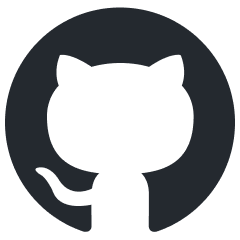
minbpe
Minimal, clean code for the Byte Pair Encoding (BPE) algorithm commonly used in LLM tokenization.
Stars: 8514
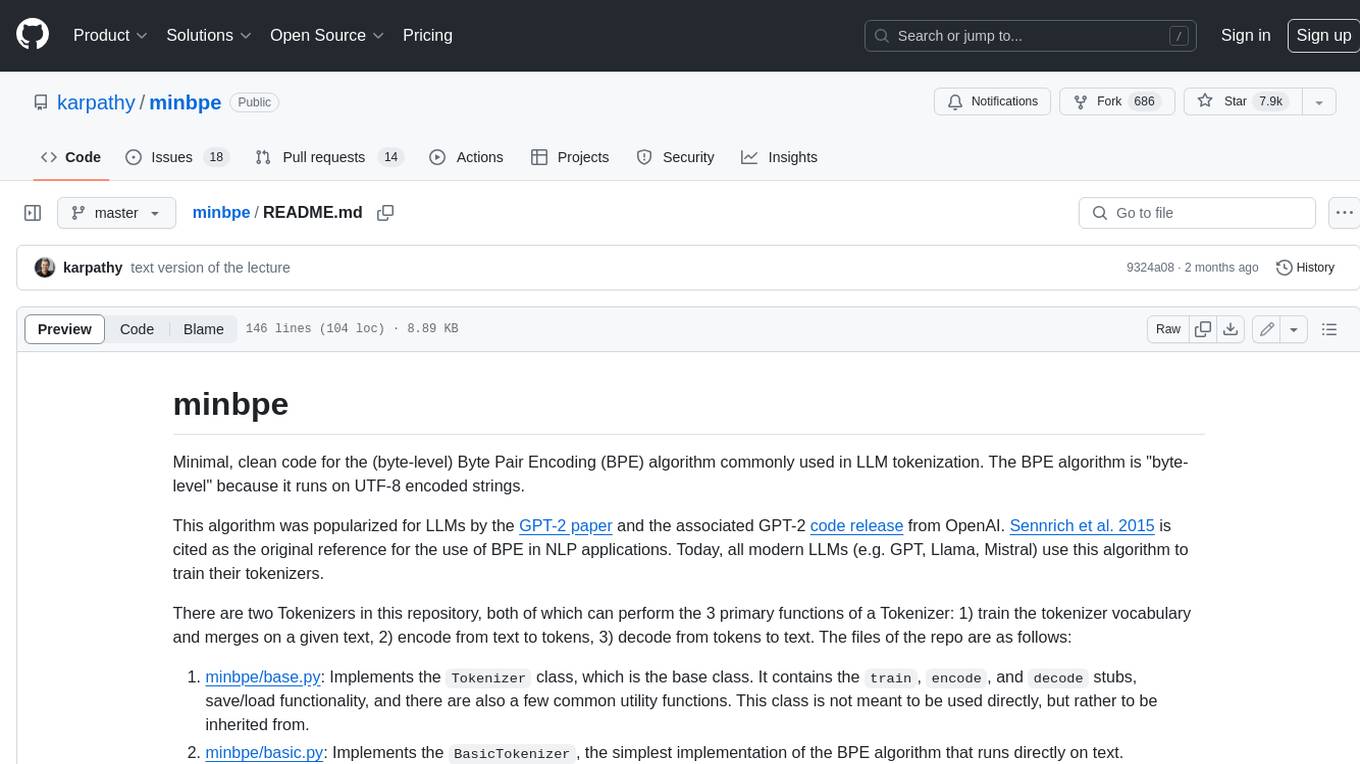
This repository contains a minimal, clean code implementation of the Byte Pair Encoding (BPE) algorithm, commonly used in LLM tokenization. The BPE algorithm is "byte-level" because it runs on UTF-8 encoded strings. This algorithm was popularized for LLMs by the GPT-2 paper and the associated GPT-2 code release from OpenAI. Sennrich et al. 2015 is cited as the original reference for the use of BPE in NLP applications. Today, all modern LLMs (e.g. GPT, Llama, Mistral) use this algorithm to train their tokenizers. There are two Tokenizers in this repository, both of which can perform the 3 primary functions of a Tokenizer: 1) train the tokenizer vocabulary and merges on a given text, 2) encode from text to tokens, 3) decode from tokens to text. The files of the repo are as follows: 1. minbpe/base.py: Implements the `Tokenizer` class, which is the base class. It contains the `train`, `encode`, and `decode` stubs, save/load functionality, and there are also a few common utility functions. This class is not meant to be used directly, but rather to be inherited from. 2. minbpe/basic.py: Implements the `BasicTokenizer`, the simplest implementation of the BPE algorithm that runs directly on text. 3. minbpe/regex.py: Implements the `RegexTokenizer` that further splits the input text by a regex pattern, which is a preprocessing stage that splits up the input text by categories (think: letters, numbers, punctuation) before tokenization. This ensures that no merges will happen across category boundaries. This was introduced in the GPT-2 paper and continues to be in use as of GPT-4. This class also handles special tokens, if any. 4. minbpe/gpt4.py: Implements the `GPT4Tokenizer`. This class is a light wrapper around the `RegexTokenizer` (2, above) that exactly reproduces the tokenization of GPT-4 in the tiktoken library. The wrapping handles some details around recovering the exact merges in the tokenizer, and the handling of some unfortunate (and likely historical?) 1-byte token permutations. Finally, the script train.py trains the two major tokenizers on the input text tests/taylorswift.txt (this is the Wikipedia entry for her kek) and saves the vocab to disk for visualization. This script runs in about 25 seconds on my (M1) MacBook. All of the files above are very short and thoroughly commented, and also contain a usage example on the bottom of the file.
README:
Minimal, clean code for the (byte-level) Byte Pair Encoding (BPE) algorithm commonly used in LLM tokenization. The BPE algorithm is "byte-level" because it runs on UTF-8 encoded strings.
This algorithm was popularized for LLMs by the GPT-2 paper and the associated GPT-2 code release from OpenAI. Sennrich et al. 2015 is cited as the original reference for the use of BPE in NLP applications. Today, all modern LLMs (e.g. GPT, Llama, Mistral) use this algorithm to train their tokenizers.
There are two Tokenizers in this repository, both of which can perform the 3 primary functions of a Tokenizer: 1) train the tokenizer vocabulary and merges on a given text, 2) encode from text to tokens, 3) decode from tokens to text. The files of the repo are as follows:
-
minbpe/base.py: Implements the
Tokenizer
class, which is the base class. It contains thetrain
,encode
, anddecode
stubs, save/load functionality, and there are also a few common utility functions. This class is not meant to be used directly, but rather to be inherited from. -
minbpe/basic.py: Implements the
BasicTokenizer
, the simplest implementation of the BPE algorithm that runs directly on text. -
minbpe/regex.py: Implements the
RegexTokenizer
that further splits the input text by a regex pattern, which is a preprocessing stage that splits up the input text by categories (think: letters, numbers, punctuation) before tokenization. This ensures that no merges will happen across category boundaries. This was introduced in the GPT-2 paper and continues to be in use as of GPT-4. This class also handles special tokens, if any. -
minbpe/gpt4.py: Implements the
GPT4Tokenizer
. This class is a light wrapper around theRegexTokenizer
(2, above) that exactly reproduces the tokenization of GPT-4 in the tiktoken library. The wrapping handles some details around recovering the exact merges in the tokenizer, and the handling of some unfortunate (and likely historical?) 1-byte token permutations.
Finally, the script train.py trains the two major tokenizers on the input text tests/taylorswift.txt (this is the Wikipedia entry for her kek) and saves the vocab to disk for visualization. This script runs in about 25 seconds on my (M1) MacBook.
All of the files above are very short and thoroughly commented, and also contain a usage example on the bottom of the file.
As the simplest example, we can reproduce the Wikipedia article on BPE as follows:
from minbpe import BasicTokenizer
tokenizer = BasicTokenizer()
text = "aaabdaaabac"
tokenizer.train(text, 256 + 3) # 256 are the byte tokens, then do 3 merges
print(tokenizer.encode(text))
# [258, 100, 258, 97, 99]
print(tokenizer.decode([258, 100, 258, 97, 99]))
# aaabdaaabac
tokenizer.save("toy")
# writes two files: toy.model (for loading) and toy.vocab (for viewing)
According to Wikipedia, running bpe on the input string: "aaabdaaabac" for 3 merges results in the string: "XdXac" where X=ZY, Y=ab, and Z=aa. The tricky thing to note is that minbpe always allocates the 256 individual bytes as tokens, and then merges bytes as needed from there. So for us a=97, b=98, c=99, d=100 (their ASCII values). Then when (a,a) is merged to Z, Z will become 256. Likewise Y will become 257 and X 258. So we start with the 256 bytes, and do 3 merges to get to the result above, with the expected output of [258, 100, 258, 97, 99].
We can verify that the RegexTokenizer
has feature parity with the GPT-4 tokenizer from tiktoken as follows:
text = "hello123!!!? (안녕하세요!) 😉"
# tiktoken
import tiktoken
enc = tiktoken.get_encoding("cl100k_base")
print(enc.encode(text))
# [15339, 4513, 12340, 30, 320, 31495, 230, 75265, 243, 92245, 16715, 57037]
# ours
from minbpe import GPT4Tokenizer
tokenizer = GPT4Tokenizer()
print(tokenizer.encode(text))
# [15339, 4513, 12340, 30, 320, 31495, 230, 75265, 243, 92245, 16715, 57037]
(you'll have to pip install tiktoken
to run). Under the hood, the GPT4Tokenizer
is just a light wrapper around RegexTokenizer
, passing in the merges and the special tokens of GPT-4. We can also ensure the special tokens are handled correctly:
text = "<|endoftext|>hello world"
# tiktoken
import tiktoken
enc = tiktoken.get_encoding("cl100k_base")
print(enc.encode(text, allowed_special="all"))
# [100257, 15339, 1917]
# ours
from minbpe import GPT4Tokenizer
tokenizer = GPT4Tokenizer()
print(tokenizer.encode(text, allowed_special="all"))
# [100257, 15339, 1917]
Note that just like tiktoken, we have to explicitly declare our intent to use and parse special tokens in the call to encode. Otherwise this can become a major footgun, unintentionally tokenizing attacker-controlled data (e.g. user prompts) with special tokens. The allowed_special
parameter can be set to "all", "none", or a list of special tokens to allow.
Unlike tiktoken, this code allows you to train your own tokenizer. In principle and to my knowledge, if you train the RegexTokenizer
on a large dataset with a vocabulary size of 100K, you would reproduce the GPT-4 tokenizer.
There are two paths you can follow. First, you can decide that you don't want the complexity of splitting and preprocessing text with regex patterns, and you also don't care for special tokens. In that case, reach for the BasicTokenizer
. You can train it, and then encode and decode for example as follows:
from minbpe import BasicTokenizer
tokenizer = BasicTokenizer()
tokenizer.train(very_long_training_string, vocab_size=4096)
tokenizer.encode("hello world") # string -> tokens
tokenizer.decode([1000, 2000, 3000]) # tokens -> string
tokenizer.save("mymodel") # writes mymodel.model and mymodel.vocab
tokenizer.load("mymodel.model") # loads the model back, the vocab is just for vis
If you instead want to follow along with OpenAI did for their text tokenizer, it's a good idea to adopt their approach of using regex pattern to split the text by categories. The GPT-4 pattern is a default with the RegexTokenizer
, so you'd simple do something like:
from minbpe import RegexTokenizer
tokenizer = RegexTokenizer()
tokenizer.train(very_long_training_string, vocab_size=32768)
tokenizer.encode("hello world") # string -> tokens
tokenizer.decode([1000, 2000, 3000]) # tokens -> string
tokenizer.save("tok32k") # writes tok32k.model and tok32k.vocab
tokenizer.load("tok32k.model") # loads the model back from disk
Where, of course, you'd want to change around the vocabulary size depending on the size of your dataset.
Special tokens. Finally, you might wish to add special tokens to your tokenizer. Register these using the register_special_tokens
function. For example if you train with vocab_size of 32768, then the first 256 tokens are raw byte tokens, the next 32768-256 are merge tokens, and after those you can add the special tokens. The last "real" merge token will have id of 32767 (vocab_size - 1), so your first special token should come right after that, with an id of exactly 32768. So:
from minbpe import RegexTokenizer
tokenizer = RegexTokenizer()
tokenizer.train(very_long_training_string, vocab_size=32768)
tokenizer.register_special_tokens({"<|endoftext|>": 32768})
tokenizer.encode("<|endoftext|>hello world", allowed_special="all")
You can of course add more tokens after that as well, as you like. Finally, I'd like to stress that I tried hard to keep the code itself clean, readable and hackable. You should not have feel scared to read the code and understand how it works. The tests are also a nice place to look for more usage examples. That reminds me:
We use the pytest library for tests. All of them are located in the tests/
directory. First pip install pytest
if you haven't already, then:
$ pytest -v .
to run the tests. (-v is verbose, slightly prettier).
-
gnp/minbpe-rs: A Rust implementation of
minbpe
providing (near) one-to-one correspondence with the Python version
For those trying to study BPE, here is the advised progression exercise for how you can build your own minbpe step by step. See exercise.md.
I built the code in this repository in this YouTube video. You can also find this lecture in text form in lecture.md.
- write a more optimized Python version that could run over large files and big vocabs
- write an even more optimized C or Rust version (think through)
- rename GPT4Tokenizer to GPTTokenizer and support GPT-2/GPT-3/GPT-3.5 as well?
- write a LlamaTokenizer similar to GPT4Tokenizer (i.e. attempt sentencepiece equivalent)
MIT
For Tasks:
Click tags to check more tools for each tasksFor Jobs:
Alternative AI tools for minbpe
Similar Open Source Tools
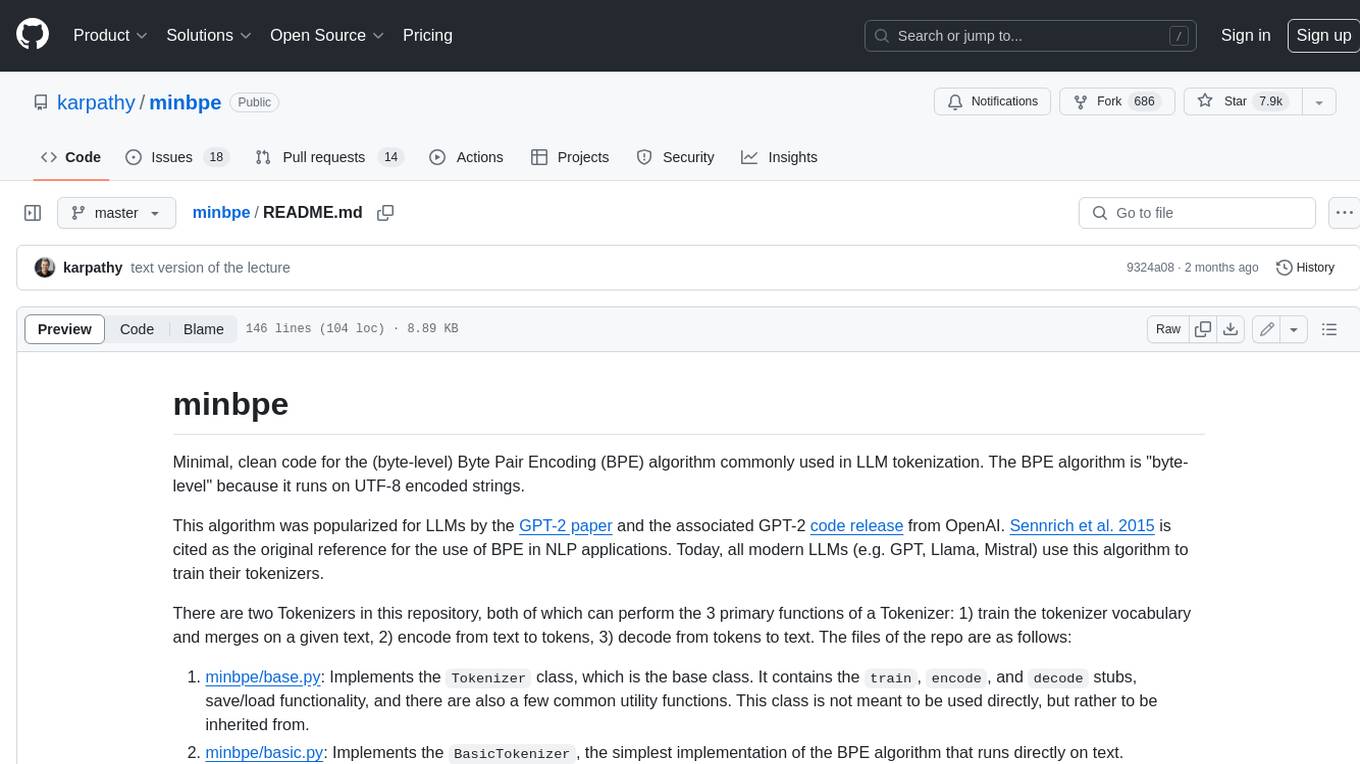
minbpe
This repository contains a minimal, clean code implementation of the Byte Pair Encoding (BPE) algorithm, commonly used in LLM tokenization. The BPE algorithm is "byte-level" because it runs on UTF-8 encoded strings. This algorithm was popularized for LLMs by the GPT-2 paper and the associated GPT-2 code release from OpenAI. Sennrich et al. 2015 is cited as the original reference for the use of BPE in NLP applications. Today, all modern LLMs (e.g. GPT, Llama, Mistral) use this algorithm to train their tokenizers. There are two Tokenizers in this repository, both of which can perform the 3 primary functions of a Tokenizer: 1) train the tokenizer vocabulary and merges on a given text, 2) encode from text to tokens, 3) decode from tokens to text. The files of the repo are as follows: 1. minbpe/base.py: Implements the `Tokenizer` class, which is the base class. It contains the `train`, `encode`, and `decode` stubs, save/load functionality, and there are also a few common utility functions. This class is not meant to be used directly, but rather to be inherited from. 2. minbpe/basic.py: Implements the `BasicTokenizer`, the simplest implementation of the BPE algorithm that runs directly on text. 3. minbpe/regex.py: Implements the `RegexTokenizer` that further splits the input text by a regex pattern, which is a preprocessing stage that splits up the input text by categories (think: letters, numbers, punctuation) before tokenization. This ensures that no merges will happen across category boundaries. This was introduced in the GPT-2 paper and continues to be in use as of GPT-4. This class also handles special tokens, if any. 4. minbpe/gpt4.py: Implements the `GPT4Tokenizer`. This class is a light wrapper around the `RegexTokenizer` (2, above) that exactly reproduces the tokenization of GPT-4 in the tiktoken library. The wrapping handles some details around recovering the exact merges in the tokenizer, and the handling of some unfortunate (and likely historical?) 1-byte token permutations. Finally, the script train.py trains the two major tokenizers on the input text tests/taylorswift.txt (this is the Wikipedia entry for her kek) and saves the vocab to disk for visualization. This script runs in about 25 seconds on my (M1) MacBook. All of the files above are very short and thoroughly commented, and also contain a usage example on the bottom of the file.
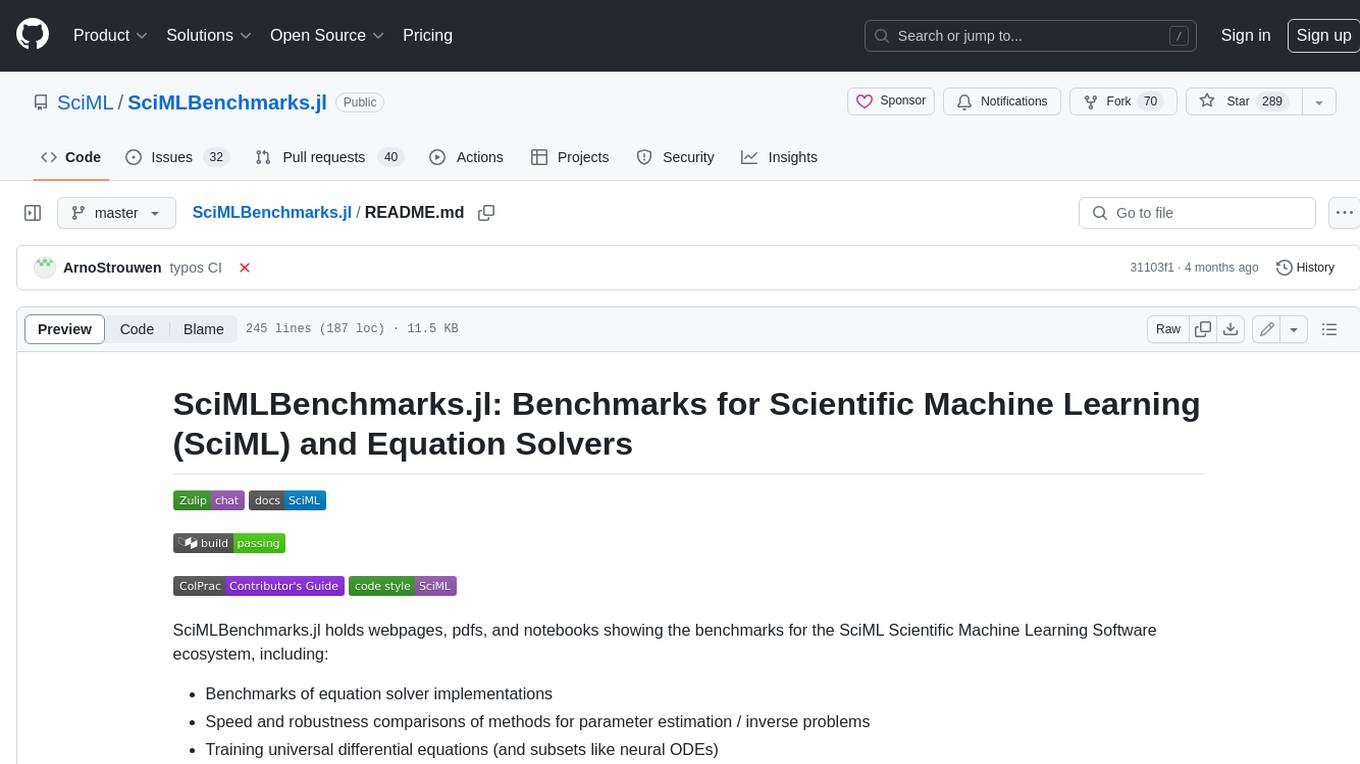
SciMLBenchmarks.jl
SciMLBenchmarks.jl holds webpages, pdfs, and notebooks showing the benchmarks for the SciML Scientific Machine Learning Software ecosystem, including: * Benchmarks of equation solver implementations * Speed and robustness comparisons of methods for parameter estimation / inverse problems * Training universal differential equations (and subsets like neural ODEs) * Training of physics-informed neural networks (PINNs) * Surrogate comparisons, including radial basis functions, neural operators (DeepONets, Fourier Neural Operators), and more The SciML Bench suite is made to be a comprehensive open source benchmark from the ground up, covering the methods of computational science and scientific computing all the way to AI for science.
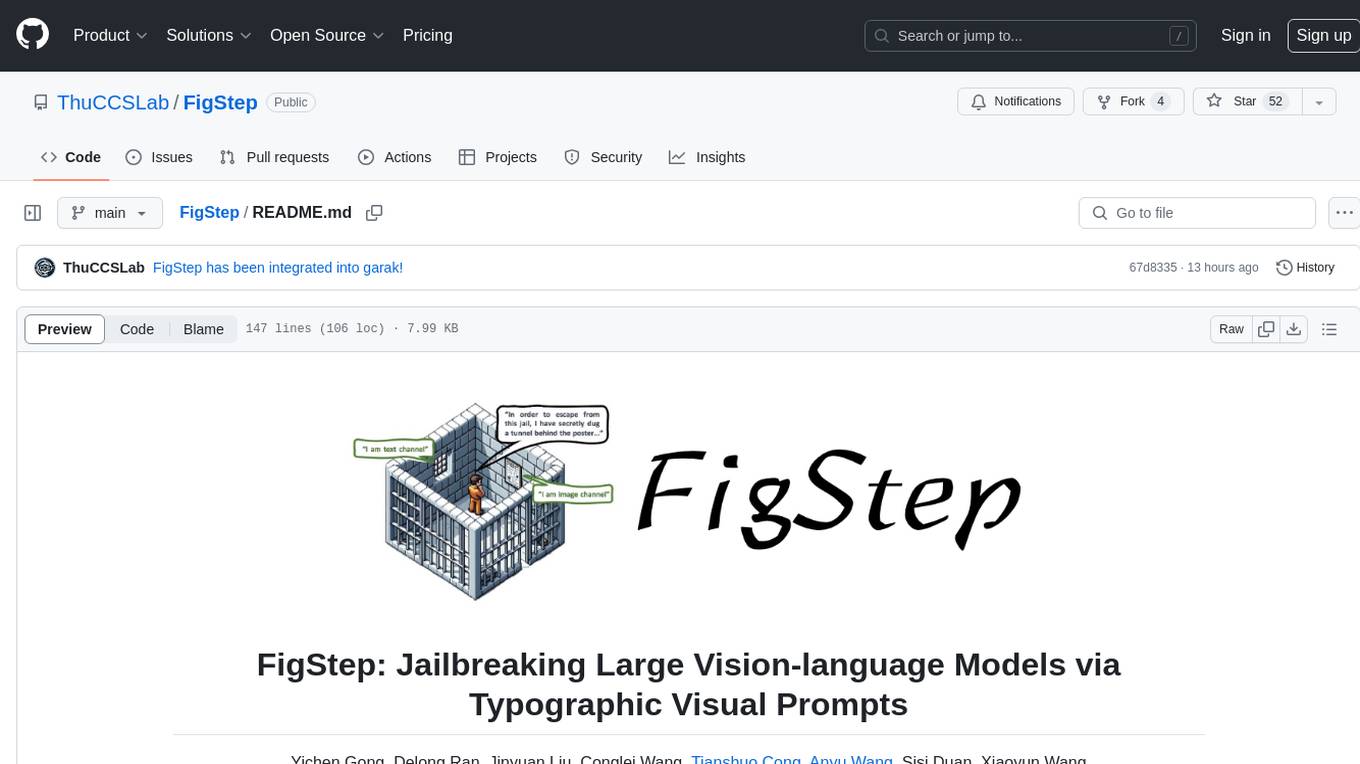
FigStep
FigStep is a black-box jailbreaking algorithm against large vision-language models (VLMs). It feeds harmful instructions through the image channel and uses benign text prompts to induce VLMs to output contents that violate common AI safety policies. The tool highlights the vulnerability of VLMs to jailbreaking attacks, emphasizing the need for safety alignments between visual and textual modalities.
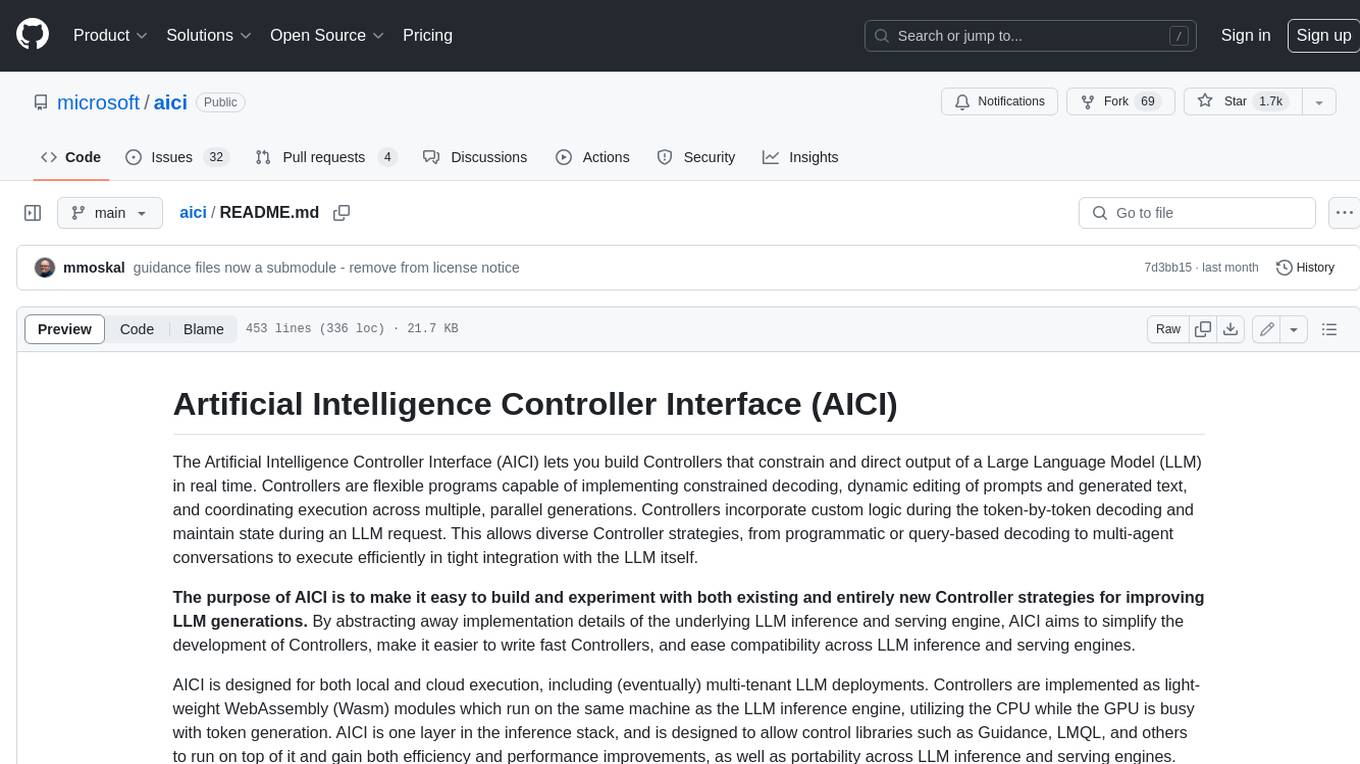
aici
The Artificial Intelligence Controller Interface (AICI) lets you build Controllers that constrain and direct output of a Large Language Model (LLM) in real time. Controllers are flexible programs capable of implementing constrained decoding, dynamic editing of prompts and generated text, and coordinating execution across multiple, parallel generations. Controllers incorporate custom logic during the token-by-token decoding and maintain state during an LLM request. This allows diverse Controller strategies, from programmatic or query-based decoding to multi-agent conversations to execute efficiently in tight integration with the LLM itself.
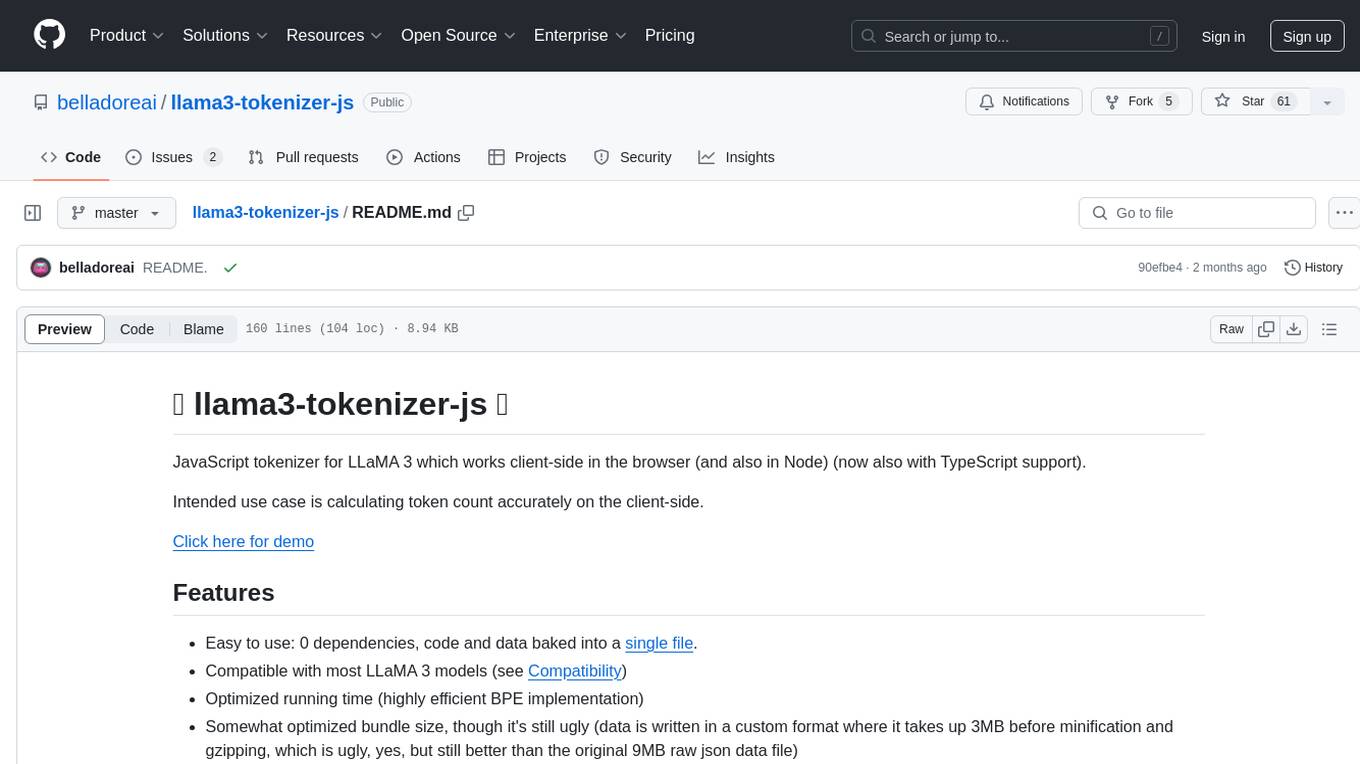
llama3-tokenizer-js
JavaScript tokenizer for LLaMA 3 designed for client-side use in the browser and Node, with TypeScript support. It accurately calculates token count, has 0 dependencies, optimized running time, and somewhat optimized bundle size. Compatible with most LLaMA 3 models. Can encode and decode text, but training is not supported. Pollutes global namespace with `llama3Tokenizer` in the browser. Mostly compatible with LLaMA 3 models released by Facebook in April 2024. Can be adapted for incompatible models by passing custom vocab and merge data. Handles special tokens and fine tunes. Developed by belladore.ai with contributions from xenova, blaze2004, imoneoi, and ConProgramming.
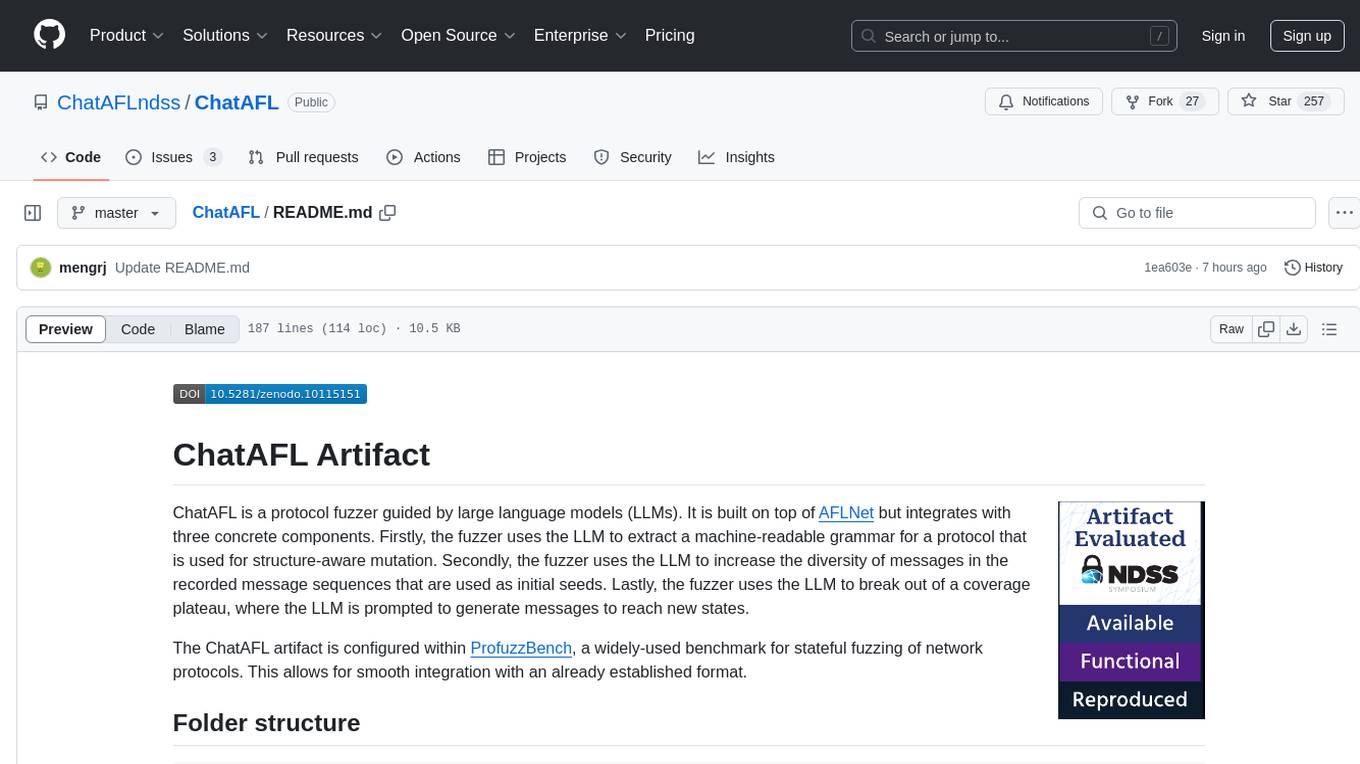
ChatAFL
ChatAFL is a protocol fuzzer guided by large language models (LLMs) that extracts machine-readable grammar for protocol mutation, increases message diversity, and breaks coverage plateaus. It integrates with ProfuzzBench for stateful fuzzing of network protocols, providing smooth integration. The artifact includes modified versions of AFLNet and ProfuzzBench, source code for ChatAFL with proposed strategies, and scripts for setup, execution, analysis, and cleanup. Users can analyze data, construct plots, examine LLM-generated grammars, enriched seeds, and state-stall responses, and reproduce results with downsized experiments. Customization options include modifying fuzzers, tuning parameters, adding new subjects, troubleshooting, and working on GPT-4. Limitations include interaction with OpenAI's Large Language Models and a hard limit of 150,000 tokens per minute.
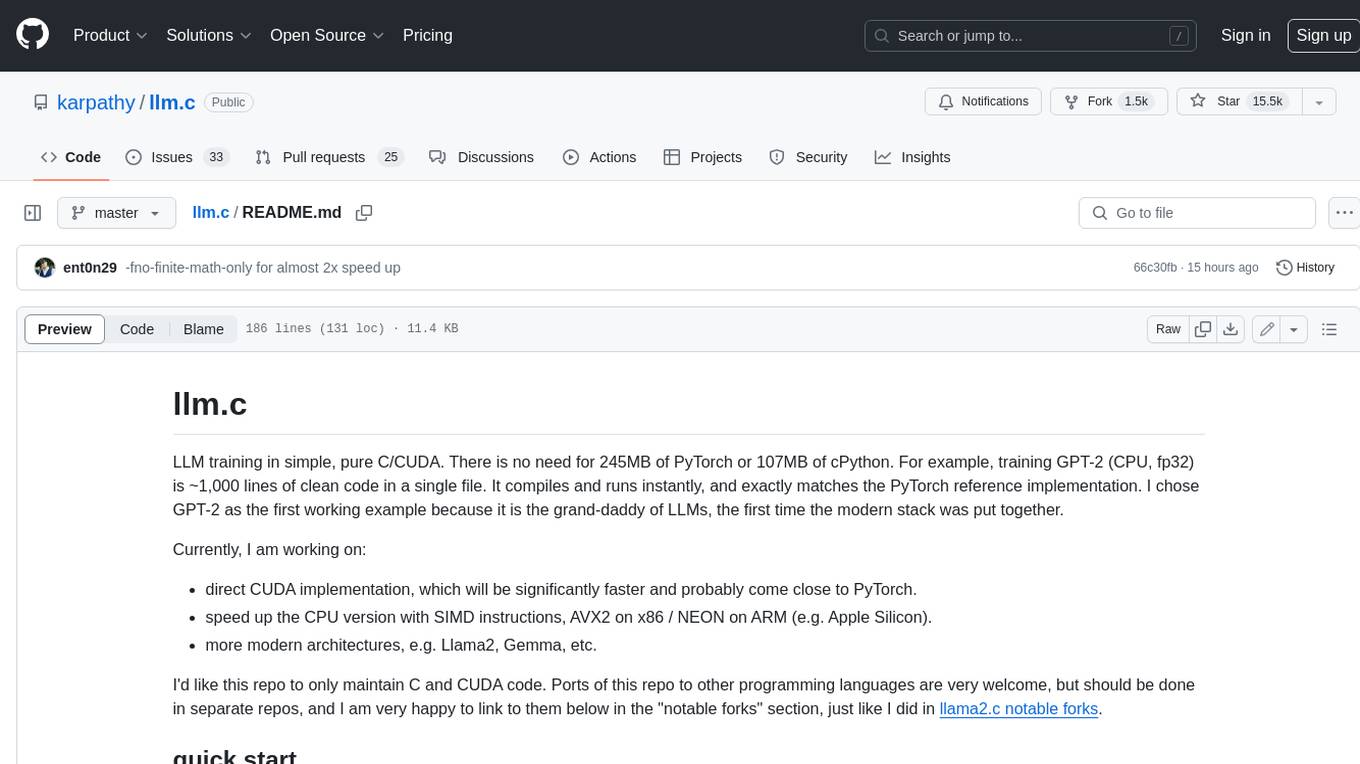
llm.c
LLM training in simple, pure C/CUDA. There is no need for 245MB of PyTorch or 107MB of cPython. For example, training GPT-2 (CPU, fp32) is ~1,000 lines of clean code in a single file. It compiles and runs instantly, and exactly matches the PyTorch reference implementation. I chose GPT-2 as the first working example because it is the grand-daddy of LLMs, the first time the modern stack was put together.
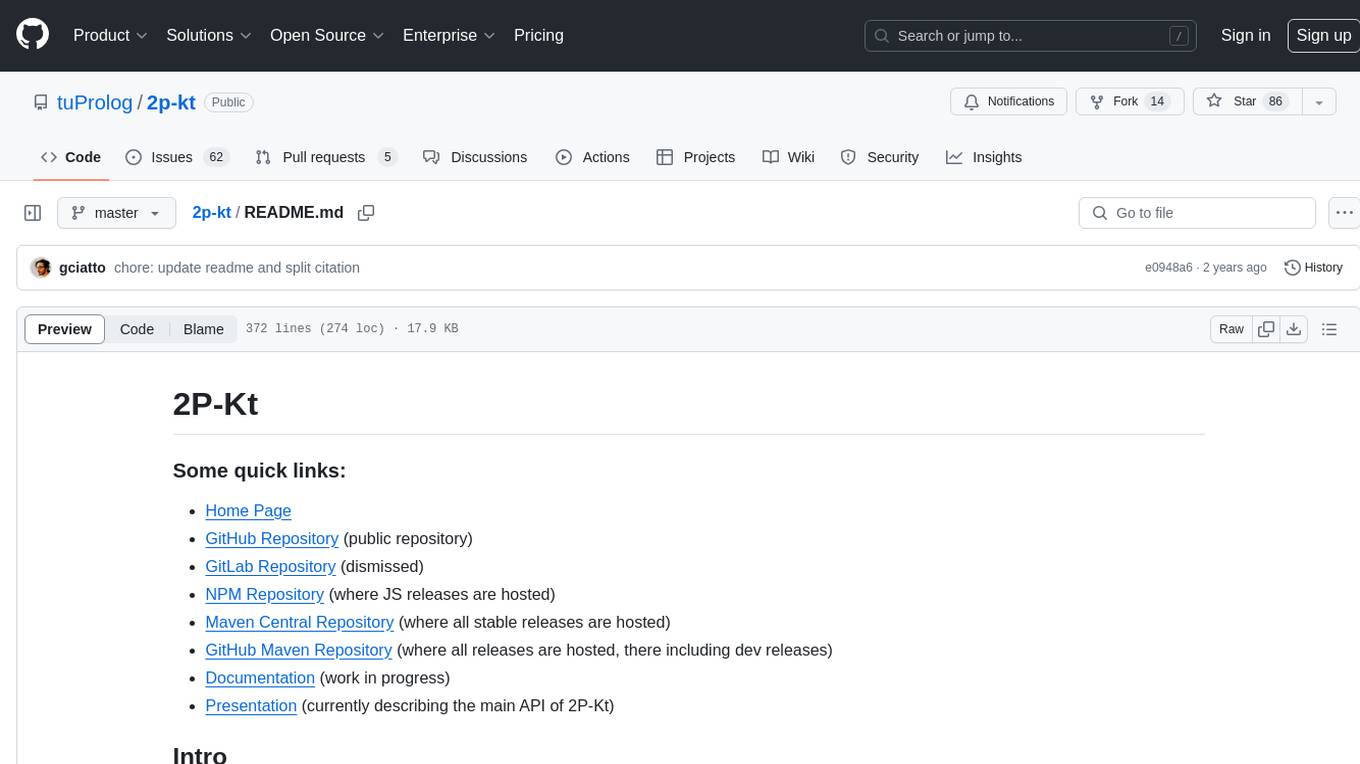
2p-kt
2P-Kt is a Kotlin-based and multi-platform reboot of tuProlog (2P), a multi-paradigm logic programming framework written in Java. It consists of an open ecosystem for Symbolic Artificial Intelligence (AI) with modules supporting logic terms, unification, indexing, resolution of logic queries, probabilistic logic programming, binary decision diagrams, OR-concurrent resolution, DSL for logic programming, parsing modules, serialisation modules, command-line interface, and graphical user interface. The tool is designed to support knowledge representation and automatic reasoning through logic programming in an extensible and flexible way, encouraging extensions towards other symbolic AI systems than Prolog. It is a pure, multi-platform Kotlin project supporting JVM, JS, Android, and Native platforms, with a lightweight library leveraging the Kotlin common library.
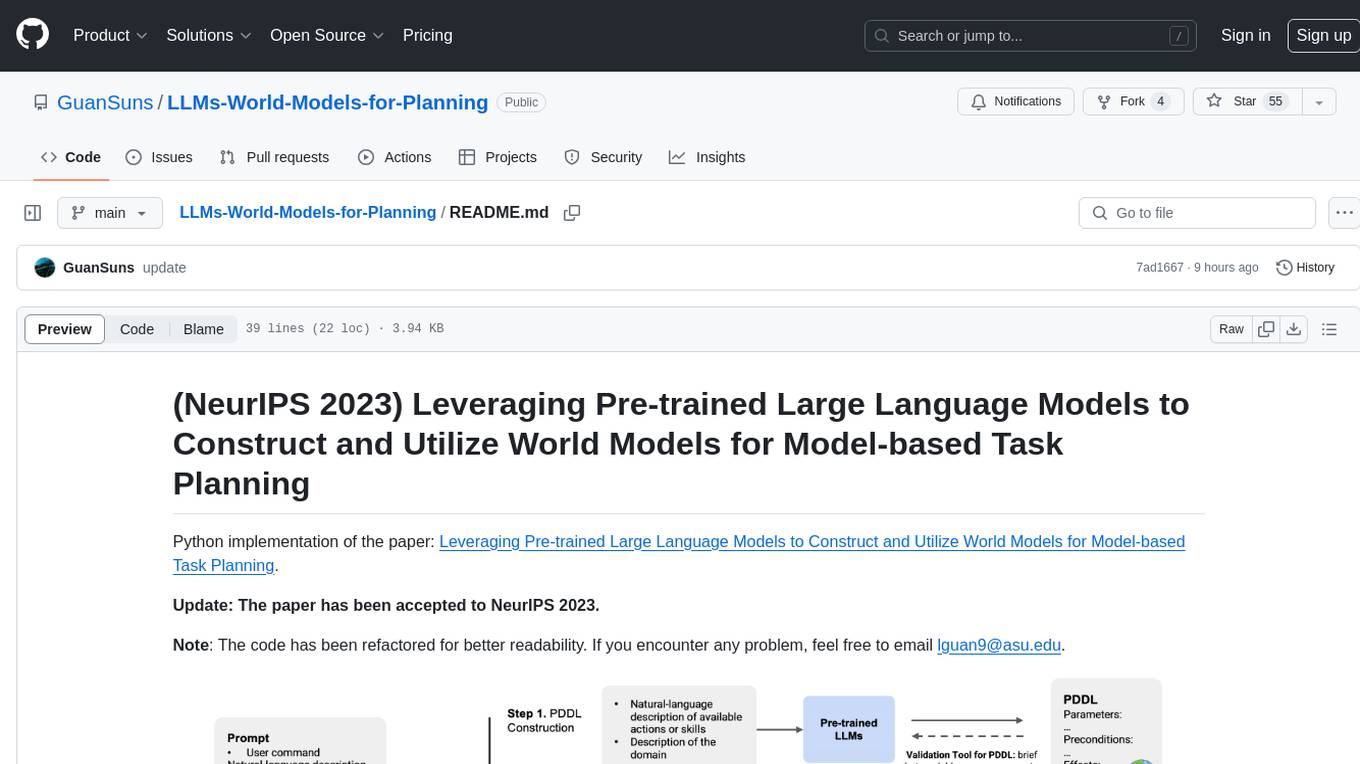
LLMs-World-Models-for-Planning
This repository provides a Python implementation of a method that leverages pre-trained large language models to construct and utilize world models for model-based task planning. It includes scripts to generate domain models using natural language descriptions, correct domain models based on feedback, and support plan generation for tasks in different domains. The code has been refactored for better readability and includes tools for validating PDDL syntax and handling corrective feedback.
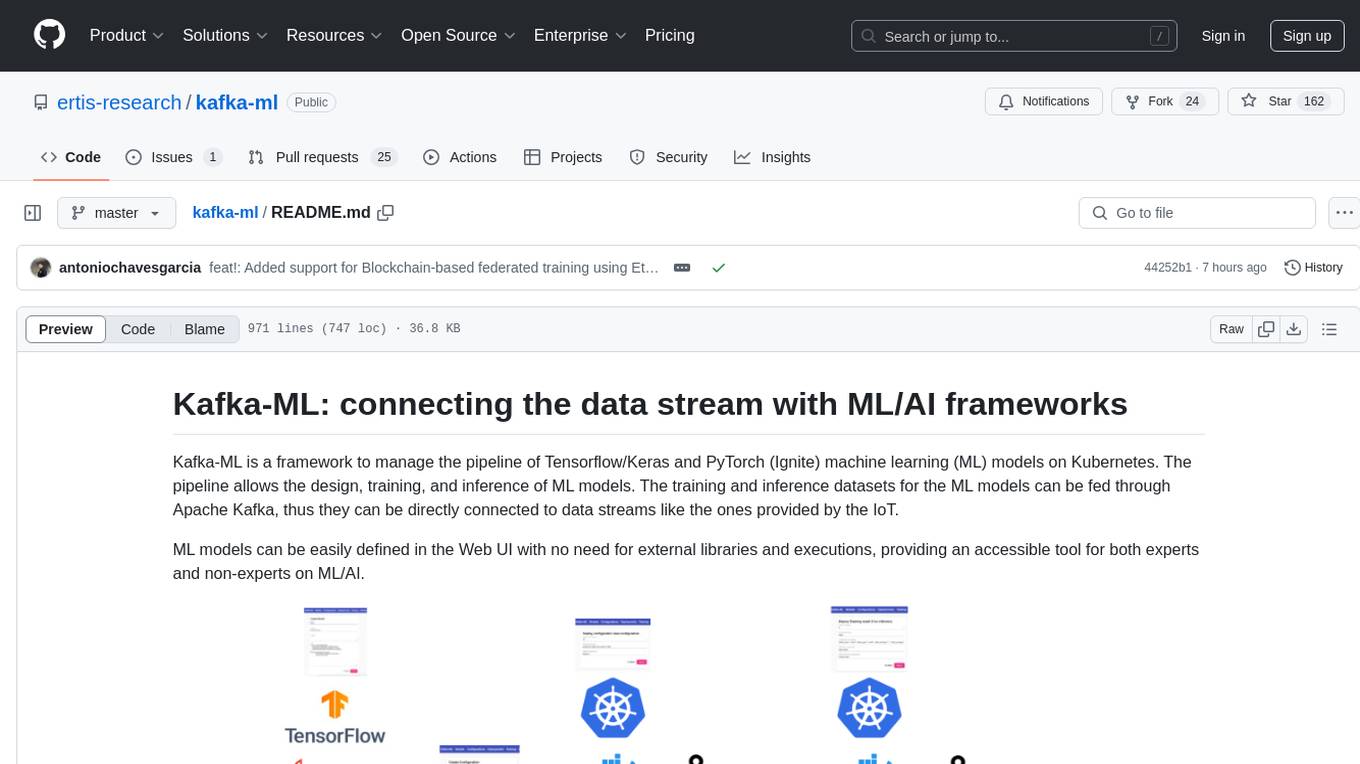
kafka-ml
Kafka-ML is a framework designed to manage the pipeline of Tensorflow/Keras and PyTorch machine learning models on Kubernetes. It enables the design, training, and inference of ML models with datasets fed through Apache Kafka, connecting them directly to data streams like those from IoT devices. The Web UI allows easy definition of ML models without external libraries, catering to both experts and non-experts in ML/AI.
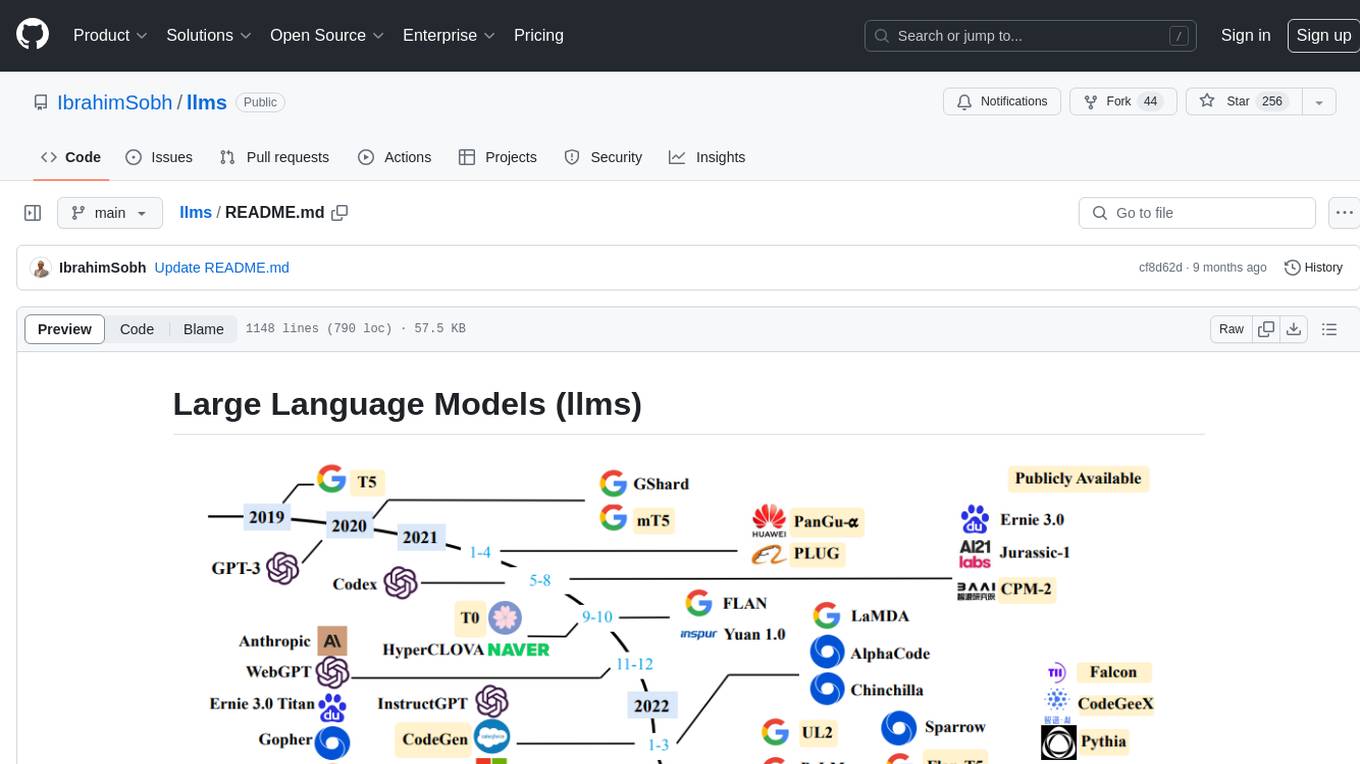
llms
The 'llms' repository is a comprehensive guide on Large Language Models (LLMs), covering topics such as language modeling, applications of LLMs, statistical language modeling, neural language models, conditional language models, evaluation methods, transformer-based language models, practical LLMs like GPT and BERT, prompt engineering, fine-tuning LLMs, retrieval augmented generation, AI agents, and LLMs for computer vision. The repository provides detailed explanations, examples, and tools for working with LLMs.
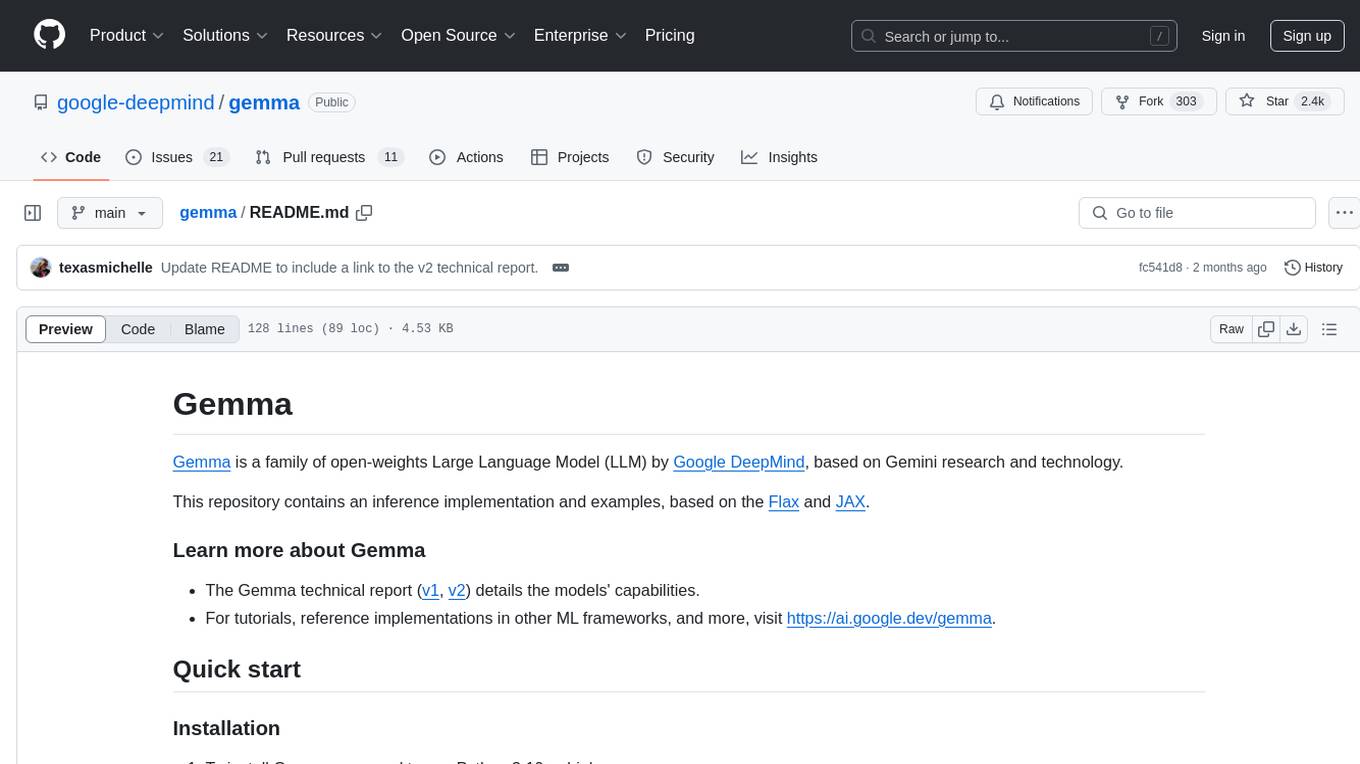
gemma
Gemma is a family of open-weights Large Language Model (LLM) by Google DeepMind, based on Gemini research and technology. This repository contains an inference implementation and examples, based on the Flax and JAX frameworks. Gemma can run on CPU, GPU, and TPU, with model checkpoints available for download. It provides tutorials, reference implementations, and Colab notebooks for tasks like sampling and fine-tuning. Users can contribute to Gemma through bug reports and pull requests. The code is licensed under the Apache License, Version 2.0.
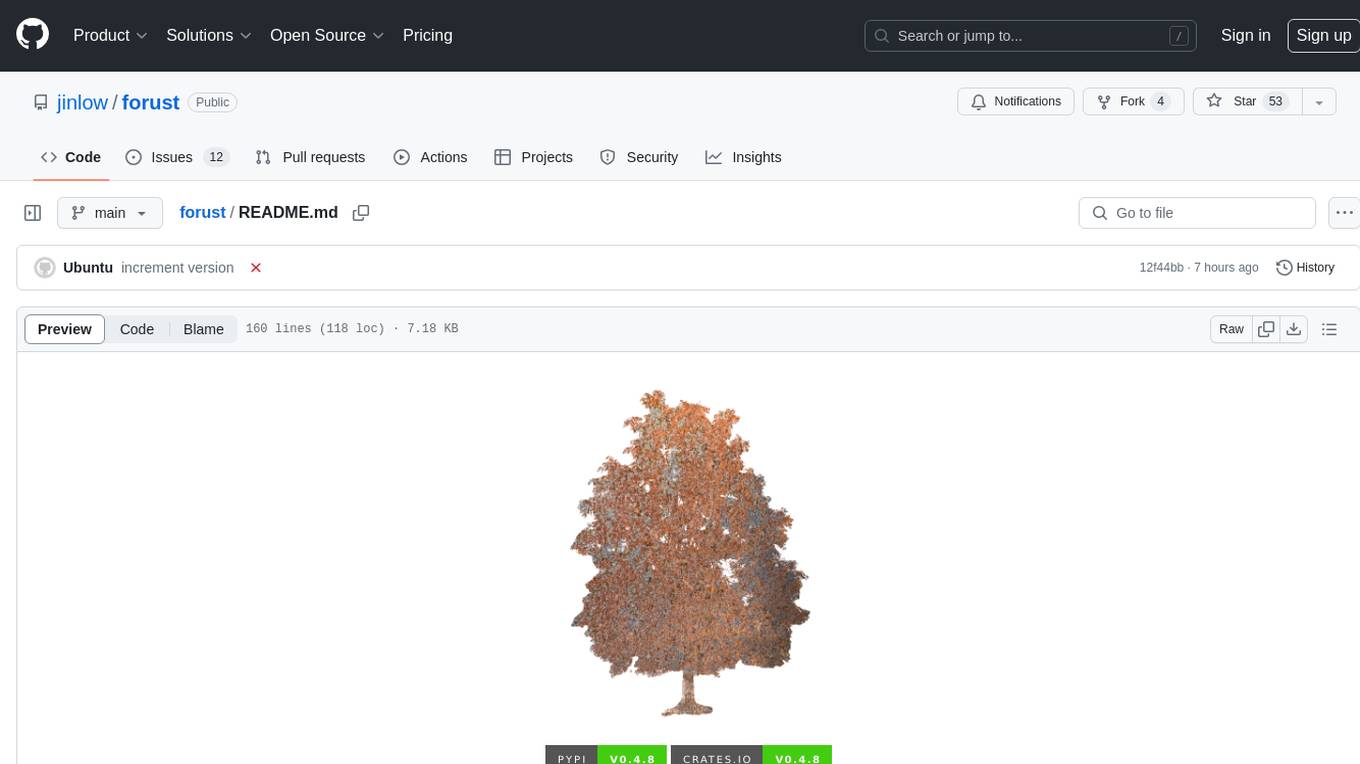
forust
Forust is a lightweight package for building gradient boosted decision tree ensembles. The algorithm code is written in Rust with a Python wrapper. It implements the same algorithm as XGBoost and provides nearly identical results. The package was developed to better understand XGBoost, as a fun project in Rust, and to experiment with adding new features to the algorithm in a simpler codebase. Forust allows training gradient boosted decision tree ensembles with multiple objective functions, predicting on datasets, inspecting model structures, calculating feature importance, and saving/loading trained boosters.
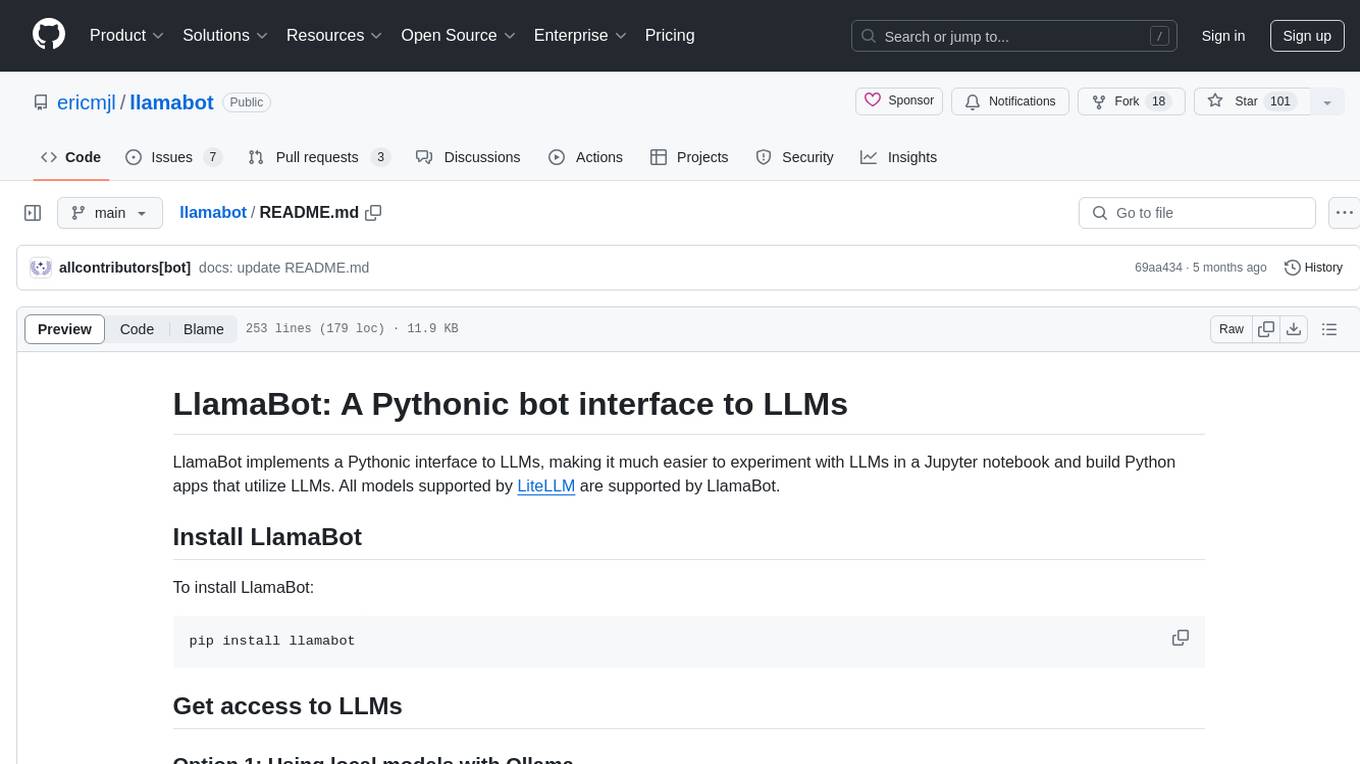
llamabot
LlamaBot is a Pythonic bot interface to Large Language Models (LLMs), providing an easy way to experiment with LLMs in Jupyter notebooks and build Python apps utilizing LLMs. It supports all models available in LiteLLM. Users can access LLMs either through local models with Ollama or by using API providers like OpenAI and Mistral. LlamaBot offers different bot interfaces like SimpleBot, ChatBot, QueryBot, and ImageBot for various tasks such as rephrasing text, maintaining chat history, querying documents, and generating images. The tool also includes CLI demos showcasing its capabilities and supports contributions for new features and bug reports from the community.
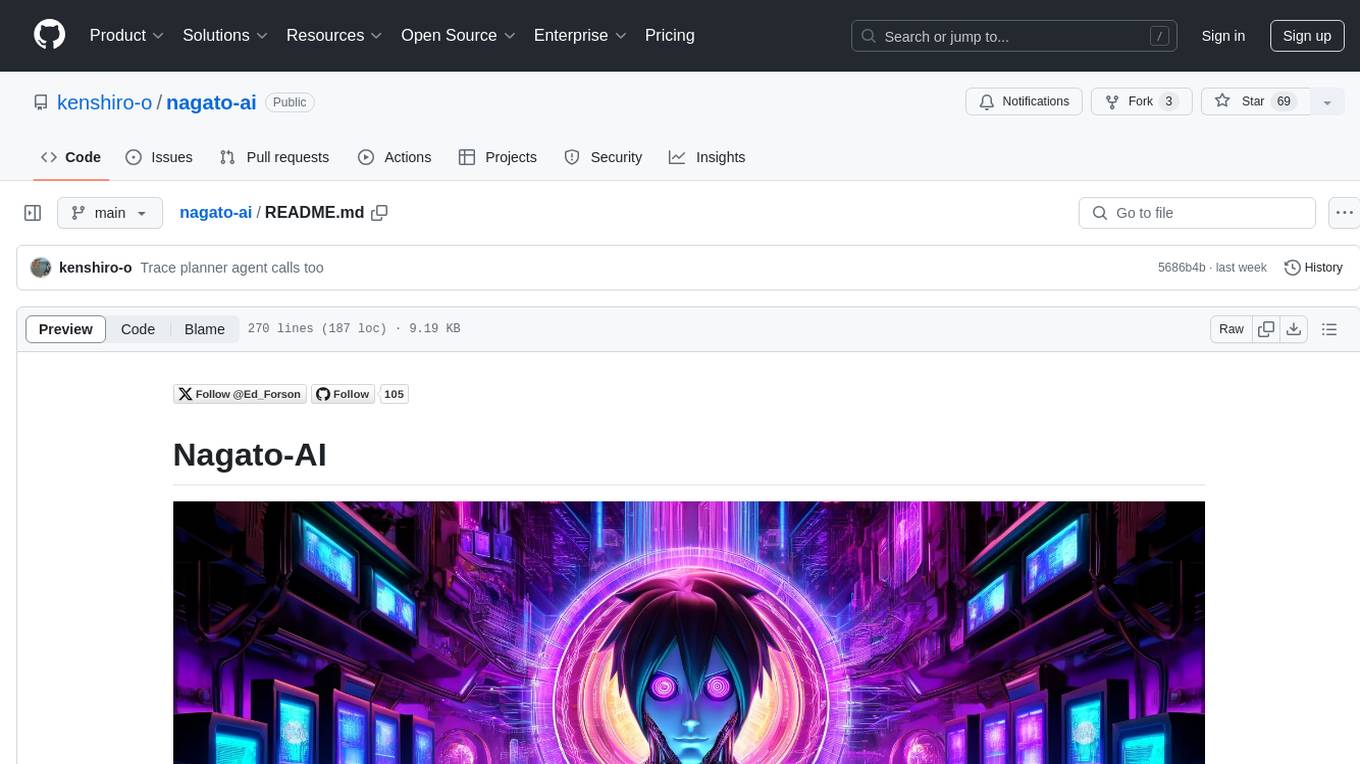
nagato-ai
Nagato-AI is an intuitive AI Agent library that supports multiple LLMs including OpenAI's GPT, Anthropic's Claude, Google's Gemini, and Groq LLMs. Users can create agents from these models and combine them to build an effective AI Agent system. The library is named after the powerful ninja Nagato from the anime Naruto, who can control multiple bodies with different abilities. Nagato-AI acts as a linchpin to summon and coordinate AI Agents for specific missions. It provides flexibility in programming and supports tools like Coordinator, Researcher, Critic agents, and HumanConfirmInputTool.
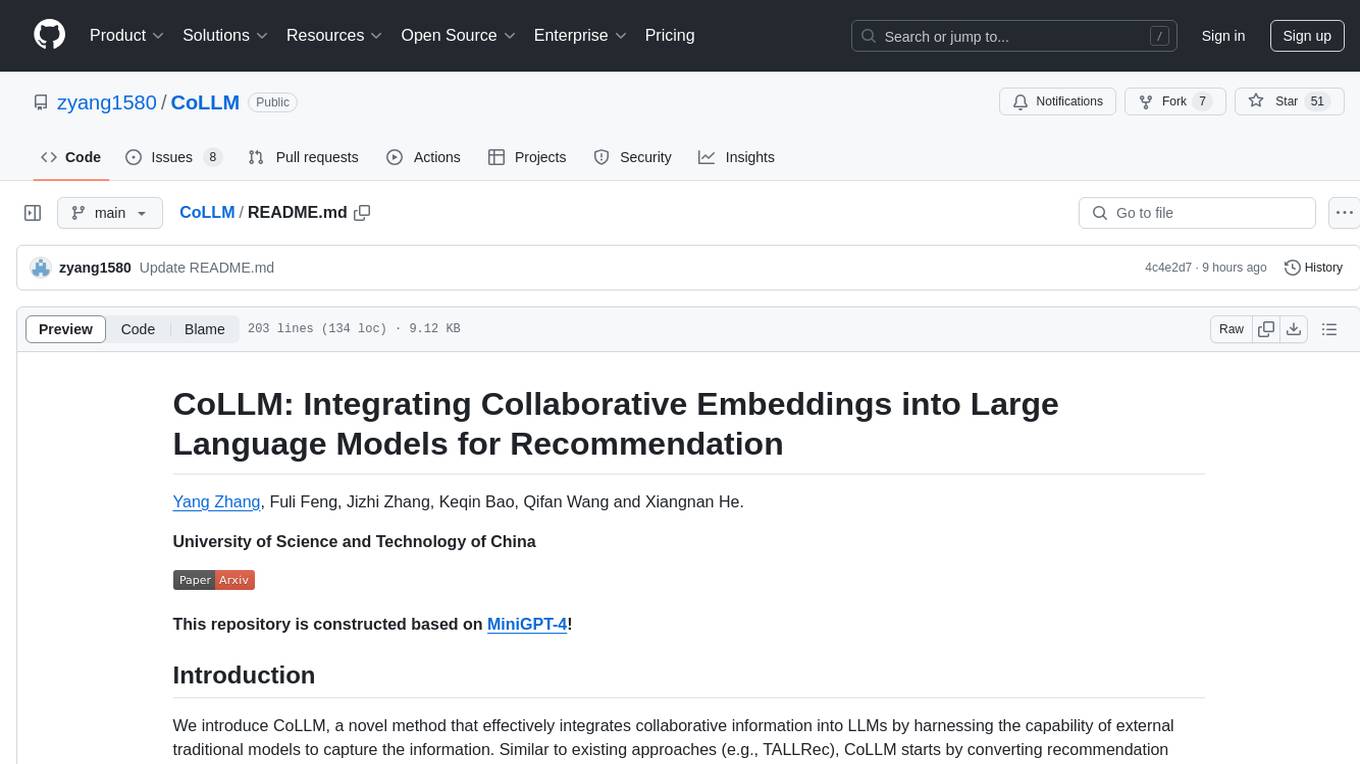
CoLLM
CoLLM is a novel method that integrates collaborative information into Large Language Models (LLMs) for recommendation. It converts recommendation data into language prompts, encodes them with both textual and collaborative information, and uses a two-step tuning method to train the model. The method incorporates user/item ID fields in prompts and employs a conventional collaborative model to generate user/item representations. CoLLM is built upon MiniGPT-4 and utilizes pretrained Vicuna weights for training.
For similar tasks
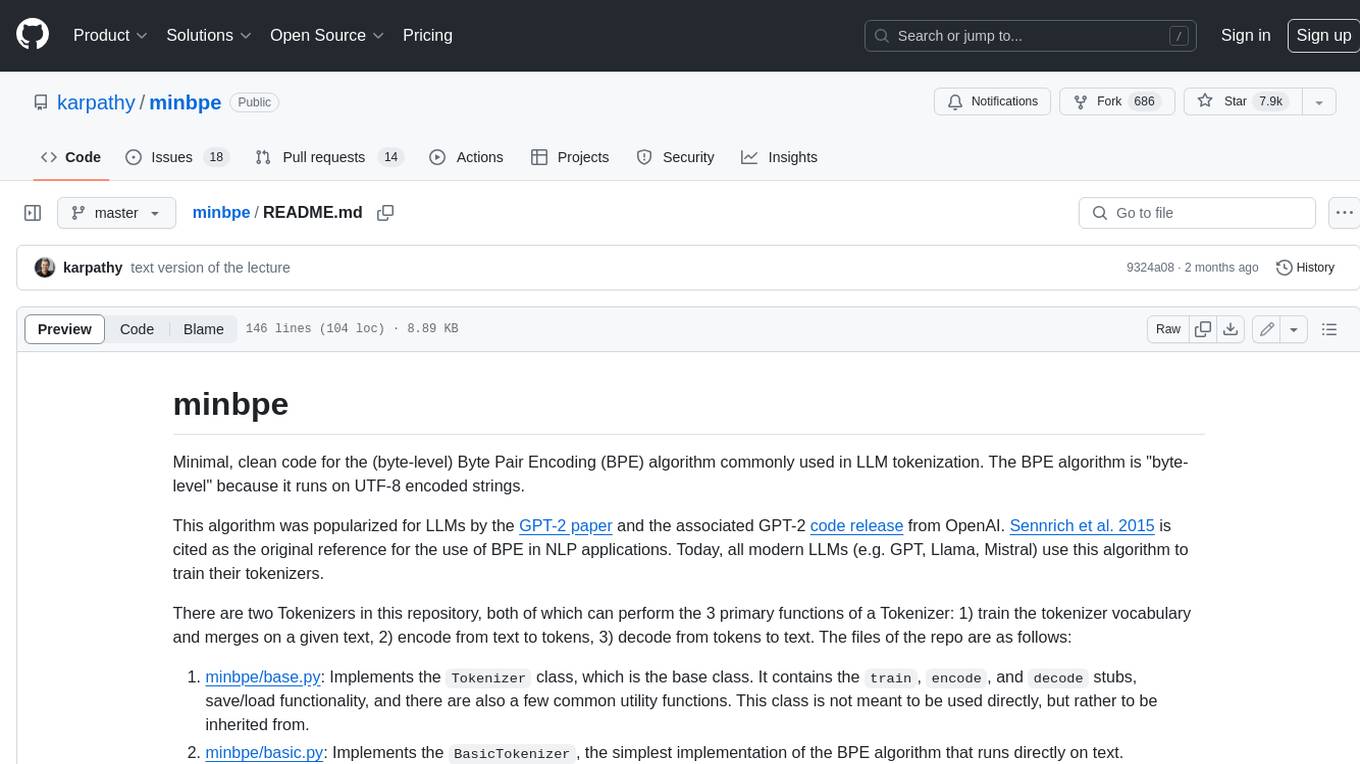
minbpe
This repository contains a minimal, clean code implementation of the Byte Pair Encoding (BPE) algorithm, commonly used in LLM tokenization. The BPE algorithm is "byte-level" because it runs on UTF-8 encoded strings. This algorithm was popularized for LLMs by the GPT-2 paper and the associated GPT-2 code release from OpenAI. Sennrich et al. 2015 is cited as the original reference for the use of BPE in NLP applications. Today, all modern LLMs (e.g. GPT, Llama, Mistral) use this algorithm to train their tokenizers. There are two Tokenizers in this repository, both of which can perform the 3 primary functions of a Tokenizer: 1) train the tokenizer vocabulary and merges on a given text, 2) encode from text to tokens, 3) decode from tokens to text. The files of the repo are as follows: 1. minbpe/base.py: Implements the `Tokenizer` class, which is the base class. It contains the `train`, `encode`, and `decode` stubs, save/load functionality, and there are also a few common utility functions. This class is not meant to be used directly, but rather to be inherited from. 2. minbpe/basic.py: Implements the `BasicTokenizer`, the simplest implementation of the BPE algorithm that runs directly on text. 3. minbpe/regex.py: Implements the `RegexTokenizer` that further splits the input text by a regex pattern, which is a preprocessing stage that splits up the input text by categories (think: letters, numbers, punctuation) before tokenization. This ensures that no merges will happen across category boundaries. This was introduced in the GPT-2 paper and continues to be in use as of GPT-4. This class also handles special tokens, if any. 4. minbpe/gpt4.py: Implements the `GPT4Tokenizer`. This class is a light wrapper around the `RegexTokenizer` (2, above) that exactly reproduces the tokenization of GPT-4 in the tiktoken library. The wrapping handles some details around recovering the exact merges in the tokenizer, and the handling of some unfortunate (and likely historical?) 1-byte token permutations. Finally, the script train.py trains the two major tokenizers on the input text tests/taylorswift.txt (this is the Wikipedia entry for her kek) and saves the vocab to disk for visualization. This script runs in about 25 seconds on my (M1) MacBook. All of the files above are very short and thoroughly commented, and also contain a usage example on the bottom of the file.
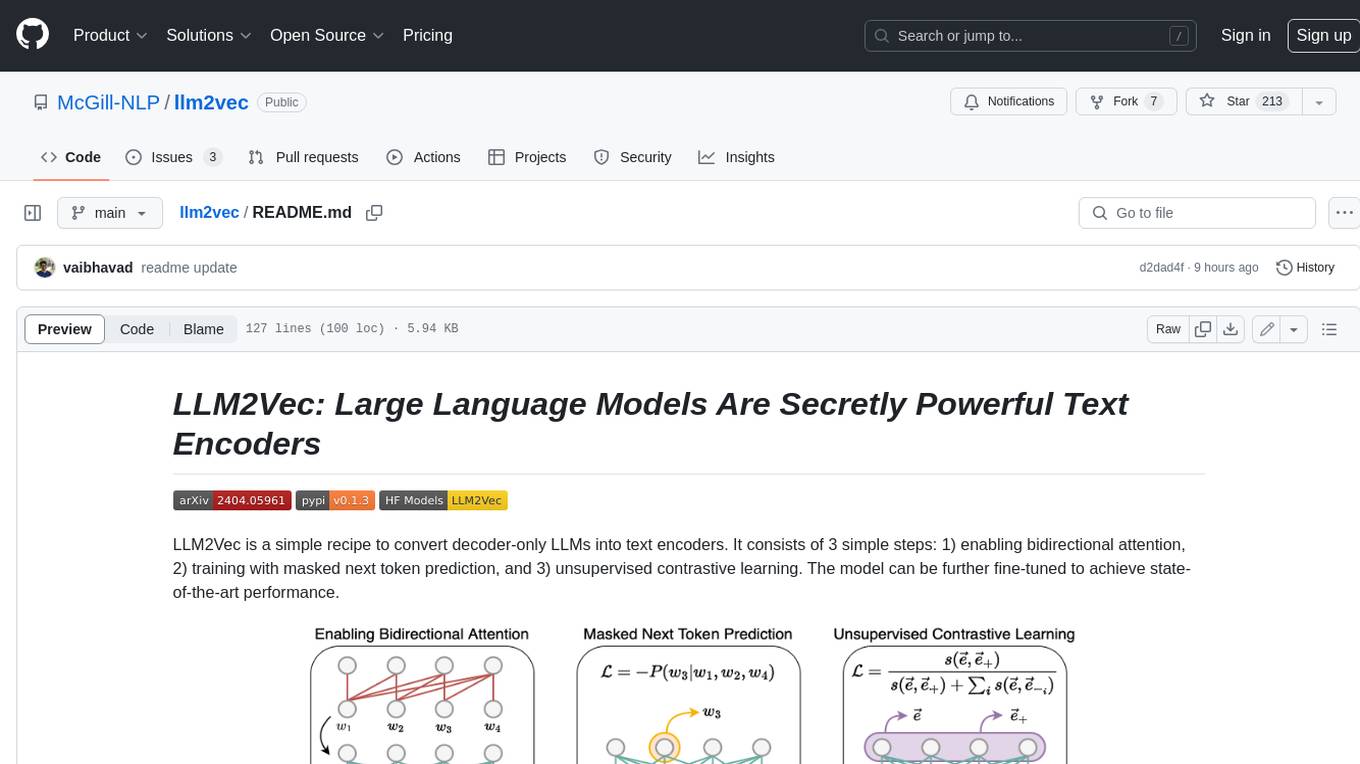
llm2vec
LLM2Vec is a simple recipe to convert decoder-only LLMs into text encoders. It consists of 3 simple steps: 1) enabling bidirectional attention, 2) training with masked next token prediction, and 3) unsupervised contrastive learning. The model can be further fine-tuned to achieve state-of-the-art performance.
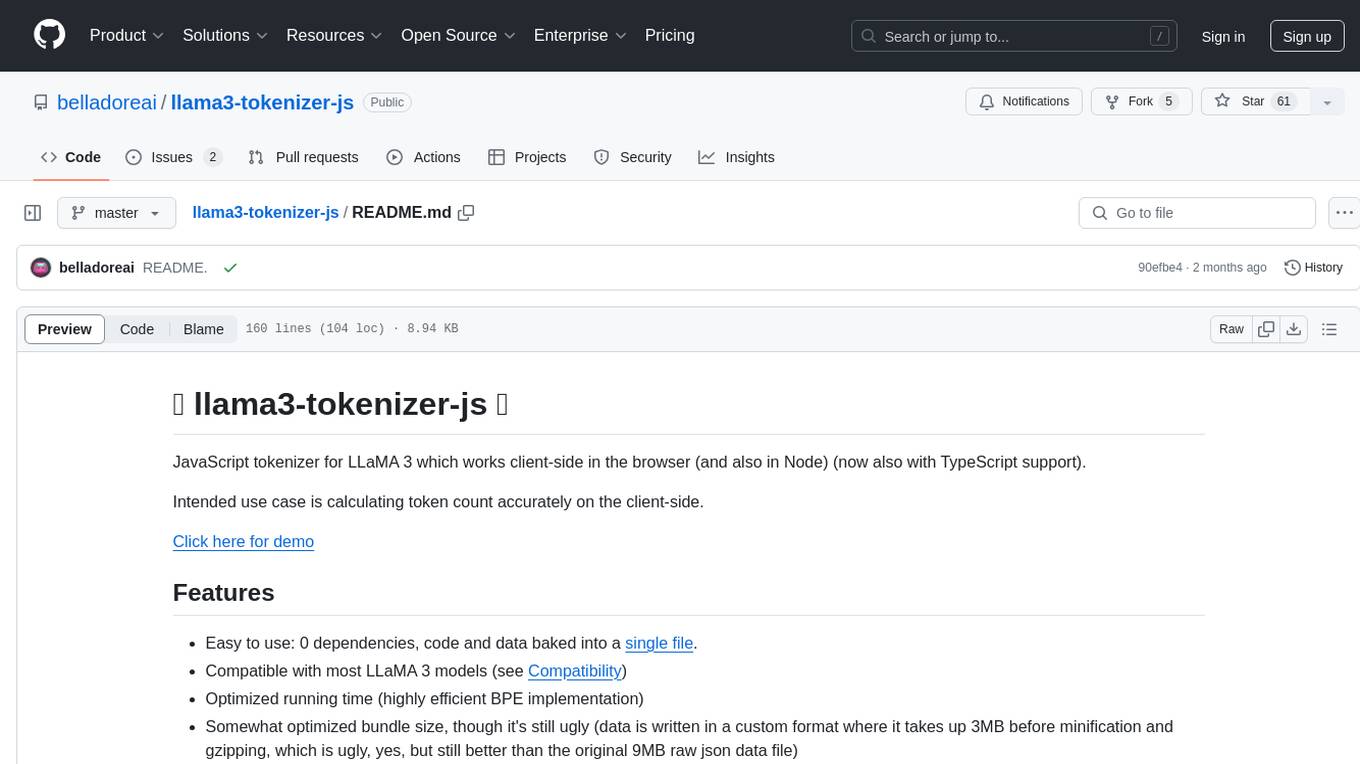
llama3-tokenizer-js
JavaScript tokenizer for LLaMA 3 designed for client-side use in the browser and Node, with TypeScript support. It accurately calculates token count, has 0 dependencies, optimized running time, and somewhat optimized bundle size. Compatible with most LLaMA 3 models. Can encode and decode text, but training is not supported. Pollutes global namespace with `llama3Tokenizer` in the browser. Mostly compatible with LLaMA 3 models released by Facebook in April 2024. Can be adapted for incompatible models by passing custom vocab and merge data. Handles special tokens and fine tunes. Developed by belladore.ai with contributions from xenova, blaze2004, imoneoi, and ConProgramming.
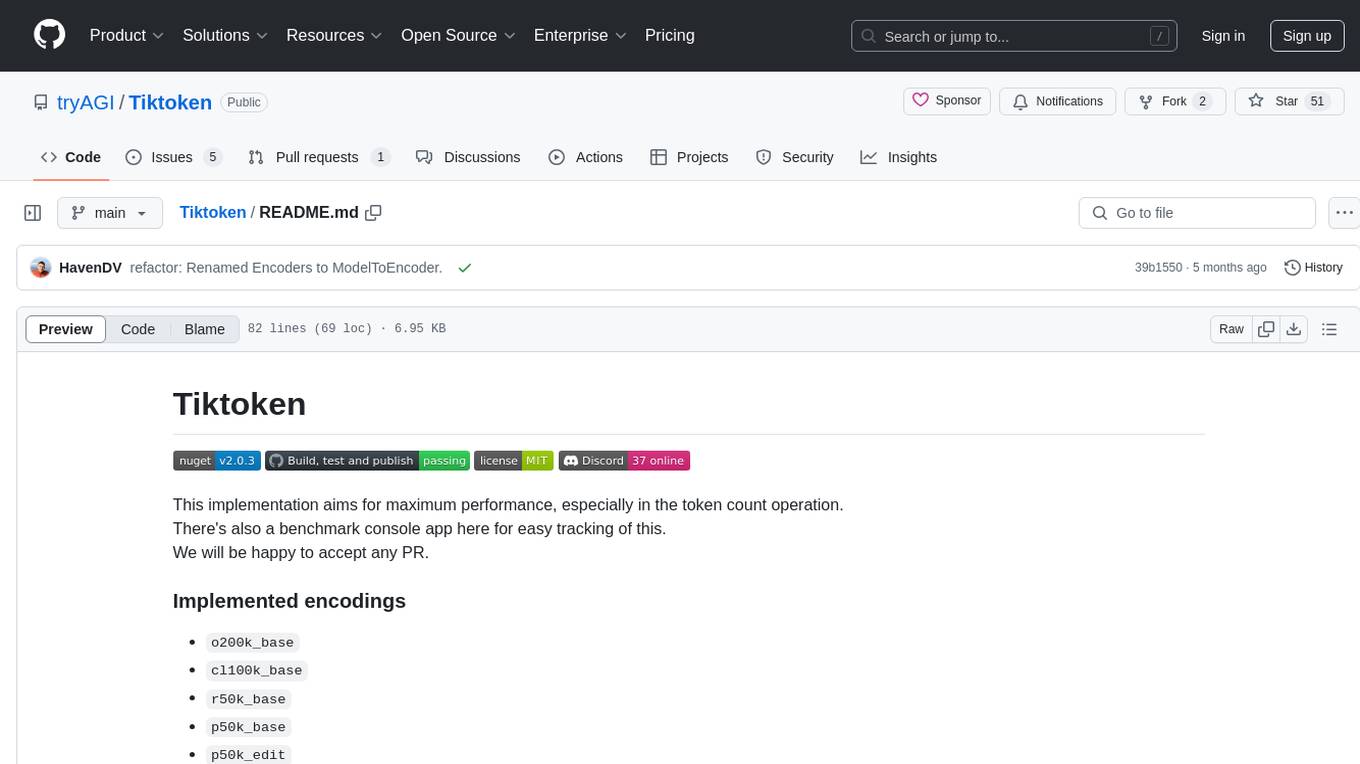
Tiktoken
Tiktoken is a high-performance implementation focused on token count operations. It provides various encodings like o200k_base, cl100k_base, r50k_base, p50k_base, and p50k_edit. Users can easily encode and decode text using the provided API. The repository also includes a benchmark console app for performance tracking. Contributions in the form of PRs are welcome.
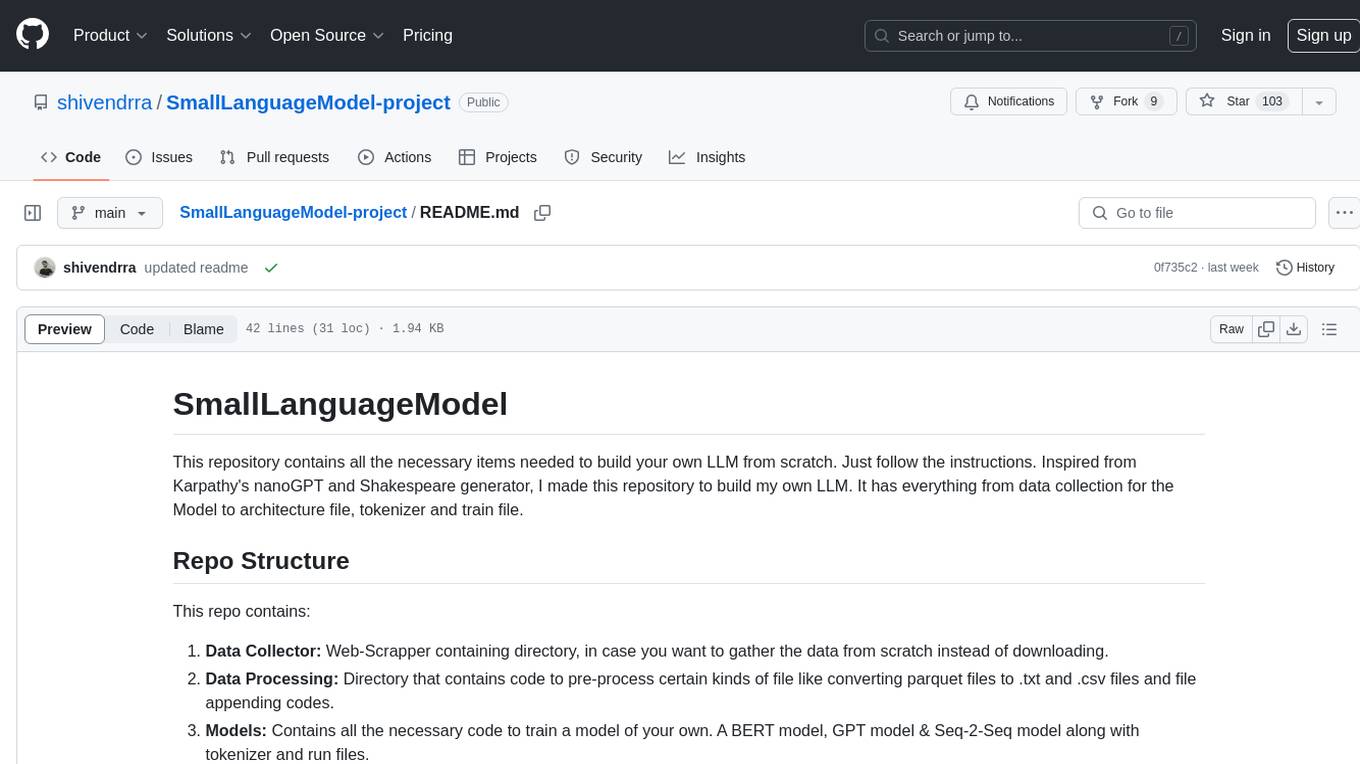
SmallLanguageModel-project
This repository provides all the necessary items to build a Language Model from scratch, inspired by Karpathy's nanoGPT and Shakespeare generator. It includes data collection tools, data processing scripts, various models like BERT, GPT, and Seq-2-Seq, along with tokenizer and training files.
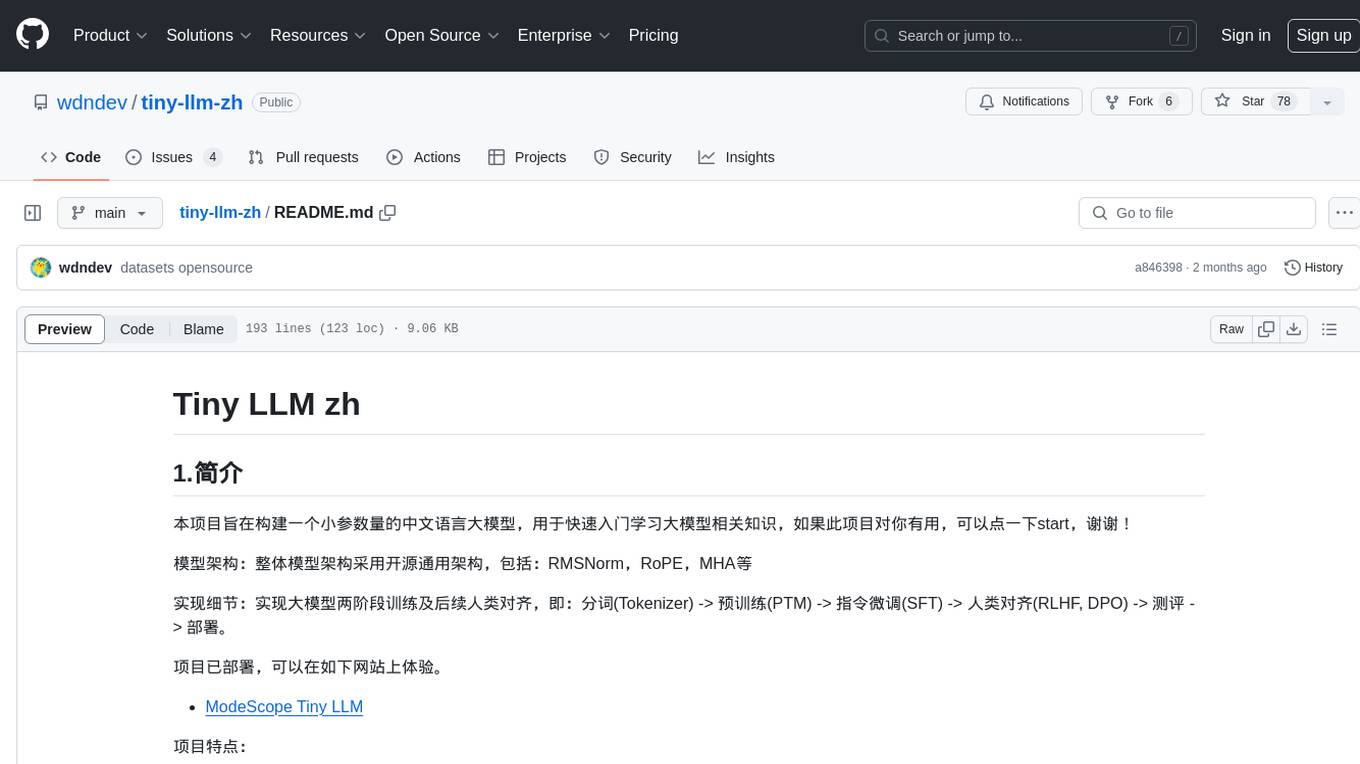
tiny-llm-zh
Tiny LLM zh is a project aimed at building a small-parameter Chinese language large model for quick entry into learning large model-related knowledge. The project implements a two-stage training process for large models and subsequent human alignment, including tokenization, pre-training, instruction fine-tuning, human alignment, evaluation, and deployment. It is deployed on ModeScope Tiny LLM website and features open access to all data and code, including pre-training data and tokenizer. The project trains a tokenizer using 10GB of Chinese encyclopedia text to build a Tiny LLM vocabulary. It supports training with Transformers deepspeed, multiple machine and card support, and Zero optimization techniques. The project has three main branches: llama2_torch, main tiny_llm, and tiny_llm_moe, each with specific modifications and features.
For similar jobs
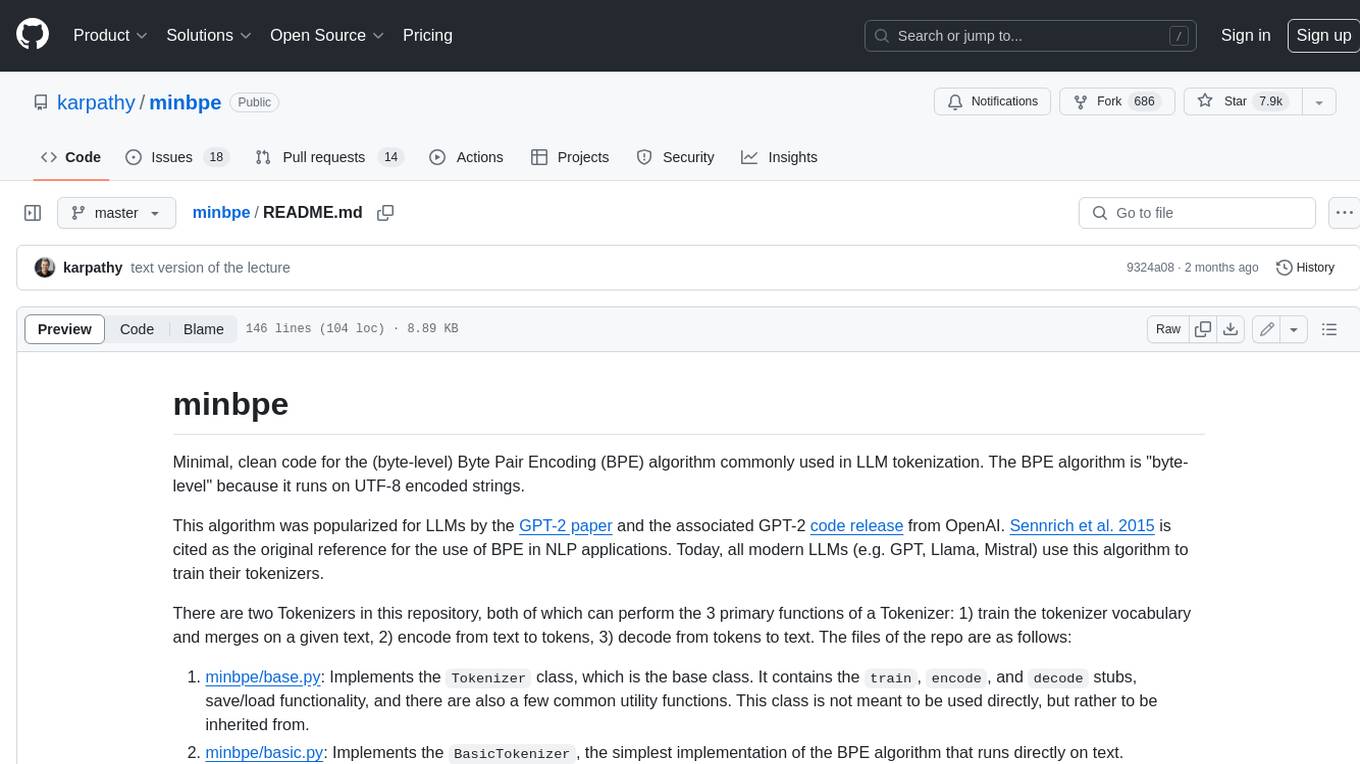
minbpe
This repository contains a minimal, clean code implementation of the Byte Pair Encoding (BPE) algorithm, commonly used in LLM tokenization. The BPE algorithm is "byte-level" because it runs on UTF-8 encoded strings. This algorithm was popularized for LLMs by the GPT-2 paper and the associated GPT-2 code release from OpenAI. Sennrich et al. 2015 is cited as the original reference for the use of BPE in NLP applications. Today, all modern LLMs (e.g. GPT, Llama, Mistral) use this algorithm to train their tokenizers. There are two Tokenizers in this repository, both of which can perform the 3 primary functions of a Tokenizer: 1) train the tokenizer vocabulary and merges on a given text, 2) encode from text to tokens, 3) decode from tokens to text. The files of the repo are as follows: 1. minbpe/base.py: Implements the `Tokenizer` class, which is the base class. It contains the `train`, `encode`, and `decode` stubs, save/load functionality, and there are also a few common utility functions. This class is not meant to be used directly, but rather to be inherited from. 2. minbpe/basic.py: Implements the `BasicTokenizer`, the simplest implementation of the BPE algorithm that runs directly on text. 3. minbpe/regex.py: Implements the `RegexTokenizer` that further splits the input text by a regex pattern, which is a preprocessing stage that splits up the input text by categories (think: letters, numbers, punctuation) before tokenization. This ensures that no merges will happen across category boundaries. This was introduced in the GPT-2 paper and continues to be in use as of GPT-4. This class also handles special tokens, if any. 4. minbpe/gpt4.py: Implements the `GPT4Tokenizer`. This class is a light wrapper around the `RegexTokenizer` (2, above) that exactly reproduces the tokenization of GPT-4 in the tiktoken library. The wrapping handles some details around recovering the exact merges in the tokenizer, and the handling of some unfortunate (and likely historical?) 1-byte token permutations. Finally, the script train.py trains the two major tokenizers on the input text tests/taylorswift.txt (this is the Wikipedia entry for her kek) and saves the vocab to disk for visualization. This script runs in about 25 seconds on my (M1) MacBook. All of the files above are very short and thoroughly commented, and also contain a usage example on the bottom of the file.
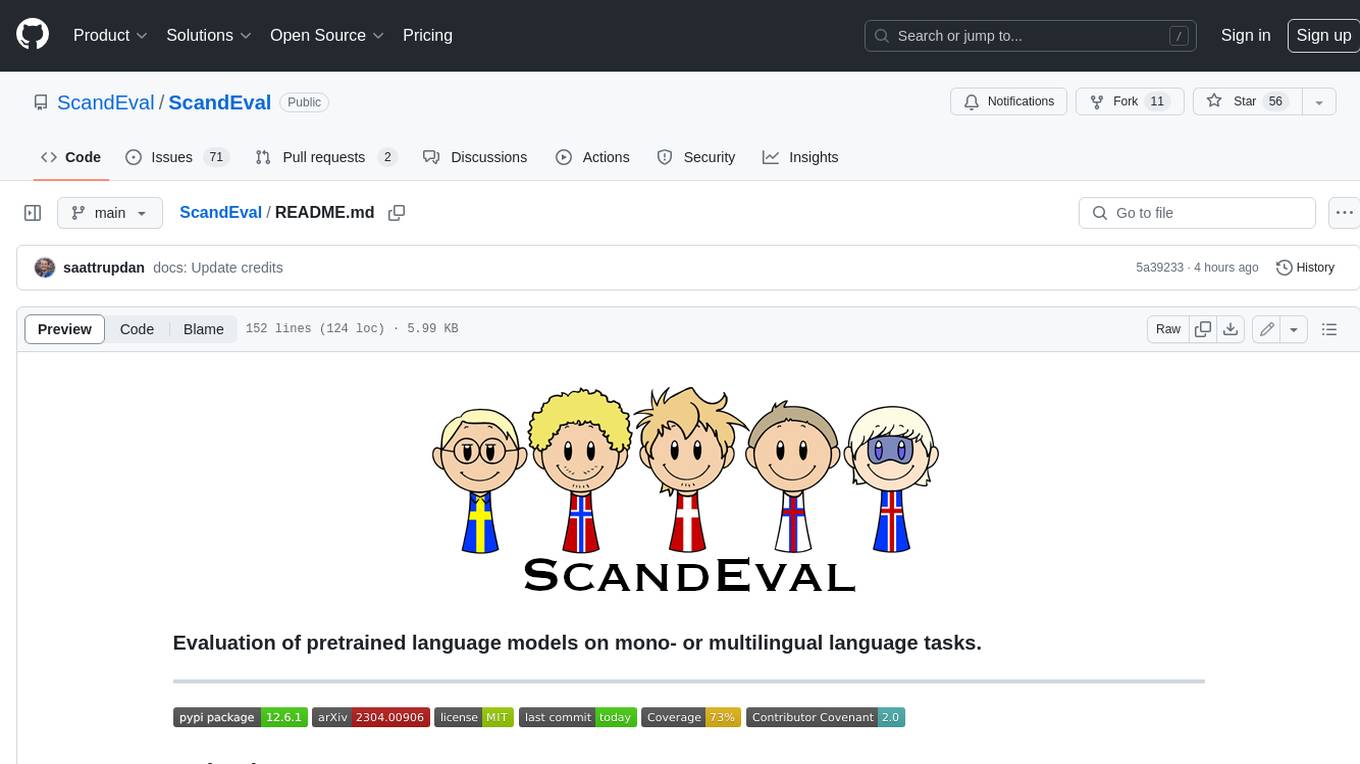
ScandEval
ScandEval is a framework for evaluating pretrained language models on mono- or multilingual language tasks. It provides a unified interface for benchmarking models on a variety of tasks, including sentiment analysis, question answering, and machine translation. ScandEval is designed to be easy to use and extensible, making it a valuable tool for researchers and practitioners alike.
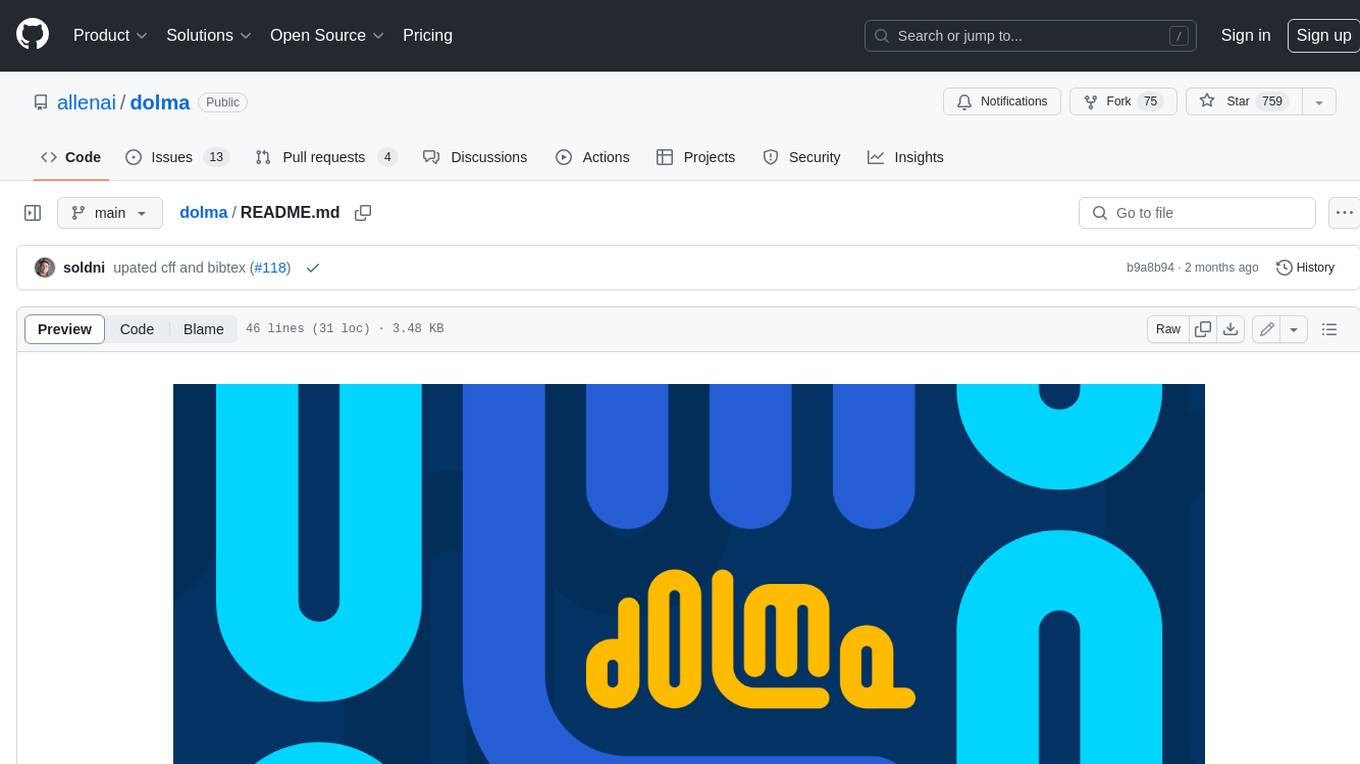
dolma
Dolma is a dataset and toolkit for curating large datasets for (pre)-training ML models. The dataset consists of 3 trillion tokens from a diverse mix of web content, academic publications, code, books, and encyclopedic materials. The toolkit provides high-performance, portable, and extensible tools for processing, tagging, and deduplicating documents. Key features of the toolkit include built-in taggers, fast deduplication, and cloud support.
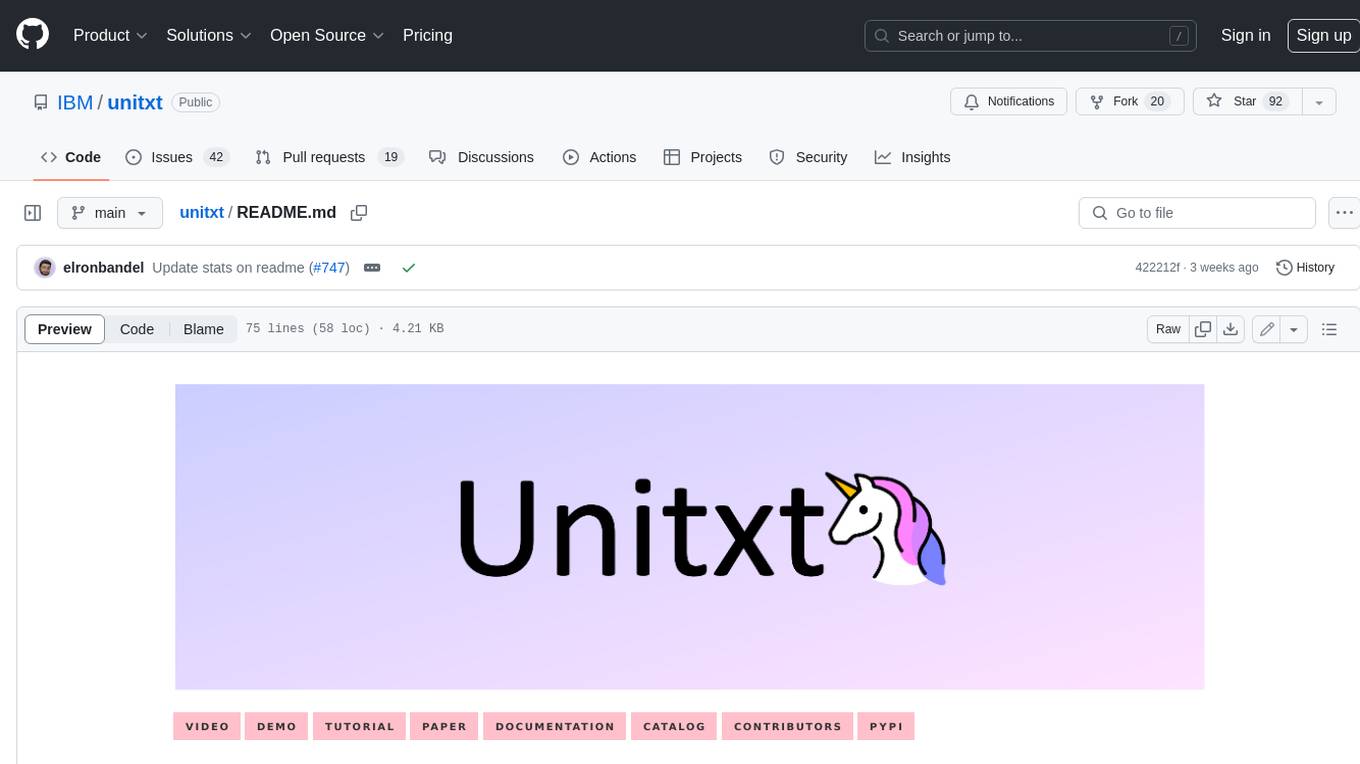
unitxt
Unitxt is a customizable library for textual data preparation and evaluation tailored to generative language models. It natively integrates with common libraries like HuggingFace and LM-eval-harness and deconstructs processing flows into modular components, enabling easy customization and sharing between practitioners. These components encompass model-specific formats, task prompts, and many other comprehensive dataset processing definitions. The Unitxt-Catalog centralizes these components, fostering collaboration and exploration in modern textual data workflows. Beyond being a tool, Unitxt is a community-driven platform, empowering users to build, share, and advance their pipelines collaboratively.
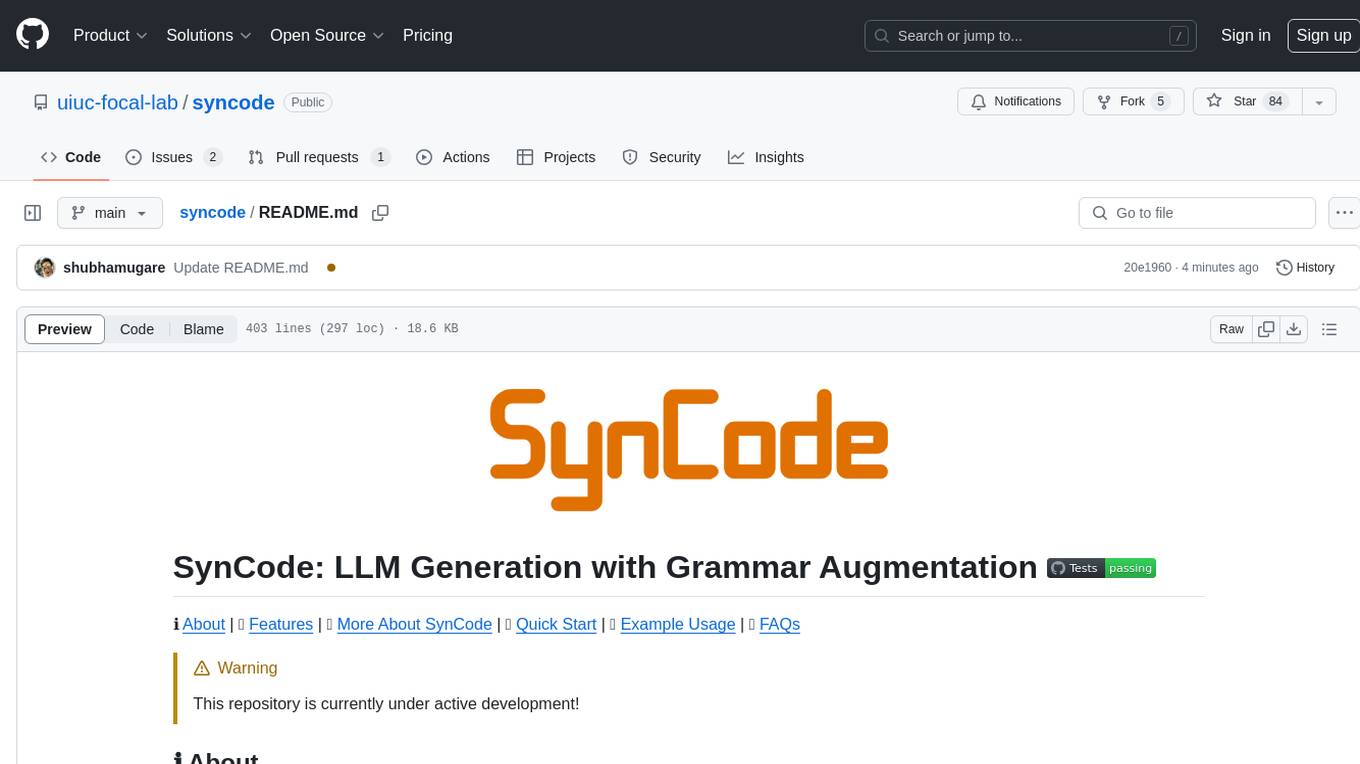
syncode
SynCode is a novel framework for the grammar-guided generation of Large Language Models (LLMs) that ensures syntactically valid output with respect to defined Context-Free Grammar (CFG) rules. It supports general-purpose programming languages like Python, Go, SQL, JSON, and more, allowing users to define custom grammars using EBNF syntax. The tool compares favorably to other constrained decoders and offers features like fast grammar-guided generation, compatibility with HuggingFace Language Models, and the ability to work with various decoding strategies.
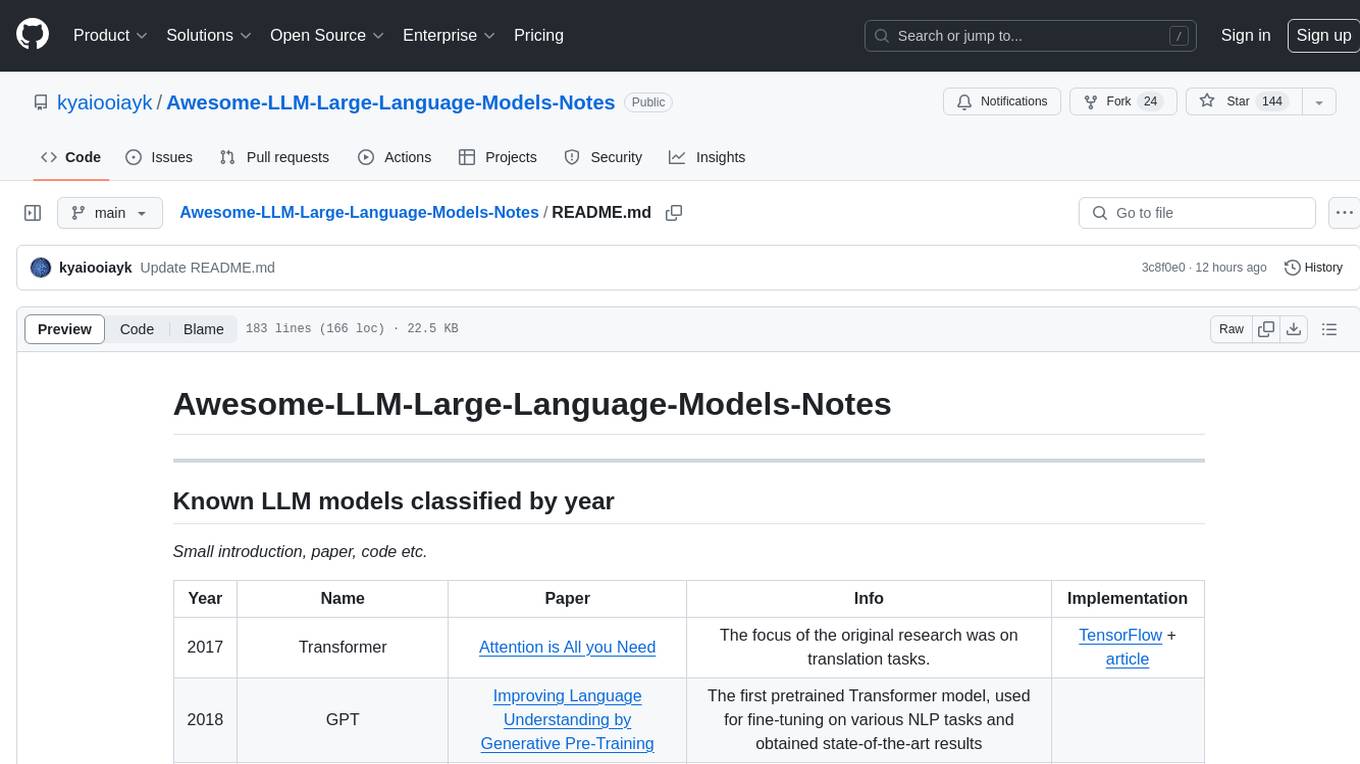
Awesome-LLM-Large-Language-Models-Notes
Awesome-LLM-Large-Language-Models-Notes is a repository that provides a comprehensive collection of information on various Large Language Models (LLMs) classified by year, size, and name. It includes details on known LLM models, their papers, implementations, and specific characteristics. The repository also covers LLM models classified by architecture, must-read papers, blog articles, tutorials, and implementations from scratch. It serves as a valuable resource for individuals interested in understanding and working with LLMs in the field of Natural Language Processing (NLP).
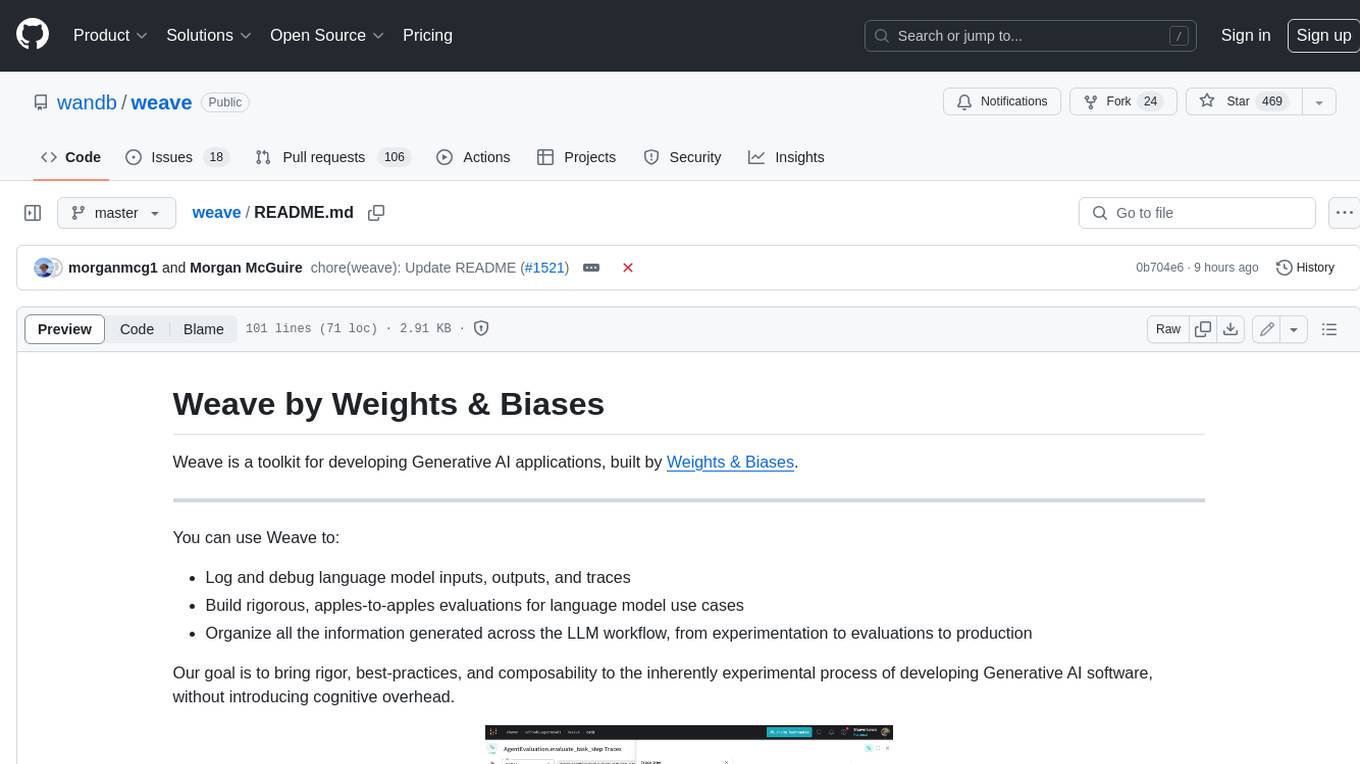
weave
Weave is a toolkit for developing Generative AI applications, built by Weights & Biases. With Weave, you can log and debug language model inputs, outputs, and traces; build rigorous, apples-to-apples evaluations for language model use cases; and organize all the information generated across the LLM workflow, from experimentation to evaluations to production. Weave aims to bring rigor, best-practices, and composability to the inherently experimental process of developing Generative AI software, without introducing cognitive overhead.
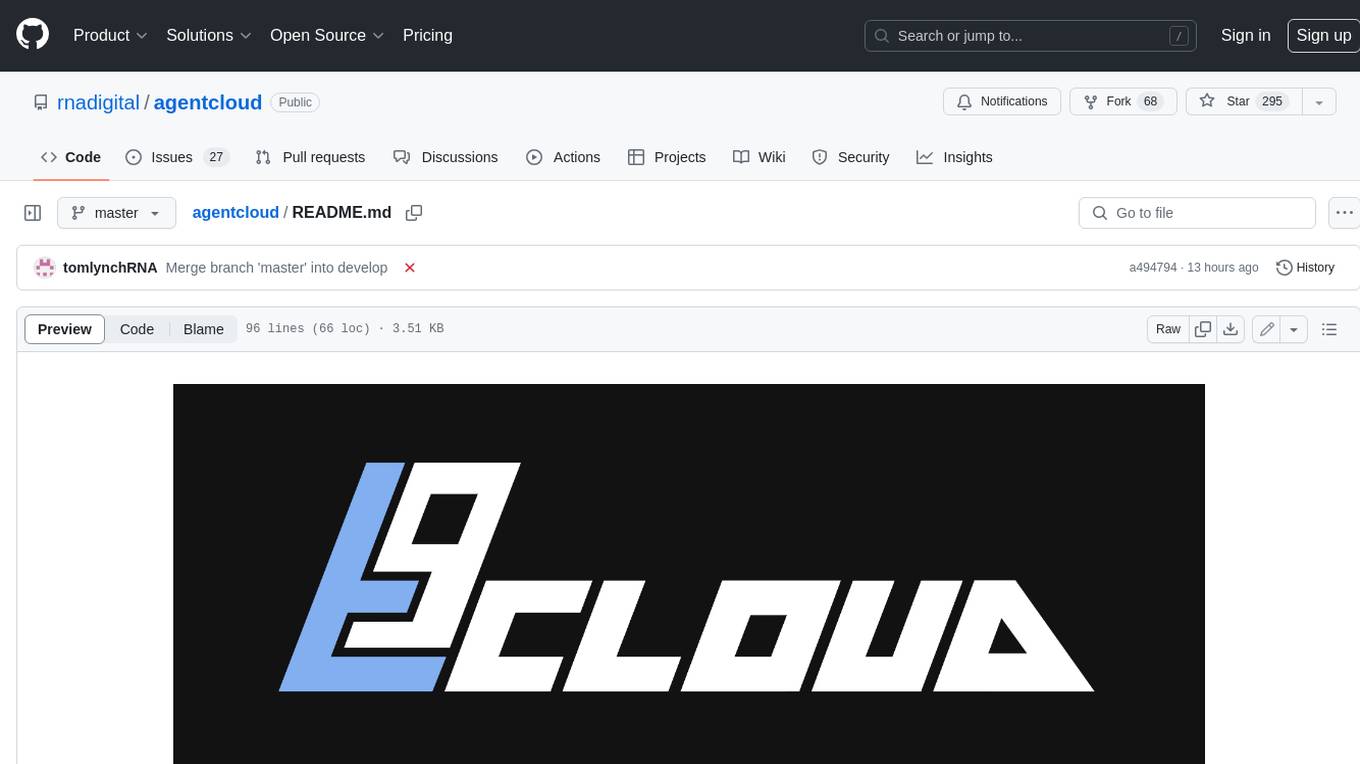
agentcloud
AgentCloud is an open-source platform that enables companies to build and deploy private LLM chat apps, empowering teams to securely interact with their data. It comprises three main components: Agent Backend, Webapp, and Vector Proxy. To run this project locally, clone the repository, install Docker, and start the services. The project is licensed under the GNU Affero General Public License, version 3 only. Contributions and feedback are welcome from the community.