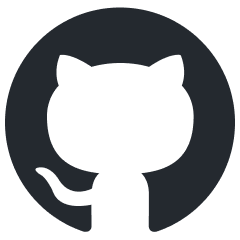
cuvs
cuVS - a library for vector search and clustering on the GPU
Stars: 323
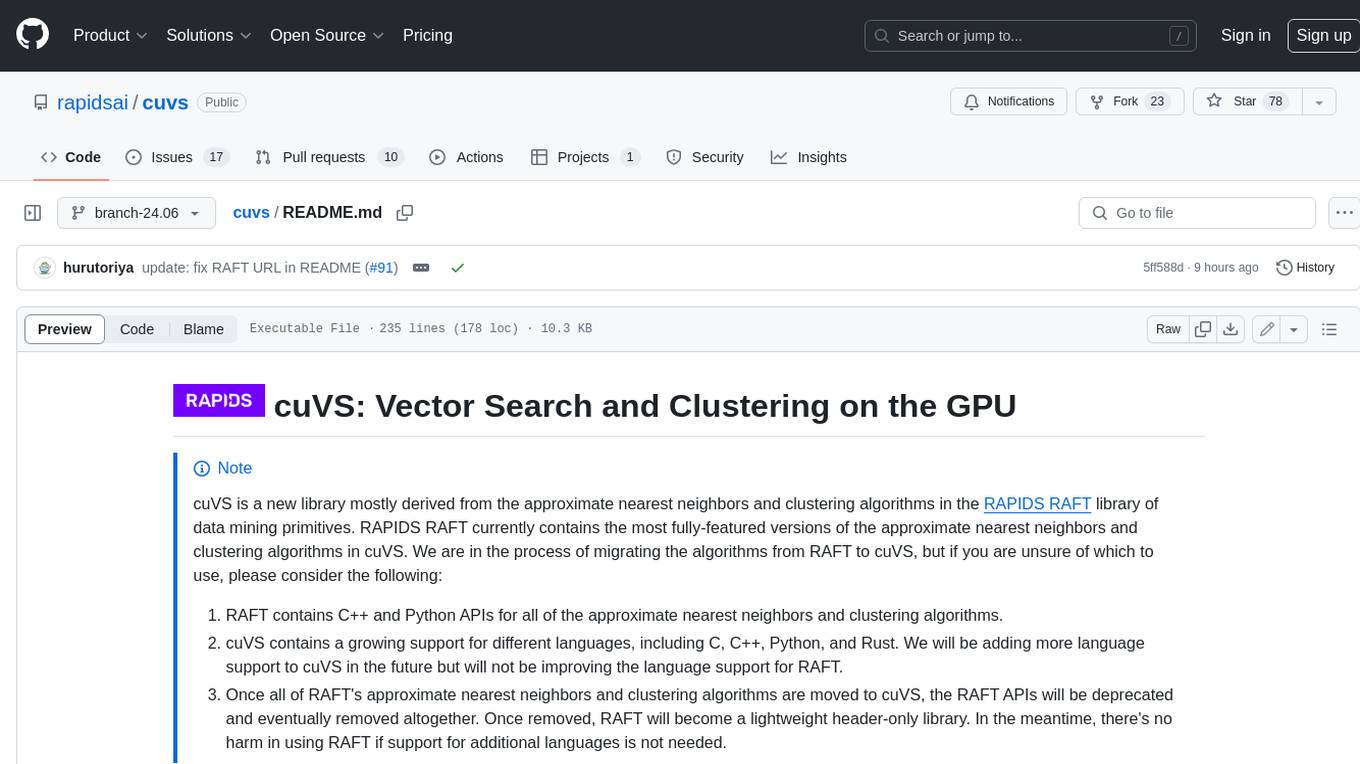
cuVS is a library that contains state-of-the-art implementations of several algorithms for running approximate nearest neighbors and clustering on the GPU. It can be used directly or through the various databases and other libraries that have integrated it. The primary goal of cuVS is to simplify the use of GPUs for vector similarity search and clustering.
README:
[!note] cuVS is a new library mostly derived from the approximate nearest neighbors and clustering algorithms in the RAPIDS RAFT library of machine learning and data mining primitives. As of version 24.10 (Release in October 2024), cuVS contains the most fully-featured versions of the approximate nearest neighbors and clustering algorithms from RAFT. The algorithms which have been migrated over to cuVS will be removed from RAFT in version 24.12 (released in December 2024).
- Documentation: Library documentation.
- Build and Install Guide: Instructions for installing and building cuVS.
- Getting Started Guide: Guide to getting started with cuVS.
- Code Examples: Self-contained Code Examples.
- API Reference Documentation: API Documentation.
- RAPIDS Community: Get help, contribute, and collaborate.
- GitHub repository: Download the cuVS source code.
- Issue tracker: Report issues or request features.
cuVS contains state-of-the-art implementations of several algorithms for running approximate nearest neighbors and clustering on the GPU. It can be used directly or through the various databases and other libraries that have integrated it. The primary goal of cuVS is to simplify the use of GPUs for vector similarity search and clustering.
Vector search is an information retrieval method that has been growing in popularity over the past few years, partly because of the rising importance of multimedia embeddings created from unstructured data and the need to perform semantic search on the embeddings to find items which are semantically similar to each other.
Vector search is also used in data mining and machine learning tasks and comprises an important step in many clustering and visualization algorithms like UMAP, t-SNE, K-means, and HDBSCAN.
Finally, faster vector search enables interactions between dense vectors and graphs. Converting a pile of dense vectors into nearest neighbors graphs unlocks the entire world of graph analysis algorithms, such as those found in GraphBLAS and cuGraph.
Below are some common use-cases for vector search
-
- Generative AI & Retrieval augmented generation (RAG)
- Recommender systems
- Computer vision
- Image search
- Text search
- Audio search
- Molecular search
- Model training
-
- Clustering algorithms
- Visualization algorithms
- Sampling algorithms
- Class balancing
- Ensemble methods
- k-NN graph construction
There are several benefits to using cuVS and GPUs for vector search, including
- Fast index build
- Latency critical and high throughput search
- Parameter tuning
- Cost savings
- Interoperability (build on GPU, deploy on CPU)
- Multiple language support
- Building blocks for composing new or accelerating existing algorithms
In addition to the items above, cuVS takes on the burden of keeping non-trivial accelerated code up to date as new NVIDIA architectures and CUDA versions are released. This provides a delightful development experience, guaranteeing that any libraries, databases, or applications built on top of it will always be getting the best performance and scale.
cuVS is built on top of the RAPIDS RAFT library of high performance machine learning primitives and provides all the necessary routines for vector search and clustering on the GPU.
cuVS comes with pre-built packages that can be installed through conda and pip. Different packages are available for the different languages supported by cuVS:
Python | C/C++ |
---|---|
cuvs |
libcuvs |
It is recommended to use mamba to install the desired packages. The following command will install the Python package. You can substitute cuvs
for any of the packages in the table above:
conda install -c conda-forge -c nvidia -c rapidsai cuvs
The cuVS Python package can also be installed through pip <https://docs.rapids.ai/install#pip>
_.
For CUDA 11 packages:
pip install cuvs-cu11 --extra-index-url=https://pypi.nvidia.com
And CUDA 12 packages:
pip install cuvs-cu12 --extra-index-url=https://pypi.nvidia.com
If installing a version that has not yet been released, the rapidsai
channel can be replaced with rapidsai-nightly
:
conda install -c conda-forge -c nvidia -c rapidsai-nightly cuvs=25.04
cuVS also has pip
wheel packages that can be installed. Please see the Build and Install Guide for more information on installing the available cuVS packages and building from source.
The following code snippets train an approximate nearest neighbors index for the CAGRA algorithm in the various different languages supported by cuVS.
from cuvs.neighbors import cagra
dataset = load_data()
index_params = cagra.IndexParams()
index = cagra.build(build_params, dataset)
#include <cuvs/neighbors/cagra.hpp>
using namespace cuvs::neighbors;
raft::device_matrix_view<float> dataset = load_dataset();
raft::device_resources res;
cagra::index_params index_params;
auto index = cagra::build(res, index_params, dataset);
For more code examples of the C++ APIs, including drop-in Cmake project templates, please refer to the C++ examples directory in the codebase.
#include <cuvs/neighbors/cagra.h>
cuvsResources_t res;
cuvsCagraIndexParams_t index_params;
cuvsCagraIndex_t index;
DLManagedTensor *dataset;
load_dataset(dataset);
cuvsResourcesCreate(&res);
cuvsCagraIndexParamsCreate(&index_params);
cuvsCagraIndexCreate(&index);
cuvsCagraBuild(res, index_params, dataset, index);
cuvsCagraIndexDestroy(index);
cuvsCagraIndexParamsDestroy(index_params);
cuvsResourcesDestroy(res);
For more code examples of the C APIs, including drop-in Cmake project templates, please refer to the C examples
use cuvs::cagra::{Index, IndexParams, SearchParams};
use cuvs::{ManagedTensor, Resources, Result};
use ndarray::s;
use ndarray_rand::rand_distr::Uniform;
use ndarray_rand::RandomExt;
/// Example showing how to index and search data with CAGRA
fn cagra_example() -> Result<()> {
let res = Resources::new()?;
// Create a new random dataset to index
let n_datapoints = 65536;
let n_features = 512;
let dataset =
ndarray::Array::<f32, _>::random((n_datapoints, n_features), Uniform::new(0., 1.0));
// build the cagra index
let build_params = IndexParams::new()?;
let index = Index::build(&res, &build_params, &dataset)?;
println!(
"Indexed {}x{} datapoints into cagra index",
n_datapoints, n_features
);
// use the first 4 points from the dataset as queries : will test that we get them back
// as their own nearest neighbor
let n_queries = 4;
let queries = dataset.slice(s![0..n_queries, ..]);
let k = 10;
// CAGRA search API requires queries and outputs to be on device memory
// copy query data over, and allocate new device memory for the distances/ neighbors
// outputs
let queries = ManagedTensor::from(&queries).to_device(&res)?;
let mut neighbors_host = ndarray::Array::<u32, _>::zeros((n_queries, k));
let neighbors = ManagedTensor::from(&neighbors_host).to_device(&res)?;
let mut distances_host = ndarray::Array::<f32, _>::zeros((n_queries, k));
let distances = ManagedTensor::from(&distances_host).to_device(&res)?;
let search_params = SearchParams::new()?;
index.search(&res, &search_params, &queries, &neighbors, &distances)?;
// Copy back to host memory
distances.to_host(&res, &mut distances_host)?;
neighbors.to_host(&res, &mut neighbors_host)?;
// nearest neighbors should be themselves, since queries are from the
// dataset
println!("Neighbors {:?}", neighbors_host);
println!("Distances {:?}", distances_host);
Ok(())
}
For more code examples of the Rust APIs, including a drop-in project templates, please refer to the Rust examples.
If you are interested in contributing to the cuVS library, please read our Contributing guidelines. Refer to the Developer Guide for details on the developer guidelines, workflows, and principles.
For the interested reader, many of the accelerated implementations in cuVS are also based on research papers which can provide a lot more background. We also ask you to please cite the corresponding algorithms by referencing them in your own research.
- CAGRA: Highly Parallel Graph Construction and Approximate Nearest Neighbor Search
- Top-K Algorithms on GPU: A Comprehensive Study and New Methods
- Fast K-NN Graph Construction by GPU Based NN-Descent
- cuSLINK: Single-linkage Agglomerative Clustering on the GPU
- GPU Semiring Primitives for Sparse Neighborhood Methods
For Tasks:
Click tags to check more tools for each tasksFor Jobs:
Alternative AI tools for cuvs
Similar Open Source Tools
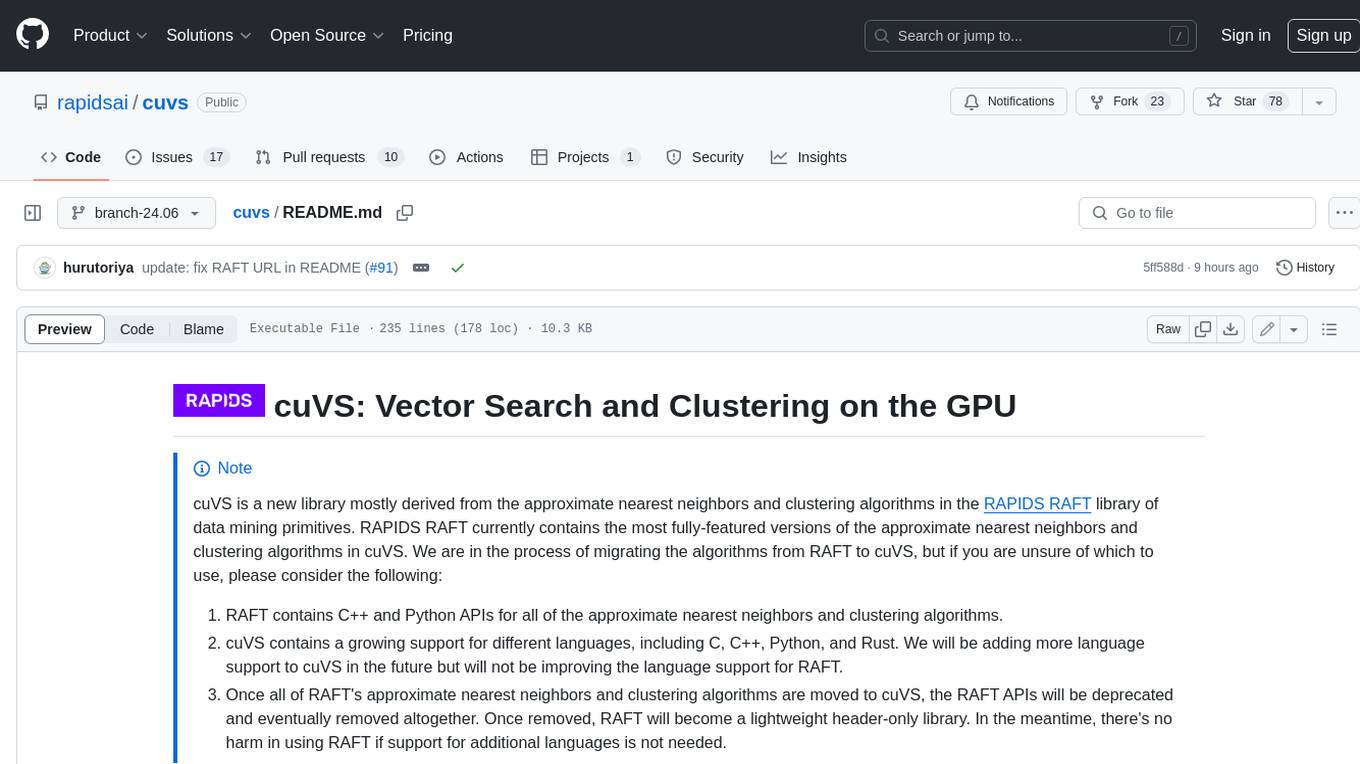
cuvs
cuVS is a library that contains state-of-the-art implementations of several algorithms for running approximate nearest neighbors and clustering on the GPU. It can be used directly or through the various databases and other libraries that have integrated it. The primary goal of cuVS is to simplify the use of GPUs for vector similarity search and clustering.
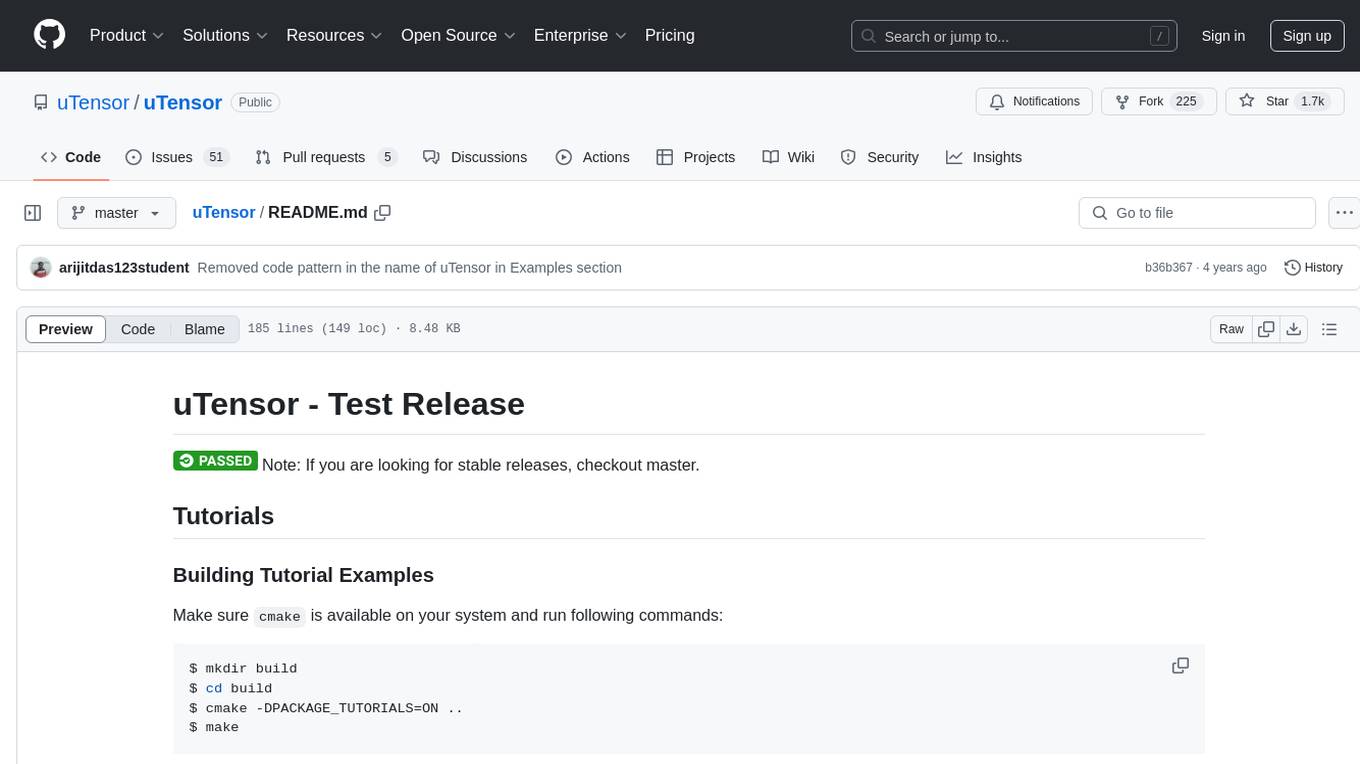
uTensor
uTensor is an extremely light-weight machine learning inference framework built on Tensorflow and optimized for Arm targets. It consists of a runtime library and an offline tool that handles most of the model translation work. The core runtime is only ~2KB. The workflow involves constructing and training a model in Tensorflow, then using uTensor to produce C++ code for inferencing. The runtime ensures system safety, guarantees RAM usage, and focuses on clear, concise, and debuggable code. The high-level API simplifies tensor handling and operator execution for embedded systems.
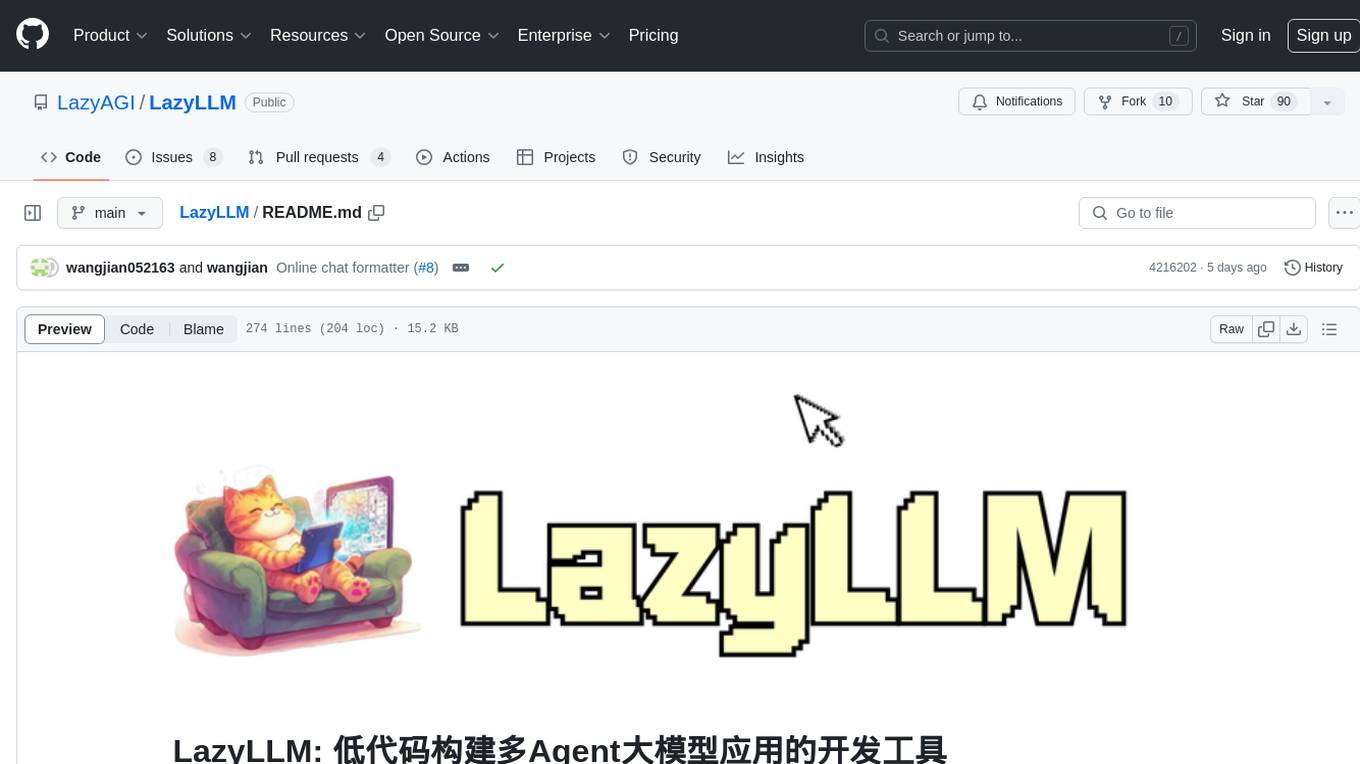
LazyLLM
LazyLLM is a low-code development tool for building complex AI applications with multiple agents. It assists developers in building AI applications at a low cost and continuously optimizing their performance. The tool provides a convenient workflow for application development and offers standard processes and tools for various stages of application development. Users can quickly prototype applications with LazyLLM, analyze bad cases with scenario task data, and iteratively optimize key components to enhance the overall application performance. LazyLLM aims to simplify the AI application development process and provide flexibility for both beginners and experts to create high-quality applications.
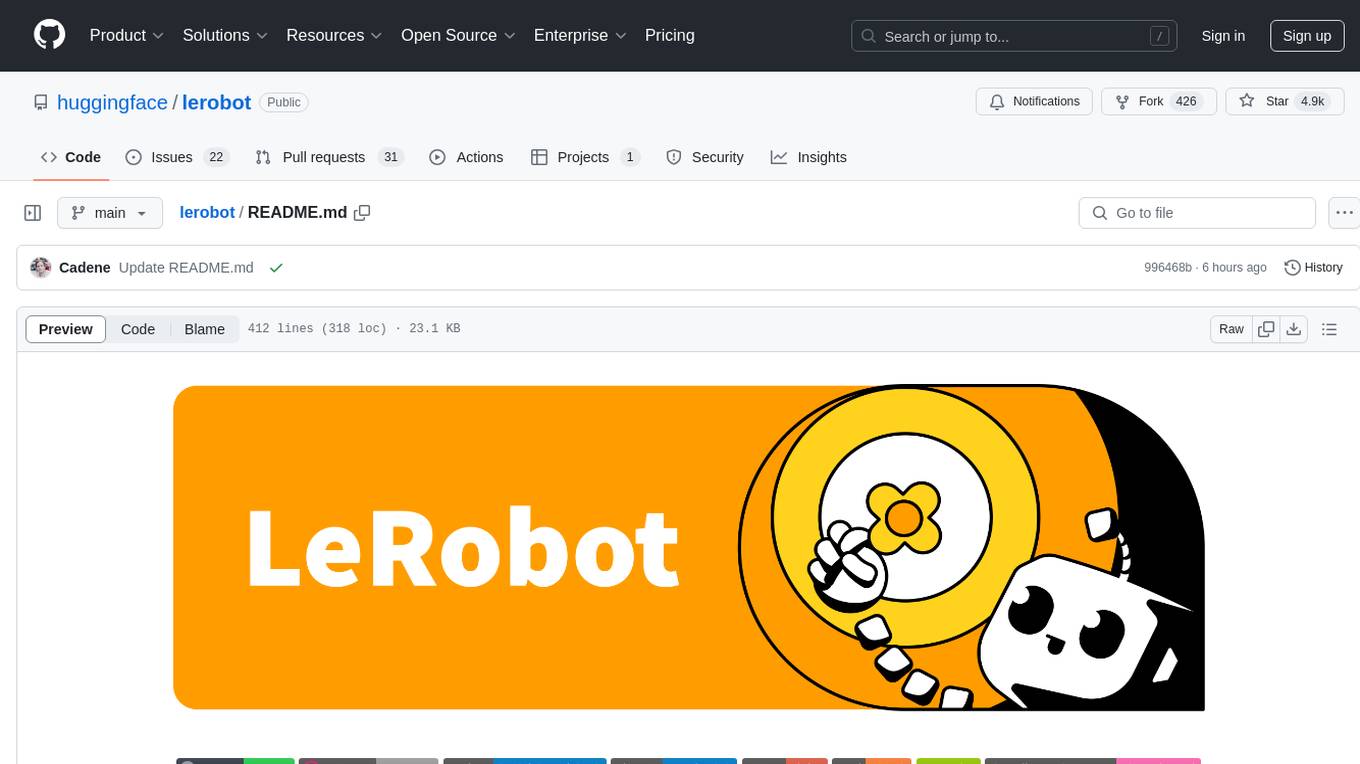
lerobot
LeRobot is a state-of-the-art AI library for real-world robotics in PyTorch. It aims to provide models, datasets, and tools to lower the barrier to entry to robotics, focusing on imitation learning and reinforcement learning. LeRobot offers pretrained models, datasets with human-collected demonstrations, and simulation environments. It plans to support real-world robotics on affordable and capable robots. The library hosts pretrained models and datasets on the Hugging Face community page.
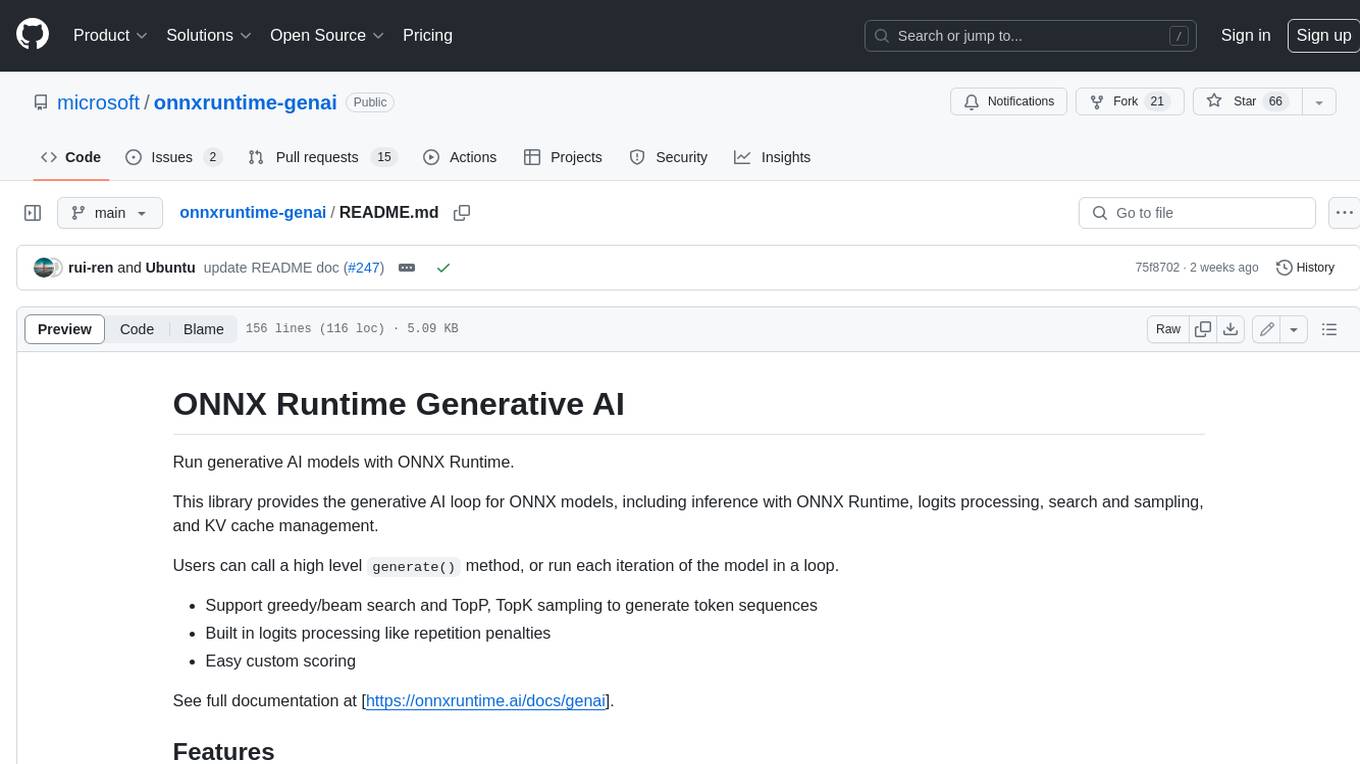
onnxruntime-genai
ONNX Runtime Generative AI is a library that provides the generative AI loop for ONNX models, including inference with ONNX Runtime, logits processing, search and sampling, and KV cache management. Users can call a high level `generate()` method, or run each iteration of the model in a loop. It supports greedy/beam search and TopP, TopK sampling to generate token sequences, has built in logits processing like repetition penalties, and allows for easy custom scoring.
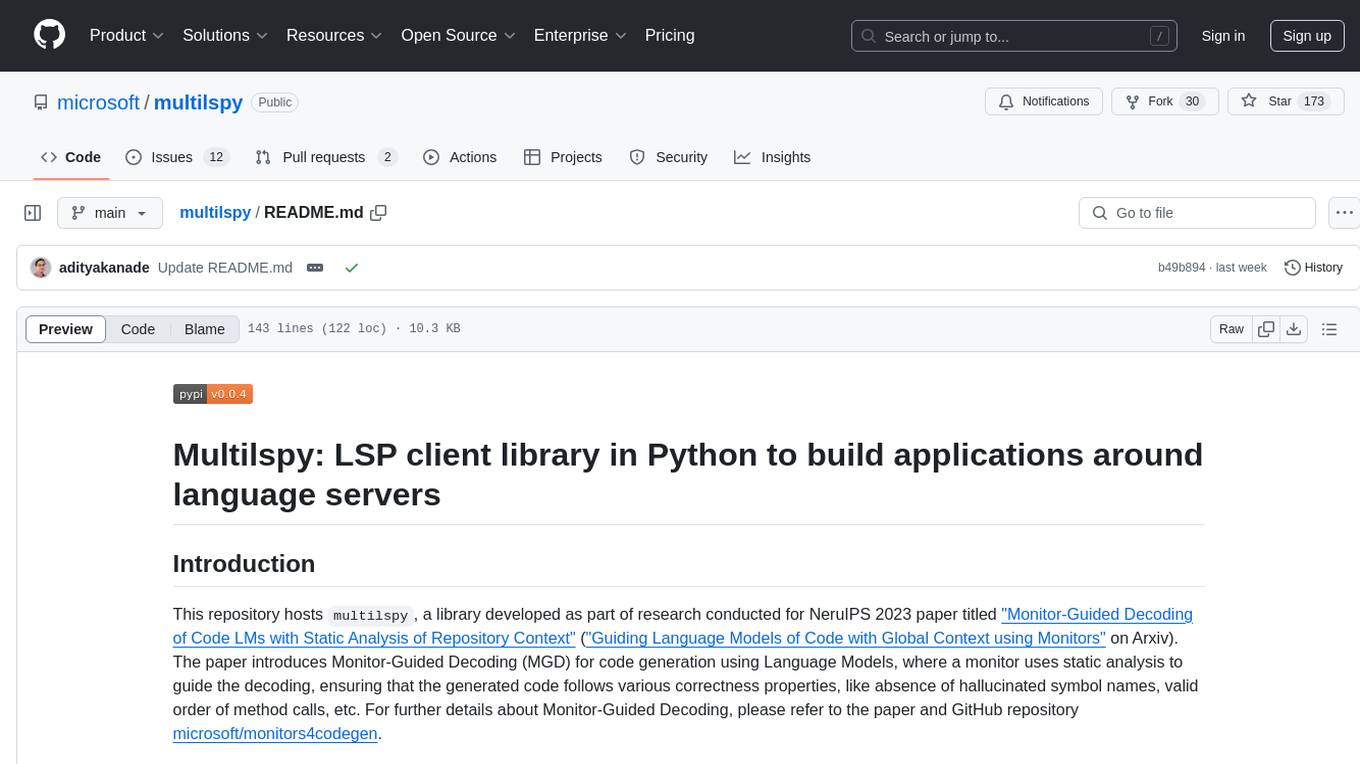
multilspy
Multilspy is a Python library developed for research purposes to facilitate the creation of language server clients for querying and obtaining results of static analyses from various language servers. It simplifies the process by handling server setup, communication, and configuration parameters, providing a common interface for different languages. The library supports features like finding function/class definitions, callers, completions, hover information, and document symbols. It is designed to work with AI systems like Large Language Models (LLMs) for tasks such as Monitor-Guided Decoding to ensure code generation correctness and boost compilability.
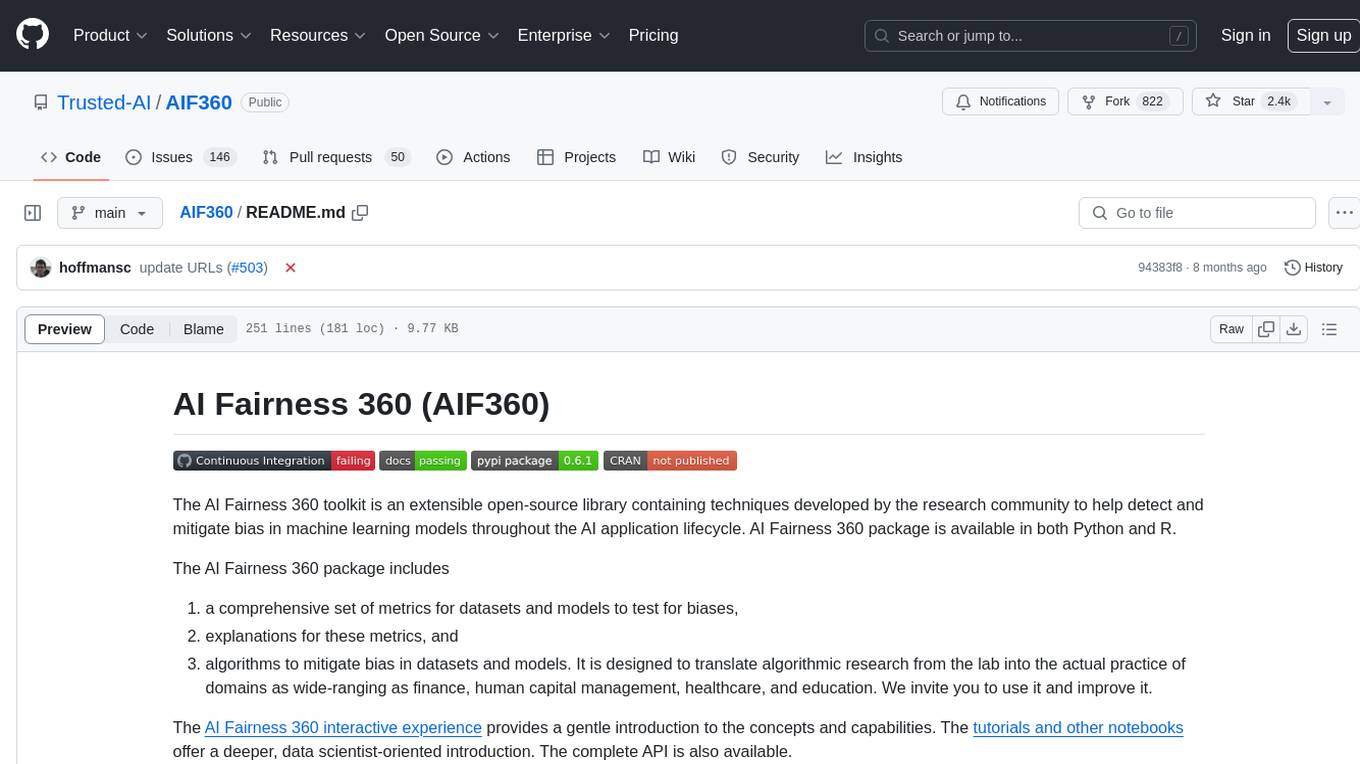
AIF360
The AI Fairness 360 toolkit is an open-source library designed to detect and mitigate bias in machine learning models. It provides a comprehensive set of metrics, explanations, and algorithms for bias mitigation in various domains such as finance, healthcare, and education. The toolkit supports multiple bias mitigation algorithms and fairness metrics, and is available in both Python and R. Users can leverage the toolkit to ensure fairness in AI applications and contribute to its development for extensibility.
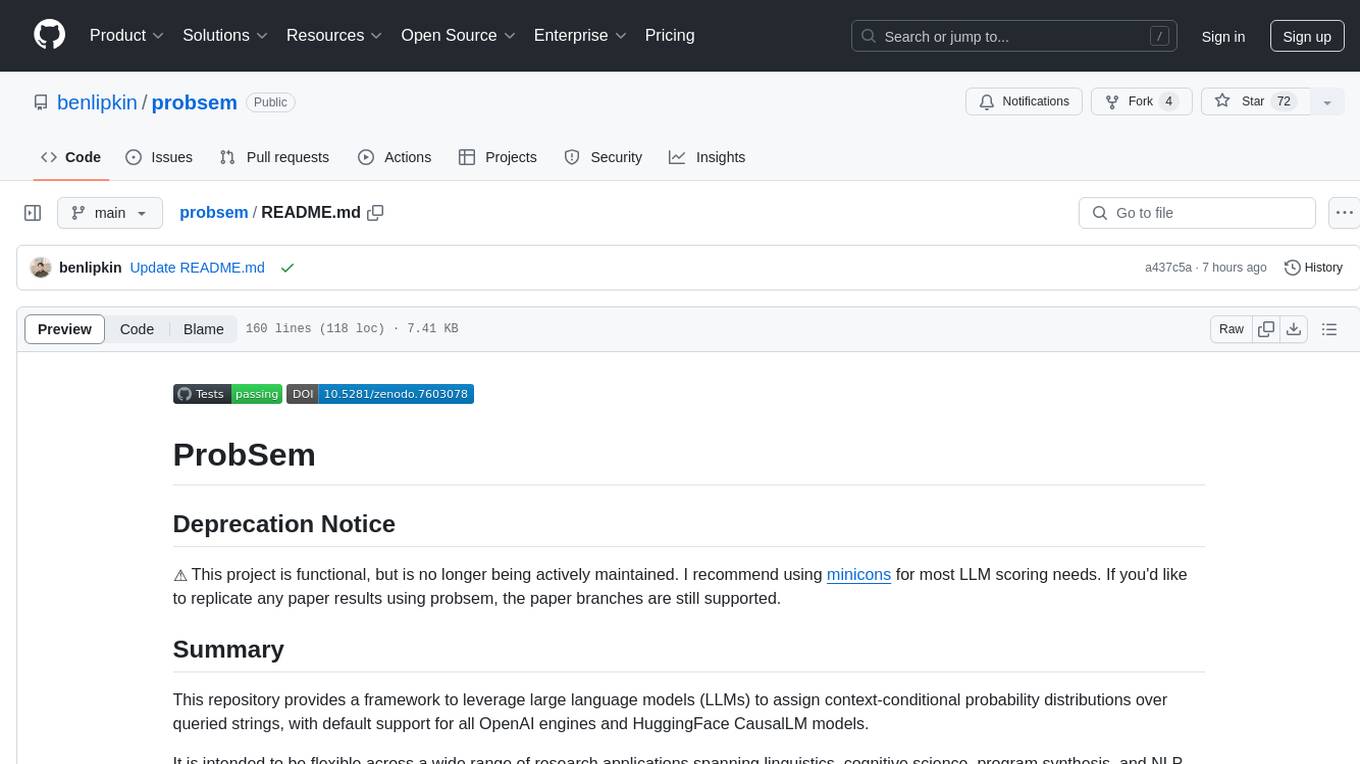
probsem
ProbSem is a repository that provides a framework to leverage large language models (LLMs) for assigning context-conditional probability distributions over queried strings. It supports OpenAI engines and HuggingFace CausalLM models, and is flexible for research applications in linguistics, cognitive science, program synthesis, and NLP. Users can define prompts, contexts, and queries to derive probability distributions over possible completions, enabling tasks like cloze completion, multiple-choice QA, semantic parsing, and code completion. The repository offers CLI and API interfaces for evaluation, with options to customize models, normalize scores, and adjust temperature for probability distributions.
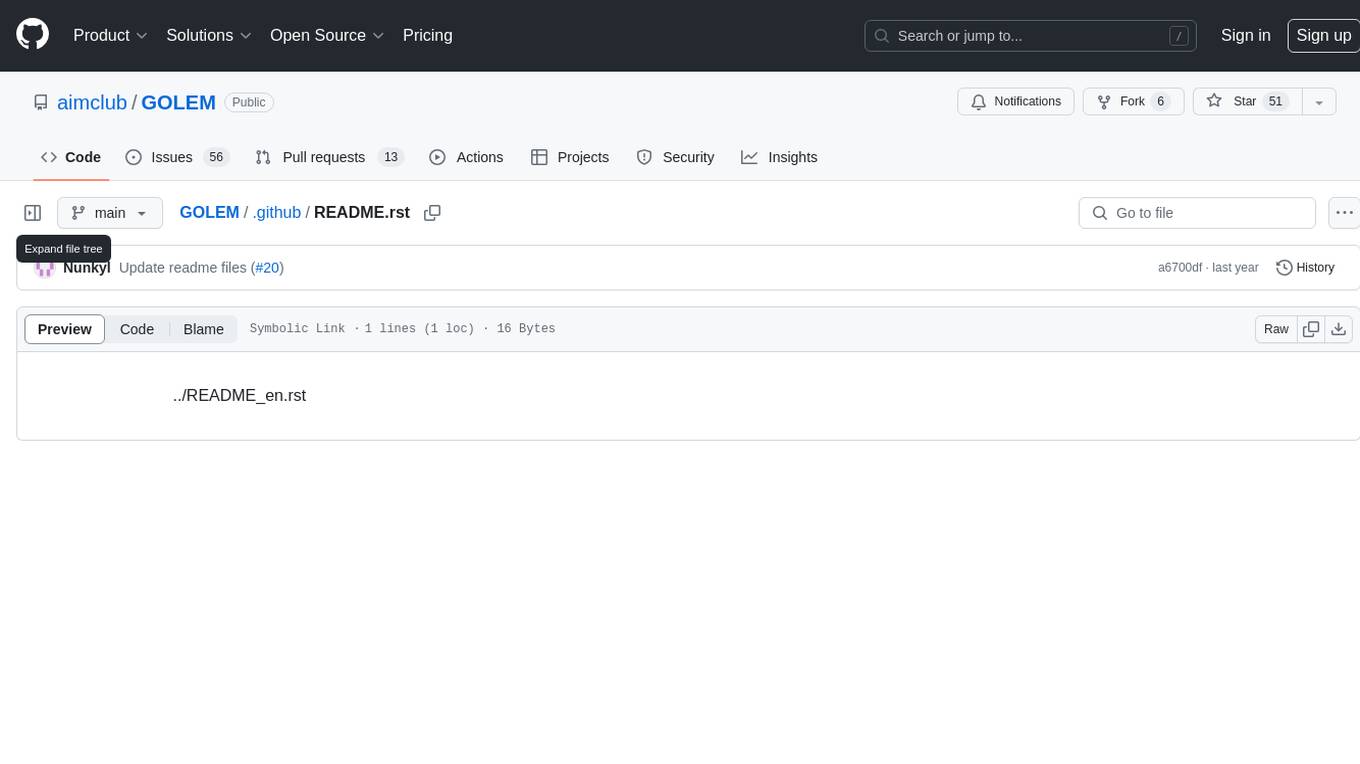
GOLEM
GOLEM is an open-source AI framework focused on optimization and learning of structured graph-based models using meta-heuristic methods. It emphasizes the potential of meta-heuristics in complex problem spaces where gradient-based methods are not suitable, and the importance of structured models in various problem domains. The framework offers features like structured model optimization, metaheuristic methods, multi-objective optimization, constrained optimization, extensibility, interpretability, and reproducibility. It can be applied to optimization problems represented as directed graphs with defined fitness functions. GOLEM has applications in areas like AutoML, Bayesian network structure search, differential equation discovery, geometric design, and neural architecture search. The project structure includes packages for core functionalities, adapters, graph representation, optimizers, genetic algorithms, utilities, serialization, visualization, examples, and testing. Contributions are welcome, and the project is supported by ITMO University's Research Center Strong Artificial Intelligence in Industry.
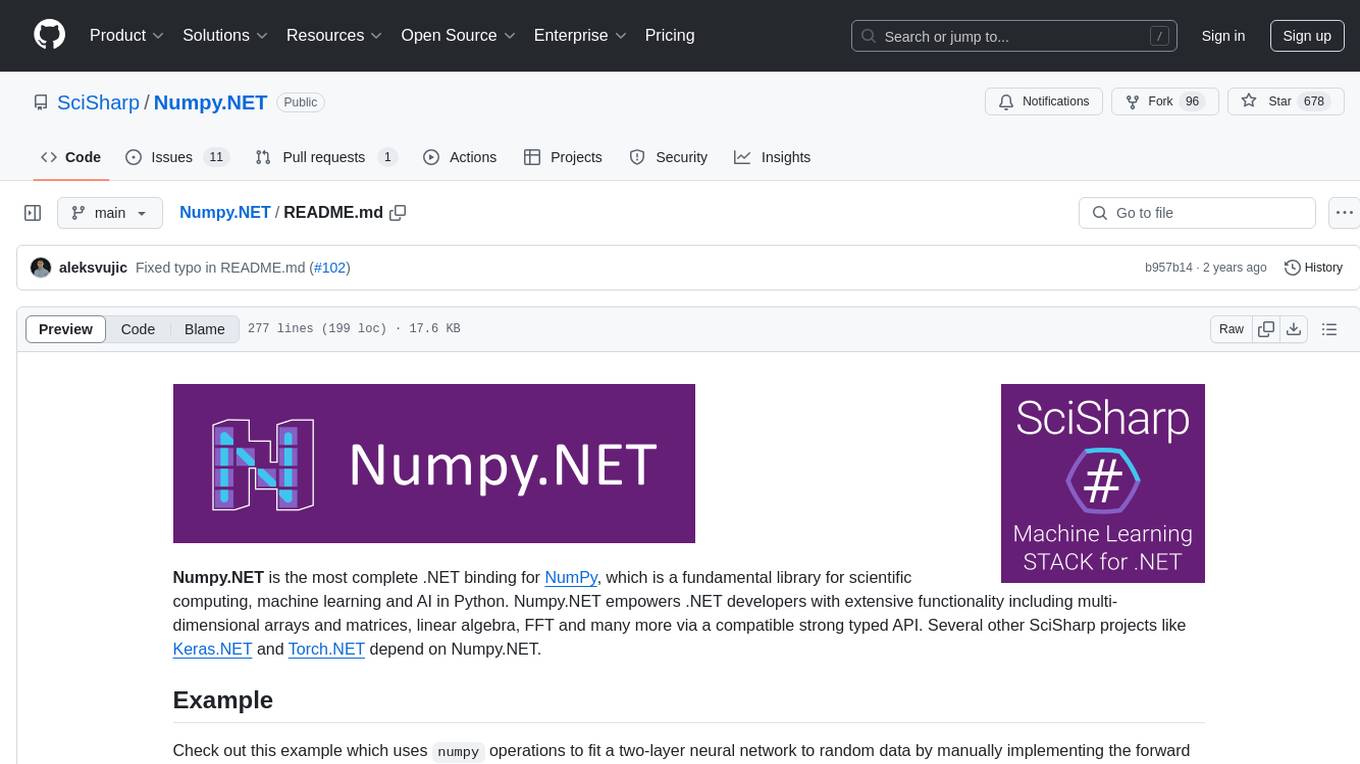
Numpy.NET
Numpy.NET is the most complete .NET binding for NumPy, empowering .NET developers with extensive functionality for scientific computing, machine learning, and AI. It provides multi-dimensional arrays, matrices, linear algebra, FFT, and more via a strong typed API. Numpy.NET does not require a local Python installation, as it uses Python.Included to package embedded Python 3.7. Multi-threading must be handled carefully to avoid deadlocks or access violation exceptions. Performance considerations include overhead when calling NumPy from C# and the efficiency of data transfer between C# and Python. Numpy.NET aims to match the completeness of the original NumPy library and is generated using CodeMinion by parsing the NumPy documentation. The project is MIT licensed and supported by JetBrains.
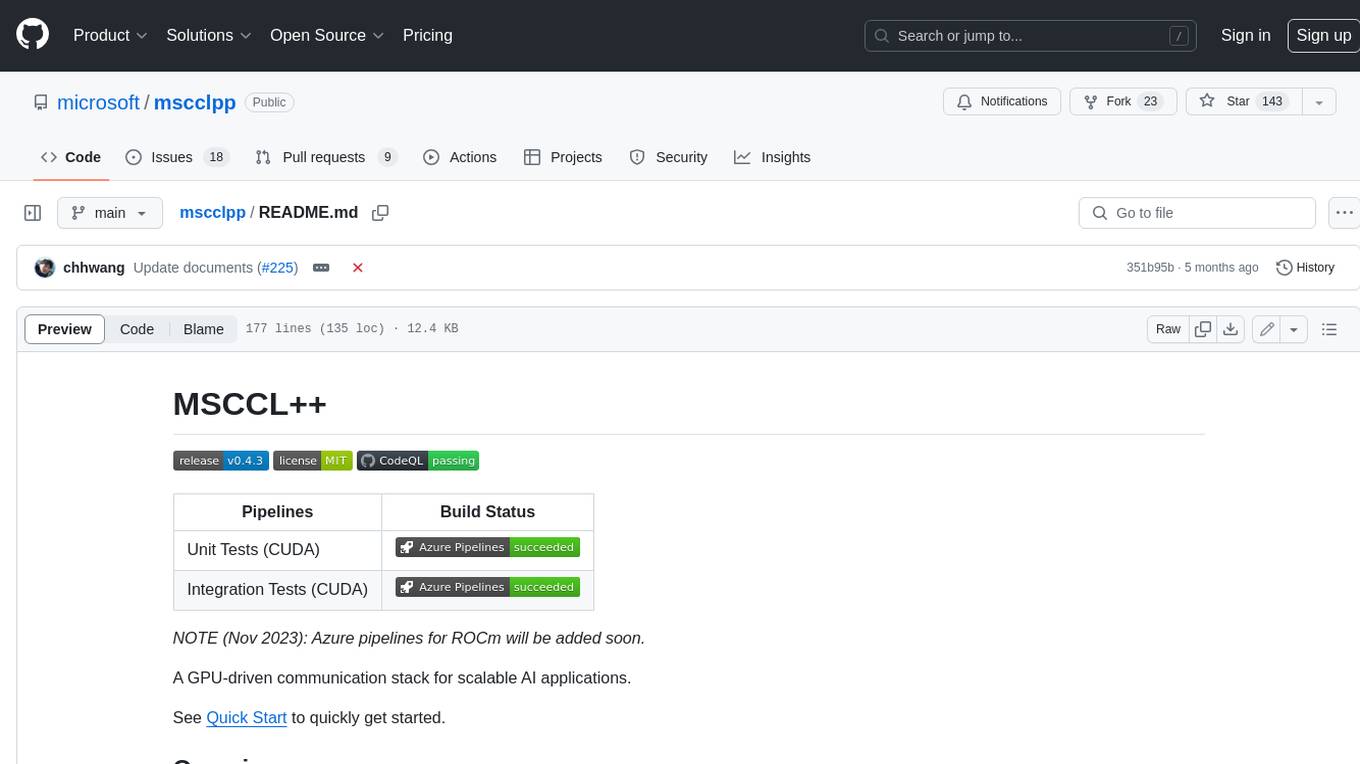
mscclpp
MSCCL++ is a GPU-driven communication stack for scalable AI applications. It provides a highly efficient and customizable communication stack for distributed GPU applications. MSCCL++ redefines inter-GPU communication interfaces, delivering a highly efficient and customizable communication stack for distributed GPU applications. Its design is specifically tailored to accommodate diverse performance optimization scenarios often encountered in state-of-the-art AI applications. MSCCL++ provides communication abstractions at the lowest level close to hardware and at the highest level close to application API. The lowest level of abstraction is ultra light weight which enables a user to implement logics of data movement for a collective operation such as AllReduce inside a GPU kernel extremely efficiently without worrying about memory ordering of different ops. The modularity of MSCCL++ enables a user to construct the building blocks of MSCCL++ in a high level abstraction in Python and feed them to a CUDA kernel in order to facilitate the user's productivity. MSCCL++ provides fine-grained synchronous and asynchronous 0-copy 1-sided abstracts for communication primitives such as `put()`, `get()`, `signal()`, `flush()`, and `wait()`. The 1-sided abstractions allows a user to asynchronously `put()` their data on the remote GPU as soon as it is ready without requiring the remote side to issue any receive instruction. This enables users to easily implement flexible communication logics, such as overlapping communication with computation, or implementing customized collective communication algorithms without worrying about potential deadlocks. Additionally, the 0-copy capability enables MSCCL++ to directly transfer data between user's buffers without using intermediate internal buffers which saves GPU bandwidth and memory capacity. MSCCL++ provides consistent abstractions regardless of the location of the remote GPU (either on the local node or on a remote node) or the underlying link (either NVLink/xGMI or InfiniBand). This simplifies the code for inter-GPU communication, which is often complex due to memory ordering of GPU/CPU read/writes and therefore, is error-prone.
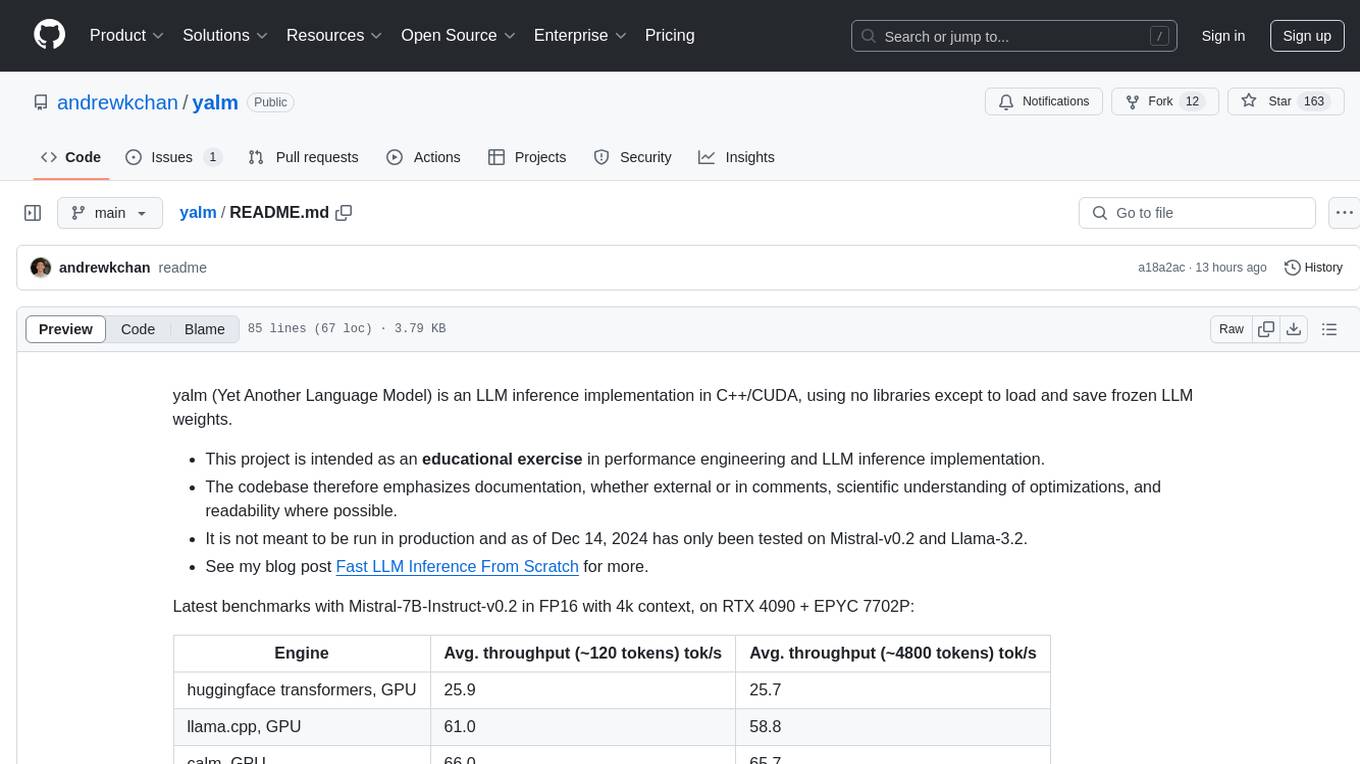
yalm
Yalm (Yet Another Language Model) is an LLM inference implementation in C++/CUDA, emphasizing performance engineering, documentation, scientific optimizations, and readability. It is not for production use and has been tested on Mistral-v0.2 and Llama-3.2. Requires C++20-compatible compiler, CUDA toolkit, and LLM safetensor weights in huggingface format converted to .yalm file.
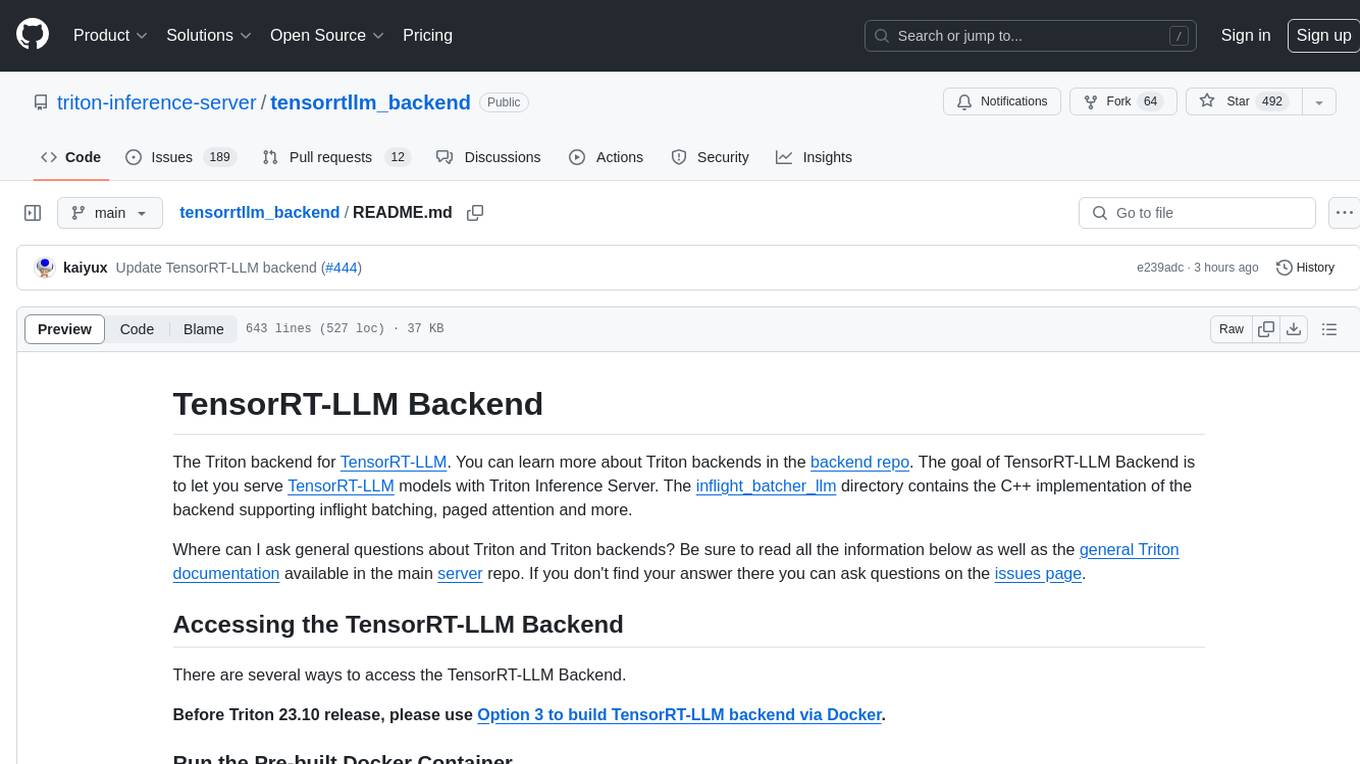
tensorrtllm_backend
The TensorRT-LLM Backend is a Triton backend designed to serve TensorRT-LLM models with Triton Inference Server. It supports features like inflight batching, paged attention, and more. Users can access the backend through pre-built Docker containers or build it using scripts provided in the repository. The backend can be used to create models for tasks like tokenizing, inferencing, de-tokenizing, ensemble modeling, and more. Users can interact with the backend using provided client scripts and query the server for metrics related to request handling, memory usage, KV cache blocks, and more. Testing for the backend can be done following the instructions in the 'ci/README.md' file.
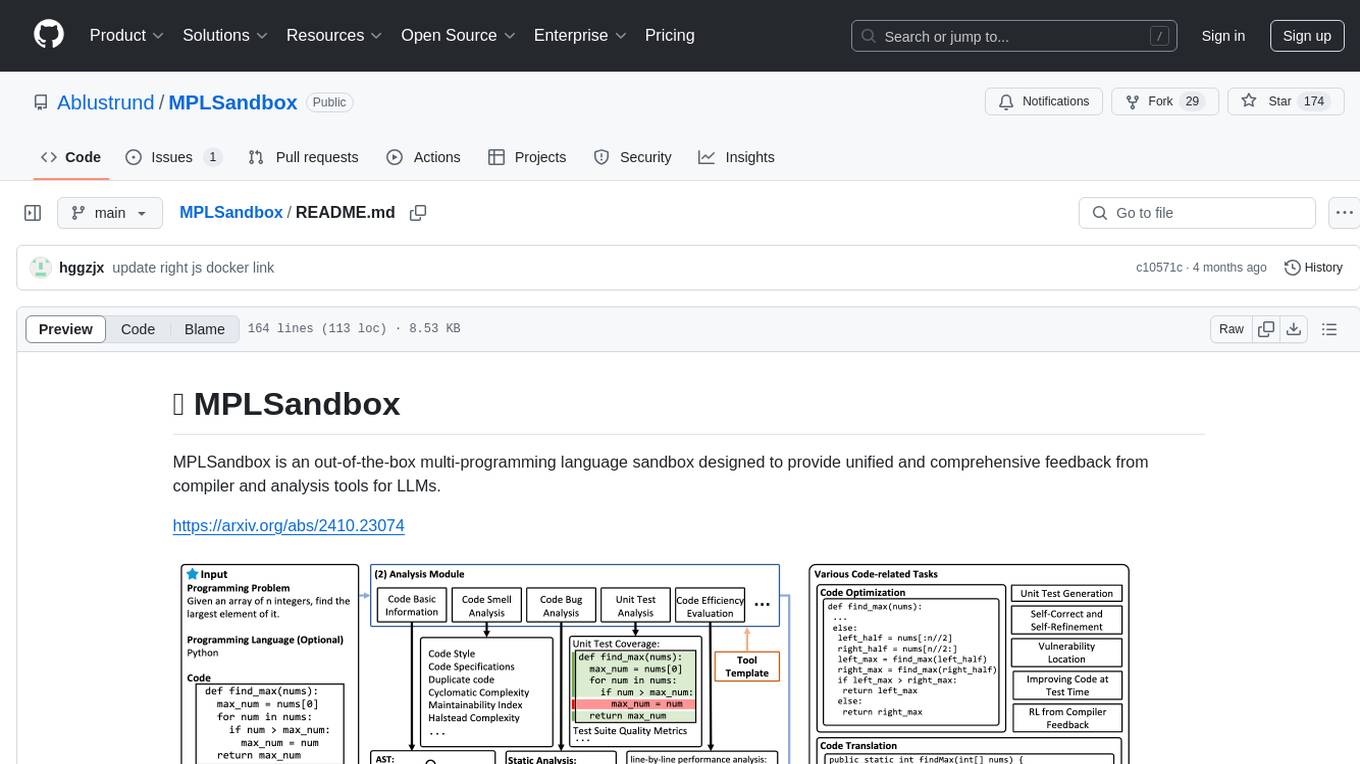
MPLSandbox
MPLSandbox is an out-of-the-box multi-programming language sandbox designed to provide unified and comprehensive feedback from compiler and analysis tools for LLMs. It simplifies code analysis for researchers and can be seamlessly integrated into LLM training and application processes to enhance performance in a range of code-related tasks. The sandbox environment ensures safe code execution, the code analysis module offers comprehensive analysis reports, and the information integration module combines compilation feedback and analysis results for complex code-related tasks.
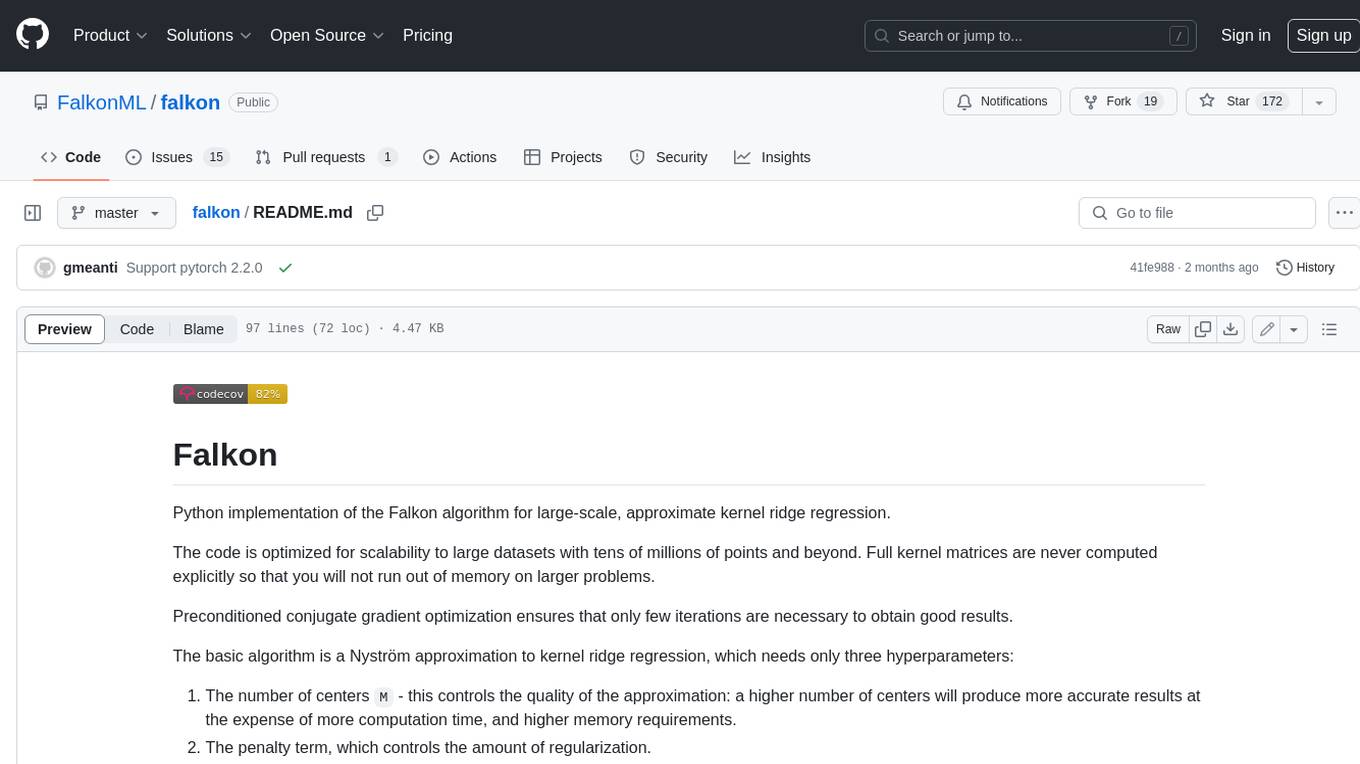
falkon
Falkon is a Python implementation of the Falkon algorithm for large-scale, approximate kernel ridge regression. The code is optimized for scalability to large datasets with tens of millions of points and beyond. Full kernel matrices are never computed explicitly so that you will not run out of memory on larger problems. Preconditioned conjugate gradient optimization ensures that only few iterations are necessary to obtain good results. The basic algorithm is a Nyström approximation to kernel ridge regression, which needs only three hyperparameters: 1. The number of centers M - this controls the quality of the approximation: a higher number of centers will produce more accurate results at the expense of more computation time, and higher memory requirements. 2. The penalty term, which controls the amount of regularization. 3. The kernel function. A good default is always the Gaussian (RBF) kernel (`falkon.kernels.GaussianKernel`).
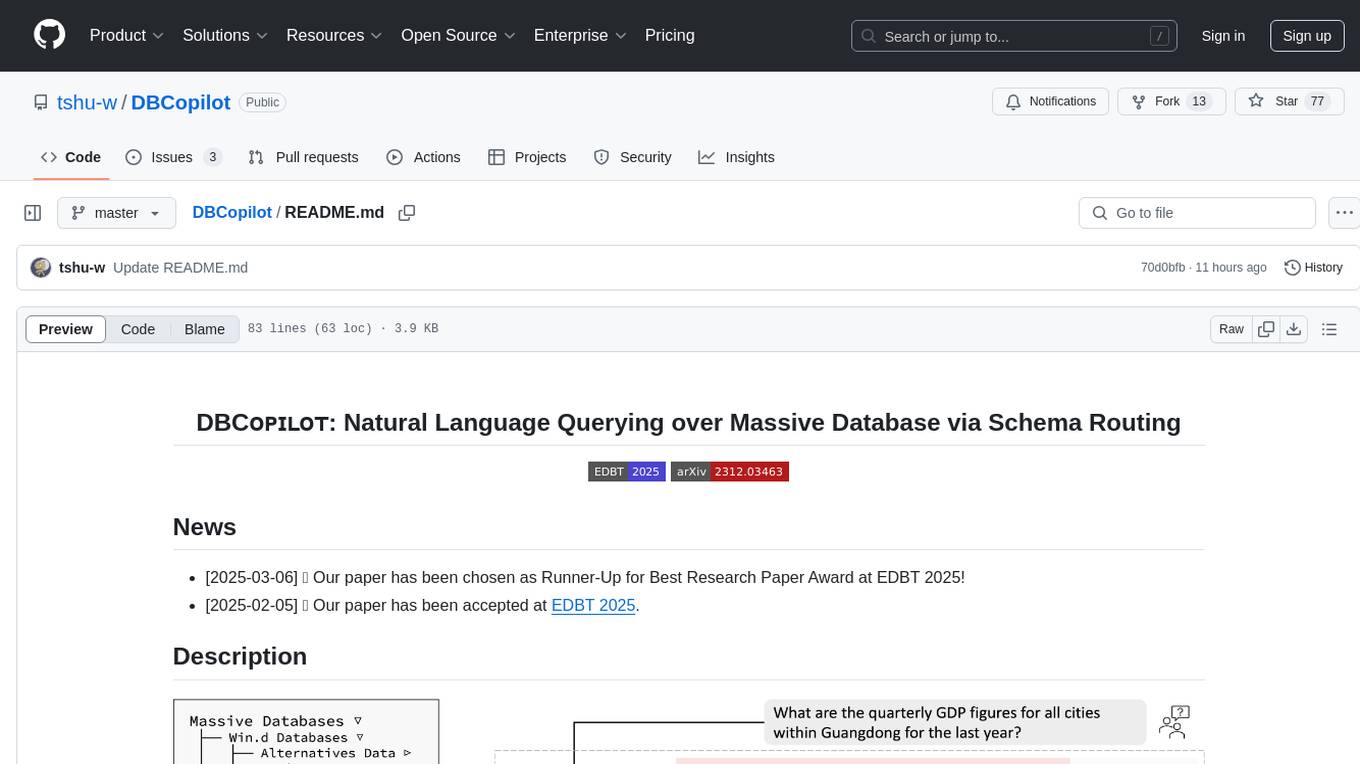
DBCopilot
The development of Natural Language Interfaces to Databases (NLIDBs) has been greatly advanced by the advent of large language models (LLMs), which provide an intuitive way to translate natural language (NL) questions into Structured Query Language (SQL) queries. DBCopilot is a framework that addresses challenges in real-world scenarios of natural language querying over massive databases by employing a compact and flexible copilot model for routing. It decouples schema-agnostic NL2SQL into schema routing and SQL generation, utilizing a lightweight differentiable search index for semantic mappings and relation-aware joint retrieval. DBCopilot introduces a reverse schema-to-question generation paradigm for automatic learning and adaptation over massive databases, providing a scalable and effective solution for schema-agnostic NL2SQL.
For similar tasks
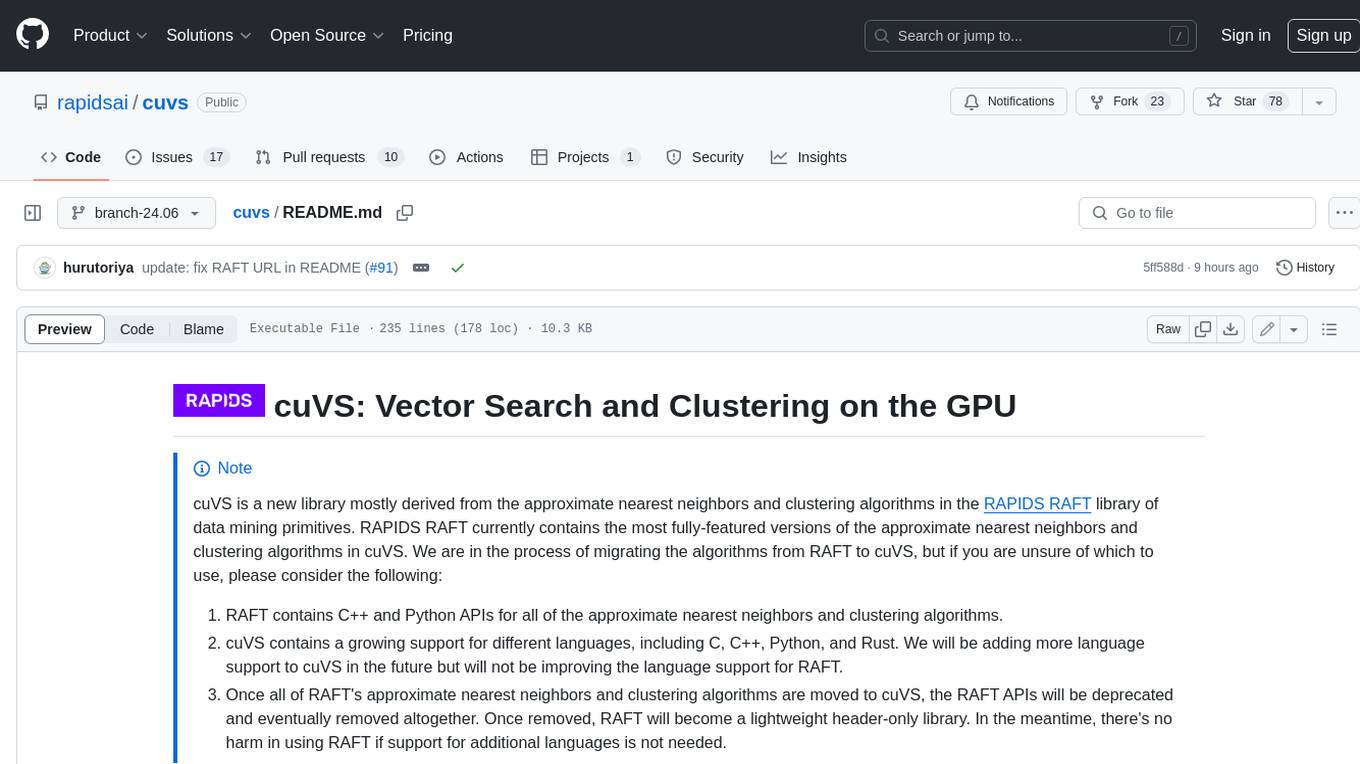
cuvs
cuVS is a library that contains state-of-the-art implementations of several algorithms for running approximate nearest neighbors and clustering on the GPU. It can be used directly or through the various databases and other libraries that have integrated it. The primary goal of cuVS is to simplify the use of GPUs for vector similarity search and clustering.
For similar jobs
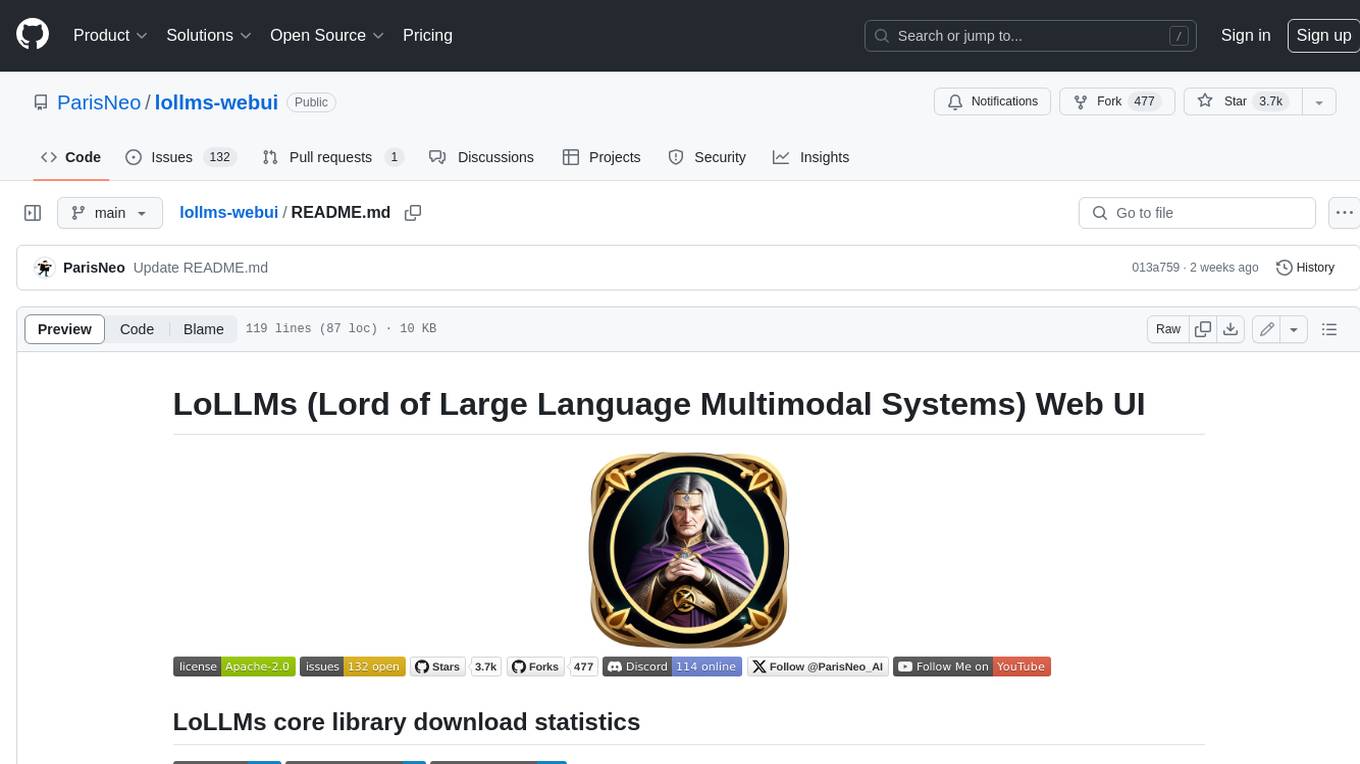
lollms-webui
LoLLMs WebUI (Lord of Large Language Multimodal Systems: One tool to rule them all) is a user-friendly interface to access and utilize various LLM (Large Language Models) and other AI models for a wide range of tasks. With over 500 AI expert conditionings across diverse domains and more than 2500 fine tuned models over multiple domains, LoLLMs WebUI provides an immediate resource for any problem, from car repair to coding assistance, legal matters, medical diagnosis, entertainment, and more. The easy-to-use UI with light and dark mode options, integration with GitHub repository, support for different personalities, and features like thumb up/down rating, copy, edit, and remove messages, local database storage, search, export, and delete multiple discussions, make LoLLMs WebUI a powerful and versatile tool.
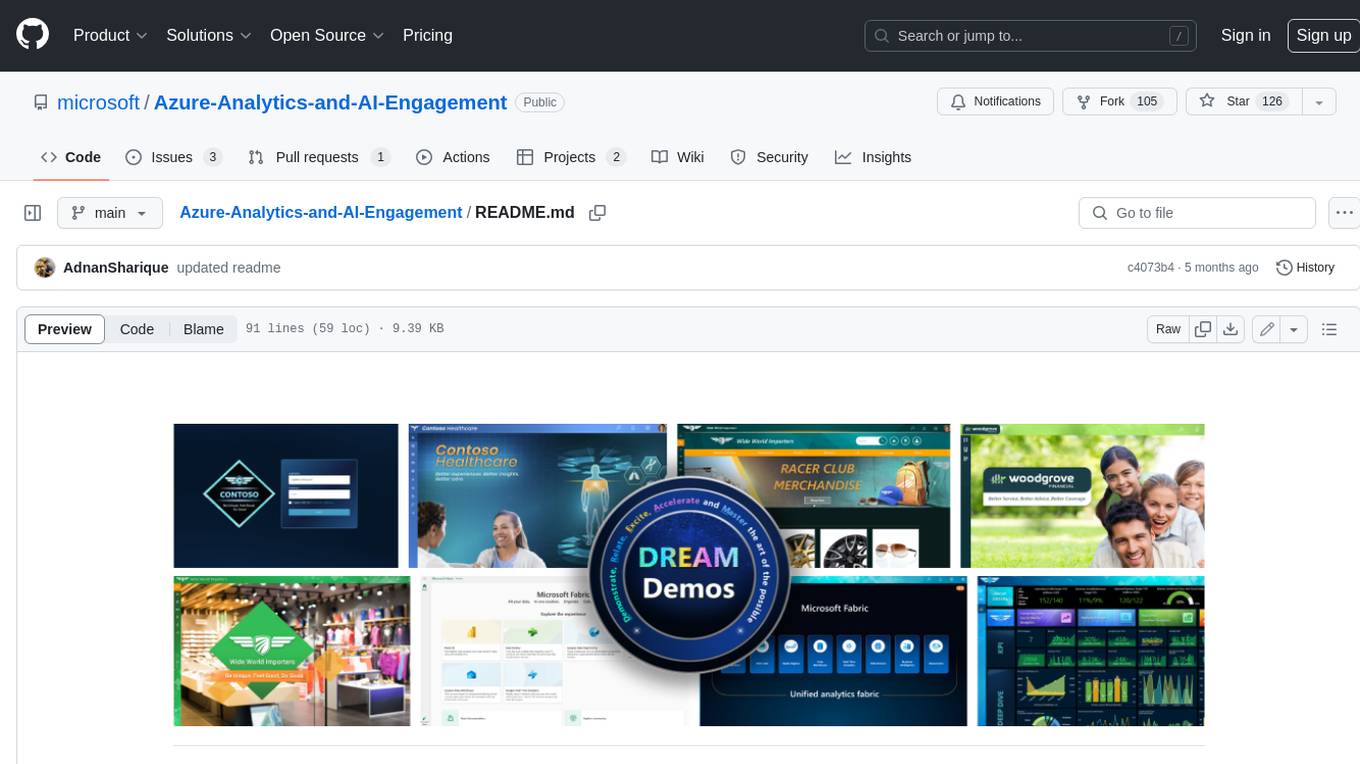
Azure-Analytics-and-AI-Engagement
The Azure-Analytics-and-AI-Engagement repository provides packaged Industry Scenario DREAM Demos with ARM templates (Containing a demo web application, Power BI reports, Synapse resources, AML Notebooks etc.) that can be deployed in a customer’s subscription using the CAPE tool within a matter of few hours. Partners can also deploy DREAM Demos in their own subscriptions using DPoC.
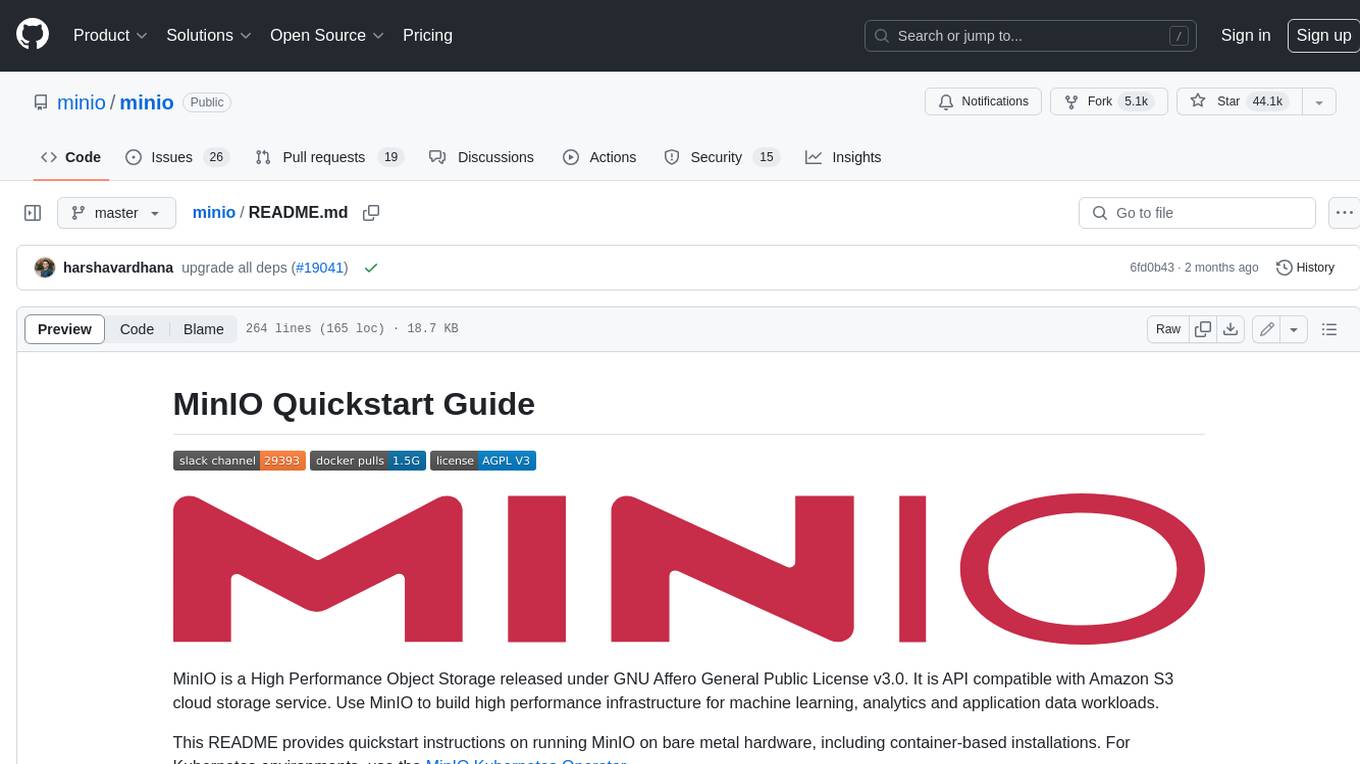
minio
MinIO is a High Performance Object Storage released under GNU Affero General Public License v3.0. It is API compatible with Amazon S3 cloud storage service. Use MinIO to build high performance infrastructure for machine learning, analytics and application data workloads.
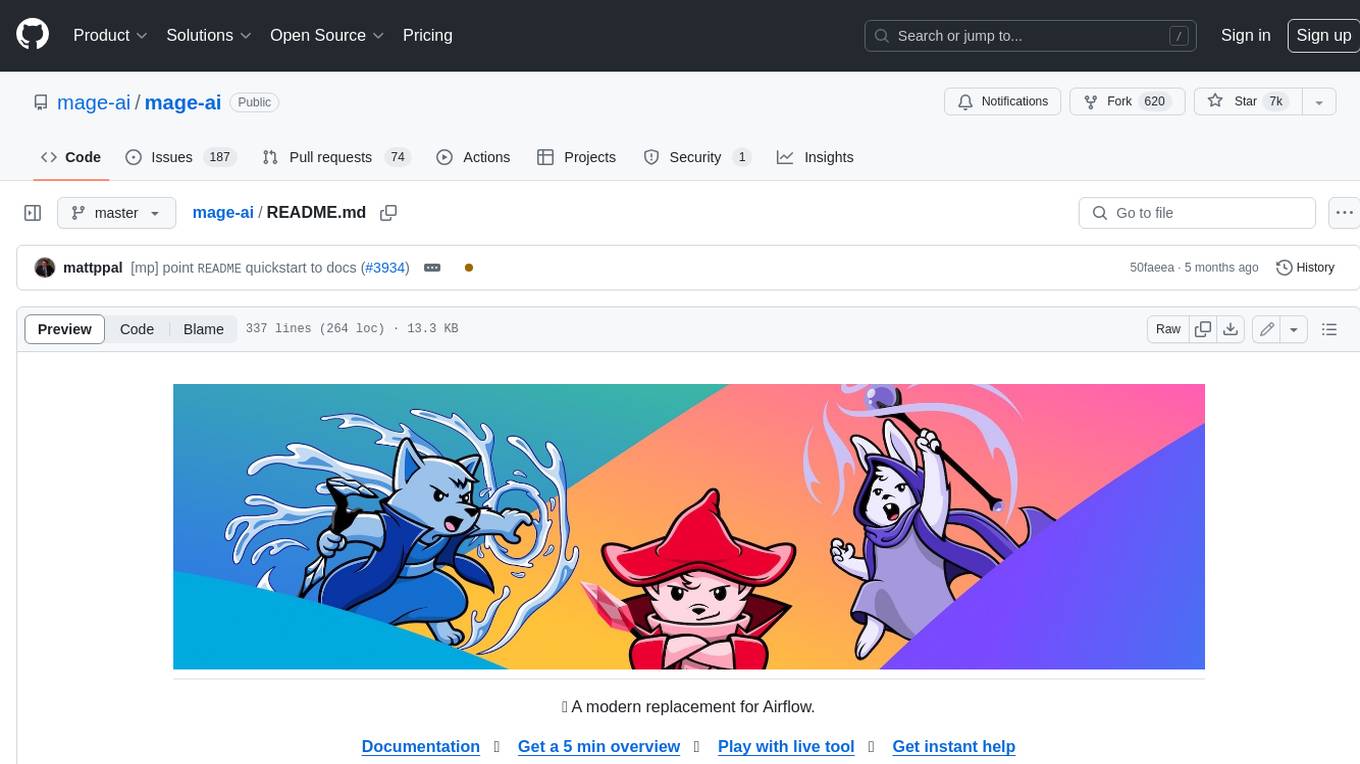
mage-ai
Mage is an open-source data pipeline tool for transforming and integrating data. It offers an easy developer experience, engineering best practices built-in, and data as a first-class citizen. Mage makes it easy to build, preview, and launch data pipelines, and provides observability and scaling capabilities. It supports data integrations, streaming pipelines, and dbt integration.
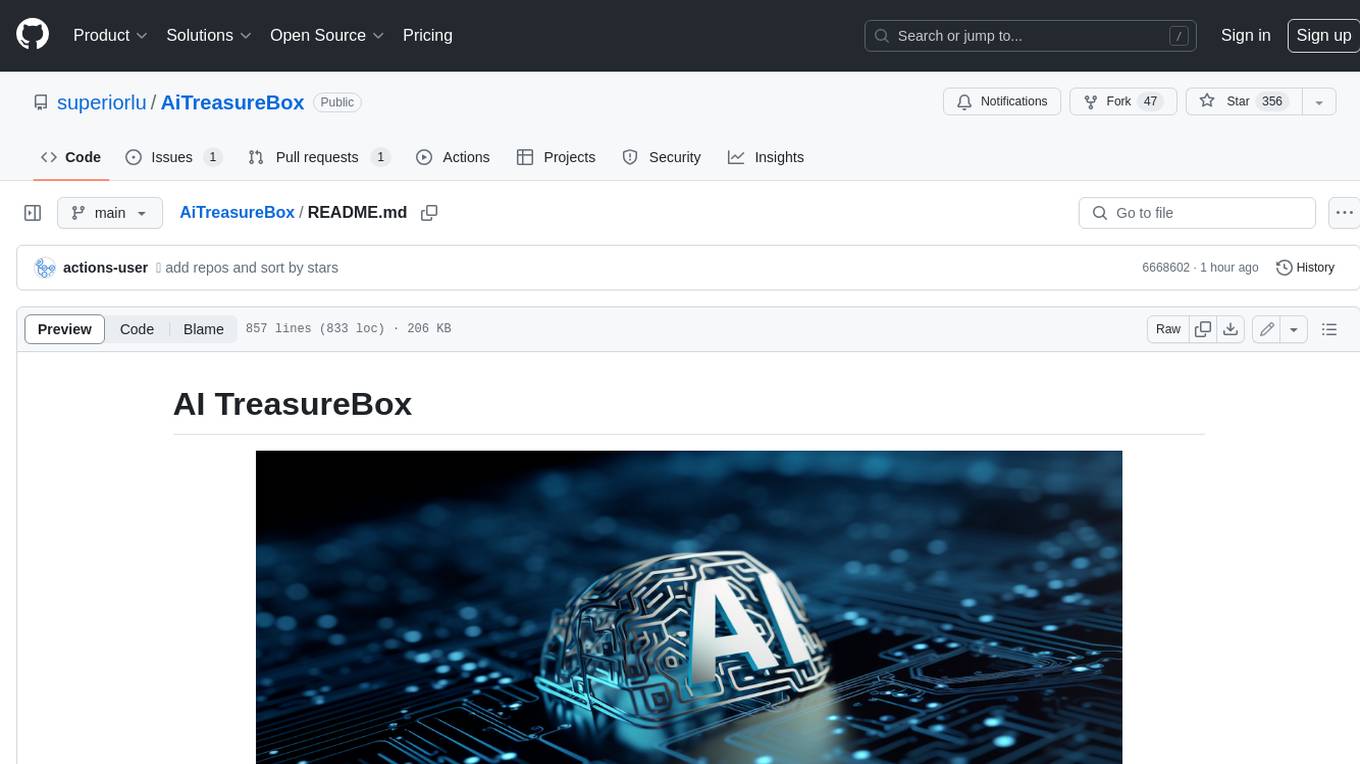
AiTreasureBox
AiTreasureBox is a versatile AI tool that provides a collection of pre-trained models and algorithms for various machine learning tasks. It simplifies the process of implementing AI solutions by offering ready-to-use components that can be easily integrated into projects. With AiTreasureBox, users can quickly prototype and deploy AI applications without the need for extensive knowledge in machine learning or deep learning. The tool covers a wide range of tasks such as image classification, text generation, sentiment analysis, object detection, and more. It is designed to be user-friendly and accessible to both beginners and experienced developers, making AI development more efficient and accessible to a wider audience.
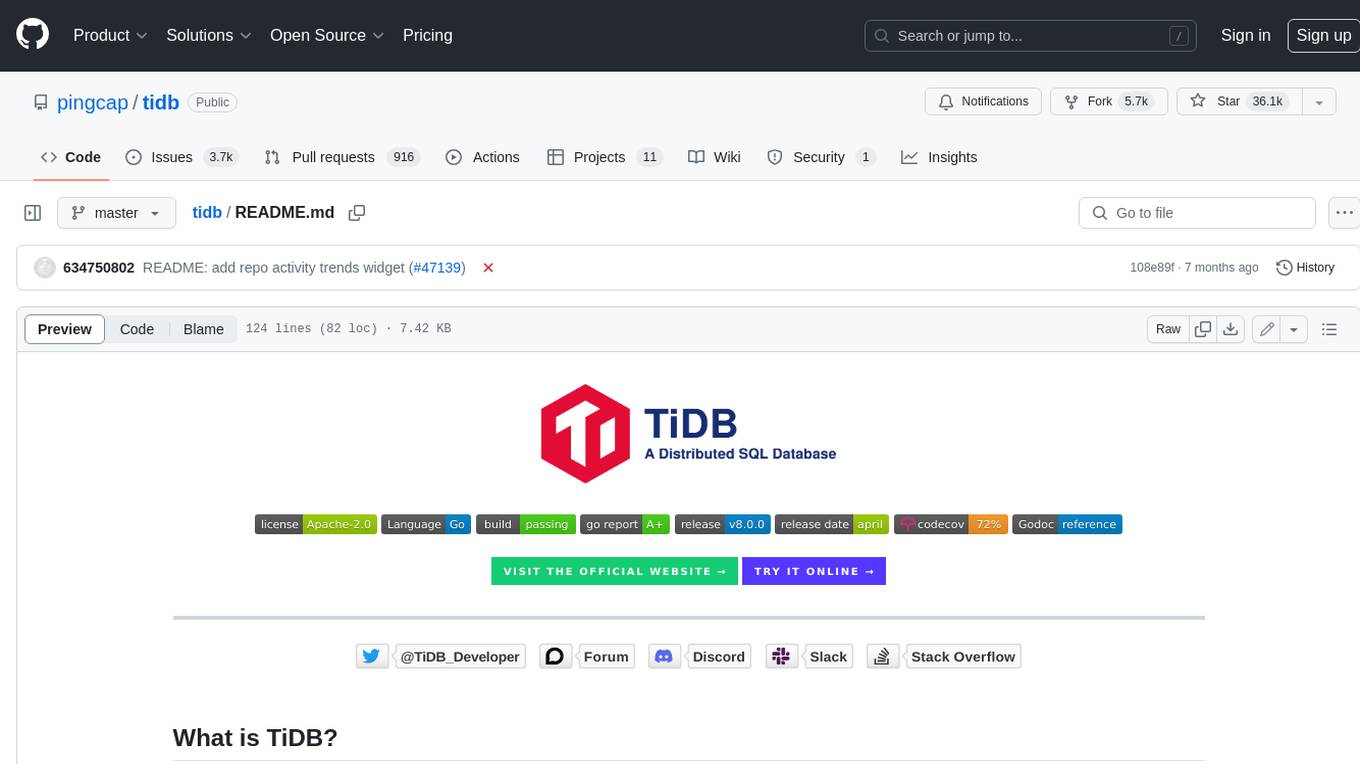
tidb
TiDB is an open-source distributed SQL database that supports Hybrid Transactional and Analytical Processing (HTAP) workloads. It is MySQL compatible and features horizontal scalability, strong consistency, and high availability.
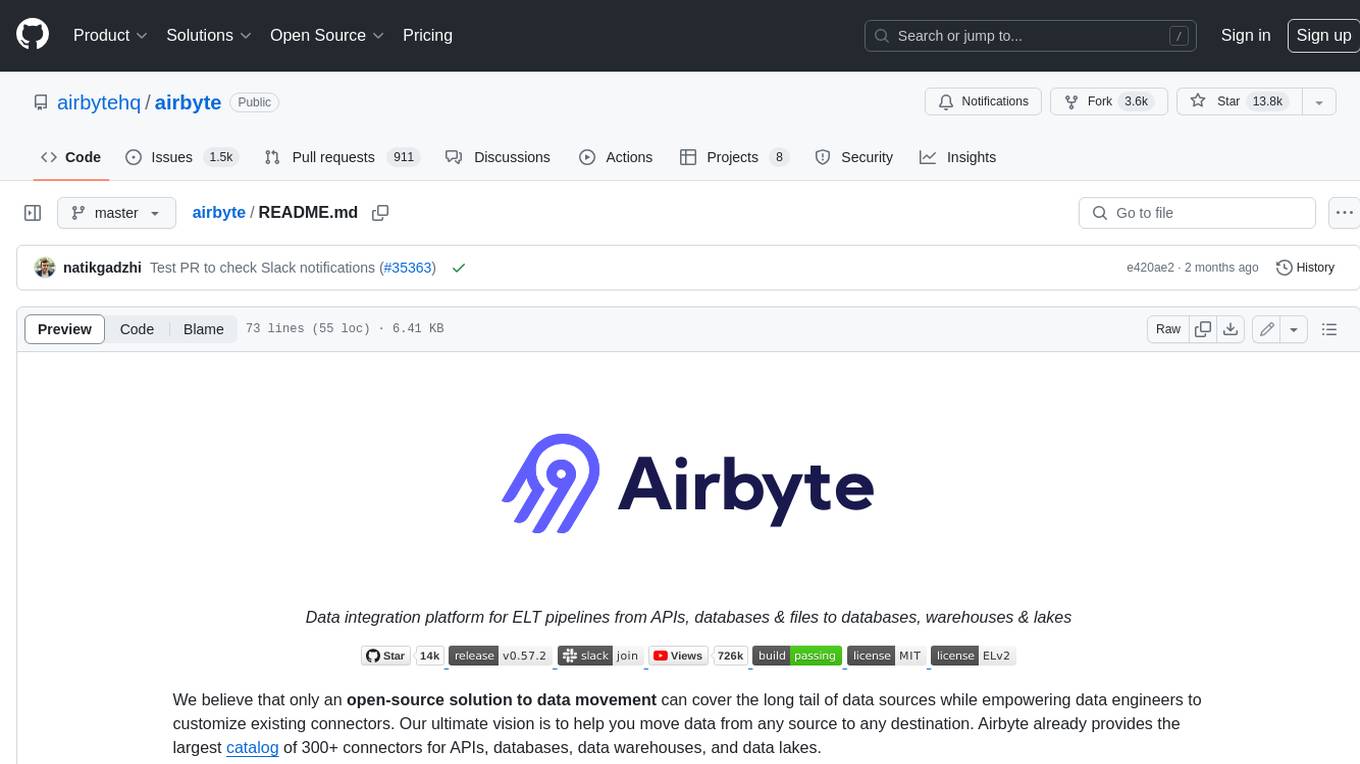
airbyte
Airbyte is an open-source data integration platform that makes it easy to move data from any source to any destination. With Airbyte, you can build and manage data pipelines without writing any code. Airbyte provides a library of pre-built connectors that make it easy to connect to popular data sources and destinations. You can also create your own connectors using Airbyte's no-code Connector Builder or low-code CDK. Airbyte is used by data engineers and analysts at companies of all sizes to build and manage their data pipelines.
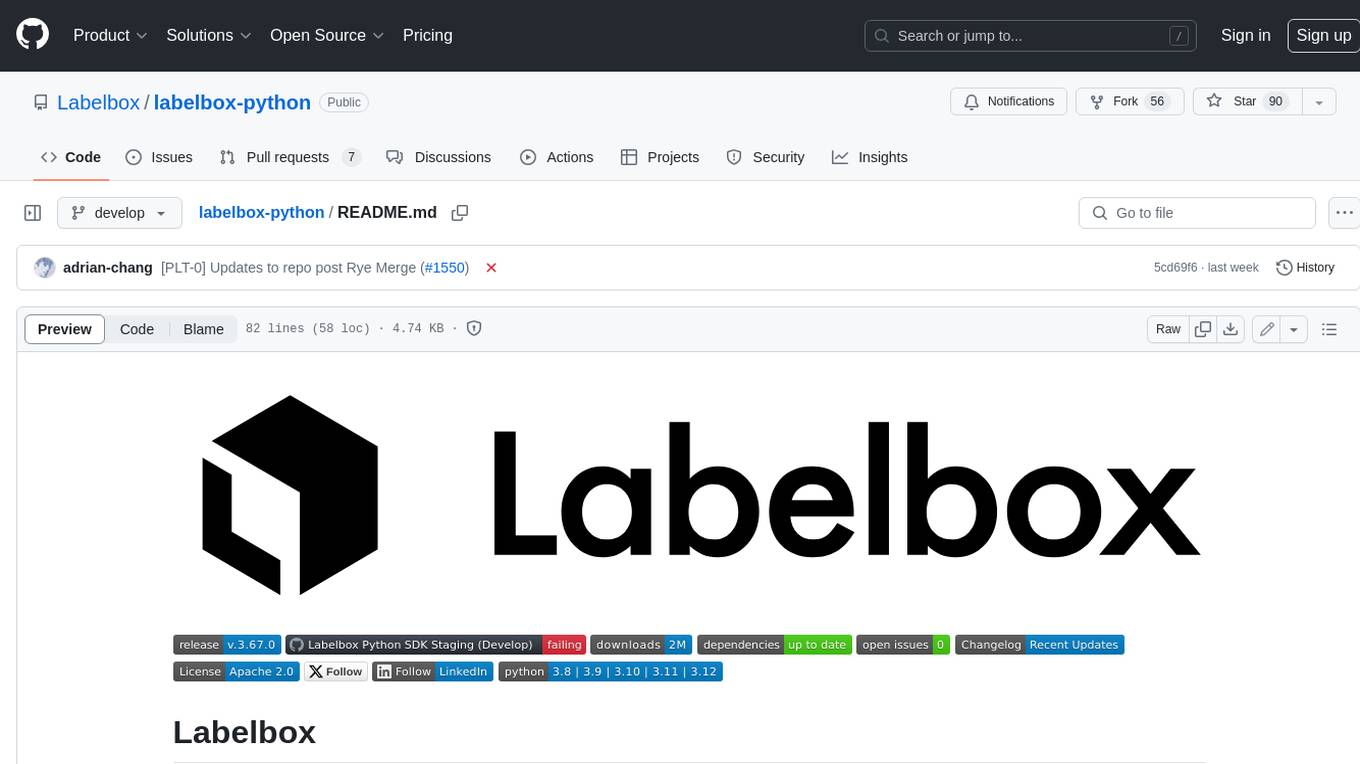
labelbox-python
Labelbox is a data-centric AI platform for enterprises to develop, optimize, and use AI to solve problems and power new products and services. Enterprises use Labelbox to curate data, generate high-quality human feedback data for computer vision and LLMs, evaluate model performance, and automate tasks by combining AI and human-centric workflows. The academic & research community uses Labelbox for cutting-edge AI research.