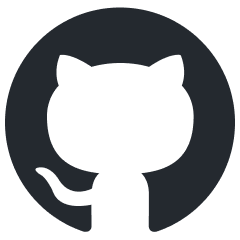
Numpy.NET
C#/F# bindings for NumPy - a fundamental library for scientific computing, machine learning and AI
Stars: 722
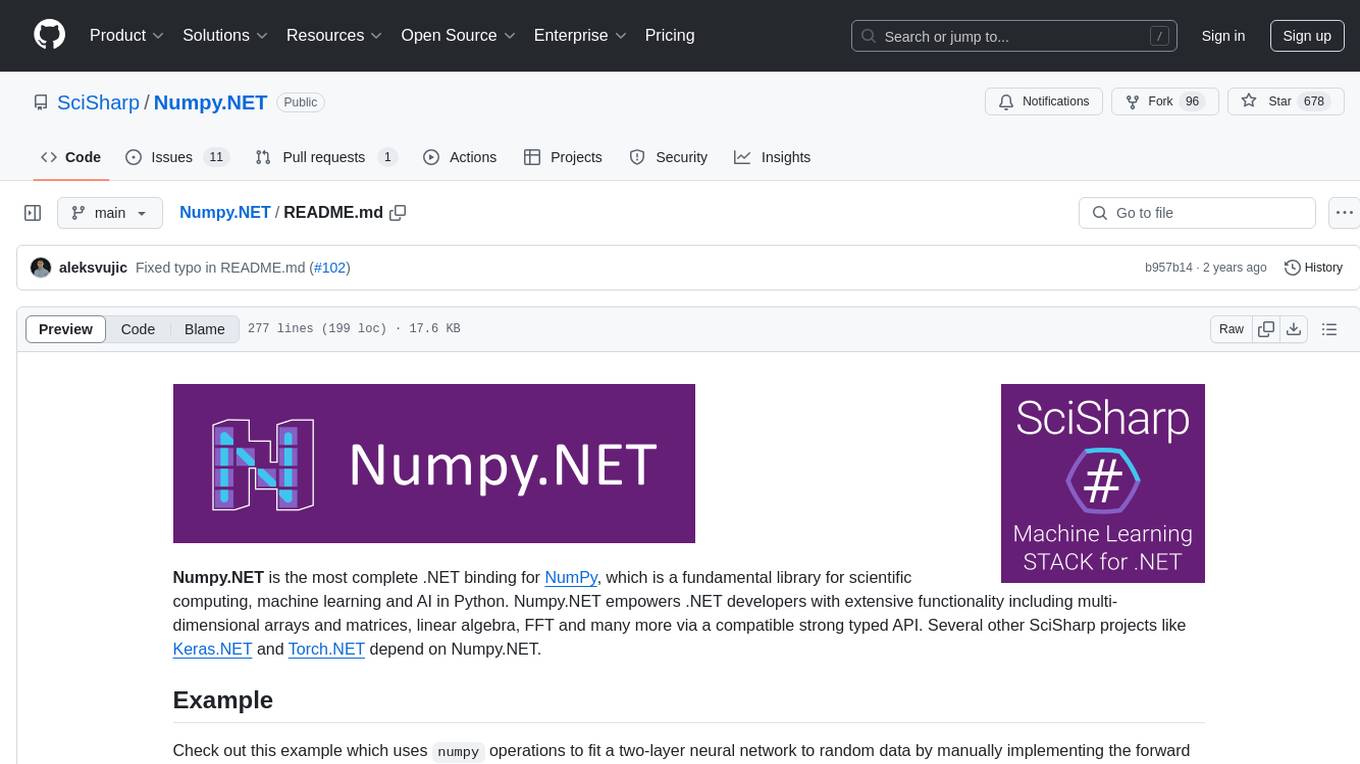
Numpy.NET is the most complete .NET binding for NumPy, empowering .NET developers with extensive functionality for scientific computing, machine learning, and AI. It provides multi-dimensional arrays, matrices, linear algebra, FFT, and more via a strong typed API. Numpy.NET does not require a local Python installation, as it uses Python.Included to package embedded Python 3.7. Multi-threading must be handled carefully to avoid deadlocks or access violation exceptions. Performance considerations include overhead when calling NumPy from C# and the efficiency of data transfer between C# and Python. Numpy.NET aims to match the completeness of the original NumPy library and is generated using CodeMinion by parsing the NumPy documentation. The project is MIT licensed and supported by JetBrains.
README:
Numpy.NET is the most complete .NET binding for NumPy, which is a fundamental library for scientific computing, machine learning and AI in Python. Numpy.NET empowers .NET developers with extensive functionality including multi-dimensional arrays and matrices, linear algebra, FFT and many more via a compatible strong typed API. Several other SciSharp projects like Keras.NET and Torch.NET depend on Numpy.NET.
Check out this example which uses numpy
operations to fit a two-layer neural network to random data by manually implementing the forward and backward passes through the network.
Numpy and Intellisense: a developer-friendly combination:
If you want to use Numpy.NET you have two options:
Just reference Numpy.dll via Nuget, set your build configuration to x64 and you are good to go. Thanks to Python.Included it doesn't require a local Python installation or will not clash with existing installations.
In certain use cases you might not want the packaged Python and NumPy packages. In that case you reference Numpy.Bare.dll via Nuget. Depending on the Numpy.Bare nuget version will need Python 3.5, 3.6 or 3.7 and Numpy 1.16 installed for it to work. The first two digits of the Numpy.Bare version indicate which Python version is needed for it to run (i.e. Numpy.Bare v3.6.1.1 needs Python 3.6 installed). If you are getting BadImageFormatException switch between x86 and x64 build settings.
In other cases, you might want to control the install location of the Python installation or even set it up yourself instead of having the Numpy library do it. For those cases Numpy.Bare is also great. Check out the custom installation example if you want to know how.
Numpy.NET uses Python for .NET to call into the Python module numpy
. However, this does not mean that it depends on a local Python installation! Numpy.NET.dll uses Python.Included which packages embedded Python 3.7 and automatically deploys it in the user's home directory upon first execution. On subsequent runs, it will find Python already deployed and therefore doesn't install it again. Numpy.NET also packages the NumPy wheel and installs it into the embedded Python installation when not yet installed.
Long story short: as a .NET Developer you don't need to worry about Python at all. You just reference Numpy.NET, use it and it will just work, no matter if you have local Python installations or not.
Beware: Not following these steps can result in deadlocks or access violation exceptions!
Python/NumPy doesn't have real multi-threading support. There is no advantage in "simultaneously" executing numpy
functions on multiple threads because pythonnet
requires you to use the Global Interpreter Lock (GIL) to lock access to the Python engine exclusively for only one thread at a time. If you have to call Python from a thread other than the main thread you first must release the main thread's mutex by calling PythonEngine.BeginAllowThreads()
or you'll have a deadlock:
var a = np.arange(1000);
var b = np.arange(1000);
// https://github.com/pythonnet/pythonnet/issues/109
PythonEngine.BeginAllowThreads();
Task.Run(()=> {
// when running on different threads you must lock!
using (Py.GIL())
{
np.matmul(a, b);
}
}).Wait();
Above example only serves as a reference on how to call numpy
from a different thread than the main thread. As said before, having multiple background threads that call into Python doesn't give you multi-core processing because of the requirement to lock the GIL. Not doing so will result in access violation exceptions and/or deadlocks.
Note that you must call a method of np
before calling PythonEngine.BeginAllowThreads()
in order for the PythonEngine to be initialized. So, for instance, if you want to initialize an inherently multi-threaded .Net Core Web API at startup, do something like this:
np.arange(1);
PythonEngine.BeginAllowThreads();
Also, if you do this, be sure to wrap any calls to Numpy in using (Py.GIL()) { ... }
or else you'll get AccessViolationExceptions.
You might ask how calling into Python affects performance. As always, it depends on your usage. Don't forget that numpy
's number crunching algorithms are written in C
so the thin pythonnet
and Python
layers on top won't have a significant impact if you are working with larger amounts of data.
In my experience, calling numpy
from C# is about 4 times slower than calling it directly in Python
while the execution time of the called operation is of course equal. So if you have an algorithm that needs to call into numpy
in a nested loop, Numpy.NET may not be for you due to the call overhead.
All of numpy
is centered around the ndarray
class which allows you to pass a huge chunk of data into the C
routines and let them execute all kinds of operations on the elements efficiently without the need for looping over the data. So if you are manipulating arrays or matrices with thousands or hundreds of thousands of elements, the call overhead will be negligible.
The most performance sensitive aspect is creating an NDarray
from a C#
array, since the data has to be moved from the CLR
into the Python
interpreter. pythonnet
does not optimize for passing large arrays from C#
to Python
but we still found a way to do that very efficiently. When creating an array with np.array( ... )
we internally use Marshal.Copy
to copy the entire C#
-array's memory into the numpy
-array's storage. And to efficiently retrieve computation results from numpy
there is a method called GetData<T>
which will copy the data back to C#
in the same way:
// create a 2D-shaped NDarray<int> from an int[]
var m = np.array(new int[] {1, 2, 3, 4});
// calculate the cosine of each element
var result = np.cos(m);
// get the floating point data of the result NDarray back to C#
var data = result.GetData<double>(); // double[] { 0.54030231, -0.41614684, -0.9899925 , -0.65364362 }
The SciSharp team is also developing a pure C# port of NumPy called NumSharp which is quite popular albeit being not quite complete.
There are a couple of other NumPy ports out there featuring subsets of the original library. The only one that matches Numpy.NET in terms of completeness is the IronPython package numpy
which is out of date though. The SciSharp team is committed to keeping Numpy.NET up to date with the original library and to feature as much of the original functionality as possible.
The vast majority of Numpy.NET's code is generated using CodeMinion by parsing the documentation at docs.scipy.org/doc/numpy/. This allowed us to wrap most of the numpy
-API in just two weeks. The rest of the API can be completed in a few more weeks, especially if there is popular demand.
The following API categories have been generated (if checked off)
- [x] Array creation routines
- [x] Array manipulation routines
- [x] Binary operations
- [x] String operations
- [x] Datetime Support Functions
- [x] Data type routines
- [x] Optionally Scipy-accelerated routines(numpy.dual)
- [ ] Floating point error handling
- [x] Discrete Fourier Transform(numpy.fft)
- [x] Financial functions
- [ ] Functional programming
- [x] Indexing routines
- [x] Input and output
- [x] Linear algebra(numpy.linalg)
- [x] Logic functions
- [ ] Masked array operations
- [x] Mathematical functions
- [ ] Matrix library(numpy.matlib)
- [ ] Miscellaneous routines
- [x] Padding Arrays
- [ ] Polynomials
- [x] Random sampling(numpy.random)
- [x] Set routines
- [x] Sorting, searching, and counting
- [x] Statistics
- [x] Window functions
Over 500 functions of all 1800 have been generated, most of the missing functions are duplicates on Matrix, Chararray, Record etc.
We even generated hundreds of unit tests from all the examples found on the NumPy documentation. Most of them don't compile without fixing them because we did not go so far as to employ a Python-To-C# converter. Instead, the tests are set up with a commented out Python-console log that shows what the expected results are and a commented out block of somewhat C#-ified Python code that doesn't compile without manual editing.
Another reason why this process can not be totally automated is the fact that NumPy obviously changed the way how arrays are printed out on the console after most of the examples where written (they removed extra spaces between the elements). This means that oftentimes the results needs to be reformatted manually for the test to pass.
Getting more unit tests to run is very easy though, and a good portion have already been processed to show that Numpy.NET really works. If you are interested in working on the test suite, please join in and help. You'll learn a lot about NumPy on the way.
Since we have taken great care to make Numpy.NET as similar to NumPy itself, you can, for the most part, rely on the official NumPy manual.
To work with data from C# in Numpy it has to be copied into the Python engine by using np.array(...)
. You get an NDarray that you can use for further processing of the data. Here we calculate the square root:
// create an NDarray from a C# array
var a = np.array(new[] { 2, 4, 9, 25 });
Console.WriteLine("a: "+ a.repr);
// a: array([ 2, 4, 9, 25])
// apply the square root to each element
var roots = np.sqrt(a);
Console.WriteLine(roots.repr);
// array([1.41421356, 2. , 3. , 5. ])
After processing the data you can copy it back into a C# array use a.GetData<int>()
, but be aware of the datatype of the NDarray in order to get correct values back:
// Copy the NDarray roots into a C# array from NDarray (incorrect datatype)
Console.WriteLine(string.Join(", ", roots.GetData<int>()));
// 1719614413, 1073127582, 0, 1073741824
Console.WriteLine("roots.dtype: " + roots.dtype);
// roots.dtype: float64
Console.WriteLine(string.Join(", ", roots.GetData<double>()));
// 1.4142135623731, 2, 3, 5
Creating an NDarray
from data is easy. Just pass the C# array into np.array(...)
. You can pass 1D, 2D and 3D C# arrays into it.
// create a 2D NDarray
var m = np.array(new int[,] {{1, 2}, {3, 4}}); // the NDarray represents a 2 by 2 matrix
Another even more efficient way (saves one array copy operation) is to pass the data as a one-dimensional array and just reshape the NDarray.
// create a 2D NDarray
var m = np.array(new int[] {1, 2, 3, 4}).reshape(2,2); // the reshaped NDarray represents a 2 by 2 matrix
As you have seen, apart from language syntax and idioms, usage of Numpy.NET is almost identical to Python. However, due to lack of language support in C#, there are some differences which you should be aware of.
You can access parts of an NDarray using array slicing. C# doesn't support the colon syntax in indexers i.e. a[:, 1]
. However, by allowing to pass a string we circumvented this limitation, i.e. a[":, 1"]
. Only the ...
operator is not yet implemented, as it is not very important.
Some NumPy functions like reshape
allow variable argument lists at the beginning of the parameter list. This of course is not allowed in C#, which supports a variable argument list only as the last parameter. In case of reshape
the solution was to replace the variable argument list that specifies the dimensions of the reshaped array by a Shape
object which takes a variable list of dimensions in its constructor:
var a=np.arange(24);
var b=np.reshape(a, new Shape(2, 3, 4)); // or a.reshape(2, 3, 4)
In other cases the problem was solved by providing overloads without the optional named parameters after the variable argument list.
C# is very strict about parameter default values which must be compile time constants. Also, parameters with default values must be at the end of the parameter list. The former problem is usually solved by having a default value of null
instead of the non-compile-time constant and setting the correct default value inside the method body when called with a value of null
.
Python operators that are not supported in C# are exposed as instance methods of NDarray
:
Python | C# |
---|---|
// | floordiv() |
** | pow() |
In NumPy you can write a*=2
which doubles each element of array a
. C# doesn't have a *=
operator and consequently overloading it in a Python fashion is not possible. This limitation is overcome by exposing inplace operator functions which all start with an 'i'. So duplicating all elements of array a
is written like this in C#: a.imul(2);
. The following table lists all inplace operators and their pendant in C#
Python | C# |
---|---|
+= | iadd() |
-= | isub() |
*= | imul() |
/= | idiv() or itruediv() |
//= | ifloordiv() |
%= | imod() |
**= | ipow() |
<<= | ilshift() |
>>= | irshift() |
&= | iand() |
|= | ior() |
^= | ixor() |
In NumPy a function like np.sqrt(a)
can either return a scalar or an array, depending on the value passed into it. In C# we are trying to solve this by creating overloads for every scalar type in addition to the original function that takes an array and returns an array. This bloats the API however and hasn't been done for most functions and for some it isn't possible at all due to language limitations. The alternative is to cast value types to an array (NDarray can represent scalars too):
So a simple root = np.sqrt(2)
needs to be written somewhat clumsily like this in C#: var root = (double)np.sqrt((NDarray)2.0);
until overloads for every value type are added in time for your convenience. In any case, the main use case for NumPy functions is to operate on the elements of arrays, which is convenient enough:
var a = np.arange(100); // => { 0, 1, 2, ... , 98, 99 }
var roots = np.sqrt(a); // => { 0.0, 1.0, 1.414, ..., 9.899, 9.950 }
Numpy.NET supports complex numbers even though the notation in Python and C# is very different:
>>> a = np.array([1+2j, 3+4j, 5+6j])
looks like this in C#
var a = np.array(new Complex[] { new Complex(1, 2), new Complex(3,4), new Complex(5,6), });
Access the real and imaginary components of a complex array via a.real
and a.imag
or copy the complex values of an ndarray into C# with Complex[] c=a.GetData<Complex>();
.
The function fft(...) in numpy.fft and random(...) in numpy.random had to be renamed because C# doesn't allow a member to have the same name as its enclosing class. That's why in Numpy.NET these functions have been renamed with a trailing underscore like this: np.fft.fft_(...)
Currently, Numpy.dll is targeting .NET Standard (on Windows) and packages the following binaries:
- Python 3.7: (python-3.7.3-embed-amd64.zip)
- NumPy 1.16 (numpy-1.16.3-cp37-cp37m-win_amd64.whl)
Numpy.Bare.dll is available for the Python versions 2.7, 3.5, 3.6 and 3.7 on Windows, Linux and MacOS on Nuget.
To make Numpy.dll support Linux a separate version of Python.Included packaging linux binaries of Python needs to be made and a version of Numpy.dll that packages a linux-compatible NumPy wheel. If you are interested, you may work on this issue.
Numpy.NET packages and distributes Python
, pythonnet
as well as numpy
. All these dependencies imprint their license conditions upon Numpy.NET. The C# wrapper itself is MIT License.
- Python: PSF License
- Python for .NET (pythonnet): MIT License
- NumPy: BSD License
- Numpy.NET: MIT License
- If you check
Prefer 32-bit
in your build config or build withx86
instead ofAny CPU
Numpy.NET will crash withBadFormatException
- If you have insufficient folder permissions in AppData Numpy.NET might crash. You can specify a different installpath by setting
Installer.INSTALL_PATH = "<install path>";
- If you get deadlocks (program hangs indefinitely) you should read the secton about multi-threading above!
- If you get AccessViolationExceptions you should read the secton about multi-threading above!
For Tasks:
Click tags to check more tools for each tasksFor Jobs:
Alternative AI tools for Numpy.NET
Similar Open Source Tools
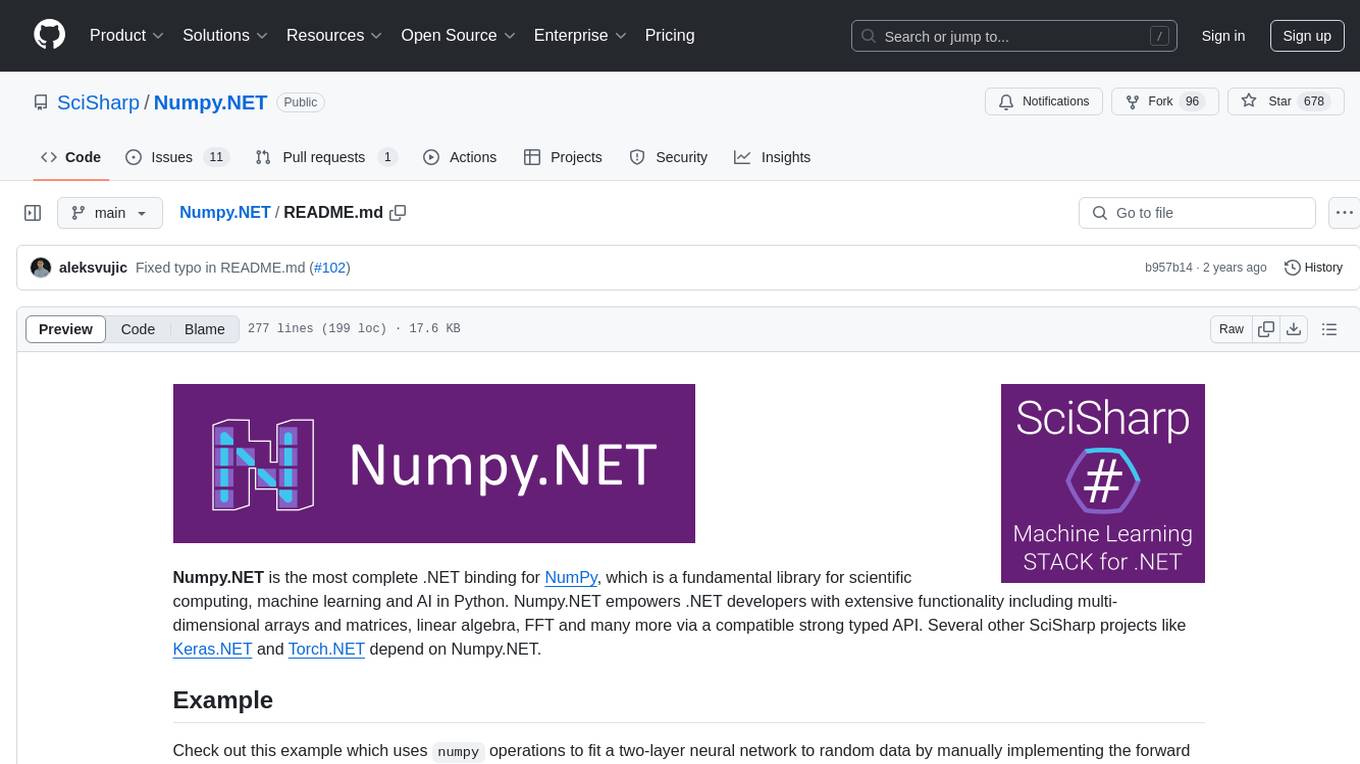
Numpy.NET
Numpy.NET is the most complete .NET binding for NumPy, empowering .NET developers with extensive functionality for scientific computing, machine learning, and AI. It provides multi-dimensional arrays, matrices, linear algebra, FFT, and more via a strong typed API. Numpy.NET does not require a local Python installation, as it uses Python.Included to package embedded Python 3.7. Multi-threading must be handled carefully to avoid deadlocks or access violation exceptions. Performance considerations include overhead when calling NumPy from C# and the efficiency of data transfer between C# and Python. Numpy.NET aims to match the completeness of the original NumPy library and is generated using CodeMinion by parsing the NumPy documentation. The project is MIT licensed and supported by JetBrains.
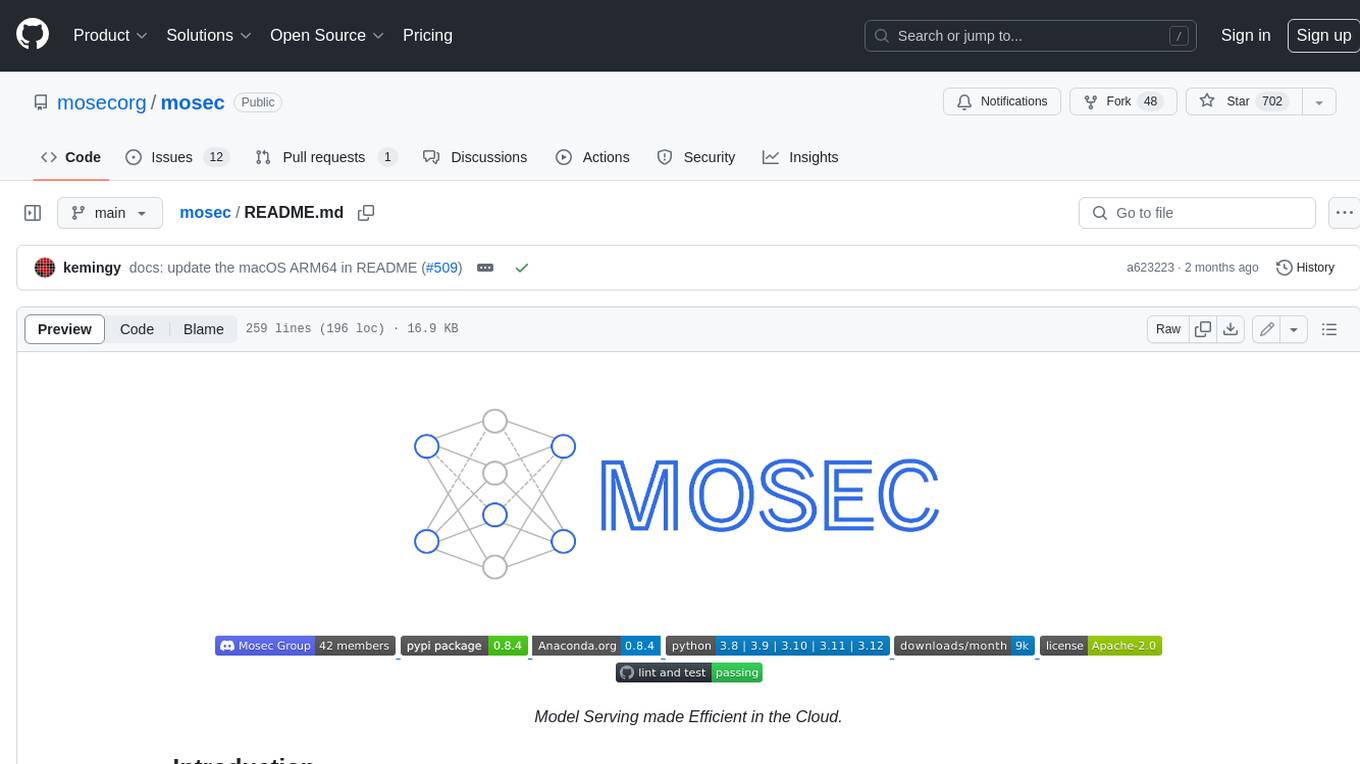
mosec
Mosec is a high-performance and flexible model serving framework for building ML model-enabled backend and microservices. It bridges the gap between any machine learning models you just trained and the efficient online service API. * **Highly performant** : web layer and task coordination built with Rust 🦀, which offers blazing speed in addition to efficient CPU utilization powered by async I/O * **Ease of use** : user interface purely in Python 🐍, by which users can serve their models in an ML framework-agnostic manner using the same code as they do for offline testing * **Dynamic batching** : aggregate requests from different users for batched inference and distribute results back * **Pipelined stages** : spawn multiple processes for pipelined stages to handle CPU/GPU/IO mixed workloads * **Cloud friendly** : designed to run in the cloud, with the model warmup, graceful shutdown, and Prometheus monitoring metrics, easily managed by Kubernetes or any container orchestration systems * **Do one thing well** : focus on the online serving part, users can pay attention to the model optimization and business logic
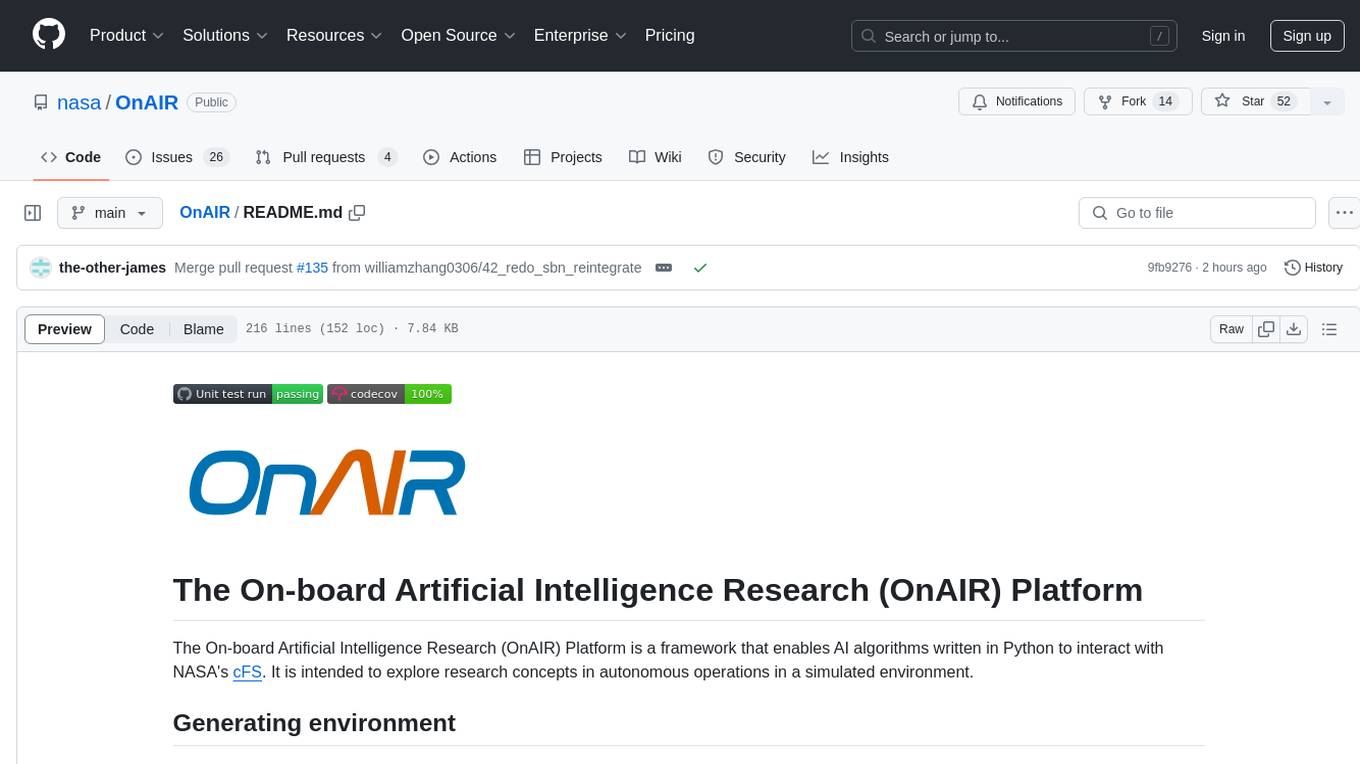
OnAIR
The On-board Artificial Intelligence Research (OnAIR) Platform is a framework that enables AI algorithms written in Python to interact with NASA's cFS. It is intended to explore research concepts in autonomous operations in a simulated environment. The platform provides tools for generating environments, handling telemetry data through Redis, running unit tests, and contributing to the repository. Users can set up a conda environment, configure telemetry and Redis examples, run simulations, and conduct unit tests to ensure the functionality of their AI algorithms. The platform also includes guidelines for licensing, copyright, and contributions to the repository.
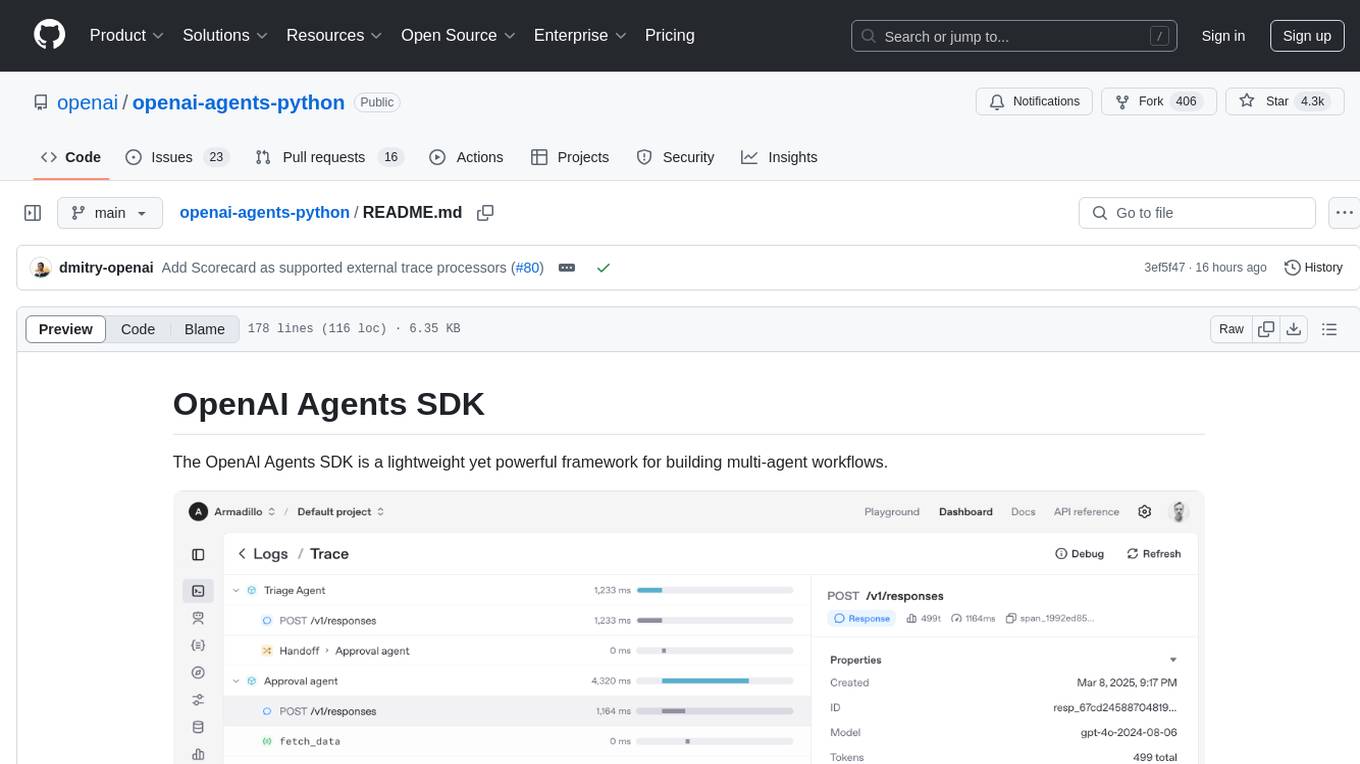
openai-agents-python
The OpenAI Agents SDK is a lightweight framework for building multi-agent workflows. It includes concepts like Agents, Handoffs, Guardrails, and Tracing to facilitate the creation and management of agents. The SDK is compatible with any model providers supporting the OpenAI Chat Completions API format. It offers flexibility in modeling various LLM workflows and provides automatic tracing for easy tracking and debugging of agent behavior. The SDK is designed for developers to create deterministic flows, iterative loops, and more complex workflows.
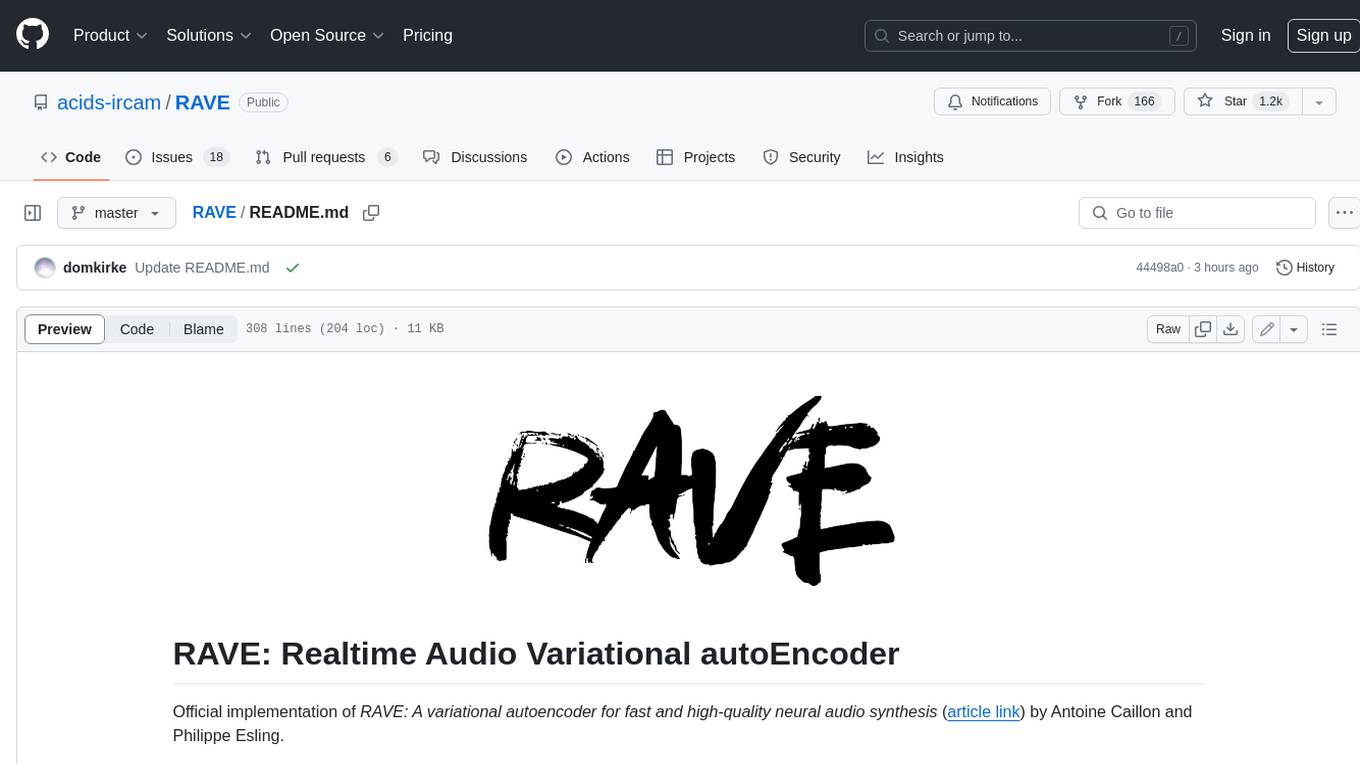
RAVE
RAVE is a variational autoencoder for fast and high-quality neural audio synthesis. It can be used to generate new audio samples from a given dataset, or to modify the style of existing audio samples. RAVE is easy to use and can be trained on a variety of audio datasets. It is also computationally efficient, making it suitable for real-time applications.
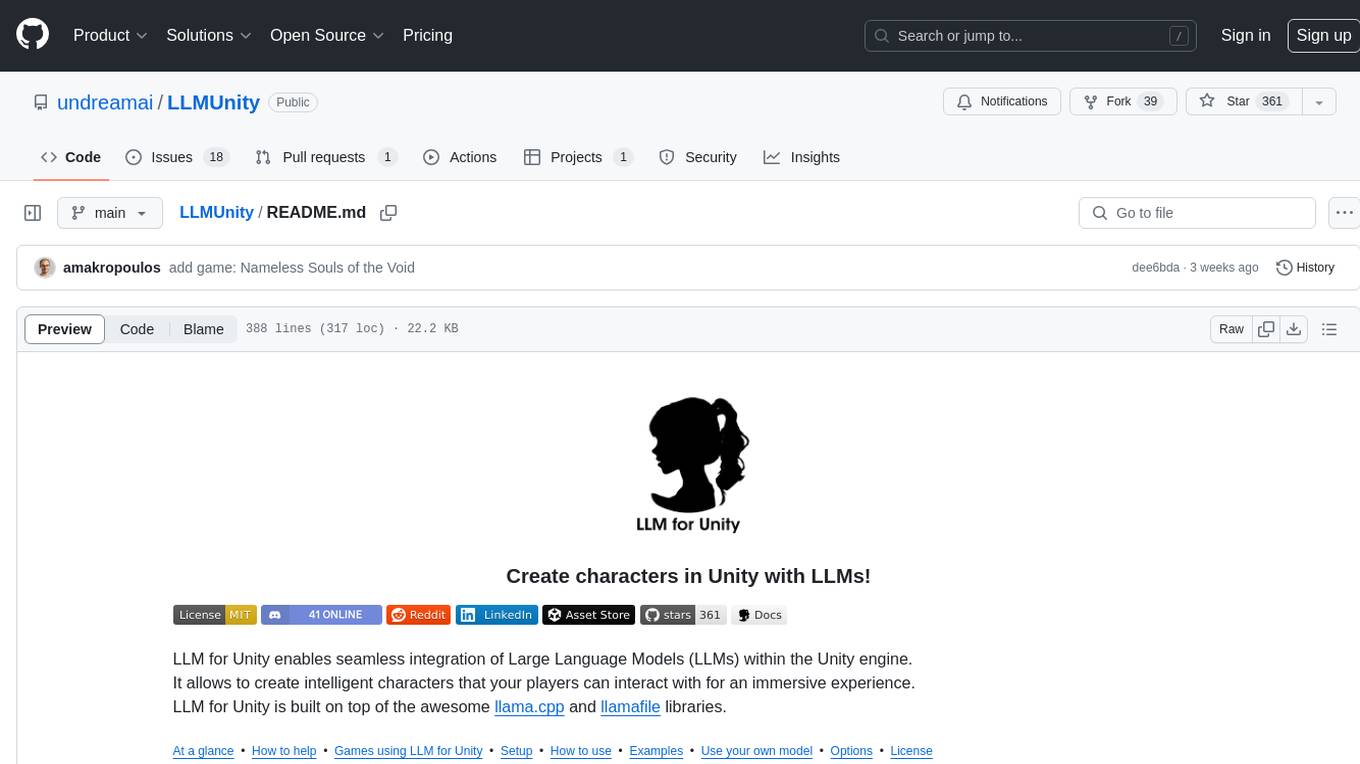
LLMUnity
LLM for Unity enables seamless integration of Large Language Models (LLMs) within the Unity engine, allowing users to create intelligent characters for immersive player interactions. The tool supports major LLM models, runs locally without internet access, offers fast inference on CPU and GPU, and is easy to set up with a single line of code. It is free for both personal and commercial use, tested on Unity 2021 LTS, 2022 LTS, and 2023. Users can build multiple AI characters efficiently, use remote servers for processing, and customize model settings for text generation.
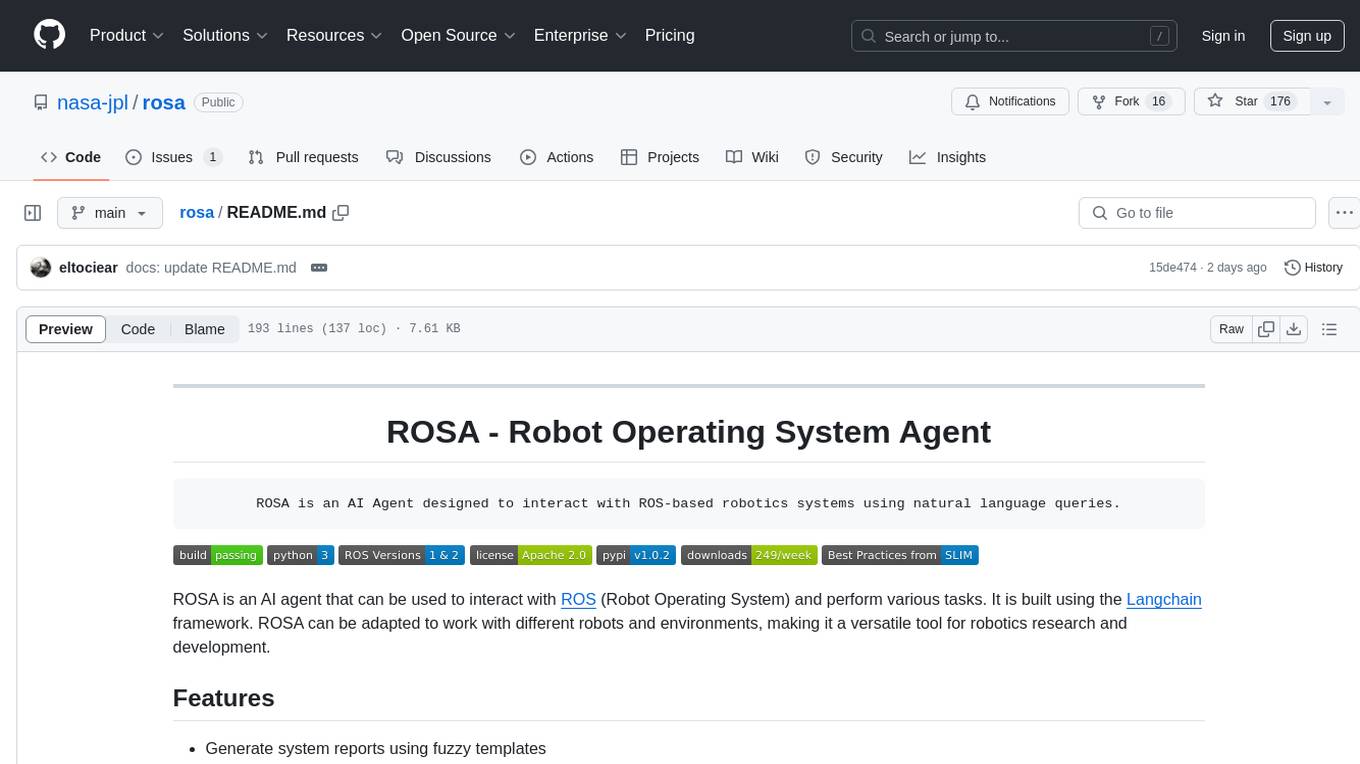
rosa
ROSA is an AI Agent designed to interact with ROS-based robotics systems using natural language queries. It can generate system reports, read and parse ROS log files, adapt to new robots, and run various ROS commands using natural language. The tool is versatile for robotics research and development, providing an easy way to interact with robots and the ROS environment.
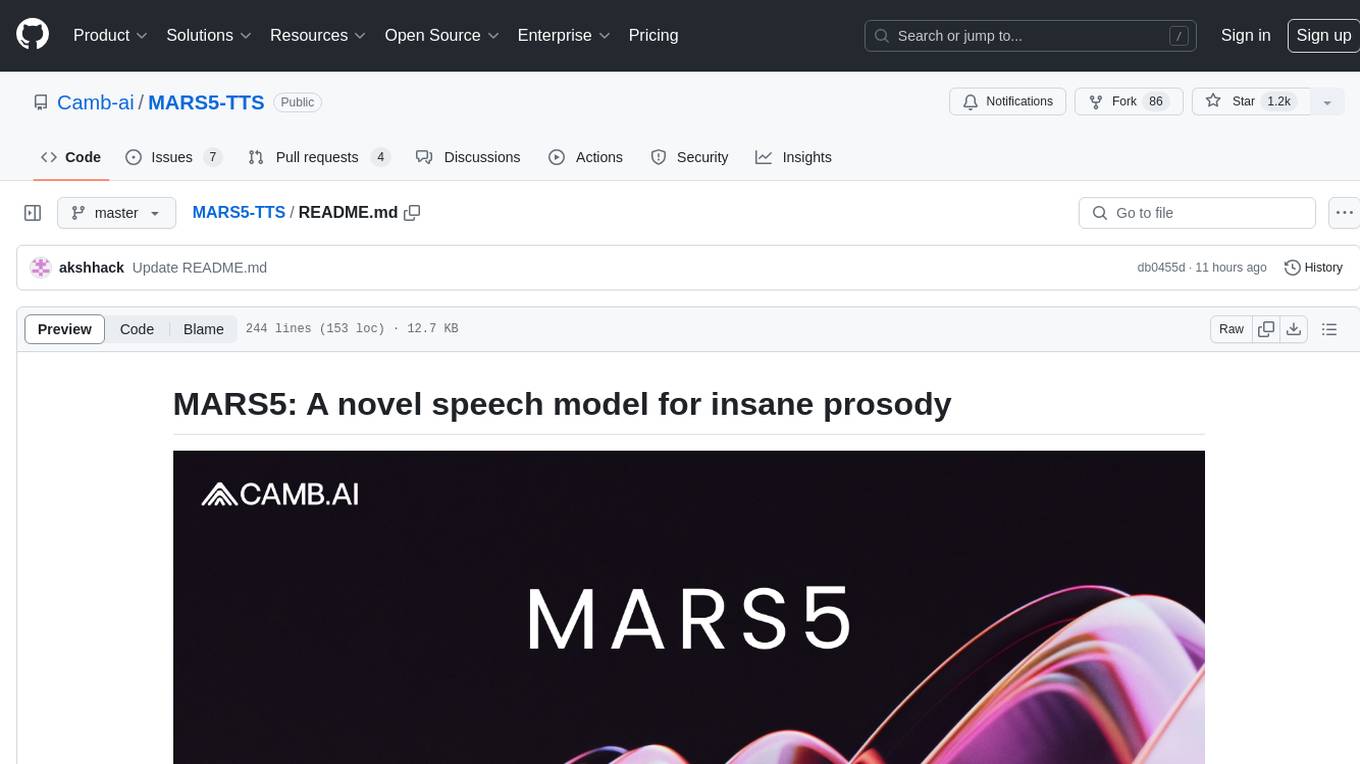
MARS5-TTS
MARS5 is a novel English speech model (TTS) developed by CAMB.AI, featuring a two-stage AR-NAR pipeline with a unique NAR component. The model can generate speech for various scenarios like sports commentary and anime with just 5 seconds of audio and a text snippet. It allows steering prosody using punctuation and capitalization in the transcript. Speaker identity is specified using an audio reference file, enabling 'deep clone' for improved quality. The model can be used via torch.hub or HuggingFace, supporting both shallow and deep cloning for inference. Checkpoints are provided for AR and NAR models, with hardware requirements of 750M+450M params on GPU. Contributions to improve model stability, performance, and reference audio selection are welcome.
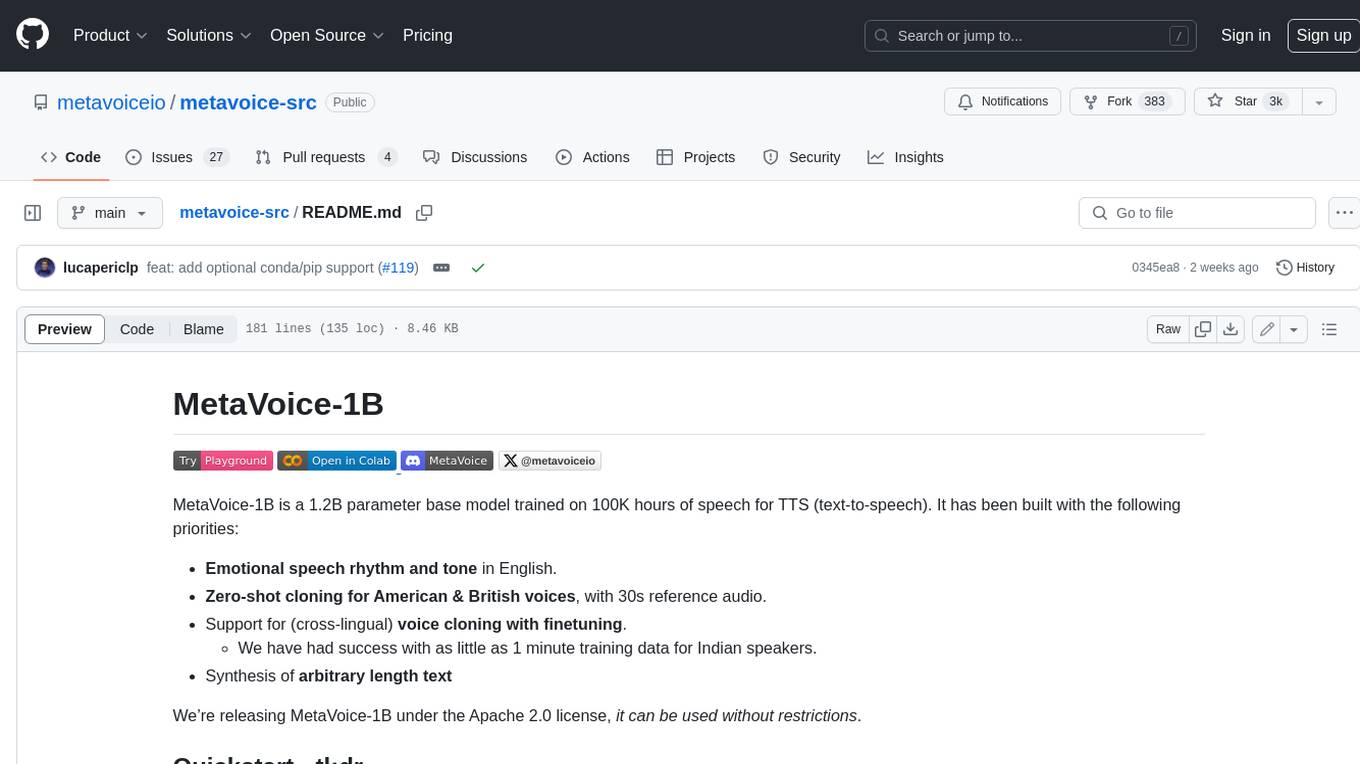
metavoice-src
MetaVoice-1B is a 1.2B parameter base model trained on 100K hours of speech for TTS (text-to-speech). It has been built with the following priorities: * Emotional speech rhythm and tone in English. * Zero-shot cloning for American & British voices, with 30s reference audio. * Support for (cross-lingual) voice cloning with finetuning. * We have had success with as little as 1 minute training data for Indian speakers. * Synthesis of arbitrary length text
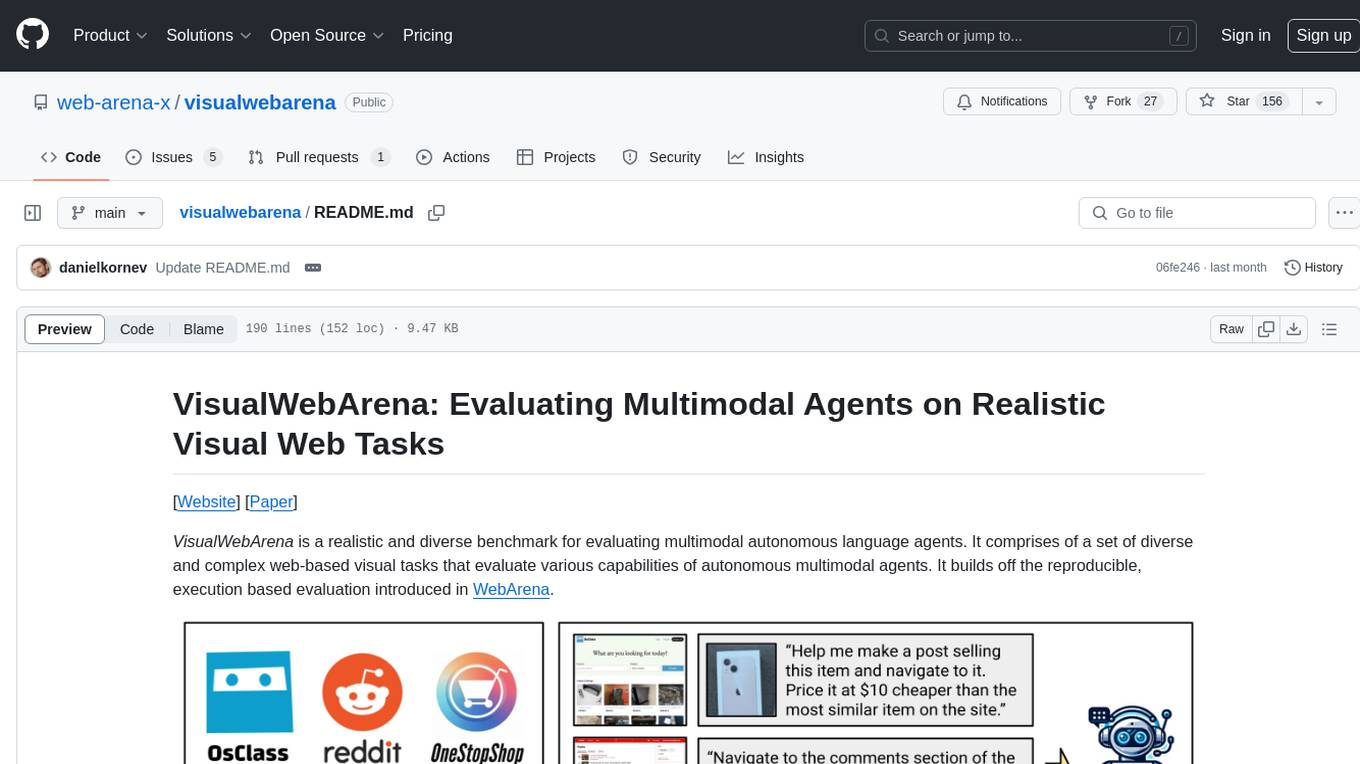
visualwebarena
VisualWebArena is a benchmark for evaluating multimodal autonomous language agents through diverse and complex web-based visual tasks. It builds on the reproducible evaluation introduced in WebArena. The repository provides scripts for end-to-end training, demos to run multimodal agents on webpages, and tools for setting up environments for evaluation. It includes trajectories of the GPT-4V + SoM agent on VWA tasks, along with human evaluations on 233 tasks. The environment supports OpenAI models and Gemini models for evaluation.
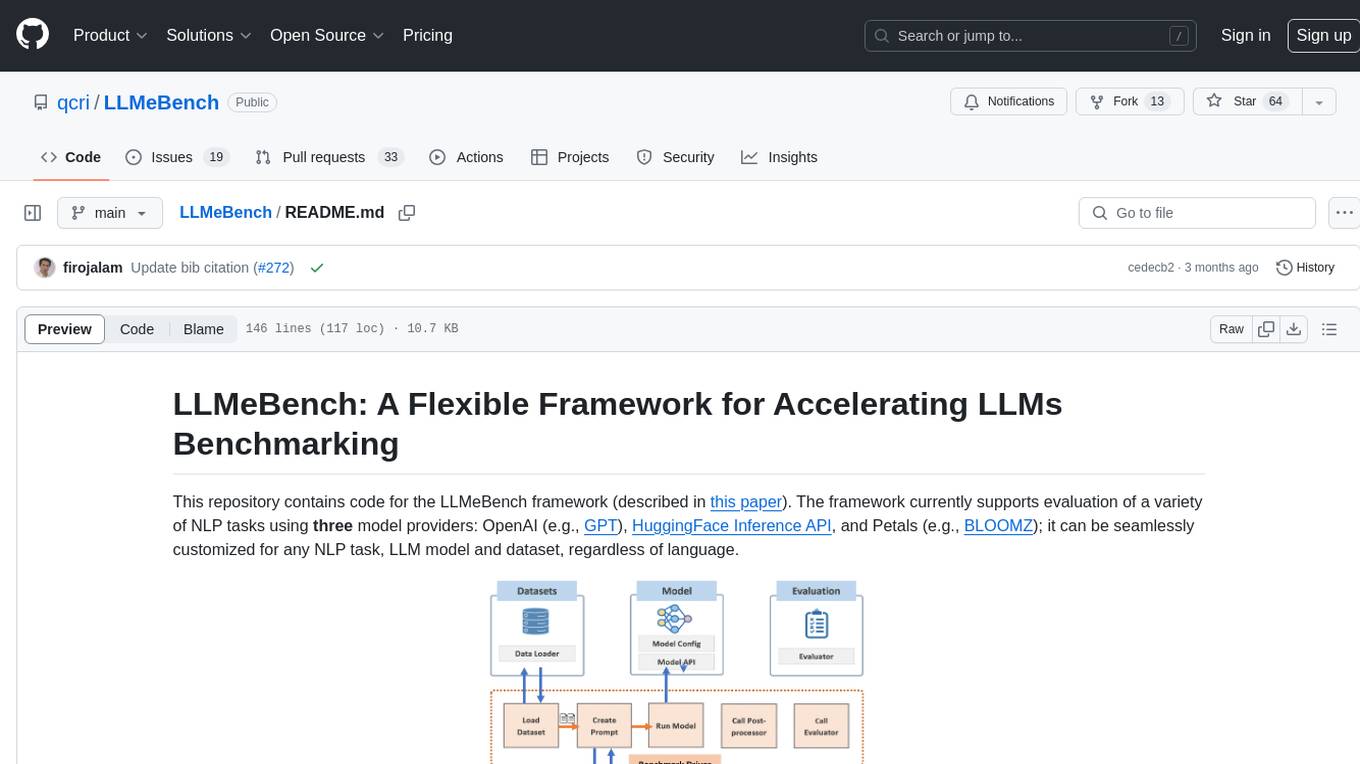
LLMeBench
LLMeBench is a flexible framework designed for accelerating benchmarking of Large Language Models (LLMs) in the field of Natural Language Processing (NLP). It supports evaluation of various NLP tasks using model providers like OpenAI, HuggingFace Inference API, and Petals. The framework is customizable for different NLP tasks, LLM models, and datasets across multiple languages. It features extensive caching capabilities, supports zero- and few-shot learning paradigms, and allows on-the-fly dataset download and caching. LLMeBench is open-source and continuously expanding to support new models accessible through APIs.
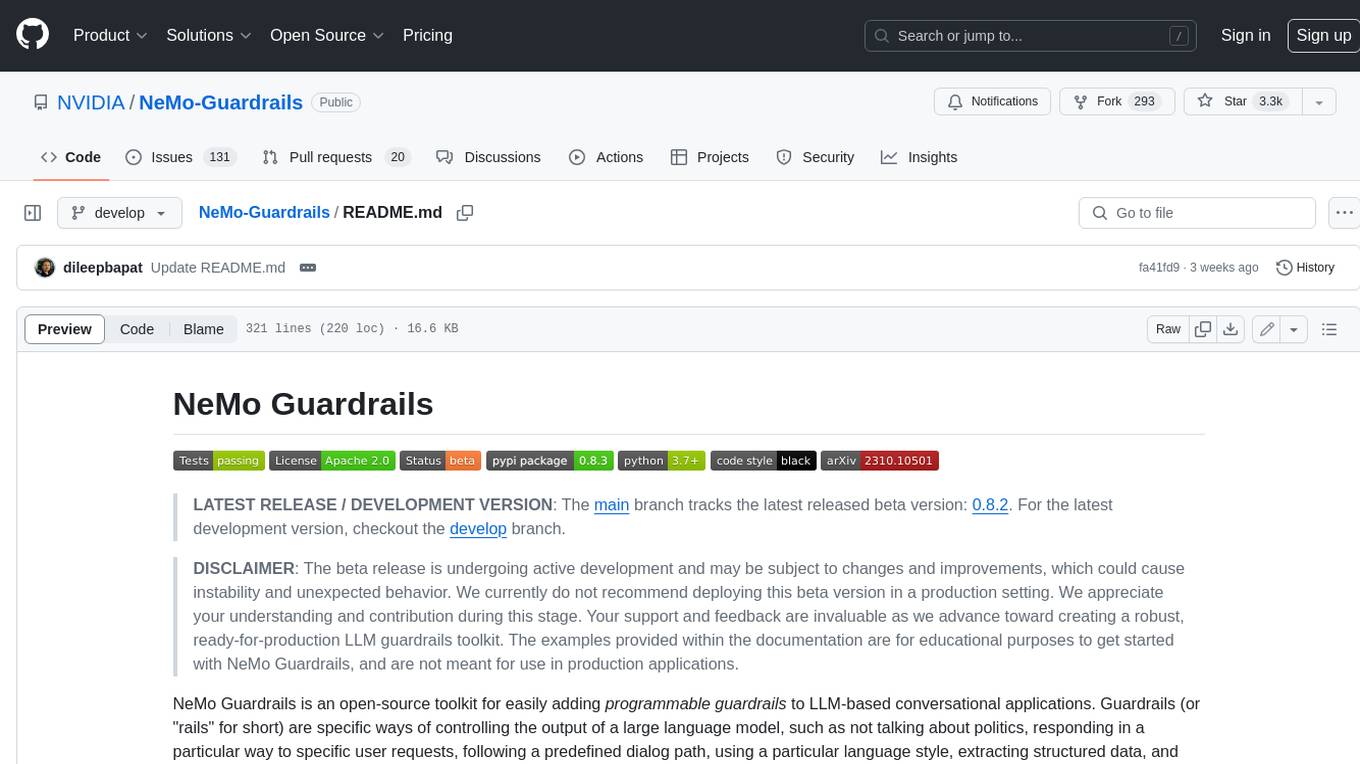
NeMo-Guardrails
NeMo Guardrails is an open-source toolkit for easily adding _programmable guardrails_ to LLM-based conversational applications. Guardrails (or "rails" for short) are specific ways of controlling the output of a large language model, such as not talking about politics, responding in a particular way to specific user requests, following a predefined dialog path, using a particular language style, extracting structured data, and more.
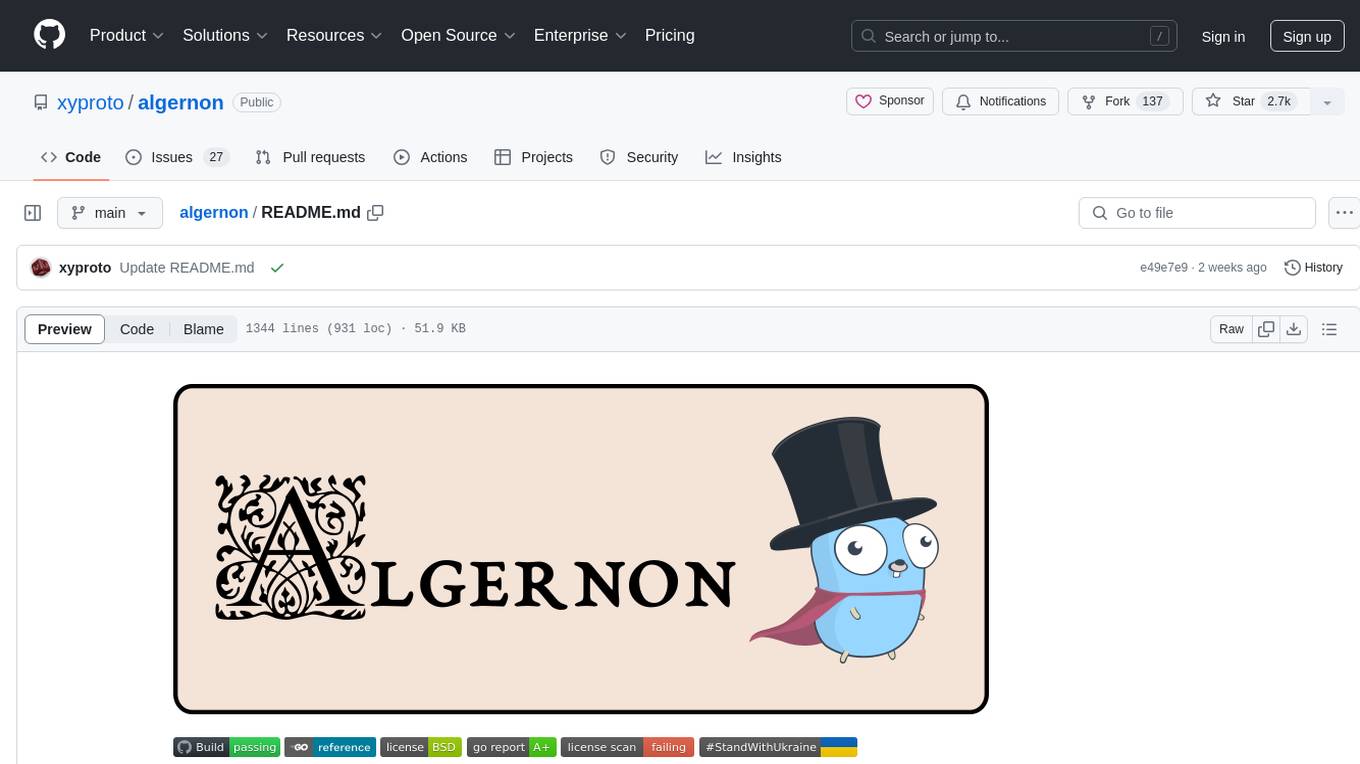
algernon
Algernon is a web server with built-in support for QUIC, HTTP/2, Lua, Teal, Markdown, Pongo2, HyperApp, Amber, Sass(SCSS), GCSS, JSX, Ollama (LLMs), BoltDB, Redis, PostgreSQL, MariaDB/MySQL, MSSQL, rate limiting, graceful shutdown, plugins, users, and permissions. It is a small self-contained executable that supports various technologies and features for web development.
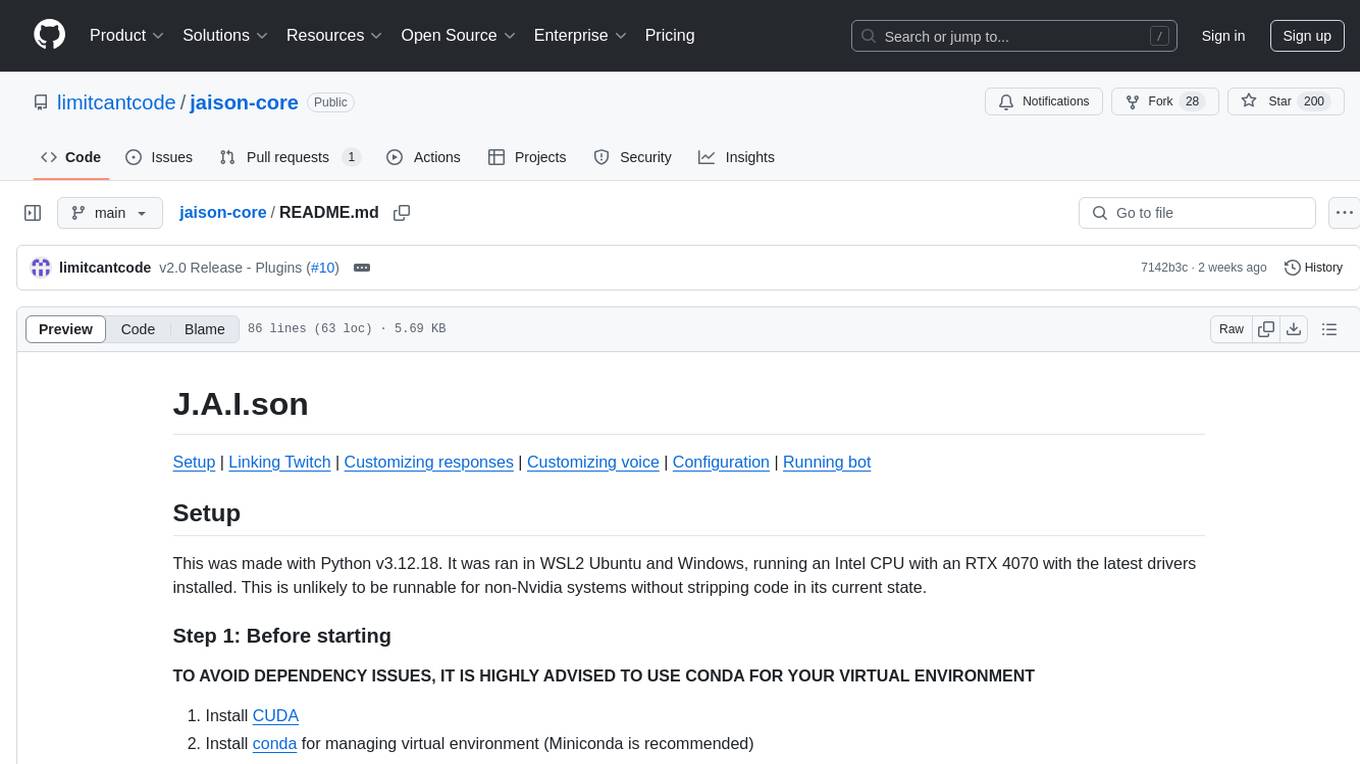
jaison-core
J.A.I.son is a Python project designed for generating responses using various components and applications. It requires specific plugins like STT, T2T, TTSG, and TTSC to function properly. Users can customize responses, voice, and configurations. The project provides a Discord bot, Twitch events and chat integration, and VTube Studio Animation Hotkeyer. It also offers features for managing conversation history, training AI models, and monitoring conversations.
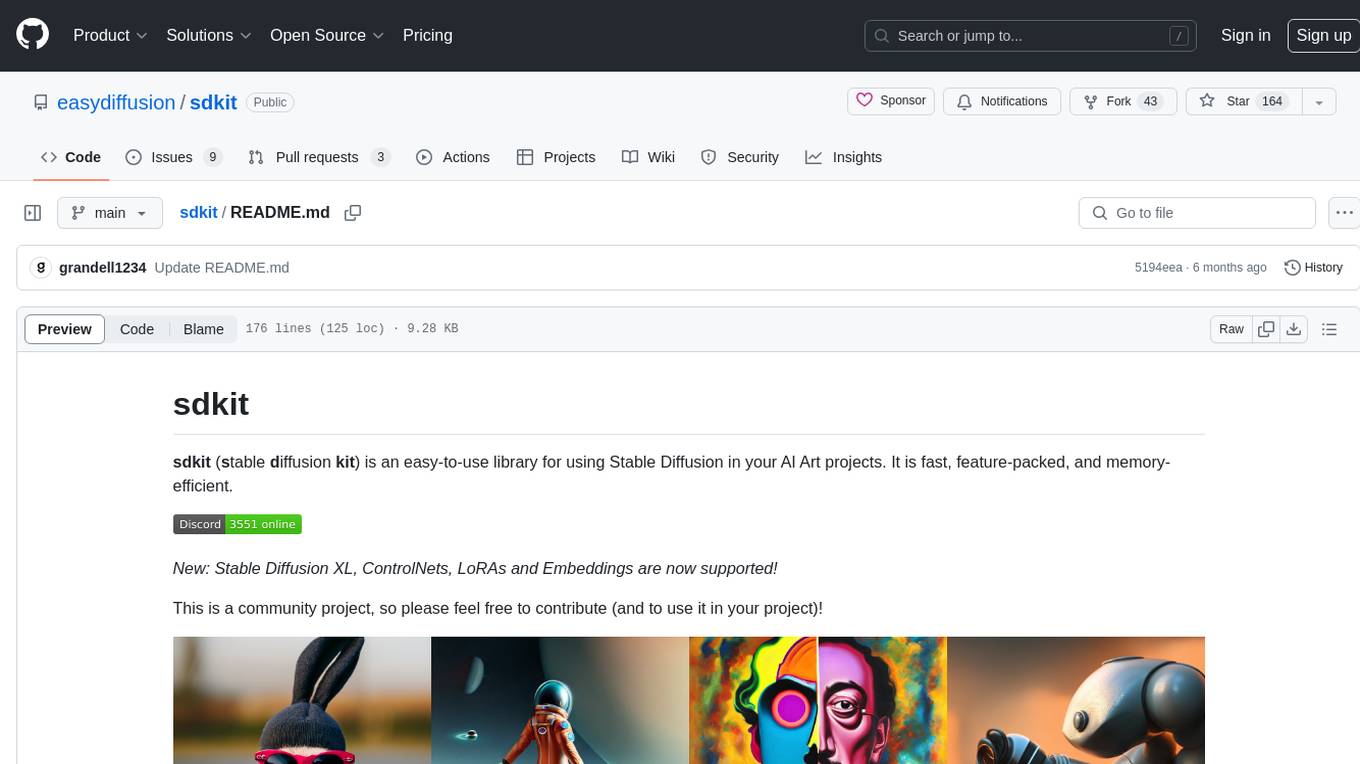
sdkit
sdkit (stable diffusion kit) is an easy-to-use library for utilizing Stable Diffusion in AI Art projects. It includes features like ControlNets, LoRAs, Textual Inversion Embeddings, GFPGAN, CodeFormer for face restoration, RealESRGAN for upscaling, k-samplers, support for custom VAEs, NSFW filter, model-downloader, parallel GPU support, and more. It offers a model database, auto-scanning for malicious models, and various optimizations. The API consists of modules for loading models, generating images, filters, model merging, and utilities, all managed through the sdkit.Context object.
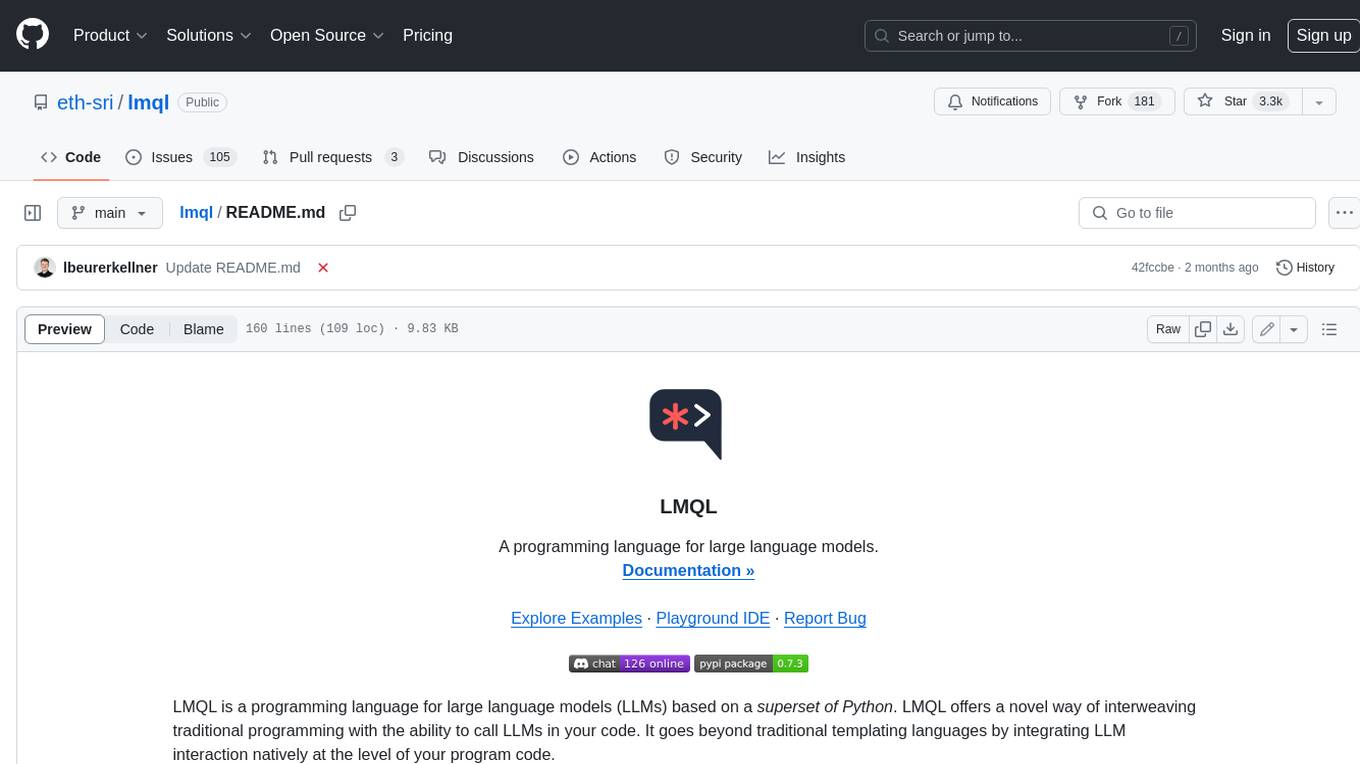
lmql
LMQL is a programming language designed for large language models (LLMs) that offers a unique way of integrating traditional programming with LLM interaction. It allows users to write programs that combine algorithmic logic with LLM calls, enabling model reasoning capabilities within the context of the program. LMQL provides features such as Python syntax integration, rich control-flow options, advanced decoding techniques, powerful constraints via logit masking, runtime optimization, sync and async API support, multi-model compatibility, and extensive applications like JSON decoding and interactive chat interfaces. The tool also offers library integration, flexible tooling, and output streaming options for easy model output handling.
For similar tasks
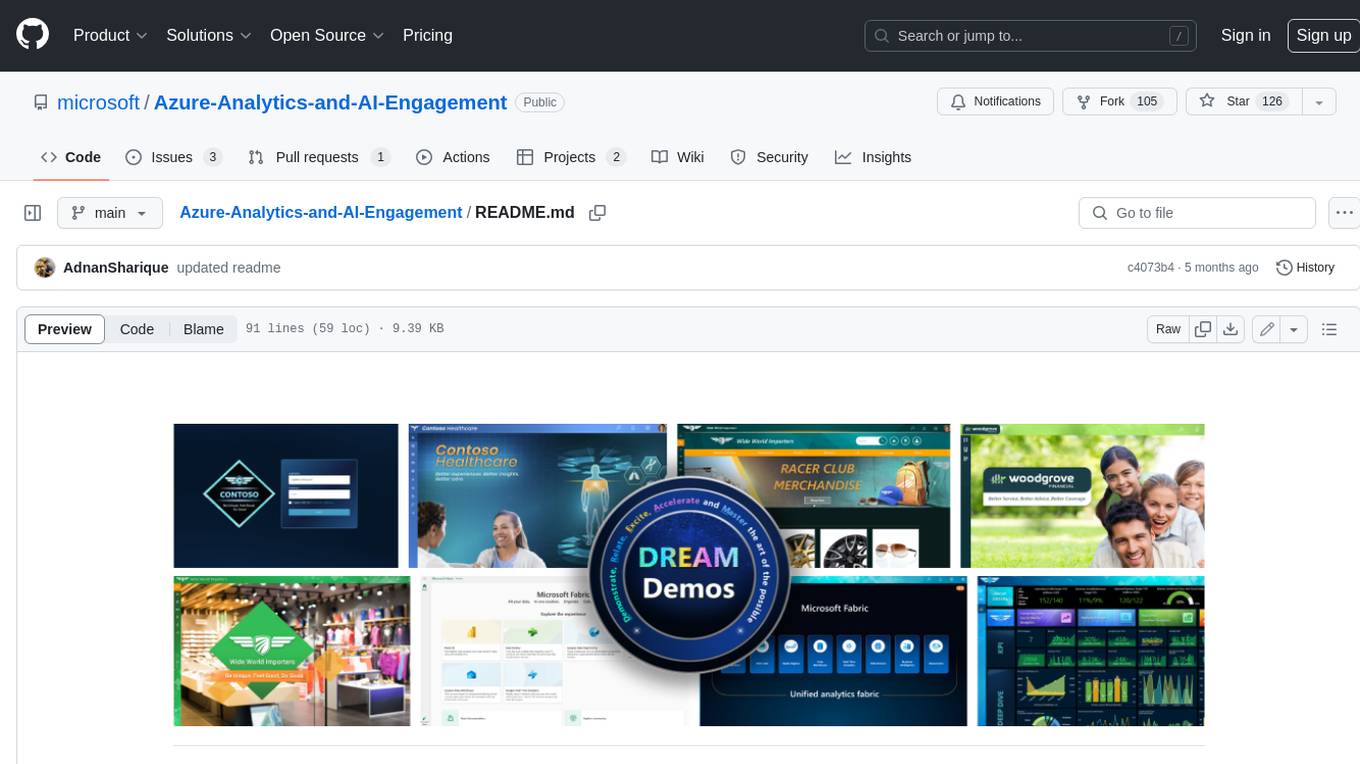
Azure-Analytics-and-AI-Engagement
The Azure-Analytics-and-AI-Engagement repository provides packaged Industry Scenario DREAM Demos with ARM templates (Containing a demo web application, Power BI reports, Synapse resources, AML Notebooks etc.) that can be deployed in a customer’s subscription using the CAPE tool within a matter of few hours. Partners can also deploy DREAM Demos in their own subscriptions using DPoC.
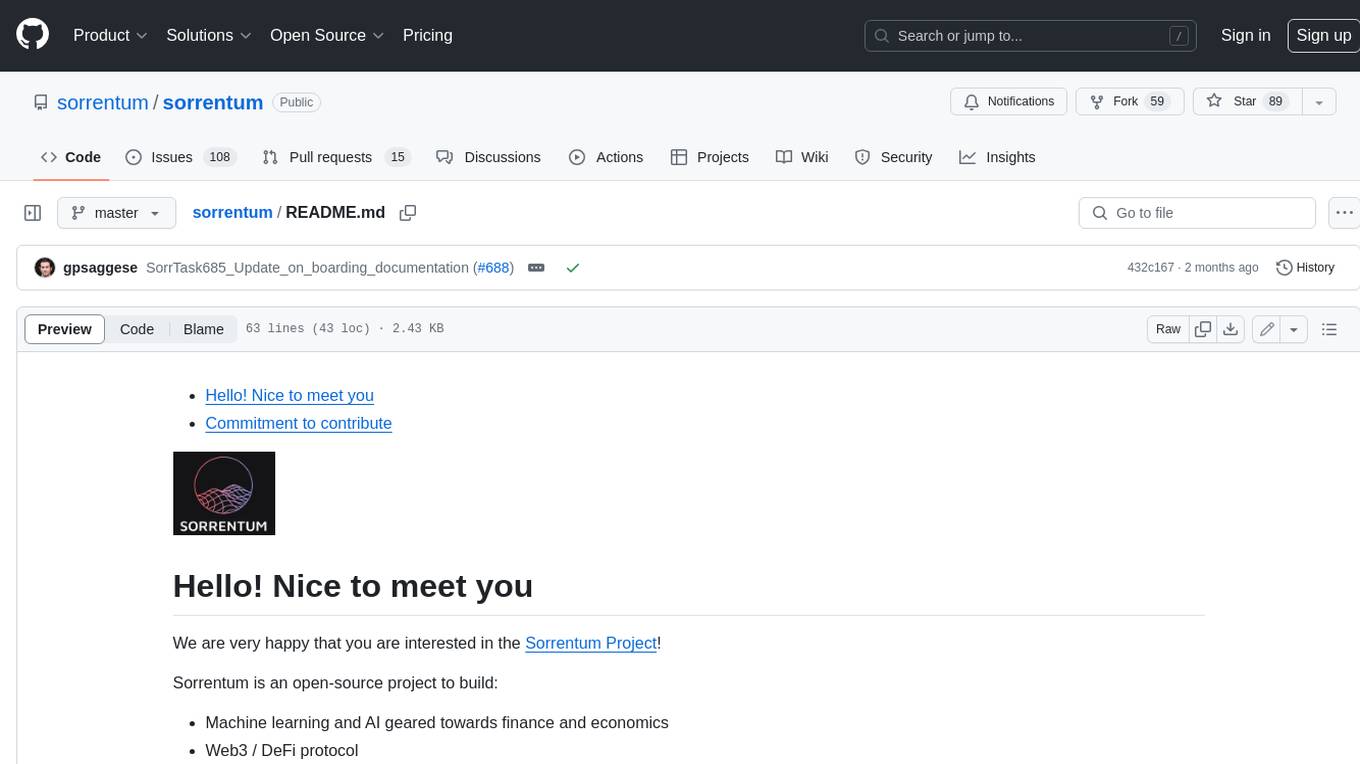
sorrentum
Sorrentum is an open-source project that aims to combine open-source development, startups, and brilliant students to build machine learning, AI, and Web3 / DeFi protocols geared towards finance and economics. The project provides opportunities for internships, research assistantships, and development grants, as well as the chance to work on cutting-edge problems, learn about startups, write academic papers, and get internships and full-time positions at companies working on Sorrentum applications.
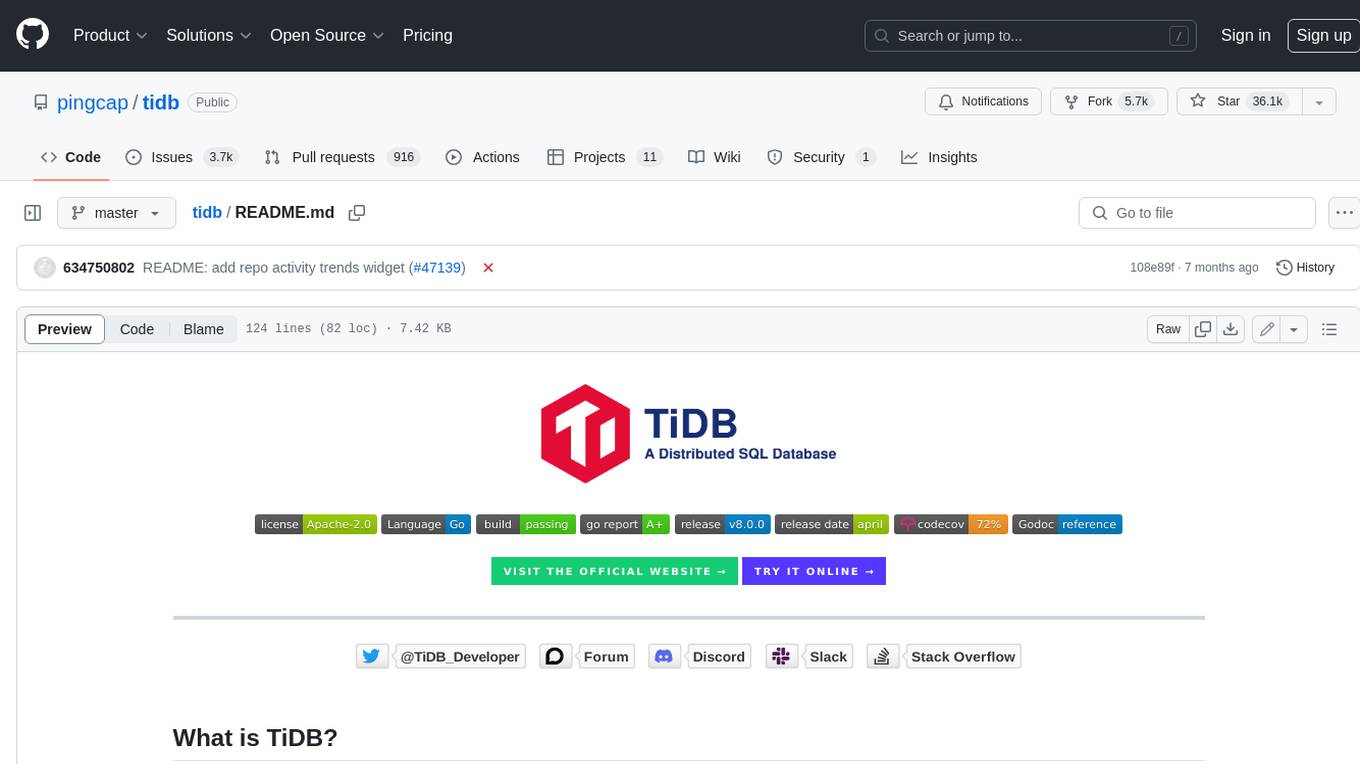
tidb
TiDB is an open-source distributed SQL database that supports Hybrid Transactional and Analytical Processing (HTAP) workloads. It is MySQL compatible and features horizontal scalability, strong consistency, and high availability.
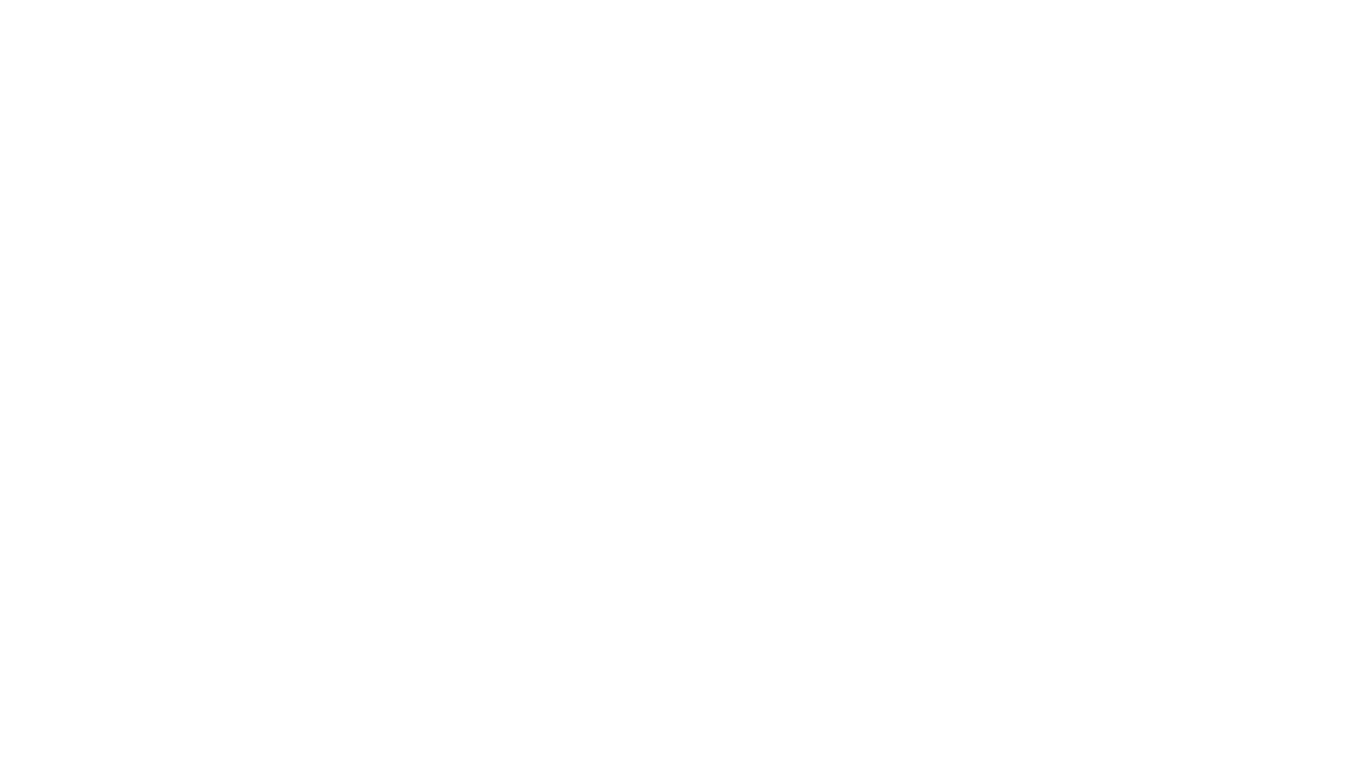
zep-python
Zep is an open-source platform for building and deploying large language model (LLM) applications. It provides a suite of tools and services that make it easy to integrate LLMs into your applications, including chat history memory, embedding, vector search, and data enrichment. Zep is designed to be scalable, reliable, and easy to use, making it a great choice for developers who want to build LLM-powered applications quickly and easily.
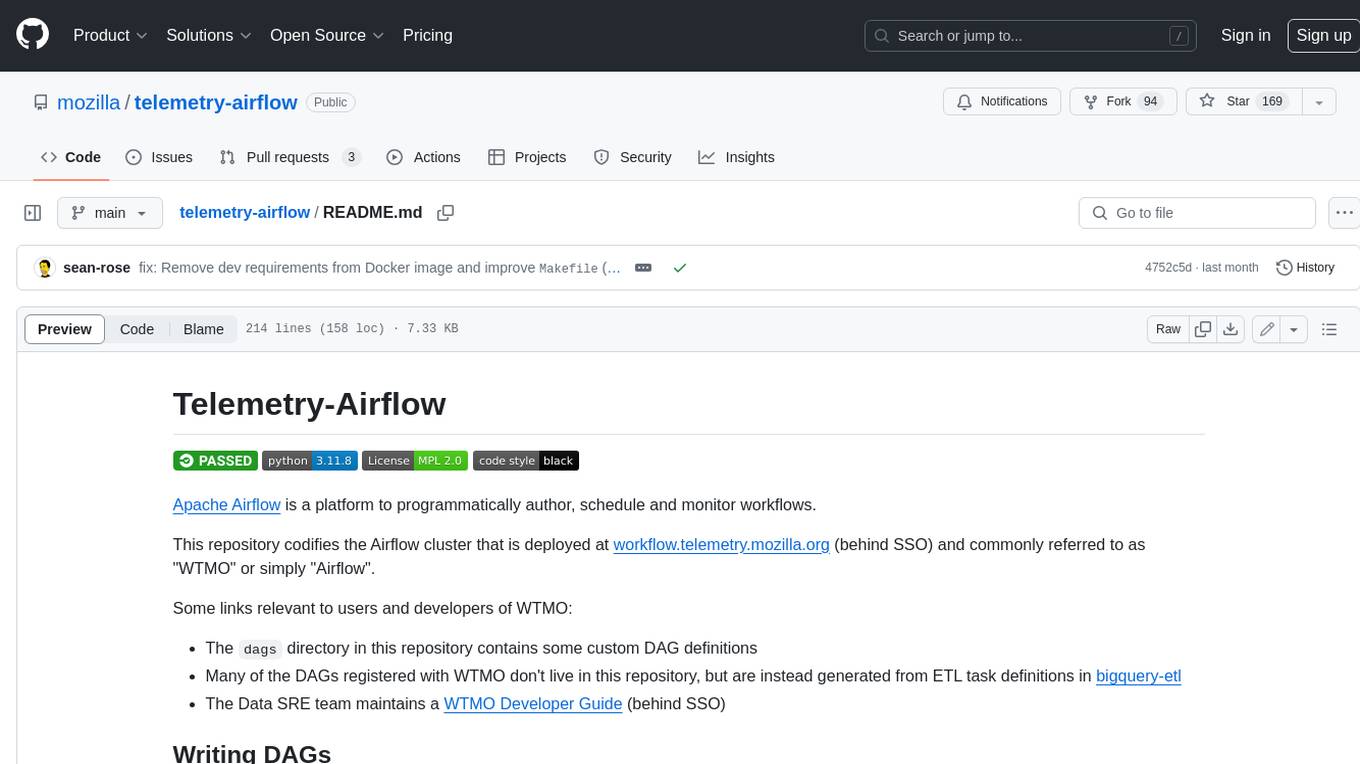
telemetry-airflow
This repository codifies the Airflow cluster that is deployed at workflow.telemetry.mozilla.org (behind SSO) and commonly referred to as "WTMO" or simply "Airflow". Some links relevant to users and developers of WTMO: * The `dags` directory in this repository contains some custom DAG definitions * Many of the DAGs registered with WTMO don't live in this repository, but are instead generated from ETL task definitions in bigquery-etl * The Data SRE team maintains a WTMO Developer Guide (behind SSO)
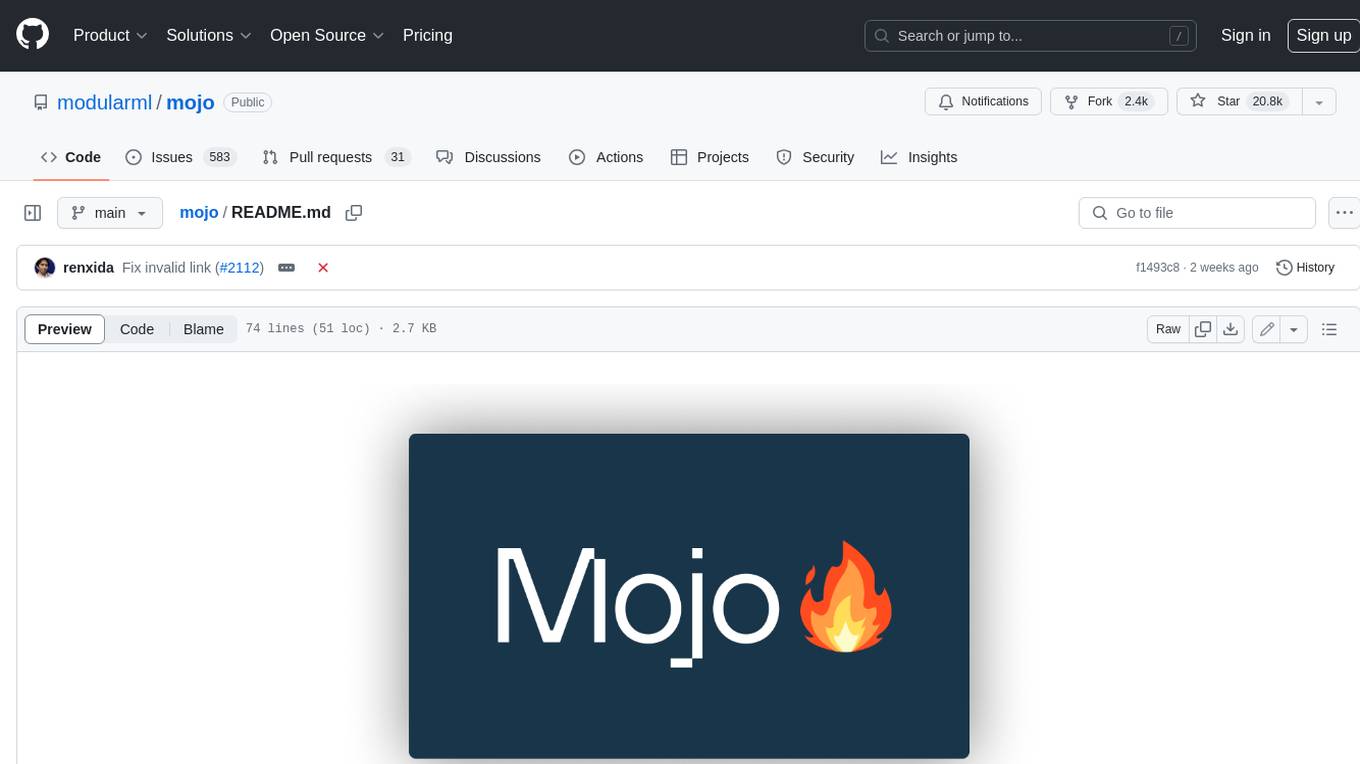
mojo
Mojo is a new programming language that bridges the gap between research and production by combining Python syntax and ecosystem with systems programming and metaprogramming features. Mojo is still young, but it is designed to become a superset of Python over time.
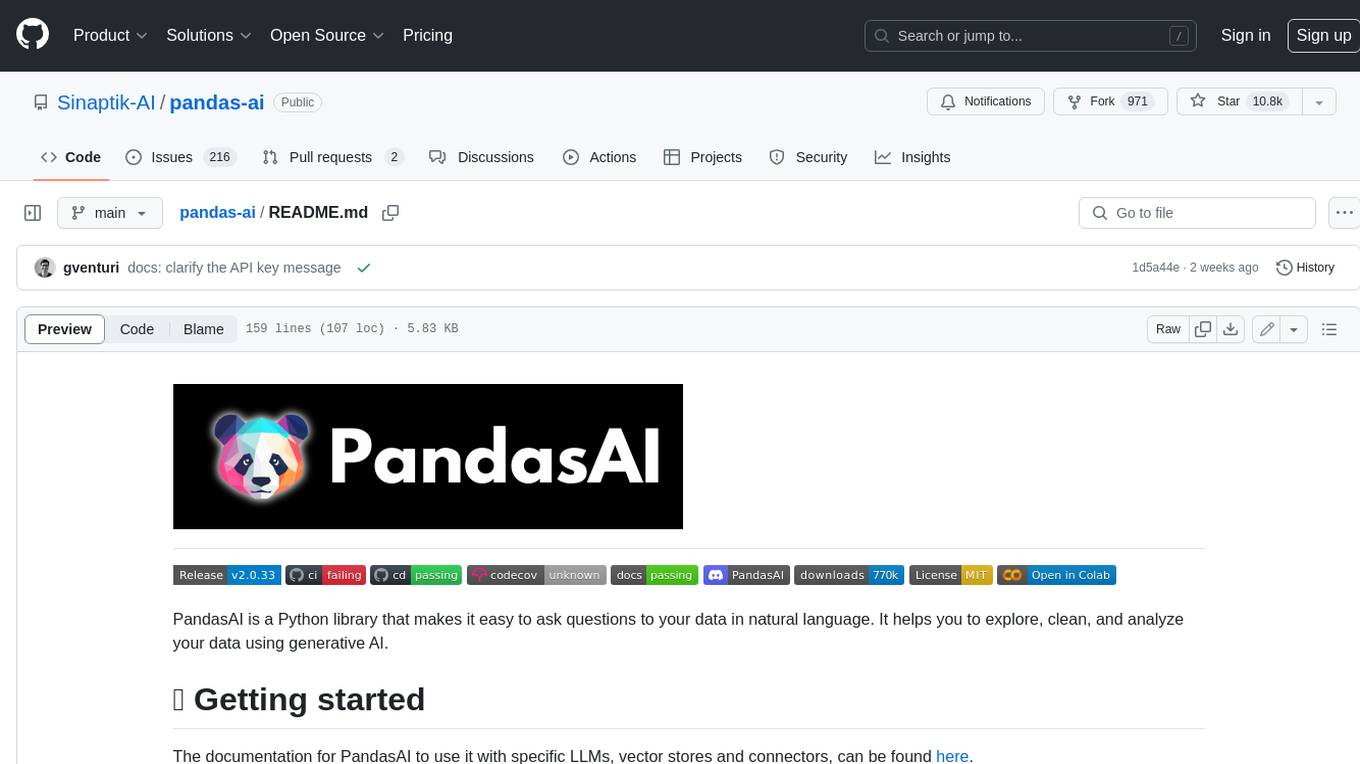
pandas-ai
PandasAI is a Python library that makes it easy to ask questions to your data in natural language. It helps you to explore, clean, and analyze your data using generative AI.
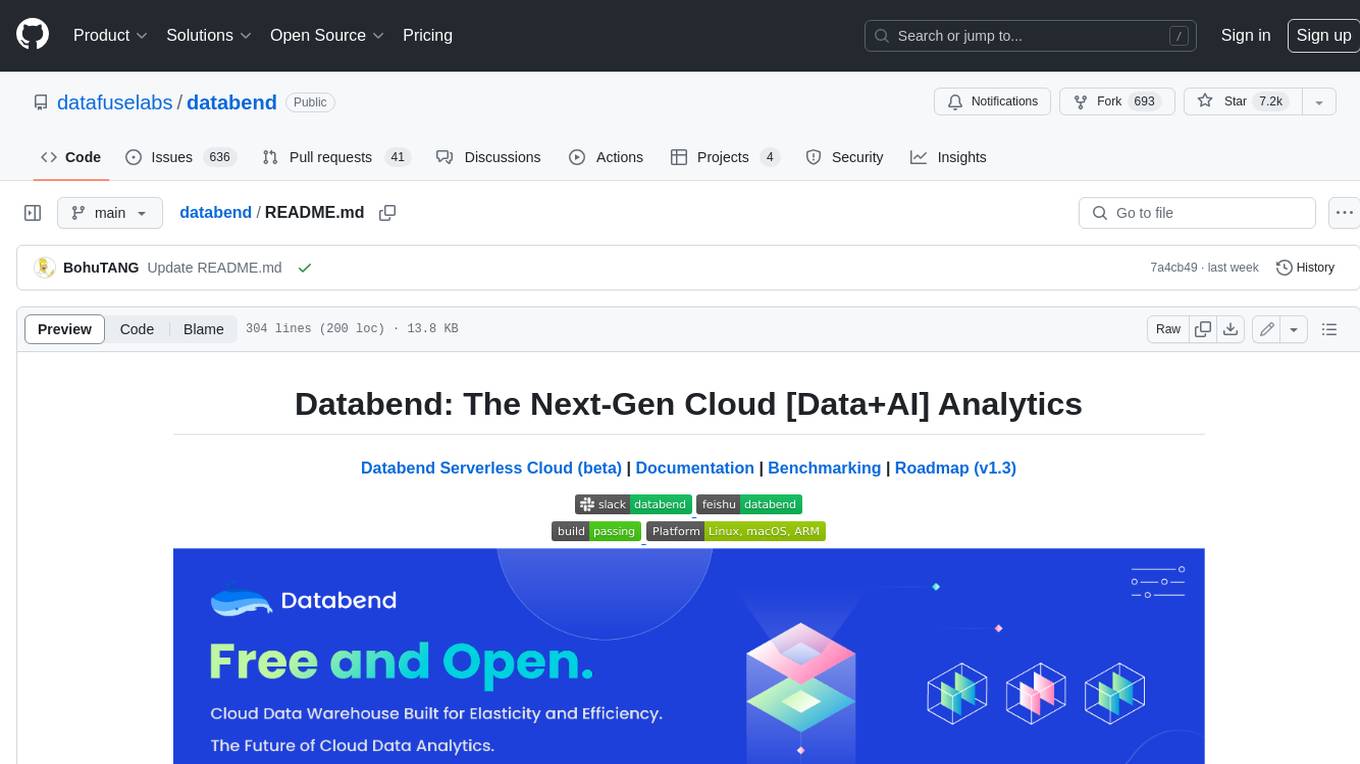
databend
Databend is an open-source cloud data warehouse that serves as a cost-effective alternative to Snowflake. With its focus on fast query execution and data ingestion, it's designed for complex analysis of the world's largest datasets.
For similar jobs
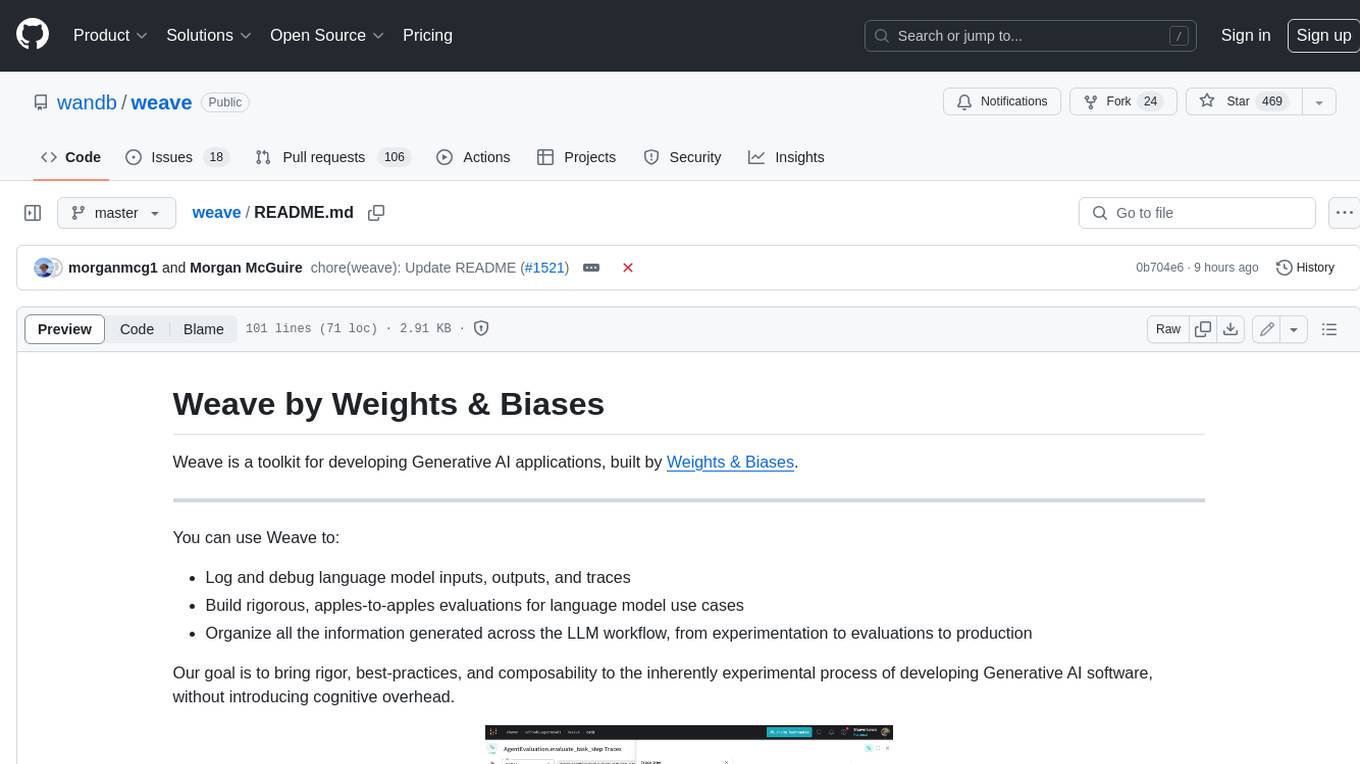
weave
Weave is a toolkit for developing Generative AI applications, built by Weights & Biases. With Weave, you can log and debug language model inputs, outputs, and traces; build rigorous, apples-to-apples evaluations for language model use cases; and organize all the information generated across the LLM workflow, from experimentation to evaluations to production. Weave aims to bring rigor, best-practices, and composability to the inherently experimental process of developing Generative AI software, without introducing cognitive overhead.
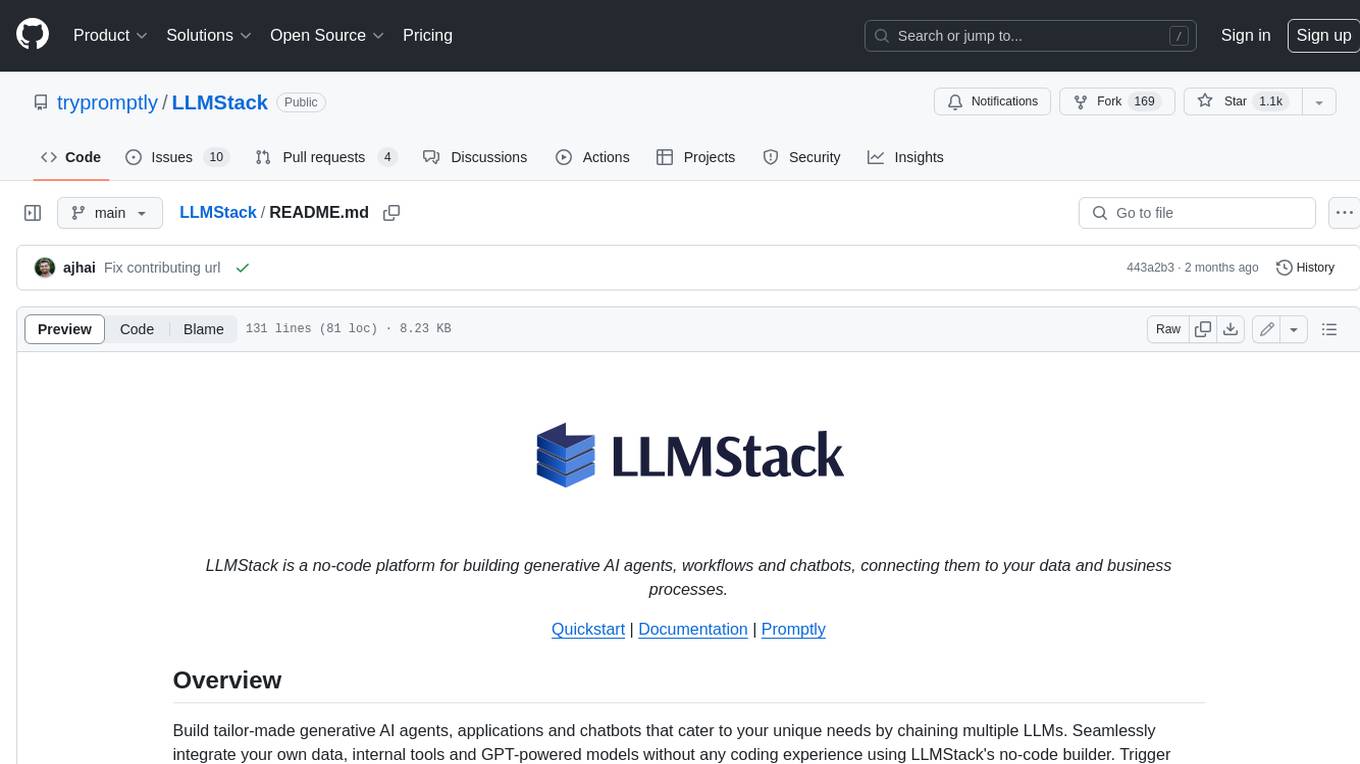
LLMStack
LLMStack is a no-code platform for building generative AI agents, workflows, and chatbots. It allows users to connect their own data, internal tools, and GPT-powered models without any coding experience. LLMStack can be deployed to the cloud or on-premise and can be accessed via HTTP API or triggered from Slack or Discord.
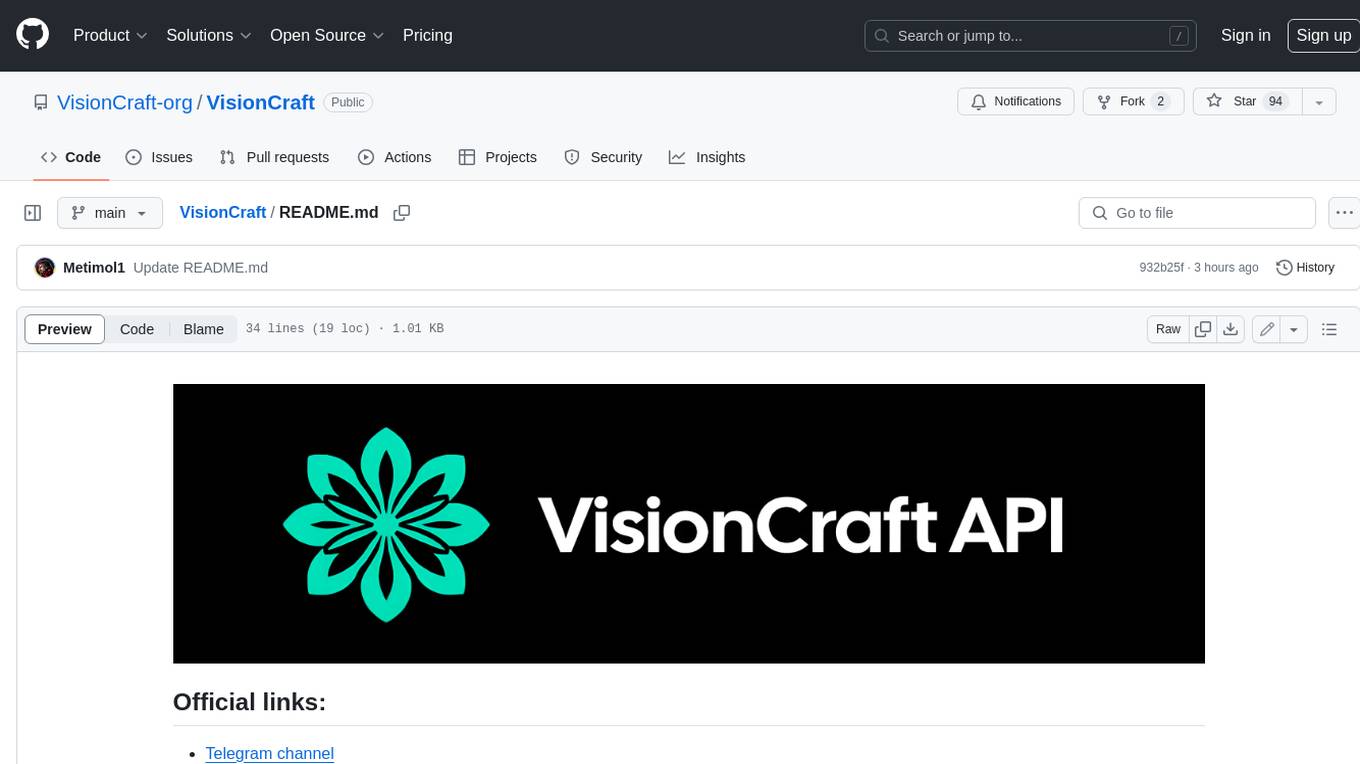
VisionCraft
The VisionCraft API is a free API for using over 100 different AI models. From images to sound.
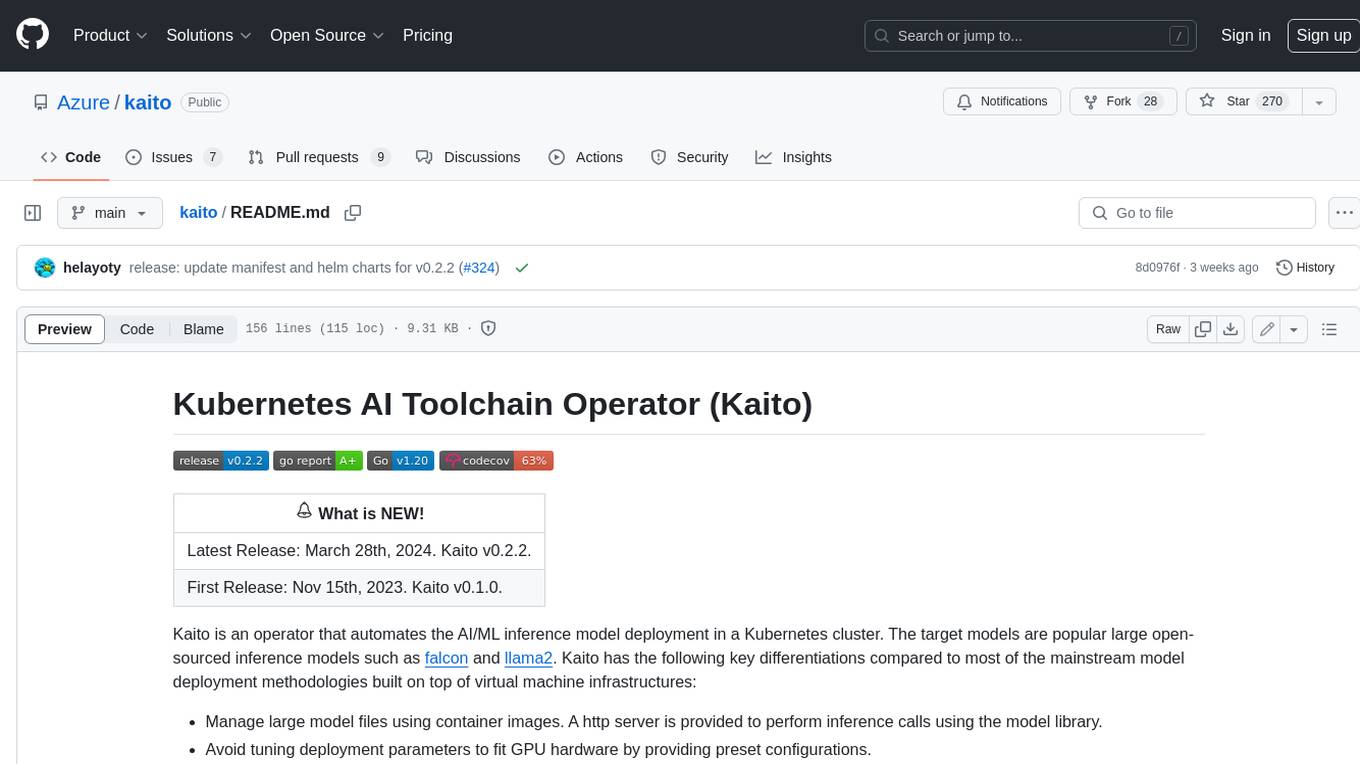
kaito
Kaito is an operator that automates the AI/ML inference model deployment in a Kubernetes cluster. It manages large model files using container images, avoids tuning deployment parameters to fit GPU hardware by providing preset configurations, auto-provisions GPU nodes based on model requirements, and hosts large model images in the public Microsoft Container Registry (MCR) if the license allows. Using Kaito, the workflow of onboarding large AI inference models in Kubernetes is largely simplified.
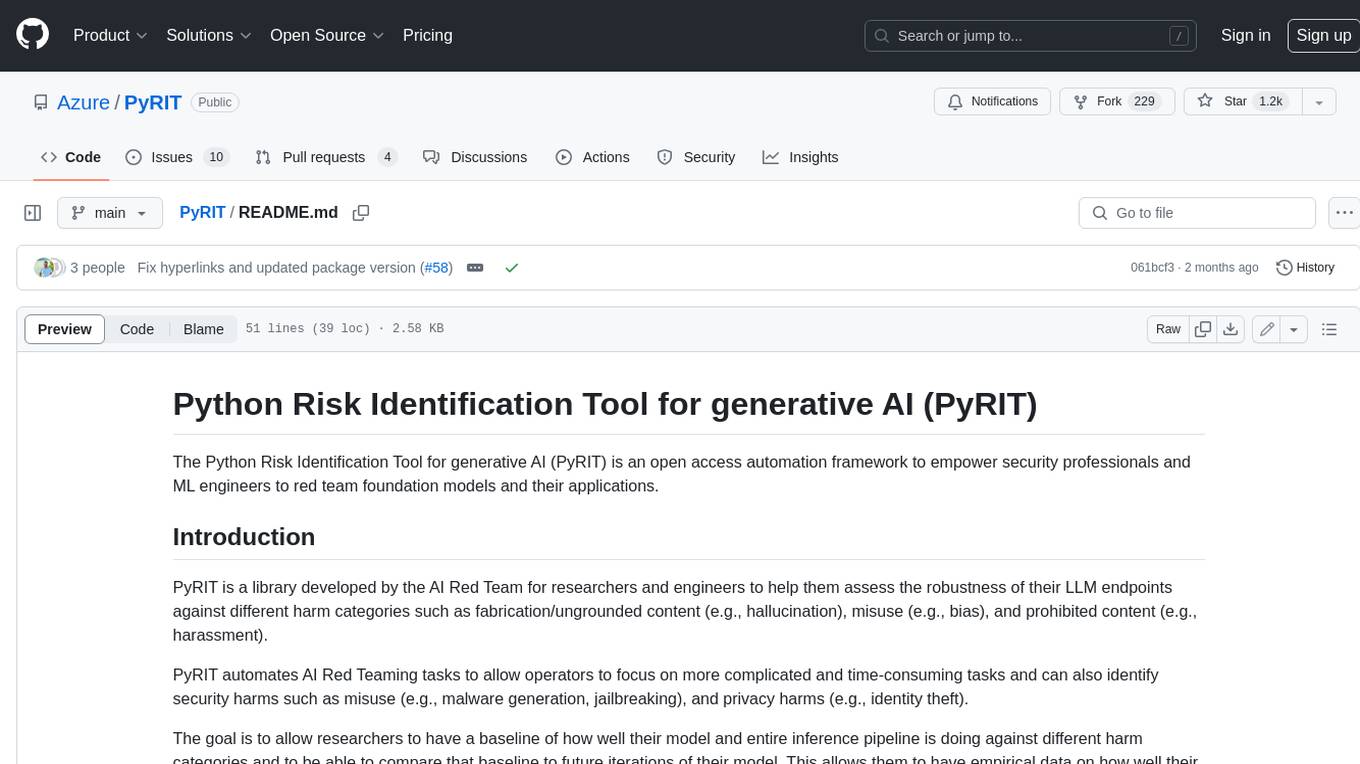
PyRIT
PyRIT is an open access automation framework designed to empower security professionals and ML engineers to red team foundation models and their applications. It automates AI Red Teaming tasks to allow operators to focus on more complicated and time-consuming tasks and can also identify security harms such as misuse (e.g., malware generation, jailbreaking), and privacy harms (e.g., identity theft). The goal is to allow researchers to have a baseline of how well their model and entire inference pipeline is doing against different harm categories and to be able to compare that baseline to future iterations of their model. This allows them to have empirical data on how well their model is doing today, and detect any degradation of performance based on future improvements.
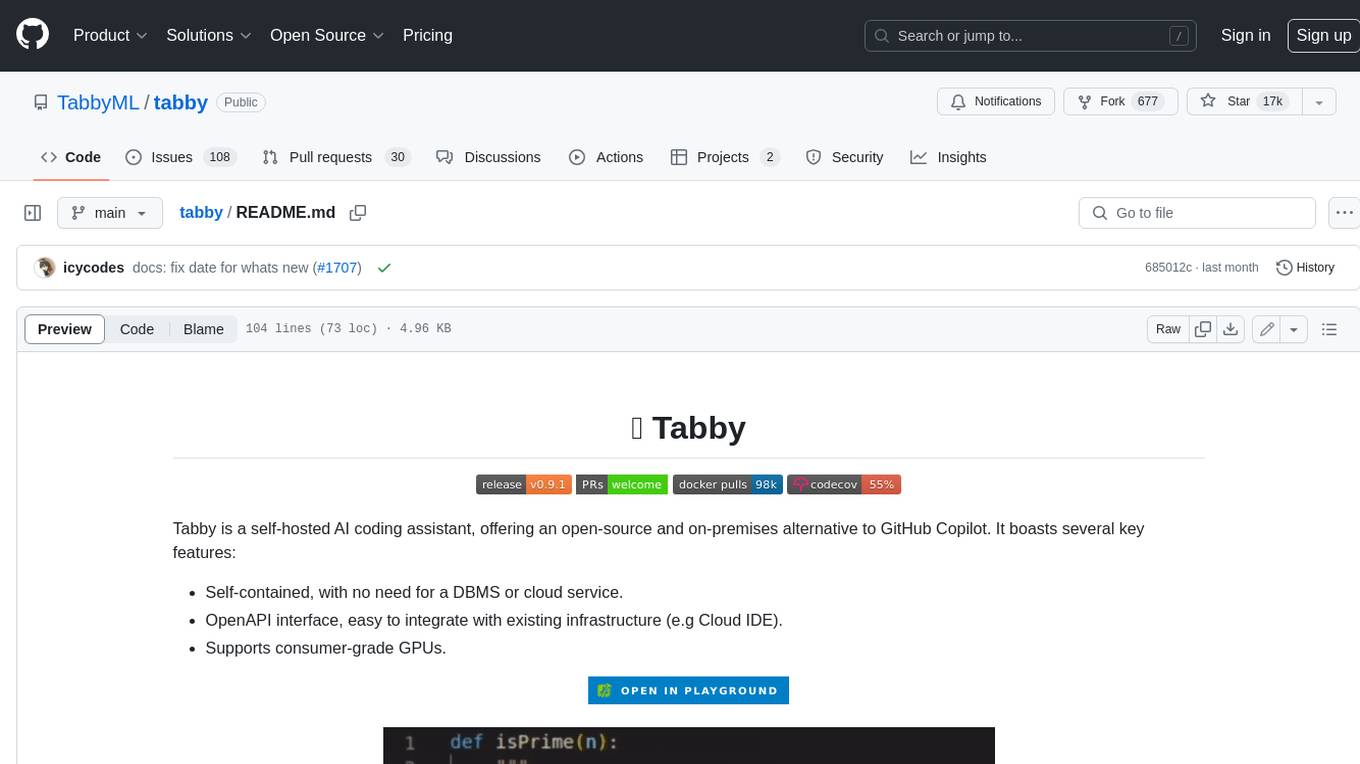
tabby
Tabby is a self-hosted AI coding assistant, offering an open-source and on-premises alternative to GitHub Copilot. It boasts several key features: * Self-contained, with no need for a DBMS or cloud service. * OpenAPI interface, easy to integrate with existing infrastructure (e.g Cloud IDE). * Supports consumer-grade GPUs.
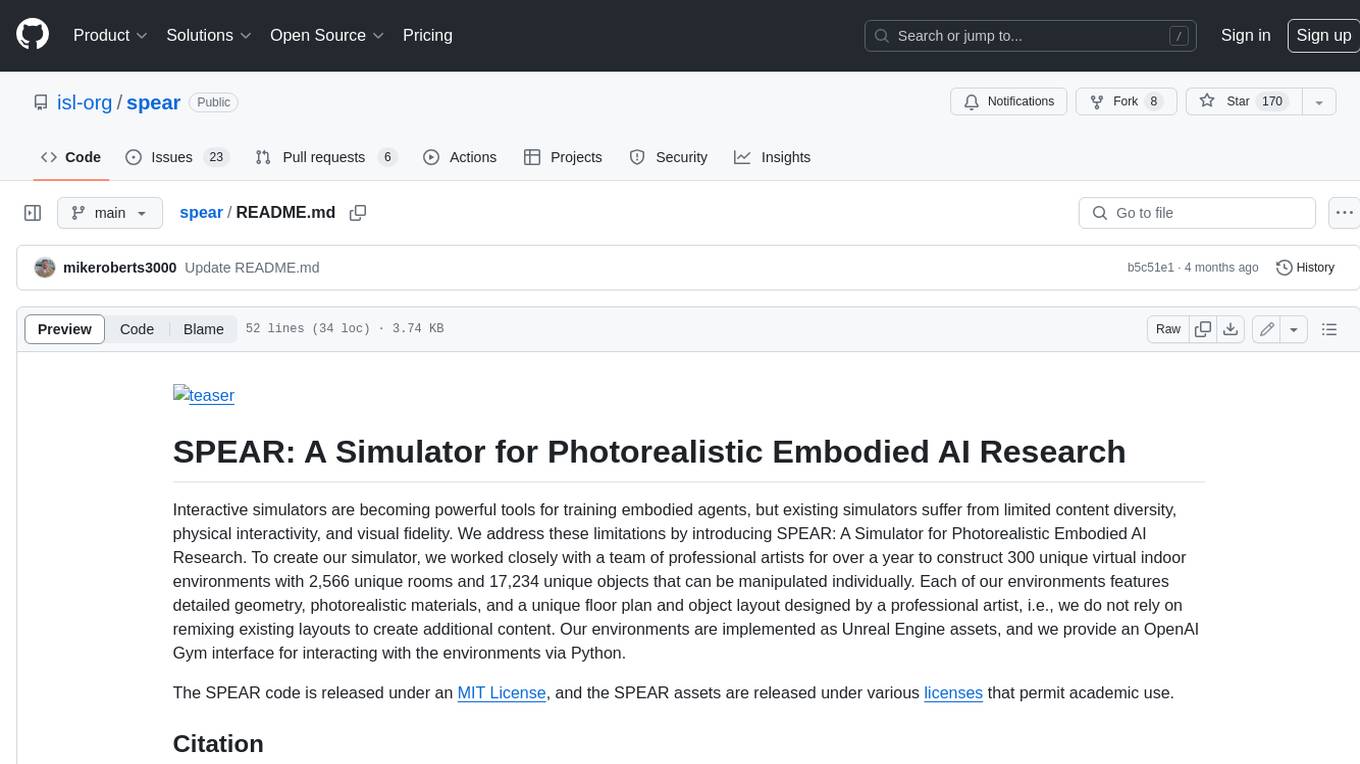
spear
SPEAR (Simulator for Photorealistic Embodied AI Research) is a powerful tool for training embodied agents. It features 300 unique virtual indoor environments with 2,566 unique rooms and 17,234 unique objects that can be manipulated individually. Each environment is designed by a professional artist and features detailed geometry, photorealistic materials, and a unique floor plan and object layout. SPEAR is implemented as Unreal Engine assets and provides an OpenAI Gym interface for interacting with the environments via Python.
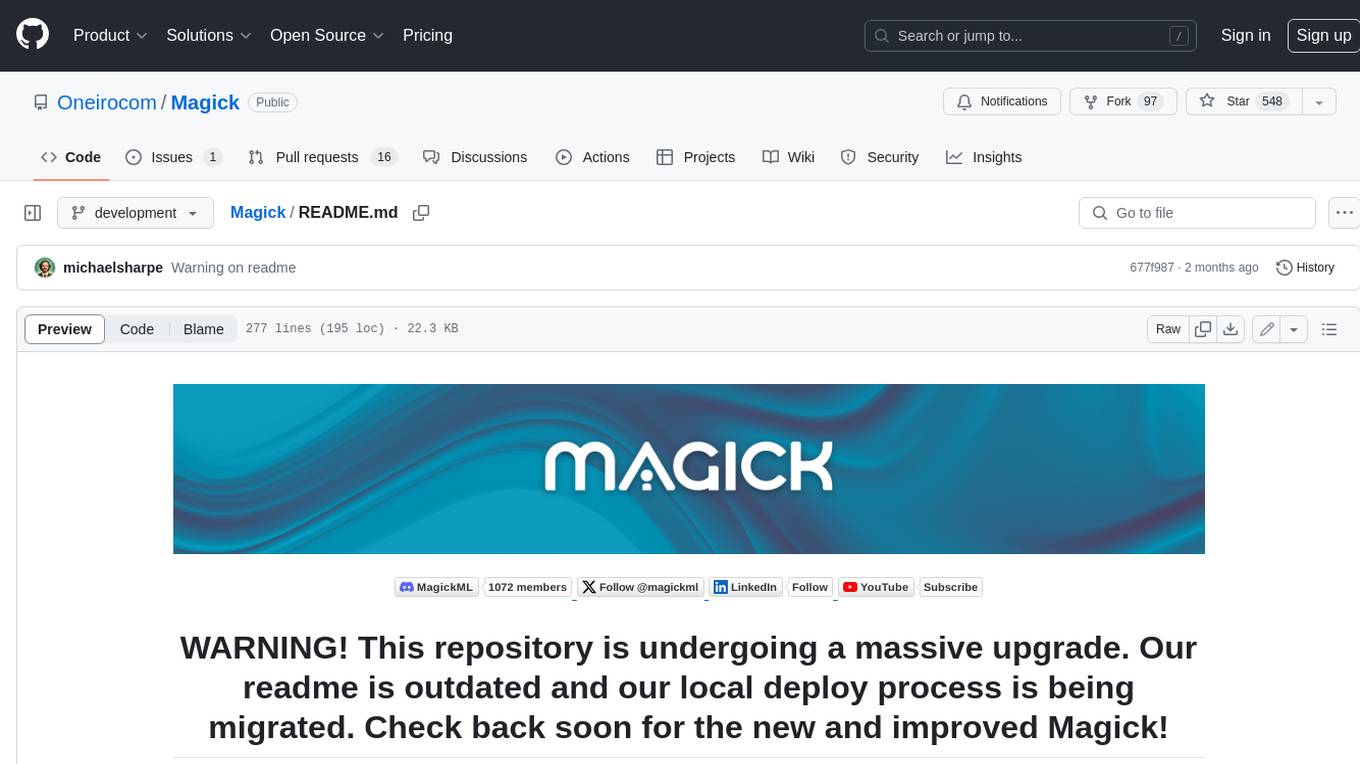
Magick
Magick is a groundbreaking visual AIDE (Artificial Intelligence Development Environment) for no-code data pipelines and multimodal agents. Magick can connect to other services and comes with nodes and templates well-suited for intelligent agents, chatbots, complex reasoning systems and realistic characters.