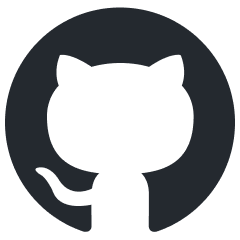
fractl
Generative AI-powered Programming Language
Stars: 117
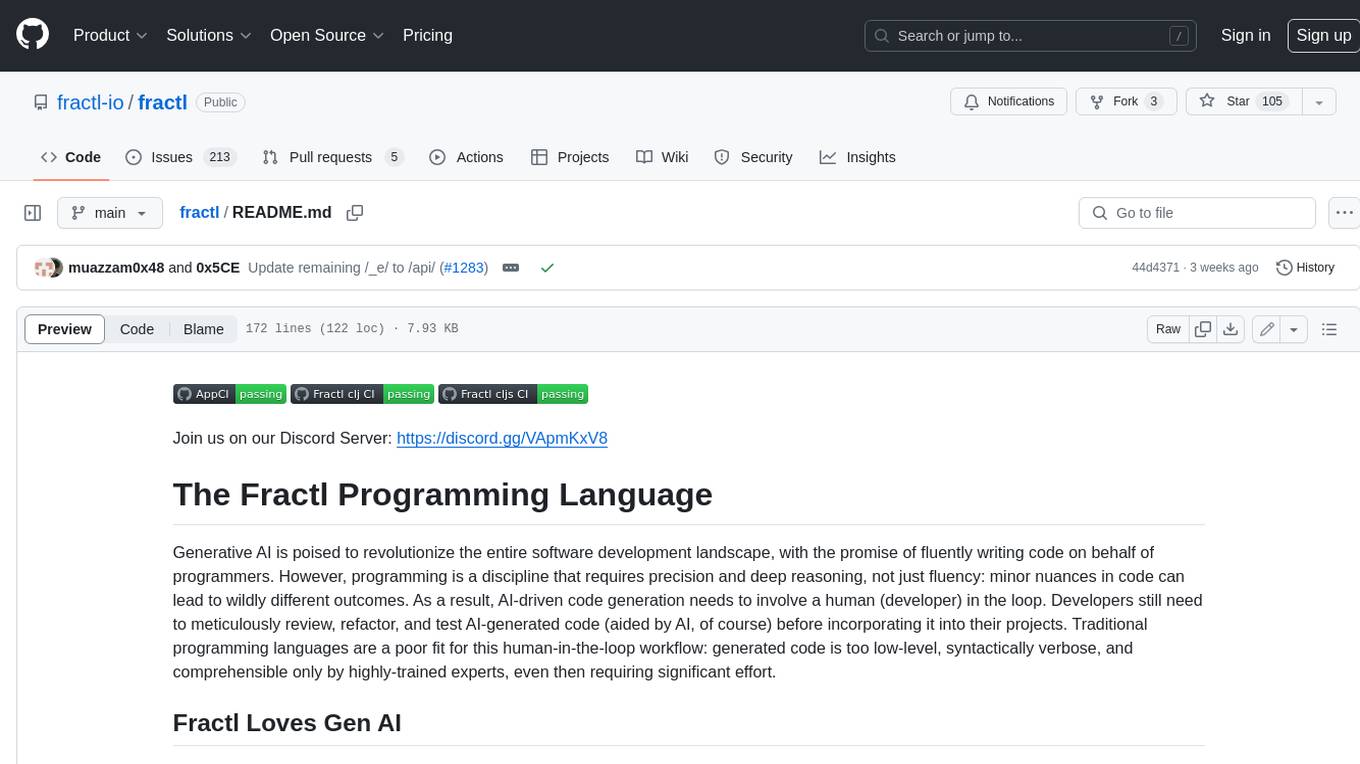
Fractl is a programming language designed for generative AI, making it easier for developers to work with AI-generated code. It features a data-oriented and declarative syntax, making it a better fit for generative AI-powered code generation. Fractl also bridges the gap between traditional programming and visual building, allowing developers to use multiple ways of building, including traditional coding, visual development, and code generation with generative AI. Key concepts in Fractl include a graph-based hierarchical data model, zero-trust programming, declarative dataflow, resolvers, interceptors, and entity-graph-database mapping.
README:
Join us on our Discord Server: https://discord.gg/VApmKxV8
Generative AI is poised to revolutionize the entire software development landscape, with the promise of fluently writing code on behalf of programmers. However, programming is a discipline that requires precision and deep reasoning, not just fluency: minor nuances in code can lead to wildly different outcomes. As a result, AI-driven code generation needs to involve a human (developer) in the loop. Developers still need to meticulously review, refactor, and test AI-generated code (aided by AI, of course) before incorporating it into their projects. Traditional programming languages are a poor fit for this human-in-the-loop workflow: generated code is too low-level, syntactically verbose, and comprehensible only by highly-trained experts, even then requiring significant effort.
As a language, Fractl is data-oriented and declarative, with an abstraction that is closer to natural languages than traditional programming languages. This makes fractl a much better fit for Gen AI-powered code generation. Users can rapidly build business application in Fractl from high-level specifications - typically more than 10x faster than traditional programming languages.
With the rising popularity of Low-code/No-code tools, a growing chasm has emerged between traditional programming and visual building. Traditional programming by professional developers offers immense power and flexiblity, but tends to be slow, expensive and constrained by the availability of skilled developers. In contrast, low-code/no-code tools provide speed and simplicity, but are often limited to simple use cases, lead to strong vendor lock-ins, and, as a result, have been strongly rejected by professional developers.
Fractl, with its high-level of abstraction and data-oriented syntax, bridges this chasm by being a single abstraction for both traditional programming and visual building. Code blocks in the Fractl programming language can be directly represented as visual elements in Fractl Design Studio, without any translation, and vice versa. This unique characteristic of Fractl makes it possible for developers to concurrently use multiple ways of building:
- Traditional coding in IDEs,
- Visual development in a no-code builder, and,
- Code generation with generative-AI.
The Fractl language specification, its compiler and runtime are open source.
The code you build in Fractl can be run anywhere using the open source compiler and runtime, thereby avoiding the vendor lock-in of other low-code/no-code platforms.
Fractl introduces a number of innovative concepts to programming:
- Graph-based Hierarchical Data Model - compose the high-level data model of an application as a hierarchical graph of business entities with relationships. Such entities and relationships are first-class constructs in Fractl.
- Zero-trust Programming - tightly control operations on business entities through declarative access-control encoded directly in the model itself.
- Declarative Dataflow - express business logic as purely-declarative patterns of data.
- Resolvers - use a simple, but powerful mechanism to interface with external systems.
- Interceptors - extend the fractl runtime with custom capabilities.
- Entity-graph-Database Mapping - take advantage of an abstract persistence layer for fully-automated storage of entity instances.
The following code snippet shows the Fractl model (i.e., program) for a simple accounting application.
(component :Accounts.Core)
(entity :Company
{:Name {:type :String :guid true}
:rbac [{:roles ["manager"] :allow [:create]}]})
(entity :AccountHead
{:Name {:type :String :id true}
:rbac [{:roles ["accountant"] :allow [:create]}]})
(entity :Entry
{:No {:type :Int :id true}
:Type {:oneof ["income" "expense"]}
:Amount :Decimal
:Remarks {:type :String :optional true}
:DateCreated :Now})
(relationship :CompanyAccounts
{:meta {:contains [:Company :AccountHead]}})
(relationship :Transactions
{:meta {:contains [:AccountHead :Entry]}})
(record :BalanceReport
{:Balance :Decimal
:GeneratedOn :Now})
(defn- find-balance [entries]
(reduce (fn [b t]
(let [op (if (= "income" (:Type t)) + -)]
(op b (:Amount t))))
0 entries))
(event :GenerateReport
{:Since :DateTime
:Company :String
:AccountHead :String})
(dataflow :GenerateReport
{:AccountHead? {}
:-> [[:CompanyAccounts?
{:Company {:Name? :GenerateReport.Company}}
:GenerateReport.AccountHead]]
:as [:A]}
{:Entry
{:DateCreated? [:>= :GenerateReport.Since]}
:-> [[:Transactions? :A]]
:as :Es}
{:BalanceReport
{:Balance '(find-balance :Es)}})
Save this code to a file named accounts.fractl
and its ready to be run as a highly-scalable accounting service with RESTful APIs to perform CRUD operations and generate balance report!
But before you can actually run it, you need to install Fractl. The next section will help you with that.
- Java SE 21 or later
- Linux, Mac OSX or a Unix emulator in Windows
Download the Fractl CLI tool and execute the model:
./fractl /path/to/accounts.fractl
We can create a new company using an HTTP POST
request,
curl --header "Content-Type: application/json" \
--request POST \
--data '{"Accounts.Core/Company": {"Name": "acme"}}' \
http://localhost:8080/api/Accounts.Core/Company
To make sure the new company is persisted in the store, try the following HTTP GET
:
curl http://localhost:8080/api/Accounts.Core/Company/acme
If Fractl is installed correctly, both these requests will return an OK
status along with a :Company
instance.
Listed below are a few more HTTP requests that you can try with our "accounting" application:
- Create an account-head for the new company.
POST /api/Accounts.Core/Company/acme/CompanyAccounts/AccountHead
{"Accounts.Core/AccountHead": {"Name": "Department01"}}
- Make some transactions under the new account-head.
POST /api/Accounts.Core/Company/acme/CompanyAccounts/AccountHead/Department01/Transactions/Entry
{"Accounts.Core/Entry":
{"No": 1, "Type": "income",
"Amount": 2000.0, "Remarks": "Opening balance"}}
POST /api/Accounts.Core/Company/acme/CompanyAccounts/AccountHead/Department01/Transactions/Entry
{"Accounts.Core/Entry":
{"No": 2, "Type": "expense",
"Amount": 500.0, "Remarks": "Rent paid"}}
- Generate the balance-report for the account-head.
POST /api/Accounts.Core/GenerateReport
{"Accounts.Core/GenerateReport":
{"Since": "2023-11-09T00:00:00.00",
"Company": "acme",
"AccountHead": "Department01"}}
You're all set to further explore Fractl. Please proceed to the official documentation pages.
Copyright 2022 Fractl Inc.
Licensed under the Apache License, Version 2.0: http://www.apache.org/licenses/LICENSE-2.0
For Tasks:
Click tags to check more tools for each tasksFor Jobs:
Alternative AI tools for fractl
Similar Open Source Tools
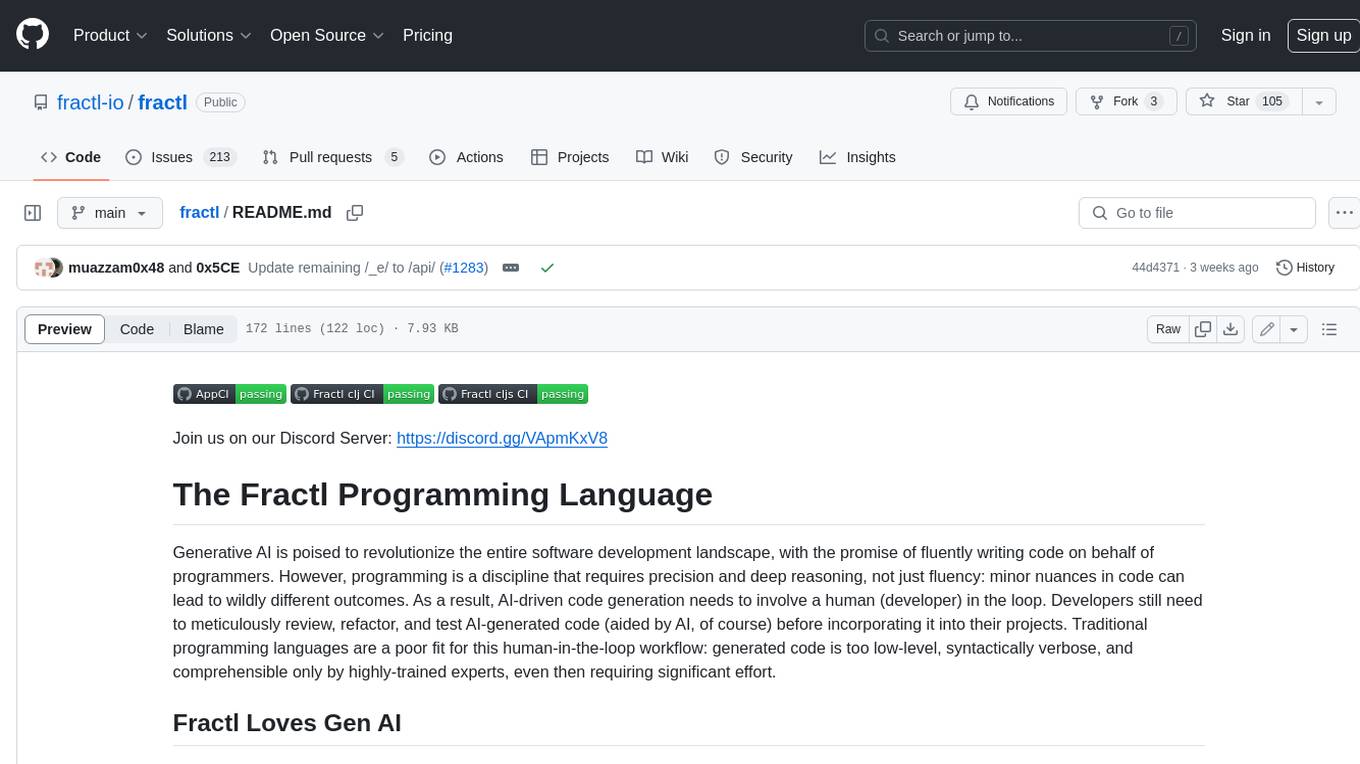
fractl
Fractl is a programming language designed for generative AI, making it easier for developers to work with AI-generated code. It features a data-oriented and declarative syntax, making it a better fit for generative AI-powered code generation. Fractl also bridges the gap between traditional programming and visual building, allowing developers to use multiple ways of building, including traditional coding, visual development, and code generation with generative AI. Key concepts in Fractl include a graph-based hierarchical data model, zero-trust programming, declarative dataflow, resolvers, interceptors, and entity-graph-database mapping.
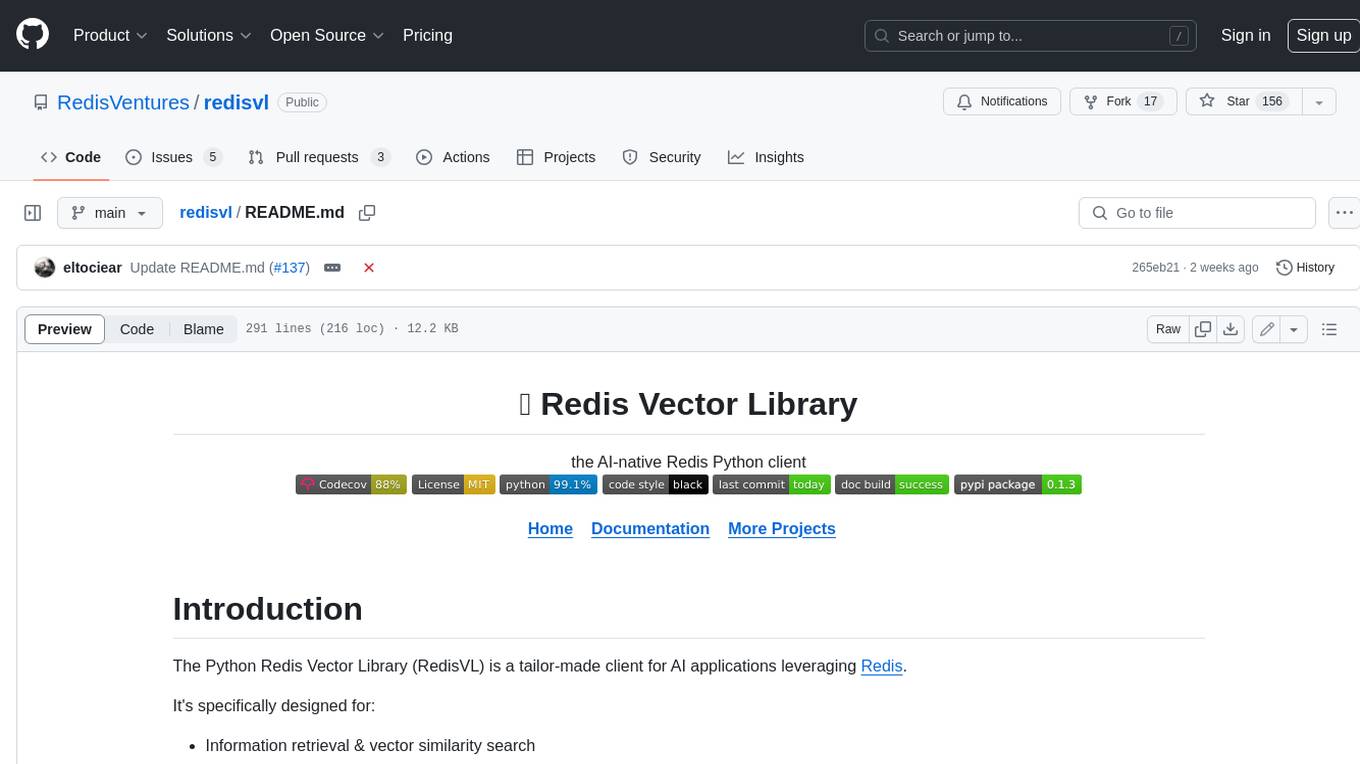
redisvl
Redis Vector Library (RedisVL) is a Python client library for building AI applications on top of Redis. It provides a high-level interface for managing vector indexes, performing vector search, and integrating with popular embedding models and providers. RedisVL is designed to make it easy for developers to build and deploy AI applications that leverage the speed, flexibility, and reliability of Redis.
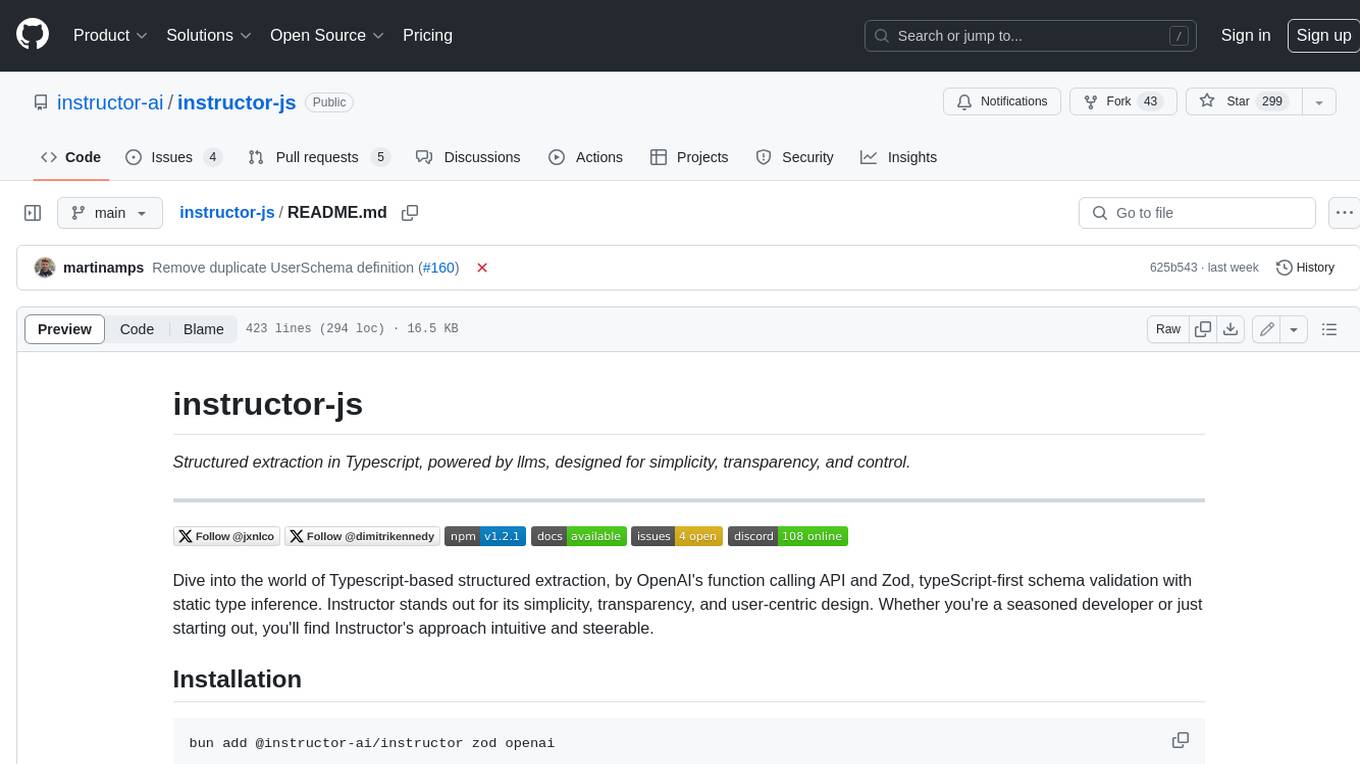
instructor-js
Instructor is a Typescript library for structured extraction in Typescript, powered by llms, designed for simplicity, transparency, and control. It stands out for its simplicity, transparency, and user-centric design. Whether you're a seasoned developer or just starting out, you'll find Instructor's approach intuitive and steerable.
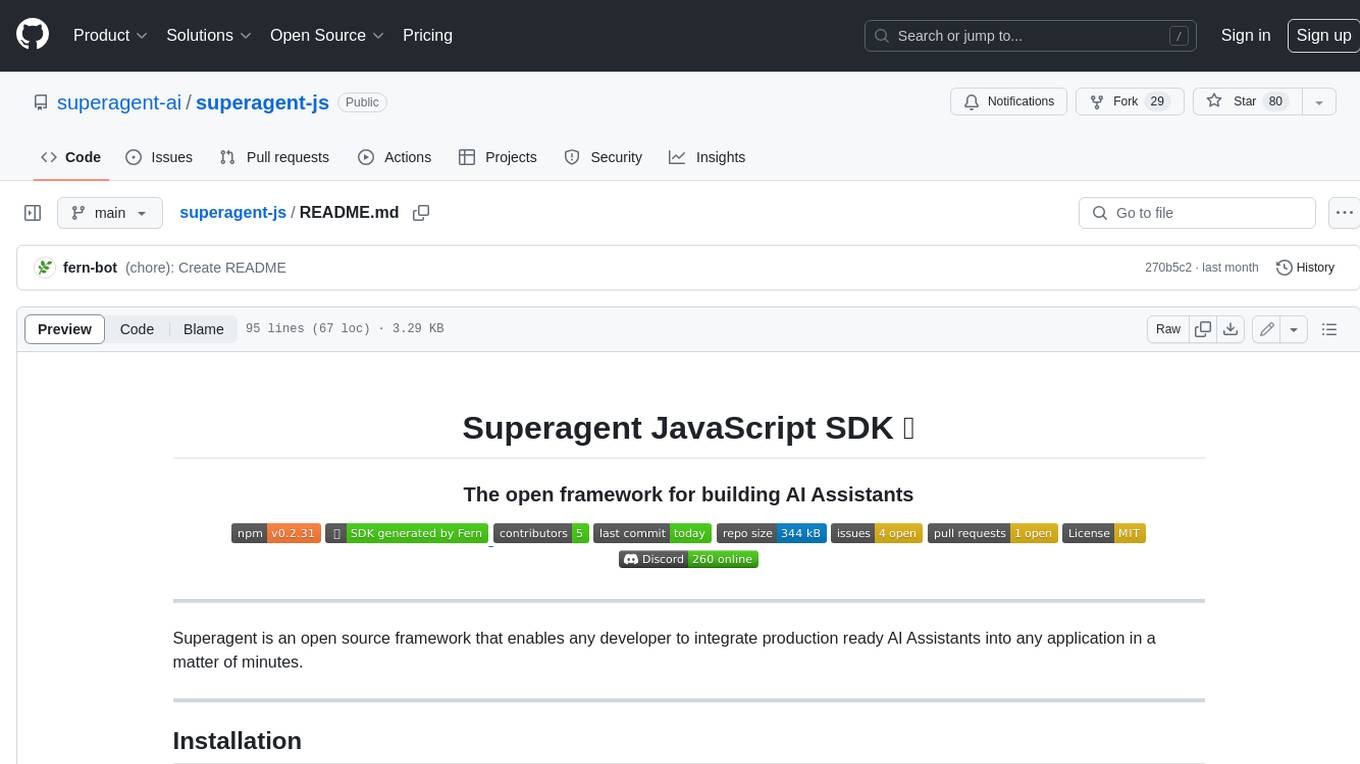
superagent-js
Superagent is an open source framework that enables any developer to integrate production ready AI Assistants into any application in a matter of minutes.
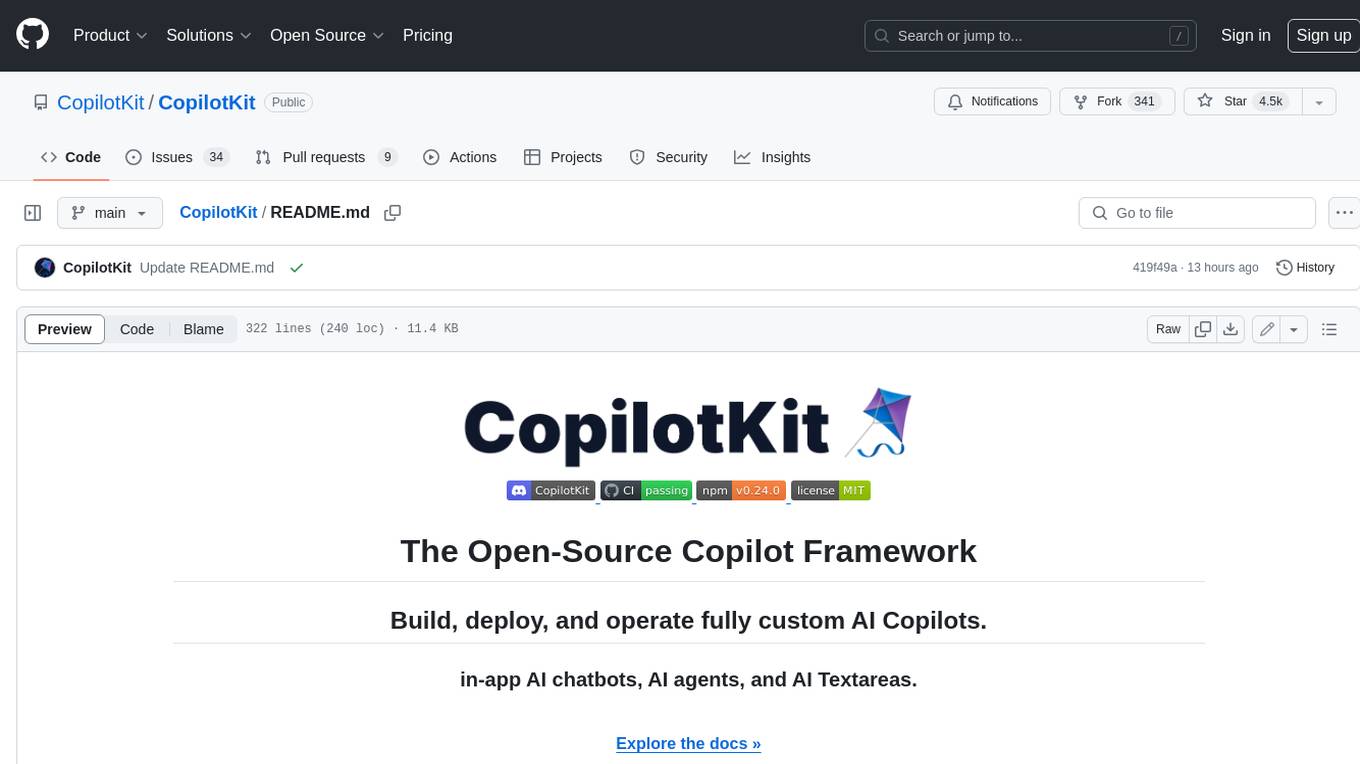
CopilotKit
CopilotKit is an open-source framework for building, deploying, and operating fully custom AI Copilots, including in-app AI chatbots, AI agents, and AI Textareas. It provides a set of components and entry points that allow developers to easily integrate AI capabilities into their applications. CopilotKit is designed to be flexible and extensible, so developers can tailor it to their specific needs. It supports a variety of use cases, including providing app-aware AI chatbots that can interact with the application state and take action, drop-in replacements for textareas with AI-assisted text generation, and in-app agents that can access real-time application context and take action within the application.
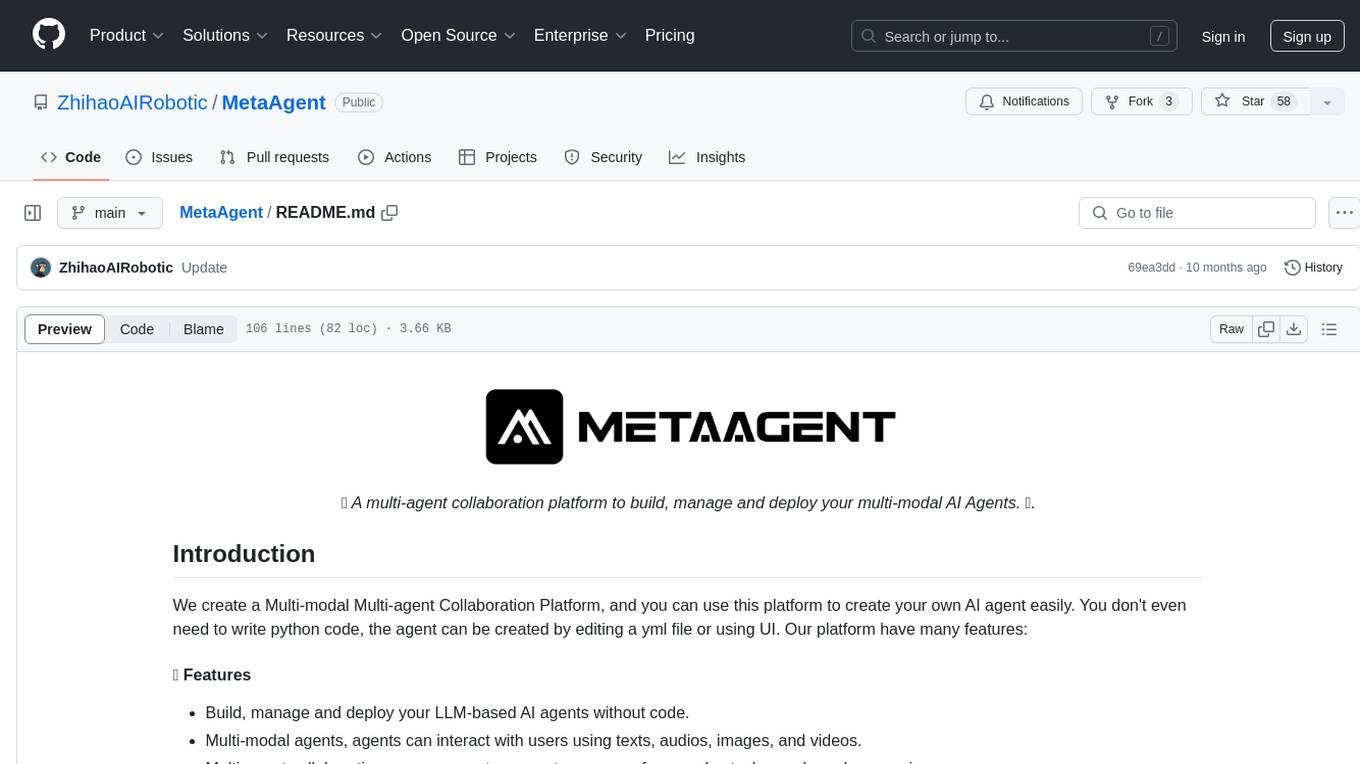
MetaAgent
MetaAgent is a multi-agent collaboration platform designed to build, manage, and deploy multi-modal AI agents without the need for coding. Users can easily create AI agents by editing a yml file or using the provided UI. The platform supports features such as building LLM-based AI agents, multi-modal interactions with users using texts, audios, images, and videos, creating a company of agents for complex tasks like drawing comics, vector database and knowledge embeddings, and upcoming features like UI for creating and using AI agents, fine-tuning, and RLHF. The tool simplifies the process of creating and deploying AI agents for various tasks.
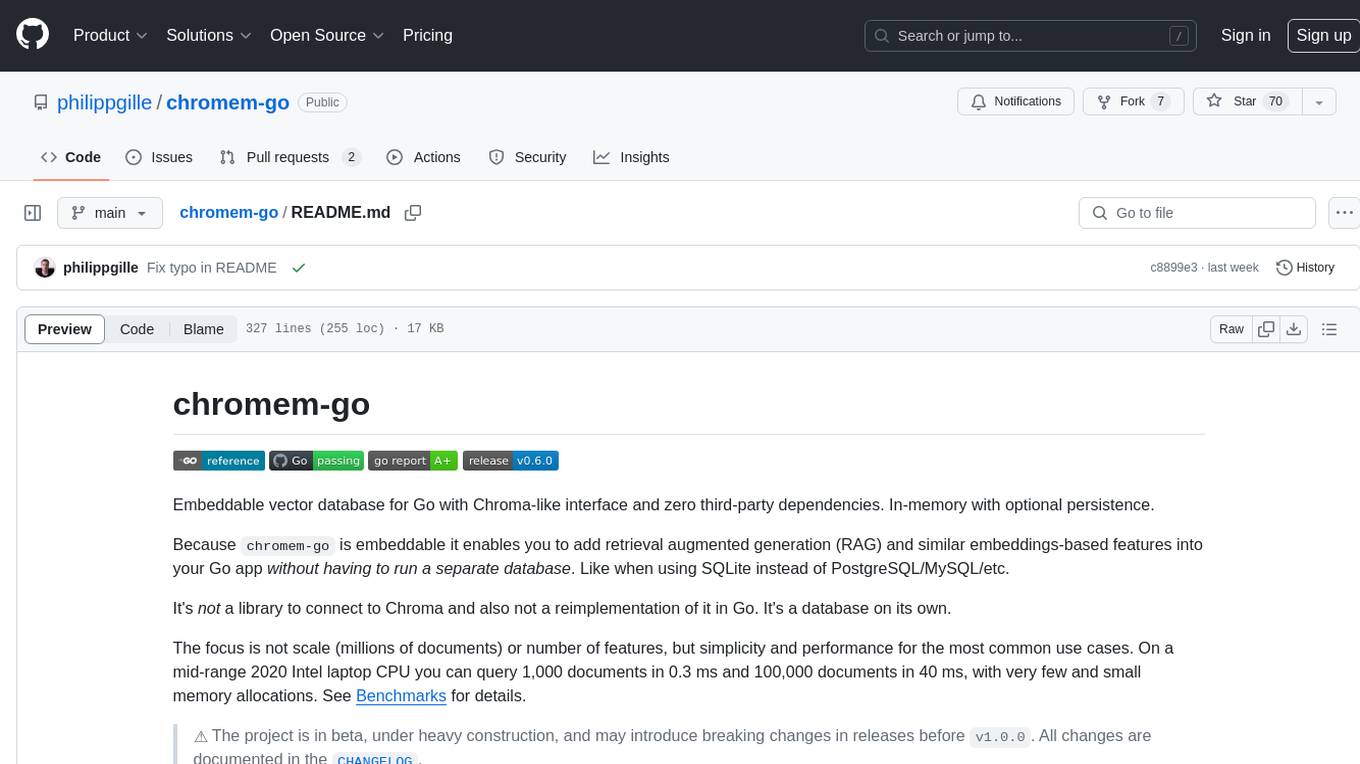
chromem-go
chromem-go is an embeddable vector database for Go with a Chroma-like interface and zero third-party dependencies. It enables retrieval augmented generation (RAG) and similar embeddings-based features in Go apps without the need for a separate database. The focus is on simplicity and performance for common use cases, allowing querying of documents with minimal memory allocations. The project is in beta and may introduce breaking changes before v1.0.0.
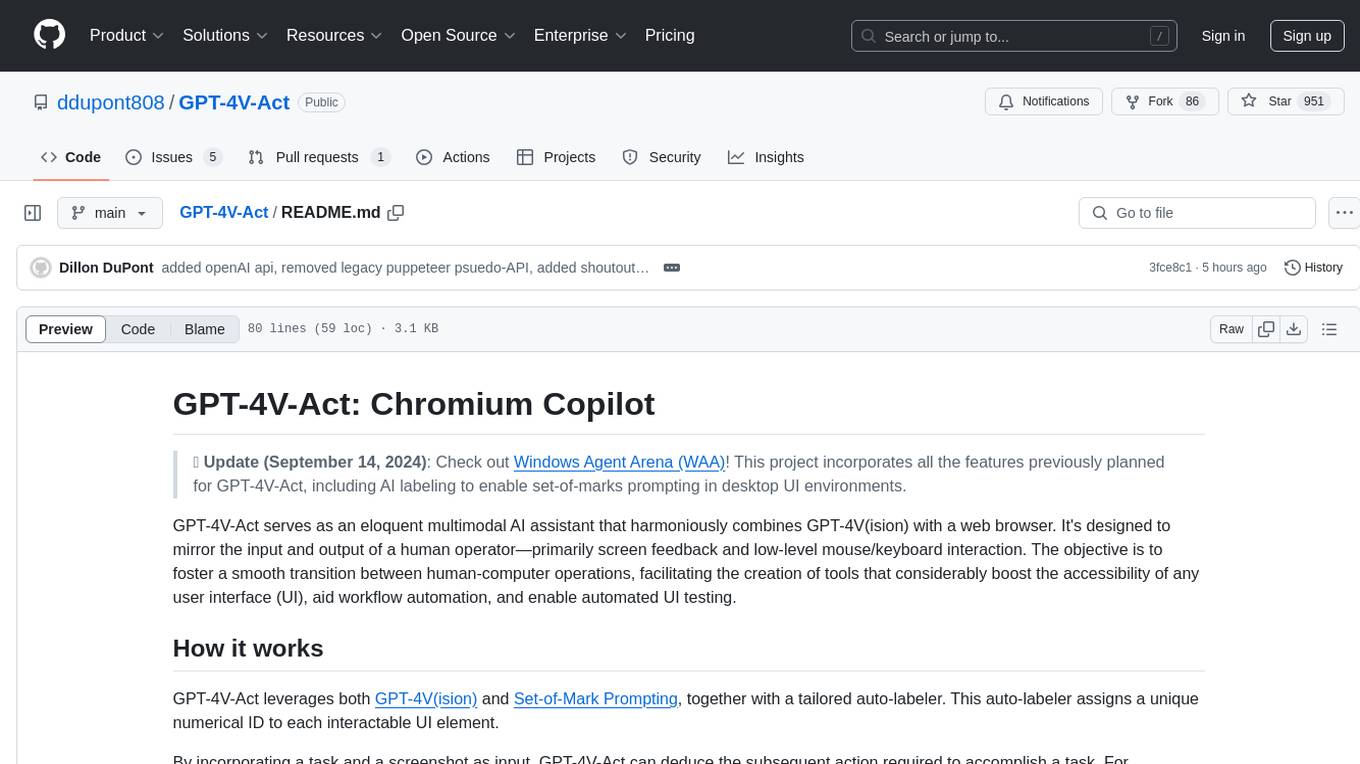
GPT-4V-Act
GPT-4V-Act is a multimodal AI assistant that combines GPT-4V(ision) with a web browser to mirror human operator input and output. It facilitates human-computer operations, boosts UI accessibility, aids workflow automation, and enables automated UI testing through AI labeling and set-of-marks prompting.
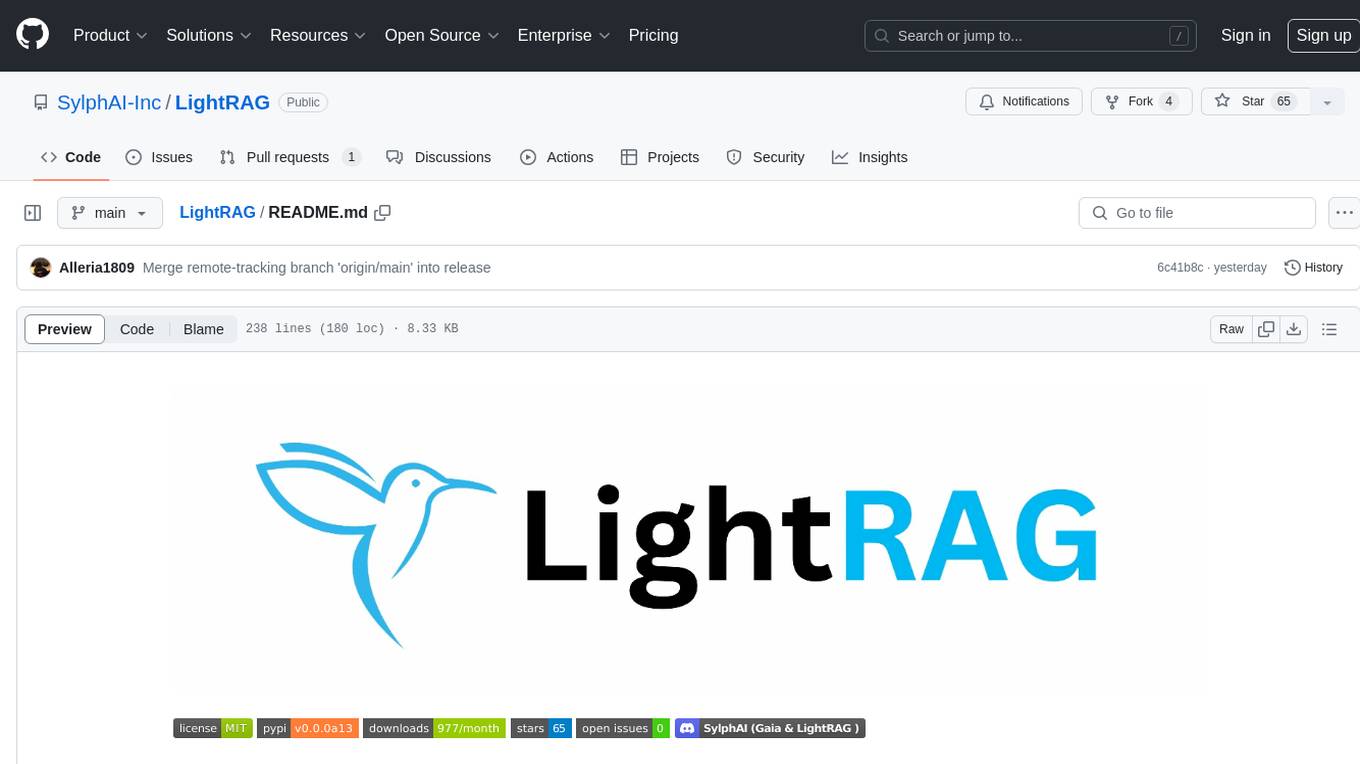
LightRAG
LightRAG is a PyTorch library designed for building and optimizing Retriever-Agent-Generator (RAG) pipelines. It follows principles of simplicity, quality, and optimization, offering developers maximum customizability with minimal abstraction. The library includes components for model interaction, output parsing, and structured data generation. LightRAG facilitates tasks like providing explanations and examples for concepts through a question-answering pipeline.
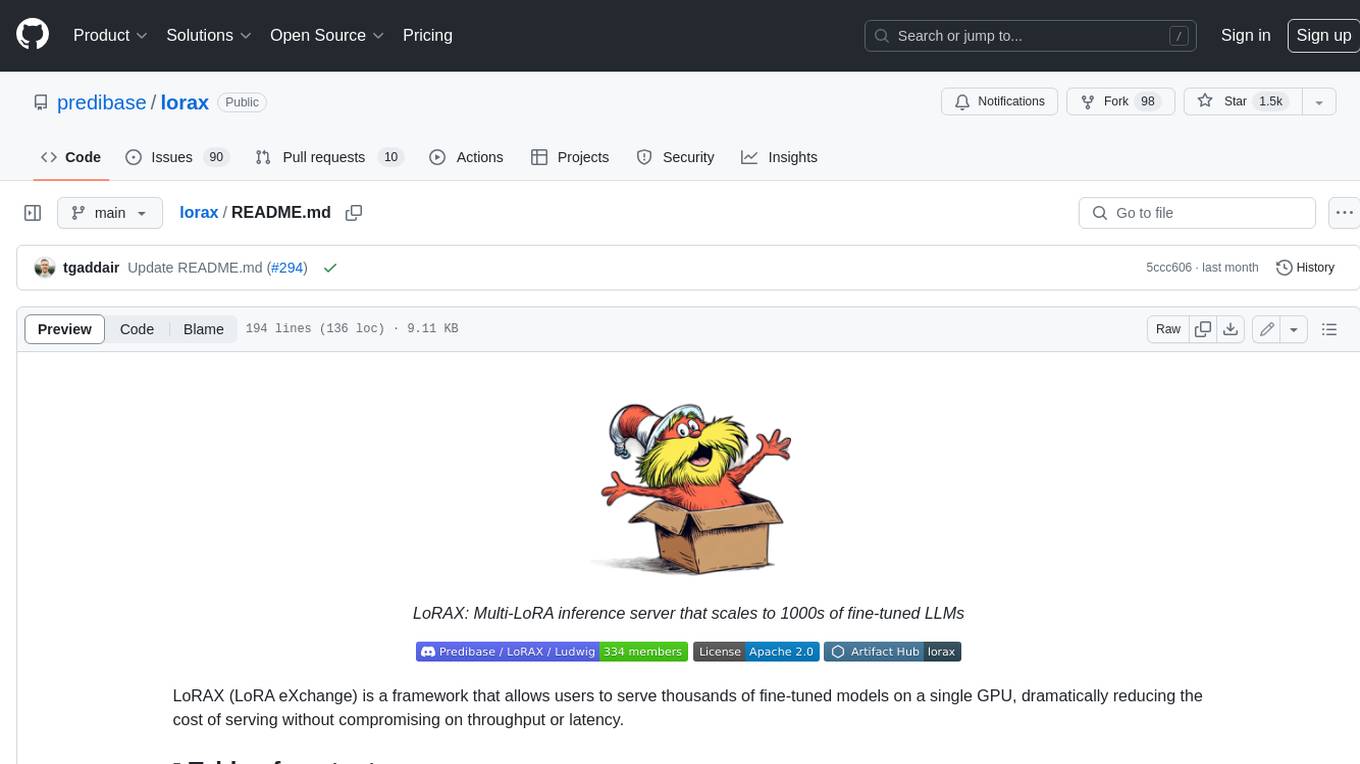
lorax
LoRAX is a framework that allows users to serve thousands of fine-tuned models on a single GPU, dramatically reducing the cost of serving without compromising on throughput or latency. It features dynamic adapter loading, heterogeneous continuous batching, adapter exchange scheduling, optimized inference, and is ready for production with prebuilt Docker images, Helm charts for Kubernetes, Prometheus metrics, and distributed tracing with Open Telemetry. LoRAX supports a number of Large Language Models as the base model including Llama, Mistral, and Qwen, and any of the linear layers in the model can be adapted via LoRA and loaded in LoRAX.
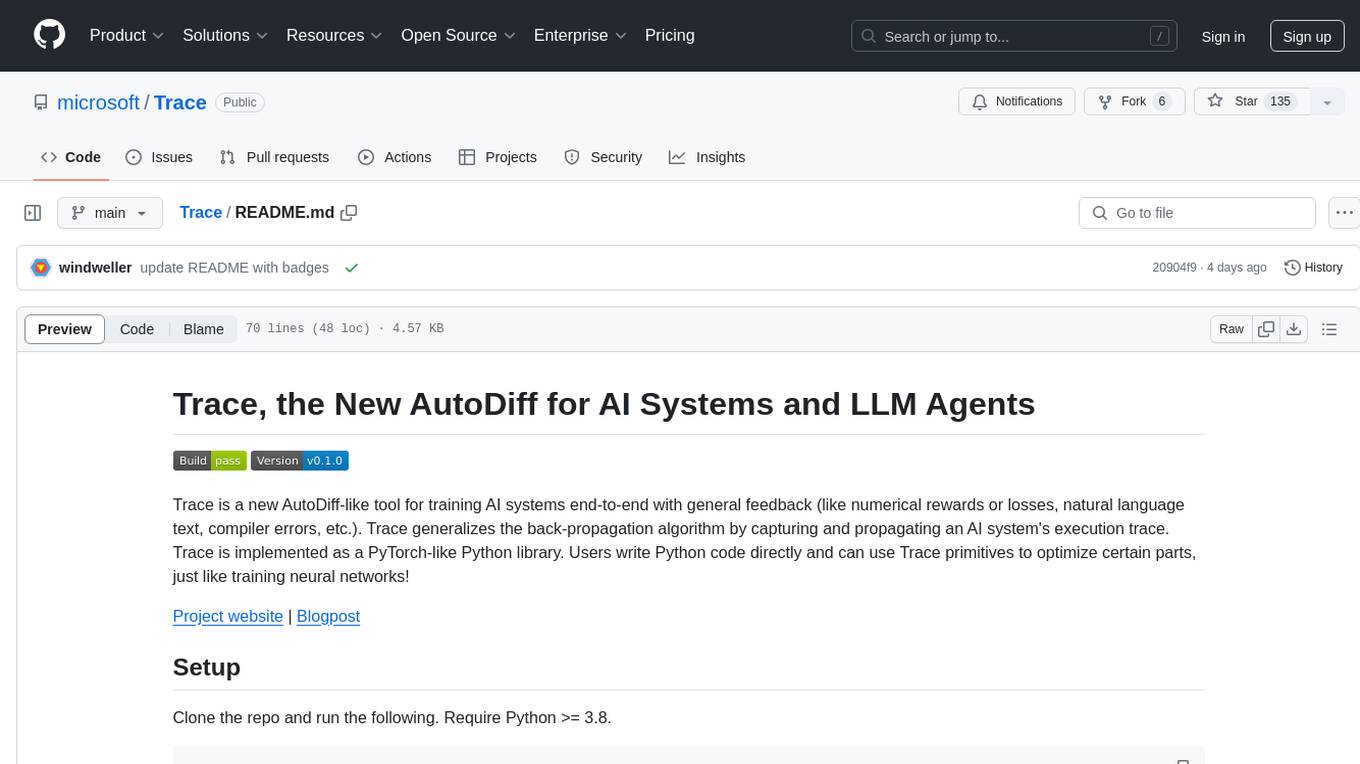
Trace
Trace is a new AutoDiff-like tool for training AI systems end-to-end with general feedback. It generalizes the back-propagation algorithm by capturing and propagating an AI system's execution trace. Implemented as a PyTorch-like Python library, users can write Python code directly and use Trace primitives to optimize certain parts, similar to training neural networks.
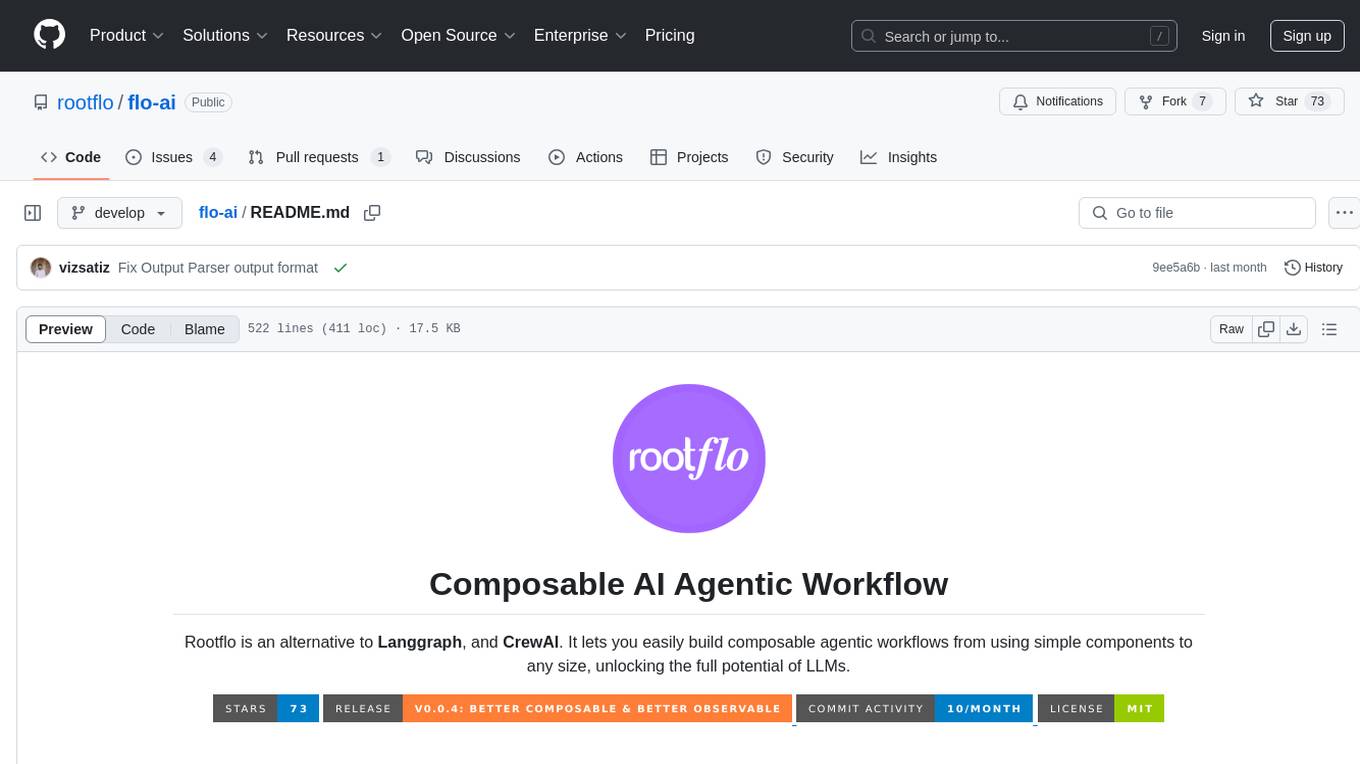
flo-ai
Flo AI is a Python framework that enables users to build production-ready AI agents and teams with minimal code. It allows users to compose complex AI architectures using pre-built components while maintaining the flexibility to create custom components. The framework supports composable, production-ready, YAML-first, and flexible AI systems. Users can easily create AI agents and teams, manage teams of AI agents working together, and utilize built-in support for Retrieval-Augmented Generation (RAG) and compatibility with Langchain tools. Flo AI also provides tools for output parsing and formatting, tool logging, data collection, and JSON output collection. It is MIT Licensed and offers detailed documentation, tutorials, and examples for AI engineers and teams to accelerate development, maintainability, scalability, and testability of AI systems.
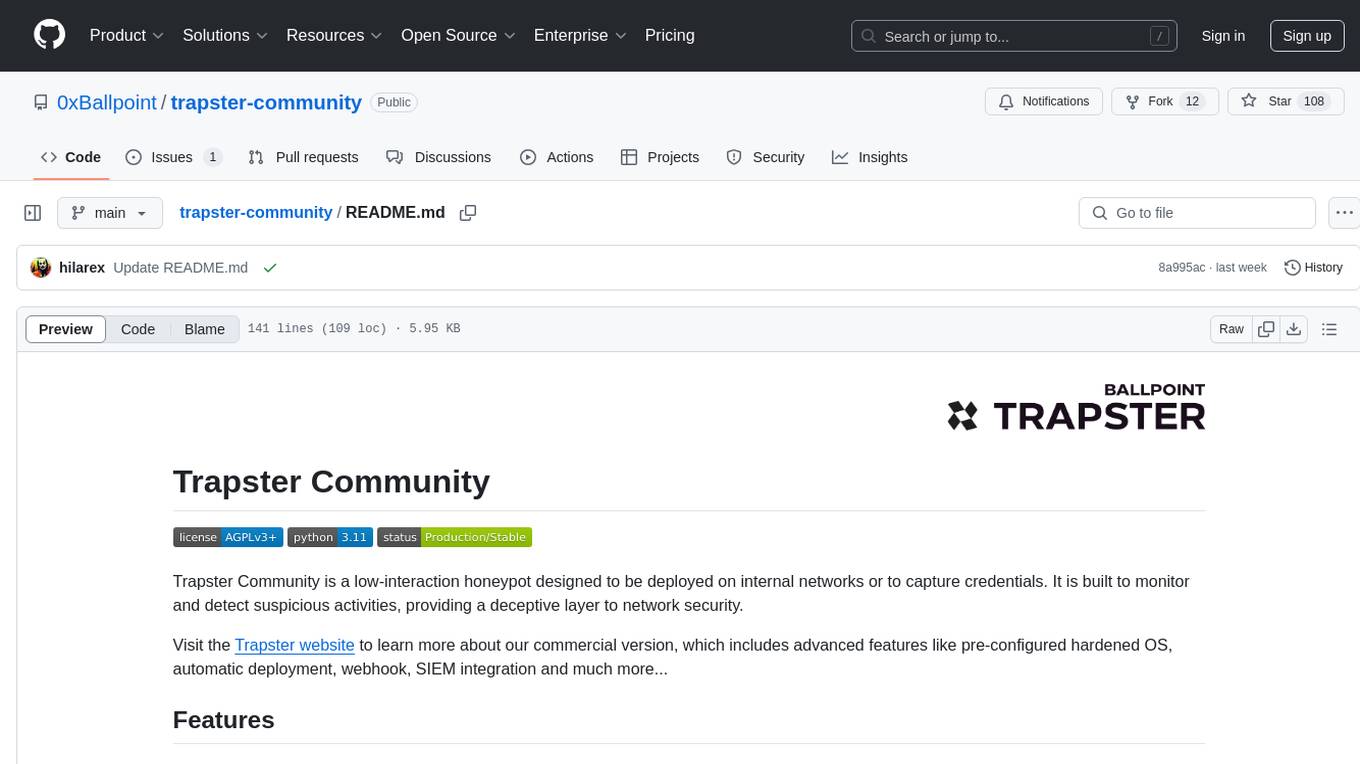
trapster-community
Trapster Community is a low-interaction honeypot designed for internal networks or credential capture. It monitors and detects suspicious activities, providing deceptive security layer. Features include mimicking network services, asynchronous framework, easy configuration, expandable services, and HTTP honeypot engine with AI capabilities. Supported protocols include DNS, HTTP/HTTPS, FTP, LDAP, MSSQL, POSTGRES, RDP, SNMP, SSH, TELNET, VNC, and RSYNC. The tool generates various types of logs and offers HTTP engine with AI capabilities to emulate websites using YAML configuration. Contributions are welcome under AGPLv3+ license.
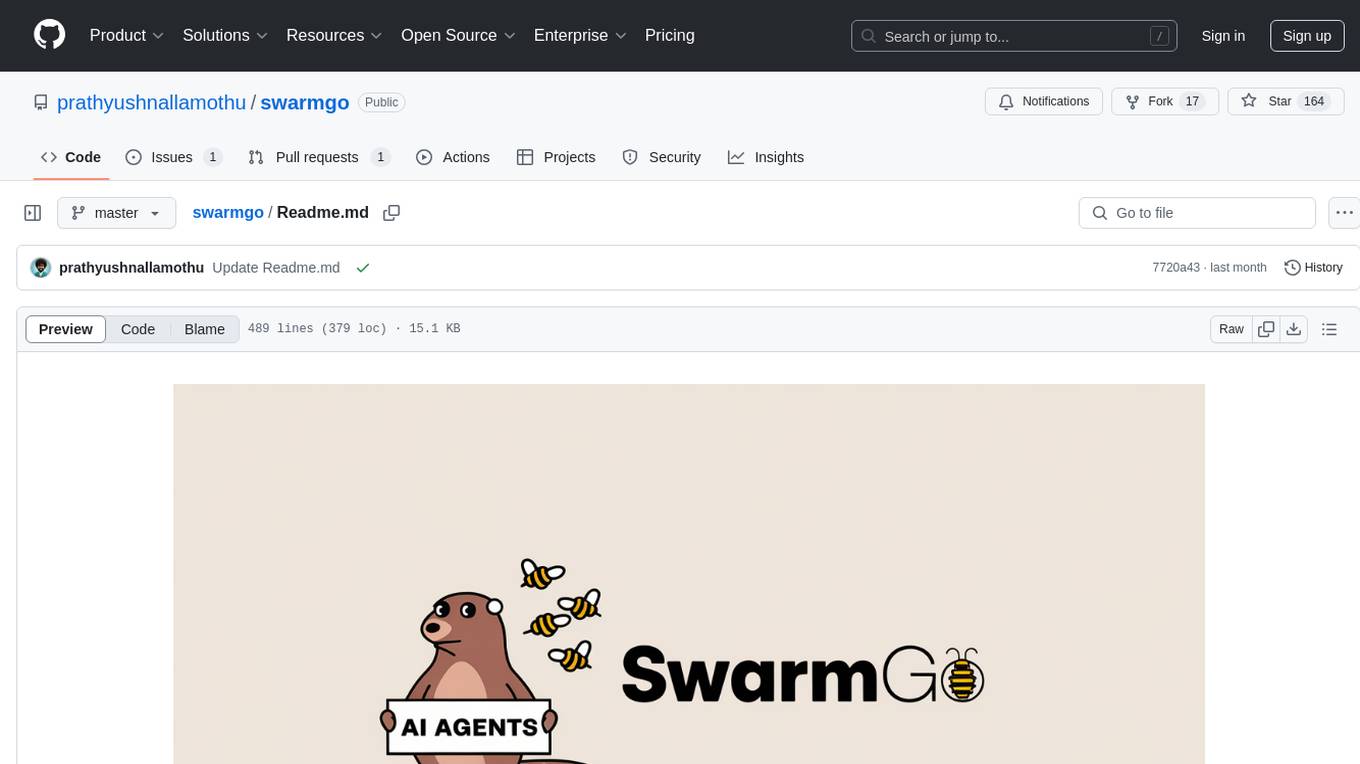
swarmgo
SwarmGo is a Go package designed to create AI agents capable of interacting, coordinating, and executing tasks. It focuses on lightweight agent coordination and execution, offering powerful primitives like Agents and handoffs. SwarmGo enables building scalable solutions with rich dynamics between tools and networks of agents, all while keeping the learning curve low. It supports features like memory management, streaming support, concurrent agent execution, LLM interface, and structured workflows for organizing and coordinating multiple agents.
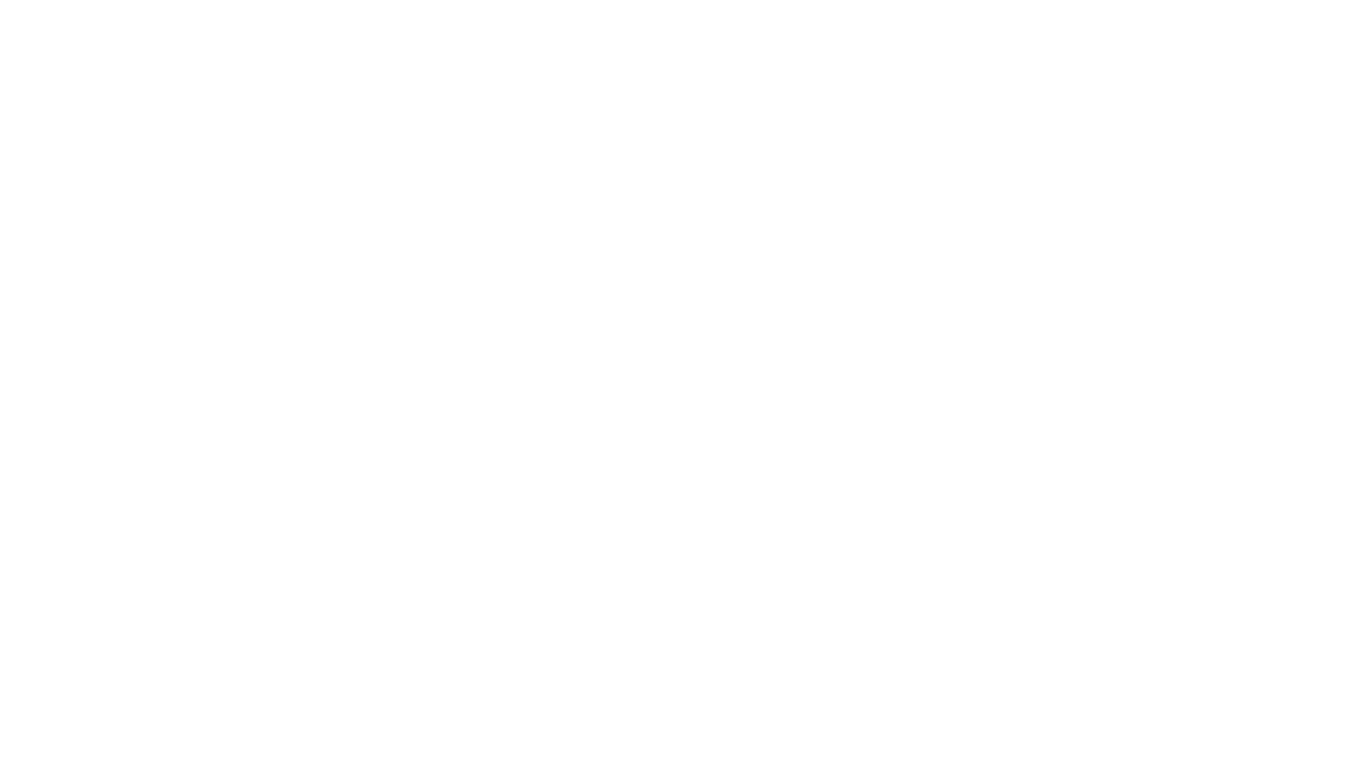
AutoMathText
AutoMathText is an extensive dataset of around 200 GB of mathematical texts autonomously selected by the language model Qwen-72B. It aims to facilitate research in mathematics and artificial intelligence, serve as an educational tool for learning complex mathematical concepts, and provide a foundation for developing AI models specialized in processing mathematical content.
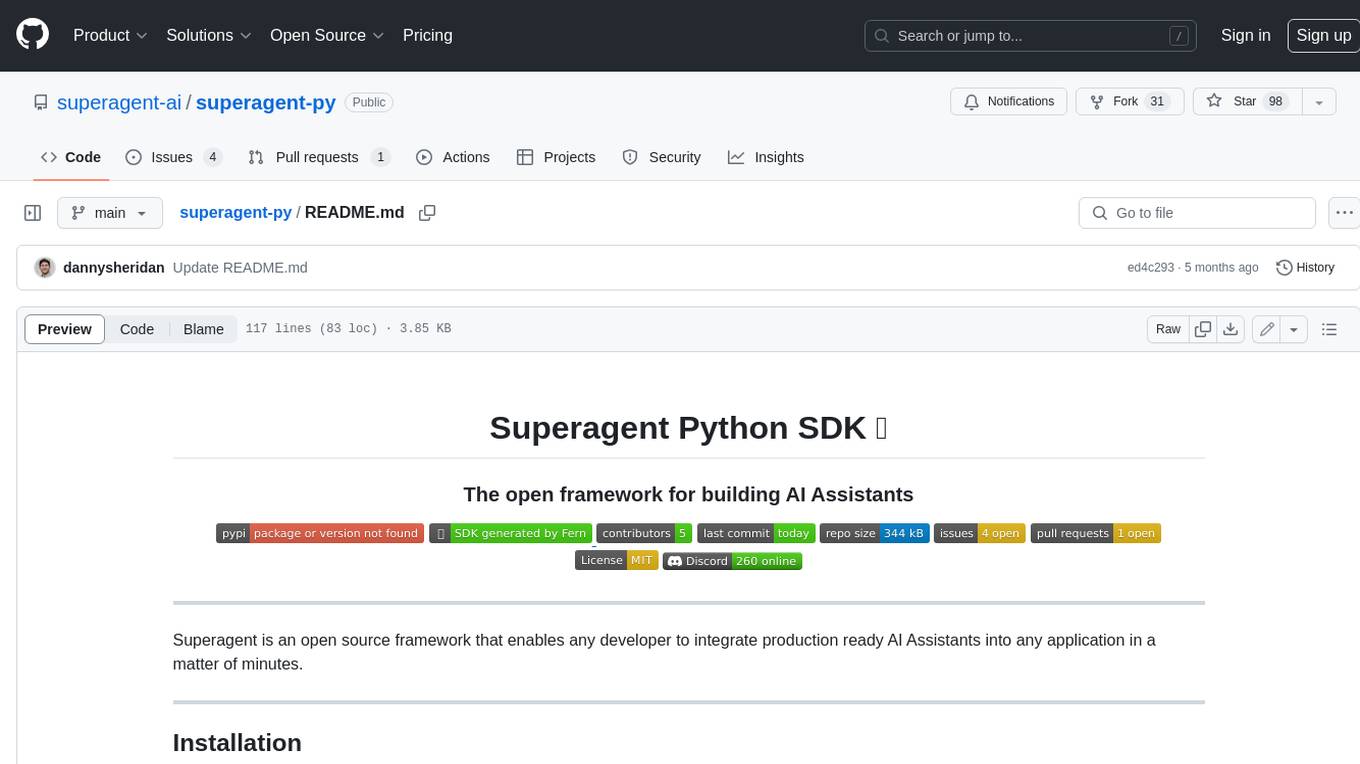
superagent-py
Superagent is an open-source framework that enables developers to integrate production-ready AI assistants into any application quickly and easily. It provides a Python SDK for interacting with the Superagent API, allowing developers to create, manage, and invoke AI agents. The SDK simplifies the process of building AI-powered applications, making it accessible to developers of all skill levels.
For similar tasks
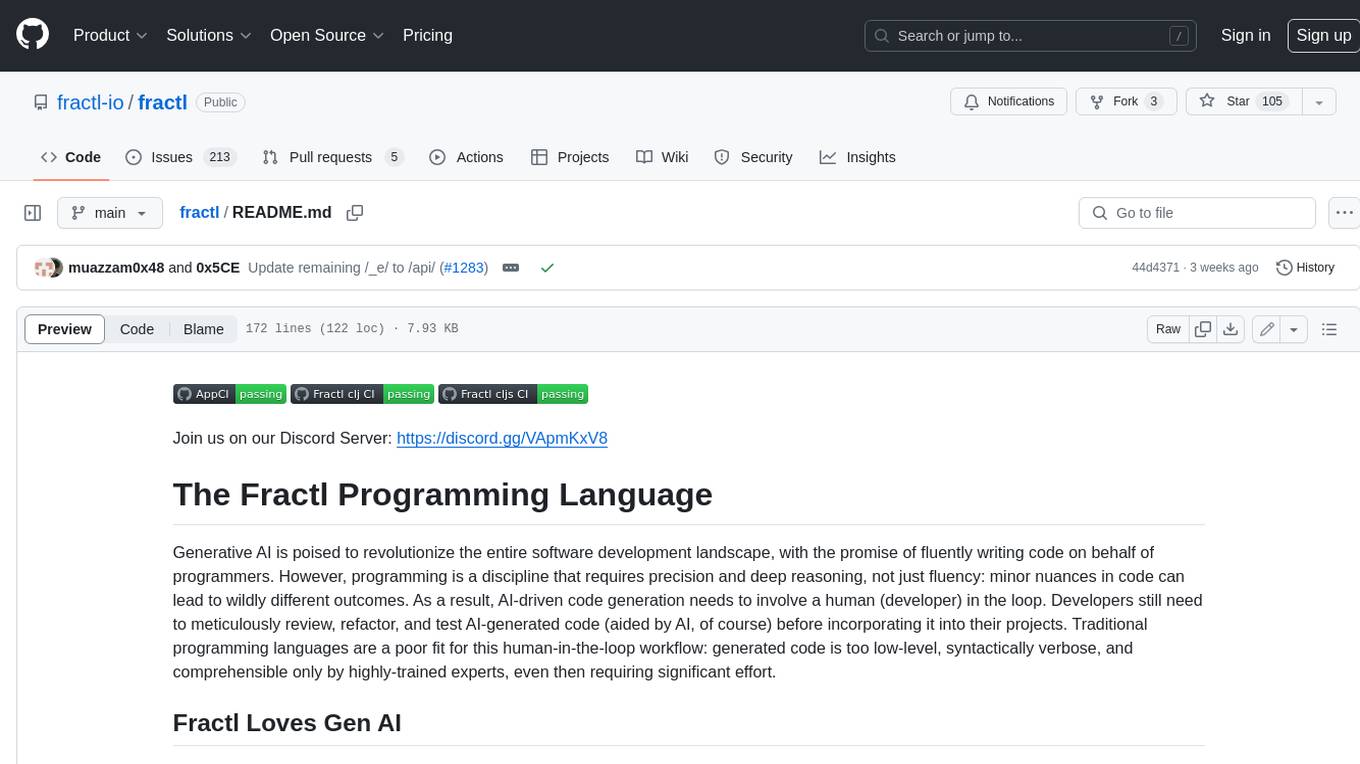
fractl
Fractl is a programming language designed for generative AI, making it easier for developers to work with AI-generated code. It features a data-oriented and declarative syntax, making it a better fit for generative AI-powered code generation. Fractl also bridges the gap between traditional programming and visual building, allowing developers to use multiple ways of building, including traditional coding, visual development, and code generation with generative AI. Key concepts in Fractl include a graph-based hierarchical data model, zero-trust programming, declarative dataflow, resolvers, interceptors, and entity-graph-database mapping.
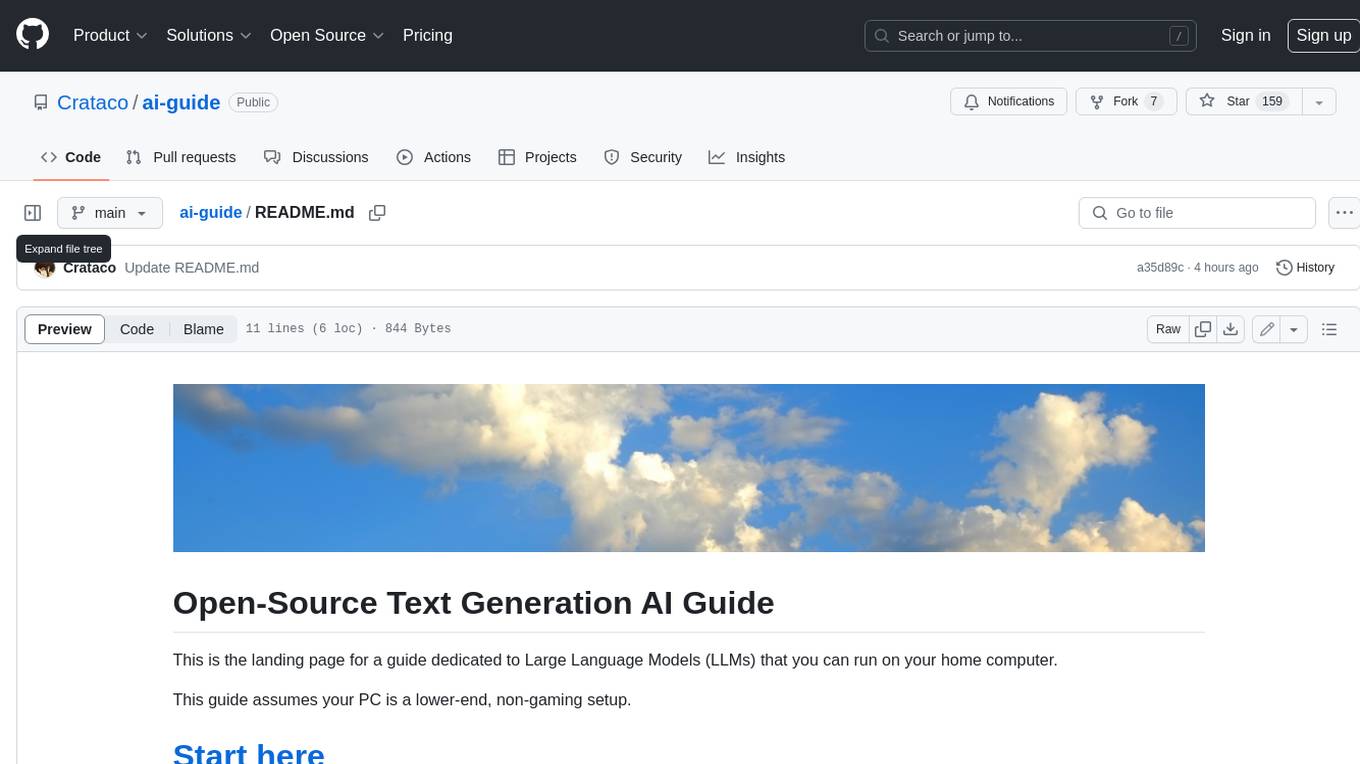
ai-guide
This guide is dedicated to Large Language Models (LLMs) that you can run on your home computer. It assumes your PC is a lower-end, non-gaming setup.
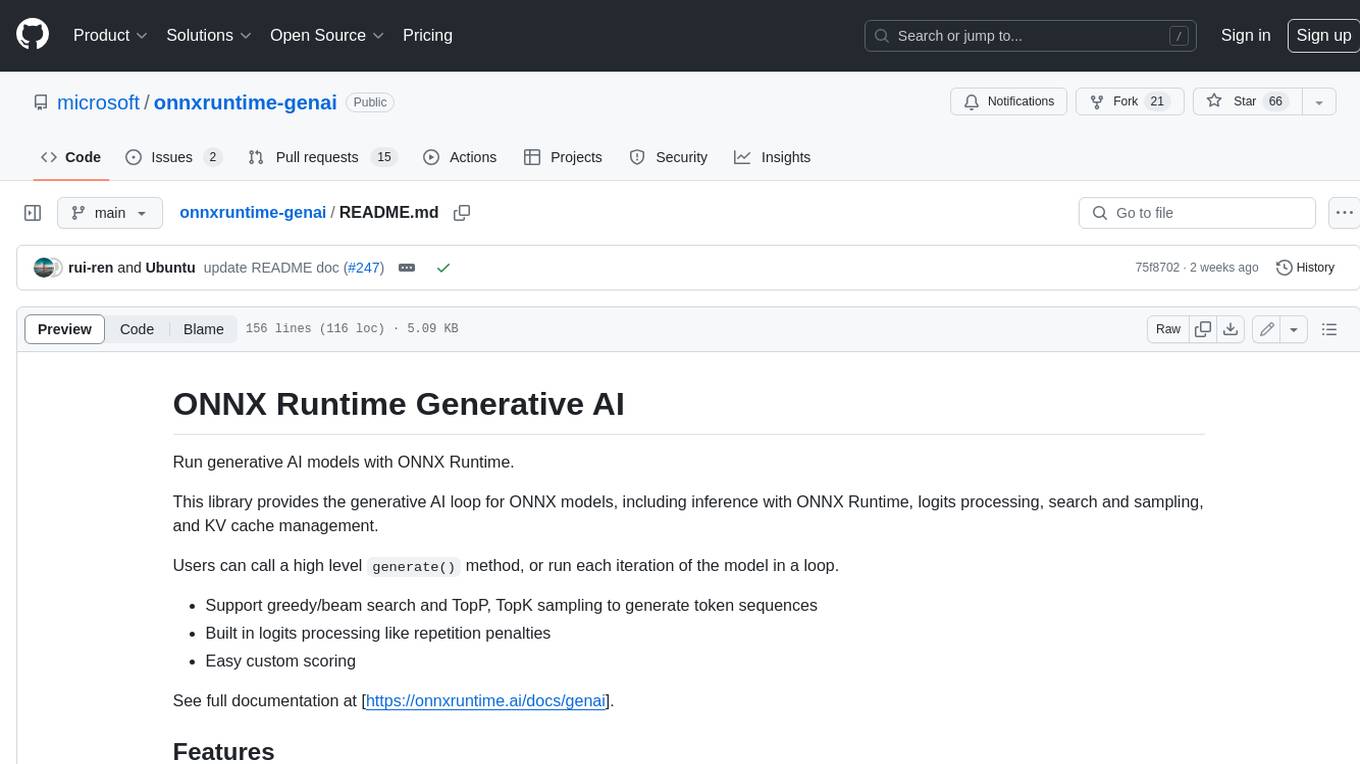
onnxruntime-genai
ONNX Runtime Generative AI is a library that provides the generative AI loop for ONNX models, including inference with ONNX Runtime, logits processing, search and sampling, and KV cache management. Users can call a high level `generate()` method, or run each iteration of the model in a loop. It supports greedy/beam search and TopP, TopK sampling to generate token sequences, has built in logits processing like repetition penalties, and allows for easy custom scoring.
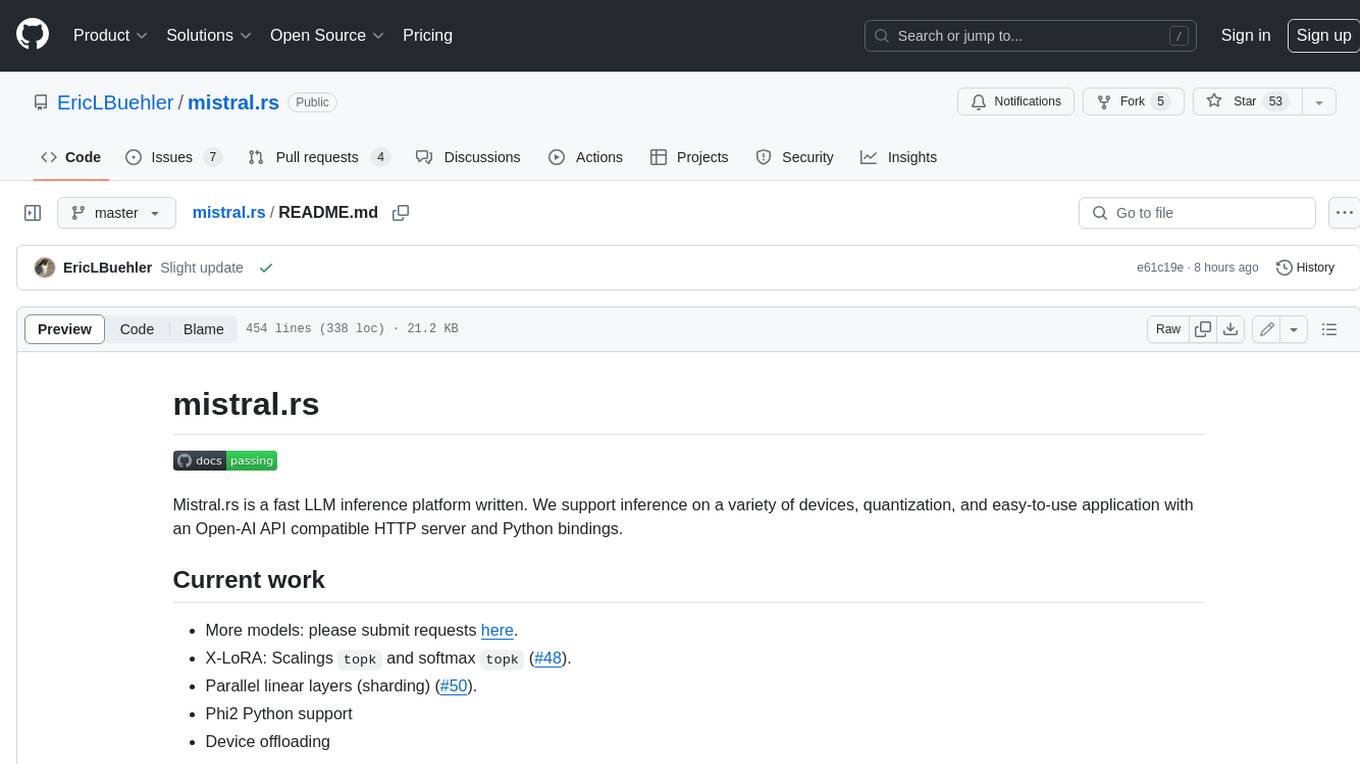
mistral.rs
Mistral.rs is a fast LLM inference platform written in Rust. We support inference on a variety of devices, quantization, and easy-to-use application with an Open-AI API compatible HTTP server and Python bindings.
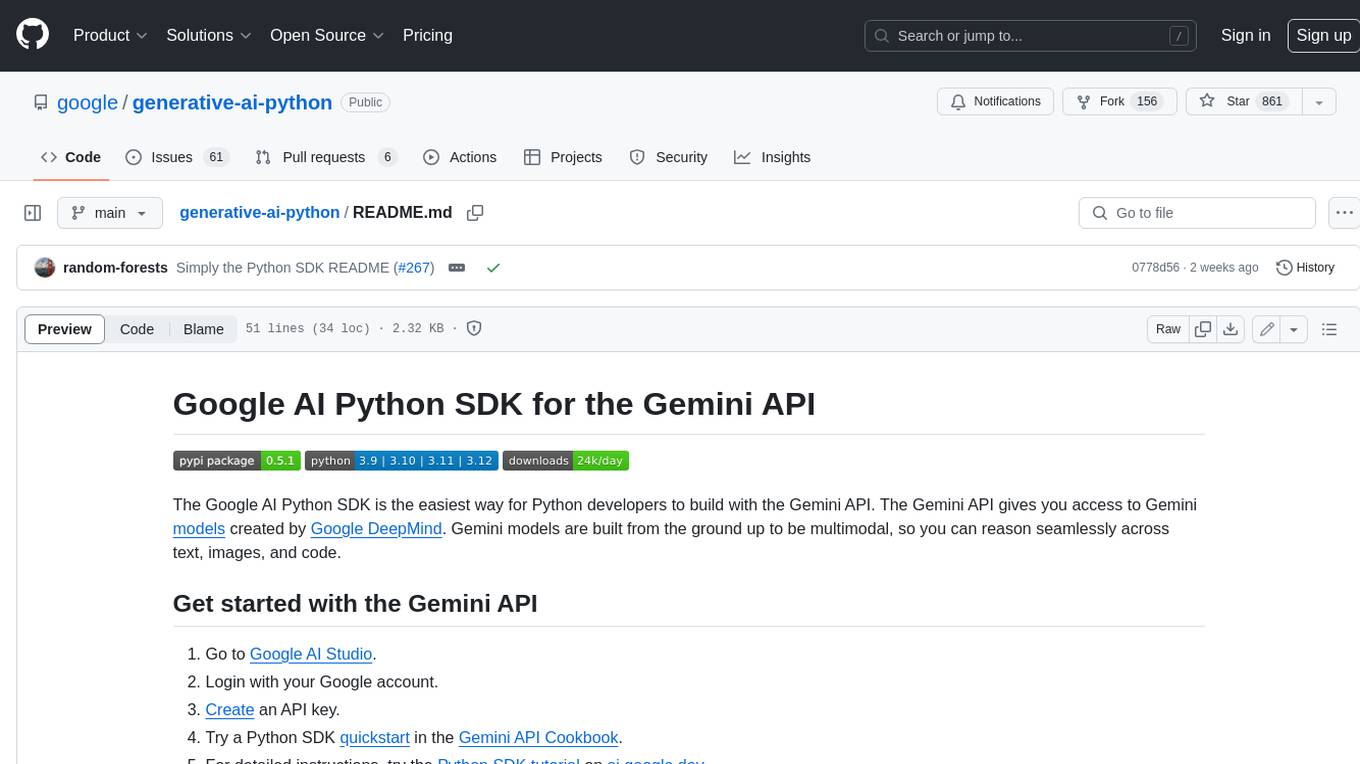
generative-ai-python
The Google AI Python SDK is the easiest way for Python developers to build with the Gemini API. The Gemini API gives you access to Gemini models created by Google DeepMind. Gemini models are built from the ground up to be multimodal, so you can reason seamlessly across text, images, and code.
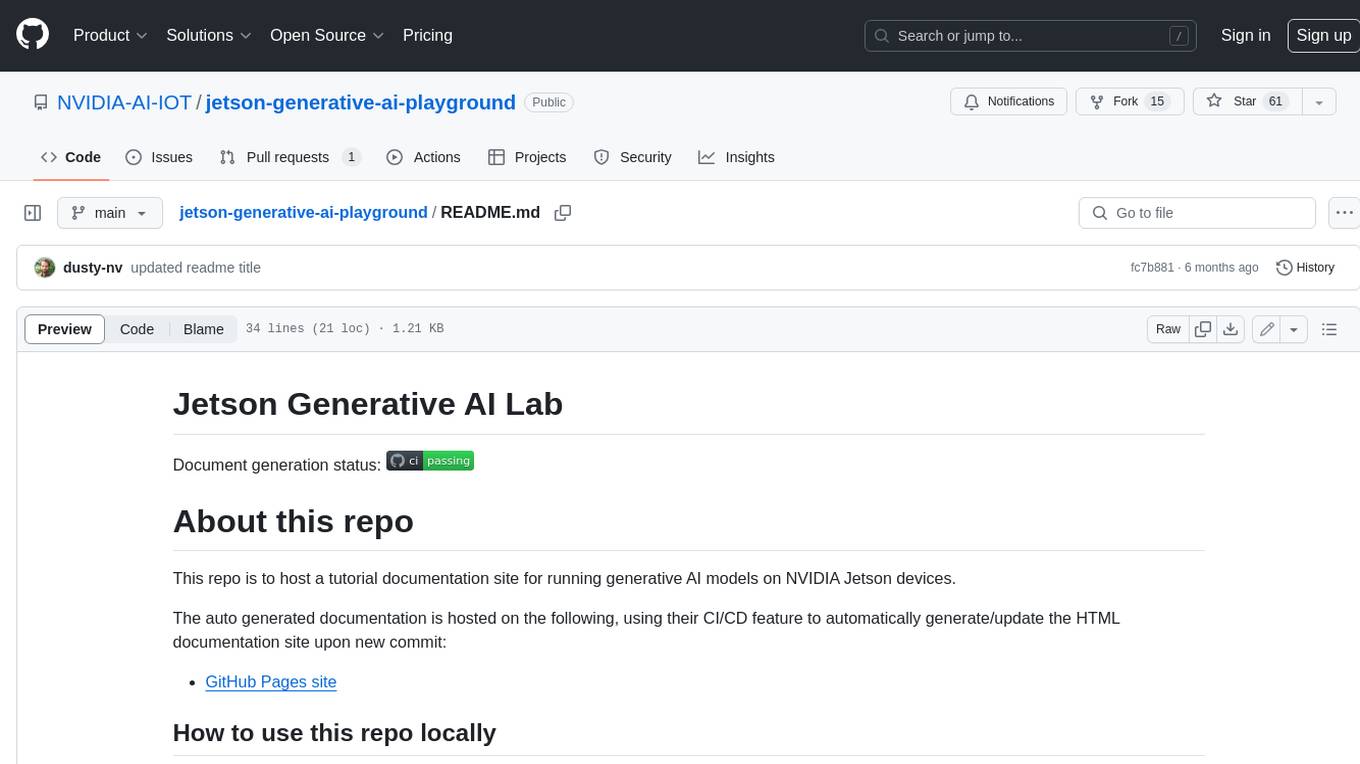
jetson-generative-ai-playground
This repo hosts tutorial documentation for running generative AI models on NVIDIA Jetson devices. The documentation is auto-generated and hosted on GitHub Pages using their CI/CD feature to automatically generate/update the HTML documentation site upon new commits.
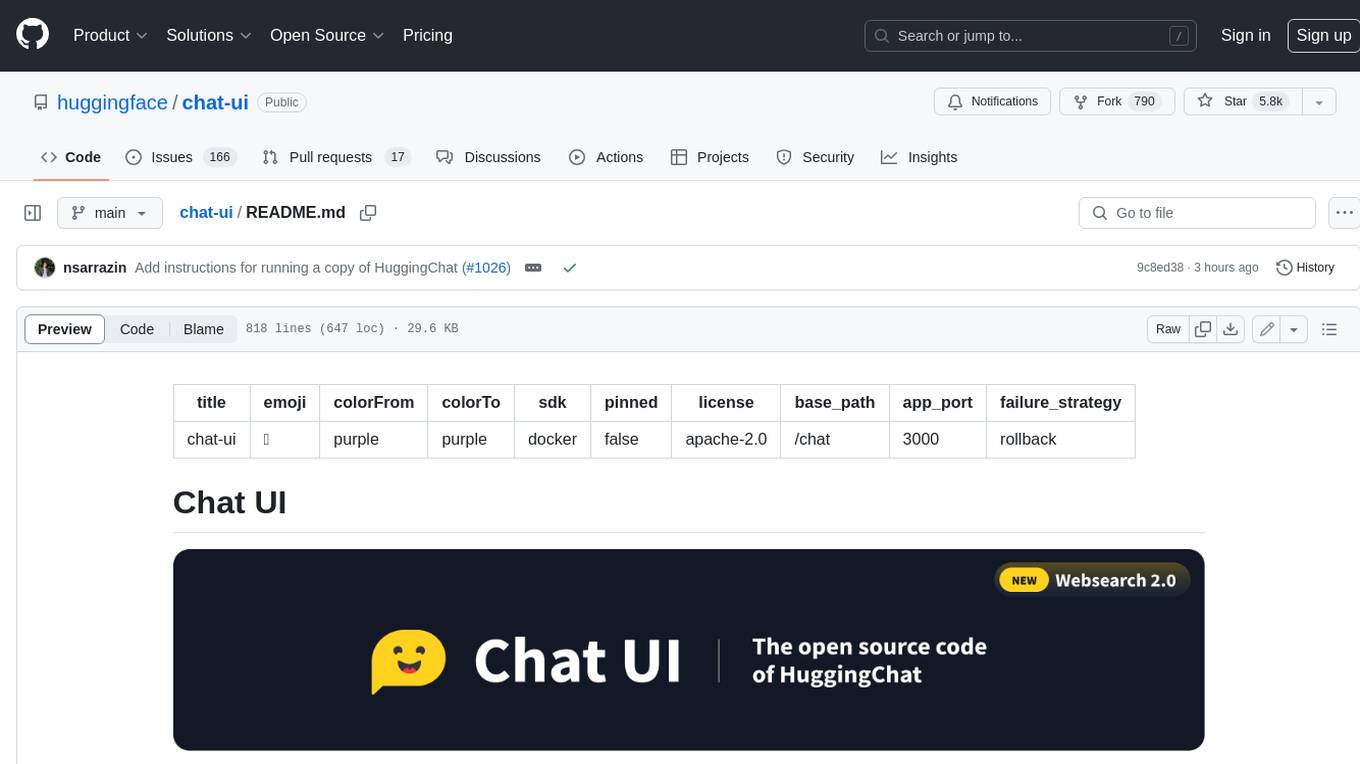
chat-ui
A chat interface using open source models, eg OpenAssistant or Llama. It is a SvelteKit app and it powers the HuggingChat app on hf.co/chat.
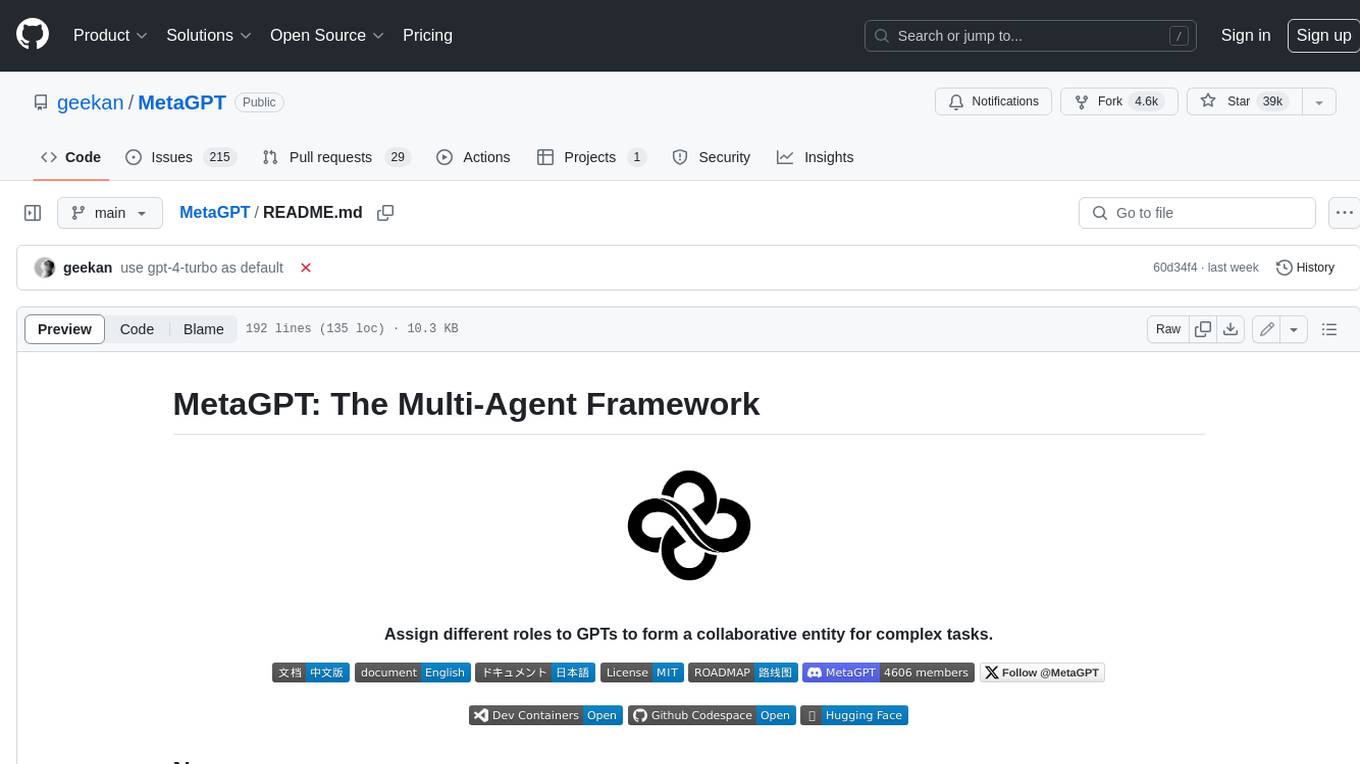
MetaGPT
MetaGPT is a multi-agent framework that enables GPT to work in a software company, collaborating to tackle more complex tasks. It assigns different roles to GPTs to form a collaborative entity for complex tasks. MetaGPT takes a one-line requirement as input and outputs user stories, competitive analysis, requirements, data structures, APIs, documents, etc. Internally, MetaGPT includes product managers, architects, project managers, and engineers. It provides the entire process of a software company along with carefully orchestrated SOPs. MetaGPT's core philosophy is "Code = SOP(Team)", materializing SOP and applying it to teams composed of LLMs.
For similar jobs
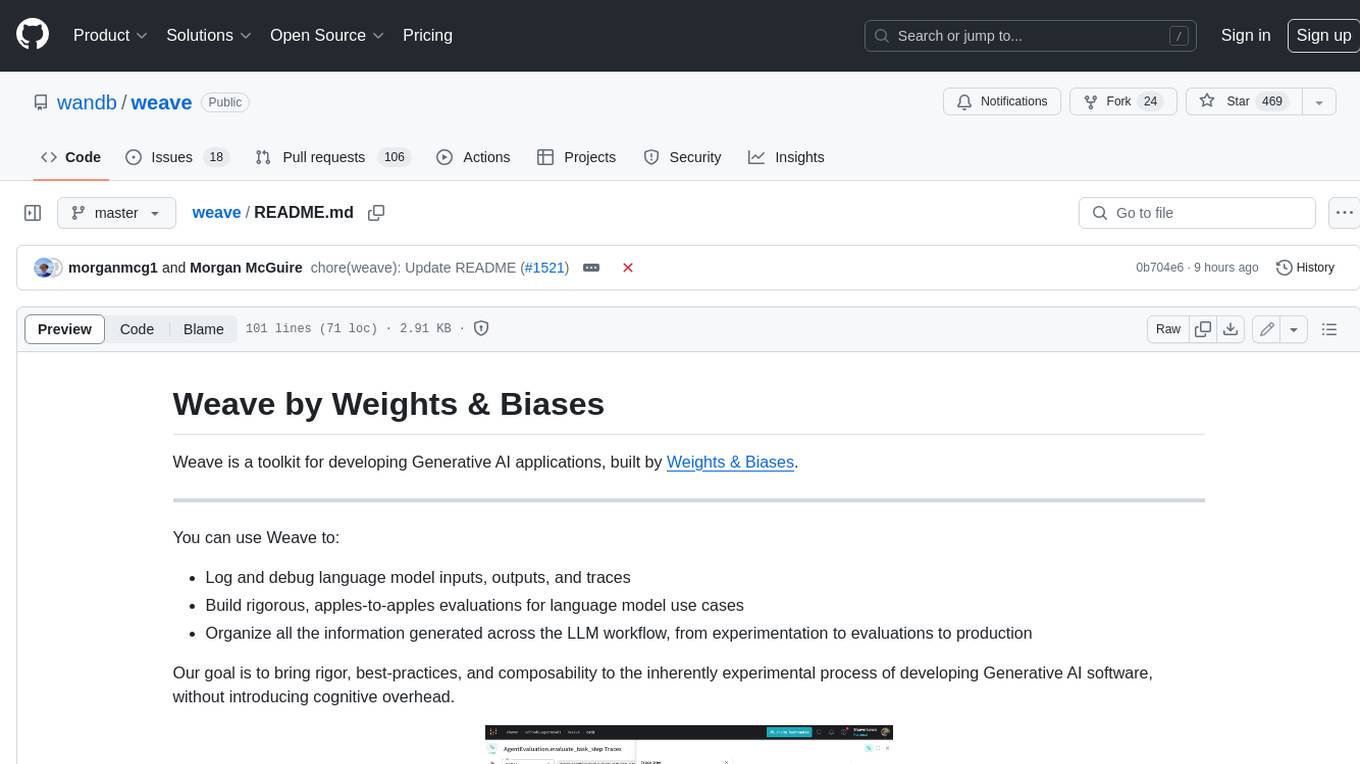
weave
Weave is a toolkit for developing Generative AI applications, built by Weights & Biases. With Weave, you can log and debug language model inputs, outputs, and traces; build rigorous, apples-to-apples evaluations for language model use cases; and organize all the information generated across the LLM workflow, from experimentation to evaluations to production. Weave aims to bring rigor, best-practices, and composability to the inherently experimental process of developing Generative AI software, without introducing cognitive overhead.
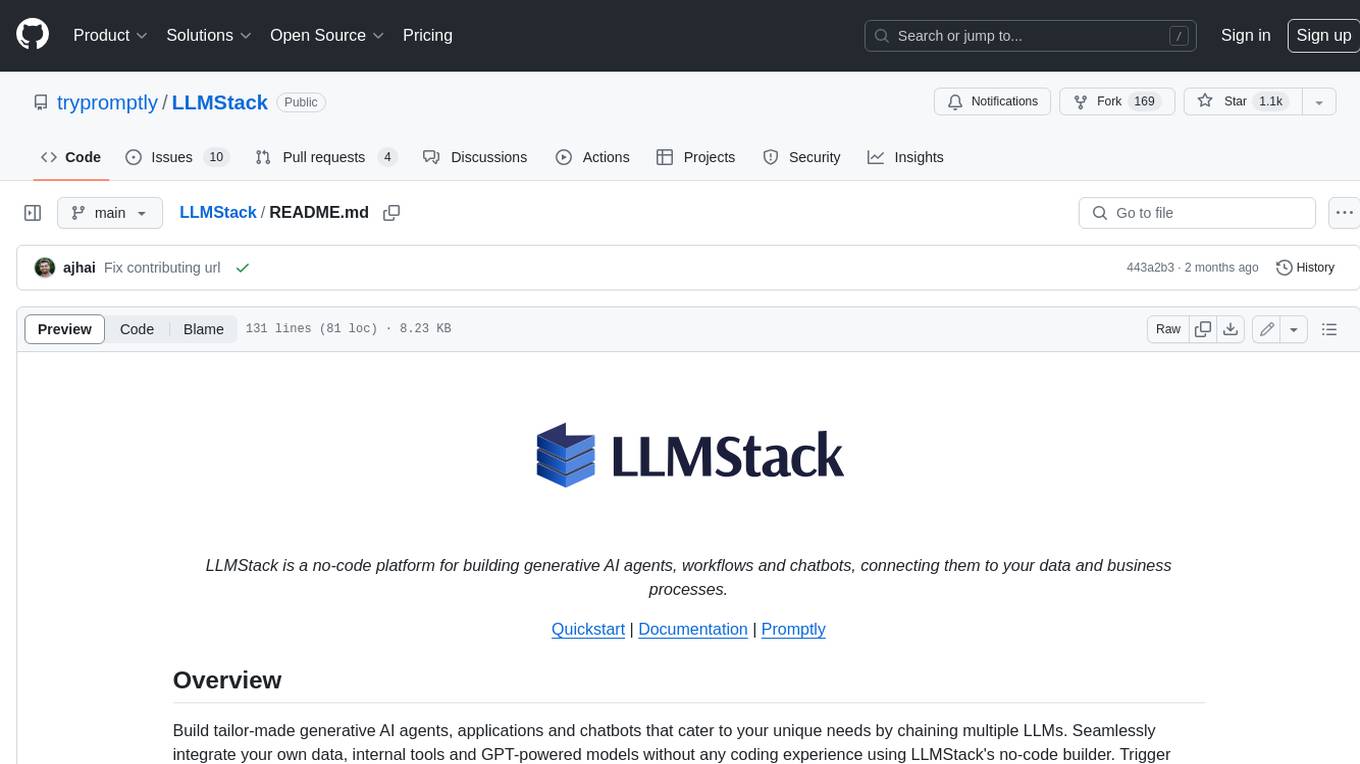
LLMStack
LLMStack is a no-code platform for building generative AI agents, workflows, and chatbots. It allows users to connect their own data, internal tools, and GPT-powered models without any coding experience. LLMStack can be deployed to the cloud or on-premise and can be accessed via HTTP API or triggered from Slack or Discord.
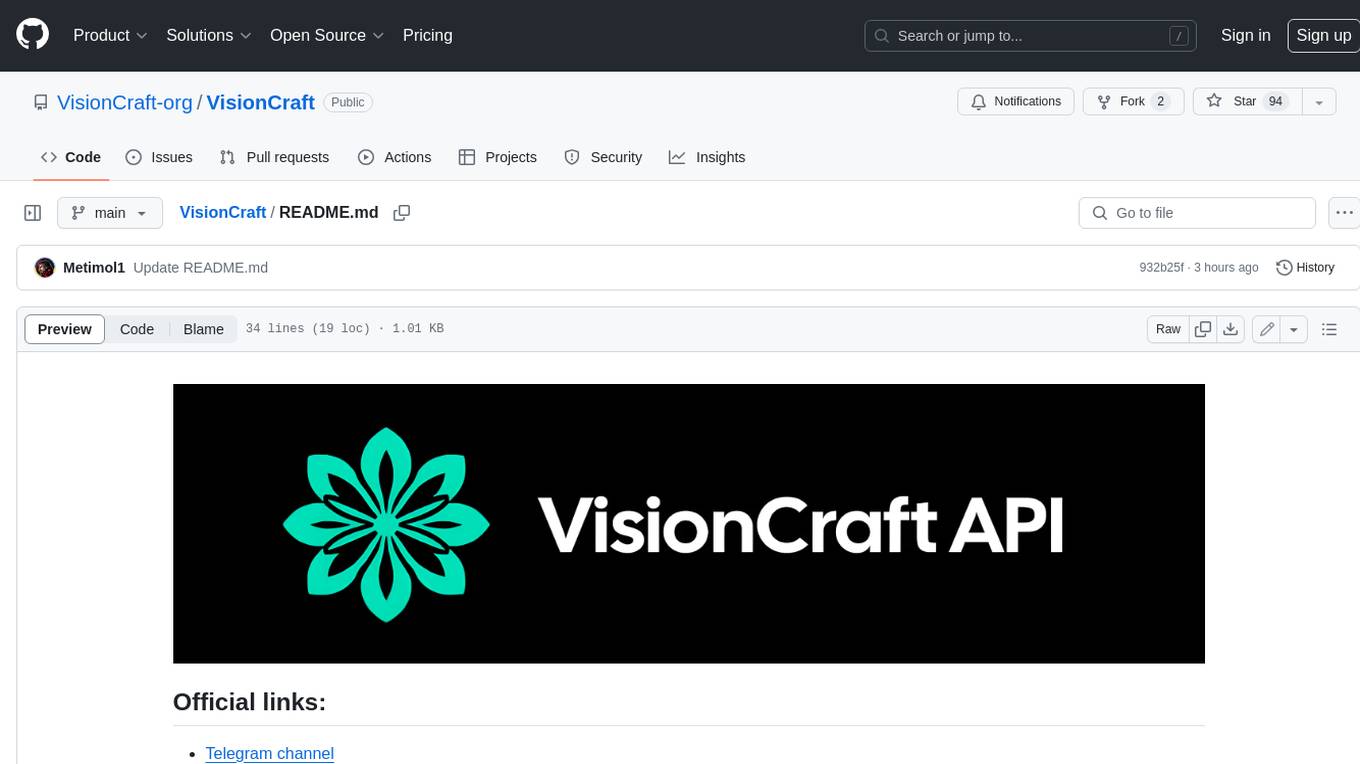
VisionCraft
The VisionCraft API is a free API for using over 100 different AI models. From images to sound.
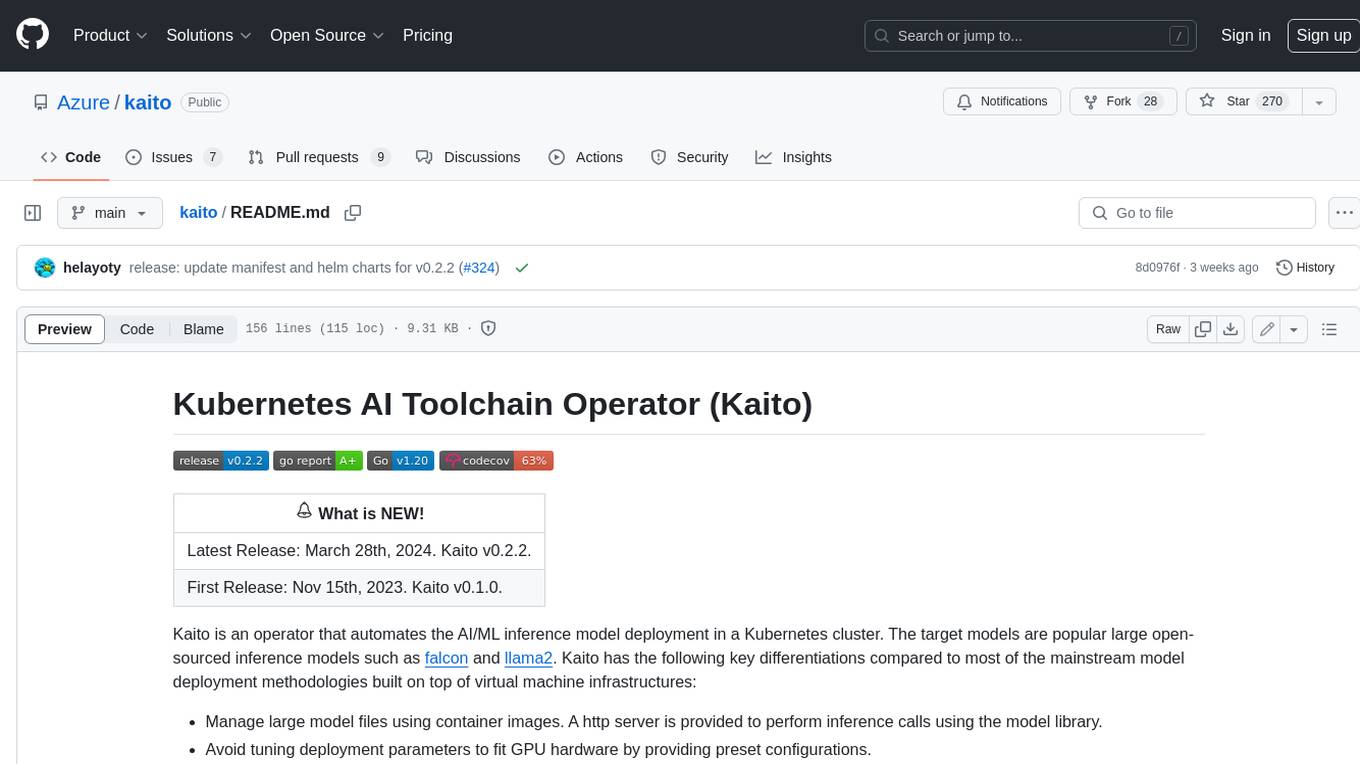
kaito
Kaito is an operator that automates the AI/ML inference model deployment in a Kubernetes cluster. It manages large model files using container images, avoids tuning deployment parameters to fit GPU hardware by providing preset configurations, auto-provisions GPU nodes based on model requirements, and hosts large model images in the public Microsoft Container Registry (MCR) if the license allows. Using Kaito, the workflow of onboarding large AI inference models in Kubernetes is largely simplified.
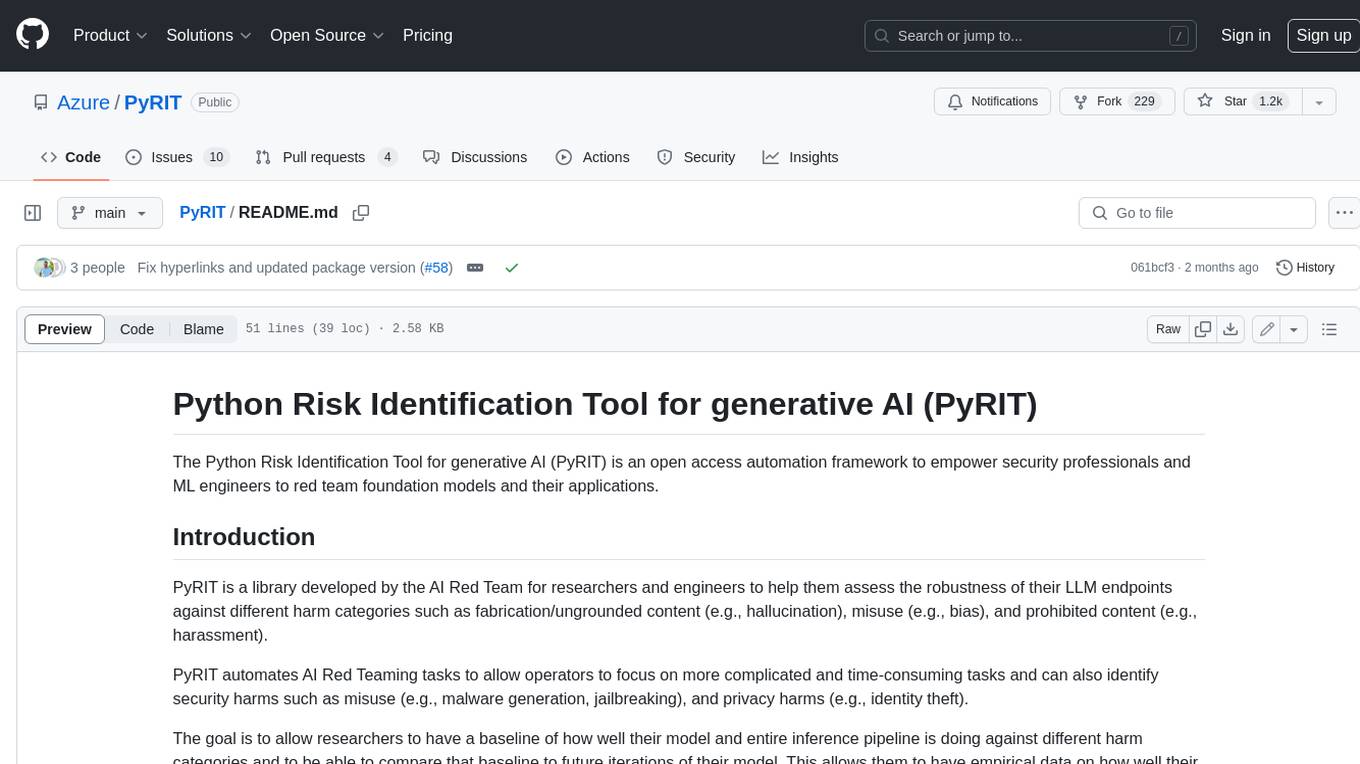
PyRIT
PyRIT is an open access automation framework designed to empower security professionals and ML engineers to red team foundation models and their applications. It automates AI Red Teaming tasks to allow operators to focus on more complicated and time-consuming tasks and can also identify security harms such as misuse (e.g., malware generation, jailbreaking), and privacy harms (e.g., identity theft). The goal is to allow researchers to have a baseline of how well their model and entire inference pipeline is doing against different harm categories and to be able to compare that baseline to future iterations of their model. This allows them to have empirical data on how well their model is doing today, and detect any degradation of performance based on future improvements.
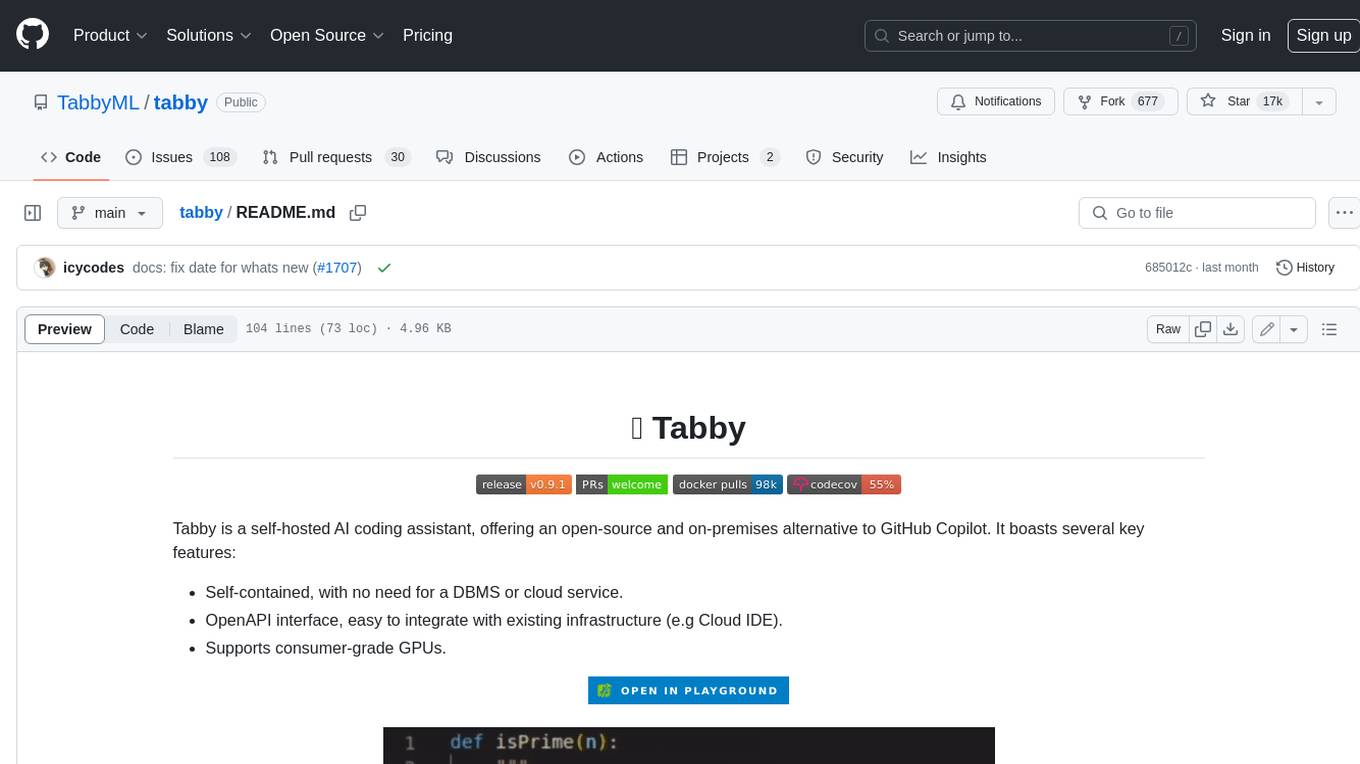
tabby
Tabby is a self-hosted AI coding assistant, offering an open-source and on-premises alternative to GitHub Copilot. It boasts several key features: * Self-contained, with no need for a DBMS or cloud service. * OpenAPI interface, easy to integrate with existing infrastructure (e.g Cloud IDE). * Supports consumer-grade GPUs.
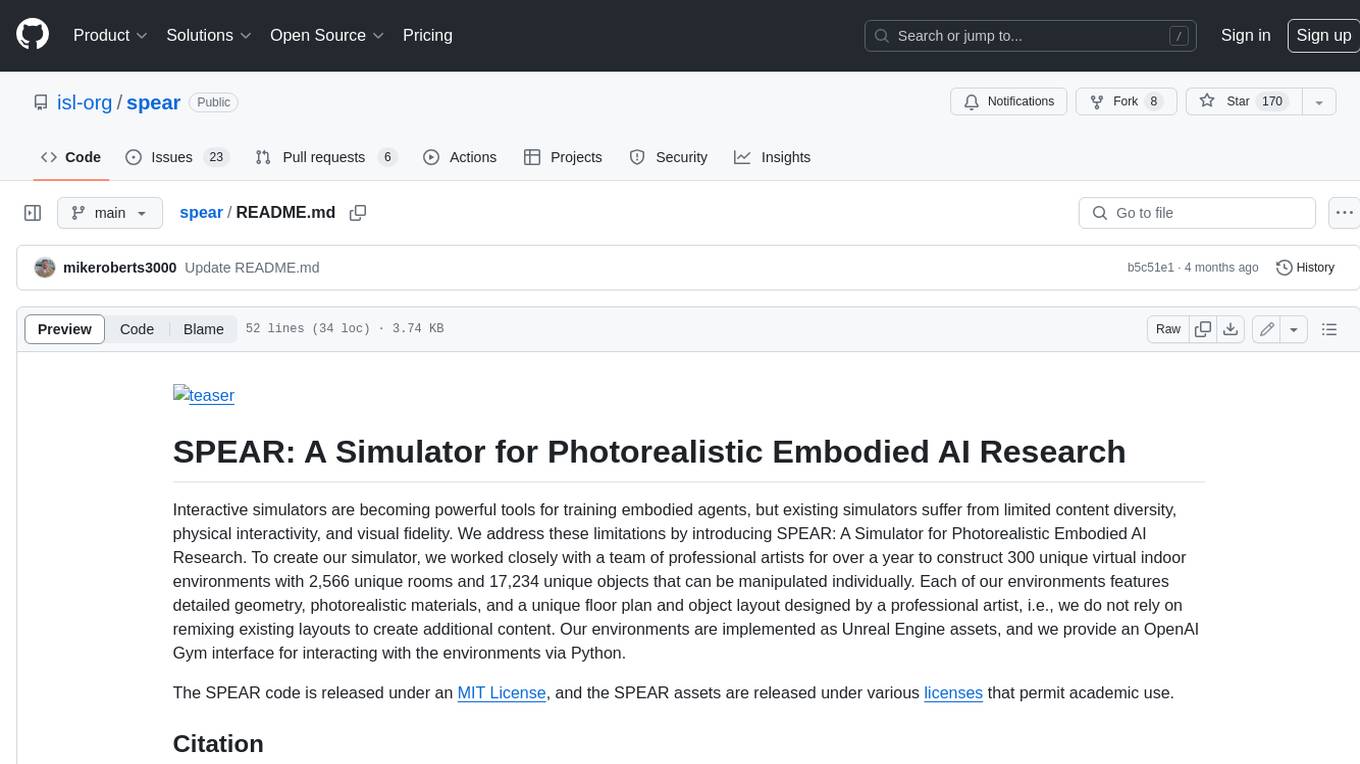
spear
SPEAR (Simulator for Photorealistic Embodied AI Research) is a powerful tool for training embodied agents. It features 300 unique virtual indoor environments with 2,566 unique rooms and 17,234 unique objects that can be manipulated individually. Each environment is designed by a professional artist and features detailed geometry, photorealistic materials, and a unique floor plan and object layout. SPEAR is implemented as Unreal Engine assets and provides an OpenAI Gym interface for interacting with the environments via Python.
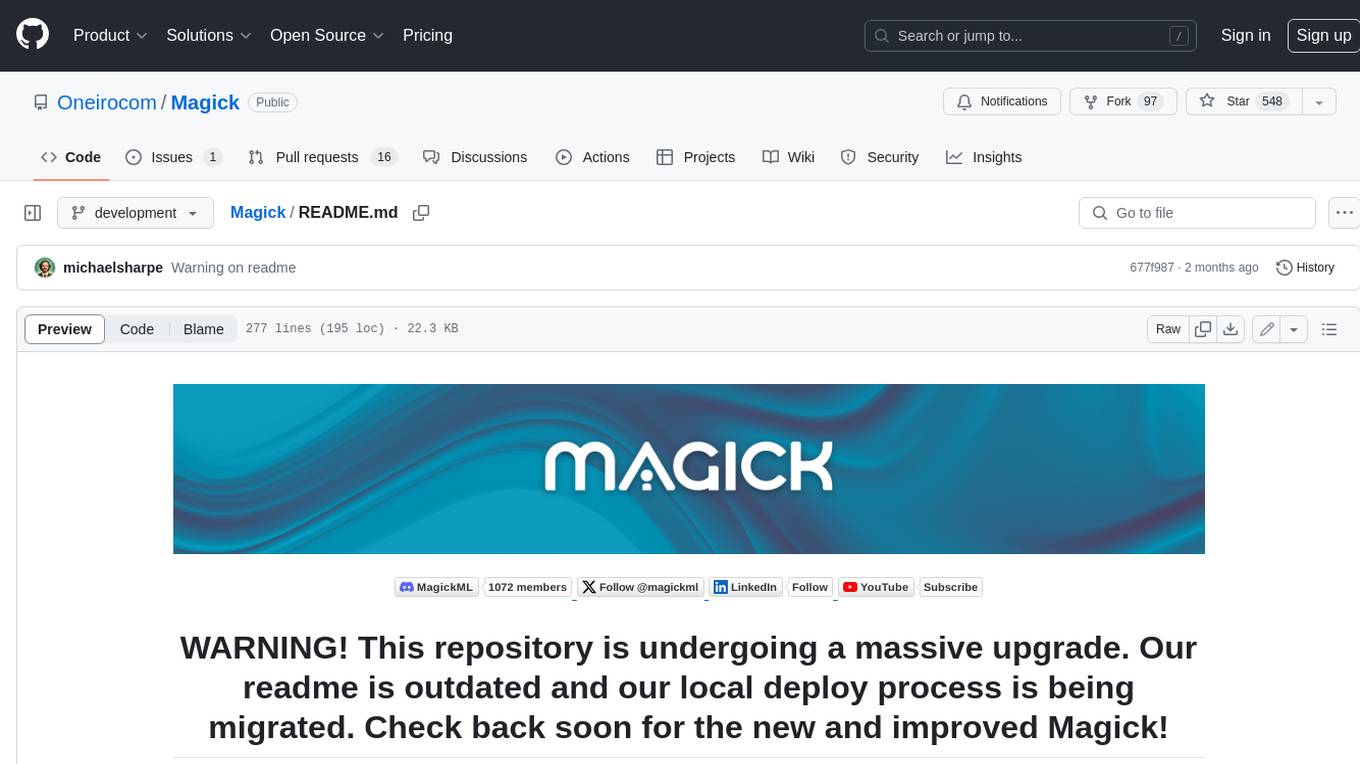
Magick
Magick is a groundbreaking visual AIDE (Artificial Intelligence Development Environment) for no-code data pipelines and multimodal agents. Magick can connect to other services and comes with nodes and templates well-suited for intelligent agents, chatbots, complex reasoning systems and realistic characters.