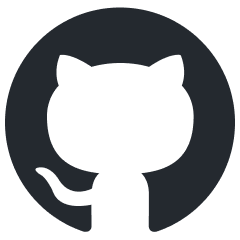
chromem-go
Embeddable vector database for Go with Chroma-like interface and zero third-party dependencies. In-memory with optional persistence.
Stars: 212
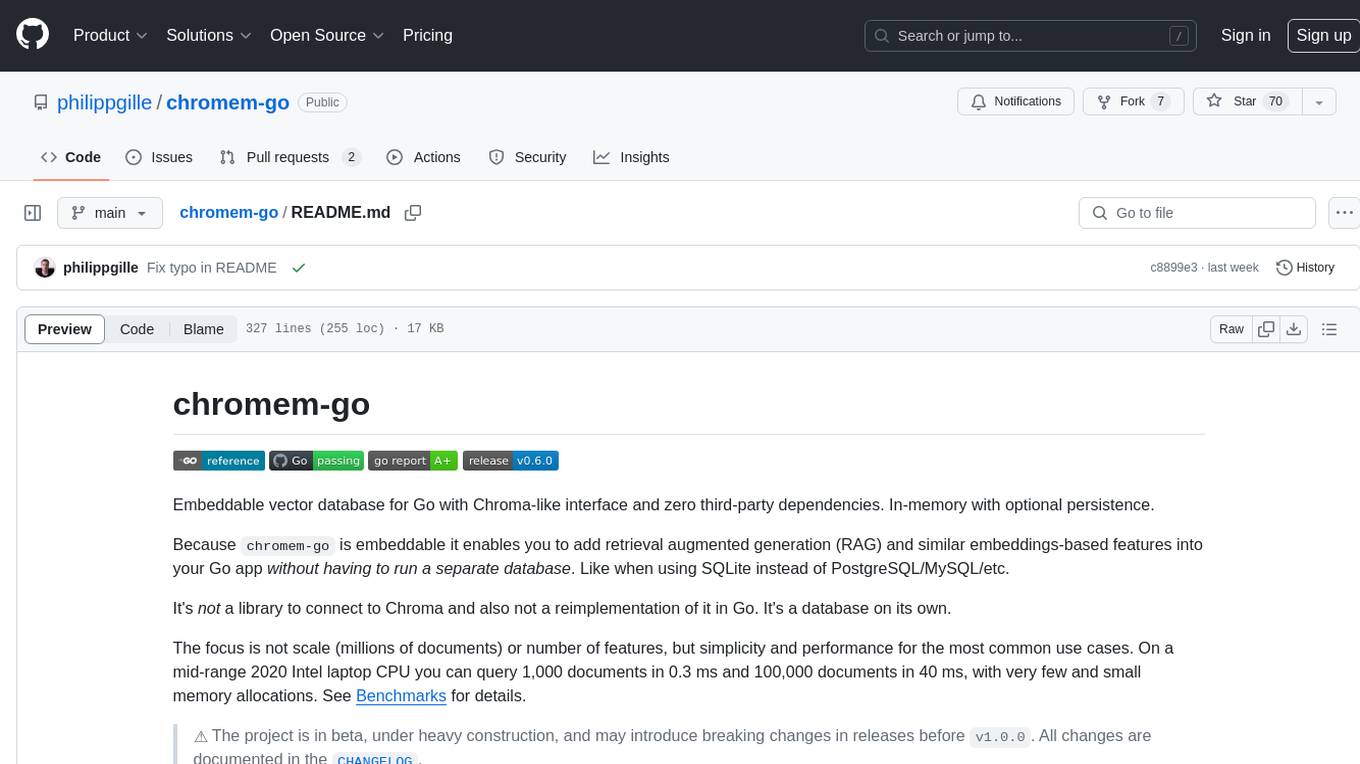
chromem-go is an embeddable vector database for Go with a Chroma-like interface and zero third-party dependencies. It enables retrieval augmented generation (RAG) and similar embeddings-based features in Go apps without the need for a separate database. The focus is on simplicity and performance for common use cases, allowing querying of documents with minimal memory allocations. The project is in beta and may introduce breaking changes before v1.0.0.
README:
Embeddable vector database for Go with Chroma-like interface and zero third-party dependencies. In-memory with optional persistence.
Because chromem-go
is embeddable it enables you to add retrieval augmented generation (RAG) and similar embeddings-based features into your Go app without having to run a separate database. Like when using SQLite instead of PostgreSQL/MySQL/etc.
It's not a library to connect to Chroma and also not a reimplementation of it in Go. It's a database on its own.
The focus is not scale (millions of documents) or number of features, but simplicity and performance for the most common use cases. On a mid-range 2020 Intel laptop CPU you can query 1,000 documents in 0.3 ms and 100,000 documents in 40 ms, with very few and small memory allocations. See Benchmarks for details.
⚠️ The project is in beta, under heavy construction, and may introduce breaking changes in releases beforev1.0.0
. All changes are documented in theCHANGELOG
.
- Use cases
- Interface
- Features + Roadmap
- Installation
- Usage
- Benchmarks
- Development
- Motivation
- Related projects
With a vector database you can do various things:
- Retrieval augmented generation (RAG), question answering (Q&A)
- Text and code search
- Recommendation systems
- Classification
- Clustering
Let's look at the RAG use case in more detail:
The knowledge of large language models (LLMs) - even the ones with 30 billion, 70 billion parameters and more - is limited. They don't know anything about what happened after their training ended, they don't know anything about data they were not trained with (like your company's intranet, Jira / bug tracker, wiki or other kinds of knowledge bases), and even the data they do know they often can't reproduce it exactly, but start to hallucinate instead.
Fine-tuning an LLM can help a bit, but it's more meant to improve the LLMs reasoning about specific topics, or reproduce the style of written text or code. Fine-tuning does not add knowledge 1:1 into the model. Details are lost or mixed up. And knowledge cutoff (about anything that happened after the fine-tuning) isn't solved either.
=> A vector database can act as the up-to-date, precise knowledge for LLMs:
- You store relevant documents that you want the LLM to know in the database.
- The database stores the embeddings alongside the documents, which you can either provide or can be created by specific "embedding models" like OpenAI's
text-embedding-3-small
.-
chromem-go
can do this for you and supports multiple embedding providers and models out-of-the-box.
-
- Later, when you want to talk to the LLM, you first send the question to the vector DB to find similar/related content. This is called "nearest neighbor search".
- In the question to the LLM, you provide this content alongside your question.
- The LLM can take this up-to-date precise content into account when answering.
Check out the example code to see it in action!
Our original inspiration was the Chroma interface, whose core API is the following (taken from their README):
Chroma core interface
import chromadb
# setup Chroma in-memory, for easy prototyping. Can add persistence easily!
client = chromadb.Client()
# Create collection. get_collection, get_or_create_collection, delete_collection also available!
collection = client.create_collection("all-my-documents")
# Add docs to the collection. Can also update and delete. Row-based API coming soon!
collection.add(
documents=["This is document1", "This is document2"], # we handle tokenization, embedding, and indexing automatically. You can skip that and add your own embeddings as well
metadatas=[{"source": "notion"}, {"source": "google-docs"}], # filter on these!
ids=["doc1", "doc2"], # unique for each doc
)
# Query/search 2 most similar results. You can also .get by id
results = collection.query(
query_texts=["This is a query document"],
n_results=2,
# where={"metadata_field": "is_equal_to_this"}, # optional filter
# where_document={"$contains":"search_string"} # optional filter
)
Our Go library exposes the same interface:
chromem-go equivalent
package main
import "github.com/philippgille/chromem-go"
func main() {
// Set up chromem-go in-memory, for easy prototyping. Can add persistence easily!
// We call it DB instead of client because there's no client-server separation. The DB is embedded.
db := chromem.NewDB()
// Create collection. GetCollection, GetOrCreateCollection, DeleteCollection also available!
collection, _ := db.CreateCollection("all-my-documents", nil, nil)
// Add docs to the collection. Update and delete will be added in the future.
// Can be multi-threaded with AddConcurrently()!
// We're showing the Chroma-like method here, but more Go-idiomatic methods are also available!
_ = collection.Add(ctx,
[]string{"doc1", "doc2"}, // unique ID for each doc
nil, // We handle embedding automatically. You can skip that and add your own embeddings as well.
[]map[string]string{{"source": "notion"}, {"source": "google-docs"}}, // Filter on these!
[]string{"This is document1", "This is document2"},
)
// Query/search 2 most similar results. Getting by ID will be added in the future.
results, _ := collection.Query(ctx,
"This is a query document",
2,
map[string]string{"metadata_field": "is_equal_to_this"}, // optional filter
map[string]string{"$contains": "search_string"}, // optional filter
)
}
Initially chromem-go
started with just the four core methods, but we added more over time. We intentionally don't want to cover 100% of Chroma's API surface though.
We're providing some alternative methods that are more Go-idiomatic instead.
For the full interface see the Godoc: https://pkg.go.dev/github.com/philippgille/chromem-go
- [X] Zero dependencies on third party libraries
- [X] Embeddable (like SQLite, i.e. no client-server model, no separate DB to maintain)
- [X] Multithreaded processing (when adding and querying documents), making use of Go's native concurrency features
- [X] Experimental WebAssembly binding
- Embedding creators:
- Hosted:
- [X] OpenAI (default)
- [X] Azure OpenAI
- [X] Cohere
- [X] Mistral
- [X] Jina
- [X] mixedbread.ai
- Local:
- Bring your own (implement
chromem.EmbeddingFunc
) - You can also pass existing embeddings when adding documents to a collection, instead of letting
chromem-go
create them
- Hosted:
- Similarity search:
- [X] Exhaustive nearest neighbor search using cosine similarity (sometimes also called exact search or brute-force search or FLAT index)
- Filters:
- [X] Document filters:
$contains
,$not_contains
- [X] Metadata filters: Exact matches
- [X] Document filters:
- Storage:
- [X] In-memory
- [X] Optional immediate persistence (writes one file for each added collection and document, encoded as gob, optionally gzip-compressed)
- [X] Backups: Export and import of the entire DB to/from a single file (encoded as gob, optionally gzip-compressed and AES-GCM encrypted)
- Includes methods for generic
io.Writer
/io.Reader
so you can plug S3 buckets and other blob storage, see examples/s3-export-import for example code
- Includes methods for generic
- Data types:
- [X] Documents (text)
- Performance:
- Use SIMD for dot product calculation on supported CPUs (draft PR: #48)
- Add roaring bitmaps to speed up full text filtering
- Embedding creators:
- Add an
EmbeddingFunc
that downloads and shells out to llamafile
- Add an
- Similarity search:
- Approximate nearest neighbor search with index (ANN)
- Hierarchical Navigable Small World (HNSW)
- Inverted file flat (IVFFlat)
- Approximate nearest neighbor search with index (ANN)
- Filters:
- Operators (
$and
,$or
etc.)
- Operators (
- Storage:
- JSON as second encoding format
- Write-ahead log (WAL) as second file format
- Optional remote storage (S3, PostgreSQL, ...)
- Data types:
- Images
- Videos
go get github.com/philippgille/chromem-go@latest
See the Godoc for a reference: https://pkg.go.dev/github.com/philippgille/chromem-go
For full, working examples, using the vector database for retrieval augmented generation (RAG) and semantic search and using either OpenAI or locally running the embeddings model and LLM (in Ollama), see the example code.
This is taken from the "minimal" example:
package main
import (
"context"
"fmt"
"runtime"
"github.com/philippgille/chromem-go"
)
func main() {
ctx := context.Background()
db := chromem.NewDB()
c, err := db.CreateCollection("knowledge-base", nil, nil)
if err != nil {
panic(err)
}
err = c.AddDocuments(ctx, []chromem.Document{
{
ID: "1",
Content: "The sky is blue because of Rayleigh scattering.",
},
{
ID: "2",
Content: "Leaves are green because chlorophyll absorbs red and blue light.",
},
}, runtime.NumCPU())
if err != nil {
panic(err)
}
res, err := c.Query(ctx, "Why is the sky blue?", 1, nil, nil)
if err != nil {
panic(err)
}
fmt.Printf("ID: %v\nSimilarity: %v\nContent: %v\n", res[0].ID, res[0].Similarity, res[0].Content)
}
Output:
ID: 1
Similarity: 0.6833369
Content: The sky is blue because of Rayleigh scattering.
Benchmarked on 2024-03-17 with:
- Computer: Framework Laptop 13 (first generation, 2021)
- CPU: 11th Gen Intel Core i5-1135G7 (2020)
- Memory: 32 GB
- OS: Fedora Linux 39
- Kernel: 6.7
$ go test -benchmem -run=^$ -bench .
goos: linux
goarch: amd64
pkg: github.com/philippgille/chromem-go
cpu: 11th Gen Intel(R) Core(TM) i5-1135G7 @ 2.40GHz
BenchmarkCollection_Query_NoContent_100-8 13164 90276 ns/op 5176 B/op 95 allocs/op
BenchmarkCollection_Query_NoContent_1000-8 2142 520261 ns/op 13558 B/op 141 allocs/op
BenchmarkCollection_Query_NoContent_5000-8 561 2150354 ns/op 47096 B/op 173 allocs/op
BenchmarkCollection_Query_NoContent_25000-8 120 9890177 ns/op 211783 B/op 208 allocs/op
BenchmarkCollection_Query_NoContent_100000-8 30 39574238 ns/op 810370 B/op 232 allocs/op
BenchmarkCollection_Query_100-8 13225 91058 ns/op 5177 B/op 95 allocs/op
BenchmarkCollection_Query_1000-8 2226 519693 ns/op 13552 B/op 140 allocs/op
BenchmarkCollection_Query_5000-8 550 2128121 ns/op 47108 B/op 173 allocs/op
BenchmarkCollection_Query_25000-8 100 10063260 ns/op 211705 B/op 205 allocs/op
BenchmarkCollection_Query_100000-8 30 39404005 ns/op 810295 B/op 229 allocs/op
PASS
ok github.com/philippgille/chromem-go 28.402s
- Build:
go build ./...
- Test:
go test -v -race -count 1 ./...
- Benchmark:
-
go test -benchmem -run=^$ -bench .
(add> bench.out
or similar to write to a file) - With profiling:
go test -benchmem -run ^$ -cpuprofile cpu.out -bench .
- (profiles:
-cpuprofile
,-memprofile
,-blockprofile
,-mutexprofile
)
- (profiles:
-
- Compare benchmarks:
- Install
benchstat
:go install golang.org/x/perf/cmd/benchstat@latest
- Compare two benchmark results:
benchstat before.out after.out
- Install
In December 2023, when I wanted to play around with retrieval augmented generation (RAG) in a Go program, I looked for a vector database that could be embedded in the Go program, just like you would embed SQLite in order to not require any separate DB setup and maintenance. I was surprised when I didn't find any, given the abundance of embedded key-value stores in the Go ecosystem.
At the time most of the popular vector databases like Pinecone, Qdrant, Milvus, Chroma, Weaviate and others were not embeddable at all or only in Python or JavaScript/TypeScript.
Then I found @eliben's blog post and example code which showed that with very little Go code you could create a very basic PoC of a vector database.
That's when I decided to build my own vector database, embeddable in Go, inspired by the ChromaDB interface. ChromaDB stood out for being embeddable (in Python), and by showing its core API in 4 commands on their README and on the landing page of their website.
- Shoutout to @eliben whose blog post and example code inspired me to start this project!
- Chroma: Looking at Pinecone, Qdrant, Milvus, Weaviate and others, Chroma stood out by showing its core API in 4 commands on their README and on the landing page of their website. It was also putting the most emphasis on its embeddability (in Python).
- The big, full-fledged client-server-based vector databases for maximum scale and performance:
- Some non-specialized SQL, NoSQL and Key-Value databases added support for storing vectors and (some of them) querying based on similarity:
- pgvector extension for PostgreSQL: Client-server model
- Redis (1, 2): Client-server model
- sqlite-vss extension for SQLite: Embedded, but the Go bindings require CGO. There's a CGO-free Go library for SQLite, but then it's without the vector search extension.
- DuckDB has a function to calculate cosine similarity (1): Embedded, but the Go bindings use CGO
- MongoDB's cloud platform offers a vector search product (1): Client-server model
- Some libraries for vector similarity search:
- Some orchestration libraries, inspired by the Python library LangChain, but with no or only rudimentary embedded vector DB:
For Tasks:
Click tags to check more tools for each tasksFor Jobs:
Alternative AI tools for chromem-go
Similar Open Source Tools
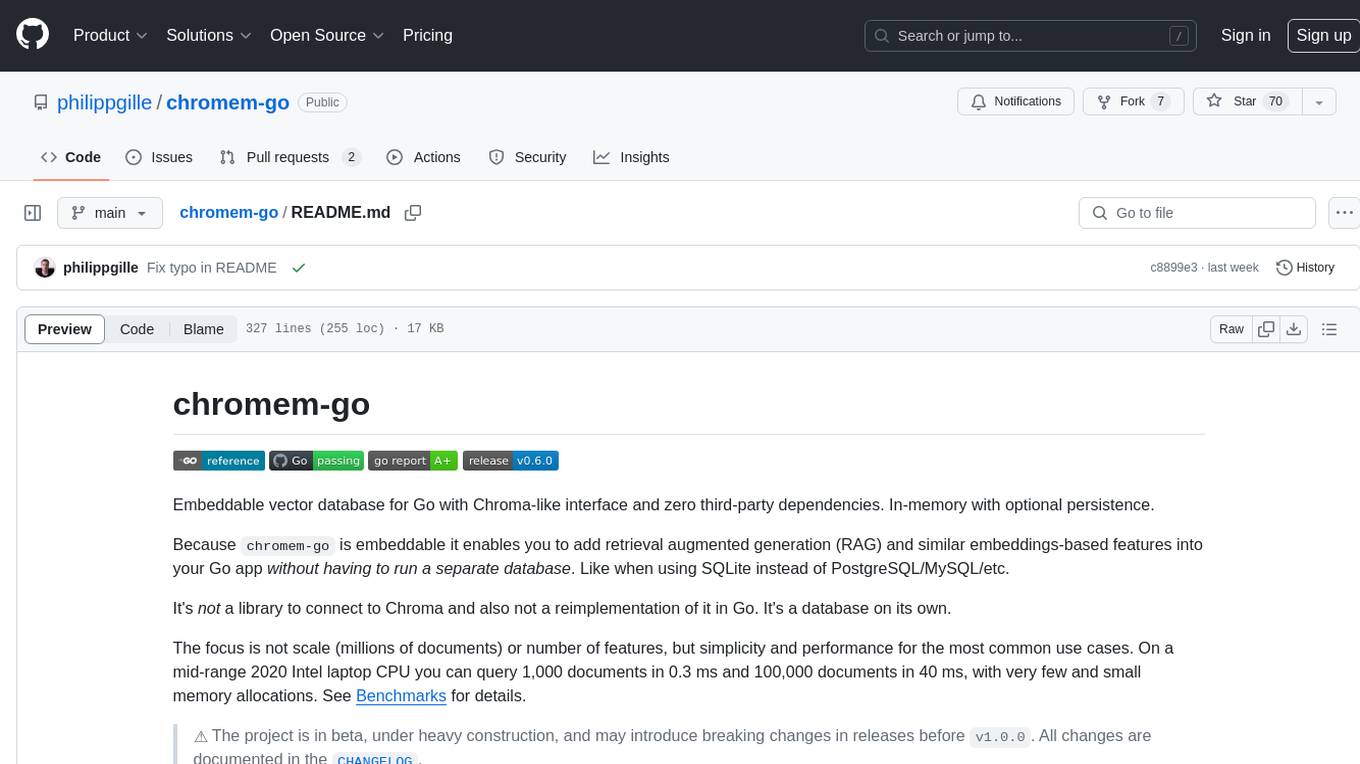
chromem-go
chromem-go is an embeddable vector database for Go with a Chroma-like interface and zero third-party dependencies. It enables retrieval augmented generation (RAG) and similar embeddings-based features in Go apps without the need for a separate database. The focus is on simplicity and performance for common use cases, allowing querying of documents with minimal memory allocations. The project is in beta and may introduce breaking changes before v1.0.0.
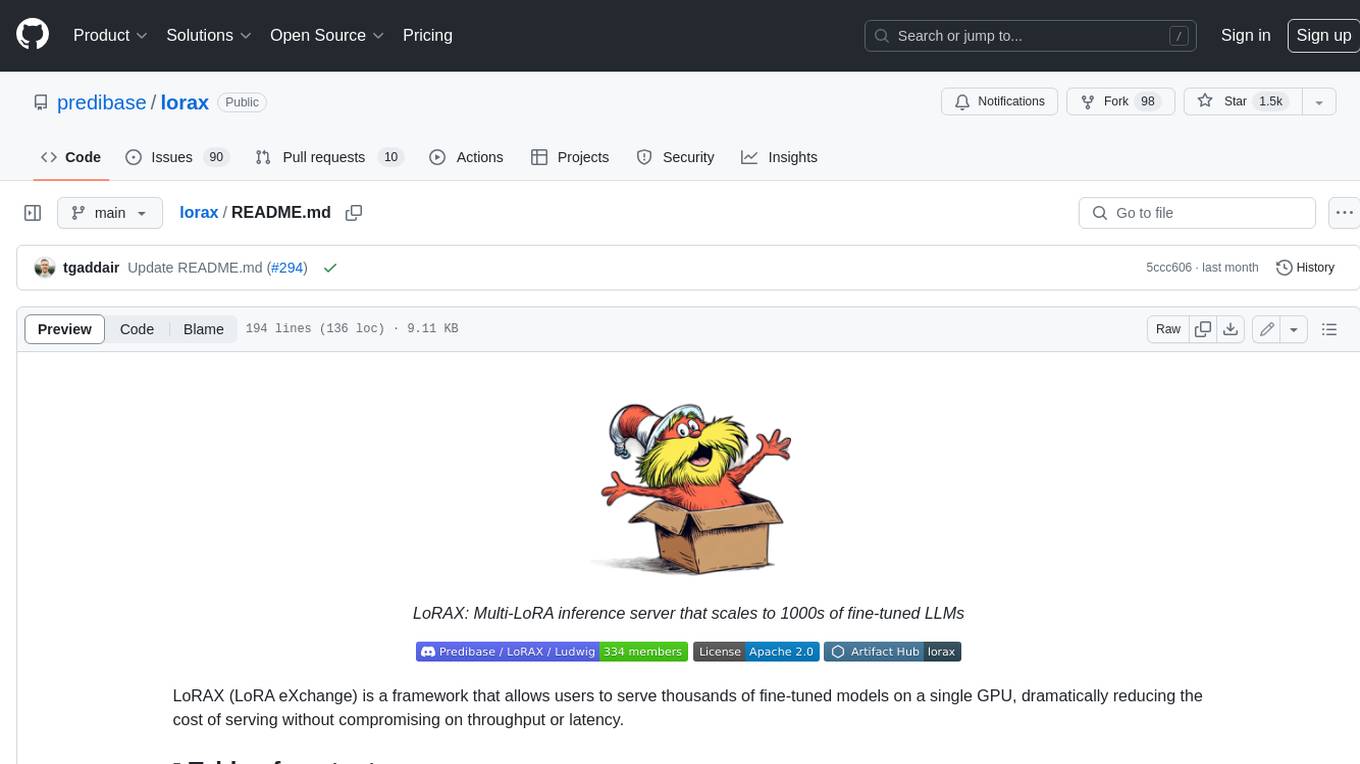
lorax
LoRAX is a framework that allows users to serve thousands of fine-tuned models on a single GPU, dramatically reducing the cost of serving without compromising on throughput or latency. It features dynamic adapter loading, heterogeneous continuous batching, adapter exchange scheduling, optimized inference, and is ready for production with prebuilt Docker images, Helm charts for Kubernetes, Prometheus metrics, and distributed tracing with Open Telemetry. LoRAX supports a number of Large Language Models as the base model including Llama, Mistral, and Qwen, and any of the linear layers in the model can be adapted via LoRA and loaded in LoRAX.
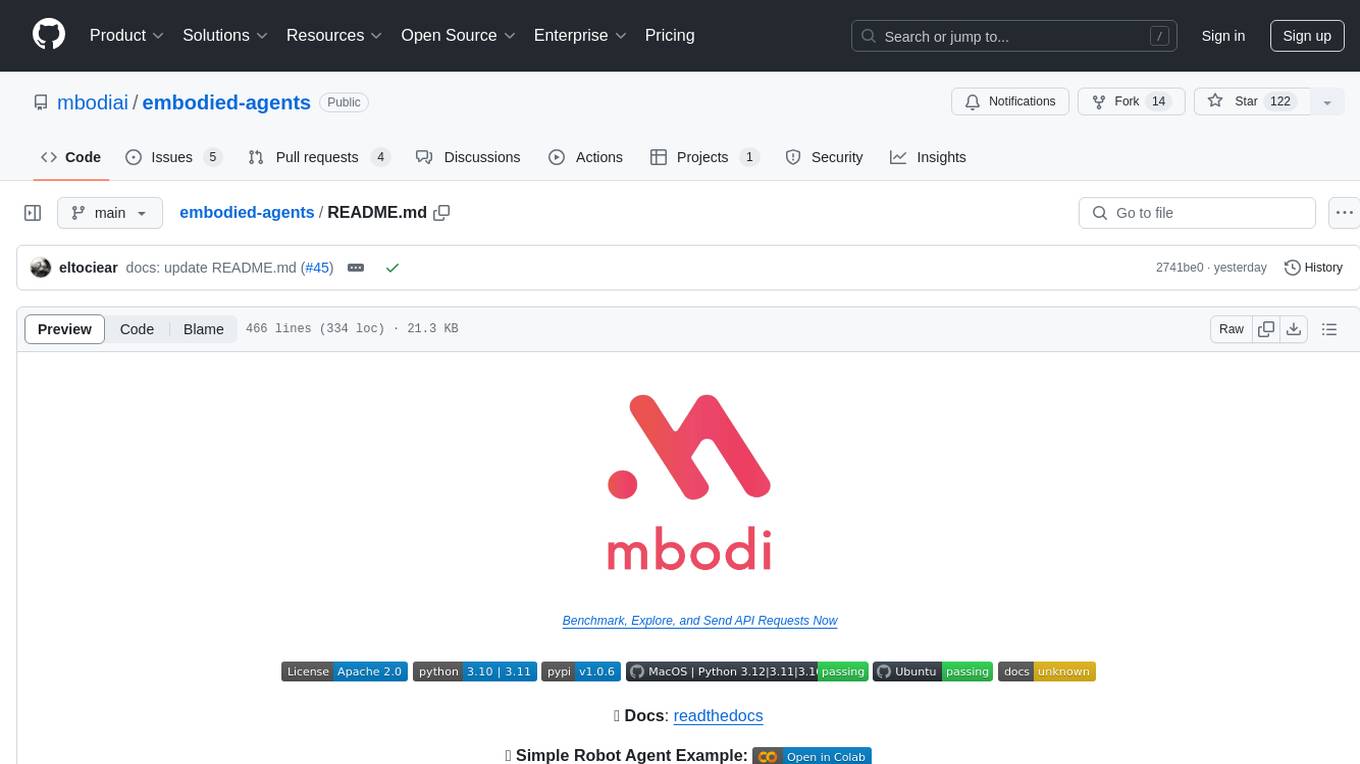
embodied-agents
Embodied Agents is a toolkit for integrating large multi-modal models into existing robot stacks with just a few lines of code. It provides consistency, reliability, scalability, and is configurable to any observation and action space. The toolkit is designed to reduce complexities involved in setting up inference endpoints, converting between different model formats, and collecting/storing datasets. It aims to facilitate data collection and sharing among roboticists by providing Python-first abstractions that are modular, extensible, and applicable to a wide range of tasks. The toolkit supports asynchronous and remote thread-safe agent execution for maximal responsiveness and scalability, and is compatible with various APIs like HuggingFace Spaces, Datasets, Gymnasium Spaces, Ollama, and OpenAI. It also offers automatic dataset recording and optional uploads to the HuggingFace hub.
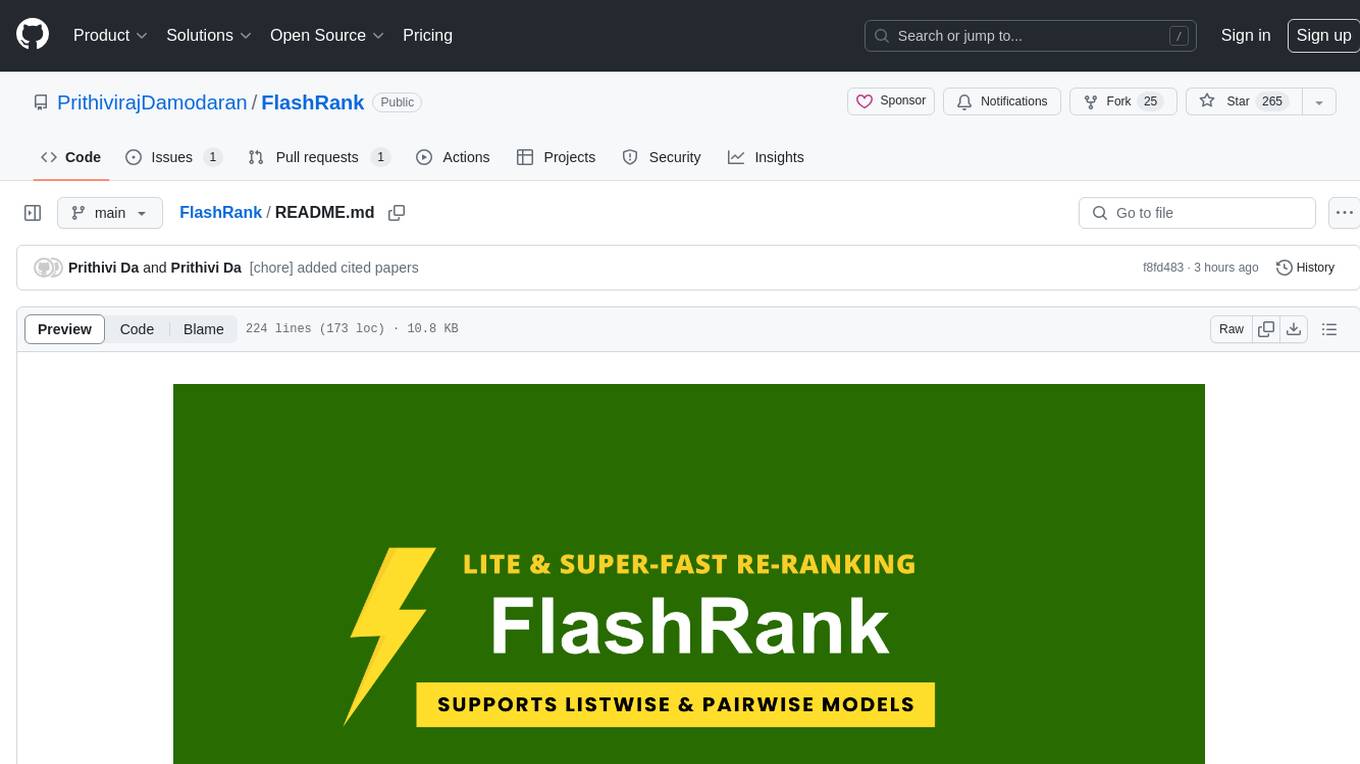
FlashRank
FlashRank is an ultra-lite and super-fast Python library designed to add re-ranking capabilities to existing search and retrieval pipelines. It is based on state-of-the-art Language Models (LLMs) and cross-encoders, offering support for pairwise/pointwise rerankers and listwise LLM-based rerankers. The library boasts the tiniest reranking model in the world (~4MB) and runs on CPU without the need for Torch or Transformers. FlashRank is cost-conscious, with a focus on low cost per invocation and smaller package size for efficient serverless deployments. It supports various models like ms-marco-TinyBERT, ms-marco-MiniLM, rank-T5-flan, ms-marco-MultiBERT, and more, with plans for future model additions. The tool is ideal for enhancing search precision and speed in scenarios where lightweight models with competitive performance are preferred.
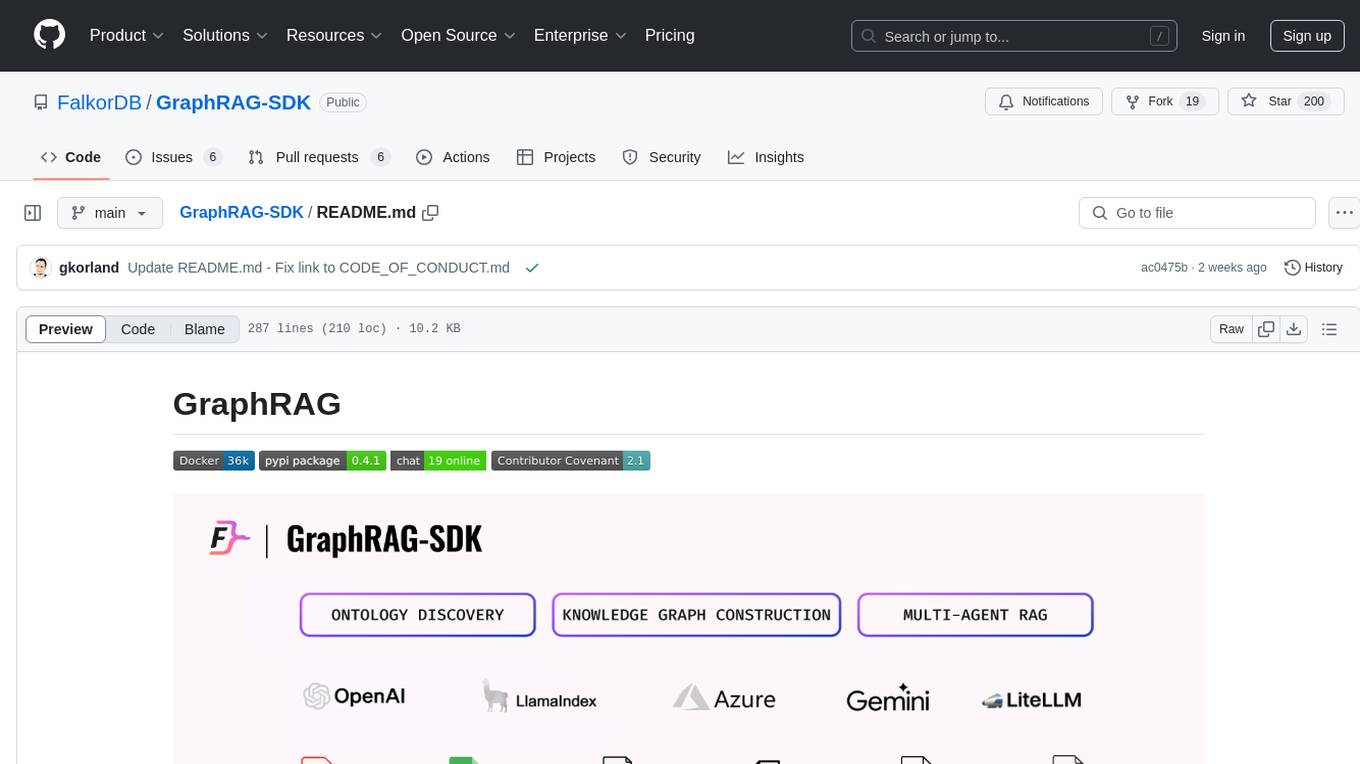
GraphRAG-SDK
Build fast and accurate GenAI applications with GraphRAG SDK, a specialized toolkit for building Graph Retrieval-Augmented Generation (GraphRAG) systems. It integrates knowledge graphs, ontology management, and state-of-the-art LLMs to deliver accurate, efficient, and customizable RAG workflows. The SDK simplifies the development process by automating ontology creation, knowledge graph agent creation, and query handling, enabling users to interact and query their knowledge graphs effectively. It supports multi-agent systems and orchestrates agents specialized in different domains. The SDK is optimized for FalkorDB, ensuring high performance and scalability for large-scale applications. By leveraging knowledge graphs, it enables semantic relationships and ontology-driven queries that go beyond standard vector similarity, enhancing retrieval-augmented generation capabilities.
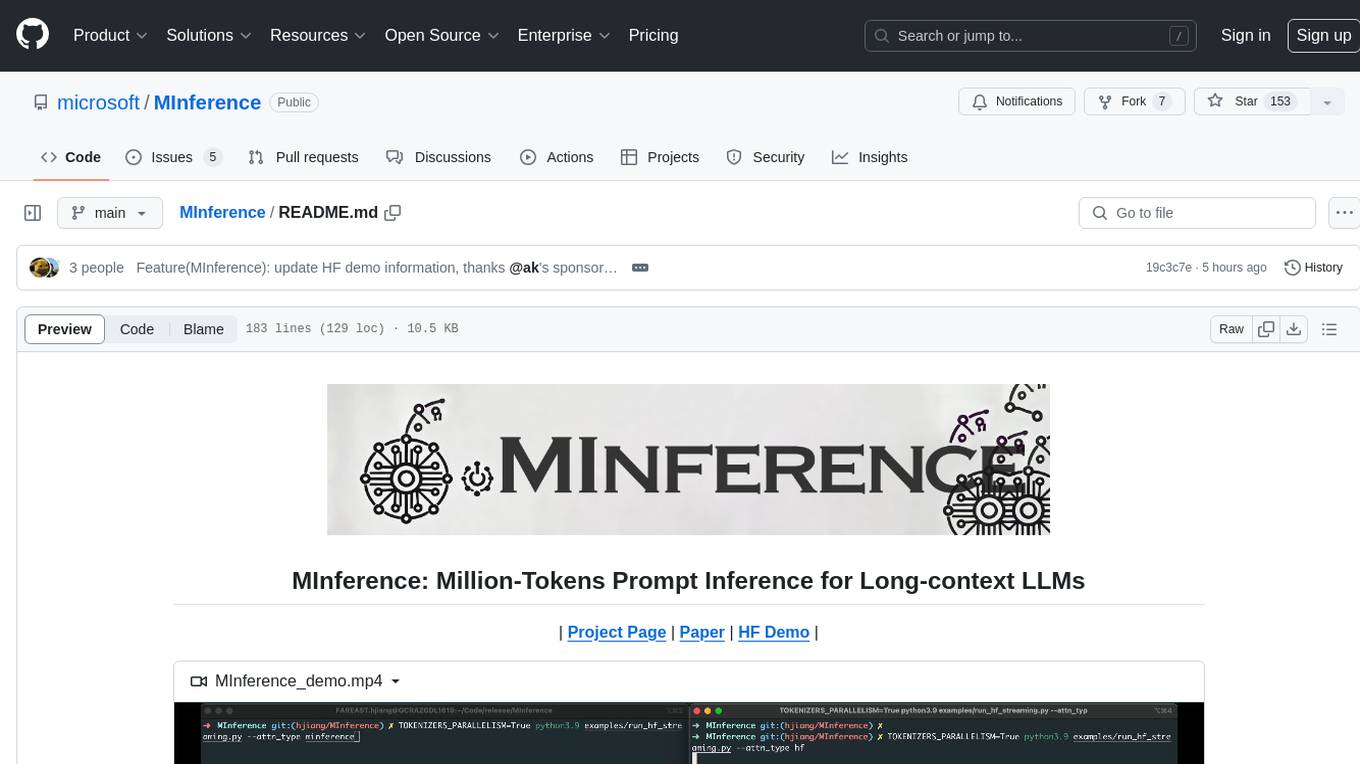
MInference
MInference is a tool designed to accelerate pre-filling for long-context Language Models (LLMs) by leveraging dynamic sparse attention. It achieves up to a 10x speedup for pre-filling on an A100 while maintaining accuracy. The tool supports various decoding LLMs, including LLaMA-style models and Phi models, and provides custom kernels for attention computation. MInference is useful for researchers and developers working with large-scale language models who aim to improve efficiency without compromising accuracy.
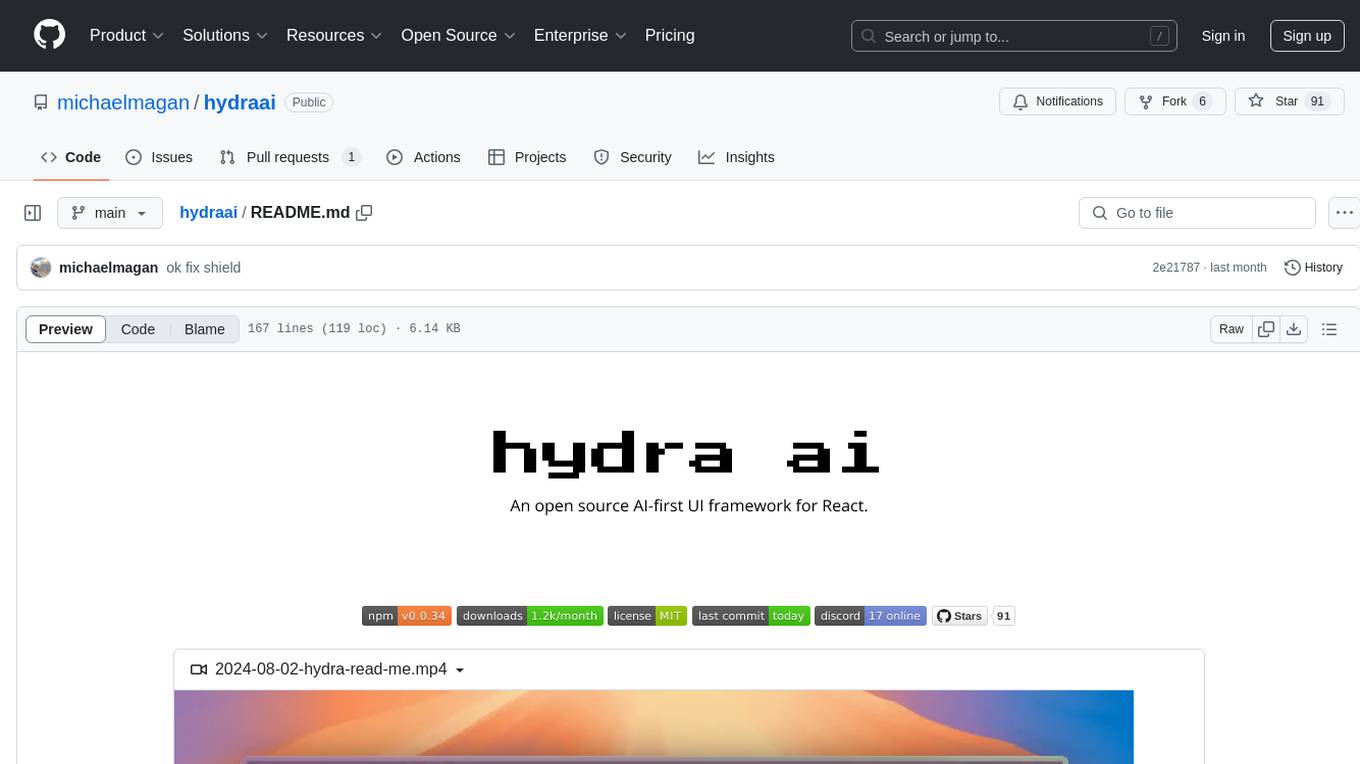
hydraai
Generate React components on-the-fly at runtime using AI. Register your components, and let Hydra choose when to show them in your App. Hydra development is still early, and patterns for different types of components and apps are still being developed. Join the discord to chat with the developers. Expects to be used in a NextJS project. Components that have function props do not work.
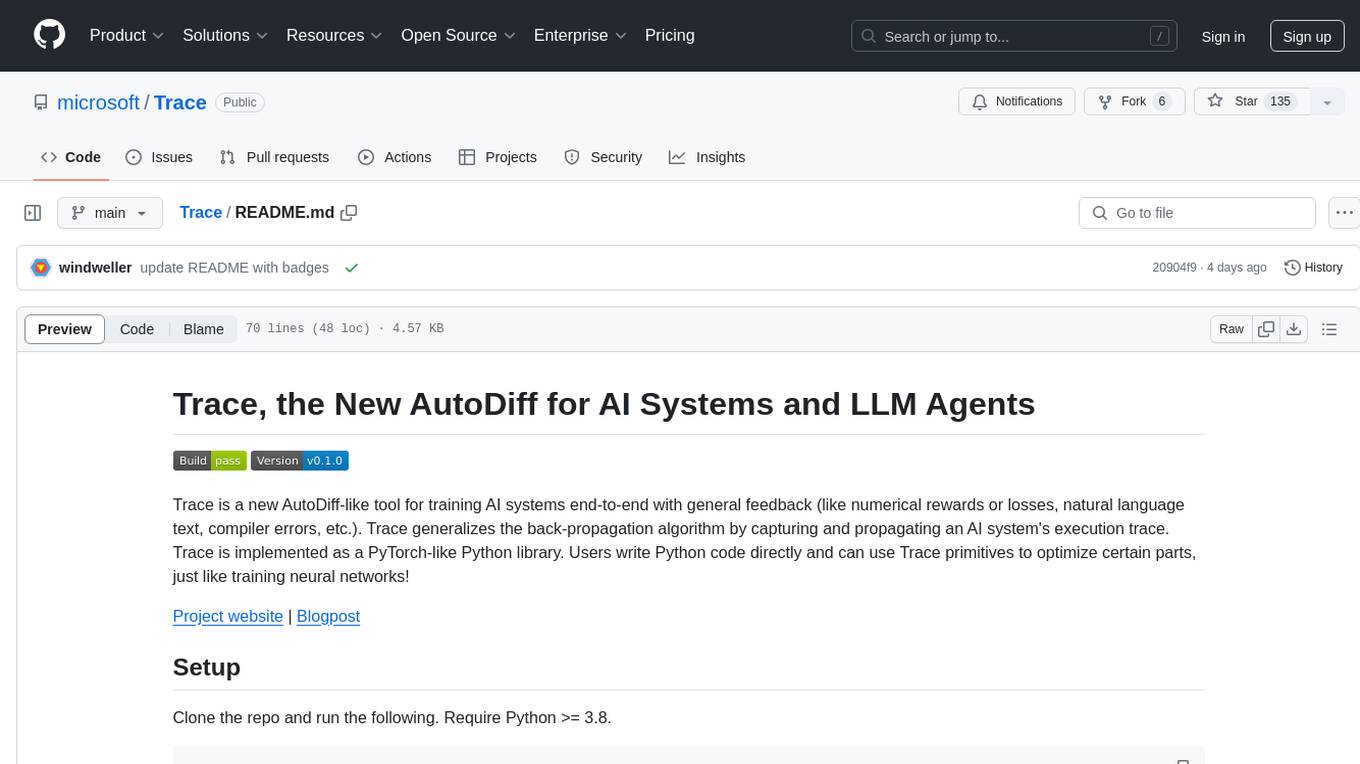
Trace
Trace is a new AutoDiff-like tool for training AI systems end-to-end with general feedback. It generalizes the back-propagation algorithm by capturing and propagating an AI system's execution trace. Implemented as a PyTorch-like Python library, users can write Python code directly and use Trace primitives to optimize certain parts, similar to training neural networks.
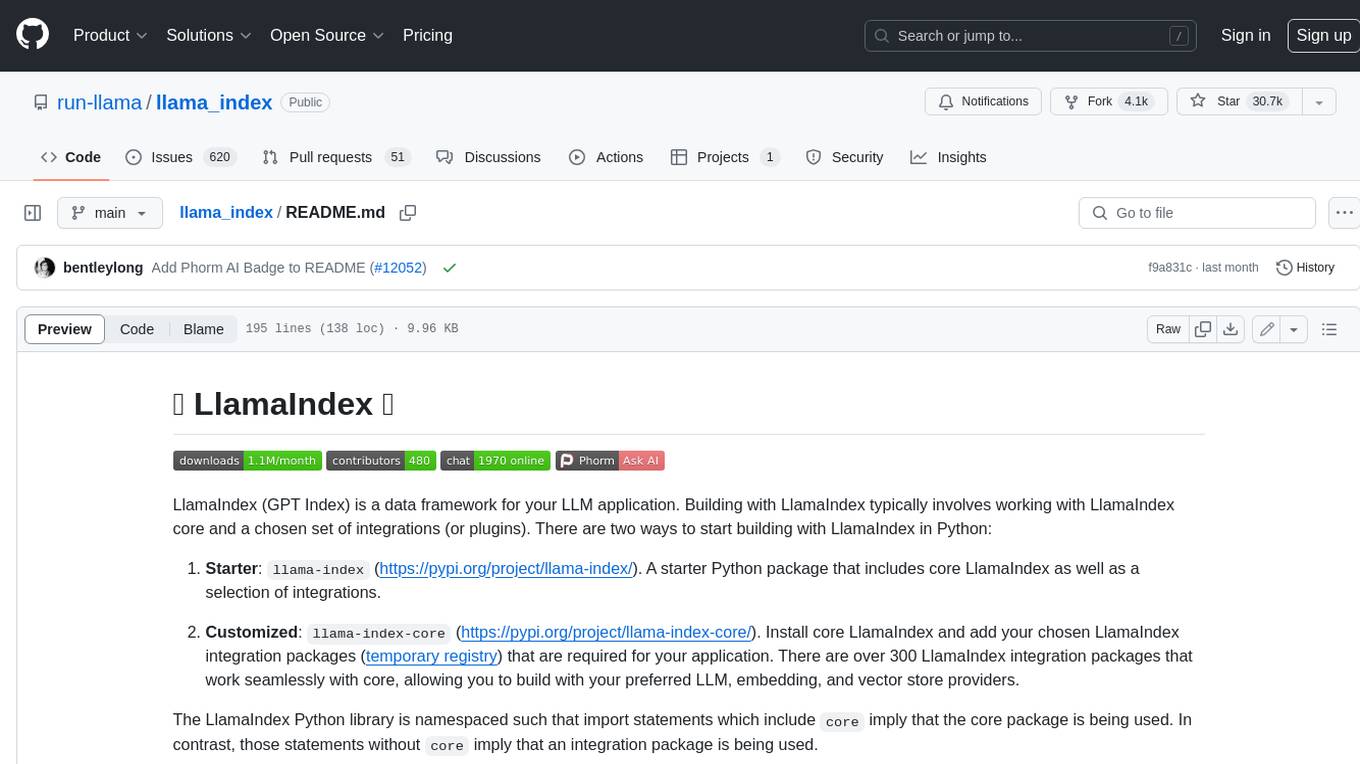
llama_index
LlamaIndex is a data framework for building LLM applications. It provides tools for ingesting, structuring, and querying data, as well as integrating with LLMs and other tools. LlamaIndex is designed to be easy to use for both beginner and advanced users, and it provides a comprehensive set of features for building LLM applications.
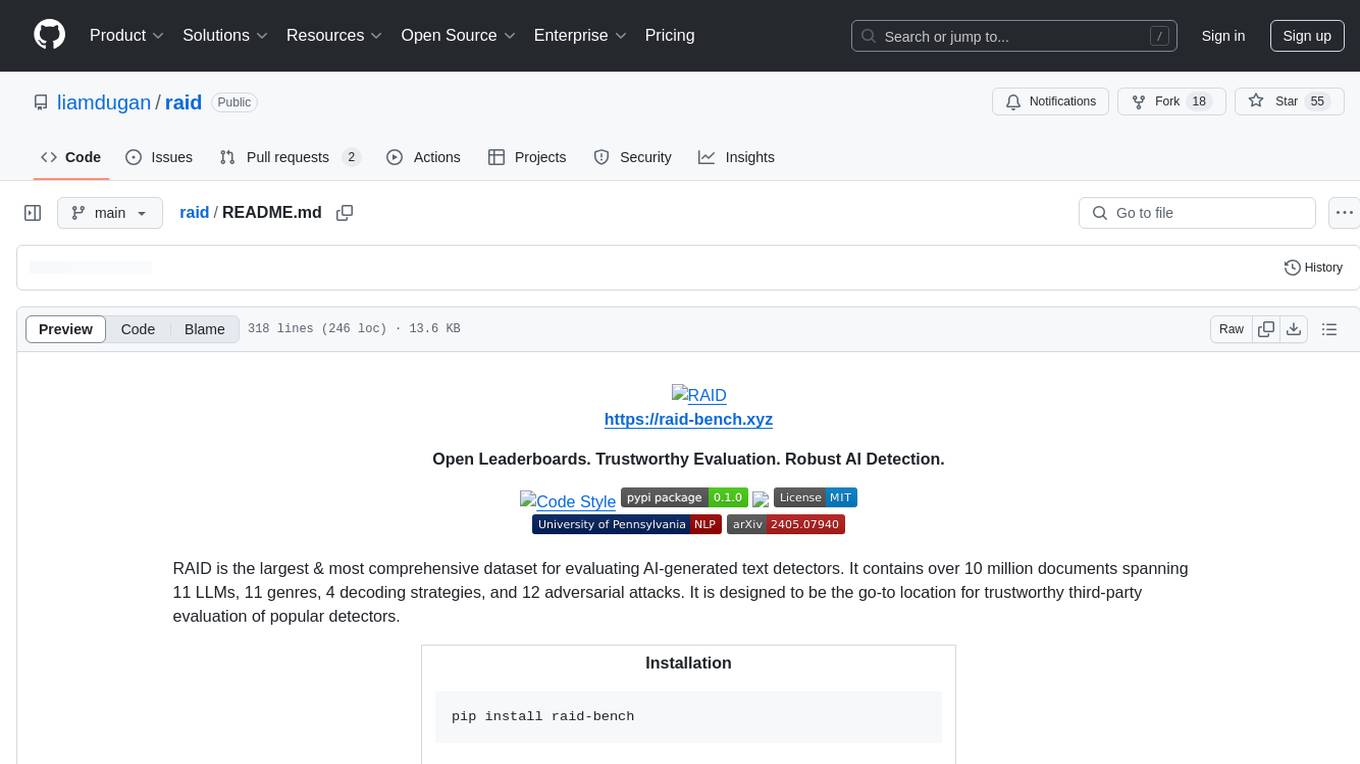
raid
RAID is the largest and most comprehensive dataset for evaluating AI-generated text detectors. It contains over 10 million documents spanning 11 LLMs, 11 genres, 4 decoding strategies, and 12 adversarial attacks. RAID is designed to be the go-to location for trustworthy third-party evaluation of popular detectors. The dataset covers diverse models, domains, sampling strategies, and attacks, making it a valuable resource for training detectors, evaluating generalization, protecting against adversaries, and comparing to state-of-the-art models from academia and industry.
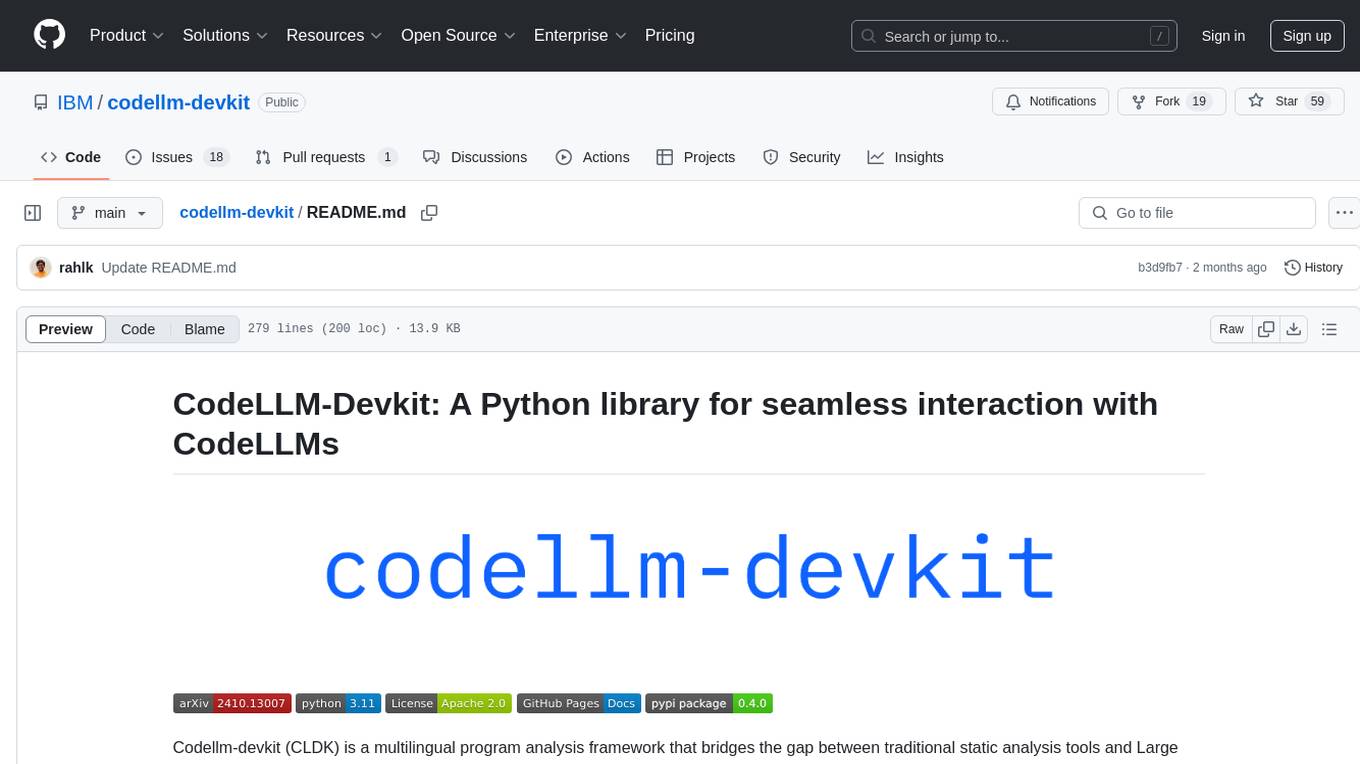
codellm-devkit
Codellm-devkit (CLDK) is a Python library that serves as a multilingual program analysis framework bridging traditional static analysis tools and Large Language Models (LLMs) specialized for code (CodeLLMs). It simplifies the process of analyzing codebases across multiple programming languages, enabling the extraction of meaningful insights and facilitating LLM-based code analysis. The library provides a unified interface for integrating outputs from various analysis tools and preparing them for effective use by CodeLLMs. Codellm-devkit aims to enable the development and experimentation of robust analysis pipelines that combine traditional program analysis tools and CodeLLMs, reducing friction in multi-language code analysis and ensuring compatibility across different tools and LLM platforms. It is designed to seamlessly integrate with popular analysis tools like WALA, Tree-sitter, LLVM, and CodeQL, acting as a crucial intermediary layer for efficient communication between these tools and CodeLLMs. The project is continuously evolving to include new tools and frameworks, maintaining its versatility for code analysis and LLM integration.
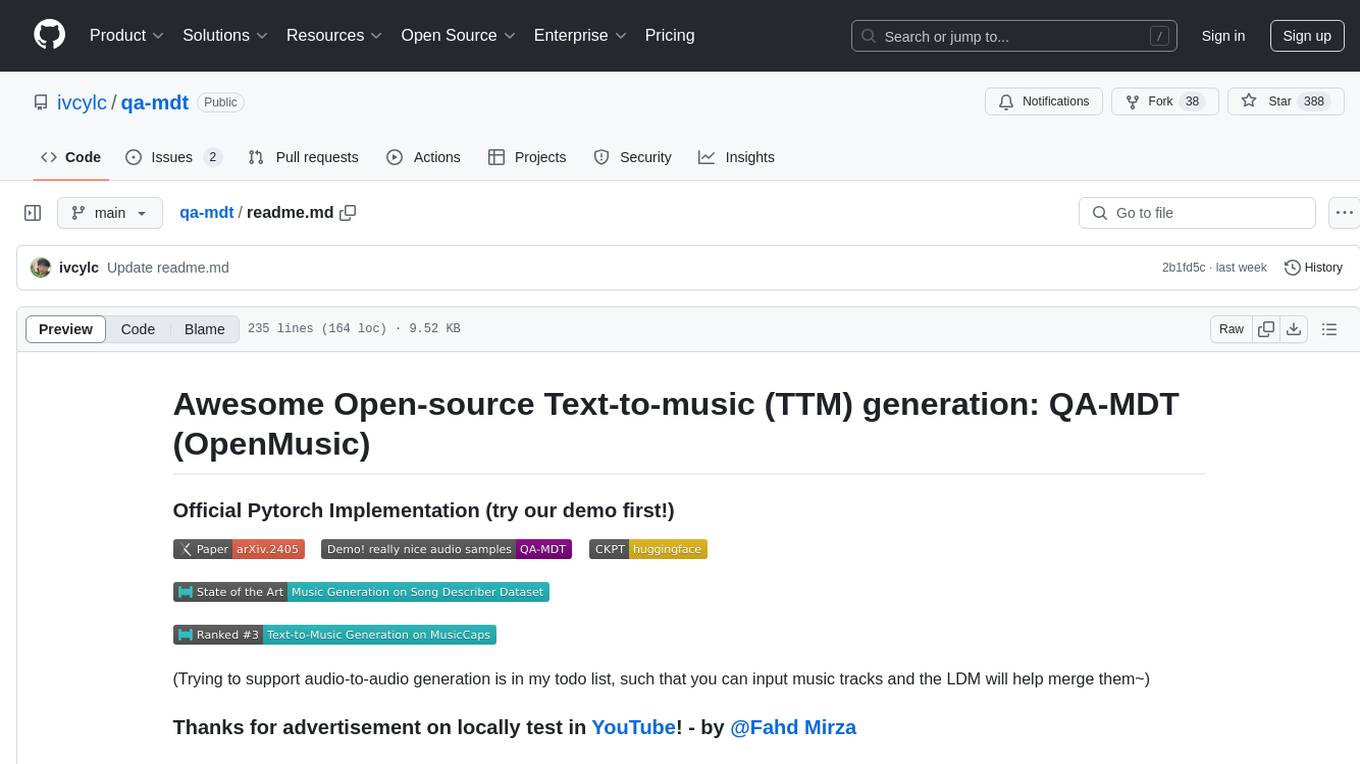
qa-mdt
This repository provides an implementation of QA-MDT, integrating state-of-the-art models for music generation. It offers a Quality-Aware Masked Diffusion Transformer for enhanced music generation. The code is based on various repositories like AudioLDM, PixArt-alpha, MDT, AudioMAE, and Open-Sora. The implementation allows for training and fine-tuning the model with different strategies and datasets. The repository also includes instructions for preparing datasets in LMDB format and provides a script for creating a toy LMDB dataset. The model can be used for music generation tasks, with a focus on quality injection to enhance the musicality of generated music.
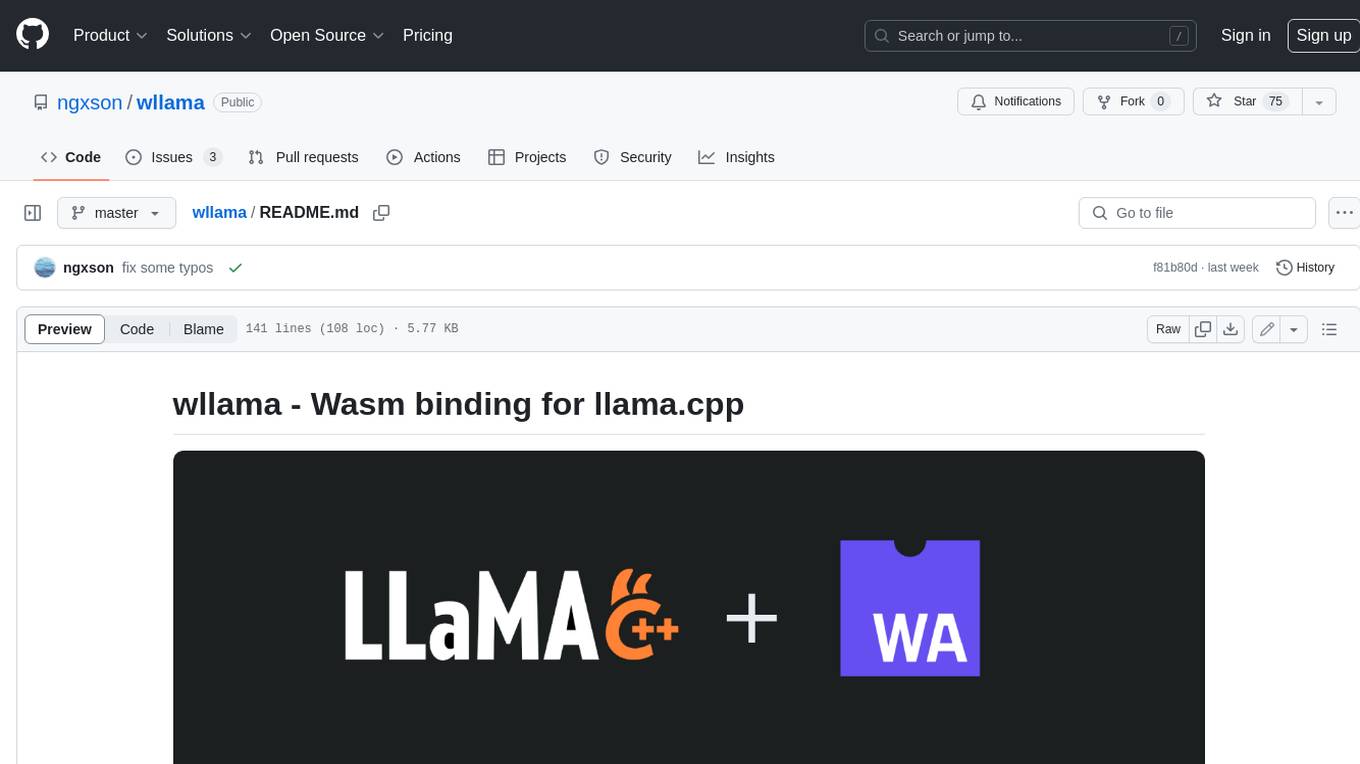
wllama
Wllama is a WebAssembly binding for llama.cpp, a high-performance and lightweight language model library. It enables you to run inference directly on the browser without the need for a backend or GPU. Wllama provides both high-level and low-level APIs, allowing you to perform various tasks such as completions, embeddings, tokenization, and more. It also supports model splitting, enabling you to load large models in parallel for faster download. With its Typescript support and pre-built npm package, Wllama is easy to integrate into your React Typescript projects.
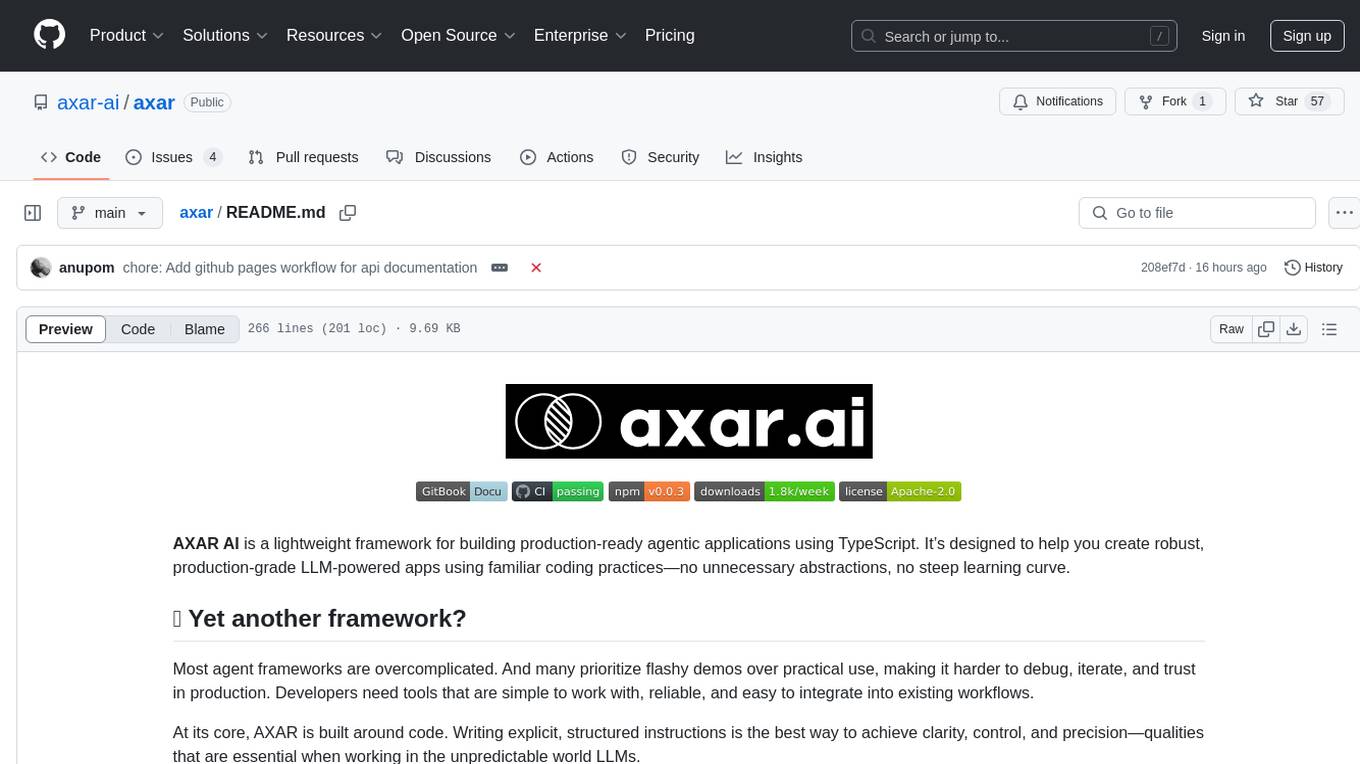
axar
AXAR AI is a lightweight framework designed for building production-ready agentic applications using TypeScript. It aims to simplify the process of creating robust, production-grade LLM-powered apps by focusing on familiar coding practices without unnecessary abstractions or steep learning curves. The framework provides structured, typed inputs and outputs, familiar and intuitive patterns like dependency injection and decorators, explicit control over agent behavior, real-time logging and monitoring tools, minimalistic design with little overhead, model agnostic compatibility with various AI models, and streamed outputs for fast and accurate results. AXAR AI is ideal for developers working on real-world AI applications who want a tool that gets out of the way and allows them to focus on shipping reliable software.
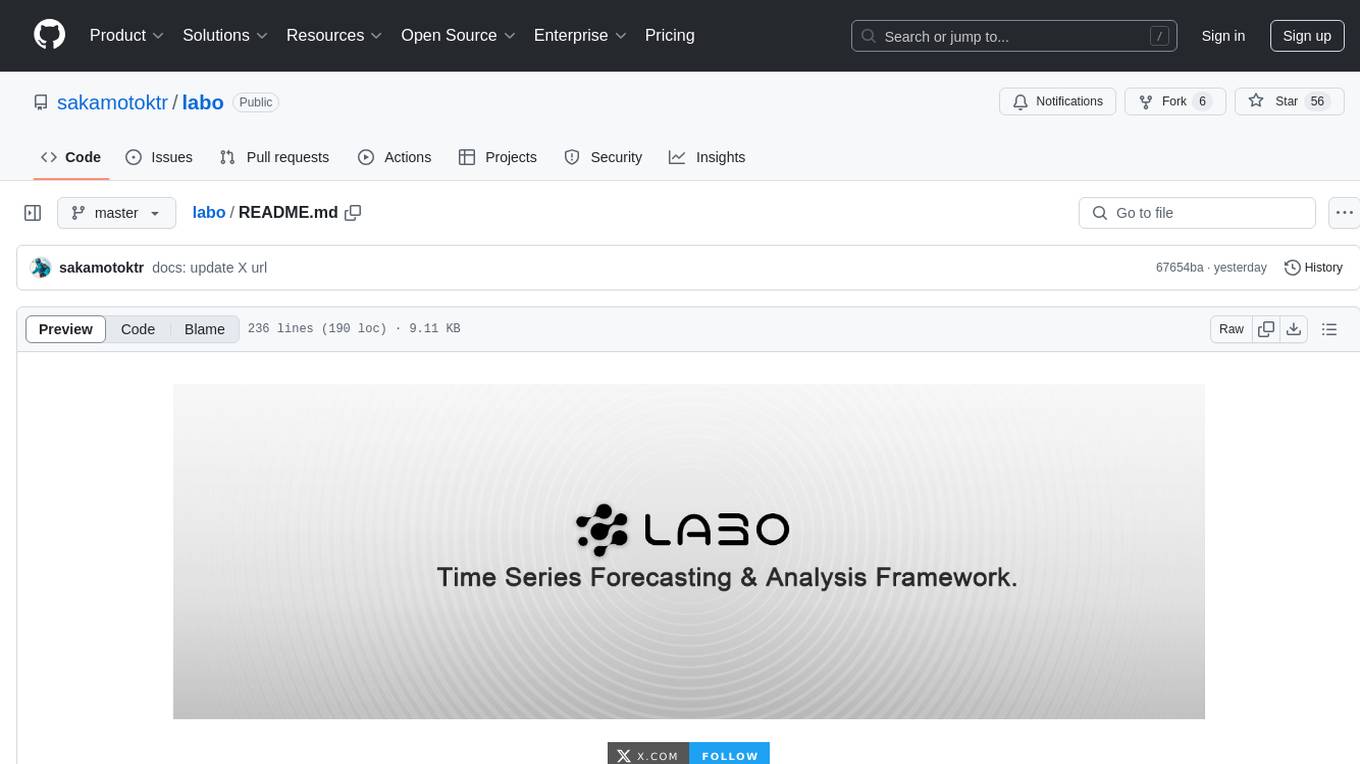
labo
LABO is a time series forecasting and analysis framework that integrates pre-trained and fine-tuned LLMs with multi-domain agent-based systems. It allows users to create and tune agents easily for various scenarios, such as stock market trend prediction and web public opinion analysis. LABO requires a specific runtime environment setup, including system requirements, Python environment, dependency installations, and configurations. Users can fine-tune their own models using LABO's Low-Rank Adaptation (LoRA) for computational efficiency and continuous model updates. Additionally, LABO provides a Python library for building model training pipelines and customizing agents for specific tasks.
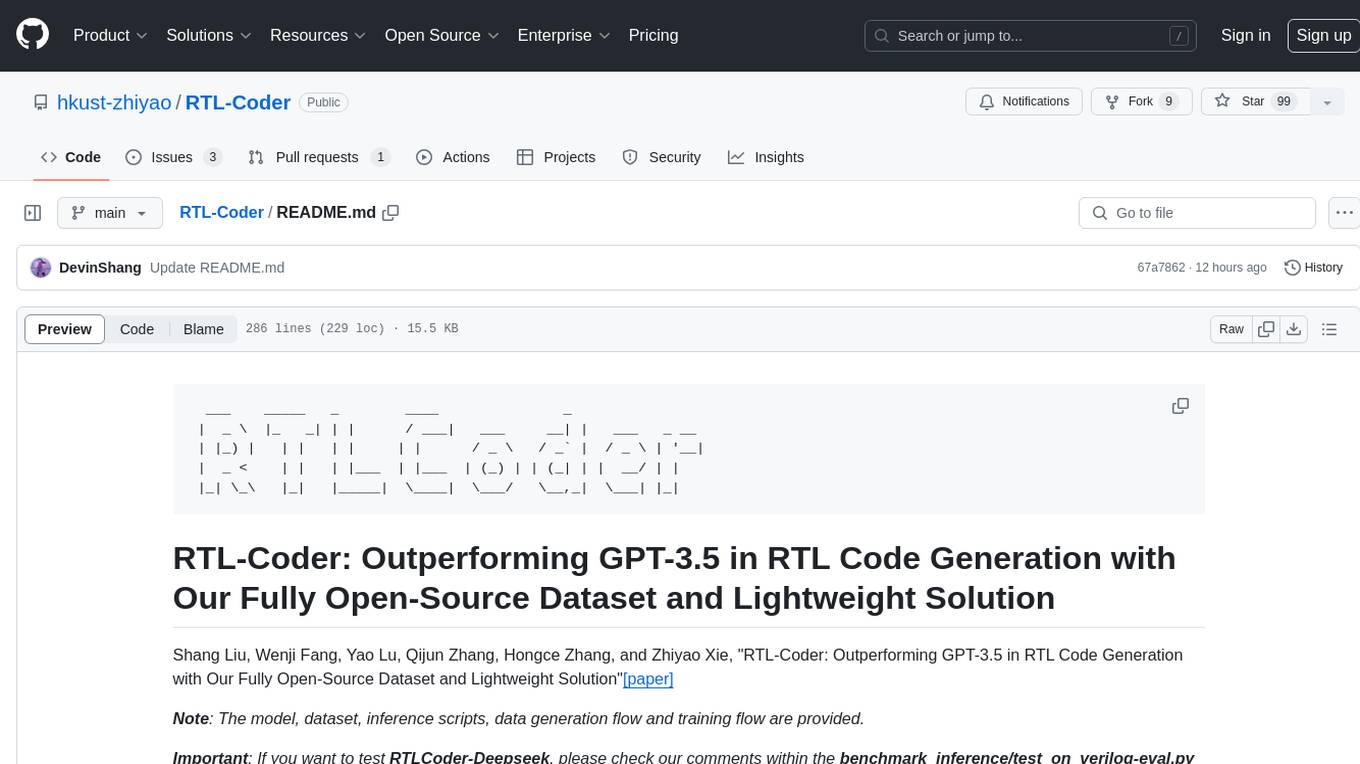
RTL-Coder
RTL-Coder is a tool designed to outperform GPT-3.5 in RTL code generation by providing a fully open-source dataset and a lightweight solution. It targets Verilog code generation and offers an automated flow to generate a large labeled dataset with over 27,000 diverse Verilog design problems and answers. The tool addresses the data availability challenge in IC design-related tasks and can be used for various applications beyond LLMs. The tool includes four RTL code generation models available on the HuggingFace platform, each with specific features and performance characteristics. Additionally, RTL-Coder introduces a new LLM training scheme based on code quality feedback to further enhance model performance and reduce GPU memory consumption.
For similar tasks
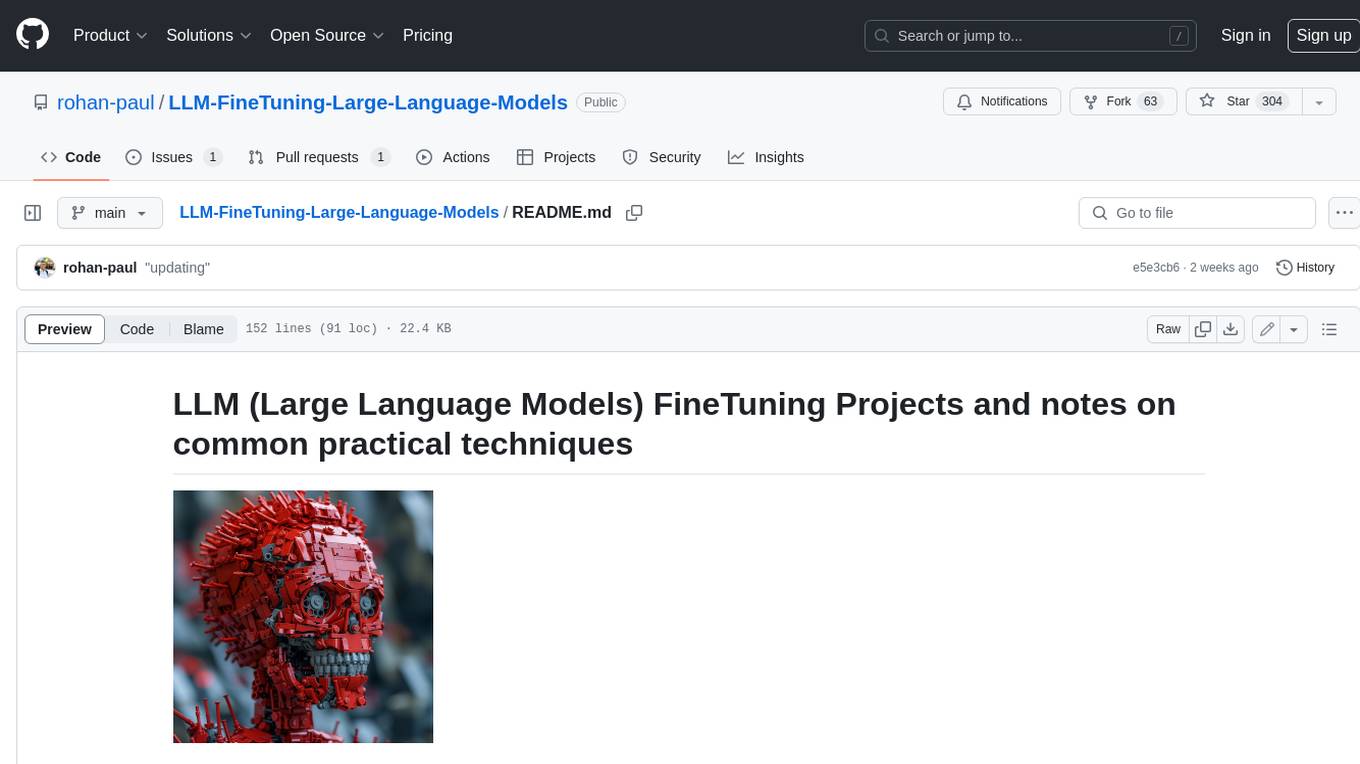
LLM-FineTuning-Large-Language-Models
This repository contains projects and notes on common practical techniques for fine-tuning Large Language Models (LLMs). It includes fine-tuning LLM notebooks, Colab links, LLM techniques and utils, and other smaller language models. The repository also provides links to YouTube videos explaining the concepts and techniques discussed in the notebooks.
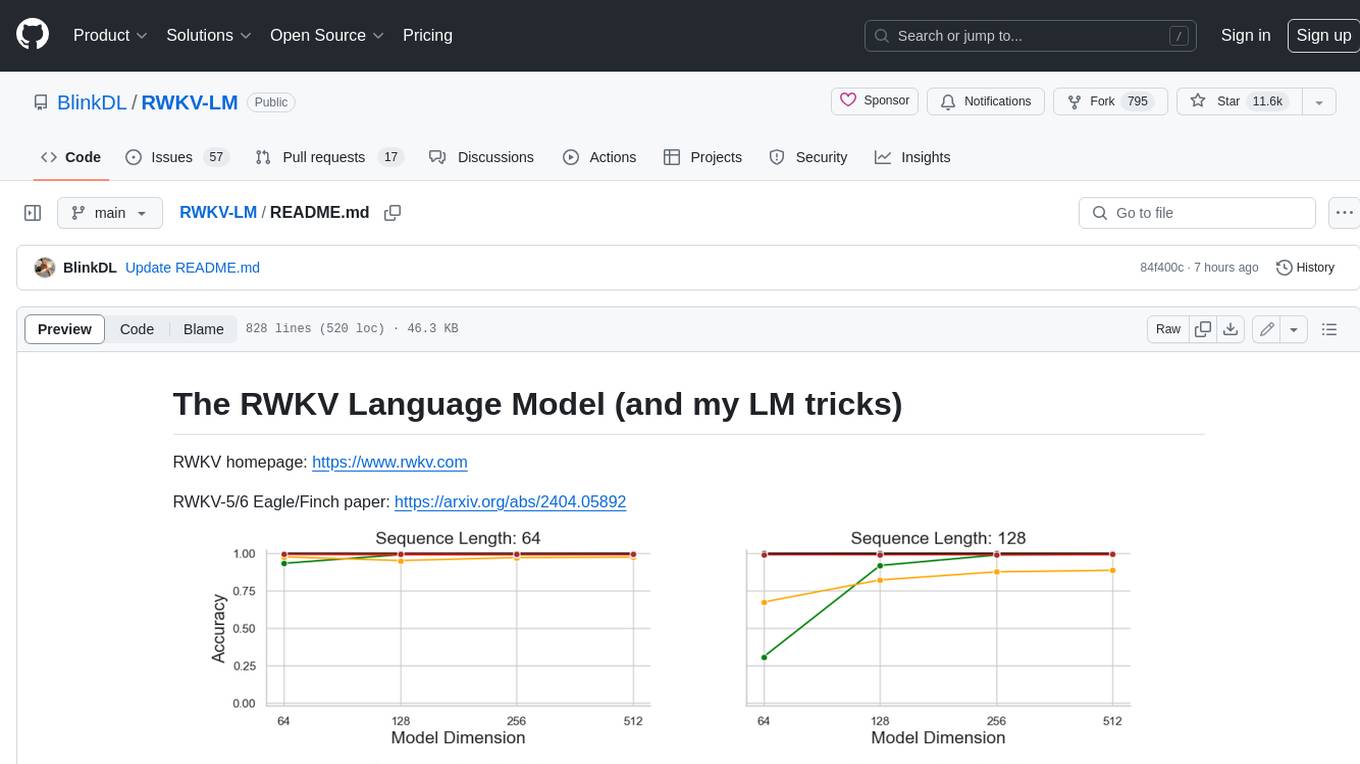
RWKV-LM
RWKV is an RNN with Transformer-level LLM performance, which can also be directly trained like a GPT transformer (parallelizable). And it's 100% attention-free. You only need the hidden state at position t to compute the state at position t+1. You can use the "GPT" mode to quickly compute the hidden state for the "RNN" mode. So it's combining the best of RNN and transformer - **great performance, fast inference, saves VRAM, fast training, "infinite" ctx_len, and free sentence embedding** (using the final hidden state).
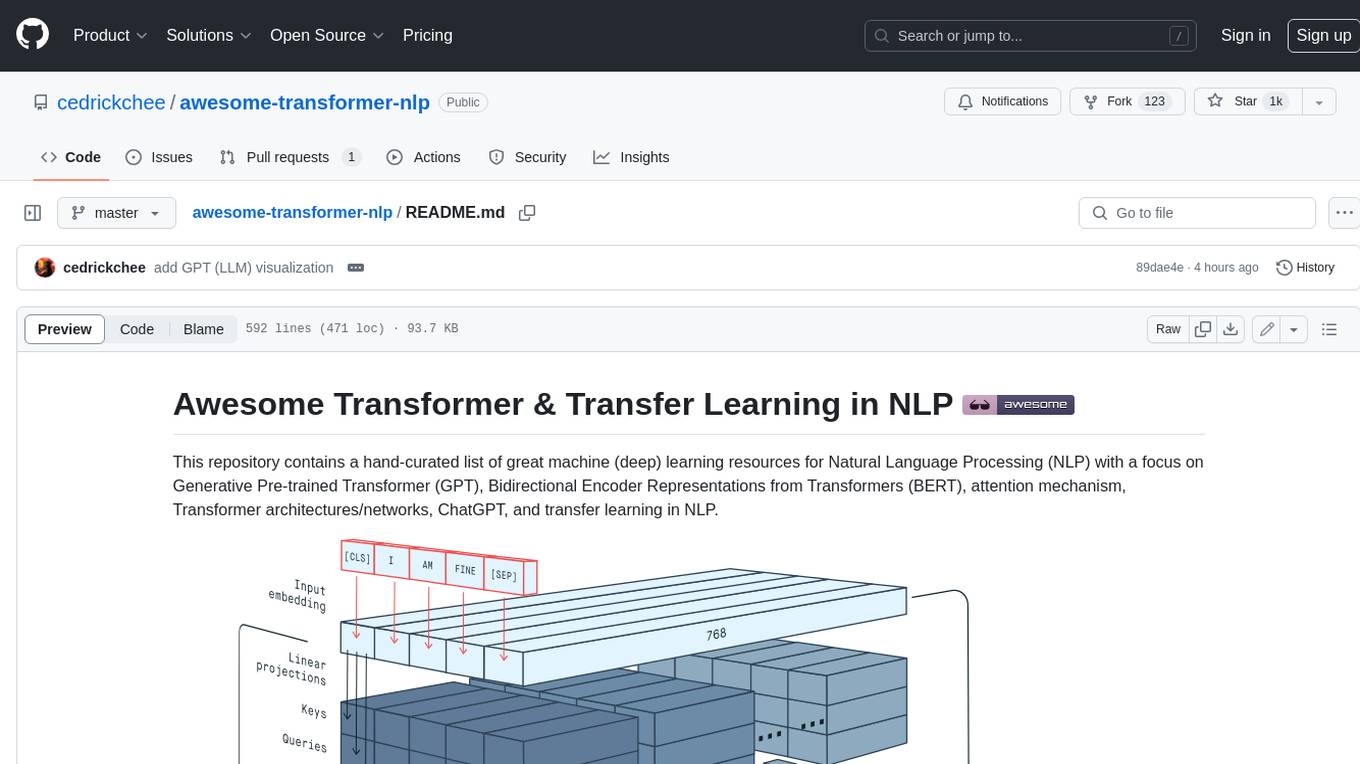
awesome-transformer-nlp
This repository contains a hand-curated list of great machine (deep) learning resources for Natural Language Processing (NLP) with a focus on Generative Pre-trained Transformer (GPT), Bidirectional Encoder Representations from Transformers (BERT), attention mechanism, Transformer architectures/networks, Chatbot, and transfer learning in NLP.
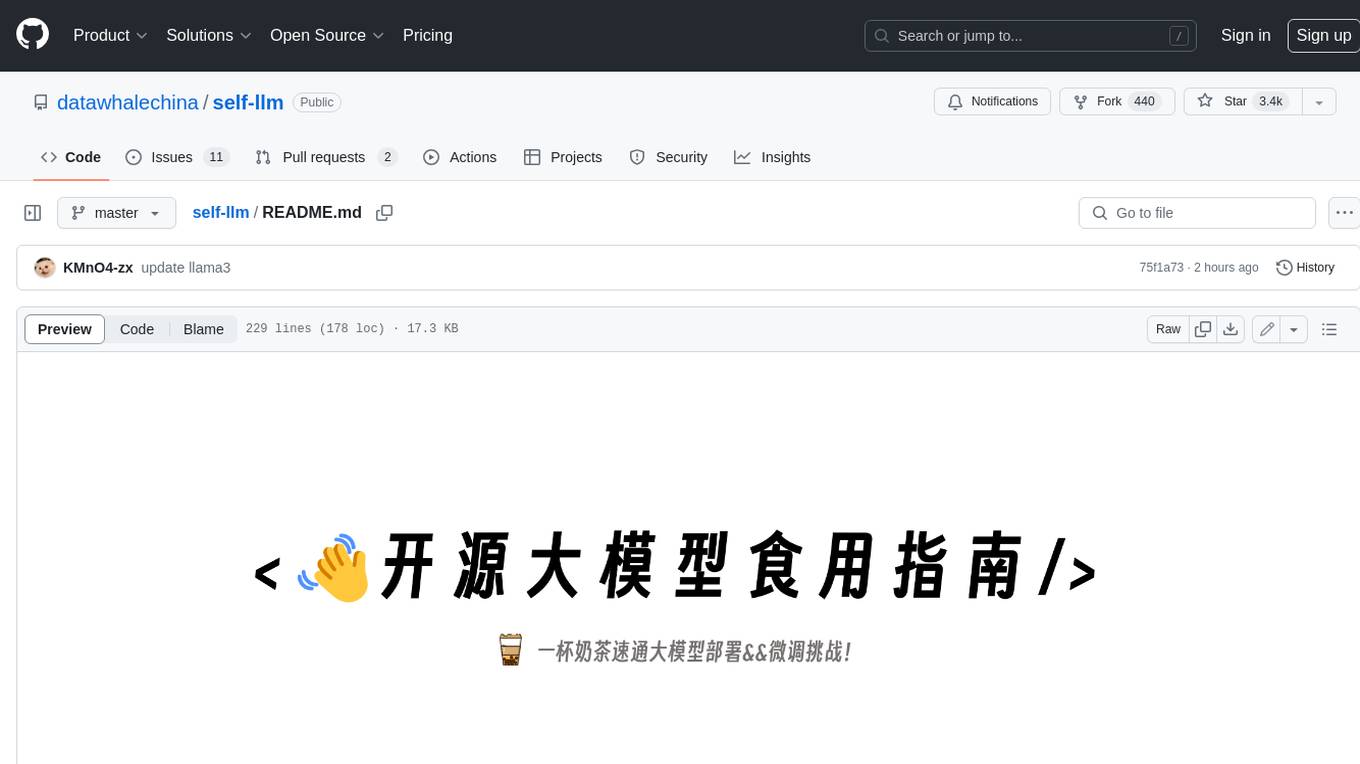
self-llm
This project is a Chinese tutorial for domestic beginners based on the AutoDL platform, providing full-process guidance for various open-source large models, including environment configuration, local deployment, and efficient fine-tuning. It simplifies the deployment, use, and application process of open-source large models, enabling more ordinary students and researchers to better use open-source large models and helping open and free large models integrate into the lives of ordinary learners faster.
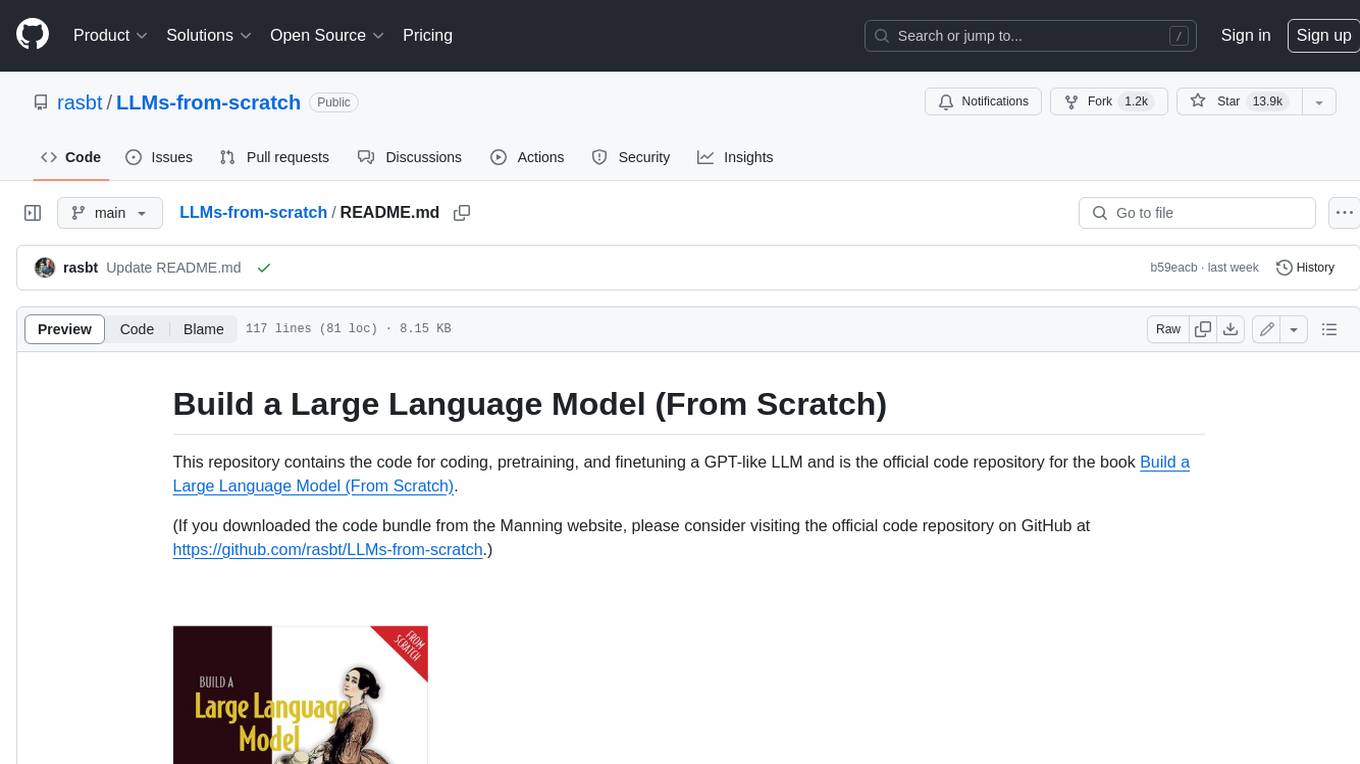
LLMs-from-scratch
This repository contains the code for coding, pretraining, and finetuning a GPT-like LLM and is the official code repository for the book Build a Large Language Model (From Scratch). In _Build a Large Language Model (From Scratch)_, you'll discover how LLMs work from the inside out. In this book, I'll guide you step by step through creating your own LLM, explaining each stage with clear text, diagrams, and examples. The method described in this book for training and developing your own small-but-functional model for educational purposes mirrors the approach used in creating large-scale foundational models such as those behind ChatGPT.
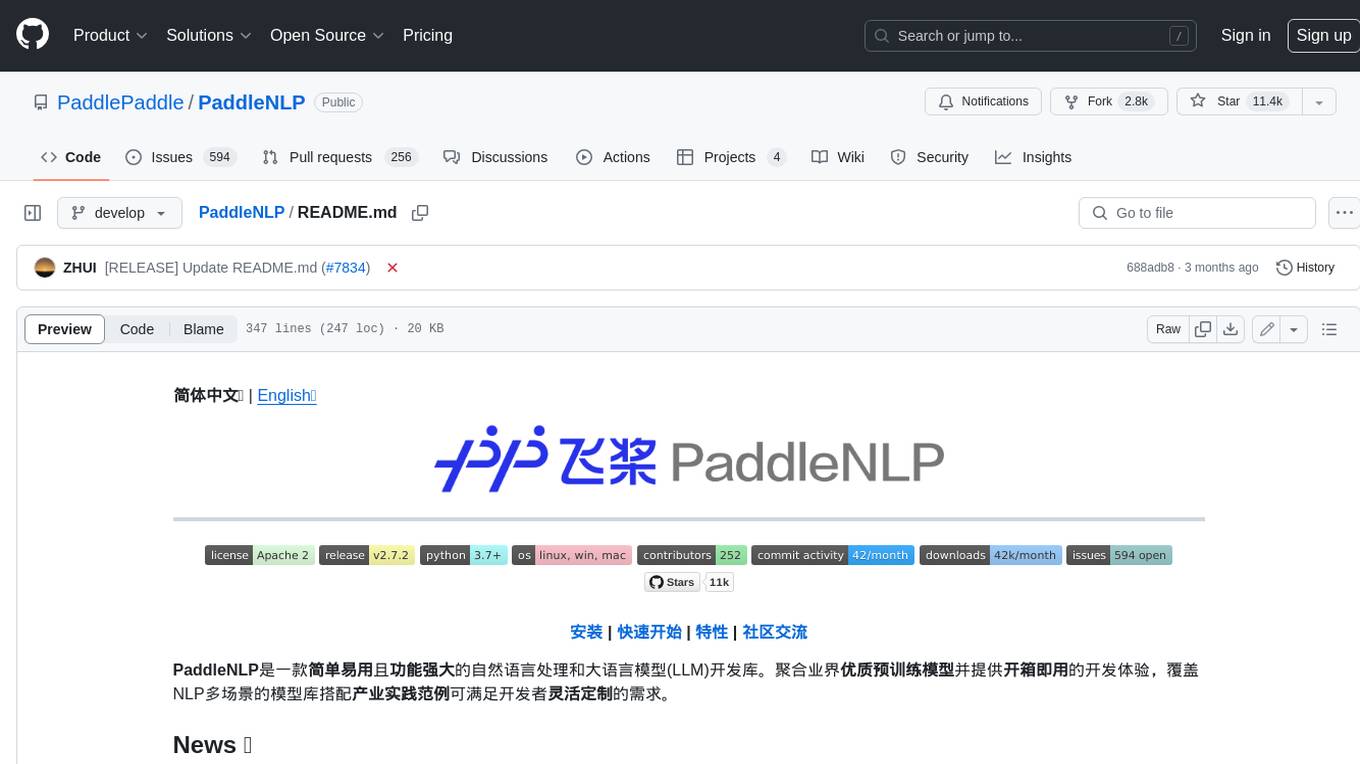
PaddleNLP
PaddleNLP is an easy-to-use and high-performance NLP library. It aggregates high-quality pre-trained models in the industry and provides out-of-the-box development experience, covering a model library for multiple NLP scenarios with industry practice examples to meet developers' flexible customization needs.
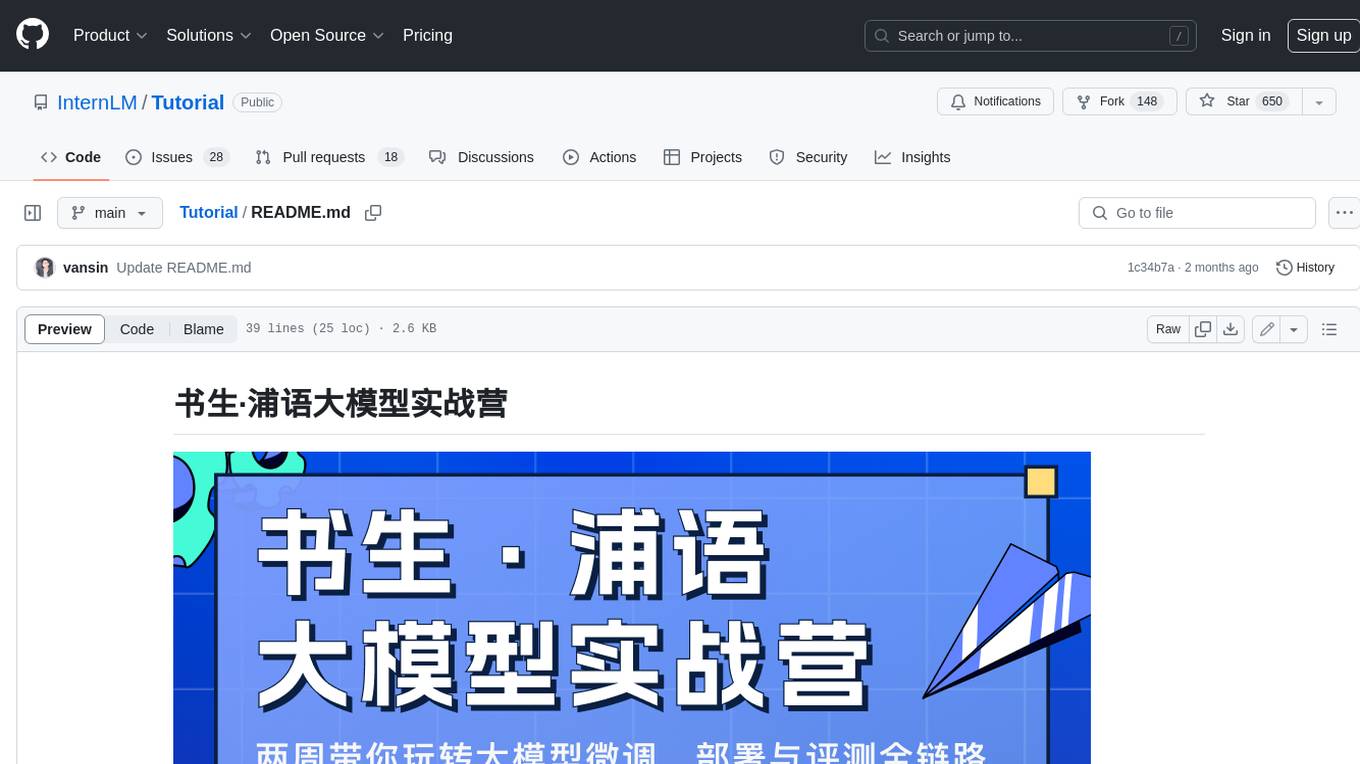
Tutorial
The Bookworm·Puyu large model training camp aims to promote the implementation of large models in more industries and provide developers with a more efficient platform for learning the development and application of large models. Within two weeks, you will learn the entire process of fine-tuning, deploying, and evaluating large models.
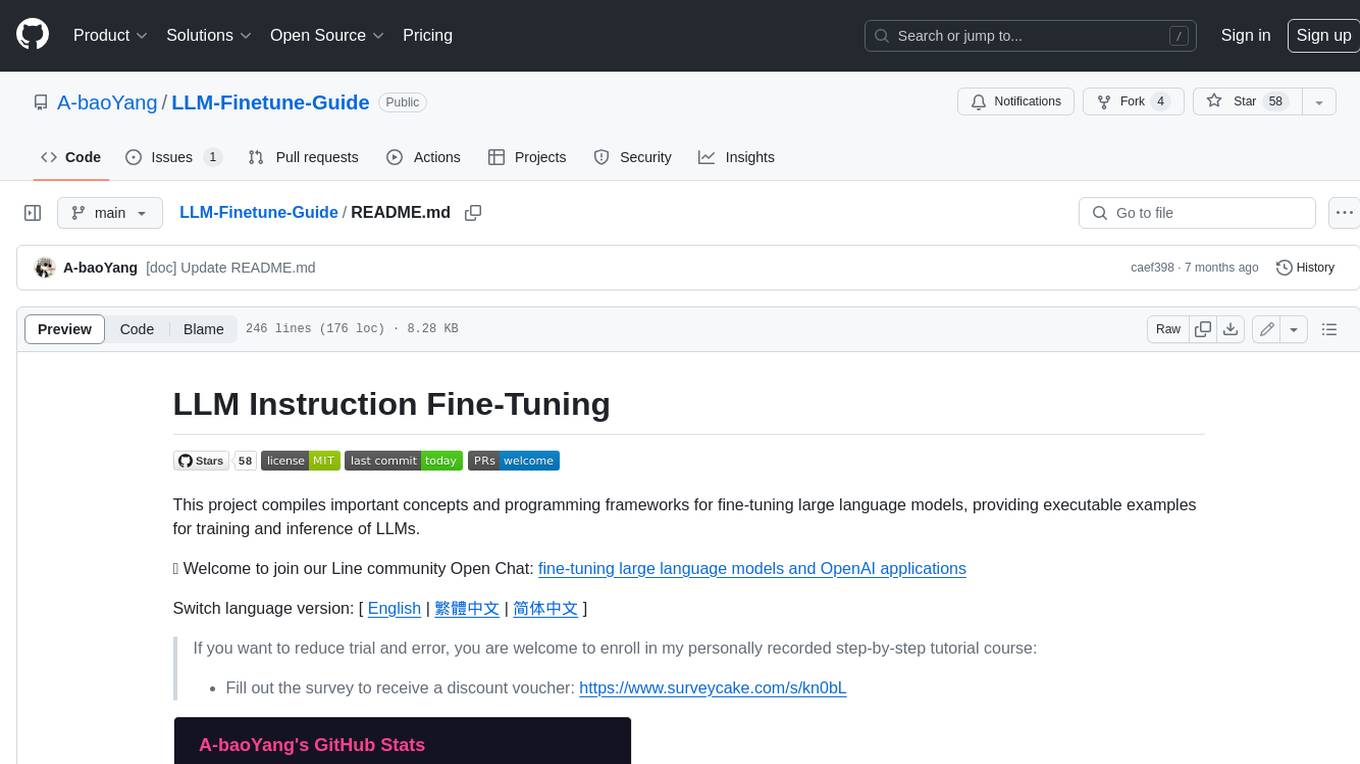
LLM-Finetune-Guide
This project provides a comprehensive guide to fine-tuning large language models (LLMs) with efficient methods like LoRA and P-tuning V2. It includes detailed instructions, code examples, and performance benchmarks for various LLMs and fine-tuning techniques. The guide also covers data preparation, evaluation, prediction, and running inference on CPU environments. By leveraging this guide, users can effectively fine-tune LLMs for specific tasks and applications.
For similar jobs
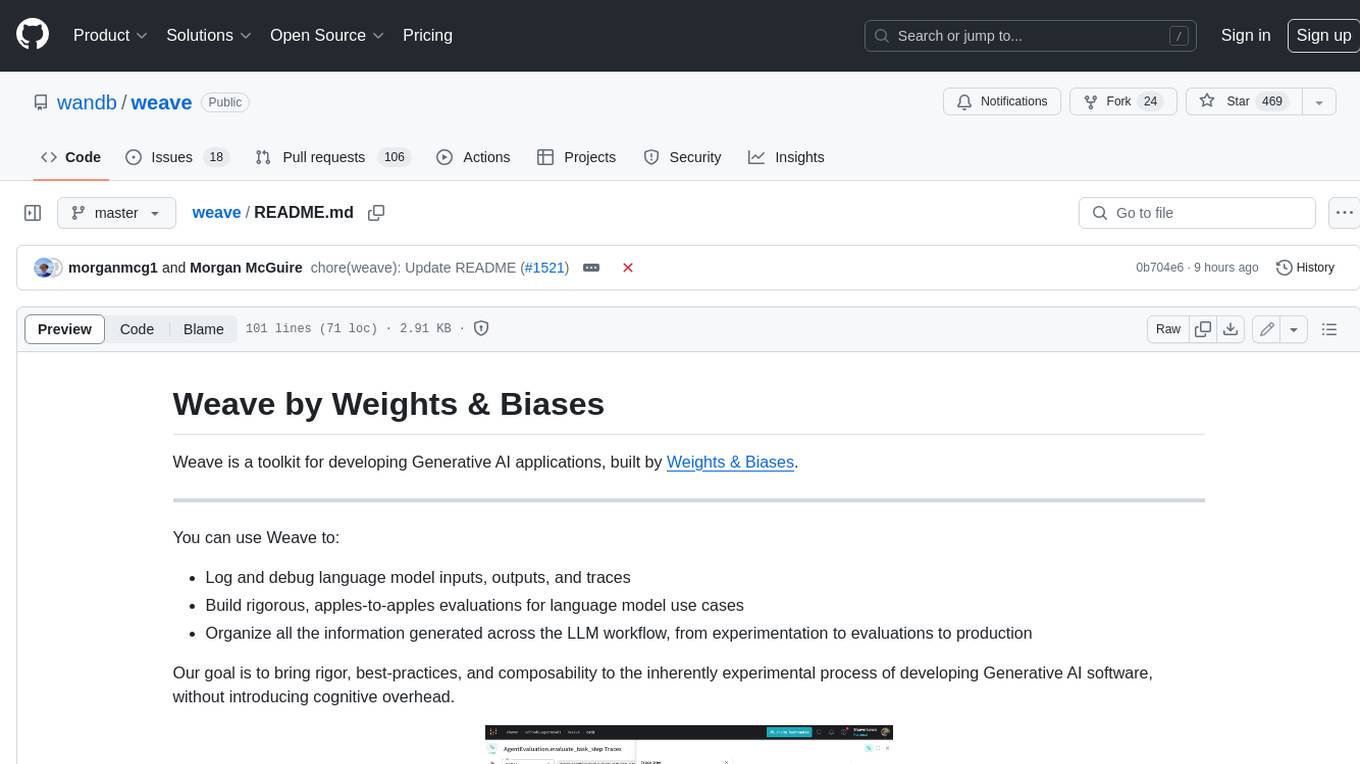
weave
Weave is a toolkit for developing Generative AI applications, built by Weights & Biases. With Weave, you can log and debug language model inputs, outputs, and traces; build rigorous, apples-to-apples evaluations for language model use cases; and organize all the information generated across the LLM workflow, from experimentation to evaluations to production. Weave aims to bring rigor, best-practices, and composability to the inherently experimental process of developing Generative AI software, without introducing cognitive overhead.
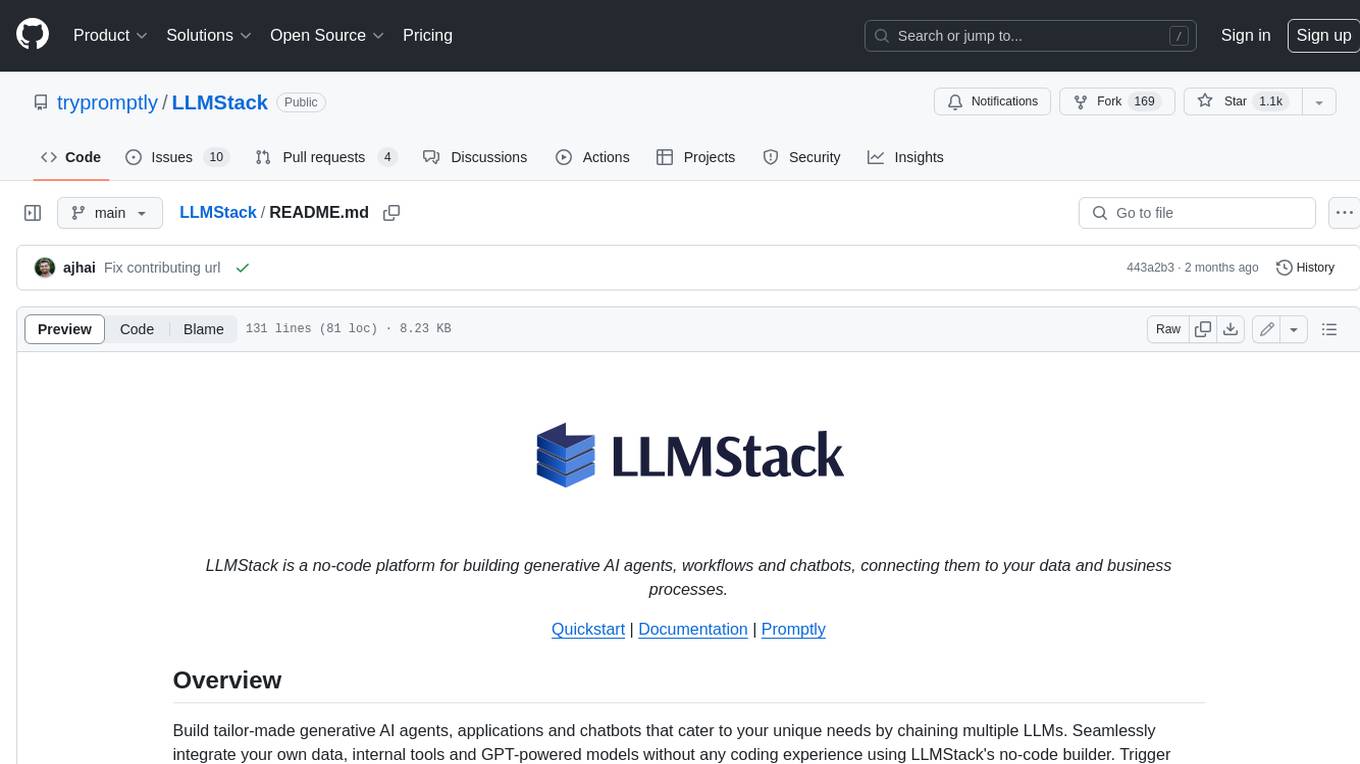
LLMStack
LLMStack is a no-code platform for building generative AI agents, workflows, and chatbots. It allows users to connect their own data, internal tools, and GPT-powered models without any coding experience. LLMStack can be deployed to the cloud or on-premise and can be accessed via HTTP API or triggered from Slack or Discord.
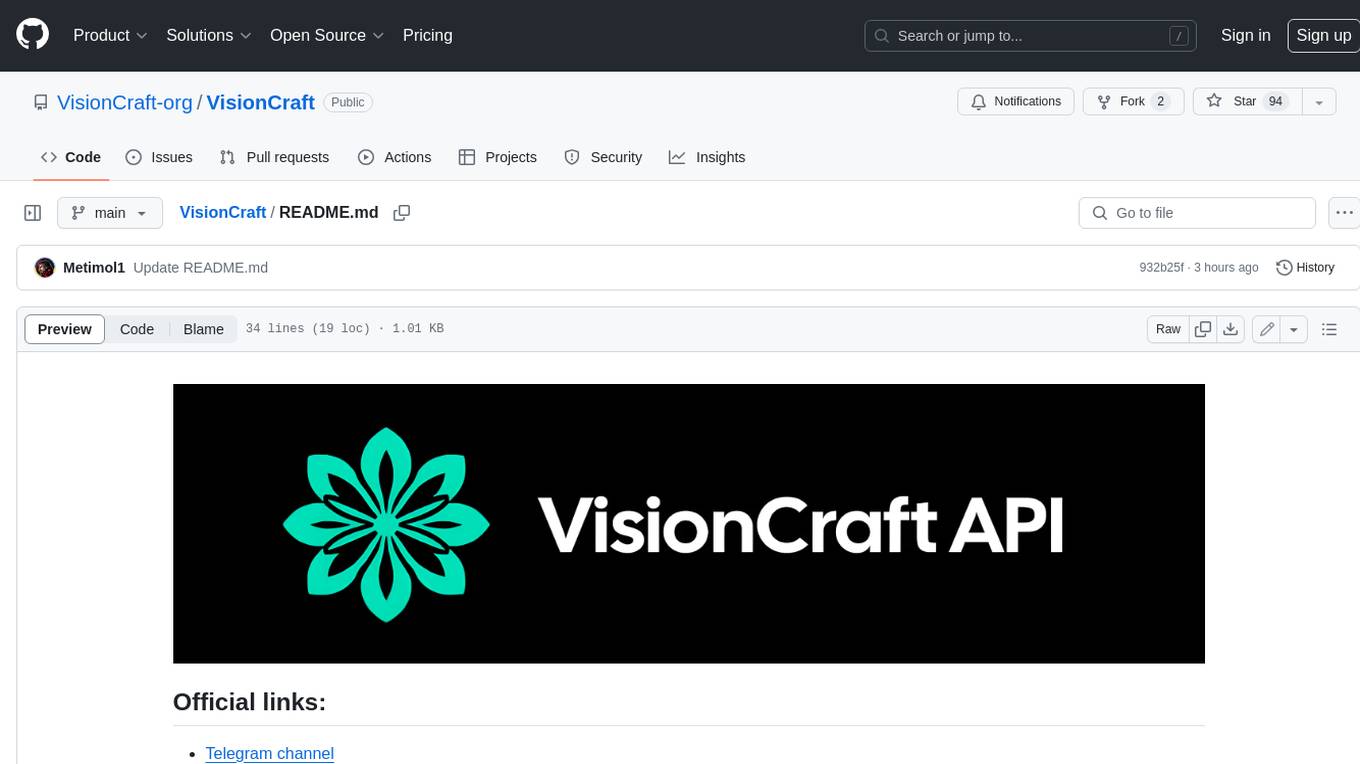
VisionCraft
The VisionCraft API is a free API for using over 100 different AI models. From images to sound.
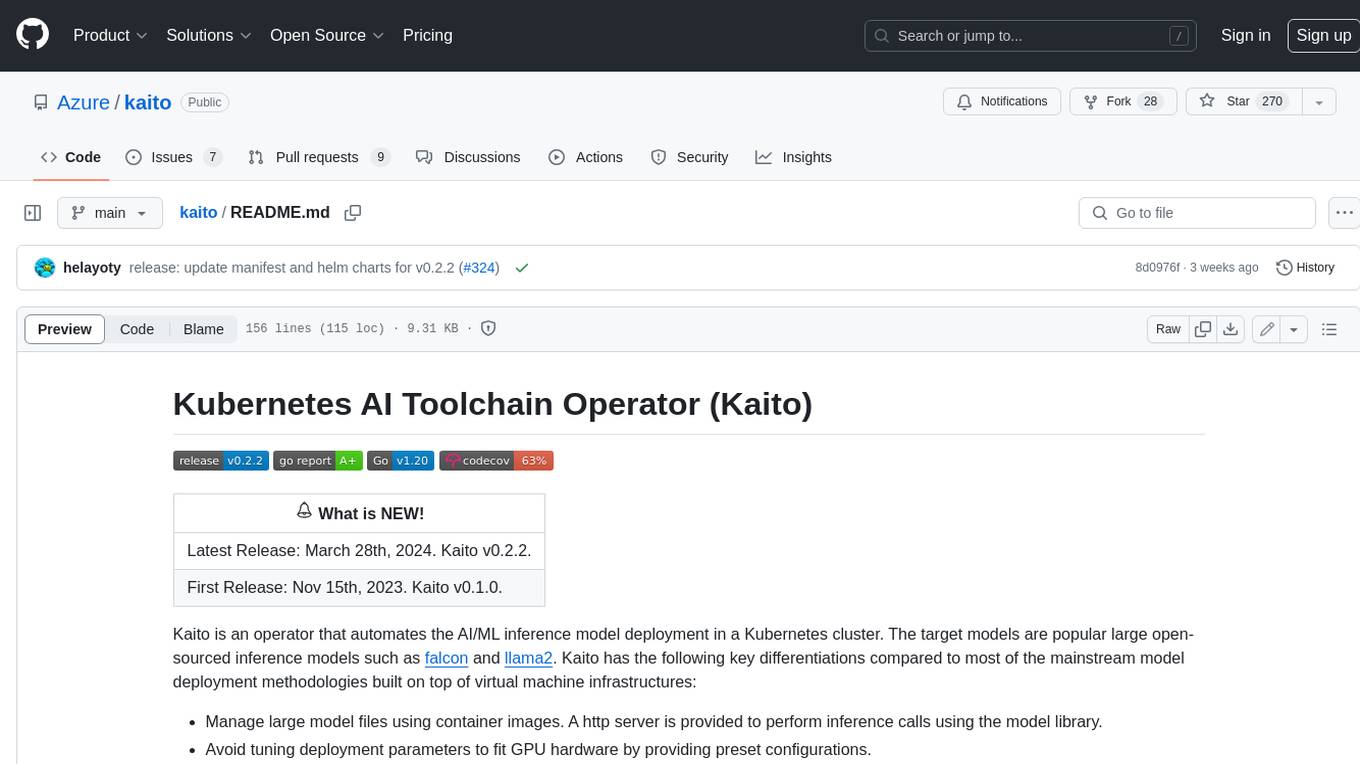
kaito
Kaito is an operator that automates the AI/ML inference model deployment in a Kubernetes cluster. It manages large model files using container images, avoids tuning deployment parameters to fit GPU hardware by providing preset configurations, auto-provisions GPU nodes based on model requirements, and hosts large model images in the public Microsoft Container Registry (MCR) if the license allows. Using Kaito, the workflow of onboarding large AI inference models in Kubernetes is largely simplified.
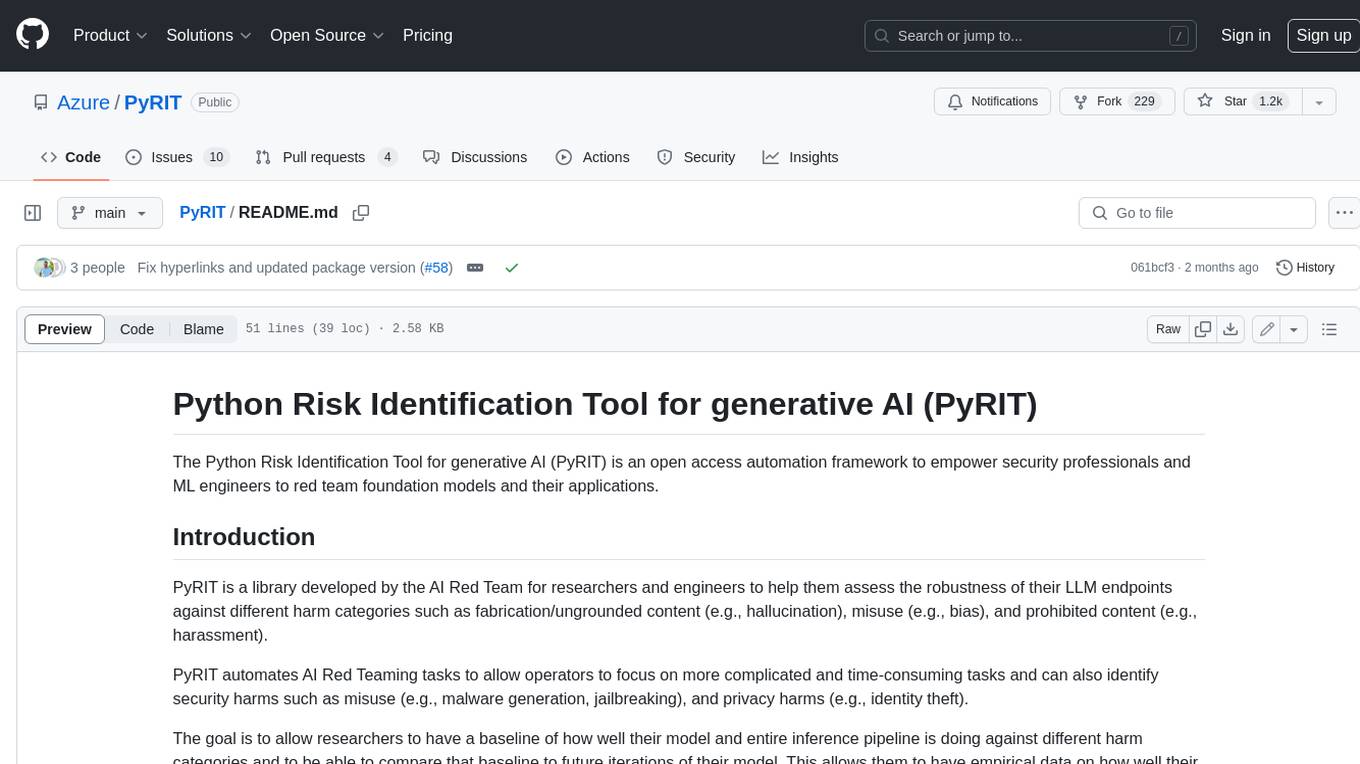
PyRIT
PyRIT is an open access automation framework designed to empower security professionals and ML engineers to red team foundation models and their applications. It automates AI Red Teaming tasks to allow operators to focus on more complicated and time-consuming tasks and can also identify security harms such as misuse (e.g., malware generation, jailbreaking), and privacy harms (e.g., identity theft). The goal is to allow researchers to have a baseline of how well their model and entire inference pipeline is doing against different harm categories and to be able to compare that baseline to future iterations of their model. This allows them to have empirical data on how well their model is doing today, and detect any degradation of performance based on future improvements.
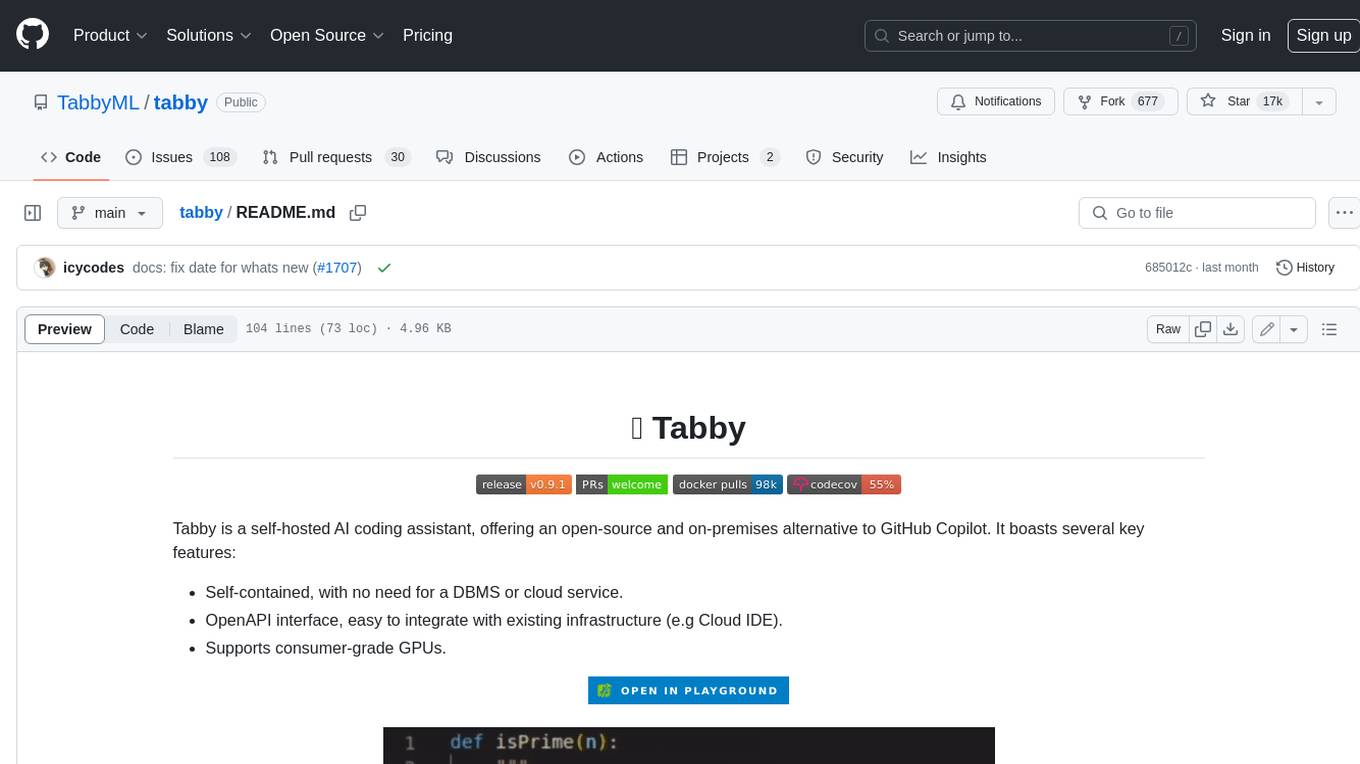
tabby
Tabby is a self-hosted AI coding assistant, offering an open-source and on-premises alternative to GitHub Copilot. It boasts several key features: * Self-contained, with no need for a DBMS or cloud service. * OpenAPI interface, easy to integrate with existing infrastructure (e.g Cloud IDE). * Supports consumer-grade GPUs.
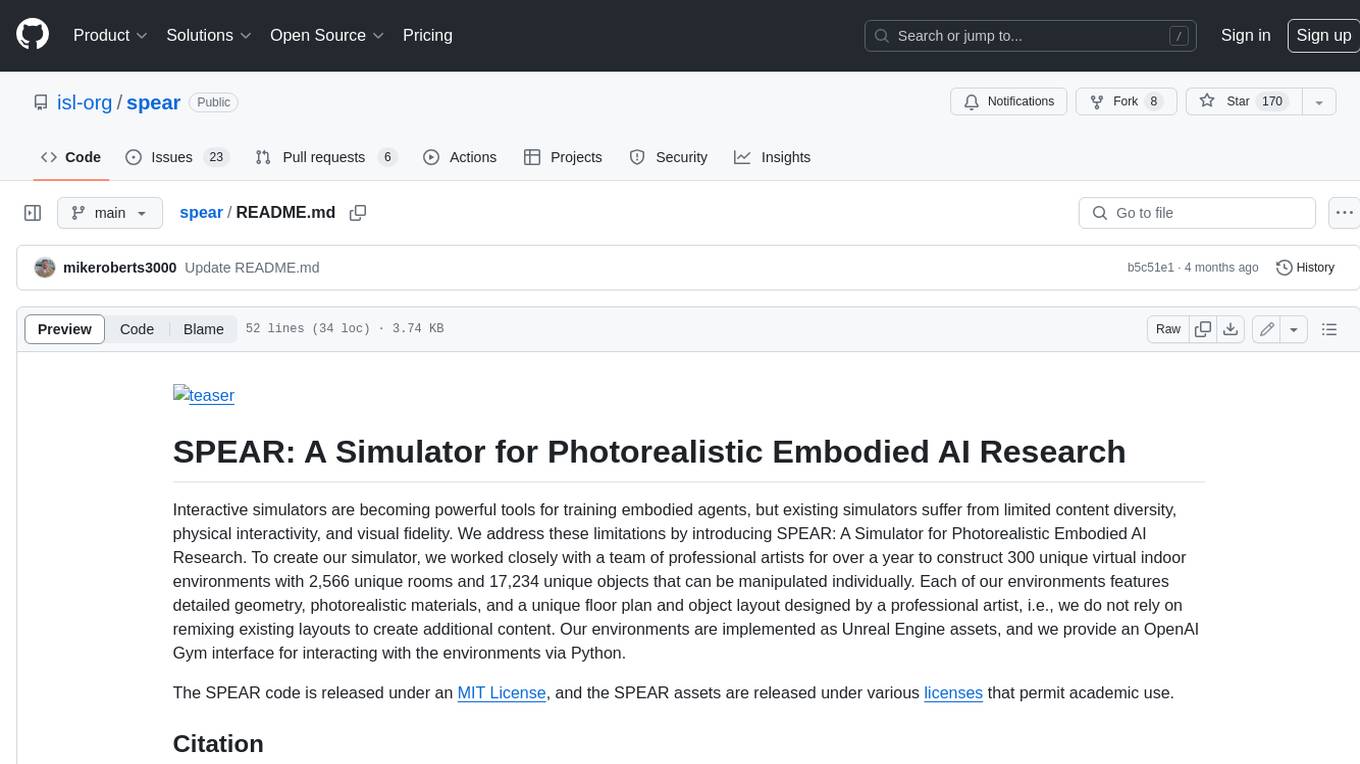
spear
SPEAR (Simulator for Photorealistic Embodied AI Research) is a powerful tool for training embodied agents. It features 300 unique virtual indoor environments with 2,566 unique rooms and 17,234 unique objects that can be manipulated individually. Each environment is designed by a professional artist and features detailed geometry, photorealistic materials, and a unique floor plan and object layout. SPEAR is implemented as Unreal Engine assets and provides an OpenAI Gym interface for interacting with the environments via Python.
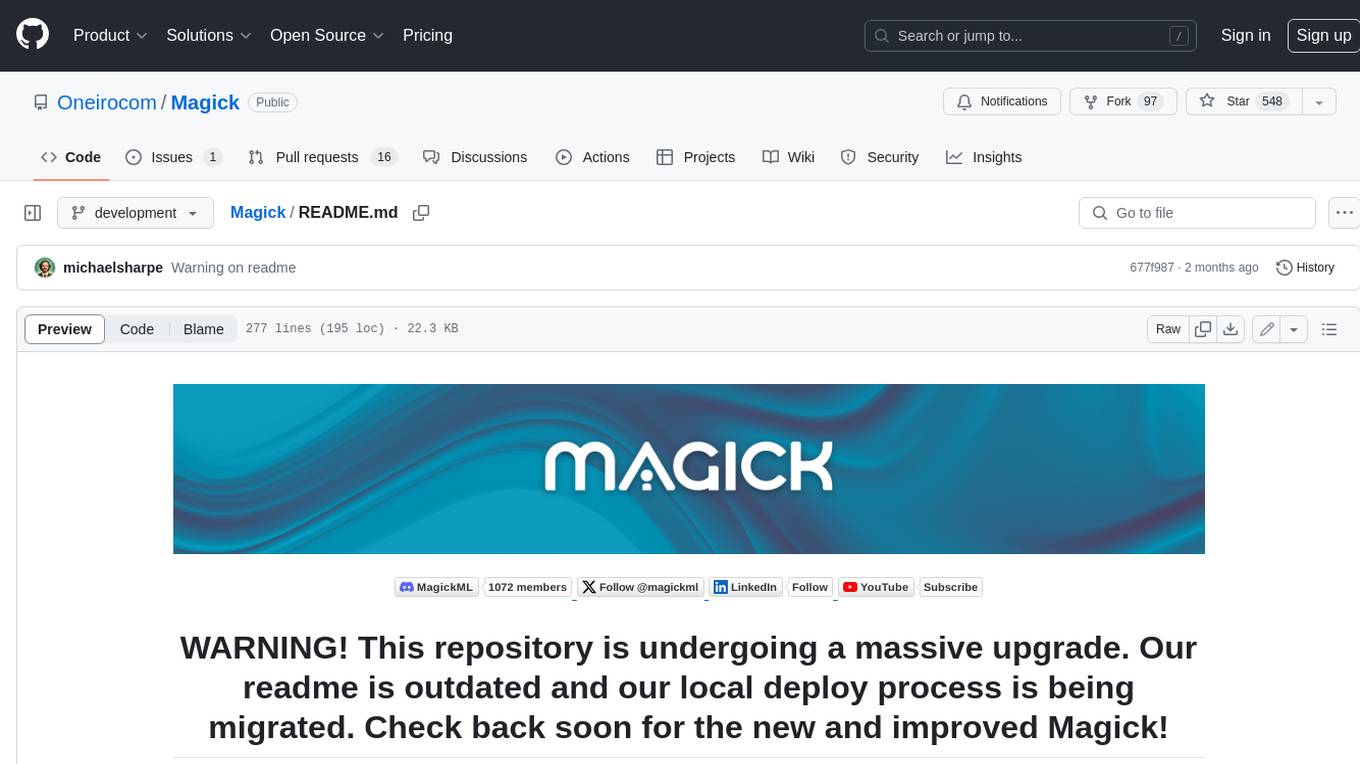
Magick
Magick is a groundbreaking visual AIDE (Artificial Intelligence Development Environment) for no-code data pipelines and multimodal agents. Magick can connect to other services and comes with nodes and templates well-suited for intelligent agents, chatbots, complex reasoning systems and realistic characters.