Best AI tools for< Encode Text >
5 - AI tool Sites
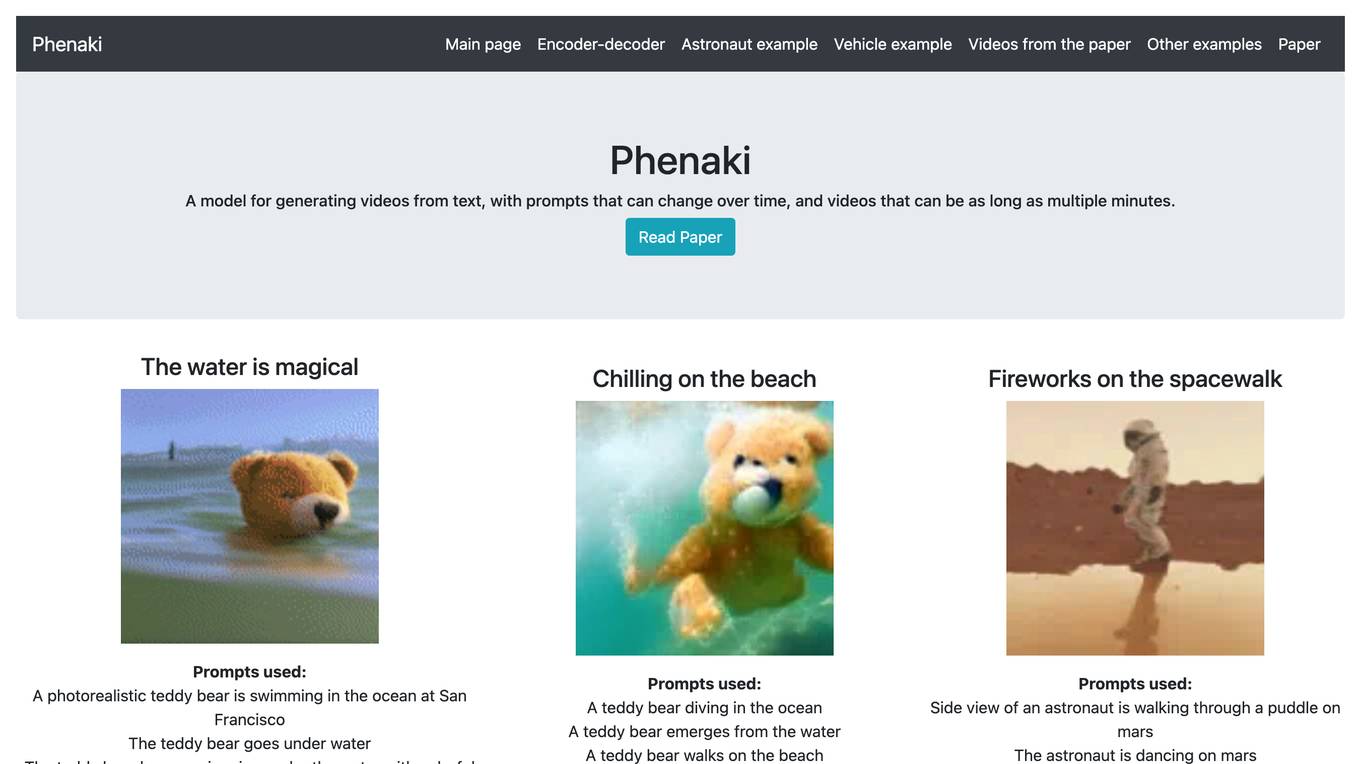
Phenaki
Phenaki is a model capable of generating realistic videos from a sequence of textual prompts. It is particularly challenging to generate videos from text due to the computational cost, limited quantities of high-quality text-video data, and variable length of videos. To address these issues, Phenaki introduces a new causal model for learning video representation, which compresses the video to a small representation of discrete tokens. This tokenizer uses causal attention in time, which allows it to work with variable-length videos. To generate video tokens from text, Phenaki uses a bidirectional masked transformer conditioned on pre-computed text tokens. The generated video tokens are subsequently de-tokenized to create the actual video. To address data issues, Phenaki demonstrates how joint training on a large corpus of image-text pairs as well as a smaller number of video-text examples can result in generalization beyond what is available in the video datasets. Compared to previous video generation methods, Phenaki can generate arbitrarily long videos conditioned on a sequence of prompts (i.e., time-variable text or a story) in an open domain. To the best of our knowledge, this is the first time a paper studies generating videos from time-variable prompts. In addition, the proposed video encoder-decoder outperforms all per-frame baselines currently used in the literature in terms of spatio-temporal quality and the number of tokens per video.
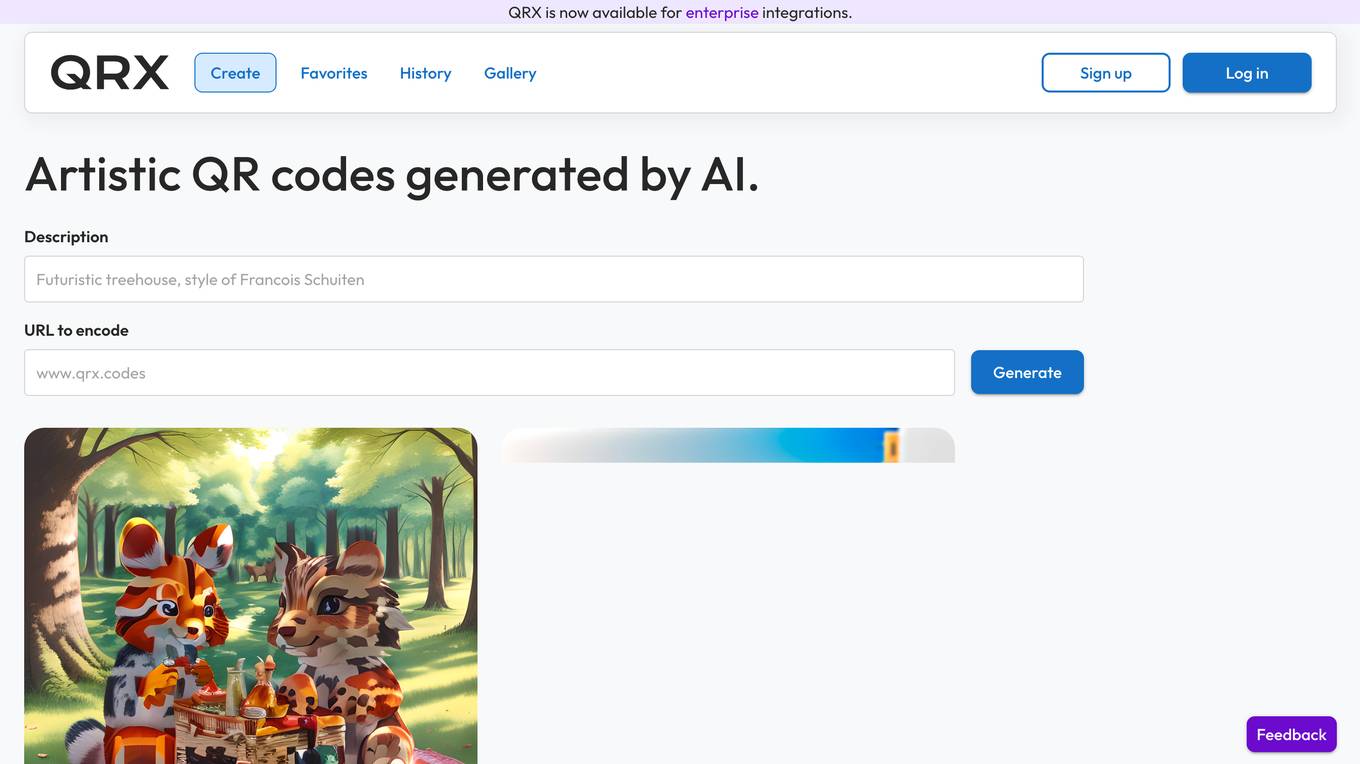
QRX Codes
QRX Codes is an AI tool that generates artistic QR codes. Users can create unique QR codes with images of woodland animals, floating castles, desert scenes, and more. The tool allows for customization of QR codes with premium designs like a dark blue Porsche, Iron Man inspired art, and underground cave themes. QRX is now available for enterprise integrations, offering a creative way to encode URLs and enhance user engagement. The tool is designed to provide a visually appealing and innovative approach to QR code generation.
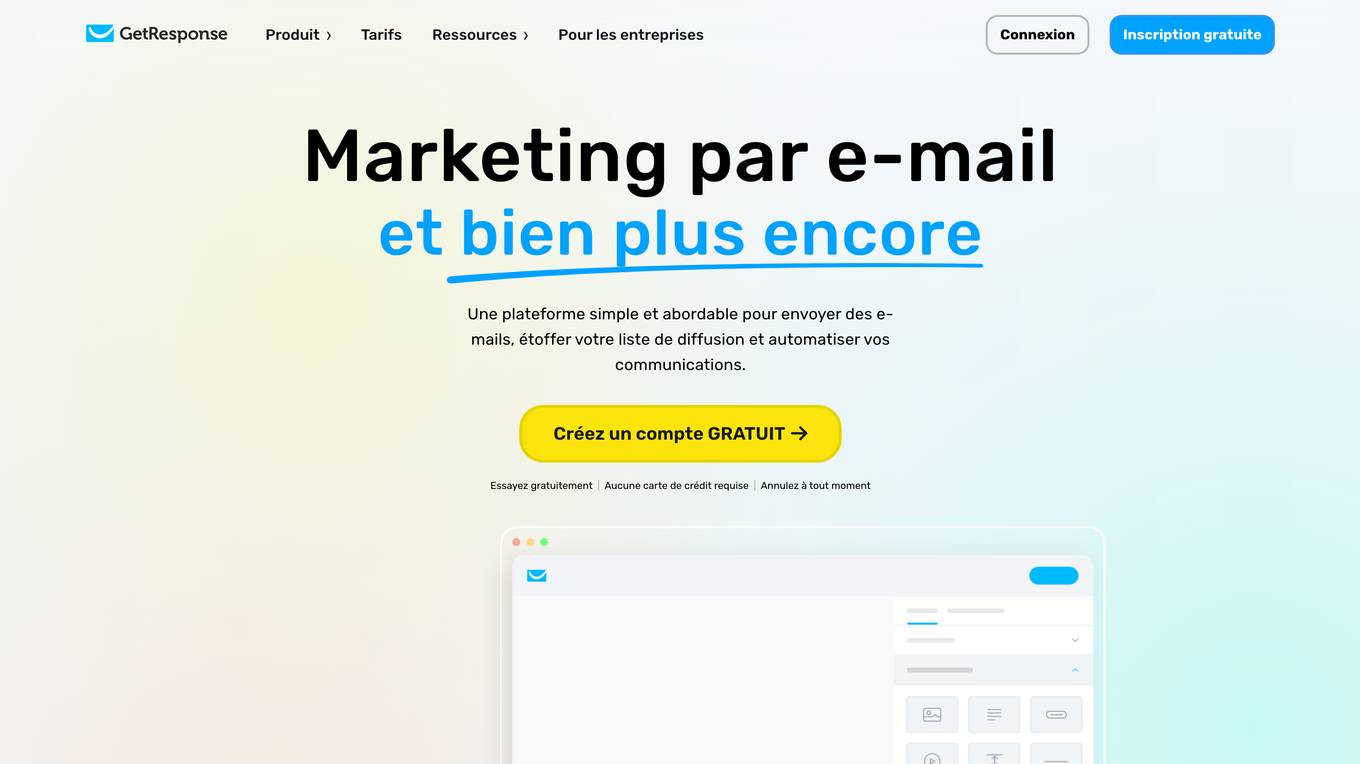
GetResponse
GetResponse is an email marketing and marketing automation platform that helps businesses of all sizes grow their audience, engage with customers, and drive sales. With a suite of powerful tools, including email marketing, landing pages, forms, and automation, GetResponse makes it easy to create and execute effective marketing campaigns. GetResponse also offers a range of integrations with other business tools, making it easy to connect your marketing efforts with your CRM, e-commerce platform, and more.
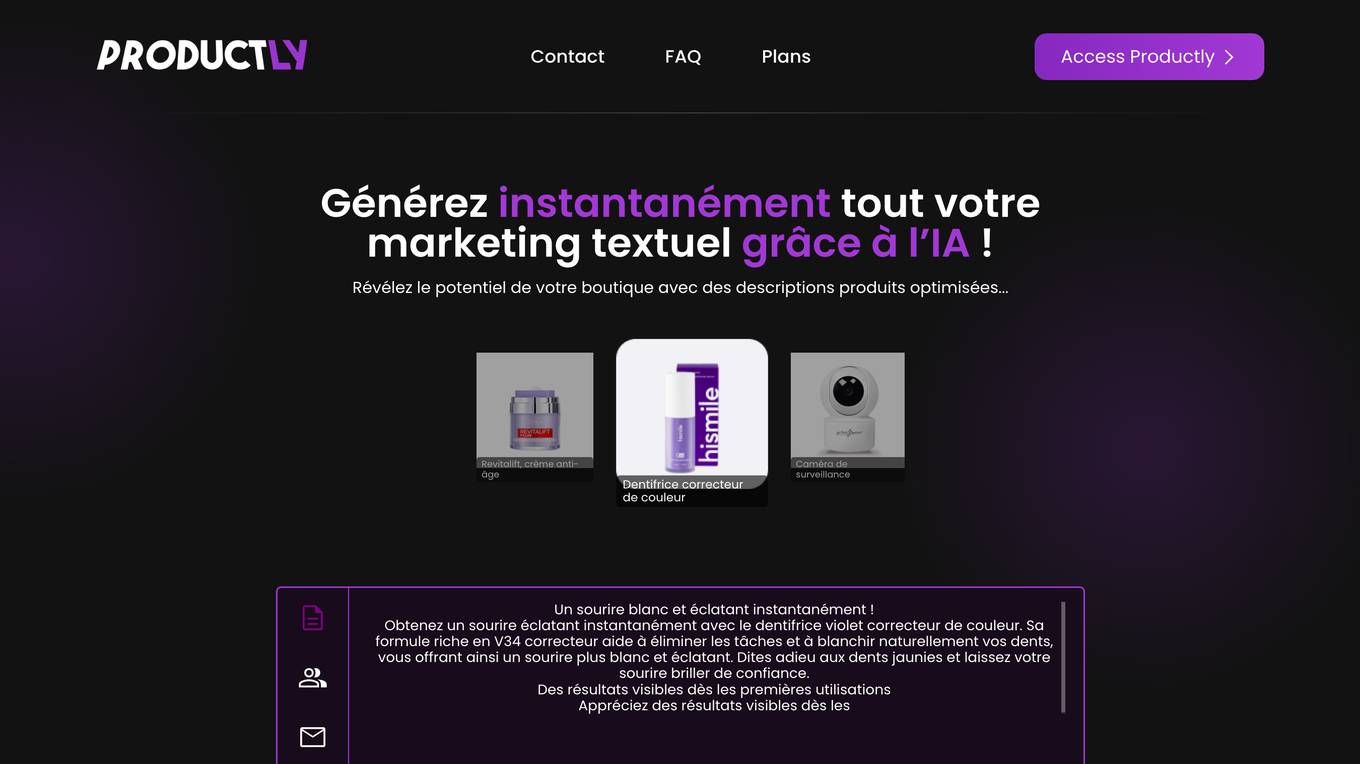
Productly
Productly is an AI-powered sales tool that helps businesses boost their sales performance. It uses machine learning to analyze customer data and identify opportunities for growth. Productly provides personalized recommendations for each customer, helping sales teams close more deals and increase revenue.
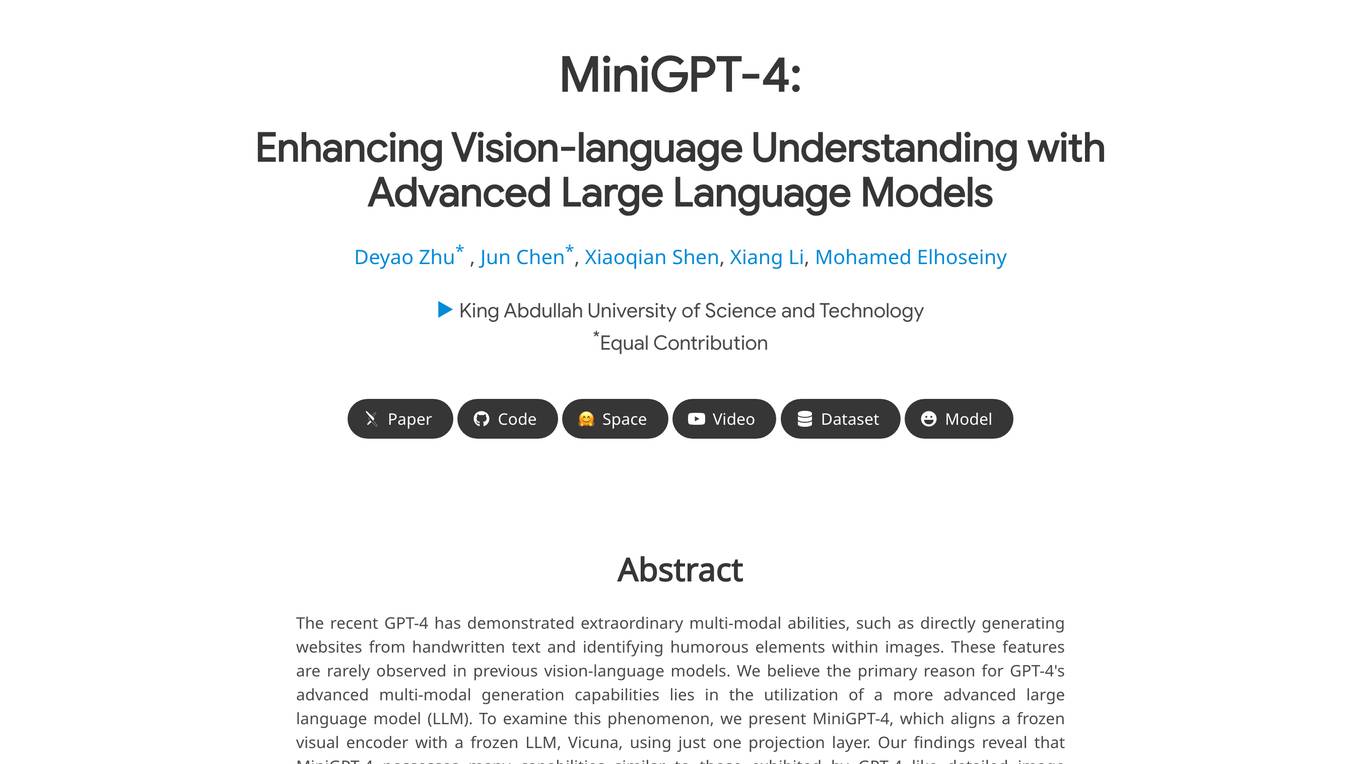
MiniGPT-4
MiniGPT-4 is a powerful AI tool that combines a vision encoder with a large language model (LLM) to enhance vision-language understanding. It can generate detailed image descriptions, create websites from handwritten drafts, write stories and poems inspired by images, provide solutions to problems shown in images, and teach users how to cook based on food photos. MiniGPT-4 is highly computationally efficient and easy to use, making it a valuable tool for a wide range of applications.
20 - Open Source AI Tools
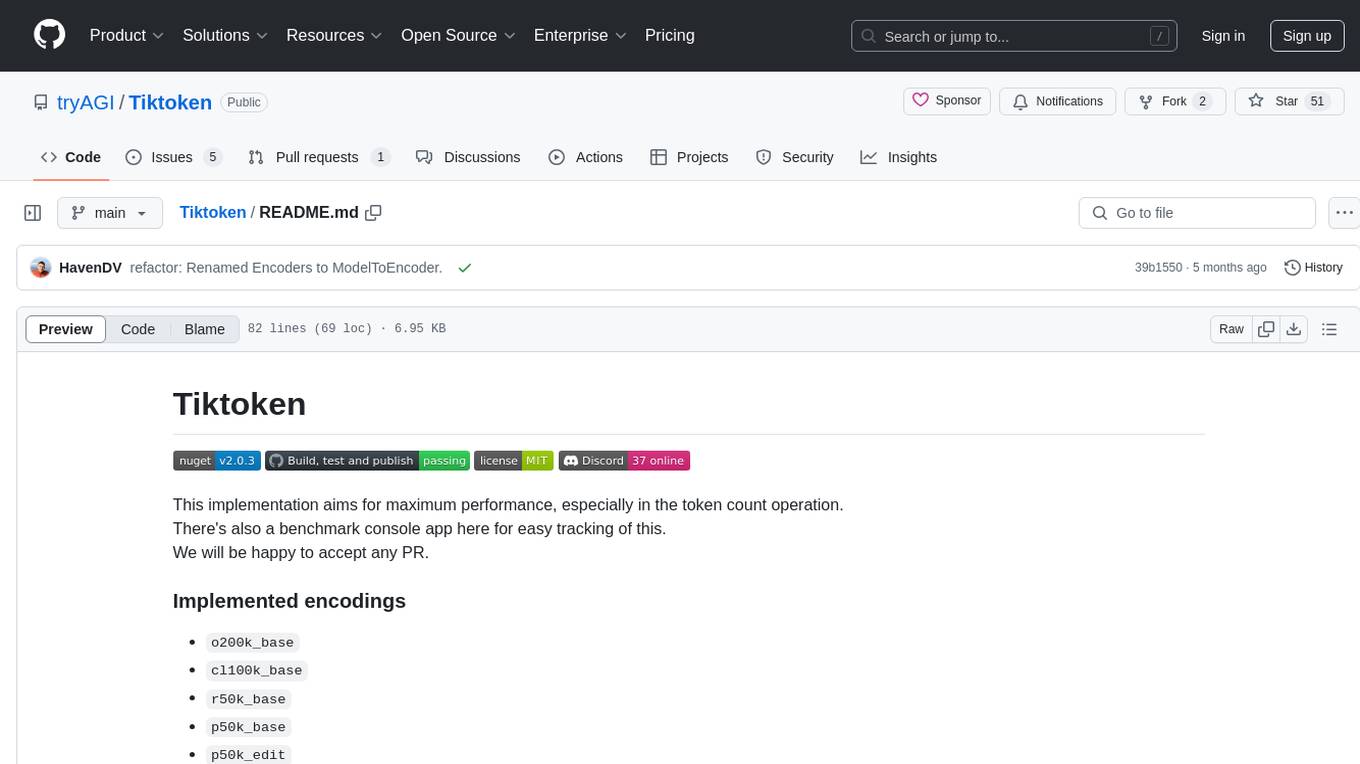
Tiktoken
Tiktoken is a high-performance implementation focused on token count operations. It provides various encodings like o200k_base, cl100k_base, r50k_base, p50k_base, and p50k_edit. Users can easily encode and decode text using the provided API. The repository also includes a benchmark console app for performance tracking. Contributions in the form of PRs are welcome.
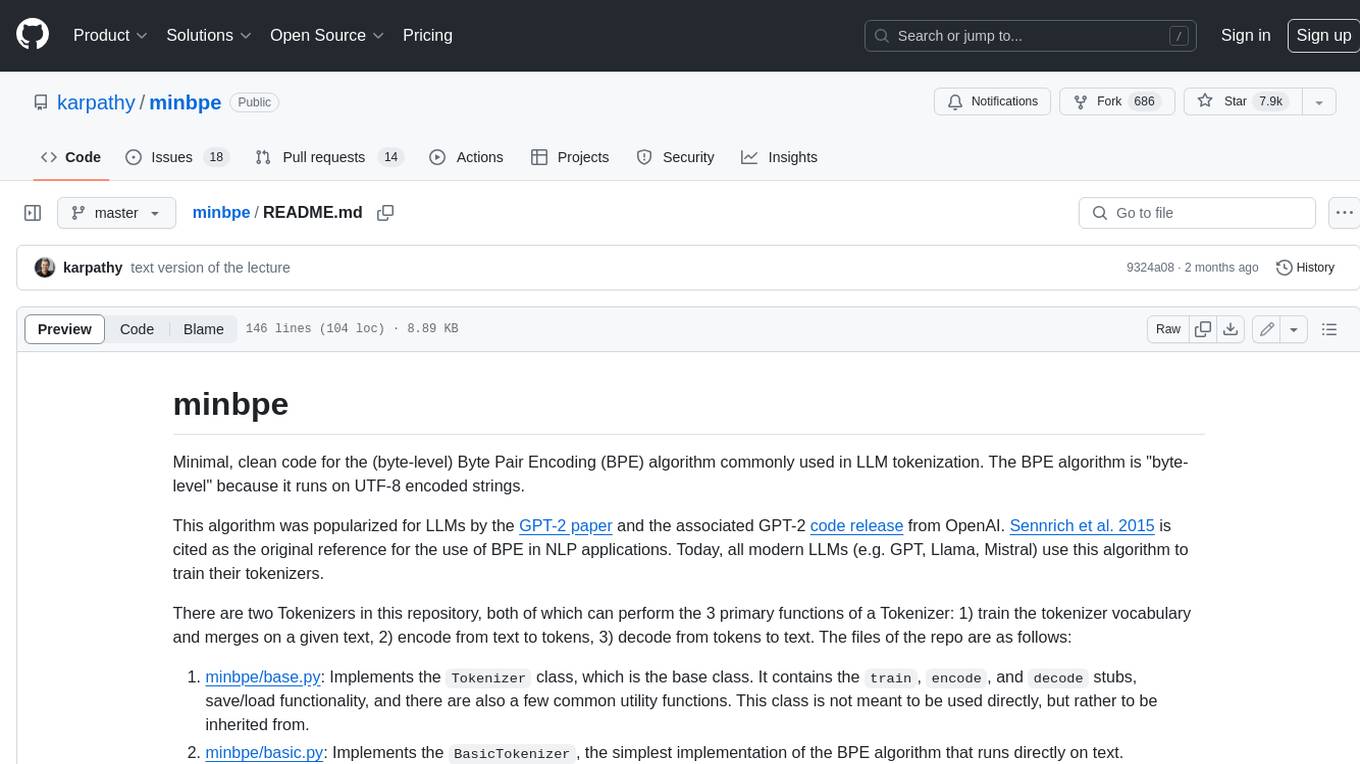
minbpe
This repository contains a minimal, clean code implementation of the Byte Pair Encoding (BPE) algorithm, commonly used in LLM tokenization. The BPE algorithm is "byte-level" because it runs on UTF-8 encoded strings. This algorithm was popularized for LLMs by the GPT-2 paper and the associated GPT-2 code release from OpenAI. Sennrich et al. 2015 is cited as the original reference for the use of BPE in NLP applications. Today, all modern LLMs (e.g. GPT, Llama, Mistral) use this algorithm to train their tokenizers. There are two Tokenizers in this repository, both of which can perform the 3 primary functions of a Tokenizer: 1) train the tokenizer vocabulary and merges on a given text, 2) encode from text to tokens, 3) decode from tokens to text. The files of the repo are as follows: 1. minbpe/base.py: Implements the `Tokenizer` class, which is the base class. It contains the `train`, `encode`, and `decode` stubs, save/load functionality, and there are also a few common utility functions. This class is not meant to be used directly, but rather to be inherited from. 2. minbpe/basic.py: Implements the `BasicTokenizer`, the simplest implementation of the BPE algorithm that runs directly on text. 3. minbpe/regex.py: Implements the `RegexTokenizer` that further splits the input text by a regex pattern, which is a preprocessing stage that splits up the input text by categories (think: letters, numbers, punctuation) before tokenization. This ensures that no merges will happen across category boundaries. This was introduced in the GPT-2 paper and continues to be in use as of GPT-4. This class also handles special tokens, if any. 4. minbpe/gpt4.py: Implements the `GPT4Tokenizer`. This class is a light wrapper around the `RegexTokenizer` (2, above) that exactly reproduces the tokenization of GPT-4 in the tiktoken library. The wrapping handles some details around recovering the exact merges in the tokenizer, and the handling of some unfortunate (and likely historical?) 1-byte token permutations. Finally, the script train.py trains the two major tokenizers on the input text tests/taylorswift.txt (this is the Wikipedia entry for her kek) and saves the vocab to disk for visualization. This script runs in about 25 seconds on my (M1) MacBook. All of the files above are very short and thoroughly commented, and also contain a usage example on the bottom of the file.
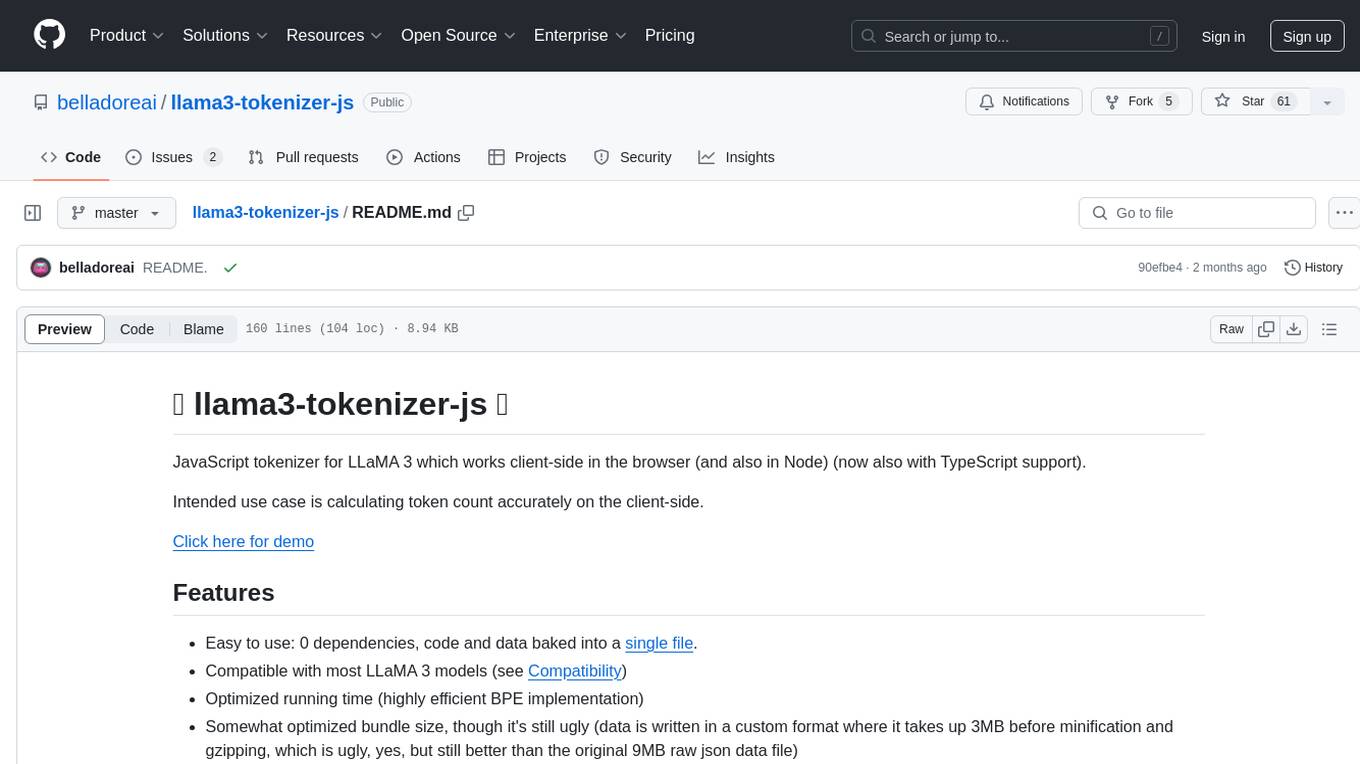
llama3-tokenizer-js
JavaScript tokenizer for LLaMA 3 designed for client-side use in the browser and Node, with TypeScript support. It accurately calculates token count, has 0 dependencies, optimized running time, and somewhat optimized bundle size. Compatible with most LLaMA 3 models. Can encode and decode text, but training is not supported. Pollutes global namespace with `llama3Tokenizer` in the browser. Mostly compatible with LLaMA 3 models released by Facebook in April 2024. Can be adapted for incompatible models by passing custom vocab and merge data. Handles special tokens and fine tunes. Developed by belladore.ai with contributions from xenova, blaze2004, imoneoi, and ConProgramming.
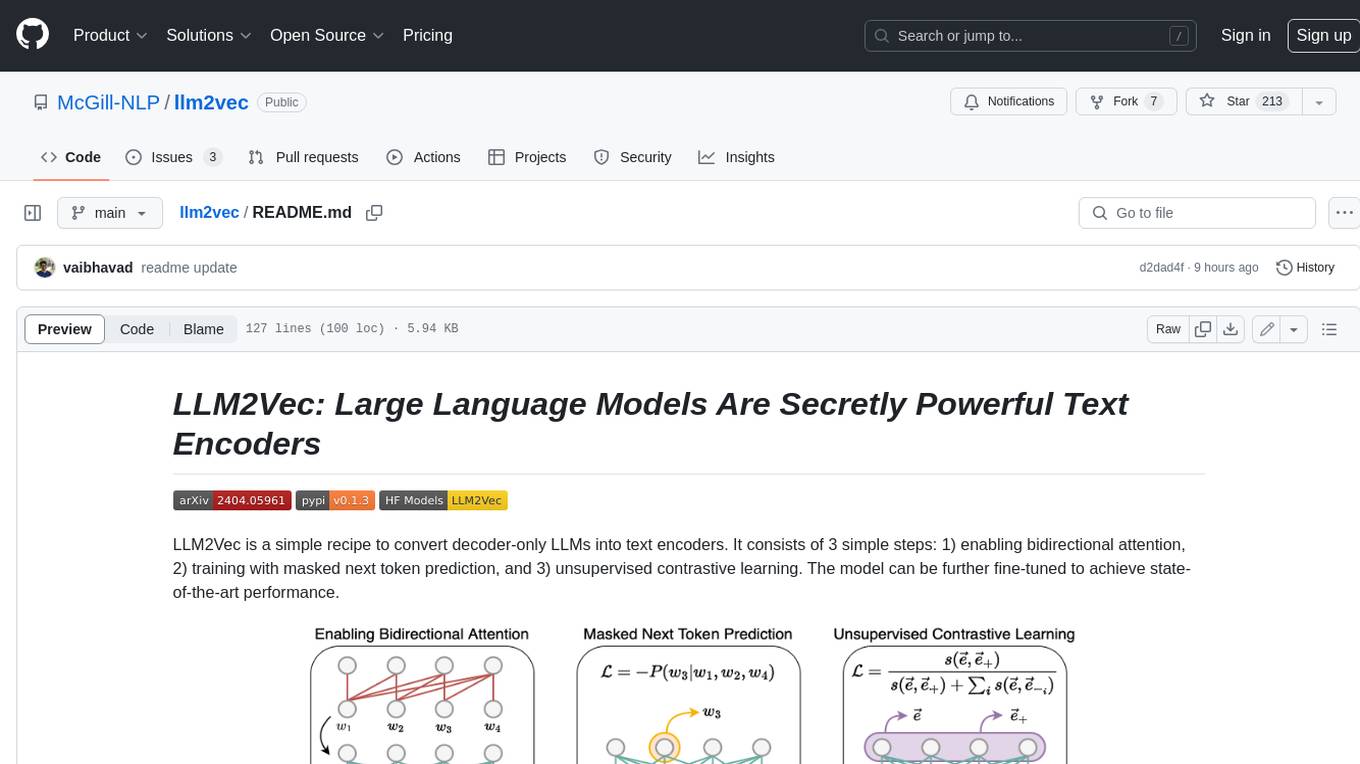
llm2vec
LLM2Vec is a simple recipe to convert decoder-only LLMs into text encoders. It consists of 3 simple steps: 1) enabling bidirectional attention, 2) training with masked next token prediction, and 3) unsupervised contrastive learning. The model can be further fine-tuned to achieve state-of-the-art performance.
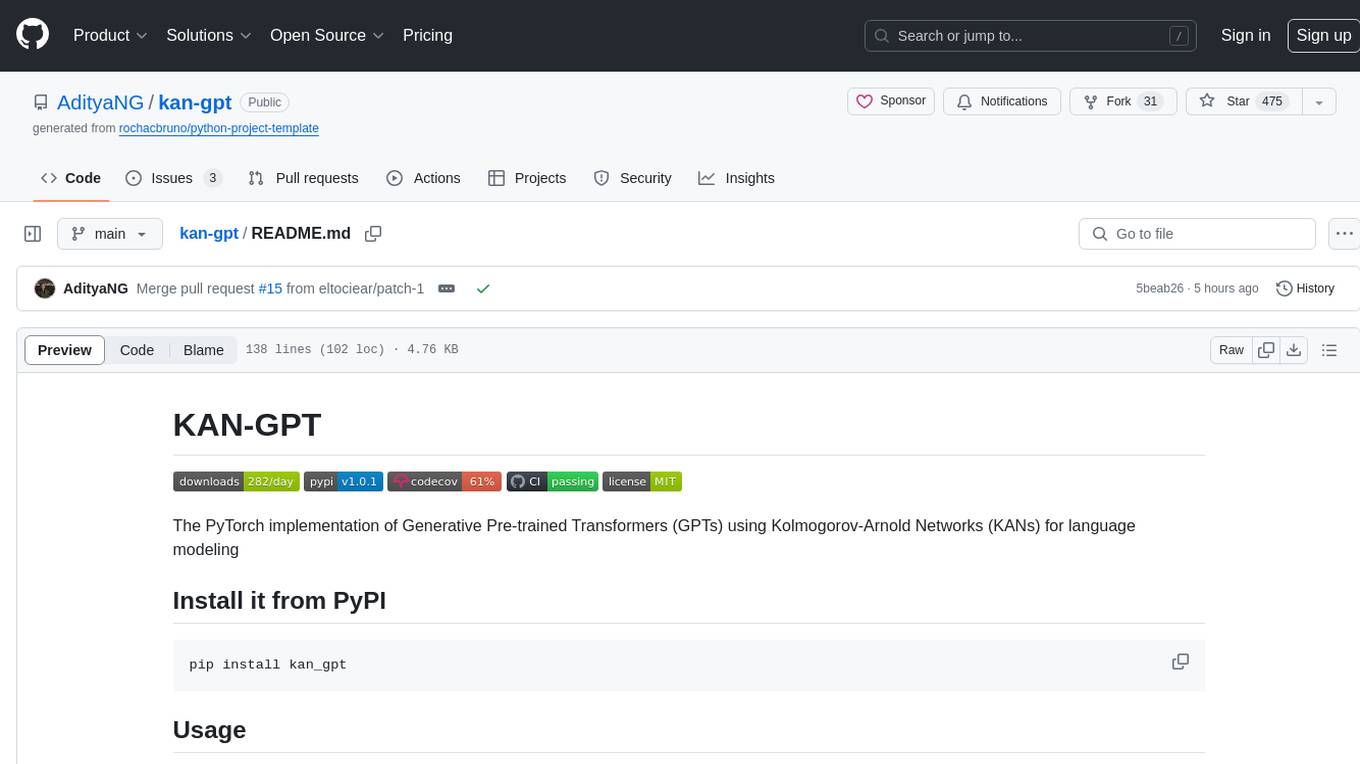
kan-gpt
The KAN-GPT repository is a PyTorch implementation of Generative Pre-trained Transformers (GPTs) using Kolmogorov-Arnold Networks (KANs) for language modeling. It provides a model for generating text based on prompts, with a focus on improving performance compared to traditional MLP-GPT models. The repository includes scripts for training the model, downloading datasets, and evaluating model performance. Development tasks include integrating with other libraries, testing, and documentation.
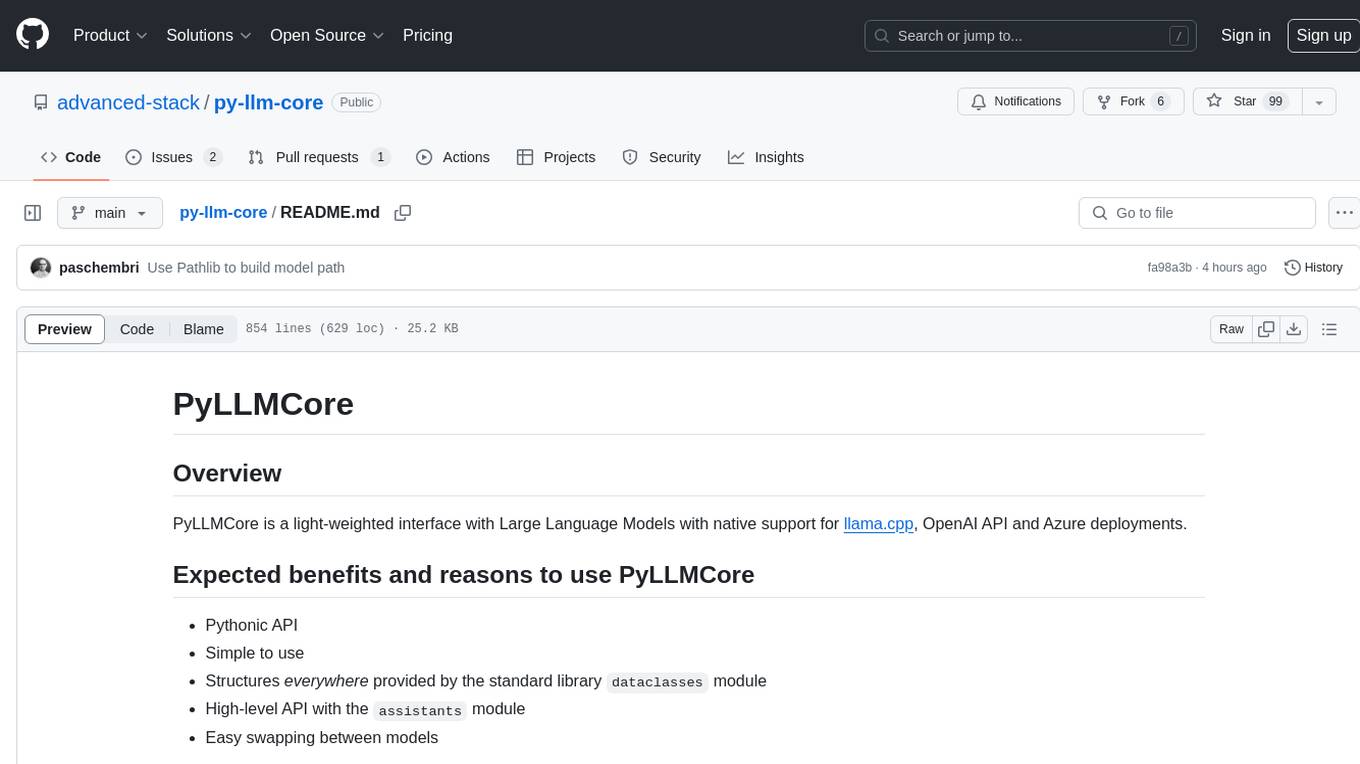
py-llm-core
PyLLMCore is a light-weighted interface with Large Language Models with native support for llama.cpp, OpenAI API, and Azure deployments. It offers a Pythonic API that is simple to use, with structures provided by the standard library dataclasses module. The high-level API includes the assistants module for easy swapping between models. PyLLMCore supports various models including those compatible with llama.cpp, OpenAI, and Azure APIs. It covers use cases such as parsing, summarizing, question answering, hallucinations reduction, context size management, and tokenizing. The tool allows users to interact with language models for tasks like parsing text, summarizing content, answering questions, reducing hallucinations, managing context size, and tokenizing text.
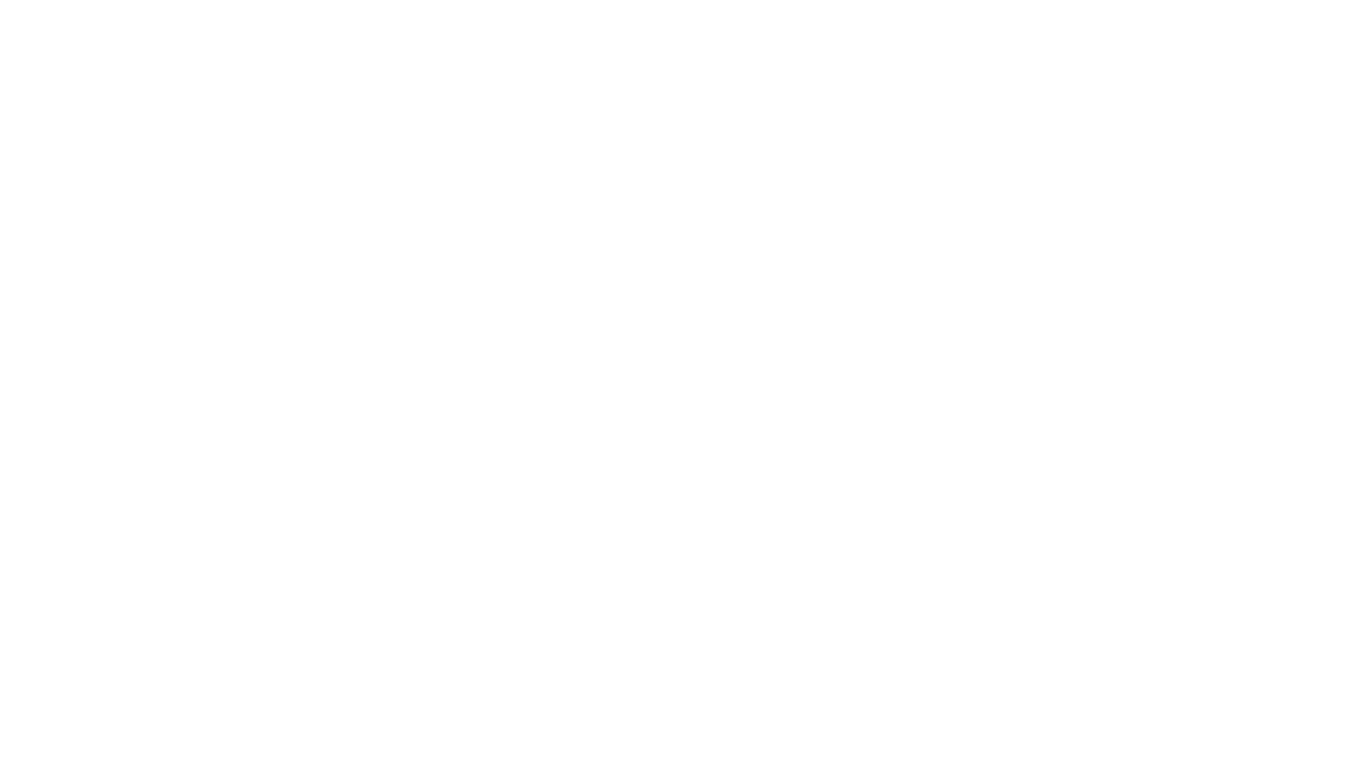
litdata
LitData is a tool designed for blazingly fast, distributed streaming of training data from any cloud storage. It allows users to transform and optimize data in cloud storage environments efficiently and intuitively, supporting various data types like images, text, video, audio, geo-spatial, and multimodal data. LitData integrates smoothly with frameworks such as LitGPT and PyTorch, enabling seamless streaming of data to multiple machines. Key features include multi-GPU/multi-node support, easy data mixing, pause & resume functionality, support for profiling, memory footprint reduction, cache size configuration, and on-prem optimizations. The tool also provides benchmarks for measuring streaming speed and conversion efficiency, along with runnable templates for different data types. LitData enables infinite cloud data processing by utilizing the Lightning.ai platform to scale data processing with optimized machines.
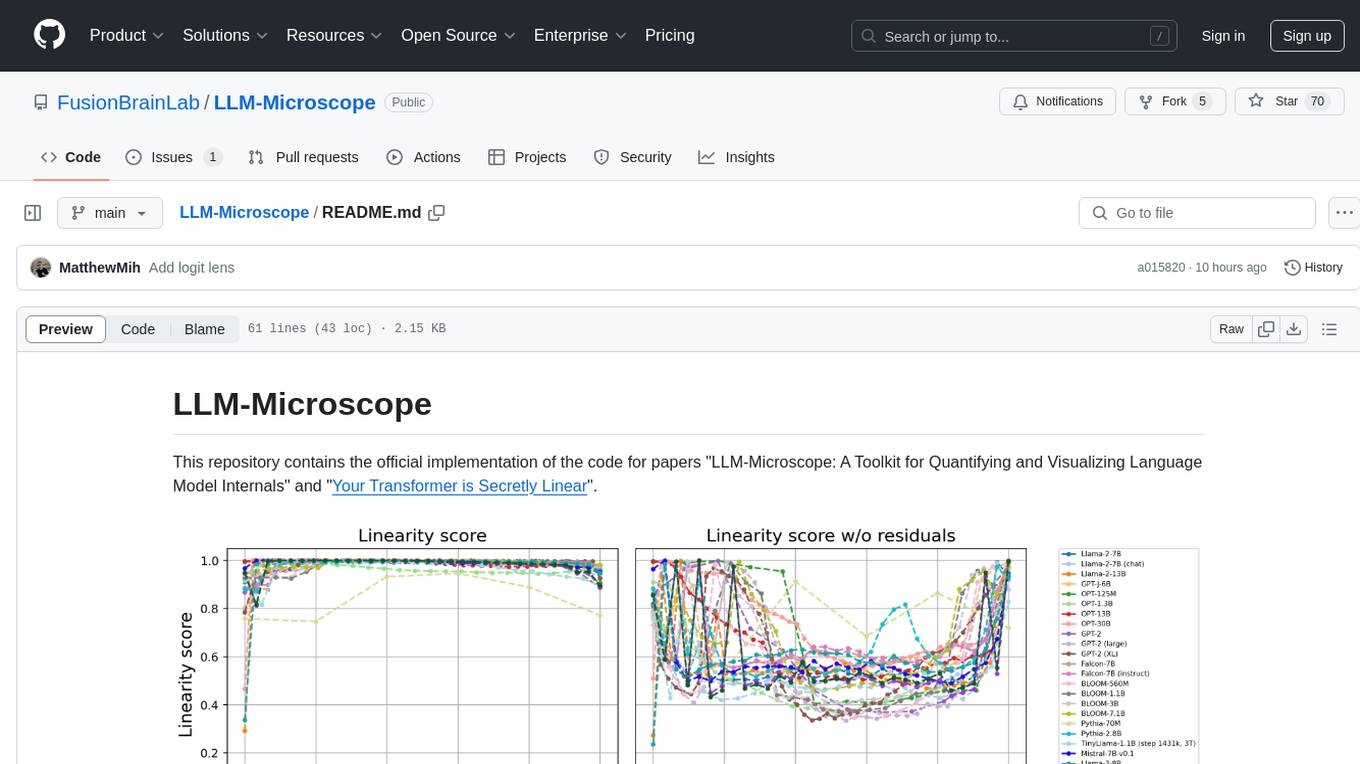
LLM-Microscope
LLM-Microscope is a toolkit designed for quantifying and visualizing language model internals. It provides functions for calculating anisotropy, intrinsic dimension, and linearity score. The toolkit also includes a Logit Lens feature for analyzing model predictions and losses. Users can easily install the toolkit using pip and explore the functionalities through provided examples.
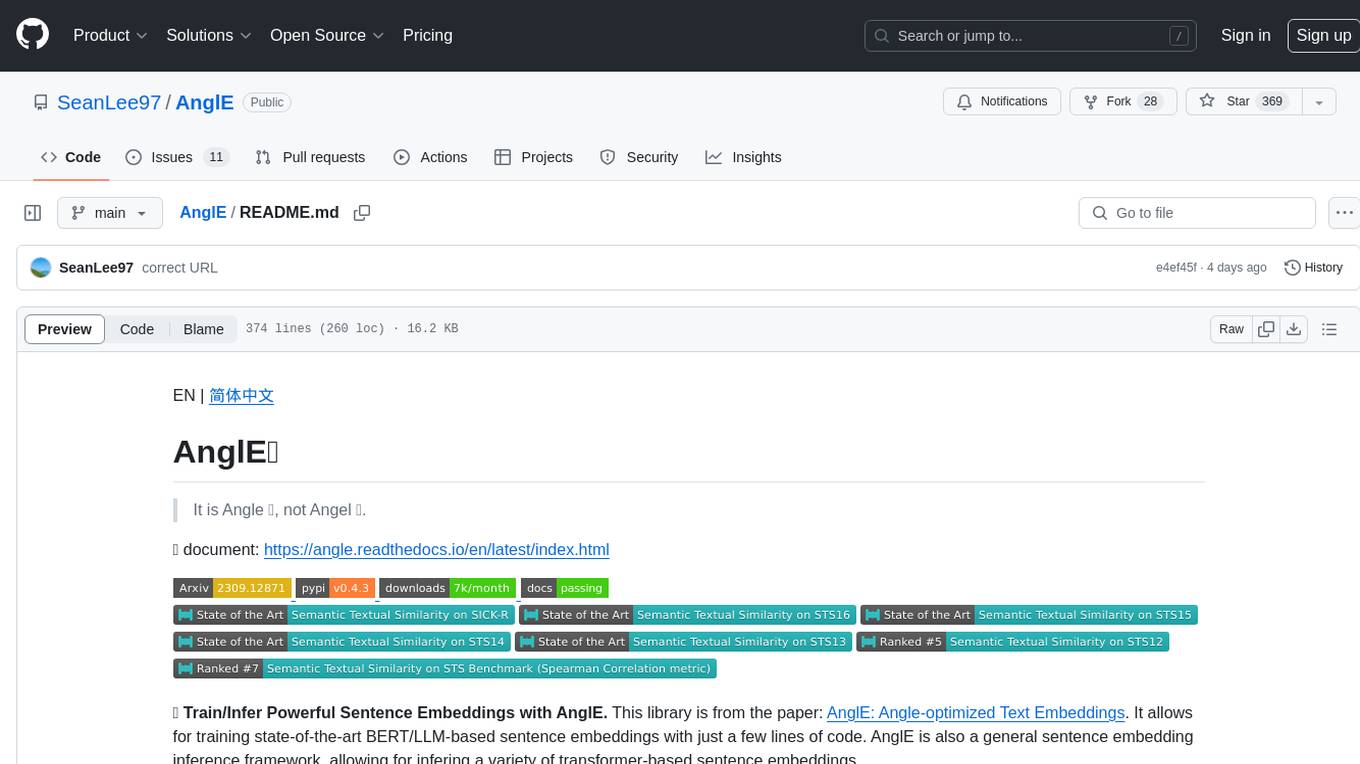
AnglE
AnglE is a library for training state-of-the-art BERT/LLM-based sentence embeddings with just a few lines of code. It also serves as a general sentence embedding inference framework, allowing for inferring a variety of transformer-based sentence embeddings. The library supports various loss functions such as AnglE loss, Contrastive loss, CoSENT loss, and Espresso loss. It provides backbones like BERT-based models, LLM-based models, and Bi-directional LLM-based models for training on single or multi-GPU setups. AnglE has achieved significant performance on various benchmarks and offers official pretrained models for both BERT-based and LLM-based models.
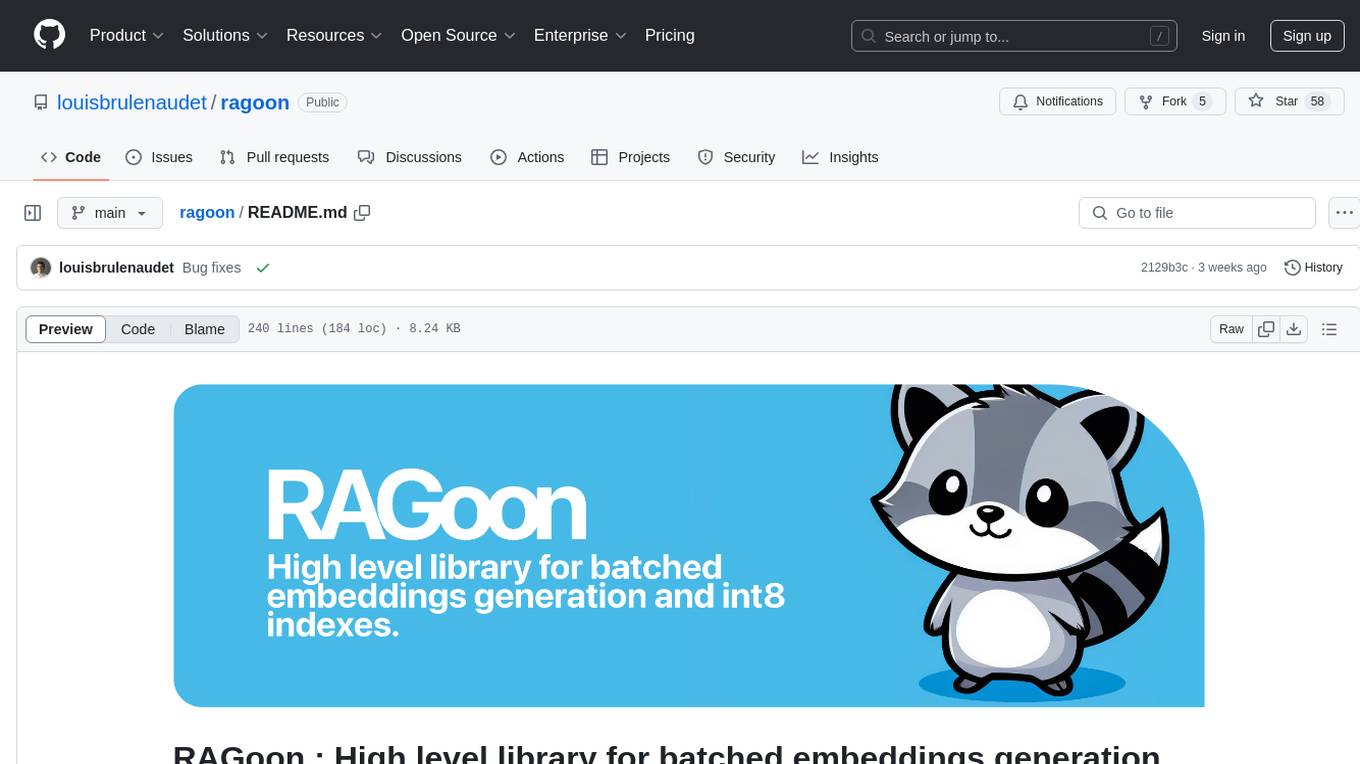
ragoon
RAGoon is a high-level library designed for batched embeddings generation, fast web-based RAG (Retrieval-Augmented Generation) processing, and quantized indexes processing. It provides NLP utilities for multi-model embedding production, high-dimensional vector visualization, and enhancing language model performance through search-based querying, web scraping, and data augmentation techniques.
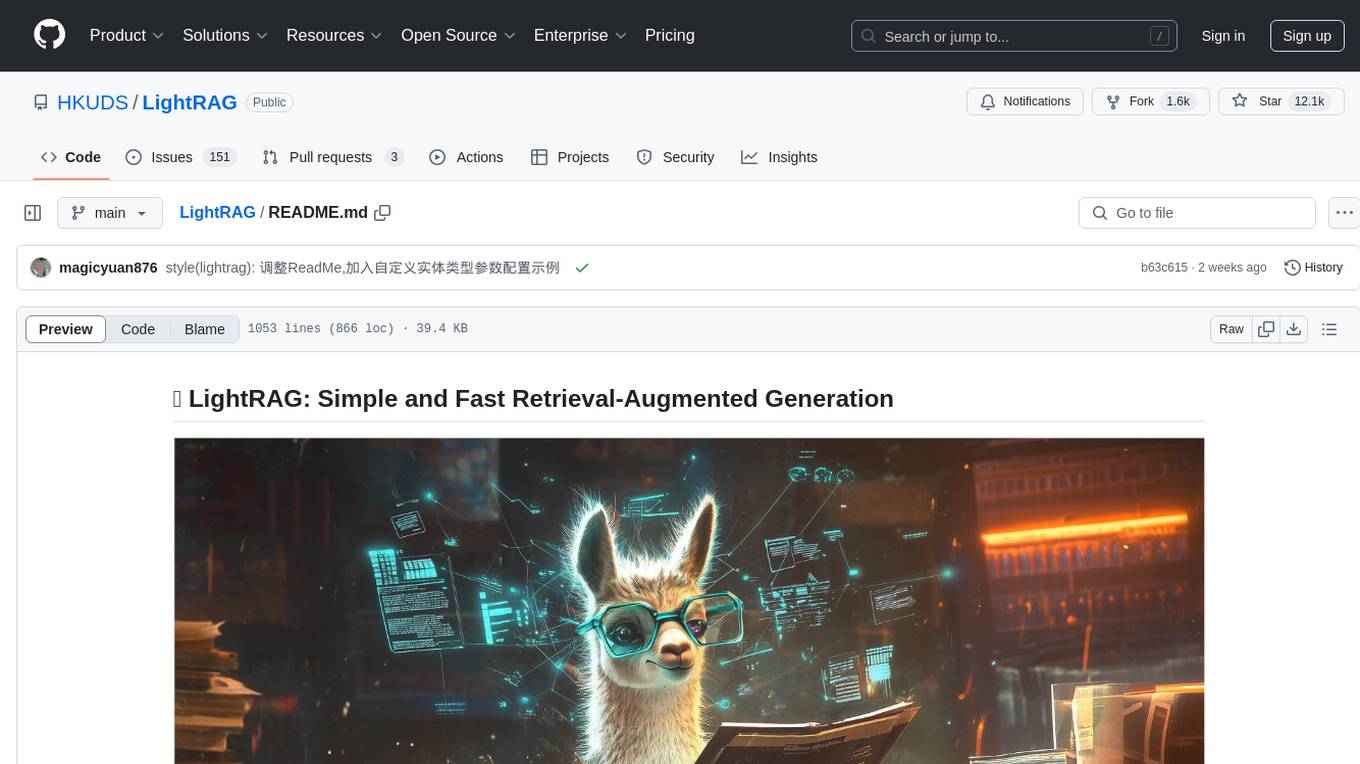
LightRAG
LightRAG is a repository hosting the code for LightRAG, a system that supports seamless integration of custom knowledge graphs, Oracle Database 23ai, Neo4J for storage, and multiple file types. It includes features like entity deletion, batch insert, incremental insert, and graph visualization. LightRAG provides an API server implementation for RESTful API access to RAG operations, allowing users to interact with it through HTTP requests. The repository also includes evaluation scripts, code for reproducing results, and a comprehensive code structure.
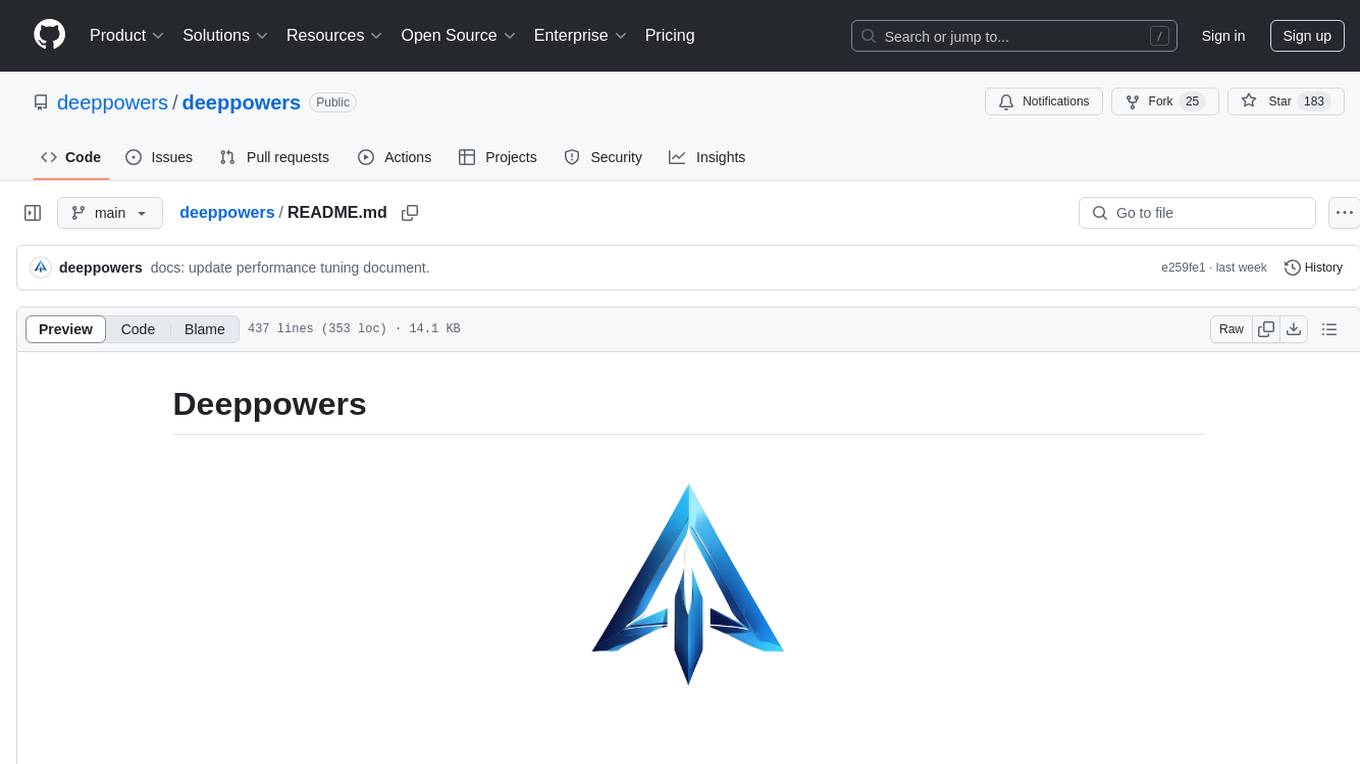
deeppowers
Deeppowers is a powerful Python library for deep learning applications. It provides a wide range of tools and utilities to simplify the process of building and training deep neural networks. With Deeppowers, users can easily create complex neural network architectures, perform efficient training and optimization, and deploy models for various tasks. The library is designed to be user-friendly and flexible, making it suitable for both beginners and experienced deep learning practitioners.
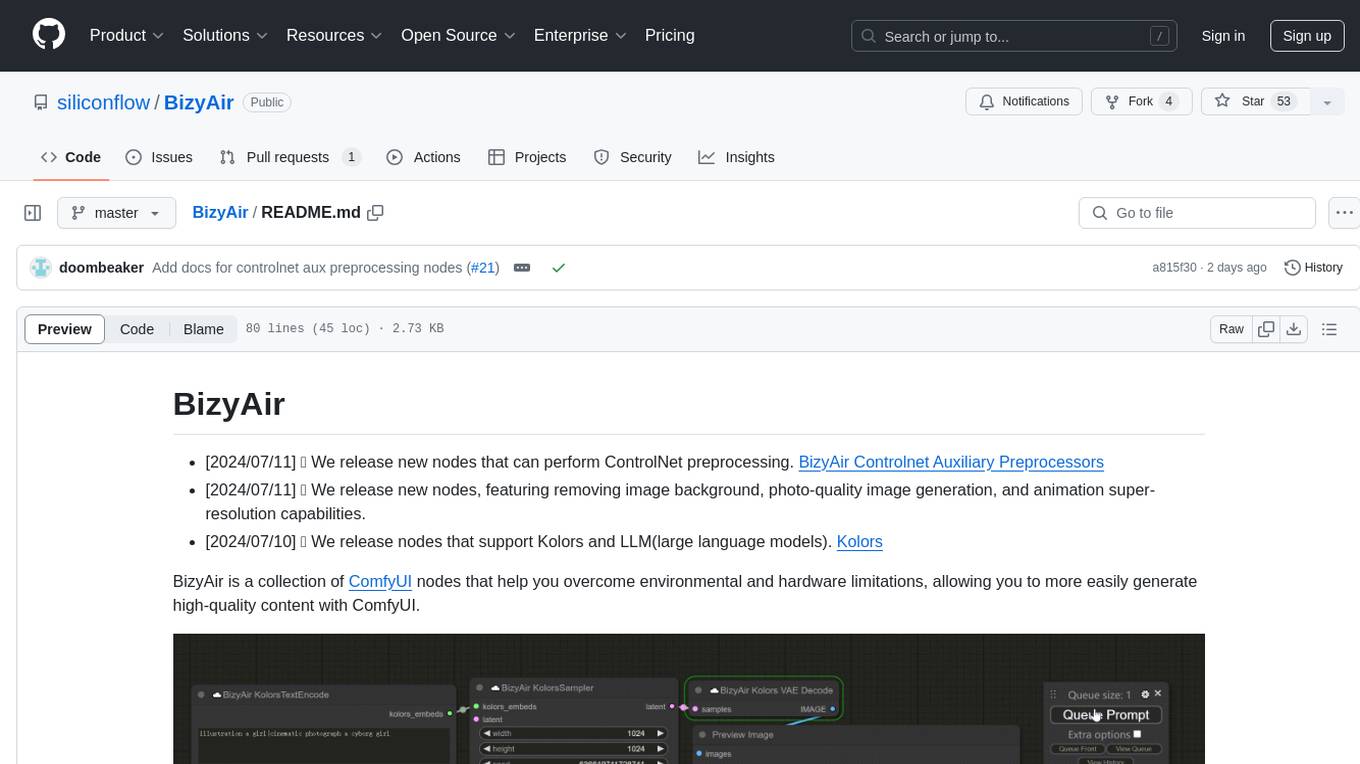
BizyAir
BizyAir is a collection of ComfyUI nodes that help users overcome environmental and hardware limitations to generate high-quality content. It includes features such as ControlNet preprocessing, image background removal, photo-quality image generation, and animation super-resolution. Users can run ComfyUI anywhere without worrying about hardware requirements. Installation methods include using ComfyUI Manager, Comfy CLI, downloading standalone packages for Windows, or cloning the BizyAir repository into the custom_nodes subdirectory of ComfyUI.
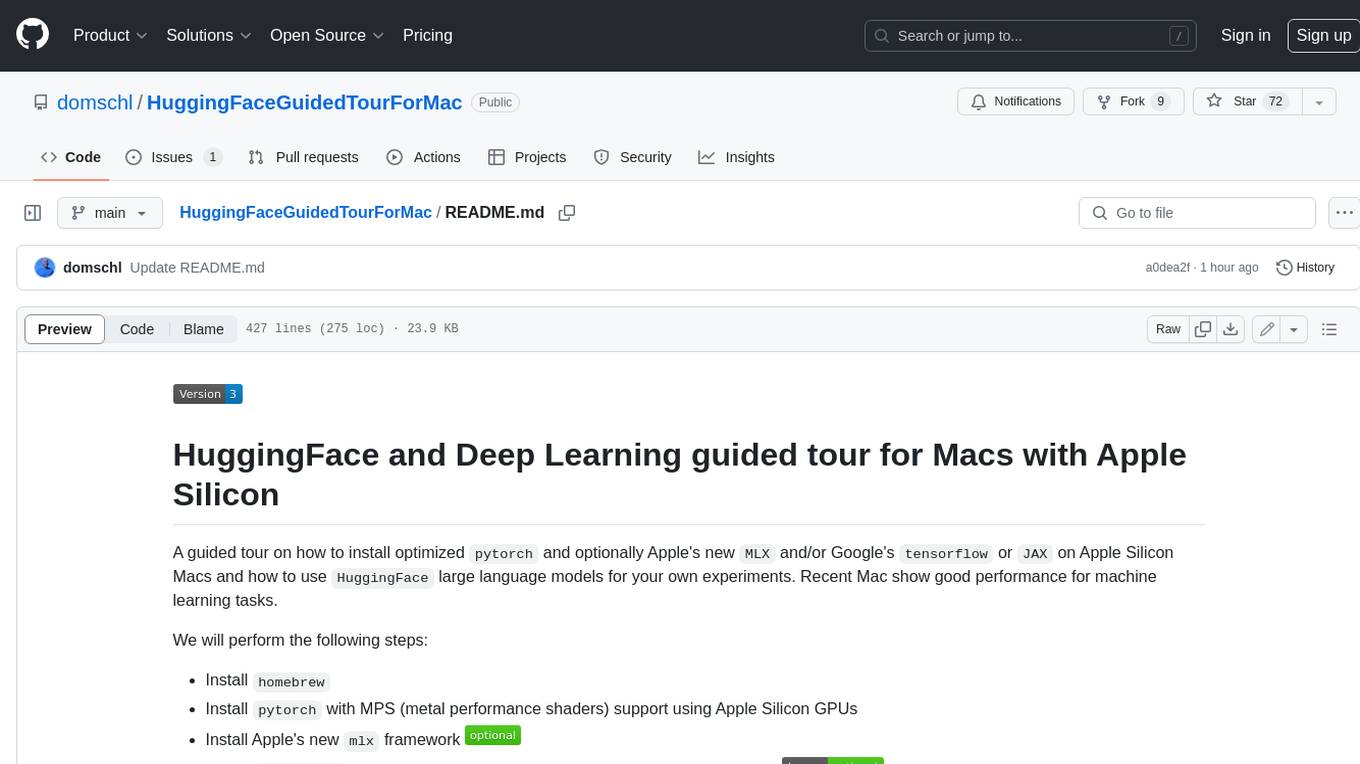
HuggingFaceGuidedTourForMac
HuggingFaceGuidedTourForMac is a guided tour on how to install optimized pytorch and optionally Apple's new MLX, JAX, and TensorFlow on Apple Silicon Macs. The repository provides steps to install homebrew, pytorch with MPS support, MLX, JAX, TensorFlow, and Jupyter lab. It also includes instructions on running large language models using HuggingFace transformers. The repository aims to help users set up their Macs for deep learning experiments with optimized performance.
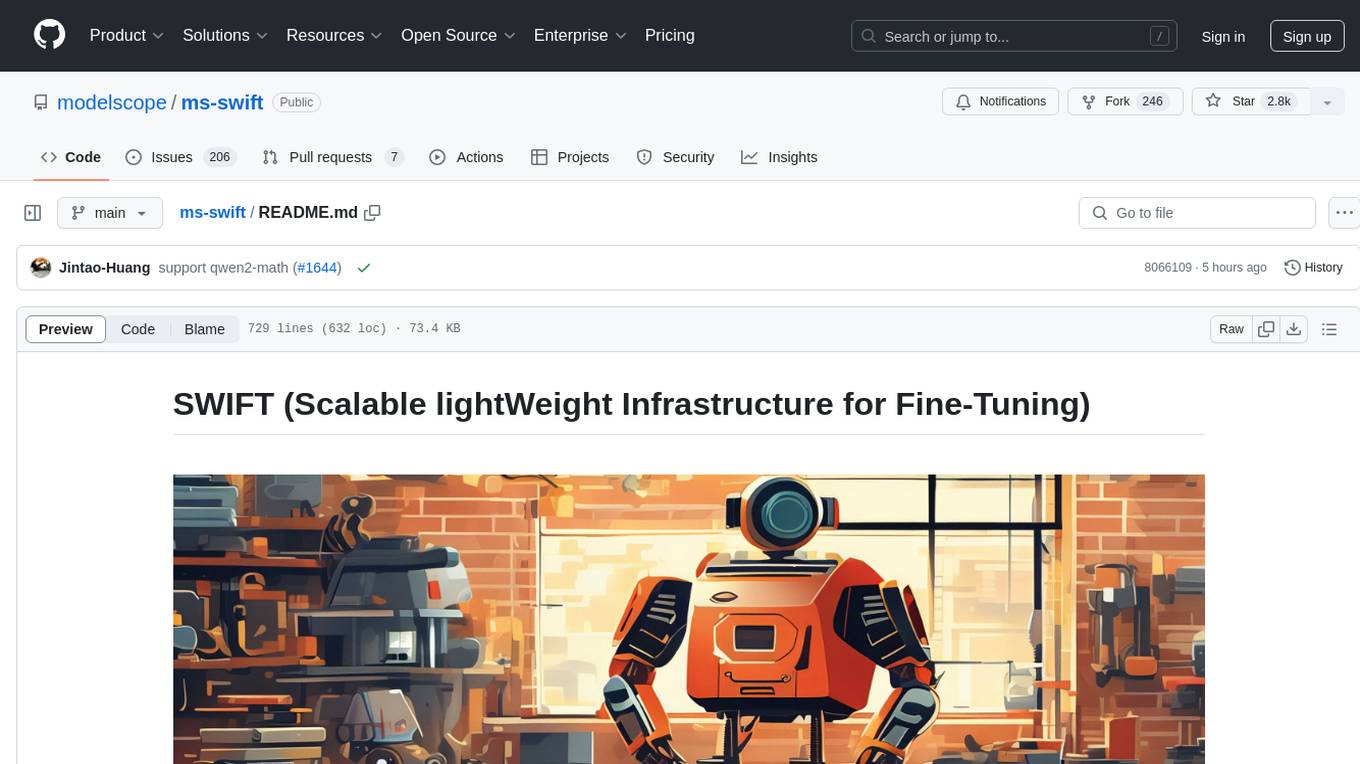
ms-swift
ms-swift is an official framework provided by the ModelScope community for fine-tuning and deploying large language models and multi-modal large models. It supports training, inference, evaluation, quantization, and deployment of over 400 large models and 100+ multi-modal large models. The framework includes various training technologies and accelerates inference, evaluation, and deployment modules. It offers a Gradio-based Web-UI interface and best practices for easy application of large models. ms-swift supports a wide range of model types, dataset types, hardware support, lightweight training methods, distributed training techniques, quantization training, RLHF training, multi-modal training, interface training, plugin and extension support, inference acceleration engines, model evaluation, and model quantization.
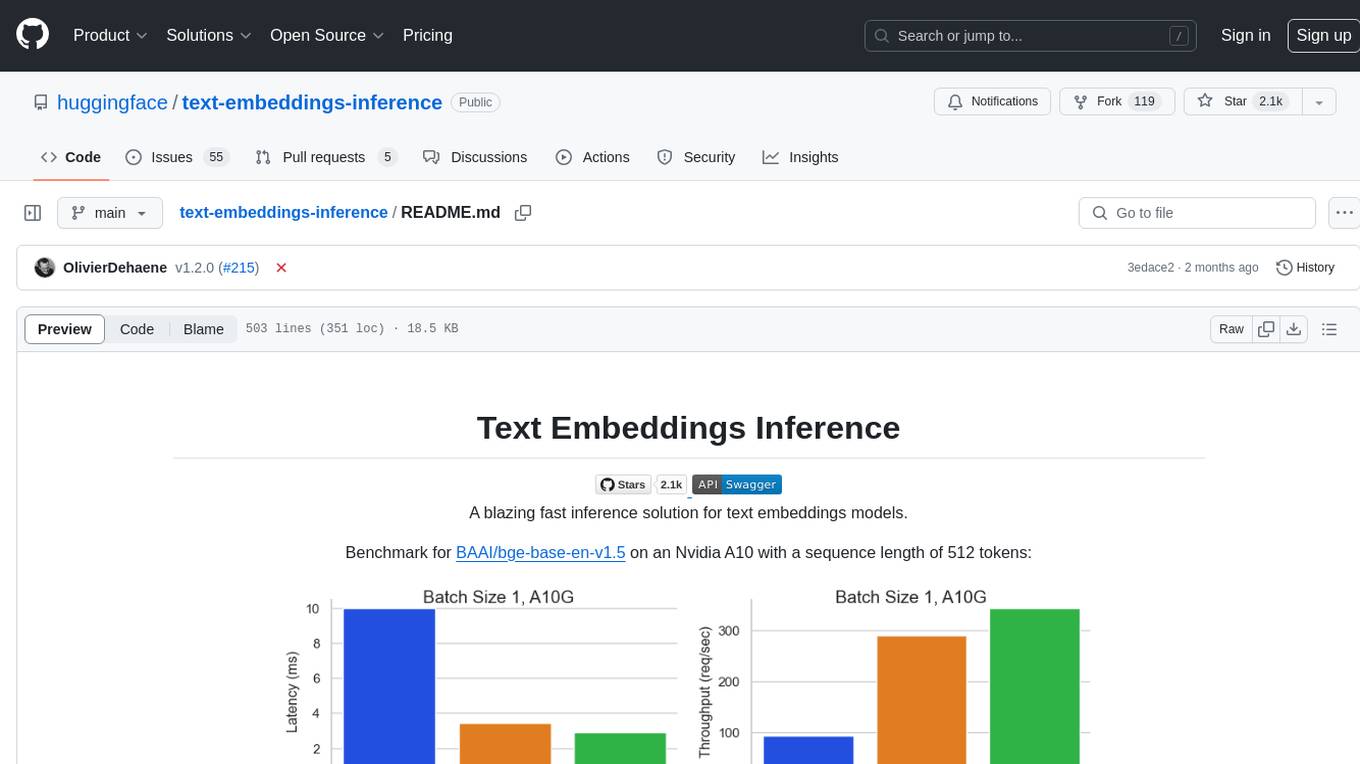
text-embeddings-inference
Text Embeddings Inference (TEI) is a toolkit for deploying and serving open source text embeddings and sequence classification models. TEI enables high-performance extraction for popular models like FlagEmbedding, Ember, GTE, and E5. It implements features such as no model graph compilation step, Metal support for local execution on Macs, small docker images with fast boot times, token-based dynamic batching, optimized transformers code for inference using Flash Attention, Candle, and cuBLASLt, Safetensors weight loading, and production-ready features like distributed tracing with Open Telemetry and Prometheus metrics.
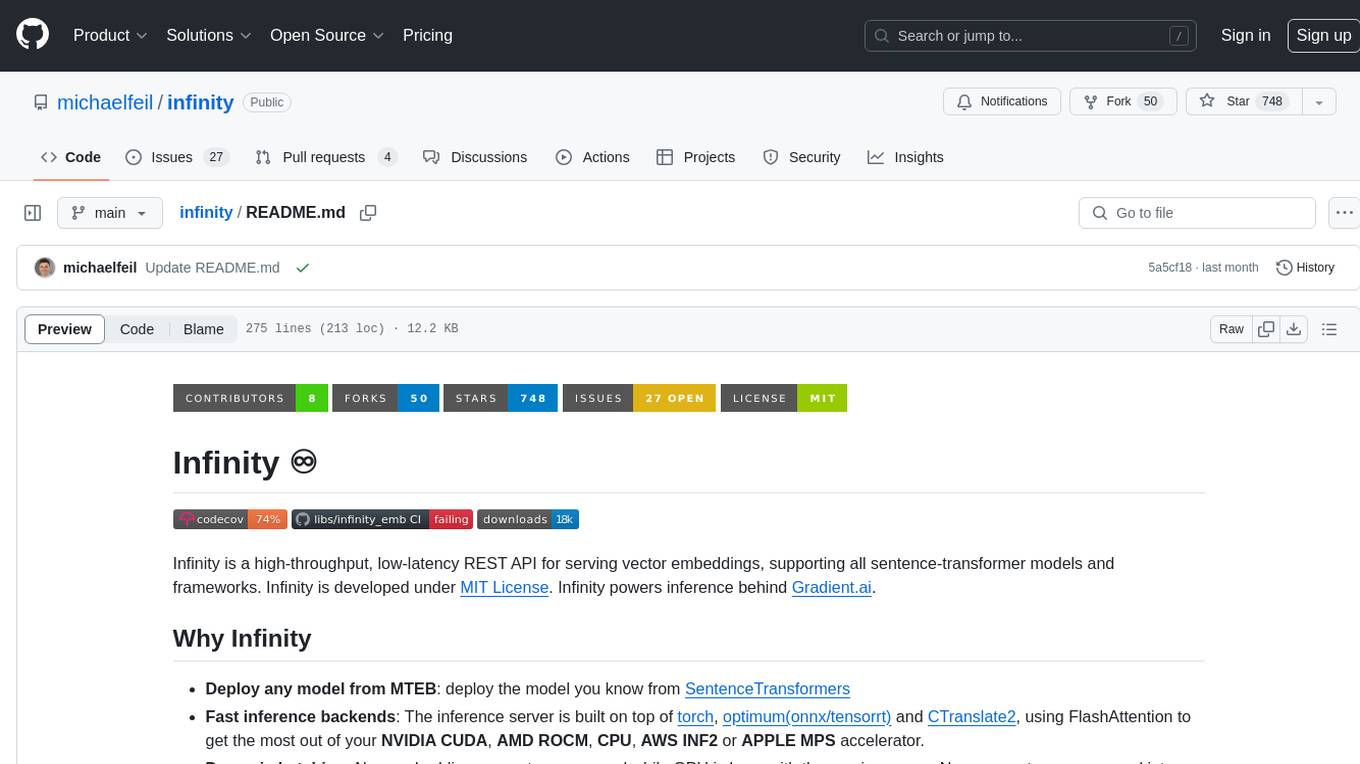
infinity
Infinity is a high-throughput, low-latency REST API for serving vector embeddings, supporting all sentence-transformer models and frameworks. It is developed under the MIT License and powers inference behind Gradient.ai. The API allows users to deploy models from SentenceTransformers, offers fast inference backends utilizing various accelerators, dynamic batching for efficient processing, correct and tested implementation, and easy-to-use API built on FastAPI with Swagger documentation. Users can embed text, rerank documents, and perform text classification tasks using the tool. Infinity supports various models from Huggingface and provides flexibility in deployment via CLI, Docker, Python API, and cloud services like dstack. The tool is suitable for tasks like embedding, reranking, and text classification.
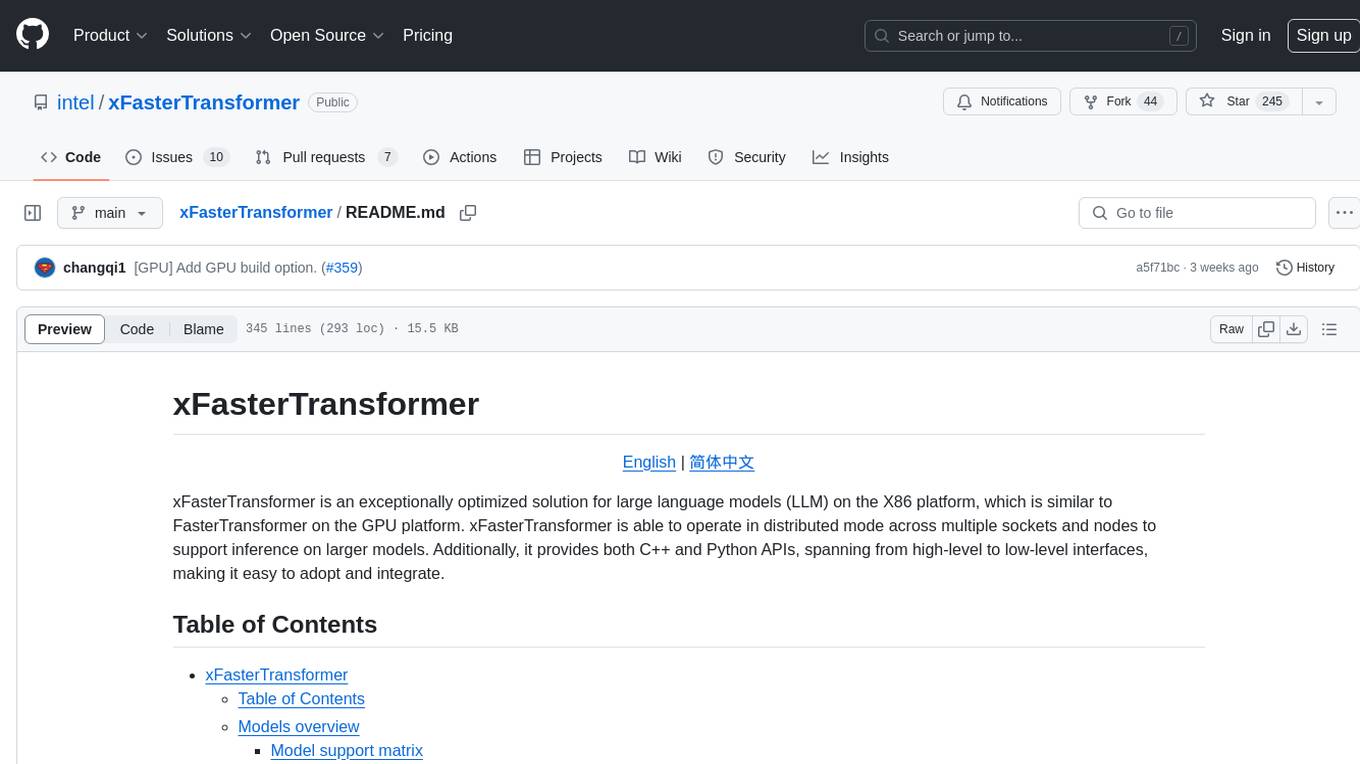
xFasterTransformer
xFasterTransformer is an optimized solution for Large Language Models (LLMs) on the X86 platform, providing high performance and scalability for inference on mainstream LLM models. It offers C++ and Python APIs for easy integration, along with example codes and benchmark scripts. Users can prepare models in a different format, convert them, and use the APIs for tasks like encoding input prompts, generating token ids, and serving inference requests. The tool supports various data types and models, and can run in single or multi-rank modes using MPI. A web demo based on Gradio is available for popular LLM models like ChatGLM and Llama2. Benchmark scripts help evaluate model inference performance quickly, and MLServer enables serving with REST and gRPC interfaces.
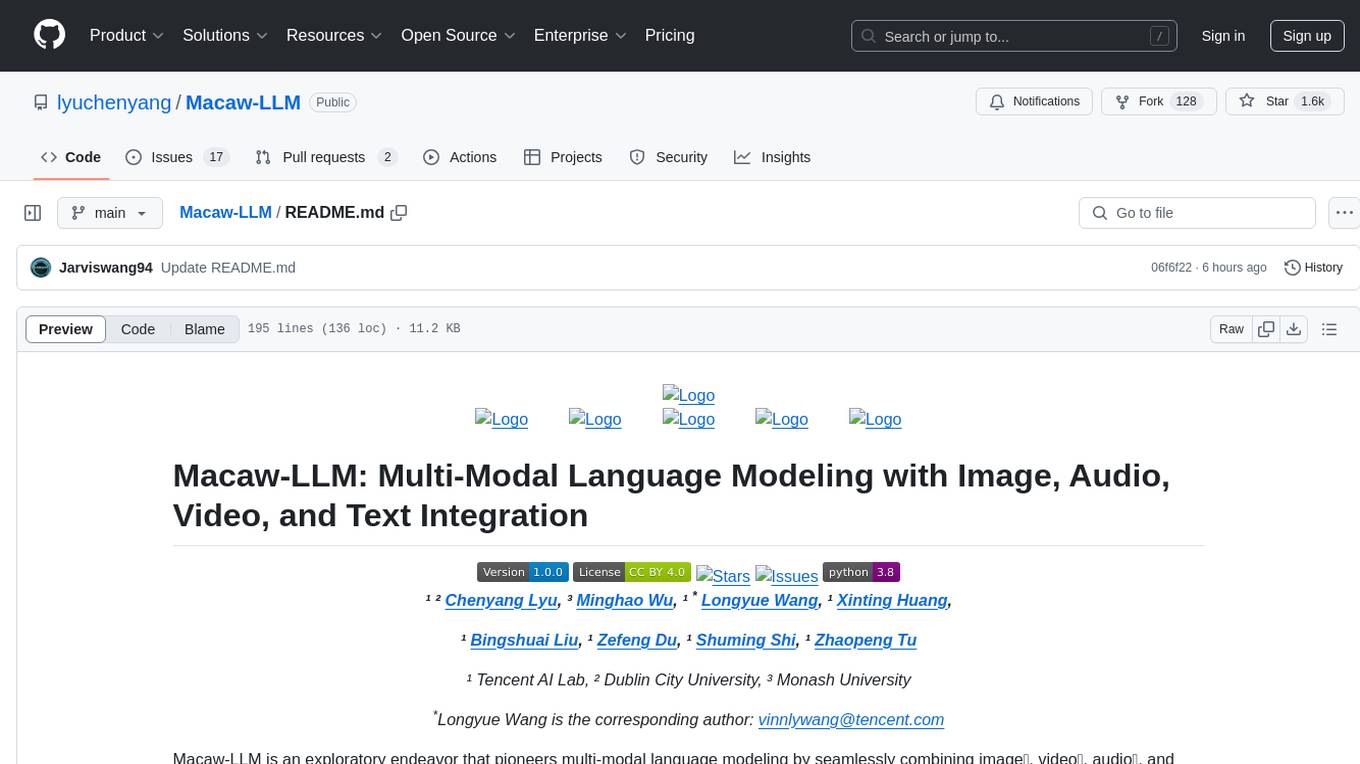
Macaw-LLM
Macaw-LLM is a pioneering multi-modal language modeling tool that seamlessly integrates image, audio, video, and text data. It builds upon CLIP, Whisper, and LLaMA models to process and analyze multi-modal information effectively. The tool boasts features like simple and fast alignment, one-stage instruction fine-tuning, and a new multi-modal instruction dataset. It enables users to align multi-modal features efficiently, encode instructions, and generate responses across different data types.
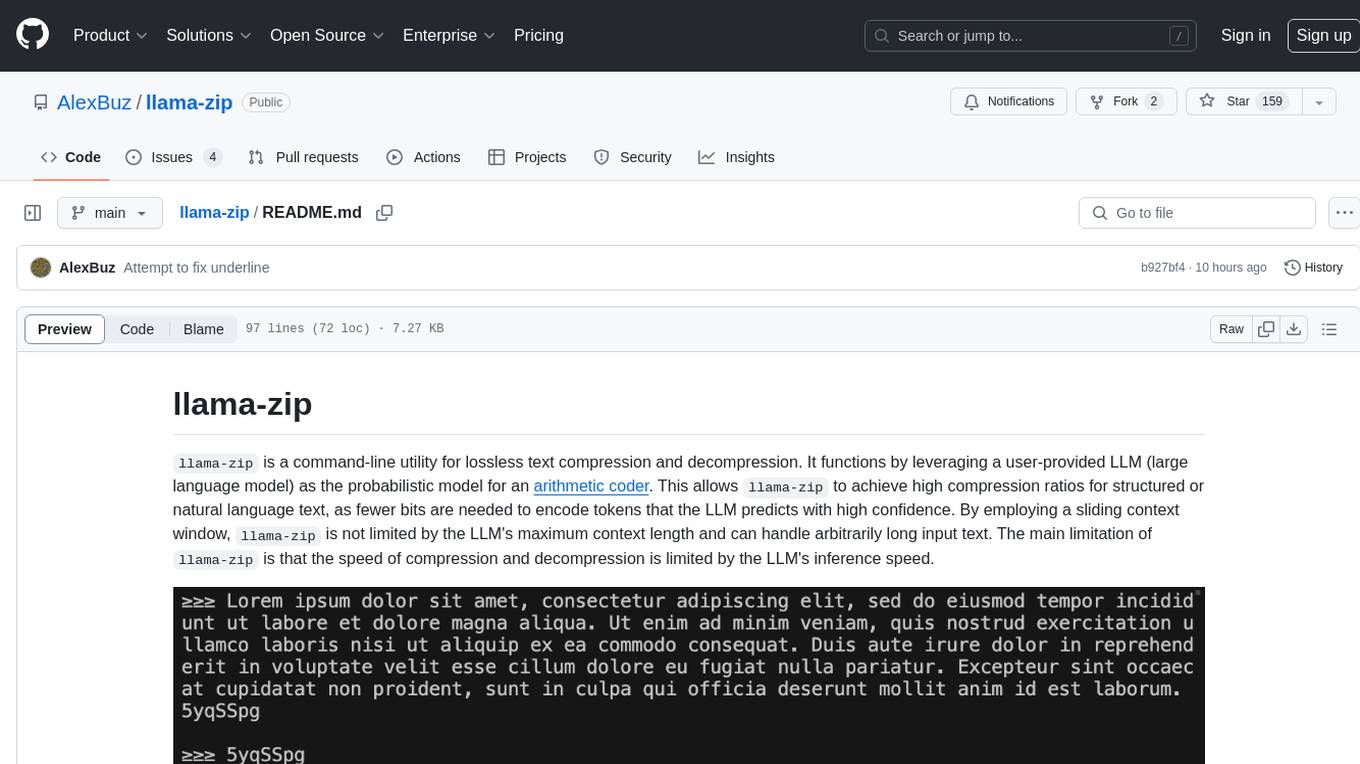
llama-zip
llama-zip is a command-line utility for lossless text compression and decompression. It leverages a user-provided large language model (LLM) as the probabilistic model for an arithmetic coder, achieving high compression ratios for structured or natural language text. The tool is not limited by the LLM's maximum context length and can handle arbitrarily long input text. However, the speed of compression and decompression is limited by the LLM's inference speed.