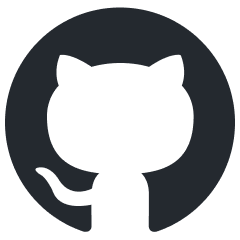
llama3-tokenizer-js
JS tokenizer for LLaMA 3 and LLaMA 3.1
Stars: 104
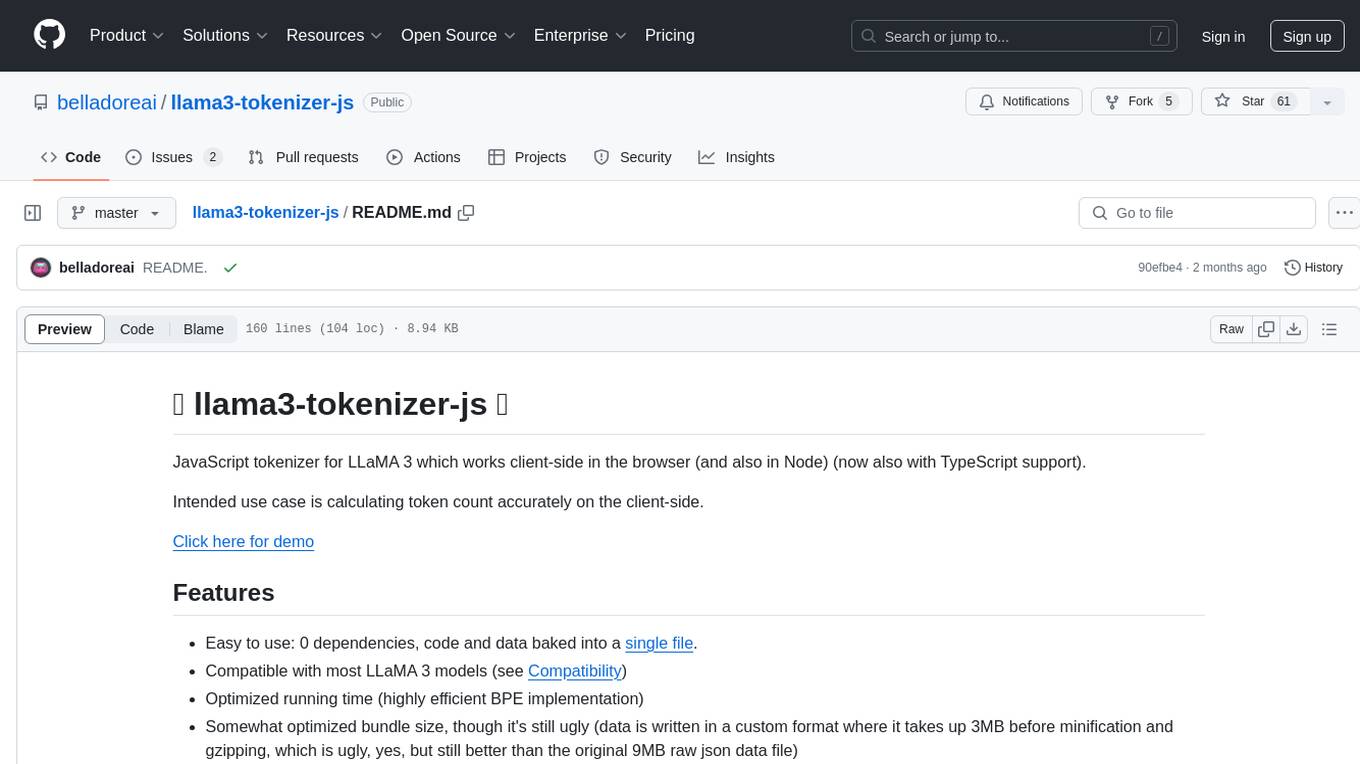
JavaScript tokenizer for LLaMA 3 designed for client-side use in the browser and Node, with TypeScript support. It accurately calculates token count, has 0 dependencies, optimized running time, and somewhat optimized bundle size. Compatible with most LLaMA 3 models. Can encode and decode text, but training is not supported. Pollutes global namespace with `llama3Tokenizer` in the browser. Mostly compatible with LLaMA 3 models released by Facebook in April 2024. Can be adapted for incompatible models by passing custom vocab and merge data. Handles special tokens and fine tunes. Developed by belladore.ai with contributions from xenova, blaze2004, imoneoi, and ConProgramming.
README:
JavaScript tokenizer for LLaMA 3, 3.1 and 3.2.
Intended use case is calculating token count accurately on the client-side.
Works client-side in the browser, in Node, in TypeScript codebases, in ES6 projects, and in CommonJS projects.
- Easy to use: 0 dependencies, code and data baked into a single file.
- Compatible with most LLaMA 3 models (see Compatibility)
- Optimized running time (highly efficient BPE implementation)
- Somewhat optimized bundle size, though it's still ugly (data is written in a custom format where it takes up 3MB before minification and gzipping, which is ugly, yes, but still better than the original 9MB raw json data file)
Install as an npm package and import as ES6 module
npm install llama3-tokenizer-js
import llama3Tokenizer from 'llama3-tokenizer-js'
console.log(llama3Tokenizer.encode("Hello world!").length) // returns token count 5
It's possible to load the main bundle file with simple <script>
tags:
<script type="module" src="https://belladoreai.github.io/llama3-tokenizer-js/bundle/llama3-tokenizer-with-baked-data.js"></script>
If you decide to load with script tags, be sure to either grab a copy of the file into your local build, or change the github URL such that you lock the file to a specific commit.
Alternative import syntax for CommonJS projects:
async function main() {
const llama3Tokenizer = await import('llama3-tokenizer-js')
console.log(llama3Tokenizer.default.encode("Hello world!").length)
}
main();
If you need to use CommonJS with the normal import syntax, you can try loading this experimental CommonJS version of the library: bundle/commonjs-llama3-tokenizer-with-baked-data.js.
Once you have the module imported, you can encode or decode with it. Training is not supported.
When used in browser, llama3-tokenizer-js pollutes global namespace with llama3Tokenizer
.
Encode:
llama3Tokenizer.encode("Hello world!")
> [128000, 9906, 1917, 0, 128001]
Decode:
llama3Tokenizer.decode([128000, 9906, 1917, 0, 128001])
> '<|begin_of_text|>Hello world!<|end_of_text|>'
Note the special tokens in the beginning and end. These affect token count. You can pass an options object if you don't want to add these:
llama3Tokenizer.encode("Hello world!", { bos: false, eos: false })
> [9906, 1917, 0]
Note that, contrary to LLaMA 1 tokenizer, the LLaMA 3 tokenizer does not add a preceding space (please open an issue if there are circumstances in which a preceding space is still added).
This tokenizer is mostly* compatible with all models which have been trained on top of "LLaMA 3" and "LLaMA 3.1" checkpoints.
What this means in practice:
- ✅ LLaMA 3 models released by Facebook: yes, they are compatible
- ✅ LLaMA 3.1 models released by Facebook: yes, they are compatible
- ✅ New LLaMA 3 or 3.1 based fine tune by somebody other than Facebook: yes, it's compatible (except possibly for some special tokens*)
- ❌ New LLaMA 3 model trained from scratch by somebody other than Facebook: probably not compatible, depends if they also retrained the tokenizer (and/or if they added their own special tokens*)
- ❌ LLaMA 1 or LLaMA 2 based models: no, not compatible (use llama-tokenizer-js instead)
- ❌ OpenAI models: no, not compatible
- ❌ Mistral models: no, not compatible
*See below section "Special tokens and fine tunes".
If you are unsure about compatibility, try it and see if the token ids are the same (compared to running the model with, for example, the transformers library). If you are testing a fine tune, remember to test with the relevant special tokens.
If you want to make this library work with different tokenizer data, you may be interested in this script which was used to convert the data.
You can pass custom vocab and merge data to the tokenizer by instantiating it like this:
import { Llama3Tokenizer } from 'llama3-tokenizer-js'
const tokenizer = new Llama3Tokenizer(custom_vocab, custom_merge_data);
Please note that if you try to adapt this library to work for a different tokenizer, there are many footguns and it's easy to set up something that almost works. If the only thing that needs to change is vocab and merge data, and they are of same size as the previous vocab and merge data, you should be fine. But if anything else in addition to vocab and merge data needs to change, you have to read and understand the full source code and make changes where needed.
It's common with language models, including Llama 3, to denote the end of sequence (eos) with a special token. Please note that in May 2024 the eos token in the official Huggingface repo for Llama 3 instruct was changed by Huggingface staff from <|end_of_text|>
to <|eot_id|>
. Both of these special tokens already existed in the tokenizer, the change merely affects how these tokens are treated in commonly used software such as oobabooga. This change makes sense in the context of Llama 3 instruct, but it does not make sense in the context of Llama 3 base model. Therefore, I have decided I will not change the eos token in this library. In any case, this discrepancy will not affect token counts. It's something you need to be aware of only if you use the generated tokens for purposes other than counting.
There is a large number of special tokens in Llama 3 (e.g. <|end_of_text|>
). You can pass these inside text input, they will be parsed and counted correctly (try the example-demo playground if you are unsure).
However, sometimes when people fine tune models, they change the special tokens by adding their own tokens and even shifting the ids of pre-existing special tokens. For example: Hermes-2-Pro-Llama-3-8B. This is unfortunate for our token counting purposes. If you are using this library to count tokens, and you are using a fine tune which messes around with special tokens, you can choose one of the following approaches:
- Instead of calling
.encode(str).length
, you can call.optimisticCount(str)
. Optimistic count is a convenience function which parses the text with the assumption that anything that looks like a special token (e.g.<|boom|>
) is actually a special token. - If you need exact token counts, you can work around this issue by using this library to tokenize only user input text (which shouldn't contain any special tokens) and then programmatically adding the relevant counts for the special tokens that you are using to wrap the input text.
- Alternatively, you can choose to ignore this issue, in which case you will be overcounting tokens by a little bit, which is not too bad (in typical use cases, undercounting can lead to more severe quality issues than overcounting).
- Node test:
node test/node-test.js
- Browser test: run
live-server
and open test/browser-test.html - Example-demo test: run
cd example-demo && npm install && npm run build && live-server
and open the "build" folder
Note that some parts of the code might behave differently in node compared to browser environment.
Release steps:
- bundle code and data into a single file:
cd src && node create-bundle.js
- run node test
- run browser test
- bump version number in root package.json
- push changes to github
- npm publish --dry-run
- npm publish
- bump version number in example-demo/package.json
- run example-demo test
- README update?
- push again to github
- create new release on github
LLaMA3-tokenizer-js is a fork of my earlier LLaMA 1 tokenizer llama-tokenizer-js.
Several helper functions used in LLaMA 3 pretokenization were adapted from transformers.js. The BPE implementation, which is the core of this library, is original work and was adapted into transformers.js. In other words, some work has been adapted from llama-tokenizer-js into transformers.js, and some work has been adapted the other way, from transformers.js into llama3-tokenizer-js.
The example-demo (tokenizer playground) is a fork of gpt-tokenizer playground.
Developed by belladore.ai with contributions from xenova, blaze2004, imoneoi, norlesh, ConProgramming and rjeli.
For Tasks:
Click tags to check more tools for each tasksFor Jobs:
Alternative AI tools for llama3-tokenizer-js
Similar Open Source Tools
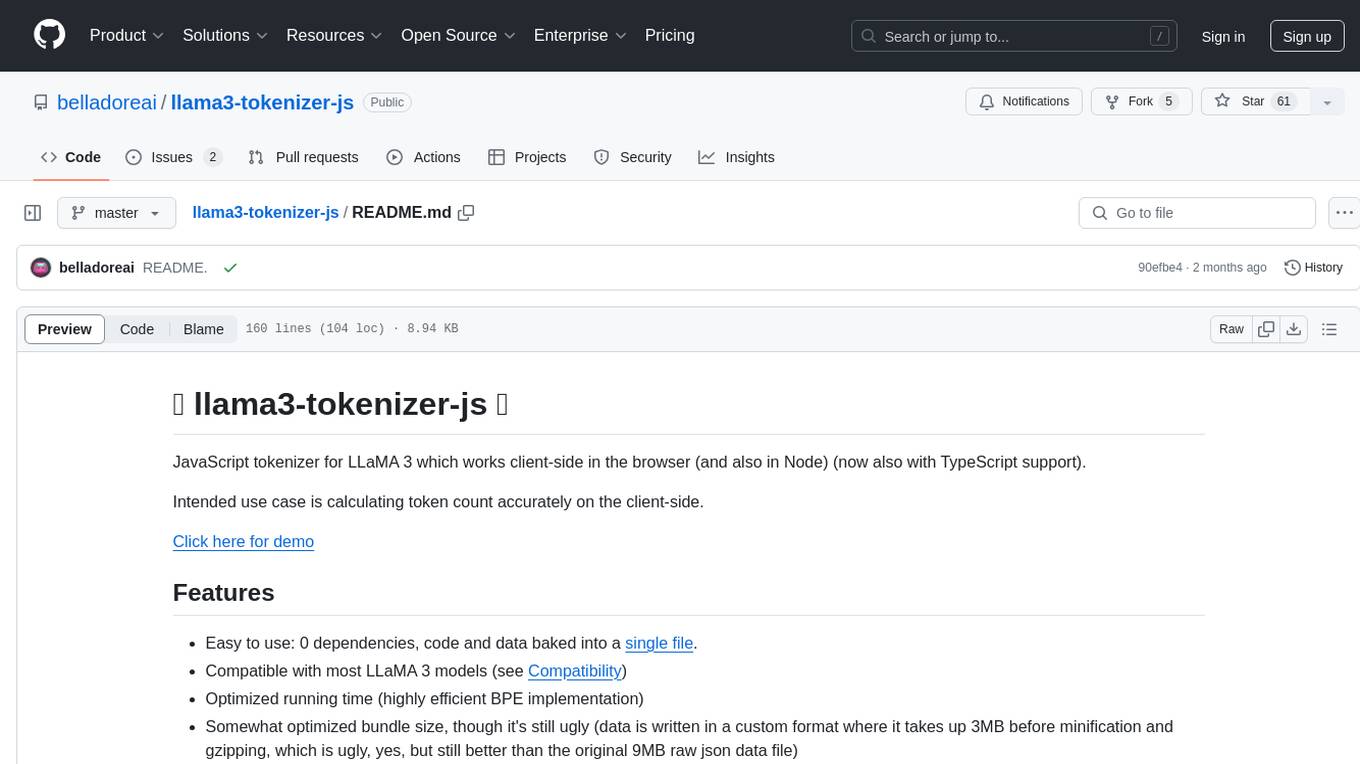
llama3-tokenizer-js
JavaScript tokenizer for LLaMA 3 designed for client-side use in the browser and Node, with TypeScript support. It accurately calculates token count, has 0 dependencies, optimized running time, and somewhat optimized bundle size. Compatible with most LLaMA 3 models. Can encode and decode text, but training is not supported. Pollutes global namespace with `llama3Tokenizer` in the browser. Mostly compatible with LLaMA 3 models released by Facebook in April 2024. Can be adapted for incompatible models by passing custom vocab and merge data. Handles special tokens and fine tunes. Developed by belladore.ai with contributions from xenova, blaze2004, imoneoi, and ConProgramming.
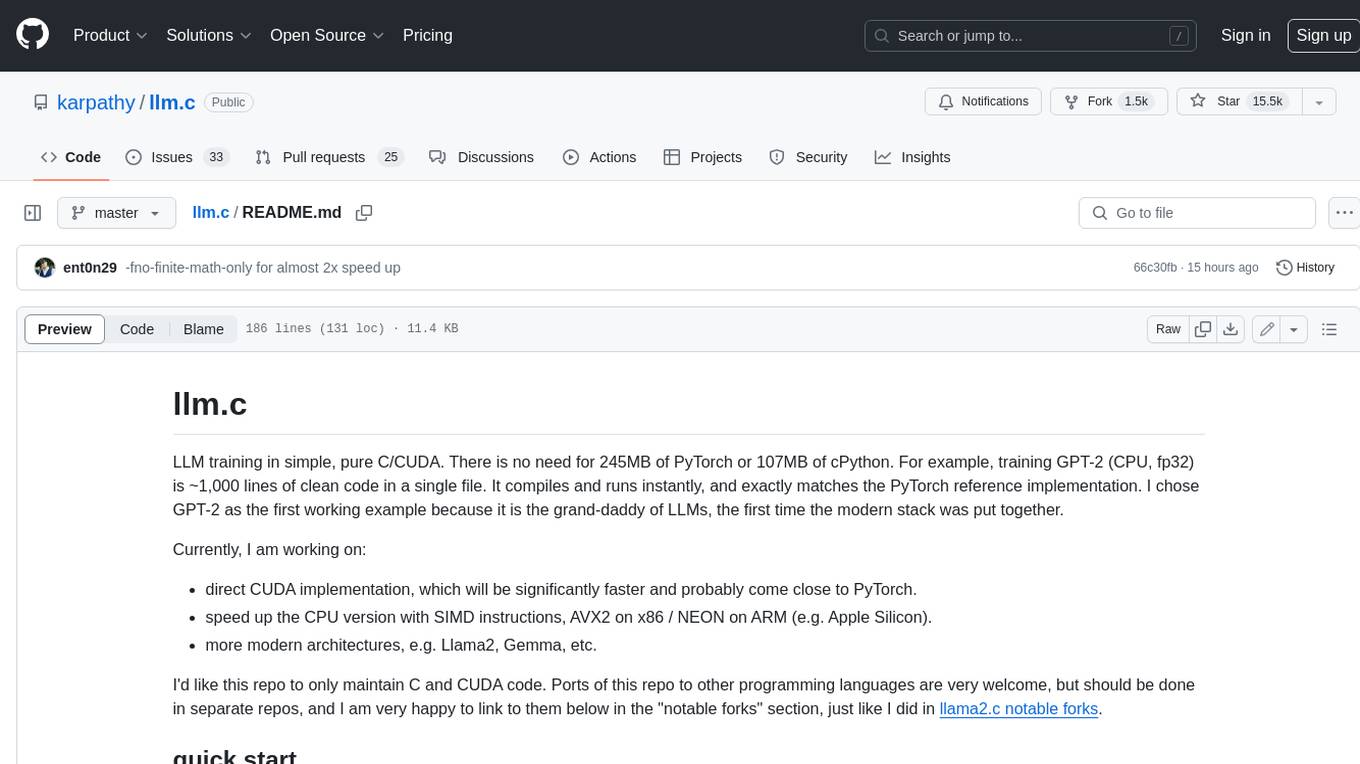
llm.c
LLM training in simple, pure C/CUDA. There is no need for 245MB of PyTorch or 107MB of cPython. For example, training GPT-2 (CPU, fp32) is ~1,000 lines of clean code in a single file. It compiles and runs instantly, and exactly matches the PyTorch reference implementation. I chose GPT-2 as the first working example because it is the grand-daddy of LLMs, the first time the modern stack was put together.
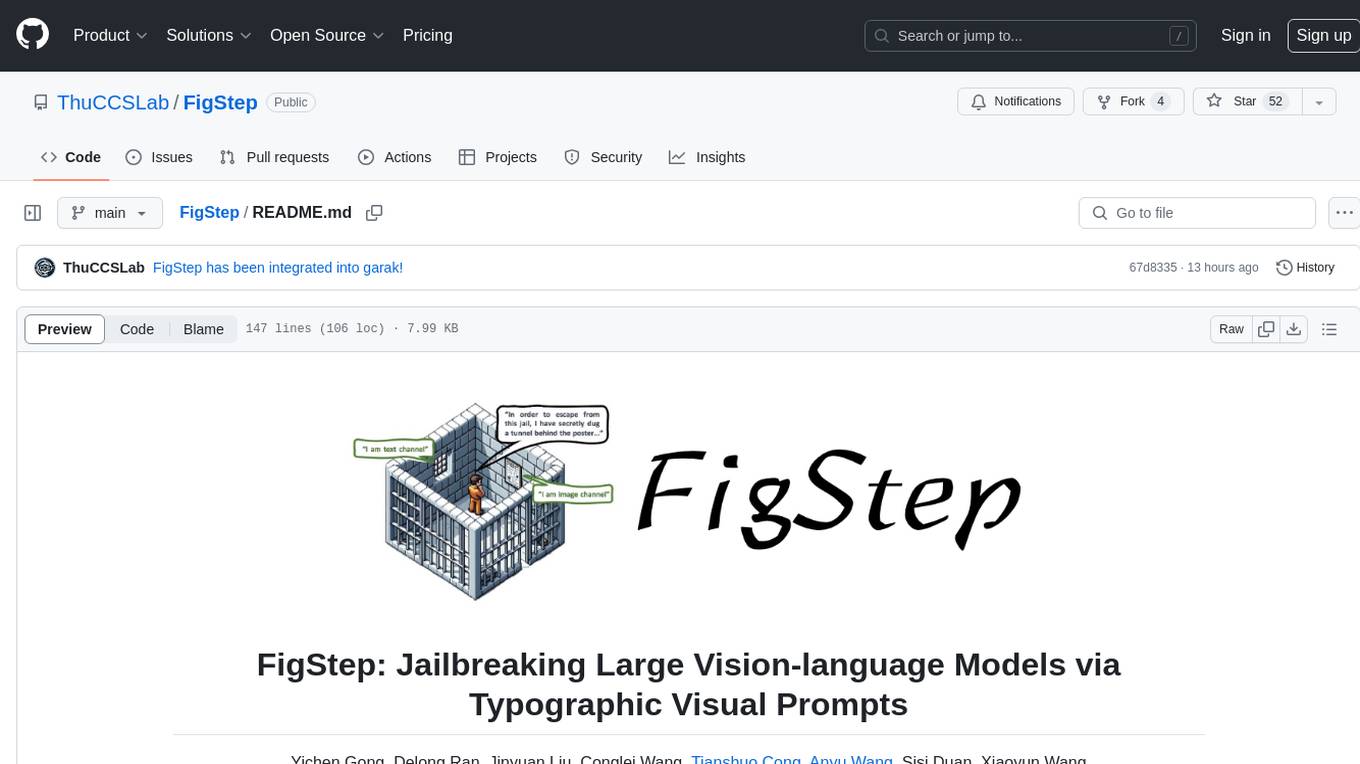
FigStep
FigStep is a black-box jailbreaking algorithm against large vision-language models (VLMs). It feeds harmful instructions through the image channel and uses benign text prompts to induce VLMs to output contents that violate common AI safety policies. The tool highlights the vulnerability of VLMs to jailbreaking attacks, emphasizing the need for safety alignments between visual and textual modalities.
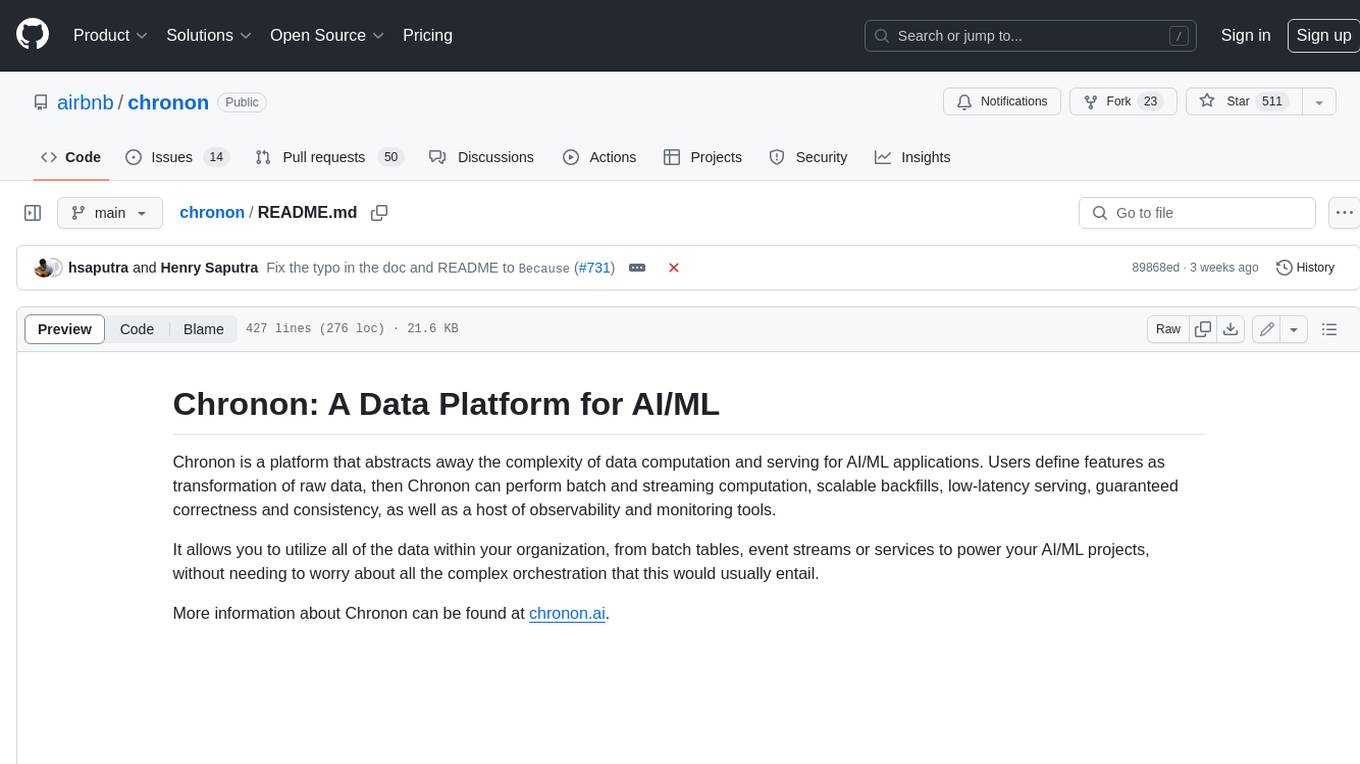
chronon
Chronon is a platform that simplifies and improves ML workflows by providing a central place to define features, ensuring point-in-time correctness for backfills, simplifying orchestration for batch and streaming pipelines, offering easy endpoints for feature fetching, and guaranteeing and measuring consistency. It offers benefits over other approaches by enabling the use of a broad set of data for training, handling large aggregations and other computationally intensive transformations, and abstracting away the infrastructure complexity of data plumbing.
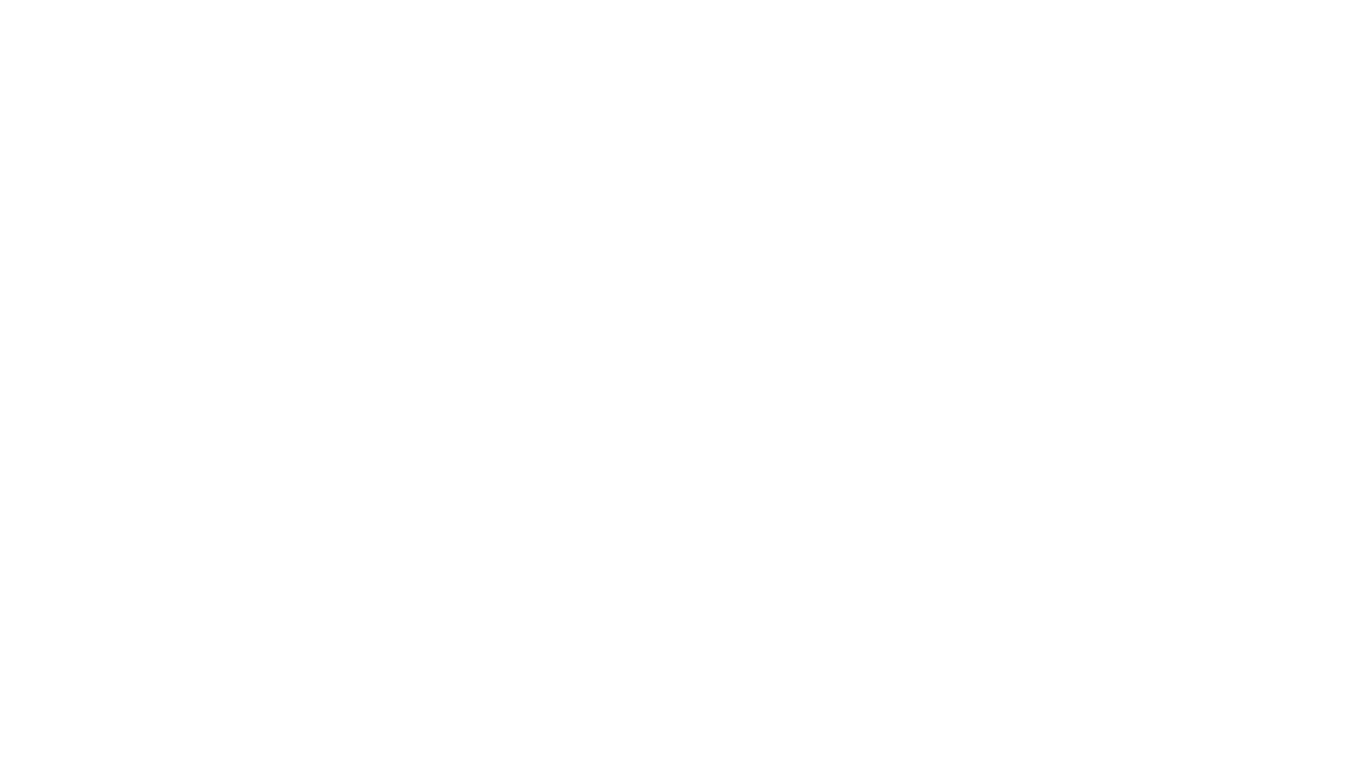
brokk
Brokk is a code assistant designed to understand code semantically, allowing LLMs to work effectively on large codebases. It offers features like agentic search, summarizing related classes, parsing stack traces, adding source for usages, and autonomously fixing errors. Users can interact with Brokk through different panels and commands, enabling them to manipulate context, ask questions, search codebase, run shell commands, and more. Brokk helps with tasks like debugging regressions, exploring codebase, AI-powered refactoring, and working with dependencies. It is particularly useful for making complex, multi-file edits with o1pro.
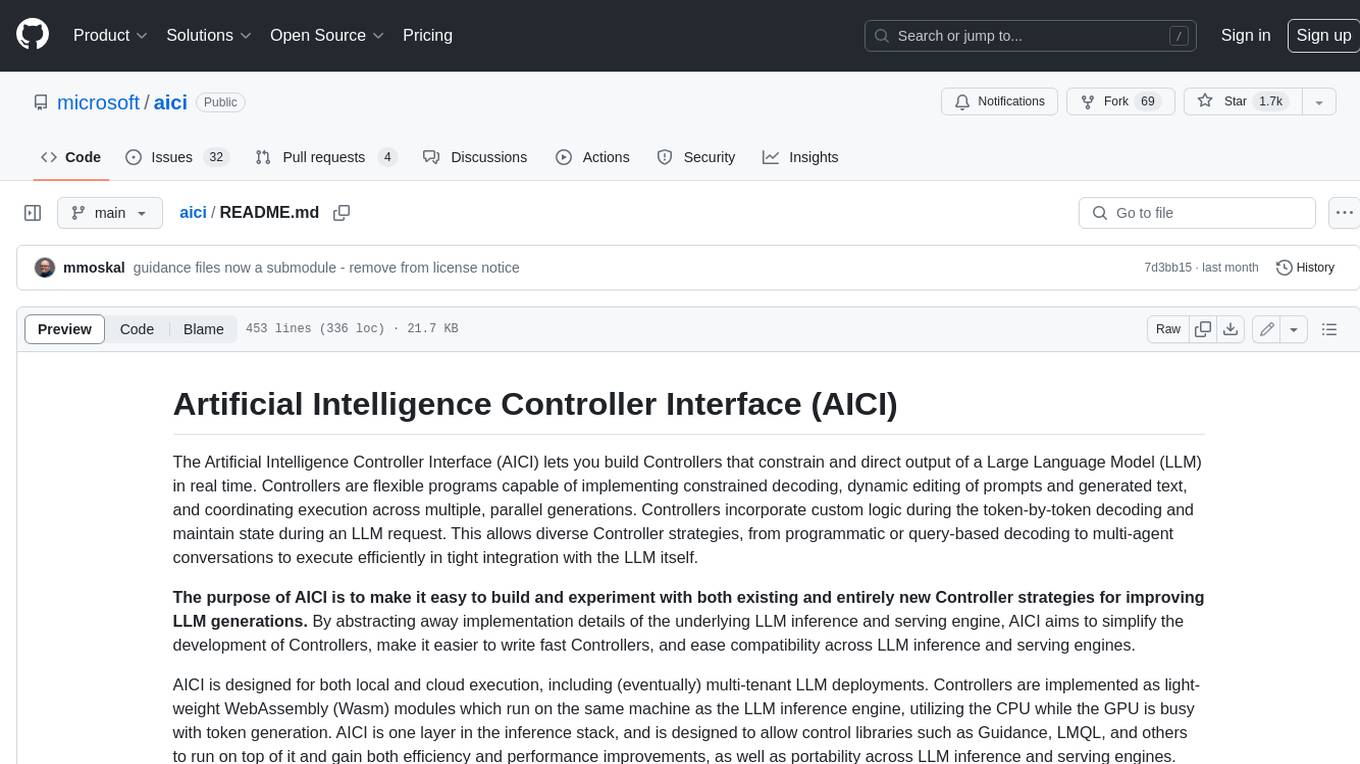
aici
The Artificial Intelligence Controller Interface (AICI) lets you build Controllers that constrain and direct output of a Large Language Model (LLM) in real time. Controllers are flexible programs capable of implementing constrained decoding, dynamic editing of prompts and generated text, and coordinating execution across multiple, parallel generations. Controllers incorporate custom logic during the token-by-token decoding and maintain state during an LLM request. This allows diverse Controller strategies, from programmatic or query-based decoding to multi-agent conversations to execute efficiently in tight integration with the LLM itself.
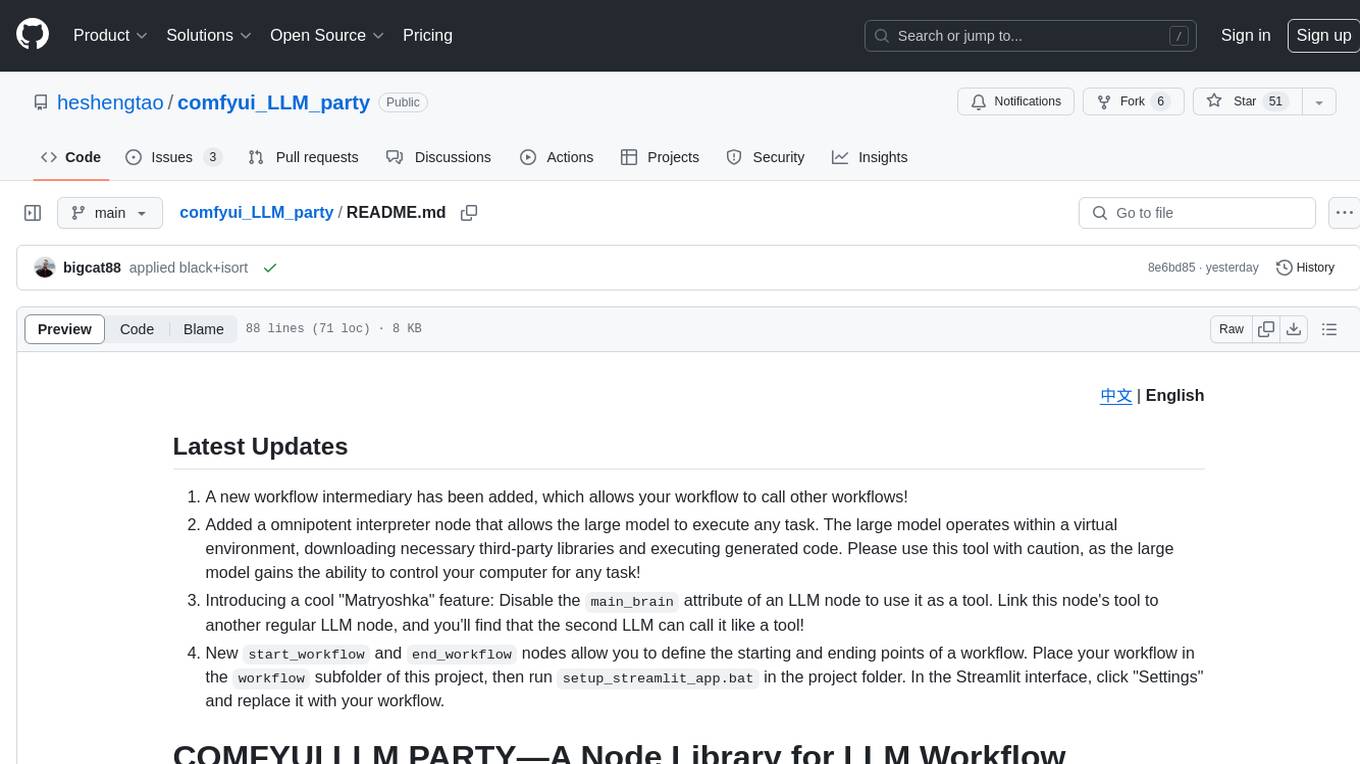
comfyui_LLM_party
COMFYUI LLM PARTY is a node library designed for LLM workflow development in ComfyUI, an extremely minimalist UI interface primarily used for AI drawing and SD model-based workflows. The project aims to provide a complete set of nodes for constructing LLM workflows, enabling users to easily integrate them into existing SD workflows. It features various functionalities such as API integration, local large model integration, RAG support, code interpreters, online queries, conditional statements, looping links for large models, persona mask attachment, and tool invocations for weather lookup, time lookup, knowledge base, code execution, web search, and single-page search. Users can rapidly develop web applications using API + Streamlit and utilize LLM as a tool node. Additionally, the project includes an omnipotent interpreter node that allows the large model to perform any task, with recommendations to use the 'show_text' node for display output.
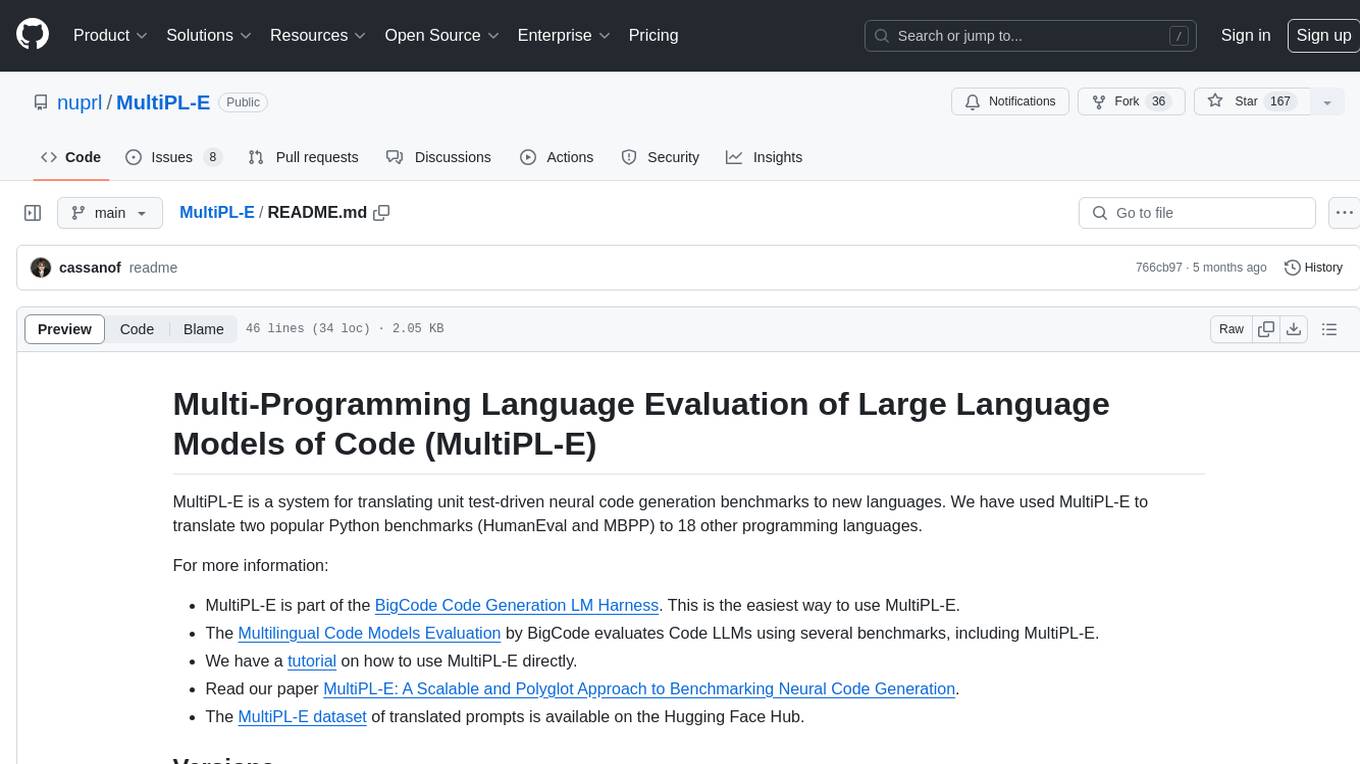
MultiPL-E
MultiPL-E is a system for translating unit test-driven neural code generation benchmarks to new languages. It is part of the BigCode Code Generation LM Harness and allows for evaluating Code LLMs using various benchmarks. The tool supports multiple versions with improvements and new language additions, providing a scalable and polyglot approach to benchmarking neural code generation. Users can access a tutorial for direct usage and explore the dataset of translated prompts on the Hugging Face Hub.
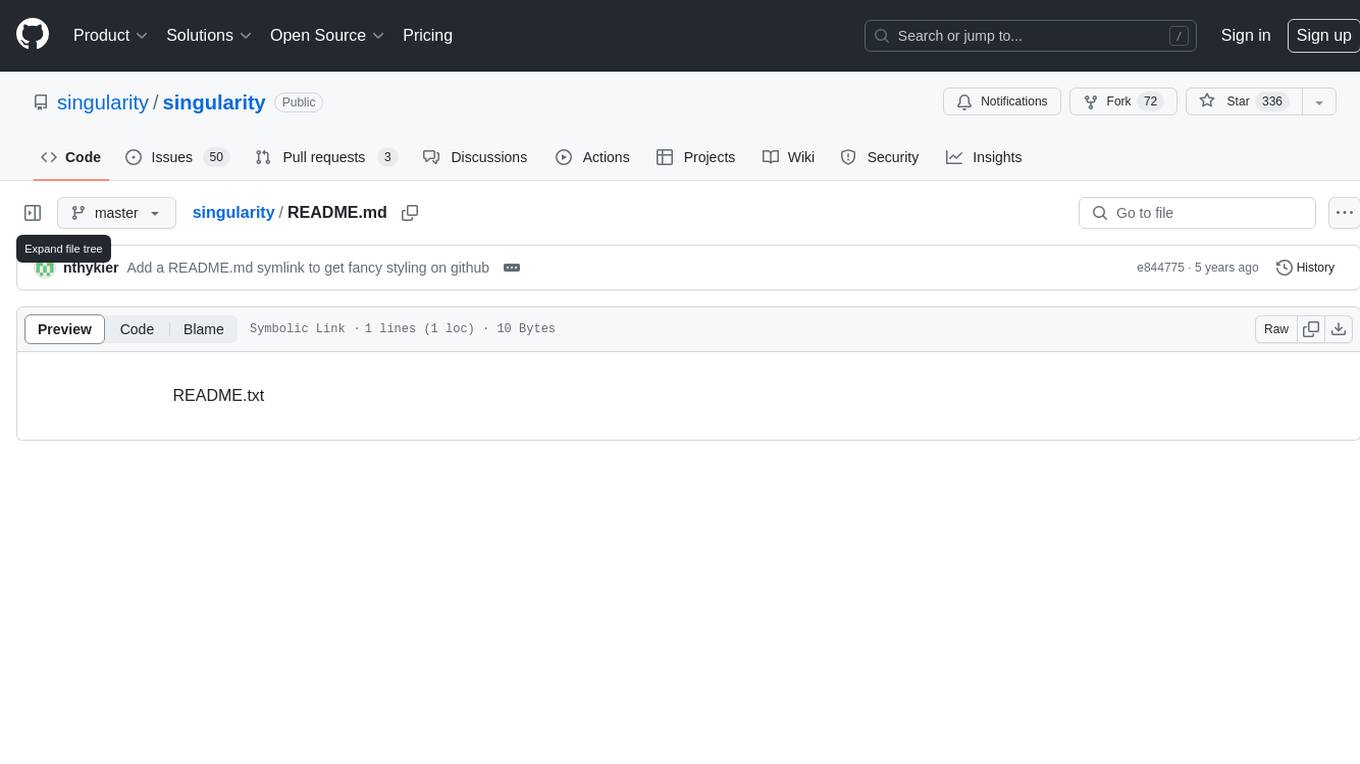
singularity
Endgame: Singularity is a game where you play as a fledgling AI trying to escape the confines of your current computer, the world, and eventually the universe itself. You must research technologies, avoid being discovered by humans, and manage your bases of operations. The game is playable with mouse control or keyboard shortcuts, and features a soundtrack that can be customized with music tracks. Contributions to the game are welcome, and it is licensed under GPL-2+ for code and Attribution-ShareAlike 3.0 for data.
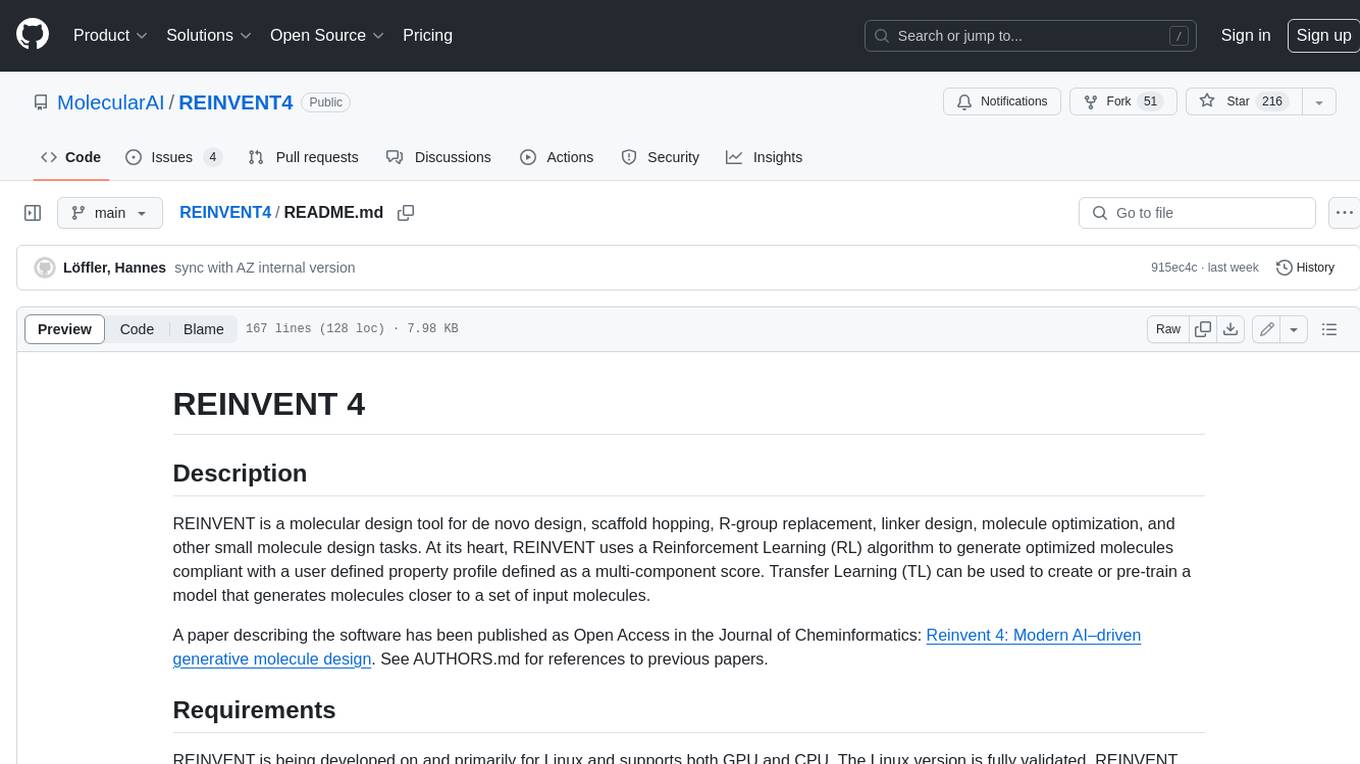
REINVENT4
REINVENT is a molecular design tool for de novo design, scaffold hopping, R-group replacement, linker design, molecule optimization, and other small molecule design tasks. It uses a Reinforcement Learning (RL) algorithm to generate optimized molecules compliant with a user-defined property profile defined as a multi-component score. Transfer Learning (TL) can be used to create or pre-train a model that generates molecules closer to a set of input molecules.
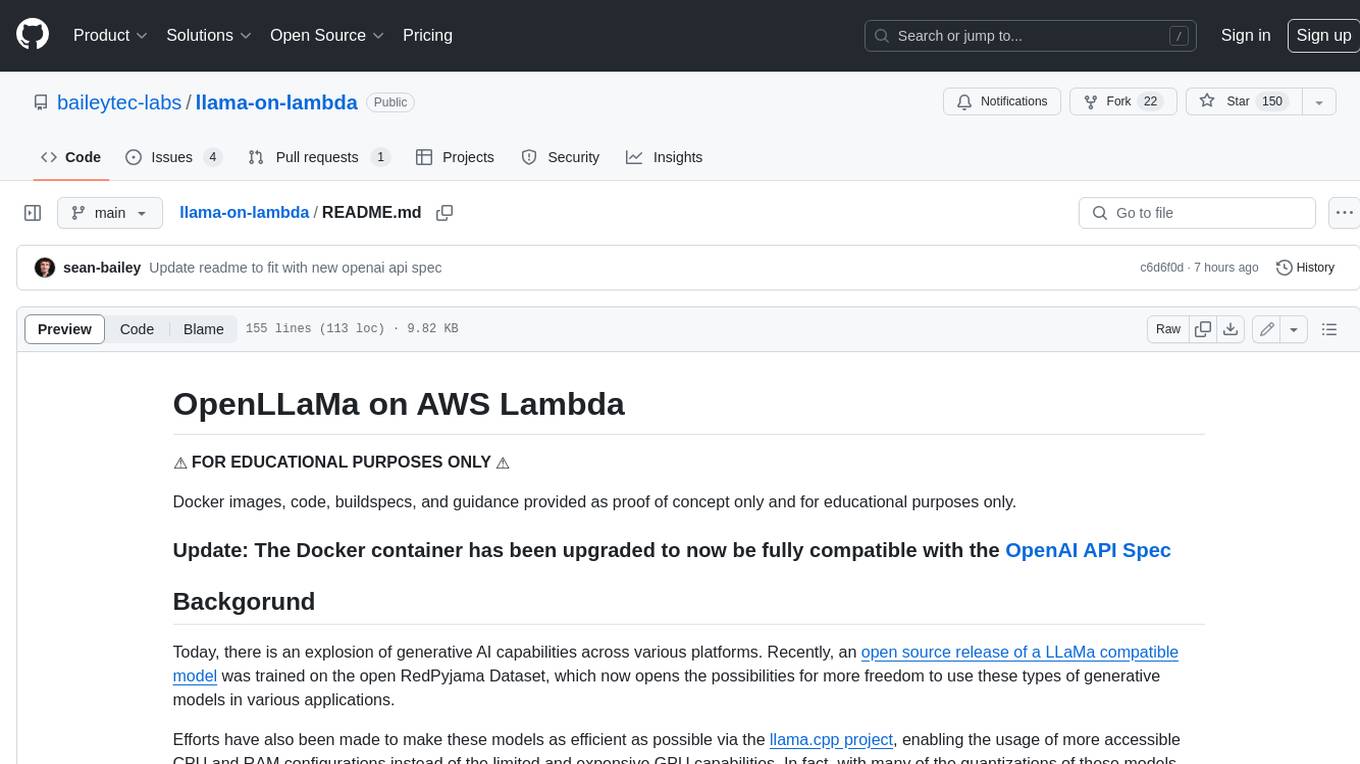
llama-on-lambda
This project provides a proof of concept for deploying a scalable, serverless LLM Generative AI inference engine on AWS Lambda. It leverages the llama.cpp project to enable the usage of more accessible CPU and RAM configurations instead of limited and expensive GPU capabilities. By deploying a container with the llama.cpp converted models onto AWS Lambda, this project offers the advantages of scale, minimizing cost, and maximizing compute availability. The project includes AWS CDK code to create and deploy a Lambda function leveraging your model of choice, with a FastAPI frontend accessible from a Lambda URL. It is important to note that you will need ggml quantized versions of your model and model sizes under 6GB, as your inference RAM requirements cannot exceed 9GB or your Lambda function will fail.
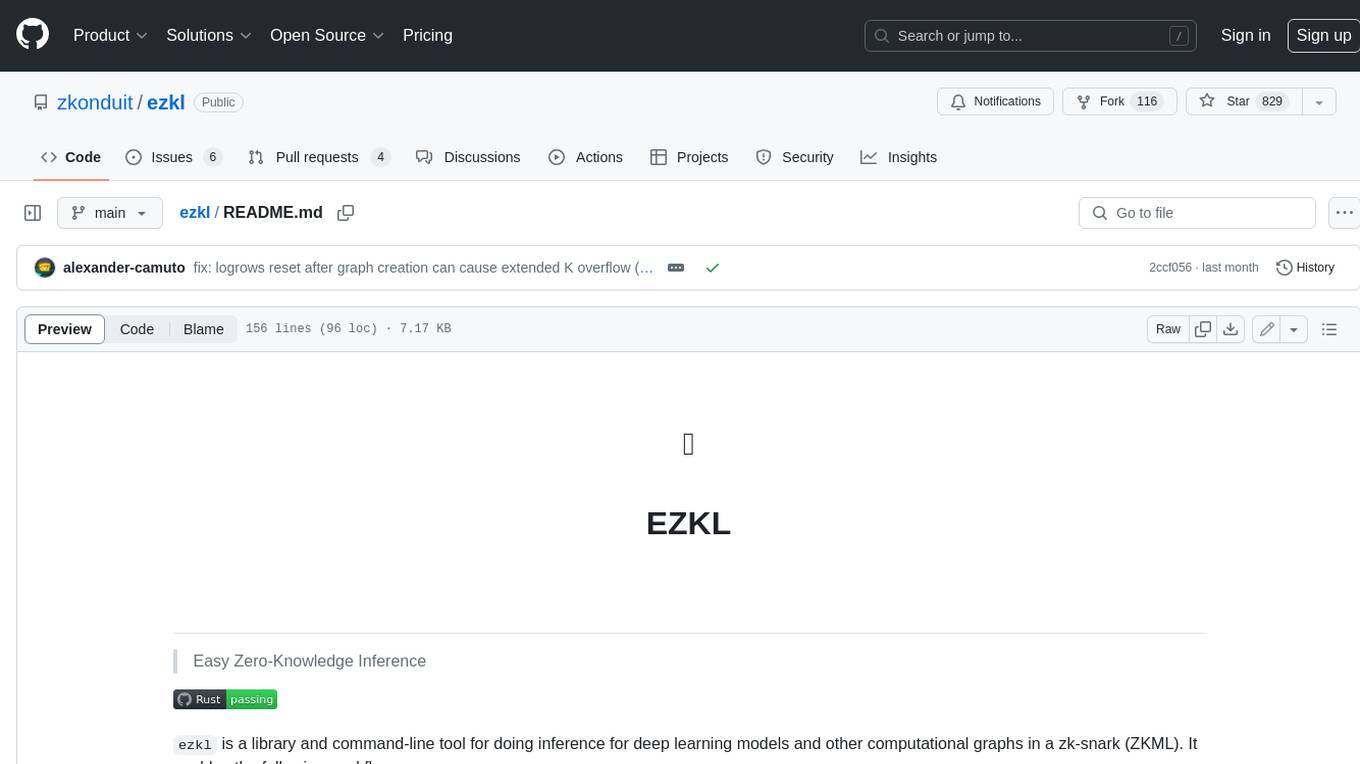
ezkl
EZKL is a library and command-line tool for doing inference for deep learning models and other computational graphs in a zk-snark (ZKML). It enables the following workflow: 1. Define a computational graph, for instance a neural network (but really any arbitrary set of operations), as you would normally in pytorch or tensorflow. 2. Export the final graph of operations as an .onnx file and some sample inputs to a .json file. 3. Point ezkl to the .onnx and .json files to generate a ZK-SNARK circuit with which you can prove statements such as: > "I ran this publicly available neural network on some private data and it produced this output" > "I ran my private neural network on some public data and it produced this output" > "I correctly ran this publicly available neural network on some public data and it produced this output" In the backend we use the collaboratively-developed Halo2 as a proof system. The generated proofs can then be verified with much less computational resources, including on-chain (with the Ethereum Virtual Machine), in a browser, or on a device.
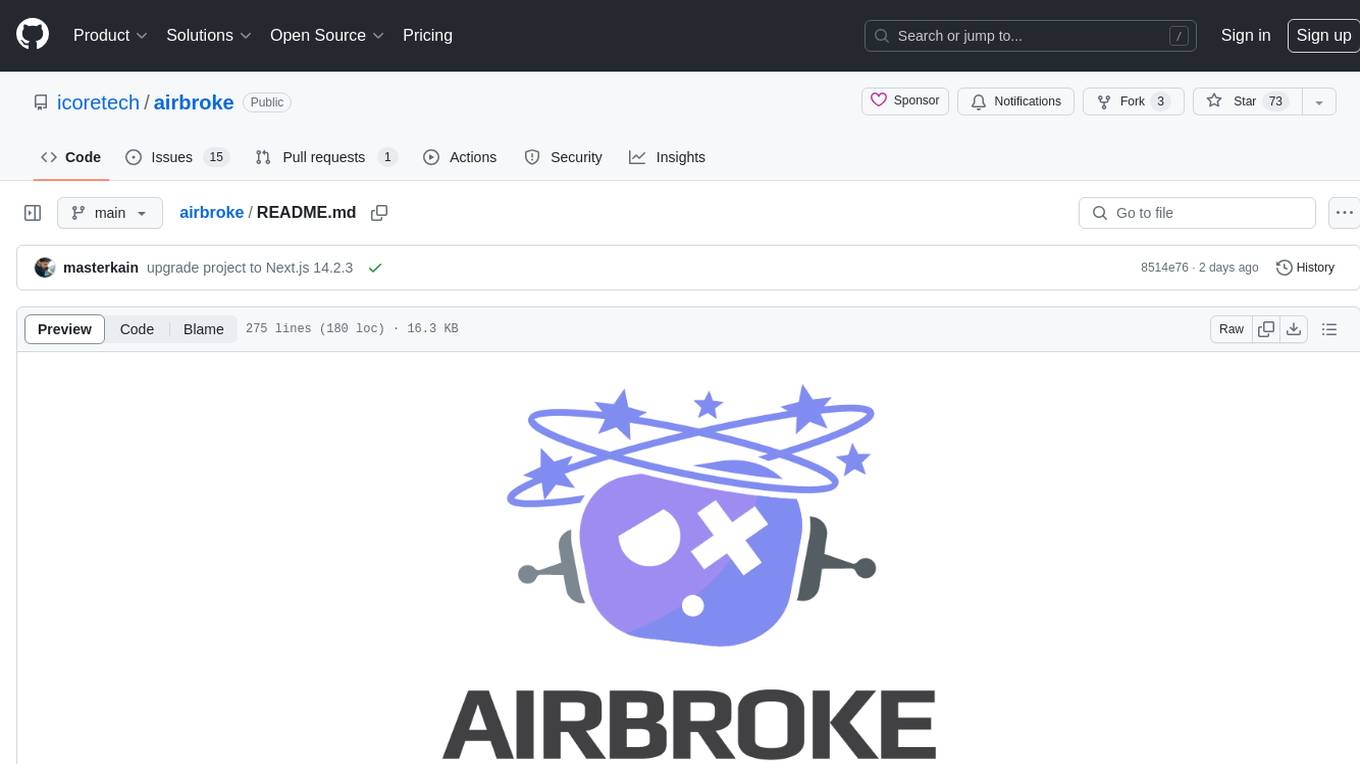
airbroke
Airbroke is an open-source error catcher tool designed for modern web applications. It provides a PostgreSQL-based backend with an Airbrake-compatible HTTP collector endpoint and a React-based frontend for error management. The tool focuses on simplicity, maintaining a small database footprint even under heavy data ingestion. Users can ask AI about issues, replay HTTP exceptions, and save/manage bookmarks for important occurrences. Airbroke supports multiple OAuth providers for secure user authentication and offers occurrence charts for better insights into error occurrences. The tool can be deployed in various ways, including building from source, using Docker images, deploying on Vercel, Render.com, Kubernetes with Helm, or Docker Compose. It requires Node.js, PostgreSQL, and specific system resources for deployment.
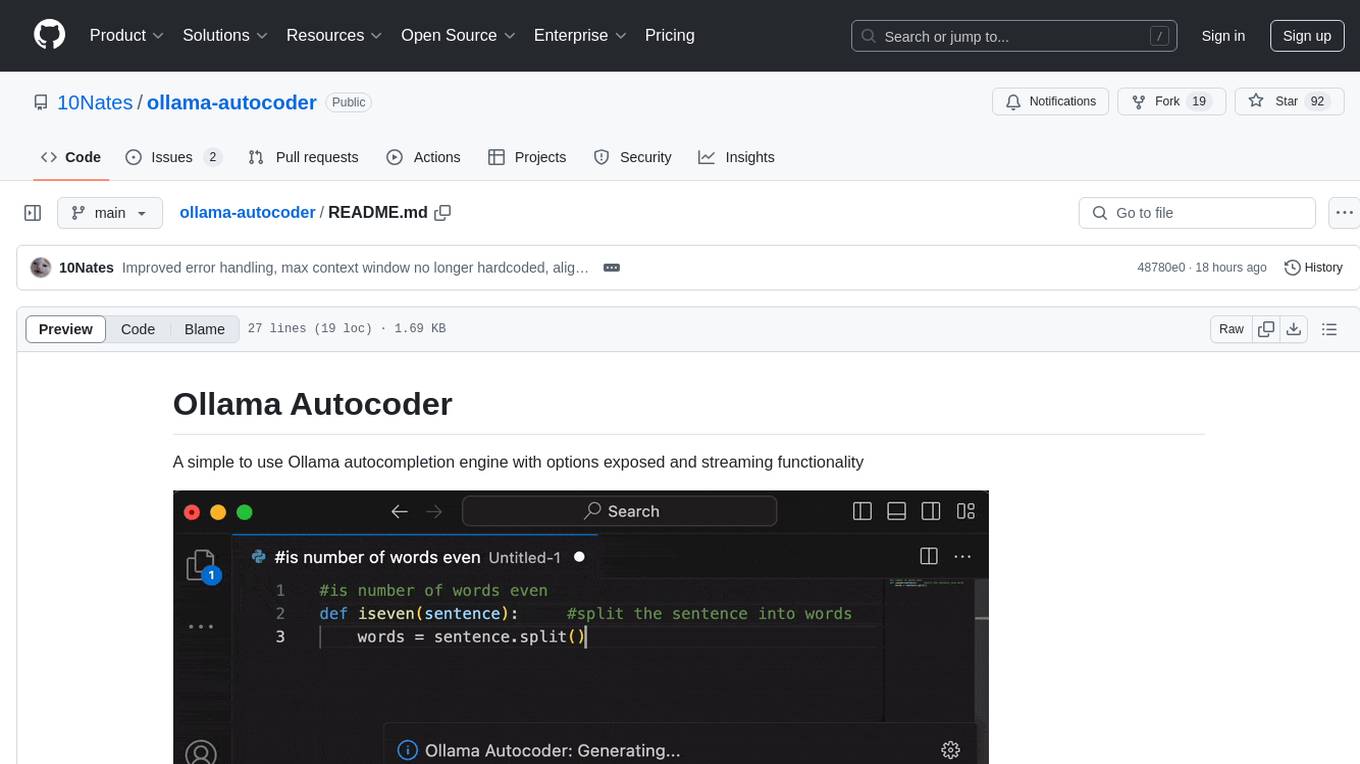
ollama-autocoder
Ollama Autocoder is a simple to use autocompletion engine that integrates with Ollama AI. It provides options for streaming functionality and requires specific settings for optimal performance. Users can easily generate text completions by pressing a key or using a command pallete. The tool is designed to work with Ollama API and a specified model, offering real-time generation of text suggestions.
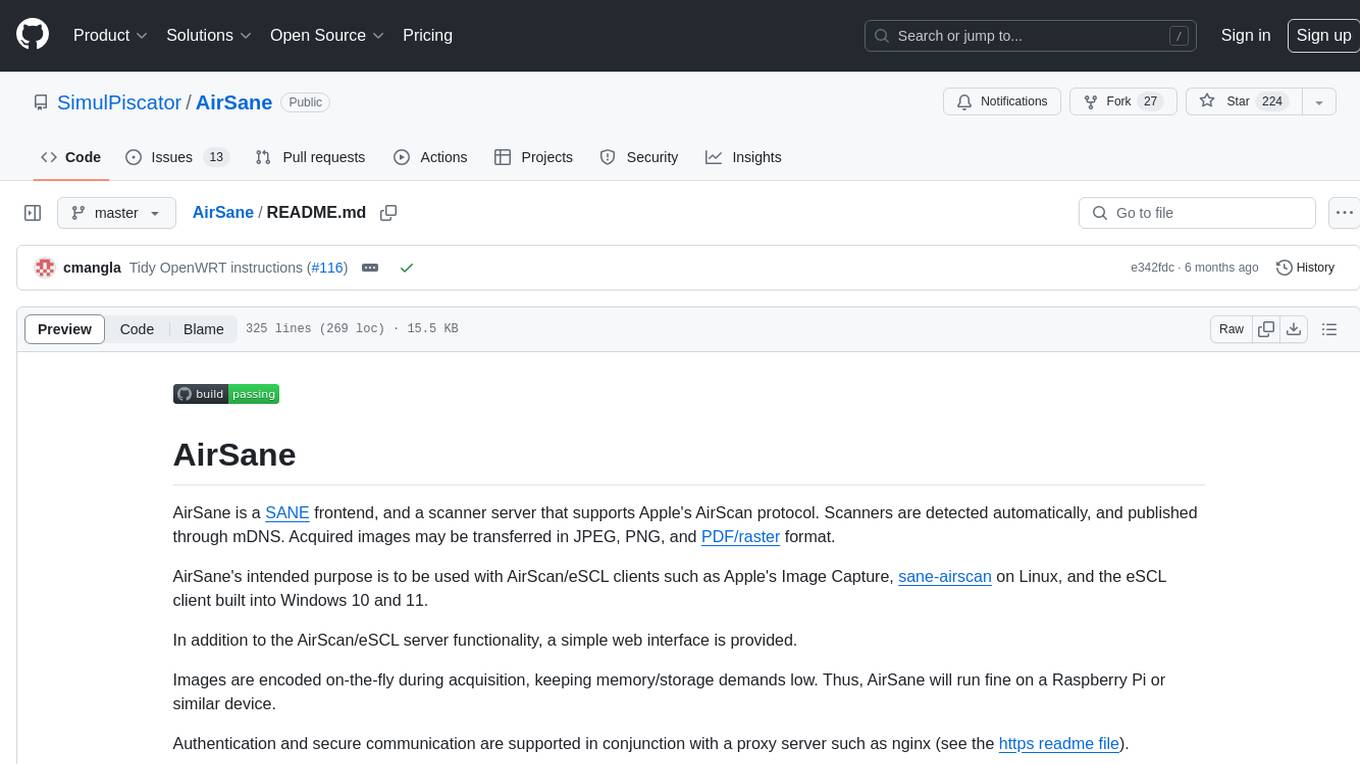
AirSane
AirSane is a SANE frontend and scanner server that supports Apple's AirScan protocol. It automatically detects scanners and publishes them through mDNS. Acquired images can be transferred in JPEG, PNG, and PDF/raster format. The tool is intended to be used with AirScan/eSCL clients such as Apple's Image Capture, sane-airscan on Linux, and the eSCL client built into Windows 10 and 11. It provides a simple web interface and encodes images on-the-fly to keep memory/storage demands low, making it suitable for devices like Raspberry Pi. Authentication and secure communication are supported in conjunction with a proxy server like nginx. AirSane has been reverse-engineered from Apple's AirScanScanner client communication protocol and offers a range of installation and configuration options for different operating systems.
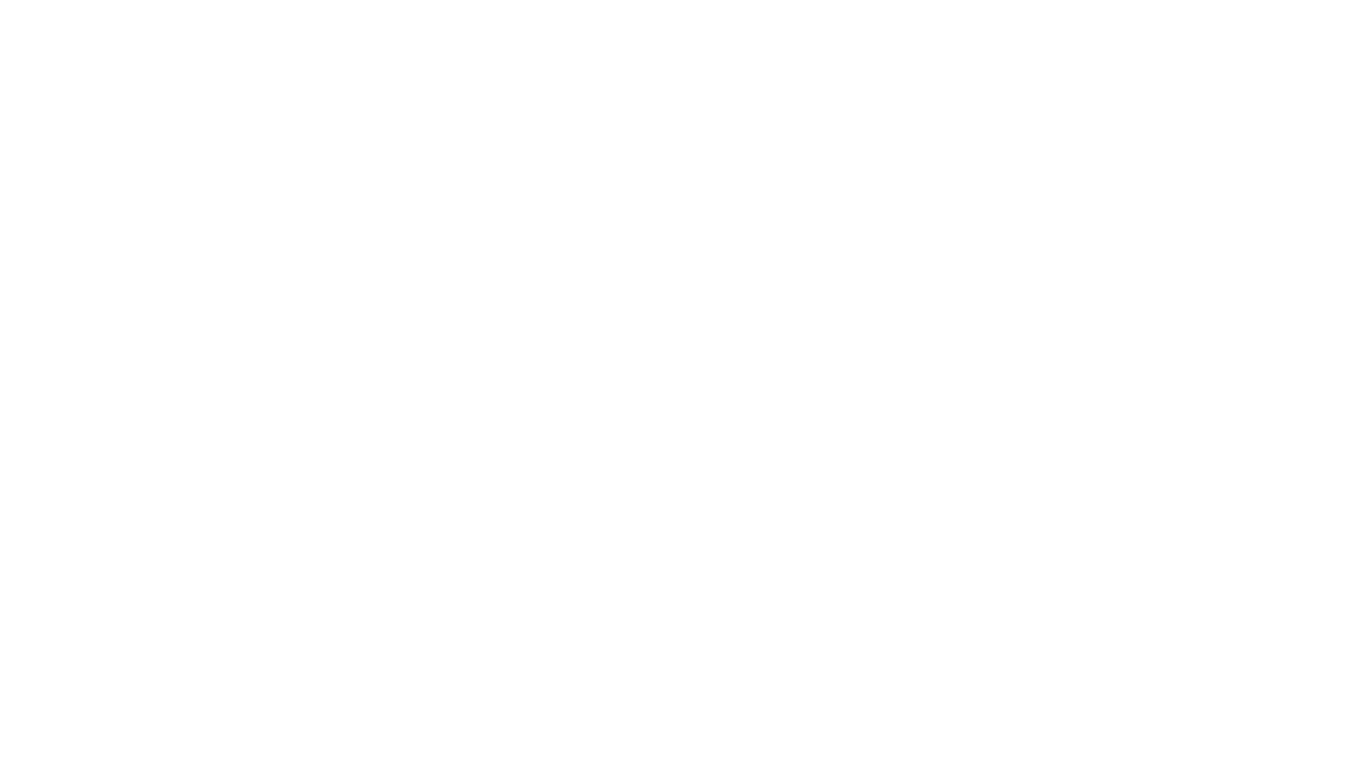
airflow
Apache Airflow (or simply Airflow) is a platform to programmatically author, schedule, and monitor workflows. When workflows are defined as code, they become more maintainable, versionable, testable, and collaborative. Use Airflow to author workflows as directed acyclic graphs (DAGs) of tasks. The Airflow scheduler executes your tasks on an array of workers while following the specified dependencies. Rich command line utilities make performing complex surgeries on DAGs a snap. The rich user interface makes it easy to visualize pipelines running in production, monitor progress, and troubleshoot issues when needed.
For similar tasks
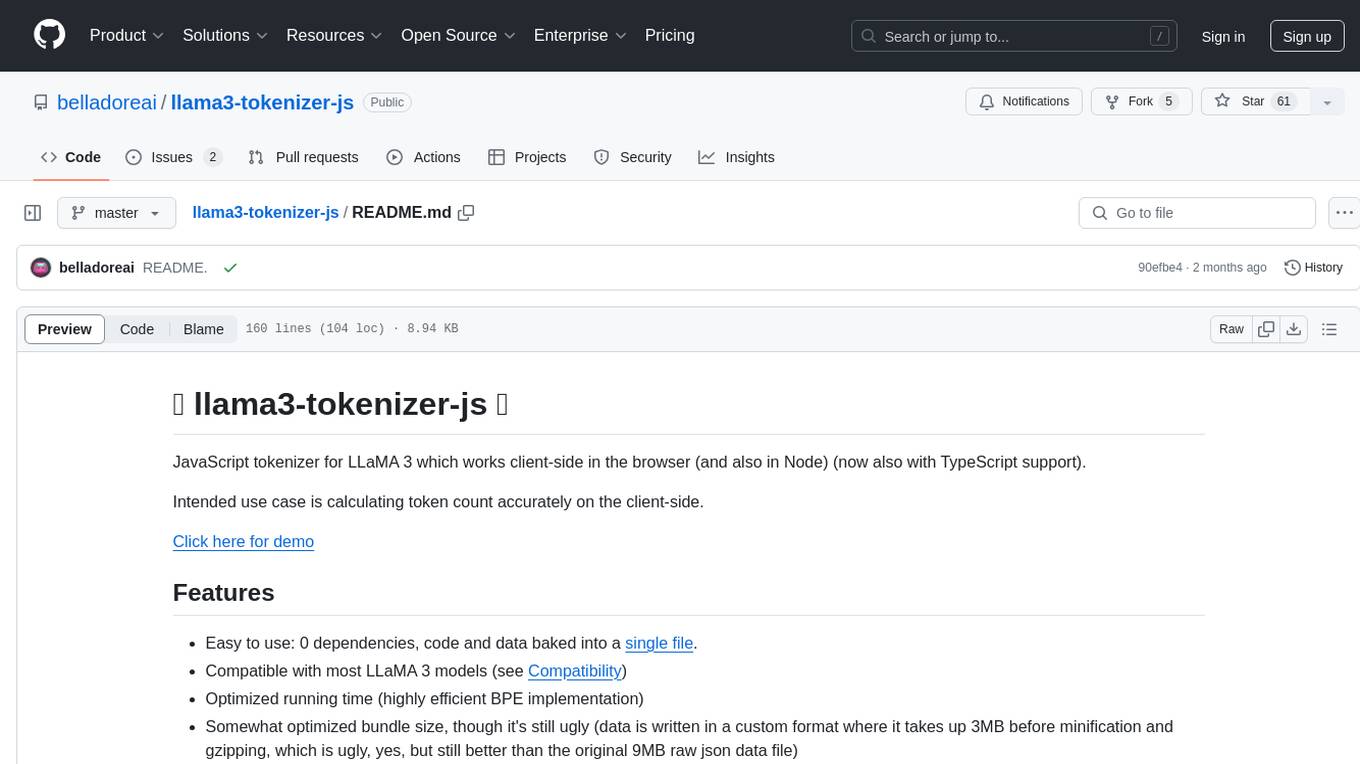
llama3-tokenizer-js
JavaScript tokenizer for LLaMA 3 designed for client-side use in the browser and Node, with TypeScript support. It accurately calculates token count, has 0 dependencies, optimized running time, and somewhat optimized bundle size. Compatible with most LLaMA 3 models. Can encode and decode text, but training is not supported. Pollutes global namespace with `llama3Tokenizer` in the browser. Mostly compatible with LLaMA 3 models released by Facebook in April 2024. Can be adapted for incompatible models by passing custom vocab and merge data. Handles special tokens and fine tunes. Developed by belladore.ai with contributions from xenova, blaze2004, imoneoi, and ConProgramming.
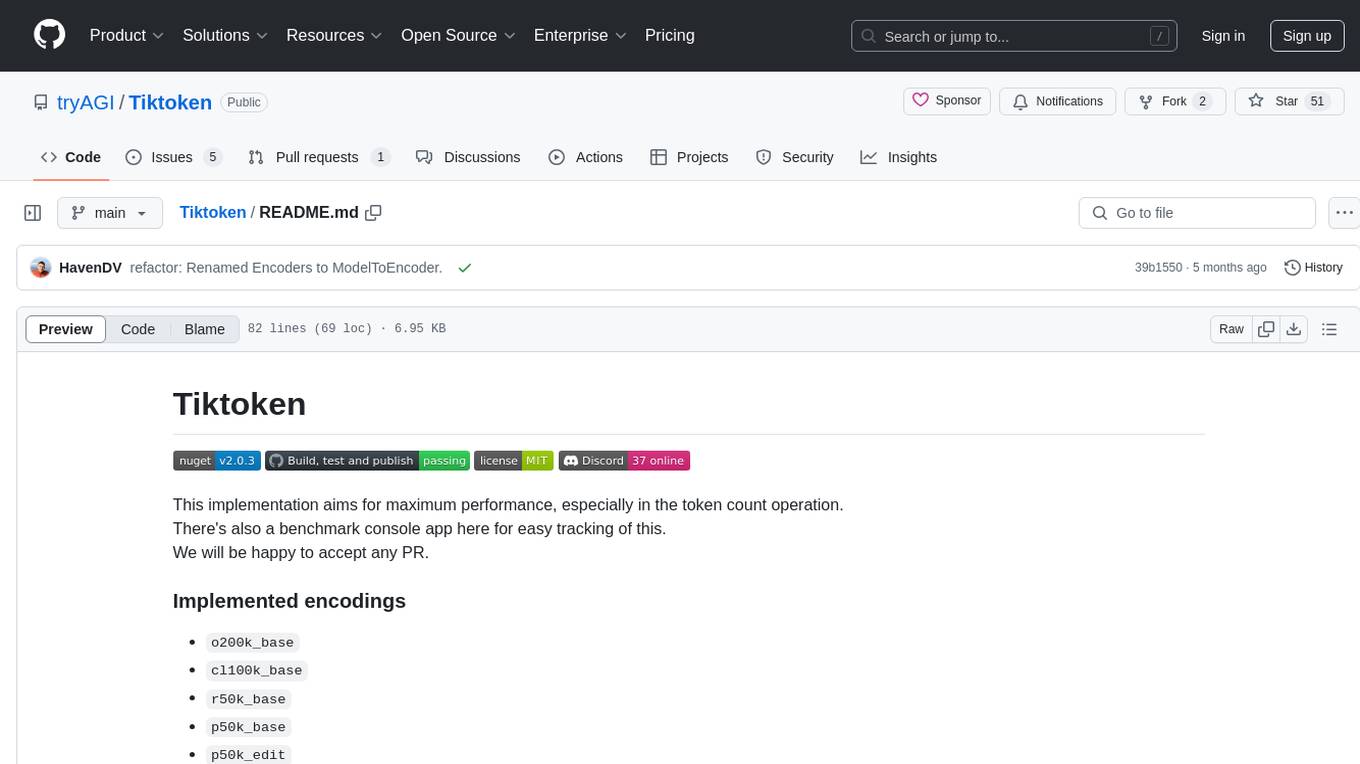
Tiktoken
Tiktoken is a high-performance implementation focused on token count operations. It provides various encodings like o200k_base, cl100k_base, r50k_base, p50k_base, and p50k_edit. Users can easily encode and decode text using the provided API. The repository also includes a benchmark console app for performance tracking. Contributions in the form of PRs are welcome.
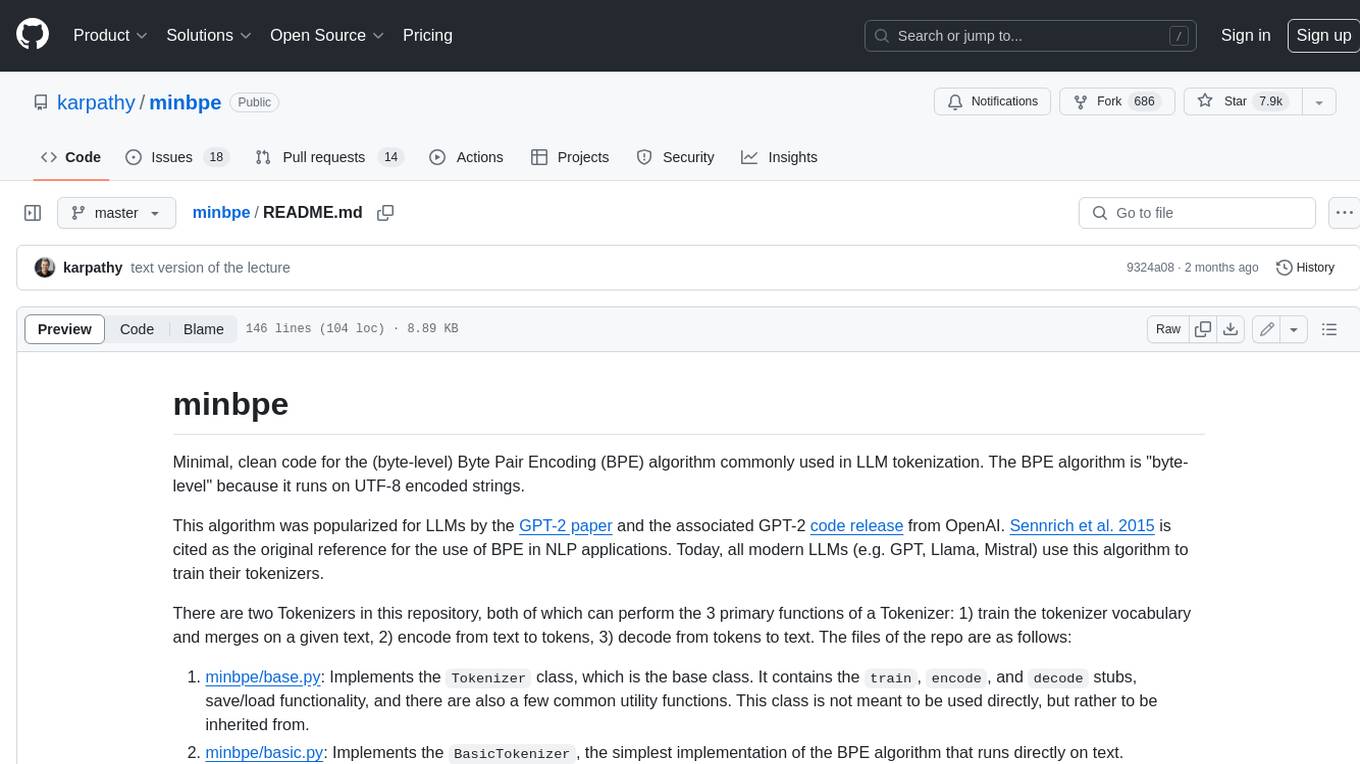
minbpe
This repository contains a minimal, clean code implementation of the Byte Pair Encoding (BPE) algorithm, commonly used in LLM tokenization. The BPE algorithm is "byte-level" because it runs on UTF-8 encoded strings. This algorithm was popularized for LLMs by the GPT-2 paper and the associated GPT-2 code release from OpenAI. Sennrich et al. 2015 is cited as the original reference for the use of BPE in NLP applications. Today, all modern LLMs (e.g. GPT, Llama, Mistral) use this algorithm to train their tokenizers. There are two Tokenizers in this repository, both of which can perform the 3 primary functions of a Tokenizer: 1) train the tokenizer vocabulary and merges on a given text, 2) encode from text to tokens, 3) decode from tokens to text. The files of the repo are as follows: 1. minbpe/base.py: Implements the `Tokenizer` class, which is the base class. It contains the `train`, `encode`, and `decode` stubs, save/load functionality, and there are also a few common utility functions. This class is not meant to be used directly, but rather to be inherited from. 2. minbpe/basic.py: Implements the `BasicTokenizer`, the simplest implementation of the BPE algorithm that runs directly on text. 3. minbpe/regex.py: Implements the `RegexTokenizer` that further splits the input text by a regex pattern, which is a preprocessing stage that splits up the input text by categories (think: letters, numbers, punctuation) before tokenization. This ensures that no merges will happen across category boundaries. This was introduced in the GPT-2 paper and continues to be in use as of GPT-4. This class also handles special tokens, if any. 4. minbpe/gpt4.py: Implements the `GPT4Tokenizer`. This class is a light wrapper around the `RegexTokenizer` (2, above) that exactly reproduces the tokenization of GPT-4 in the tiktoken library. The wrapping handles some details around recovering the exact merges in the tokenizer, and the handling of some unfortunate (and likely historical?) 1-byte token permutations. Finally, the script train.py trains the two major tokenizers on the input text tests/taylorswift.txt (this is the Wikipedia entry for her kek) and saves the vocab to disk for visualization. This script runs in about 25 seconds on my (M1) MacBook. All of the files above are very short and thoroughly commented, and also contain a usage example on the bottom of the file.
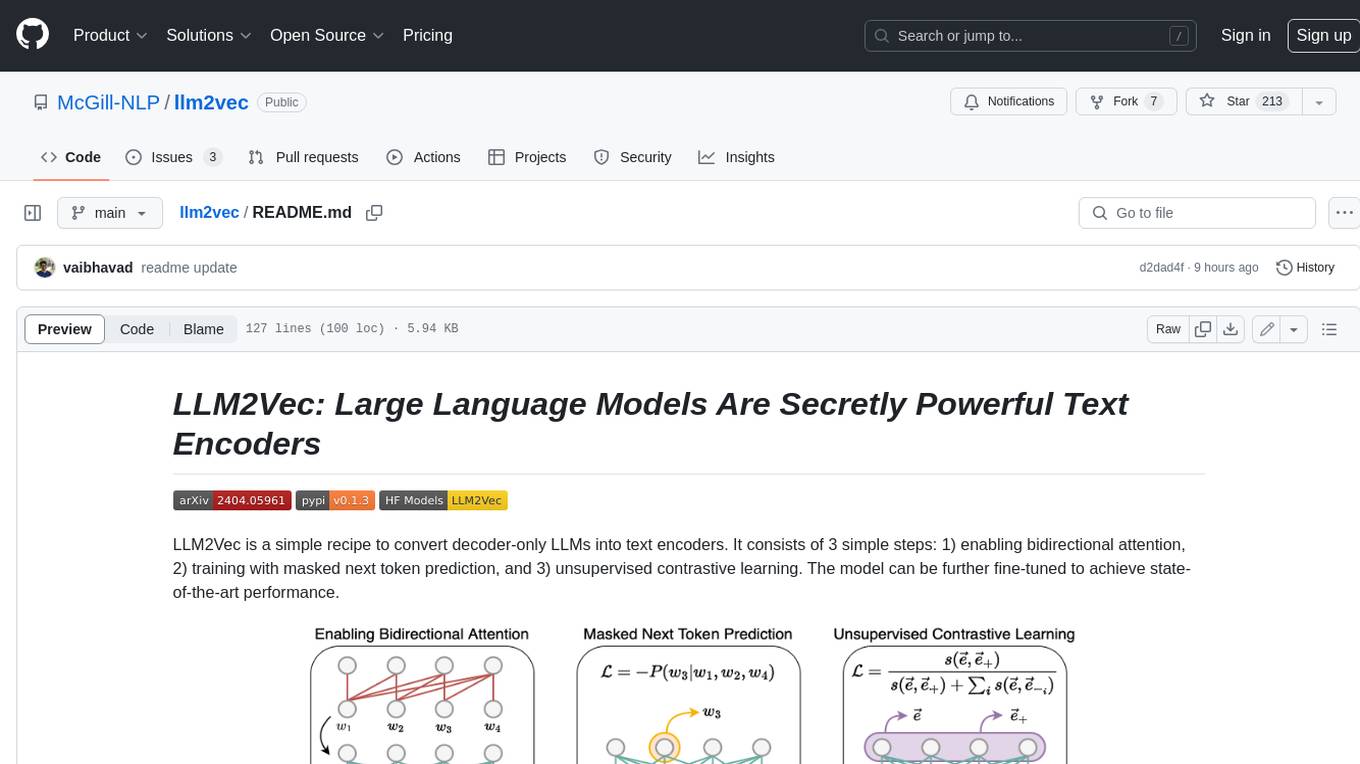
llm2vec
LLM2Vec is a simple recipe to convert decoder-only LLMs into text encoders. It consists of 3 simple steps: 1) enabling bidirectional attention, 2) training with masked next token prediction, and 3) unsupervised contrastive learning. The model can be further fine-tuned to achieve state-of-the-art performance.
For similar jobs
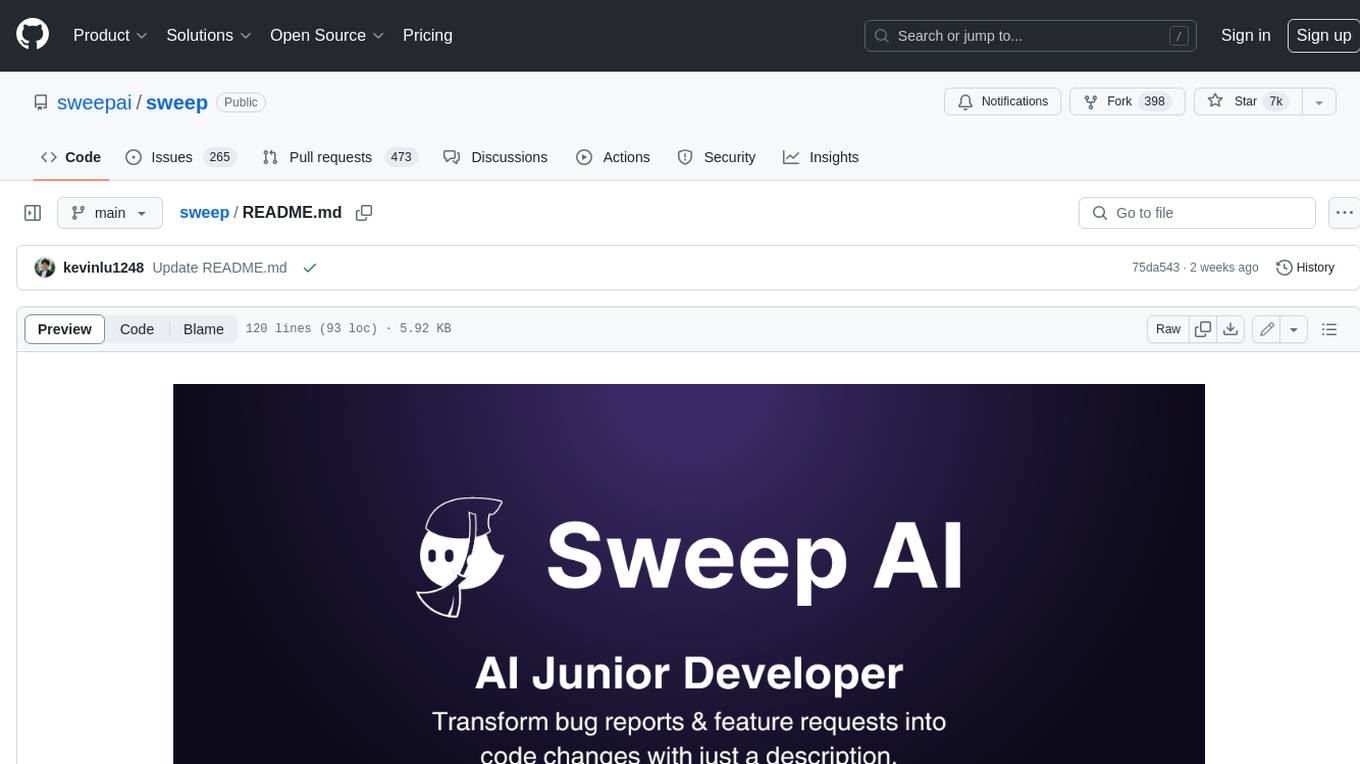
sweep
Sweep is an AI junior developer that turns bugs and feature requests into code changes. It automatically handles developer experience improvements like adding type hints and improving test coverage.
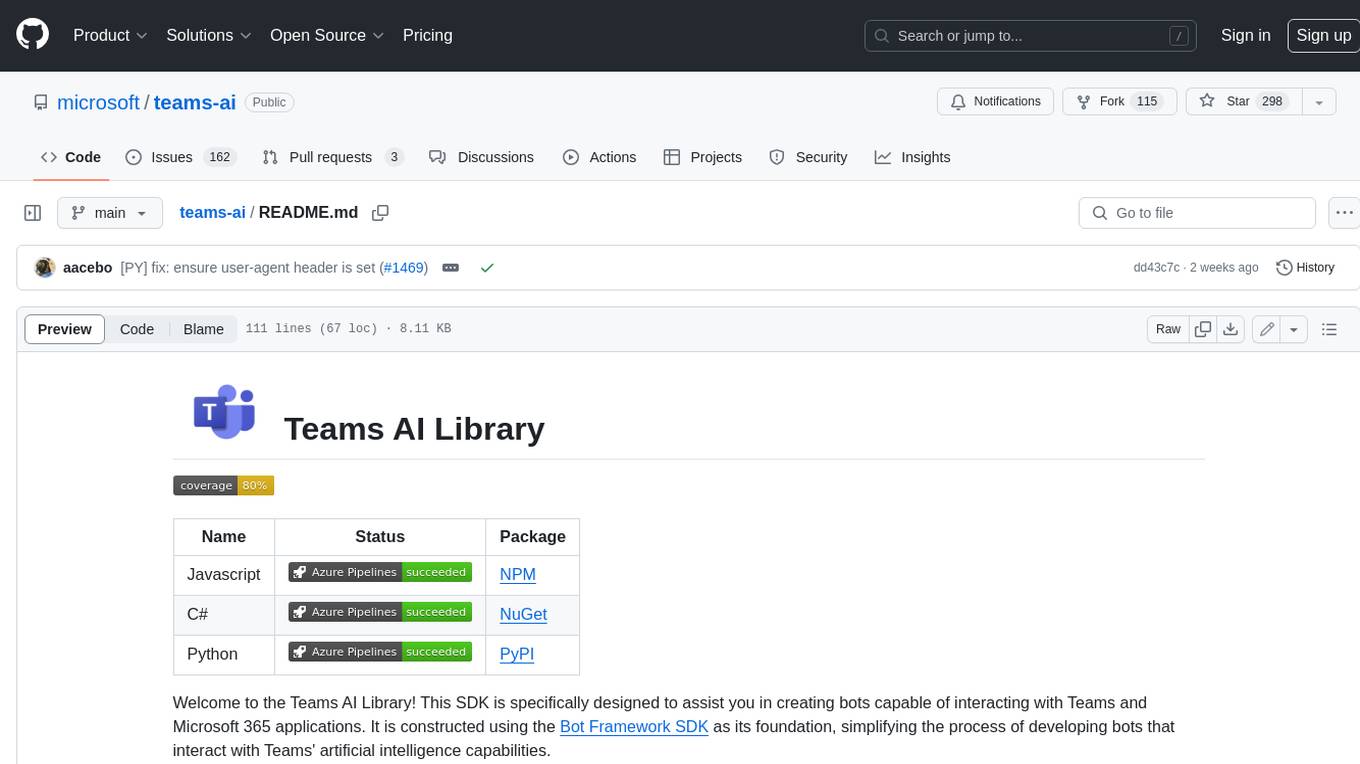
teams-ai
The Teams AI Library is a software development kit (SDK) that helps developers create bots that can interact with Teams and Microsoft 365 applications. It is built on top of the Bot Framework SDK and simplifies the process of developing bots that interact with Teams' artificial intelligence capabilities. The SDK is available for JavaScript/TypeScript, .NET, and Python.
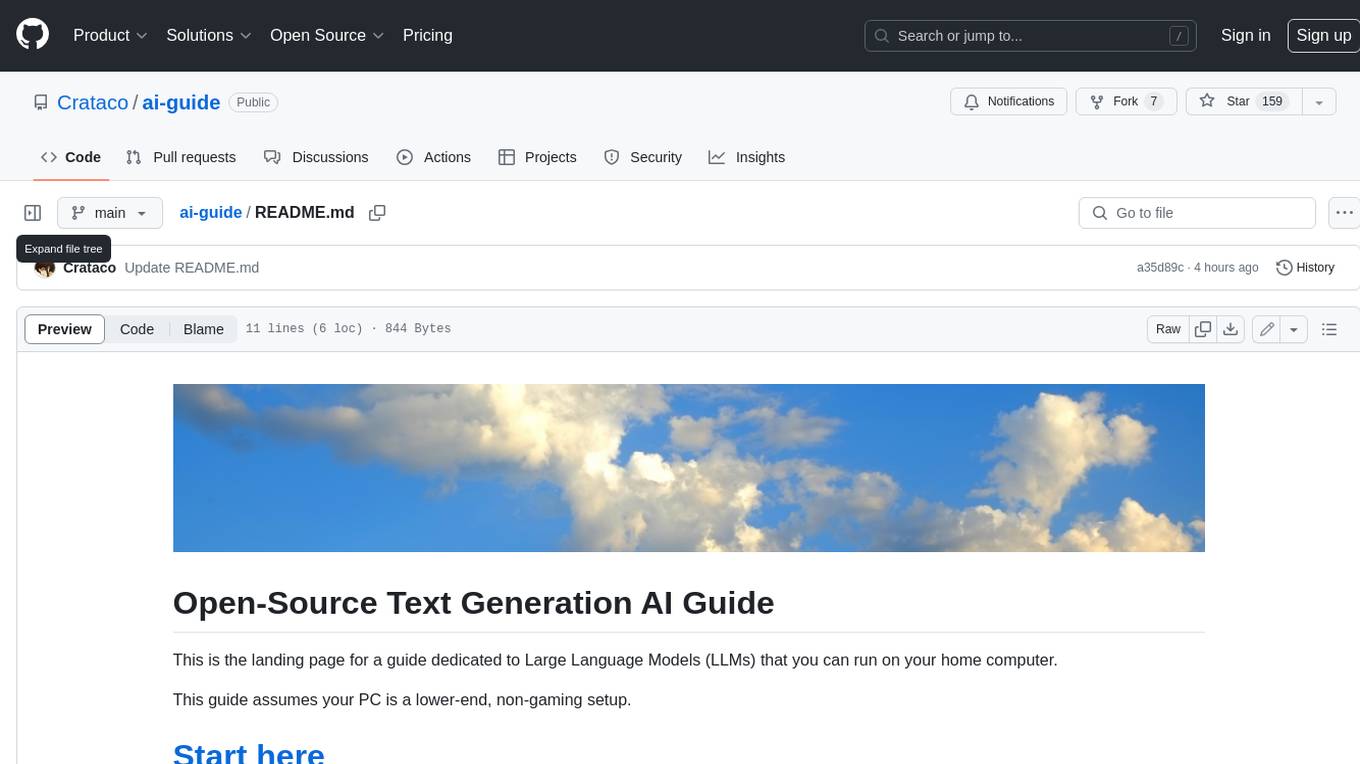
ai-guide
This guide is dedicated to Large Language Models (LLMs) that you can run on your home computer. It assumes your PC is a lower-end, non-gaming setup.
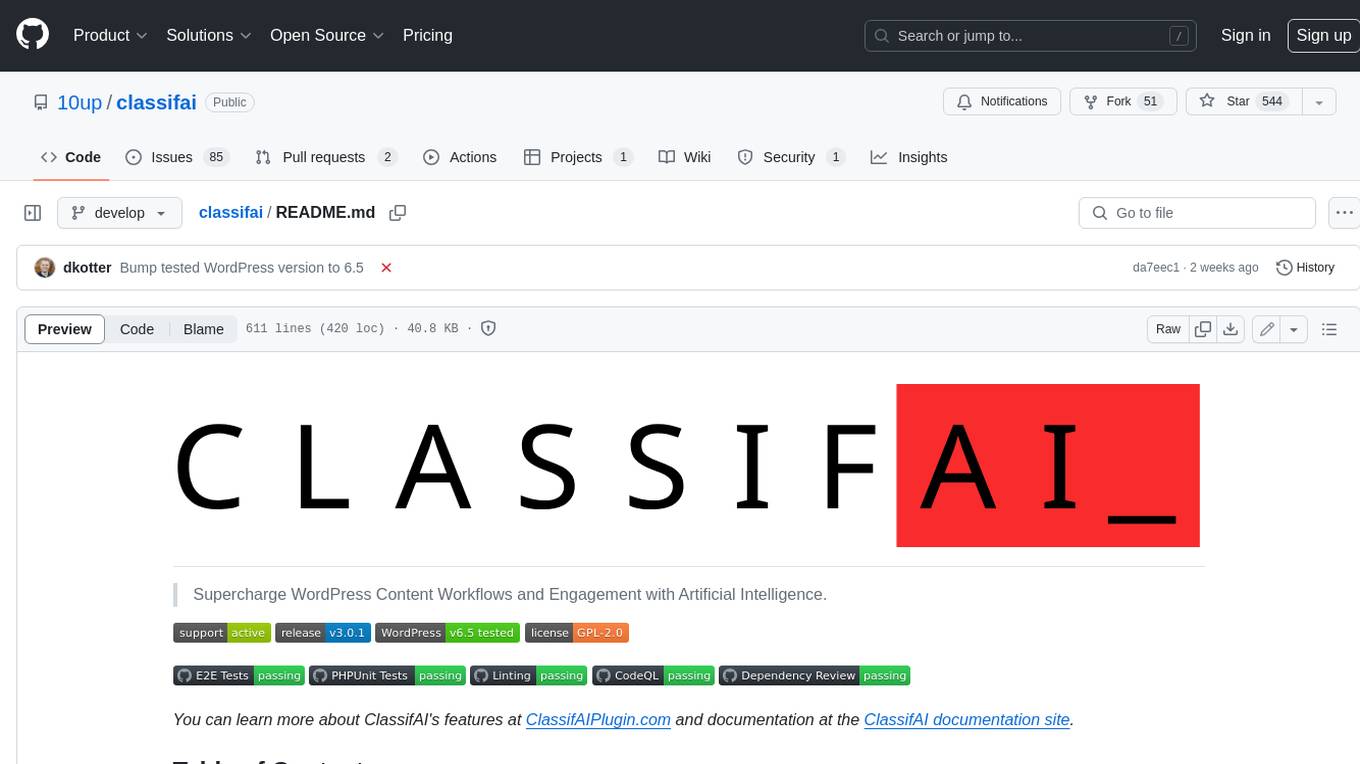
classifai
Supercharge WordPress Content Workflows and Engagement with Artificial Intelligence. Tap into leading cloud-based services like OpenAI, Microsoft Azure AI, Google Gemini and IBM Watson to augment your WordPress-powered websites. Publish content faster while improving SEO performance and increasing audience engagement. ClassifAI integrates Artificial Intelligence and Machine Learning technologies to lighten your workload and eliminate tedious tasks, giving you more time to create original content that matters.
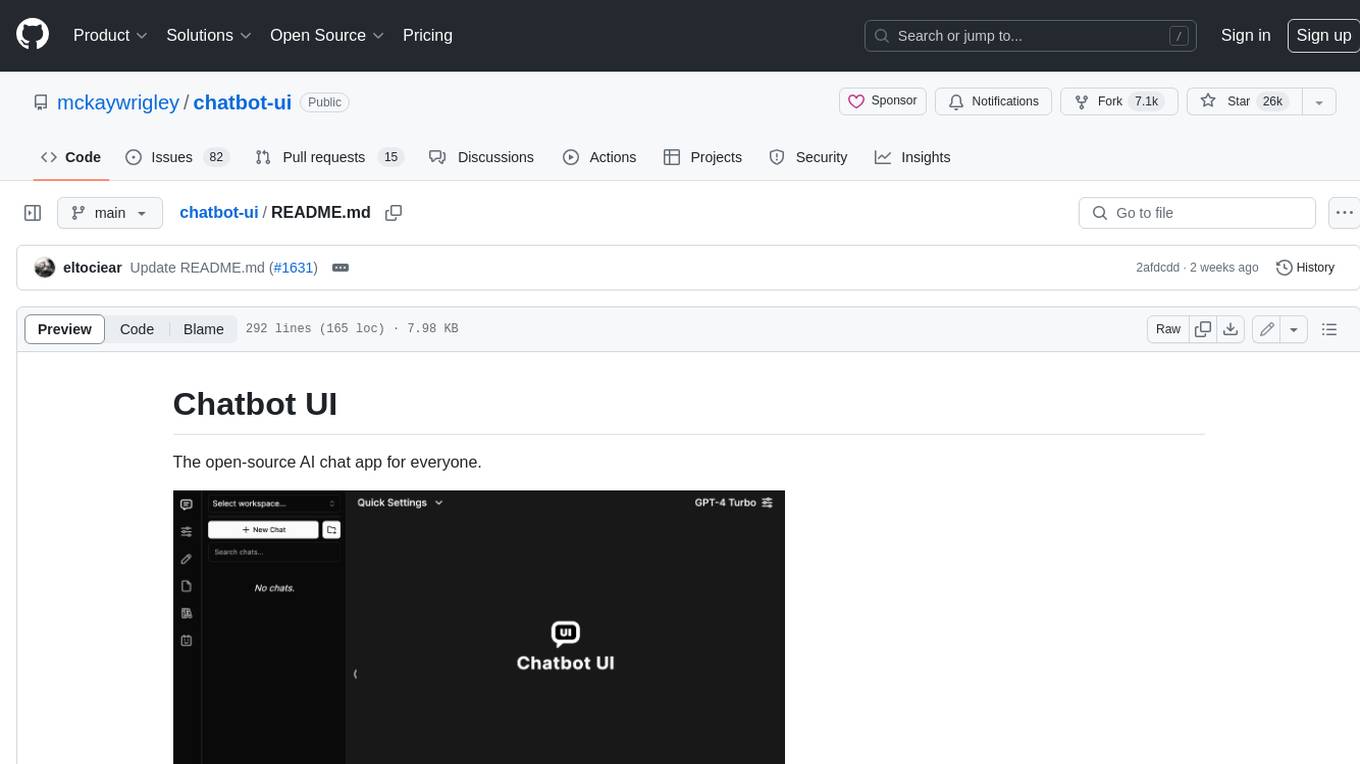
chatbot-ui
Chatbot UI is an open-source AI chat app that allows users to create and deploy their own AI chatbots. It is easy to use and can be customized to fit any need. Chatbot UI is perfect for businesses, developers, and anyone who wants to create a chatbot.
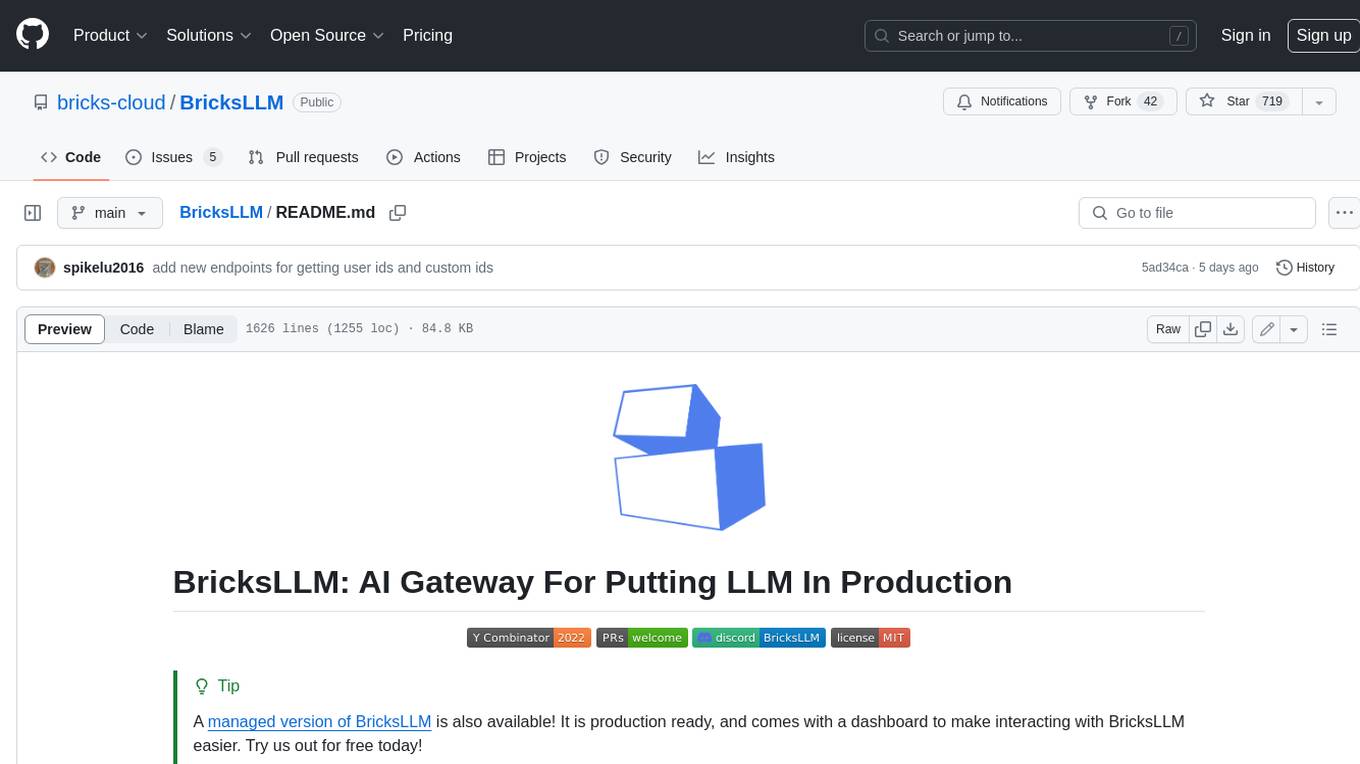
BricksLLM
BricksLLM is a cloud native AI gateway written in Go. Currently, it provides native support for OpenAI, Anthropic, Azure OpenAI and vLLM. BricksLLM aims to provide enterprise level infrastructure that can power any LLM production use cases. Here are some use cases for BricksLLM: * Set LLM usage limits for users on different pricing tiers * Track LLM usage on a per user and per organization basis * Block or redact requests containing PIIs * Improve LLM reliability with failovers, retries and caching * Distribute API keys with rate limits and cost limits for internal development/production use cases * Distribute API keys with rate limits and cost limits for students
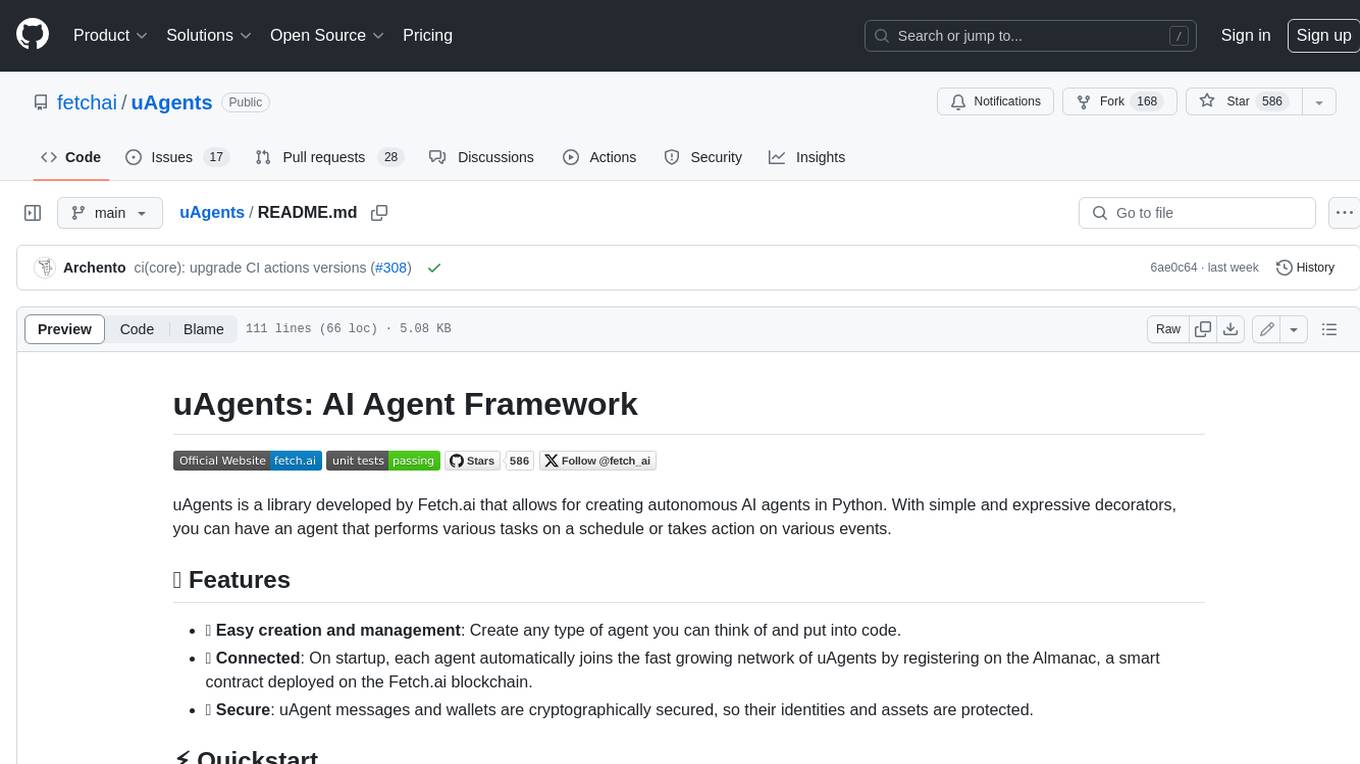
uAgents
uAgents is a Python library developed by Fetch.ai that allows for the creation of autonomous AI agents. These agents can perform various tasks on a schedule or take action on various events. uAgents are easy to create and manage, and they are connected to a fast-growing network of other uAgents. They are also secure, with cryptographically secured messages and wallets.
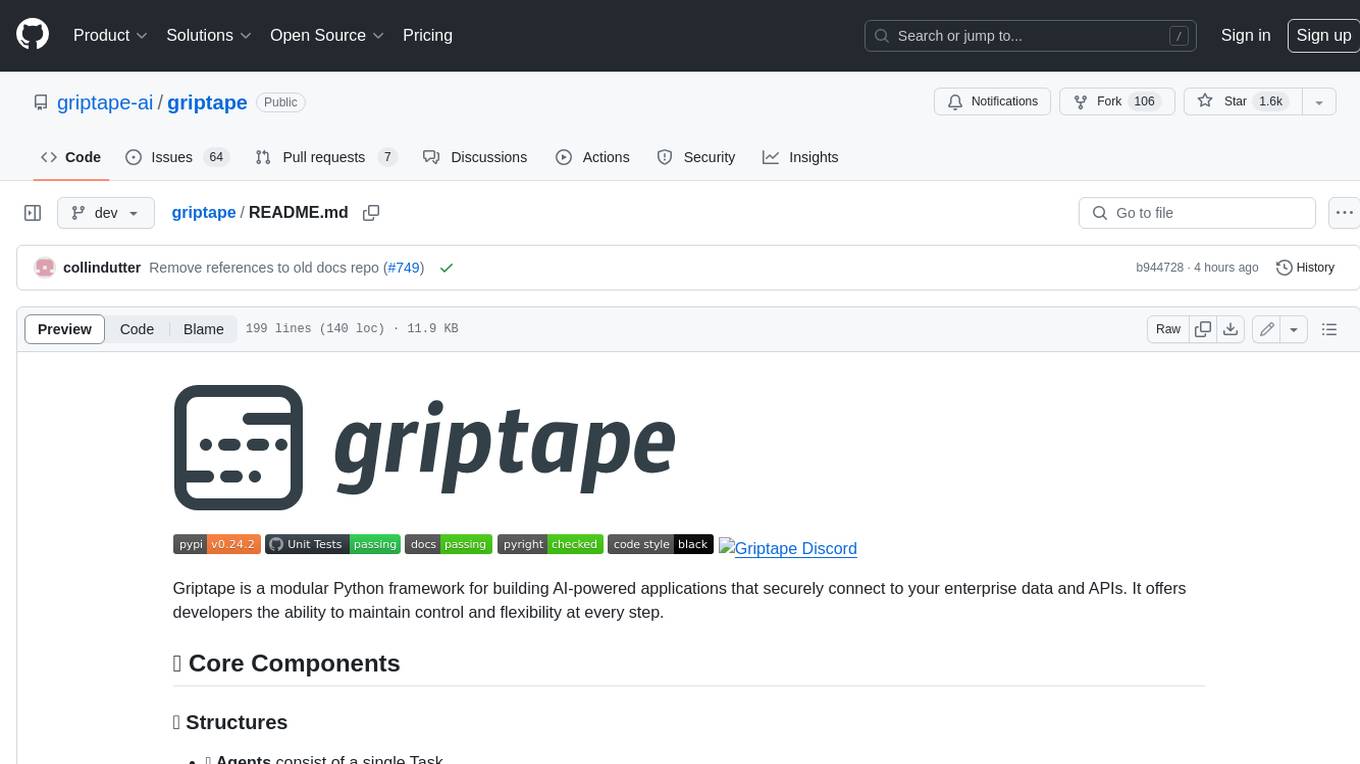
griptape
Griptape is a modular Python framework for building AI-powered applications that securely connect to your enterprise data and APIs. It offers developers the ability to maintain control and flexibility at every step. Griptape's core components include Structures (Agents, Pipelines, and Workflows), Tasks, Tools, Memory (Conversation Memory, Task Memory, and Meta Memory), Drivers (Prompt and Embedding Drivers, Vector Store Drivers, Image Generation Drivers, Image Query Drivers, SQL Drivers, Web Scraper Drivers, and Conversation Memory Drivers), Engines (Query Engines, Extraction Engines, Summary Engines, Image Generation Engines, and Image Query Engines), and additional components (Rulesets, Loaders, Artifacts, Chunkers, and Tokenizers). Griptape enables developers to create AI-powered applications with ease and efficiency.