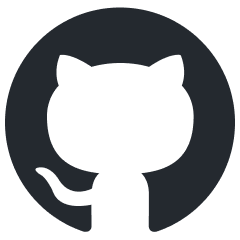
falkon
Large-scale, multi-GPU capable, kernel solver
Stars: 172
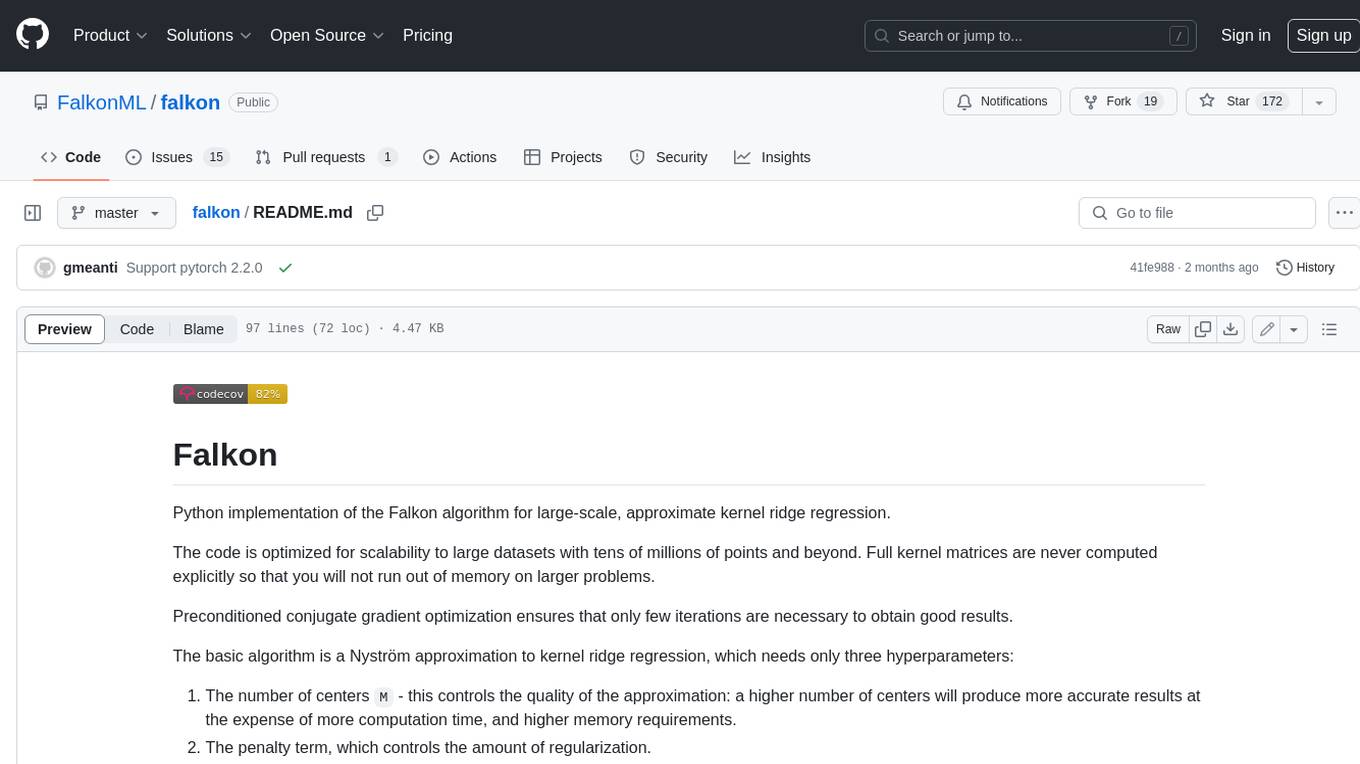
Falkon is a Python implementation of the Falkon algorithm for large-scale, approximate kernel ridge regression. The code is optimized for scalability to large datasets with tens of millions of points and beyond. Full kernel matrices are never computed explicitly so that you will not run out of memory on larger problems. Preconditioned conjugate gradient optimization ensures that only few iterations are necessary to obtain good results. The basic algorithm is a Nyström approximation to kernel ridge regression, which needs only three hyperparameters: 1. The number of centers M - this controls the quality of the approximation: a higher number of centers will produce more accurate results at the expense of more computation time, and higher memory requirements. 2. The penalty term, which controls the amount of regularization. 3. The kernel function. A good default is always the Gaussian (RBF) kernel (`falkon.kernels.GaussianKernel`).
README:
Python implementation of the Falkon algorithm for large-scale, approximate kernel ridge regression.
The code is optimized for scalability to large datasets with tens of millions of points and beyond. Full kernel matrices are never computed explicitly so that you will not run out of memory on larger problems.
Preconditioned conjugate gradient optimization ensures that only few iterations are necessary to obtain good results.
The basic algorithm is a Nyström approximation to kernel ridge regression, which needs only three hyperparameters:
- The number of centers
M
- this controls the quality of the approximation: a higher number of centers will produce more accurate results at the expense of more computation time, and higher memory requirements. - The penalty term, which controls the amount of regularization.
- The kernel function. A good default is always the Gaussian (RBF) kernel
(
falkon.kernels.GaussianKernel
).
For more information about the algorithm and the optimized solver, you can download our paper: Kernel methods through the roof: handling billions of points efficiently
The API is sklearn-like, so that Falkon should be easy to integrate in your existing code. Several worked-through examples are provided in the docs, and for more advanced usage, extensive documentation is available at https://falkonml.github.io/falkon/.
If you find a bug, please open a new issue on GitHub!
Dependencies:
- Please install PyTorch first.
-
cmake
and a C++ compiler are also needed for KeOps acceleration (optional but strongly recommended).
To install from source, you can run
pip install --no-build-isolation git+https://github.com/FalkonML/falkon.git
We alternatively provide pre-built pip wheels for the following combinations of PyTorch and CUDA:
Linux | cu117 |
cu118 |
cu121 |
---|---|---|---|
torch 2.0.0 | ✅ | ✅ | |
torch 2.1.0 | ✅ | ✅ | |
torch 2.2.0 | ✅ | ✅ |
For other combinations, and previous versions of Falkon, please check here for a list of supported wheels.
To install a wheel for a specific PyTorch + CUDA combination, you can run
# e.g., torch 2.2.0 + CUDA 12.1
pip install falkon -f https://falkon.dibris.unige.it/torch-2.2.0_cu121.html
Similarly for CPU-only packages
# e.g., torch 2.1.0 + cpu
pip install falkon -f https://falkon.dibris.unige.it/torch-2.1.0_cpu.html
More detailed installation instructions are available in the documentation.
New! We added a new module for automatic hyperparameter optimization.
The falkon.hopt
module contains the implementation
of several objective functions which can be minimized with respect to Falkon's hyperparameters (notably the penalty,
the kernel parameters and the centers themselves).
For more details check out our paper: Efficient Hyperparameter Tuning for Large Scale Kernel Ridge Regression, and the automatic hyperparameter tuning notebook.
If you find this library useful for your work, please cite the following publications:
@inproceedings{falkonlibrary2020,
title = {Kernel methods through the roof: handling billions of points efficiently},
author = {Meanti, Giacomo and Carratino, Luigi and Rosasco, Lorenzo and Rudi, Alessandro},
year = {2020},
booktitle = {Advances in Neural Information Processing Systems 32}
}
@inproceedings{falkonhopt2022,
title = {Efficient Hyperparameter Tuning for Large Scale Kernel Ridge Regression},
author = {Meanti, Giacomo and Carratino, Luigi and De Vito, Ernesto and Rosasco, Lorenzo},
year = {2022},
booktitle = {Proceedings of The 25th International Conference on Artificial Intelligence and Statistics}
}
For Tasks:
Click tags to check more tools for each tasksFor Jobs:
Alternative AI tools for falkon
Similar Open Source Tools
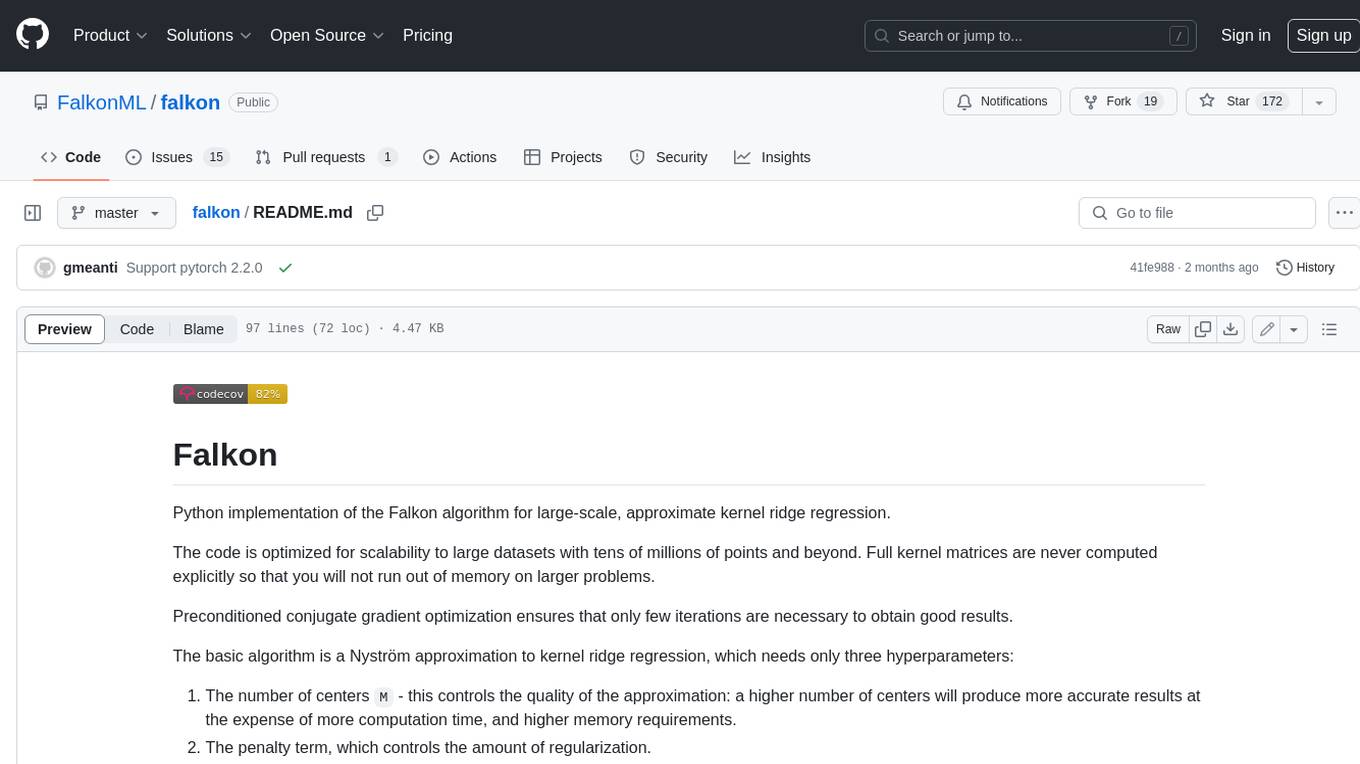
falkon
Falkon is a Python implementation of the Falkon algorithm for large-scale, approximate kernel ridge regression. The code is optimized for scalability to large datasets with tens of millions of points and beyond. Full kernel matrices are never computed explicitly so that you will not run out of memory on larger problems. Preconditioned conjugate gradient optimization ensures that only few iterations are necessary to obtain good results. The basic algorithm is a Nyström approximation to kernel ridge regression, which needs only three hyperparameters: 1. The number of centers M - this controls the quality of the approximation: a higher number of centers will produce more accurate results at the expense of more computation time, and higher memory requirements. 2. The penalty term, which controls the amount of regularization. 3. The kernel function. A good default is always the Gaussian (RBF) kernel (`falkon.kernels.GaussianKernel`).
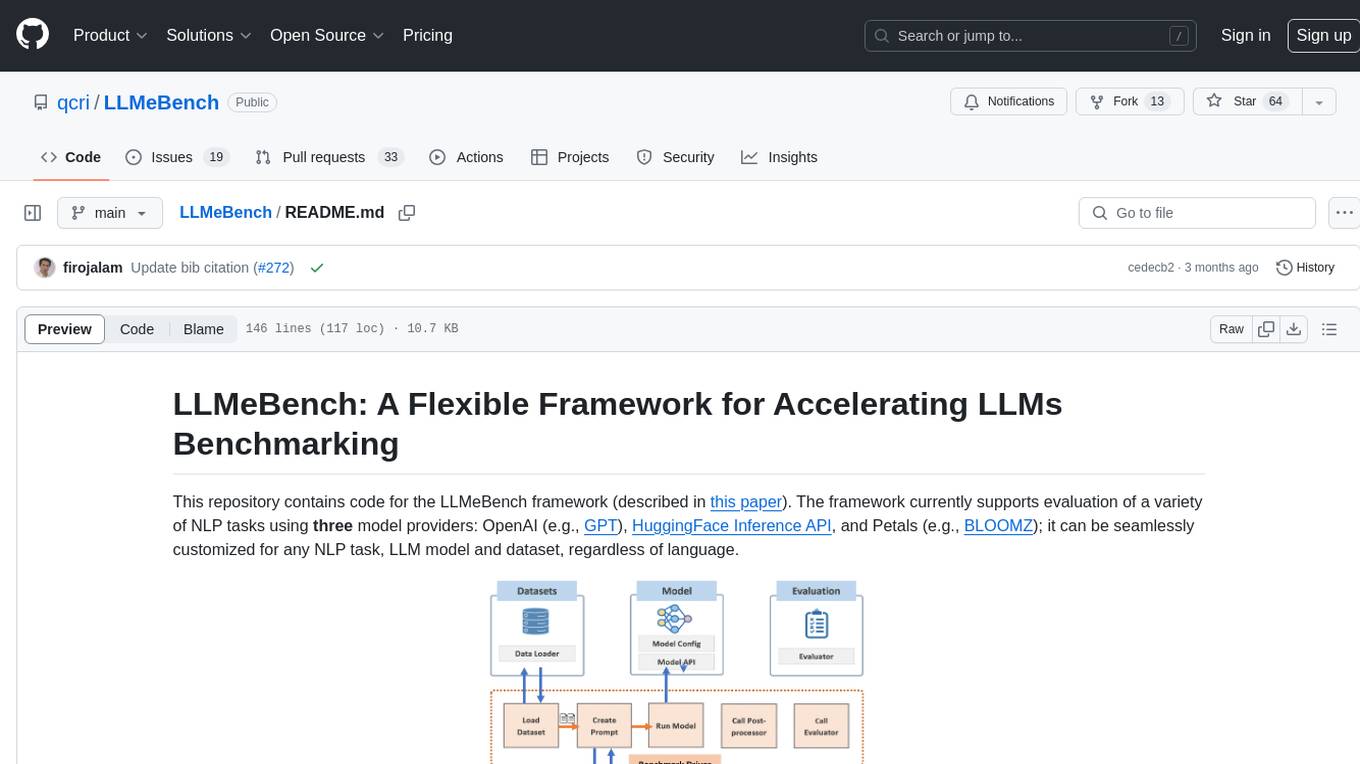
LLMeBench
LLMeBench is a flexible framework designed for accelerating benchmarking of Large Language Models (LLMs) in the field of Natural Language Processing (NLP). It supports evaluation of various NLP tasks using model providers like OpenAI, HuggingFace Inference API, and Petals. The framework is customizable for different NLP tasks, LLM models, and datasets across multiple languages. It features extensive caching capabilities, supports zero- and few-shot learning paradigms, and allows on-the-fly dataset download and caching. LLMeBench is open-source and continuously expanding to support new models accessible through APIs.
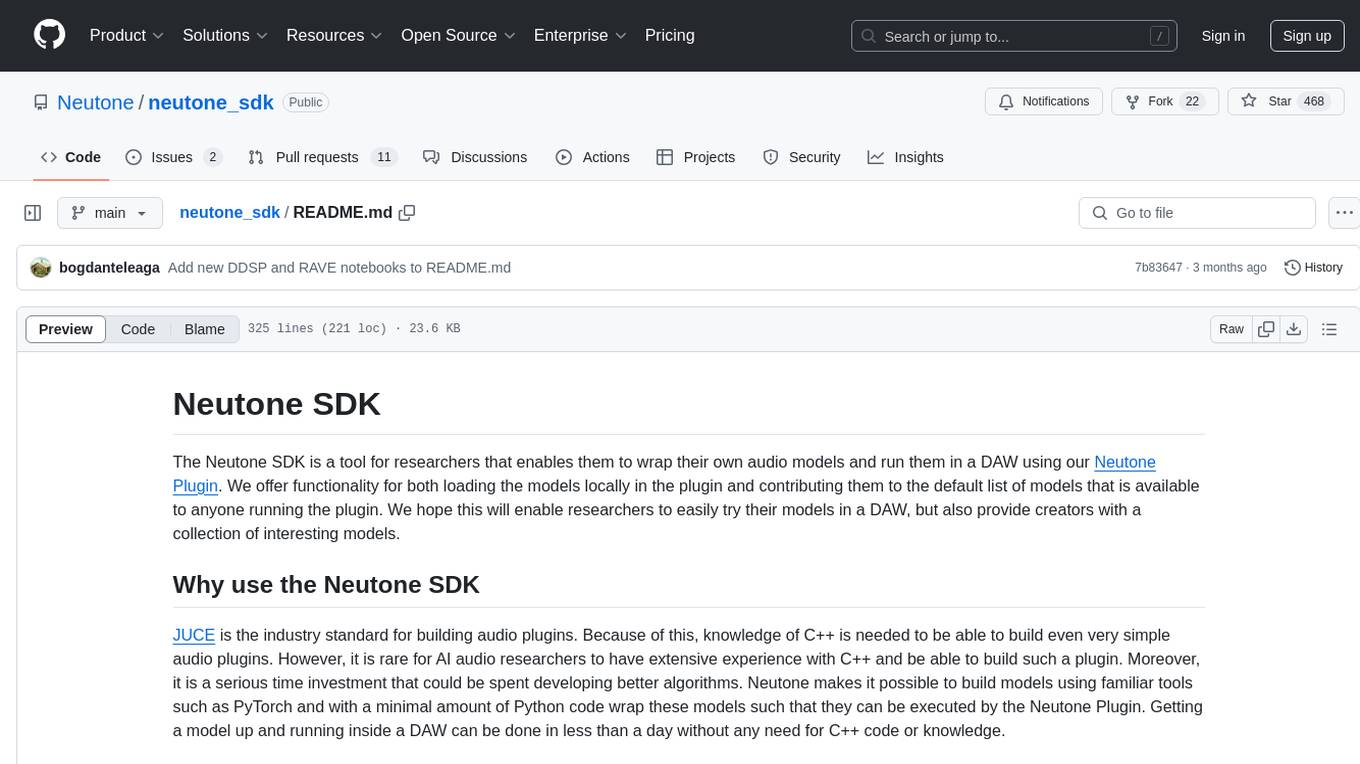
neutone_sdk
The Neutone SDK is a tool designed for researchers to wrap their own audio models and run them in a DAW using the Neutone Plugin. It simplifies the process by allowing models to be built using PyTorch and minimal Python code, eliminating the need for extensive C++ knowledge. The SDK provides support for buffering inputs and outputs, sample rate conversion, and profiling tools for model performance testing. It also offers examples, notebooks, and a submission process for sharing models with the community.
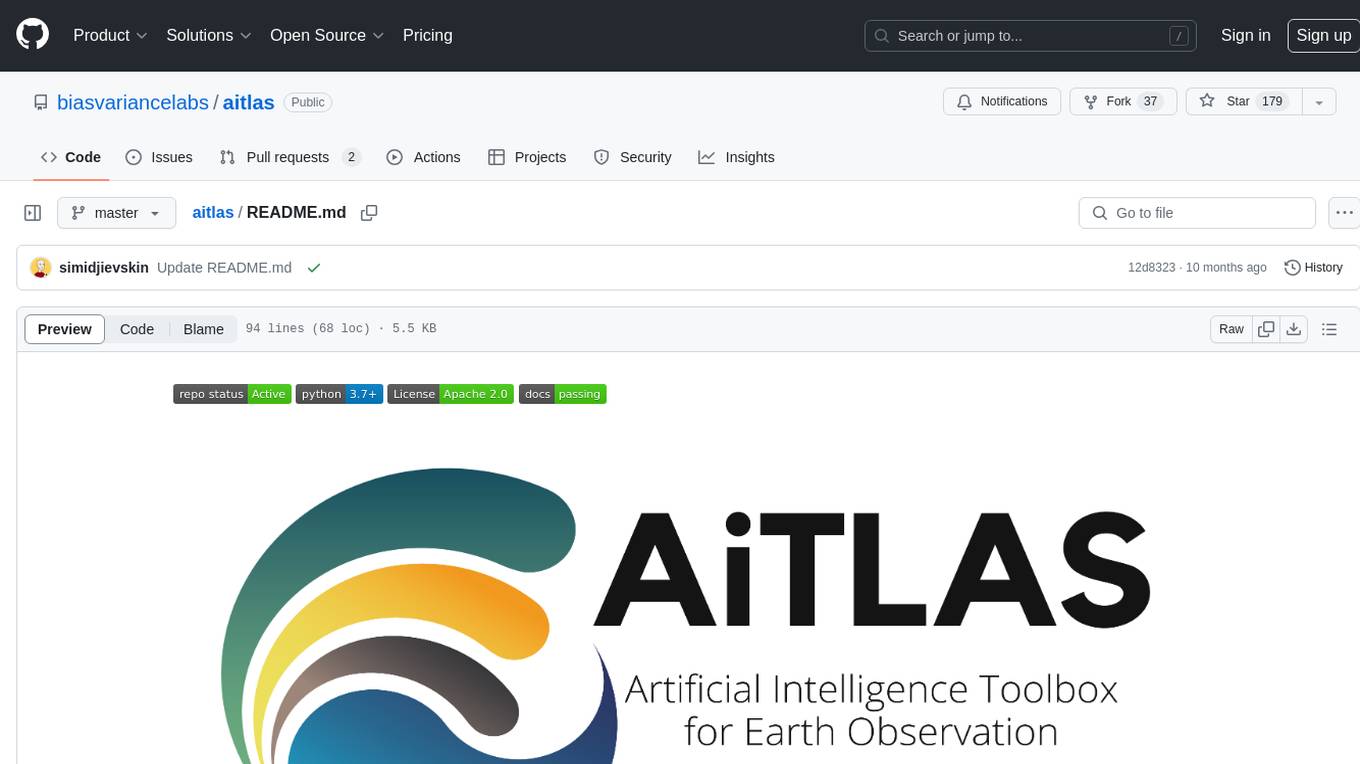
aitlas
The AiTLAS toolbox (Artificial Intelligence Toolbox for Earth Observation) includes state-of-the-art machine learning methods for exploratory and predictive analysis of satellite imagery as well as a repository of AI-ready Earth Observation (EO) datasets. It can be easily applied for a variety of Earth Observation tasks, such as land use and cover classification, crop type prediction, localization of specific objects (semantic segmentation), etc. The main goal of AiTLAS is to facilitate better usability and adoption of novel AI methods (and models) by EO experts, while offering easy access and standardized format of EO datasets to AI experts which allows benchmarking of various existing and novel AI methods tailored for EO data.
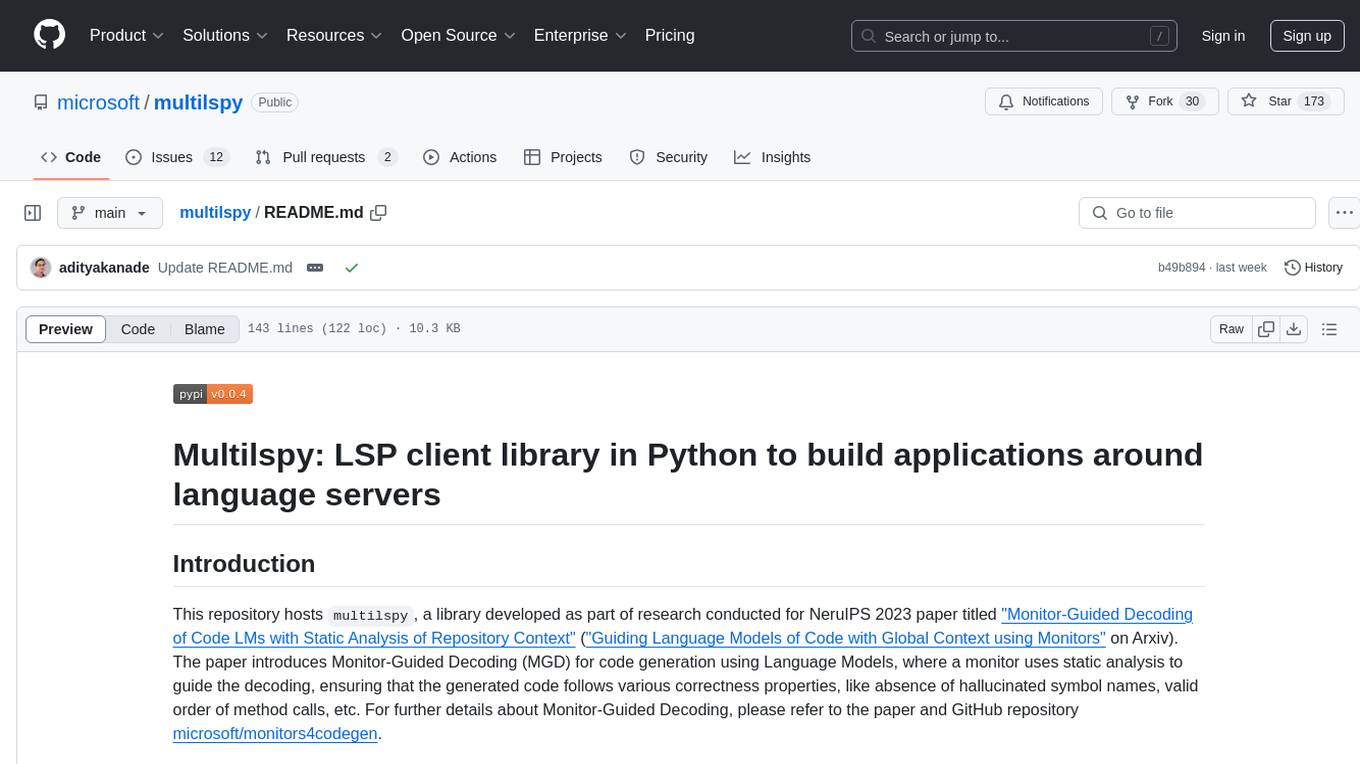
multilspy
Multilspy is a Python library developed for research purposes to facilitate the creation of language server clients for querying and obtaining results of static analyses from various language servers. It simplifies the process by handling server setup, communication, and configuration parameters, providing a common interface for different languages. The library supports features like finding function/class definitions, callers, completions, hover information, and document symbols. It is designed to work with AI systems like Large Language Models (LLMs) for tasks such as Monitor-Guided Decoding to ensure code generation correctness and boost compilability.
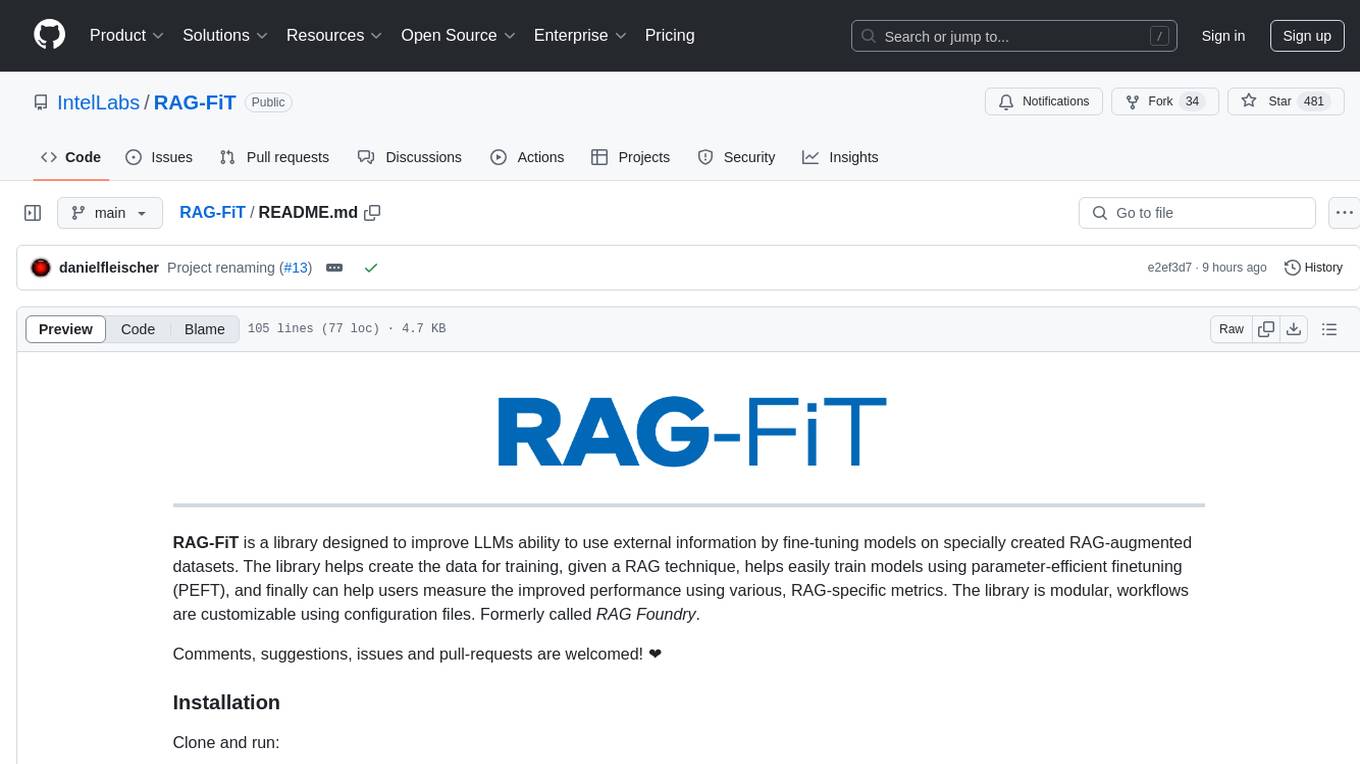
RAG-FiT
RAG-FiT is a library designed to improve Language Models' ability to use external information by fine-tuning models on specially created RAG-augmented datasets. The library assists in creating training data, training models using parameter-efficient finetuning (PEFT), and evaluating performance using RAG-specific metrics. It is modular, customizable via configuration files, and facilitates fast prototyping and experimentation with various RAG settings and configurations.
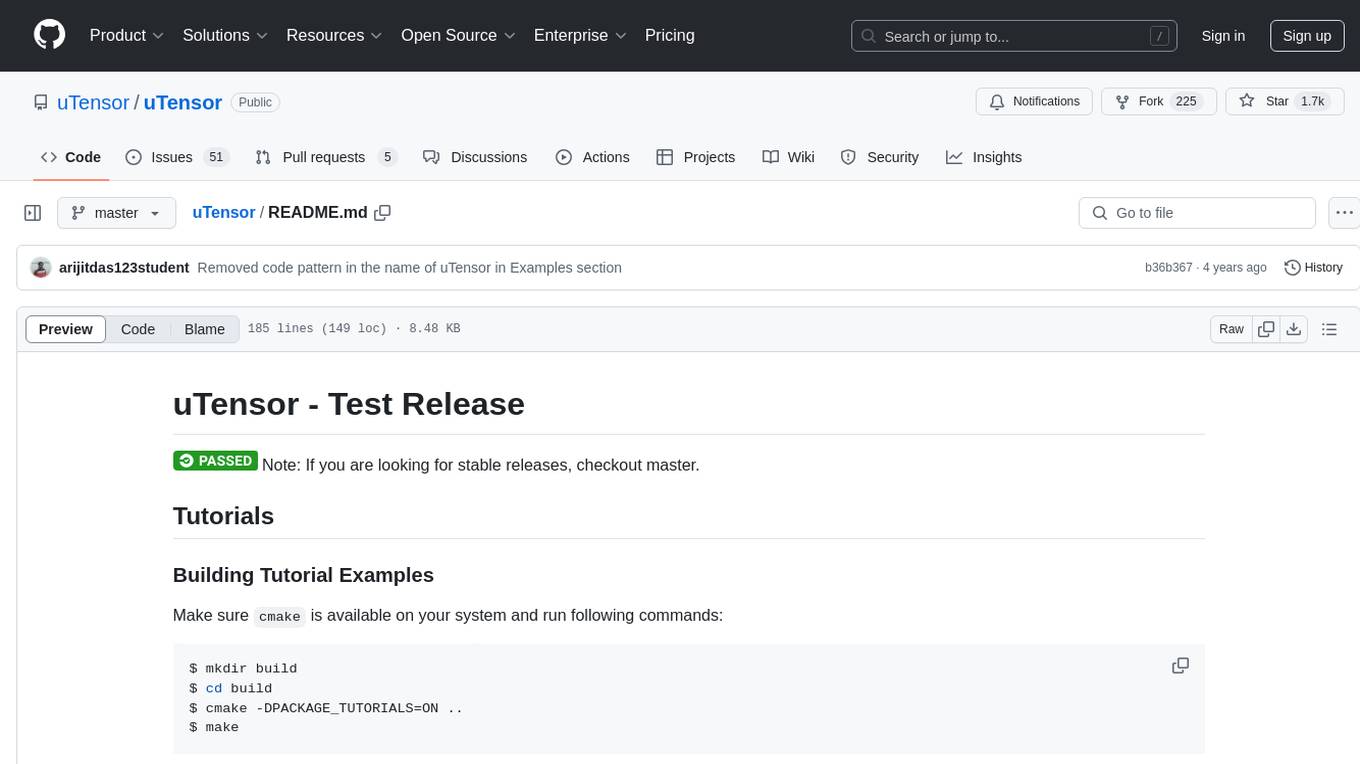
uTensor
uTensor is an extremely light-weight machine learning inference framework built on Tensorflow and optimized for Arm targets. It consists of a runtime library and an offline tool that handles most of the model translation work. The core runtime is only ~2KB. The workflow involves constructing and training a model in Tensorflow, then using uTensor to produce C++ code for inferencing. The runtime ensures system safety, guarantees RAM usage, and focuses on clear, concise, and debuggable code. The high-level API simplifies tensor handling and operator execution for embedded systems.
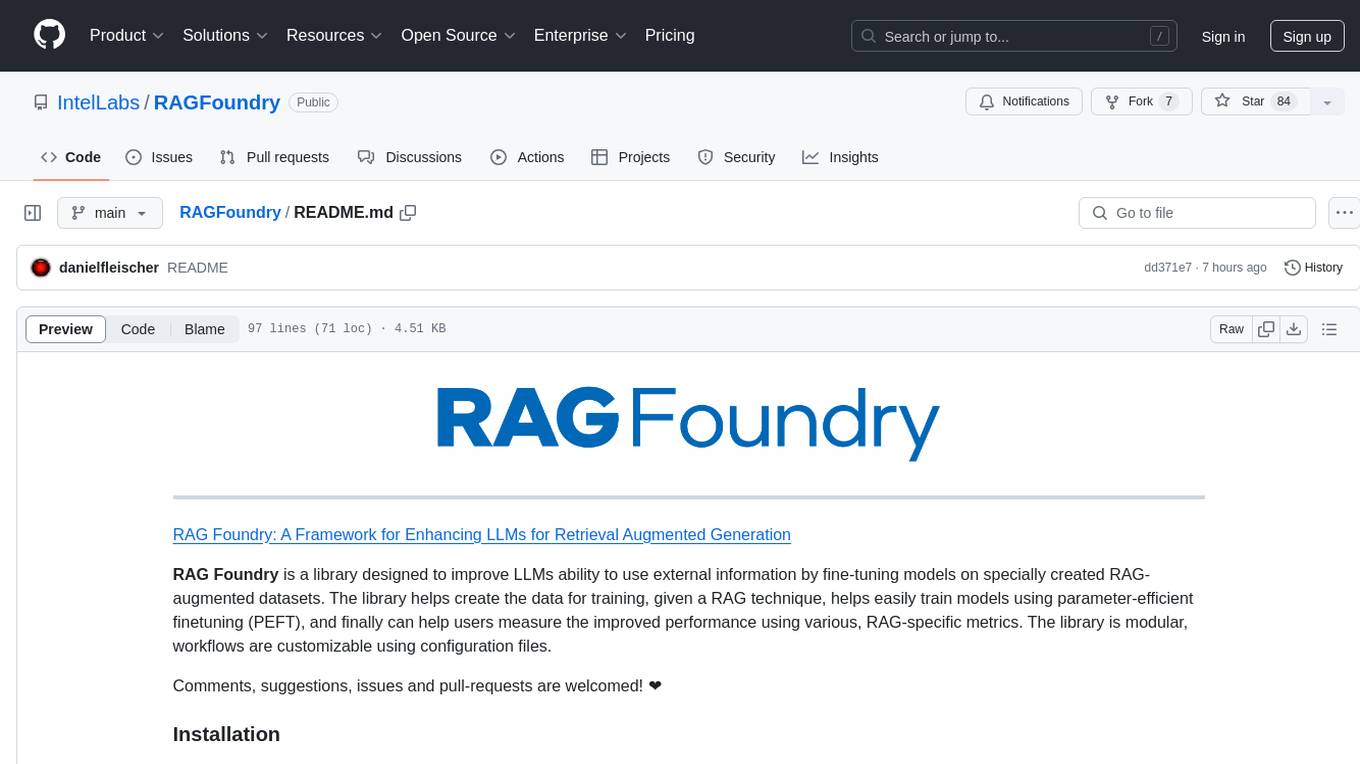
RAGFoundry
RAG Foundry is a library designed to enhance Large Language Models (LLMs) by fine-tuning models on RAG-augmented datasets. It helps create training data, train models using parameter-efficient finetuning (PEFT), and measure performance using RAG-specific metrics. The library is modular, customizable using configuration files, and facilitates prototyping with various RAG settings and configurations for tasks like data processing, retrieval, training, inference, and evaluation.
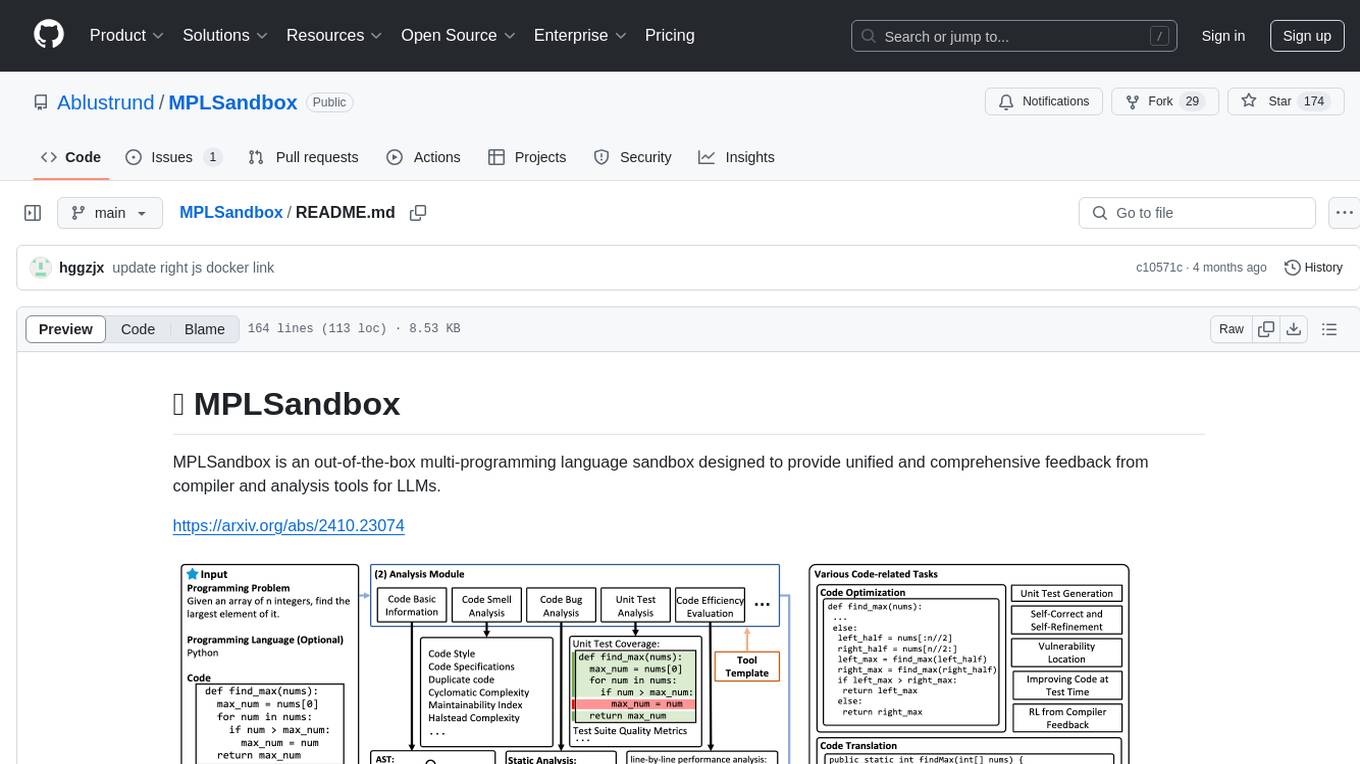
MPLSandbox
MPLSandbox is an out-of-the-box multi-programming language sandbox designed to provide unified and comprehensive feedback from compiler and analysis tools for LLMs. It simplifies code analysis for researchers and can be seamlessly integrated into LLM training and application processes to enhance performance in a range of code-related tasks. The sandbox environment ensures safe code execution, the code analysis module offers comprehensive analysis reports, and the information integration module combines compilation feedback and analysis results for complex code-related tasks.
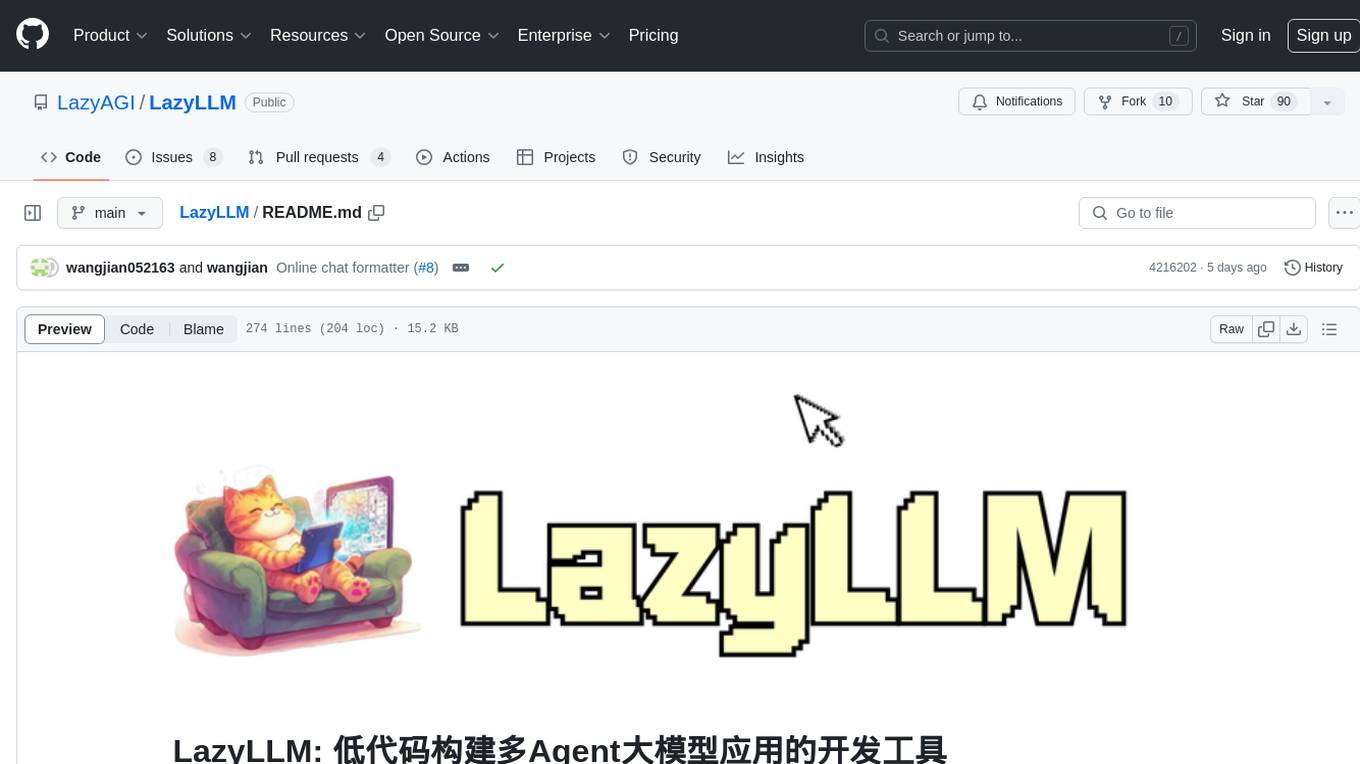
LazyLLM
LazyLLM is a low-code development tool for building complex AI applications with multiple agents. It assists developers in building AI applications at a low cost and continuously optimizing their performance. The tool provides a convenient workflow for application development and offers standard processes and tools for various stages of application development. Users can quickly prototype applications with LazyLLM, analyze bad cases with scenario task data, and iteratively optimize key components to enhance the overall application performance. LazyLLM aims to simplify the AI application development process and provide flexibility for both beginners and experts to create high-quality applications.
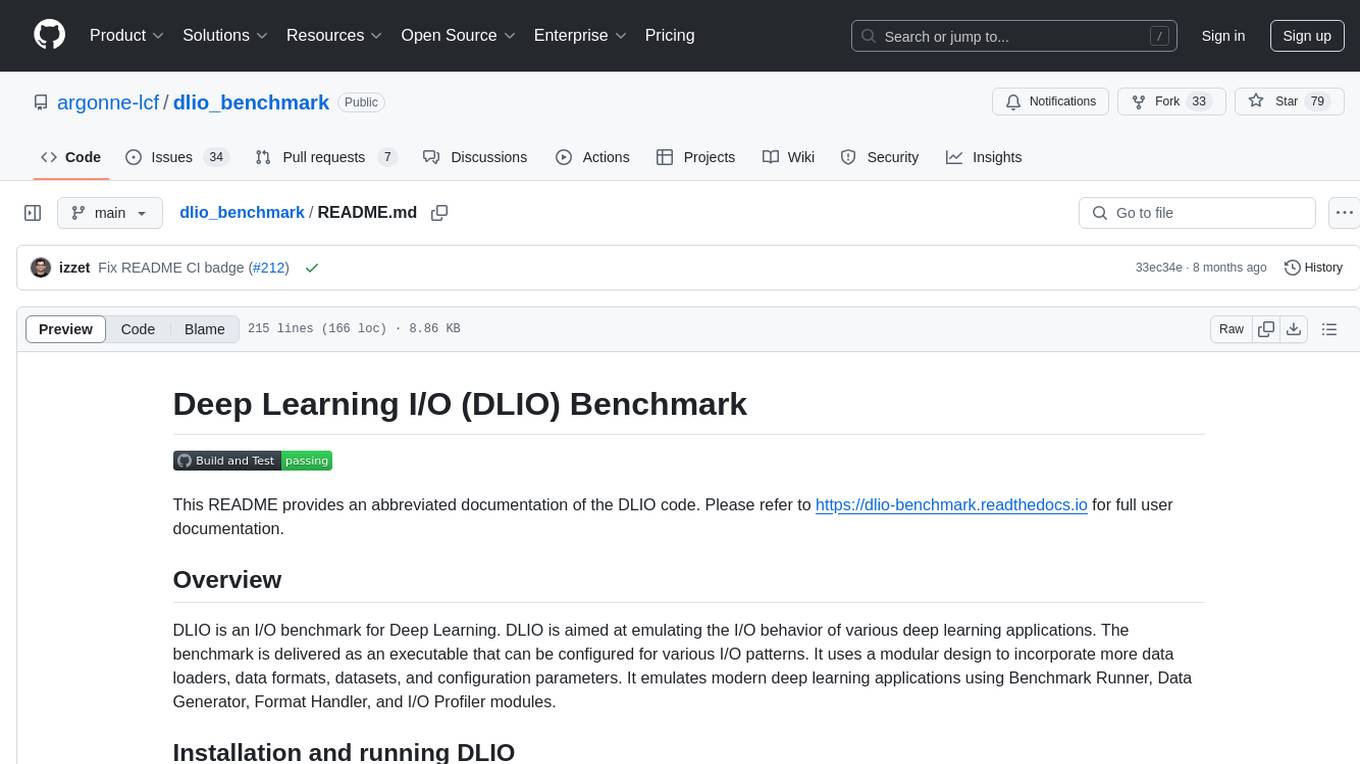
dlio_benchmark
DLIO is an I/O benchmark tool designed for Deep Learning applications. It emulates modern deep learning applications using Benchmark Runner, Data Generator, Format Handler, and I/O Profiler modules. Users can configure various I/O patterns, data loaders, data formats, datasets, and parameters. The tool is aimed at emulating the I/O behavior of deep learning applications and provides a modular design for flexibility and customization.
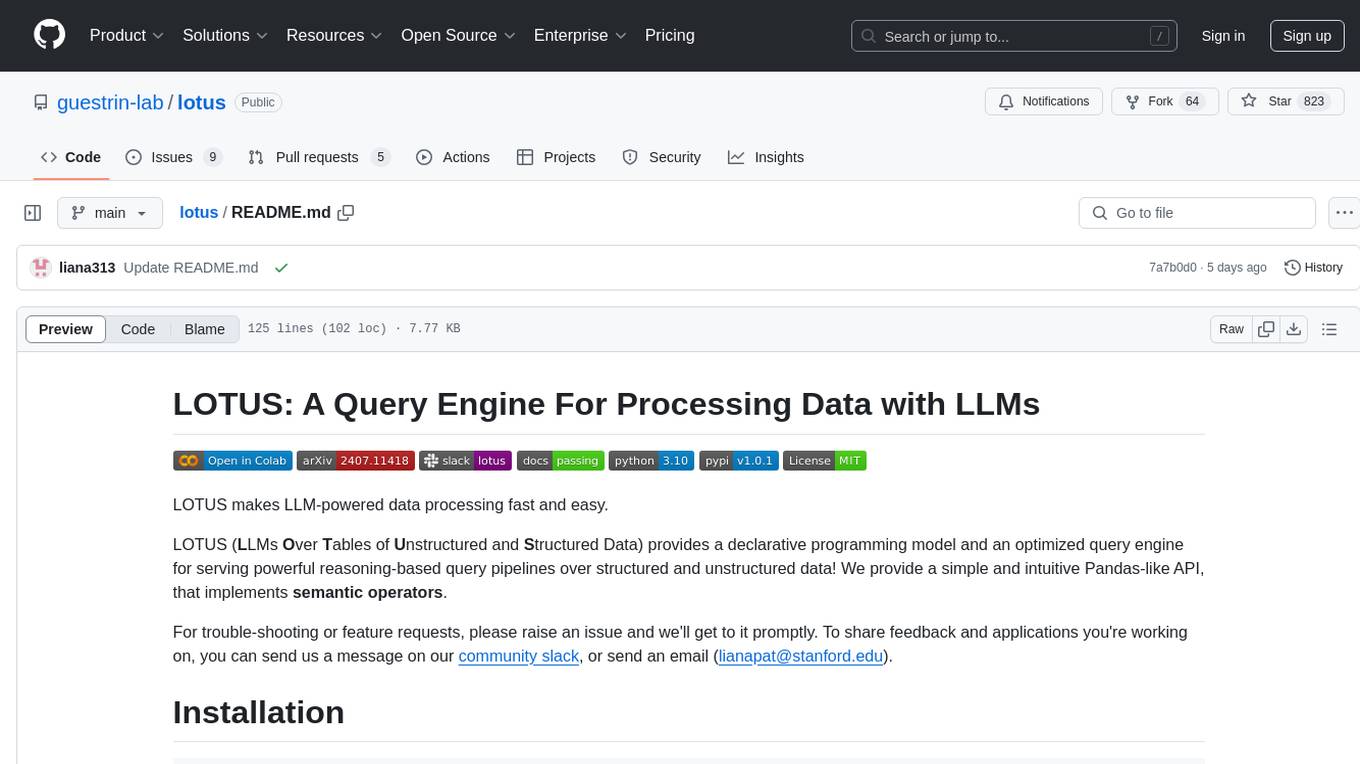
lotus
LOTUS (LLMs Over Tables of Unstructured and Structured Data) is a query engine that provides a declarative programming model and an optimized query engine for reasoning-based query pipelines over structured and unstructured data. It offers a simple and intuitive Pandas-like API with semantic operators for fast and easy LLM-powered data processing. The tool implements a semantic operator programming model, allowing users to write AI-based pipelines with high-level logic and leaving the rest of the work to the query engine. LOTUS supports various semantic operators like sem_map, sem_filter, sem_extract, sem_agg, sem_topk, sem_join, sem_sim_join, and sem_search, enabling users to perform tasks like mapping records, filtering data, aggregating records, and more. The tool also supports different model classes such as LM, RM, and Reranker for language modeling, retrieval, and reranking tasks respectively.
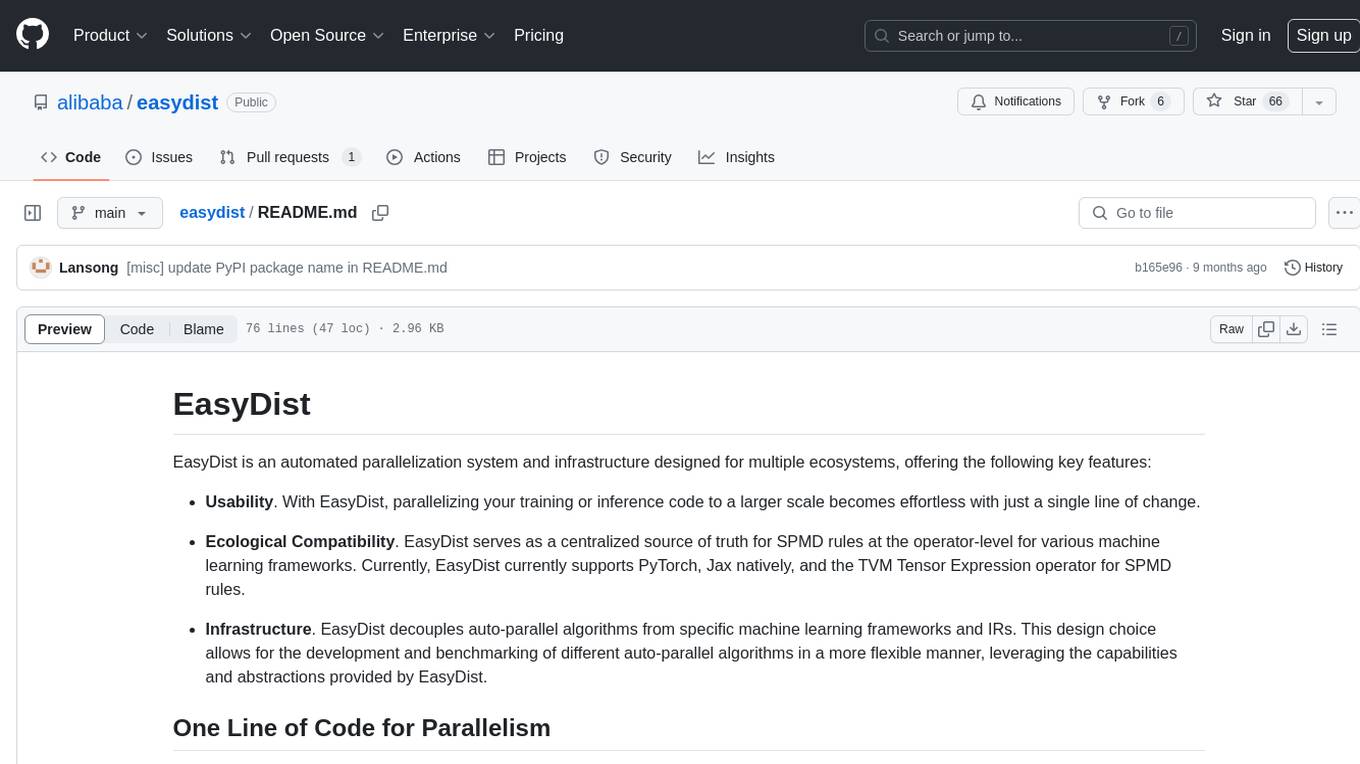
easydist
EasyDist is an automated parallelization system and infrastructure designed for multiple ecosystems. It offers usability by making parallelizing training or inference code effortless with just a single line of change. It ensures ecological compatibility by serving as a centralized source of truth for SPMD rules at the operator-level for various machine learning frameworks. EasyDist decouples auto-parallel algorithms from specific frameworks and IRs, allowing for the development and benchmarking of different auto-parallel algorithms in a flexible manner. The architecture includes MetaOp, MetaIR, and the ShardCombine Algorithm for SPMD sharding rules without manual annotations.
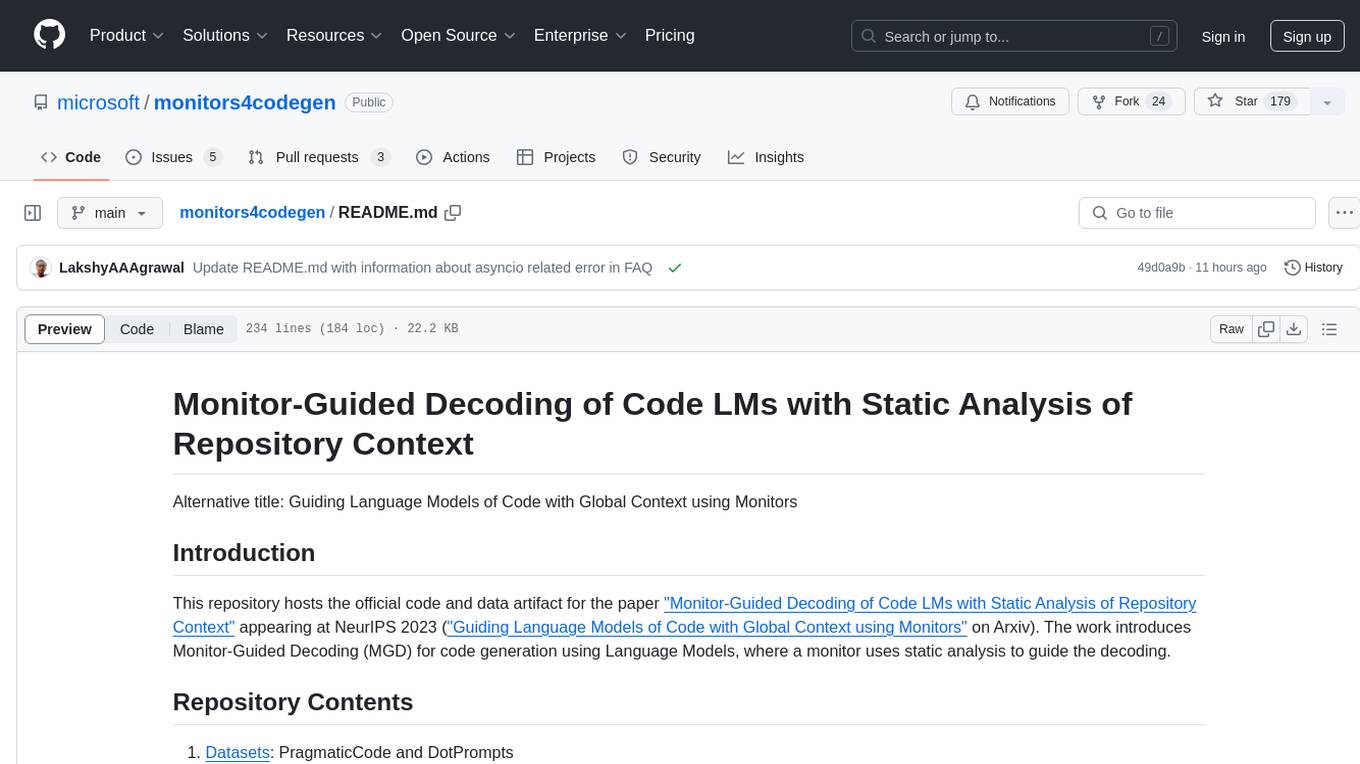
monitors4codegen
This repository hosts the official code and data artifact for the paper 'Monitor-Guided Decoding of Code LMs with Static Analysis of Repository Context'. It introduces Monitor-Guided Decoding (MGD) for code generation using Language Models, where a monitor uses static analysis to guide the decoding. The repository contains datasets, evaluation scripts, inference results, a language server client 'multilspy' for static analyses, and implementation of various monitors monitoring for different properties in 3 programming languages. The monitors guide Language Models to adhere to properties like valid identifier dereferences, correct number of arguments to method calls, typestate validity of method call sequences, and more.
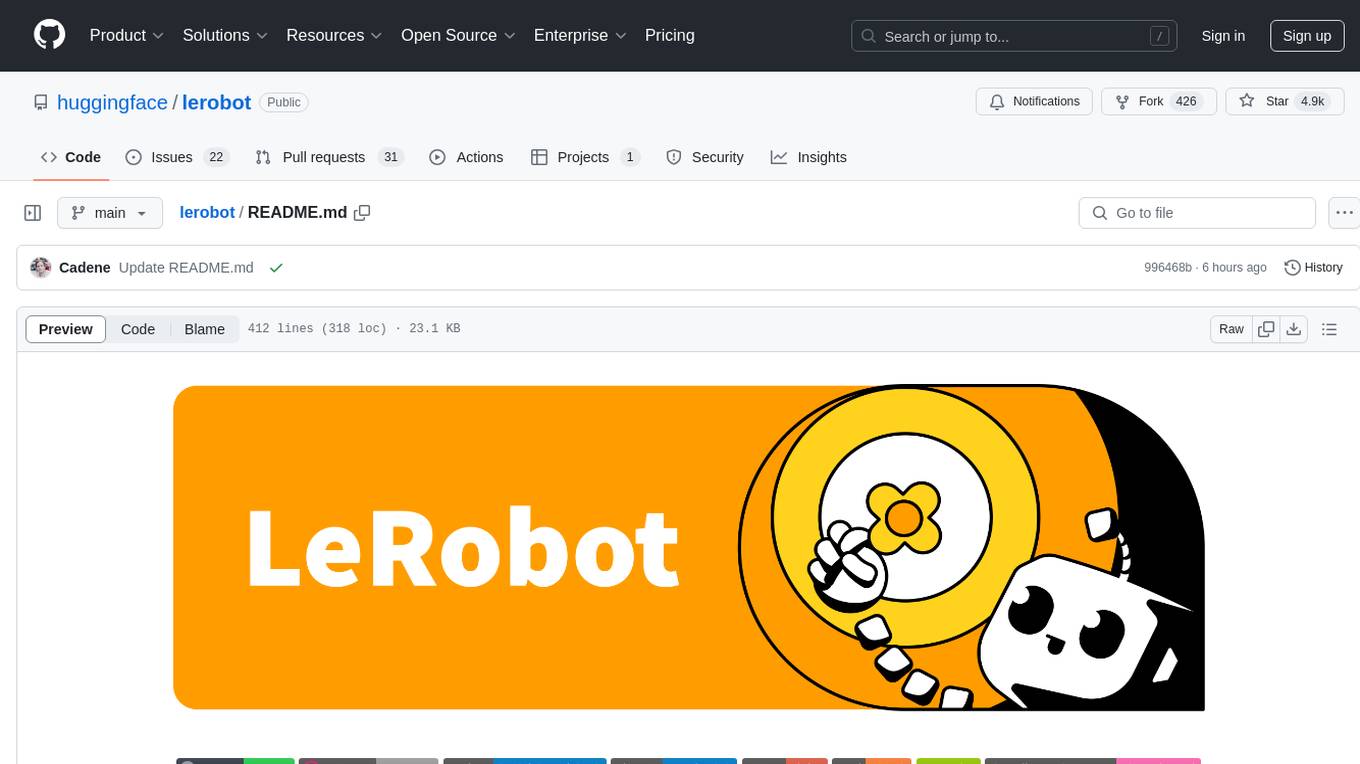
lerobot
LeRobot is a state-of-the-art AI library for real-world robotics in PyTorch. It aims to provide models, datasets, and tools to lower the barrier to entry to robotics, focusing on imitation learning and reinforcement learning. LeRobot offers pretrained models, datasets with human-collected demonstrations, and simulation environments. It plans to support real-world robotics on affordable and capable robots. The library hosts pretrained models and datasets on the Hugging Face community page.
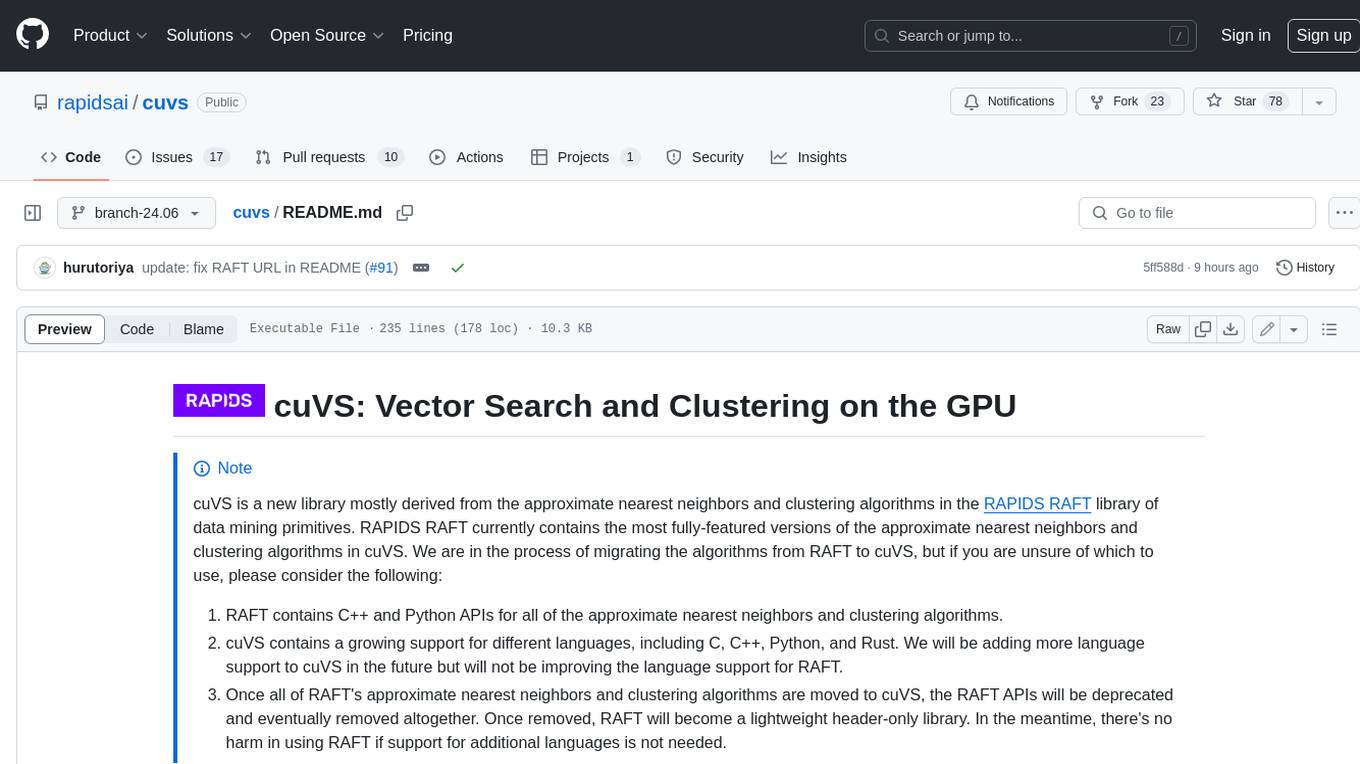
cuvs
cuVS is a library that contains state-of-the-art implementations of several algorithms for running approximate nearest neighbors and clustering on the GPU. It can be used directly or through the various databases and other libraries that have integrated it. The primary goal of cuVS is to simplify the use of GPUs for vector similarity search and clustering.
For similar tasks
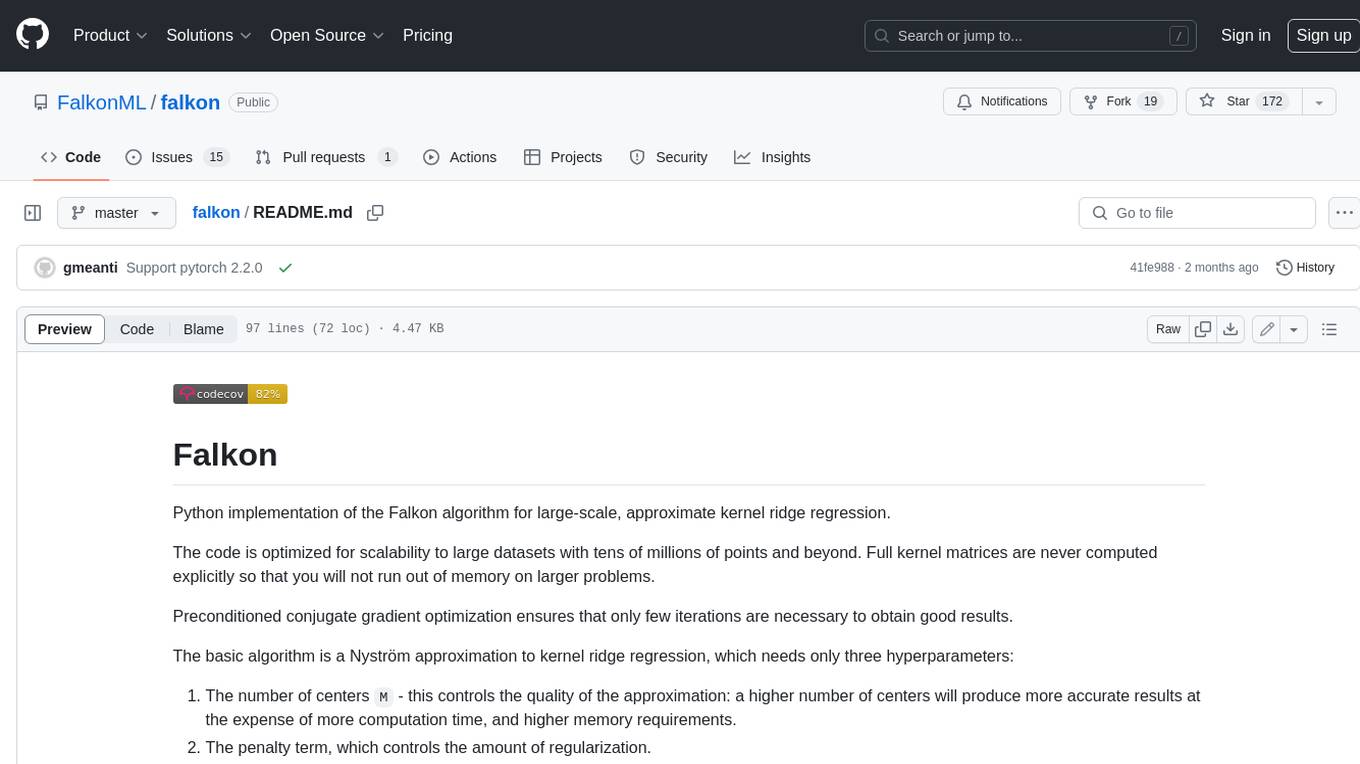
falkon
Falkon is a Python implementation of the Falkon algorithm for large-scale, approximate kernel ridge regression. The code is optimized for scalability to large datasets with tens of millions of points and beyond. Full kernel matrices are never computed explicitly so that you will not run out of memory on larger problems. Preconditioned conjugate gradient optimization ensures that only few iterations are necessary to obtain good results. The basic algorithm is a Nyström approximation to kernel ridge regression, which needs only three hyperparameters: 1. The number of centers M - this controls the quality of the approximation: a higher number of centers will produce more accurate results at the expense of more computation time, and higher memory requirements. 2. The penalty term, which controls the amount of regularization. 3. The kernel function. A good default is always the Gaussian (RBF) kernel (`falkon.kernels.GaussianKernel`).
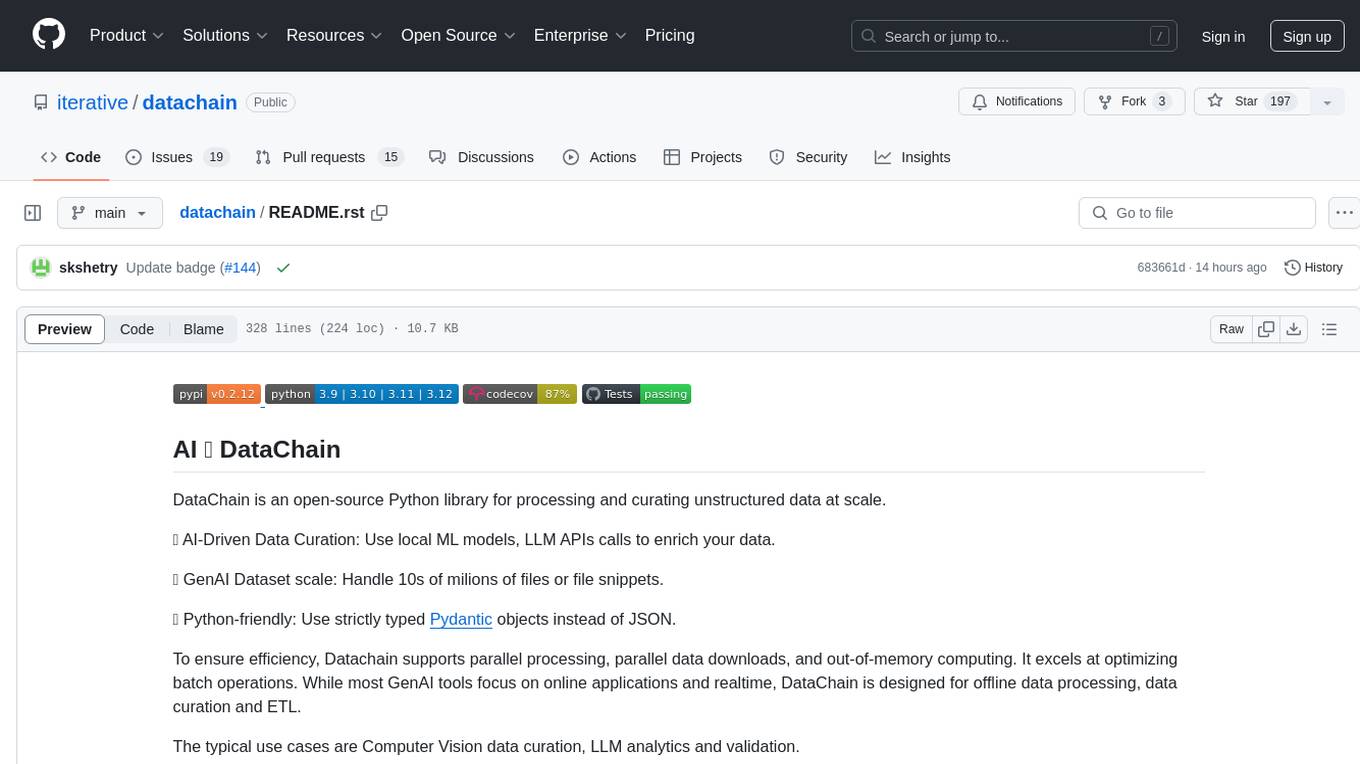
datachain
DataChain is an open-source Python library for processing and curating unstructured data at scale. It supports AI-driven data curation using local ML models and LLM APIs, handles large datasets, and is Python-friendly with Pydantic objects. It excels at optimizing batch operations and is designed for offline data processing, curation, and ETL. Typical use cases include Computer Vision data curation, LLM analytics, and validation.
For similar jobs
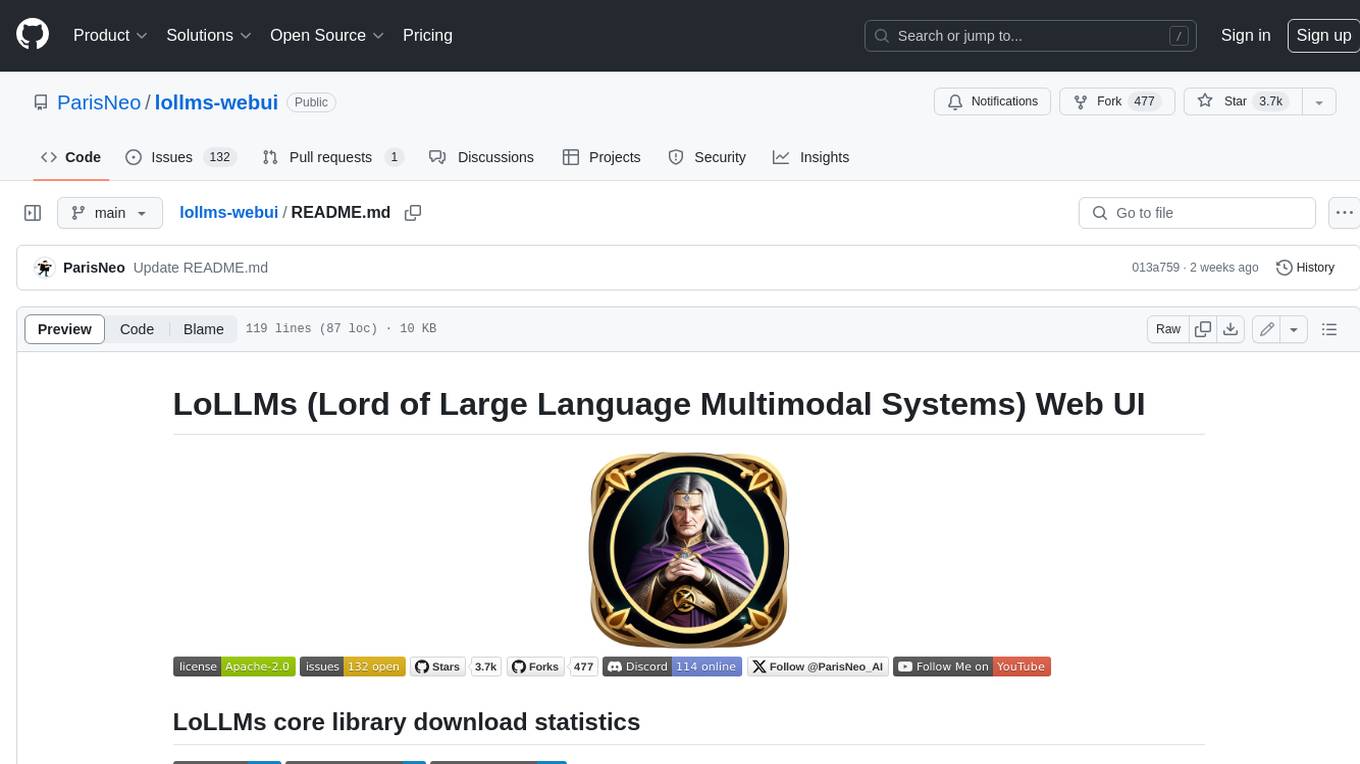
lollms-webui
LoLLMs WebUI (Lord of Large Language Multimodal Systems: One tool to rule them all) is a user-friendly interface to access and utilize various LLM (Large Language Models) and other AI models for a wide range of tasks. With over 500 AI expert conditionings across diverse domains and more than 2500 fine tuned models over multiple domains, LoLLMs WebUI provides an immediate resource for any problem, from car repair to coding assistance, legal matters, medical diagnosis, entertainment, and more. The easy-to-use UI with light and dark mode options, integration with GitHub repository, support for different personalities, and features like thumb up/down rating, copy, edit, and remove messages, local database storage, search, export, and delete multiple discussions, make LoLLMs WebUI a powerful and versatile tool.
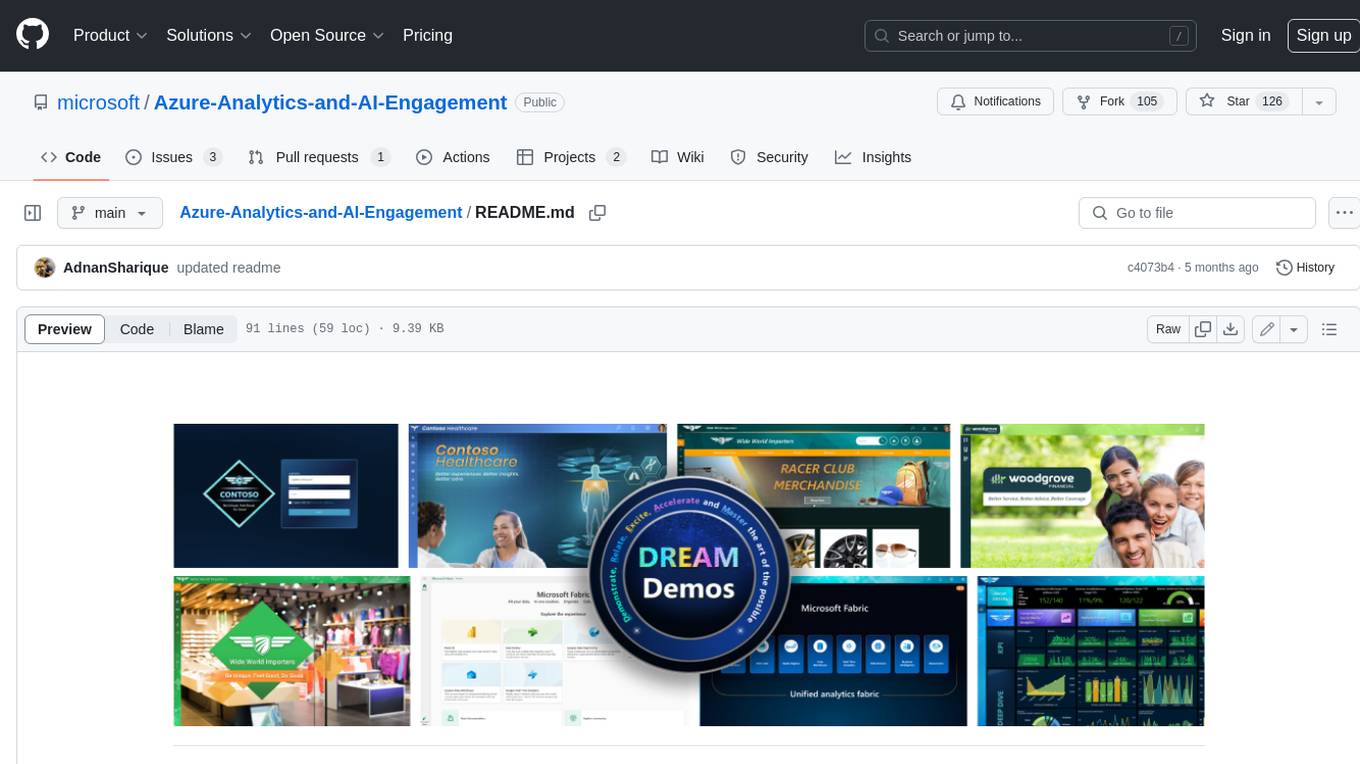
Azure-Analytics-and-AI-Engagement
The Azure-Analytics-and-AI-Engagement repository provides packaged Industry Scenario DREAM Demos with ARM templates (Containing a demo web application, Power BI reports, Synapse resources, AML Notebooks etc.) that can be deployed in a customer’s subscription using the CAPE tool within a matter of few hours. Partners can also deploy DREAM Demos in their own subscriptions using DPoC.
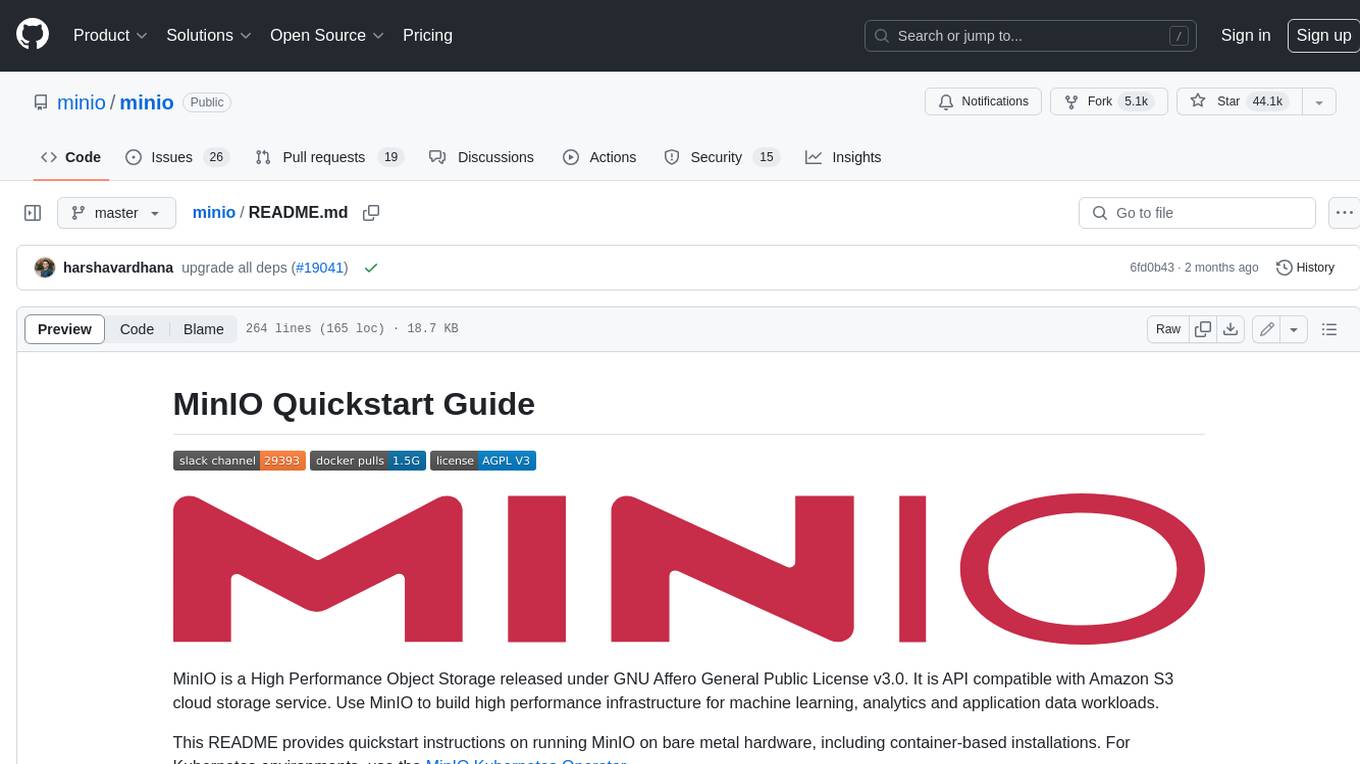
minio
MinIO is a High Performance Object Storage released under GNU Affero General Public License v3.0. It is API compatible with Amazon S3 cloud storage service. Use MinIO to build high performance infrastructure for machine learning, analytics and application data workloads.
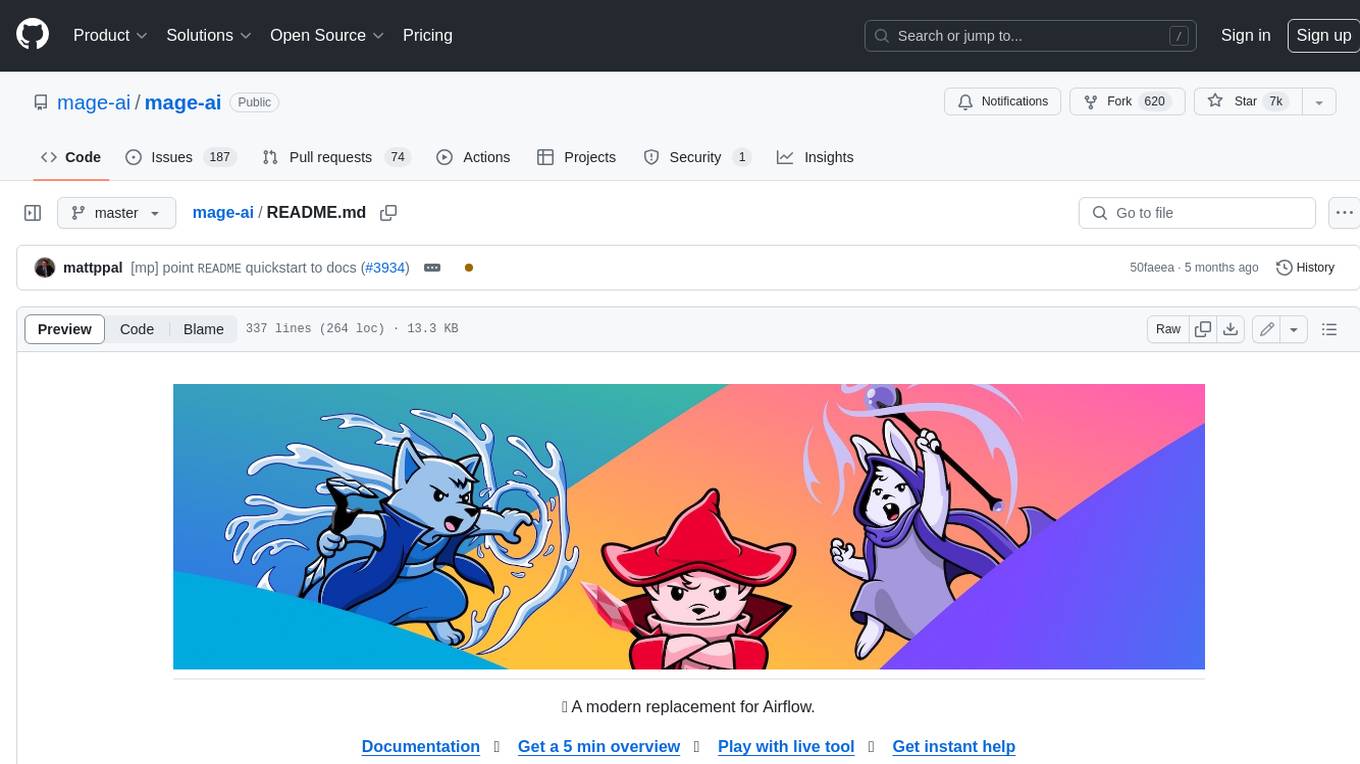
mage-ai
Mage is an open-source data pipeline tool for transforming and integrating data. It offers an easy developer experience, engineering best practices built-in, and data as a first-class citizen. Mage makes it easy to build, preview, and launch data pipelines, and provides observability and scaling capabilities. It supports data integrations, streaming pipelines, and dbt integration.
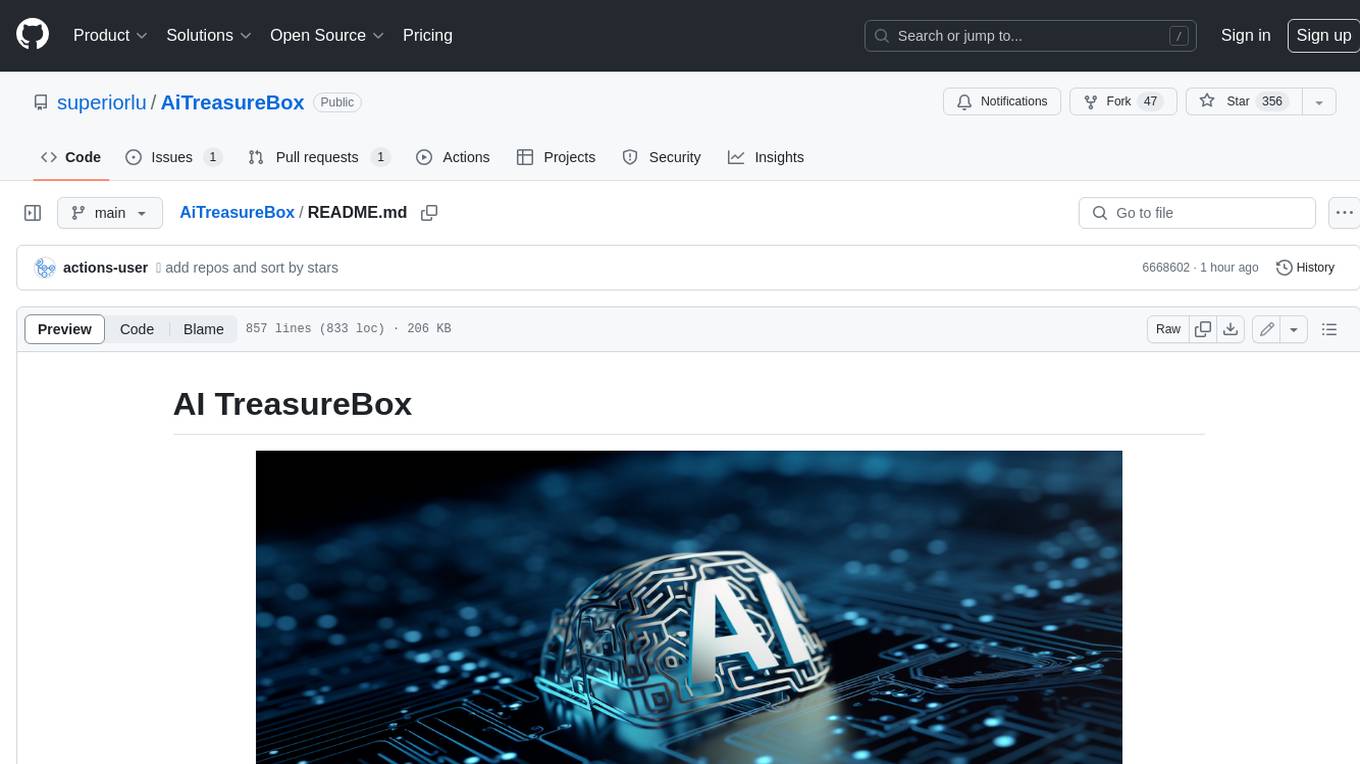
AiTreasureBox
AiTreasureBox is a versatile AI tool that provides a collection of pre-trained models and algorithms for various machine learning tasks. It simplifies the process of implementing AI solutions by offering ready-to-use components that can be easily integrated into projects. With AiTreasureBox, users can quickly prototype and deploy AI applications without the need for extensive knowledge in machine learning or deep learning. The tool covers a wide range of tasks such as image classification, text generation, sentiment analysis, object detection, and more. It is designed to be user-friendly and accessible to both beginners and experienced developers, making AI development more efficient and accessible to a wider audience.
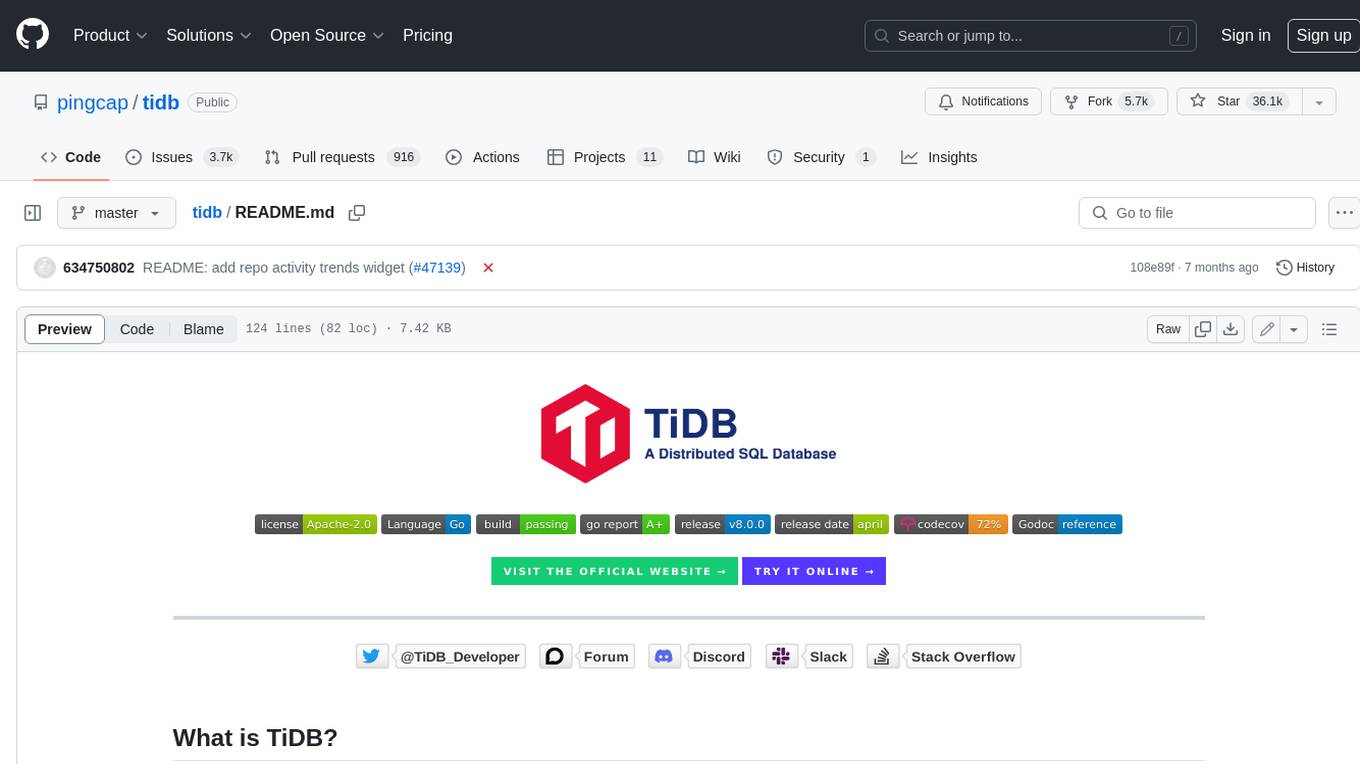
tidb
TiDB is an open-source distributed SQL database that supports Hybrid Transactional and Analytical Processing (HTAP) workloads. It is MySQL compatible and features horizontal scalability, strong consistency, and high availability.
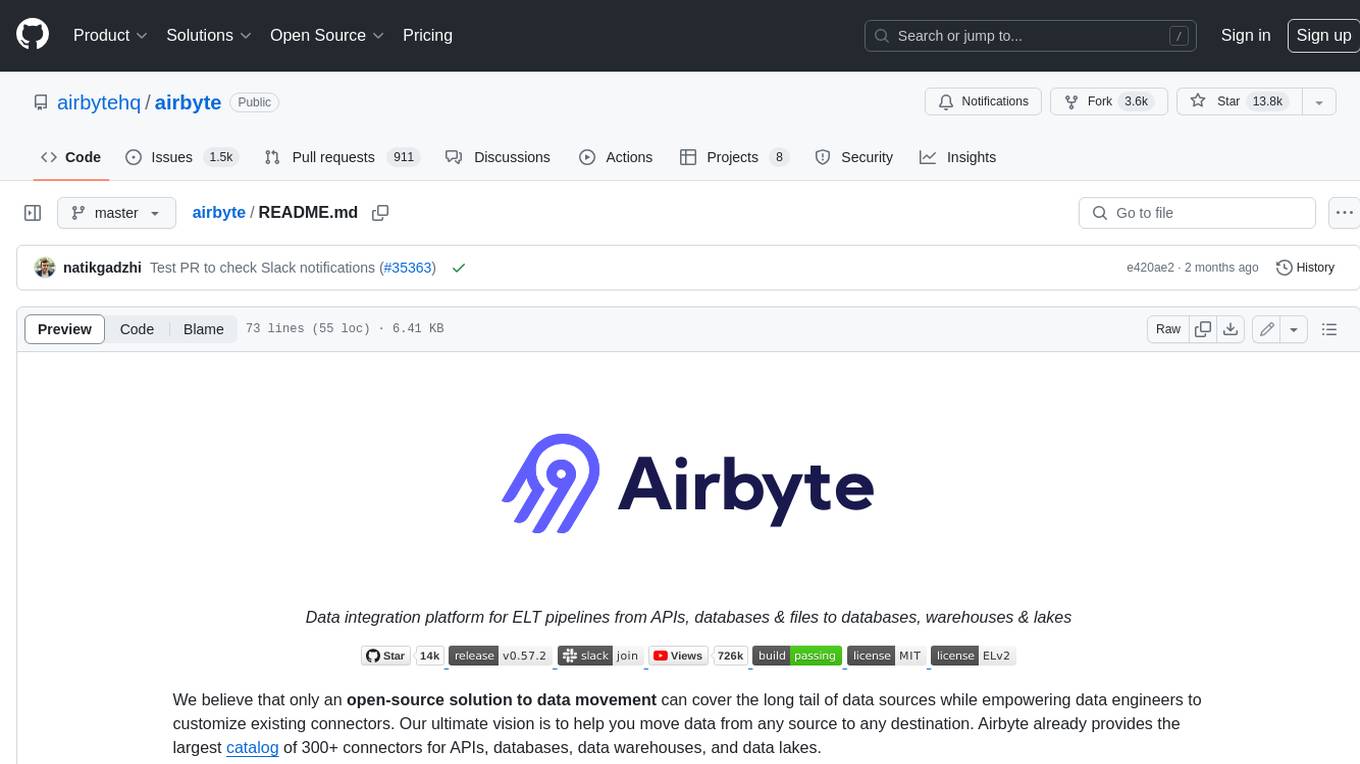
airbyte
Airbyte is an open-source data integration platform that makes it easy to move data from any source to any destination. With Airbyte, you can build and manage data pipelines without writing any code. Airbyte provides a library of pre-built connectors that make it easy to connect to popular data sources and destinations. You can also create your own connectors using Airbyte's no-code Connector Builder or low-code CDK. Airbyte is used by data engineers and analysts at companies of all sizes to build and manage their data pipelines.
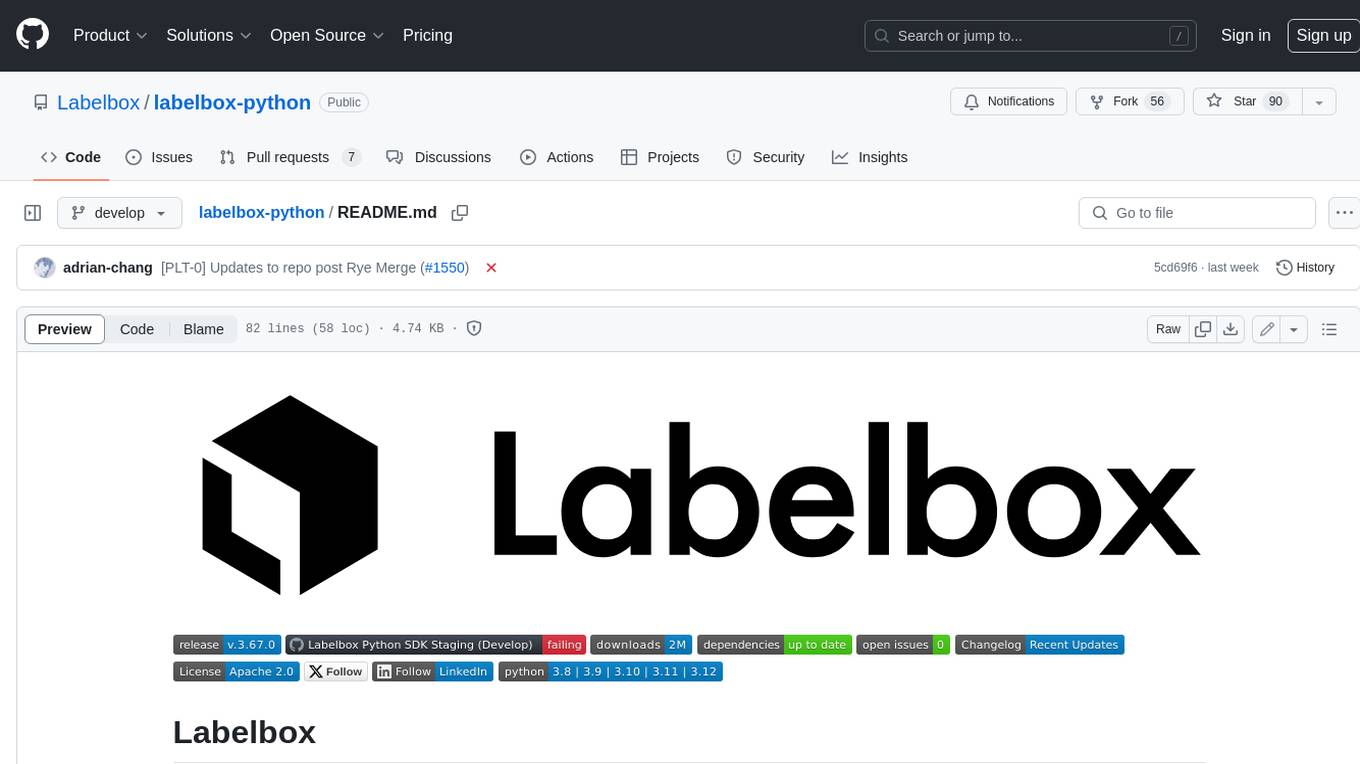
labelbox-python
Labelbox is a data-centric AI platform for enterprises to develop, optimize, and use AI to solve problems and power new products and services. Enterprises use Labelbox to curate data, generate high-quality human feedback data for computer vision and LLMs, evaluate model performance, and automate tasks by combining AI and human-centric workflows. The academic & research community uses Labelbox for cutting-edge AI research.