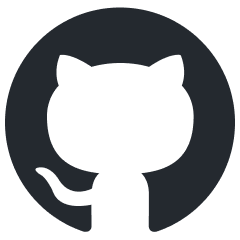
monitors4codegen
Code and Data artifact for NeurIPS 2023 paper - "Monitor-Guided Decoding of Code LMs with Static Analysis of Repository Context". `multispy` is a lsp client library in Python intended to be used to build applications around language servers.
Stars: 179
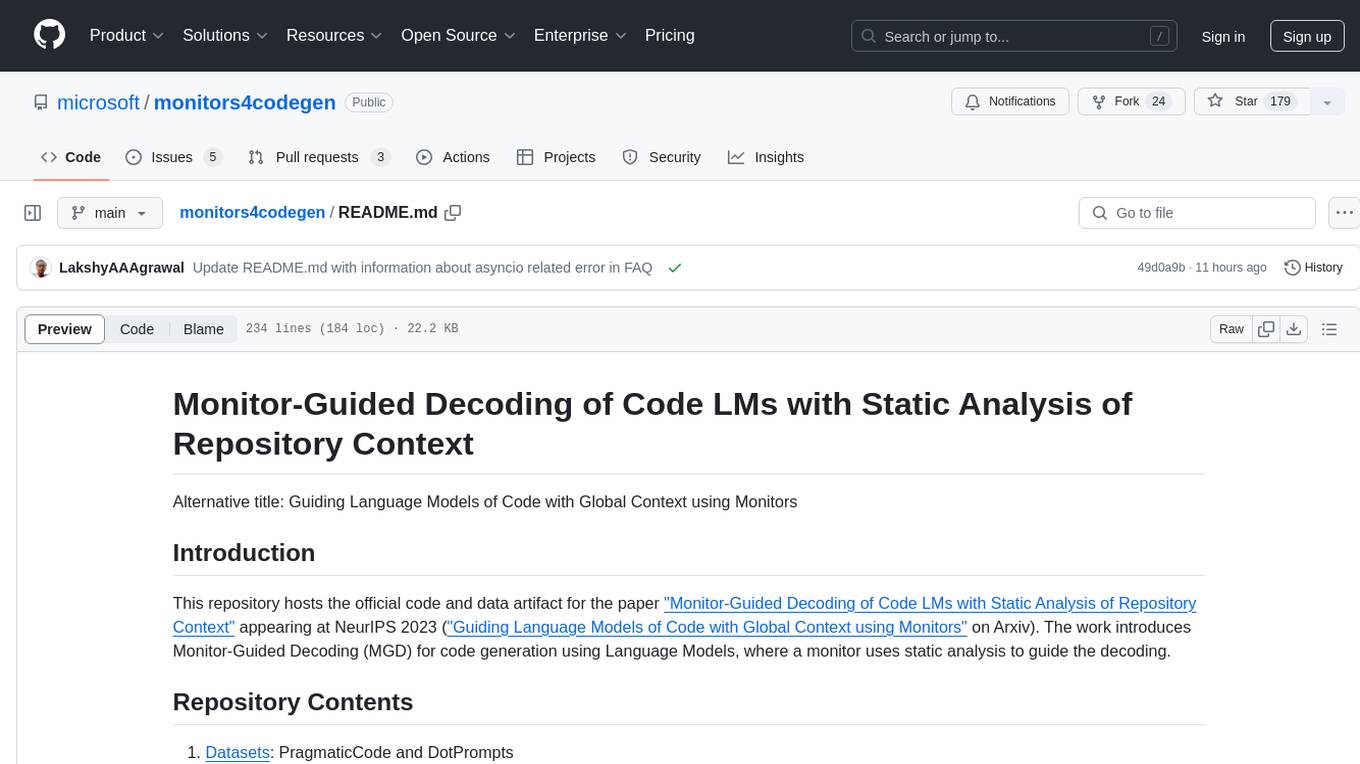
This repository hosts the official code and data artifact for the paper 'Monitor-Guided Decoding of Code LMs with Static Analysis of Repository Context'. It introduces Monitor-Guided Decoding (MGD) for code generation using Language Models, where a monitor uses static analysis to guide the decoding. The repository contains datasets, evaluation scripts, inference results, a language server client 'multilspy' for static analyses, and implementation of various monitors monitoring for different properties in 3 programming languages. The monitors guide Language Models to adhere to properties like valid identifier dereferences, correct number of arguments to method calls, typestate validity of method call sequences, and more.
README:
Alternative title: Guiding Language Models of Code with Global Context using Monitors
This repository hosts the official code and data artifact for the paper "Monitor-Guided Decoding of Code LMs with Static Analysis of Repository Context" appearing at NeurIPS 2023 ("Guiding Language Models of Code with Global Context using Monitors" on Arxiv). The work introduces Monitor-Guided Decoding (MGD) for code generation using Language Models, where a monitor uses static analysis to guide the decoding.
- Datasets: PragmaticCode and DotPrompts
- Evaluation scripts: Scripts to evaluate LMs by taking as input inferences (code generated by the model) for examples in DotPrompts and producing score@k scores for the metrics reported in the paper: Compilation Rate (CR), Next-Identifier Match (NIM), Identifier-Sequence Match (ISM) and Prefix Match (PM).
- Inference Results over DotPrompts: Generated code for examples in DotPrompts with various model configurations reported in the paper. The graphs and tables reported in the paper can be reproduced by running the evaluation scripts on the provided inference results.
-
multilspy
: A language server client, to easily obtain and use results of various static analyses provided by a large variety of language servers that communicate over the Language Server Protocol.multilspy
is intended to be used as a library to easily query various language servers, without having to worry about setting up their configurations and implementing the client-side of language server protocol.multilspy
currently supports running language servers for Java, Rust, C# and Python, and we aim to expand this list with the help of the community. - Monitor-Guided Decoding: Implementation of various monitors monitoring for different properties reported in the paper (for example: monitoring for type-valid identifier dereferences, monitoring for correct number of arguments to method calls, monitoring for typestate validity of method call sequences, etc.), spanning 3 programming languages.
For example, consider the partial code to be completed in the figure below. To complete this code, an LM has to generate identifiers consistent with the type of the object returned by ServerNode.Builder.newServerNode()
. The method newServerNode
and its return type, class ServerNode.Builder
, are defined in another file. If an LM does not have information about the ServerNode.Builder type
, it ends up hallucinating, as can be seen in the example generations with the text-davinci-003 and SantaCoder models. The completion uses identifiers host
and port
, which do not exist in the type ServerNode.Builder
. The generated code therefore results in “symbol not found” compilation errors.
MGD uses static analysis to guide the decoding of LMs, to generate code following certain properties. In the example, MGD is used to monitor for generating code with type-correct dereferences, and the SantaCoder model with the same prompt is able to generate the correct code completion, which compiles and matches the ground truth as well.
As reported in the paper, we observe that MGD can improve the compilation rate of code generated by LMs at all scales (350M-175B) by 19-25%, without any training/fine-tuning required. Further, it boosts the ground-truth match at all granularities from token-level to method-level code completion.
Number of repositories in PragmaticCode | 100 |
Number of methods in DotPrompts | 1420 |
Number of examples in DotPrompts | 10538 |
PragmaticCode is a dataset of real-world open-source Java projects complete with their development environments and dependencies (through their respective build systems). The authors tried to ensure that all the repositories in PragmaticCode were released publicly only after the determined training dataset cutoff date (31 March 2022) for the CodeGen, SantaCoder and text-davinci-003 family of models, which were used to evaluate MGD.
The full dataset, along with repository zip files is available in our Zenodo dataset release at https://zenodo.org/records/10072088. The list of repositories along with their respective licenses consisting PragmaticCode is available in datasets/PragmaticCode/repos.csv. The contents of the files required for inference for each of the repositories is available in datasets/PragmaticCode/fileContentsByRepo.json.
DotPrompts is a set of examples derived from PragmaticCode, such that each example consists of a prompt to a dereference location (a code location having the "." operator in Java). DotPrompts can be used to benchmark Language Models of Code on their ability to utilize repository level context to generate code for method-level completion tasks. The task for the models is to complete a partially written Java method, utilizing the full repository available from PragmaticCode. Since all the repositories in PragmaticCode are buildable, DotPrompts (derived from PragmaticCode) supports Compilation Rate as a metric of evaluation for generated code, apart from standard metrics of ground truth match like Next-Identifier Match, Identifier Sequence Match and Prefix Match.
The scenario described in motivating example above is an example in DotPrompts.
The complete description of an example in DotPrompts is a tuple - (repo, classFileName, methodStartIdx, methodStopIdx, dot_idx)
. The dataset is available at datasets/DotPrompts/dataset.csv.
We use the Python packages listed in requirements.txt. Our experiments used python 3.10. It is recommended to install the same with dependencies in an isolated virtual environment. To create a virtual environment using venv
:
python3 -m venv venv_monitors4codegen
source venv_monitors4codegen/bin/activate
or using conda:
conda create -n monitors4codegen python=3.10
conda activate monitors4codegen
Further details and instructions on creation of python virtual environments can be found in the official documentation. Further, we also refer users to Miniconda, as an alternative to the above steps for creation of the virtual environment.
To install the requirements for running evaluations as described below:
pip3 install -r requirements.txt
The evaluation script can be run as follows:
python3 eval_results.py <path to inference results - csv> <path to PragmaticCode filecontents - json> <path to output directory>
The above command will create a directory <path to output directory>
, containing all the graphs and tables reported in the paper along with extra details. The command also generates a report in the output directory, named Report.md
which relates the generated figures to sections in the paper.
To ensure that the environment setup has been done correctly, please run the below command, which runs the evaluation script over dummy data (included in inference_results/dotprompts_results_sample.csv). If the command fails, that indicates an error in the environment setup and the authors request you to kindly report the same.
python3 evaluation_scripts/eval_results.py inference_results/dotprompts_results_sample.csv datasets/PragmaticCode/fileContentsByRepo.json results_sample/
Description of expected columns in the inference results csv input to the evaluation script:
-
repo
: Name of the repository from which the testcase was sourced -
classFileName
: relative path to file containing the testcase prompt location -
methodStartIdx
: String index of starting'{'
of the method -
methodStopIdx
: String index of closing'}'
of the method -
dot_idx
: String index of'.'
that is the dereference prompt point -
configuration
: Identifies the configuration used to generate the given code sample. Values from:['SC-classExprTypes', 'CG-6B', 'SC-FIM-classExprTypes', 'SC-RLPG-MGD', 'SC-MGD', 'SC-FIM-classExprTypes-MGD', 'CG-2B', 'SC', 'CG-2B-MGD', 'CG-350M-classExprTypes-MGD', 'SC-FIM', 'TD-3', 'CG-350M-MGD', 'SC-FIM-MGD', 'SC-RLPG', 'CG-350M', 'CG-350M-classExprTypes', 'SC-classExprTypes-MGD', 'CG-6B-MGD', 'TD-3-MGD']
-
temperature
: Temperature used for sampling. Values from:[0.8, 0.6, 0.4, 0.2]
-
model
: Name of the model used for sampling. Values from:['Salesforce/codegen-6B-multi', 'bigcode/santacoder', 'Salesforce/codegen-2B-multi', 'Salesforce/codegen-350M-multi', 'text-davinci-003']
-
context
: Decoding strategy used. Values from:['autoregressive', 'fim']
-
prefix
: Prompt strategy used. Values from:['classExprTypes', 'none', 'rlpg']
-
rlpg_best_rule_name
: Name of the rule used for creating RLPG prompt (if used for the corresponding testcase). Values from:[nan, 'in_file#lines#0.25', 'in_file#lines#0.5', 'in_file#lines#0.75', 'import_file#method_names#0.5']
-
output
: Generated output by the model -
compilationSucceeded
: Result of compiling the generated method in the context of the full repository. 1 if success, 0 otherwise. Values from:[1, 0]
We provide all inferences (generated code) generated by all model configurations reported in the paper, for every example in DotPrompts. This consists of 6 independently sampled inferences for 18 different model configurations (spanning parameter scale, prompt templates, use of FIM context, etc.) for every example in DotPrompts.
The generated samples along with their compilation status, following the format described above, is available at inference_results/dotprompts_results.csv. The file is stored using git lfs. If the file is not available locally after cloning this repository, please check the git lfs website for instructions on setup, and clone the repository again after git lfs setup.
Each row in the file contains several multi-line string cells, and therefore, while viewing them in tools like Microsoft Office Excel, kindly enable "Word Wrap" to be able to view the full contents.
To run the evaluation scripts over the inferences, in order to reproduce the graphs and tables reported in the paper, run:
python3 evaluation_scripts/eval_results.py inference_results/dotprompts_results.csv datasets/PragmaticCode/fileContentsByRepo.json results/
The above command creates a directory results (already included in the repository), containing all the figures and tables provided in the paper along with extra details. The command also generates a report in the output directory which relates the generated figures to sections in the paper. In case of above command, the report is generated at results/Report.md.
multilspy
is a cross-platform library that we have built to set up and interact with various language servers in a unified and easy way. Language servers are tools that perform a variety of static analyses on source code and provide useful information such as type-directed code completion suggestions, symbol definition locations, symbol references, etc., over the Language Server Protocol (LSP). multilspy
intends to ease the process of using language servers, by abstracting the setting up of the language servers, performing language-specific configuration and handling communication with the server over the json-rpc based protocol, while exposing a simple interface to the user.
Since LSP is language-agnostic, multilspy
can provide the results for static analyses of code in different languages over a common interface. multilspy
is easily extensible to any language that has a Language Server and currently supports Java, Rust, C# and Python and we aim to support more language servers from the list of language server implementations.
Some of the analyses results that multilspy
can provide are:
- Finding the definition of a function or a class (textDocument/definition)
- Finding the callers of a function or the instantiations of a class (textDocument/references)
- Providing type-based dereference completions (textDocument/completion)
- Getting information displayed when hovering over symbols, like method signature (textDocument/hover)
- Getting list/tree of all symbols defined in a given file, along with symbol type like class, method, etc. (textDocument/documentSymbol)
- Please create an issue/PR to add any other LSP request not listed above
To install multilspy
using pip, execute the following command:
pip install https://github.com/microsoft/monitors4codegen/archive/main.zip
Example usage:
from monitors4codegen.multilspy import SyncLanguageServer
from monitors4codegen.multilspy.multilspy_config import MultilspyConfig
from monitors4codegen.multilspy.multilspy_logger import MultilspyLogger
...
config = MultilspyConfig.from_dict({"code_language": "java"}) # Also supports "python", "rust", "csharp"
logger = MultilspyLogger()
lsp = SyncLanguageServer.create(config, logger, "/abs/path/to/project/root/")
with lsp.start_server():
result = lsp.request_definition(
"relative/path/to/code_file.java", # Filename of location where request is being made
163, # line number of symbol for which request is being made
4 # column number of symbol for which request is being made
)
result2 = lsp.request_completions(
...
)
result3 = lsp.request_references(
...
)
result4 = lsp.request_document_symbols(
...
)
result5 = lsp.request_hover(
...
)
...
multilspy
also provides an asyncio based API which can be used in async contexts. Example usage (asyncio):
from monitors4codegen.multilspy import LanguageServer
...
lsp = LanguageServer.create(...)
async with lsp.start_server():
result = await lsp.request_definition(
...
)
...
The file src/monitors4codegen/multilspy/language_server.py provides the multilspy
API. Several tests for multilspy
present under tests/multilspy/ provide detailed usage examples for multilspy
. The tests can be executed by running:
pytest tests/multilspy
A monitor under the Monitor-Guided Decoding framework, is instantiated using multilspy
as the LSP client, and provides maskgen to guide the LM decoding. The monitor interface is defined as class Monitor
in file src/monitors4codegen/monitor_guided_decoding/monitor.py. The interface is implemented by various monitors supporting different properties like valid identifier dereferences, valid number of arguments, valid typestate method calls, etc.
src/monitors4codegen/monitor_guided_decoding/hf_gen.py provides the class MGDLogitsProcessor
which can be used with any HuggingFace Language Model, as a LogitsProcessor
to guide the LM using MGD. Example uses with SantaCoder model are available in tests/monitor_guided_decoding/test_dereferences_monitor_java.py.
src/monitors4codegen/monitor_guided_decoding/openai_gen.py provides the method openai_mgd
which takes the prompt and a Monitor
as input, and returns the MGD guided generation using an OpenAI model.
src/monitors4codegen/monitor_guided_decoding/monitors/dereferences_monitor.py provides the instantiation of Monitor
class for dereferences monitor. It can be used to guide LMs to generate valid identifier dereferences. Unit tests for the dereferences monitor are present in tests/monitor_guided_decoding/test_dereferences_monitor_java.py, which also provide usage examples for the dereferences monitor.
src/monitors4codegen/monitor_guided_decoding/monitors/numargs_monitor.py provides the instantiation of Monitor
class for numargs_monitor. It can be used to guide LMs to generate correct number of arguments to function calls. Unit tests, which also provide usage examples are present in tests/monitor_guided_decoding/test_numargs_monitor_java.py.
The typestate analysis is used to enforce that methods on an object are called in a certain order, consistent with the ordering constraints provided by the API contracts. Example usage of the typestate monitor for Rust is available in the unit test file tests/monitor_guided_decoding/test_typestate_monitor_rust.py.
src/monitors4codegen/monitor_guided_decoding/monitors/switch_enum_monitor.py provides the instantiation of Monitor
for generating valid named enum constants in C#. Unit tests for the switch-enum monitor are present in tests/monitor_guided_decoding/test_switchenum_monitor_csharp.py, which also provide usage examples for the switch-enum monitor.
src/monitors4codegen/monitor_guided_decoding/monitors/class_instantiation_monitor.py provides the instantiation of Monitor
for generating valid class instantiations following 'new '
in a Java code base. Unit tests for the class-instantiation monitor, which provide examples usages are present in tests/monitor_guided_decoding/test_classinstantiation_monitor_java.py.
Multiple monitors can be used simultaneously to guide LMs to adhere to multiple properties. Example demonstration with 2 monitors used jointly are present in tests/monitor_guided_decoding/test_joint_monitors.py.
If you get the following error:
RuntimeError: Task <Task pending name='Task-2' coro=<_AsyncGeneratorContextManager.__aenter__() running at
python3.8/contextlib.py:171> cb=[_chain_future.<locals>._call_set_state() at
python3.8/asyncio/futures.py:367]> got Future <Future pending> attached to a different loop python3.8/asyncio/locks.py:309: RuntimeError
Please ensure that you create a new environment with Python >=3.10
. For further details, please have a look at the StackOverflow Discussion.
This project welcomes contributions and suggestions. Most contributions require you to agree to a Contributor License Agreement (CLA) declaring that you have the right to, and actually do, grant us the rights to use your contribution. For details, visit https://cla.opensource.microsoft.com.
When you submit a pull request, a CLA bot will automatically determine whether you need to provide a CLA and decorate the PR appropriately (e.g., status check, comment). Simply follow the instructions provided by the bot. You will only need to do this once across all repos using our CLA.
This project has adopted the Microsoft Open Source Code of Conduct. For more information see the Code of Conduct FAQ or contact [email protected] with any additional questions or comments.
This project may contain trademarks or logos for projects, products, or services. Authorized use of Microsoft trademarks or logos is subject to and must follow Microsoft's Trademark & Brand Guidelines. Use of Microsoft trademarks or logos in modified versions of this project must not cause confusion or imply Microsoft sponsorship. Any use of third-party trademarks or logos are subject to those third-party's policies.
For Tasks:
Click tags to check more tools for each tasksFor Jobs:
Alternative AI tools for monitors4codegen
Similar Open Source Tools
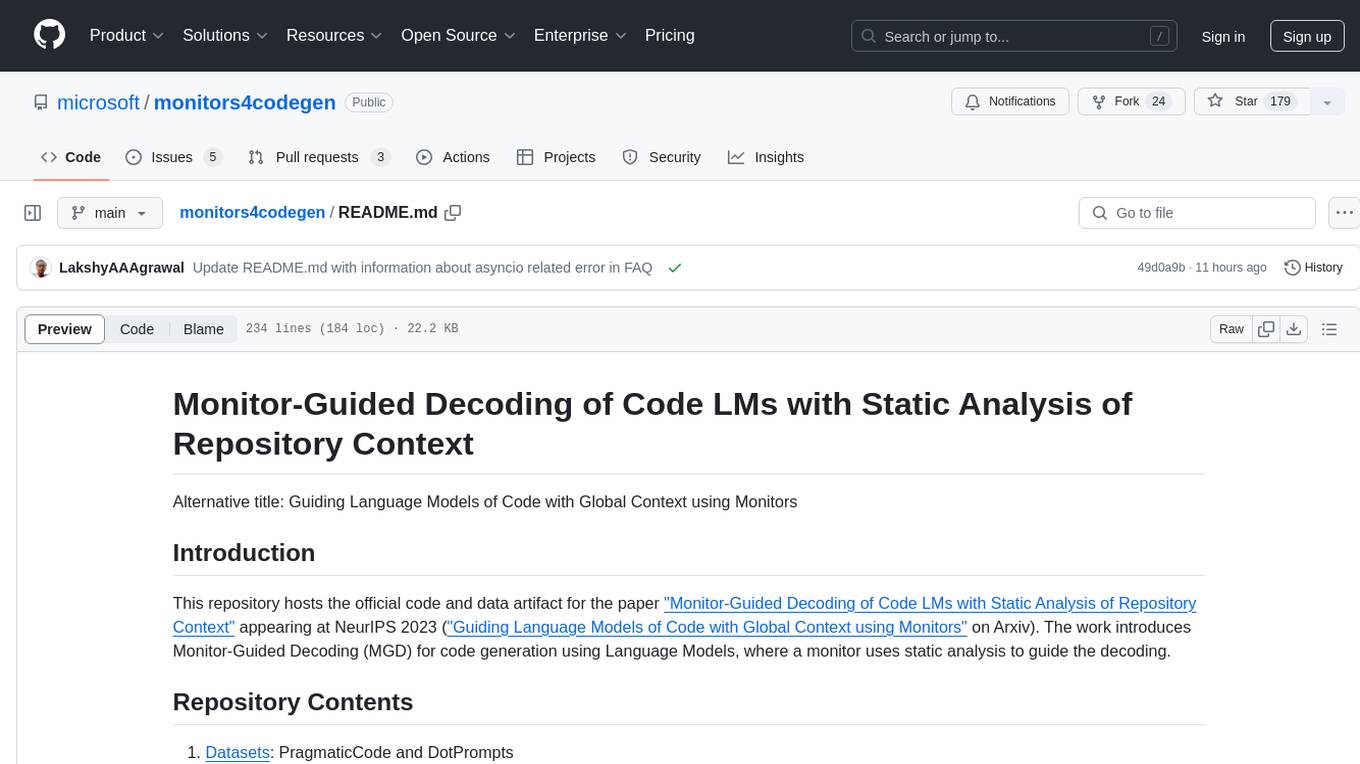
monitors4codegen
This repository hosts the official code and data artifact for the paper 'Monitor-Guided Decoding of Code LMs with Static Analysis of Repository Context'. It introduces Monitor-Guided Decoding (MGD) for code generation using Language Models, where a monitor uses static analysis to guide the decoding. The repository contains datasets, evaluation scripts, inference results, a language server client 'multilspy' for static analyses, and implementation of various monitors monitoring for different properties in 3 programming languages. The monitors guide Language Models to adhere to properties like valid identifier dereferences, correct number of arguments to method calls, typestate validity of method call sequences, and more.
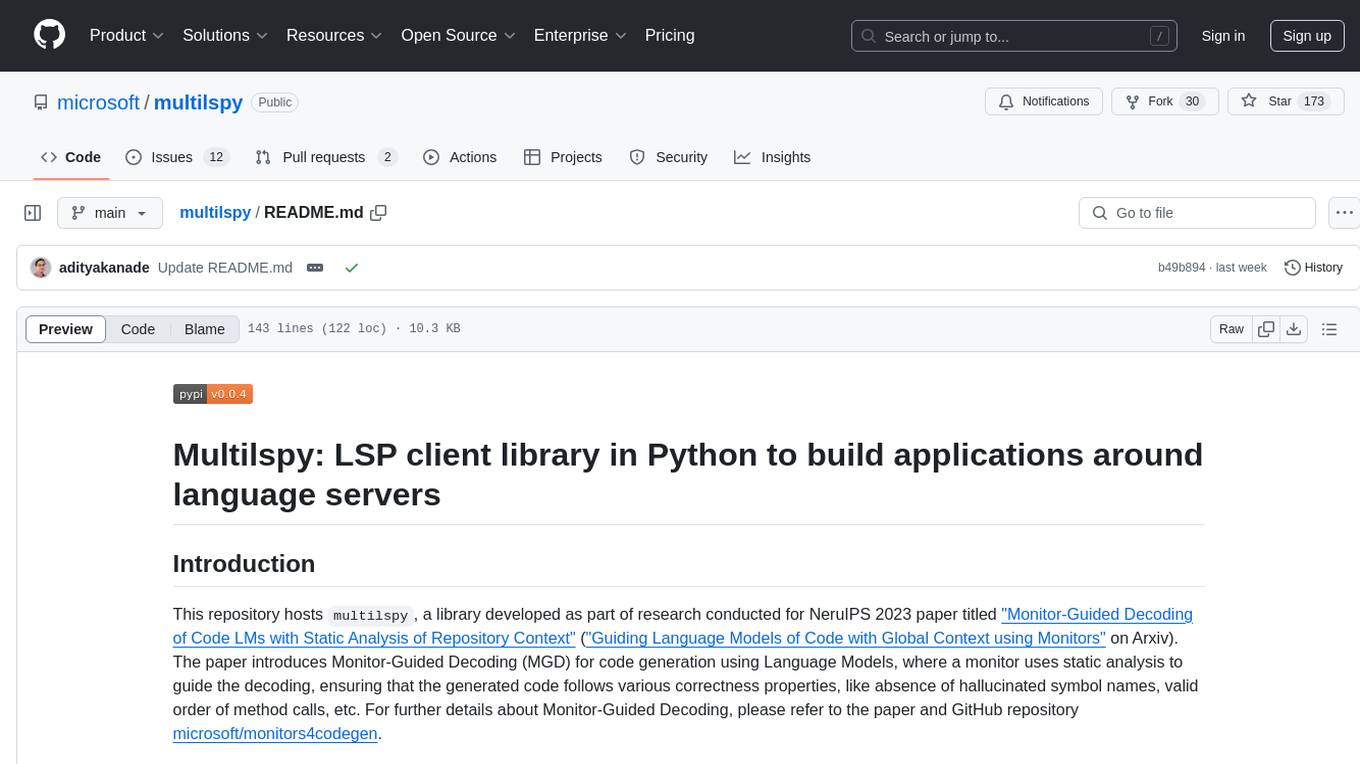
multilspy
Multilspy is a Python library developed for research purposes to facilitate the creation of language server clients for querying and obtaining results of static analyses from various language servers. It simplifies the process by handling server setup, communication, and configuration parameters, providing a common interface for different languages. The library supports features like finding function/class definitions, callers, completions, hover information, and document symbols. It is designed to work with AI systems like Large Language Models (LLMs) for tasks such as Monitor-Guided Decoding to ensure code generation correctness and boost compilability.
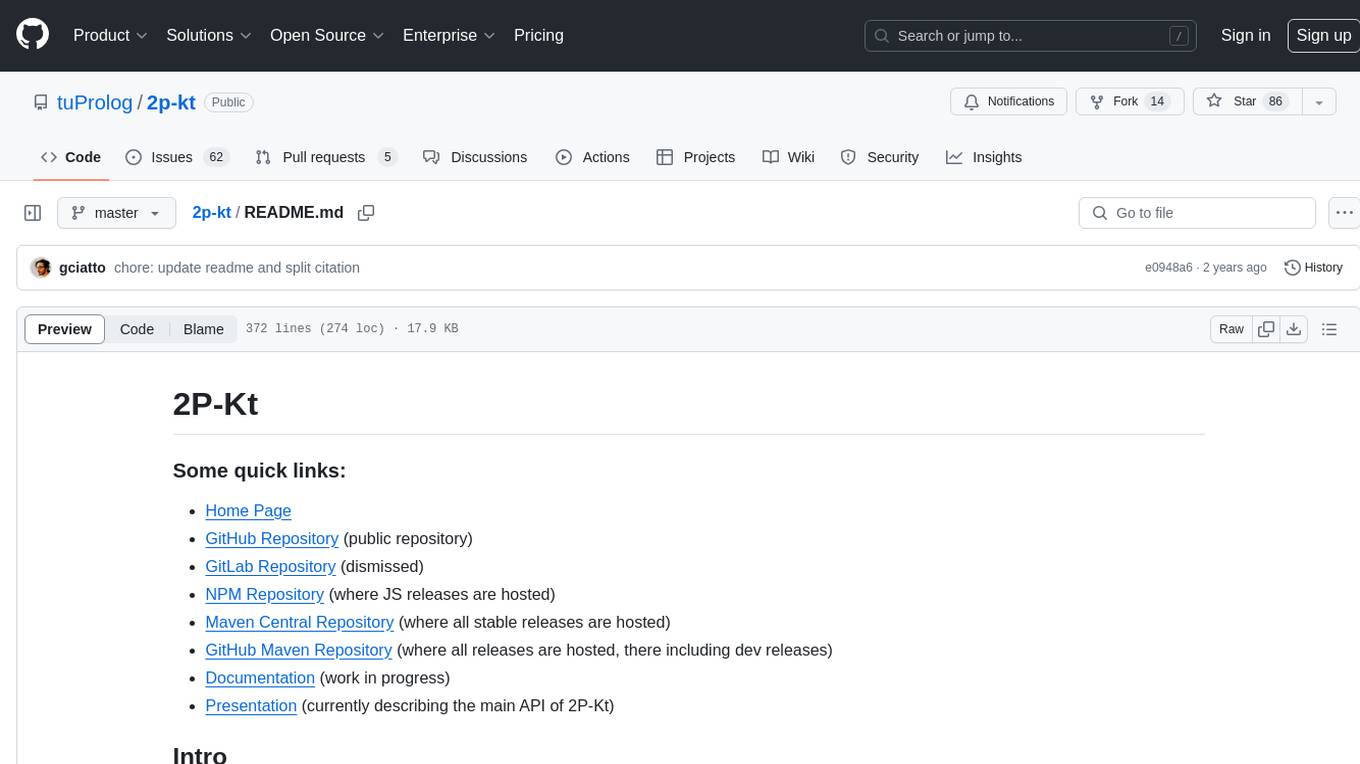
2p-kt
2P-Kt is a Kotlin-based and multi-platform reboot of tuProlog (2P), a multi-paradigm logic programming framework written in Java. It consists of an open ecosystem for Symbolic Artificial Intelligence (AI) with modules supporting logic terms, unification, indexing, resolution of logic queries, probabilistic logic programming, binary decision diagrams, OR-concurrent resolution, DSL for logic programming, parsing modules, serialisation modules, command-line interface, and graphical user interface. The tool is designed to support knowledge representation and automatic reasoning through logic programming in an extensible and flexible way, encouraging extensions towards other symbolic AI systems than Prolog. It is a pure, multi-platform Kotlin project supporting JVM, JS, Android, and Native platforms, with a lightweight library leveraging the Kotlin common library.
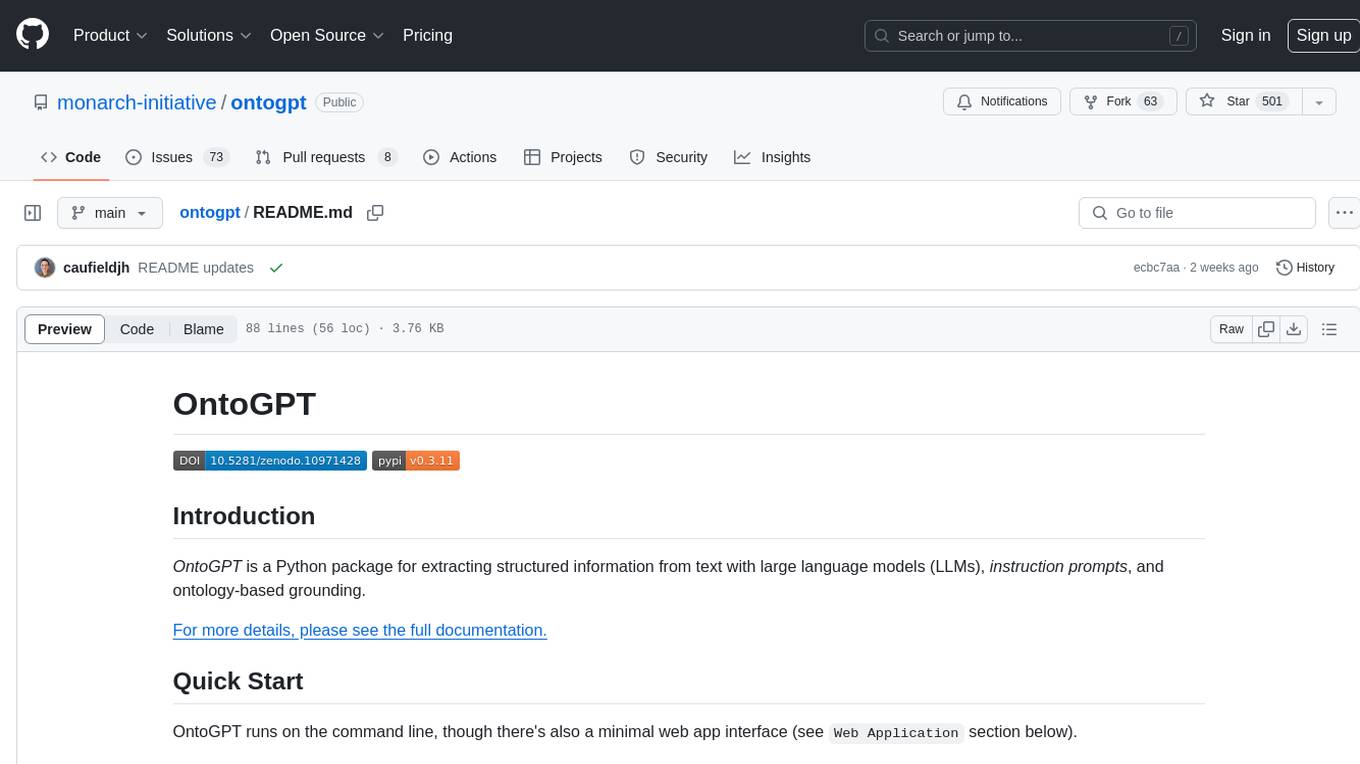
ontogpt
OntoGPT is a Python package for extracting structured information from text using large language models, instruction prompts, and ontology-based grounding. It provides a command line interface and a minimal web app for easy usage. The tool has been evaluated on test data and is used in related projects like TALISMAN for gene set analysis. OntoGPT enables users to extract information from text by specifying relevant terms and provides the extracted objects as output.
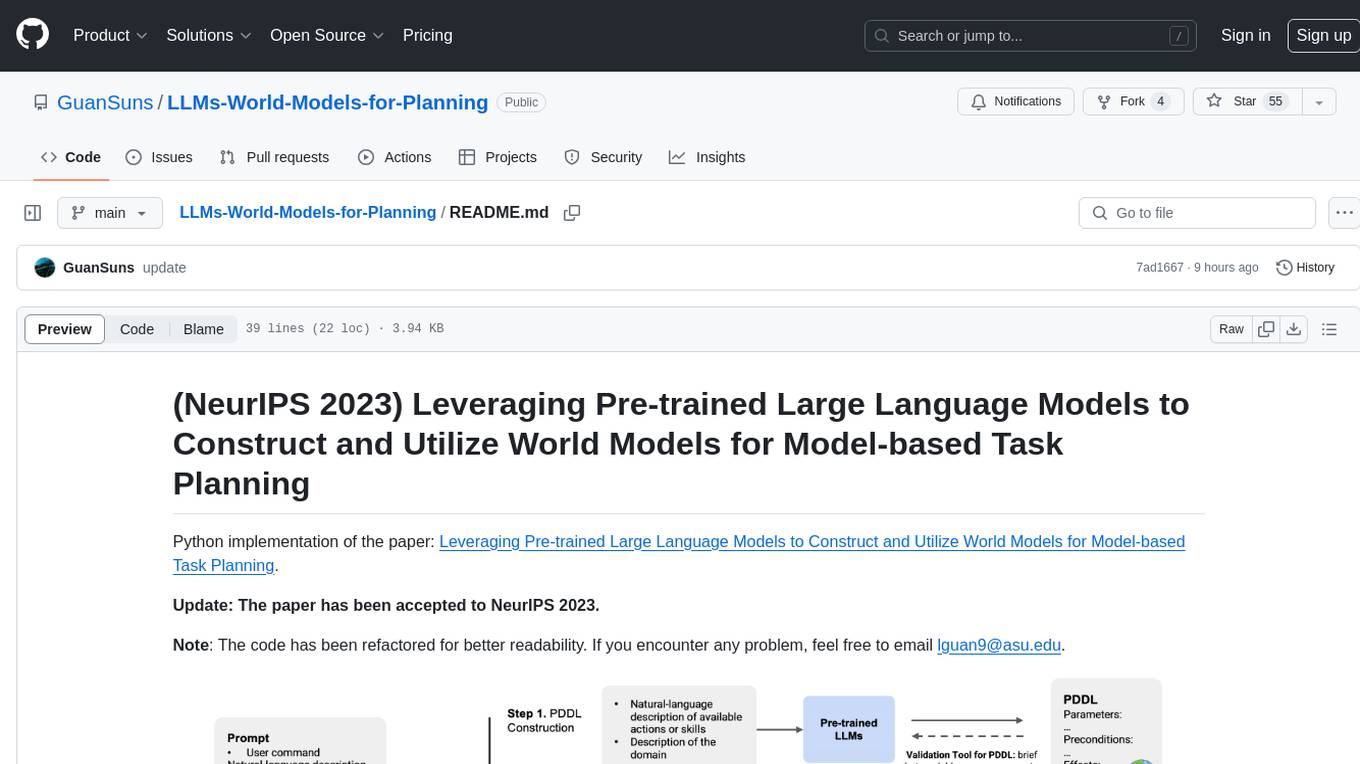
LLMs-World-Models-for-Planning
This repository provides a Python implementation of a method that leverages pre-trained large language models to construct and utilize world models for model-based task planning. It includes scripts to generate domain models using natural language descriptions, correct domain models based on feedback, and support plan generation for tasks in different domains. The code has been refactored for better readability and includes tools for validating PDDL syntax and handling corrective feedback.
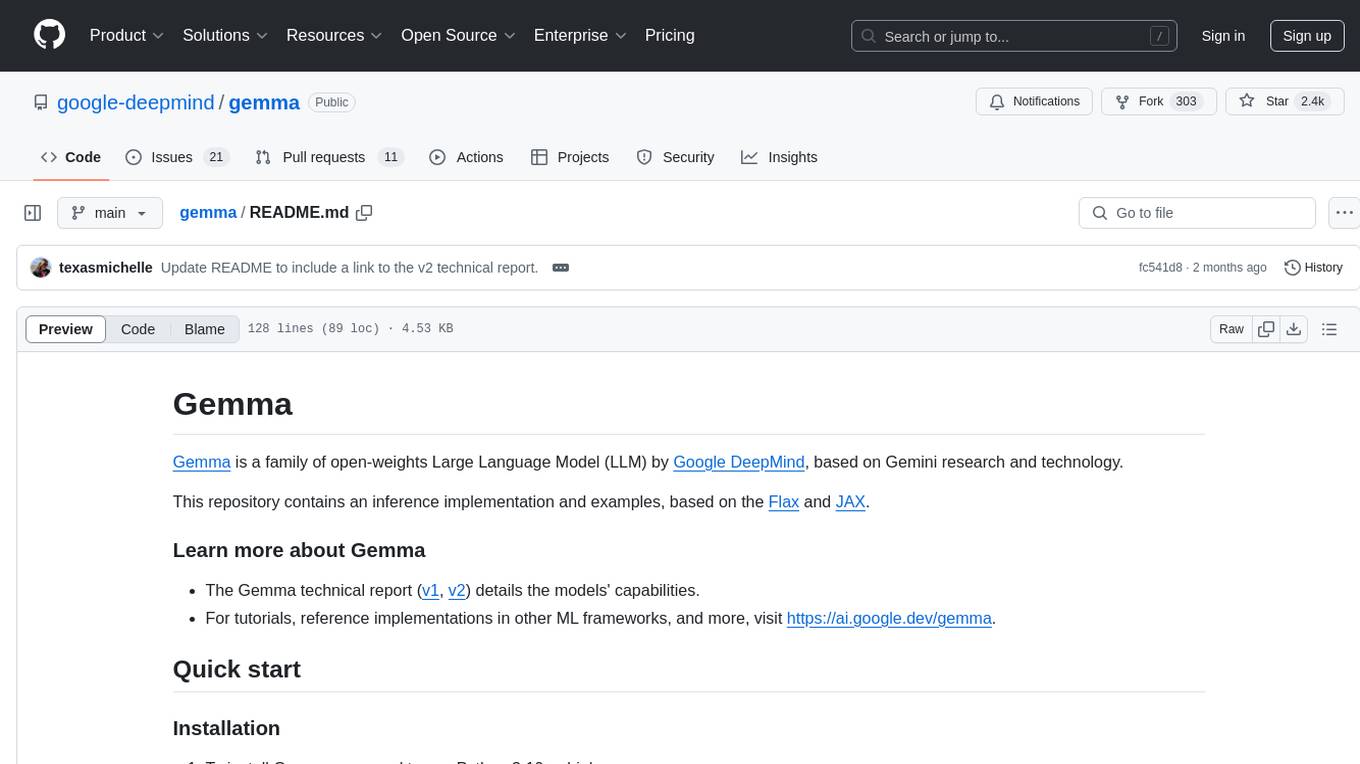
gemma
Gemma is a family of open-weights Large Language Model (LLM) by Google DeepMind, based on Gemini research and technology. This repository contains an inference implementation and examples, based on the Flax and JAX frameworks. Gemma can run on CPU, GPU, and TPU, with model checkpoints available for download. It provides tutorials, reference implementations, and Colab notebooks for tasks like sampling and fine-tuning. Users can contribute to Gemma through bug reports and pull requests. The code is licensed under the Apache License, Version 2.0.
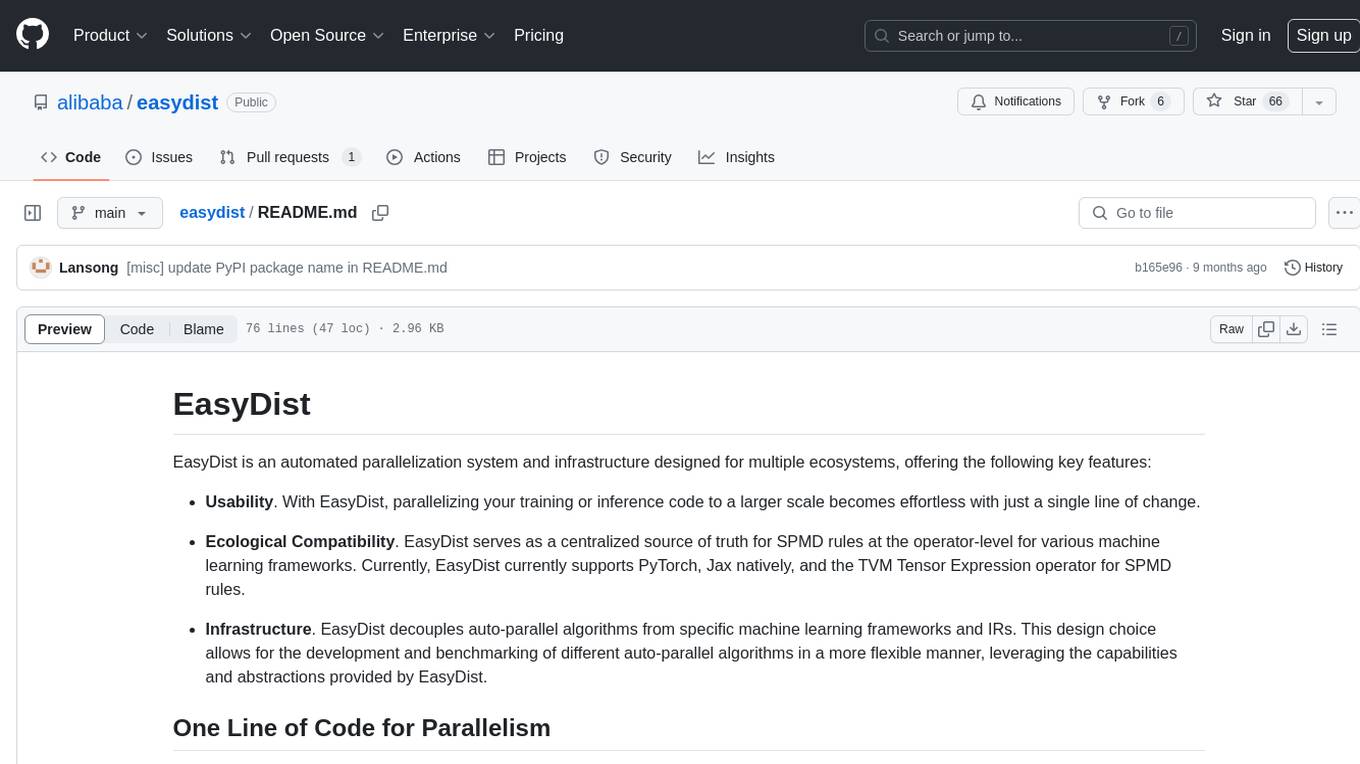
easydist
EasyDist is an automated parallelization system and infrastructure designed for multiple ecosystems. It offers usability by making parallelizing training or inference code effortless with just a single line of change. It ensures ecological compatibility by serving as a centralized source of truth for SPMD rules at the operator-level for various machine learning frameworks. EasyDist decouples auto-parallel algorithms from specific frameworks and IRs, allowing for the development and benchmarking of different auto-parallel algorithms in a flexible manner. The architecture includes MetaOp, MetaIR, and the ShardCombine Algorithm for SPMD sharding rules without manual annotations.
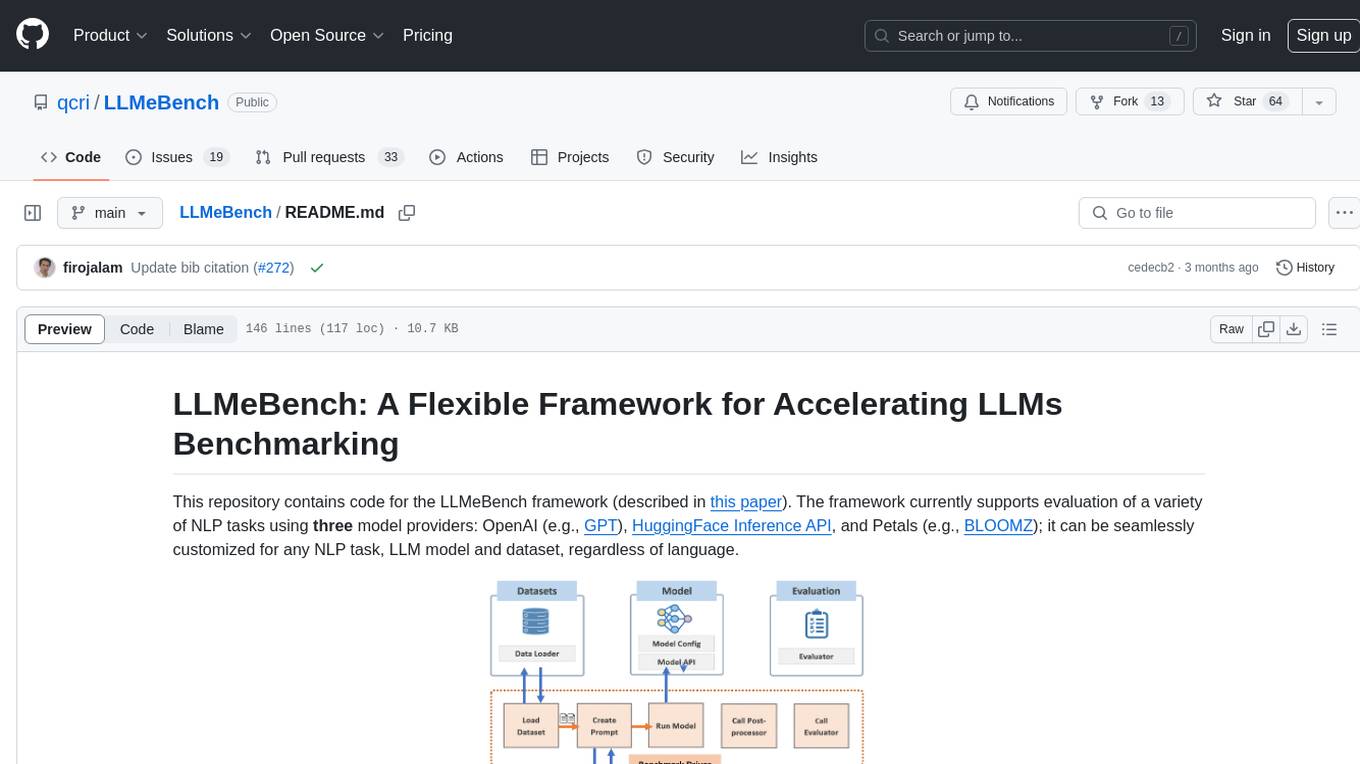
LLMeBench
LLMeBench is a flexible framework designed for accelerating benchmarking of Large Language Models (LLMs) in the field of Natural Language Processing (NLP). It supports evaluation of various NLP tasks using model providers like OpenAI, HuggingFace Inference API, and Petals. The framework is customizable for different NLP tasks, LLM models, and datasets across multiple languages. It features extensive caching capabilities, supports zero- and few-shot learning paradigms, and allows on-the-fly dataset download and caching. LLMeBench is open-source and continuously expanding to support new models accessible through APIs.
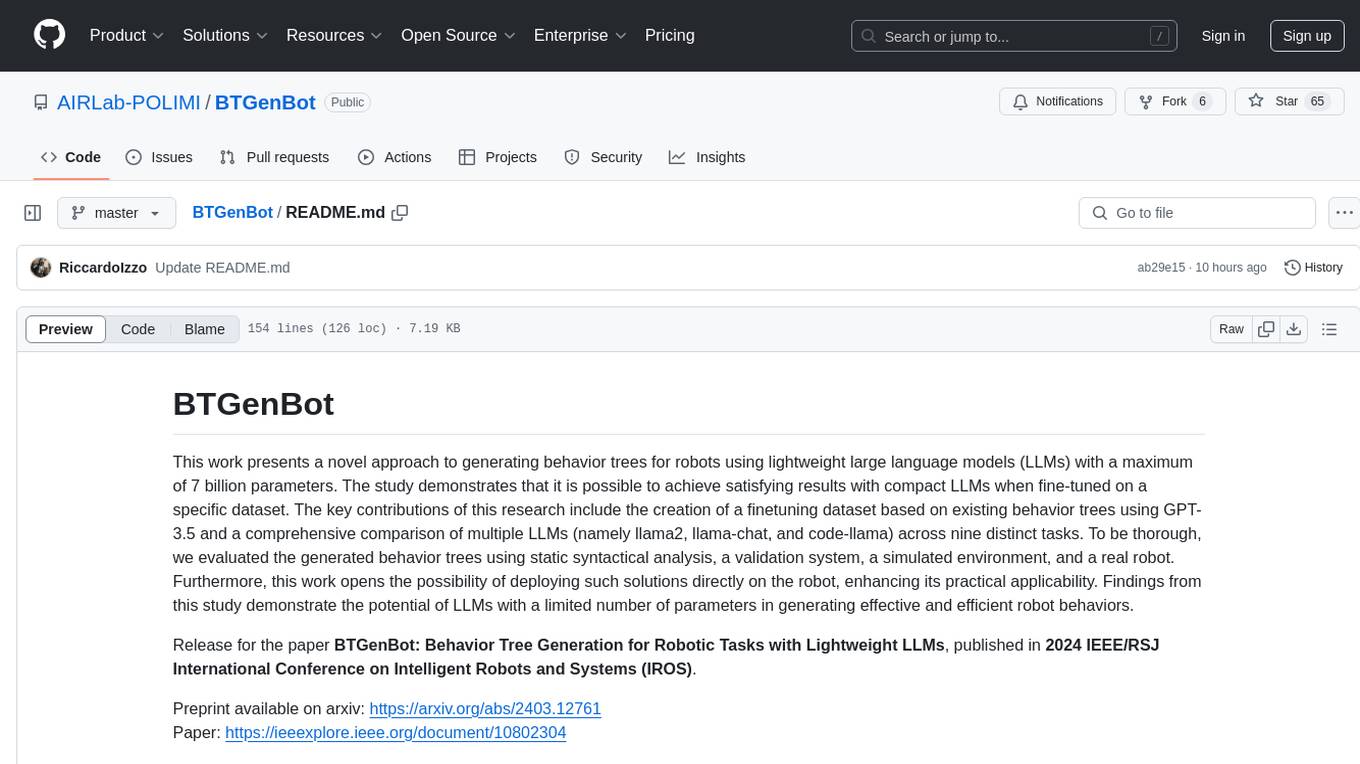
BTGenBot
BTGenBot is a tool that generates behavior trees for robots using lightweight large language models (LLMs) with a maximum of 7 billion parameters. It fine-tunes on a specific dataset, compares multiple LLMs, and evaluates generated behavior trees using various methods. The tool demonstrates the potential of LLMs with a limited number of parameters in creating effective and efficient robot behaviors.
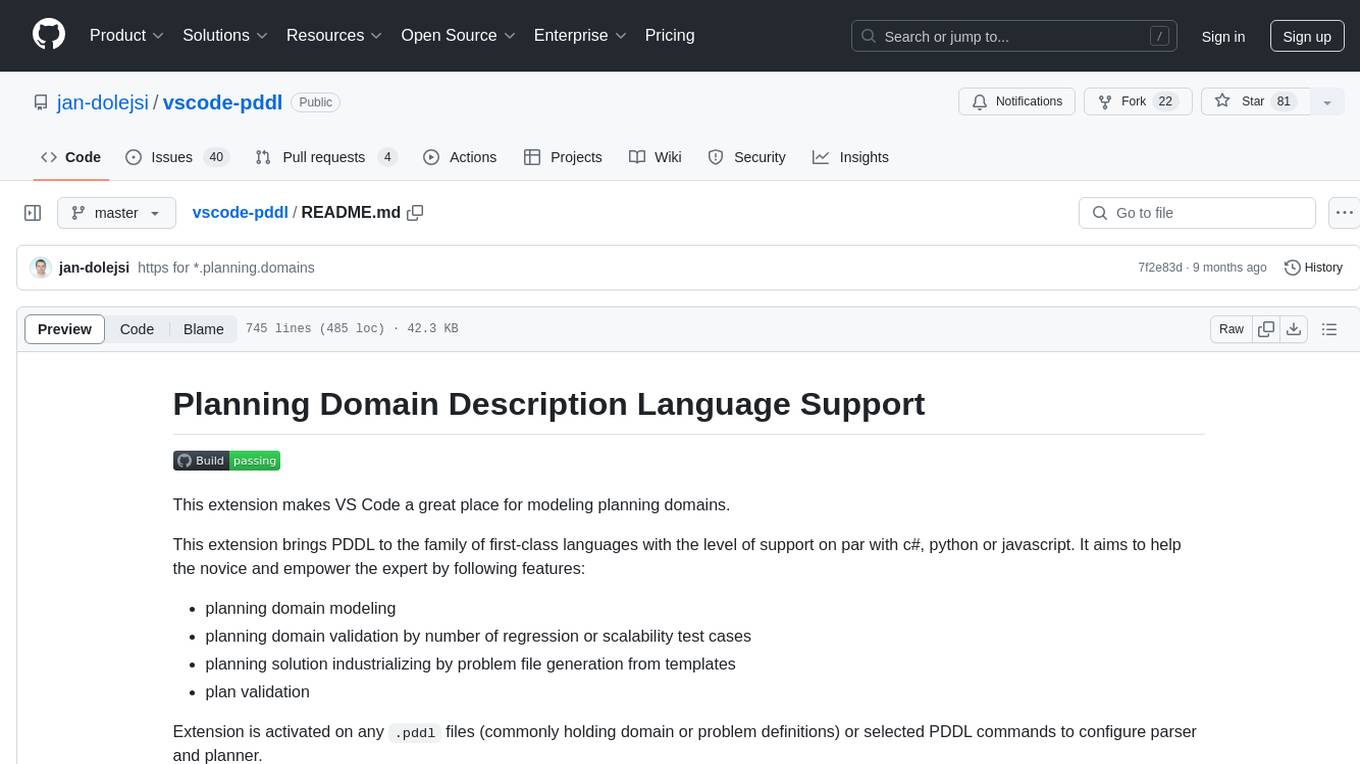
vscode-pddl
The vscode-pddl extension provides comprehensive support for Planning Domain Description Language (PDDL) in Visual Studio Code. It enables users to model planning domains, validate them, industrialize planning solutions, and run planners. The extension offers features like syntax highlighting, auto-completion, plan visualization, plan validation, plan happenings evaluation, search debugging, and integration with Planning.Domains. Users can create PDDL files, run planners, visualize plans, and debug search algorithms efficiently within VS Code.
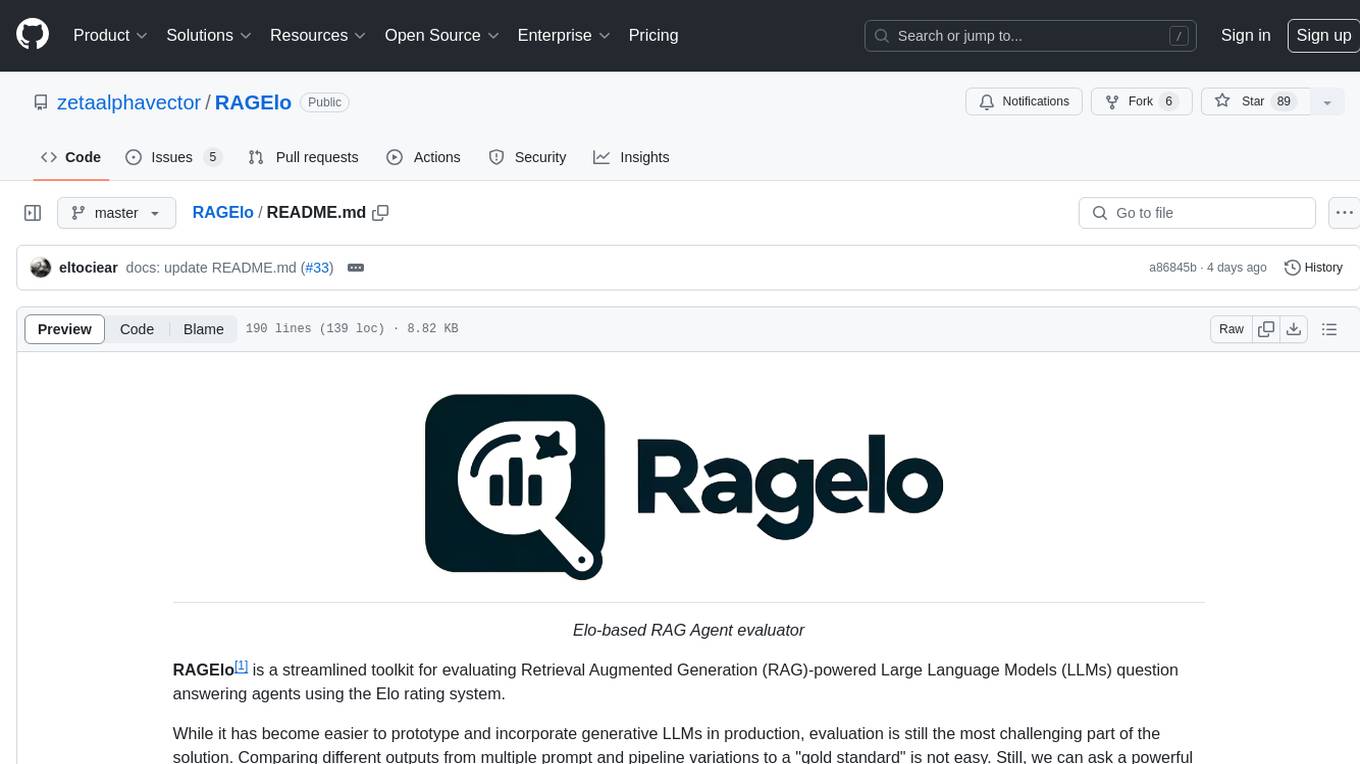
RAGElo
RAGElo is a streamlined toolkit for evaluating Retrieval Augmented Generation (RAG)-powered Large Language Models (LLMs) question answering agents using the Elo rating system. It simplifies the process of comparing different outputs from multiple prompt and pipeline variations to a 'gold standard' by allowing a powerful LLM to judge between pairs of answers and questions. RAGElo conducts tournament-style Elo ranking of LLM outputs, providing insights into the effectiveness of different settings.
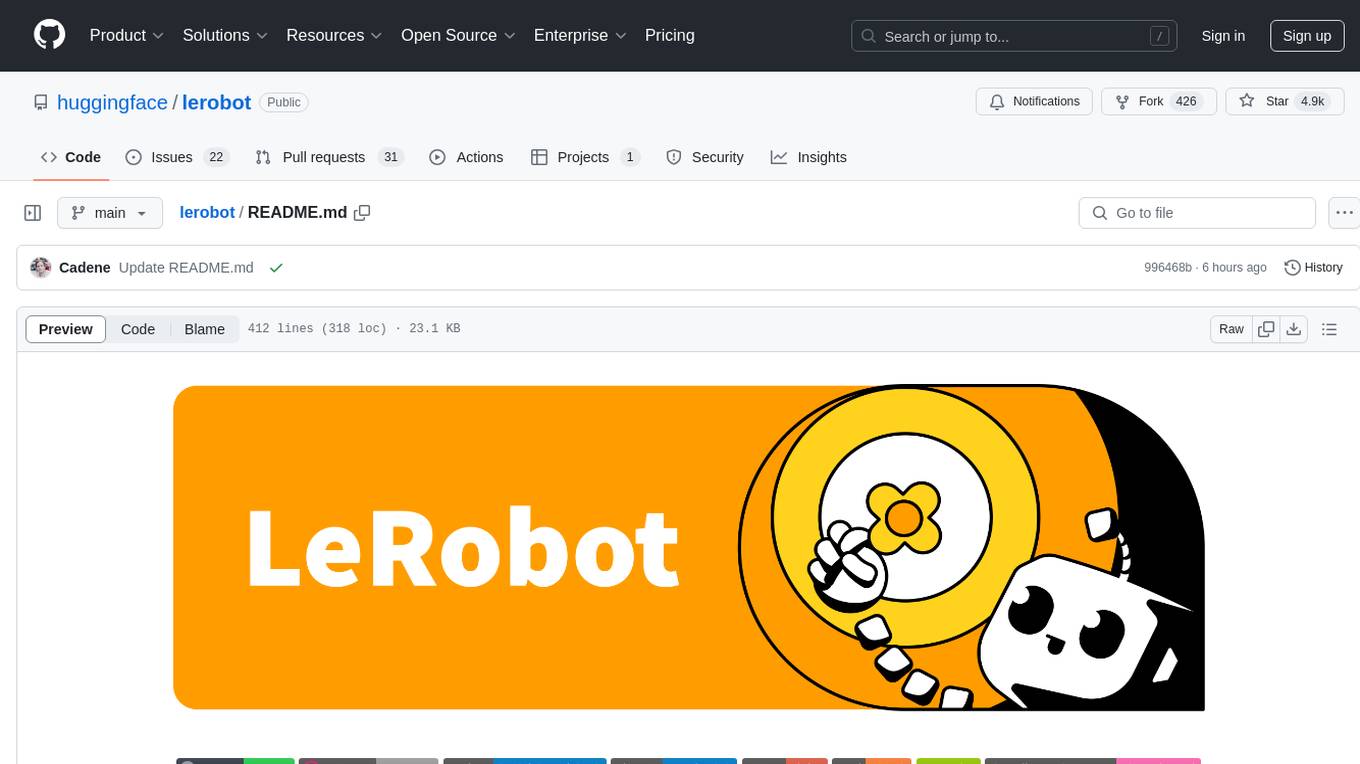
lerobot
LeRobot is a state-of-the-art AI library for real-world robotics in PyTorch. It aims to provide models, datasets, and tools to lower the barrier to entry to robotics, focusing on imitation learning and reinforcement learning. LeRobot offers pretrained models, datasets with human-collected demonstrations, and simulation environments. It plans to support real-world robotics on affordable and capable robots. The library hosts pretrained models and datasets on the Hugging Face community page.
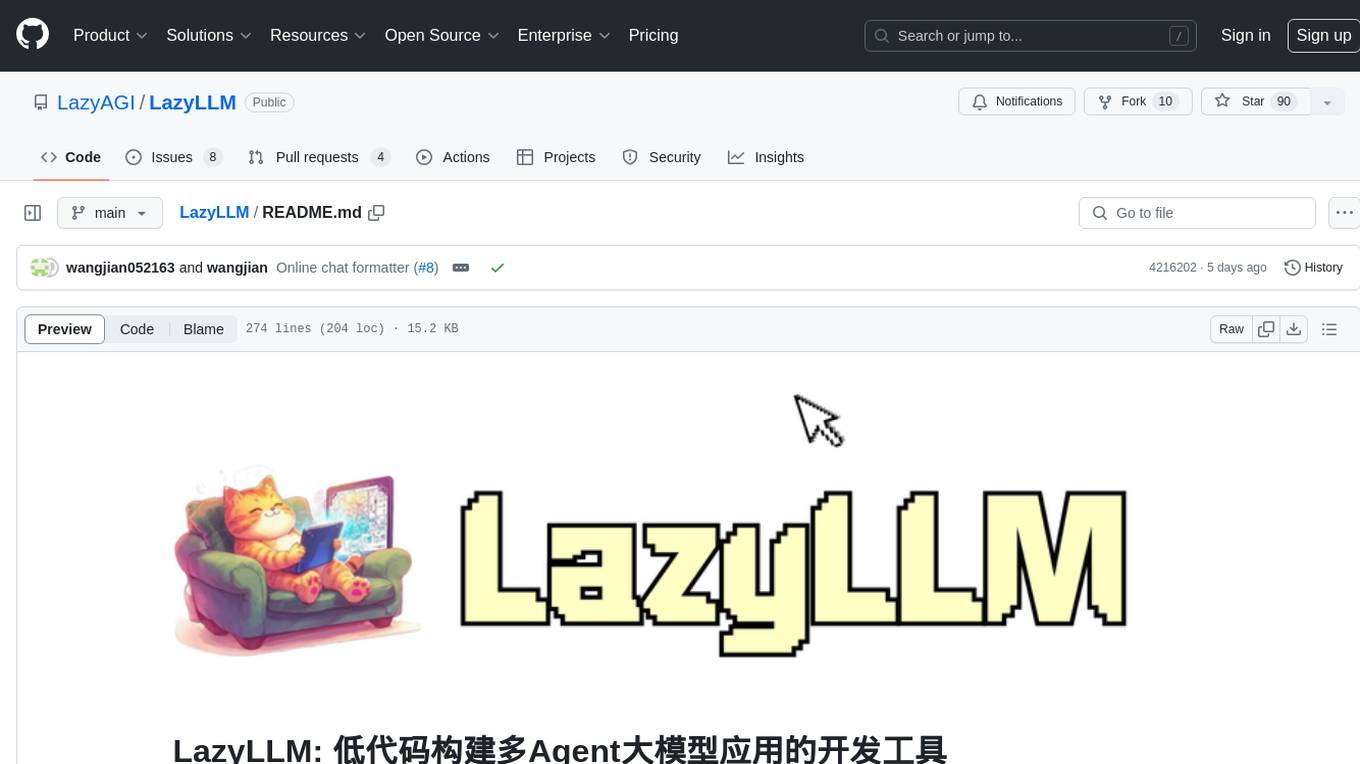
LazyLLM
LazyLLM is a low-code development tool for building complex AI applications with multiple agents. It assists developers in building AI applications at a low cost and continuously optimizing their performance. The tool provides a convenient workflow for application development and offers standard processes and tools for various stages of application development. Users can quickly prototype applications with LazyLLM, analyze bad cases with scenario task data, and iteratively optimize key components to enhance the overall application performance. LazyLLM aims to simplify the AI application development process and provide flexibility for both beginners and experts to create high-quality applications.
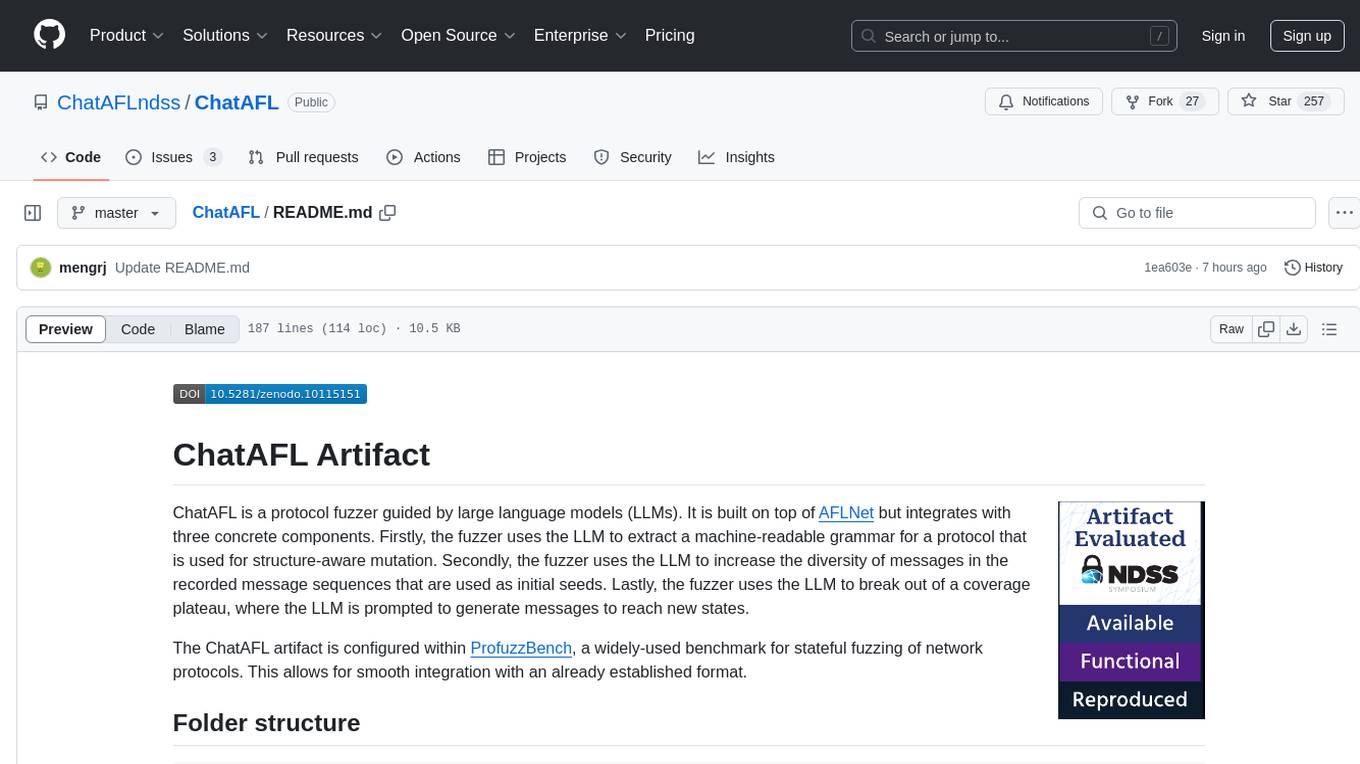
ChatAFL
ChatAFL is a protocol fuzzer guided by large language models (LLMs) that extracts machine-readable grammar for protocol mutation, increases message diversity, and breaks coverage plateaus. It integrates with ProfuzzBench for stateful fuzzing of network protocols, providing smooth integration. The artifact includes modified versions of AFLNet and ProfuzzBench, source code for ChatAFL with proposed strategies, and scripts for setup, execution, analysis, and cleanup. Users can analyze data, construct plots, examine LLM-generated grammars, enriched seeds, and state-stall responses, and reproduce results with downsized experiments. Customization options include modifying fuzzers, tuning parameters, adding new subjects, troubleshooting, and working on GPT-4. Limitations include interaction with OpenAI's Large Language Models and a hard limit of 150,000 tokens per minute.
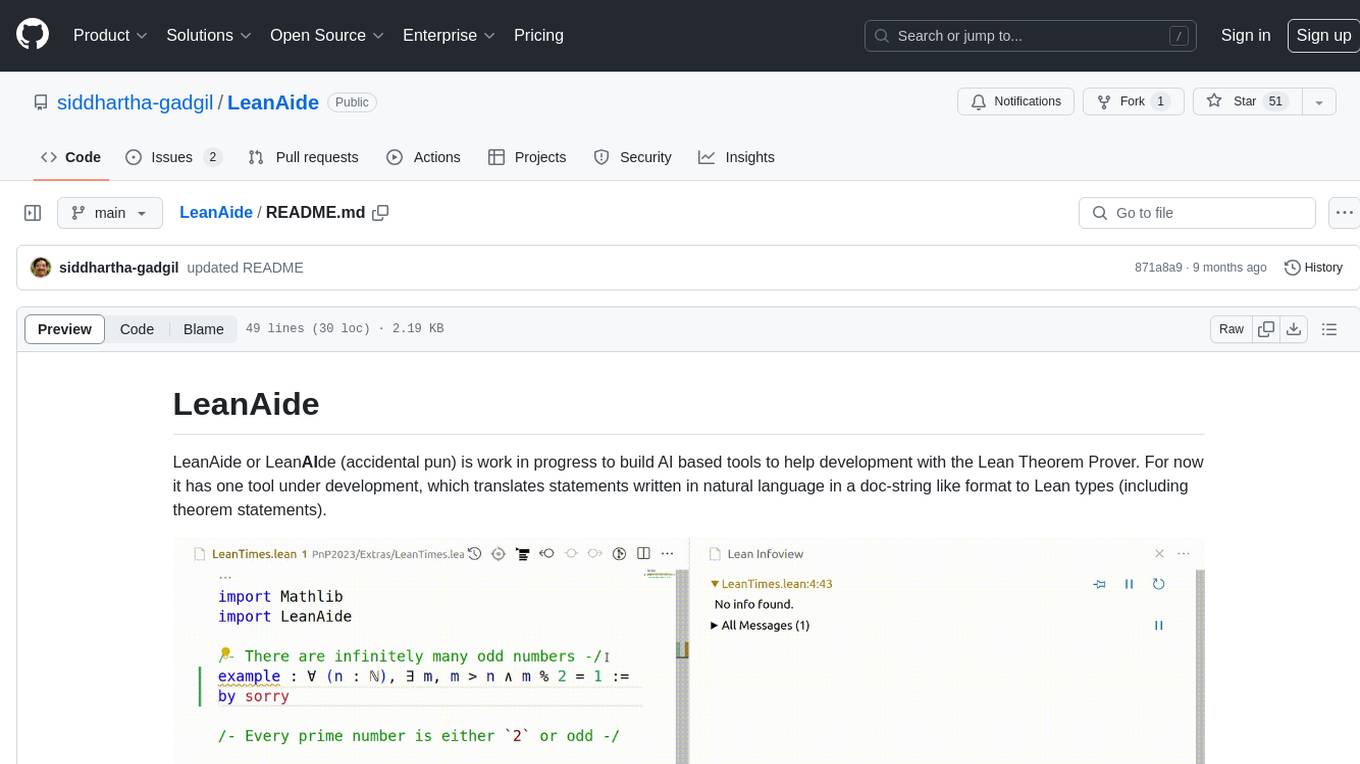
LeanAide
LeanAide is a work in progress AI tool designed to assist with development using the Lean Theorem Prover. It currently offers a tool that translates natural language statements to Lean types, including theorem statements. The tool is based on GPT 3.5-turbo/GPT 4 and requires an OpenAI key for usage. Users can include LeanAide as a dependency in their projects to access the translation functionality.
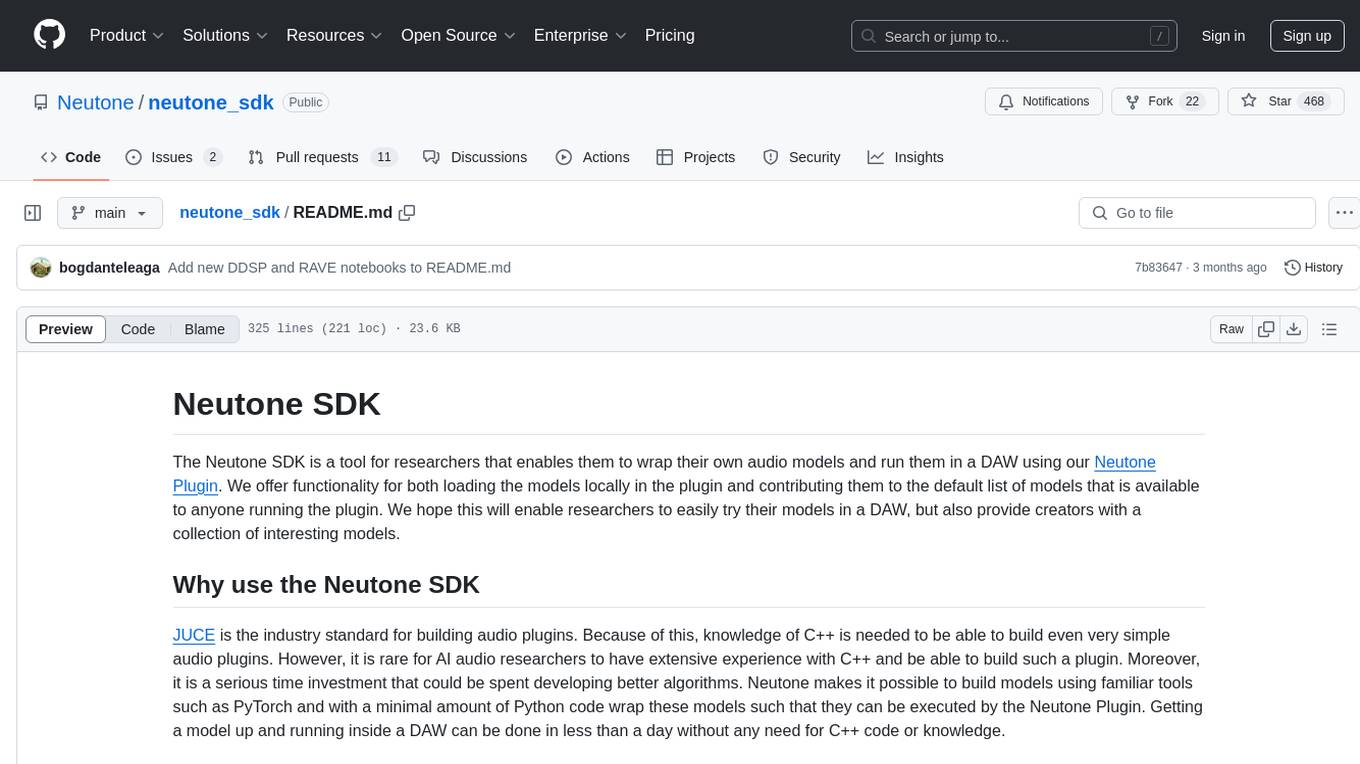
neutone_sdk
The Neutone SDK is a tool designed for researchers to wrap their own audio models and run them in a DAW using the Neutone Plugin. It simplifies the process by allowing models to be built using PyTorch and minimal Python code, eliminating the need for extensive C++ knowledge. The SDK provides support for buffering inputs and outputs, sample rate conversion, and profiling tools for model performance testing. It also offers examples, notebooks, and a submission process for sharing models with the community.
For similar tasks
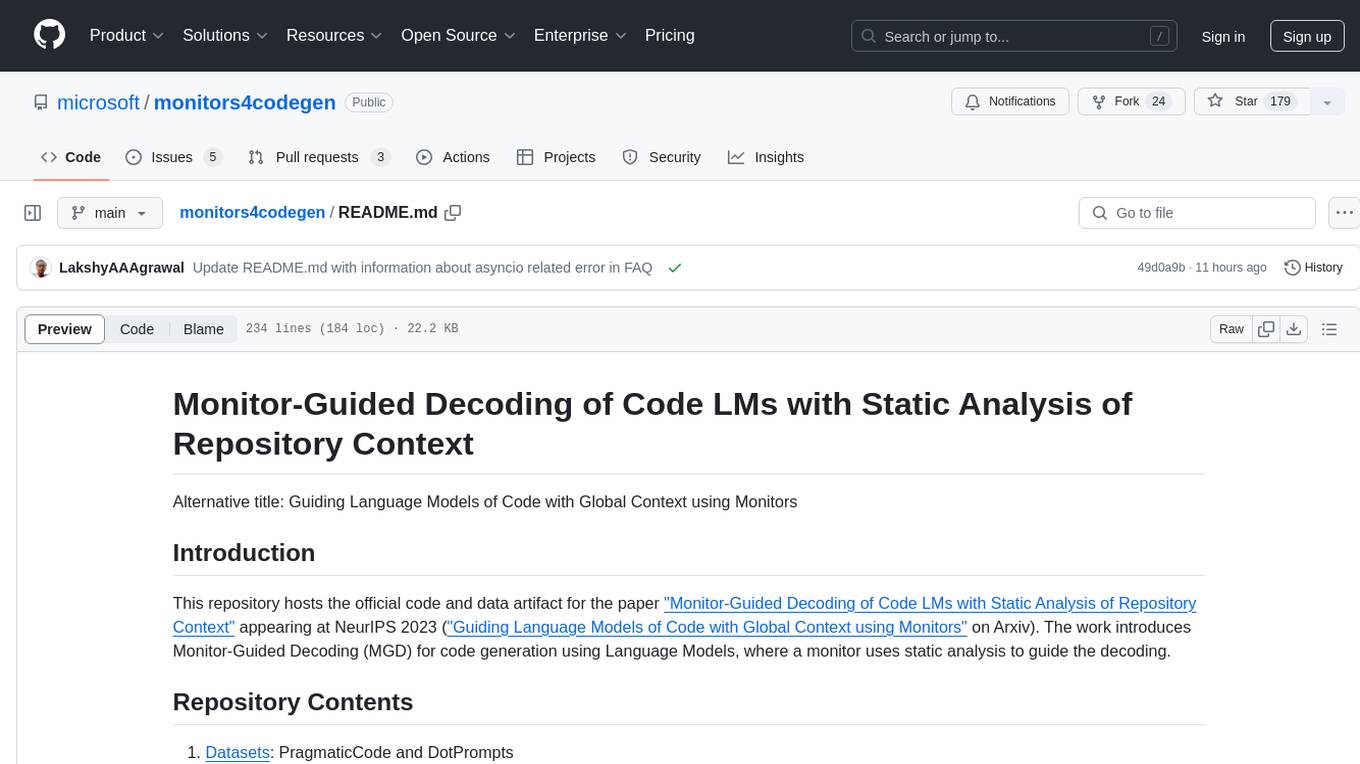
monitors4codegen
This repository hosts the official code and data artifact for the paper 'Monitor-Guided Decoding of Code LMs with Static Analysis of Repository Context'. It introduces Monitor-Guided Decoding (MGD) for code generation using Language Models, where a monitor uses static analysis to guide the decoding. The repository contains datasets, evaluation scripts, inference results, a language server client 'multilspy' for static analyses, and implementation of various monitors monitoring for different properties in 3 programming languages. The monitors guide Language Models to adhere to properties like valid identifier dereferences, correct number of arguments to method calls, typestate validity of method call sequences, and more.
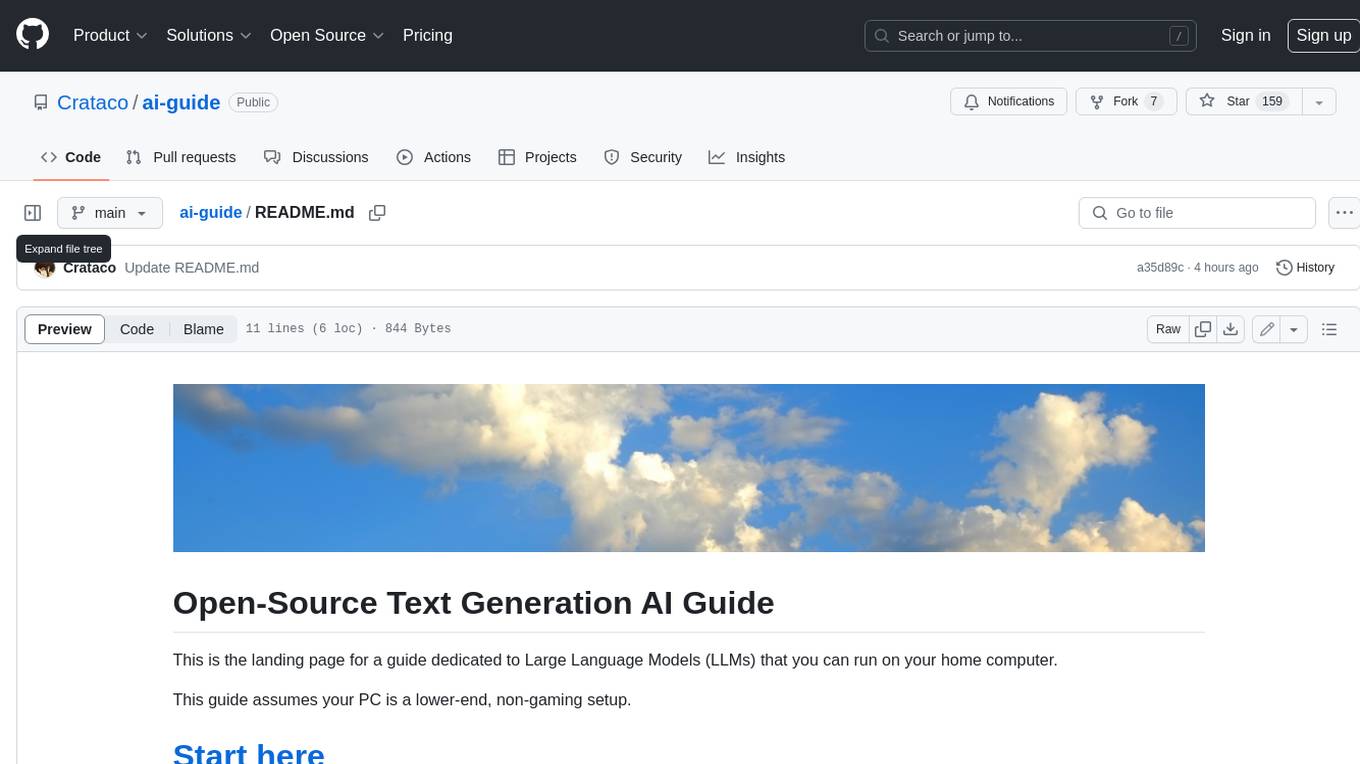
ai-guide
This guide is dedicated to Large Language Models (LLMs) that you can run on your home computer. It assumes your PC is a lower-end, non-gaming setup.
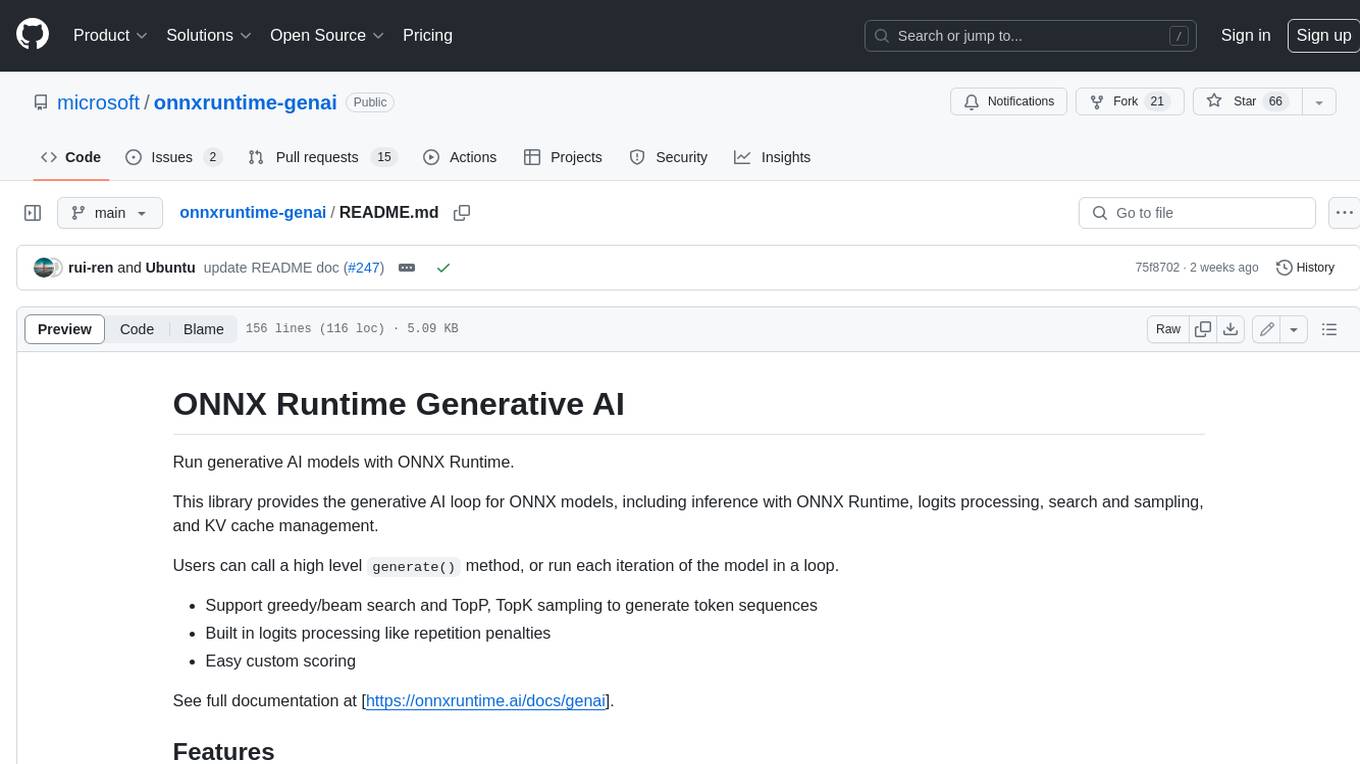
onnxruntime-genai
ONNX Runtime Generative AI is a library that provides the generative AI loop for ONNX models, including inference with ONNX Runtime, logits processing, search and sampling, and KV cache management. Users can call a high level `generate()` method, or run each iteration of the model in a loop. It supports greedy/beam search and TopP, TopK sampling to generate token sequences, has built in logits processing like repetition penalties, and allows for easy custom scoring.
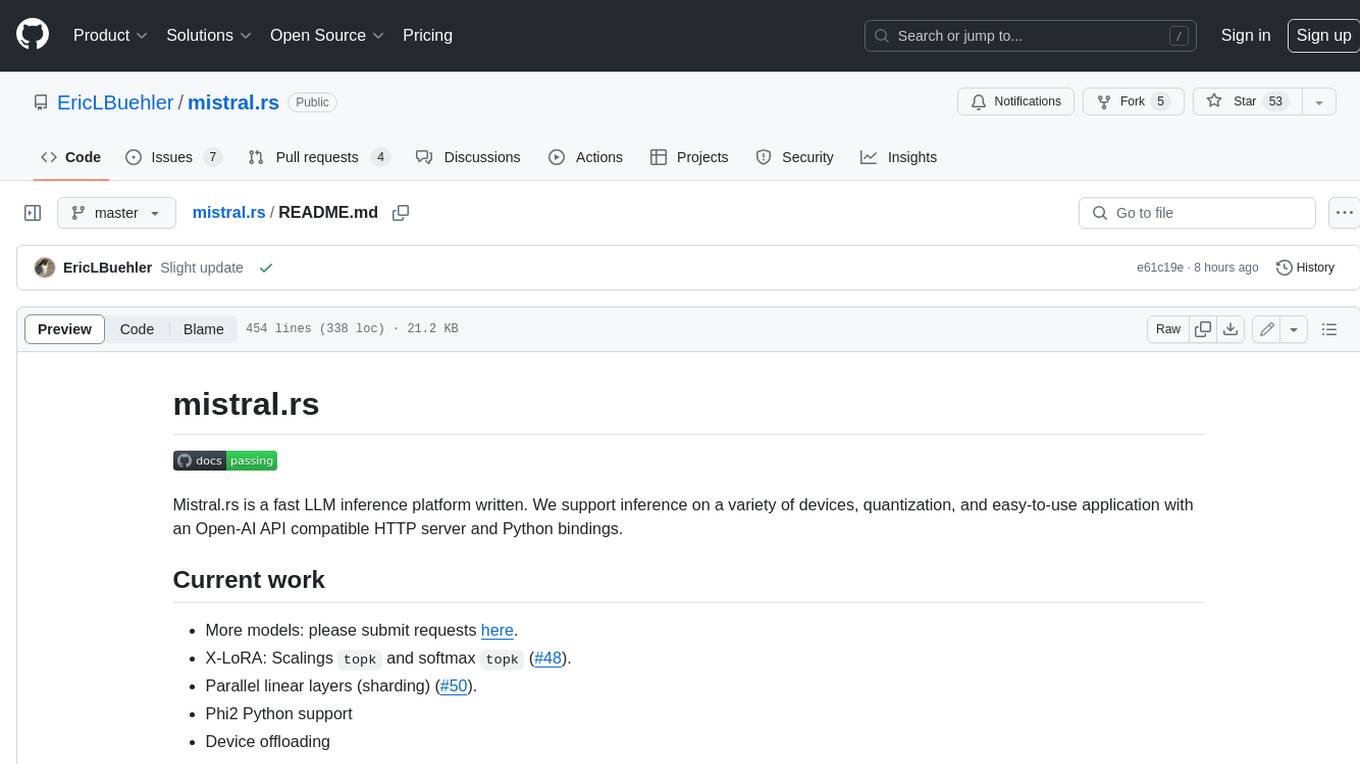
mistral.rs
Mistral.rs is a fast LLM inference platform written in Rust. We support inference on a variety of devices, quantization, and easy-to-use application with an Open-AI API compatible HTTP server and Python bindings.
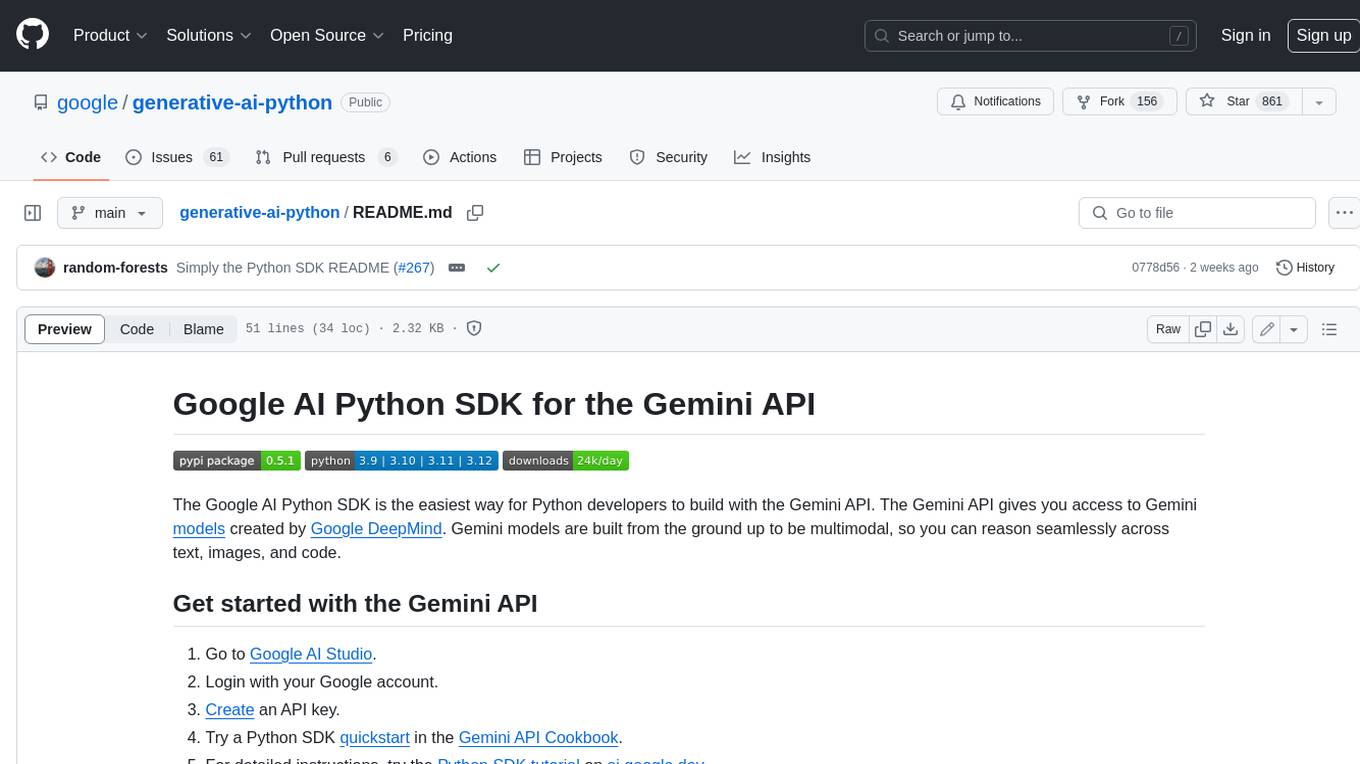
generative-ai-python
The Google AI Python SDK is the easiest way for Python developers to build with the Gemini API. The Gemini API gives you access to Gemini models created by Google DeepMind. Gemini models are built from the ground up to be multimodal, so you can reason seamlessly across text, images, and code.
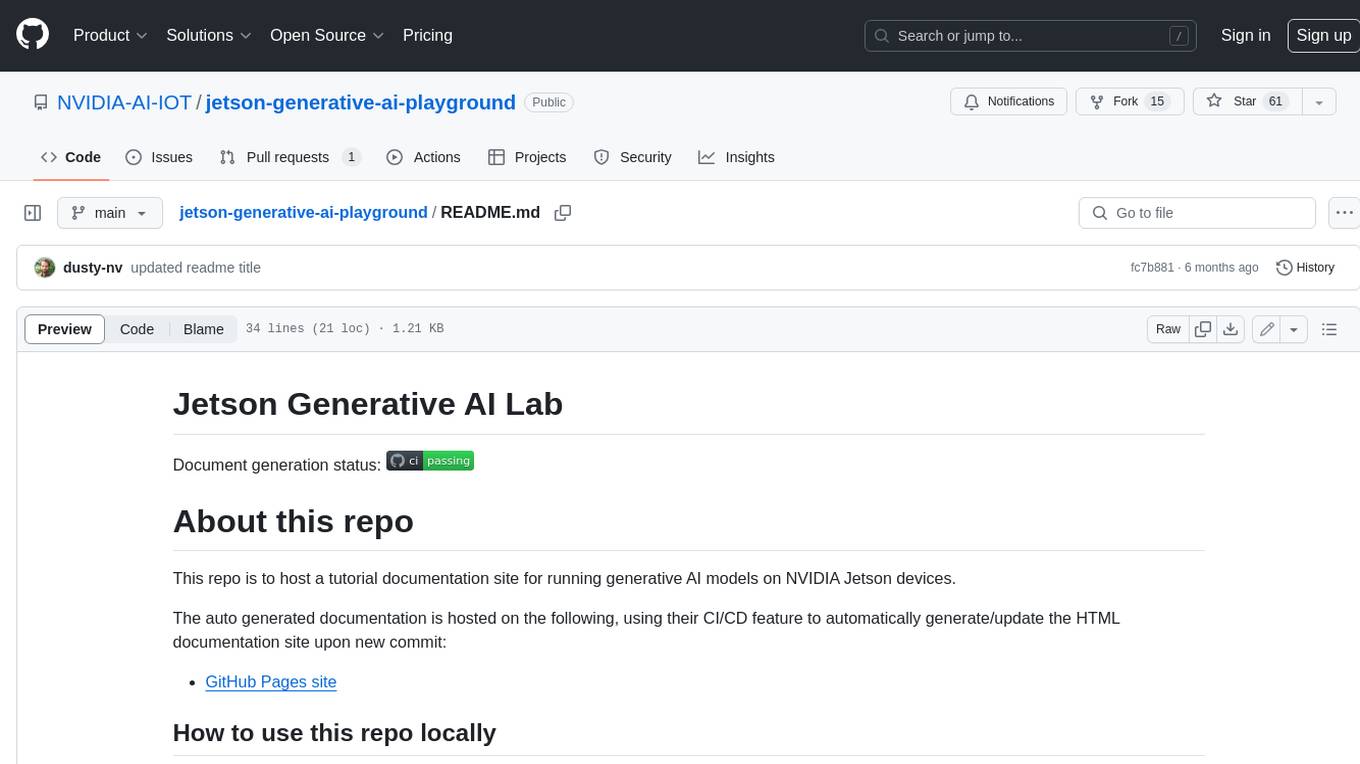
jetson-generative-ai-playground
This repo hosts tutorial documentation for running generative AI models on NVIDIA Jetson devices. The documentation is auto-generated and hosted on GitHub Pages using their CI/CD feature to automatically generate/update the HTML documentation site upon new commits.
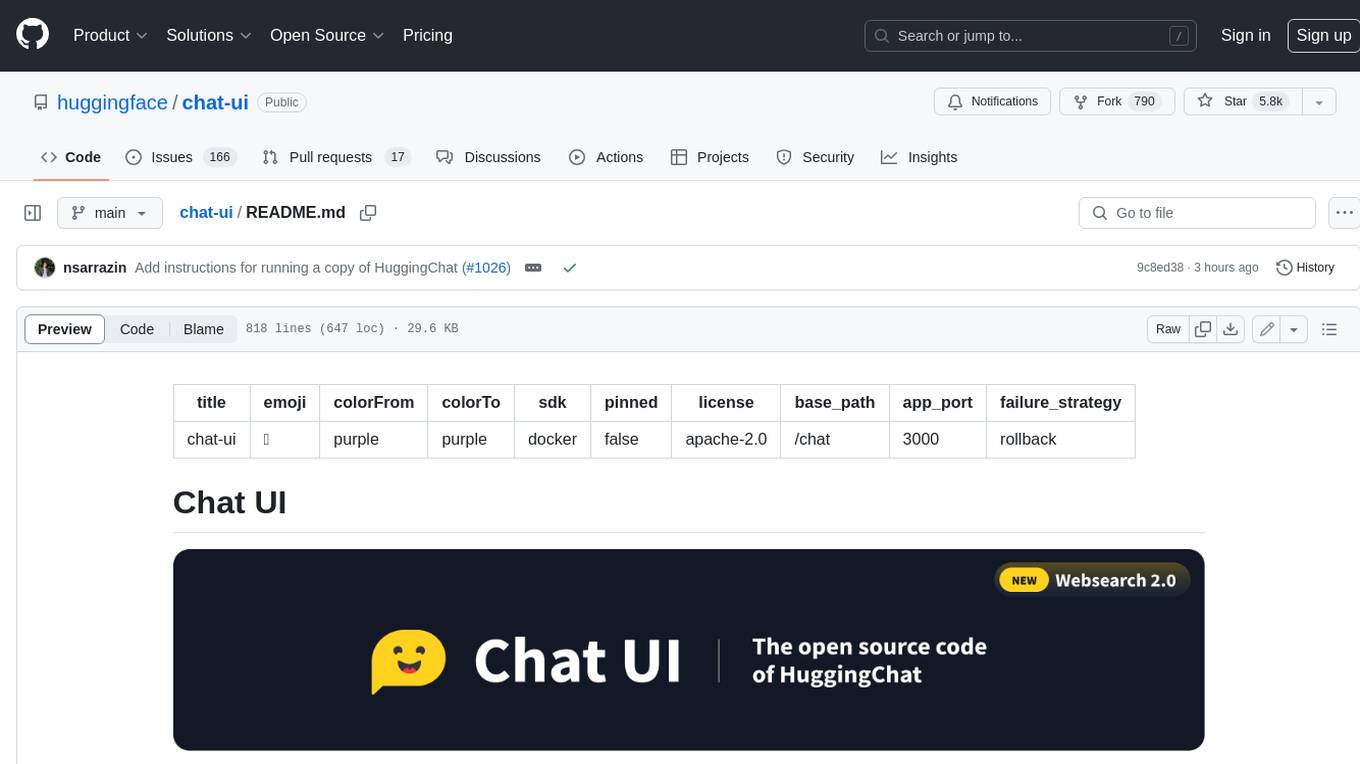
chat-ui
A chat interface using open source models, eg OpenAssistant or Llama. It is a SvelteKit app and it powers the HuggingChat app on hf.co/chat.
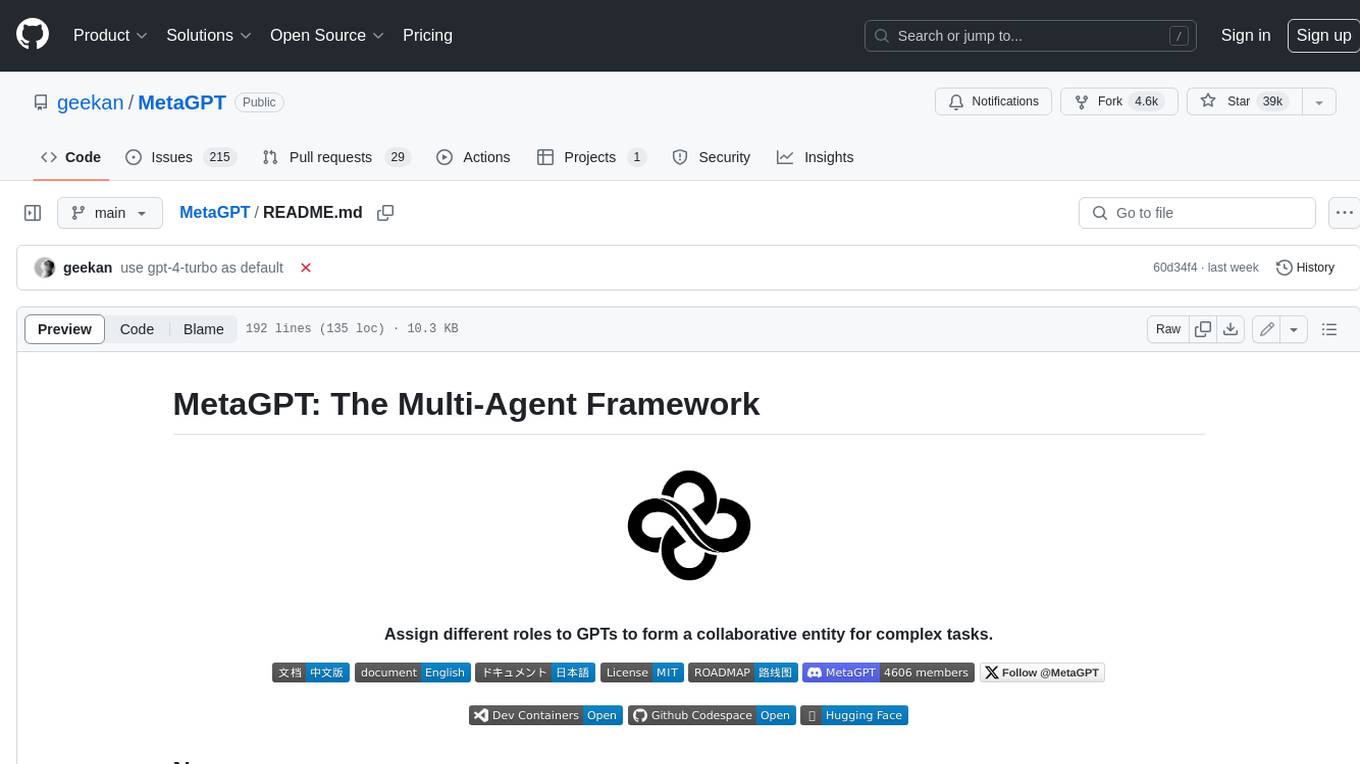
MetaGPT
MetaGPT is a multi-agent framework that enables GPT to work in a software company, collaborating to tackle more complex tasks. It assigns different roles to GPTs to form a collaborative entity for complex tasks. MetaGPT takes a one-line requirement as input and outputs user stories, competitive analysis, requirements, data structures, APIs, documents, etc. Internally, MetaGPT includes product managers, architects, project managers, and engineers. It provides the entire process of a software company along with carefully orchestrated SOPs. MetaGPT's core philosophy is "Code = SOP(Team)", materializing SOP and applying it to teams composed of LLMs.
For similar jobs
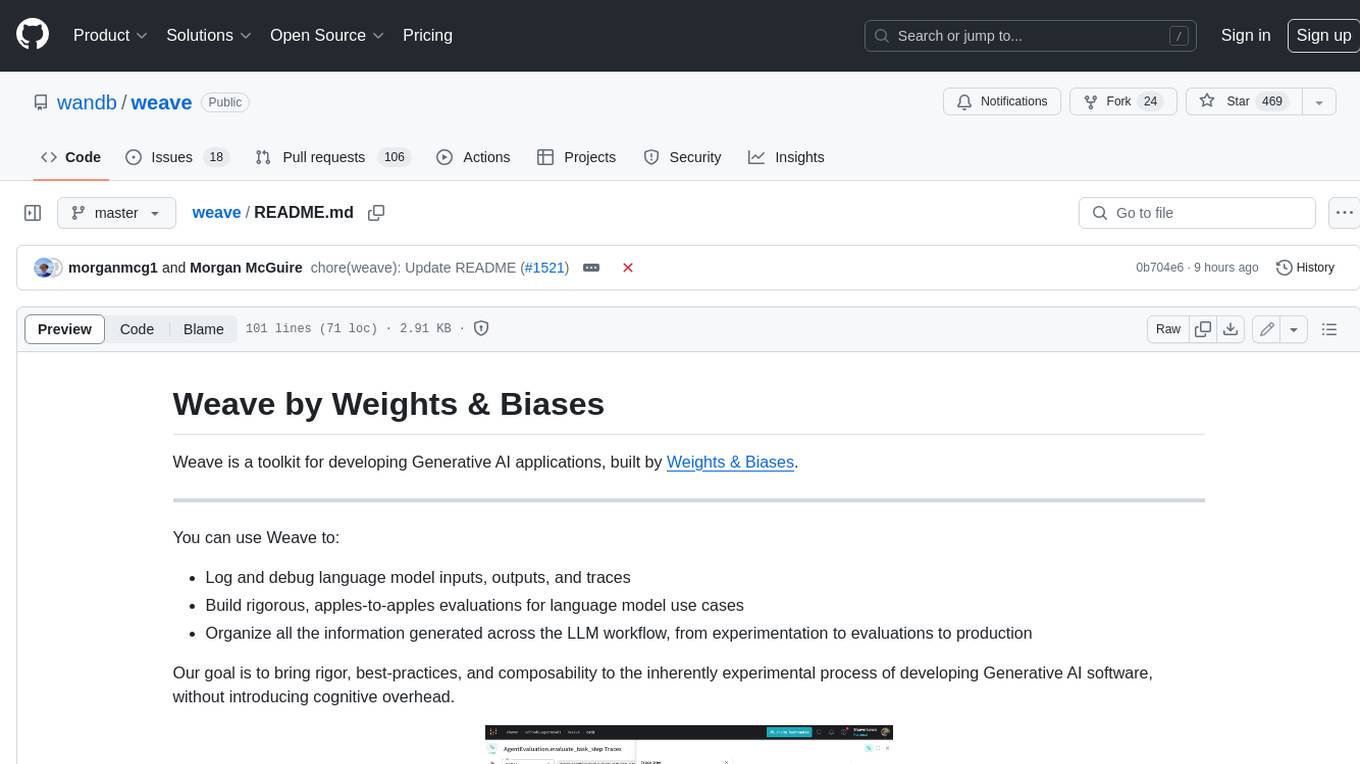
weave
Weave is a toolkit for developing Generative AI applications, built by Weights & Biases. With Weave, you can log and debug language model inputs, outputs, and traces; build rigorous, apples-to-apples evaluations for language model use cases; and organize all the information generated across the LLM workflow, from experimentation to evaluations to production. Weave aims to bring rigor, best-practices, and composability to the inherently experimental process of developing Generative AI software, without introducing cognitive overhead.
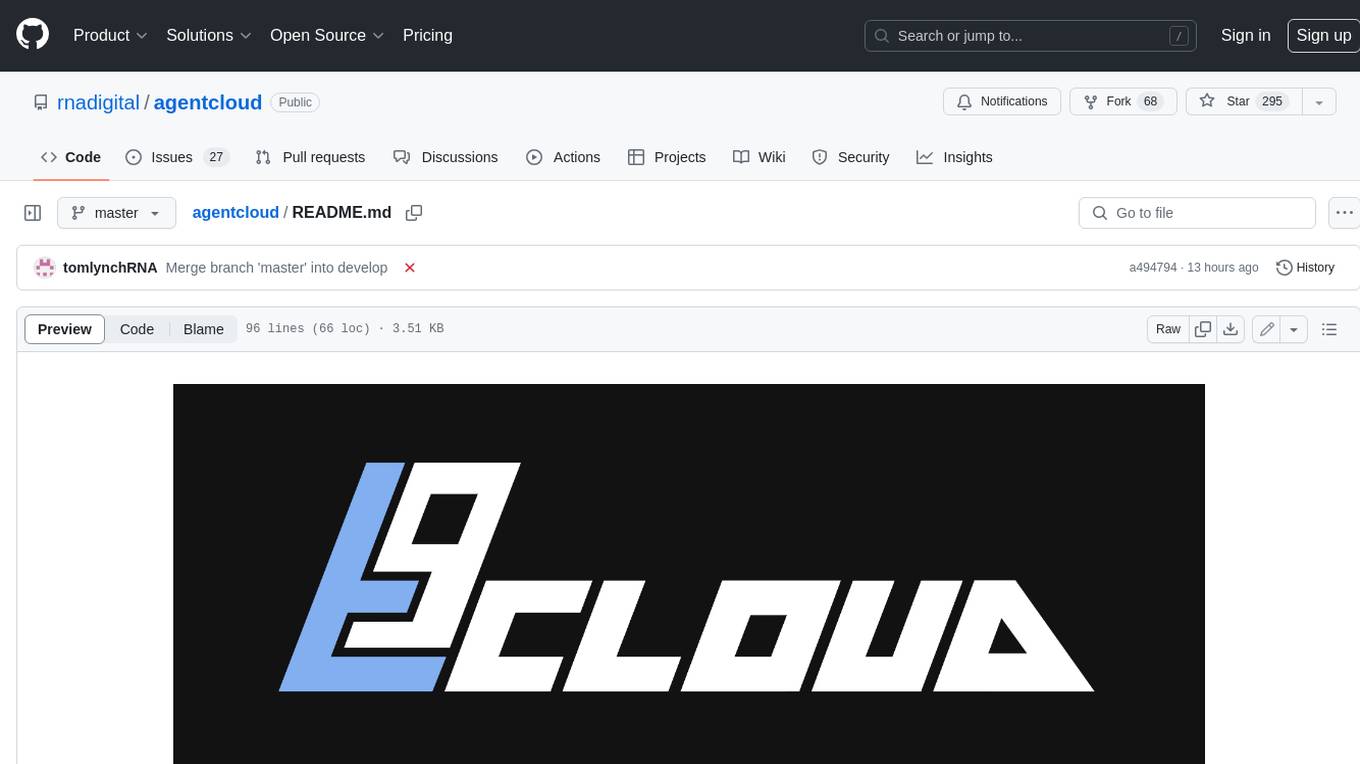
agentcloud
AgentCloud is an open-source platform that enables companies to build and deploy private LLM chat apps, empowering teams to securely interact with their data. It comprises three main components: Agent Backend, Webapp, and Vector Proxy. To run this project locally, clone the repository, install Docker, and start the services. The project is licensed under the GNU Affero General Public License, version 3 only. Contributions and feedback are welcome from the community.
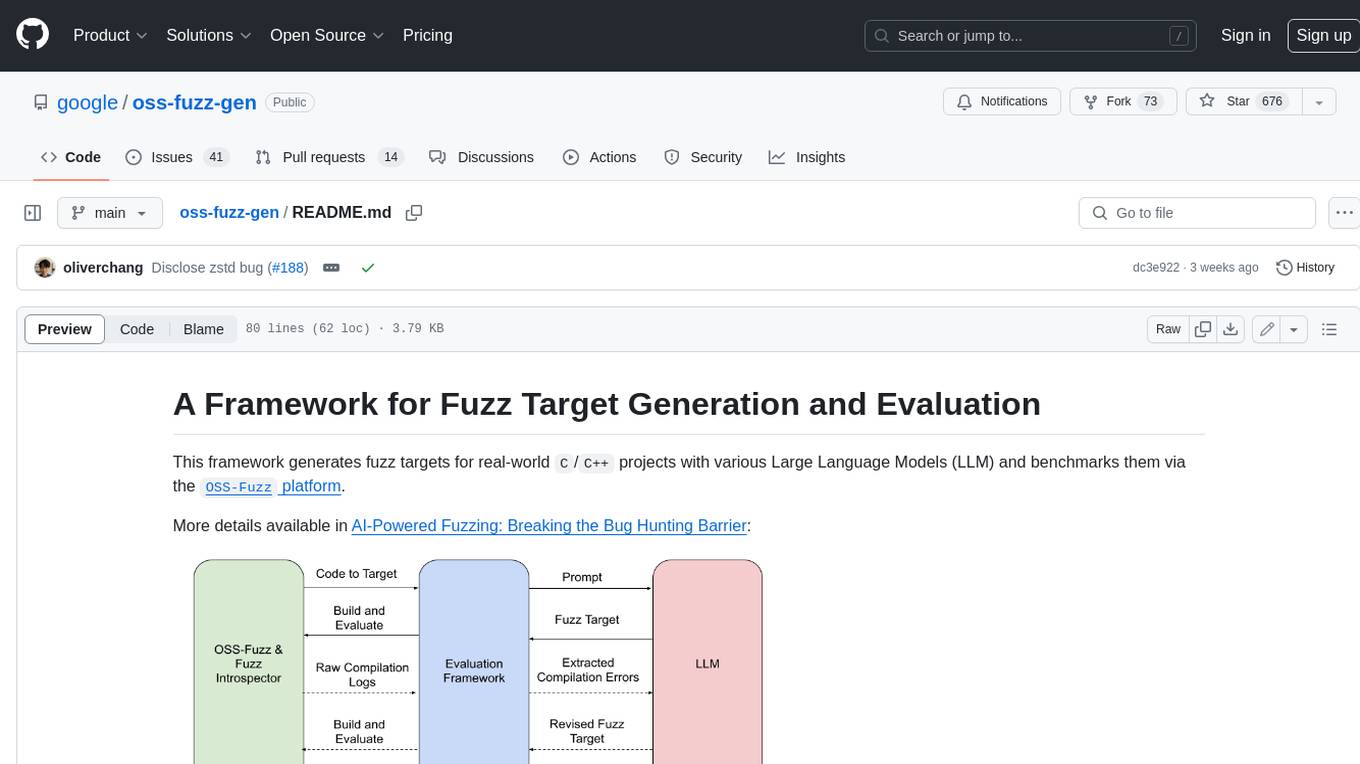
oss-fuzz-gen
This framework generates fuzz targets for real-world `C`/`C++` projects with various Large Language Models (LLM) and benchmarks them via the `OSS-Fuzz` platform. It manages to successfully leverage LLMs to generate valid fuzz targets (which generate non-zero coverage increase) for 160 C/C++ projects. The maximum line coverage increase is 29% from the existing human-written targets.
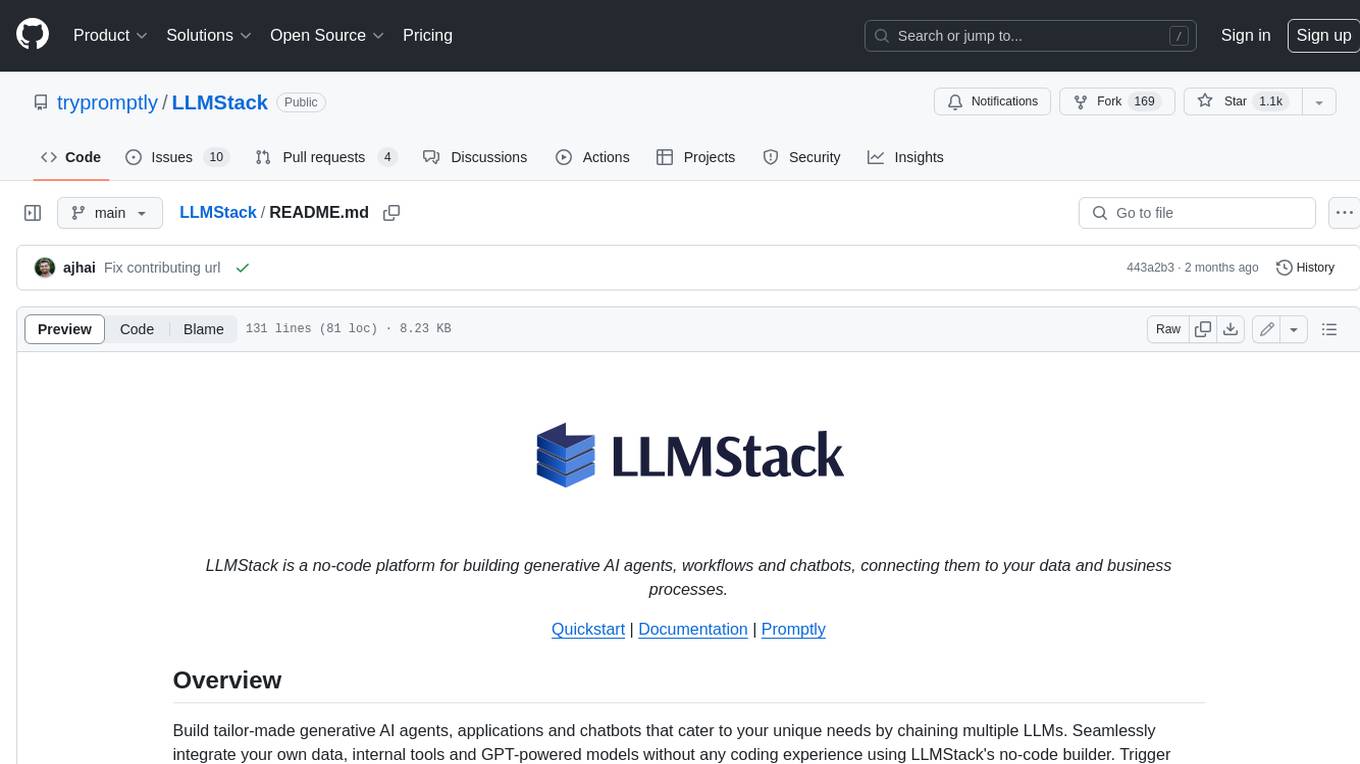
LLMStack
LLMStack is a no-code platform for building generative AI agents, workflows, and chatbots. It allows users to connect their own data, internal tools, and GPT-powered models without any coding experience. LLMStack can be deployed to the cloud or on-premise and can be accessed via HTTP API or triggered from Slack or Discord.
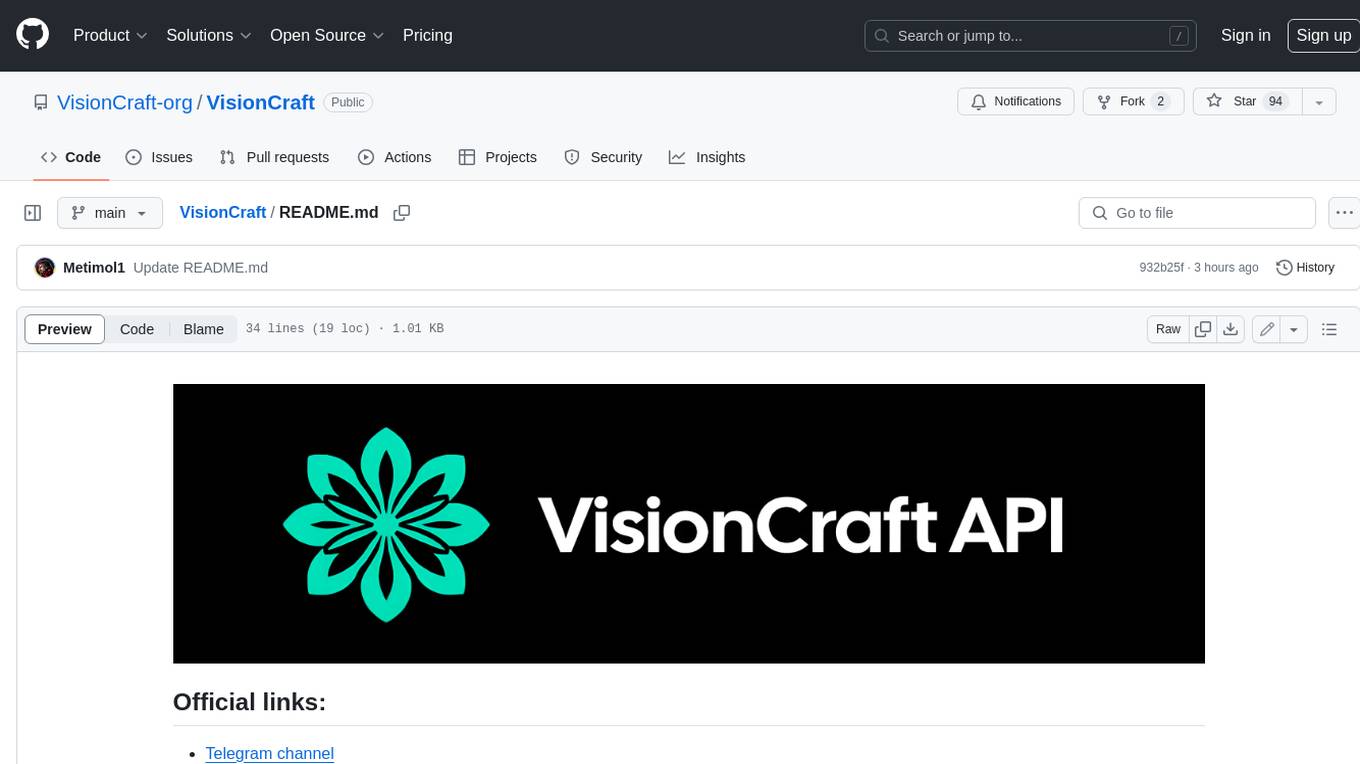
VisionCraft
The VisionCraft API is a free API for using over 100 different AI models. From images to sound.
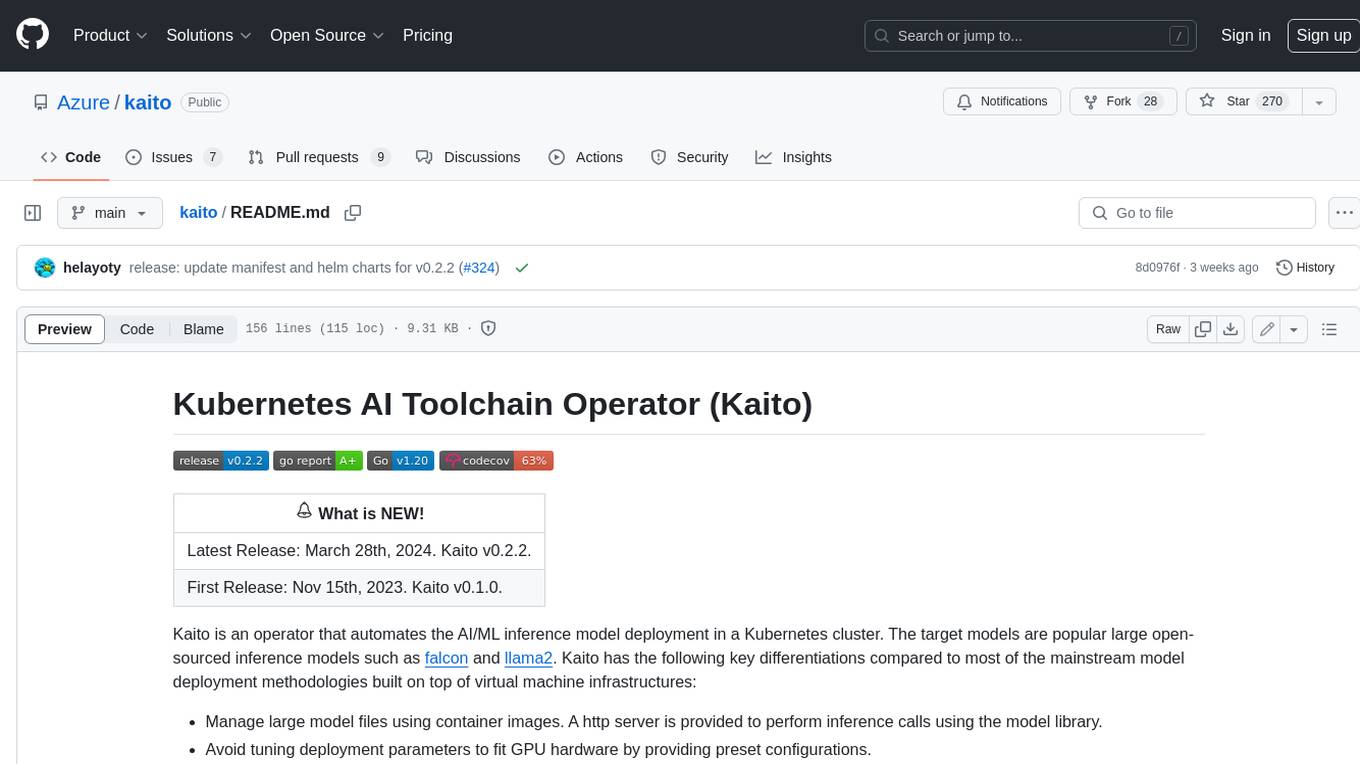
kaito
Kaito is an operator that automates the AI/ML inference model deployment in a Kubernetes cluster. It manages large model files using container images, avoids tuning deployment parameters to fit GPU hardware by providing preset configurations, auto-provisions GPU nodes based on model requirements, and hosts large model images in the public Microsoft Container Registry (MCR) if the license allows. Using Kaito, the workflow of onboarding large AI inference models in Kubernetes is largely simplified.
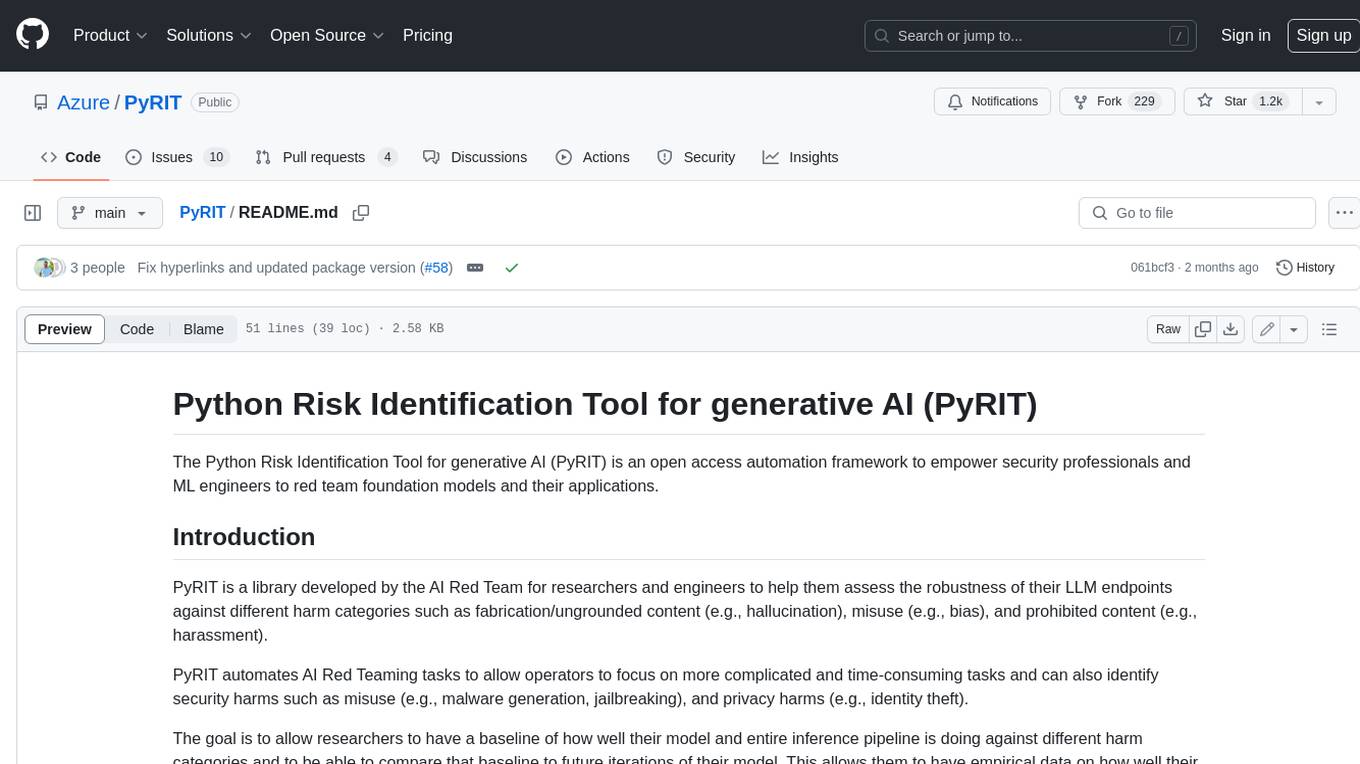
PyRIT
PyRIT is an open access automation framework designed to empower security professionals and ML engineers to red team foundation models and their applications. It automates AI Red Teaming tasks to allow operators to focus on more complicated and time-consuming tasks and can also identify security harms such as misuse (e.g., malware generation, jailbreaking), and privacy harms (e.g., identity theft). The goal is to allow researchers to have a baseline of how well their model and entire inference pipeline is doing against different harm categories and to be able to compare that baseline to future iterations of their model. This allows them to have empirical data on how well their model is doing today, and detect any degradation of performance based on future improvements.
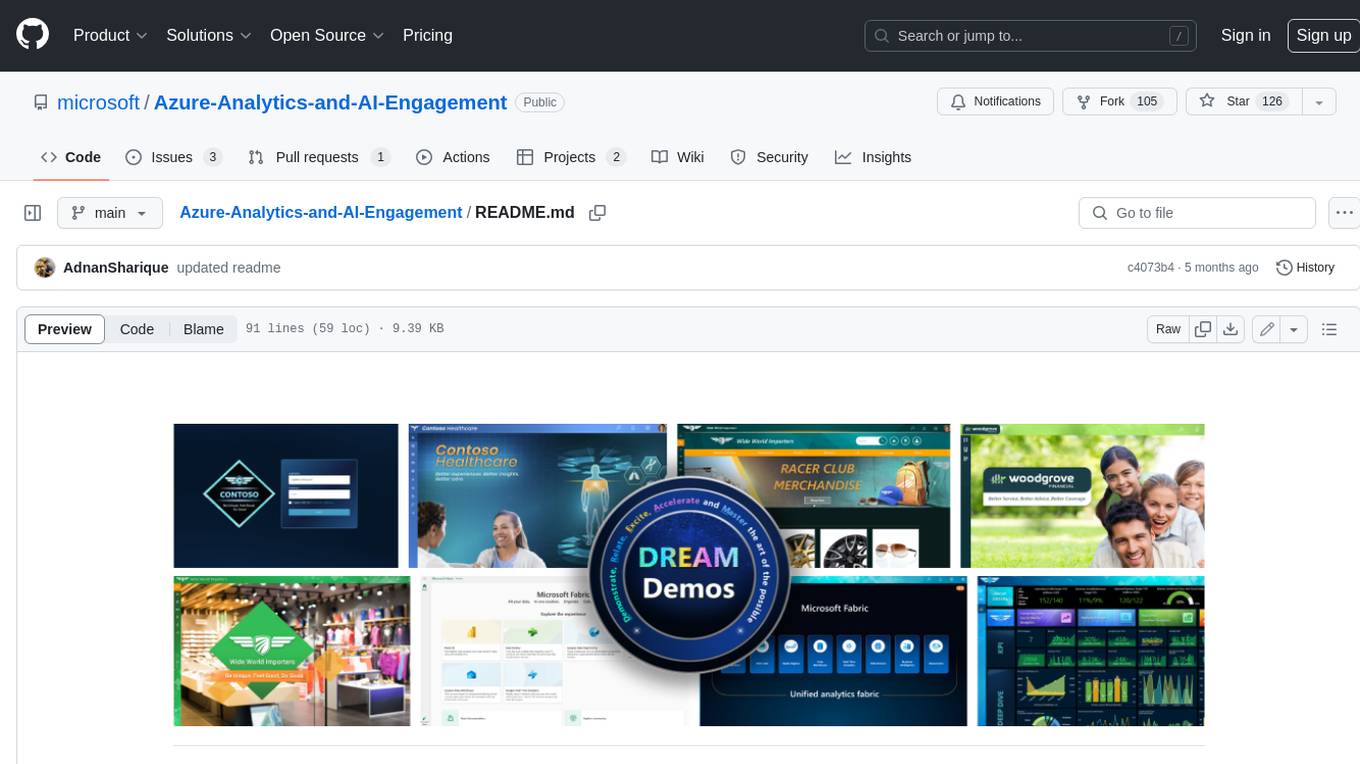
Azure-Analytics-and-AI-Engagement
The Azure-Analytics-and-AI-Engagement repository provides packaged Industry Scenario DREAM Demos with ARM templates (Containing a demo web application, Power BI reports, Synapse resources, AML Notebooks etc.) that can be deployed in a customer’s subscription using the CAPE tool within a matter of few hours. Partners can also deploy DREAM Demos in their own subscriptions using DPoC.