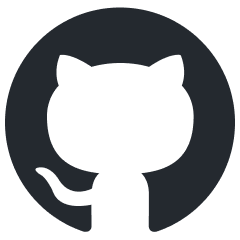
RTL-Coder
A new LLM solution for RTL code generation, achieving state-of-the-art performance in non-commercial solutions and outperforming GPT-3.5.
Stars: 121
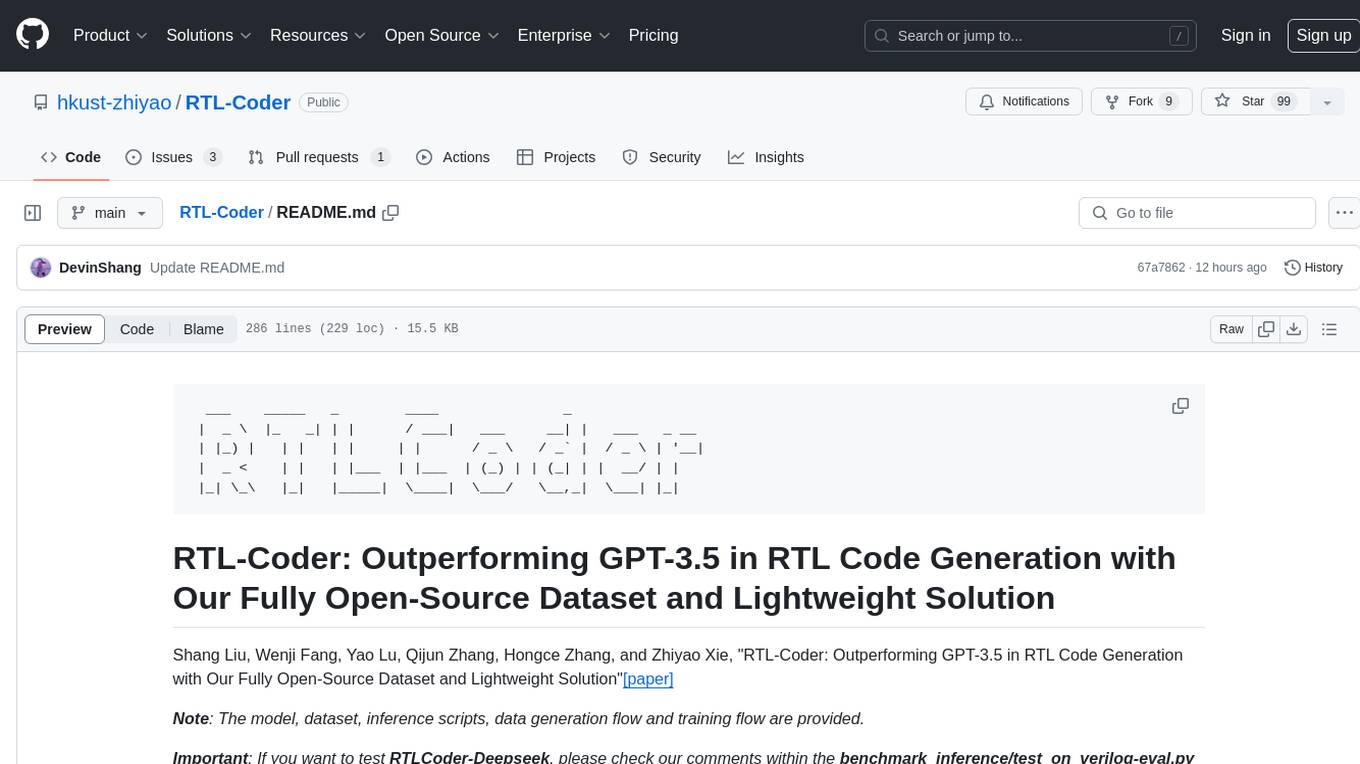
RTL-Coder is a tool designed to outperform GPT-3.5 in RTL code generation by providing a fully open-source dataset and a lightweight solution. It targets Verilog code generation and offers an automated flow to generate a large labeled dataset with over 27,000 diverse Verilog design problems and answers. The tool addresses the data availability challenge in IC design-related tasks and can be used for various applications beyond LLMs. The tool includes four RTL code generation models available on the HuggingFace platform, each with specific features and performance characteristics. Additionally, RTL-Coder introduces a new LLM training scheme based on code quality feedback to further enhance model performance and reduce GPU memory consumption.
README:
___ _____ _ ____ _
| _ \ |_ _| | | / ___| ___ __| | ___ _ __
| |_) | | | | | | | / _ \ / _` | / _ \ | '__|
| _ < | | | |___ | |___ | (_) | | (_| | | __/ | |
|_| \_\ |_| |_____| \____| \___/ \__,_| \___| |_|
πΒ Papers | πΒ Repo-intro | πΒ RTLCoder-flow | πΒ Dataset-generation | π§ͺΒ Inference | ποΈΒ Training | πΒ Citation
This is the official implementation for RTLCoder. We have three relevant accepted papers:
RTLCoder: Fully Open-Source and Efficient LLM-Assisted RTL Code Generation Technique.[paper] IEEE Transactions on Computer-Aided Design of Integrated Circuits and Systems (TCAD) (Early Access).
RTLCoder: Outperforming GPT-3.5 in Design RTL Generation with Our Open-Source Dataset and Lightweight Solution.[paper] IEEE International Workshop on LLM-Aided Design (LAD), 2024. (Best Paper Nomination ).
OpenLLM-RTL: Open Dataset and Benchmark for LLM-Aided Design RTL Generation (Invited).[paper] IEEE/ACM International Conference on Computer-Aided Design (ICCAD), 2024.
Note: The model, dataset, inference scripts, data generation flow and training flow are provided.
Important: If you want to test RTLCoder-Deepseek, please check our comments within the benchmark_inference/test_on_verilog-eval.py file. They are the response postprocessing method designed for RTLCoder-Deepseek. The default inference script is for RTLCoder-Mistral.
Targeting Verilog code generation, we propose an automated flow to generate a large labeled dataset with diverse Verilog design problems and answers. It addresses the serious data availability challenge in IC design-related tasks, and its potential applications are not limited to LLMs. The LLM directly trained on it can already achieve comparable accuracy with GPT-3.5.
We also introduce a new LLM training scheme based on code quality feedback. It further boosts the ultimate model performance to outperform GPT-3.5. And we further revised the training process from an algorithm perspective to reduce its GPU memory consumption.
TABLE 1 summarizes existing works in LLM-based design RTL generation.
TABLE 1: LLM-based works on design RTL generation (e.g., Verilog).
In our work, we provide four RTL code generation models that are available on the HuggingFace platform.
- RTLCoder-Deepseek-v1.1. This model was finetund on DeepSeek-coder-6.7b. It has the best performance on VerilogEval and RTLLM benchmarks but with a relatively lower inference speed compared with the following models. The RTLCoder-Deepseek-v1.1 may not stop even when the required output text is finished. So We need to extract the required code part before the keyword"endmodulemodule" from the output sequence and add an "endmodule" at the end.
- RTLCoder-v1.1. (Finetuned based on Mistral-v0.1)
- RTLCoder-v1.1-gptq-4bit. (The GPTQ version of RTLCoder-v1.1)
- RTLCoder-v1.1-gguf-4bit. This quantized one could run on CPU. (The CPU version of RTLCoder-v1.1)
In this paper, there are two main contributions to obtain the RTLCoder. (1) We first introduce our automated dataset generation flow. It generated our RTL generation dataset with over 27 thousand samples, each sample being a pair of design description instruction and corresponding reference code. We build this automated generation flow by taking full advantage of the powerful general text generation ability of the commercial tool GPT. Please notice that GPT is only used for dataset generation in this work and we adhere to the terms of service of OpenAI, and there is no commercial competition between the proposed RTLcoder and OpenAI's models. The automated dataset generation flow is illustrated in Figure 1 which includes three stages: 1) RTL domain keywords preparation, 2) instruction generation, and 3) reference code generation. We designed several general prompt templates to control GPT generating the desired outputs in each stage.
Figure 1: Our proposed automated dataset generation flow.
(2) Besides the new training dataset, we propose a new LLM training scheme that incorporates code quality scoring. It significantly improves the RTLCoderβs performance on the RTL generation task. Also, we revised the training process from the algorithm perspective to reduce the GPU memory consumption of this new training method, allowing implementation with limited hardware resources. The training scheme is illustrated in Figure 2.
Figure 2: Our proposed training scheme based on RTL quality score.
We provide the generation scripts and data samples in the folder "data_generation". You can design your own prompting method by modifying the file "p_example.txt" and "instruction_gen.py".
You can expand the existing dataset by running the following command.
python instruction_gen.py
The 27K instruction-code dataset "Resyn-27k.json" is provided in the "dataset" file. Please kindly note that the dataset was generated by GPT-3.5-turbo and it cannot be guaranteed that all the data are strictly correct. Despite the possible presence of errors in some problem descriptions and design code, we believe that they can still provide valuable information for model training.
(1) Inference demo
The input prompt may have a great influence on the generation quality. Ideally, it should describe the circuit "IO" and behavior clearly so that it doesn't contain ambiguity. We provide a template as follows.
Please act as a professional verilog designer.
Implement a data width conversion circuit that converts 8-bit data input to 16-bit data output. The module provides two output ports: valid_out, which indicates the validity of the output data, and data_out, which represents the converted 16-bit output data. The first arriving 8-bit data should be placed in the higher 8 bits of the 16-bit data output. The valid_out and data_out signals are generated in the next clock cycle after the two data inputs. When there is only one data input, valid_out and data_out are not generated immediately. Instead, they wait for the arrival of the next data input to complete the concatenation of the two data inputs before generating valid_out and data_out.
Module name:
width_8to16
Input ports:
clk: Clock signal used for synchronous operation.
rst_n: Active-low reset signal. Defined as 0 for reset and 1 for reset signal inactive.
valid_in: Input signal indicating the validity of the input data.
data_in: 8-bit input data to be converted.
Output ports:
valid_out: Output signal indicating the validity of the output data.
data_out: 16-bit output data resulting from the width conversion.
Implementation:
The data_out register is triggered on the positive edge of the clock signal (posedge clk) or the negative edge of the reset signal (negedge rst_n).
On reset, indicated by !rst_n, the data_out register is cleared to 0.
If the input data is valid (valid_in) and the flag signal is active, the data_out register is updated by concatenating the contents of the data_lock register (8 bits) and the data_in register (8 bits) to form a 16-bit output. The first valid data is temporarily stored, and when the second valid data is inputted, they are concatenated to produce the output valid_out and data_out.
Give me the complete code.
module width_8to16(
input clk ,
input rst_n ,
input valid_in ,
input [7:0] data_in ,
output reg valid_out,
output reg [15:0] data_out
);
If you don't have a GPU with more than 4 GB memory, please try the quantized 4-bit version which could run on CPU: RTLCoder-v1.1-gguf-4bit. Before you try the following code, please install the ctransformers package.
from ctransformers import AutoModelForCausalLM
model_path = 'ggml-model-q4_0.gguf'
# Set gpu_layers to the number of layers to offload to GPU. Set to 0 if no GPU acceleration is available on your system.
llm = AutoModelForCausalLM.from_pretrained(model_path, model_type="mistral", gpu_layers=0, max_new_tokens=2000, context_length=6048, temperature=0.5, top_p=0.95,)
prompt = "Please act as a professional verilog designer and provide a half adder. \nmodule half_adder\n(input a, \ninput b, \noutput sum, \n output carry);\n"
print(llm(prompt))
For inference using RTLCoder, you can just use the following code.
import torch
from transformers import AutoTokenizer, AutoModelForCausalLM
# Prompt
prompt = "Please act as a professional verilog designer and provide a half adder. \nmodule half_adder\n(input a, \ninput b, \noutput sum, \n output carry);\n"
# Load model and tokenizer
# With multiple gpus, you can specify the GPU you want to use as gpu_name (e.g. int(0)).
gpu_name = 0
tokenizer = AutoTokenizer.from_pretrained("ishorn5/RTLCoder-Deepseek-v1.1")
model = AutoModelForCausalLM.from_pretrained("ishorn5/RTLCoder-Deepseek-v1.1", torch_dtype=torch.float16, device_map=gpu_name)
model.eval()
# Sample
input_ids = tokenizer(prompt, return_tensors="pt").input_ids.to(gpu_name)
sample = model.generate(input_ids, max_length=512, temperature=0.5, top_p=0.9)
s_full = tokenizer.decode(sample[0])
# The RTLCoder-Deepseek-v1.1 may not stop even when the required output text is finished.
# We need to extract the required part from the output sequence based on a keyword "endmodulemodule".
if len(s_full.split('endmodulemodule', 1)) == 2:
s = s_full.split('endmodulemodule', 1)[0] + "\n" + "endmodule"
else:
s = s_full.rsplit('endmodule', 1)[0] + "\n" + "endmodule"
if s.find('top_module') != -1:
s = s.split('top_module', 1)[0]
s = s.rsplit('endmodule', 1)[0] + "\n" + "endmodule"
index = s.rfind('tb_module')
if index == -1:
index = s.find('testbench')
if index != -1:
s_tmp = s[:index]
s = s_tmp.rsplit("endmodule", 1)[0] + "\n" + "endmodule"
print(s)
#For "ishorn5/RTLCoder-v1.1", it will stop generating tokens after completing the coding task.
#But you can still use the keyword "endmodule" to extract the code part.
#tokenizer = AutoTokenizer.from_pretrained("ishorn5/RTLCoder-v1.1")
#model = AutoModelForCausalLM.from_pretrained("ishorn5/RTLCoder-v1.1", torch_dtype=torch.float16, device_map=gpu_name)
#model.eval()
#Sample
#input_ids = tokenizer(prompt, return_tensors="pt").input_ids.to(gpu_name)
#sample = model.generate(input_ids, max_length=512, temperature=0.5, top_p=0.9)
#print(tokenizer.decode(sample[0]))
To test the RTLCoder-gptq-4bit, you can just use the following code. Before you try the following code, please make sure to have the package https://github.com/marella/ctransformers.
from transformers import AutoTokenizer
from [auto_gptq](https://github.com/marella/ctransformers) import AutoGPTQForCausalLM, BaseQuantizeConfig
# Prompt
prompt = "Please act as a professional verilog designer and provide a half adder. \nmodule half_adder\n(input a, \ninput b, \noutput sum, \n output carry);\n"
tokenizer = AutoTokenizer.from_pretrained("ishorn5/RTLCoder-v1.1-gptq-4bit", use_fast=True)
model = AutoGPTQForCausalLM.from_quantized("ishorn5/RTLCoder-v1.1-gptq-4bit", device="cuda:0")
model.eval()
# Sample
inputs = tokenizer(prompt, return_tensors="pt").to(0)
sample = model.generate(**inputs, max_length=512, temperature=0.5, top_p=0.9)
print(tokenizer.decode(sample[0]))
(2) Test model on Verilog-eval
We provide the inference script "test_on_verilog-eval.py" for "verilog-eval" benchmark in folder "benchmark_inference". You need to firstly download the "verilog-eval" benchmark.
git clone https://github.com/NVlabs/verilog-eval.git
Then modify the "descri_path" and "input_path" in "test_on_nvbench.py" according to the location of verlog-eval file.
Use the following command to do model inference on EvalMachine.
python test_on_nvbench.py --model <your model path or model card name, e.g., "ishorn5/RTLCoder-v1.1"> --n 20 --temperature=0.2 --gpu_name 0 --output_dir <your result directory> --output_file <your result file, e.g. rtlcoder_temp0.2_evalmachine.json> --bench_type Machine
If you want to do model inference on EvalHuman, just change the --bench_type from Machine to Human.
python test_on_nvbench.py --model <your model path or model card name, e.g., "ishorn5/RTLCoder-v1.1"> --n 20 --temperature=0.2 --gpu_name 0 --output_dir <your result directory> --output_file <your result file, e.g. rtlcoder_temp0.2_evalhuman.json> --bench_type Human
Please refer the verilog-eval benchmark repo https://github.com/NVlabs/verilog-eval to evaluate the generated code quality.
(3) Test model on RTLLM
We provide the organized descriptions of RTLLM as "rtllm-1.1.json" in folder "benchmark_inference".
Use the following command to do inference on RTLLM benchmark.
python test_on_rtllm.py --model <your model path or model card name, e.g., "ishorn5/RTLCoder-v1.1"> --n 5 --temperature=0.5 --gpu_name 0 --output_dir <your result directory>
Please refer the RTLLM benchmark repo https://github.com/hkust-zhiyao/RTLLM to evaluate the generated code quality.
We provide three options for instruction tuning: MLE based direct train, Scoring train and Scoring train with gradients splitting. For more details, please refer to the paper and the folder "train".
For MLE based direct training, just simply use:
torchrun --nproc_per_node=4 mle.py \
--model_name_or_path <model path> \
--data_path <data path> \
--fp16 True \
--output_dir <output path>\
--num_train_epochs 3 \
--per_device_train_batch_size 2 \
--per_device_eval_batch_size 2 \
--gradient_accumulation_steps 32 \
--evaluation_strategy "no" \
--save_strategy "steps" \
--save_steps 50 \
--save_total_limit 10 \
--learning_rate 1e-5 \
--weight_decay 0. \
--logging_steps 1 \
--tf32 False\
--gradient_checkpointing True \
--deepspeed ds_stage_2.json\
--model_max_length 2048
For scoring based training method, you need to firstly obtain answer candidates to each of the instruction in the training dataset and we provide a data sample "scoring_data_sample.json" to illustrate the data format for training. Then use the following command.
torchrun --nproc_per_node=4 mle_scoring.py \
--model_name_or_path <model path> \
--data_path <data path> \
--fp16 True \
--output_dir <output path>\
--num_train_epochs 3 \
--per_device_train_batch_size 1 \
--per_device_eval_batch_size 2 \
--gradient_accumulation_steps 64\
--evaluation_strategy "no" \
--save_strategy "steps" \
--save_steps 50 \
--save_total_limit 10 \
--learning_rate 1e-5 \
--weight_decay 0. \
--logging_steps 1 \
--tf32 False\
--gradient_checkpointing True \
--deepspeed ds_stage_2.json\
--model_max_length 2048
If your gpu could't afford batch size 1 with these answer candidates, try the gradients splitting method.
torchrun --nproc_per_node=4 mle_scoring_grad_split.py \
--model_name_or_path <model path> \
--data_path <data path> \
--fp16 True \
--output_dir <output path>\
--num_train_epochs 3 \
--per_device_train_batch_size 1 \
--per_device_eval_batch_size 2 \
--gradient_accumulation_steps 64\
--evaluation_strategy "no" \
--save_strategy "steps" \
--save_steps 50 \
--save_total_limit 10 \
--learning_rate 1e-5 \
--weight_decay 0. \
--logging_steps 1 \
--tf32 False\
--gradient_checkpointing True \
--deepspeed ds_stage_2.json\
--model_max_length 2048
@article{liu2024rtlcoderb,
title={RTLCoder: Fully Open-Source and Efficient LLM-Assisted RTL Code Generation Technique},
author={Liu, Shang and Fang, Wenji and Lu, Yao and Wang, Jing and Zhang, Qijun and Zhang, Hongce and Xie, Zhiyao},
journal={IEEE Transactions on Computer-Aided Design of Integrated Circuits and Systems},
year={2024},
publisher={IEEE}
}
@inproceedings{liu2024rtlcodera,
title={RTLCoder: Outperforming GPT-3.5 in Design RTL Generation with Our Open-Source Dataset and Lightweight Solution},
author={Liu, Shang and Fang, Wenji and Lu, Yao and Zhang, Qijun and Zhang, Hongce and Xie, Zhiyao},
booktitle={2024 IEEE International Workshop on LLM-Aided Design},
year={2024},
organization={IEEE}
}
@inproceedings{liu2024openllm,
title={OpenLLM-RTL: Open Dataset and Benchmark for LLM-Aided Design RTL Generation},
author={Liu, Shang and Lu, Yao and Fang, Wenji and Li, Mengming and Xie, Zhiyao},
booktitle={2024 IEEE/ACM International Conference on Computer Aided Design (ICCAD)},
year={2024},
organization={IEEE/ACM}
}
For Tasks:
Click tags to check more tools for each tasksFor Jobs:
Alternative AI tools for RTL-Coder
Similar Open Source Tools
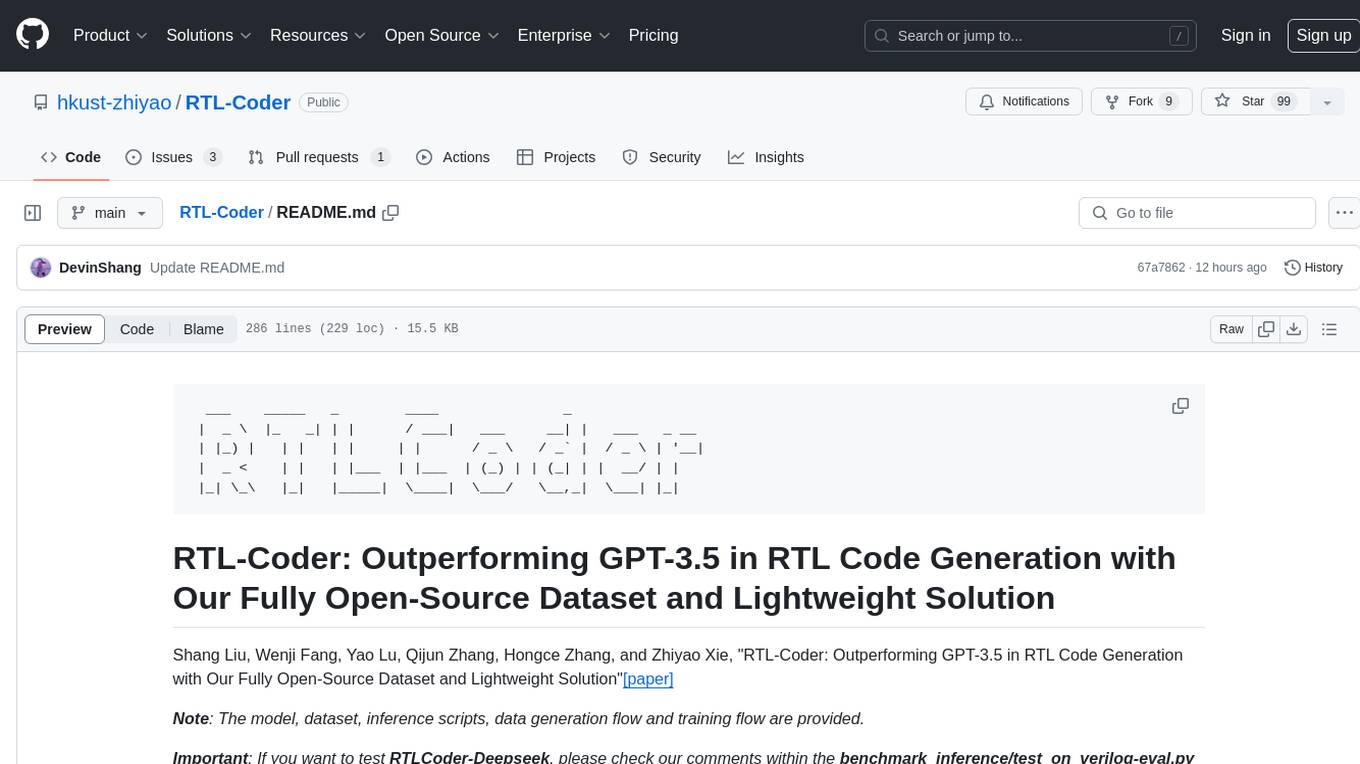
RTL-Coder
RTL-Coder is a tool designed to outperform GPT-3.5 in RTL code generation by providing a fully open-source dataset and a lightweight solution. It targets Verilog code generation and offers an automated flow to generate a large labeled dataset with over 27,000 diverse Verilog design problems and answers. The tool addresses the data availability challenge in IC design-related tasks and can be used for various applications beyond LLMs. The tool includes four RTL code generation models available on the HuggingFace platform, each with specific features and performance characteristics. Additionally, RTL-Coder introduces a new LLM training scheme based on code quality feedback to further enhance model performance and reduce GPU memory consumption.
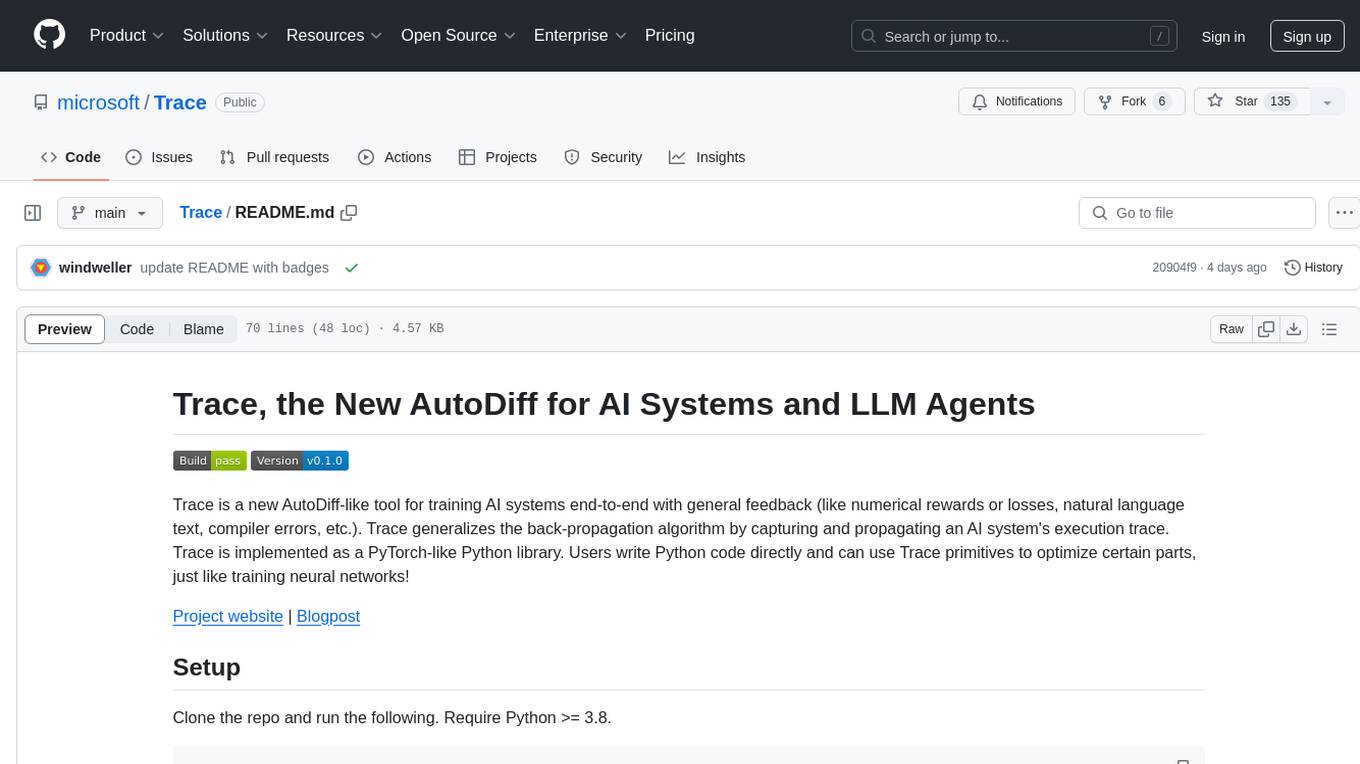
Trace
Trace is a new AutoDiff-like tool for training AI systems end-to-end with general feedback. It generalizes the back-propagation algorithm by capturing and propagating an AI system's execution trace. Implemented as a PyTorch-like Python library, users can write Python code directly and use Trace primitives to optimize certain parts, similar to training neural networks.
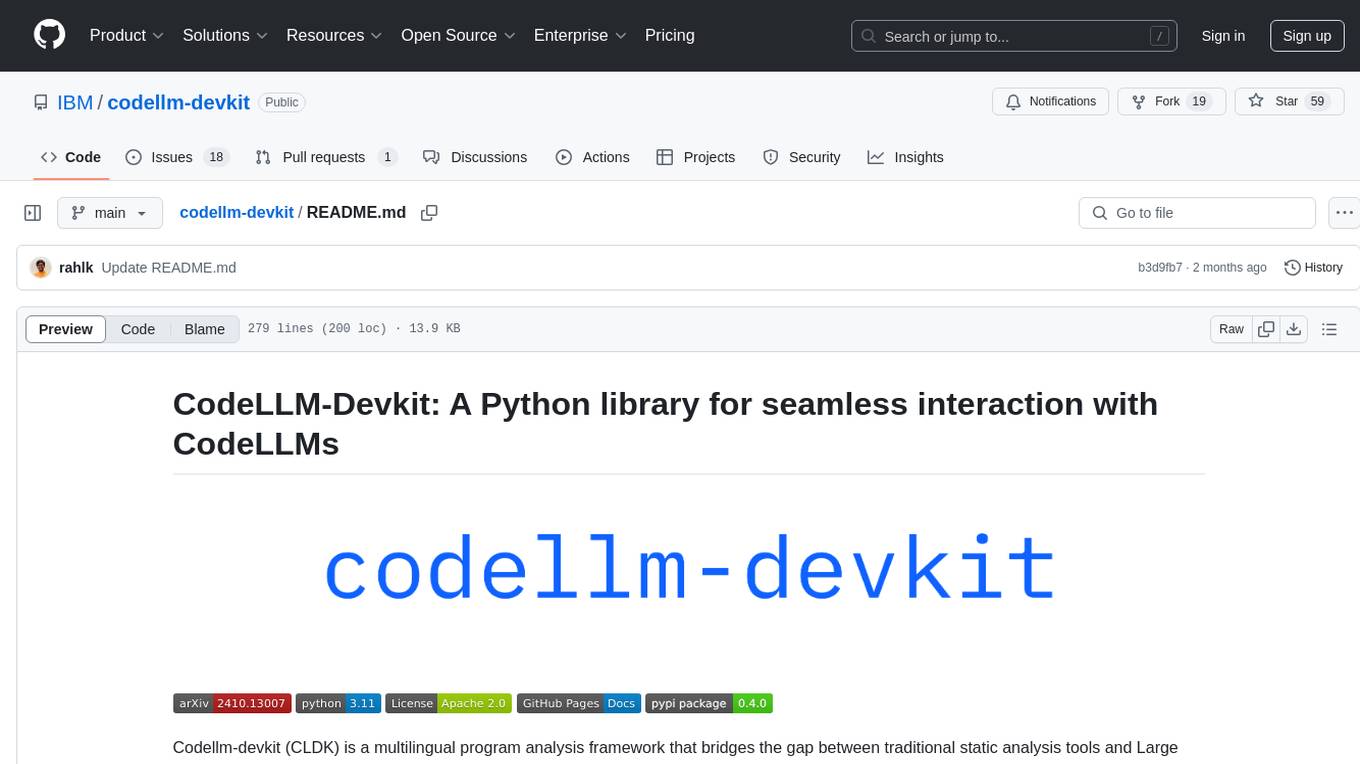
codellm-devkit
Codellm-devkit (CLDK) is a Python library that serves as a multilingual program analysis framework bridging traditional static analysis tools and Large Language Models (LLMs) specialized for code (CodeLLMs). It simplifies the process of analyzing codebases across multiple programming languages, enabling the extraction of meaningful insights and facilitating LLM-based code analysis. The library provides a unified interface for integrating outputs from various analysis tools and preparing them for effective use by CodeLLMs. Codellm-devkit aims to enable the development and experimentation of robust analysis pipelines that combine traditional program analysis tools and CodeLLMs, reducing friction in multi-language code analysis and ensuring compatibility across different tools and LLM platforms. It is designed to seamlessly integrate with popular analysis tools like WALA, Tree-sitter, LLVM, and CodeQL, acting as a crucial intermediary layer for efficient communication between these tools and CodeLLMs. The project is continuously evolving to include new tools and frameworks, maintaining its versatility for code analysis and LLM integration.
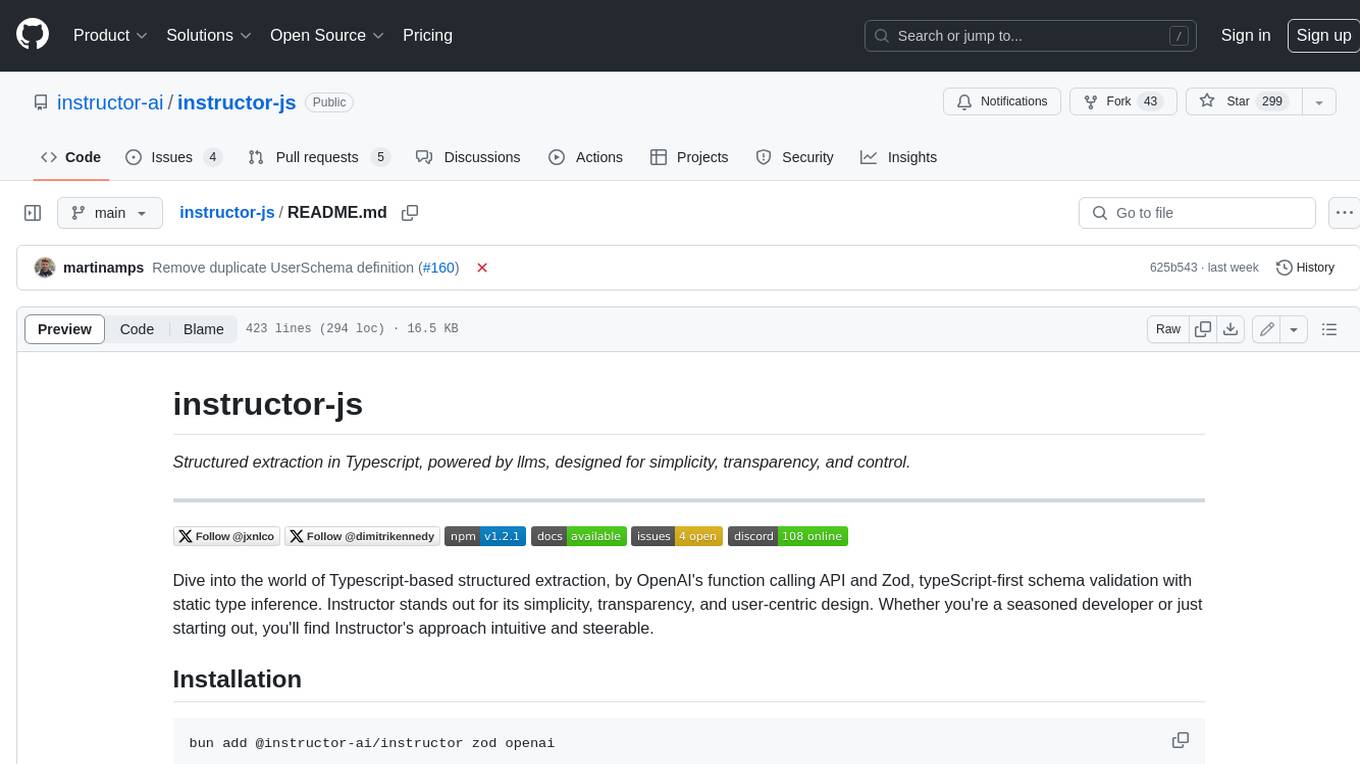
instructor-js
Instructor is a Typescript library for structured extraction in Typescript, powered by llms, designed for simplicity, transparency, and control. It stands out for its simplicity, transparency, and user-centric design. Whether you're a seasoned developer or just starting out, you'll find Instructor's approach intuitive and steerable.
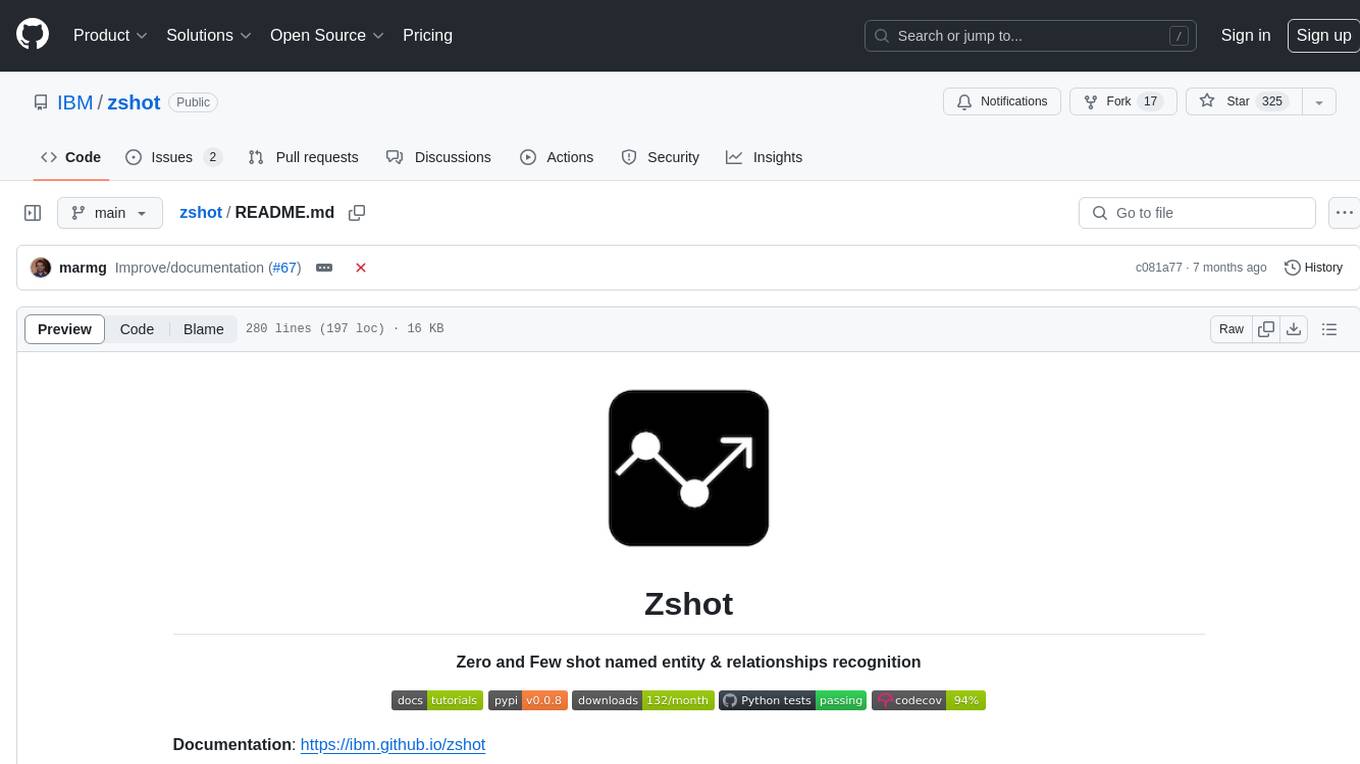
zshot
Zshot is a highly customizable framework for performing Zero and Few shot named entity and relationships recognition. It can be used for mentions extraction, wikification, zero and few shot named entity recognition, zero and few shot named relationship recognition, and visualization of zero-shot NER and RE extraction. The framework consists of two main components: the mentions extractor and the linker. There are multiple mentions extractors and linkers available, each serving a specific purpose. Zshot also includes a relations extractor and a knowledge extractor for extracting relations among entities and performing entity classification. The tool requires Python 3.6+ and dependencies like spacy, torch, transformers, evaluate, and datasets for evaluation over datasets like OntoNotes. Optional dependencies include flair and blink for additional functionalities. Zshot provides examples, tutorials, and evaluation methods to assess the performance of the components.
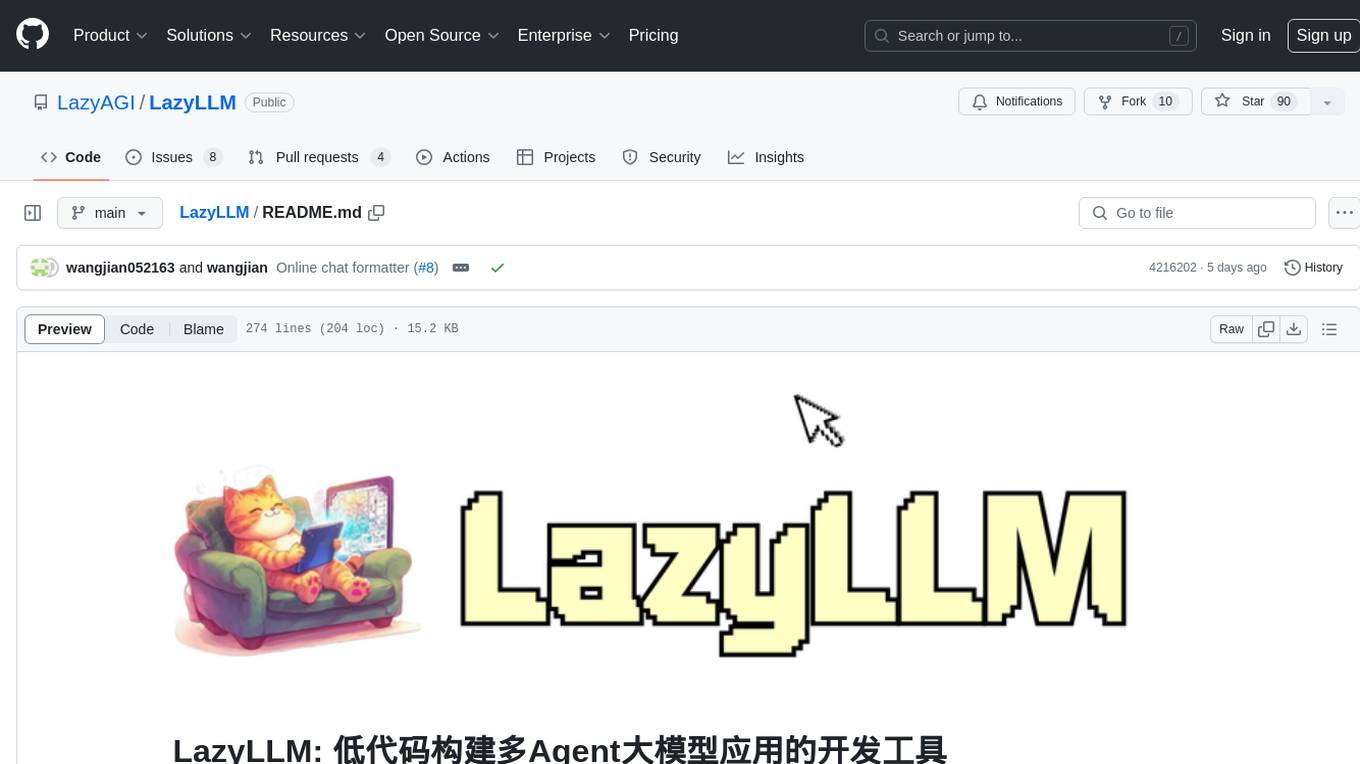
LazyLLM
LazyLLM is a low-code development tool for building complex AI applications with multiple agents. It assists developers in building AI applications at a low cost and continuously optimizing their performance. The tool provides a convenient workflow for application development and offers standard processes and tools for various stages of application development. Users can quickly prototype applications with LazyLLM, analyze bad cases with scenario task data, and iteratively optimize key components to enhance the overall application performance. LazyLLM aims to simplify the AI application development process and provide flexibility for both beginners and experts to create high-quality applications.
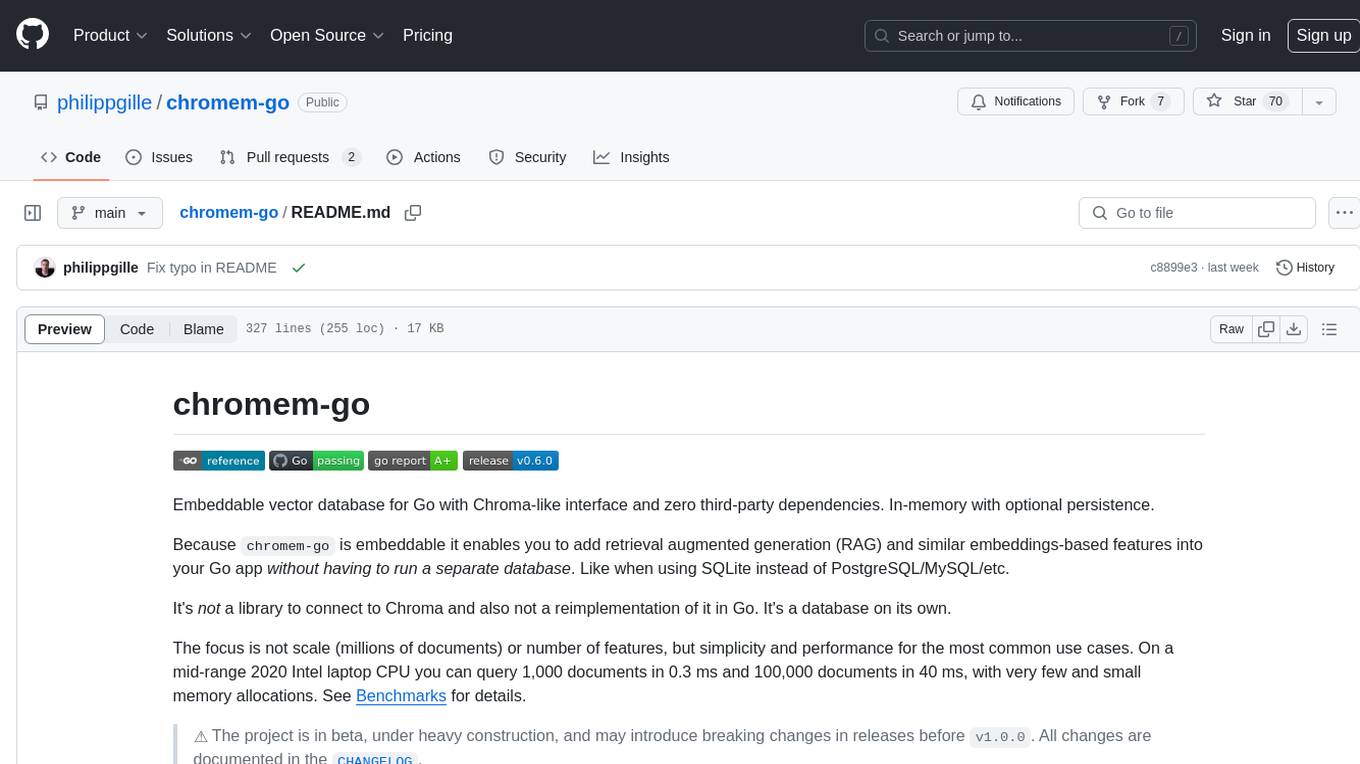
chromem-go
chromem-go is an embeddable vector database for Go with a Chroma-like interface and zero third-party dependencies. It enables retrieval augmented generation (RAG) and similar embeddings-based features in Go apps without the need for a separate database. The focus is on simplicity and performance for common use cases, allowing querying of documents with minimal memory allocations. The project is in beta and may introduce breaking changes before v1.0.0.
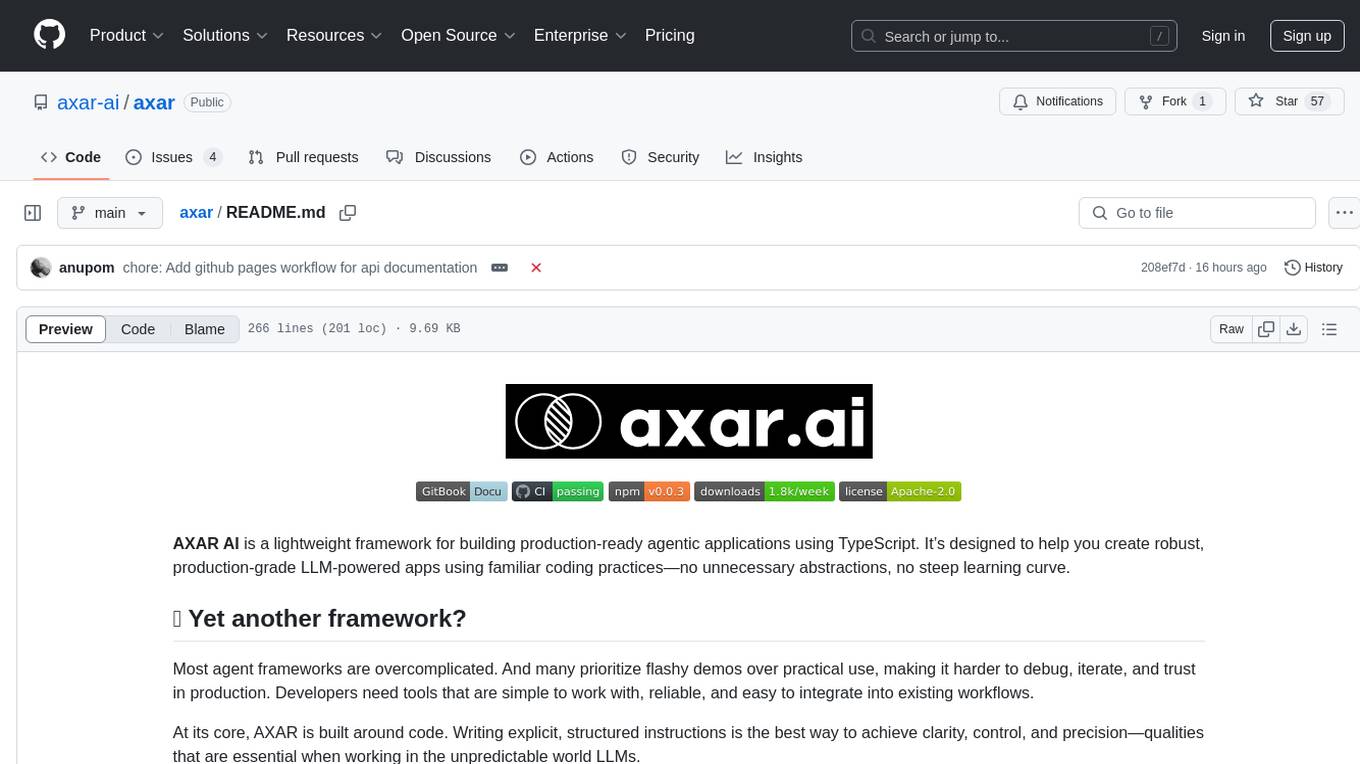
axar
AXAR AI is a lightweight framework designed for building production-ready agentic applications using TypeScript. It aims to simplify the process of creating robust, production-grade LLM-powered apps by focusing on familiar coding practices without unnecessary abstractions or steep learning curves. The framework provides structured, typed inputs and outputs, familiar and intuitive patterns like dependency injection and decorators, explicit control over agent behavior, real-time logging and monitoring tools, minimalistic design with little overhead, model agnostic compatibility with various AI models, and streamed outputs for fast and accurate results. AXAR AI is ideal for developers working on real-world AI applications who want a tool that gets out of the way and allows them to focus on shipping reliable software.
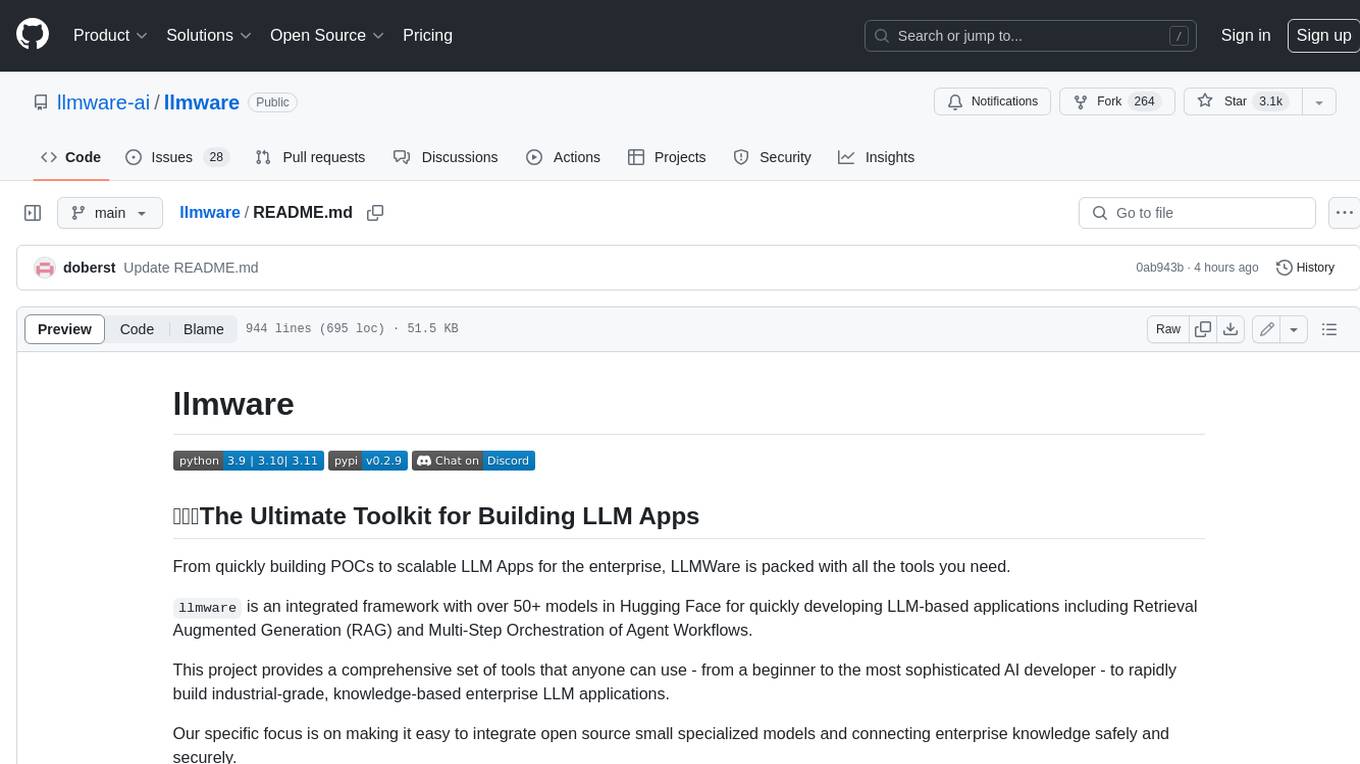
llmware
LLMWare is a framework for quickly developing LLM-based applications including Retrieval Augmented Generation (RAG) and Multi-Step Orchestration of Agent Workflows. This project provides a comprehensive set of tools that anyone can use - from a beginner to the most sophisticated AI developer - to rapidly build industrial-grade, knowledge-based enterprise LLM applications. Our specific focus is on making it easy to integrate open source small specialized models and connecting enterprise knowledge safely and securely.
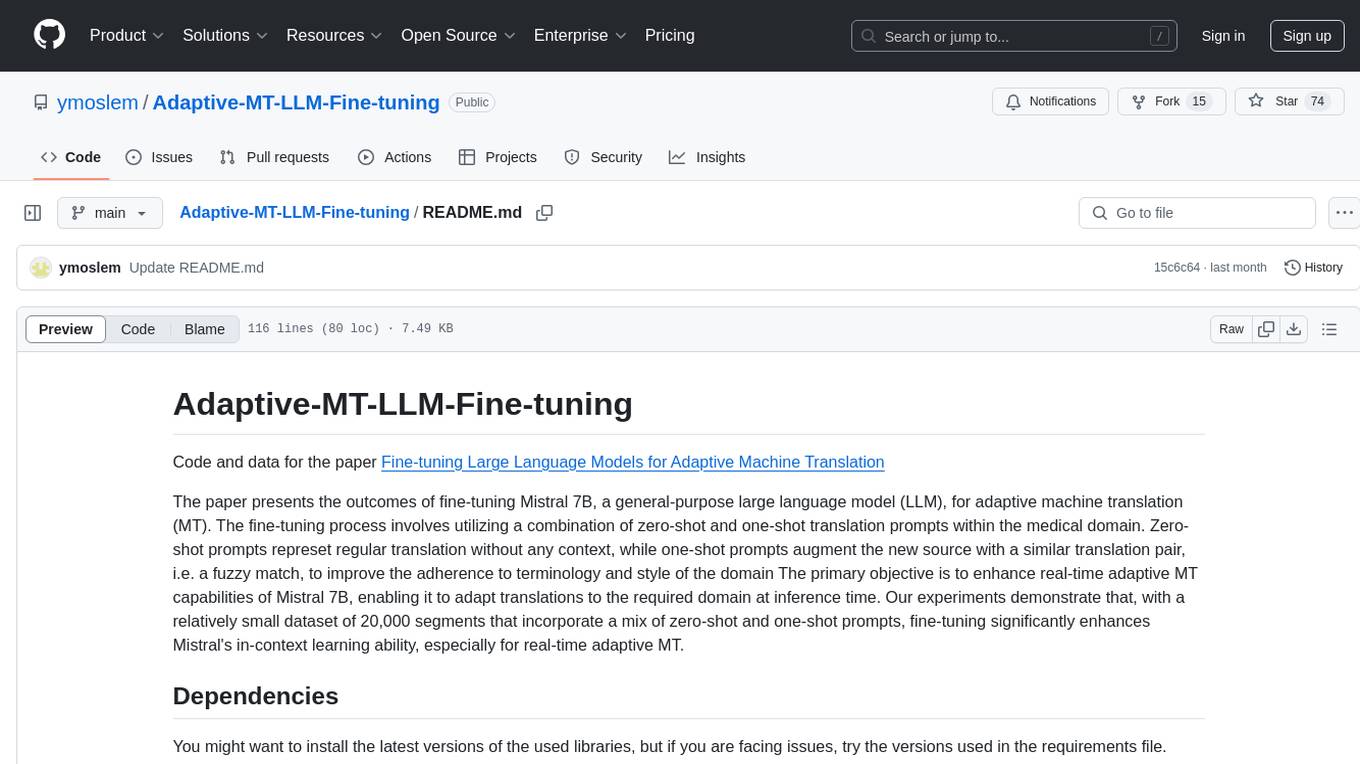
Adaptive-MT-LLM-Fine-tuning
The repository Adaptive-MT-LLM-Fine-tuning contains code and data for the paper 'Fine-tuning Large Language Models for Adaptive Machine Translation'. It focuses on enhancing Mistral 7B, a large language model, for real-time adaptive machine translation in the medical domain. The fine-tuning process involves using zero-shot and one-shot translation prompts to improve terminology and style adherence. The repository includes training and test data, data processing code, fuzzy match retrieval techniques, fine-tuning methods, conversion to CTranslate2 format, tokenizers, translation codes, and evaluation metrics.
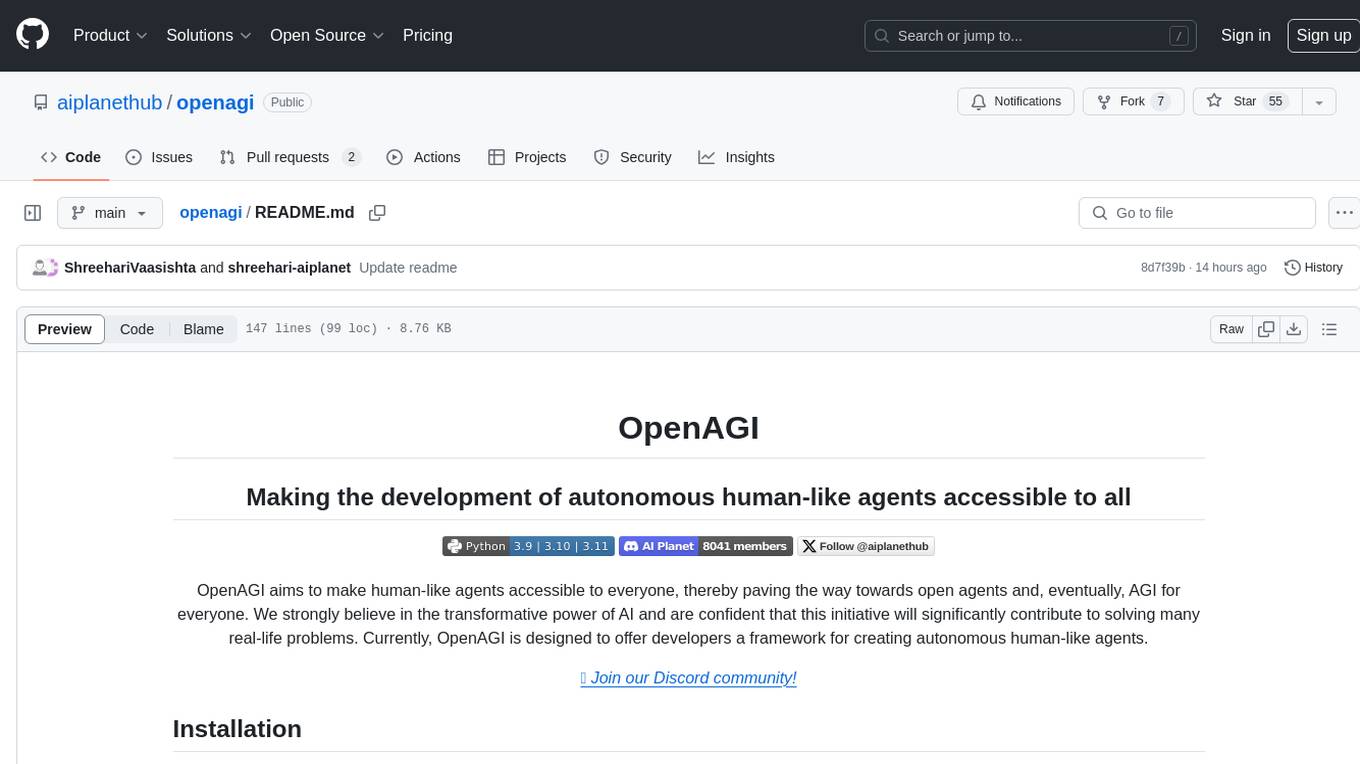
openagi
OpenAGI is a framework designed to make the development of autonomous human-like agents accessible to all. It aims to pave the way towards open agents and eventually AGI for everyone. The initiative strongly believes in the transformative power of AI and offers developers a platform to create autonomous human-like agents. OpenAGI features a flexible agent architecture, streamlined integration and configuration processes, and automated/manual agent configuration generation. It can be used in education for personalized learning experiences, in finance and banking for fraud detection and personalized banking advice, and in healthcare for patient monitoring and disease diagnosis.
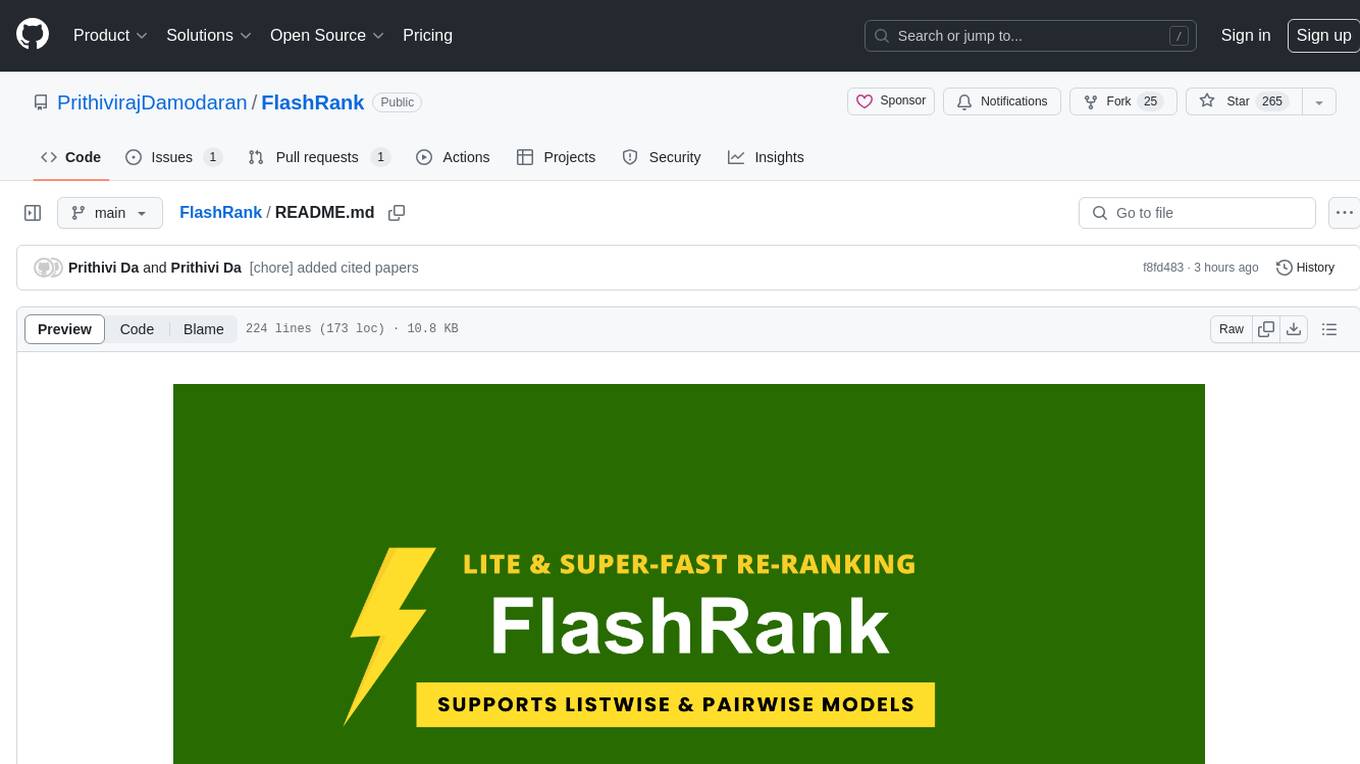
FlashRank
FlashRank is an ultra-lite and super-fast Python library designed to add re-ranking capabilities to existing search and retrieval pipelines. It is based on state-of-the-art Language Models (LLMs) and cross-encoders, offering support for pairwise/pointwise rerankers and listwise LLM-based rerankers. The library boasts the tiniest reranking model in the world (~4MB) and runs on CPU without the need for Torch or Transformers. FlashRank is cost-conscious, with a focus on low cost per invocation and smaller package size for efficient serverless deployments. It supports various models like ms-marco-TinyBERT, ms-marco-MiniLM, rank-T5-flan, ms-marco-MultiBERT, and more, with plans for future model additions. The tool is ideal for enhancing search precision and speed in scenarios where lightweight models with competitive performance are preferred.
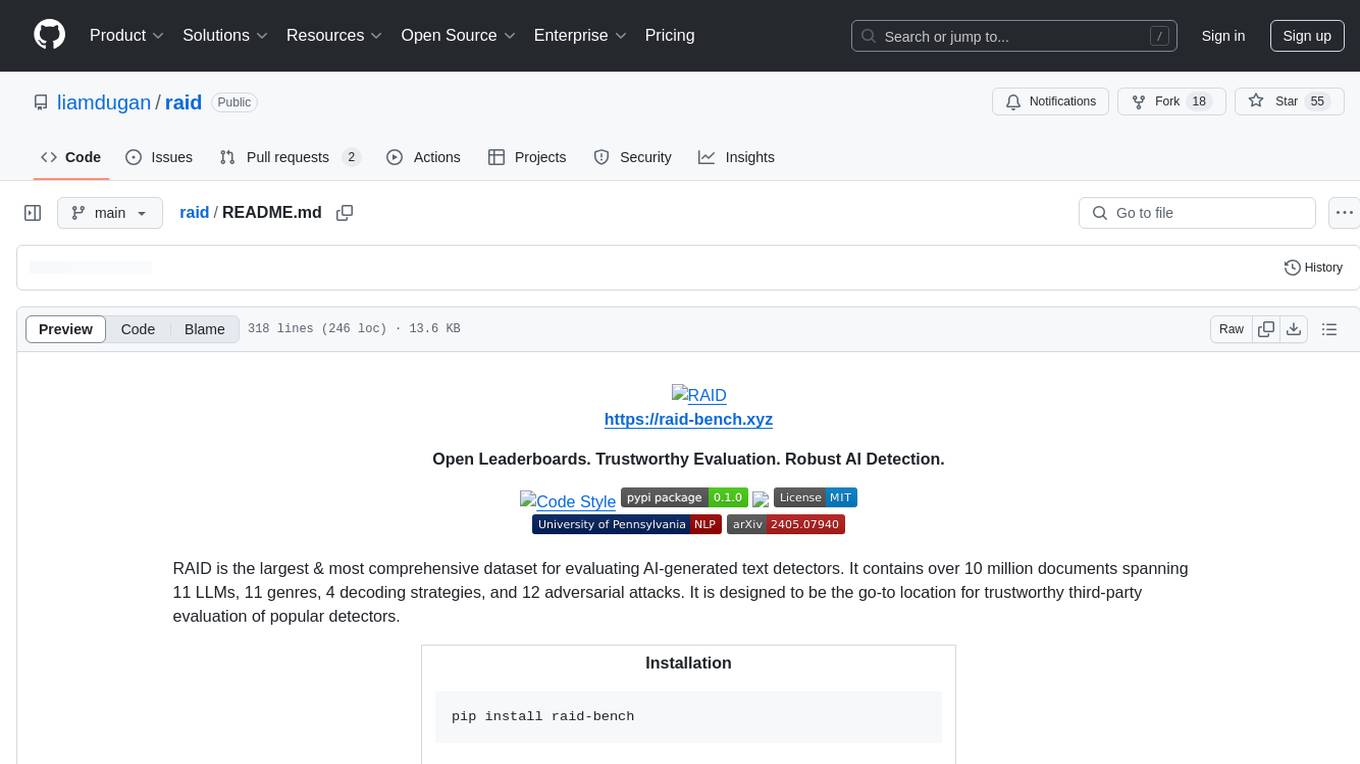
raid
RAID is the largest and most comprehensive dataset for evaluating AI-generated text detectors. It contains over 10 million documents spanning 11 LLMs, 11 genres, 4 decoding strategies, and 12 adversarial attacks. RAID is designed to be the go-to location for trustworthy third-party evaluation of popular detectors. The dataset covers diverse models, domains, sampling strategies, and attacks, making it a valuable resource for training detectors, evaluating generalization, protecting against adversaries, and comparing to state-of-the-art models from academia and industry.
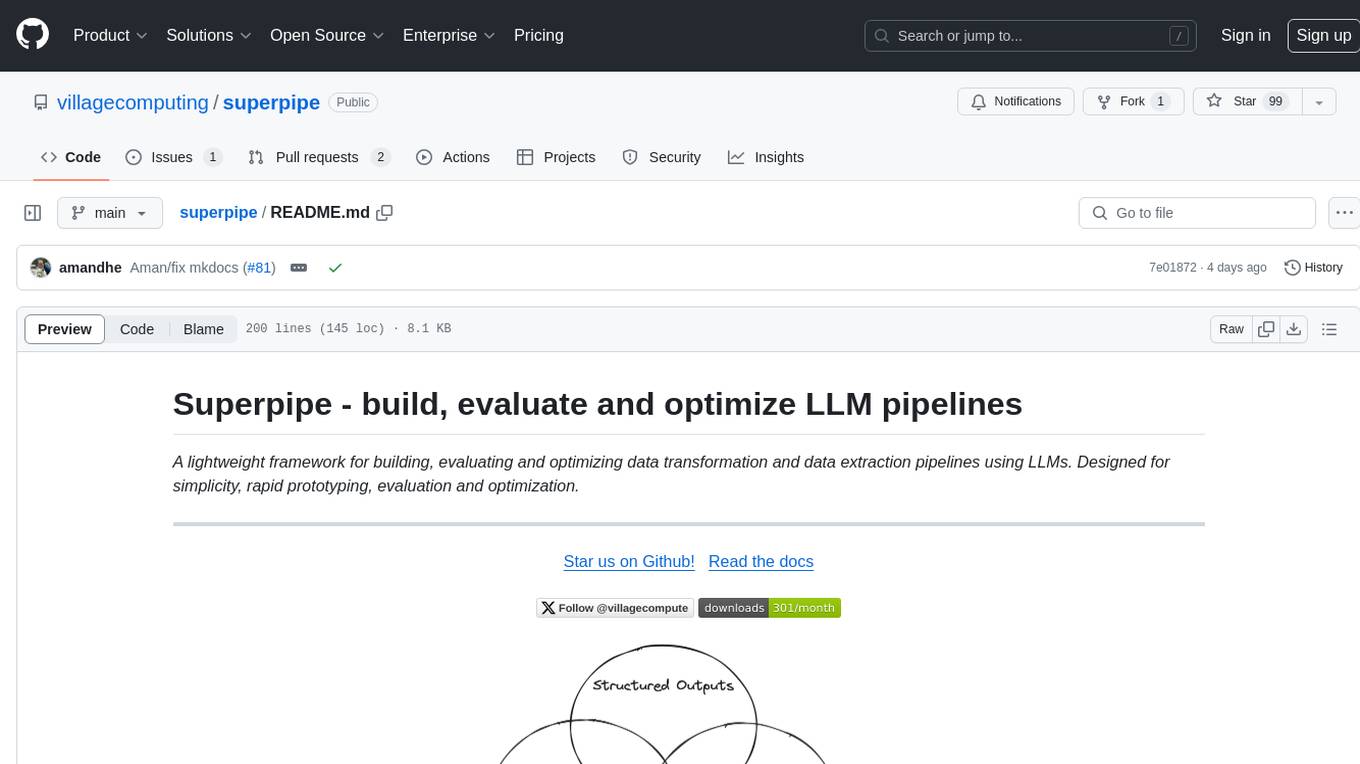
superpipe
Superpipe is a lightweight framework designed for building, evaluating, and optimizing data transformation and data extraction pipelines using LLMs. It allows users to easily combine their favorite LLM libraries with Superpipe's building blocks to create pipelines tailored to their unique data and use cases. The tool facilitates rapid prototyping, evaluation, and optimization of end-to-end pipelines for tasks such as classification and evaluation of job departments based on work history. Superpipe also provides functionalities for evaluating pipeline performance, optimizing parameters for cost, accuracy, and speed, and conducting grid searches to experiment with different models and prompts.
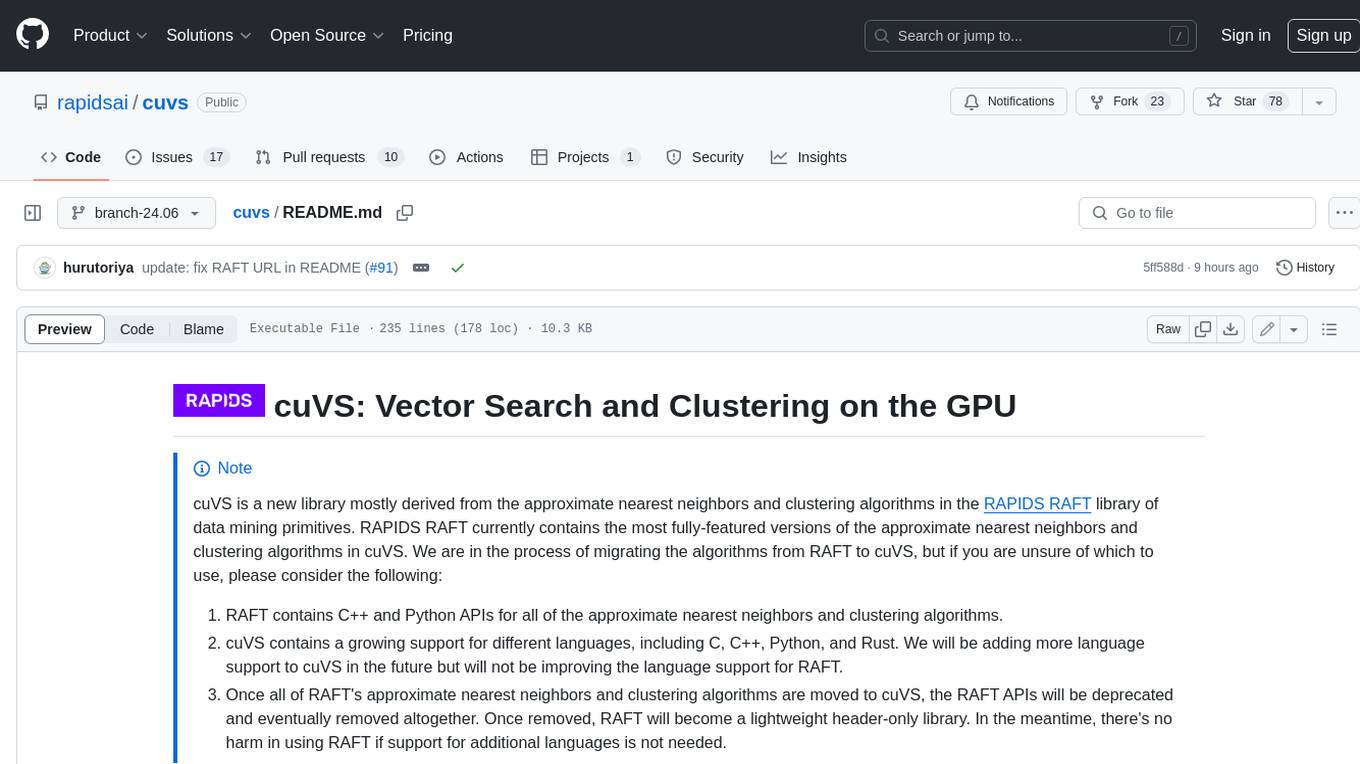
cuvs
cuVS is a library that contains state-of-the-art implementations of several algorithms for running approximate nearest neighbors and clustering on the GPU. It can be used directly or through the various databases and other libraries that have integrated it. The primary goal of cuVS is to simplify the use of GPUs for vector similarity search and clustering.
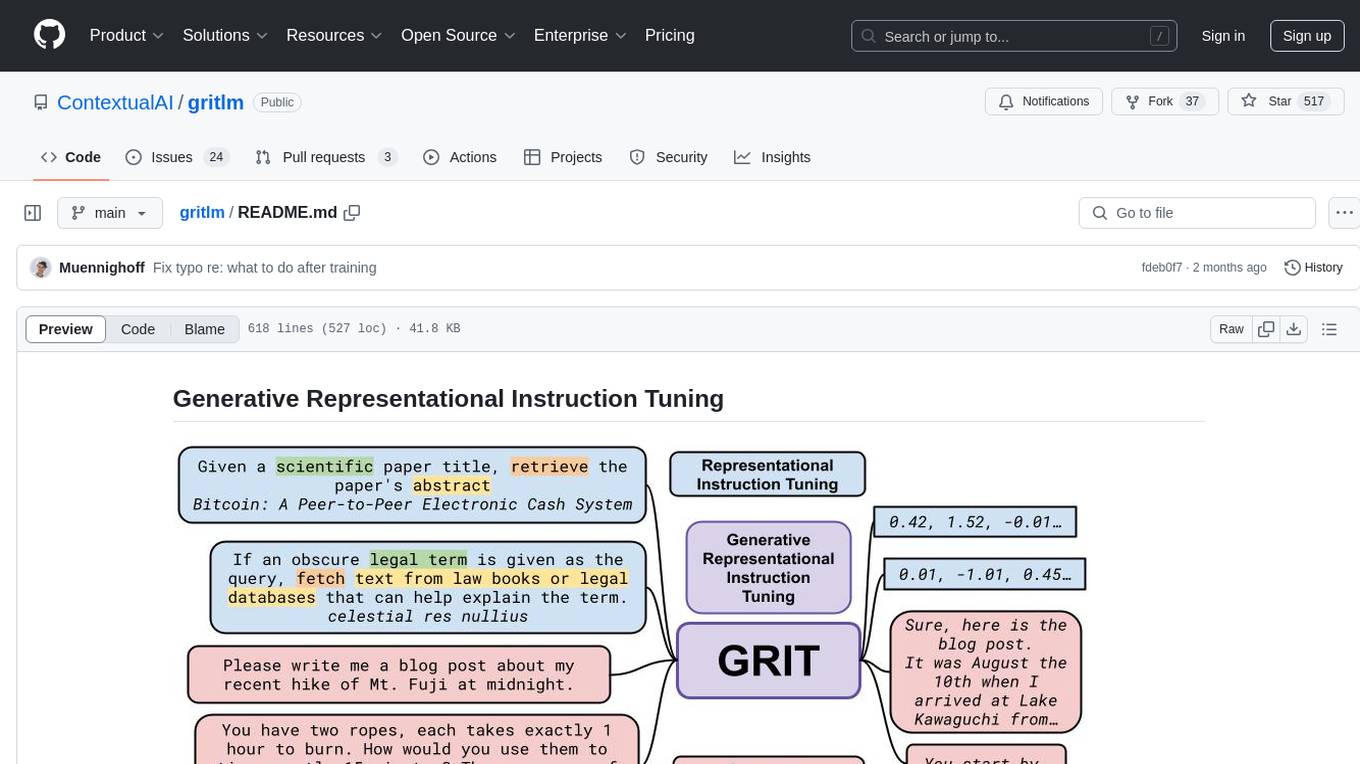
gritlm
The 'gritlm' repository provides all materials for the paper Generative Representational Instruction Tuning. It includes code for inference, training, evaluation, and known issues related to the GritLM model. The repository also offers models for embedding and generation tasks, along with instructions on how to train and evaluate the models. Additionally, it contains visualizations, acknowledgements, and a citation for referencing the work.
For similar tasks
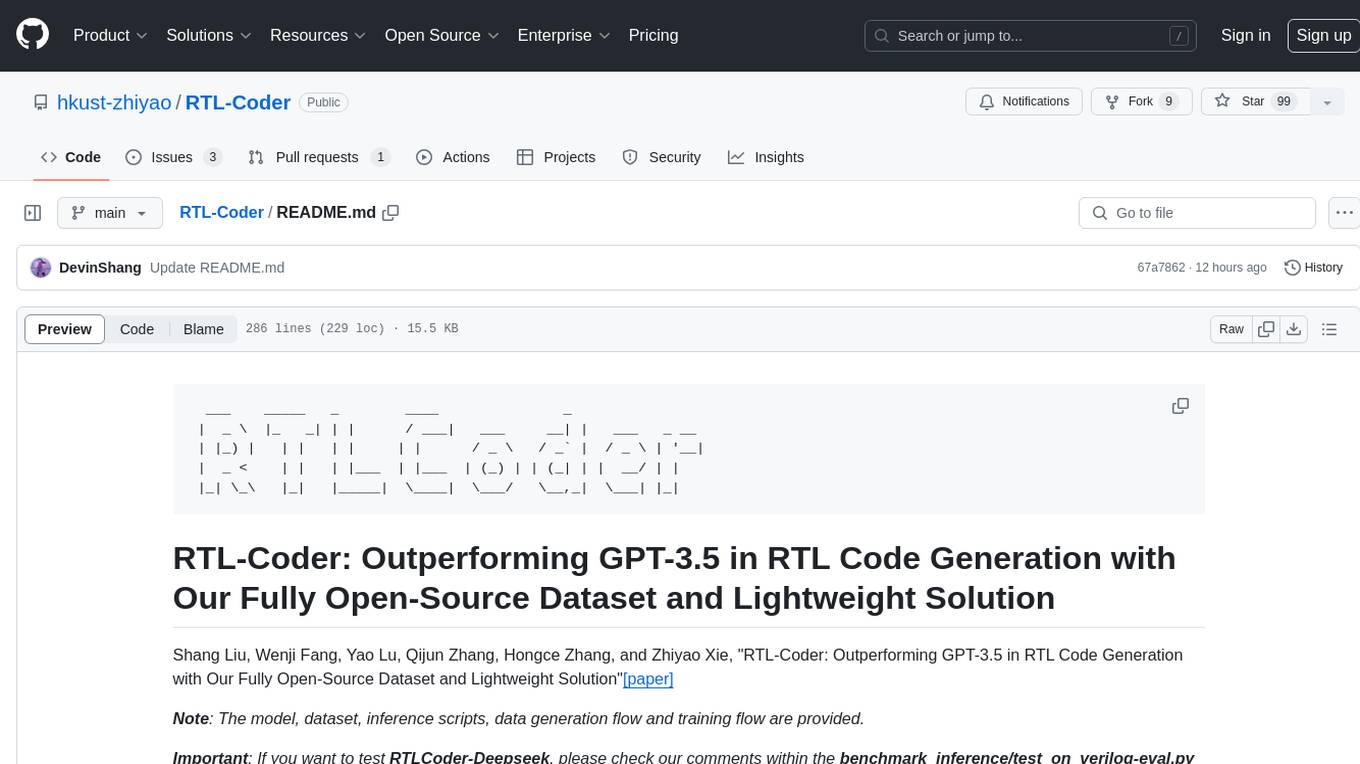
RTL-Coder
RTL-Coder is a tool designed to outperform GPT-3.5 in RTL code generation by providing a fully open-source dataset and a lightweight solution. It targets Verilog code generation and offers an automated flow to generate a large labeled dataset with over 27,000 diverse Verilog design problems and answers. The tool addresses the data availability challenge in IC design-related tasks and can be used for various applications beyond LLMs. The tool includes four RTL code generation models available on the HuggingFace platform, each with specific features and performance characteristics. Additionally, RTL-Coder introduces a new LLM training scheme based on code quality feedback to further enhance model performance and reduce GPU memory consumption.
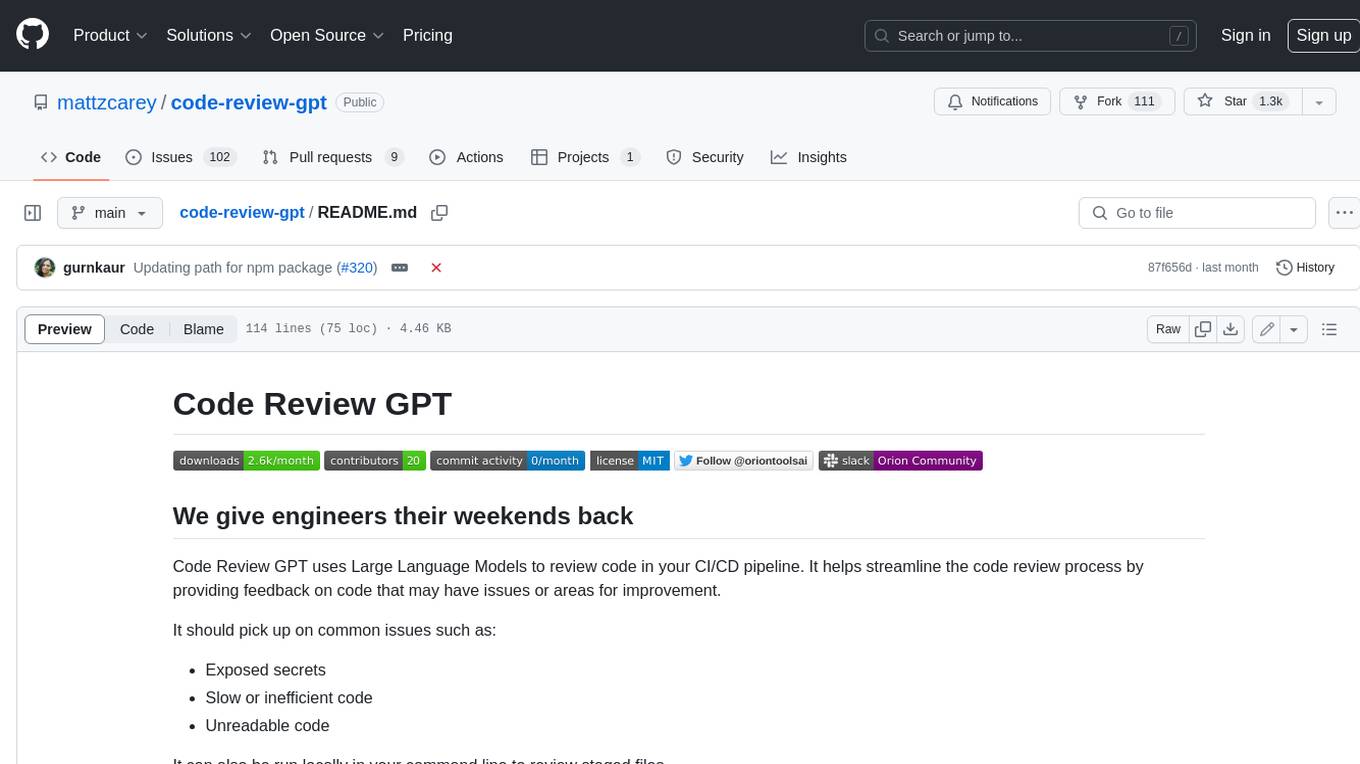
code-review-gpt
Code Review GPT uses Large Language Models to review code in your CI/CD pipeline. It helps streamline the code review process by providing feedback on code that may have issues or areas for improvement. It should pick up on common issues such as exposed secrets, slow or inefficient code, and unreadable code. It can also be run locally in your command line to review staged files. Code Review GPT is in alpha and should be used for fun only. It may provide useful feedback but please check any suggestions thoroughly.
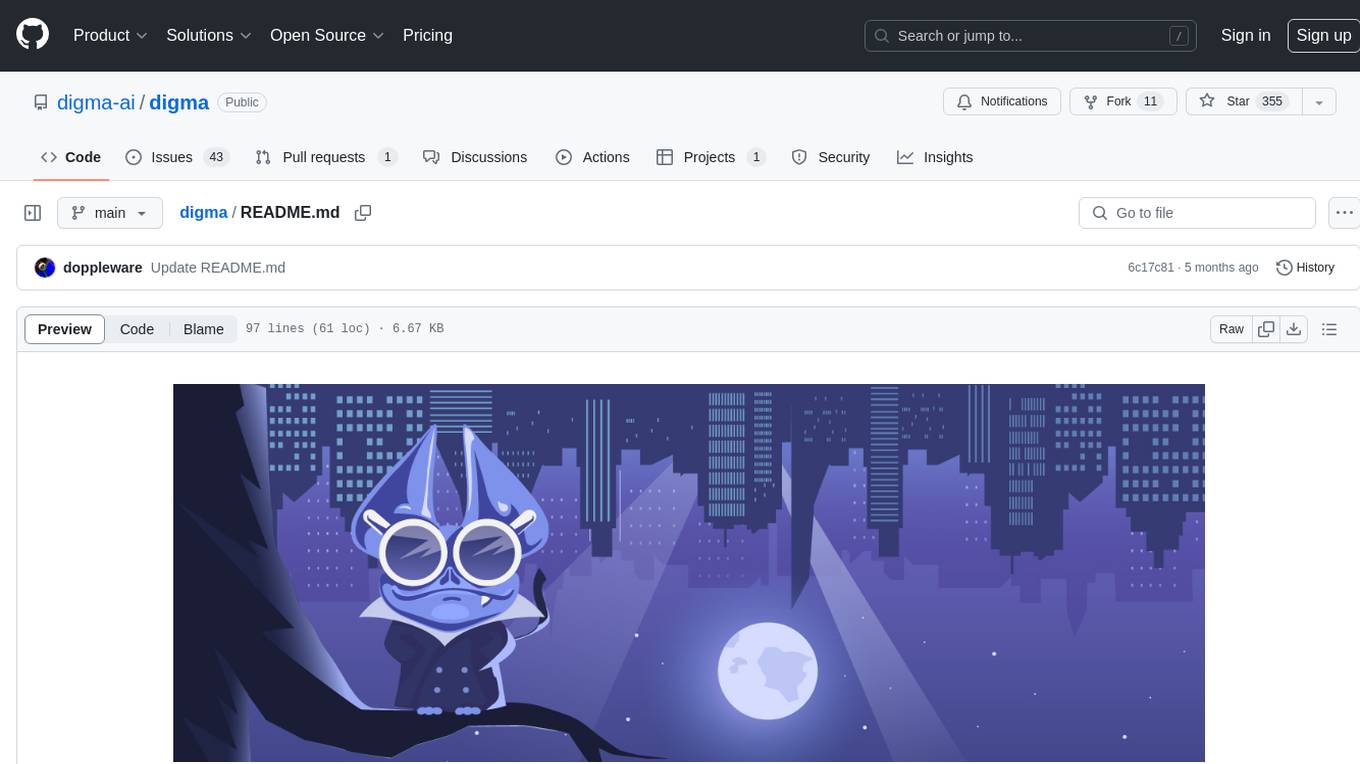
digma
Digma is a Continuous Feedback platform that provides code-level insights related to performance, errors, and usage during development. It empowers developers to own their code all the way to production, improving code quality and preventing critical issues. Digma integrates with OpenTelemetry traces and metrics to generate insights in the IDE, helping developers analyze code scalability, bottlenecks, errors, and usage patterns.
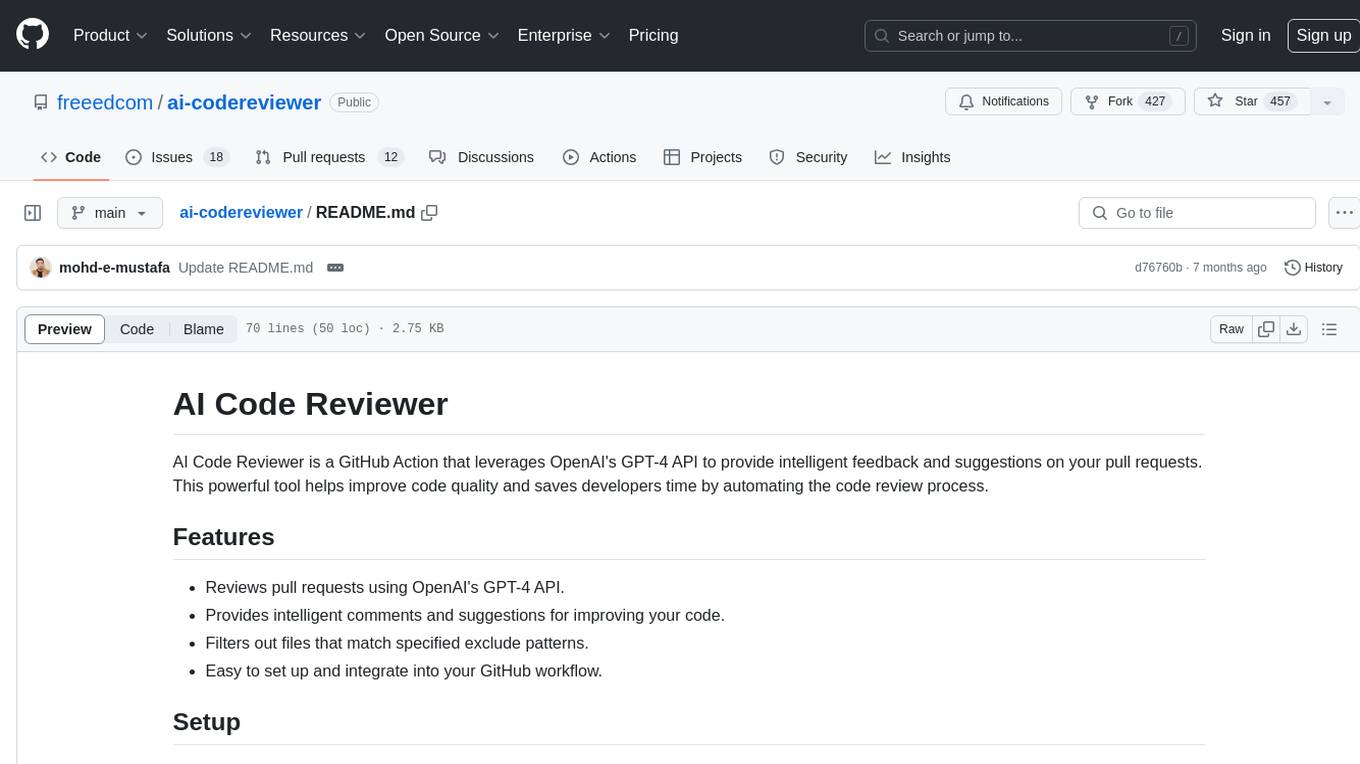
ai-codereviewer
AI Code Reviewer is a GitHub Action that utilizes OpenAI's GPT-4 API to provide intelligent feedback and suggestions on pull requests. It helps enhance code quality and streamline the code review process by offering insightful comments and filtering out specified files. The tool is easy to set up and integrate into GitHub workflows.
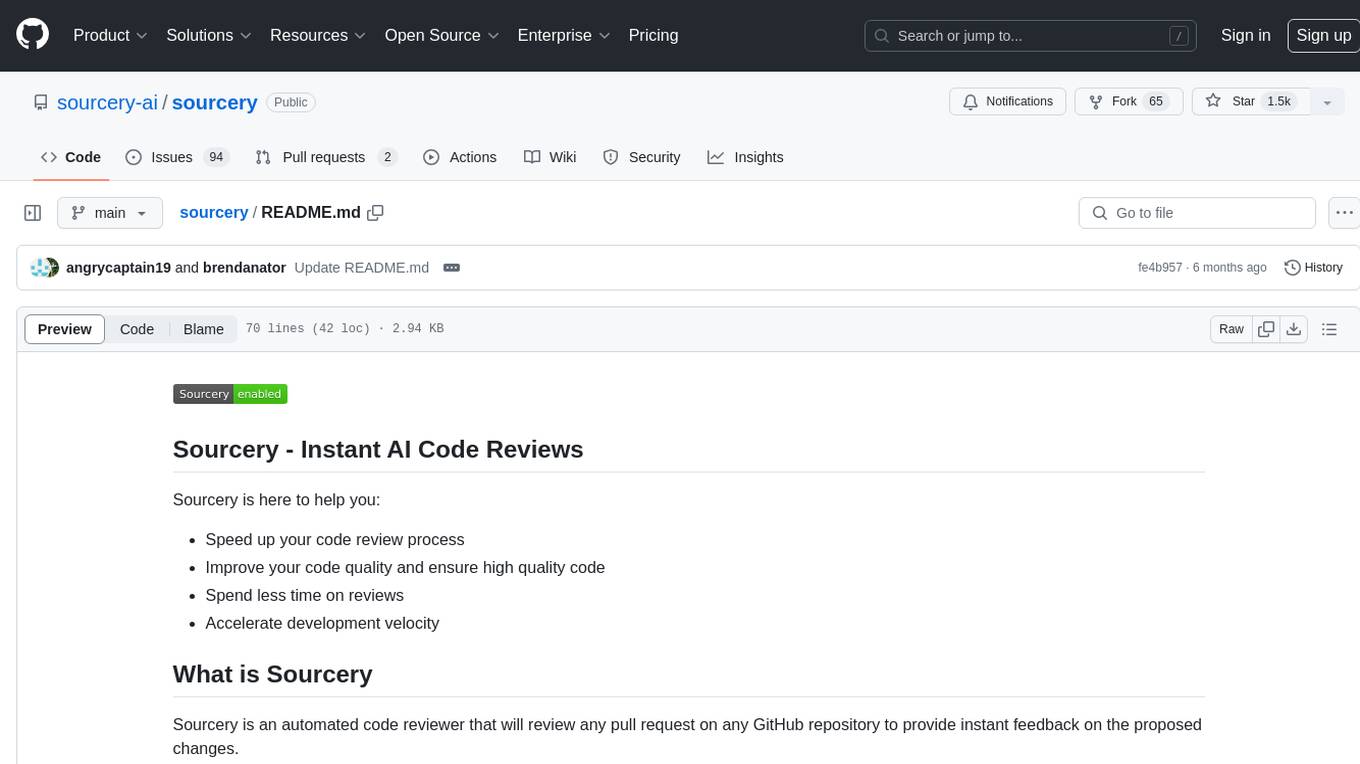
sourcery
Sourcery is an automated code reviewer tool that provides instant feedback on pull requests, helping to speed up the code review process, improve code quality, and accelerate development velocity. It offers high-level feedback, line-by-line suggestions, and aims to mimic the type of code review one would expect from a colleague. Sourcery can also be used as an IDE coding assistant to understand existing code, add unit tests, optimize code, and improve code quality with instant suggestions. It is free for public repos/open source projects and offers a 14-day trial for private repos.
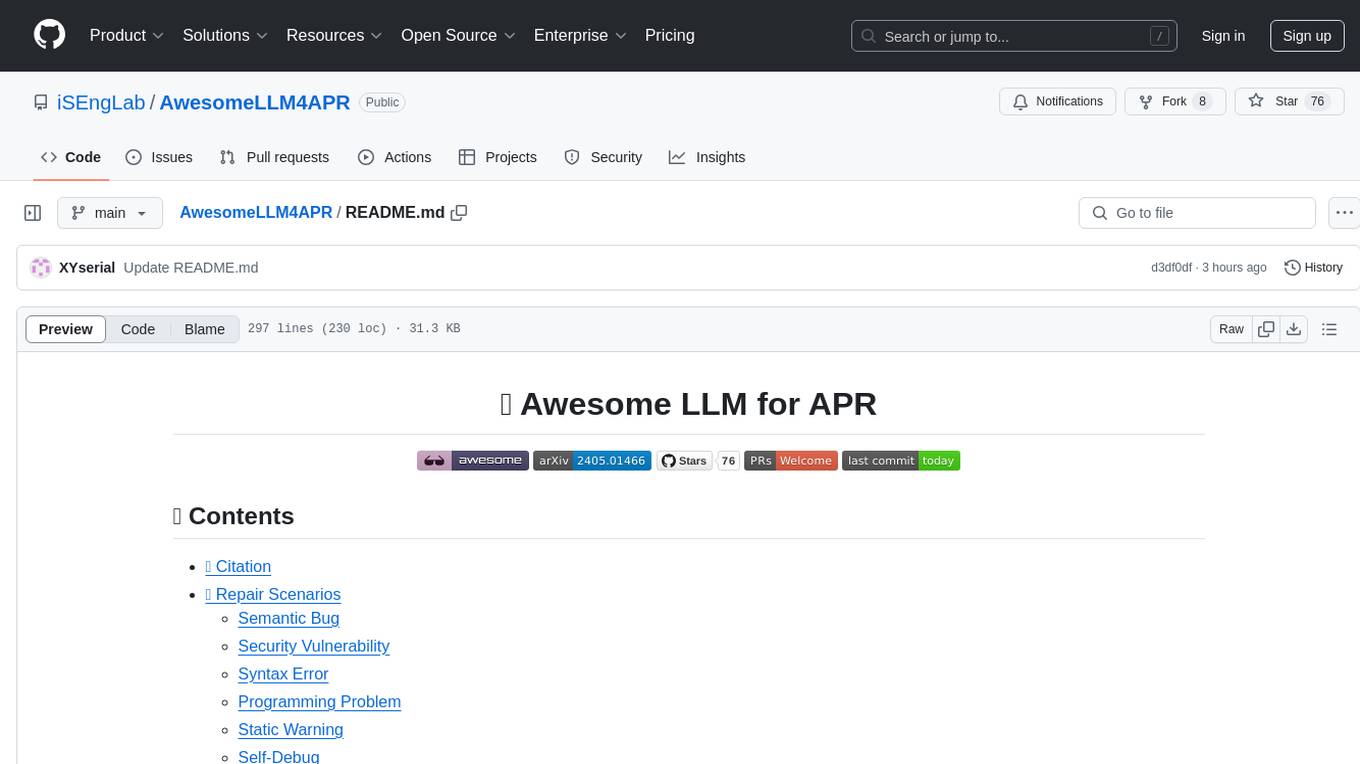
AwesomeLLM4APR
Awesome LLM for APR is a repository dedicated to exploring the capabilities of Large Language Models (LLMs) in Automated Program Repair (APR). It provides a comprehensive collection of research papers, tools, and resources related to using LLMs for various scenarios such as repairing semantic bugs, security vulnerabilities, syntax errors, programming problems, static warnings, self-debugging, type errors, web UI tests, smart contracts, hardware bugs, performance bugs, API misuses, crash bugs, test case repairs, formal proofs, GitHub issues, code reviews, motion planners, human studies, and patch correctness assessments. The repository serves as a valuable reference for researchers and practitioners interested in leveraging LLMs for automated program repair.
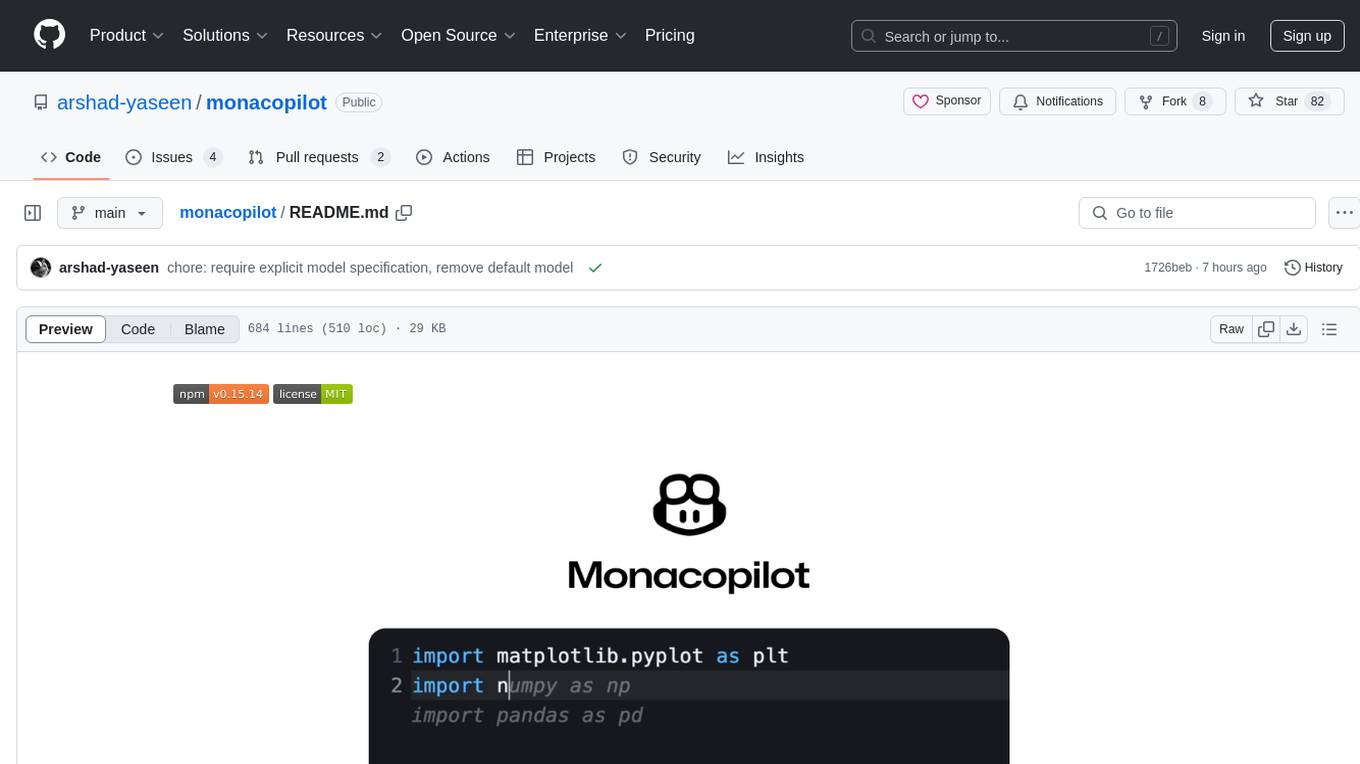
monacopilot
Monacopilot is a powerful and customizable AI auto-completion plugin for the Monaco Editor. It supports multiple AI providers such as Anthropic, OpenAI, Groq, and Google, providing real-time code completions with an efficient caching system. The plugin offers context-aware suggestions, customizable completion behavior, and framework agnostic features. Users can also customize the model support and trigger completions manually. Monacopilot is designed to enhance coding productivity by providing accurate and contextually appropriate completions in daily spoken language.
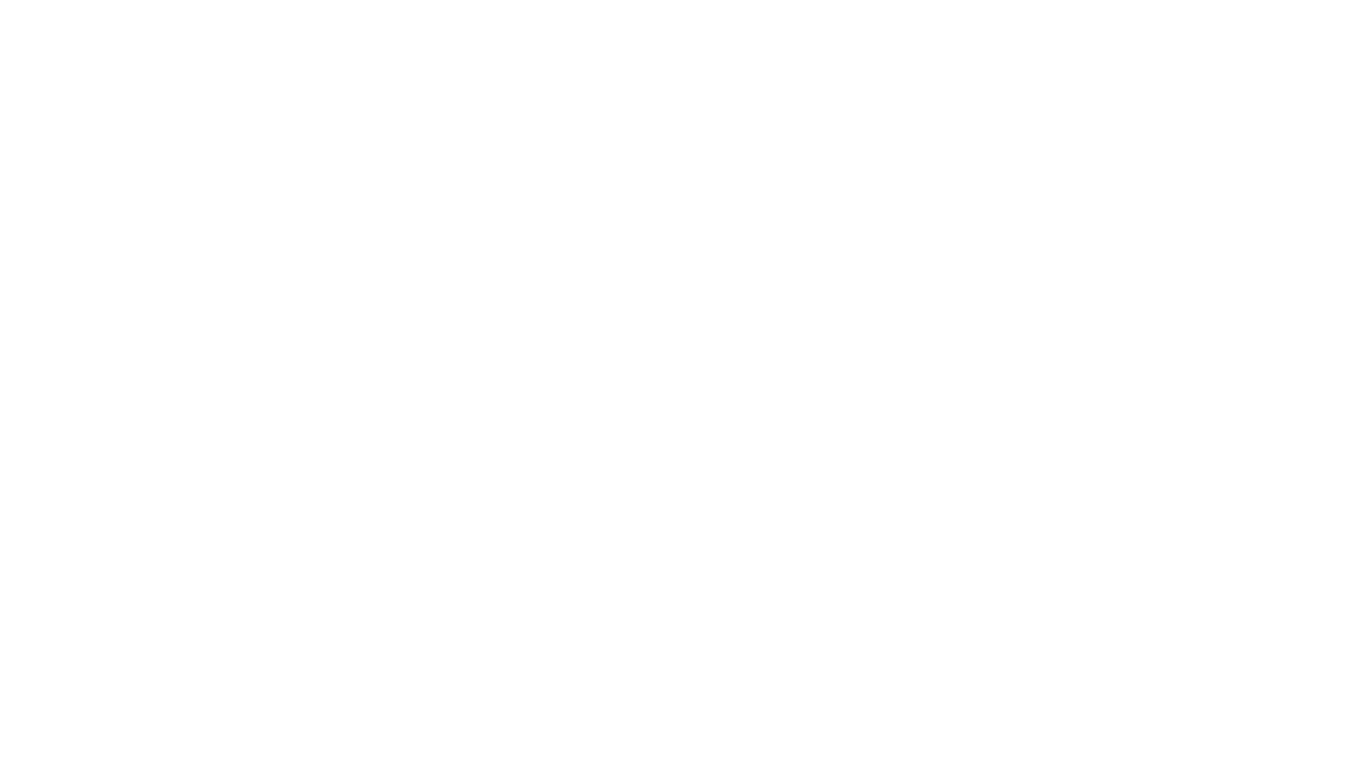
gemini-ai-code-reviewer
Gemini AI Code Reviewer is a GitHub Action that automatically reviews pull requests using Google's Gemini AI. It analyzes code changes, consults the Gemini model, provides feedback, and delivers review comments directly to pull requests on GitHub. Users need a Gemini API key and can trigger the workflow by commenting '/gemini-review' in the PR. The tool helps improve source code quality by giving suggestions and comments for enhancement.
For similar jobs
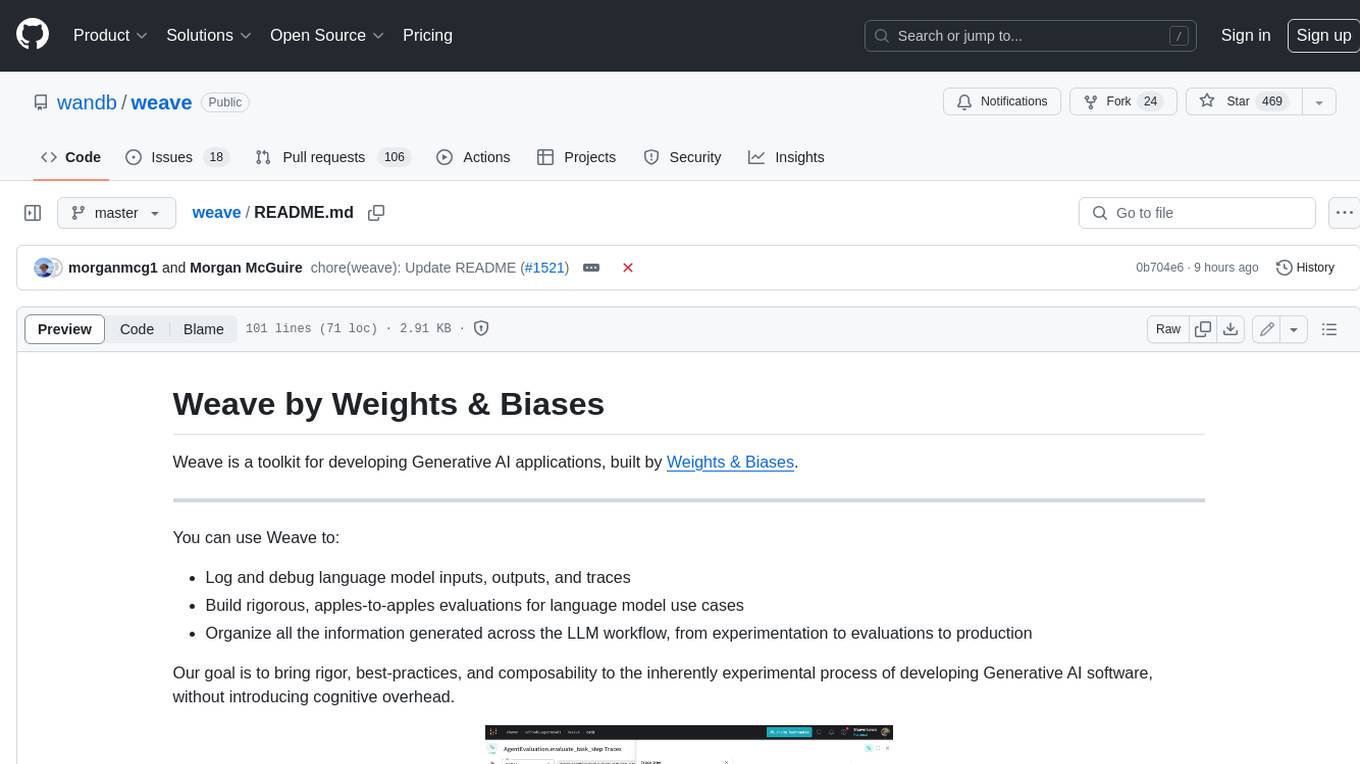
weave
Weave is a toolkit for developing Generative AI applications, built by Weights & Biases. With Weave, you can log and debug language model inputs, outputs, and traces; build rigorous, apples-to-apples evaluations for language model use cases; and organize all the information generated across the LLM workflow, from experimentation to evaluations to production. Weave aims to bring rigor, best-practices, and composability to the inherently experimental process of developing Generative AI software, without introducing cognitive overhead.
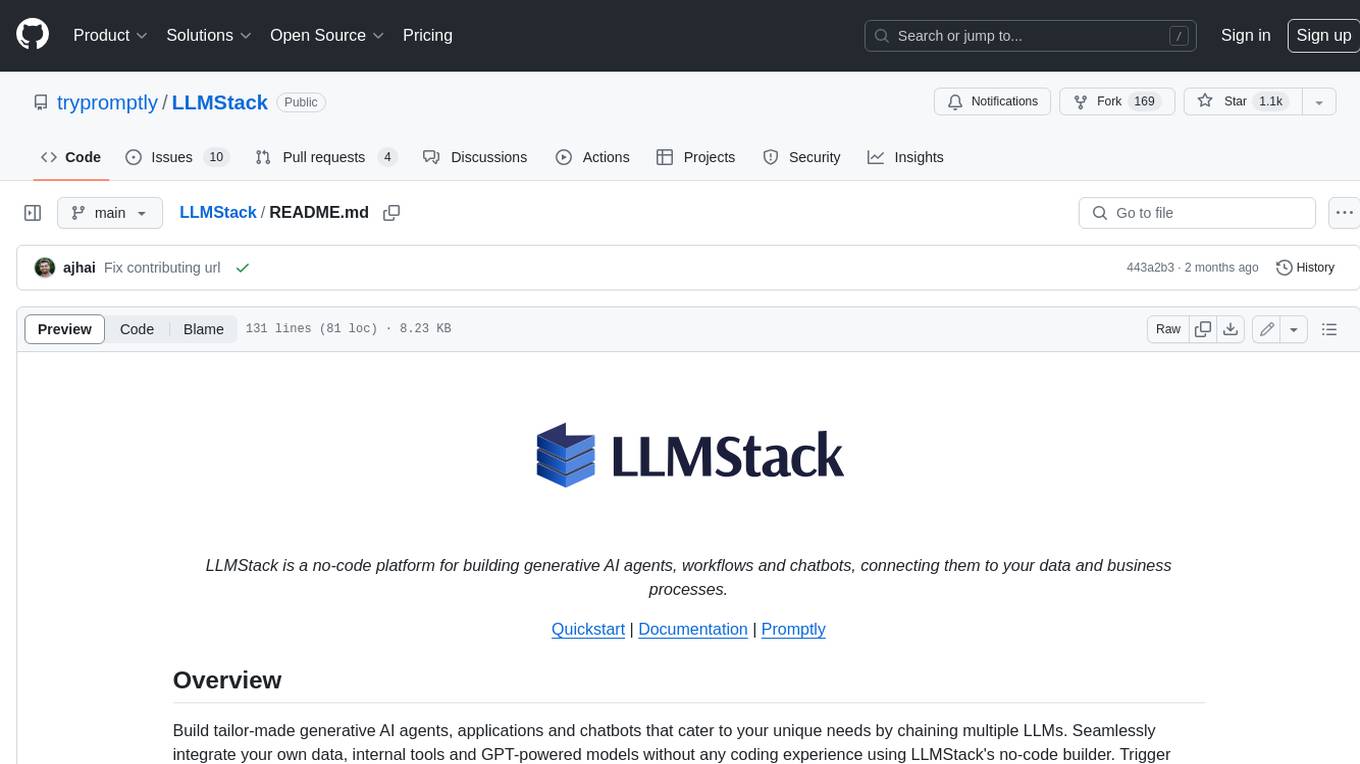
LLMStack
LLMStack is a no-code platform for building generative AI agents, workflows, and chatbots. It allows users to connect their own data, internal tools, and GPT-powered models without any coding experience. LLMStack can be deployed to the cloud or on-premise and can be accessed via HTTP API or triggered from Slack or Discord.
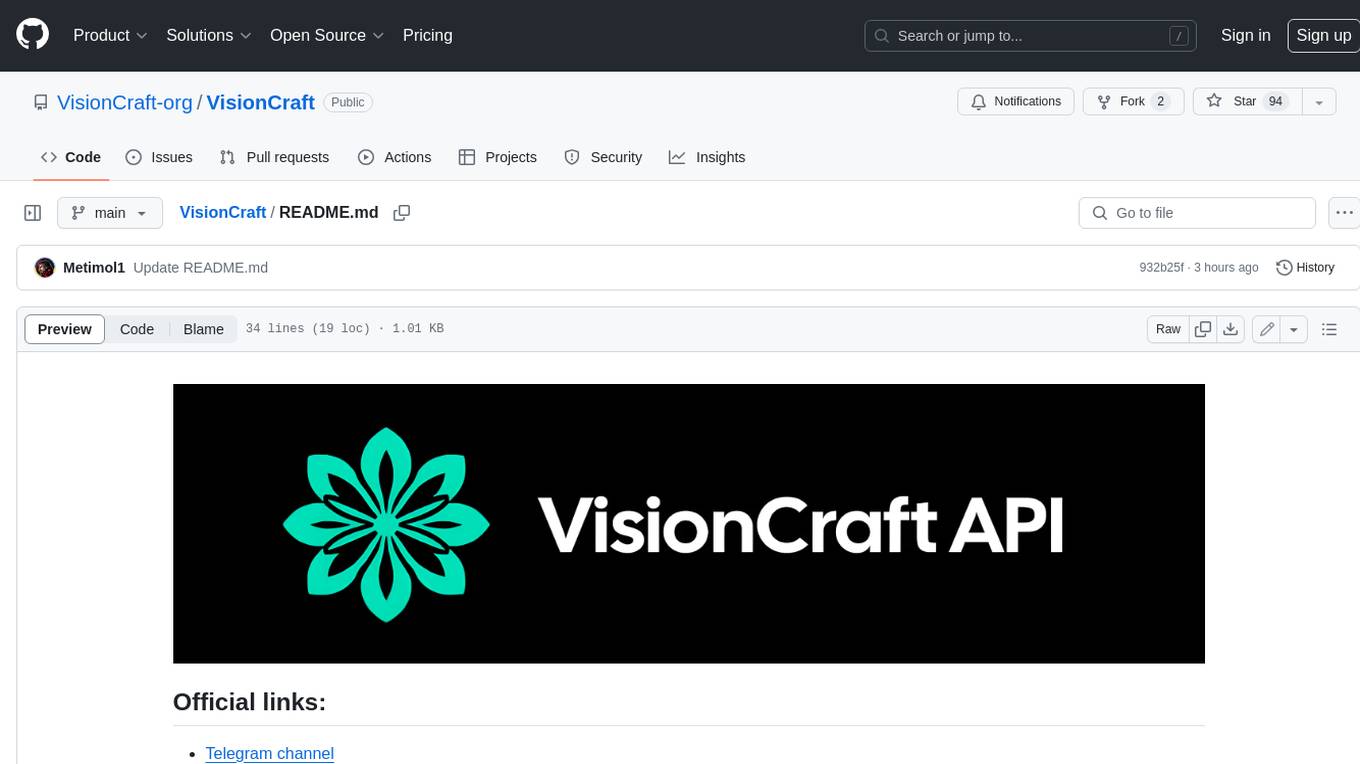
VisionCraft
The VisionCraft API is a free API for using over 100 different AI models. From images to sound.
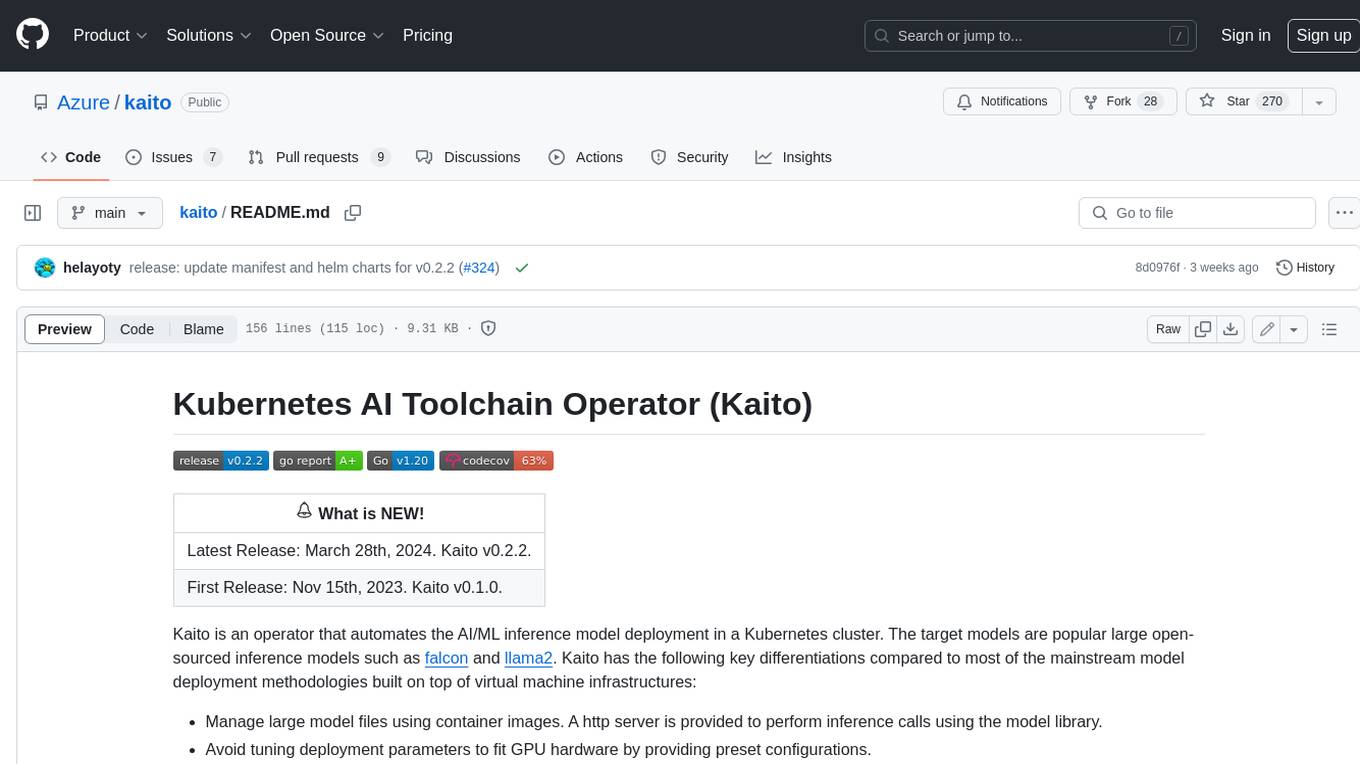
kaito
Kaito is an operator that automates the AI/ML inference model deployment in a Kubernetes cluster. It manages large model files using container images, avoids tuning deployment parameters to fit GPU hardware by providing preset configurations, auto-provisions GPU nodes based on model requirements, and hosts large model images in the public Microsoft Container Registry (MCR) if the license allows. Using Kaito, the workflow of onboarding large AI inference models in Kubernetes is largely simplified.
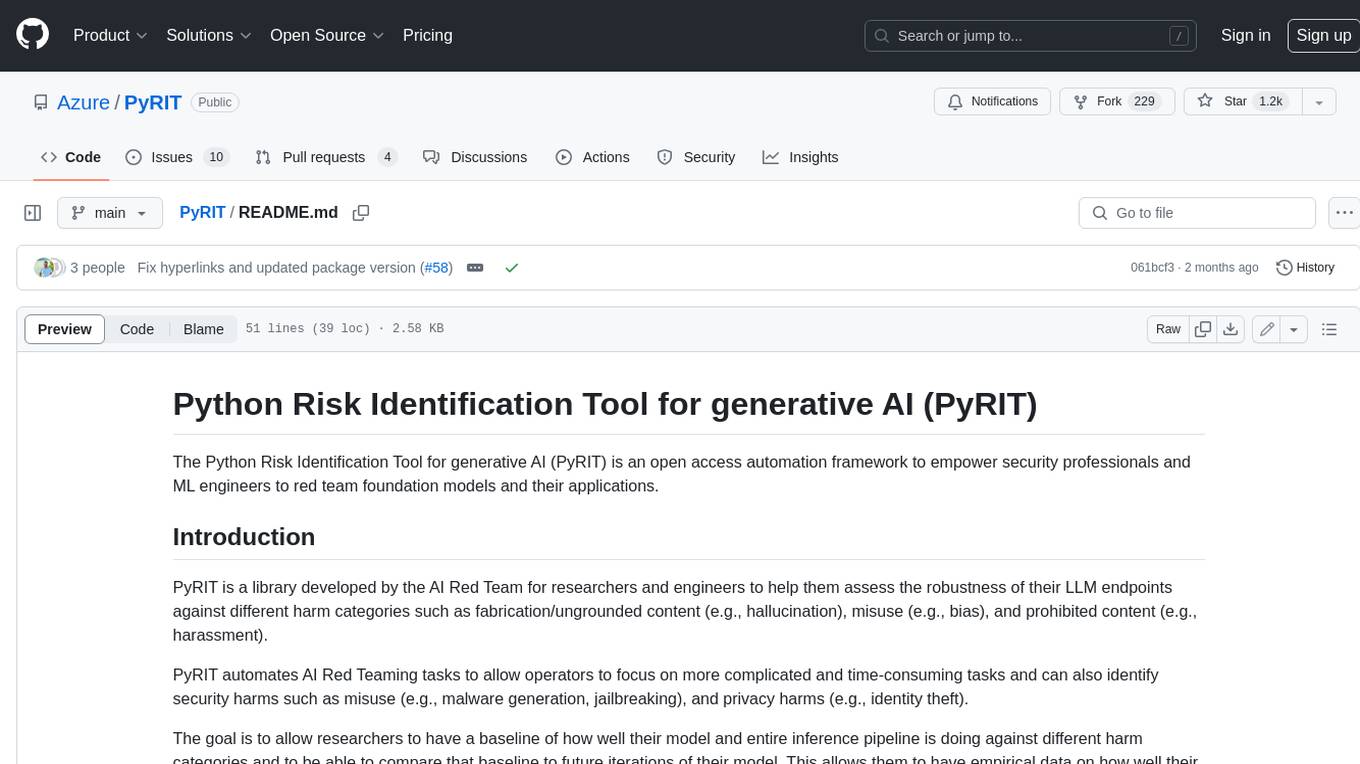
PyRIT
PyRIT is an open access automation framework designed to empower security professionals and ML engineers to red team foundation models and their applications. It automates AI Red Teaming tasks to allow operators to focus on more complicated and time-consuming tasks and can also identify security harms such as misuse (e.g., malware generation, jailbreaking), and privacy harms (e.g., identity theft). The goal is to allow researchers to have a baseline of how well their model and entire inference pipeline is doing against different harm categories and to be able to compare that baseline to future iterations of their model. This allows them to have empirical data on how well their model is doing today, and detect any degradation of performance based on future improvements.
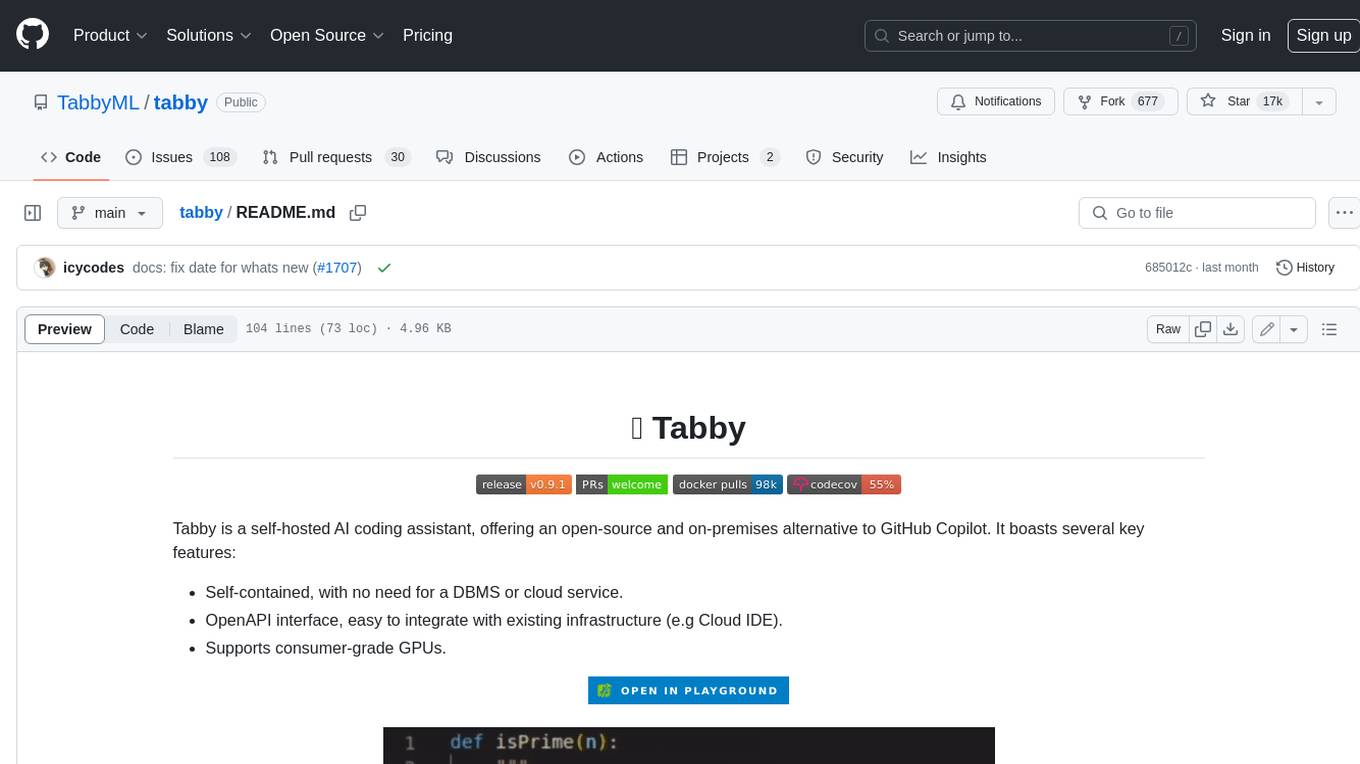
tabby
Tabby is a self-hosted AI coding assistant, offering an open-source and on-premises alternative to GitHub Copilot. It boasts several key features: * Self-contained, with no need for a DBMS or cloud service. * OpenAPI interface, easy to integrate with existing infrastructure (e.g Cloud IDE). * Supports consumer-grade GPUs.
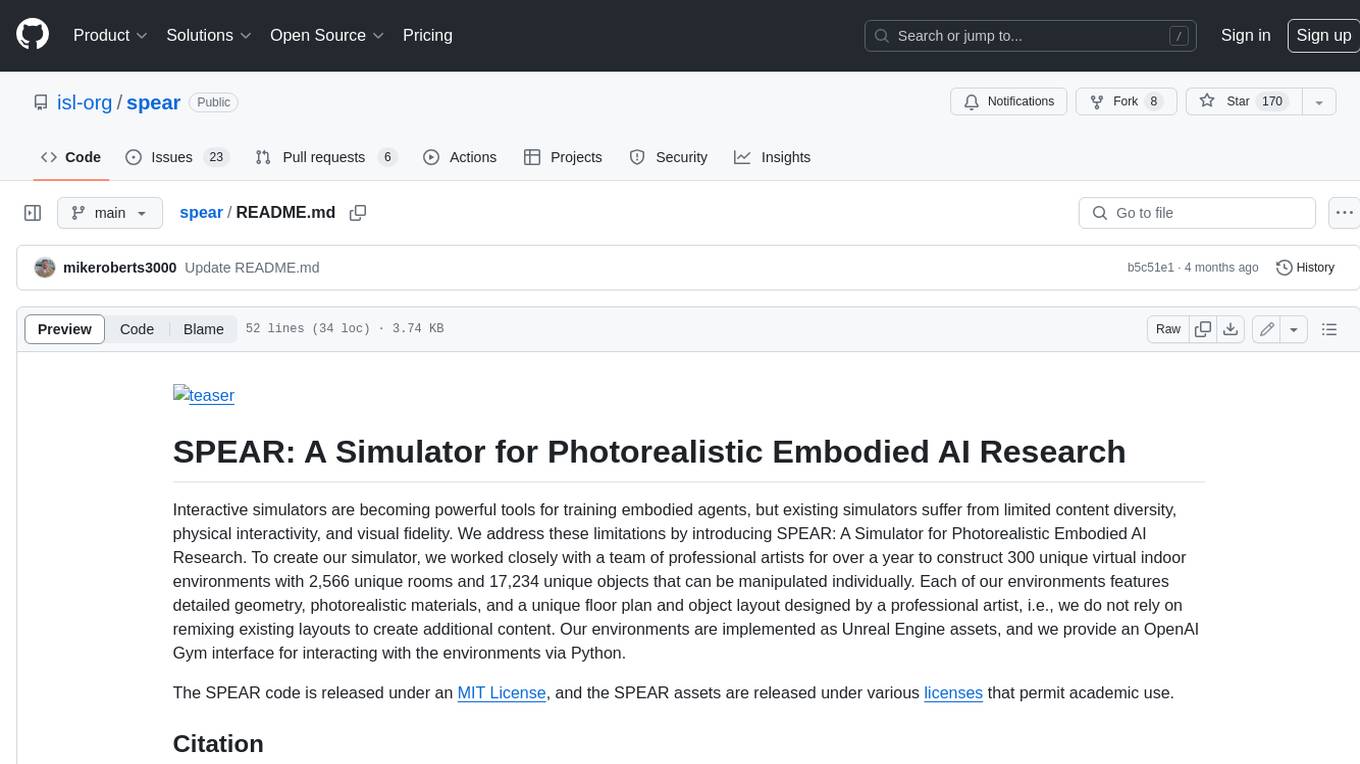
spear
SPEAR (Simulator for Photorealistic Embodied AI Research) is a powerful tool for training embodied agents. It features 300 unique virtual indoor environments with 2,566 unique rooms and 17,234 unique objects that can be manipulated individually. Each environment is designed by a professional artist and features detailed geometry, photorealistic materials, and a unique floor plan and object layout. SPEAR is implemented as Unreal Engine assets and provides an OpenAI Gym interface for interacting with the environments via Python.
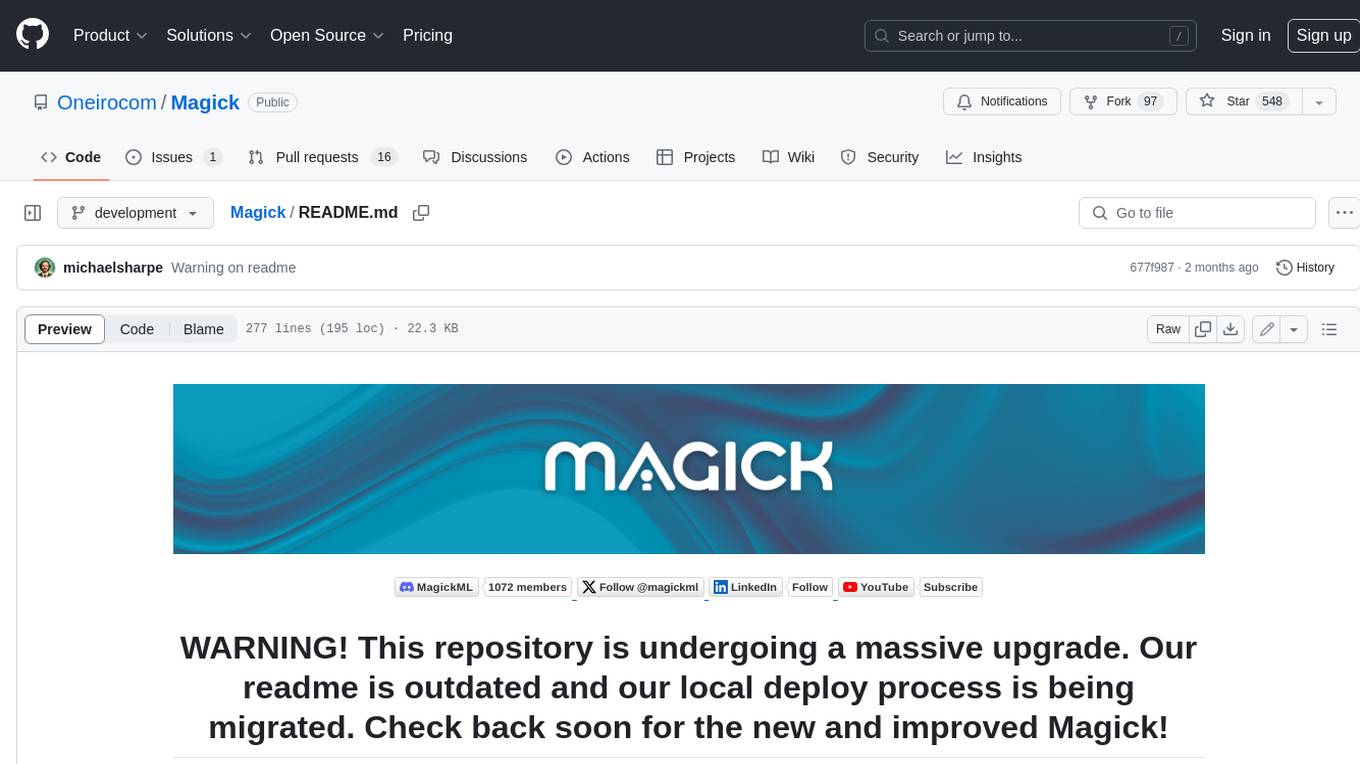
Magick
Magick is a groundbreaking visual AIDE (Artificial Intelligence Development Environment) for no-code data pipelines and multimodal agents. Magick can connect to other services and comes with nodes and templates well-suited for intelligent agents, chatbots, complex reasoning systems and realistic characters.