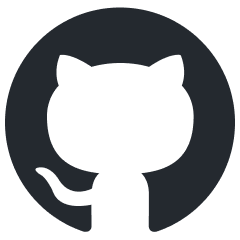
unitxt
π¦ Unitxt: a python library for getting data fired up and set for training and evaluation
Stars: 172
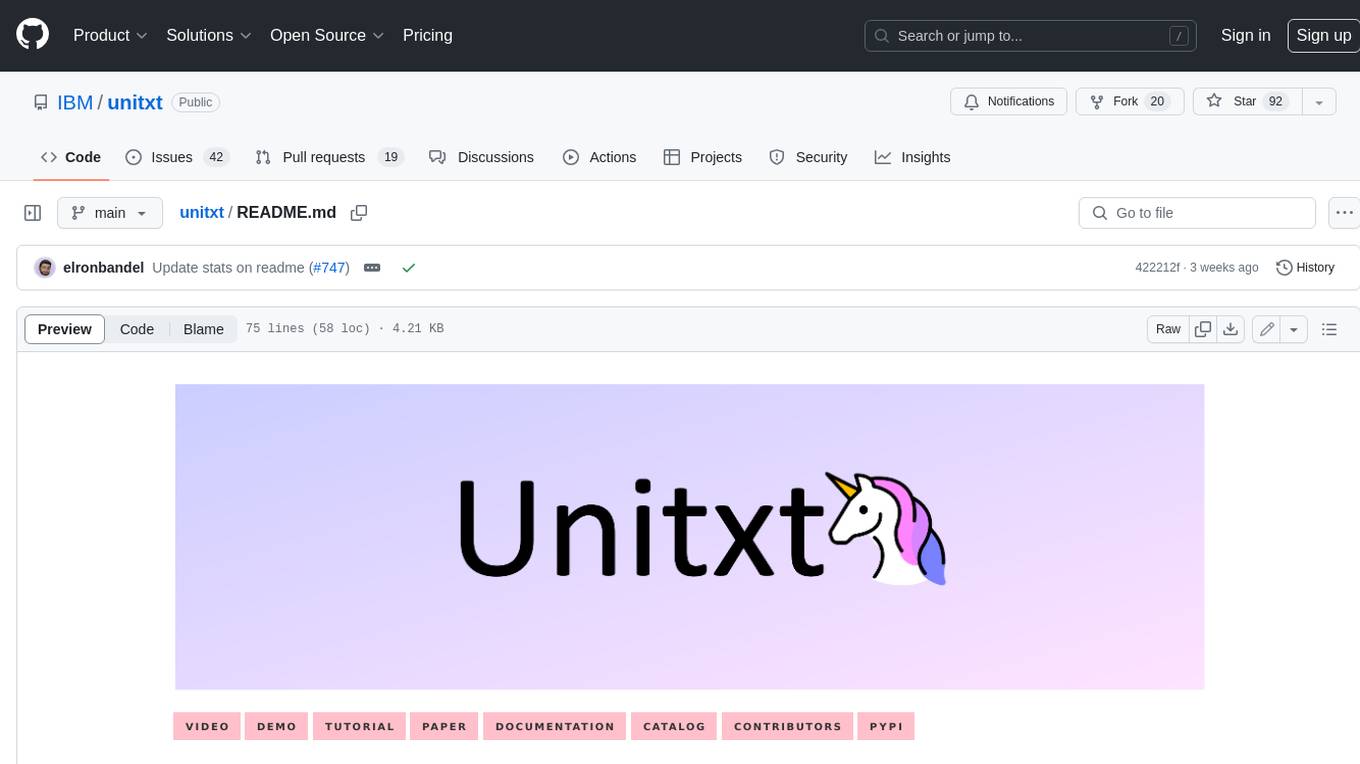
Unitxt is a customizable library for textual data preparation and evaluation tailored to generative language models. It natively integrates with common libraries like HuggingFace and LM-eval-harness and deconstructs processing flows into modular components, enabling easy customization and sharing between practitioners. These components encompass model-specific formats, task prompts, and many other comprehensive dataset processing definitions. The Unitxt-Catalog centralizes these components, fostering collaboration and exploration in modern textual data workflows. Beyond being a tool, Unitxt is a community-driven platform, empowering users to build, share, and advance their pipelines collaboratively.
README:
In the dynamic landscape of generative NLP, traditional text processing pipelines limit research flexibility and reproducibility, as they are tailored to specific dataset, task, and model combinations. The escalating complexity, involving system prompts, model-specific formats, instructions, and more, calls for a shift to a structured, modular, and customizable solution.
Addressing this need, we present Unitxt, an innovative library for customizable textual data preparation and evaluation tailored to generative language models. Unitxt natively integrates with common libraries like HuggingFace and LM-eval-harness and deconstructs processing flows into modular components, enabling easy customization and sharing between practitioners. These components encompass model-specific formats, task prompts, and many other comprehensive dataset processing definitions. The Unitxt-Catalog centralizes these components, fostering collaboration and exploration in modern textual data workflows. Beyond being a tool, Unitxt is a community-driven platform, empowering users to build, share, and advance their pipelines collaboratively.
https://github.com/IBM/unitxt/assets/23455264/baef9131-39d4-4164-90b2-05da52919fdf
To launch unitxt graphical user interface first install unitxt with ui requirements:
pip install unitxt[ui]
Then launch the ui by running:
unitxt-explore
This is a simple example of running end-to-end evaluation in self contained python code over user data.
See more examples in examples subdirectory.
# Import required components
from unitxt import evaluate, create_dataset
from unitxt.blocks import Task, InputOutputTemplate
from unitxt.inference import HFAutoModelInferenceEngine
# Question-answer dataset
data = [
{"question": "What is the capital of Texas?", "answer": "Austin"},
{"question": "What is the color of the sky?", "answer": "Blue"},
]
# Define the task and evaluation metric
task = Task(
input_fields={"question": str},
reference_fields={"answer": str},
prediction_type=str,
metrics=["metrics.accuracy"],
)
# Create a template to format inputs and outputs
template = InputOutputTemplate(
instruction="Answer the following question.",
input_format="{question}",
output_format="{answer}",
postprocessors=["processors.lower_case"],
)
# Prepare the dataset
dataset = create_dataset(
task=task,
template=template,
format="formats.chat_api",
test_set=data,
split="test",
)
# Set up the model (supports Hugging Face, WatsonX, OpenAI, etc.)
model = HFAutoModelInferenceEngine(
model_name="Qwen/Qwen1.5-0.5B-Chat", max_new_tokens=32
)
# Generate predictions and evaluate
predictions = model(dataset)
results = evaluate(predictions=predictions, data=dataset)
# Print results
print("Global Results:\n", results.global_scores.summary)
print("Instance Results:\n", results.instance_scores.summary)
Please install Unitxt from source by:
git clone [email protected]:IBM/unitxt.git
cd unitxt
pip install -e ".[dev]"
pre-commit install
If you use Unitxt in your research, please cite our paper:
@inproceedings{bandel-etal-2024-unitxt,
title = "Unitxt: Flexible, Shareable and Reusable Data Preparation and Evaluation for Generative {AI}",
author = "Bandel, Elron and
Perlitz, Yotam and
Venezian, Elad and
Friedman, Roni and
Arviv, Ofir and
Orbach, Matan and
Don-Yehiya, Shachar and
Sheinwald, Dafna and
Gera, Ariel and
Choshen, Leshem and
Shmueli-Scheuer, Michal and
Katz, Yoav",
editor = "Chang, Kai-Wei and
Lee, Annie and
Rajani, Nazneen",
booktitle = "Proceedings of the 2024 Conference of the North American Chapter of the Association for Computational Linguistics: Human Language Technologies (Volume 3: System Demonstrations)",
month = jun,
year = "2024",
address = "Mexico City, Mexico",
publisher = "Association for Computational Linguistics",
url = "https://aclanthology.org/2024.naacl-demo.21",
pages = "207--215",
abstract = "In the dynamic landscape of generative NLP, traditional text processing pipelines limit research flexibility and reproducibility, as they are tailored to specific dataset, task, and model combinations. The escalating complexity, involving system prompts, model-specific formats, instructions, and more, calls for a shift to a structured, modular, and customizable solution.Addressing this need, we present Unitxt, an innovative library for customizable textual data preparation and evaluation tailored to generative language models. Unitxt natively integrates with common libraries like HuggingFace and LM-eval-harness and deconstructs processing flows into modular components, enabling easy customization and sharing between practitioners. These components encompass model-specific formats, task prompts, and many other comprehensive dataset processing definitions. The Unitxt Catalog centralizes these components, fostering collaboration and exploration in modern textual data workflows. Beyond being a tool, Unitxt is a community-driven platform, empowering users to build, share, and advance their pipelines collaboratively. Join the Unitxt community at https://github.com/IBM/unitxt",
}
Unitxt emoji designed by OpenMoji - the open-source emoji and icon project. License: CC BY-SA 4.0
For Tasks:
Click tags to check more tools for each tasksFor Jobs:
Alternative AI tools for unitxt
Similar Open Source Tools
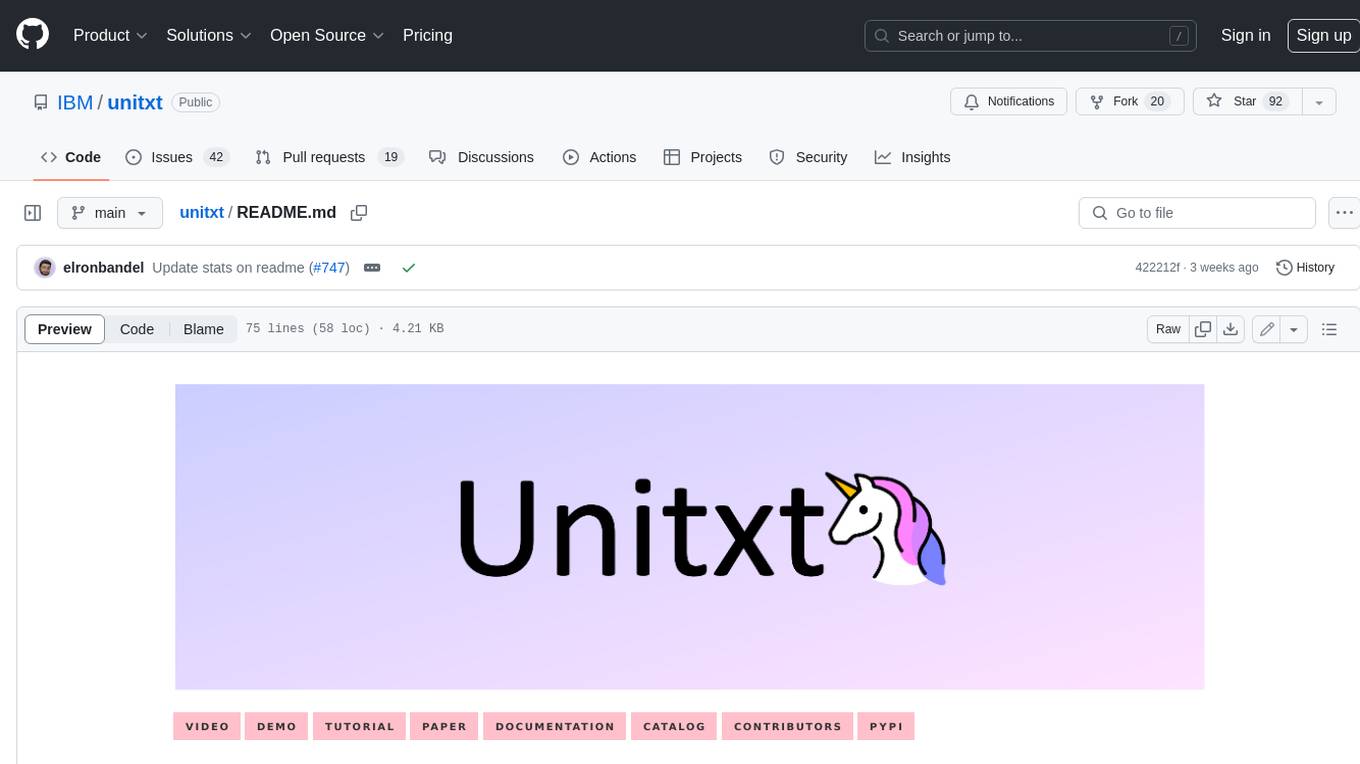
unitxt
Unitxt is a customizable library for textual data preparation and evaluation tailored to generative language models. It natively integrates with common libraries like HuggingFace and LM-eval-harness and deconstructs processing flows into modular components, enabling easy customization and sharing between practitioners. These components encompass model-specific formats, task prompts, and many other comprehensive dataset processing definitions. The Unitxt-Catalog centralizes these components, fostering collaboration and exploration in modern textual data workflows. Beyond being a tool, Unitxt is a community-driven platform, empowering users to build, share, and advance their pipelines collaboratively.
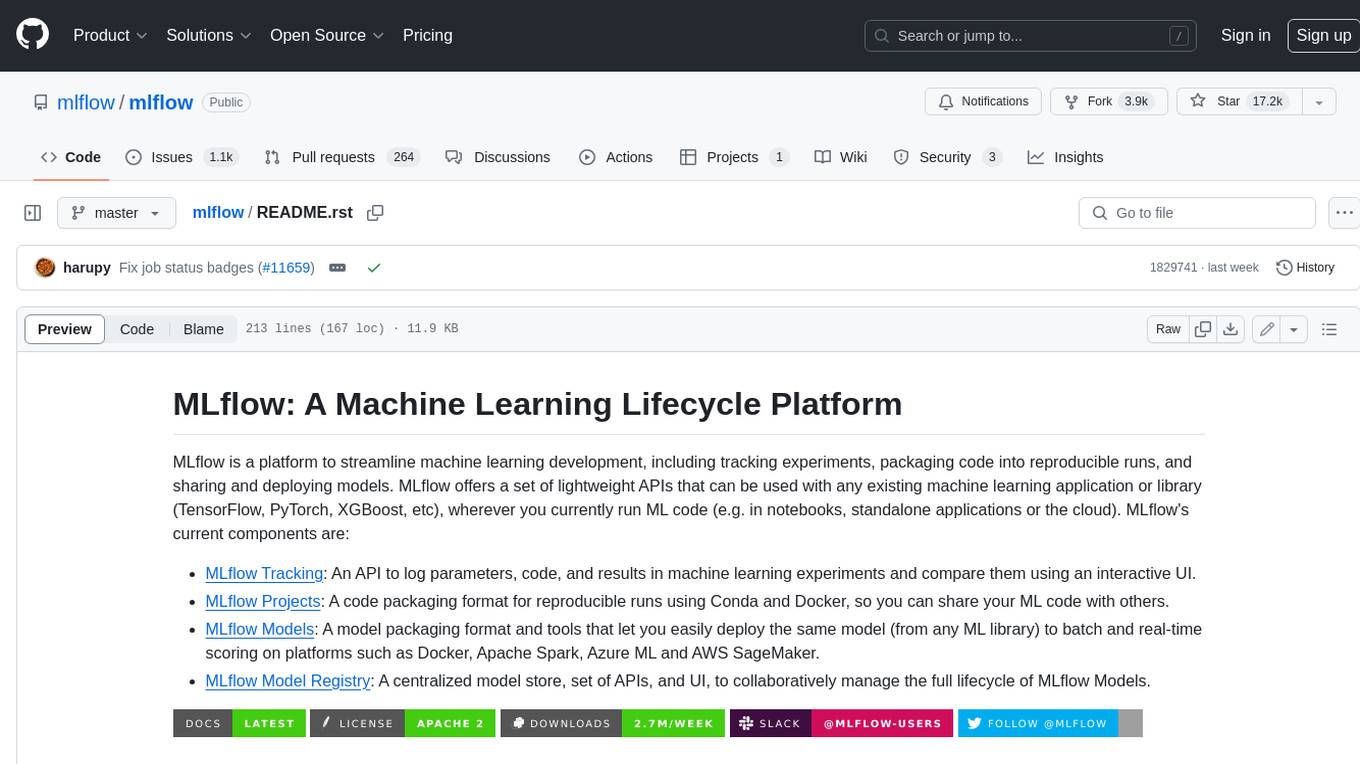
mlflow
MLflow is a platform to streamline machine learning development, including tracking experiments, packaging code into reproducible runs, and sharing and deploying models. MLflow offers a set of lightweight APIs that can be used with any existing machine learning application or library (TensorFlow, PyTorch, XGBoost, etc), wherever you currently run ML code (e.g. in notebooks, standalone applications or the cloud). MLflow's current components are:
* `MLflow Tracking
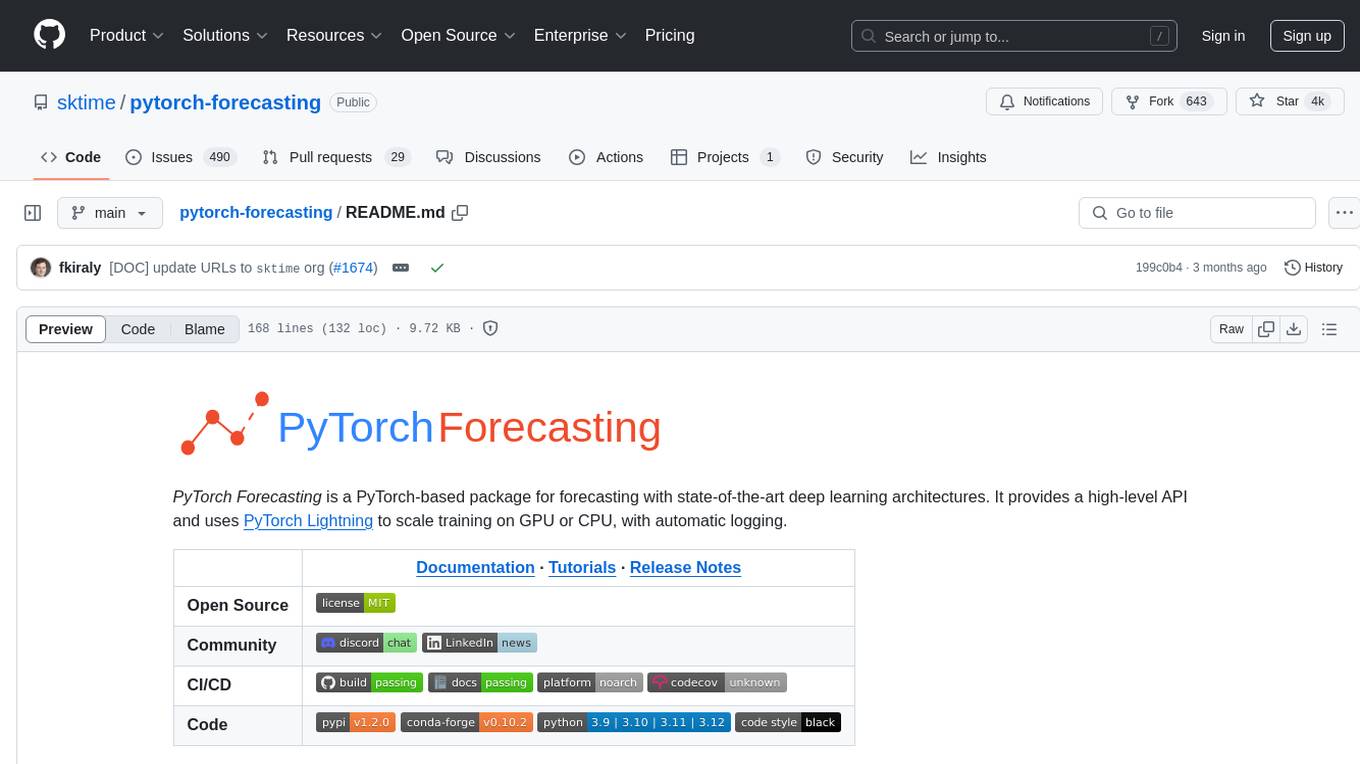
pytorch-forecasting
PyTorch Forecasting is a PyTorch-based package designed for state-of-the-art timeseries forecasting using deep learning architectures. It offers a high-level API and leverages PyTorch Lightning for efficient training on GPU or CPU with automatic logging. The package aims to simplify timeseries forecasting tasks by providing a flexible API for professionals and user-friendly defaults for beginners. It includes features such as a timeseries dataset class for handling data transformations, missing values, and subsampling, various neural network architectures optimized for real-world deployment, multi-horizon timeseries metrics, and hyperparameter tuning with optuna. Built on pytorch-lightning, it supports training on CPUs, single GPUs, and multiple GPUs out-of-the-box.
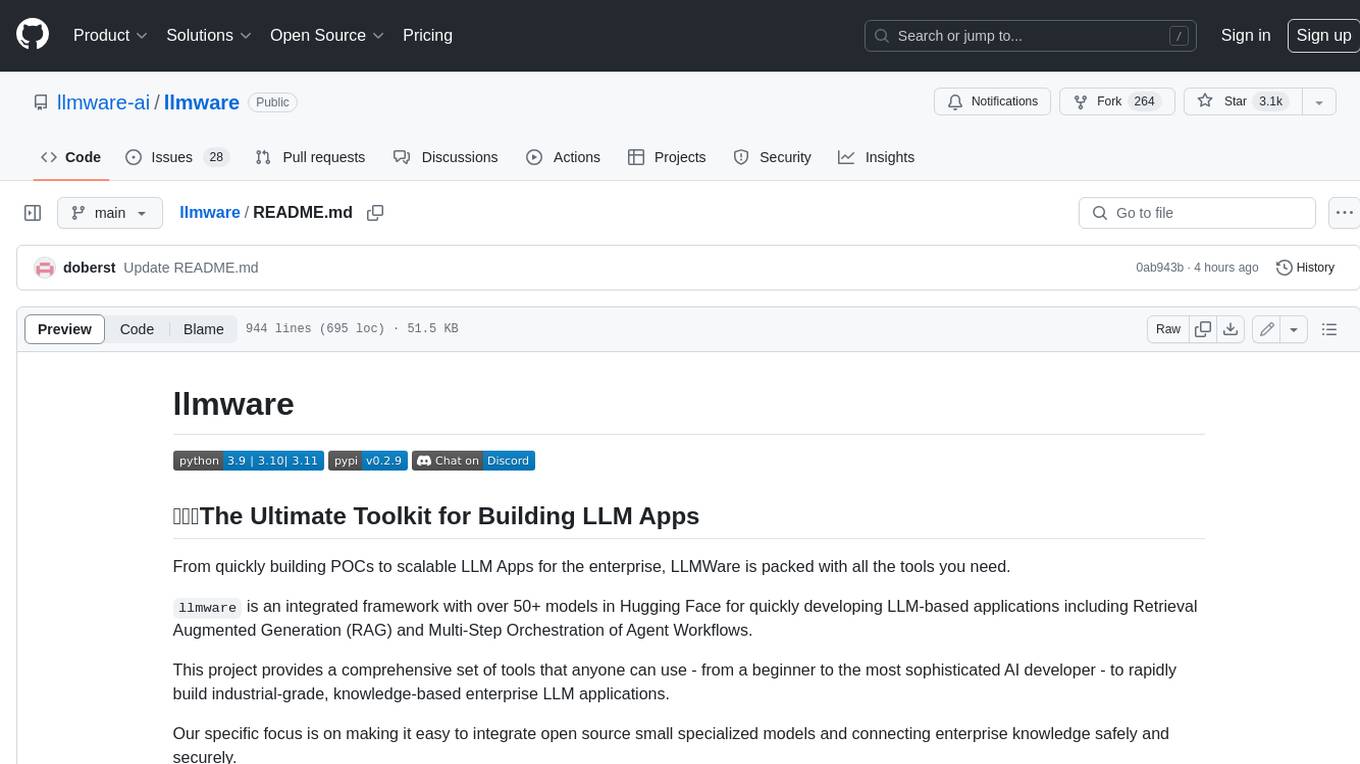
llmware
LLMWare is a framework for quickly developing LLM-based applications including Retrieval Augmented Generation (RAG) and Multi-Step Orchestration of Agent Workflows. This project provides a comprehensive set of tools that anyone can use - from a beginner to the most sophisticated AI developer - to rapidly build industrial-grade, knowledge-based enterprise LLM applications. Our specific focus is on making it easy to integrate open source small specialized models and connecting enterprise knowledge safely and securely.
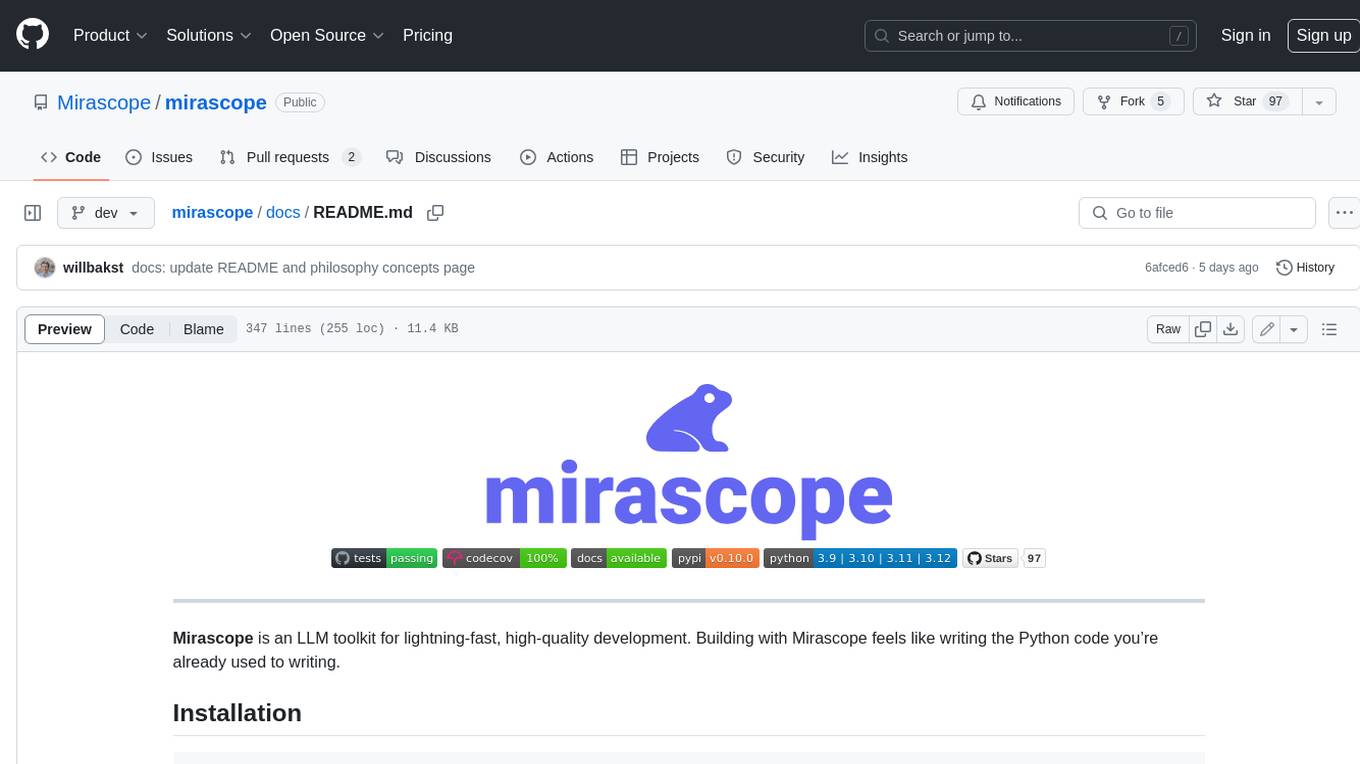
mirascope
Mirascope is an LLM toolkit for lightning-fast, high-quality development. Building with Mirascope feels like writing the Python code youβre already used to writing.
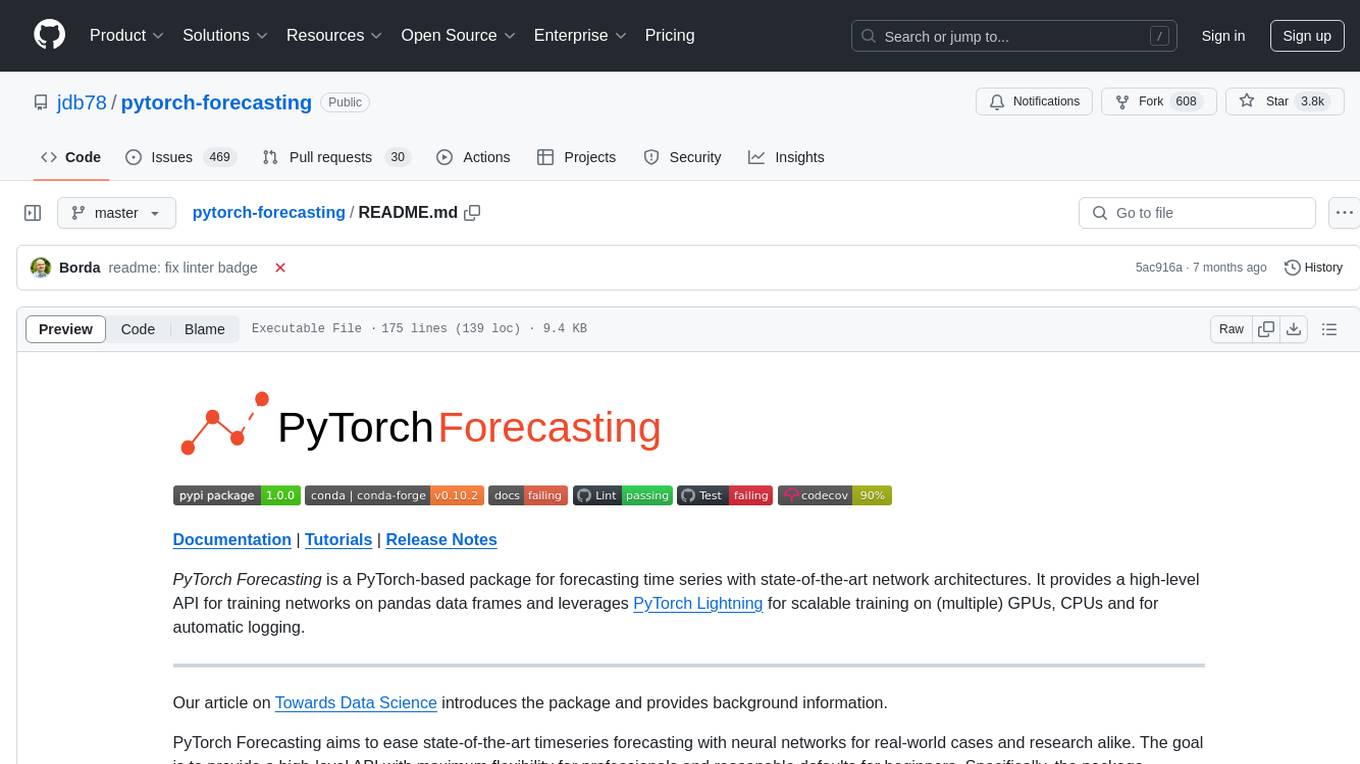
pytorch-forecasting
PyTorch Forecasting is a PyTorch-based package for time series forecasting with state-of-the-art network architectures. It offers a high-level API for training networks on pandas data frames and utilizes PyTorch Lightning for scalable training on GPUs and CPUs. The package aims to simplify time series forecasting with neural networks by providing a flexible API for professionals and default settings for beginners. It includes a timeseries dataset class, base model class, multiple neural network architectures, multi-horizon timeseries metrics, and hyperparameter tuning with optuna. PyTorch Forecasting is built on pytorch-lightning for easy training on various hardware configurations.
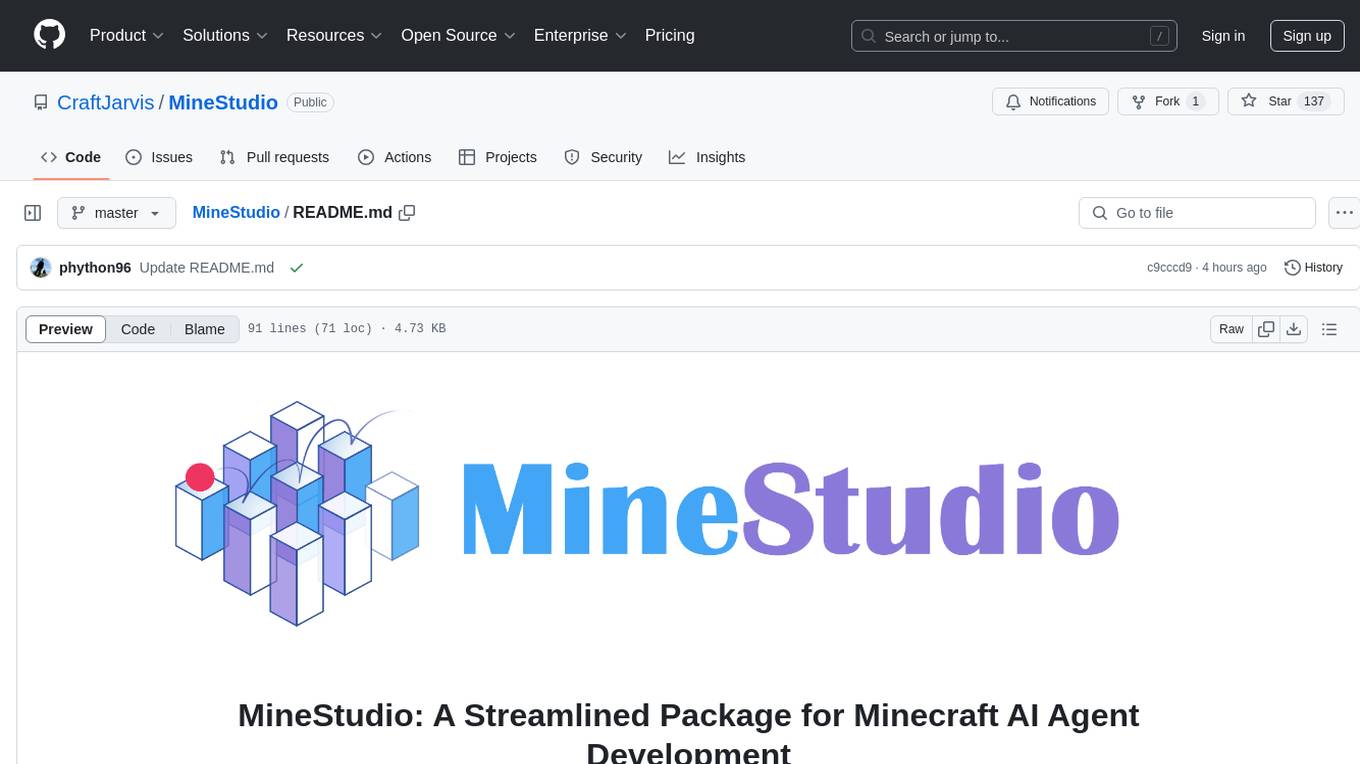
MineStudio
MineStudio is a simple and efficient Minecraft development kit for AI research. It contains tools and APIs for developing Minecraft AI agents, including a customizable simulator, trajectory data structure, policy models, offline and online training pipelines, inference framework, and benchmarking automation. The repository is under development and welcomes contributions and suggestions.
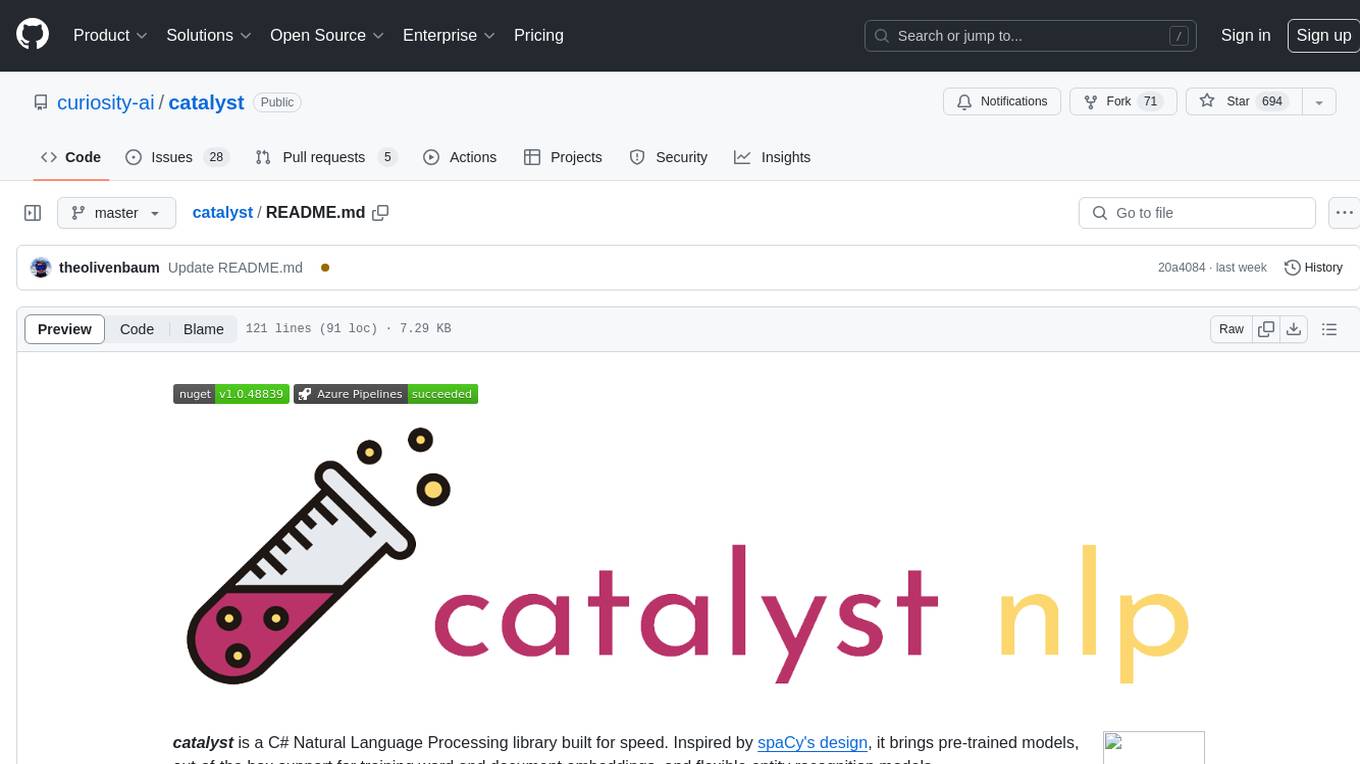
catalyst
Catalyst is a C# Natural Language Processing library designed for speed, inspired by spaCy's design. It provides pre-trained models, support for training word and document embeddings, and flexible entity recognition models. The library is fast, modern, and pure-C#, supporting .NET standard 2.0. It is cross-platform, running on Windows, Linux, macOS, and ARM. Catalyst offers non-destructive tokenization, named entity recognition, part-of-speech tagging, language detection, and efficient binary serialization. It includes pre-built models for language packages and lemmatization. Users can store and load models using streams. Getting started with Catalyst involves installing its NuGet Package and setting the storage to use the online repository. The library supports lazy loading of models from disk or online. Users can take advantage of C# lazy evaluation and native multi-threading support to process documents in parallel. Training a new FastText word2vec embedding model is straightforward, and Catalyst also provides algorithms for fast embedding search and dimensionality reduction.
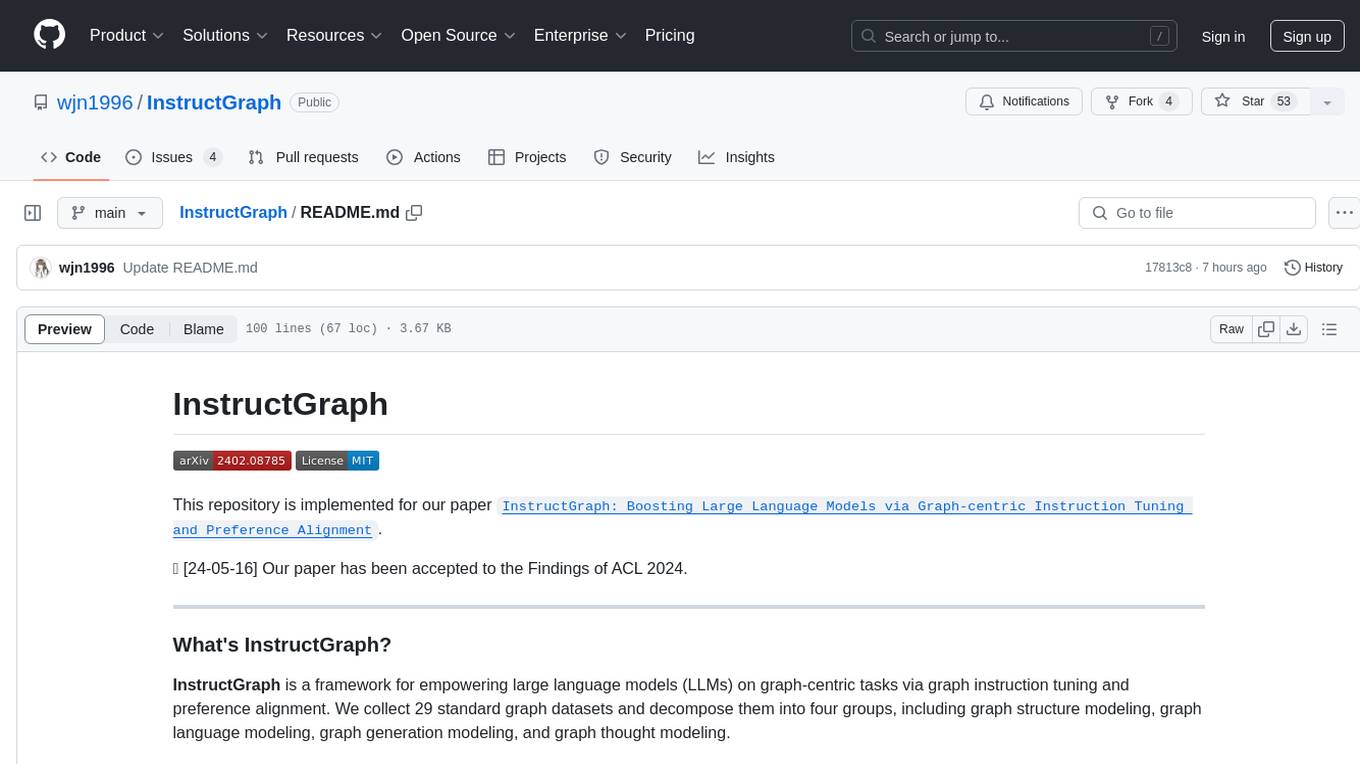
InstructGraph
InstructGraph is a framework designed to enhance large language models (LLMs) for graph-centric tasks by utilizing graph instruction tuning and preference alignment. The tool collects and decomposes 29 standard graph datasets into four groups, enabling LLMs to better understand and generate graph data. It introduces a structured format verbalizer to transform graph data into a code-like format, facilitating code understanding and generation. Additionally, it addresses hallucination problems in graph reasoning and generation through direct preference optimization (DPO). The tool aims to bridge the gap between textual LLMs and graph data, offering a comprehensive solution for graph-related tasks.
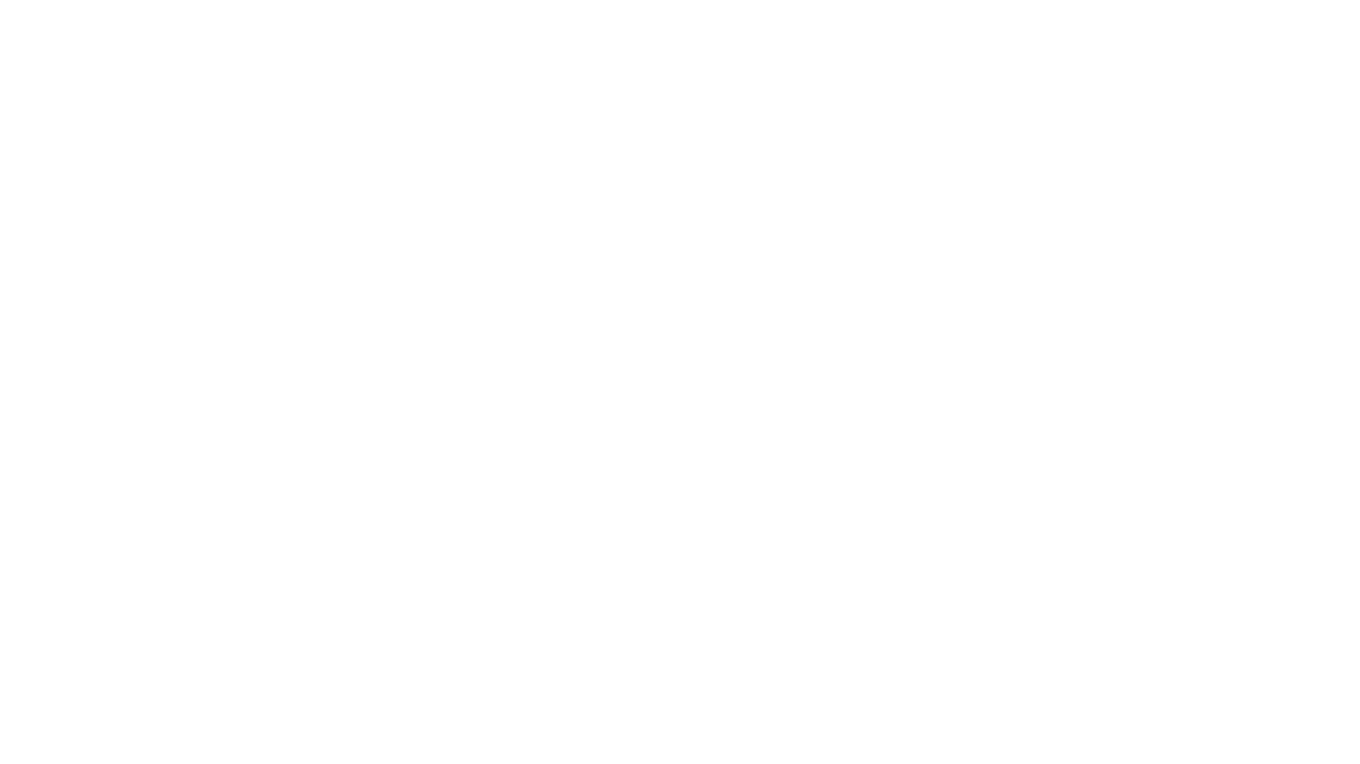
only_train_once
Only Train Once (OTO) is an automatic, architecture-agnostic DNN training and compression framework that allows users to train a general DNN from scratch or a pretrained checkpoint to achieve high performance and slimmer architecture simultaneously in a one-shot manner without fine-tuning. The framework includes features for automatic structured pruning and erasing operators, as well as hybrid structured sparse optimizers for efficient model compression. OTO provides tools for pruning zero-invariant group partitioning, constructing pruned models, and visualizing pruning and erasing dependency graphs. It supports the HESSO optimizer and offers a sanity check for compliance testing on various DNNs. The repository also includes publications, installation instructions, quick start guides, and a roadmap for future enhancements and collaborations.
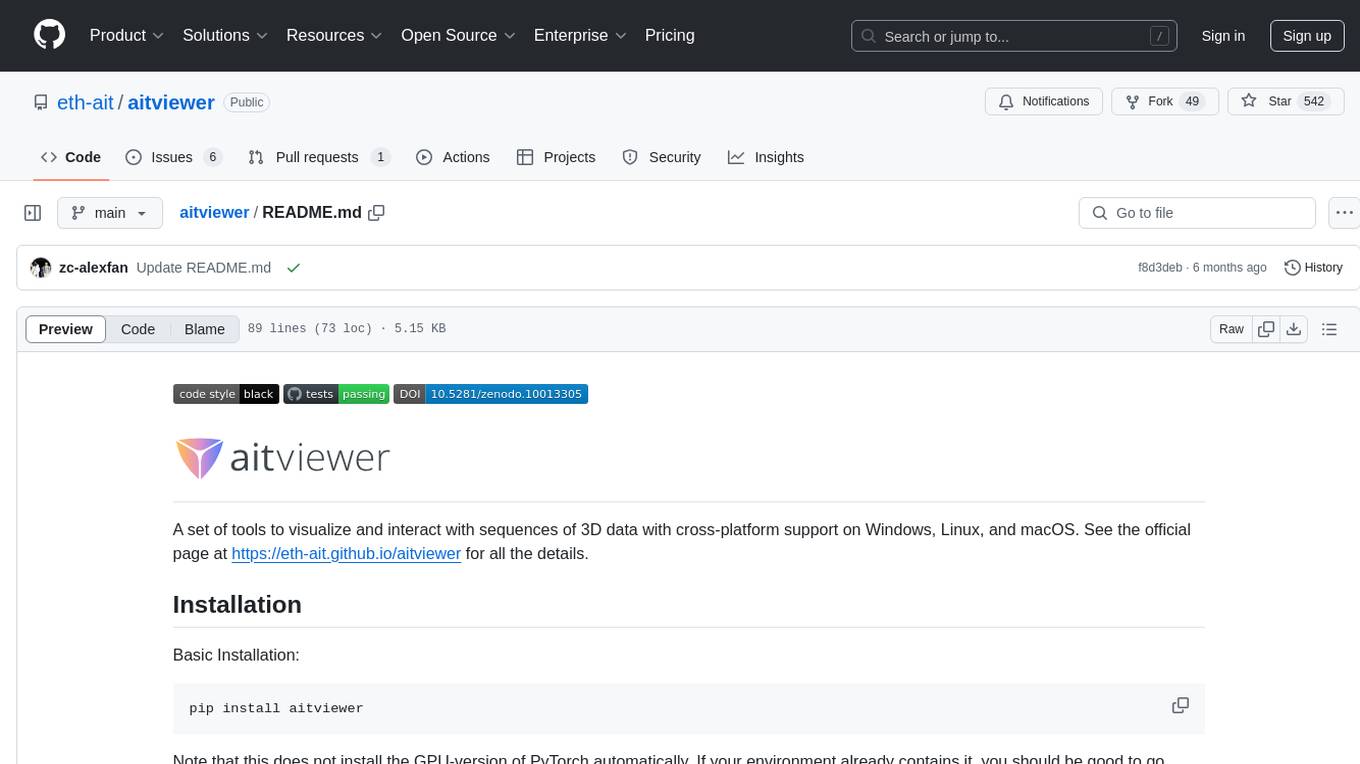
aitviewer
A set of tools to visualize and interact with sequences of 3D data with cross-platform support on Windows, Linux, and macOS. It provides a native Python interface for loading and displaying SMPL[-H/-X], MANO, FLAME, STAR, and SUPR sequences in an interactive viewer. Users can render 3D data on top of images, edit SMPL sequences and poses, export screenshots and videos, and utilize a high-performance ModernGL-based rendering pipeline. The tool is designed for easy use and hacking, with features like headless mode, remote mode, animatable camera paths, and a built-in extensible GUI.
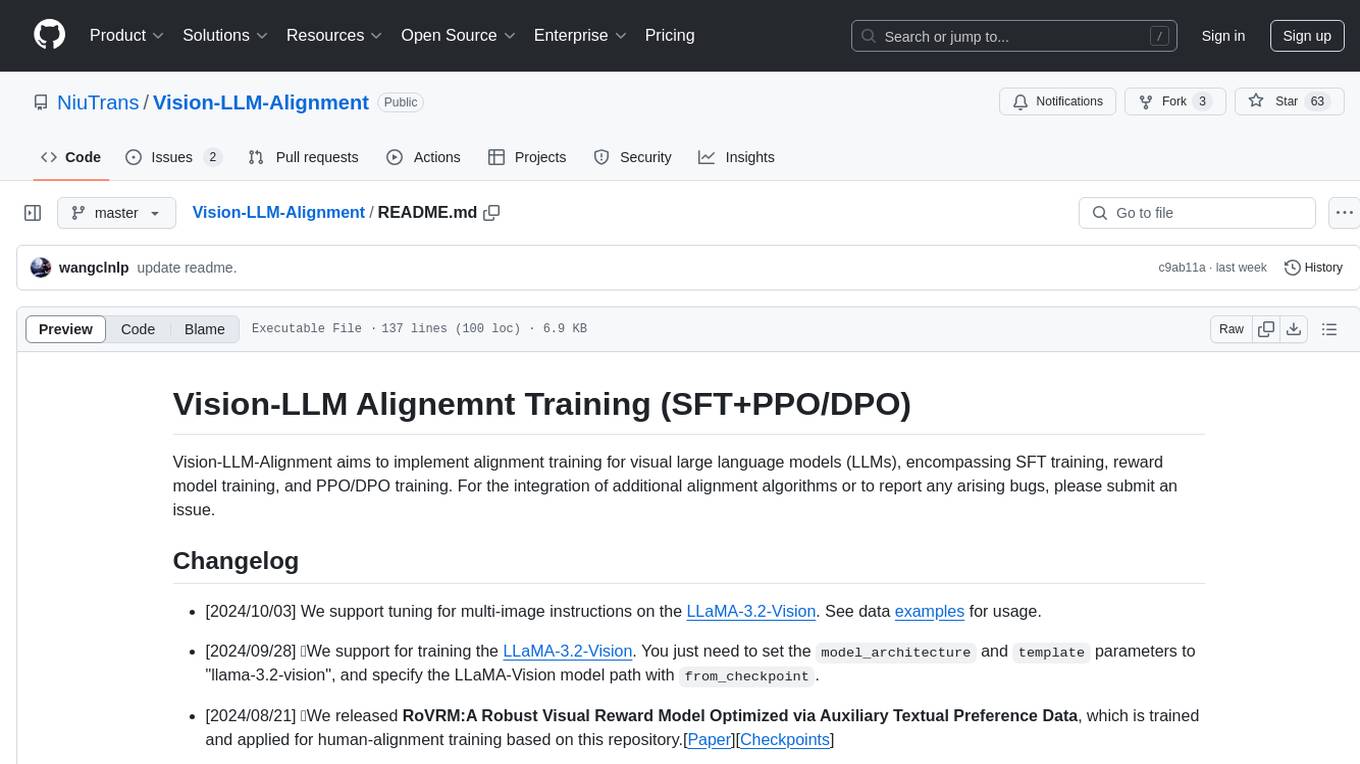
Vision-LLM-Alignment
Vision-LLM-Alignment is a repository focused on implementing alignment training for visual large language models (LLMs), including SFT training, reward model training, and PPO/DPO training. It supports various model architectures and provides datasets for training. The repository also offers benchmark results and installation instructions for users.
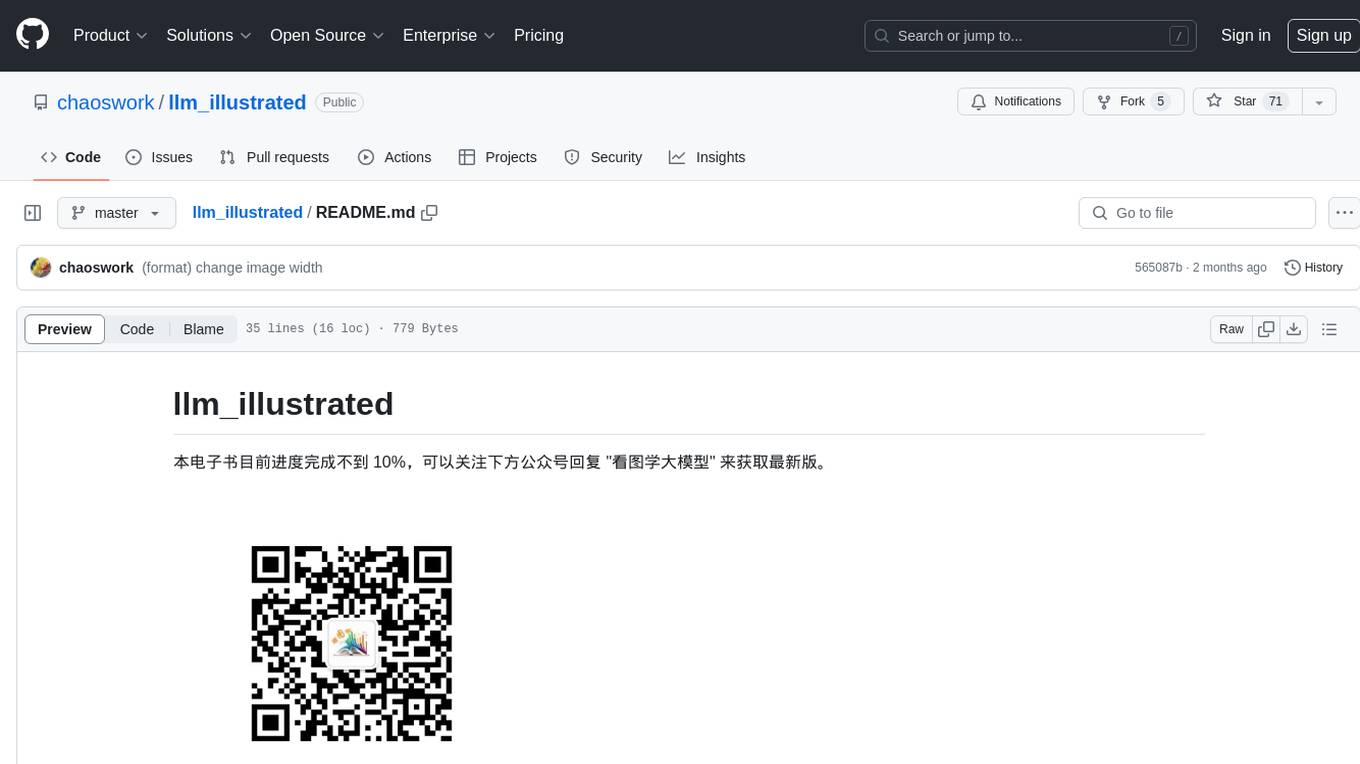
llm_illustrated
llm_illustrated is an electronic book that visually explains various technical aspects of large language models using clear and easy-to-understand images. The book covers topics such as self-attention structure and code, absolute position encoding, KV cache visualization, transformers composition, and a relationship graph of participants in the Dartmouth Conference. The progress of the book is less than 10%, and readers can stay updated by following the WeChat official account and replying 'learn large models through images'. The PDF layout and Latex formatting are still being adjusted.
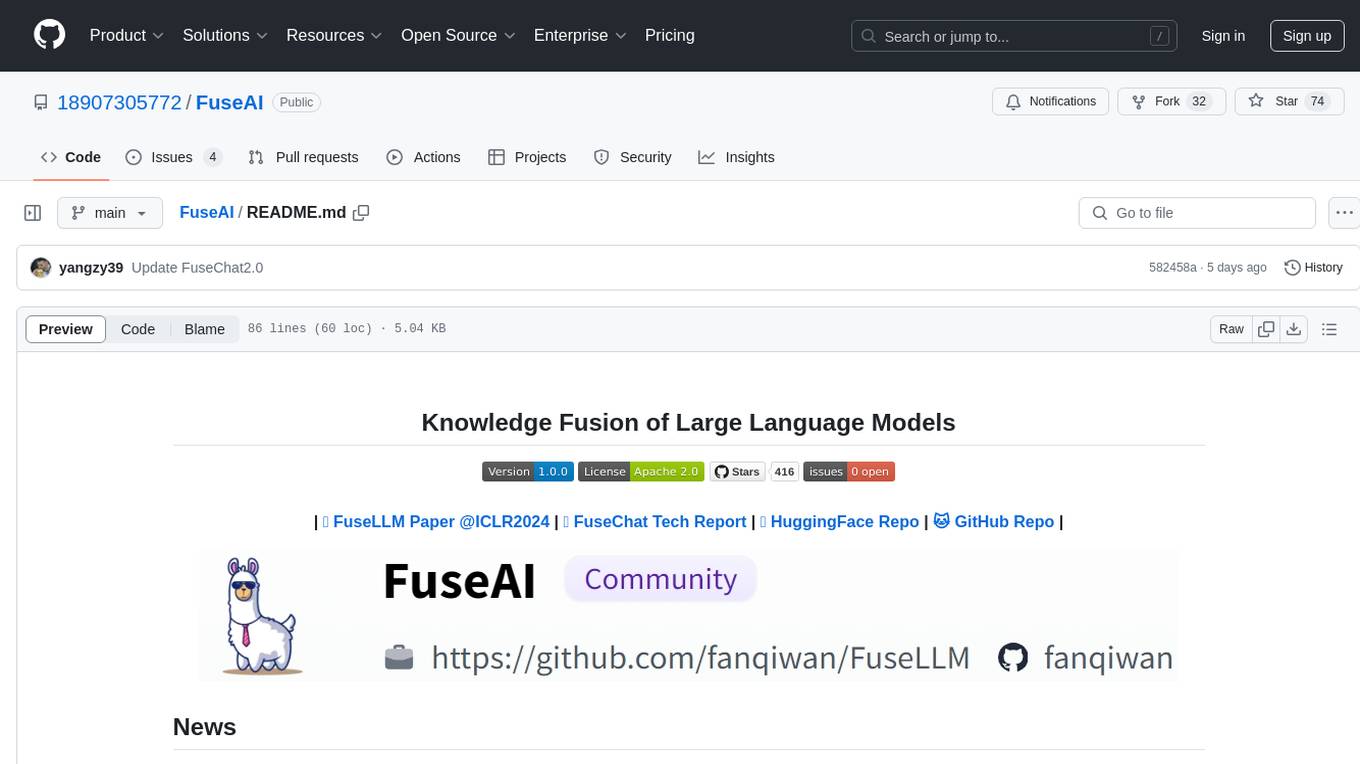
FuseAI
FuseAI is a repository that focuses on knowledge fusion of large language models. It includes FuseChat, a state-of-the-art 7B LLM on MT-Bench, and FuseLLM, which surpasses Llama-2-7B by fusing three open-source foundation LLMs. The repository provides tech reports, releases, and datasets for FuseChat and FuseLLM, showcasing their performance and advancements in the field of chat models and large language models.
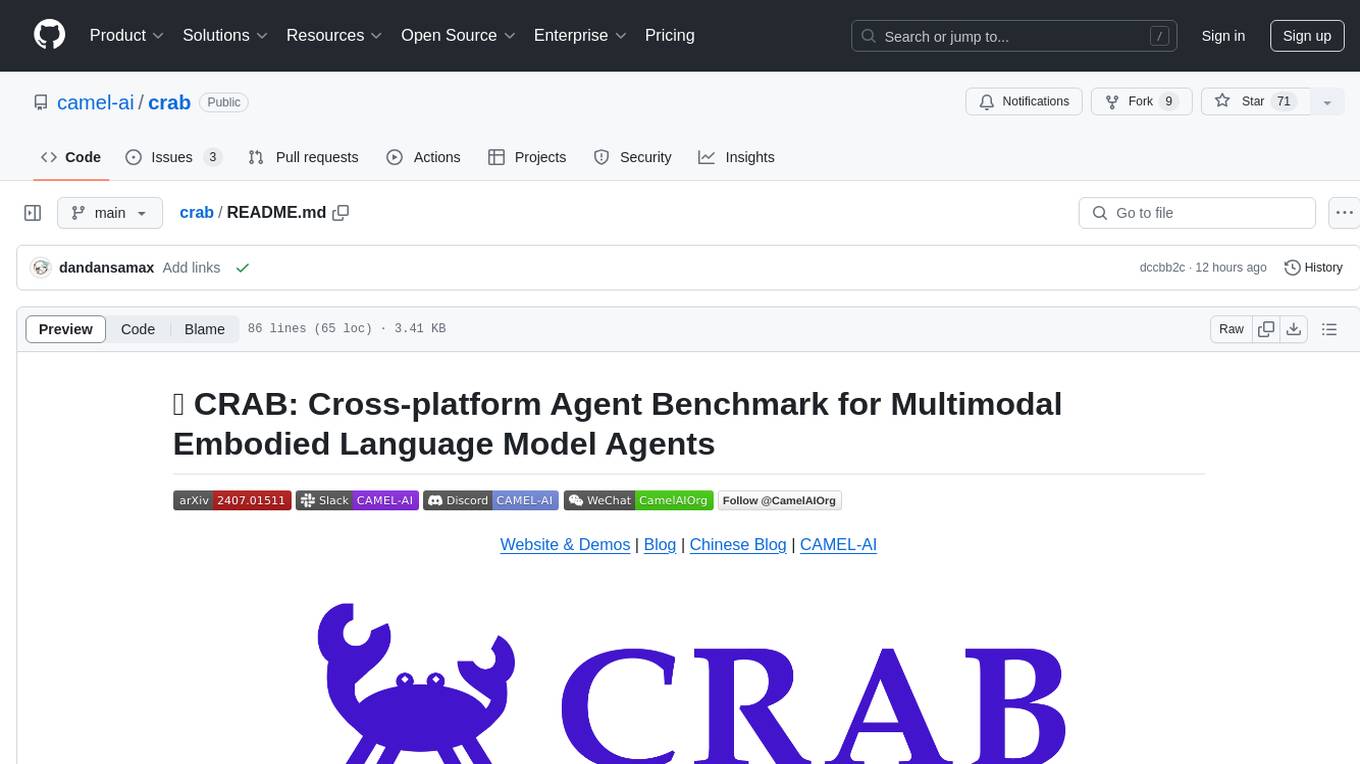
crab
CRAB is a framework for building LLM agent benchmark environments in a Python-centric way. It is cross-platform and multi-environment, allowing the creation of agent environments supporting various deployment options. The framework offers easy-to-use configuration with the ability to add new actions and define environments seamlessly. CRAB also provides a novel benchmarking suite with tasks and evaluators defined in Python, along with a unique graph evaluator method for detailed metrics.
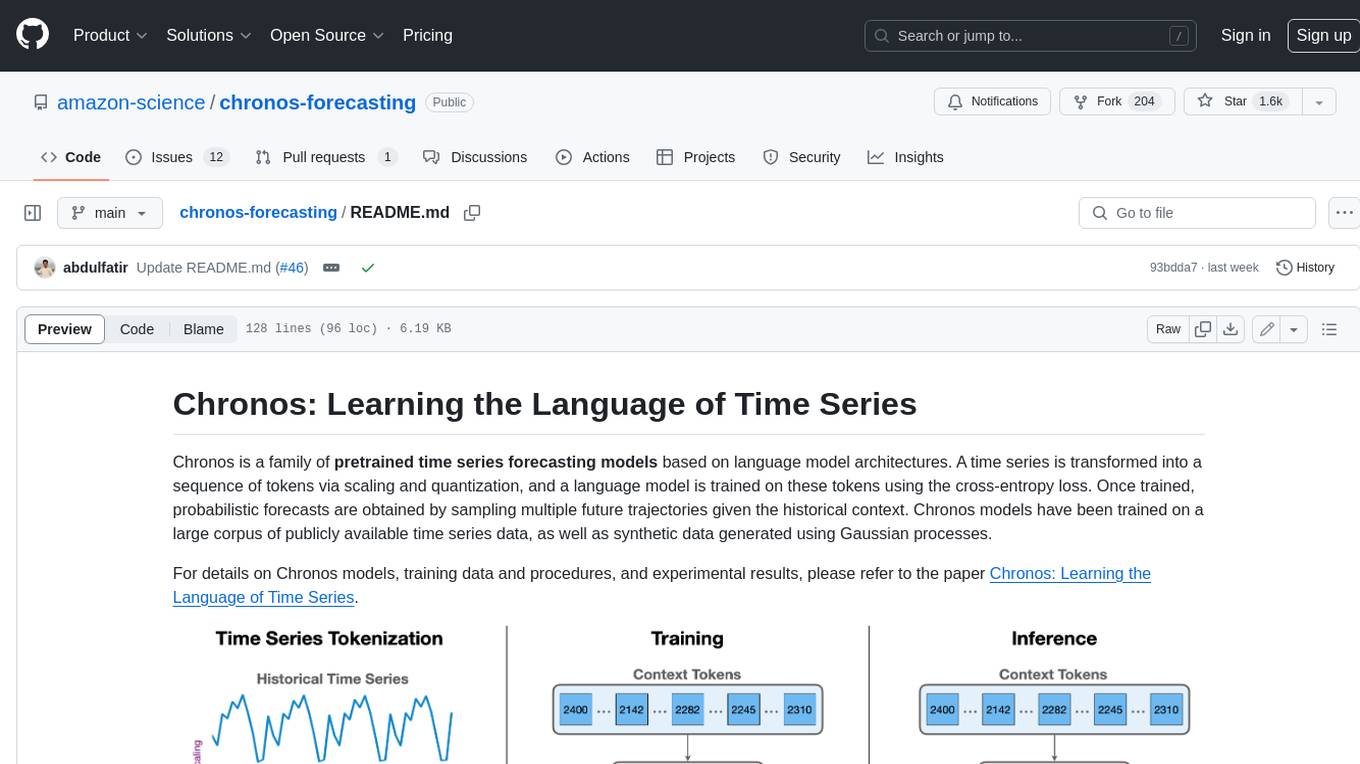
chronos-forecasting
Chronos is a family of pretrained time series forecasting models based on language model architectures. A time series is transformed into a sequence of tokens via scaling and quantization, and a language model is trained on these tokens using the cross-entropy loss. Once trained, probabilistic forecasts are obtained by sampling multiple future trajectories given the historical context. Chronos models have been trained on a large corpus of publicly available time series data, as well as synthetic data generated using Gaussian processes.
For similar tasks
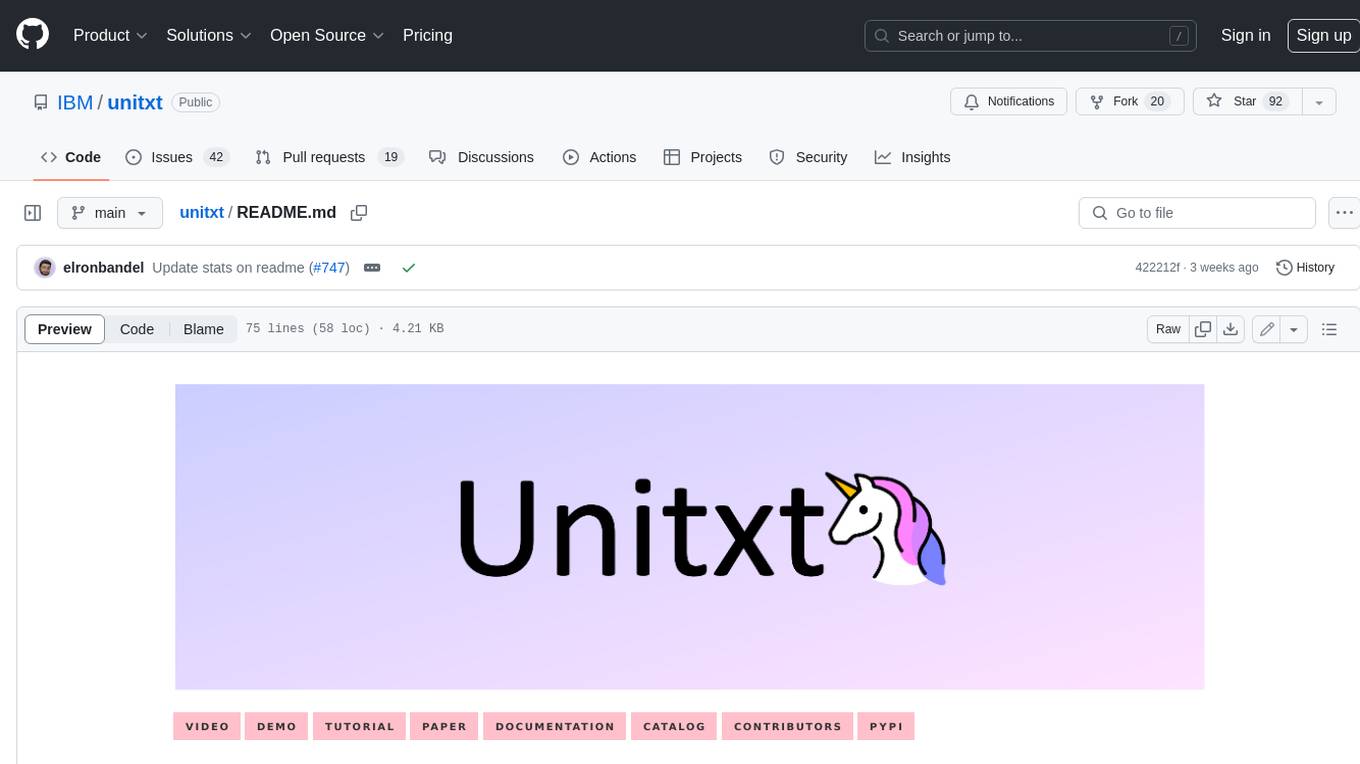
unitxt
Unitxt is a customizable library for textual data preparation and evaluation tailored to generative language models. It natively integrates with common libraries like HuggingFace and LM-eval-harness and deconstructs processing flows into modular components, enabling easy customization and sharing between practitioners. These components encompass model-specific formats, task prompts, and many other comprehensive dataset processing definitions. The Unitxt-Catalog centralizes these components, fostering collaboration and exploration in modern textual data workflows. Beyond being a tool, Unitxt is a community-driven platform, empowering users to build, share, and advance their pipelines collaboratively.
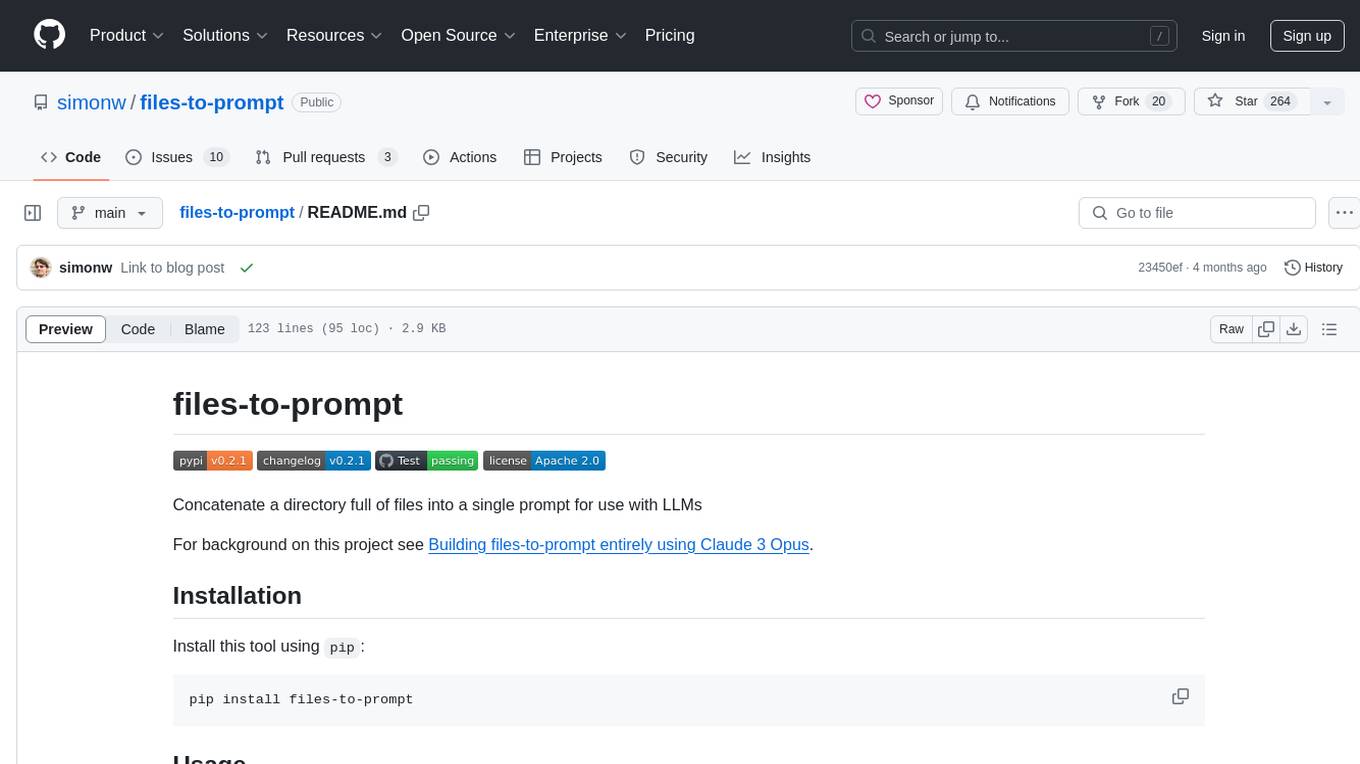
files-to-prompt
files-to-prompt is a tool that concatenates a directory full of files into a single prompt for use with Language Models (LLMs). It allows users to provide the path to one or more files or directories for processing, outputting the contents of each file with relative paths and separators. The tool offers options to include hidden files, ignore specific patterns, and exclude files specified in .gitignore. It is designed to streamline the process of preparing text data for LLMs by simplifying file concatenation and customization.
For similar jobs
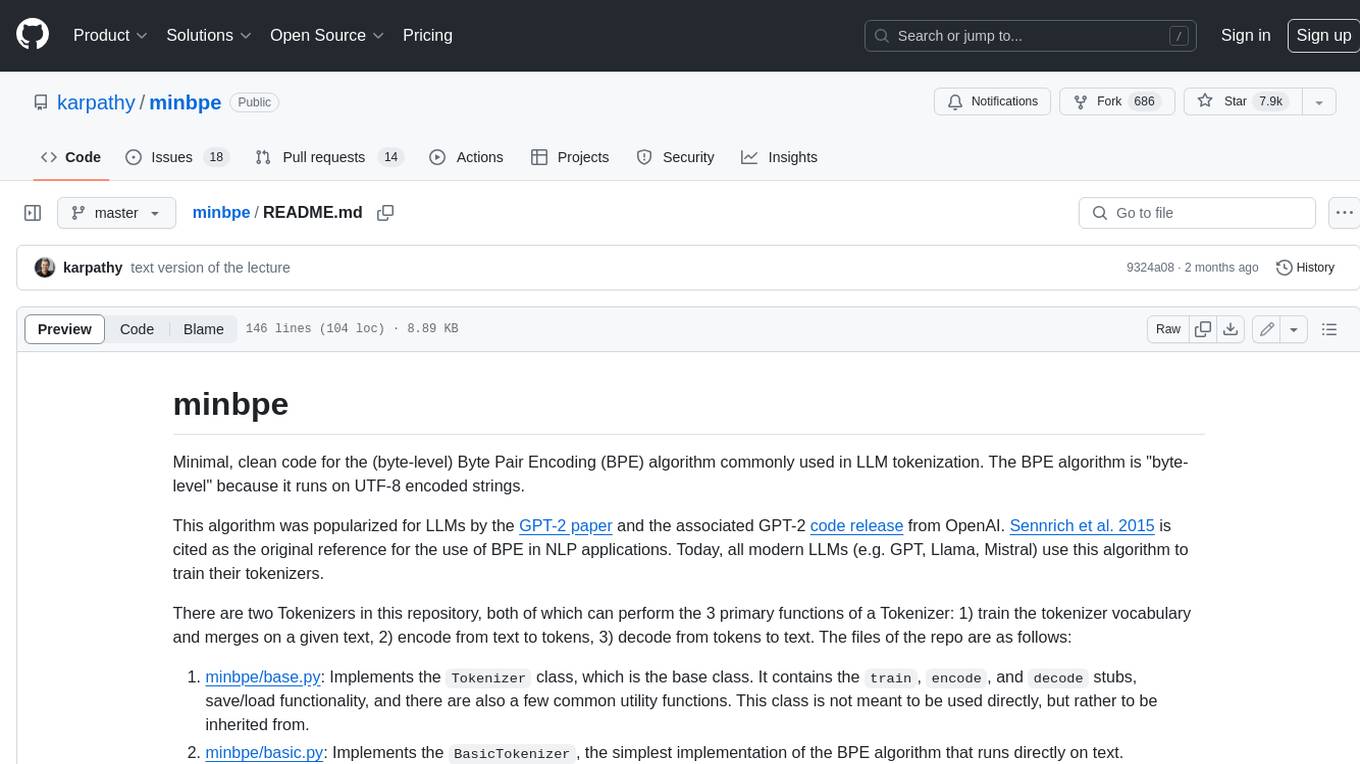
minbpe
This repository contains a minimal, clean code implementation of the Byte Pair Encoding (BPE) algorithm, commonly used in LLM tokenization. The BPE algorithm is "byte-level" because it runs on UTF-8 encoded strings. This algorithm was popularized for LLMs by the GPT-2 paper and the associated GPT-2 code release from OpenAI. Sennrich et al. 2015 is cited as the original reference for the use of BPE in NLP applications. Today, all modern LLMs (e.g. GPT, Llama, Mistral) use this algorithm to train their tokenizers. There are two Tokenizers in this repository, both of which can perform the 3 primary functions of a Tokenizer: 1) train the tokenizer vocabulary and merges on a given text, 2) encode from text to tokens, 3) decode from tokens to text. The files of the repo are as follows: 1. minbpe/base.py: Implements the `Tokenizer` class, which is the base class. It contains the `train`, `encode`, and `decode` stubs, save/load functionality, and there are also a few common utility functions. This class is not meant to be used directly, but rather to be inherited from. 2. minbpe/basic.py: Implements the `BasicTokenizer`, the simplest implementation of the BPE algorithm that runs directly on text. 3. minbpe/regex.py: Implements the `RegexTokenizer` that further splits the input text by a regex pattern, which is a preprocessing stage that splits up the input text by categories (think: letters, numbers, punctuation) before tokenization. This ensures that no merges will happen across category boundaries. This was introduced in the GPT-2 paper and continues to be in use as of GPT-4. This class also handles special tokens, if any. 4. minbpe/gpt4.py: Implements the `GPT4Tokenizer`. This class is a light wrapper around the `RegexTokenizer` (2, above) that exactly reproduces the tokenization of GPT-4 in the tiktoken library. The wrapping handles some details around recovering the exact merges in the tokenizer, and the handling of some unfortunate (and likely historical?) 1-byte token permutations. Finally, the script train.py trains the two major tokenizers on the input text tests/taylorswift.txt (this is the Wikipedia entry for her kek) and saves the vocab to disk for visualization. This script runs in about 25 seconds on my (M1) MacBook. All of the files above are very short and thoroughly commented, and also contain a usage example on the bottom of the file.
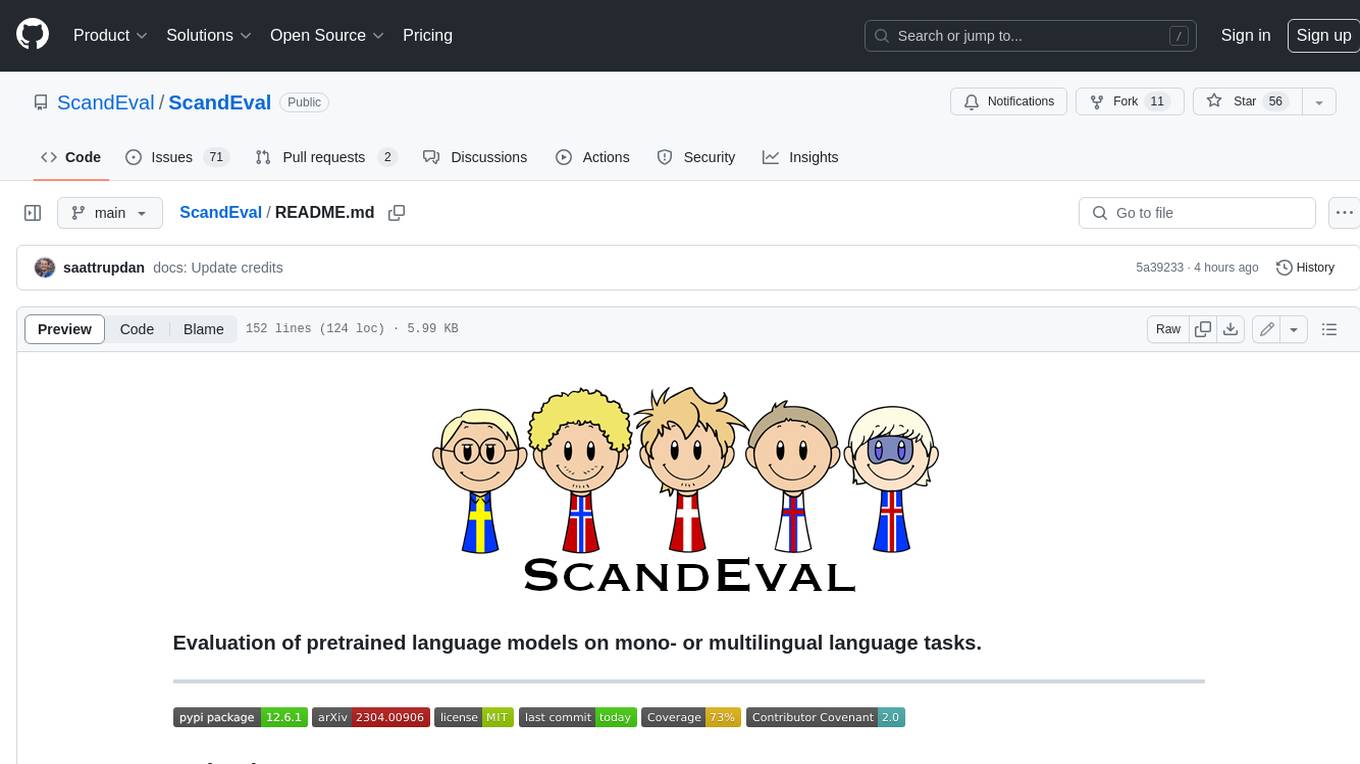
ScandEval
ScandEval is a framework for evaluating pretrained language models on mono- or multilingual language tasks. It provides a unified interface for benchmarking models on a variety of tasks, including sentiment analysis, question answering, and machine translation. ScandEval is designed to be easy to use and extensible, making it a valuable tool for researchers and practitioners alike.
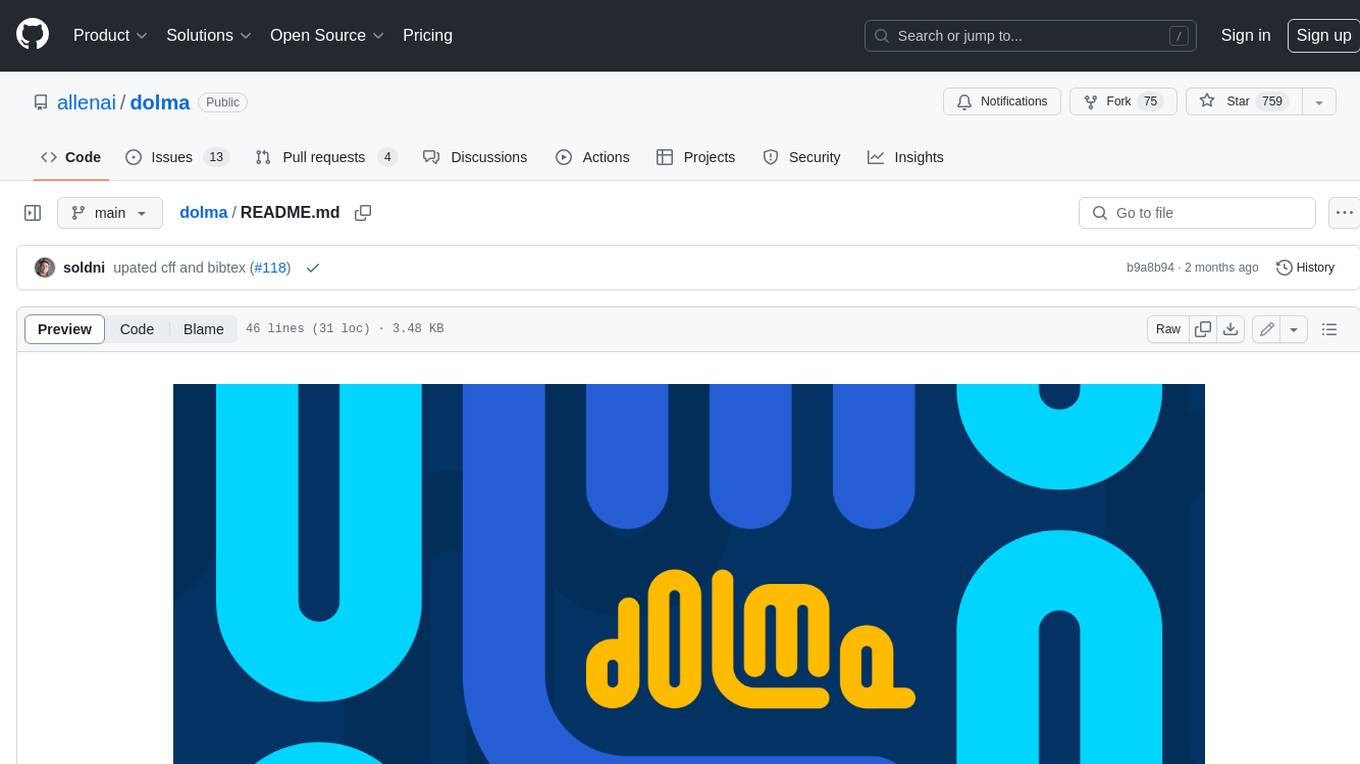
dolma
Dolma is a dataset and toolkit for curating large datasets for (pre)-training ML models. The dataset consists of 3 trillion tokens from a diverse mix of web content, academic publications, code, books, and encyclopedic materials. The toolkit provides high-performance, portable, and extensible tools for processing, tagging, and deduplicating documents. Key features of the toolkit include built-in taggers, fast deduplication, and cloud support.
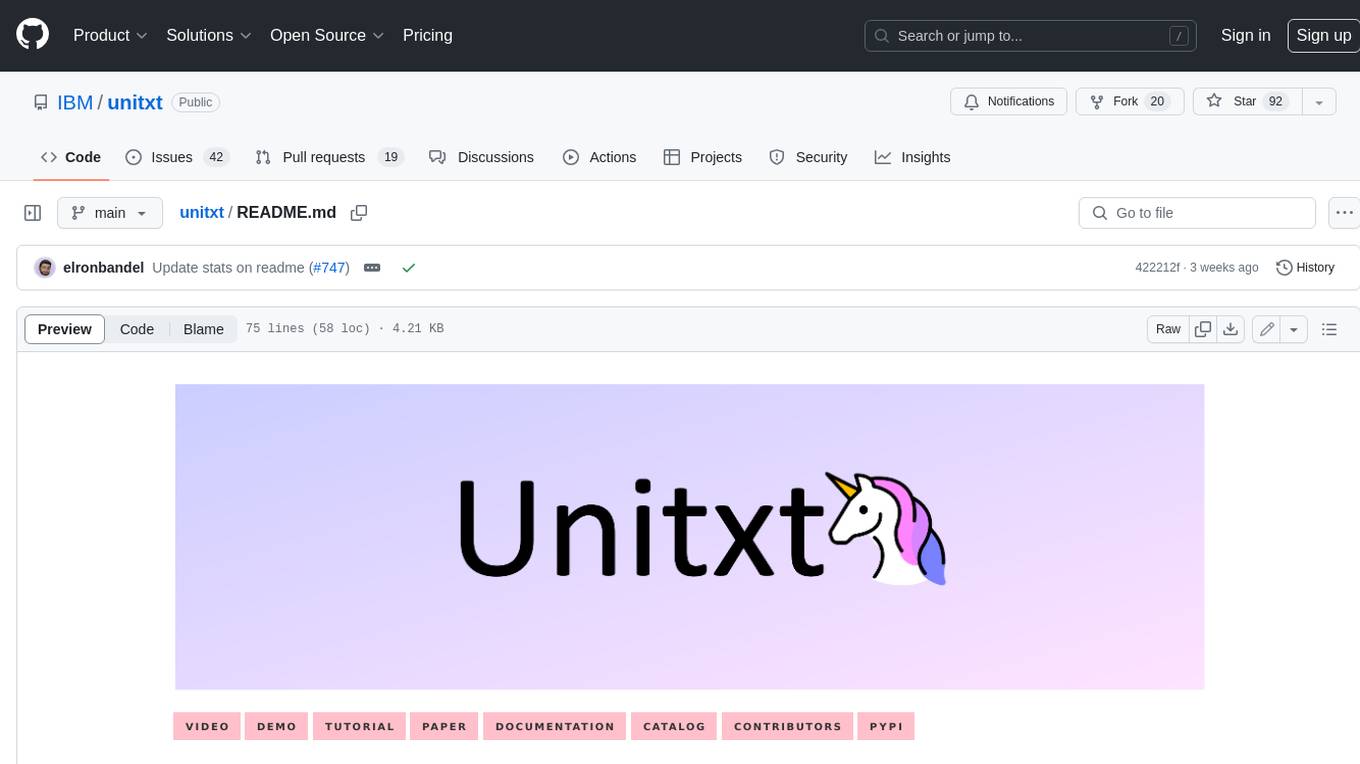
unitxt
Unitxt is a customizable library for textual data preparation and evaluation tailored to generative language models. It natively integrates with common libraries like HuggingFace and LM-eval-harness and deconstructs processing flows into modular components, enabling easy customization and sharing between practitioners. These components encompass model-specific formats, task prompts, and many other comprehensive dataset processing definitions. The Unitxt-Catalog centralizes these components, fostering collaboration and exploration in modern textual data workflows. Beyond being a tool, Unitxt is a community-driven platform, empowering users to build, share, and advance their pipelines collaboratively.
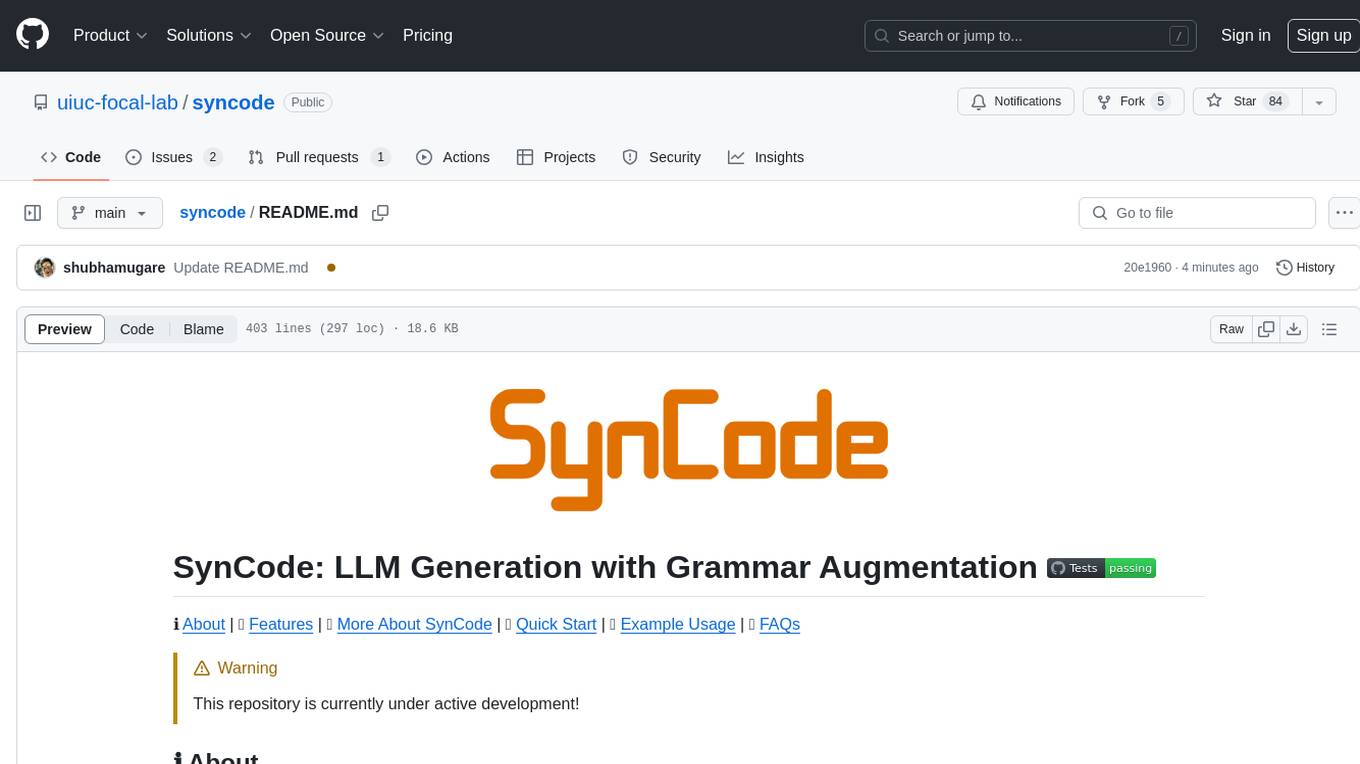
syncode
SynCode is a novel framework for the grammar-guided generation of Large Language Models (LLMs) that ensures syntactically valid output with respect to defined Context-Free Grammar (CFG) rules. It supports general-purpose programming languages like Python, Go, SQL, JSON, and more, allowing users to define custom grammars using EBNF syntax. The tool compares favorably to other constrained decoders and offers features like fast grammar-guided generation, compatibility with HuggingFace Language Models, and the ability to work with various decoding strategies.
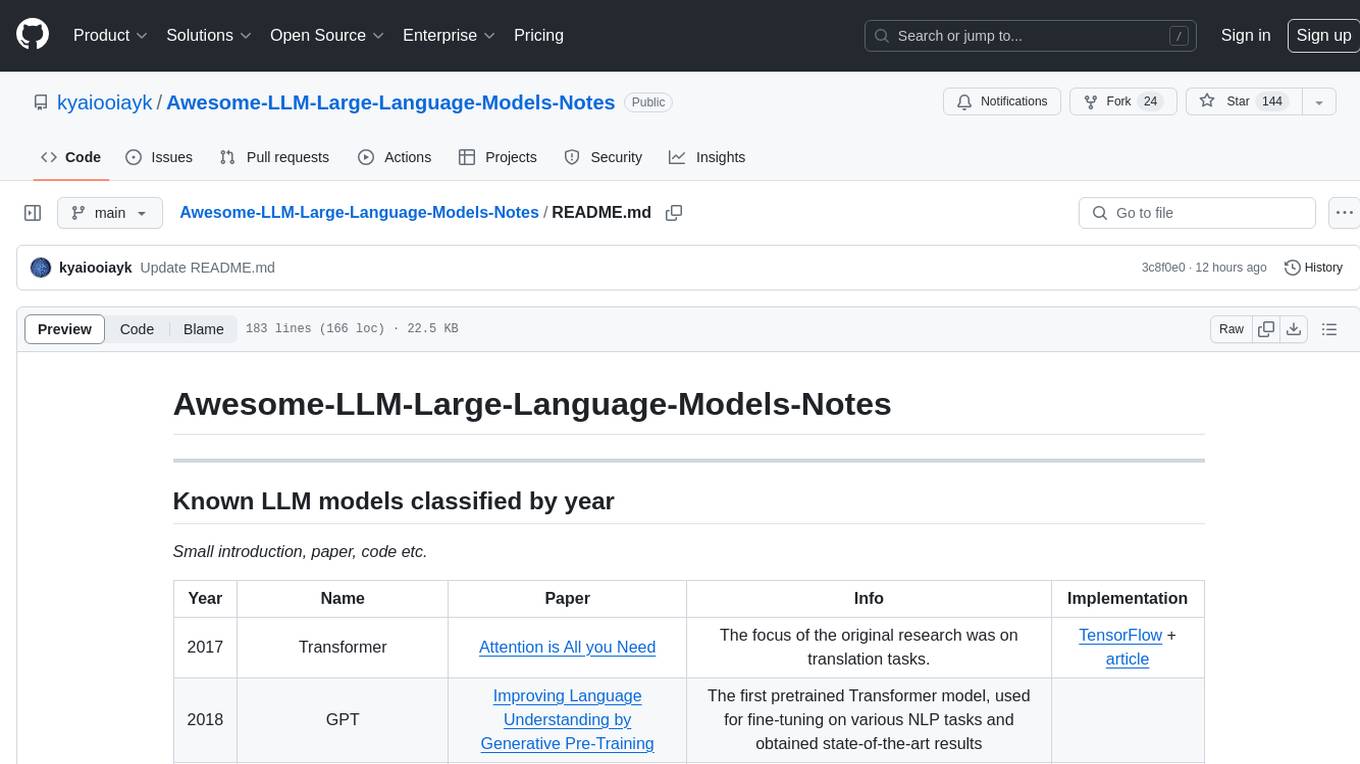
Awesome-LLM-Large-Language-Models-Notes
Awesome-LLM-Large-Language-Models-Notes is a repository that provides a comprehensive collection of information on various Large Language Models (LLMs) classified by year, size, and name. It includes details on known LLM models, their papers, implementations, and specific characteristics. The repository also covers LLM models classified by architecture, must-read papers, blog articles, tutorials, and implementations from scratch. It serves as a valuable resource for individuals interested in understanding and working with LLMs in the field of Natural Language Processing (NLP).
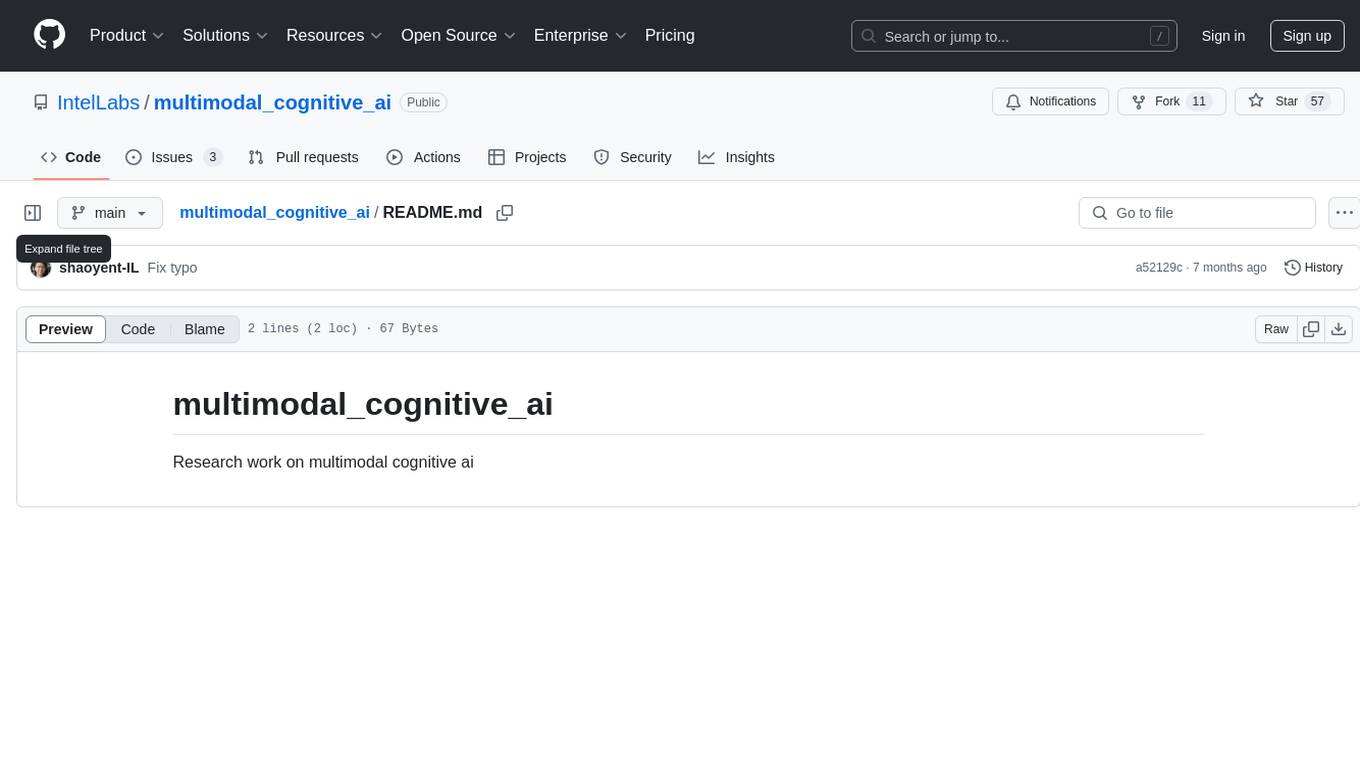
multimodal_cognitive_ai
The multimodal cognitive AI repository focuses on research work related to multimodal cognitive artificial intelligence. It explores the integration of multiple modes of data such as text, images, and audio to enhance AI systems' cognitive capabilities. The repository likely contains code, datasets, and research papers related to multimodal AI applications, including natural language processing, computer vision, and audio processing. Researchers and developers interested in advancing AI systems' understanding of multimodal data can find valuable resources and insights in this repository.
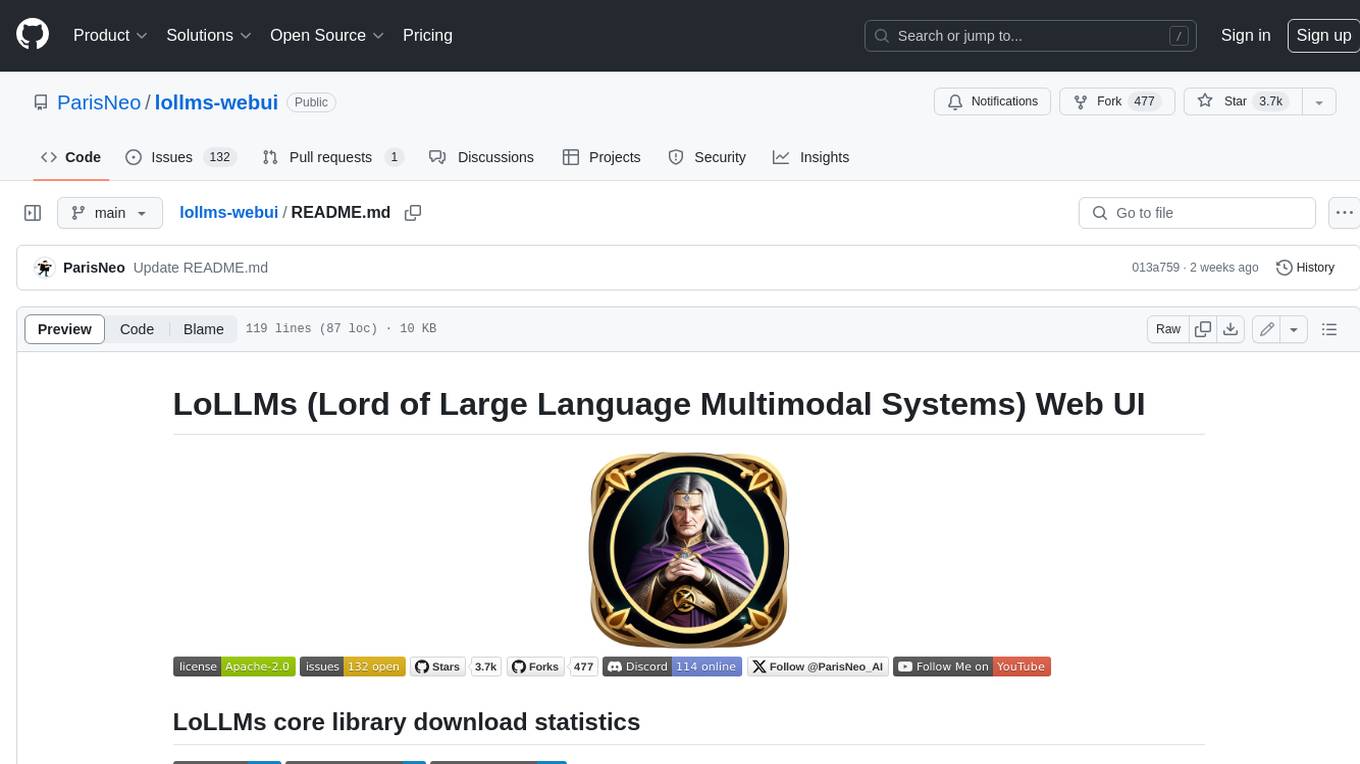
lollms-webui
LoLLMs WebUI (Lord of Large Language Multimodal Systems: One tool to rule them all) is a user-friendly interface to access and utilize various LLM (Large Language Models) and other AI models for a wide range of tasks. With over 500 AI expert conditionings across diverse domains and more than 2500 fine tuned models over multiple domains, LoLLMs WebUI provides an immediate resource for any problem, from car repair to coding assistance, legal matters, medical diagnosis, entertainment, and more. The easy-to-use UI with light and dark mode options, integration with GitHub repository, support for different personalities, and features like thumb up/down rating, copy, edit, and remove messages, local database storage, search, export, and delete multiple discussions, make LoLLMs WebUI a powerful and versatile tool.