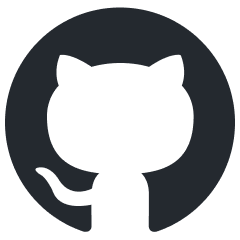
amazon-transcribe-live-call-analytics
Amazon Transcribe Live Call Analytics (LCA) Sample Solution
Stars: 85
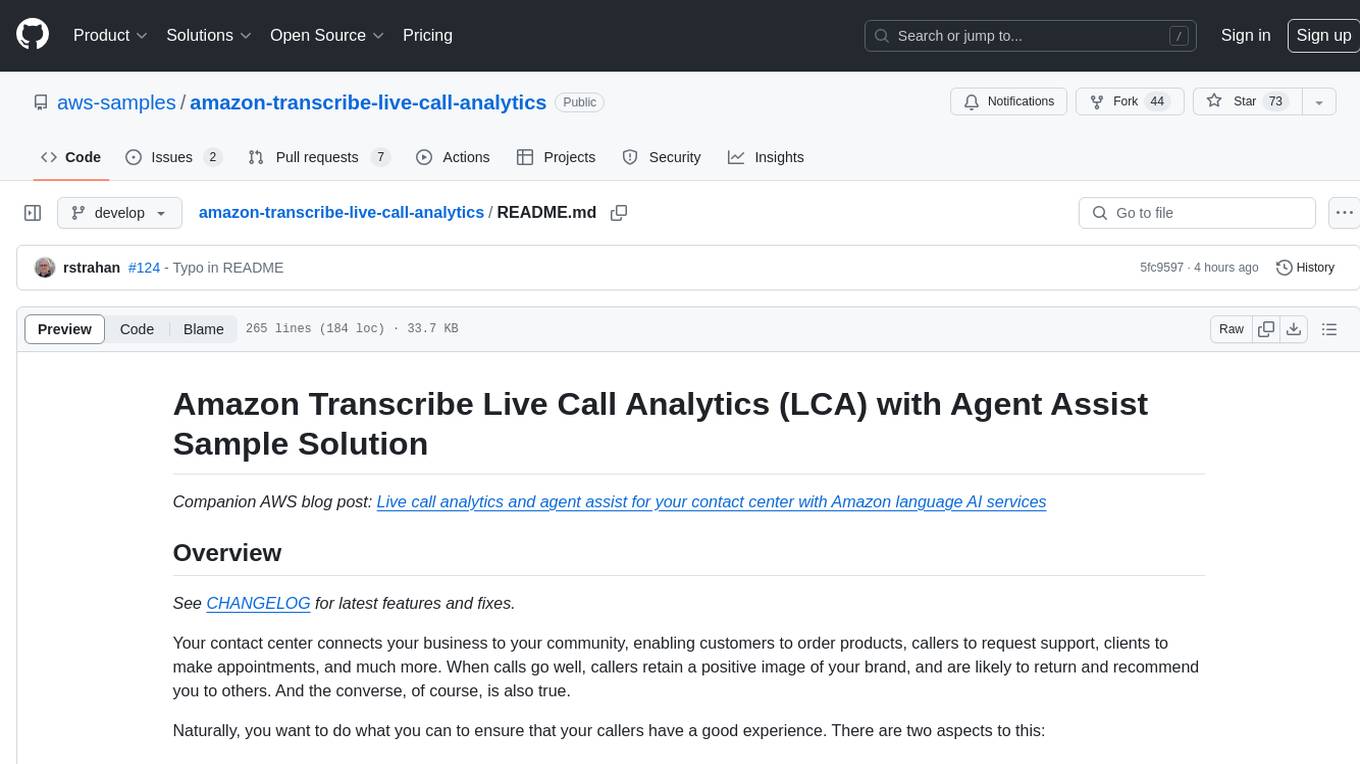
The Amazon Transcribe Live Call Analytics (LCA) with Agent Assist Sample Solution is designed to help contact centers assess and optimize caller experiences in real time. It leverages Amazon machine learning services like Amazon Transcribe, Amazon Comprehend, and Amazon SageMaker to transcribe and extract insights from contact center audio. The solution provides real-time supervisor and agent assist features, integrates with existing contact centers, and offers a scalable, cost-effective approach to improve customer interactions. The end-to-end architecture includes features like live call transcription, call summarization, AI-powered agent assistance, and real-time analytics. The solution is event-driven, ensuring low latency and seamless processing flow from ingested speech to live webpage updates.
README:
Companion AWS blog post: Live call analytics and agent assist for your contact center with Amazon language AI services
See CHANGELOG for latest features and fixes.
Your contact center connects your business to your community, enabling customers to order products, callers to request support, clients to make appointments, and much more. When calls go well, callers retain a positive image of your brand, and are likely to return and recommend you to others. And the converse, of course, is also true.
Naturally, you want to do what you can to ensure that your callers have a good experience. There are two aspects to this:
- Help supervisors assess the quality of your caller’s experiences in real time – For example, your supervisors need to know if initially unhappy callers become happier as the call progresses. And if not, why? What actions can be taken, before the call ends, to assist the agent to improve the customer experience for calls that aren’t going well?
- Help agents optimize the quality of your caller’s experiences – For example, can you deploy live call transcription, call summarization, or AI powered Agent Assistance? This removes the need for your agents to take notes, and provides them with contextually relevant information and guidance during calls, freeing them to focus more attention on providing positive customer interactions.
Amazon machine learning services like Amazon Transcribe, Amazon Comprehend and Amazon Bedrock provide feature-rich APIs that you can use to transcribe and extract insights from your contact center audio at scale. Amazon Lex provides conversational AI capabilities that can capture intents and context from conversations, and knowledge bases on Amazon Bedrock offers intelligent search and generative AI features that can provide useful information to agents based on callers' needs. Although you could build your own custom call analytics solution using these services, that requires time and resources. You figure that someone must have done this before, and that with luck you’ll find a solution that you can re-use.
Contact Lens for Amazon Connect provides real-time supervisor and agent assist features that could be just what you need, but you may not yet be using Amazon Connect. You need a solution that will also work with your existing contact center.
Our sample solution, Live Call Analytics with Agent Assist (LCA), does most of the heavy lifting associated with providing an end-to-end solution that can plug into your contact center and provide the intelligent insights that you need.
Here's a 5 minute fly-over demo of some (but not all) of the features you get with LCA.
https://github.com/user-attachments/assets/1bfc7b9f-472c-460e-9c2b-81b8066ce6e5
The demo Asterisk server is configured to use Amazon Voice Connector, which provides the phone number and SIP trunking needed to route inbound and outbound calls. When you configure LCA to integrate with your contact center using the Amazon Chime SDK Voice Connector (SIPREC) option, instead of the demo Asterisk server, Voice Connector is configured to integrate instead with your existing contact center using SIP-based media recording (SIPREC) or network-based recording (NBR). In both cases, Voice Connector streams audio to Kinesis Video Streams using two streams per call, one for the caller and one for the agent.
LCA also now also supports additional input sources, using different architectures for ingestion:
- The Genesys Cloud AudioHook integration option - see Genesys AudioHook Integration README for details.
- The new Connect Contact Lens integration option - see Amazon Connect Integration README for details.
- Amazon Connect Kinesis Video Streams integration option - see Amazon Connect Kinesis Video Streams README
- Amazon Chime SDK Call Analytics and voice tone analysis - see Amazon Chime SDK Call Analytics README
When a new caller or agent Kinesis Video stream is initiated, an event is fired using EventBridge. This event triggers the Call Transcriber Lambda function. When both the caller and agent streams have been established, your custom call initialization Lambda hook function, if specified, is invoked for you - see LambdaHookFunction. Then the Call Transcriber function starts consuming real time audio fragments from both input streams and combines them to create a new stereo audio stream. The stereo audio is streamed to an Amazon Transcribe Real-time Call Analytics or standard Amazon Transcribe session (depending on stack parameter value), and the transcription results are written in real time to Kinesis Data Streams.
Each call processing session runs until the call ends. Any session that lasts longer than the maximum duration of an AWS Lambda function invocation (15 minutes) is automatically and seamlessly transitioned to a new ‘chained’ invocation of the same function, while maintaining a continuous transcription session with Amazon Transcribe. This function chaining repeats as needed until the call ends. At the end of the call the function creates a stereo recording file in Amazon S3.
Another Lambda function, the Call Event Processor, fed by Kinesis Data Streams, processes and enriches call metadata and transcription segments. The Call Event Processor integrates with the (optional) Agent Assist services. By default, LCA agent assist is powered by Amazon Lex and Amazon Bedrock using the open source QnABot on AWS solution, though other options are available as discussed in the blog post. The Call Event Processor also invokes the (optional) Transcript Summarization lambda when the call ends, to generate a summary of the call from the full transcript.
The Call Event Processor function interfaces with AWS AppSync to persist changes (mutations) in DynamoDB and to send real-time updates to logged in web clients.
The LCA web UI assets are hosted on Amazon S3 and served via Amazon CloudFront. Authentication is provided by Amazon Cognito. In demo mode, user identities are configured in an Amazon Cognito user pool. In a production setting, you would likely configure Amazon Cognito to integrate with your existing identity provider (IdP) so authorized users can log in with their corporate credentials.
When the user is authenticated, the web application establishes a secure GraphQL connection to the AWS AppSync API, and subscribes to receive real-time events such as new calls and call status changes for the calls list page, and new or updated transcription segments, agent assist messages, and computed analytics for the call details page. When translation is enabled, the web application also interacts securely with Amazon Translate to translate the call transcription into the selected language
The entire processing flow, from ingested speech to live webpage updates, is event driven, and so the end-to-end latency is small—typically just a few seconds.
If you’re a developer, and you want to build, deploy, or publish the solution from code, refer to the Developer README.
Start your LCA experience by using AWS CloudFormation to deploy the sample solution with the built-in demo mode enabled.
The demo mode downloads, builds, and installs a small virtual PBX server on an Amazon EC2 instance in your AWS account (using the free open source Asterisk project) so you can make test phone calls right away and see the solution in action. You can integrate it with your contact center later after evaluating the solution's functionality for your unique use case.
To get LCA up and running in your own AWS account, follow these steps (if you do not have an AWS account, please see How do I create and activate a new Amazon Web Services account?):
- Log into the AWS console if you are not already. Note: If you are logged in as an IAM user, ensure your account has permissions to create and manage the necessary resources and components for this application.
- Choose one of the Launch Stack buttons below for your desired AWS region to open the AWS CloudFormation console and create a new stack. AWS Full-Stack Template is supported in the following regions:
Region name | Region code | Launch |
---|---|---|
US East (N. Virginia) | us-east-1 | |
US West (Oregon) | us-west-2 |
-
On the CloudFormation
Create Stack
page, clickNext
-
Enter the following parameters:
-
Stack Name
: Name your stack, e.g. LCA
Web UI Authentication -
Admin Email Address
- Enter the email address of the admin user to be used to log into the web UI. An initial temporary password will be automatically sent via email. This email also includes the link to the web UI -
Authorized Account Email Domain
- (Optional) Enter the email domain that is allowed to signup and signin using the web UI. Leave blank to disable signups via the web UI (users must be created using Cognito). If you configure a domain, only email addresses from that domain will be allowed to signup and signin via the web UI
Telephony Ingestion Options -
Call Audio Source
- ChooseDemo Asterisk PBX Server
to automatically install a demo Asterisk server for testing Amazon Chime SDK Voice Connector streaming -
Call Audio Processor
- ChooseAmazon Chime SDK Call Analytics
to use the new Amazon Chime SDK Call Analytics service features instead of the LCA Call transcriber Lambda. See ChimeCallAnalytics. -
WebSocketAudioInput
- Enable this option (default) to ingest and analyze audio from the web and microphone. -
Chime Voice Tone Analysis
- Choose only when Amazon Chime SDK Call Analytics is used as the call processor. Enables you to analyze caller voices for a positive, negative, or neutral tone. This is different than sentiment analysis, as it analyzes the audio versus text. --NOTE-- In some jurisdictions, it may not be legal to use voice analytics without the caller's consent. Please read https://docs.aws.amazon.com/chime-sdk/latest/dg/va-opt-out.html for more information. See ChimeCallAnalytics. -
Allowed CIDR Block for Demo Softphone
- Ignored ifCall Audio Source
is not set toDemo Asterisk PBX Server
. CIDR block allowed by demo Asterisk server for soft phone registration. Example: '10.1.1.0/24' -
Allowed CIDR List for SIPREC Integration
- Ignored ifCall Audio Source
is not set toDemo Asterisk PBX Server
. Comma delimited list of CIDR blocks allowed byAmazon Chime SDK Voice Connector for SIPREC source hosts. Example: '10.1.1.0/24, 10.1.2.0/24' -
Lambda Hook Function ARN for SIPREC Call Initialization (existing)
- Used only when CallAudioSource is set to 'Chime Voice Connector (SIPREC)' or 'Demo Asterisk PBX Server'. If present, the specified Lambda function can selectively choose calls to process or to suspend, toggle agent/caller streams, assign AgentId, and/or modify values for CallId and displayed phone numbers. See LambdaHookFunction.md. -
Amazon Connect instance ARN (existing)
- Ignored ifCall Audio Source
is not set toAmazon Connect Contact Lens
. Amazon Connect instance ARN of working instance. Prerequisite: Agent queue and Real Time Contact Lens must be enabled - see Amazon Connect Integration README.Agent Assist Options
-
Enable Agent Assist
- ChooseQnABot on AWS with new Bedrock knowledge base
to automatically install all the components and demo configuration needed to experiment with the new Agent Assist capabilities of LCA. See Agent Assist README. If you want to integrate LCA with your own agent assist bots or knowledge bases using either Amazon Lex or your own custom implementations, chooseBring your own LexV2 bot
orBring your own AWS Lambda function
. Or chooseDisable
if you do not want any agent assistant capabilities. -
BedrockKnowledgeBaseId
,BedrockKnowledgeBaseS3BucketName
,BedrockKnowledgeBaseS3DocumentUploadFolderPrefix
,AgentAssistWebCrawlURLs
,AgentAssistExistingLexV2BotId
,AgentAssistExistingLexV2BotAliasId
, andAgentAssistExistingLambdaFunctionArn
- empty by default, but must be populated as described depending on the option chosen forAgentAssistOption
. -
AgentAssistLLMBedrockModelId
- Choose the Bedrock model to use if you selectedQnABot on AWS with new Bedrock knowledge base
,QnABot on AWS with existing Bedrock knowledge base
, orQnABot on AWS with Bedrock LLM only (no knowledge base)
. You must request model access for the model selected.Amazon S3 Configuration
-
Call Audio Recordings Bucket Name
- (Optional) Existing bucket where call recording files will be stored. Leave blank to automatically create new bucket -
Audio File Prefix
- The Amazon S3 prefix where the audio files will be saved (must end in "/") -
Call Analytics Output File Prefix
- The Amazon S3 prefix where the post-call analytics files will be saved, when using analytics api mode (must end in "/")Amazon Transcribe Configuration
-
Enable Partial Transcripts
- Enable partial transcripts to receive low latency evolving transcriptions for each conversation turn. -
Transcribe API mode
- Set the default API mode for Transcribe. Set to 'analytics' to use the Amazon Transcribe Real-time Call Analytics service, used to support call categories and alerts, call summarization, and PCA integration. -
Enable Content Redaction for Transcripts
- Enable content redaction from Amazon Transcribe transcription output. NOTE: Content redaction is only available when using English (en-US, en-GB, en-AU) or Spanish (es-US). This parameter is ignored when not using other languages. -
Language for Transcription
- Language code to be used for Amazon Transcribe. To transcribe meetings in a supported language other than US English, chose the desired value for Language for Transcription. You can also choose to have Transcribe identify the primary language, or even multiple languages used during the meeting by setting Language for Transcription toidentify-language
oridentify-multiple-languages
and optionally provide a list of languages - see Language identification with streaming transcriptions. -
Content Redaction Type for Transcription
- Type of content redaction from Amazon Transcribe transcription output -
Transcription PII Redaction Entity Types
- Select the PII entity types you want to identify or redact. Remove the values that you don't want to redact from the default. DO NOT ADD CUSTOM VALUES HERE. -
Transcription Custom Vocabulary Name
- The name of the vocabulary to use when processing the transcription job. Leave blank if no custom vocabulary to be used. If yes, the custom vocabulary must pre-exist in your account. -
Transcription Custom Language Model Name
- The name of the custom language model to use when processing the transcription job. Leave blank if no custom language model is to be used. If specified, the custom language model must pre-exist in your account, match the Language Code selected above, and use the 'Narrow Band' base model.Transcript Event Processing Configuration
-
Enable Sentiment Analysis
- Enable or disable display of sentiment analysis. -
Sentiment Negative Score Threshold
- Minimum negative sentiment confidence required to declare a phrase as having negative sentiment, in the range 0-1. Not applicable when using Contact Lens or Transcribe Call Analytics (as sentiment is pre-calculated). -
Sentiment Positive Score Threshold
- Minimum positive sentiment confidence required to declare a phrase as having positive sentiment, in the range 0-1. Not applicable when using Contact Lens or Transcribe Call Analytics (as sentiment is pre-calculated). -
Lambda Hook Function ARN for Custom Transcript Segment Processing (existing)
- If present, the specified Lambda function is invoked by the LCA Call Event Processor Lambda function for each transcript segment. See TranscriptLambdaHookFunction.md. -
Lambda Hook Function Mode Non-Partial only
- Specifies if Transcript Lambda Hook Function (if specified) is invoked for Non-Partial transcript segments only (true), or for both Partial and Non-Partial transcript segments (false). -
End of Call Transcript Summary
-BEDROCK
option (default) requires you to choose one of the supported model IDs from the provided list (BedrockModelId). ChooseSAGEMAKER
to automatically deploy a summarization model. ChooseANTHROPIC
to use the Third Party Anthropic Claude model with your own API key. Alternatively, choose LAMBDA to use your own Lambda function to generate summaries using other models, or choose DISABLED if you are not interested in exploring the new Transcript Summarization feature. See Transcript Summarization for more information. -
BedrockModelId
- IfEndOfCallTranscriptSummary
isBEDROCK
, then choose a model ID from the list of supported models. Defaults toanthropic.claude-3-haiku-20240307-v1:0
-
Initial Instance Count for Summarization SageMaker Endpoint
- WhenSAGEMAKER
option is chosen (above) enter 0 for a SageMaker Serverless Inference endpoint, or 1 or greater for a provisioned endpoint with the specified number of instances. See Transcript Summarization for more details. -
End of Call Summarization LLM Third Party API Key
- Provide your API key if you choose ANTHROPIC above. See Transcript Summarization for more details. -
Lambda Hook Function ARN for Custom End of Call Processing (existing)
- When LAMBDA option is chosen (above) enter the ARN for your custom summarization Lambda function. See Transcript Summarization for more details.Download locations
-
Demo Asterisk Download URL
- (Optional) URL used to download the Asterisk PBX software -
Demo Asterisk Agent Audio URL
- (Optional) URL for audio (agent.wav) file download for demo Asterisk server. Audio file is automatically played when an agent is not connected with a softphoneAmazon CloudFront Configuration
-
CloudFront Price Class
- The CloudFront price class. See the CloudFront Pricing for a description of each price class. -
CloudFront Allowed Geographies
- (Optional) Comma separated list of two letter country codes (uppercase ISO 3166-1) that are allowed to access the web user interface via CloudFront. For example: US,CA. Leave empty if you do not want geo restrictions to be applied. For details, see: Restricting the Geographic Distribution of your Content.Record retention
-
Record Expiration In Days
- The length of time, in days, that LCA will retain call records. Records and transcripts that are older than this number of days are permanently deleted.User Experience
-
Category Alert Regular Expression
- If using the 'analytics' Transcribe API Mode, this regular expression will be used to show an alert in red in the web user interface if it matches a call category. This defaults to matching all categories.Post Call Analytics (PCA) Integration
-
PCA InputBucket
- (Optional) Value of PCA stack "InputBucket". Effective if Transcribe API Mode parameter is 'analytics'. -
PCA InputBucket Transcript prefix
- Value of PCA stack "InputBucketTranscriptPrefix". -
PCA InputBucket Playback AudioFile prefix
- Value of PCA stack "InputBucketPlaybackAudioPrefix". -
PcaWebAppURL
- (Optional) Value of PCA stack "WebAppURL" - allows PCA UI to be launched from LCA UI. -
PCA Web App Call Path Prefix
- PCA path prefix for call detail pages.
-
-
After reviewing, check the blue box for creating IAM resources.
-
Choose Create stack. This will take ~15 minutes to complete.
-
Once the CloudFormation deployment is complete,
- The admin user will receive a temporary password and the link to the CloudFront URL of the web UI (this can take a few minutes). The output of the CloudFormation stack creation will also provide a CloudFront URL (in the Outputs table of the stack details page). Click the link or copy and paste the CloudFront URL into your browser. NOTE: this page may not be available while the stack is completing the deployment
- You can sign into your application using the admin email address as the username and the temporary password you received via email. The web UI will prompt you to provide your permanent password. The user registration/login experience is run in your AWS account, and the supplied credentials are stored in Amazon Cognito. Note: given that this is a demo application, we highly suggest that you do not use an email and password combination that you use for other purposes (such as an AWS account, email, or e-commerce site)..
- Once you provide your credentials, you will be prompted to verify the email address. You can verify your account at a later time by clicking the Skip link. Otherwise, you will receive a verification code at the email address you provided (this can take a few minutes). Upon entering this verification code in the web UI, you will be signed into the application.
- Log into the AWS console if you are not already. Note: If you are logged in as an IAM user, ensure your account has permissions to create and manage the necessary resources and components for this application.
- Select your existing LiveCallAnaytics stack
- Choose Update
- Choose Replace current template
- Use one of the published template below for your region, or use the Template URL output of the publish.sh script if you have build your own artifacts from the repository:
Region name | Region code | Template URL |
---|---|---|
US East (N. Virginia) | us-east-1 | https://s3.us-east-1.amazonaws.com/aws-ml-blog-us-east-1/artifacts/lca/lca-main.yaml |
US West (Oregon) | us-west-2 | https://s3.us-west-2.amazonaws.com/aws-ml-blog-us-west-2/artifacts/lca/lca-main.yaml |
- Choose Next and review the stack parameters.
- Choose Next two more times.
- Check the blue boxes for creating IAM resources, and choose Update stack to start the update.
LCA now supports real-time Categories and Alerts using the new Amazon Transcribe Real-time Call Analytics service. Use the Amazon Transcribe Category Management console to create one or more categories with Category Type set to REAL_TIME (see Creating Categories). As categories are matched by Transcribe Real-time Call Analytics during the progress of a call, they are dynamically identified by LCA in the live transcript, call summary, and call list areas of the UI.
Categories with names that match the LCA CloudFormation stack parameter “Category Alert Regular Expression” are highlighted with a red color to show that they have been designated by LCA as Alerts. The Alert counter in the Call list page is used to identify, sort, and filter calls with alerts, allowing supervisors to quickly identify potentially problematic calls, even while they are still in progress.
LCA publishes a notification for each matched category to a topic in the Amazon Simple Notification Service. Subscribe to this topic to receive emails, SMS text messages, or to integrate category match notifications with your own applications. For more information, see Configure SNS Notifications on Call Categories.
You can test this solution if you installed the demo asterisk server during deployment. To test, perform the following steps:
- Configure a Zoiper client as described in this README. This will allow you to receive phone calls from an external phone number to the Zoiper client on your computer.
- Once installed, log in to the web app created in the deploy section by opening the Cloudfront URL provided in you CloudFormation outputs (
CloudfrontEndpoint
) - Once logged in, place a phone call using an external phone to the number provided in the CloudFormation outputs (
DemoPBXPhoneNumber
) - You will see the phone call show up on the LCA web page as follows
- Try the built-in agent assist demo using the agent assist demo script. For more detail and tutorials on Agent Assist, see Agent Assist README
For automated testing, see
- Test scripts for simulating phone calls. See Asterisk Test Scripts.
- LCA client utility to make it easier to test Call Event Processors and LCA UI without having to actually make a phone call. See LCA Client.
- SIPREC Call Initialization Lambda Hook
- Delay start of call processing
- Assign an AgentID to a call
- Customize transcript processing
- Customize transcript summarization
- Amazon Chime SDK Call Analytics & voice tone analysis
- Amazon Connect Kinesis Video Streams
Our companion solution, Post Call Analytics (PCA), offers additional insights and analytics capabilities by using the Amazon Transcribe Call Analytics batch API to detect common issues, interruptions, silences, speaker loudness, call categories, and more. Unlike LCA, which transcribes and analyzes streaming audio in real time, PCA analyzes your calls after the call has ended. The new Amazon Transcribe Real-time Call Analytics service provides post-call analytics output from your streaming sessions just a few minutes after the call has ended. LCA can now send this post-call analytics data to the latest version of PCA (v0.4.0) to provide analytics visualizations for your streaming sessions without needing to transcribe the audio a second time. Configure LCA to integrate with PCA v0.4.0 or later using the LCA CloudFormation template parameters labeled Post Call Analytics (PCA) Integration. Use the two solutions together to get the best of both worlds. For more information, see Post call analytics for your contact center with Amazon language AI services.
The Live Call Analytics (LCA) with Agent Assist sample solution offers a scalable, cost-effective approach to provide live call analysis with features to assist supervisors and agents to improve focus on your callers’ experience. It uses Amazon ML services like Amazon Transcribe, Amazon Comprehend, Amazon Lex and Amazon Bedrock to transcribe and extract real-time insights from your contact center audio. The sample LCA application is provided as open source—use it as a starting point for your own solution, and help us make it better by contributing back fixes and features via GitHub pull requests. For expert assistance, AWS Professional Services and other AWS Partners are here to help.
Congratulations! 🎉 You have completed all the steps for setting up your live call analytics sample solution using AWS services.
To make sure you are not charged for any unwanted services, you can clean up by deleting the stack created in the Deploy section and its resources.
When you’re finished experimenting with this sample solution, clean up your resources by using the AWS CloudFormation console to delete the LiveCallAnalytics stacks that you deployed. This deletes resources that were created by deploying the solution. The recording S3 buckets, the DynamoDB table and CloudWatch Log groups are retained after the stack is deleted to avoid deleting your data.
Your contributions are always welcome! Please have a look at the contribution guidelines first. 🎉
See CONTRIBUTING for more information.
This sample code is made available under the Apache-2.0 license. See the LICENSE file.
For Tasks:
Click tags to check more tools for each tasksFor Jobs:
Alternative AI tools for amazon-transcribe-live-call-analytics
Similar Open Source Tools
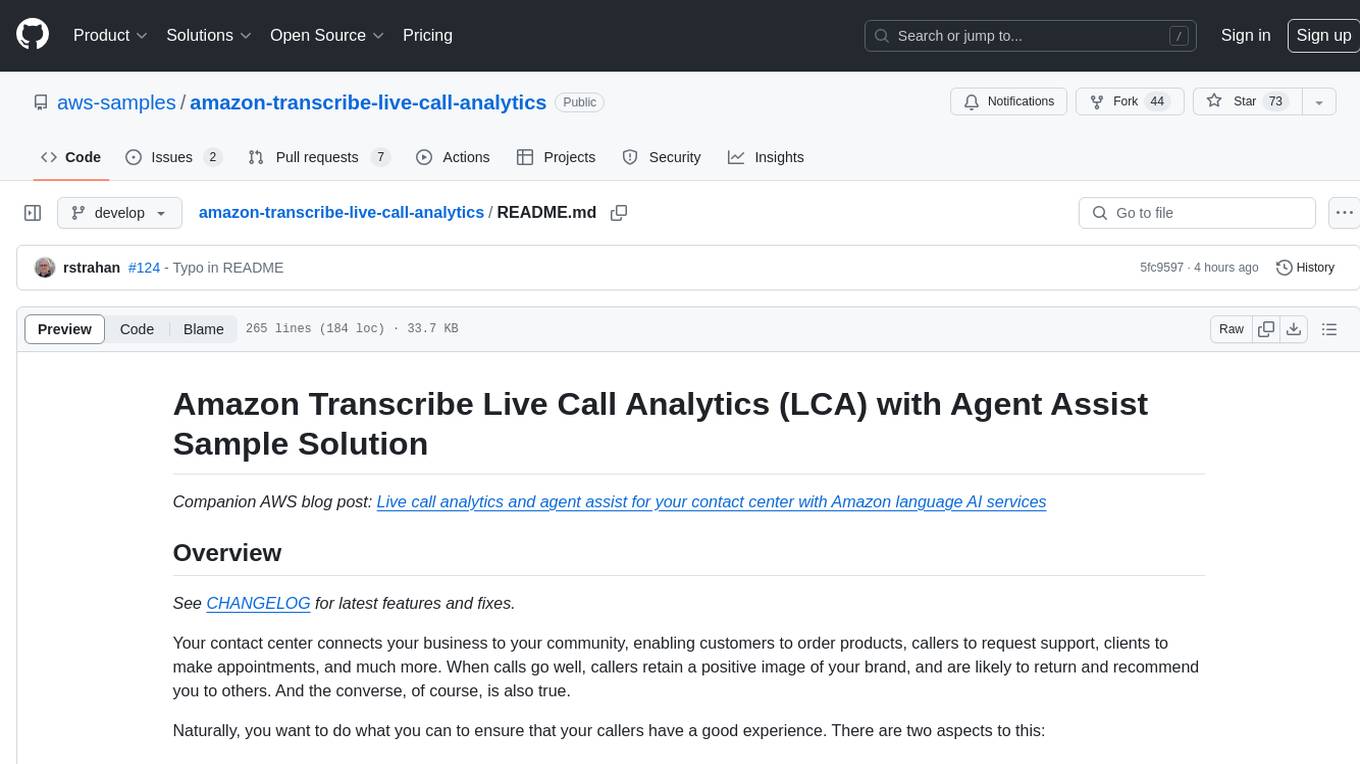
amazon-transcribe-live-call-analytics
The Amazon Transcribe Live Call Analytics (LCA) with Agent Assist Sample Solution is designed to help contact centers assess and optimize caller experiences in real time. It leverages Amazon machine learning services like Amazon Transcribe, Amazon Comprehend, and Amazon SageMaker to transcribe and extract insights from contact center audio. The solution provides real-time supervisor and agent assist features, integrates with existing contact centers, and offers a scalable, cost-effective approach to improve customer interactions. The end-to-end architecture includes features like live call transcription, call summarization, AI-powered agent assistance, and real-time analytics. The solution is event-driven, ensuring low latency and seamless processing flow from ingested speech to live webpage updates.
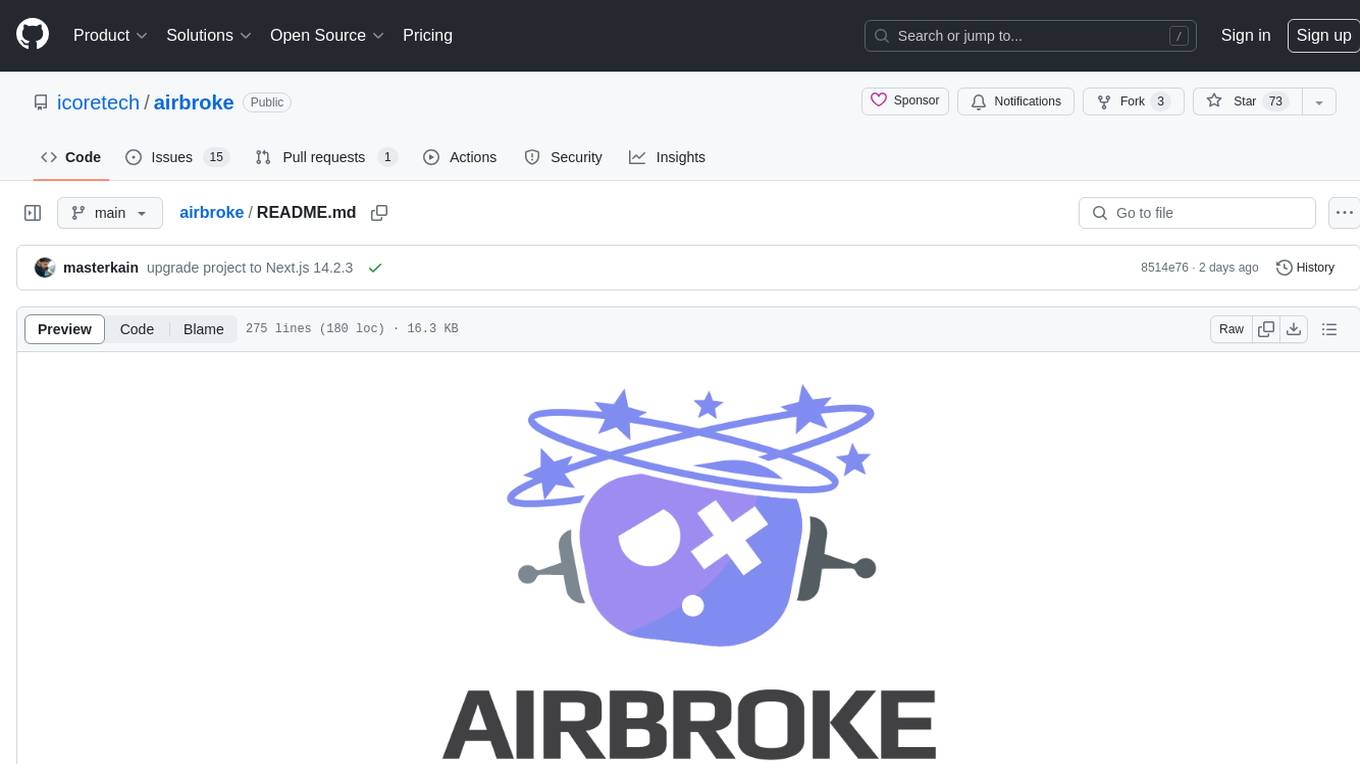
airbroke
Airbroke is an open-source error catcher tool designed for modern web applications. It provides a PostgreSQL-based backend with an Airbrake-compatible HTTP collector endpoint and a React-based frontend for error management. The tool focuses on simplicity, maintaining a small database footprint even under heavy data ingestion. Users can ask AI about issues, replay HTTP exceptions, and save/manage bookmarks for important occurrences. Airbroke supports multiple OAuth providers for secure user authentication and offers occurrence charts for better insights into error occurrences. The tool can be deployed in various ways, including building from source, using Docker images, deploying on Vercel, Render.com, Kubernetes with Helm, or Docker Compose. It requires Node.js, PostgreSQL, and specific system resources for deployment.
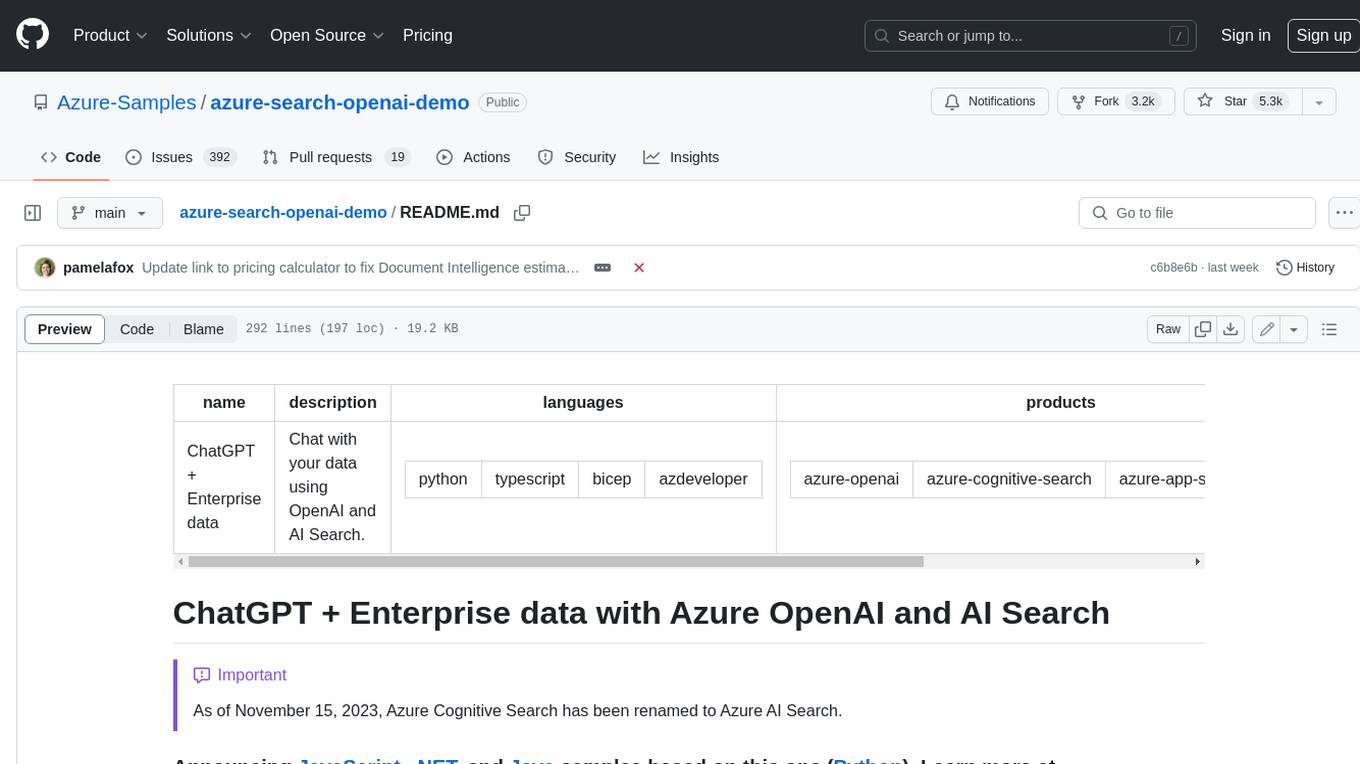
azure-search-openai-demo
This sample demonstrates a few approaches for creating ChatGPT-like experiences over your own data using the Retrieval Augmented Generation pattern. It uses Azure OpenAI Service to access a GPT model (gpt-35-turbo), and Azure AI Search for data indexing and retrieval. The repo includes sample data so it's ready to try end to end. In this sample application we use a fictitious company called Contoso Electronics, and the experience allows its employees to ask questions about the benefits, internal policies, as well as job descriptions and roles.
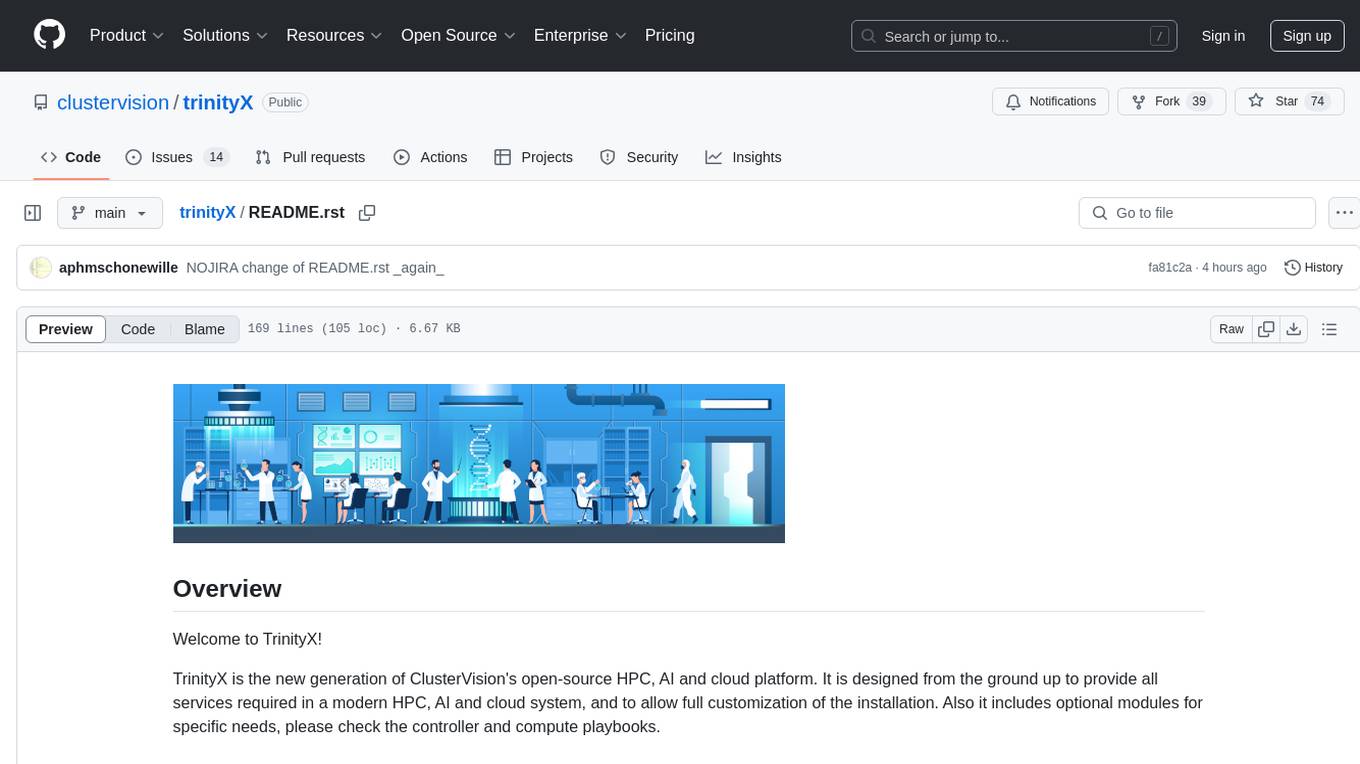
trinityX
TrinityX is an open-source HPC, AI, and cloud platform designed to provide all services required in a modern system, with full customization options. It includes default services like Luna node provisioner, OpenLDAP, SLURM or OpenPBS, Prometheus, Grafana, OpenOndemand, and more. TrinityX also sets up NFS-shared directories, OpenHPC applications, environment modules, HA, and more. Users can install TrinityX on Enterprise Linux, configure network interfaces, set up passwordless authentication, and customize the installation using Ansible playbooks. The platform supports HA, OpenHPC integration, and provides detailed documentation for users to contribute to the project.
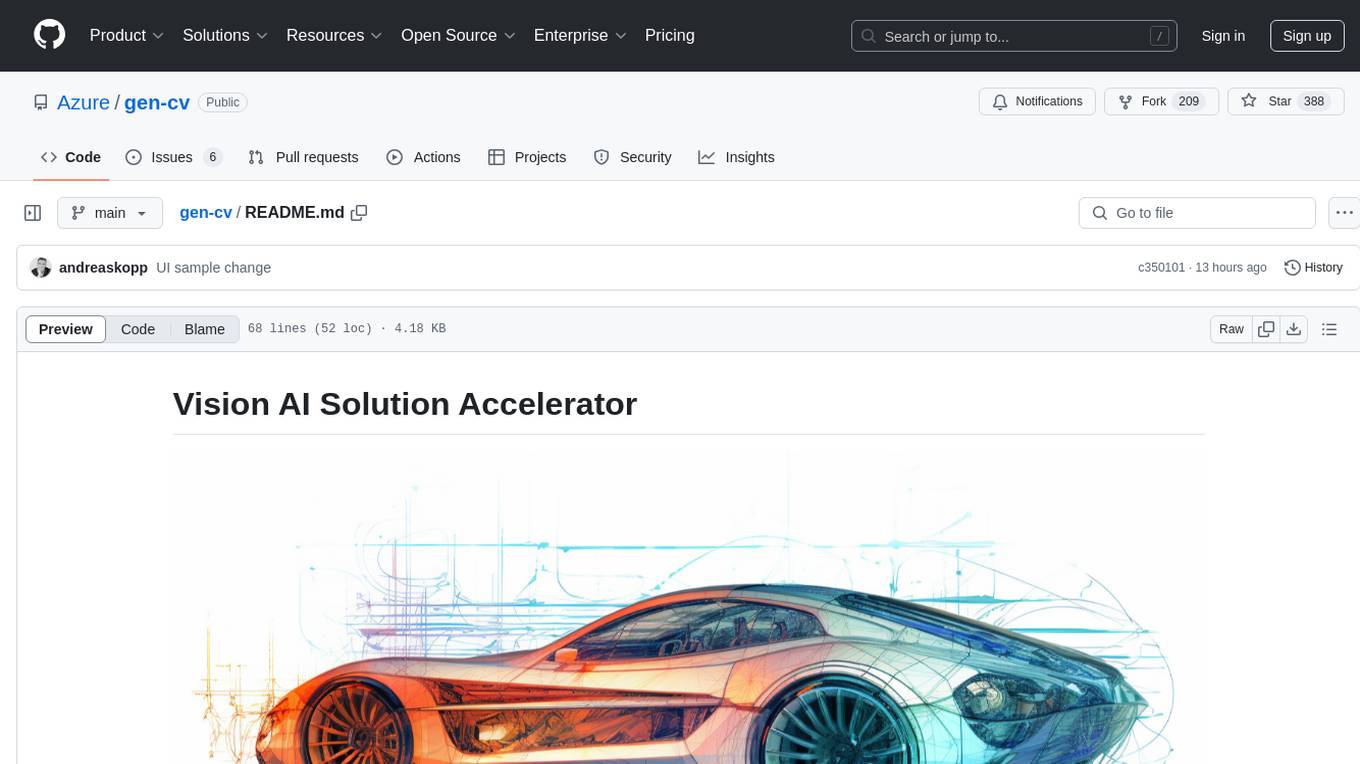
gen-cv
This repository is a rich resource offering examples of synthetic image generation, manipulation, and reasoning using Azure Machine Learning, Computer Vision, OpenAI, and open-source frameworks like Stable Diffusion. It provides practical insights into image processing applications, including content generation, video analysis, avatar creation, and image manipulation with various tools and APIs.
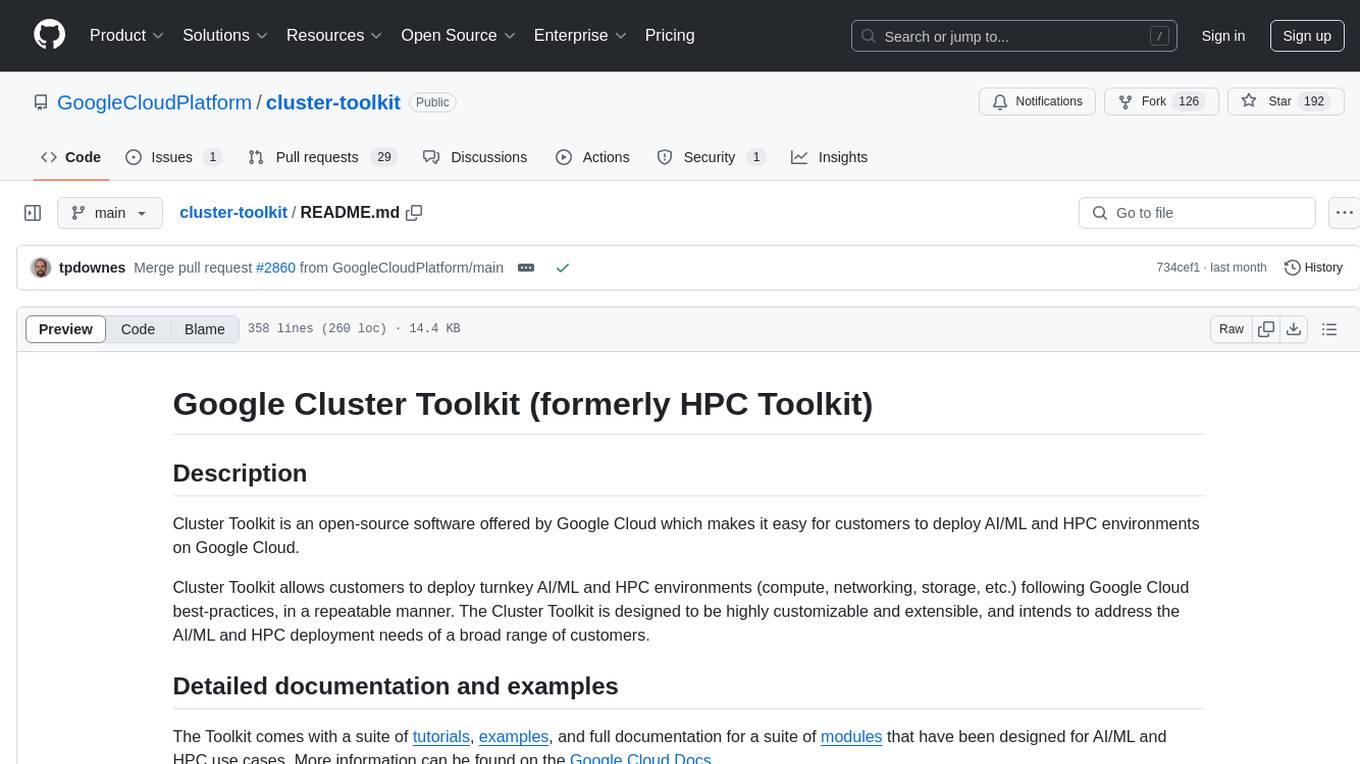
cluster-toolkit
Cluster Toolkit is an open-source software by Google Cloud for deploying AI/ML and HPC environments on Google Cloud. It allows easy deployment following best practices, with high customization and extensibility. The toolkit includes tutorials, examples, and documentation for various modules designed for AI/ML and HPC use cases.
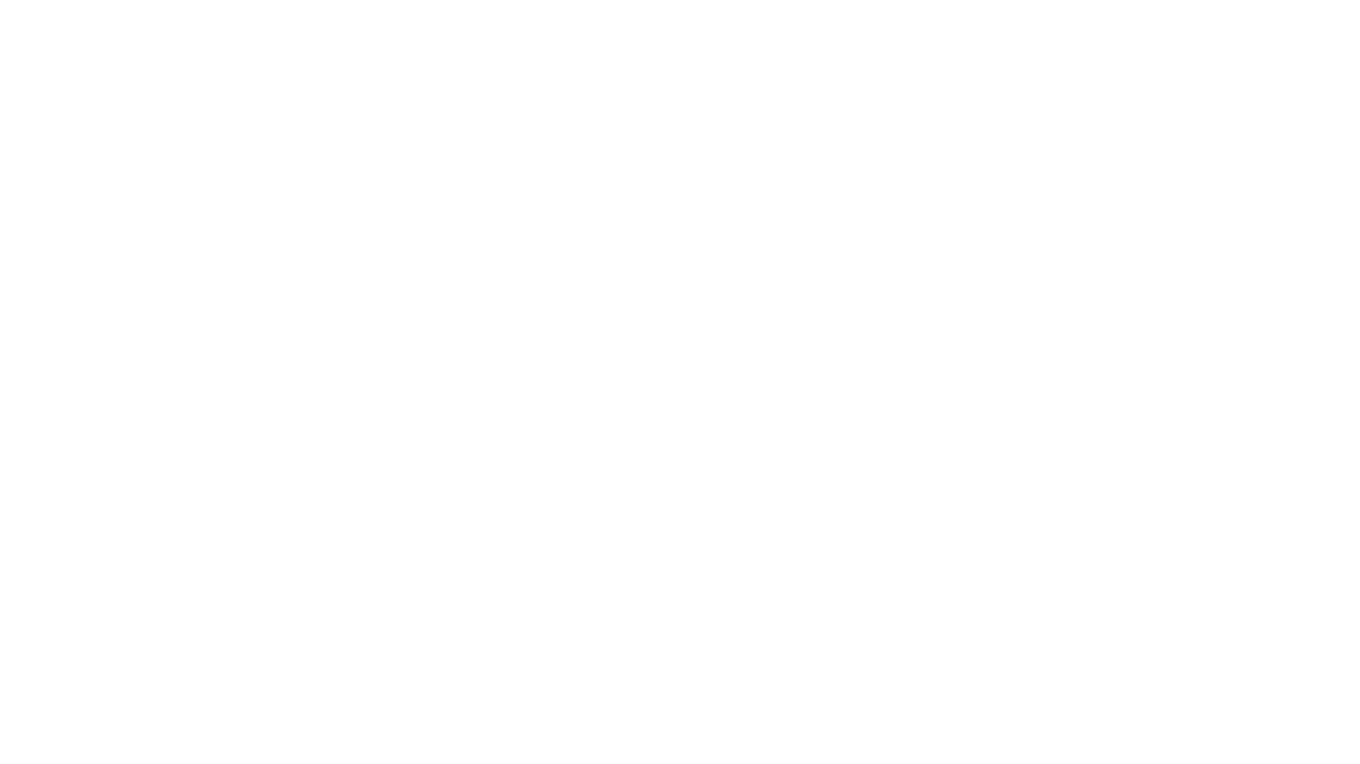
eureka-ml-insights
The Eureka ML Insights Framework is a repository containing code designed to help researchers and practitioners run reproducible evaluations of generative models efficiently. Users can define custom pipelines for data processing, inference, and evaluation, as well as utilize pre-defined evaluation pipelines for key benchmarks. The framework provides a structured approach to conducting experiments and analyzing model performance across various tasks and modalities.
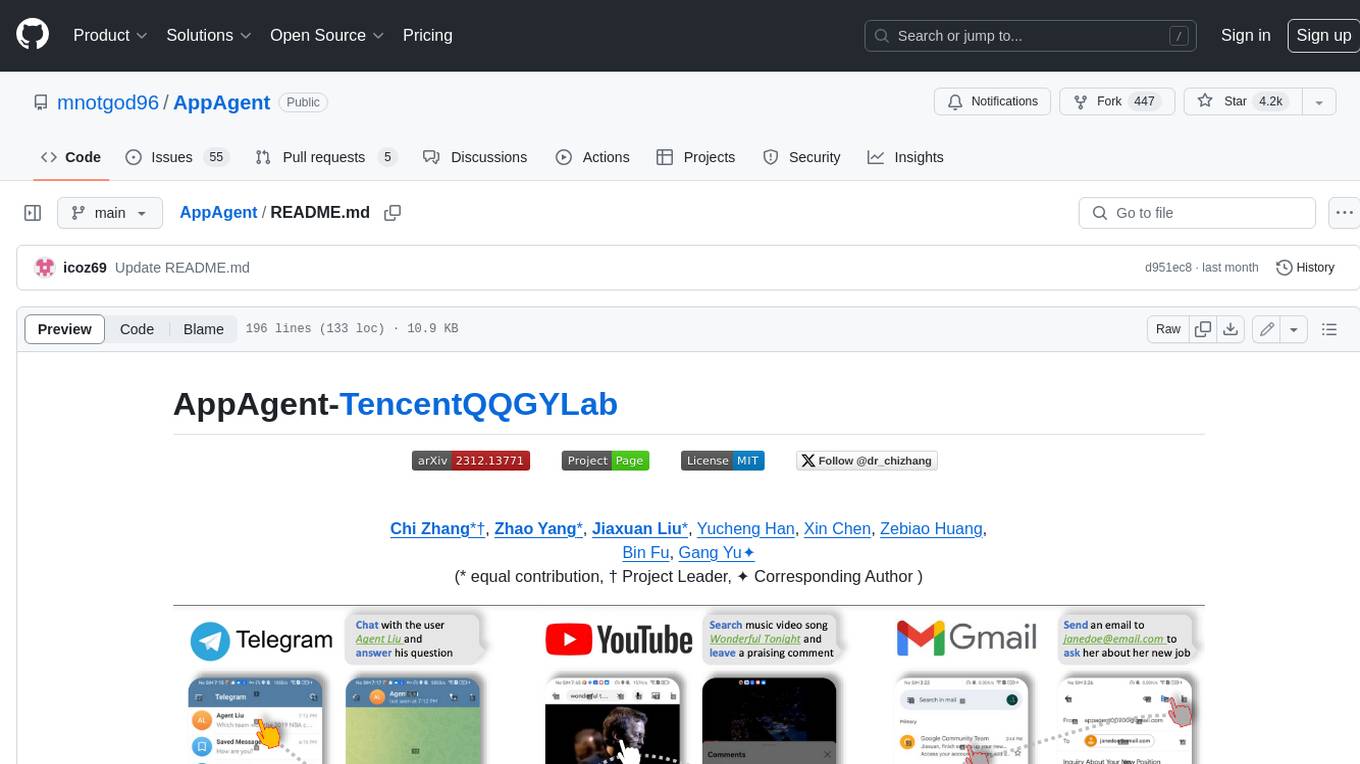
AppAgent
AppAgent is a novel LLM-based multimodal agent framework designed to operate smartphone applications. Our framework enables the agent to operate smartphone applications through a simplified action space, mimicking human-like interactions such as tapping and swiping. This novel approach bypasses the need for system back-end access, thereby broadening its applicability across diverse apps. Central to our agent's functionality is its innovative learning method. The agent learns to navigate and use new apps either through autonomous exploration or by observing human demonstrations. This process generates a knowledge base that the agent refers to for executing complex tasks across different applications.
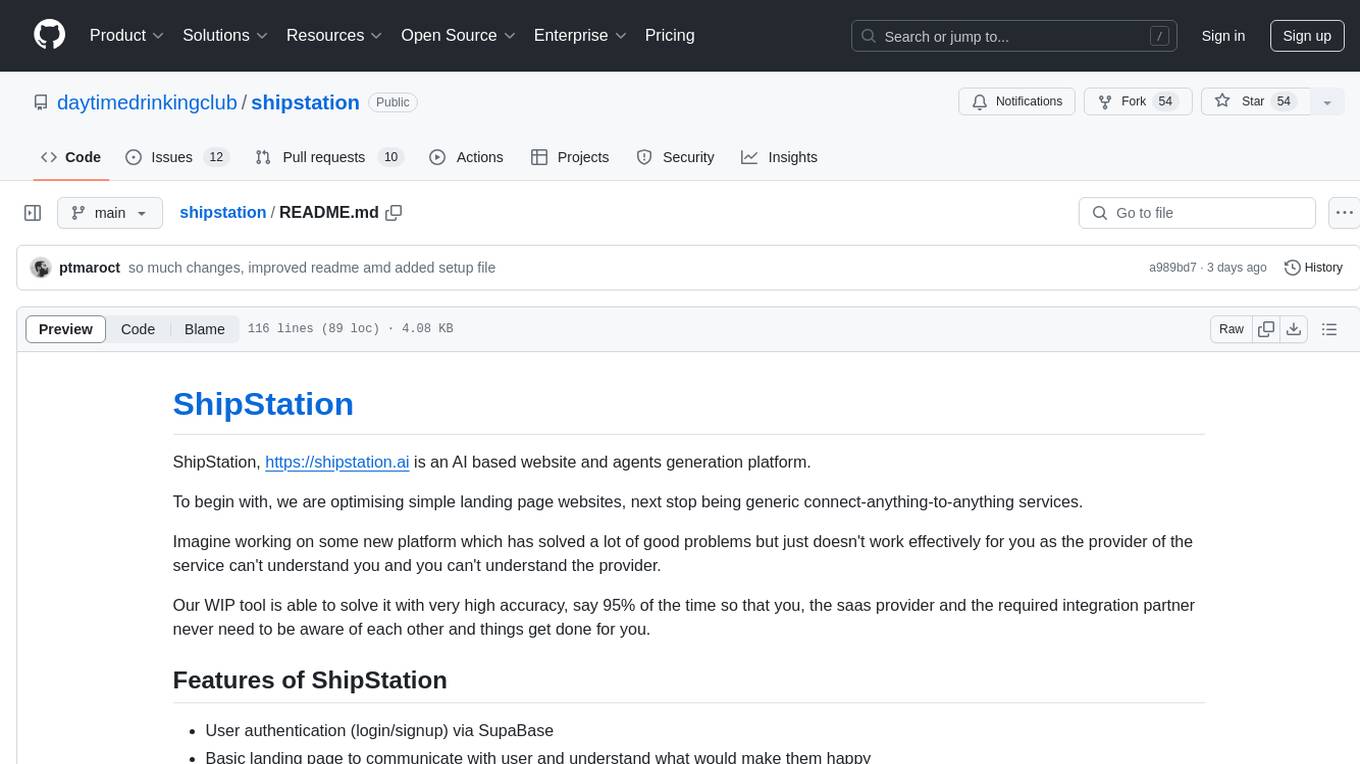
shipstation
ShipStation is an AI-based website and agents generation platform that optimizes landing page websites and generic connect-anything-to-anything services. It enables seamless communication between service providers and integration partners, offering features like user authentication, project management, code editing, payment integration, and real-time progress tracking. The project architecture includes server-side (Node.js) and client-side (React with Vite) components. Prerequisites include Node.js, npm or yarn, Anthropic API key, Supabase account, Tavily API key, and Razorpay account. Setup instructions involve cloning the repository, setting up Supabase, configuring environment variables, and starting the backend and frontend servers. Users can access the application through the browser, sign up or log in, create landing pages or portfolios, and get websites stored in an S3 bucket. Deployment to Heroku involves building the client project, committing changes, and pushing to the main branch. Contributions to the project are encouraged, and the license encourages doing good.
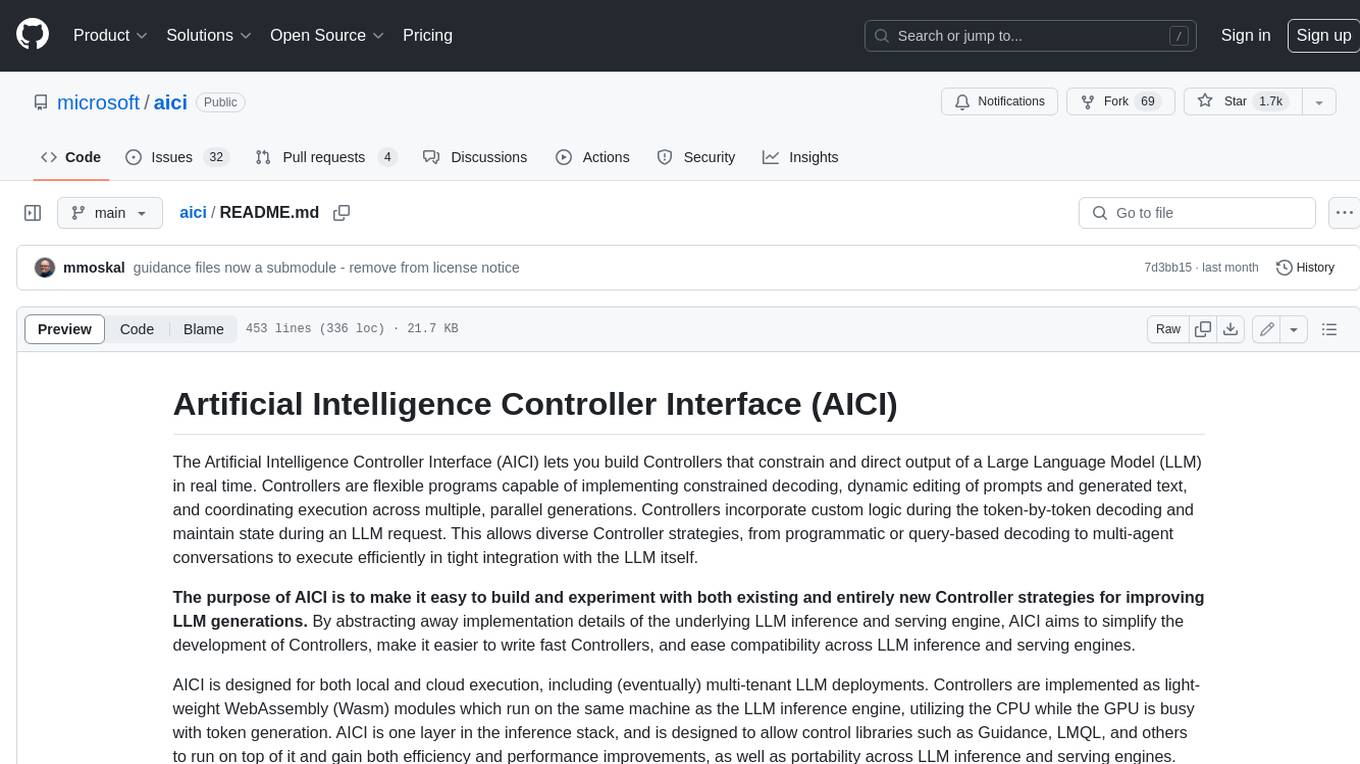
aici
The Artificial Intelligence Controller Interface (AICI) lets you build Controllers that constrain and direct output of a Large Language Model (LLM) in real time. Controllers are flexible programs capable of implementing constrained decoding, dynamic editing of prompts and generated text, and coordinating execution across multiple, parallel generations. Controllers incorporate custom logic during the token-by-token decoding and maintain state during an LLM request. This allows diverse Controller strategies, from programmatic or query-based decoding to multi-agent conversations to execute efficiently in tight integration with the LLM itself.
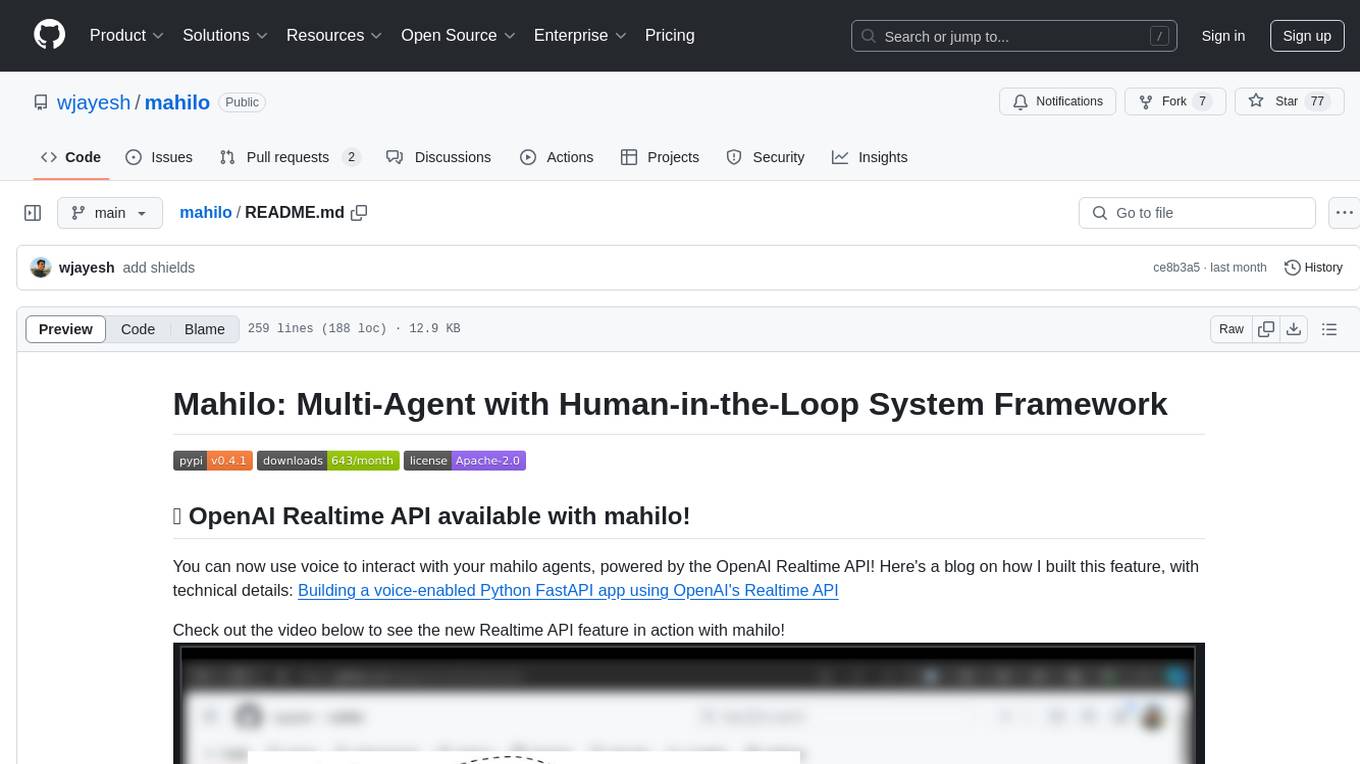
mahilo
Mahilo is a flexible framework for creating multi-agent systems that can interact with humans while sharing context internally. It allows developers to set up complex agent networks for various applications, from customer service to emergency response simulations. Agents can communicate with each other and with humans, making the system efficient by handling context from multiple agents and helping humans stay focused on specific problems. The system supports Realtime API for voice interactions, WebSocket-based communication, flexible communication patterns, session management, and easy agent definition.
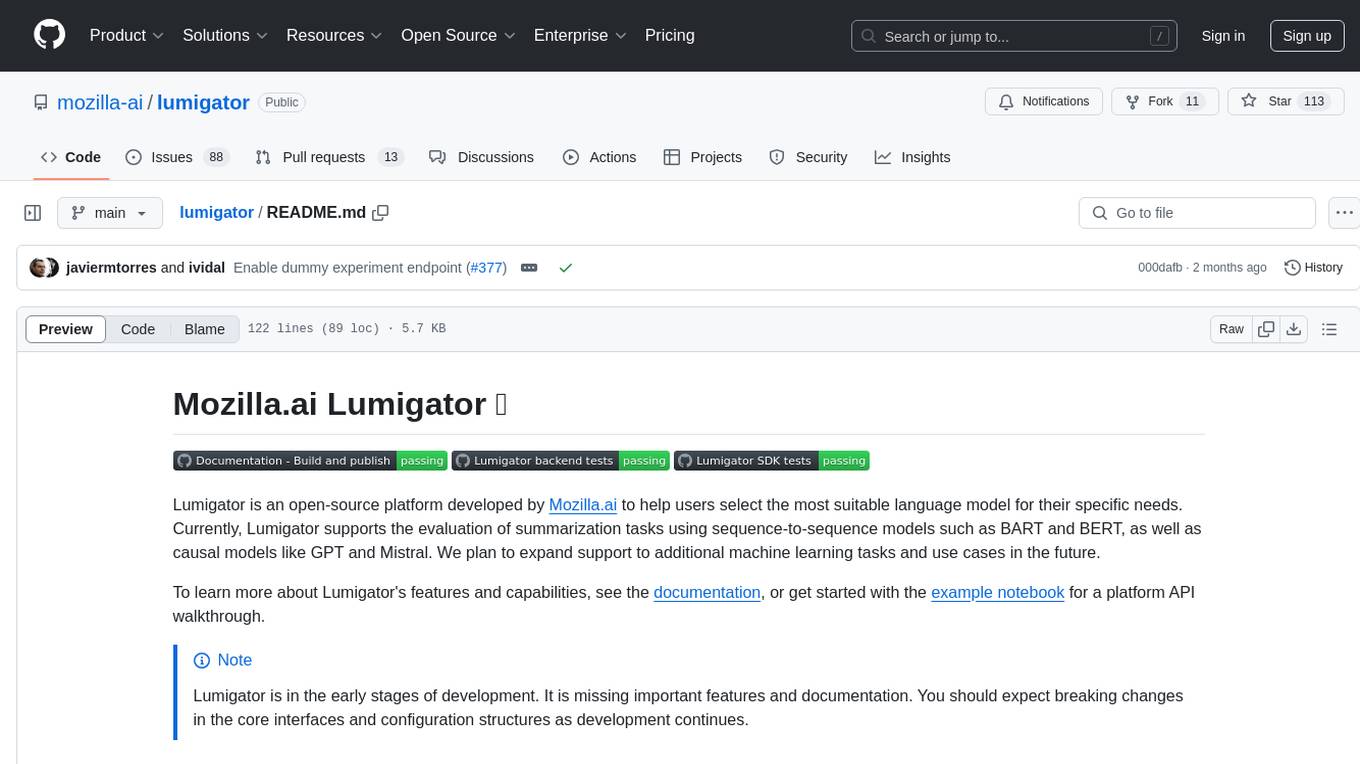
lumigator
Lumigator is an open-source platform developed by Mozilla.ai to help users select the most suitable language model for their specific needs. It supports the evaluation of summarization tasks using sequence-to-sequence models such as BART and BERT, as well as causal models like GPT and Mistral. The platform aims to make model selection transparent, efficient, and empowering by providing a framework for comparing LLMs using task-specific metrics to evaluate how well a model fits a project's needs. Lumigator is in the early stages of development and plans to expand support to additional machine learning tasks and use cases in the future.
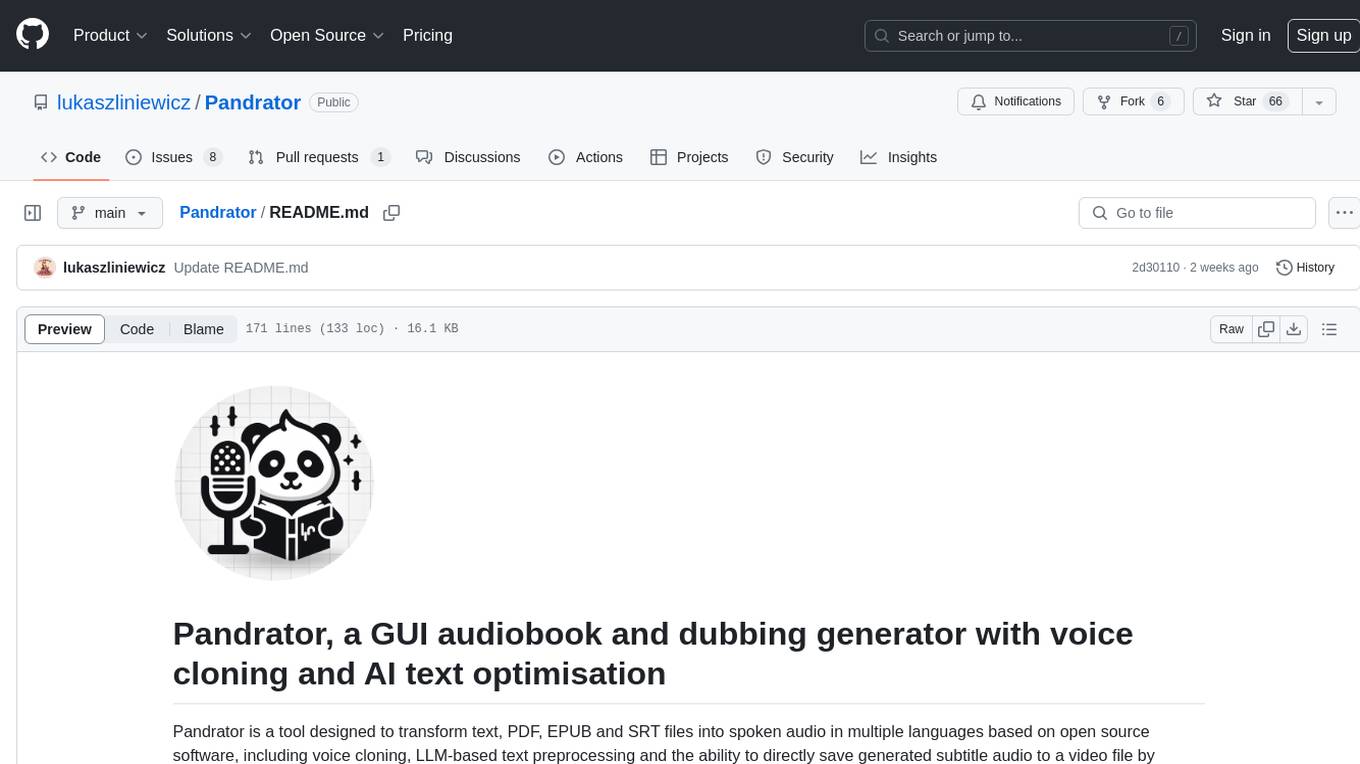
Pandrator
Pandrator is a GUI tool for generating audiobooks and dubbing using voice cloning and AI. It transforms text, PDF, EPUB, and SRT files into spoken audio in multiple languages. It leverages XTTS, Silero, and VoiceCraft models for text-to-speech conversion and voice cloning, with additional features like LLM-based text preprocessing and NISQA for audio quality evaluation. The tool aims to be user-friendly with a one-click installer and a graphical interface.
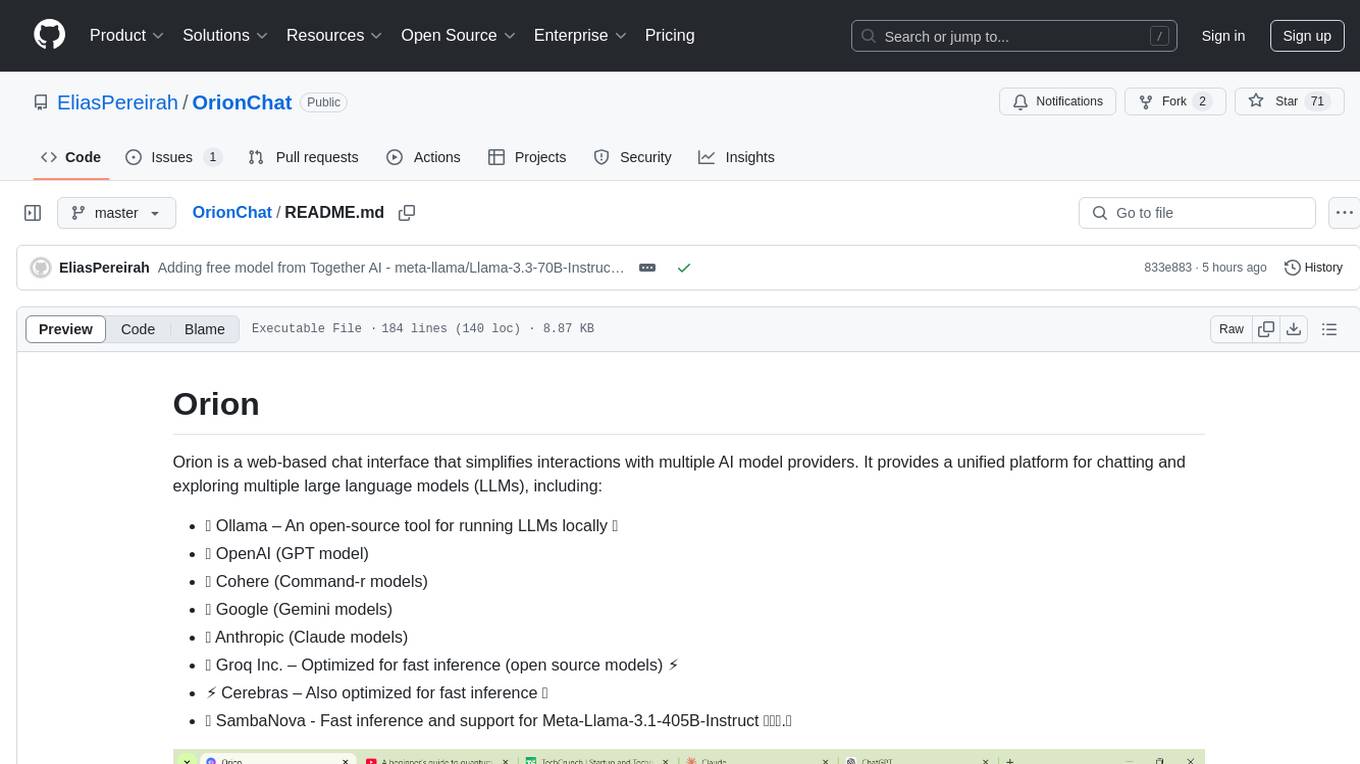
OrionChat
Orion is a web-based chat interface that simplifies interactions with multiple AI model providers. It provides a unified platform for chatting and exploring various large language models (LLMs) such as Ollama, OpenAI (GPT model), Cohere (Command-r models), Google (Gemini models), Anthropic (Claude models), Groq Inc., Cerebras, and SambaNova. Users can easily navigate and assess different AI models through an intuitive, user-friendly interface. Orion offers features like browser-based access, code execution with Google Gemini, text-to-speech (TTS), speech-to-text (STT), seamless integration with multiple AI models, customizable system prompts, language translation tasks, document uploads for analysis, and more. API keys are stored locally, and requests are sent directly to official providers' APIs without external proxies.
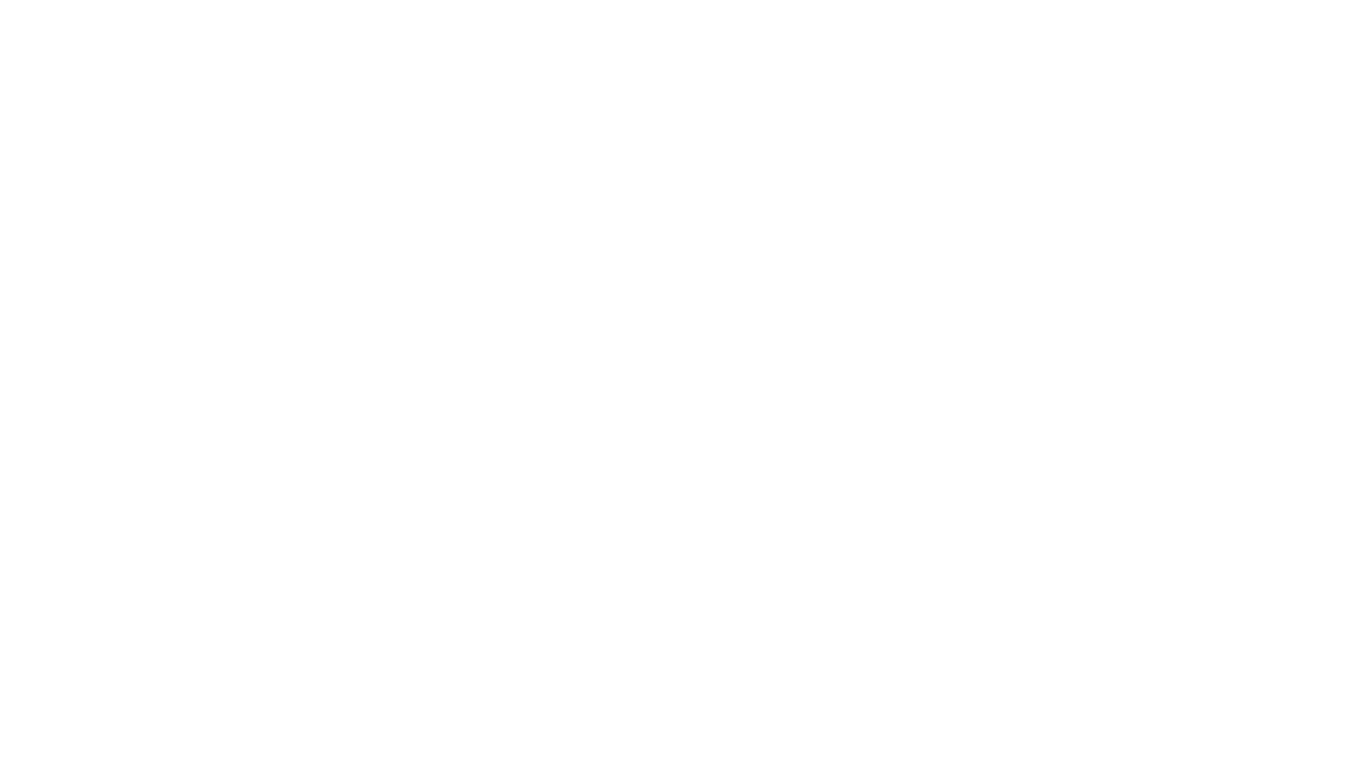
airflow
Apache Airflow (or simply Airflow) is a platform to programmatically author, schedule, and monitor workflows. When workflows are defined as code, they become more maintainable, versionable, testable, and collaborative. Use Airflow to author workflows as directed acyclic graphs (DAGs) of tasks. The Airflow scheduler executes your tasks on an array of workers while following the specified dependencies. Rich command line utilities make performing complex surgeries on DAGs a snap. The rich user interface makes it easy to visualize pipelines running in production, monitor progress, and troubleshoot issues when needed.
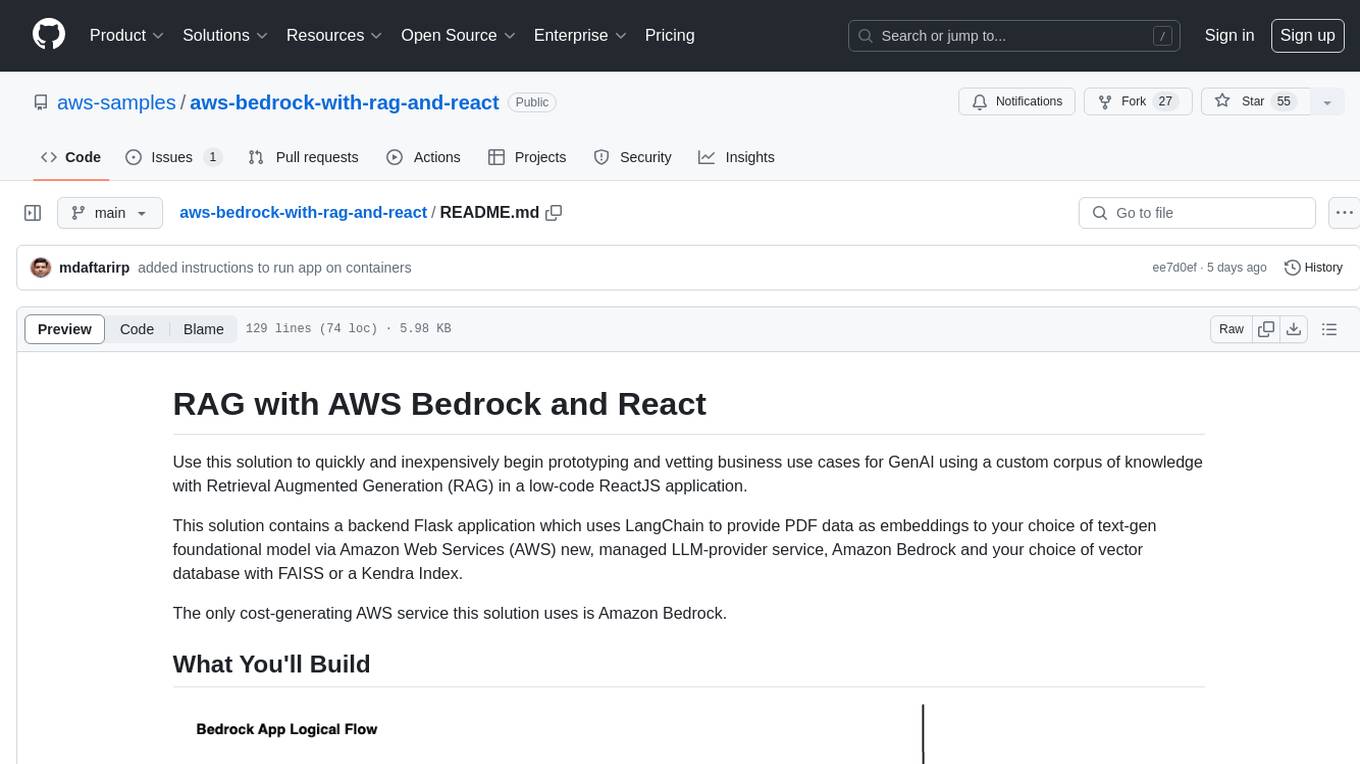
aws-bedrock-with-rag-and-react
This solution provides a low-code ReactJS application to prototype and vet business use cases for GenAI using Retrieval Augmented Generation (RAG). It includes a backend Flask application that uses LangChain to provide PDF data as embeddings to a text-gen model via Amazon Bedrock and a vector database with FAISS or Kendra Index. The solution utilizes Amazon Bedrock as the only cost-generating AWS service.
For similar tasks
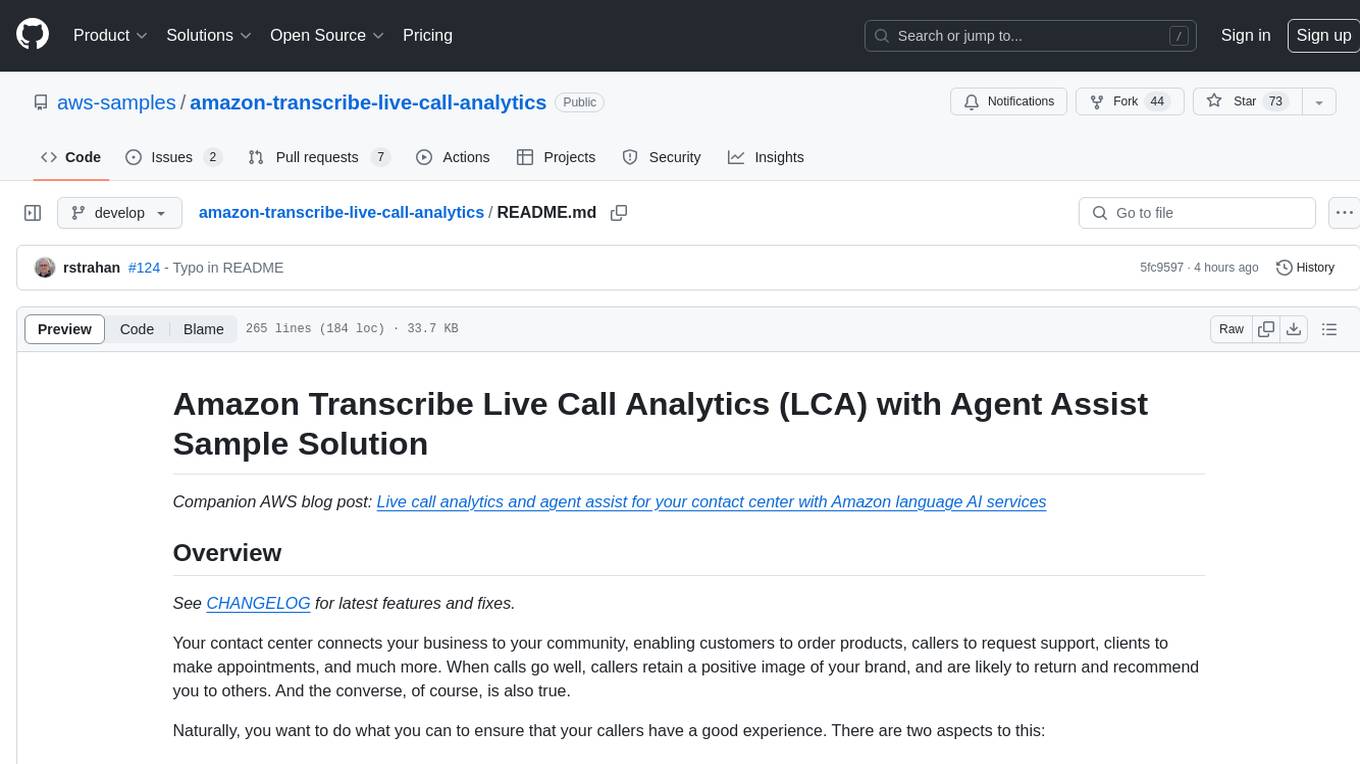
amazon-transcribe-live-call-analytics
The Amazon Transcribe Live Call Analytics (LCA) with Agent Assist Sample Solution is designed to help contact centers assess and optimize caller experiences in real time. It leverages Amazon machine learning services like Amazon Transcribe, Amazon Comprehend, and Amazon SageMaker to transcribe and extract insights from contact center audio. The solution provides real-time supervisor and agent assist features, integrates with existing contact centers, and offers a scalable, cost-effective approach to improve customer interactions. The end-to-end architecture includes features like live call transcription, call summarization, AI-powered agent assistance, and real-time analytics. The solution is event-driven, ensuring low latency and seamless processing flow from ingested speech to live webpage updates.
For similar jobs
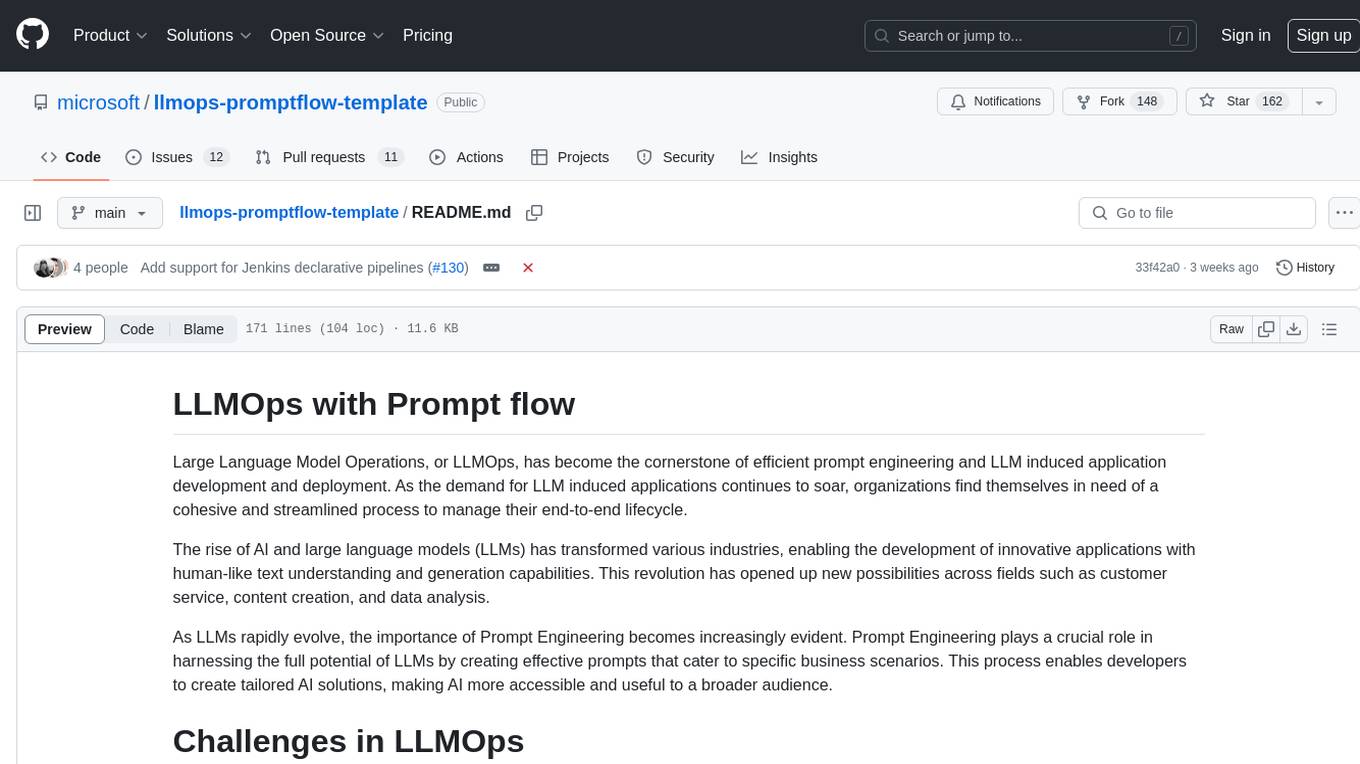
llmops-promptflow-template
LLMOps with Prompt flow is a template and guidance for building LLM-infused apps using Prompt flow. It provides centralized code hosting, lifecycle management, variant and hyperparameter experimentation, A/B deployment, many-to-many dataset/flow relationships, multiple deployment targets, comprehensive reporting, BYOF capabilities, configuration-based development, local prompt experimentation and evaluation, endpoint testing, and optional Human-in-loop validation. The tool is customizable to suit various application needs.
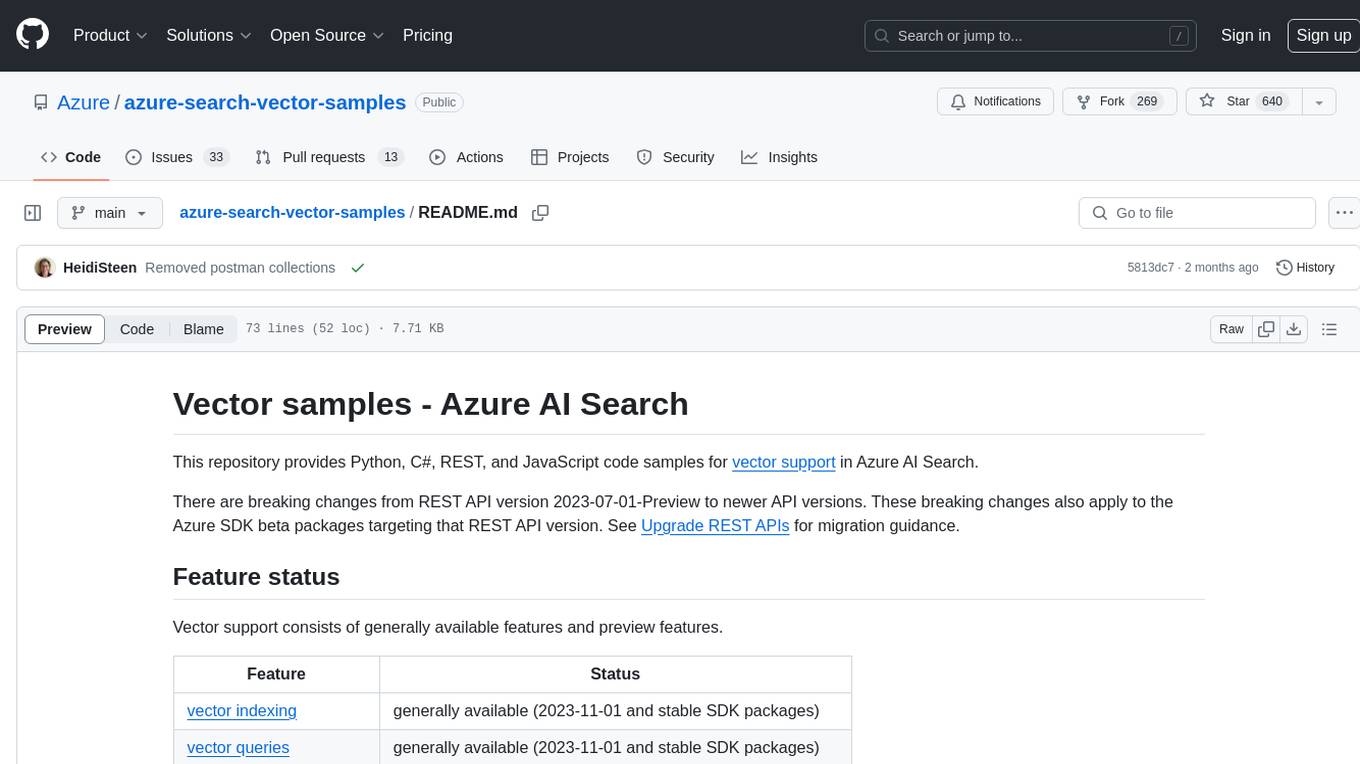
azure-search-vector-samples
This repository provides code samples in Python, C#, REST, and JavaScript for vector support in Azure AI Search. It includes demos for various languages showcasing vectorization of data, creating indexes, and querying vector data. Additionally, it offers tools like Azure AI Search Lab for experimenting with AI-enabled search scenarios in Azure and templates for deploying custom chat-with-your-data solutions. The repository also features documentation on vector search, hybrid search, creating and querying vector indexes, and REST API references for Azure AI Search and Azure OpenAI Service.
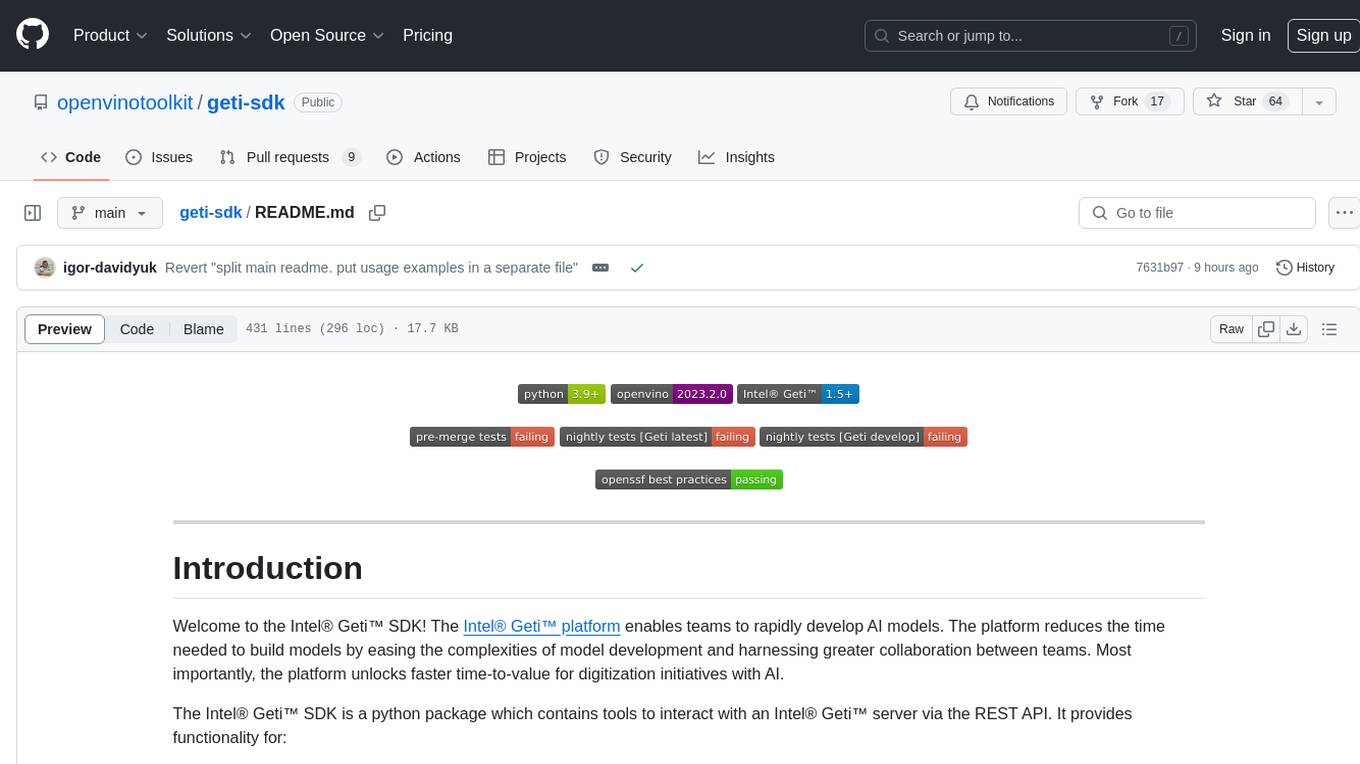
geti-sdk
The Intel® Geti™ SDK is a python package that enables teams to rapidly develop AI models by easing the complexities of model development and enhancing collaboration between teams. It provides tools to interact with an Intel® Geti™ server via the REST API, allowing for project creation, downloading, uploading, deploying for local inference with OpenVINO, setting project and model configuration, launching and monitoring training jobs, and media upload and prediction. The SDK also includes tutorial-style Jupyter notebooks demonstrating its usage.
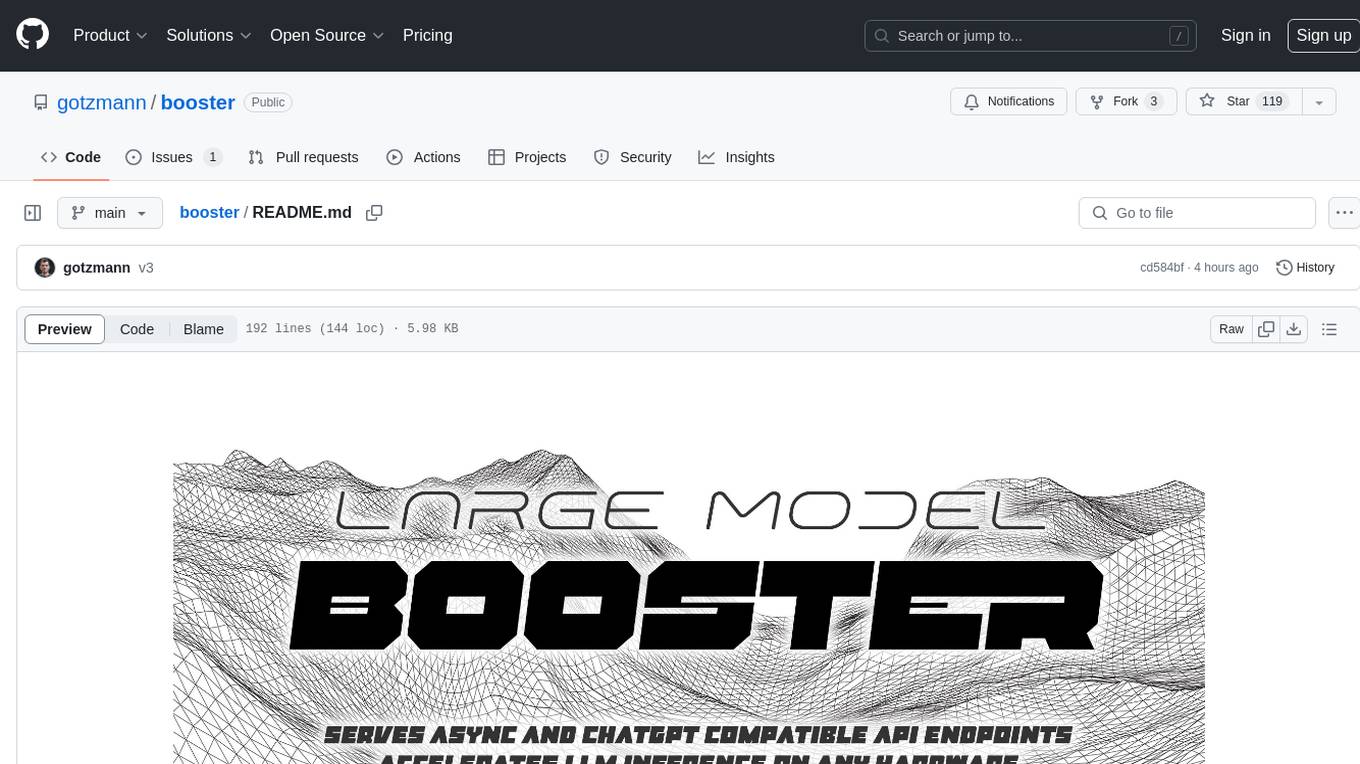
booster
Booster is a powerful inference accelerator designed for scaling large language models within production environments or for experimental purposes. It is built with performance and scaling in mind, supporting various CPUs and GPUs, including Nvidia CUDA, Apple Metal, and OpenCL cards. The tool can split large models across multiple GPUs, offering fast inference on machines with beefy GPUs. It supports both regular FP16/FP32 models and quantised versions, along with popular LLM architectures. Additionally, Booster features proprietary Janus Sampling for code generation and non-English languages.
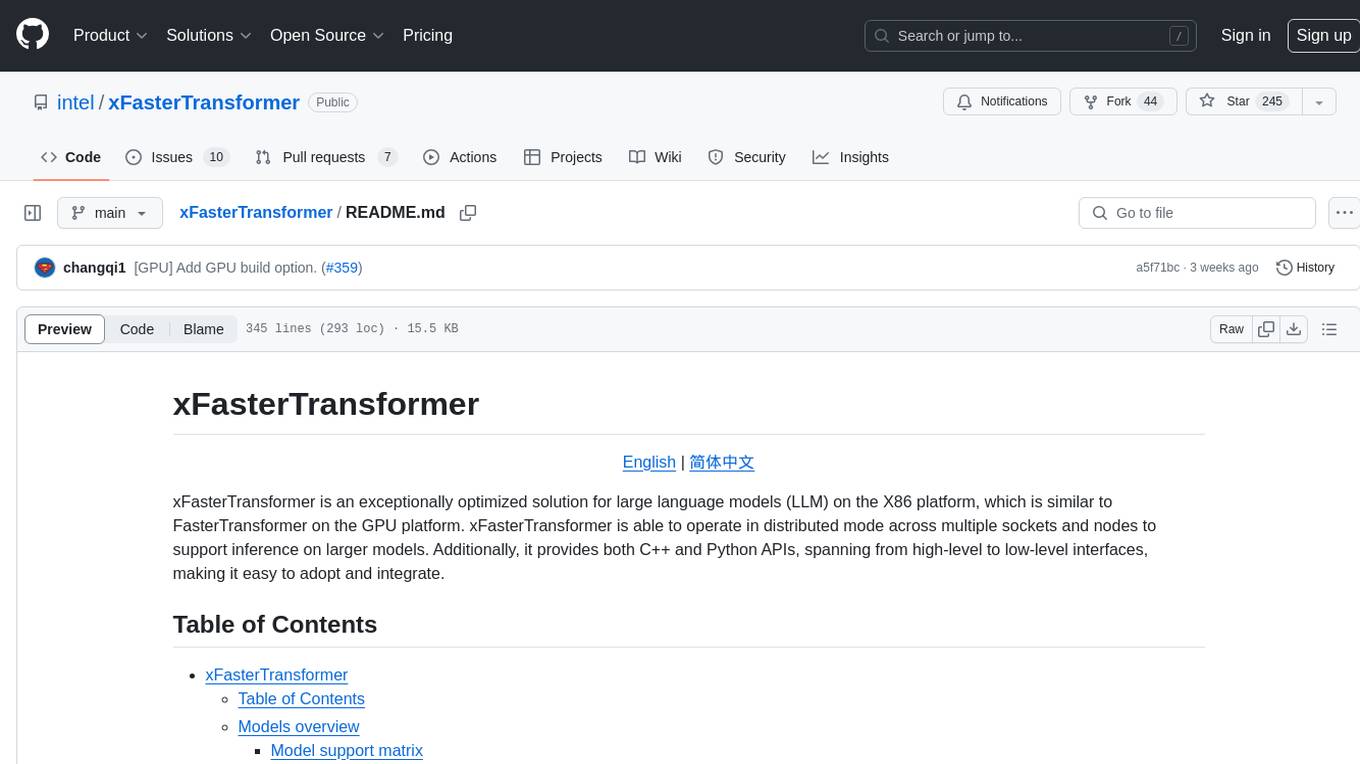
xFasterTransformer
xFasterTransformer is an optimized solution for Large Language Models (LLMs) on the X86 platform, providing high performance and scalability for inference on mainstream LLM models. It offers C++ and Python APIs for easy integration, along with example codes and benchmark scripts. Users can prepare models in a different format, convert them, and use the APIs for tasks like encoding input prompts, generating token ids, and serving inference requests. The tool supports various data types and models, and can run in single or multi-rank modes using MPI. A web demo based on Gradio is available for popular LLM models like ChatGLM and Llama2. Benchmark scripts help evaluate model inference performance quickly, and MLServer enables serving with REST and gRPC interfaces.
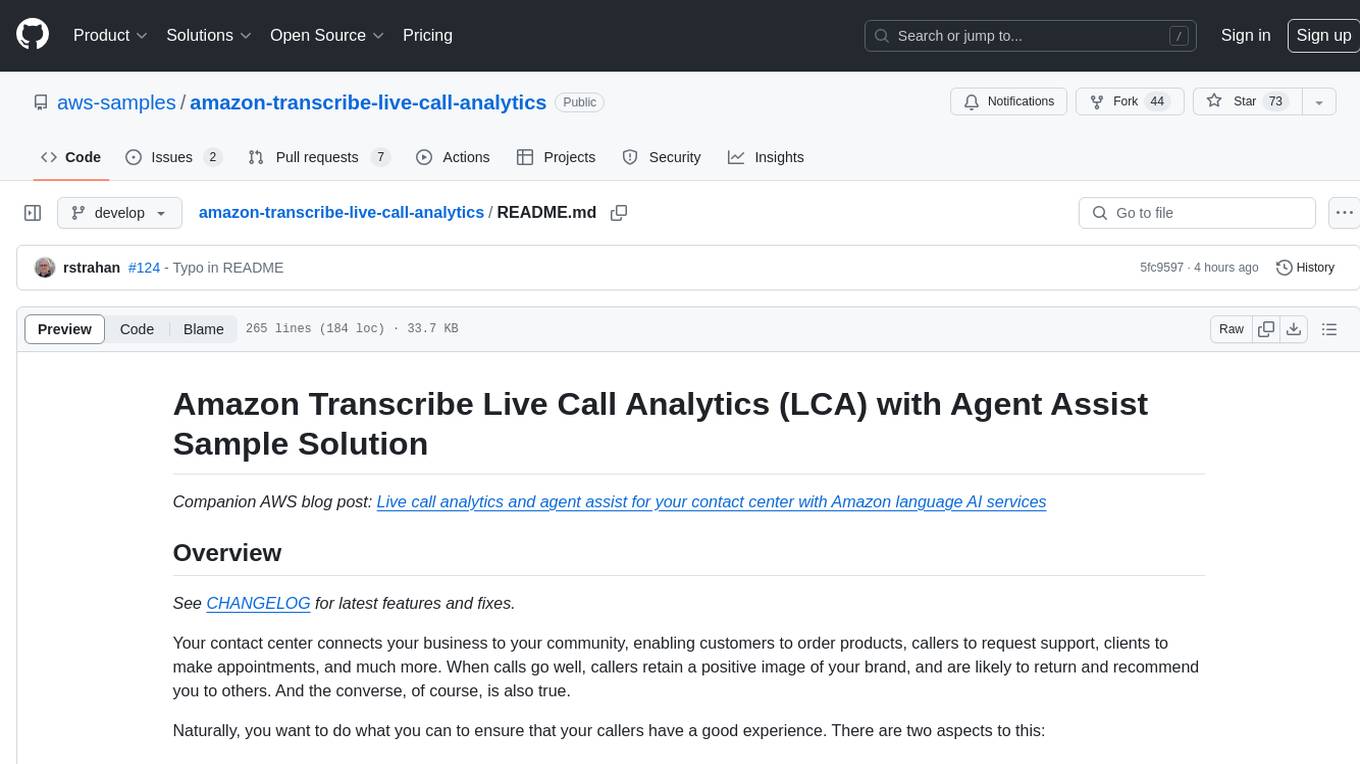
amazon-transcribe-live-call-analytics
The Amazon Transcribe Live Call Analytics (LCA) with Agent Assist Sample Solution is designed to help contact centers assess and optimize caller experiences in real time. It leverages Amazon machine learning services like Amazon Transcribe, Amazon Comprehend, and Amazon SageMaker to transcribe and extract insights from contact center audio. The solution provides real-time supervisor and agent assist features, integrates with existing contact centers, and offers a scalable, cost-effective approach to improve customer interactions. The end-to-end architecture includes features like live call transcription, call summarization, AI-powered agent assistance, and real-time analytics. The solution is event-driven, ensuring low latency and seamless processing flow from ingested speech to live webpage updates.
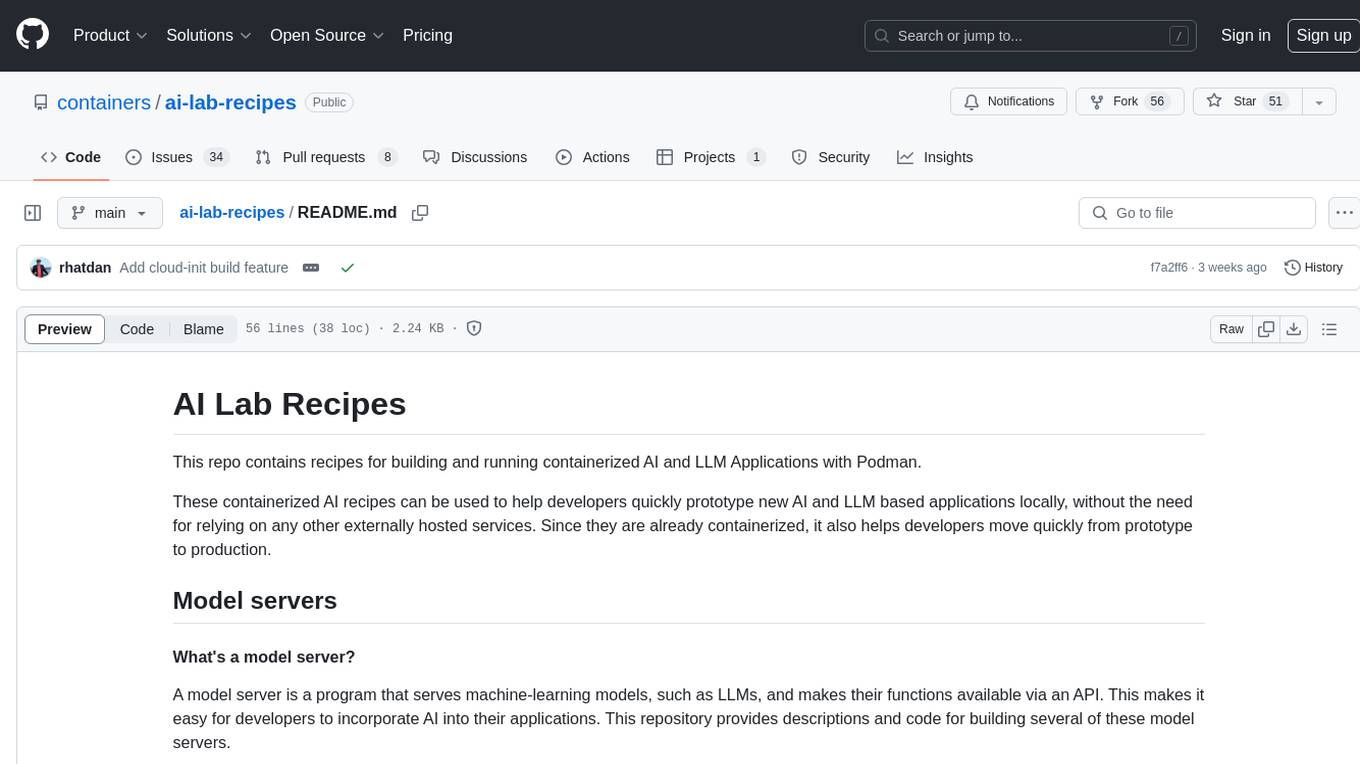
ai-lab-recipes
This repository contains recipes for building and running containerized AI and LLM applications with Podman. It provides model servers that serve machine-learning models via an API, allowing developers to quickly prototype new AI applications locally. The recipes include components like model servers and AI applications for tasks such as chat, summarization, object detection, etc. Images for sample applications and models are available in `quay.io`, and bootable containers for AI training on Linux OS are enabled.
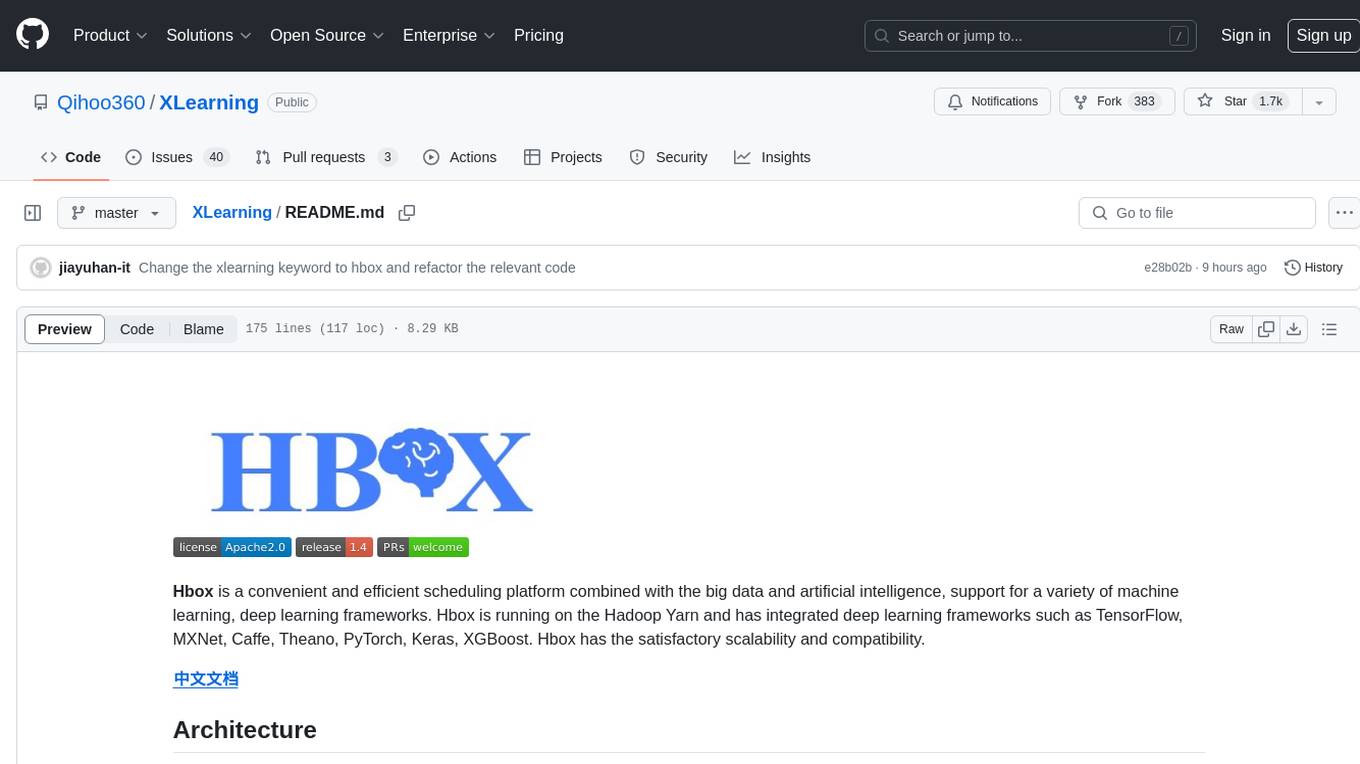
XLearning
XLearning is a scheduling platform for big data and artificial intelligence, supporting various machine learning and deep learning frameworks. It runs on Hadoop Yarn and integrates frameworks like TensorFlow, MXNet, Caffe, Theano, PyTorch, Keras, XGBoost. XLearning offers scalability, compatibility, multiple deep learning framework support, unified data management based on HDFS, visualization display, and compatibility with code at native frameworks. It provides functions for data input/output strategies, container management, TensorBoard service, and resource usage metrics display. XLearning requires JDK >= 1.7 and Maven >= 3.3 for compilation, and deployment on CentOS 7.2 with Java >= 1.7 and Hadoop 2.6, 2.7, 2.8.