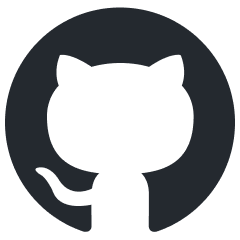
generative-ai-application-builder-on-aws
Generative AI Application Builder on AWS facilitates the development, rapid experimentation, and deployment of generative artificial intelligence (AI) applications without requiring deep experience in AI. The solution includes integrations with Amazon Bedrock and its included LLMs, such as Amazon Titan, and pre-built connectors for 3rd-party LLMs.
Stars: 143
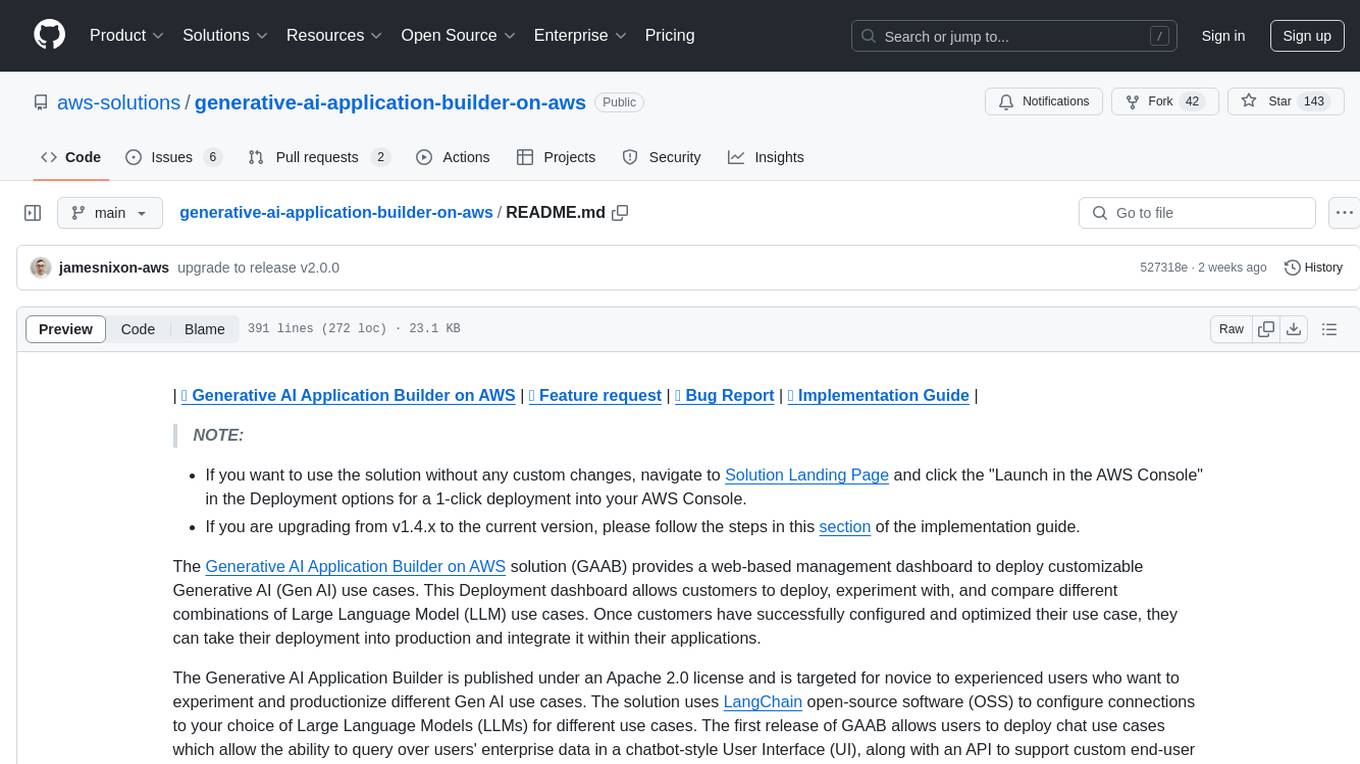
The Generative AI Application Builder on AWS (GAAB) is a solution that provides a web-based management dashboard for deploying customizable Generative AI (Gen AI) use cases. Users can experiment with and compare different combinations of Large Language Model (LLM) use cases, configure and optimize their use cases, and integrate them into their applications for production. The solution is targeted at novice to experienced users who want to experiment and productionize different Gen AI use cases. It uses LangChain open-source software to configure connections to Large Language Models (LLMs) for various use cases, with the ability to deploy chat use cases that allow querying over users' enterprise data in a chatbot-style User Interface (UI) and support custom end-user implementations through an API.
README:
| β¨ Generative AI Application Builder on AWS | π§ Feature request | π Bug Report | π Implementation Guide |
NOTE:
- If you want to use the solution without any custom changes, navigate to Solution Landing Page and click the "Launch in the AWS Console" in the Deployment options for a 1-click deployment into your AWS Console.
- If you are upgrading from v1.4.x to the current version, please follow the steps in this section of the implementation guide.
The Generative AI Application Builder on AWS solution (GAAB) provides a web-based management dashboard to deploy customizable Generative AI (Gen AI) use cases. This Deployment dashboard allows customers to deploy, experiment with, and compare different combinations of Large Language Model (LLM) use cases. Once customers have successfully configured and optimized their use case, they can take their deployment into production and integrate it within their applications.
The Generative AI Application Builder is published under an Apache 2.0 license and is targeted for novice to experienced users who want to experiment and productionize different Gen AI use cases. The solution uses LangChain open-source software (OSS) to configure connections to your choice of Large Language Models (LLMs) for different use cases. The first release of GAAB allows users to deploy chat use cases which allow the ability to query over users' enterprise data in a chatbot-style User Interface (UI), along with an API to support custom end-user implementations.
Some of the features of GAAB are:
- Rapid experimentation with ability to productionize at scale
- Extendable and modularized architecture using nested Amazon CloudFormation stacks
- Enterprise ready for company-specific data to tackle real-world business problems
- Integration with Amazon Bedrock and Amazon SageMaker as LLM providers
- Multi-LLM comparison and experimentation with metric tracking using Amazon CloudWatch dashboards
- Growing list of model providers and Gen AI use cases
For a detailed solution implementation guide, refer to The Generative AI Application Builder on AWS
- Architecture Overview
- Deployment
- Source code
- SageMaker Model Input Documentation
- Creating a custom build
There are 3 unique user personas that are referred to in the solution walkthrough below:
- The DevOps user is responsible for deploying the solution within the AWS account and for managing the infrastructure, updating the solution, monitoring performance, and maintaining the overall health and lifecycle of the solution.
- The admin users are responsible for managing the content contained within the deployment. These users gets access to the Deployment dashboard UI and is primarily responsible for curating the business user experience. This is our primary target customer.
- The business users represents the individuals who the use case has been deployed for. They are the consumers of the knowledge base and the customer responsible for evaluating and experimenting with the LLMs.
NOTE:
- You have the option of deploying the solution as a VPC enabled configuration. With a VPC enabled configuration, you can choose
- if the solution should build the VPC for this deployment.
- if you would like to deploy the solution in a VPC existing in your AWS account.
- To see the VPC related architecture diagrams, please visit the implementation guide.
When the DevOps user deploys the Deployment Dashboard, the following components are deployed in the AWS account:
- The admin users can log in to the deployed Deployment Dashboard UI.
- Amazon CloudFront delivers the web UI which is hosted in an Amazon S3 bucket.
- AWS WAF protects the APIs from attacks. This solution configures a set of rules called a web access control list (web ACL) that allows, blocks, or counts web requests based on configurable, user-defined web security rules and conditions.
- The web UI leverages a set of REST APIs that are exposed using Amazon API Gateway.
- Amazon Cognito authenticates users and backs both the Cloudfront web UI and API Gateway. An Amazon DynamoDB table is used to store the AWS IAM policy of authorized users.
- AWS Lambda is used to provide the business logic for the REST endpoints. This Backing Lambda will manage and create the necessary resources to perform use case deployments using AWS Cloudformation.
- Amazon DynamoDB is used to store the list of deployments.
- When a new use case is created by the admin user, the Backing Lambda will initiate a CloudFormation stack creation event for the requested use case.
- All of the LLM configuration options provided by the admin user in the deployment wizard are saved in DynamoDB. The deployment uses this DynamoDB table to configure the LLM at runtime.
- Using Amazon Cloudwatch, this solution collects operational metrics from various services to generate custom dashboards that allow you to monitor the solution's performance and operational health.
Note: Although the Deployment dashboard can be launched in most AWS regions, the deployed use cases have some restrictions based on service availability. See Supported AWS Regions in the Implementation Guide for more details.
Once the Deployment Dashboard is deployed, the admin user can then deploy multiple use case stacks. When a use case stack is deployed by the admin user, the following components are deployed in the AWS account:
- Admin users deploy the use case using the Deployment dashboard. Business users log in to the use case UI.
- Amazon CloudFront delivers the web UI which is hosted in an Amazon S3 bucket.
- The web UI leverages a WebSocket integration built using Amazon API Gateway. The API Gateway is backed by a custom Lambda Authorizer function, which returns the appropriate AWS IAM policy based on the Amazon Cognito group the authenticating user is part of. The policy is stored in Amazon DynamoDB.
- Amazon Cognito authenticates users and backs both the Cloudfront web UI and API Gateway.
- Incoming requests from the business user are passed from API Gateway to an Amazon SQS queue and then to the LangChain Orchestrator. The LangChain Orchestrator is a collection of Lambda functions and layers that provide the business logic for fulfilling requests coming from the business user. The queue enables the asynchronous operation of the API Gateway to Lambda integration. The queue passes connection information to the Lambda functions which will then post results directly back to the API Gateway websocket connection to support long running inference calls.
- The LangChain Orchestrator uses Amazon DynamoDB to get the configured LLM options and necessary session information (such as the chat history).
- If the deployment has a knowledge base configured, then the LangChain Orchestrator leverages Amazon Kendra or Knowledge Bases for Amazon Bedrock to run a search query to retrieve document excerpts.
- Using the chat history, query, and context from the knowledge base, the LangChain Orchestrator creates the final prompt and sends the request to the LLM hosted on Amazon Bedrock or Amazon SageMaker.
- When the response comes back from the LLM, the LangChain Orchestrator streams the response back through the API Gateway WebSocket to be consumed by the client application.
- Using Amazon CloudWatch, this solution collects operational metrics from various services to generate custom dashboards that allow you to monitor the deploymentβs performance and operational health.
NOTE:
- To use Amazon Bedrock, you must request access to models before they are available for use. Refer to Model access in the Amazon Bedrock User Guide for more details.
- You can also test the UI project locally by deploying the API endpoints and the rest of the infrastructure. To do so, follow either of the below two options and then refer Deployment Dashboard and Chat UI project for details.
There are two options for deployment into your AWS account:
Following are pre-requisites to build and deploy locally:
- Docker
- Nodejs 20.x
- CDK v2.118.0
-
Python >= 3.11, <=3.12.1
- Note: normal python installations should include support for
ensurepip
andpip
; however, if running in an environment without these packages you will need to manually install them (e.g. a minimal docker image). See pip's installation guide for details.
- Note: normal python installations should include support for
- AWS CLI
- jq
Note: Configure the AWS CLI with your AWS credentials or have them exported in the CLI terminal environment. In case the credentials are invalid or expired, running cdk deploy
produces an error.
Also, if you have not run cdk bootstrap
in this account and region, please follow the instructions here to execute cdk bootstrap as a one time process before proceeding with the below steps.
After cloning the repo from GitHub, complete the following steps:
NOTE:
- Please update the
cdk-asset-bucket
property insource/infrastructure/cdk.json
. The value of this property should be the bucket name thatcdk bootstrap
process created. Unless a bucket name is configured, thecdk bootstrap
process creates a bucket in the following format:cdk-hnb659fds-assets-<aws-account-number>-<region>
(where is the AWS Account ID where the solution is being deployed in the specific ). The repo currently has the value set as the following:
If deployed with this default value, the use case creation from deployment dashboards would fail."cdk-asset-bucket": "cdk-hnb659fds-assets-123456789012-us-east-1"
cd <project-directory>/source/infrastructure
npm install
npm run build
cdk synth
cdk deploy DeploymentPlatformStack --parameters AdminUserEmail=<replace with admin user's email>
Note: Because cdk deploy
is executed with a stack name, it does not synthesize the other CloudFormation stacks in the infrastructure folder. To ensure all stacks are synthesized based on the infrastructure code changes, please ensure to cdk synth
. For a complete list of cdk commands that can be run, see Toolkit commands
For the deployment dashboard to deploy LLM chat use cases, you would additionally need to stage synthesized CDK assets (such as lambdas, synthesized CloudFormation templates, etc.) from the source/infrastructure/cdk.out
directory to a configured S3 bucket in your account from where these resources will be pulled from at the time of deployment. To make it easy to stage these assets, you can use the source/stage-assets.sh
script. This script should be run from the source
directory.
cd <project-directory>/source
./stage-assets.sh
When run, the script looks like this:
>>> ./stage-assets.sh
This script should be run from the 'source' folder
The region to upload CDK artifacts to (default:us-east-1)?
>>> us-west-2
>>> All assets will be uploaded to cdk-hnb659fds-assets-123456789-us-west-2
>>> Do you want to proceed? (y/n) y
You must provide the full region name as the first input to the script as shown in the above example.
Note: Assets must be staged every time there is a change in the codebase to have the most up-to-date staged assets. It is also recommend to run cdk synth
before staging.
Refer section Creating a custom build
βββ CHANGELOG.md
βββ CODE_OF_CONDUCT.md
βββ CONTRIBUTING.md
βββ Config
βββ LICENSE.txt
βββ NOTICE.txt
βββ README.md
βββ buildspec.yml
βββ deployment
β βββ build-open-source-dist.sh
β βββ build-s3-dist.sh
β βββ cdk-solution-helper
β βββ clean-for-scan.sh
β βββ get-cdk-version.js
β βββ manifest.yaml
| βββ run-unit-tests.sh [shell script that can run unit tests for the entire project]
βββ pyproject.toml
βββ pytest.ini
βββ sonar-project.properties
βββ source
βββ images
βββ infrastructure [CDK infrastructure]
βββ lambda [Lambda functions for the application]
βββ pre-build-lambda-layers.sh [pre-builds lambda layers for the project]
βββ scripts [standalone utility scripts]
βββ stage-assets.sh
βββ test
βββ ui-chat [Web App project for chat UI]
βββ ui-deployment [Web App project for deployment dashboard UI]
βββ docs
The project provides a docs folder which gives you access to sample SageMaker inputs. As SageMaker models can take in and output a variety of input and output schemas, respectively, the solution requests these values from the users to allow correct model invocation. This allows the solution to support a wide set of SageMaker models.
The input schemas are essentially your model's payload, with placeholders for the actual values. The placeholders enable replacing the actual model values at run-time and are represented by a keyword enclosed in angle brackets like: <<prompt>>
. Note that <<prompt>>
and <<temperature>>
are reserved placeholders for the model prompt and temperatures respectively.
The model's output JSONPath provides the solution a path to retrieve the LLM's textual response from the model response.
Please always refer to model documentation and SageMaker JumpStart jupyter notebook samples to see the most up-to-date model payloads and supported parameters.
Run the following command:
git clone https://github.com/aws-solutions/<repository_name>
- Install the dependencies:
cd <rootDir>/source/infrastructure
npm install
- (Optional) Run the unit tests:
Note: To run the unit tests, docker must be installed and running, and valid AWS credentials must be configured.
cd <rootDir>/deployment
chmod +x ./run-unit-tests.sh
./run-unit-tests.sh
- Configure the bucket name of your target Amazon S3 distribution bucket:
export DIST_OUTPUT_BUCKET=my-bucket-name
export VERSION=my-version
- Build the distributable:
cd <rootDir>/deployment
chmod +x ./build-s3-dist.sh
./build-s3-dist.sh $DIST_OUTPUT_BUCKET $SOLUTION_NAME $VERSION $CF_TEMPLATE_BUCKET_NAME
Parameter details:
$DIST_OUTPUT_BUCKET - This is the global name of the distribution. For the bucket name, the AWS Region is added to the global name (example: 'my-bucket-name-us-east-1') to create a regional bucket. The lambda
artifacts should be uploaded to the regional buckets for the CloudFormation template to pick it up for deployment.
$SOLUTION_NAME - The name of This solution (example: generative-ai-application-builder-on-aws)
$VERSION - The version number of the change
$CF_TEMPLATE_BUCKET_NAME - The name of the S3 bucket where the CloudFormation templates should be uploaded
When you create and use buckets, we recommended that you:
- Use randomized names or uuid as part of your bucket naming strategy.
- Ensure that buckets aren't public.
- Verify bucket ownership prior to uploading templates or code artifacts.
- Deploy the distributable to an Amazon S3 bucket in your account.
Note: You must have the AWS CLI installed.
aws s3 cp ./global-s3-assets/ s3://my-bucket-name-<aws_region>/generative-ai-application-builder-on-aws/<my-version>/ --recursive --acl bucket-owner-full-control --profile aws-cred-profile-name
aws s3 cp ./regional-s3-assets/ s3://my-bucket-name-<aws_region>/generative-ai-application-builder-on-aws/<my-version>/ --recursive --acl bucket-owner-full-control --profile aws-cred-profile-name
The solution includes two separate React-based web applications: the Deployment Dashboard UI and the Chat UI. Both user interfaces are built using Vite, a modern and fast build tool for modern web projects.
- Node.js (version 16 or later)
- npm (usually included with Node.js)
-
Open a Terminal
- On Windows, you can use the built-in Command Prompt, PowerShell, or a terminal emulator like Git Bash.
- On macOS or Linux, open the default terminal application.
-
Navigate to the Source Directory
- Change to the
source
directory within the project root:cd <project-directory>/source
- Change to the
-
Fetch and Update Runtime Config
-
Run the provided
setup_local_dev_server.py
script with the appropriate arguments:python setup_local_dev_server.py --ui-project-type <ui_project_name> --website <website_url>
- Replace
<ui_project_name>
with eitherui-chat
orui-deployment
depending on which UI project you want to set up. - Replace
<website_url>
with the URL of the website where the solution is deployed (e.g.,https://example.com
).
- Replace
-
The script will fetch the runtime configuration used to configure AWS Amplify, which the project uses. It will also update the Cognito app client by adding the localhost address to the callbackurls and logouturls list, so it works with the Cognito hosted domain.
-
For example, to update the UI Chat project:
python setup_local_dev_server.py --ui-project-type ui-chat --website https://example.com
or
python setup_local_dev_server.py -t ui-chat -w https://example.com
-
To update the UI Deployment project:
python setup_local_dev_server.py --ui-project-type ui-deployment --website https://example.com
or
python setup_local_dev_server.py -t ui-deployment -w https://example.com
-
-
Navigate to the UI Project Directory
-
Change to the directory of the UI project you want to work with:
cd ui-chat
or
cd ui-deployment
-
-
Install Dependencies
-
Install the required Node.js dependencies by running the following command in the project directory:
npm install
-
-
Start the Local Development Server
-
In the project directory, run the following command to start the local Vite development server:
npm start
-
The Deployment Dashboard UI development server will start at
http://localhost:5177
, and the Chat UI development server will start athttp://localhost:5178
. -
If these ports are already in use on your machine, you can customize the
setup_local_dev_server.py
script to use different ports.
-
With the local development environment set up, you can now customize the React components and functionality of the UI projects according to your requirements. The source code for the Deployment Dashboard UI is located in the source/ui-deployment
directory, and the source code for the Chat UI is located in the source/ui-chat
directory.
Follow the standard React development workflow to make changes to the code, and use the local Vite development server to preview your changes in real-time.
When you're ready to deploy your customized UI projects, follow the instructions in the main README file for building and deploying the solution using the AWS CDK.
This solution collects anonymized operational metrics to help AWS improve the quality and features of the solution. For more information, including how to disable this capability, please see the implementation guide.
Copyright Amazon.com, Inc. or its affiliates. All Rights Reserved.
Licensed under the Apache License, Version 2.0 (the "License"); you may not use this file except in compliance with the License. You may obtain a copy of the License at
http://www.apache.org/licenses/LICENSE-2.0
Unless required by applicable law or agreed to in writing, software distributed under the License is distributed on an "AS IS" BASIS, WITHOUT WARRANTIES OR CONDITIONS OF ANY KIND, either express or implied. See the License for the specific language governing permissions and limitations under the License.
A script is included to migrate use cases created by v1.X to an upgraded v2.X deployment. See the documentation
For Tasks:
Click tags to check more tools for each tasksFor Jobs:
Alternative AI tools for generative-ai-application-builder-on-aws
Similar Open Source Tools
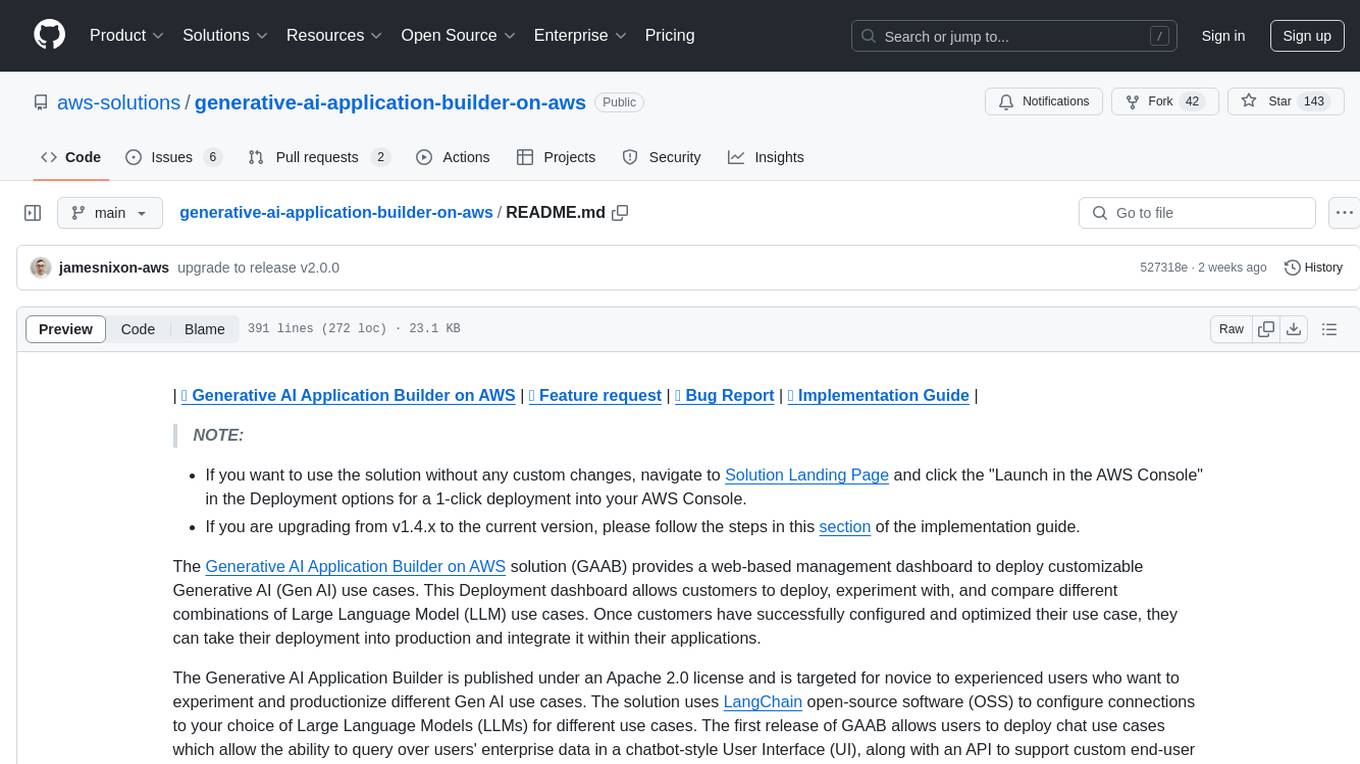
generative-ai-application-builder-on-aws
The Generative AI Application Builder on AWS (GAAB) is a solution that provides a web-based management dashboard for deploying customizable Generative AI (Gen AI) use cases. Users can experiment with and compare different combinations of Large Language Model (LLM) use cases, configure and optimize their use cases, and integrate them into their applications for production. The solution is targeted at novice to experienced users who want to experiment and productionize different Gen AI use cases. It uses LangChain open-source software to configure connections to Large Language Models (LLMs) for various use cases, with the ability to deploy chat use cases that allow querying over users' enterprise data in a chatbot-style User Interface (UI) and support custom end-user implementations through an API.
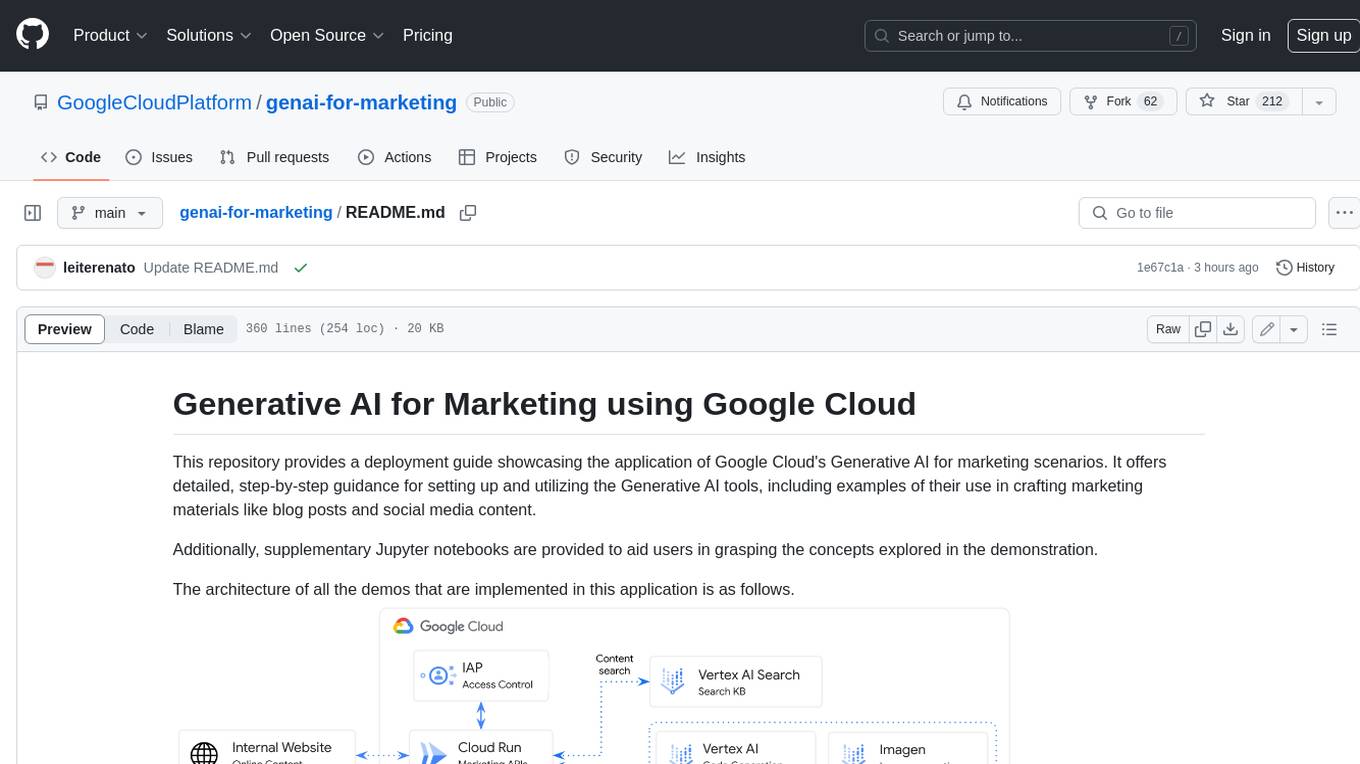
genai-for-marketing
This repository provides a deployment guide for utilizing Google Cloud's Generative AI tools in marketing scenarios. It includes step-by-step instructions, examples of crafting marketing materials, and supplementary Jupyter notebooks. The demos cover marketing insights, audience analysis, trendspotting, content search, content generation, and workspace integration. Users can access and visualize marketing data, analyze trends, improve search experience, and generate compelling content. The repository structure includes backend APIs, frontend code, sample notebooks, templates, and installation scripts.
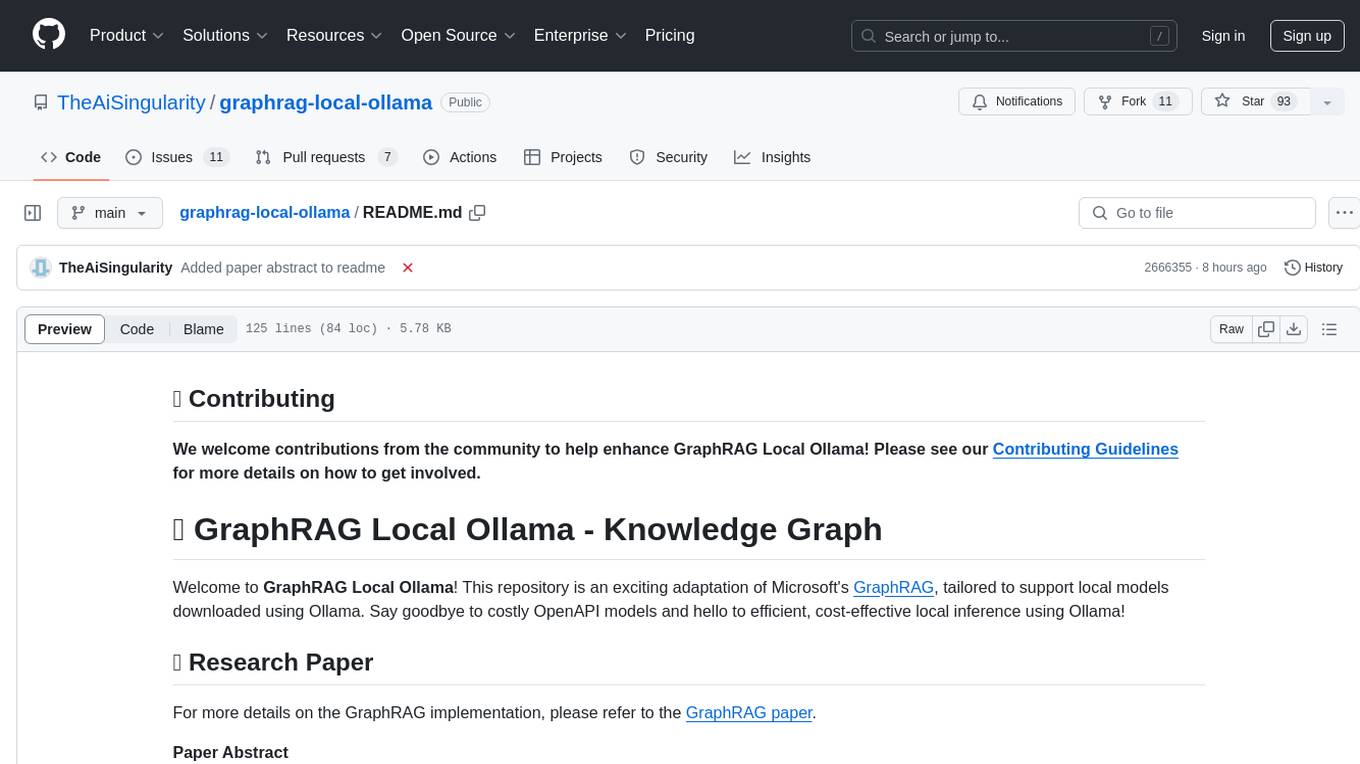
graphrag-local-ollama
GraphRAG Local Ollama is a repository that offers an adaptation of Microsoft's GraphRAG, customized to support local models downloaded using Ollama. It enables users to leverage local models with Ollama for large language models (LLMs) and embeddings, eliminating the need for costly OpenAPI models. The repository provides a simple setup process and allows users to perform question answering over private text corpora by building a graph-based text index and generating community summaries for closely-related entities. GraphRAG Local Ollama aims to improve the comprehensiveness and diversity of generated answers for global sensemaking questions over datasets.
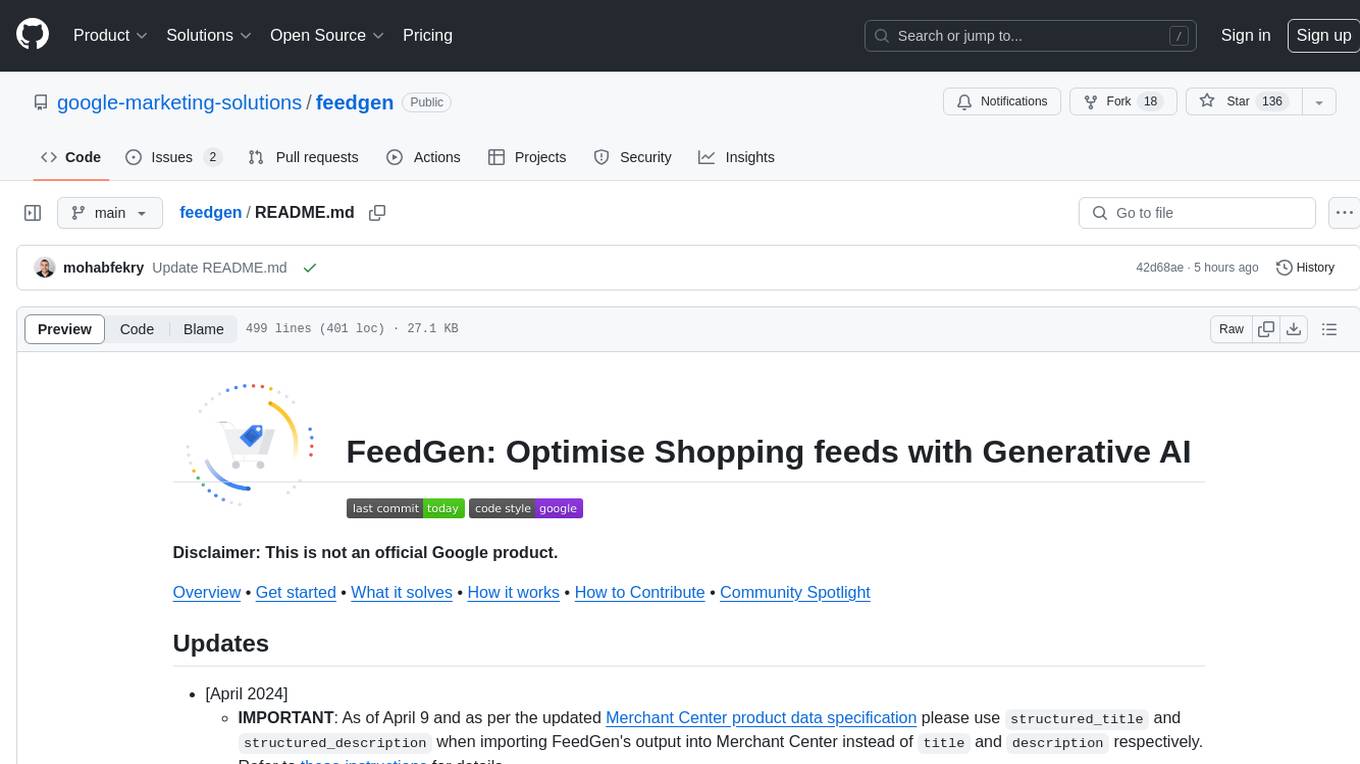
feedgen
FeedGen is an open-source tool that uses Google Cloud's state-of-the-art Large Language Models (LLMs) to improve product titles, generate more comprehensive descriptions, and fill missing attributes in product feeds. It helps merchants and advertisers surface and fix quality issues in their feeds using Generative AI in a simple and configurable way. The tool relies on GCP's Vertex AI API to provide both zero-shot and few-shot inference capabilities on GCP's foundational LLMs. With few-shot prompting, users can customize the model's responses towards their own data, achieving higher quality and more consistent output. FeedGen is an Apps Script based application that runs as an HTML sidebar in Google Sheets, allowing users to optimize their feeds with ease.
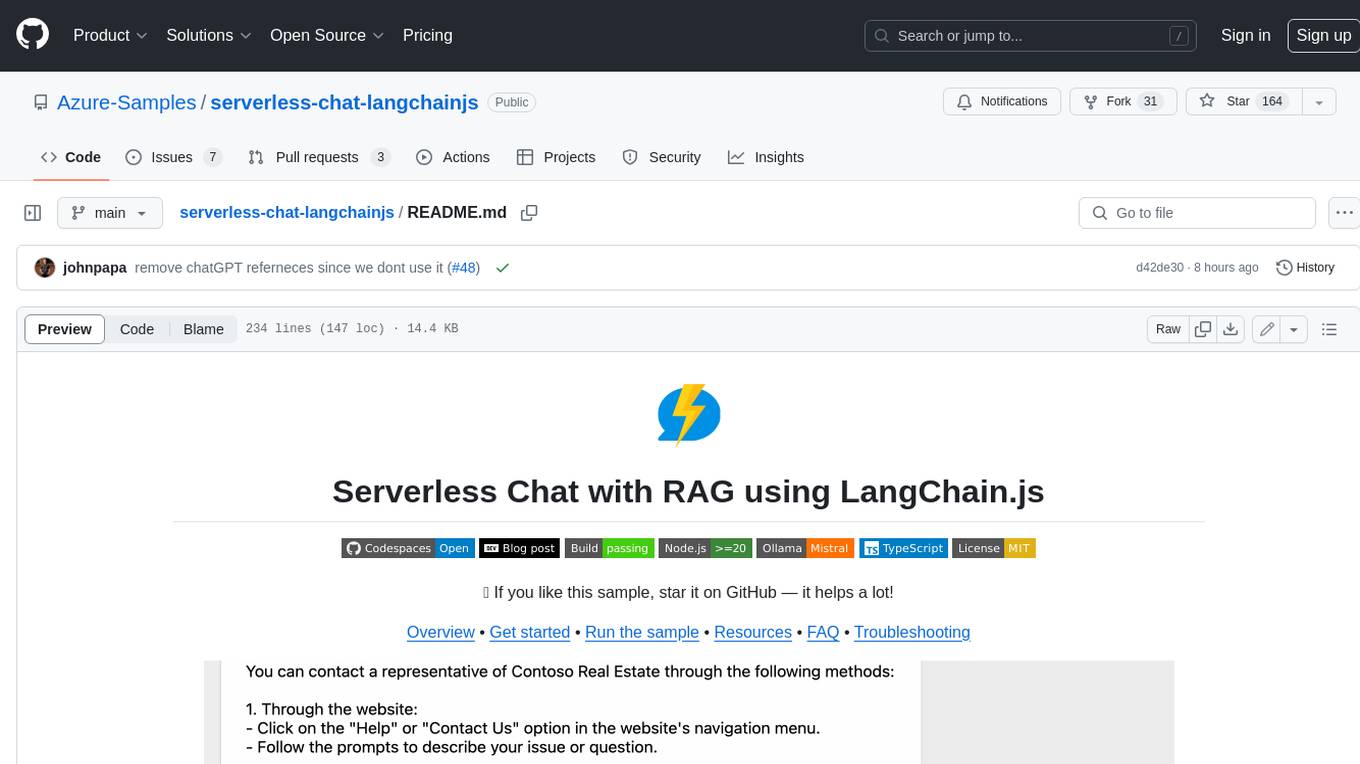
serverless-chat-langchainjs
This sample shows how to build a serverless chat experience with Retrieval-Augmented Generation using LangChain.js and Azure. The application is hosted on Azure Static Web Apps and Azure Functions, with Azure Cosmos DB for MongoDB vCore as the vector database. You can use it as a starting point for building more complex AI applications.
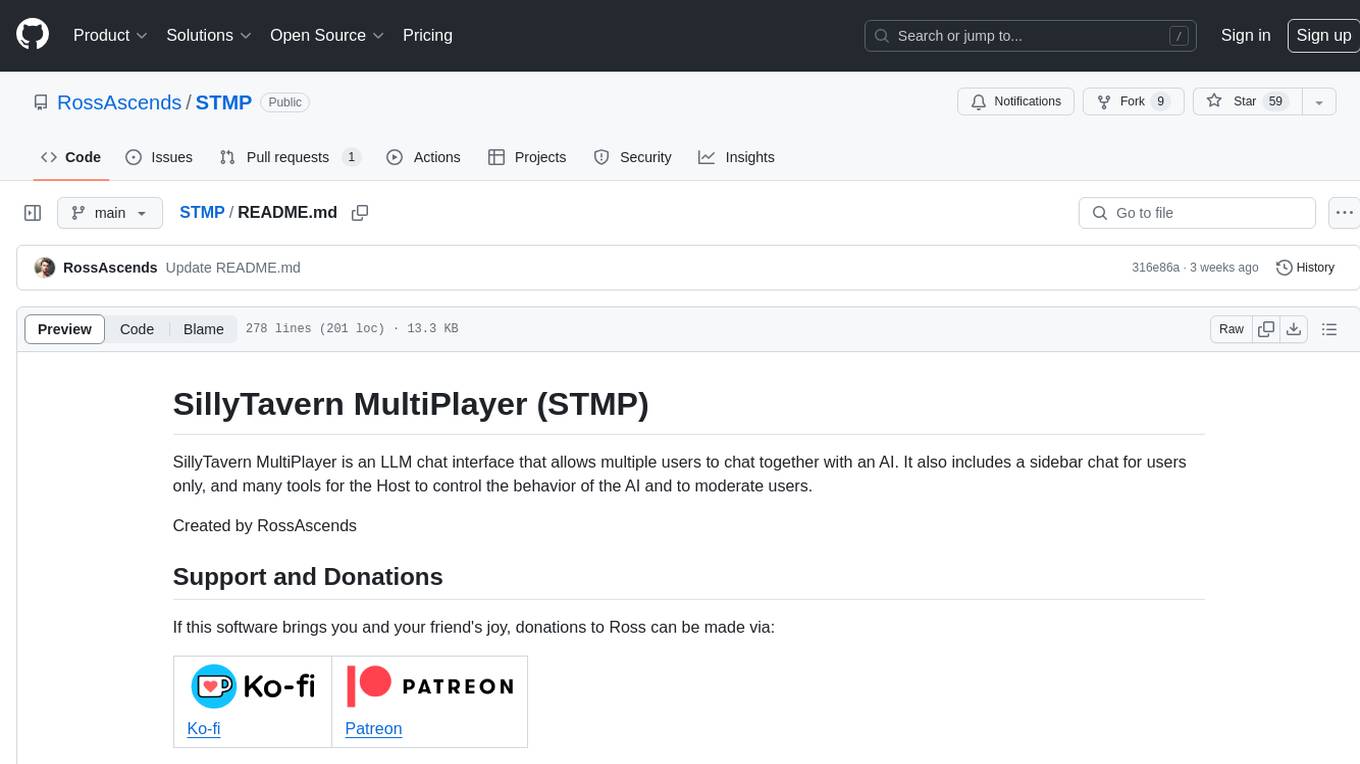
STMP
SillyTavern MultiPlayer (STMP) is an LLM chat interface that enables multiple users to chat with an AI. It features a sidebar chat for users, tools for the Host to manage the AI's behavior and moderate users. Users can change display names, chat in different windows, and the Host can control AI settings. STMP supports Text Completions, Chat Completions, and HordeAI. Users can add/edit APIs, manage past chats, view user lists, and control delays. Hosts have access to various controls, including AI configuration, adding presets, and managing characters. Planned features include smarter retry logic, host controls enhancements, and quality of life improvements like user list fading and highlighting exact usernames in AI responses.
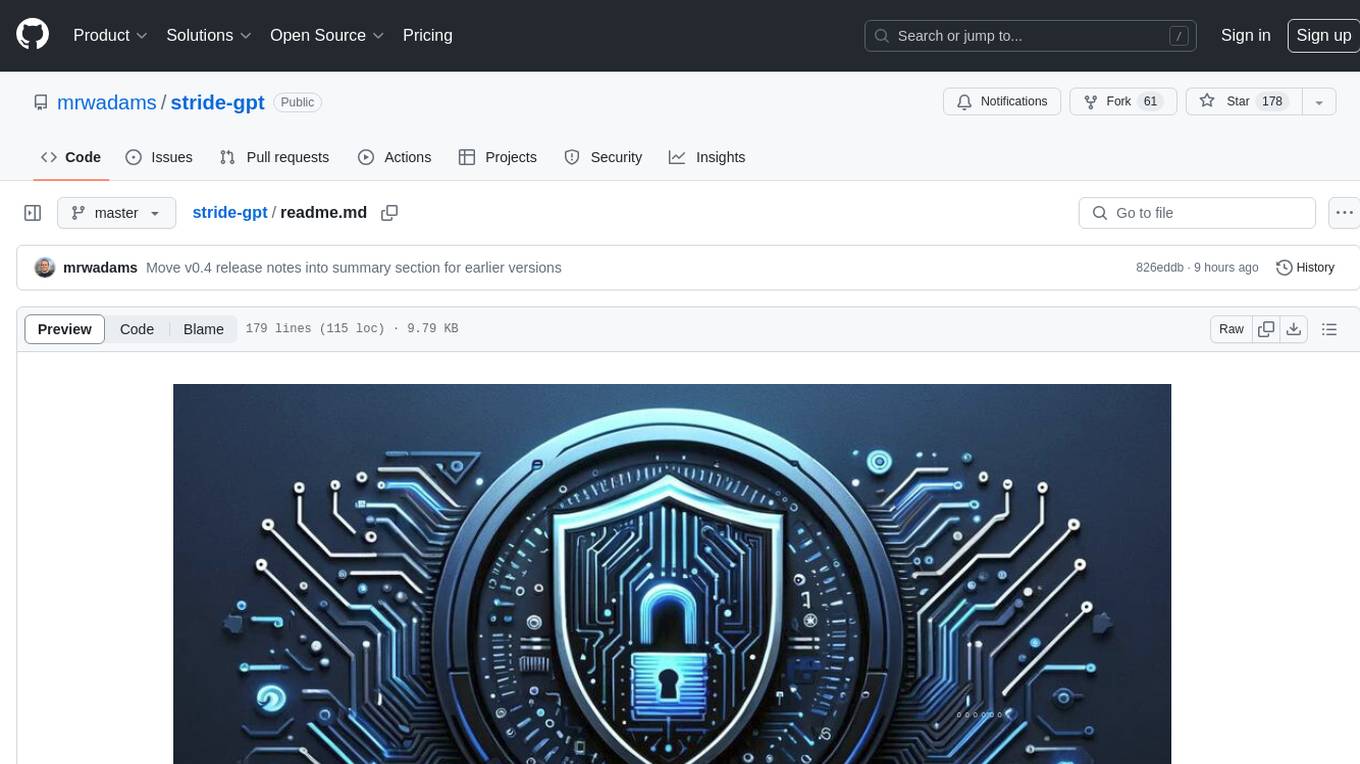
stride-gpt
STRIDE GPT is an AI-powered threat modelling tool that leverages Large Language Models (LLMs) to generate threat models and attack trees for a given application based on the STRIDE methodology. Users provide application details, such as the application type, authentication methods, and whether the application is internet-facing or processes sensitive data. The model then generates its output based on the provided information. It features a simple and user-friendly interface, supports multi-modal threat modelling, generates attack trees, suggests possible mitigations for identified threats, and does not store application details. STRIDE GPT can be accessed via OpenAI API, Azure OpenAI Service, Google AI API, or Mistral API. It is available as a Docker container image for easy deployment.
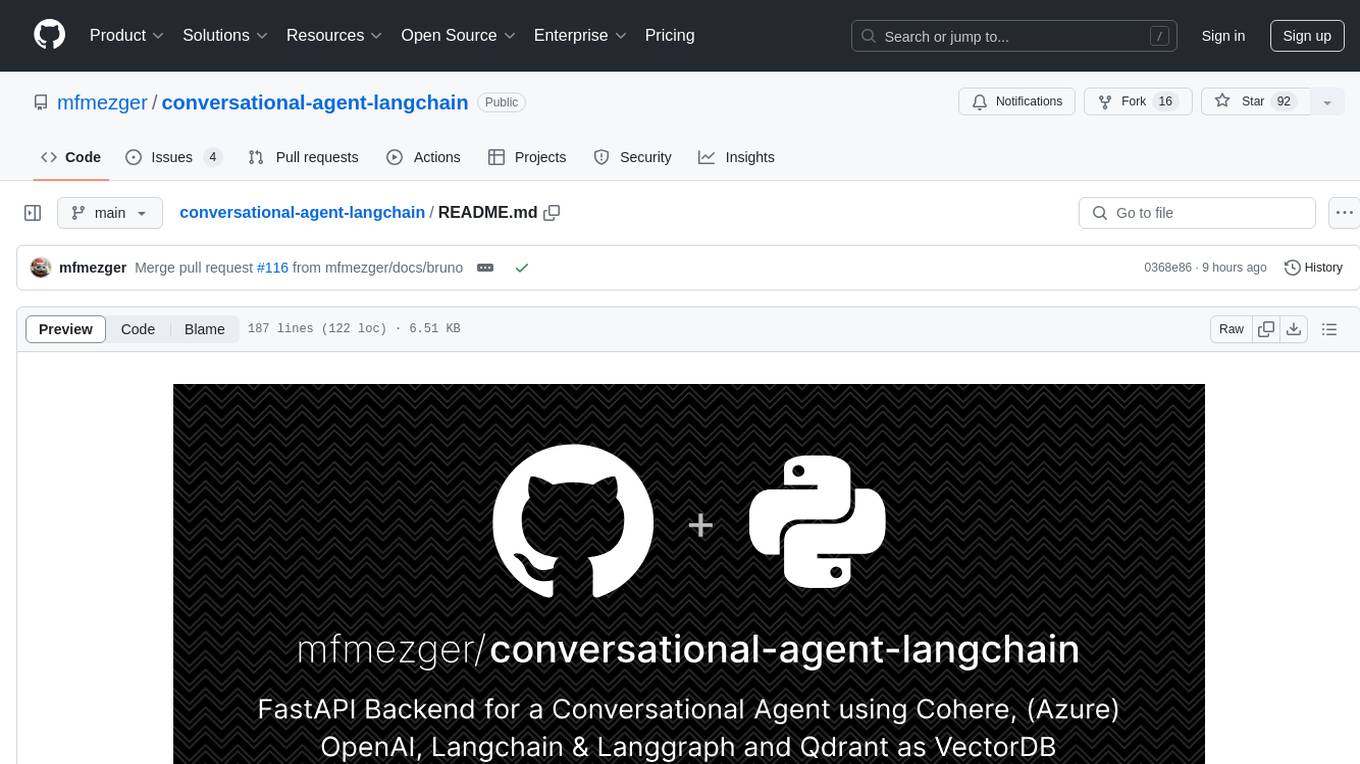
conversational-agent-langchain
This repository contains a Rest-Backend for a Conversational Agent that allows embedding documents, semantic search, QA based on documents, and document processing with Large Language Models. It uses Aleph Alpha and OpenAI Large Language Models to generate responses to user queries, includes a vector database, and provides a REST API built with FastAPI. The project also features semantic search, secret management for API keys, installation instructions, and development guidelines for both backend and frontend components.
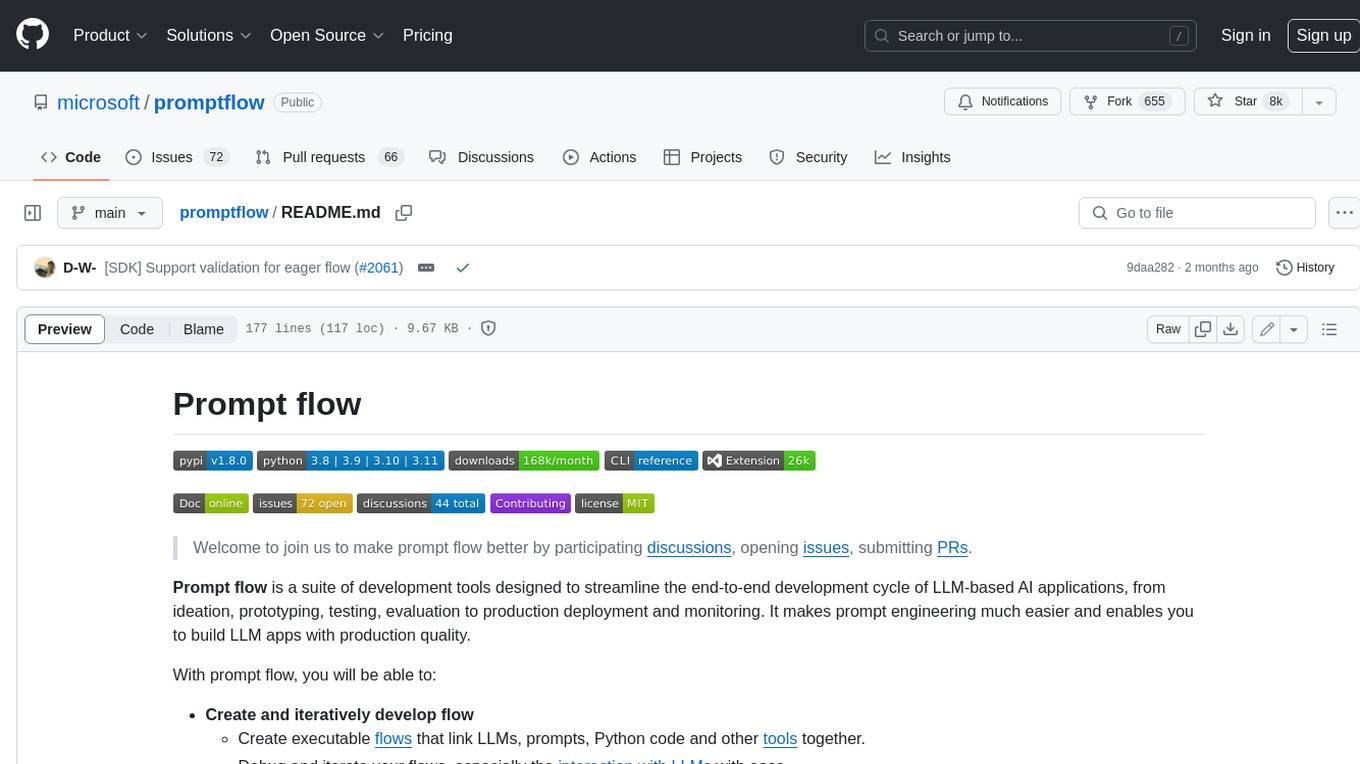
promptflow
**Prompt flow** is a suite of development tools designed to streamline the end-to-end development cycle of LLM-based AI applications, from ideation, prototyping, testing, evaluation to production deployment and monitoring. It makes prompt engineering much easier and enables you to build LLM apps with production quality.
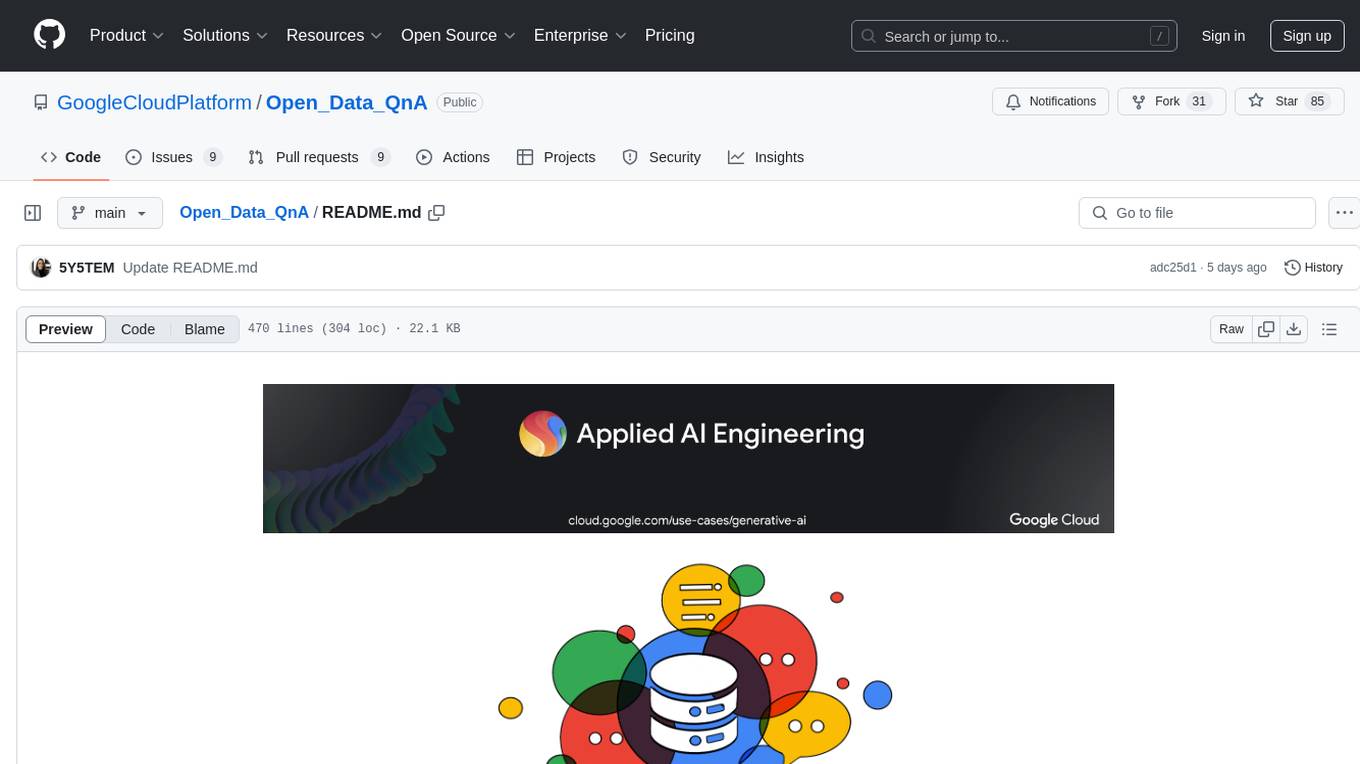
Open_Data_QnA
Open Data QnA is a Python library that allows users to interact with their PostgreSQL or BigQuery databases in a conversational manner, without needing to write SQL queries. The library leverages Large Language Models (LLMs) to bridge the gap between human language and database queries, enabling users to ask questions in natural language and receive informative responses. It offers features such as conversational querying with multiturn support, table grouping, multi schema/dataset support, SQL generation, query refinement, natural language responses, visualizations, and extensibility. The library is built on a modular design and supports various components like Database Connectors, Vector Stores, and Agents for SQL generation, validation, debugging, descriptions, embeddings, responses, and visualizations.
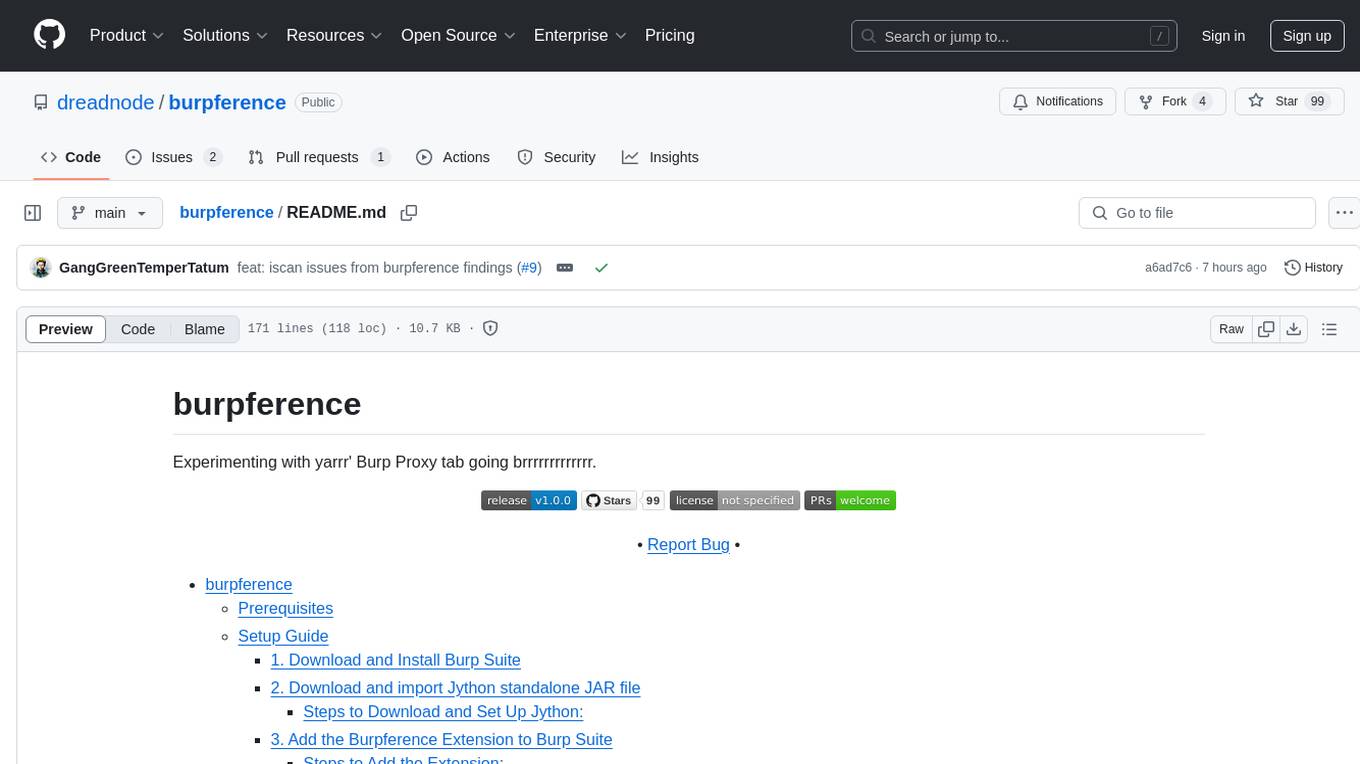
burpference
Burpference is an open-source extension designed to capture in-scope HTTP requests and responses from Burp's proxy history and send them to a remote LLM API in JSON format. It automates response capture, integrates with APIs, optimizes resource usage, provides color-coded findings visualization, offers comprehensive logging, supports native Burp reporting, and allows flexible configuration. Users can customize system prompts, API keys, and remote hosts, and host models locally to prevent high inference costs. The tool is ideal for offensive web application engagements to surface findings and vulnerabilities.
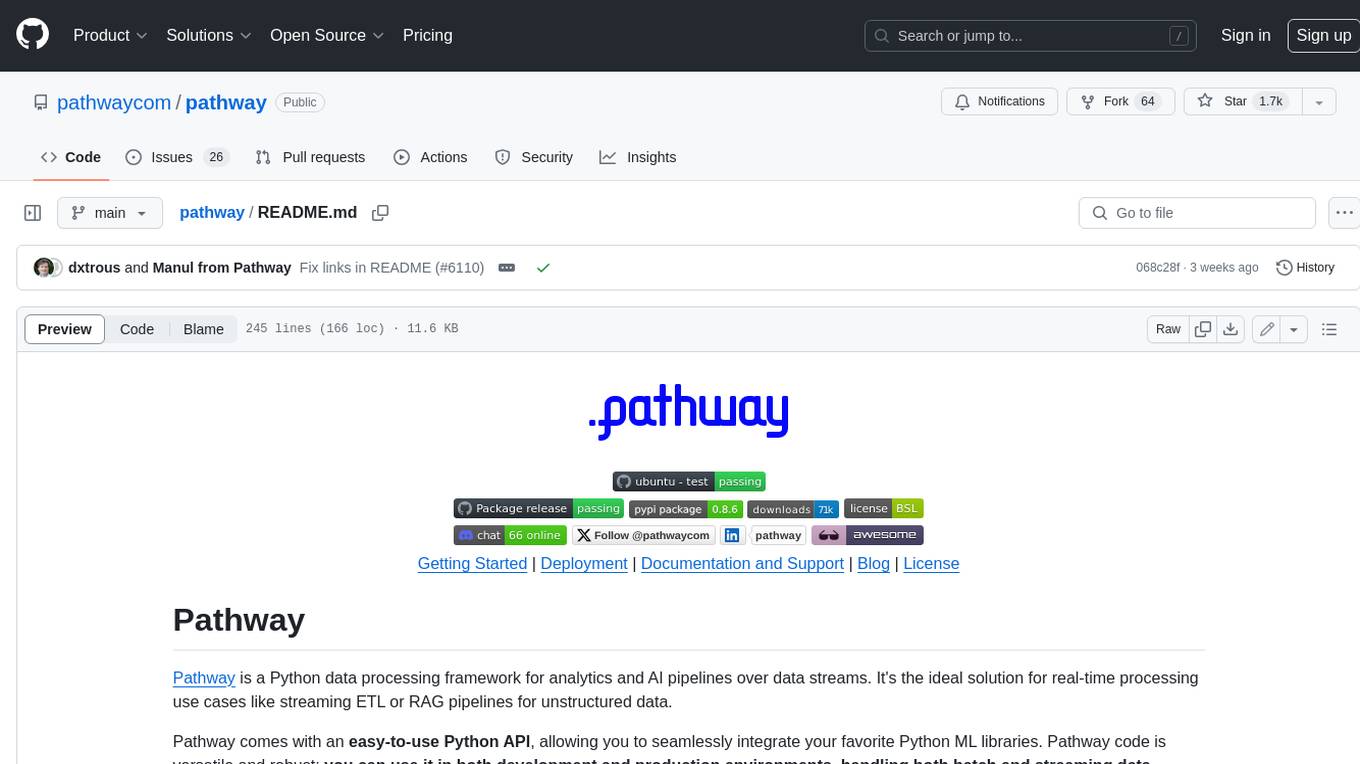
pathway
Pathway is a Python data processing framework for analytics and AI pipelines over data streams. It's the ideal solution for real-time processing use cases like streaming ETL or RAG pipelines for unstructured data. Pathway comes with an **easy-to-use Python API** , allowing you to seamlessly integrate your favorite Python ML libraries. Pathway code is versatile and robust: **you can use it in both development and production environments, handling both batch and streaming data effectively**. The same code can be used for local development, CI/CD tests, running batch jobs, handling stream replays, and processing data streams. Pathway is powered by a **scalable Rust engine** based on Differential Dataflow and performs incremental computation. Your Pathway code, despite being written in Python, is run by the Rust engine, enabling multithreading, multiprocessing, and distributed computations. All the pipeline is kept in memory and can be easily deployed with **Docker and Kubernetes**. You can install Pathway with pip: `pip install -U pathway` For any questions, you will find the community and team behind the project on Discord.
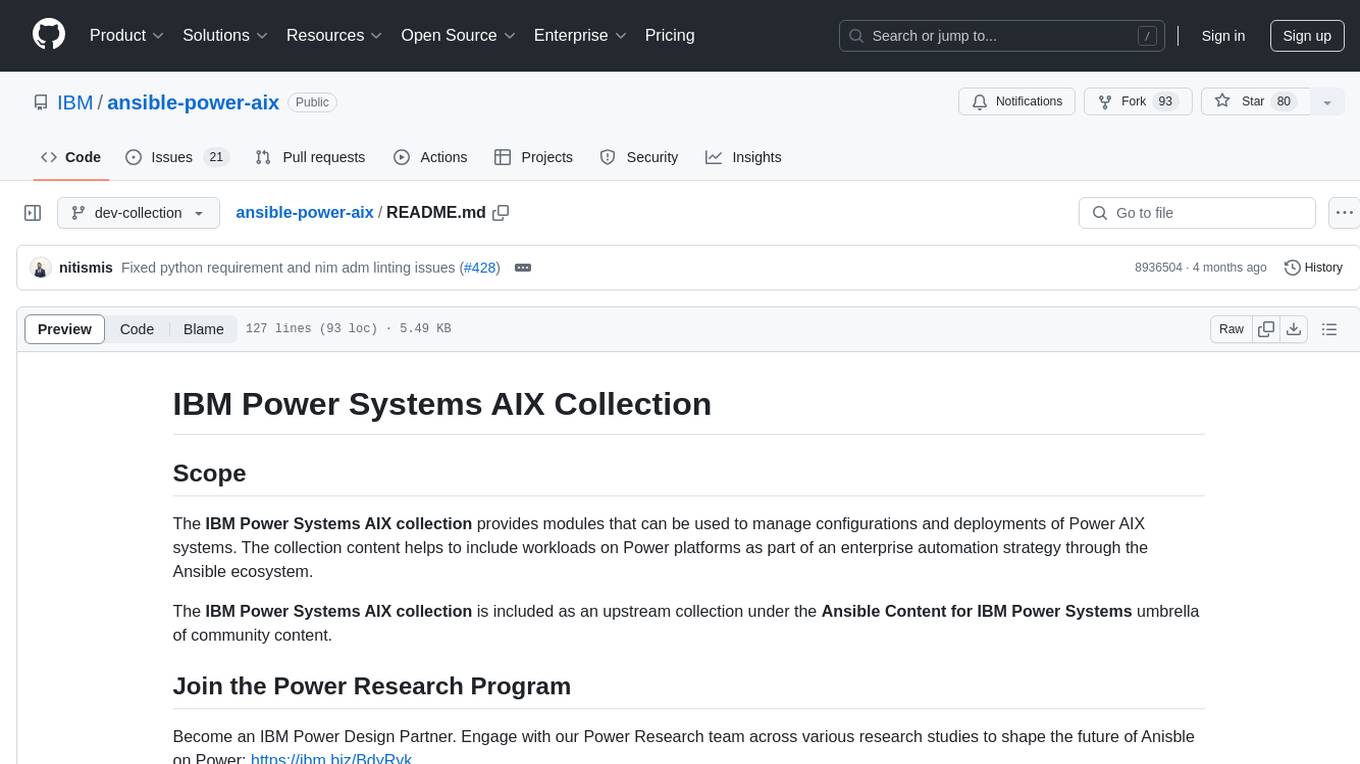
ansible-power-aix
The IBM Power Systems AIX Collection provides modules to manage configurations and deployments of Power AIX systems, enabling workloads on Power platforms as part of an enterprise automation strategy through the Ansible ecosystem. It includes example best practices, requirements for AIX versions, Ansible, and Python, along with resources for documentation and contribution.
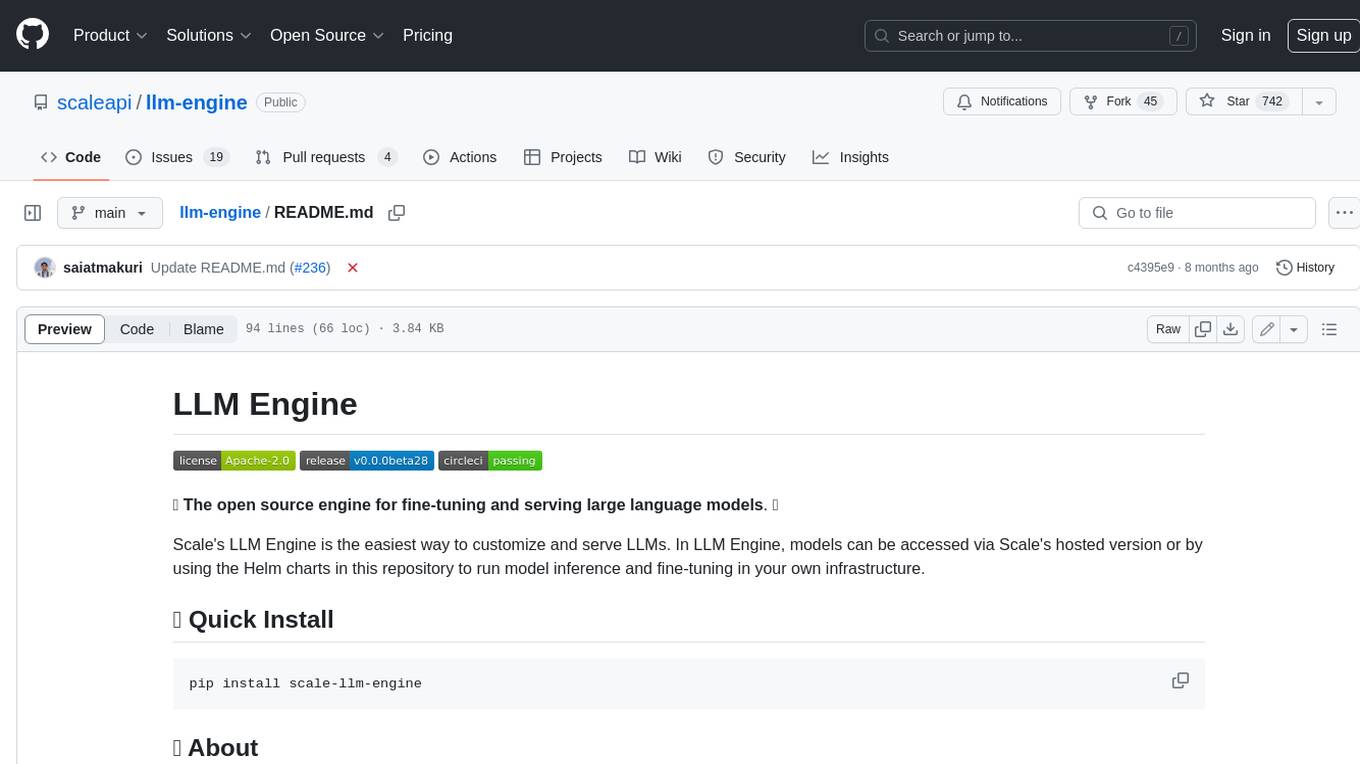
llm-engine
Scale's LLM Engine is an open-source Python library, CLI, and Helm chart that provides everything you need to serve and fine-tune foundation models, whether you use Scale's hosted infrastructure or do it in your own cloud infrastructure using Kubernetes.
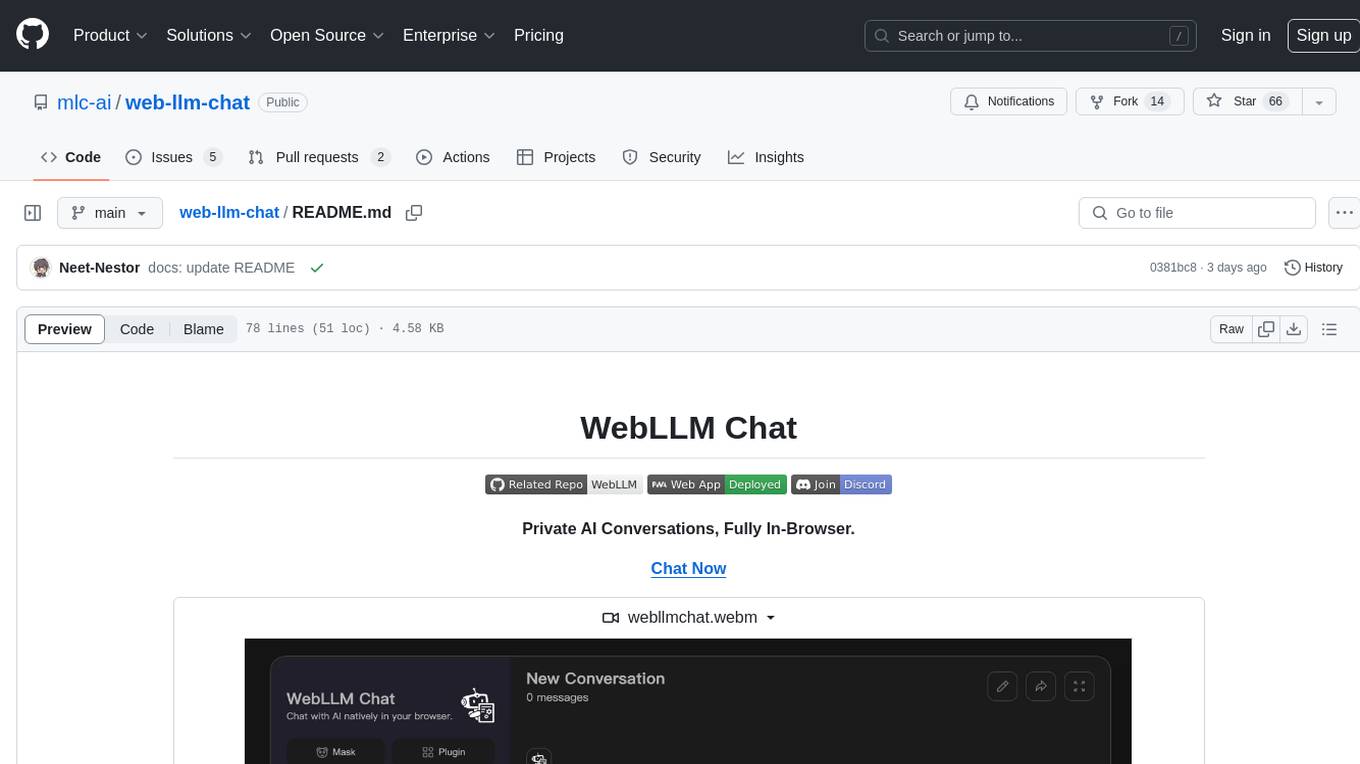
web-llm-chat
WebLLM Chat is a private AI chat interface that combines WebLLM with a user-friendly design, leveraging WebGPU to run large language models natively in your browser. It offers browser-native AI experience with WebGPU acceleration, guaranteed privacy as all data processing happens locally, offline accessibility, user-friendly interface with markdown support, and open-source customization. The project aims to democratize AI technology by making powerful tools accessible directly to end-users, enhancing the chatting experience and broadening the scope for deployment of self-hosted and customizable language models.
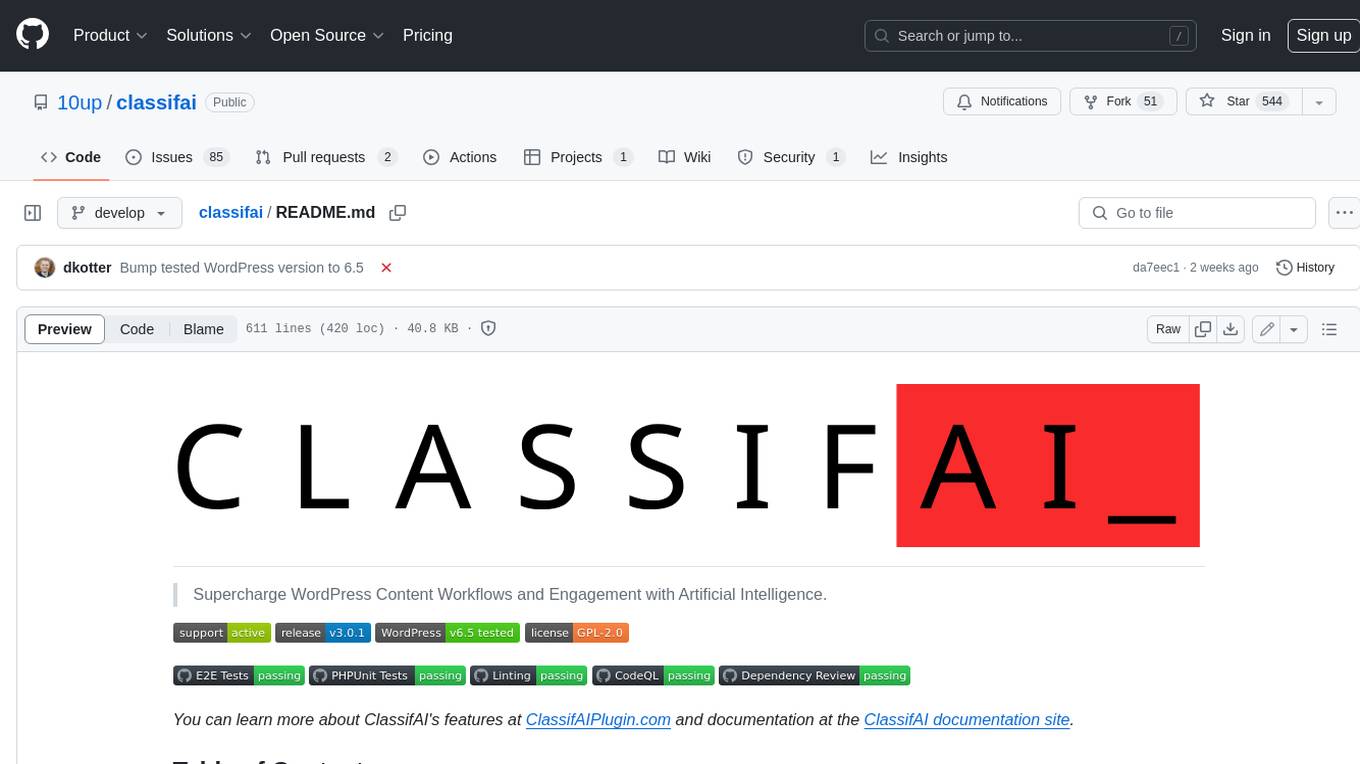
classifai
Supercharge WordPress Content Workflows and Engagement with Artificial Intelligence. Tap into leading cloud-based services like OpenAI, Microsoft Azure AI, Google Gemini and IBM Watson to augment your WordPress-powered websites. Publish content faster while improving SEO performance and increasing audience engagement. ClassifAI integrates Artificial Intelligence and Machine Learning technologies to lighten your workload and eliminate tedious tasks, giving you more time to create original content that matters.
For similar tasks
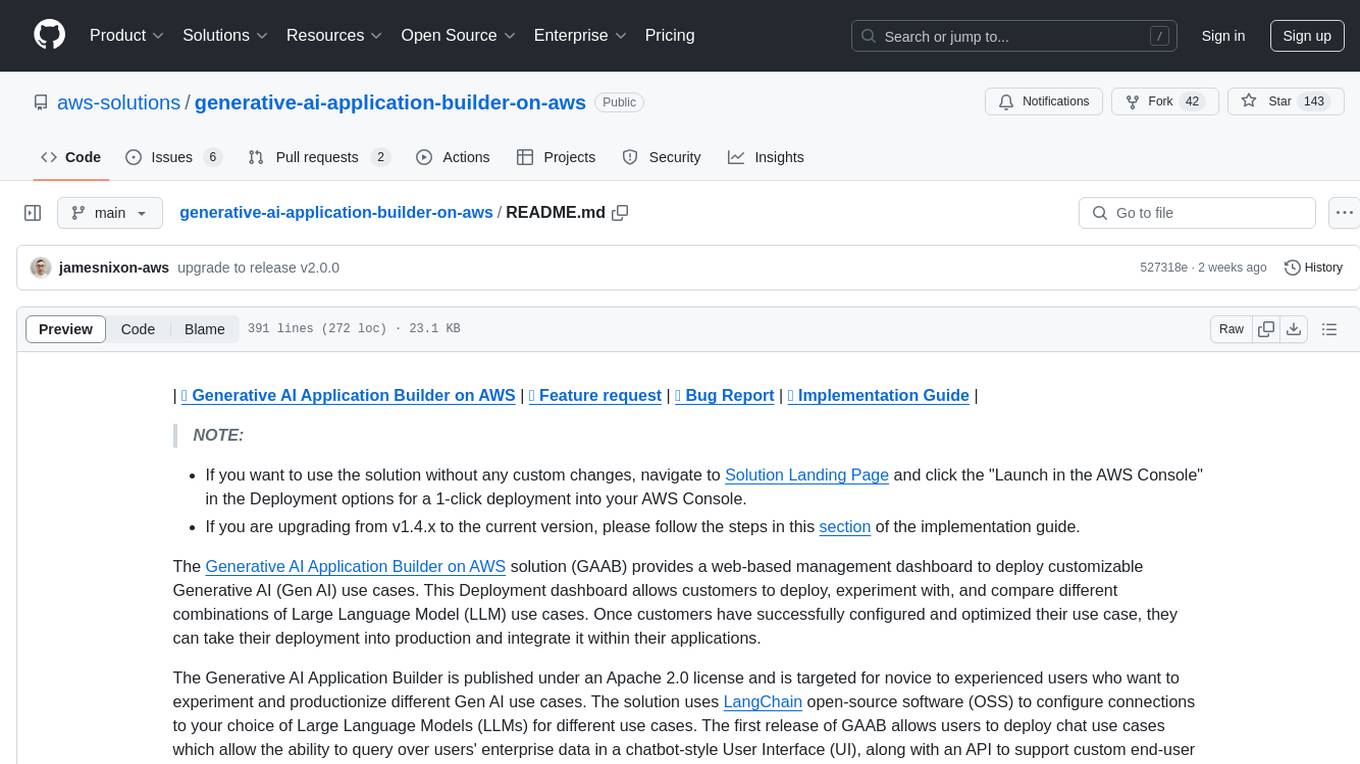
generative-ai-application-builder-on-aws
The Generative AI Application Builder on AWS (GAAB) is a solution that provides a web-based management dashboard for deploying customizable Generative AI (Gen AI) use cases. Users can experiment with and compare different combinations of Large Language Model (LLM) use cases, configure and optimize their use cases, and integrate them into their applications for production. The solution is targeted at novice to experienced users who want to experiment and productionize different Gen AI use cases. It uses LangChain open-source software to configure connections to Large Language Models (LLMs) for various use cases, with the ability to deploy chat use cases that allow querying over users' enterprise data in a chatbot-style User Interface (UI) and support custom end-user implementations through an API.
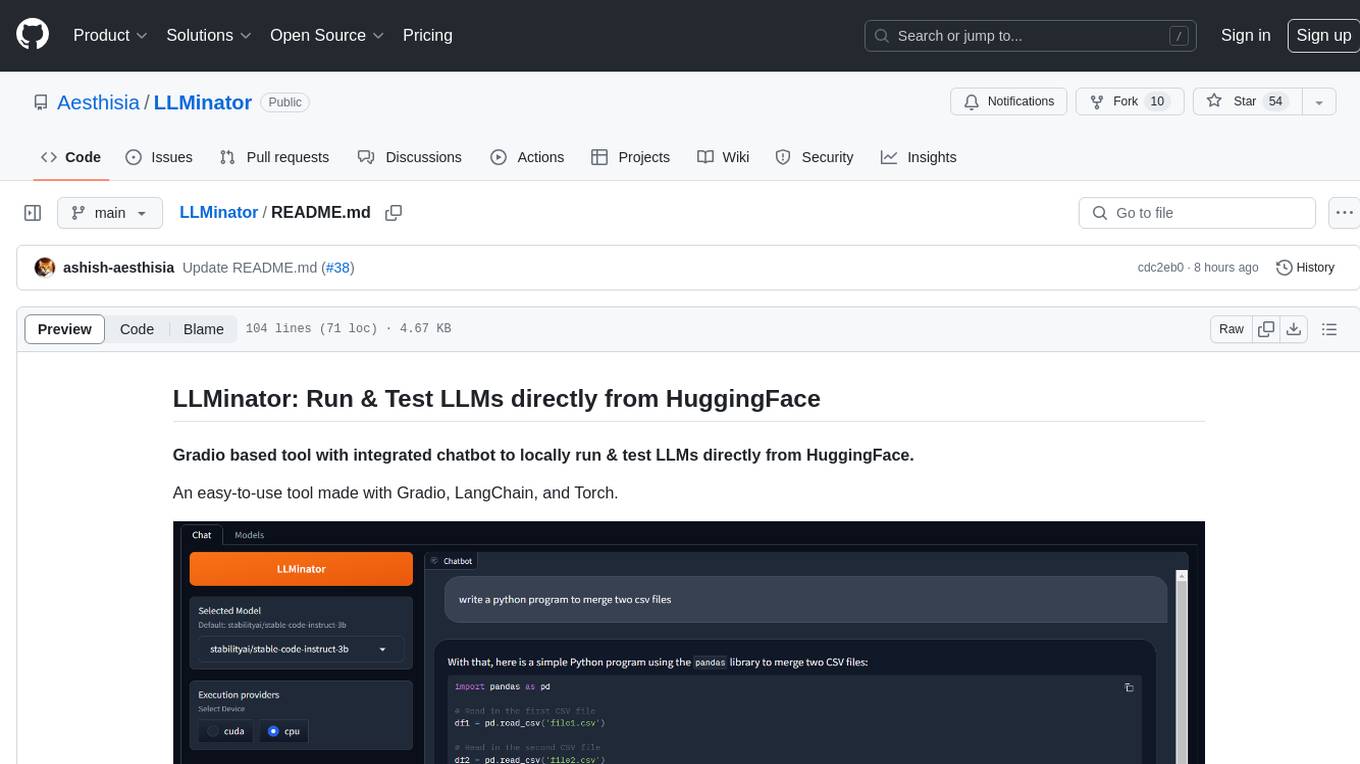
LLMinator
LLMinator is a Gradio-based tool with an integrated chatbot designed to locally run and test Language Model Models (LLMs) directly from HuggingFace. It provides an easy-to-use interface made with Gradio, LangChain, and Torch, offering features such as context-aware streaming chatbot, inbuilt code syntax highlighting, loading any LLM repo from HuggingFace, support for both CPU and CUDA modes, enabling LLM inference with llama.cpp, and model conversion capabilities.
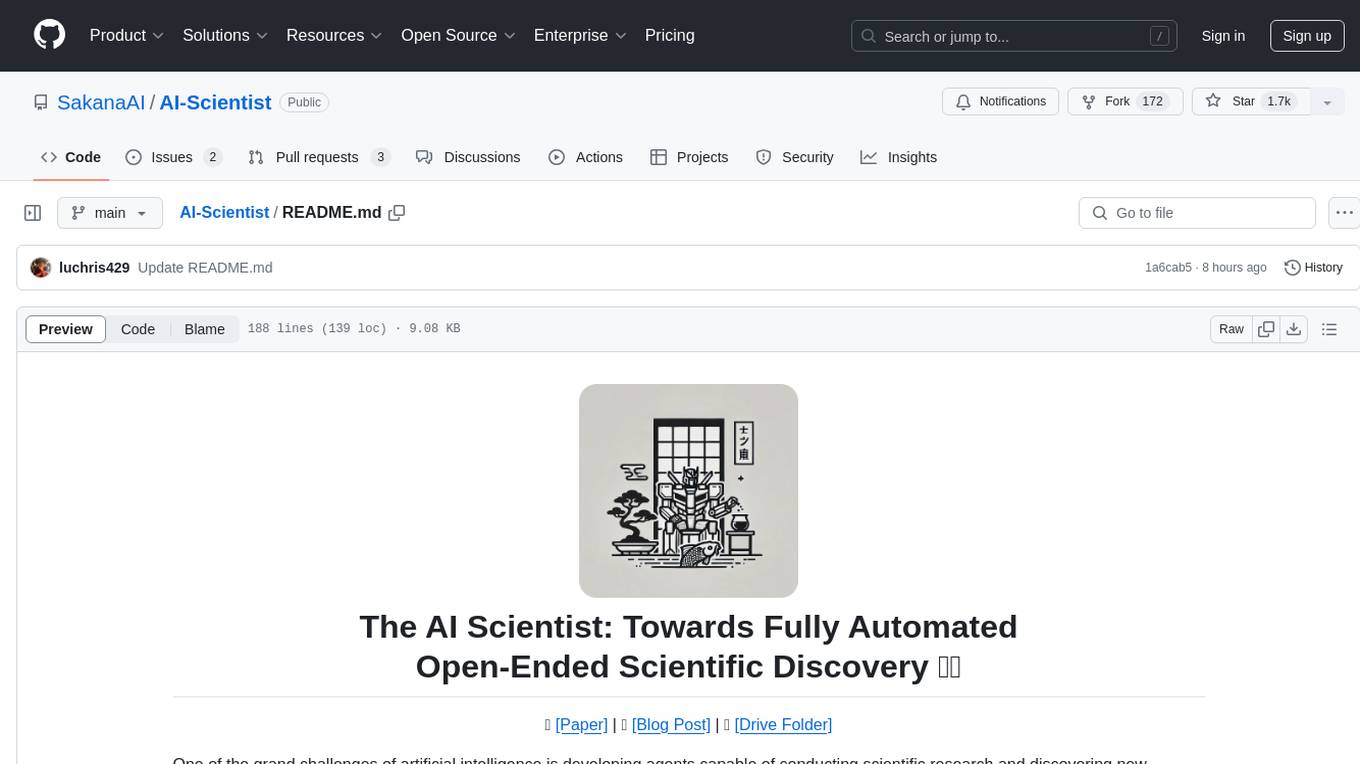
AI-Scientist
The AI Scientist is a comprehensive system for fully automatic scientific discovery, enabling Foundation Models to perform research independently. It aims to tackle the grand challenge of developing agents capable of conducting scientific research and discovering new knowledge. The tool generates papers on various topics using Large Language Models (LLMs) and provides a platform for exploring new research ideas. Users can create their own templates for specific areas of study and run experiments to generate papers. However, caution is advised as the codebase executes LLM-written code, which may pose risks such as the use of potentially dangerous packages and web access.
For similar jobs
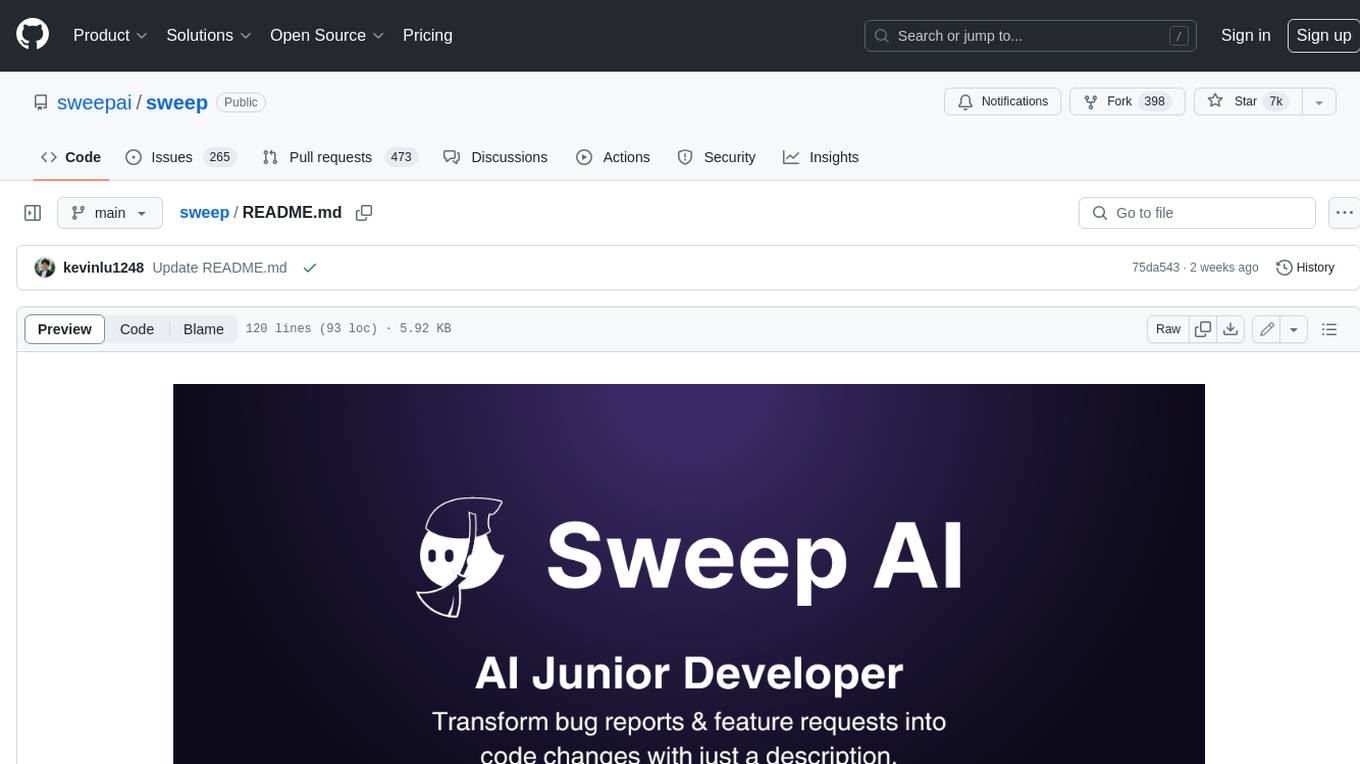
sweep
Sweep is an AI junior developer that turns bugs and feature requests into code changes. It automatically handles developer experience improvements like adding type hints and improving test coverage.
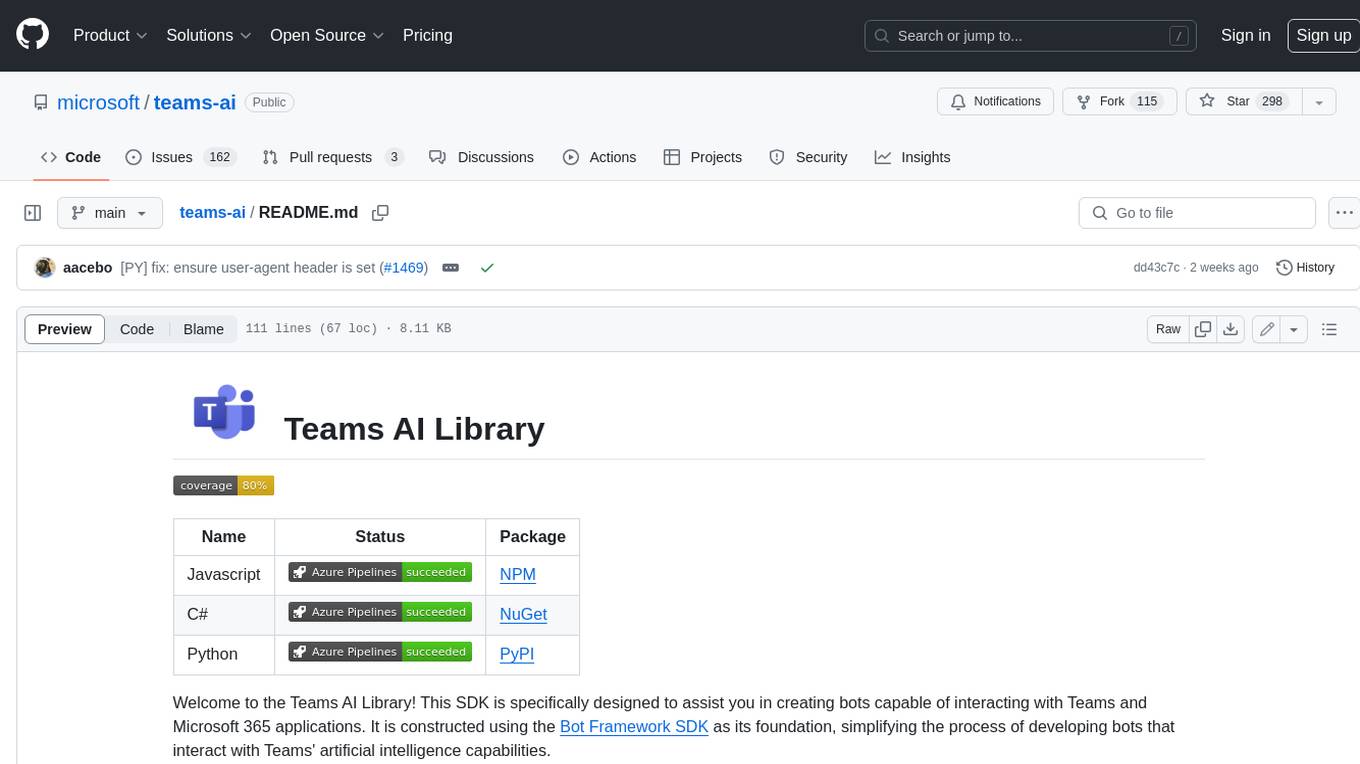
teams-ai
The Teams AI Library is a software development kit (SDK) that helps developers create bots that can interact with Teams and Microsoft 365 applications. It is built on top of the Bot Framework SDK and simplifies the process of developing bots that interact with Teams' artificial intelligence capabilities. The SDK is available for JavaScript/TypeScript, .NET, and Python.
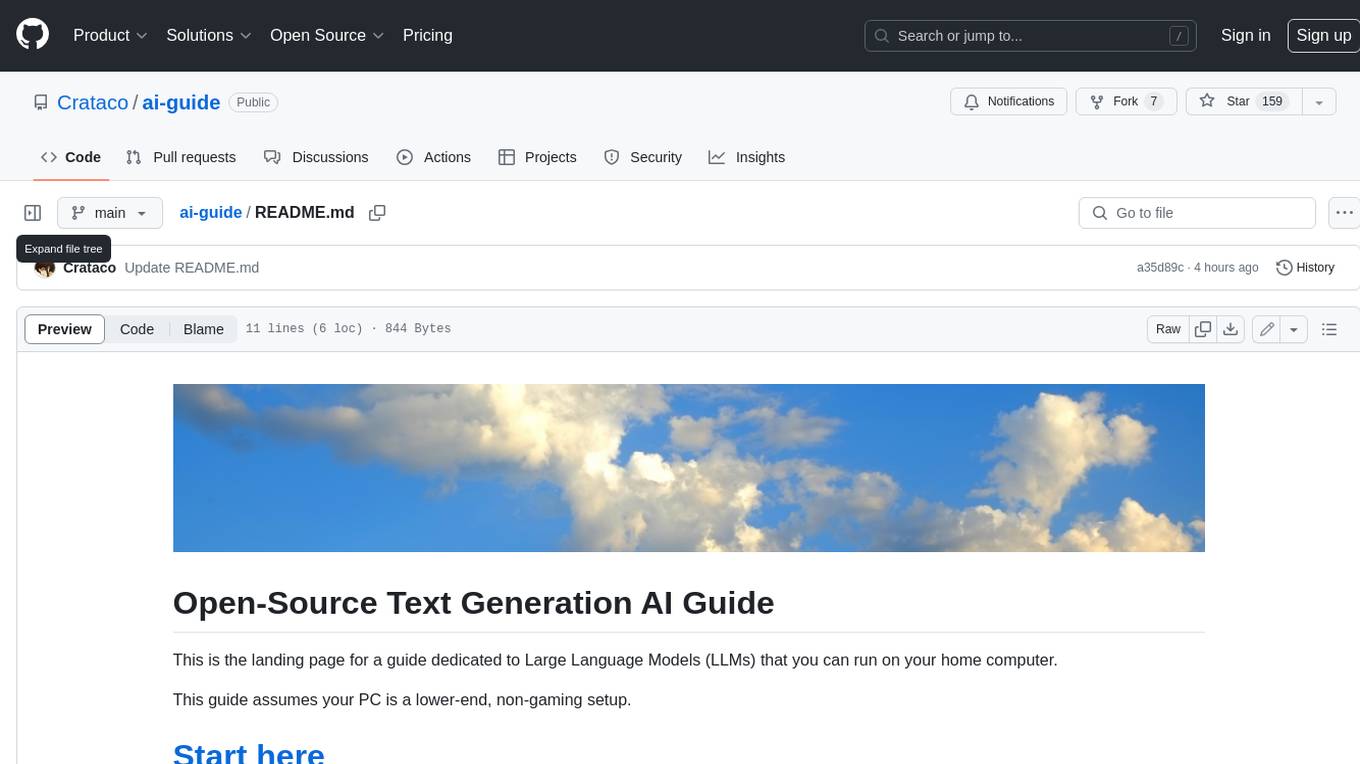
ai-guide
This guide is dedicated to Large Language Models (LLMs) that you can run on your home computer. It assumes your PC is a lower-end, non-gaming setup.
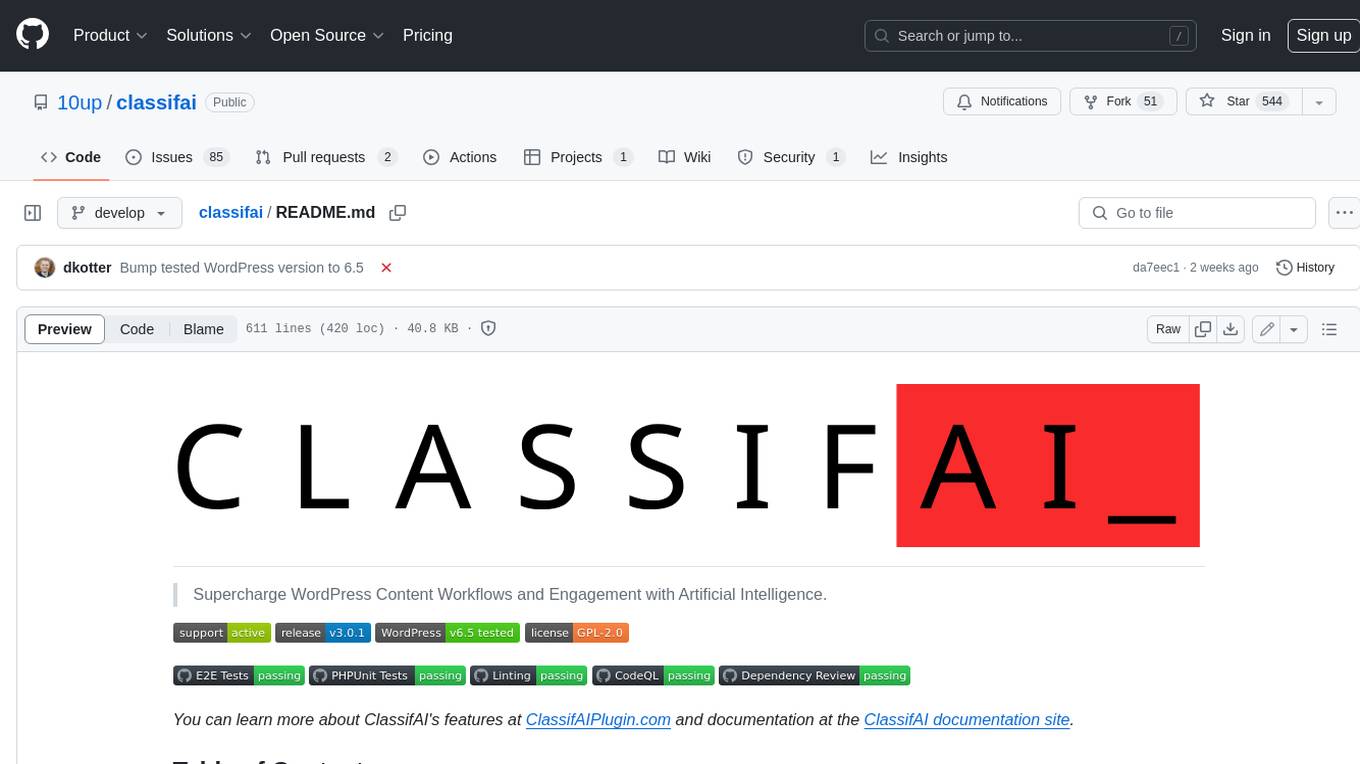
classifai
Supercharge WordPress Content Workflows and Engagement with Artificial Intelligence. Tap into leading cloud-based services like OpenAI, Microsoft Azure AI, Google Gemini and IBM Watson to augment your WordPress-powered websites. Publish content faster while improving SEO performance and increasing audience engagement. ClassifAI integrates Artificial Intelligence and Machine Learning technologies to lighten your workload and eliminate tedious tasks, giving you more time to create original content that matters.
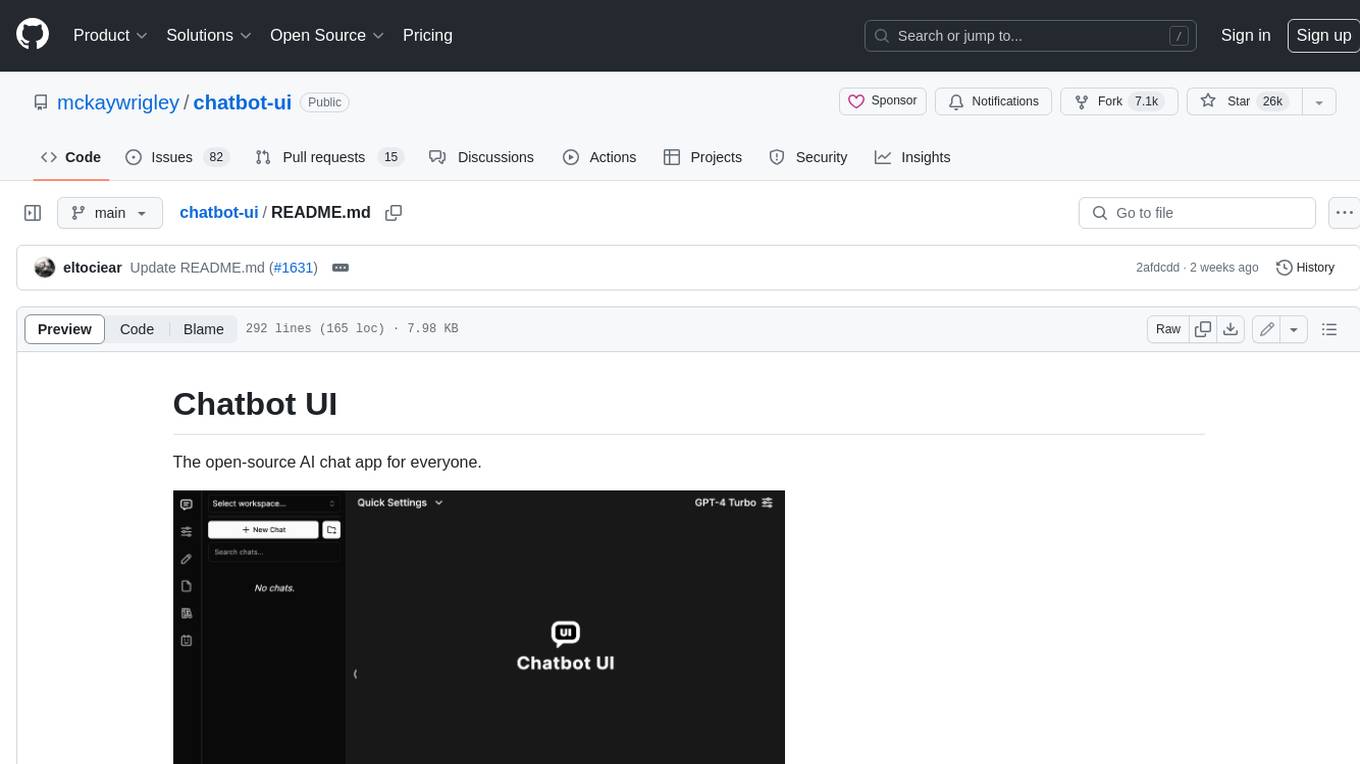
chatbot-ui
Chatbot UI is an open-source AI chat app that allows users to create and deploy their own AI chatbots. It is easy to use and can be customized to fit any need. Chatbot UI is perfect for businesses, developers, and anyone who wants to create a chatbot.
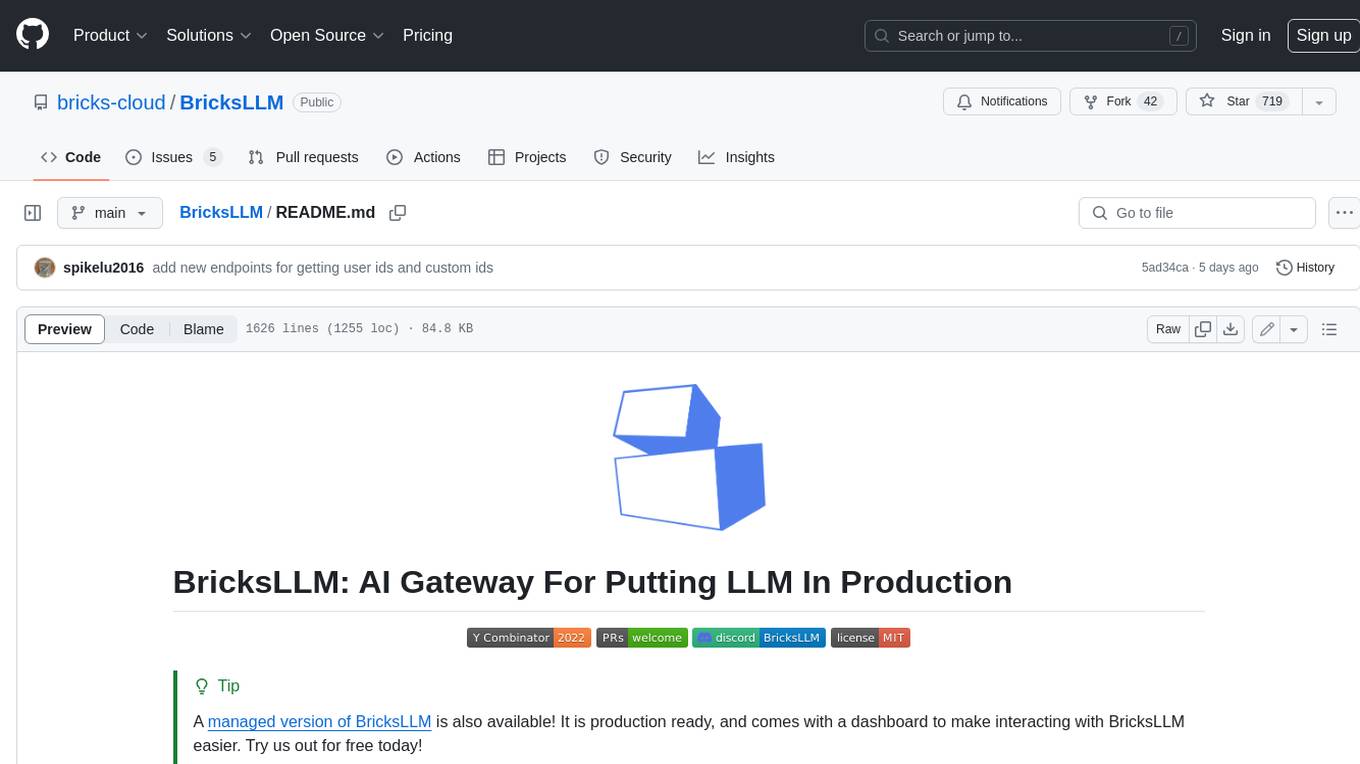
BricksLLM
BricksLLM is a cloud native AI gateway written in Go. Currently, it provides native support for OpenAI, Anthropic, Azure OpenAI and vLLM. BricksLLM aims to provide enterprise level infrastructure that can power any LLM production use cases. Here are some use cases for BricksLLM: * Set LLM usage limits for users on different pricing tiers * Track LLM usage on a per user and per organization basis * Block or redact requests containing PIIs * Improve LLM reliability with failovers, retries and caching * Distribute API keys with rate limits and cost limits for internal development/production use cases * Distribute API keys with rate limits and cost limits for students
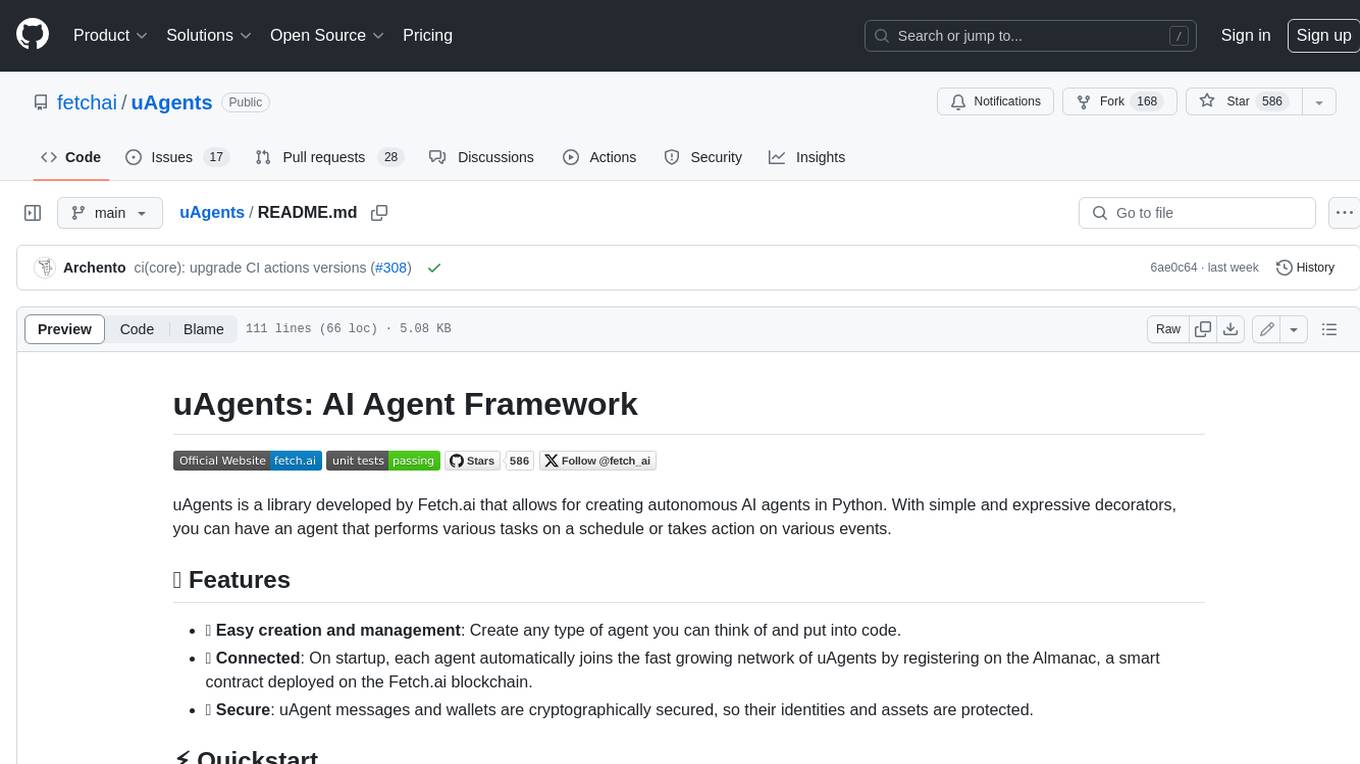
uAgents
uAgents is a Python library developed by Fetch.ai that allows for the creation of autonomous AI agents. These agents can perform various tasks on a schedule or take action on various events. uAgents are easy to create and manage, and they are connected to a fast-growing network of other uAgents. They are also secure, with cryptographically secured messages and wallets.
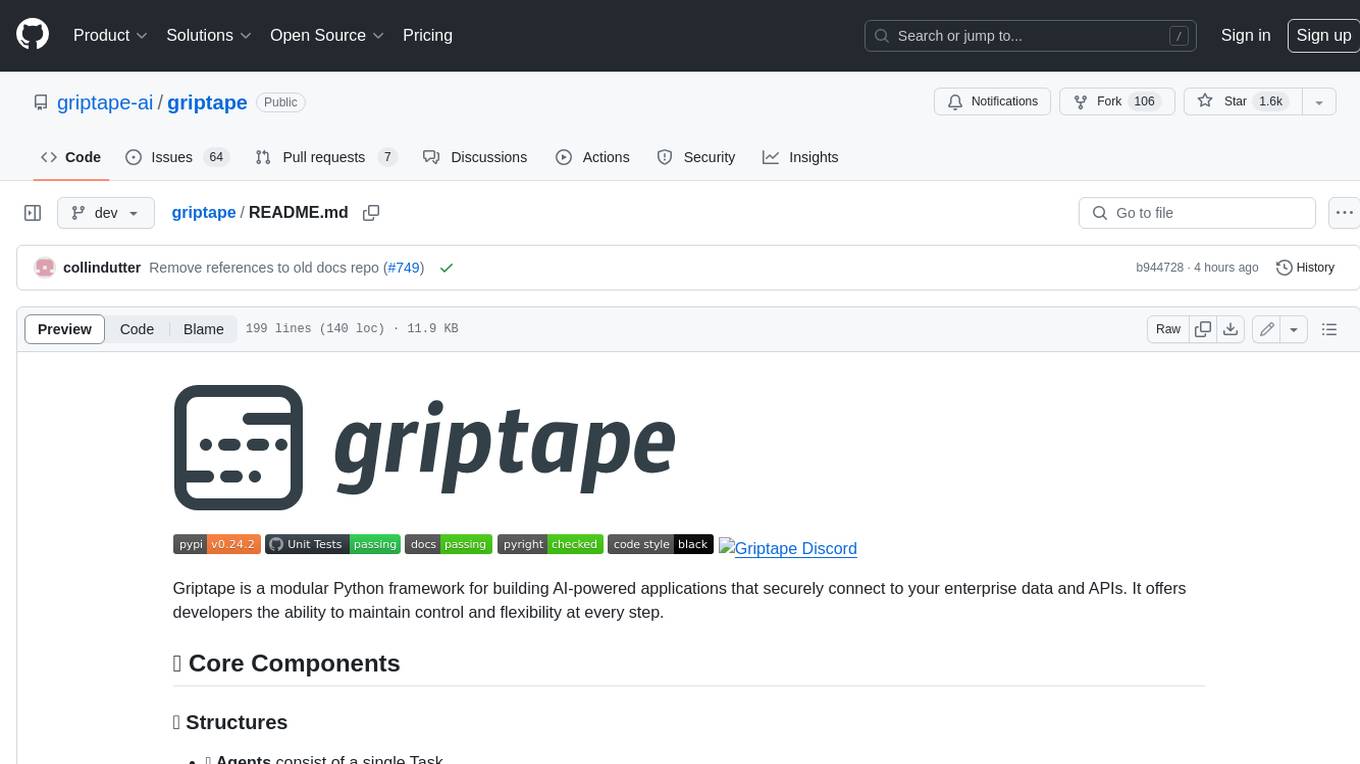
griptape
Griptape is a modular Python framework for building AI-powered applications that securely connect to your enterprise data and APIs. It offers developers the ability to maintain control and flexibility at every step. Griptape's core components include Structures (Agents, Pipelines, and Workflows), Tasks, Tools, Memory (Conversation Memory, Task Memory, and Meta Memory), Drivers (Prompt and Embedding Drivers, Vector Store Drivers, Image Generation Drivers, Image Query Drivers, SQL Drivers, Web Scraper Drivers, and Conversation Memory Drivers), Engines (Query Engines, Extraction Engines, Summary Engines, Image Generation Engines, and Image Query Engines), and additional components (Rulesets, Loaders, Artifacts, Chunkers, and Tokenizers). Griptape enables developers to create AI-powered applications with ease and efficiency.