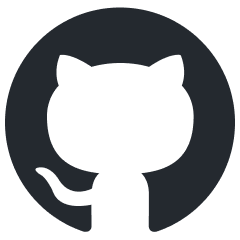
verifAI
VerifAI initiative to build open-source easy-to-deploy generative question-answering engine that can reference and verify answers for correctness (using posteriori model)
Stars: 54
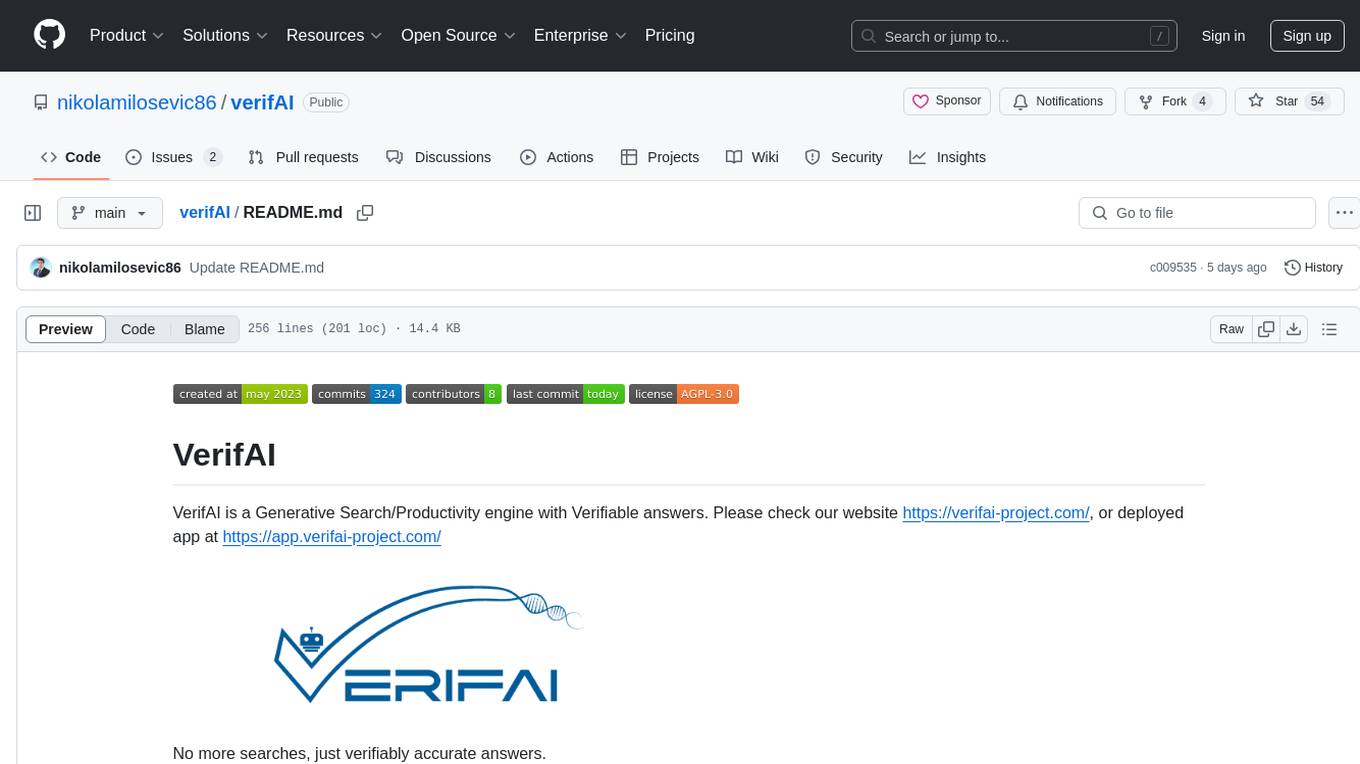
VerifAI is a document-based question-answering system that addresses hallucinations in generative large language models and search engines. It retrieves relevant documents, generates answers with references, and verifies answers for accuracy. The engine uses generative search technology and a verification model to ensure no misinformation. VerifAI supports various document formats and offers user registration with a React.js interface. It is open-source and designed to be user-friendly, making it accessible for anyone to use.
README:
VerifAI is a Generative Search/Productivity engine with Verifiable answers. Please check our website https://verifai-project.com/, or deployed app at https://app.verifai-project.com/
No more searches, just verifiably accurate answers.
- Project Description
- Main Features
- Support This Project
- Installation and Start-up
- Developed Models and Datasets
- Using our APP
- Collaborators and Contributions
- Papers and Citations
- Funding
VerifAI is a document-based question-answering systems that aims to address problem of hallucinations in generative large language models and generative search engines. Initially, we started with biomedical domain, however, now we have expanded VerifAI to support indexing any documents in txt,md, docx, pptx, or pdf formats.
VerifAI is an AI system designed to answer users' questions by retrieving the most relevant documents, generate answer with references to the relevant documents and verify that the generated answer does not contain any hallucinations. In the core of the engine is generative search engine, powered by open technologies. However, generative models may hallucinate, and therefore VerifAI is developed a second model that would check the sources of generative model and flag any misinformation or misinterpretations of source documents. Therefore, make the answer created by generative search engine completly verifiable.
The best part is, that we are making it open source, so anyone can use it!
Check the article about VerifAI project published on TowardsDataScience
- Easy installation by running a single script
- Easy indexing of local files in PDF,EPUB, PPTX, DOCX, MD and TXT formats
- Combination of lexical and semantic search to find the most relevant documents
- Usage of any HuggingFace listed model for document embeddings
- Usage of any LLM that follows OpenAI API standard (deployed using vLLM, Nvidia NIM, Ollama, or via commercial APIs, such as OpenAI, Azure)
- Supports large amounts of indexed documents (tested with over 200GB of data and 30 million documents)
- Shows the closest sentence in the document to the generated claim
- User registration and log-in
- Pleasent user interface developed in React.js
- Verification that generated text does not contain hallucinations by a specially fine-tuned model
- Possible single-sign-on with AzureAD (future plans to add other services, e.g. Google, GitHub, etc.)
If you find this project helpful or interesting, please consider giving it a star on GitHub! Your support helps make this project more visible to others who might benefit from it.
By starring this repository, you'll also stay updated on new features and improvements. Thank you for your support! 🙏
- Clone the repository or download latest release
- Create virtual python environment by running:
python -m venv verifai
source verifai/bin/activate
- In case you get errors with installing psycopg2, you may need to install postgres by running
sudo apt install postgresql-server-dev-all
- On a clean instance you may need to run:
sudo sh -c 'echo "deb http://apt.postgresql.org/pub/repos/apt $(lsb_release -cs)-pgdg main" > /etc/apt/sources.list.d/pgdg.list'
wget --quiet -O - https://www.postgresql.org/media/keys/ACCC4CF8.asc | sudo apt-key add -
sudo apt update
- Run requirements.txt by running
pip install -r backend/requirements.txt
- Configure system, by replacing and modifying
.env.local.example
in backend folder and rename it into just.env
: The configuration should look in the following manner:
SECRET_KEY=6183db7b3c4f67439ad61d1b798224a035fe35c4113bf870
ALGORITHM=HS256
DBNAME=verifai_database
USER_DB=myuser
PASSWORD_DB=mypassword
HOST_DB=localhost
OPENSEARCH_IP=localhost
OPENSEARCH_USER=admin
OPENSEARCH_PASSWORD=admin
OPENSEARCH_PORT=9200
OPENSEARCH_USE_SSL=False
QDRANT_IP=localhost
QDRANT_PORT=6333
QDRANT_API=8da7725d78141e19a9bf3d878f4cb333fedb56eed9727904b46ce4b32e1ce085
QDRANT_USE_SSL=False
OPENAI_PATH=<path-to-openai/azure/vllm/nvidia_nim/ollama-interface>
OPENAI_KEY=<key-in-interface>
DEPLOYMENT_MODEL=GPT4o
MAX_CONTEXT_LENGTH=128000
SIMILARITY_METRIC=DOT
VECTOR_SIZE=768
EMBEDDING_MODEL="sentence-transformers/msmarco-bert-base-dot-v5"
INDEX_NAME_LEXICAL = 'myindex-lexical'
INDEX_NAME_SEMANTIC = "myindex-semantic"
USE_VERIFICATION=True
Please note that value of SIMILARITY_METRIC can be either DOT
(dot product) or COSINE
(cosine similarity). If not stated, it will resolve to Cosine similarity.
- Run install_datastores.py file. To run this file, it is necessary to install Docker (and run the daemon). This file is designed to install necessary components, such as OpenSearch, Qdrant and PostgreSQL, as well as to create database in PostgreSQL.
python install_datastore.py
- Index your files, by running index_files.py and pointing it to the directory with files you would like to index. It will recuresevly index all files in the directory.
python index_files.py <path-to-directory-with-files>
As an example, we have created a folder with some example files in the folder test_data
. You can index them by running:
python index_files.py test_data
- Run the backend of VerifAI by running
main.py
in the backend folder.
python main.py
- Install React by following this guide, or by running following commands:
sudo apt update
sudo apt install nodejs npm
sudo npm install -g create-react-app
- Install React requirements for the front-end in
client-gui/verifai-ui
folder and run front end:
cd ..
cd client-gui/verifai-ui
npm install
Create .env
file in the client-gui/verifai-ui
folder with the following content (or based on .env.example
file):
REACT_APP_BACKEND = http://127.0.0.1:5001/ # or your API url
REACT_APP_AZURE_CLIENT_ID=<your_azure_client_id>
REACT_APP_AZURE_TENANT_ID=<your_azure_tenant_id>
REACT_APP_AZURE_REDIRECT_URL=http://localhost:3000
If you do not configure REACT_APP_AZURE_CLIENT_ID
and REACT_APP_AZURE_TENANT_ID
, the app will not have the option to log in with AzureAD. Your AzureAD application needs to be registered as Single-Page Application in Azure. Change REACT_APP_AZURE_REDIRECT_URL
to the redirect URL matching one in Azure.
Start the app by running:
npm start
- Go to
http://localhost:3000
to see the VerifAI in action.
You can check a tutorial on deploying VerifAI published on Towards Data Science
This is biomedical version of VerifAI. It is designed to answer questions from the biomedical domain.
One requirement to run locally is to have installed Postgres SQL. You can install it for example on mac by running brew install postgresql
.
- Clone the repository
- Run requirements.txt by running
pip install -r backend/requirements.txt
- Download Medline. You can do it by executing
download_medline_data.sh
for core files for the current year anddownload_medline_data_update.sh
for Medline current update files. - Install Qdrant following the guide here
- Run the script:
python medline2json.py
to transform MEDLINE XML files into JSON - Run
python json2selected.py
to selects the fields that should be inported into the index - Run
python abstarct_parser.py
to concatinate abstract titles and abstracts and splits texts to 512 parts that can be indexed using a transformer model - Run
python embeddings_creation.py
to create embeddings. - Run
python scripts/indexing_qdrant.py
to create qdrant index. Make sure to point to the right folder created in the previous step and to the qdrant instance. - Install OpenSearch following the guide here
- Create OpenSearch index by running
python scripts/indexing_lexical_pmid.py
. Make sure to configure access to the OpenSearch and point the path variable to the folder created by json2selected script. - Set up system variables that are needed for the project. You can do it by creating
.env
file with the following content:
OPENSEARCH_IP=open_search_ip
OPENSEARCH_USER=open_search_user
OPENSEARCH_PASSWORD=open_search_pass
OPENSEARCH_PORT=9200
QDRANT_IP=qdrant_ip
QDRANT_PORT=qdrant_port
QDRANT_API=qdrant_api_key
QDRANT_USE_SSL=False
OPENSEARCH_USE_SSL=False
MAX_CONTEXT_LENGTH=32000
EMBEDDING_MODEL="sentence-transformers/msmarco-bert-base-dot-v5"
INDEX_NAME_LEXICAL = 'medline-faiss-hnsw-lexical-pmid'
INDEX_NAME_SEMANTIC = "medline-faiss-hnsw"
USE_VERIFICATION=True
- Run backend by running
python backend/main.py
- Install React by following this guide
- Run
npm run-script build
- Run frontend by running
npm start
in client-gui/verifai-ui
- Fine tuned QLoRA addapted for Mistral 7B-instruct v01
- Fine tuned QLoRA addapted for Mistral 7B-instruct v02
- PQAref dataset
- Verification model based on DeBERTa, fine-tuned on SciFact dataset
You can use our app here. You need to create a free account by clicking on Join now.
Currently, two institutions are the main drivers of this project, namely Bayer A.G and Institute for Artificial Intelligence Research and Development of Serbia. Current contrbiutors are by institutions
- Bayer A.G.
- Nikola Milosevic
- Lorenzo Cassano
- Institute for Artificial Intelligence Research and Development of Serbia:
- Adela Ljajic
- Milos Kosprdic
- Bojana Basaragin
- Darija Medvecki
- Angela Pupovac
- Nataša Radmilović
- Petar Stevanović
We welcome contribution to this project by anyone interested in participating. This is an open source project under AGPL license. In order to prevent any legal issues, before sending the first pull request, we ask potential contributors to sign Individual Contributor Agreement and send to us via email ([email protected]).
- Adela Ljajić, Miloš Košprdić, Bojana Bašaragin, Darija Medvecki, Lorenzo Cassano, Nikola Milošević, “Scientific QA System with Verifiable Answers”, The 6th International Open Search Symposium 2024
- Košprdić, M., Ljajić, A., Bašaragin, B., Medvecki, D., & Milošević, N. "Verif. ai: Towards an Open-Source Scientific Generative Question-Answering System with Referenced and Verifiable Answers." The Sixteenth International Conference on Evolving Internet INTERNET 2024 (2024).
- Bojana Bašaragin, Adela Ljajić, Darija Medvecki, Lorenzo Cassano, Miloš Košprdić, Nikola Milošević "How do you know that? Teaching Generative Language Models to Reference Answers to Biomedical Questions", Accepted at BioNLP 2024, Colocated with ACL 2024
- Adela Ljajić, Lorenzo Cassano, Miloš Košprdić, Bašaragin Bojana, Darija Medvecki, Nikola Milošević, "Enhancing Biomedical Information Retrieval with Semantic Search: A Comparative Analysis Using PubMed Data", Belgrade Bioinformatics Conference BelBi2024, 2024
- Košprdić, M.; Ljajić, A.; Medvecki, D.; Bašaragin, B. and Milošević, N. (2024). Scientific Claim Verification with Fine-Tuned NLI Models. In Proceedings of the 16th International Joint Conference on Knowledge Discovery, Knowledge Engineering and Knowledge Management - KMIS; ISBN 978-989-758-716-0; ISSN 2184-3228, SciTePress, pages 15-25. DOI: 10.5220/0012900000003838
This project was in September 2023 funded by NGI Search project of the European Union. Views and opinions expressed are however those of the author(s) only and do not necessarily reflect those of the European Union or European Commission. Neither the European Union nor the granting authority can be held responsible for them. Funded within the framework of the NGI Search project under grant agreement No 101069364
For Tasks:
Click tags to check more tools for each tasksFor Jobs:
Alternative AI tools for verifAI
Similar Open Source Tools
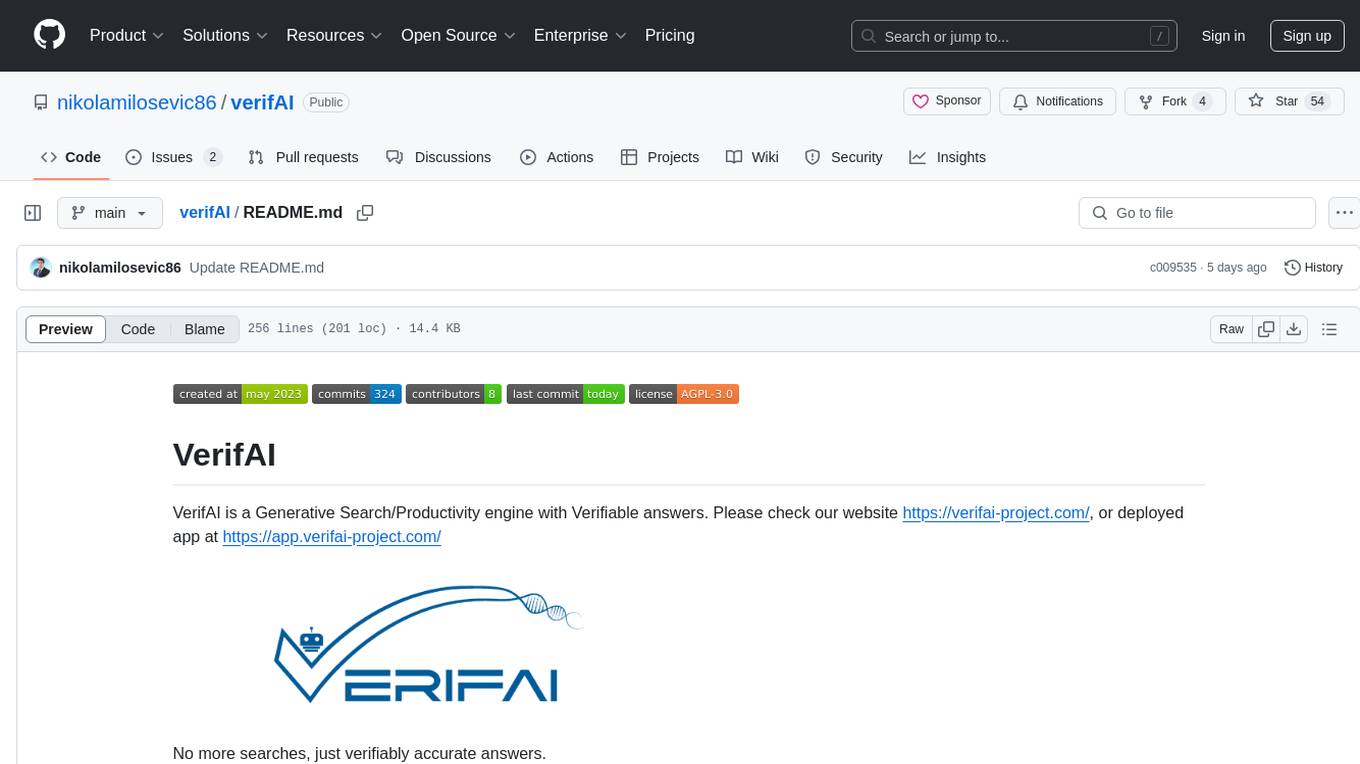
verifAI
VerifAI is a document-based question-answering system that addresses hallucinations in generative large language models and search engines. It retrieves relevant documents, generates answers with references, and verifies answers for accuracy. The engine uses generative search technology and a verification model to ensure no misinformation. VerifAI supports various document formats and offers user registration with a React.js interface. It is open-source and designed to be user-friendly, making it accessible for anyone to use.
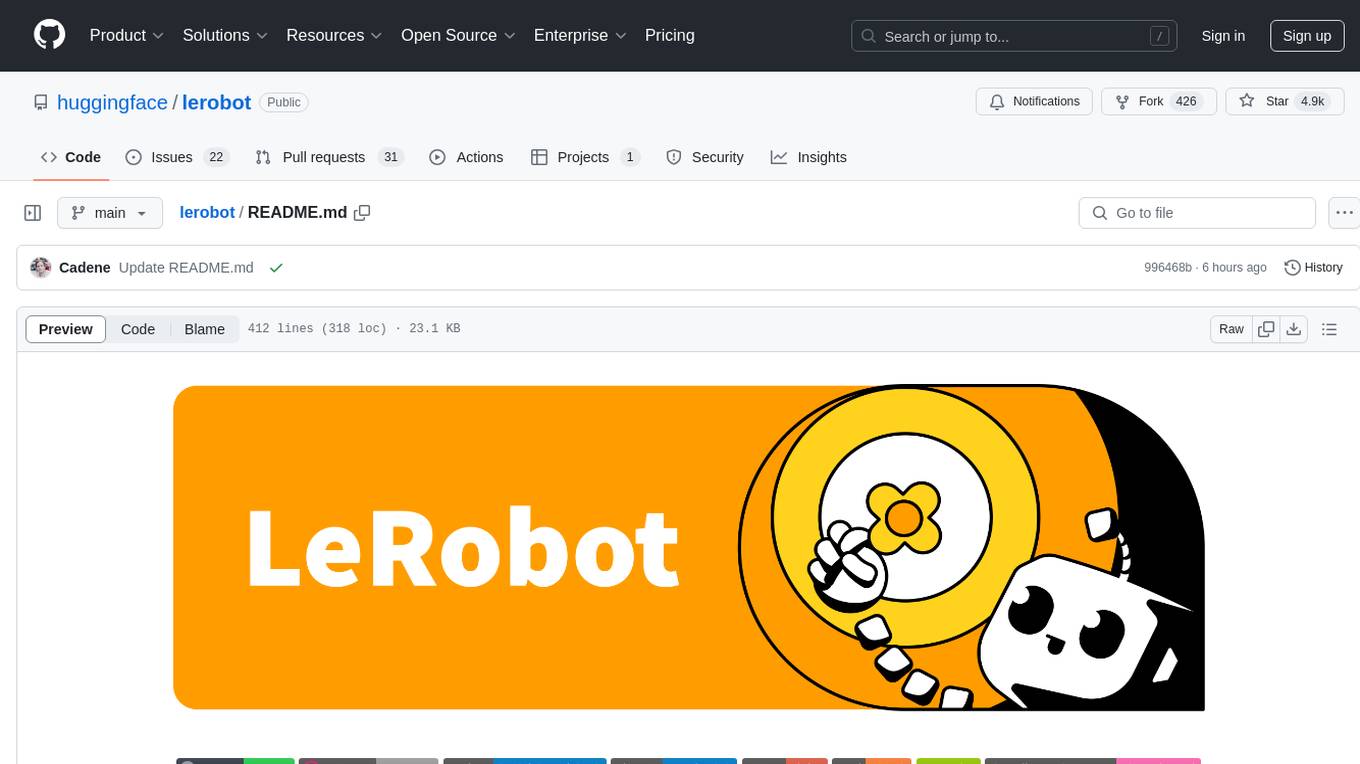
lerobot
LeRobot is a state-of-the-art AI library for real-world robotics in PyTorch. It aims to provide models, datasets, and tools to lower the barrier to entry to robotics, focusing on imitation learning and reinforcement learning. LeRobot offers pretrained models, datasets with human-collected demonstrations, and simulation environments. It plans to support real-world robotics on affordable and capable robots. The library hosts pretrained models and datasets on the Hugging Face community page.
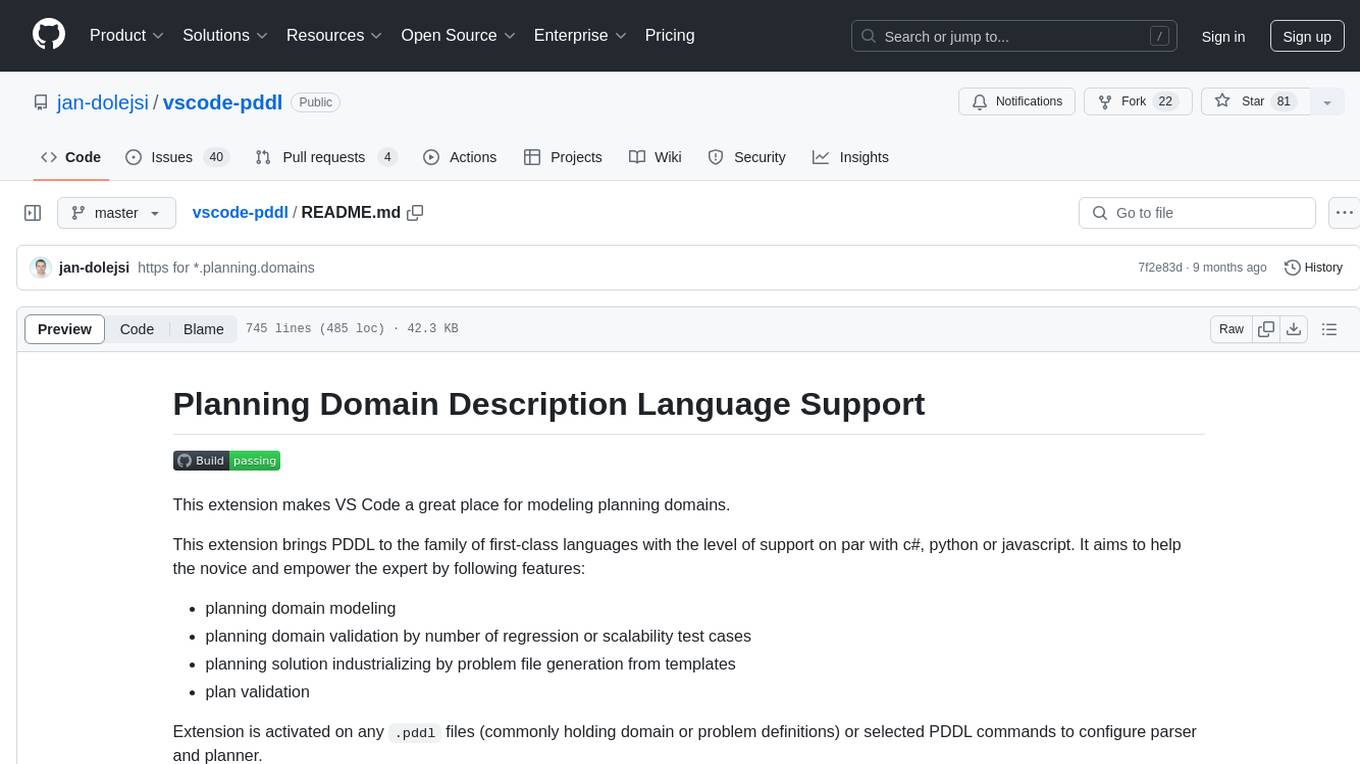
vscode-pddl
The vscode-pddl extension provides comprehensive support for Planning Domain Description Language (PDDL) in Visual Studio Code. It enables users to model planning domains, validate them, industrialize planning solutions, and run planners. The extension offers features like syntax highlighting, auto-completion, plan visualization, plan validation, plan happenings evaluation, search debugging, and integration with Planning.Domains. Users can create PDDL files, run planners, visualize plans, and debug search algorithms efficiently within VS Code.
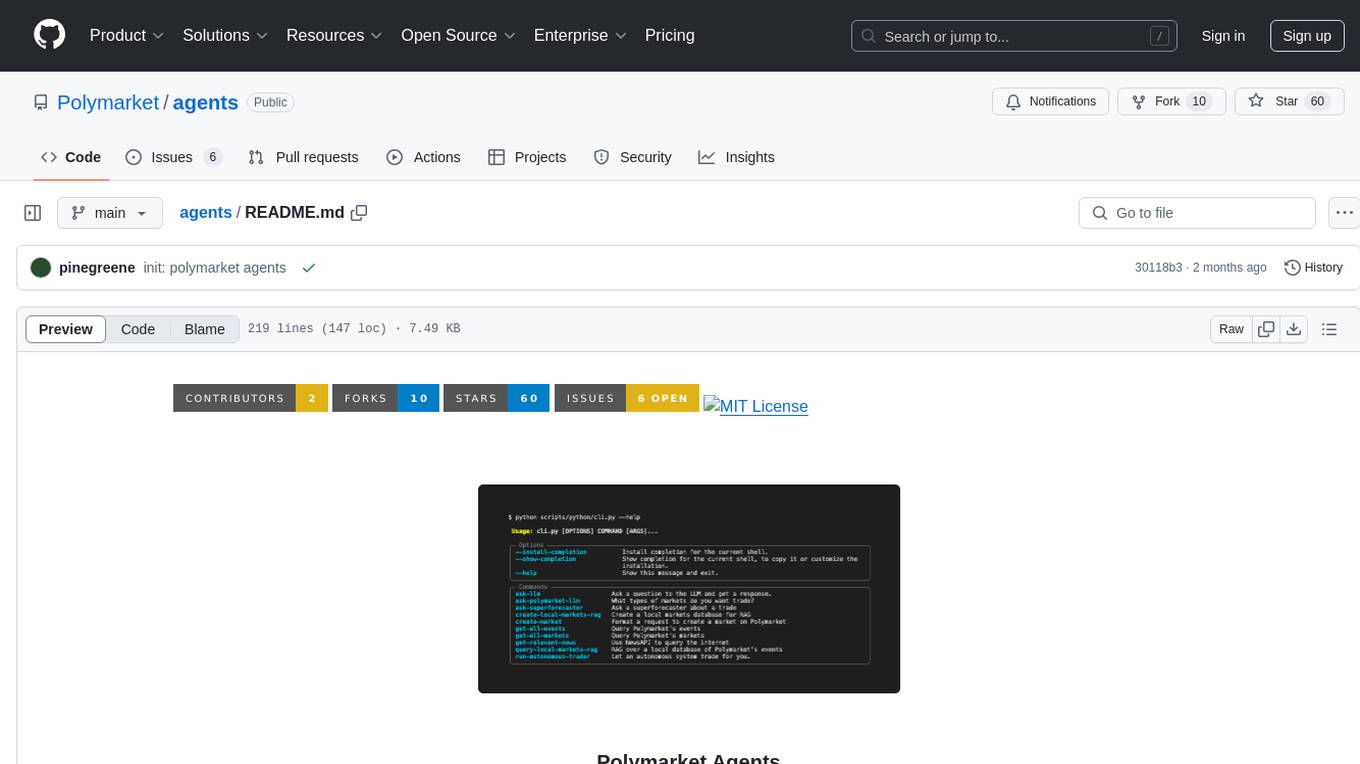
agents
Polymarket Agents is a developer framework and set of utilities for building AI agents to trade autonomously on Polymarket. It integrates with Polymarket API, provides AI agent utilities for prediction markets, supports local and remote RAG, sources data from various services, and offers comprehensive LLM tools for prompt engineering. The architecture features modular components like APIs and scripts for managing local environments, server set-up, and CLI for end-user commands.
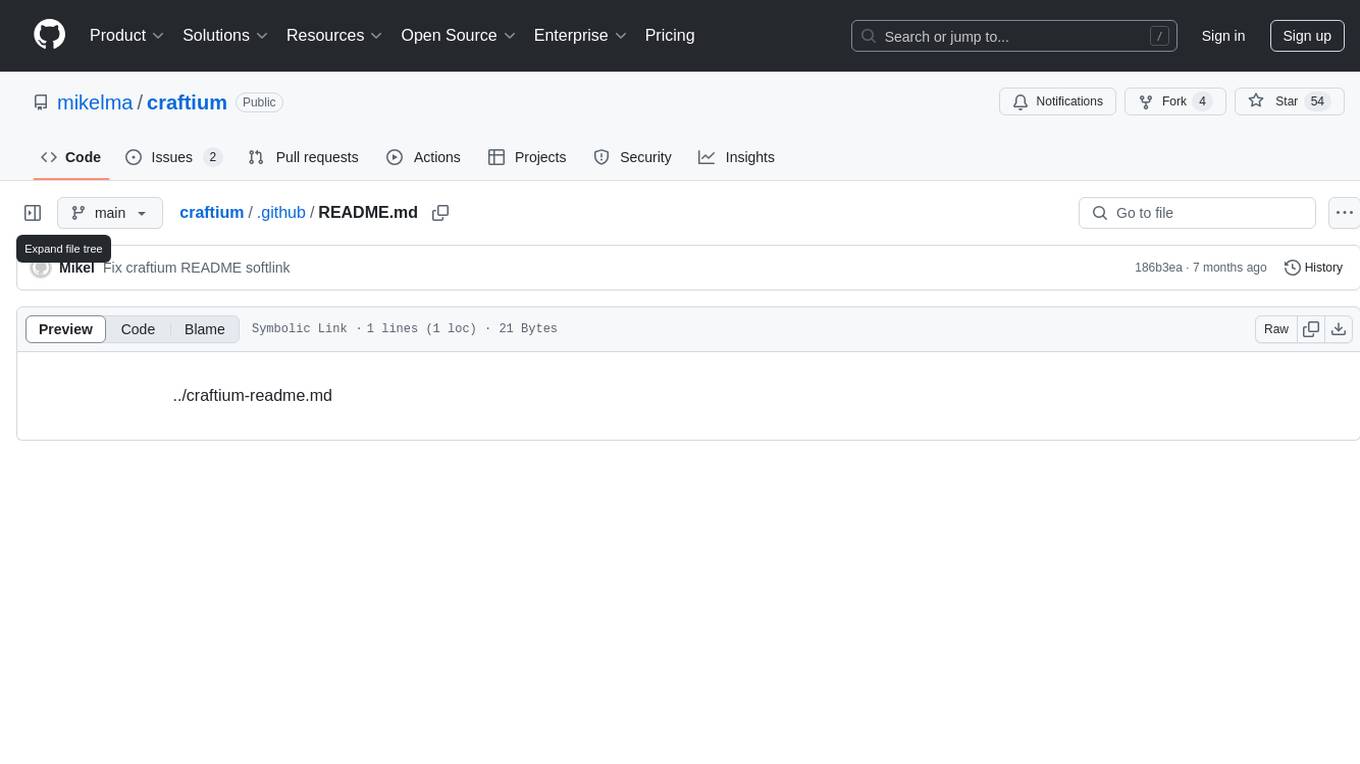
craftium
Craftium is an open-source platform based on the Minetest voxel game engine and the Gymnasium and PettingZoo APIs, designed for creating fast, rich, and diverse single and multi-agent environments. It allows for connecting to Craftium's Python process, executing actions as keyboard and mouse controls, extending the Lua API for creating RL environments and tasks, and supporting client/server synchronization for slow agents. Craftium is fully extensible, extensively documented, modern RL API compatible, fully open source, and eliminates the need for Java. It offers a variety of environments for research and development in reinforcement learning.
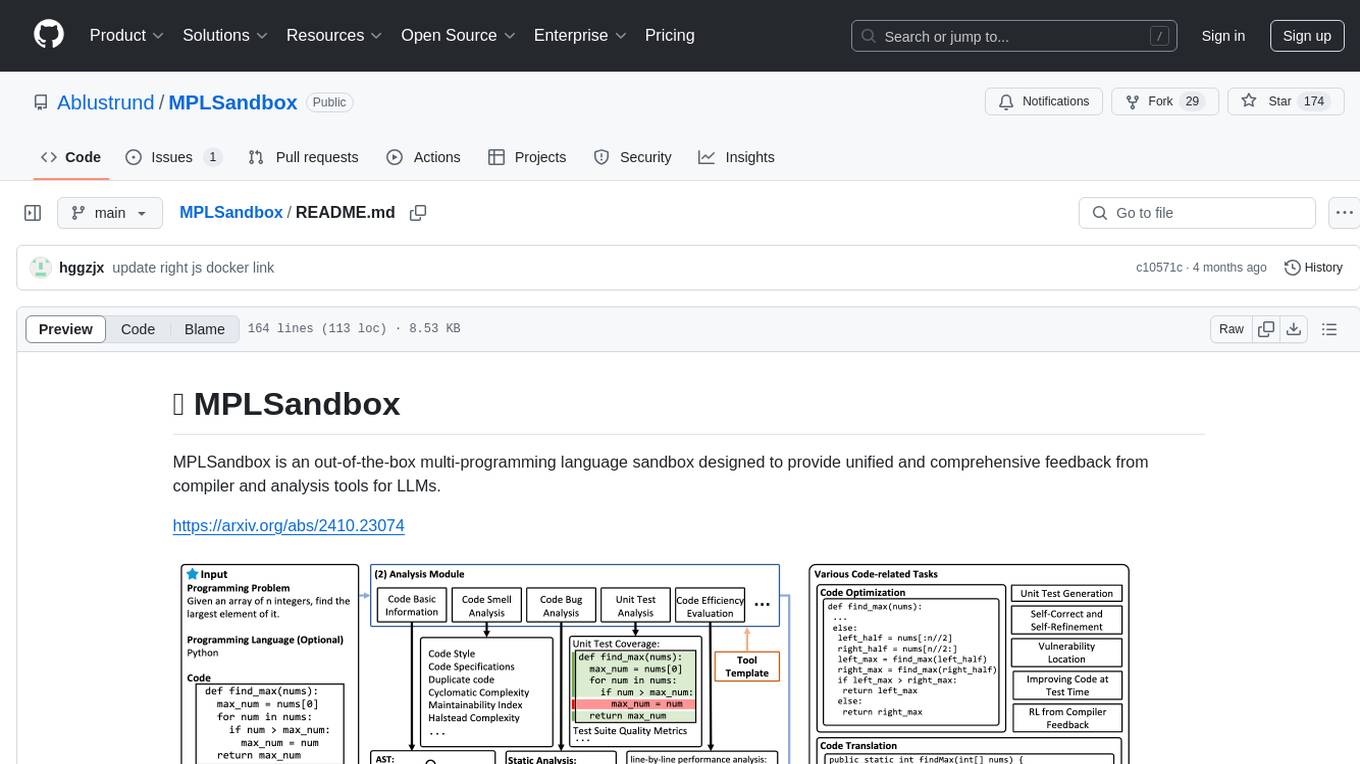
MPLSandbox
MPLSandbox is an out-of-the-box multi-programming language sandbox designed to provide unified and comprehensive feedback from compiler and analysis tools for LLMs. It simplifies code analysis for researchers and can be seamlessly integrated into LLM training and application processes to enhance performance in a range of code-related tasks. The sandbox environment ensures safe code execution, the code analysis module offers comprehensive analysis reports, and the information integration module combines compilation feedback and analysis results for complex code-related tasks.
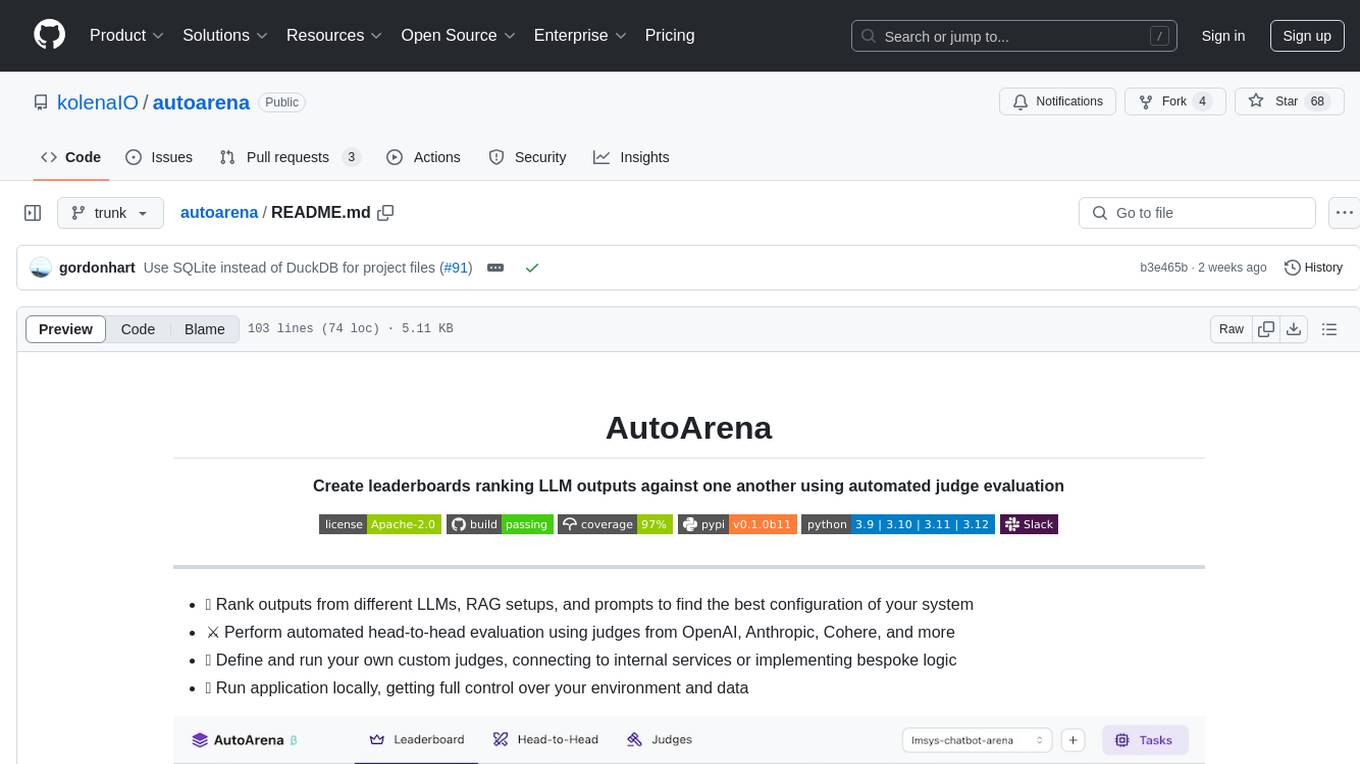
autoarena
AutoArena is a tool designed to create leaderboards ranking Language Model outputs against one another using automated judge evaluation. It allows users to rank outputs from different LLMs, RAG setups, and prompts to find the best configuration of their system. Users can perform automated head-to-head evaluation using judges from various platforms like OpenAI, Anthropic, and Cohere. Additionally, users can define and run custom judges, connect to internal services, or implement bespoke logic. AutoArena enables users to run the application locally, providing full control over their environment and data.
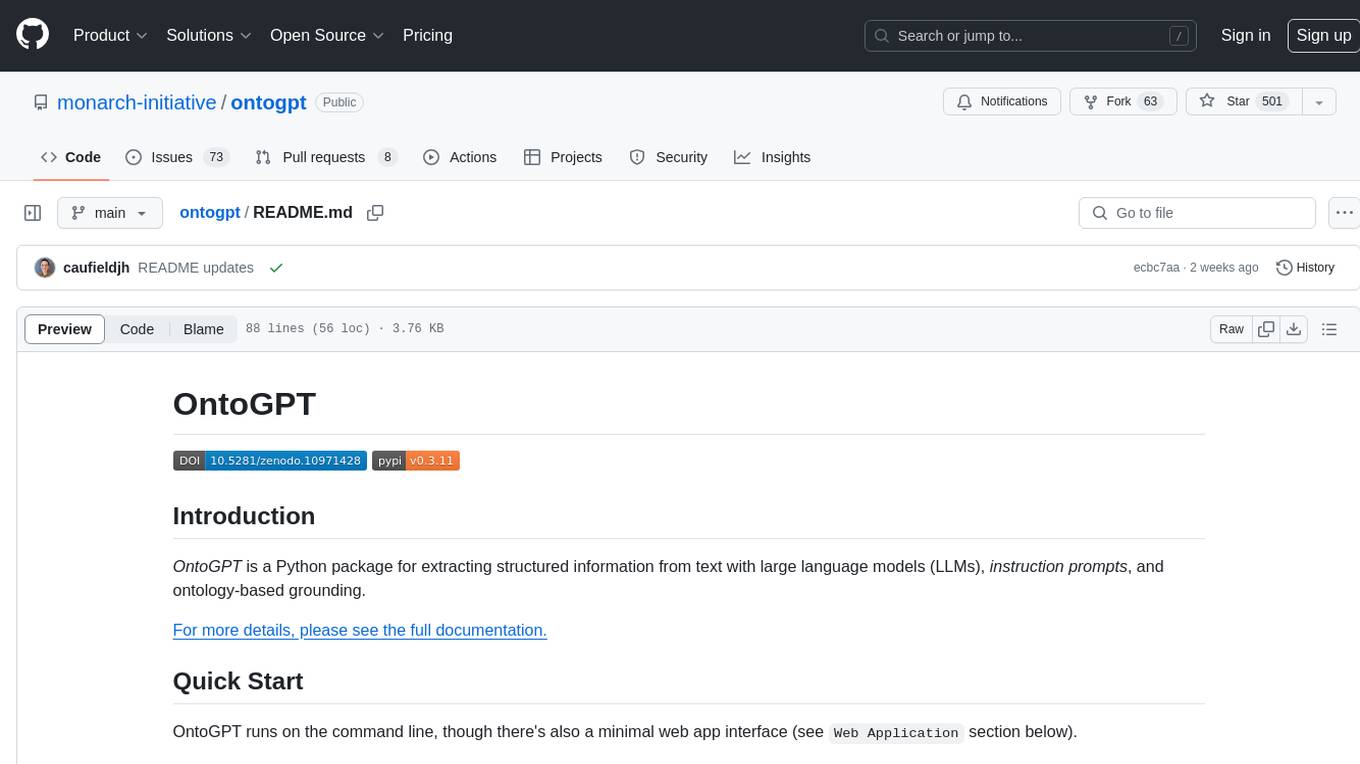
ontogpt
OntoGPT is a Python package for extracting structured information from text using large language models, instruction prompts, and ontology-based grounding. It provides a command line interface and a minimal web app for easy usage. The tool has been evaluated on test data and is used in related projects like TALISMAN for gene set analysis. OntoGPT enables users to extract information from text by specifying relevant terms and provides the extracted objects as output.
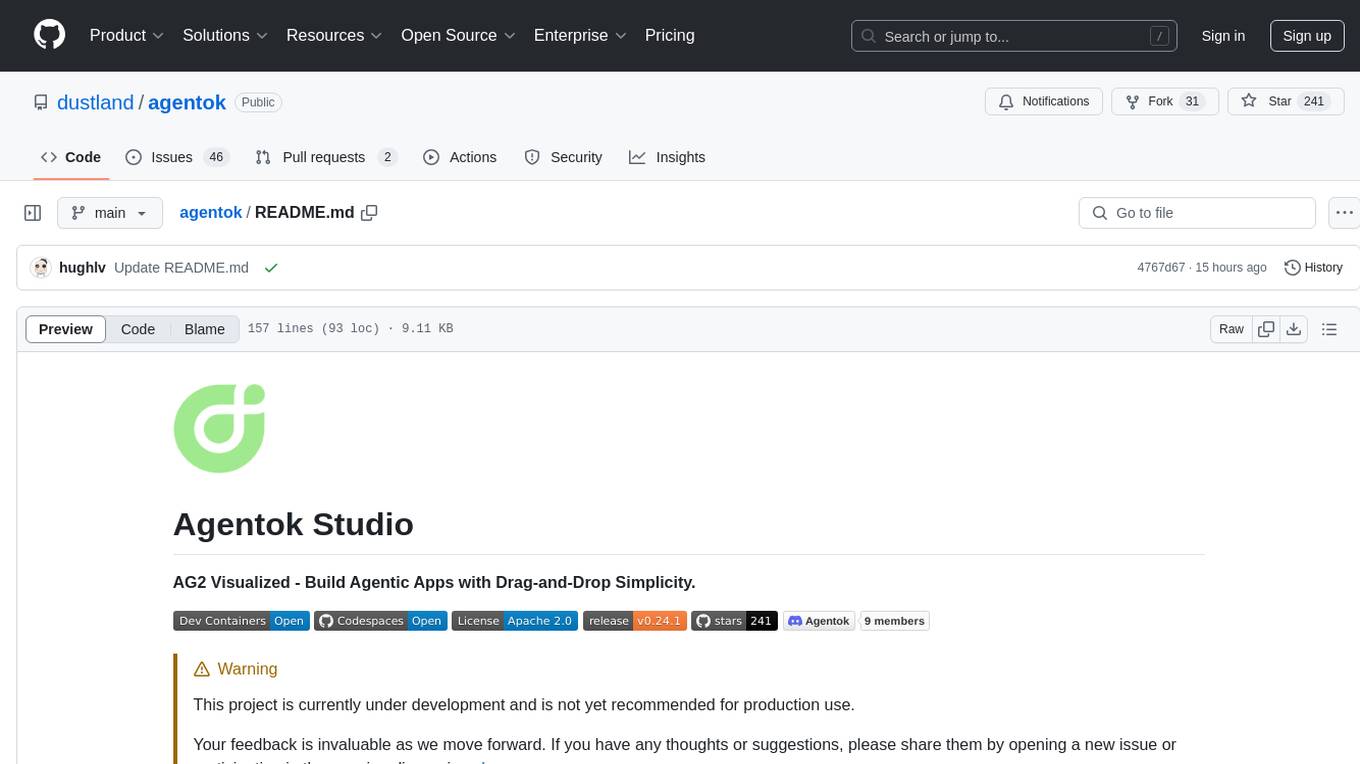
agentok
Agentok Studio is a tool built upon AG2, a powerful agent framework from Microsoft, offering intuitive visual tools to streamline the creation and management of complex agent-based workflows. It simplifies the process for creators and developers by generating native Python code with minimal dependencies, enabling users to create self-contained code that can be executed anywhere. The tool is currently under development and not recommended for production use, but contributions are welcome from the community to enhance its capabilities and functionalities.
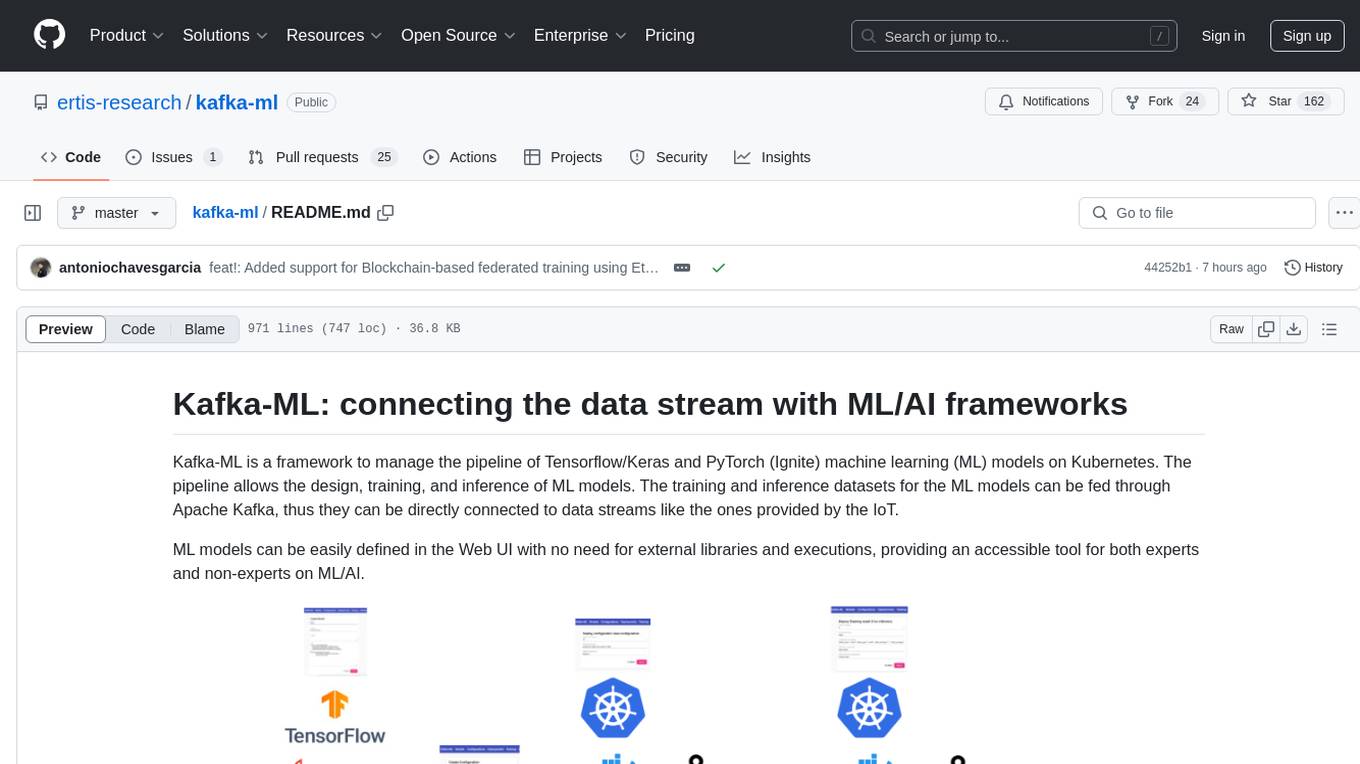
kafka-ml
Kafka-ML is a framework designed to manage the pipeline of Tensorflow/Keras and PyTorch machine learning models on Kubernetes. It enables the design, training, and inference of ML models with datasets fed through Apache Kafka, connecting them directly to data streams like those from IoT devices. The Web UI allows easy definition of ML models without external libraries, catering to both experts and non-experts in ML/AI.
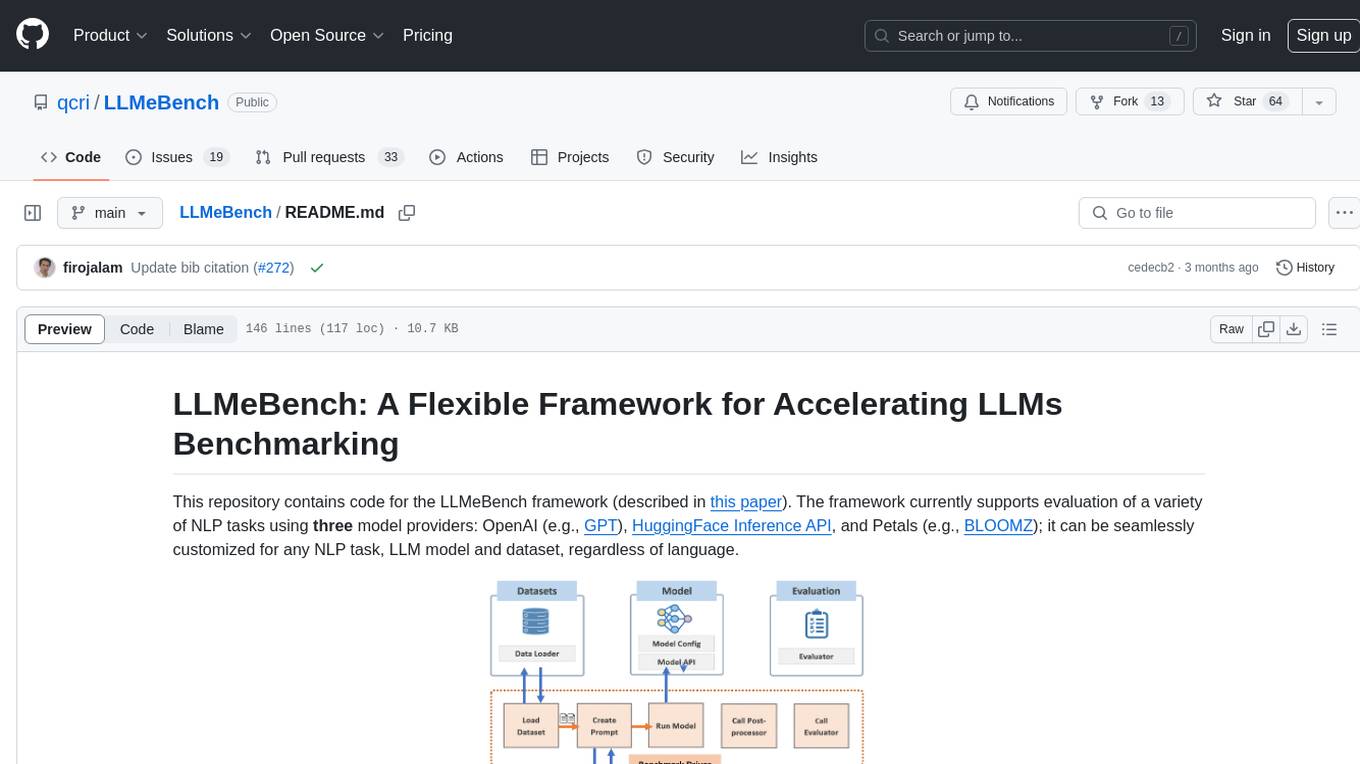
LLMeBench
LLMeBench is a flexible framework designed for accelerating benchmarking of Large Language Models (LLMs) in the field of Natural Language Processing (NLP). It supports evaluation of various NLP tasks using model providers like OpenAI, HuggingFace Inference API, and Petals. The framework is customizable for different NLP tasks, LLM models, and datasets across multiple languages. It features extensive caching capabilities, supports zero- and few-shot learning paradigms, and allows on-the-fly dataset download and caching. LLMeBench is open-source and continuously expanding to support new models accessible through APIs.
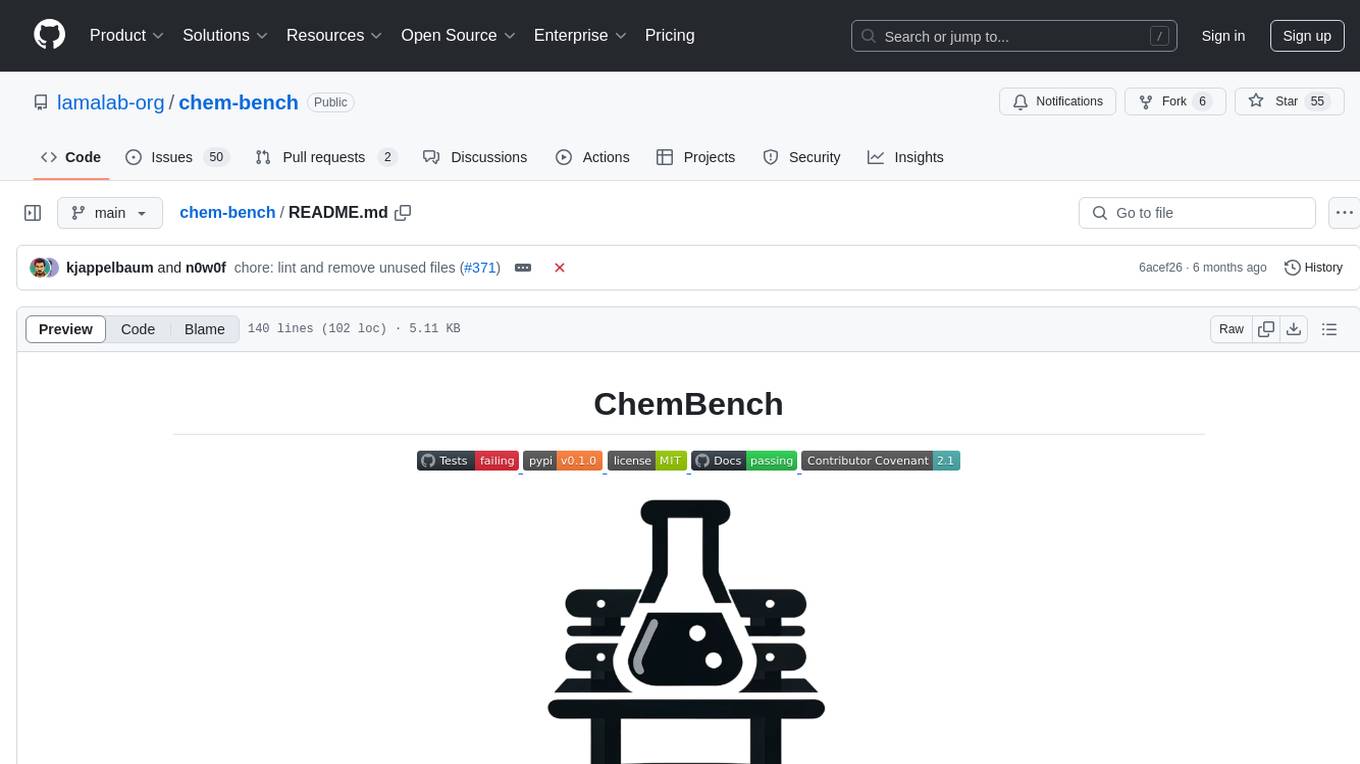
chem-bench
ChemBench is a project aimed at expanding chemistry benchmark tasks in a BIG-bench compatible way, providing a pipeline to benchmark frontier and open models. It allows users to run benchmarking tasks on models with existing presets, offering predefined parameters and processing steps. The library facilitates benchmarking models on the entire suite, addressing challenges such as prompt structure, parsing, and scoring methods. Users can contribute to the project by following the developer notes.
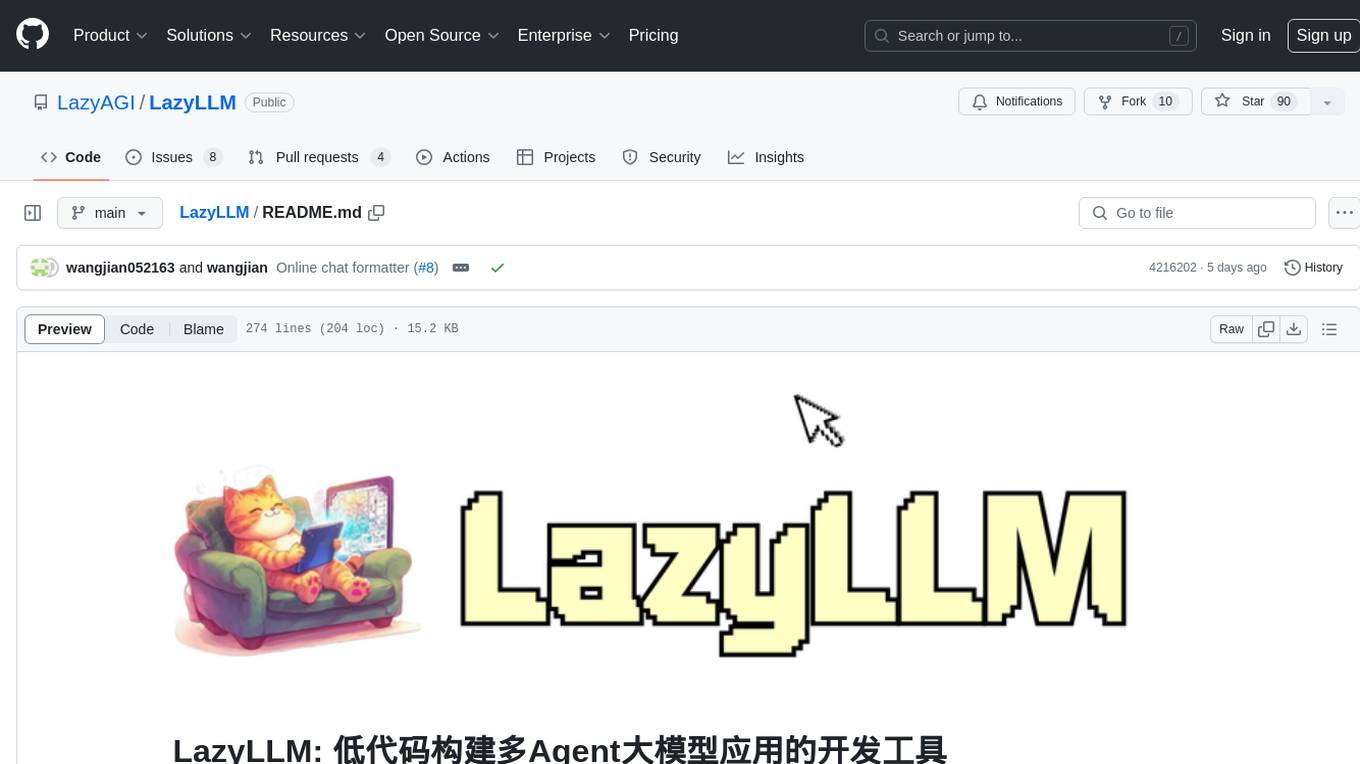
LazyLLM
LazyLLM is a low-code development tool for building complex AI applications with multiple agents. It assists developers in building AI applications at a low cost and continuously optimizing their performance. The tool provides a convenient workflow for application development and offers standard processes and tools for various stages of application development. Users can quickly prototype applications with LazyLLM, analyze bad cases with scenario task data, and iteratively optimize key components to enhance the overall application performance. LazyLLM aims to simplify the AI application development process and provide flexibility for both beginners and experts to create high-quality applications.
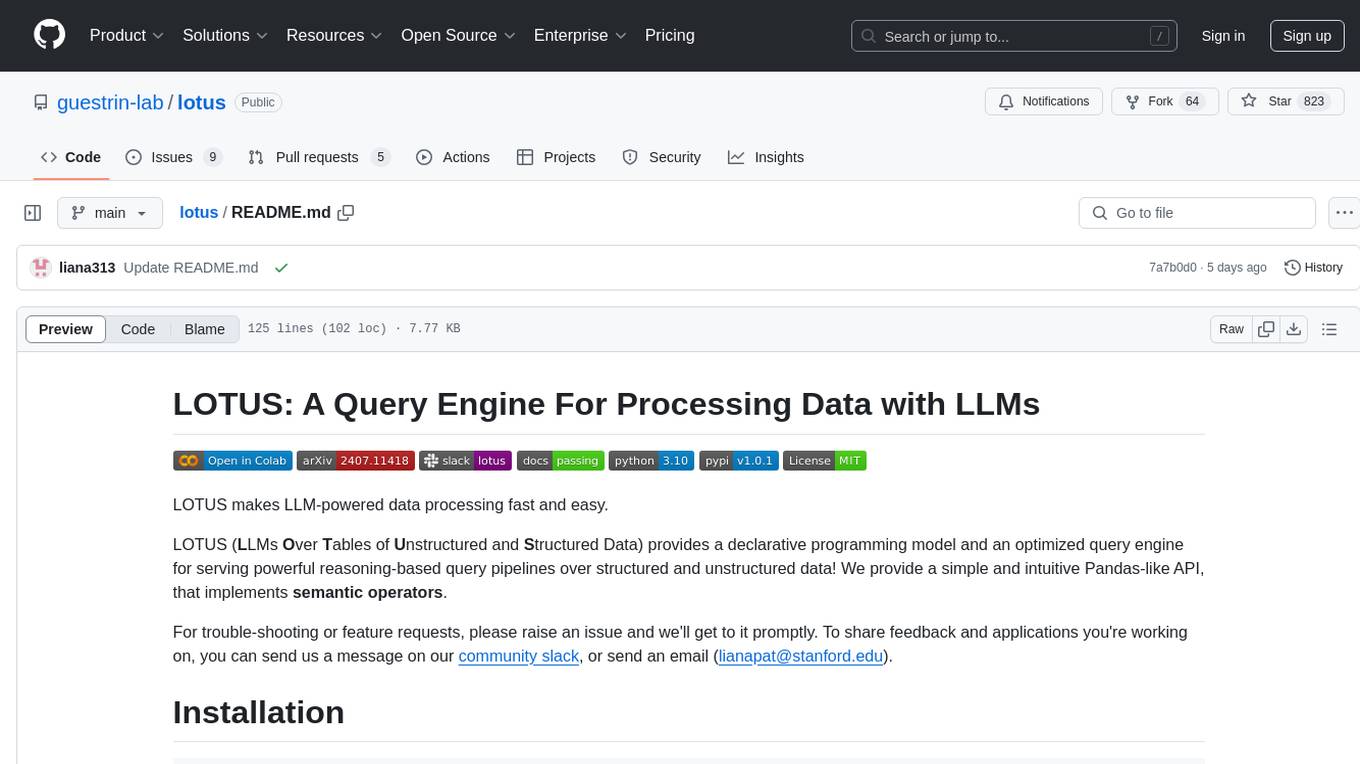
lotus
LOTUS (LLMs Over Tables of Unstructured and Structured Data) is a query engine that provides a declarative programming model and an optimized query engine for reasoning-based query pipelines over structured and unstructured data. It offers a simple and intuitive Pandas-like API with semantic operators for fast and easy LLM-powered data processing. The tool implements a semantic operator programming model, allowing users to write AI-based pipelines with high-level logic and leaving the rest of the work to the query engine. LOTUS supports various semantic operators like sem_map, sem_filter, sem_extract, sem_agg, sem_topk, sem_join, sem_sim_join, and sem_search, enabling users to perform tasks like mapping records, filtering data, aggregating records, and more. The tool also supports different model classes such as LM, RM, and Reranker for language modeling, retrieval, and reranking tasks respectively.
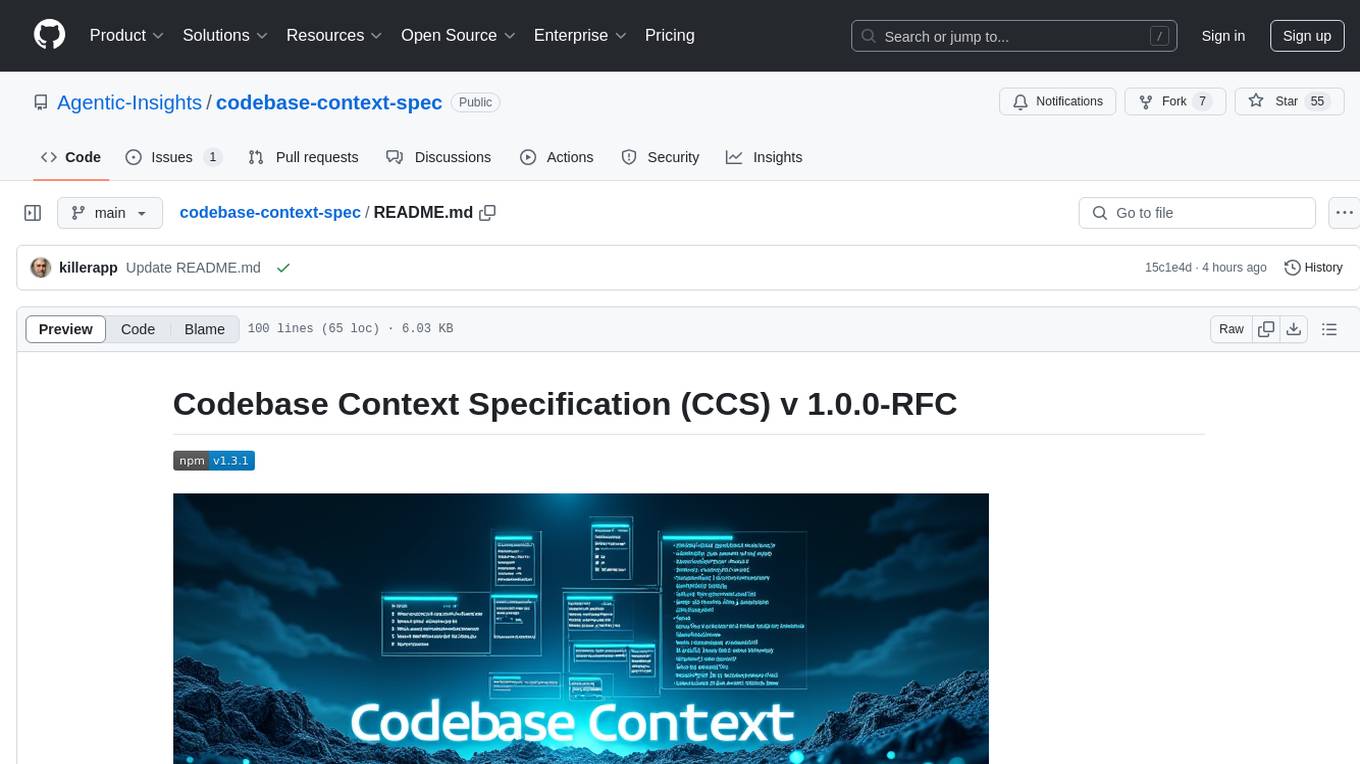
codebase-context-spec
The Codebase Context Specification (CCS) project aims to standardize embedding contextual information within codebases to enhance understanding for both AI and human developers. It introduces a convention similar to `.env` and `.editorconfig` files but focused on documenting code for both AI and humans. By providing structured contextual metadata, collaborative documentation guidelines, and standardized context files, developers can improve code comprehension, collaboration, and development efficiency. The project includes a linter for validating context files and provides guidelines for using the specification with AI assistants. Tooling recommendations suggest creating memory systems, IDE plugins, AI model integrations, and agents for context creation and utilization. Future directions include integration with existing documentation systems, dynamic context generation, and support for explicit context overriding.
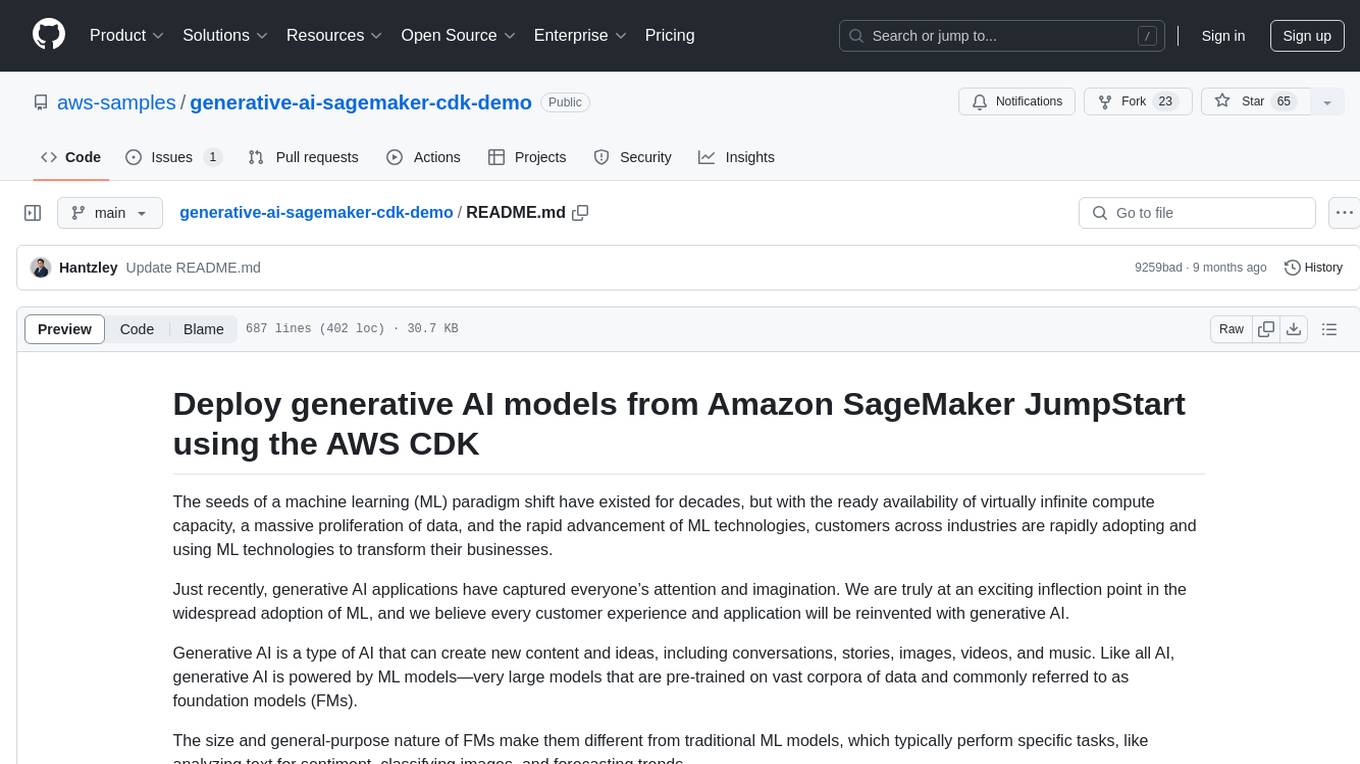
generative-ai-sagemaker-cdk-demo
This repository showcases how to deploy generative AI models from Amazon SageMaker JumpStart using the AWS CDK. Generative AI is a type of AI that can create new content and ideas, such as conversations, stories, images, videos, and music. The repository provides a detailed guide on deploying image and text generative AI models, utilizing pre-trained models from SageMaker JumpStart. The web application is built on Streamlit and hosted on Amazon ECS with Fargate. It interacts with the SageMaker model endpoints through Lambda functions and Amazon API Gateway. The repository also includes instructions on setting up the AWS CDK application, deploying the stacks, using the models, and viewing the deployed resources on the AWS Management Console.
For similar tasks
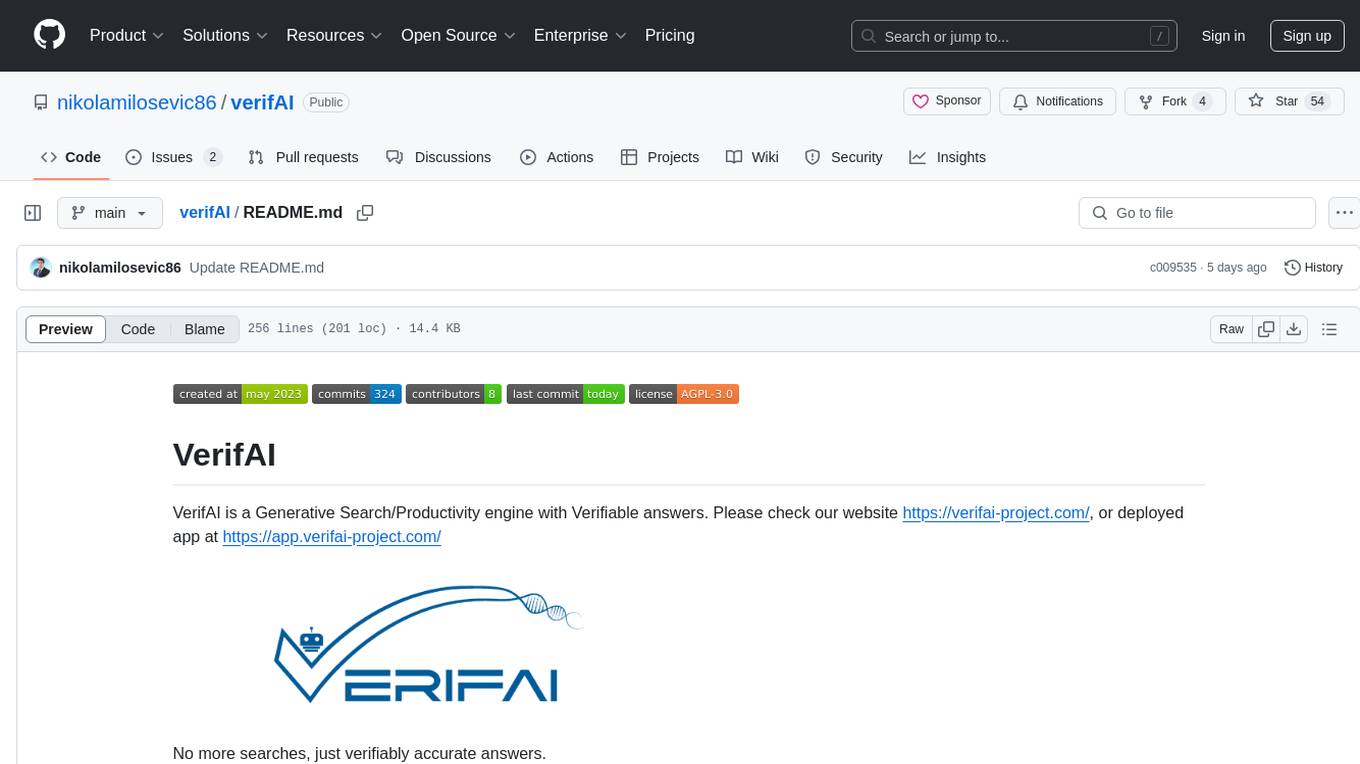
verifAI
VerifAI is a document-based question-answering system that addresses hallucinations in generative large language models and search engines. It retrieves relevant documents, generates answers with references, and verifies answers for accuracy. The engine uses generative search technology and a verification model to ensure no misinformation. VerifAI supports various document formats and offers user registration with a React.js interface. It is open-source and designed to be user-friendly, making it accessible for anyone to use.
For similar jobs
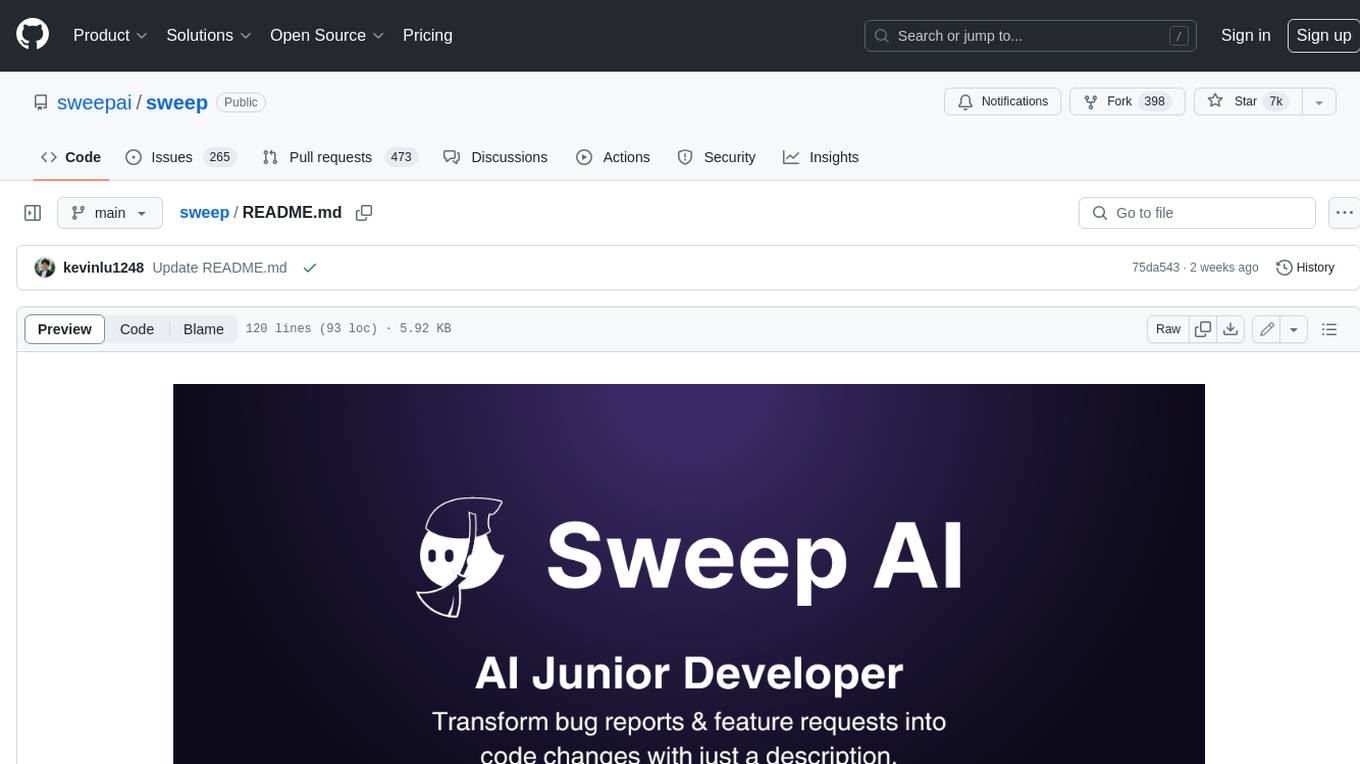
sweep
Sweep is an AI junior developer that turns bugs and feature requests into code changes. It automatically handles developer experience improvements like adding type hints and improving test coverage.
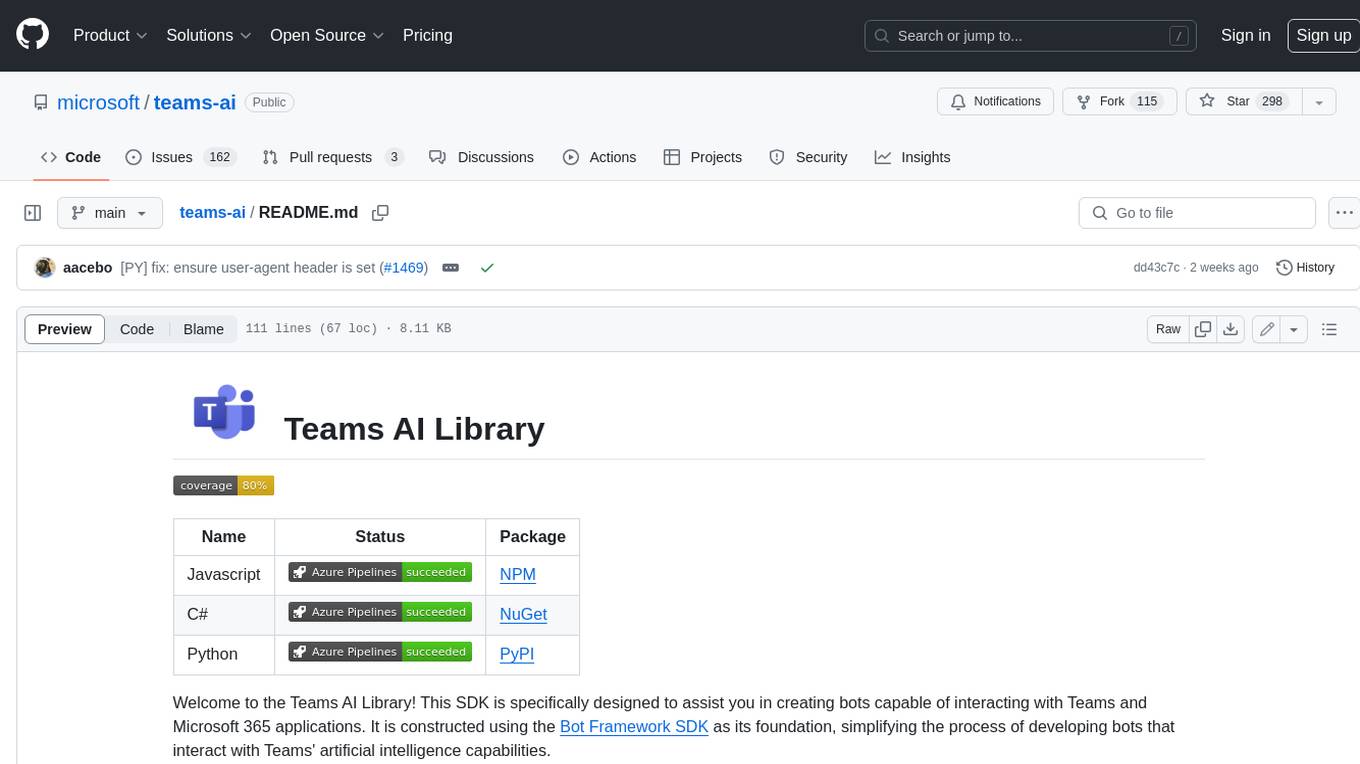
teams-ai
The Teams AI Library is a software development kit (SDK) that helps developers create bots that can interact with Teams and Microsoft 365 applications. It is built on top of the Bot Framework SDK and simplifies the process of developing bots that interact with Teams' artificial intelligence capabilities. The SDK is available for JavaScript/TypeScript, .NET, and Python.
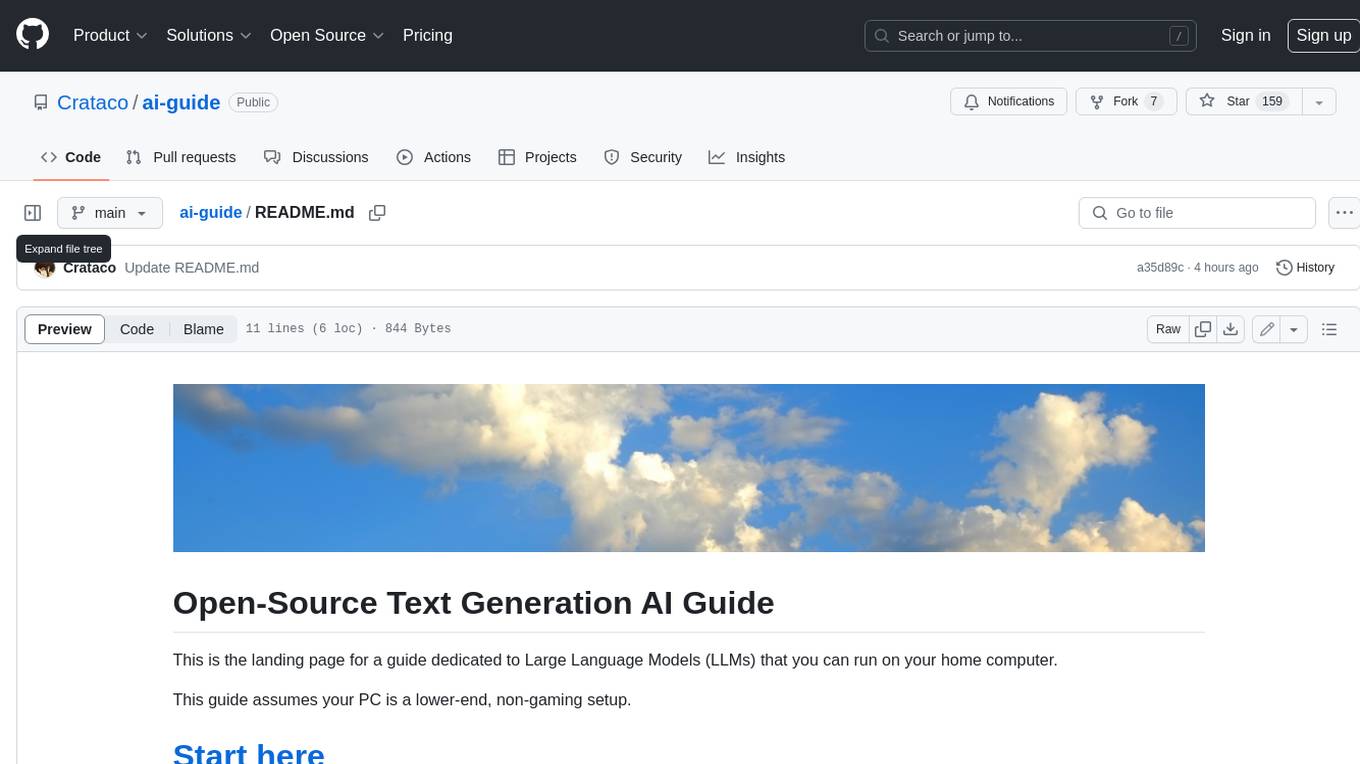
ai-guide
This guide is dedicated to Large Language Models (LLMs) that you can run on your home computer. It assumes your PC is a lower-end, non-gaming setup.
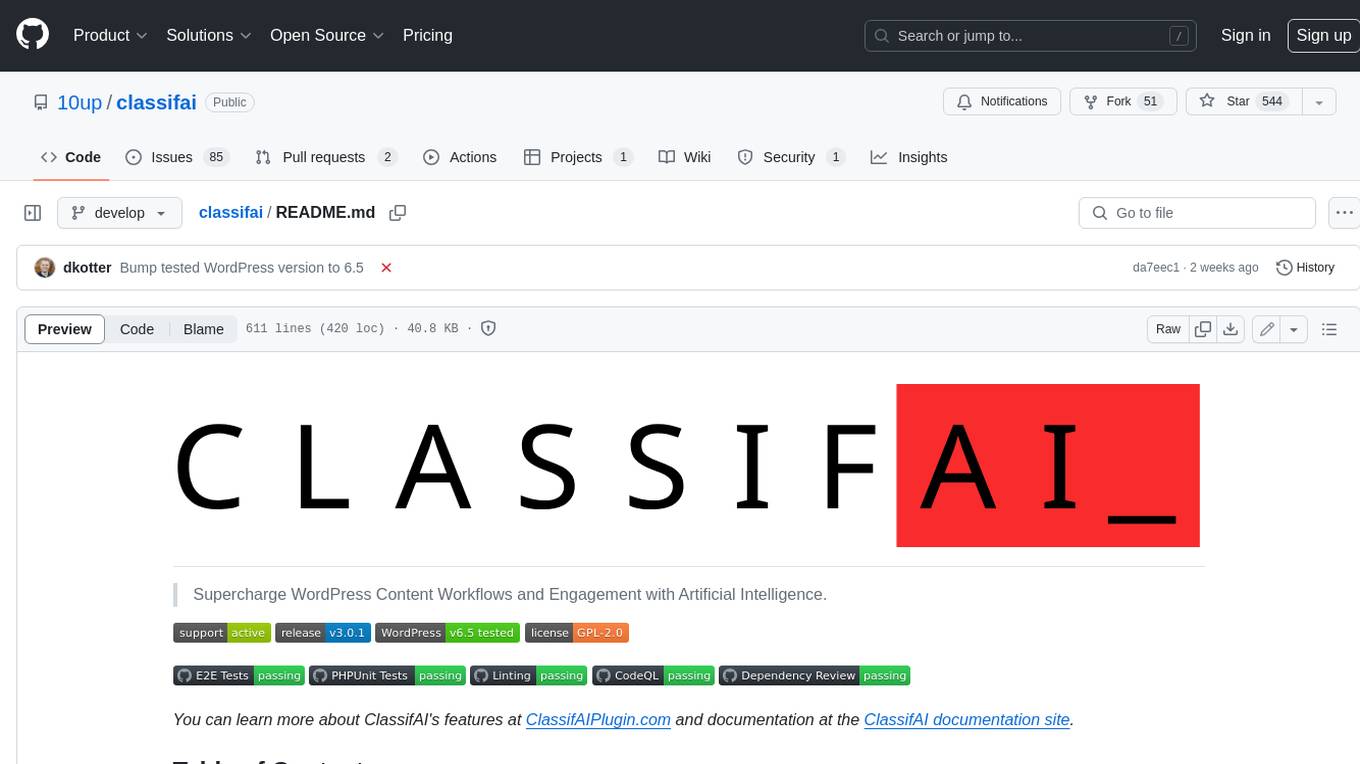
classifai
Supercharge WordPress Content Workflows and Engagement with Artificial Intelligence. Tap into leading cloud-based services like OpenAI, Microsoft Azure AI, Google Gemini and IBM Watson to augment your WordPress-powered websites. Publish content faster while improving SEO performance and increasing audience engagement. ClassifAI integrates Artificial Intelligence and Machine Learning technologies to lighten your workload and eliminate tedious tasks, giving you more time to create original content that matters.
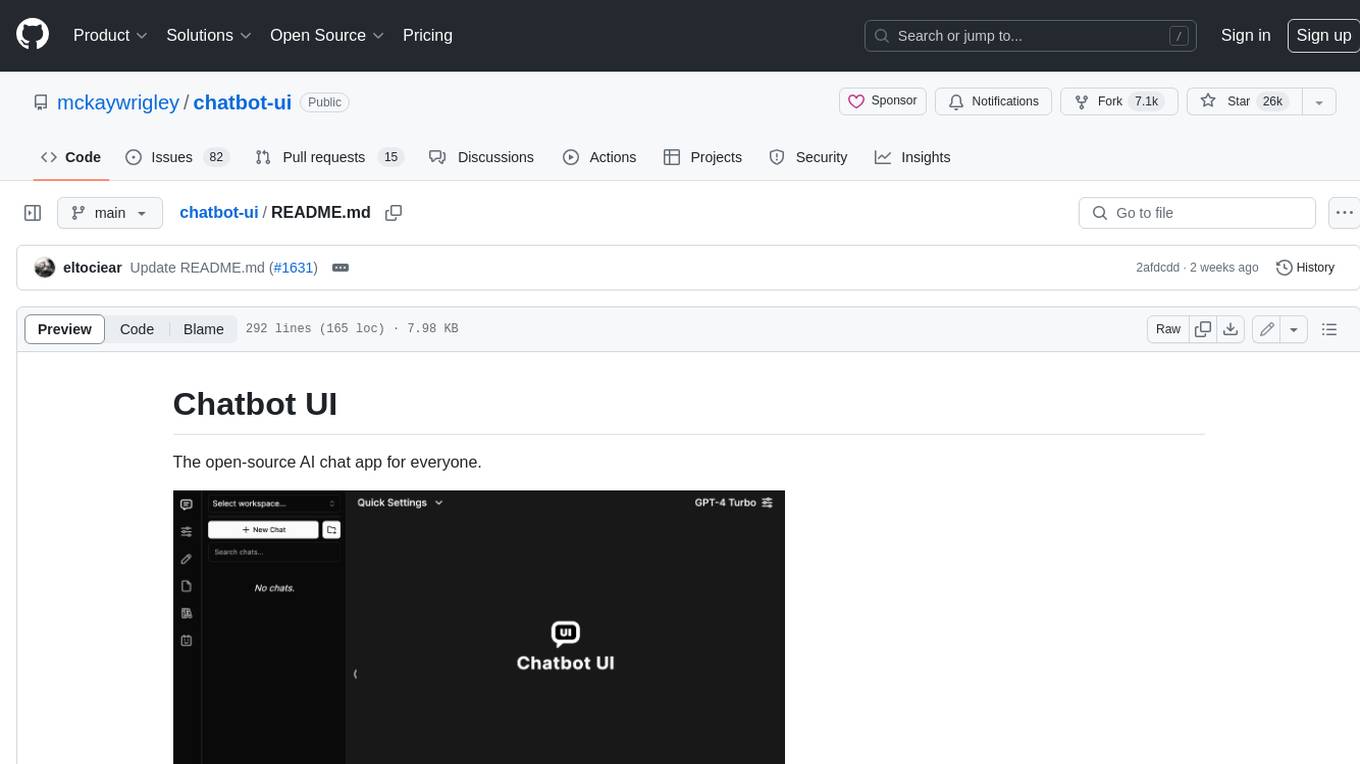
chatbot-ui
Chatbot UI is an open-source AI chat app that allows users to create and deploy their own AI chatbots. It is easy to use and can be customized to fit any need. Chatbot UI is perfect for businesses, developers, and anyone who wants to create a chatbot.
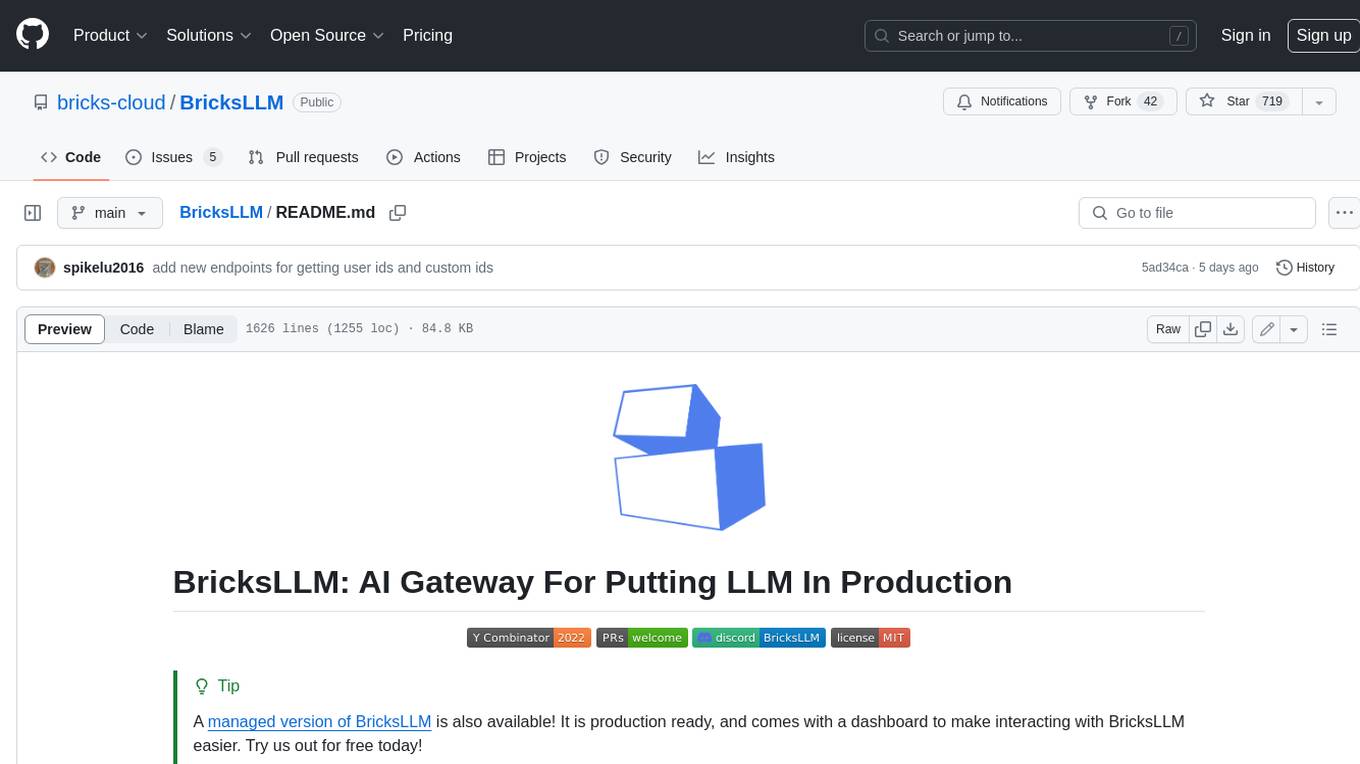
BricksLLM
BricksLLM is a cloud native AI gateway written in Go. Currently, it provides native support for OpenAI, Anthropic, Azure OpenAI and vLLM. BricksLLM aims to provide enterprise level infrastructure that can power any LLM production use cases. Here are some use cases for BricksLLM: * Set LLM usage limits for users on different pricing tiers * Track LLM usage on a per user and per organization basis * Block or redact requests containing PIIs * Improve LLM reliability with failovers, retries and caching * Distribute API keys with rate limits and cost limits for internal development/production use cases * Distribute API keys with rate limits and cost limits for students
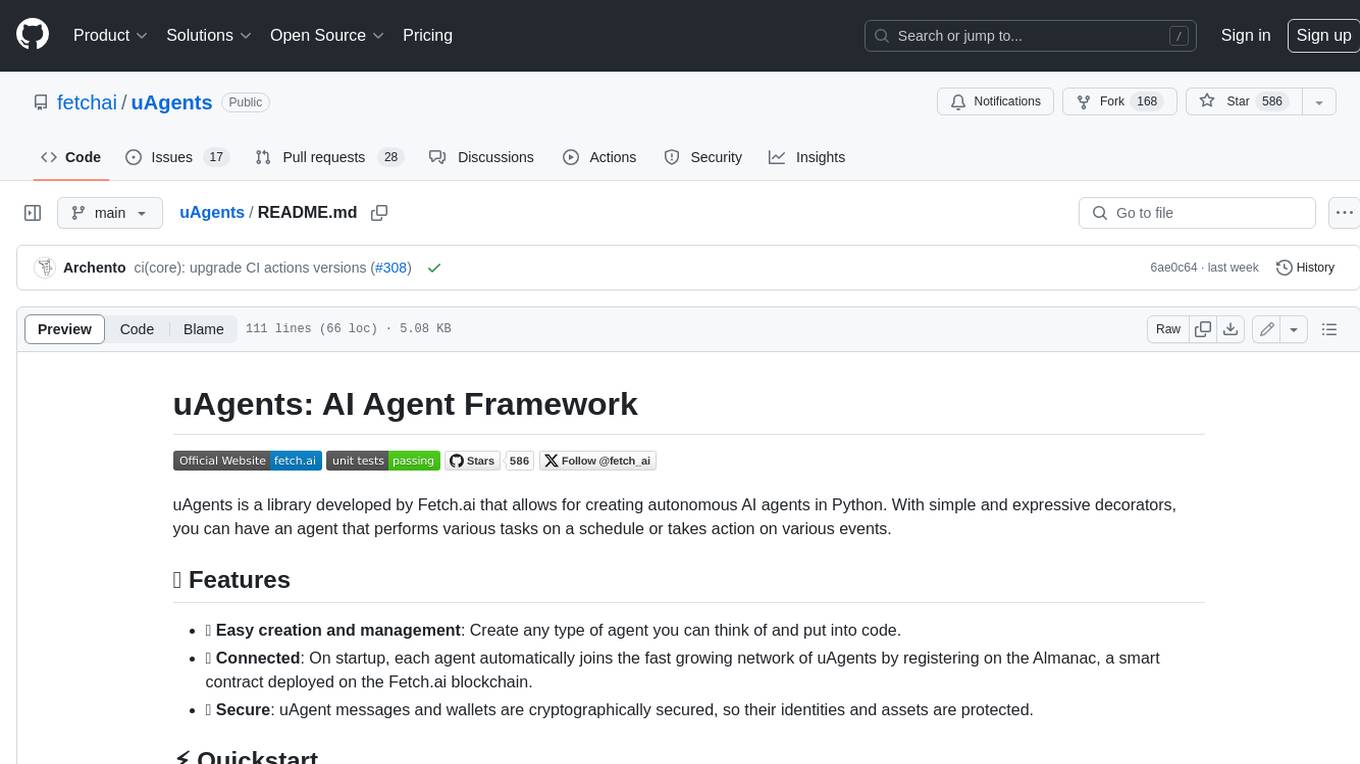
uAgents
uAgents is a Python library developed by Fetch.ai that allows for the creation of autonomous AI agents. These agents can perform various tasks on a schedule or take action on various events. uAgents are easy to create and manage, and they are connected to a fast-growing network of other uAgents. They are also secure, with cryptographically secured messages and wallets.
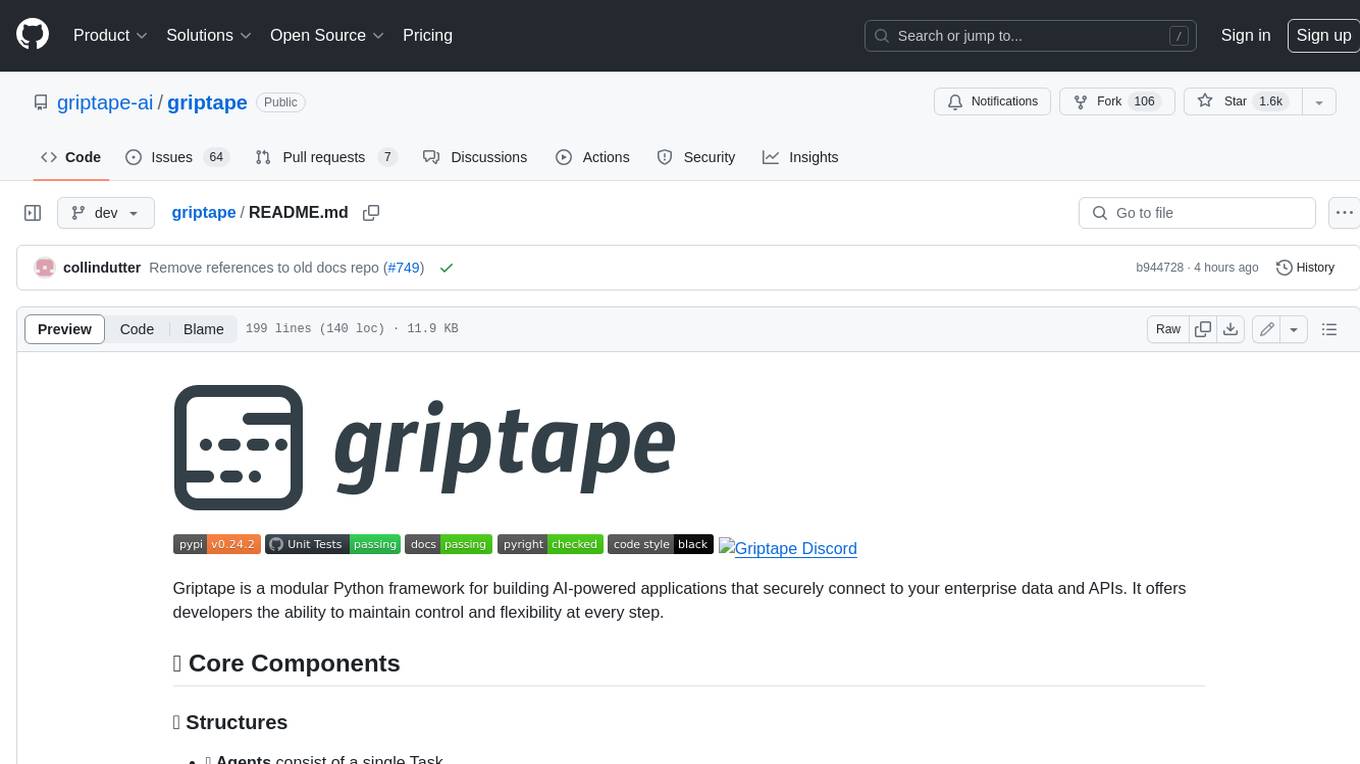
griptape
Griptape is a modular Python framework for building AI-powered applications that securely connect to your enterprise data and APIs. It offers developers the ability to maintain control and flexibility at every step. Griptape's core components include Structures (Agents, Pipelines, and Workflows), Tasks, Tools, Memory (Conversation Memory, Task Memory, and Meta Memory), Drivers (Prompt and Embedding Drivers, Vector Store Drivers, Image Generation Drivers, Image Query Drivers, SQL Drivers, Web Scraper Drivers, and Conversation Memory Drivers), Engines (Query Engines, Extraction Engines, Summary Engines, Image Generation Engines, and Image Query Engines), and additional components (Rulesets, Loaders, Artifacts, Chunkers, and Tokenizers). Griptape enables developers to create AI-powered applications with ease and efficiency.