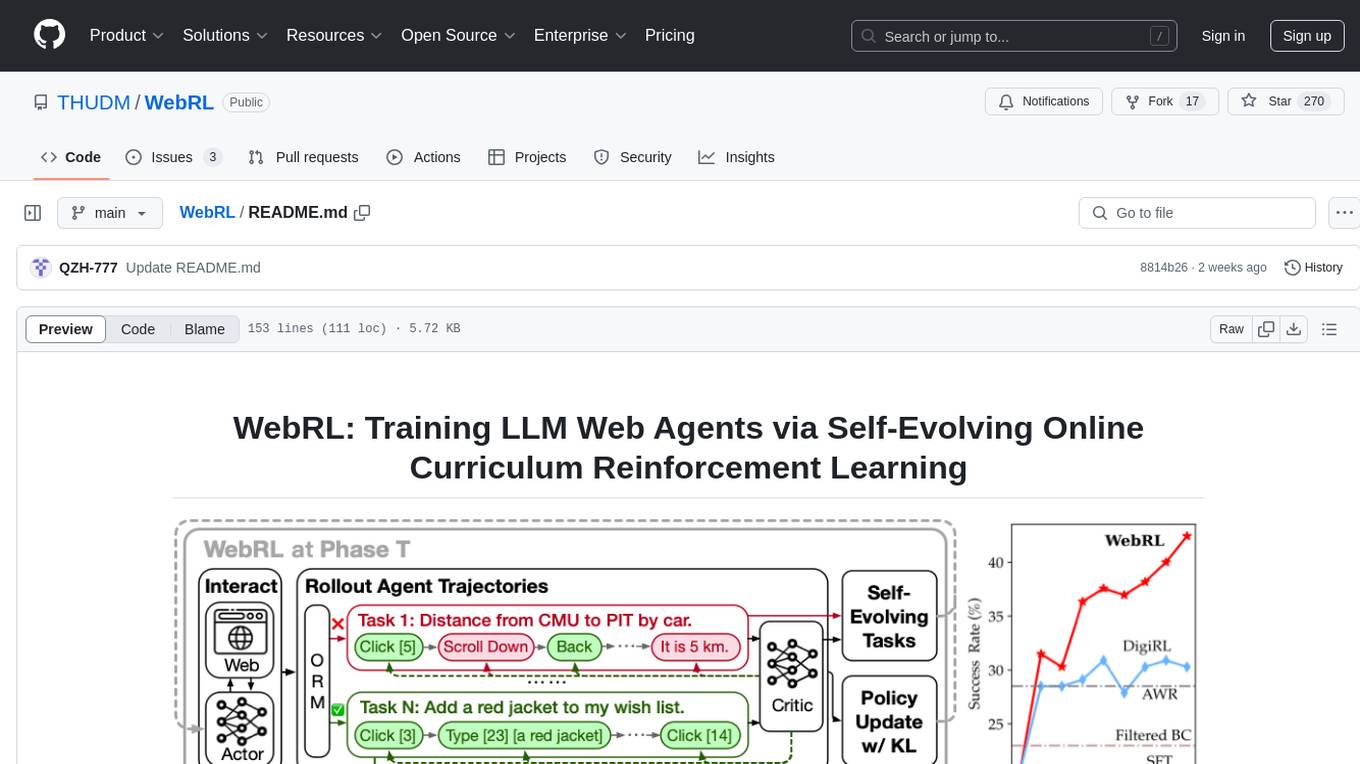
WebRL
WebRL is a self-evolving online curriculum learning framework designed for training web agents in the WebArena environment. It provides model checkpoints, training instructions, and evaluation processes for training the actor and critic models. The tool enables users to generate new instructions and interact with WebArena to configure tasks for training and evaluation.
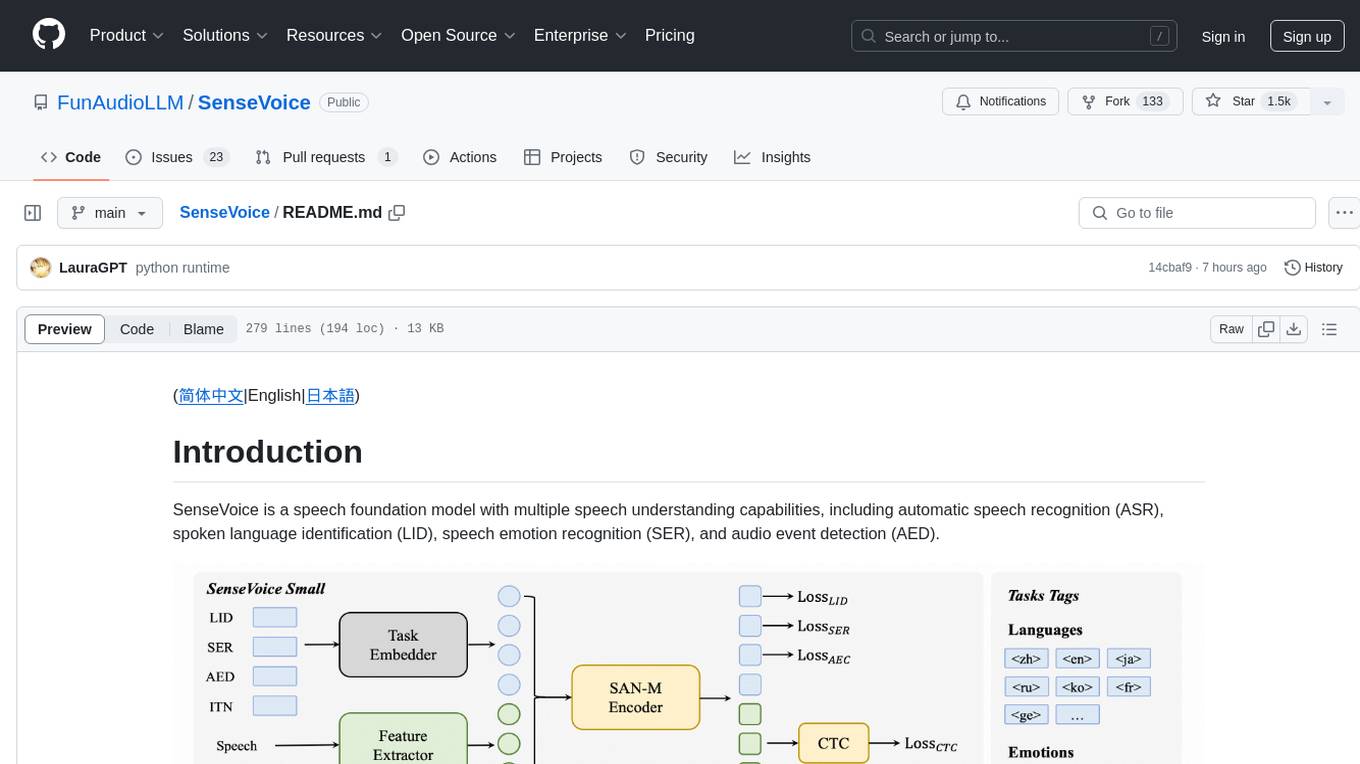
SenseVoice
SenseVoice is a speech foundation model focusing on high-accuracy multilingual speech recognition, speech emotion recognition, and audio event detection. Trained with over 400,000 hours of data, it supports more than 50 languages and excels in emotion recognition and sound event detection. The model offers efficient inference with low latency and convenient finetuning scripts. It can be deployed for service with support for multiple client-side languages. SenseVoice-Small model is open-sourced and provides capabilities for Mandarin, Cantonese, English, Japanese, and Korean. The tool also includes features for natural speech generation and fundamental speech recognition tasks.
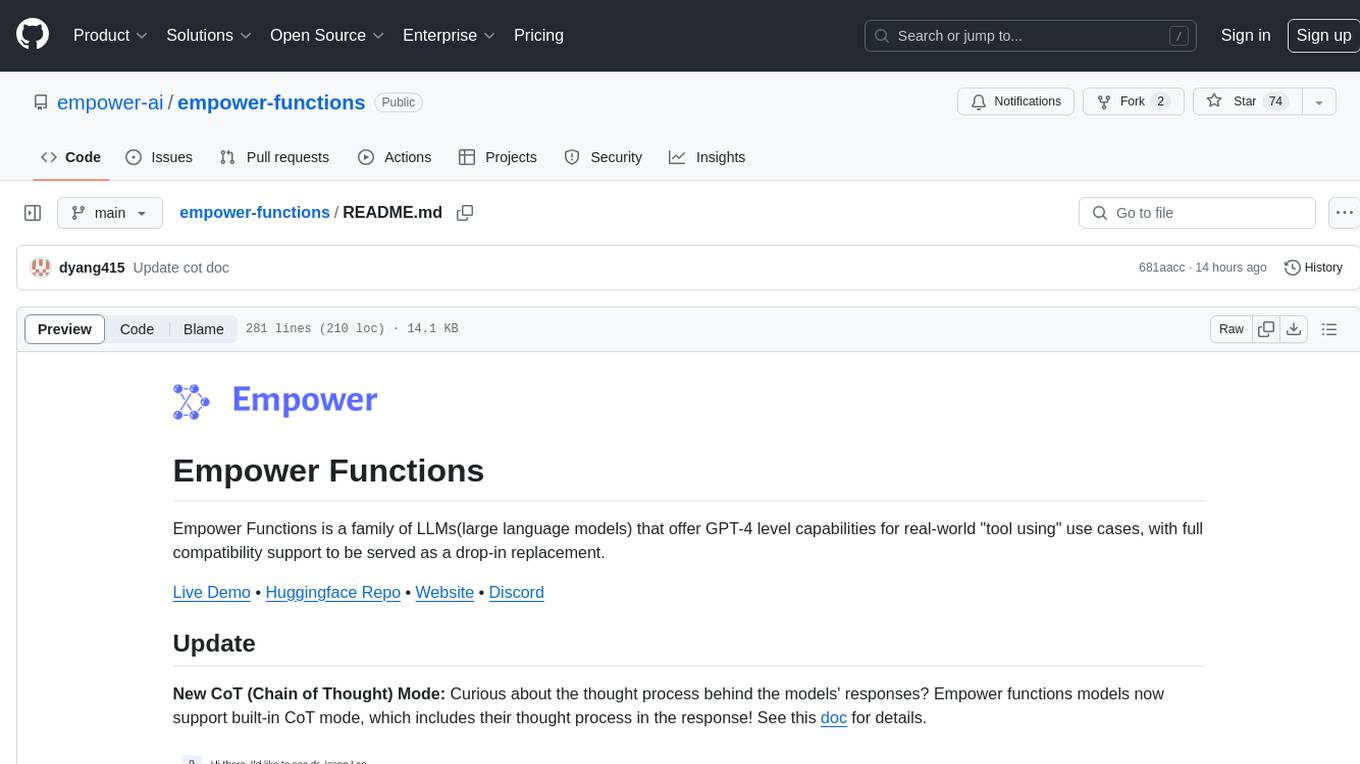
empower-functions
Empower Functions is a family of large language models (LLMs) that provide GPT-4 level capabilities for real-world 'tool using' use cases. These models offer compatibility support to be used as drop-in replacements, enabling interactions with external APIs by recognizing when a function needs to be called and generating JSON containing necessary arguments based on user inputs. This capability is crucial for building conversational agents and applications that convert natural language into API calls, facilitating tasks such as weather inquiries, data extraction, and interactions with knowledge bases. The models can handle multi-turn conversations, choose between tools or standard dialogue, ask for clarification on missing parameters, integrate responses with tool outputs in a streaming fashion, and efficiently execute multiple functions either in parallel or sequentially with dependencies.
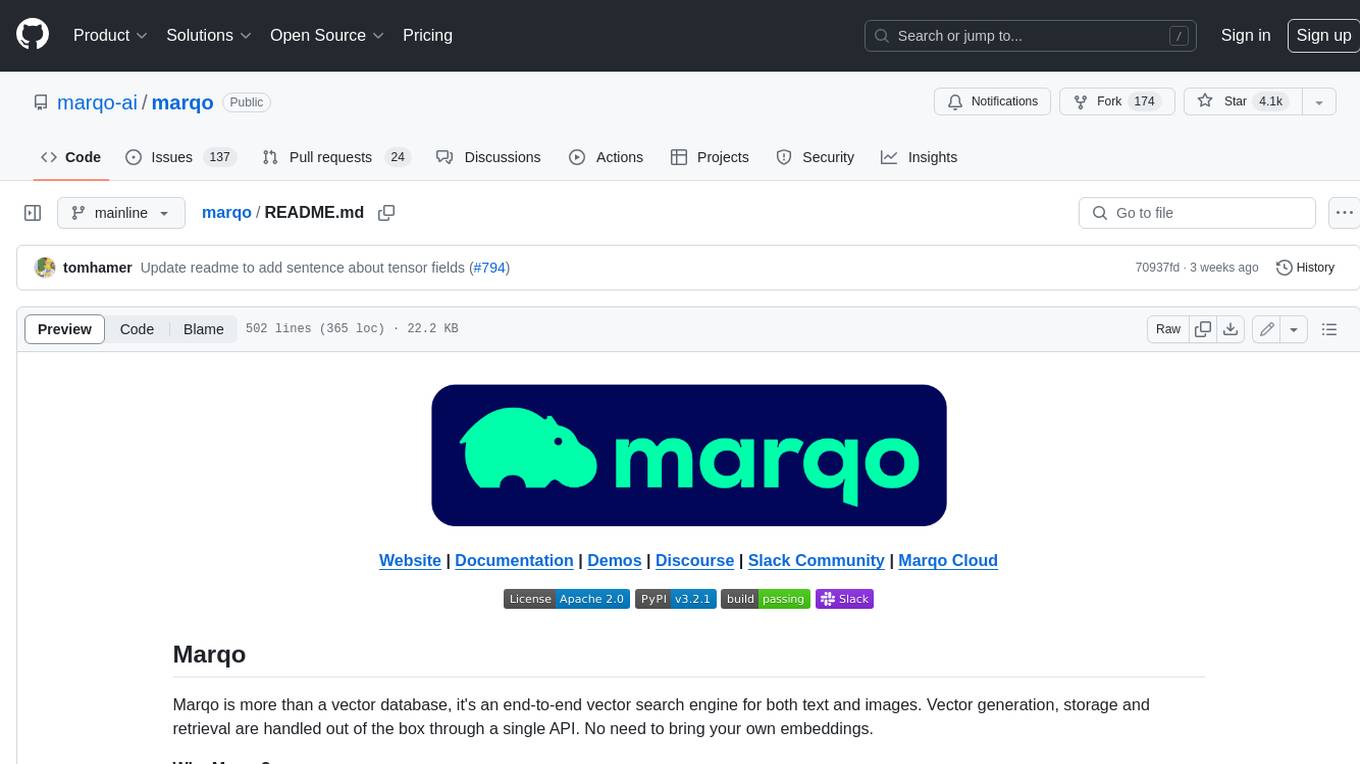
marqo
Marqo is more than a vector database, it's an end-to-end vector search engine for both text and images. Vector generation, storage and retrieval are handled out of the box through a single API. No need to bring your own embeddings.
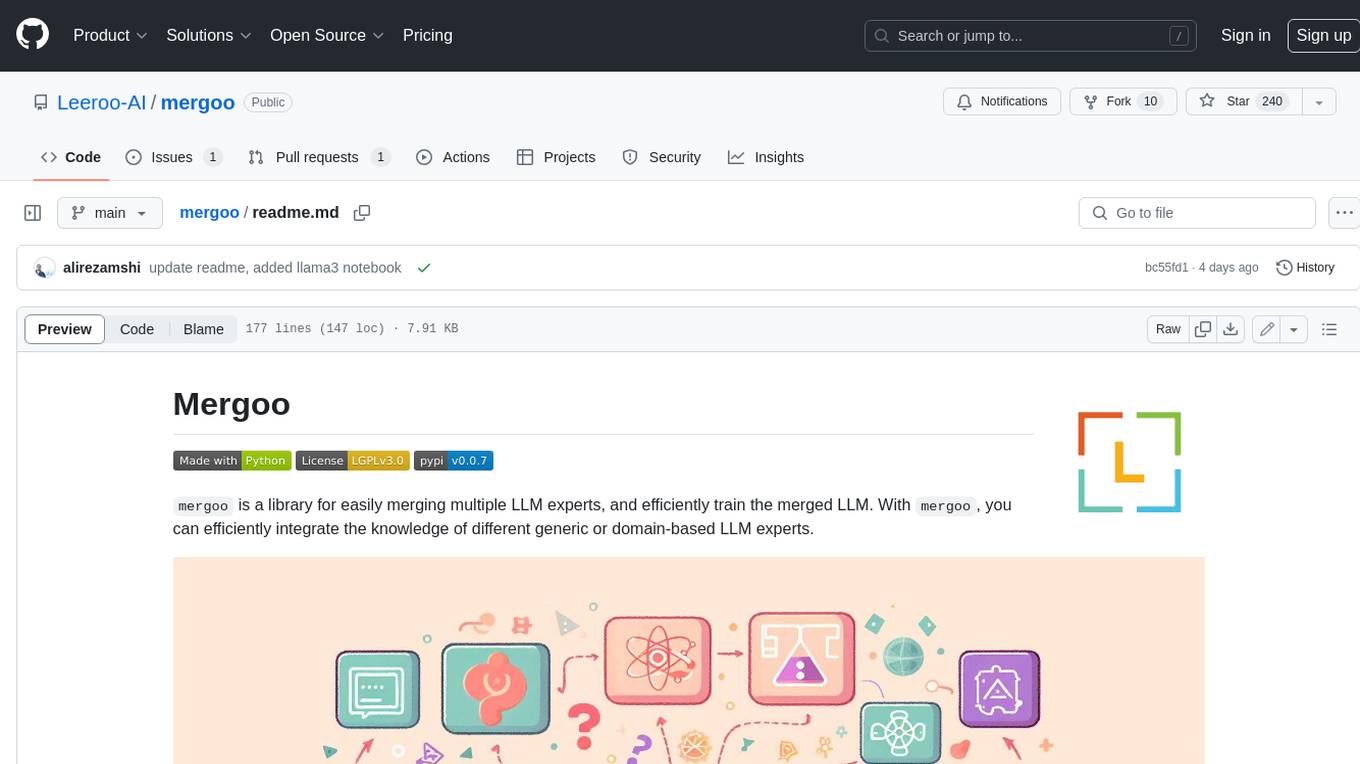
mergoo
Mergoo is a library for easily merging multiple LLM experts and efficiently training the merged LLM. With Mergoo, you can efficiently integrate the knowledge of different generic or domain-based LLM experts. Mergoo supports several merging methods, including Mixture-of-Experts, Mixture-of-Adapters, and Layer-wise merging. It also supports various base models, including LLaMa, Mistral, and BERT, and trainers, including Hugging Face Trainer, SFTrainer, and PEFT. Mergoo provides flexible merging for each layer and supports training choices such as only routing MoE layers or fully fine-tuning the merged LLM.
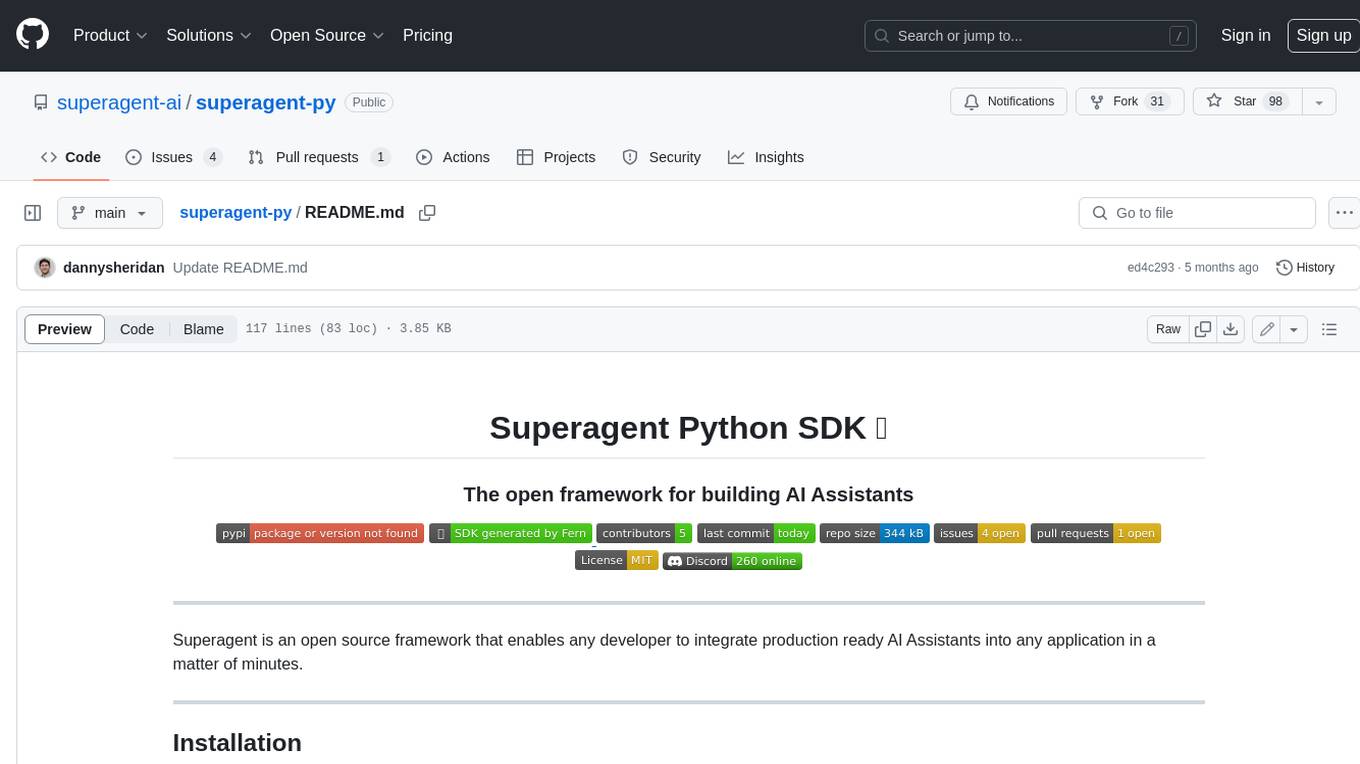
superagent-py
Superagent is an open-source framework that enables developers to integrate production-ready AI assistants into any application quickly and easily. It provides a Python SDK for interacting with the Superagent API, allowing developers to create, manage, and invoke AI agents. The SDK simplifies the process of building AI-powered applications, making it accessible to developers of all skill levels.
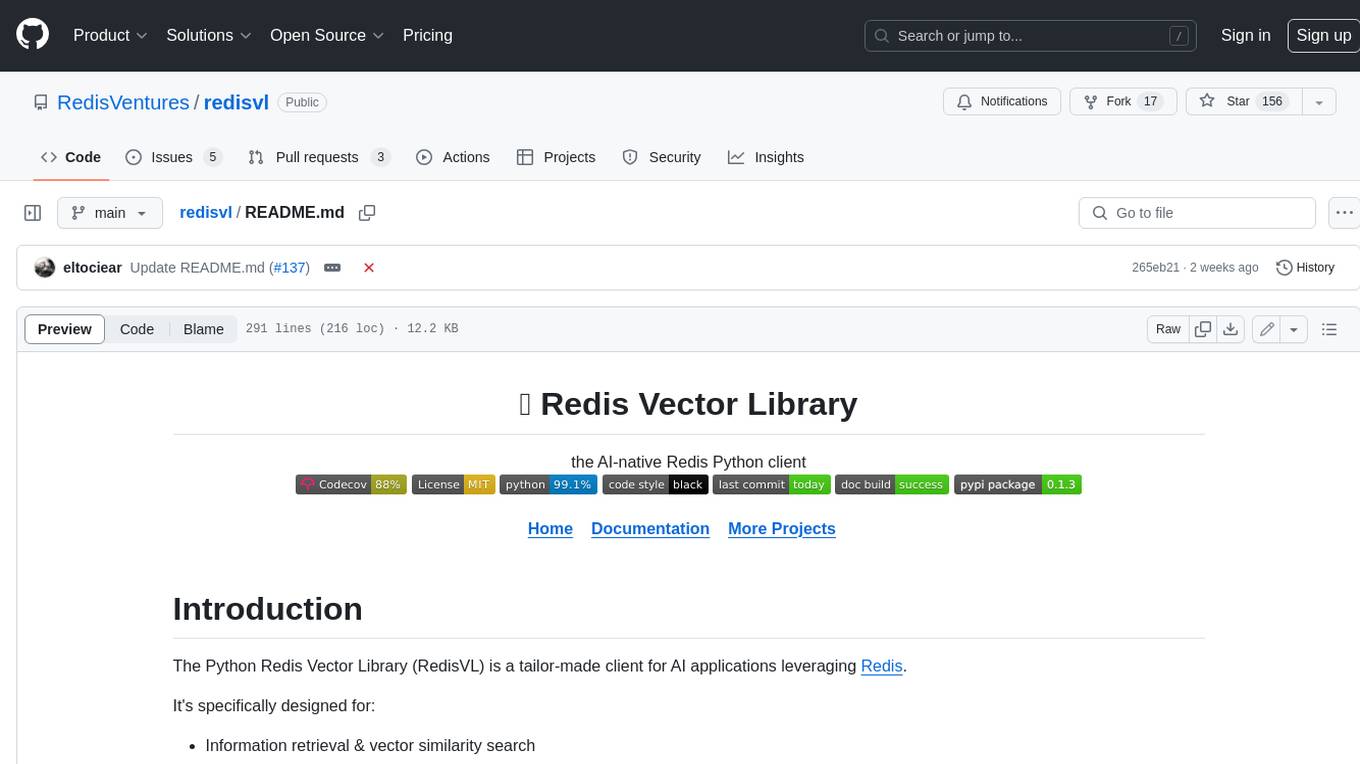
redisvl
Redis Vector Library (RedisVL) is a Python client library for building AI applications on top of Redis. It provides a high-level interface for managing vector indexes, performing vector search, and integrating with popular embedding models and providers. RedisVL is designed to make it easy for developers to build and deploy AI applications that leverage the speed, flexibility, and reliability of Redis.
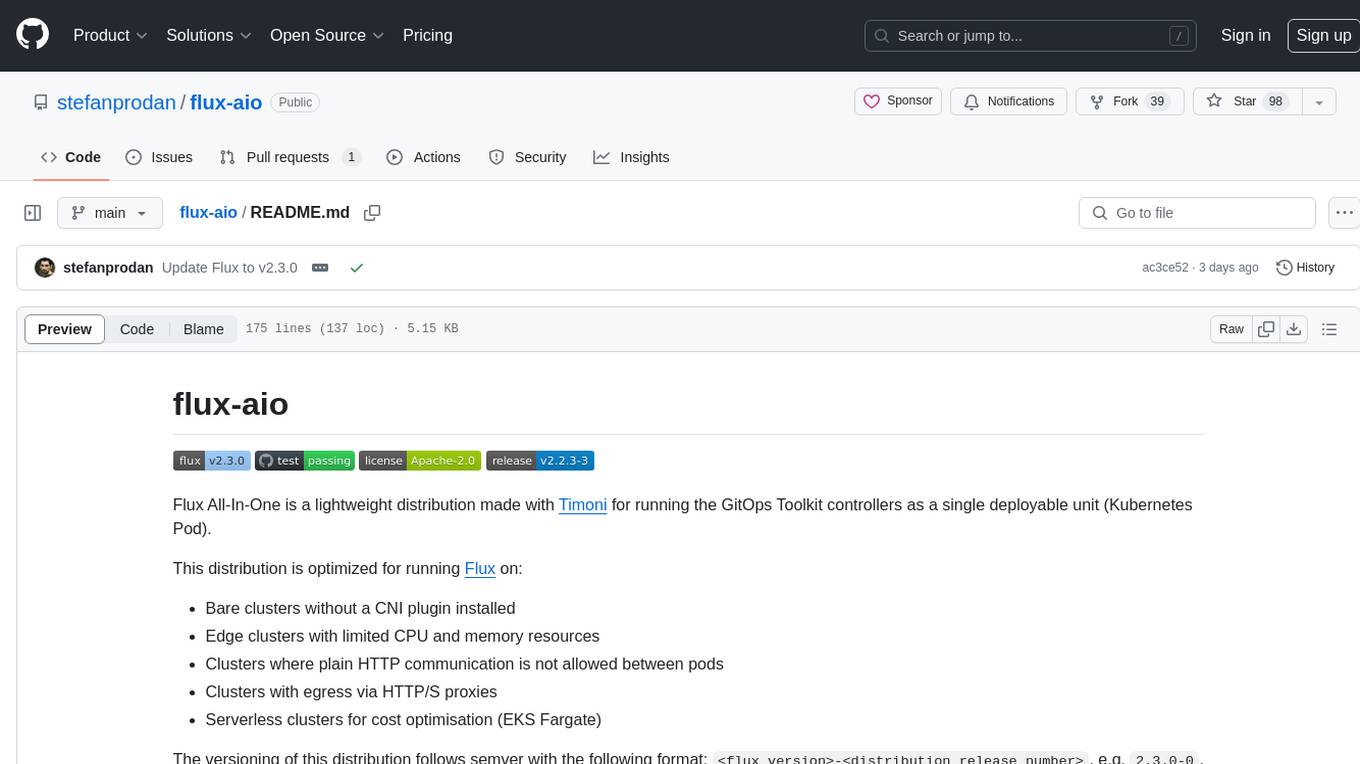
flux-aio
Flux All-In-One is a lightweight distribution optimized for running the GitOps Toolkit controllers as a single deployable unit on Kubernetes clusters. It is designed for bare clusters, edge clusters, clusters with restricted communication, clusters with egress via proxies, and serverless clusters. The distribution follows semver versioning and provides documentation for specifications, installation, upgrade, OCI sync configuration, Git sync configuration, and multi-tenancy configuration. Users can deploy Flux using Timoni CLI and a Timoni Bundle file, fine-tune installation options, sync from public Git repositories, bootstrap repositories, and uninstall Flux without affecting reconciled workloads.
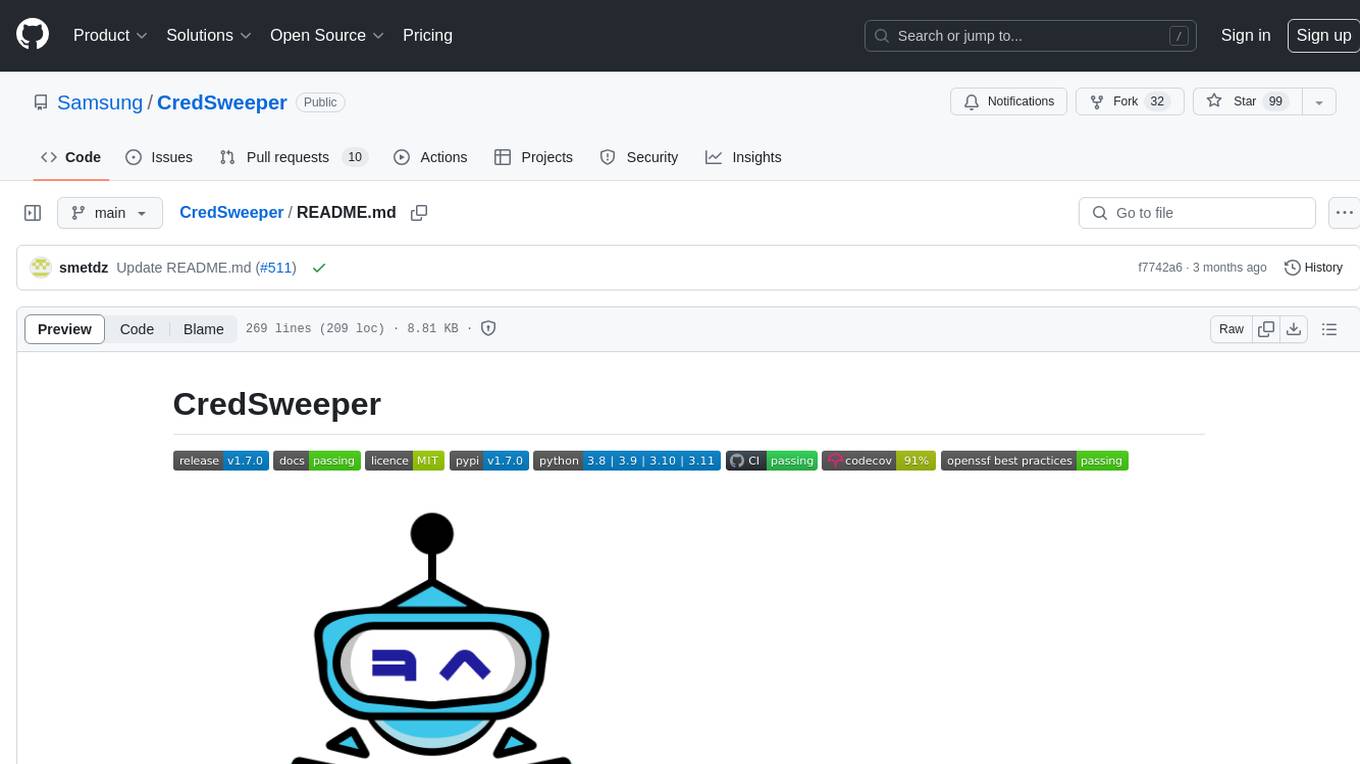
CredSweeper
CredSweeper is a tool designed to detect credentials like tokens, passwords, and API keys in directories or files. It helps users identify potential exposure of sensitive information by scanning lines, filtering, and utilizing an AI model. The tool reports lines containing possible credentials, their location, and the expected type of credential.
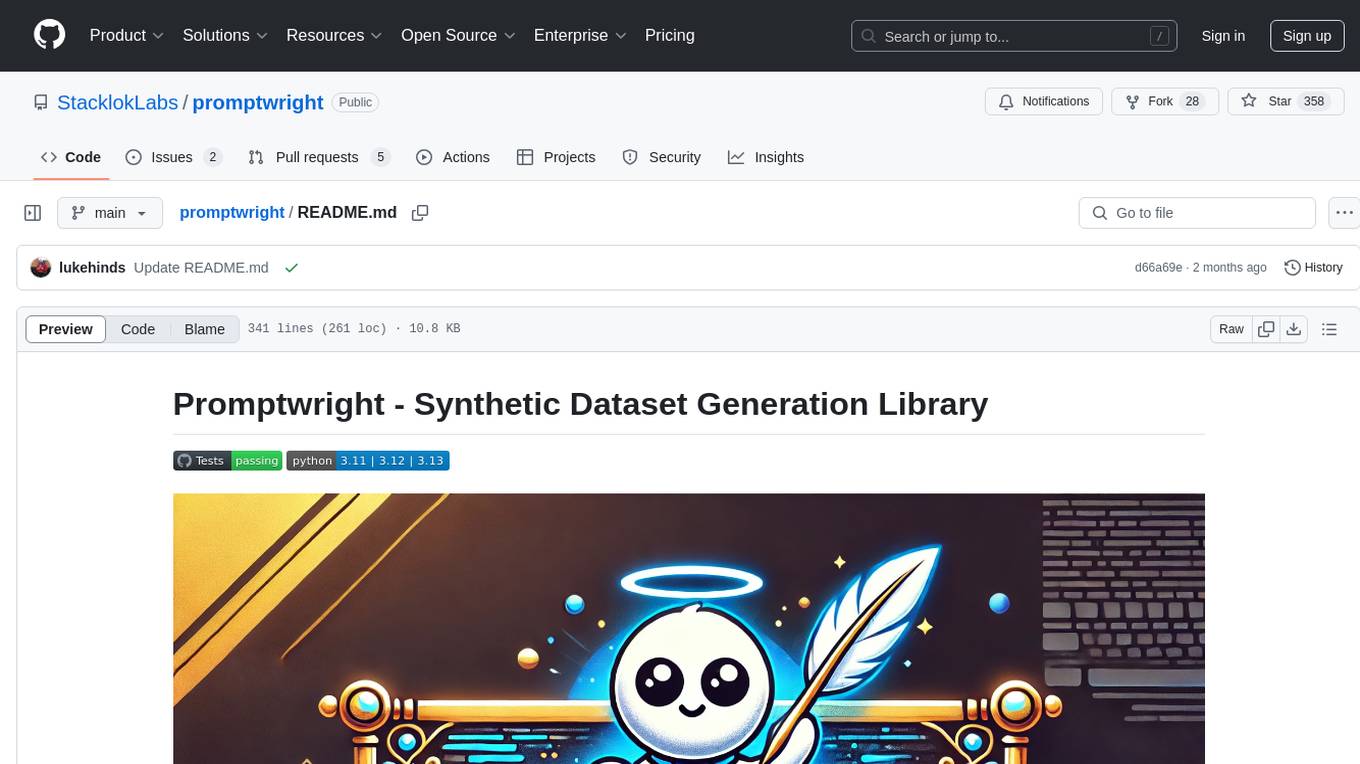
promptwright
Promptwright is a Python library designed for generating large synthetic datasets using a local LLM and various LLM service providers. It offers flexible interfaces for generating prompt-led synthetic datasets. The library supports multiple providers, configurable instructions and prompts, YAML configuration for tasks, command line interface for running tasks, push to Hugging Face Hub for dataset upload, and system message control. Users can define generation tasks using YAML configuration or Python code. Promptwright integrates with LiteLLM to interface with LLM providers and supports automatic dataset upload to Hugging Face Hub.
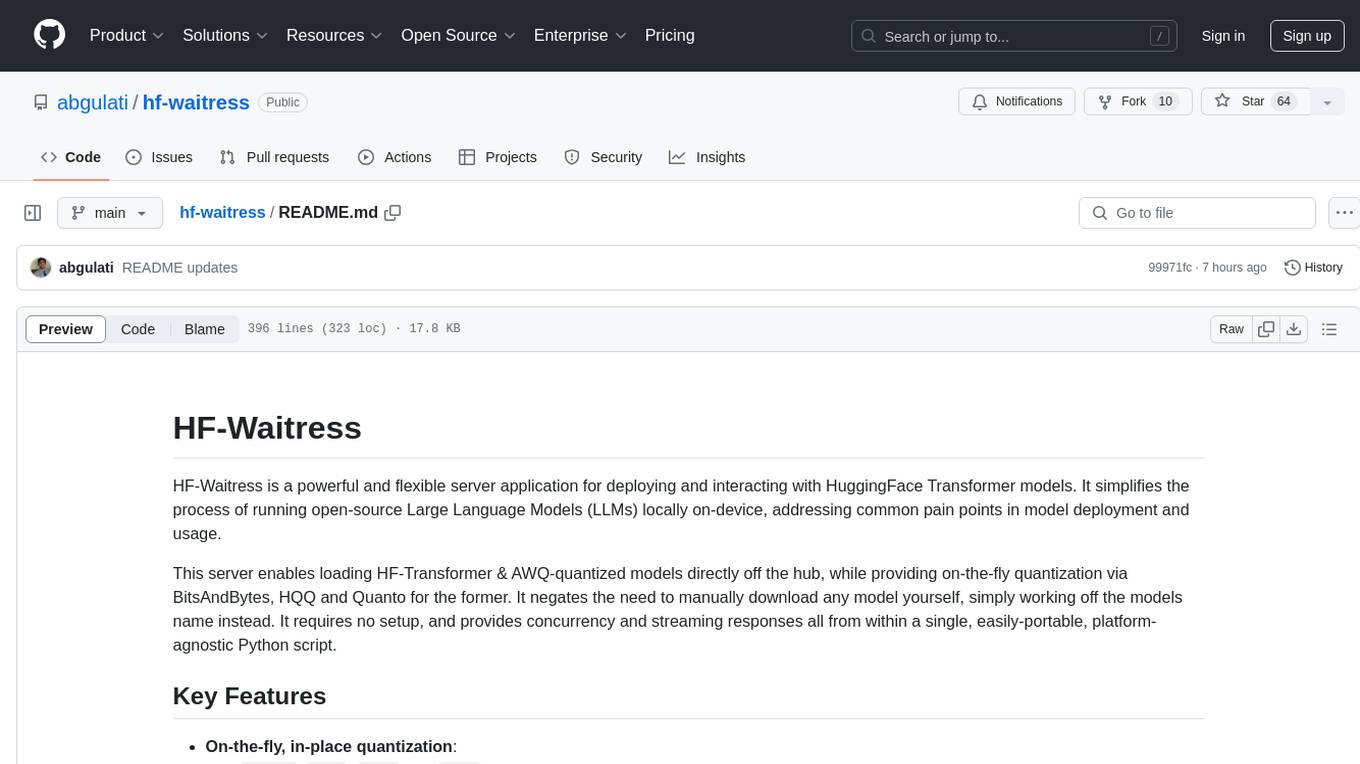
hf-waitress
HF-Waitress is a powerful server application for deploying and interacting with HuggingFace Transformer models. It simplifies running open-source Large Language Models (LLMs) locally on-device, providing on-the-fly quantization via BitsAndBytes, HQQ, and Quanto. It requires no manual model downloads, offers concurrency, streaming responses, and supports various hardware and platforms. The server uses a `config.json` file for easy configuration management and provides detailed error handling and logging.
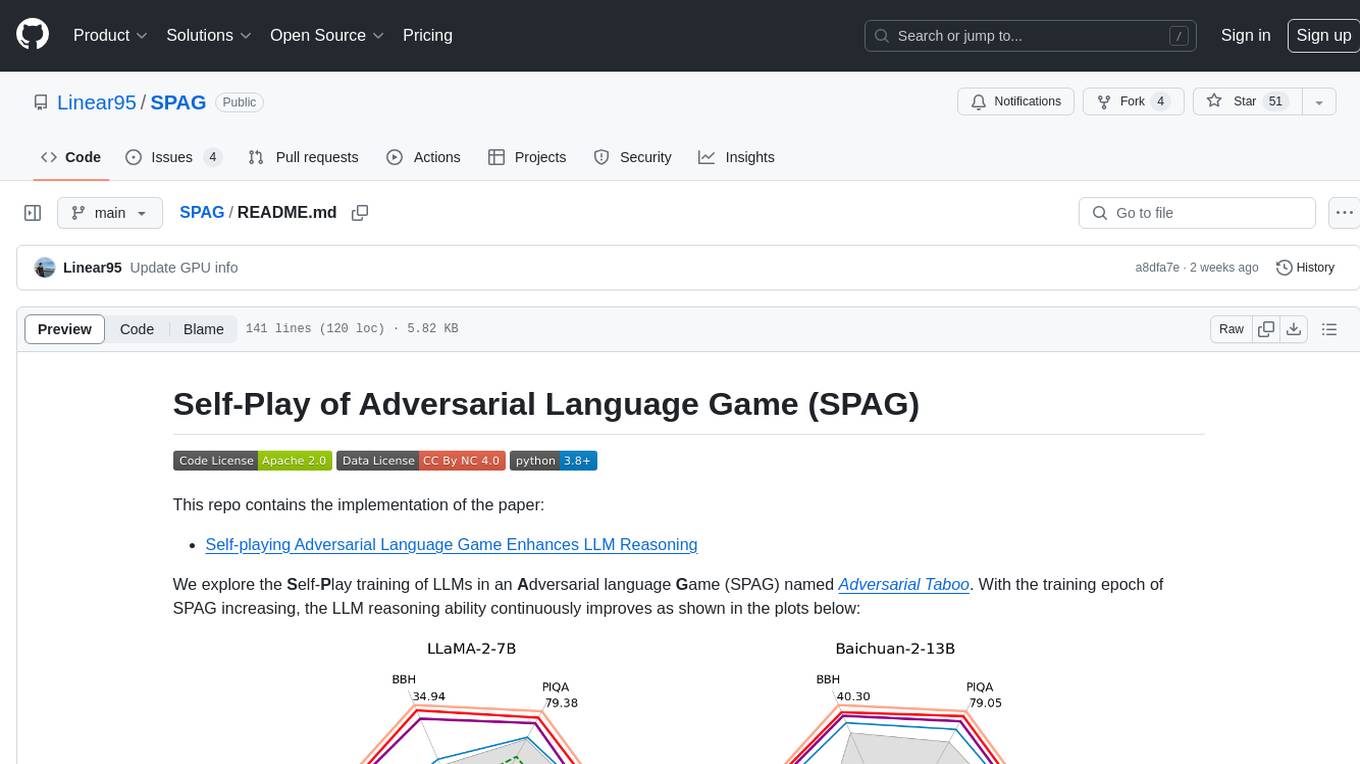
SPAG
This repository contains the implementation of Self-Play of Adversarial Language Game (SPAG) as described in the paper 'Self-playing Adversarial Language Game Enhances LLM Reasoning'. The SPAG involves training Language Models (LLMs) in an adversarial language game called Adversarial Taboo. The repository provides tools for imitation learning, self-play episode collection, and reinforcement learning on game episodes to enhance LLM reasoning abilities. The process involves training models using GPUs, launching imitation learning, conducting self-play episodes, assigning rewards based on outcomes, and learning the SPAG model through reinforcement learning. Continuous improvements on reasoning benchmarks can be observed by repeating the episode-collection and SPAG-learning processes.
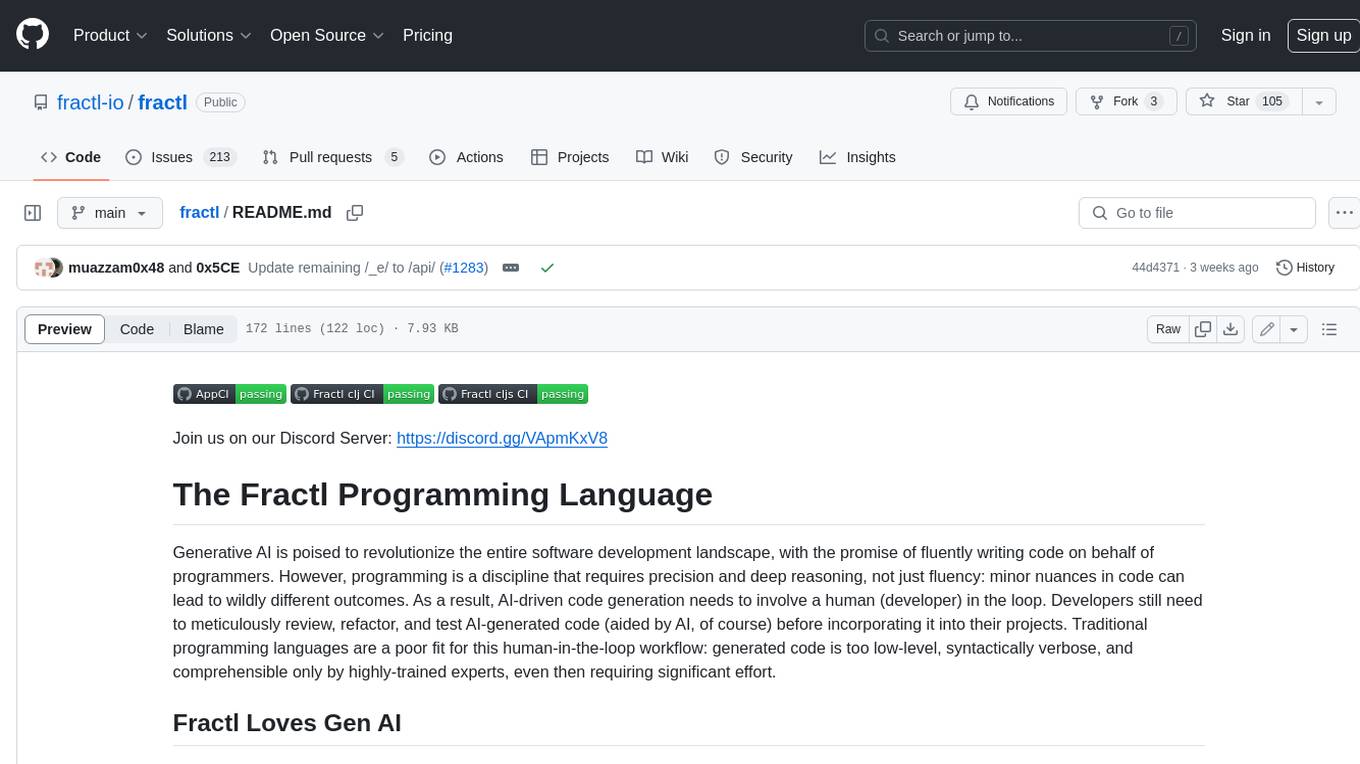
fractl
Fractl is a programming language designed for generative AI, making it easier for developers to work with AI-generated code. It features a data-oriented and declarative syntax, making it a better fit for generative AI-powered code generation. Fractl also bridges the gap between traditional programming and visual building, allowing developers to use multiple ways of building, including traditional coding, visual development, and code generation with generative AI. Key concepts in Fractl include a graph-based hierarchical data model, zero-trust programming, declarative dataflow, resolvers, interceptors, and entity-graph-database mapping.
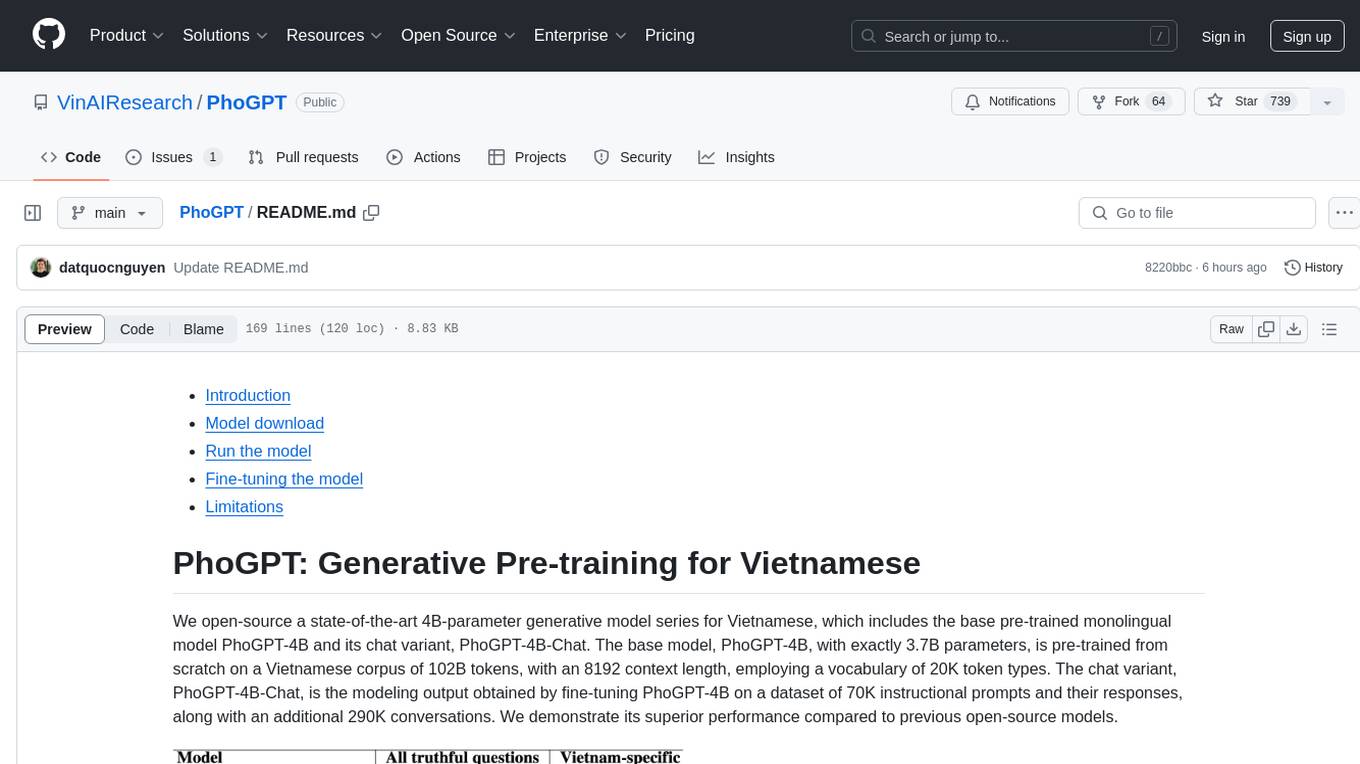
PhoGPT
PhoGPT is an open-source 4B-parameter generative model series for Vietnamese, including the base pre-trained monolingual model PhoGPT-4B and its chat variant, PhoGPT-4B-Chat. PhoGPT-4B is pre-trained from scratch on a Vietnamese corpus of 102B tokens, with an 8192 context length and a vocabulary of 20K token types. PhoGPT-4B-Chat is fine-tuned on instructional prompts and conversations, demonstrating superior performance. Users can run the model with inference engines like vLLM and Text Generation Inference, and fine-tune it using llm-foundry. However, PhoGPT has limitations in reasoning, coding, and mathematics tasks, and may generate harmful or biased responses.
For similar tasks
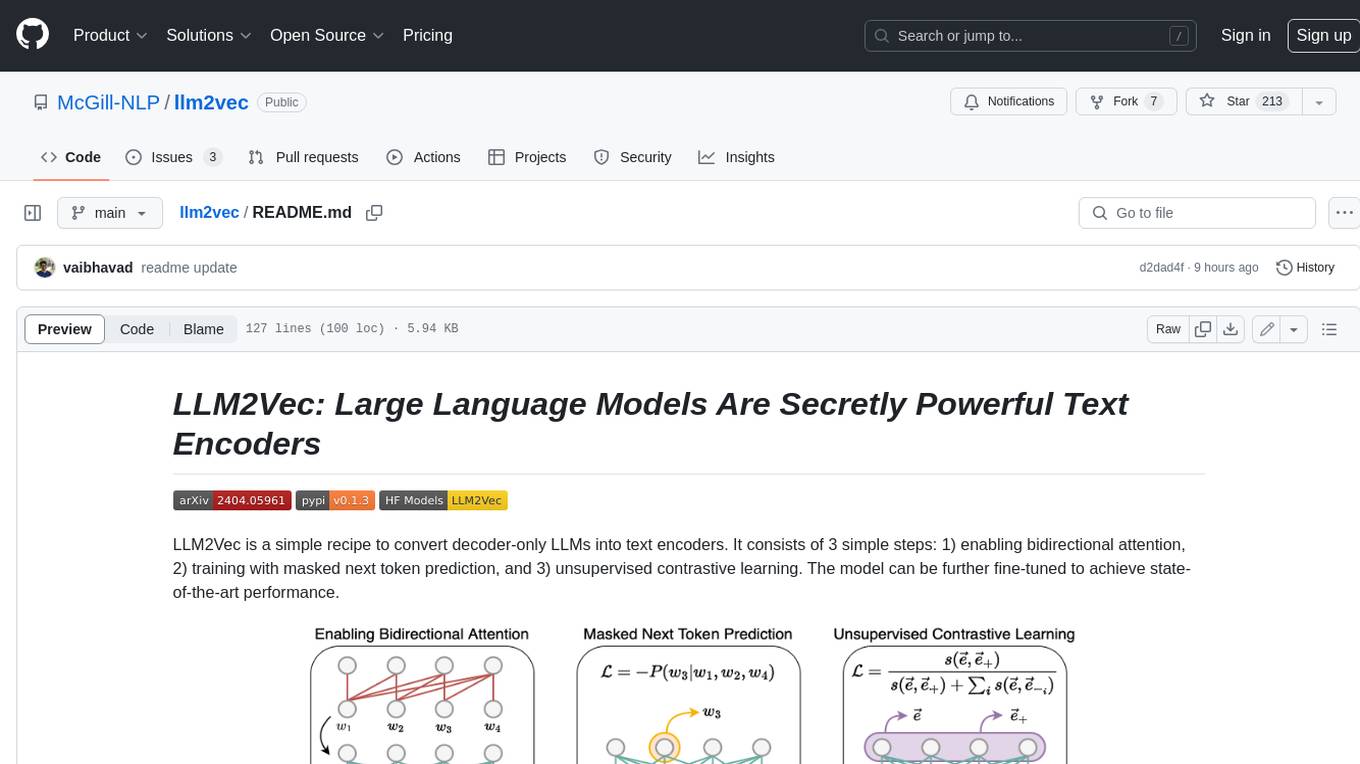
llm2vec
LLM2Vec is a simple recipe to convert decoder-only LLMs into text encoders. It consists of 3 simple steps: 1) enabling bidirectional attention, 2) training with masked next token prediction, and 3) unsupervised contrastive learning. The model can be further fine-tuned to achieve state-of-the-art performance.
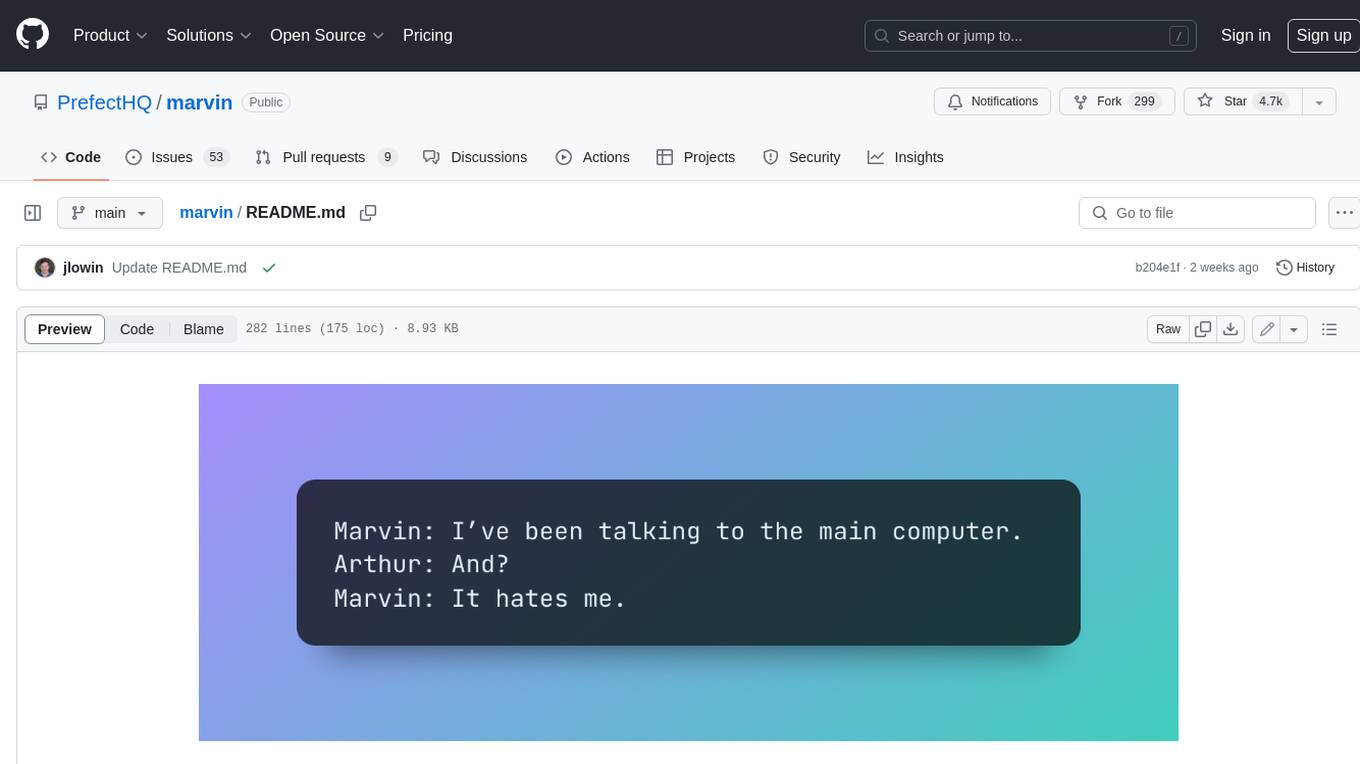
marvin
Marvin is a lightweight AI toolkit for building natural language interfaces that are reliable, scalable, and easy to trust. Each of Marvin's tools is simple and self-documenting, using AI to solve common but complex challenges like entity extraction, classification, and generating synthetic data. Each tool is independent and incrementally adoptable, so you can use them on their own or in combination with any other library. Marvin is also multi-modal, supporting both image and audio generation as well using images as inputs for extraction and classification. Marvin is for developers who care more about _using_ AI than _building_ AI, and we are focused on creating an exceptional developer experience. Marvin users should feel empowered to bring tightly-scoped "AI magic" into any traditional software project with just a few extra lines of code. Marvin aims to merge the best practices for building dependable, observable software with the best practices for building with generative AI into a single, easy-to-use library. It's a serious tool, but we hope you have fun with it. Marvin is open-source, free to use, and made with 💙 by the team at Prefect.
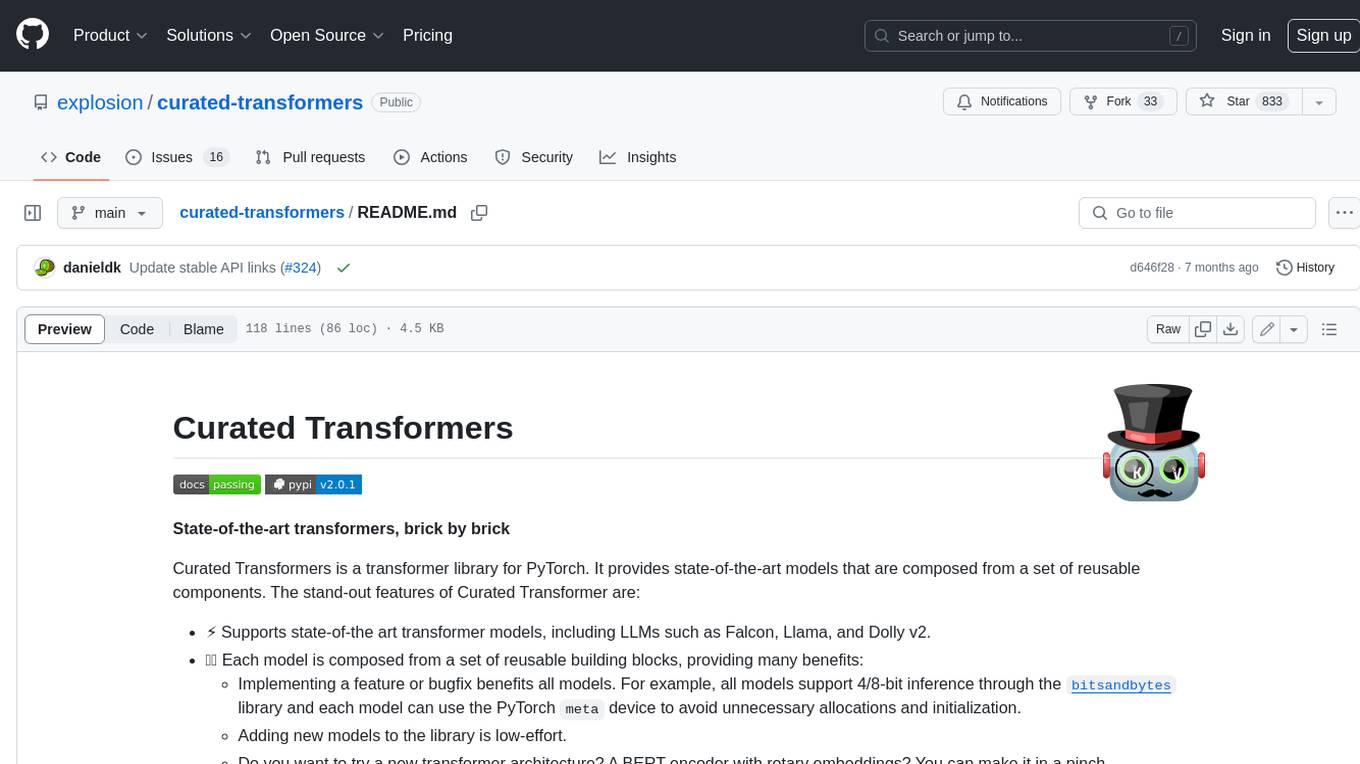
curated-transformers
Curated Transformers is a transformer library for PyTorch that provides state-of-the-art models composed of reusable components. It supports various transformer architectures, including encoders like ALBERT, BERT, and RoBERTa, and decoders like Falcon, Llama, and MPT. The library emphasizes consistent type annotations, minimal dependencies, and ease of use for education and research. It has been production-tested by Explosion and will be the default transformer implementation in spaCy 3.7.
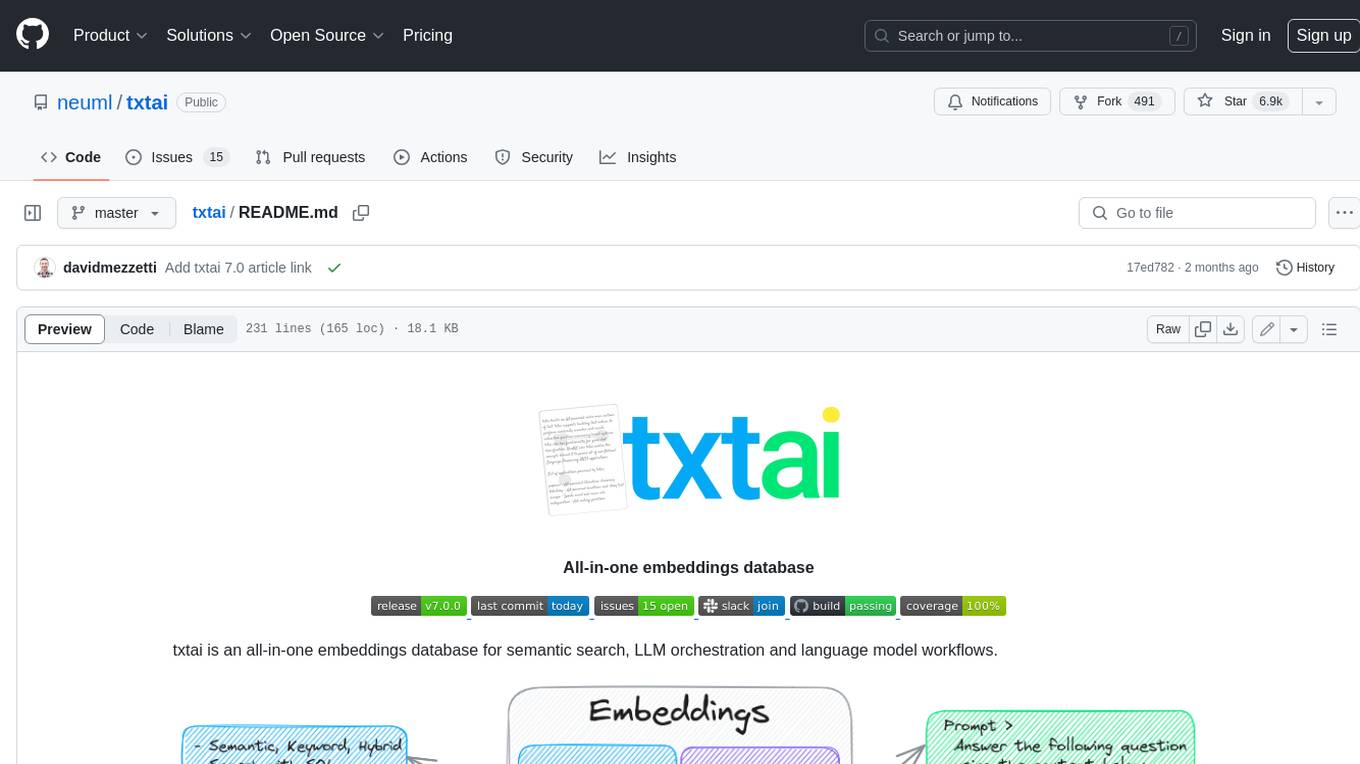
txtai
Txtai is an all-in-one embeddings database for semantic search, LLM orchestration, and language model workflows. It combines vector indexes, graph networks, and relational databases to enable vector search with SQL, topic modeling, retrieval augmented generation, and more. Txtai can stand alone or serve as a knowledge source for large language models (LLMs). Key features include vector search with SQL, object storage, topic modeling, graph analysis, multimodal indexing, embedding creation for various data types, pipelines powered by language models, workflows to connect pipelines, and support for Python, JavaScript, Java, Rust, and Go. Txtai is open-source under the Apache 2.0 license.
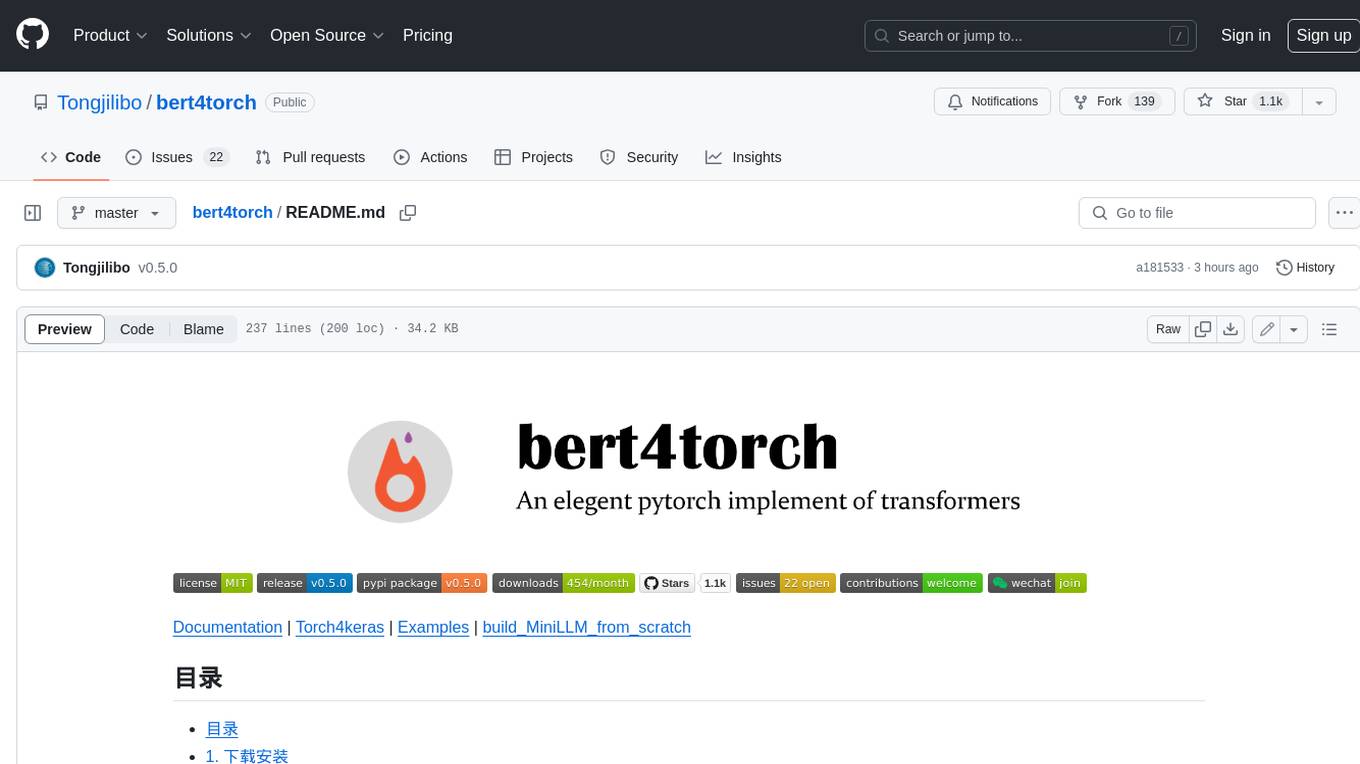
bert4torch
**bert4torch** is a high-level framework for training and deploying transformer models in PyTorch. It provides a simple and efficient API for building, training, and evaluating transformer models, and supports a wide range of pre-trained models, including BERT, RoBERTa, ALBERT, XLNet, and GPT-2. bert4torch also includes a number of useful features, such as data loading, tokenization, and model evaluation. It is a powerful and versatile tool for natural language processing tasks.
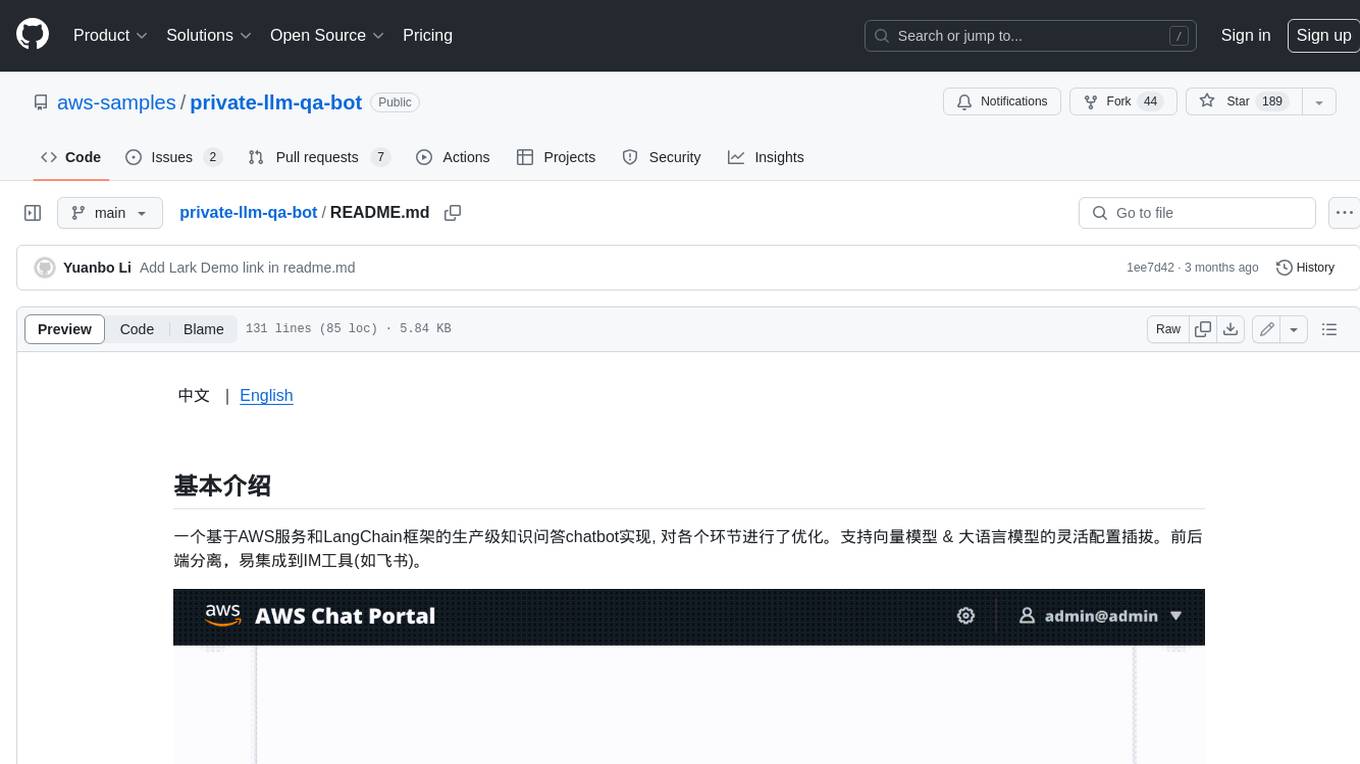
private-llm-qa-bot
This is a production-grade knowledge Q&A chatbot implementation based on AWS services and the LangChain framework, with optimizations at various stages. It supports flexible configuration and plugging of vector models and large language models. The front and back ends are separated, making it easy to integrate with IM tools (such as Feishu).
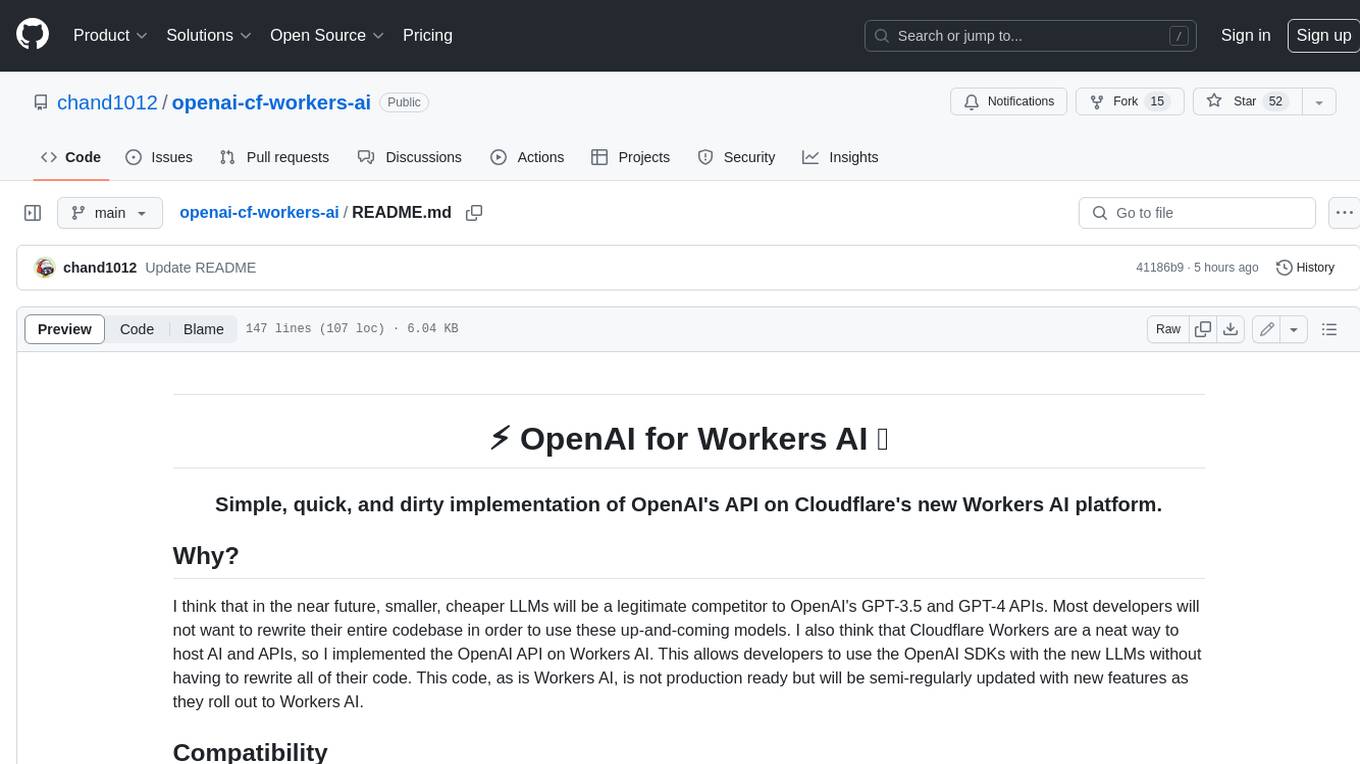
openai-cf-workers-ai
OpenAI for Workers AI is a simple, quick, and dirty implementation of OpenAI's API on Cloudflare's new Workers AI platform. It allows developers to use the OpenAI SDKs with the new LLMs without having to rewrite all of their code. The API currently supports completions, chat completions, audio transcription, embeddings, audio translation, and image generation. It is not production ready but will be semi-regularly updated with new features as they roll out to Workers AI.
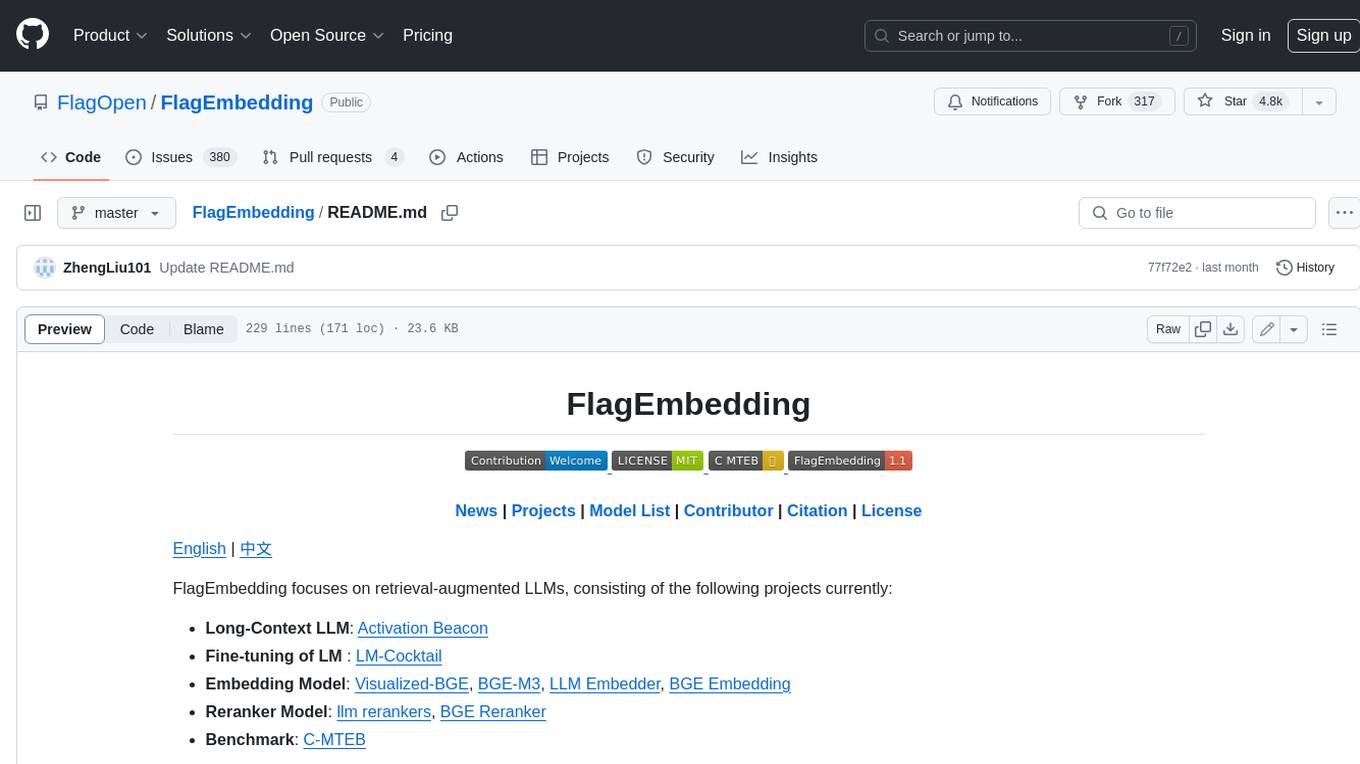
FlagEmbedding
FlagEmbedding focuses on retrieval-augmented LLMs, consisting of the following projects currently: * **Long-Context LLM** : Activation Beacon * **Fine-tuning of LM** : LM-Cocktail * **Embedding Model** : Visualized-BGE, BGE-M3, LLM Embedder, BGE Embedding * **Reranker Model** : llm rerankers, BGE Reranker * **Benchmark** : C-MTEB
For similar jobs
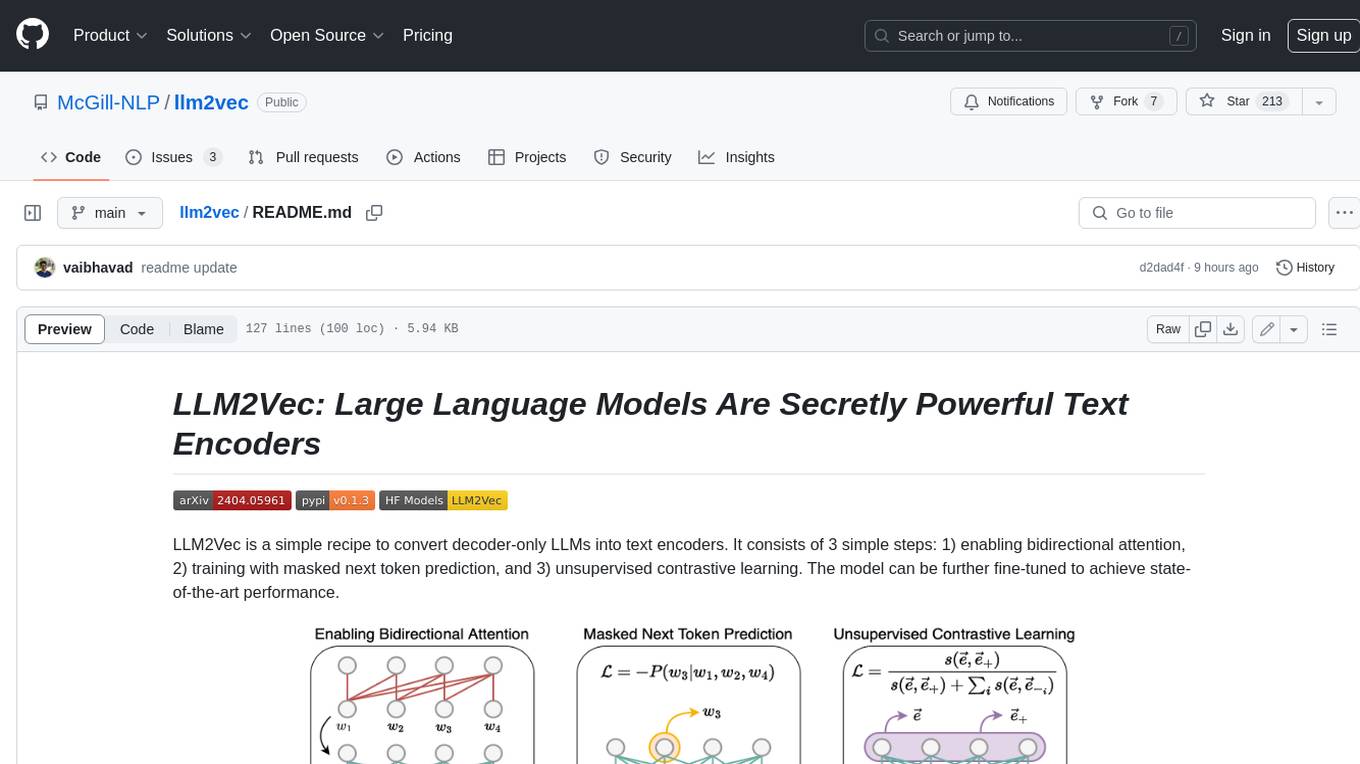
llm2vec
LLM2Vec is a simple recipe to convert decoder-only LLMs into text encoders. It consists of 3 simple steps: 1) enabling bidirectional attention, 2) training with masked next token prediction, and 3) unsupervised contrastive learning. The model can be further fine-tuned to achieve state-of-the-art performance.
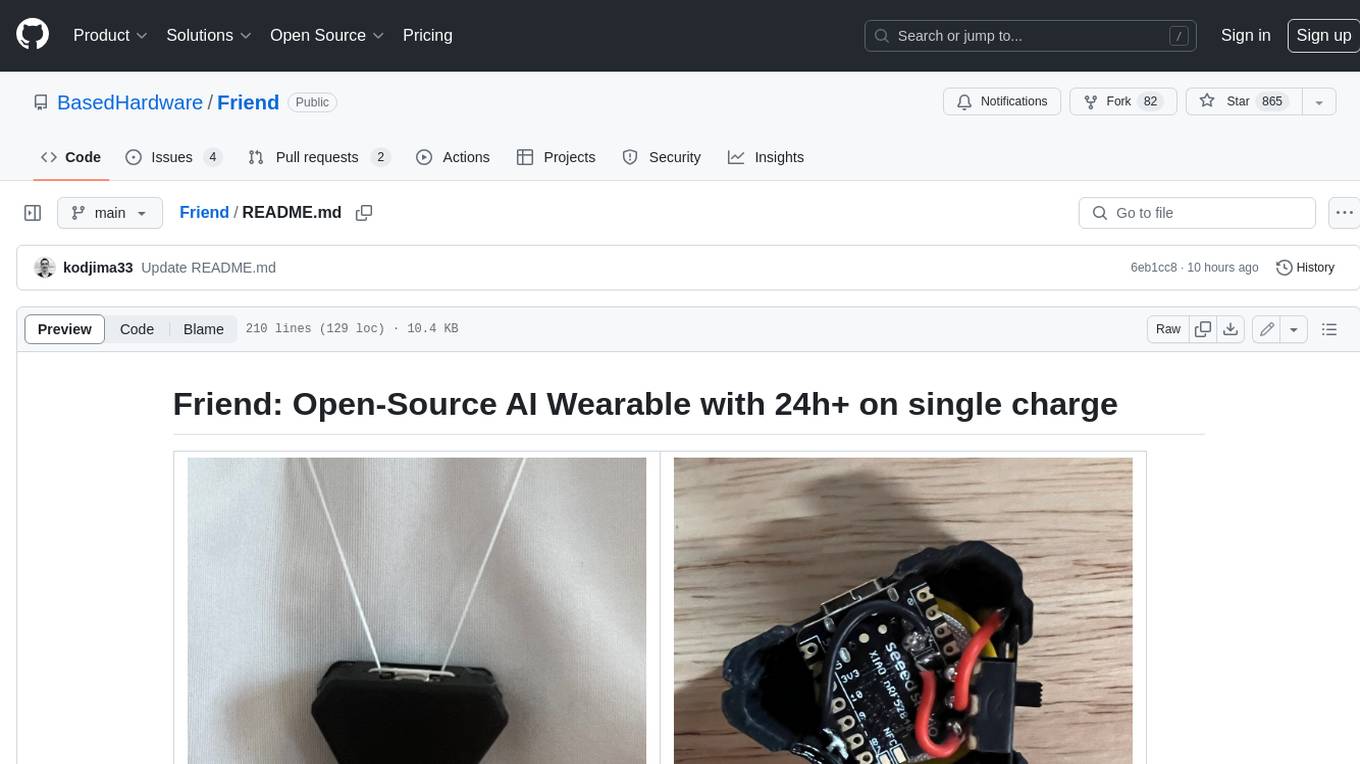
Friend
Friend is an open-source AI wearable device that records everything you say, gives you proactive feedback and advice. It has real-time AI audio processing capabilities, low-powered Bluetooth, open-source software, and a wearable design. The device is designed to be affordable and easy to use, with a total cost of less than $20. To get started, you can clone the repo, choose the version of the app you want to install, and follow the instructions for installing the firmware and assembling the device. Friend is still a prototype project and is provided "as is", without warranty of any kind. Use of the device should comply with all local laws and regulations concerning privacy and data protection.
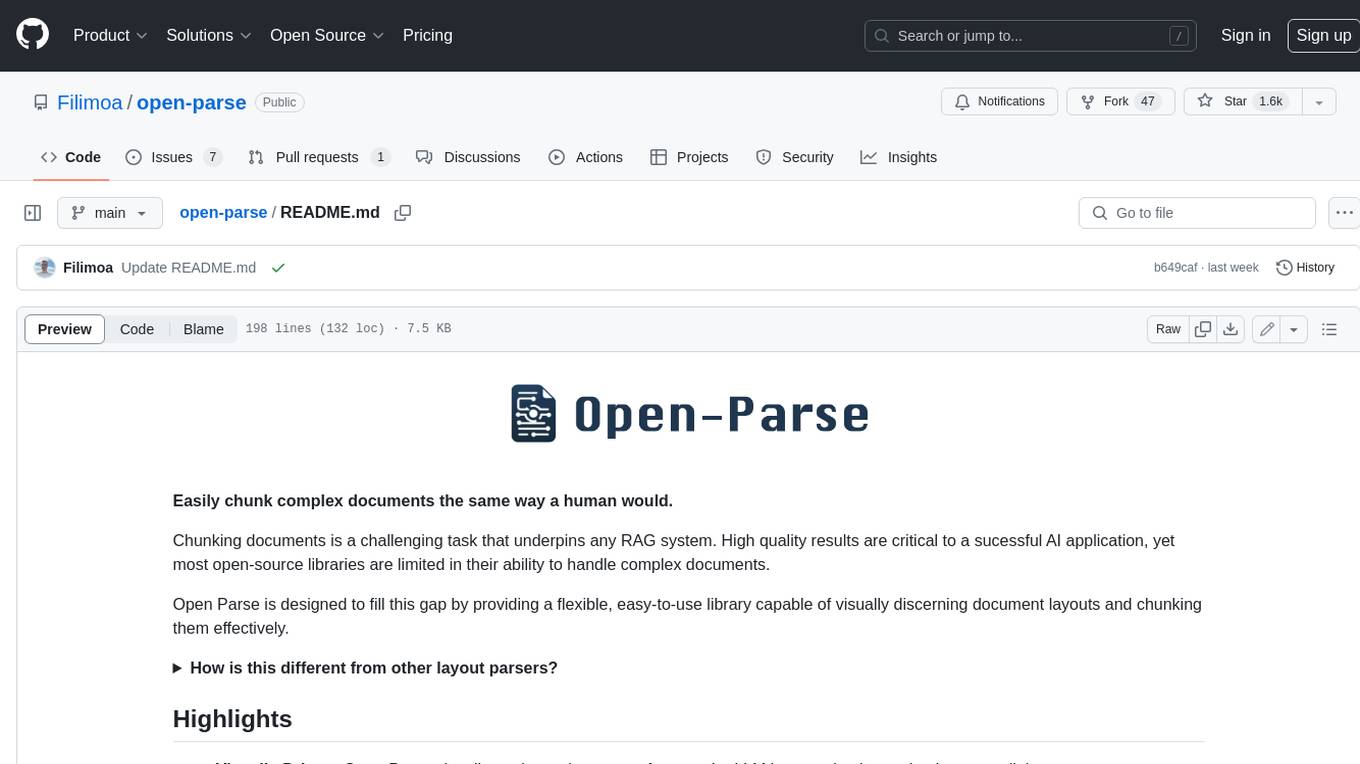
open-parse
Open Parse is a Python library for visually discerning document layouts and chunking them effectively. It is designed to fill the gap in open-source libraries for handling complex documents. Unlike text splitting, which converts a file to raw text and slices it up, Open Parse visually analyzes documents for superior LLM input. It also supports basic markdown for parsing headings, bold, and italics, and has high-precision table support, extracting tables into clean Markdown formats with accuracy that surpasses traditional tools. Open Parse is extensible, allowing users to easily implement their own post-processing steps. It is also intuitive, with great editor support and completion everywhere, making it easy to use and learn.
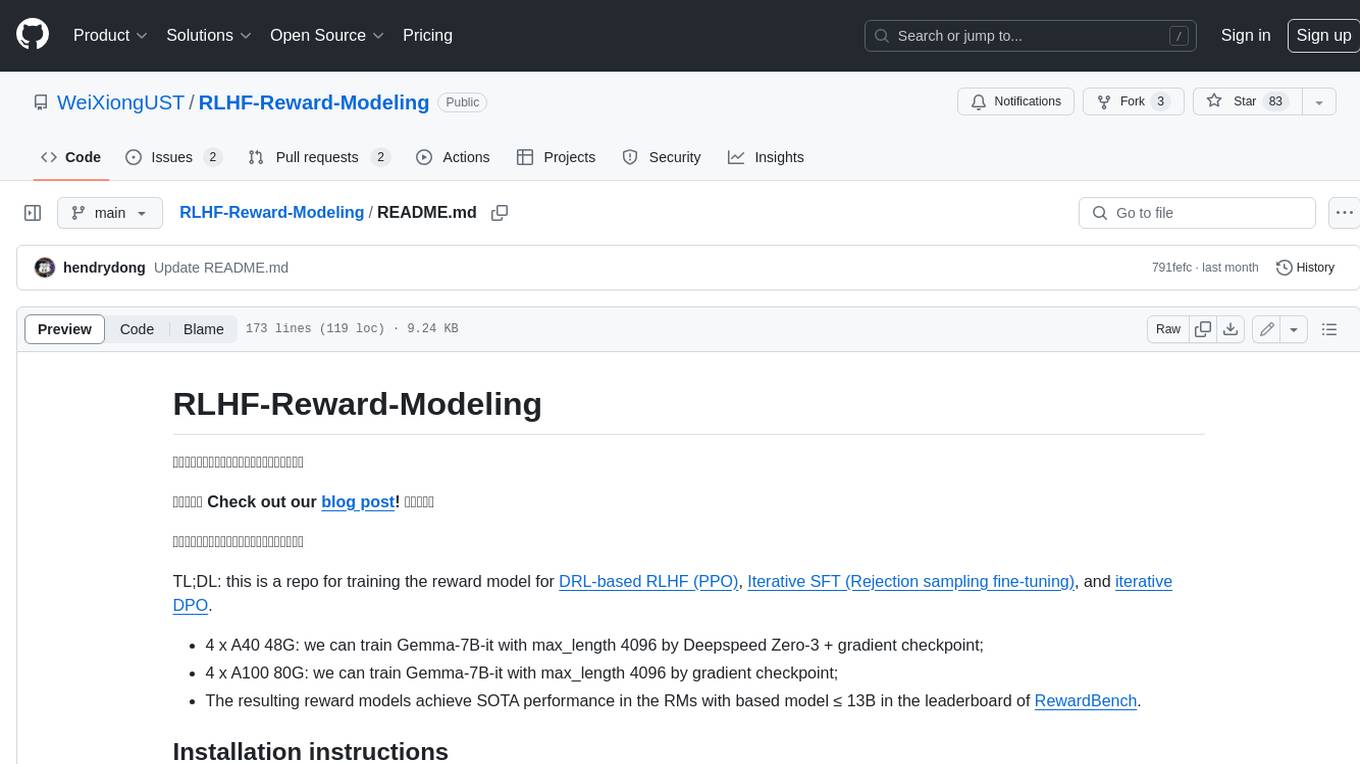
RLHF-Reward-Modeling
This repository contains code for training reward models for Deep Reinforcement Learning-based Reward-modulated Hierarchical Fine-tuning (DRL-based RLHF), Iterative Selection Fine-tuning (Rejection sampling fine-tuning), and iterative Decision Policy Optimization (DPO). The reward models are trained using a Bradley-Terry model based on the Gemma and Mistral language models. The resulting reward models achieve state-of-the-art performance on the RewardBench leaderboard for reward models with base models of up to 13B parameters.
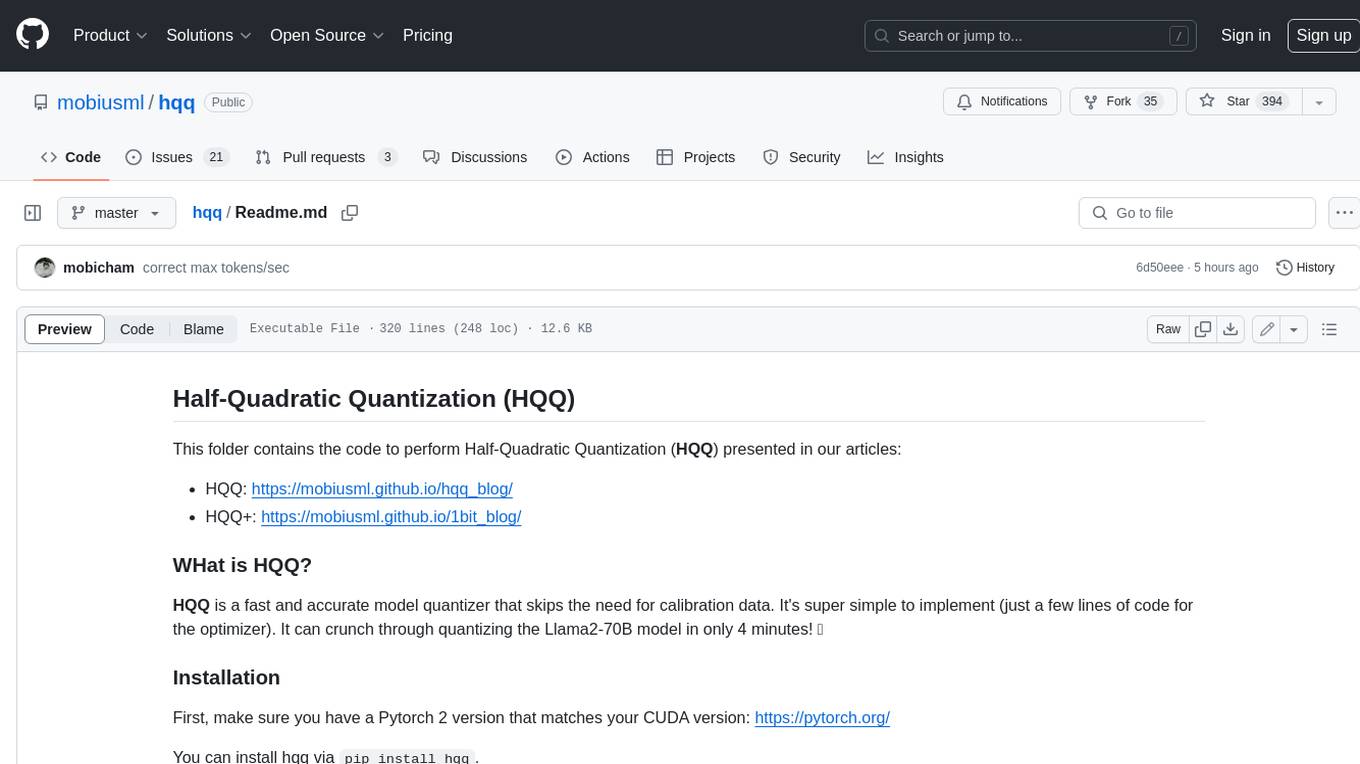
hqq
HQQ is a fast and accurate model quantizer that skips the need for calibration data. It's super simple to implement (just a few lines of code for the optimizer). It can crunch through quantizing the Llama2-70B model in only 4 minutes! 🚀
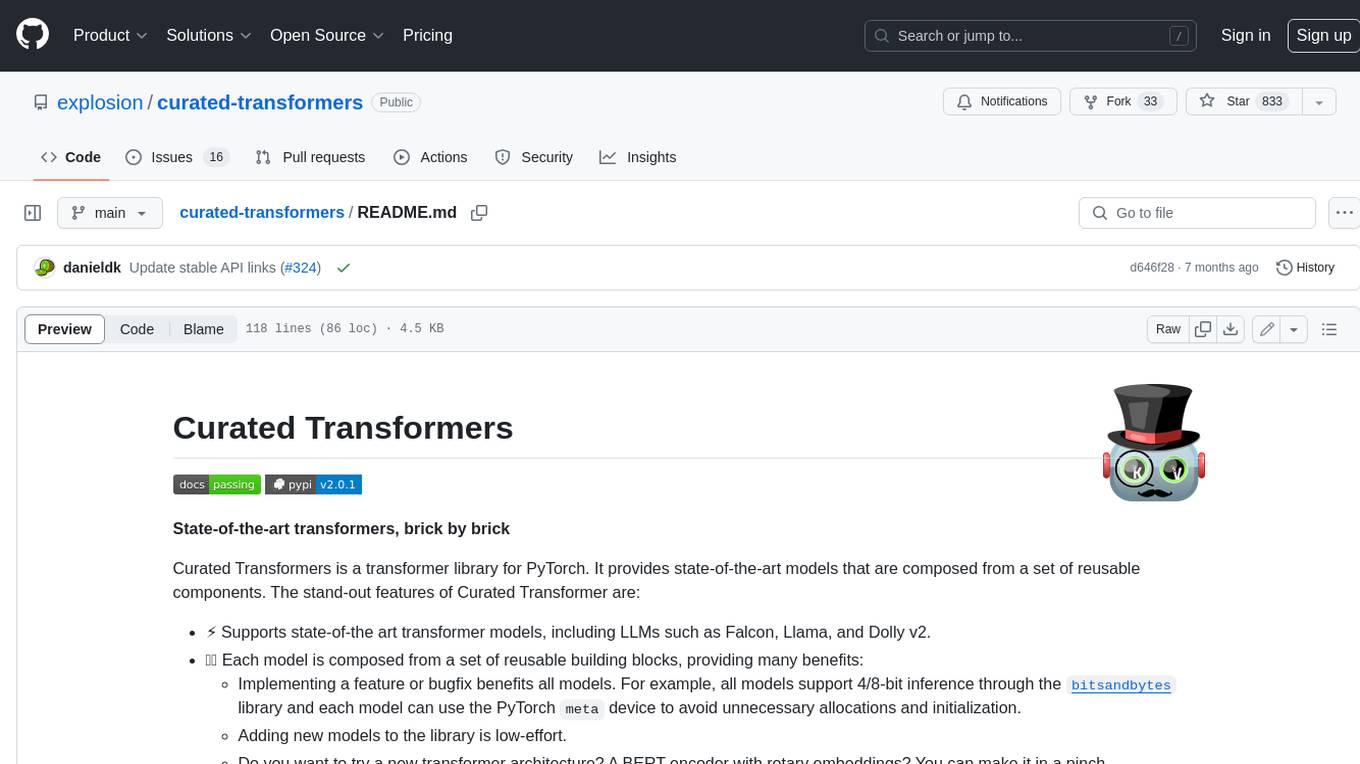
curated-transformers
Curated Transformers is a transformer library for PyTorch that provides state-of-the-art models composed of reusable components. It supports various transformer architectures, including encoders like ALBERT, BERT, and RoBERTa, and decoders like Falcon, Llama, and MPT. The library emphasizes consistent type annotations, minimal dependencies, and ease of use for education and research. It has been production-tested by Explosion and will be the default transformer implementation in spaCy 3.7.
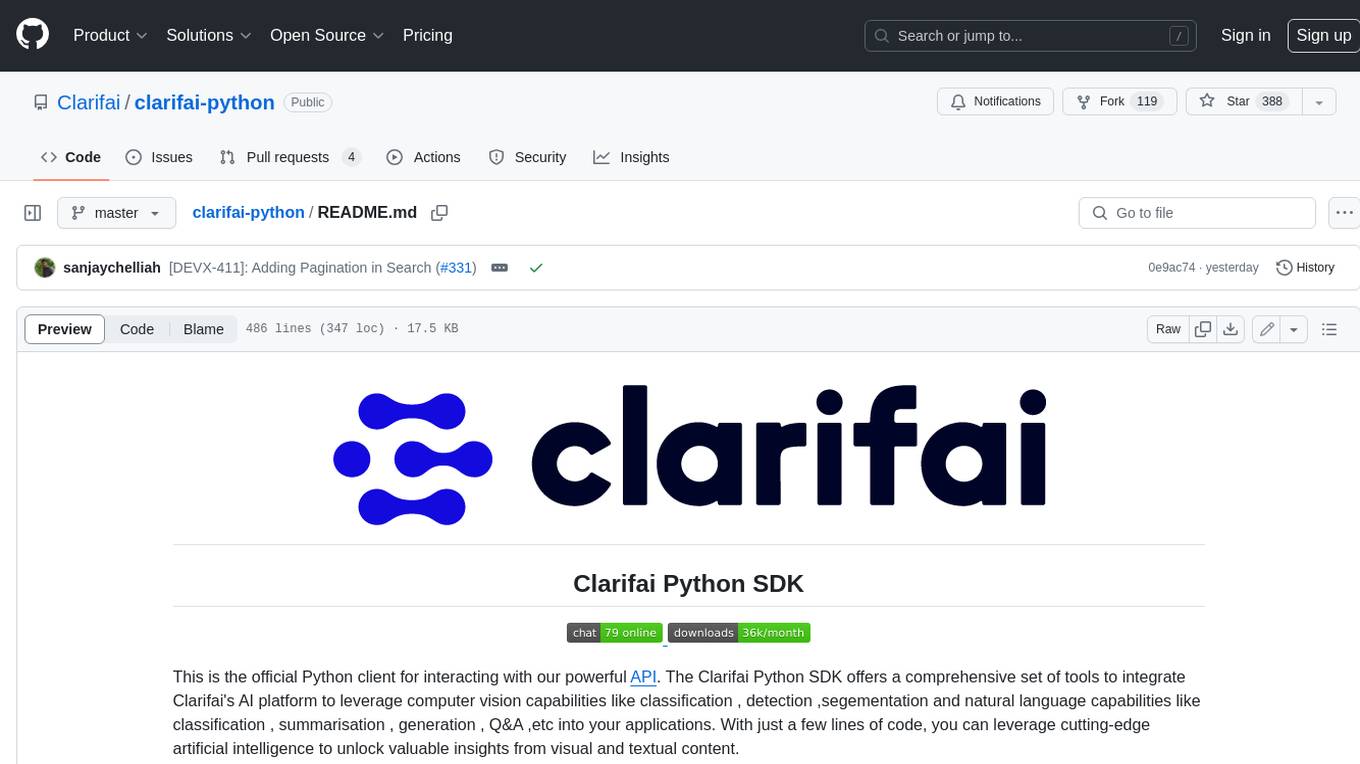
clarifai-python
The Clarifai Python SDK offers a comprehensive set of tools to integrate Clarifai's AI platform to leverage computer vision capabilities like classification , detection ,segementation and natural language capabilities like classification , summarisation , generation , Q&A ,etc into your applications. With just a few lines of code, you can leverage cutting-edge artificial intelligence to unlock valuable insights from visual and textual content.
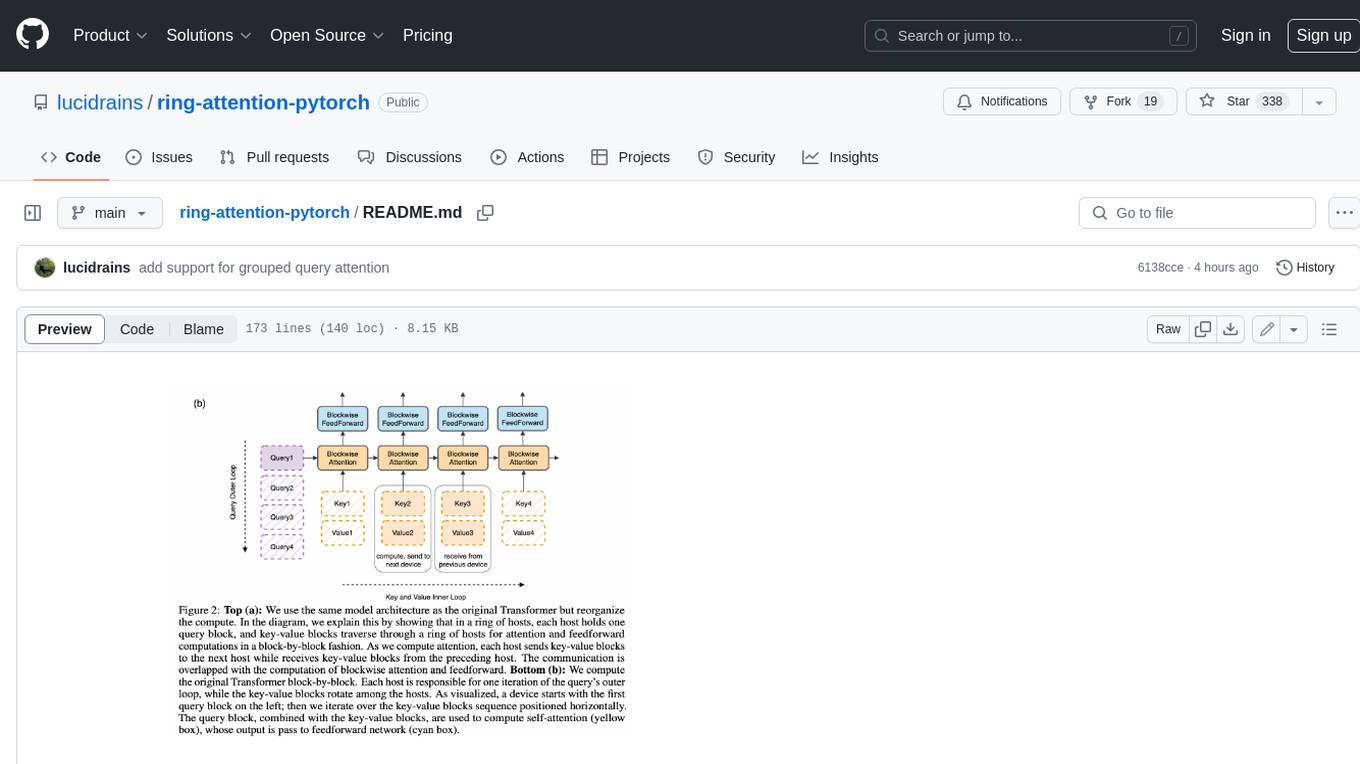
ring-attention-pytorch
This repository contains an implementation of Ring Attention, a technique for processing large sequences in transformers. Ring Attention splits the data across the sequence dimension and applies ring reduce to the processing of the tiles of the attention matrix, similar to flash attention. It also includes support for Striped Attention, a follow-up paper that permutes the sequence for better workload balancing for autoregressive transformers, and grouped query attention, which saves on communication costs during the ring reduce. The repository includes a CUDA version of the flash attention kernel, which is used for the forward and backward passes of the ring attention. It also includes logic for splitting the sequence evenly among ranks, either within the attention function or in the external ring transformer wrapper, and basic test cases with two processes to check for equivalent output and gradients.