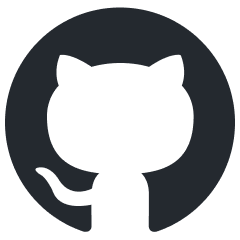
XLearning
AI on Hadoop
Stars: 1733
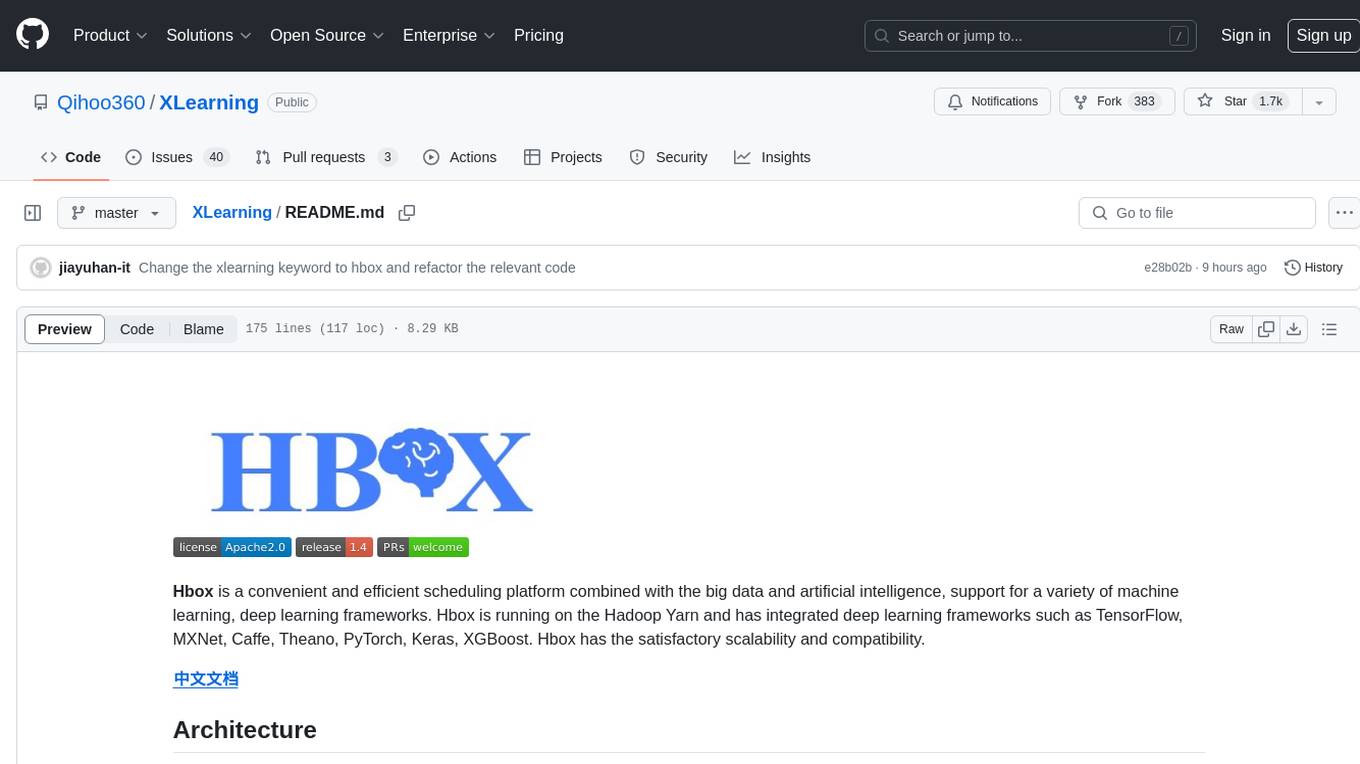
XLearning is a scheduling platform for big data and artificial intelligence, supporting various machine learning and deep learning frameworks. It runs on Hadoop Yarn and integrates frameworks like TensorFlow, MXNet, Caffe, Theano, PyTorch, Keras, XGBoost. XLearning offers scalability, compatibility, multiple deep learning framework support, unified data management based on HDFS, visualization display, and compatibility with code at native frameworks. It provides functions for data input/output strategies, container management, TensorBoard service, and resource usage metrics display. XLearning requires JDK >= 1.7 and Maven >= 3.3 for compilation, and deployment on CentOS 7.2 with Java >= 1.7 and Hadoop 2.6, 2.7, 2.8.
README:
Hbox is a convenient and efficient scheduling platform combined with the big data and artificial intelligence, support for a variety of machine learning, deep learning frameworks. Hbox is running on the Hadoop Yarn and has integrated deep learning frameworks such as TensorFlow, MXNet, Caffe, Theano, PyTorch, Keras, XGBoost. Hbox has the satisfactory scalability and compatibility.
There are three essential components in Hbox:
- Client: start and get the state of the application.
- ApplicationMaster(AM): the role for the internal schedule and lifecycle manager, including the input data distribution and containers management.
- Container: the actual executor of the application to start the progress of Worker or PS(Parameter Server), monitor and report the status of the progress to AM, and save the output, especially start the TensorBoard service for TensorFlow application.
Besides the distributed mode of TensorFlow and MXNet frameworks, Hbox supports the standalone mode of all deep learning frameworks such as Caffe, Theano, PyTorch. Moreover, Hbox allows the custom versions and multi-version of frameworks flexibly.
Hbox is enable to specify the input strategy for the input data --input
by setting the --input-strategy
parameter or hbox.input.strategy
configuration. Hbox support three ways to read the HDFS input data:
- Download: AM traverses all files under the specified HDFS path and distributes data to workers in files. Each worker download files from the remote to local.
- Placeholder: The difference with Download mode is that AM send the related HDFS file list to workers. The process in worker read the data from HDFS directly.
- InputFormat: Integrated the InputFormat function of MapReduce, Hbox allows the user to specify any of the implementation of InputFormat for the input data. AM splits the input data and assigns fragments to the different workers. Each worker passes the assigned fragments through the pipeline to the execution progress.
Similar with the read strategy, Hbox allows to specify the output strategy for the output data --output
by setting the --output-strategy
parameter or hbox.output.strategy
configuration. There are two kinds of result output modes:
- Upload: After the program finished, each worker upload the local directory of the output to specified HDFS path directly. The button, "Saved Model", on the web interface allows user to upload the intermediate result to remote during the execution.
- OutputFormat: Integrated the OutputFormat function of MapReduce, Hbox allows the user to specify any of the implementation of OutputFormat for saving the result to HDFS.
More detail see data management
The application interface can be divided into four parts:
- All Containers:display the container list and corresponding information, including the container host, container role, current state of container, start time, finish time, current progress.
- View TensorBoard:If set to start the service of TensorBoard when the type of application is TensorFlow, provide the link to enter the TensorBoard for real-time view.
- Save Model:If the application has the output, user can upload the intermediate output to specified HDFS path during the execution of the application through the button of "Save Model". After the upload finished, display the list of the intermediate saved path.
-
Worker Metrix:display the resource usage information metrics of each worker.
As shown below:
Except the automatic construction of the ClusterSpec at the distributed mode TensorFlow framework, the program at standalone mode TensorFlow and other deep learning frameworks can be executed at Hbox directly.
- jdk >= 1.7
- Maven >= 3.3
Run the following command in the root directory of the source code:
mvn package
After compiling, a distribution package named hbox-1.1-dist.tar.gz
will be generated under target
in the root directory.
Unpacking the distribution package, the following subdirectories will be generated under the root directory:
- bin: scripts for application commit
- lib: jars for Hbox and dependencies
- conf: configuration files
- sbin: scripts for history service
- data: data and files for examples
- examples: Hbox examples
- CentOS 7.2
- Java >= 1.7
- Hadoop = 2.6, 2.7, 2.8
- [optional] Dependent environment for deep learning frameworks at the cluster nodes, such as TensorFlow, numpy, Caffe.
Under the "conf" directory of the unpacking distribution package "$HBOX_HOME", configure the related files:
-
hbox-env.sh: set the environment variables, such as:
- JAVA_HOME
- HADOOP_CONF_DIR
-
hbox-site.xml: configure related properties. Note that the properties associated with the history service needs to be consistent with what has configured when the history service started.For more details, please see the Configuration part。
-
log4j.properties:configure the log level
- run
$HBOX_HOME/sbin/start-history-server.sh
.
Use $HBOX_HOME/bin/xl-submit
to submit the application to cluster in the Hbox client.
Here are the submit example for the TensorFlow application.
upload the "data" directory under the root of unpacking distribution package to HDFS
cd $HBOX_HOME
hadoop fs -put data /tmp/
cd $HBOX_HOME/examples/tensorflow
$HBOX_HOME/bin/xl-submit \
--app-type "tensorflow" \
--app-name "tf-demo" \
--input /tmp/data/tensorflow#data \
--output /tmp/tensorflow_model#model \
--files demo.py,dataDeal.py \
--launch-cmd "python demo.py --data_path=./data --save_path=./model --log_dir=./eventLog --training_epochs=10" \
--worker-memory 10G \
--worker-num 2 \
--worker-cores 3 \
--ps-memory 1G \
--ps-num 1 \
--ps-cores 2 \
--queue default \
The meaning of the parameters are as follows:
Property Name | Meaning |
---|---|
app-name | application name as "tf-demo" |
app-type | application type as "tensorflow" |
input | input file, HDFS path is "/tmp/data/tensorflow" related to local dir "./data" |
output | output file,HDFS path is "/tmp/tensorflow_model" related to local dir "./model" |
files | application program and required local files, including demo.py, dataDeal.py |
launch-cmd | execute command |
worker-memory | amount of memory to use for the worker process is 10GB |
worker-num | number of worker containers to use for the application is 2 |
worker-cores | number of cores to use for the worker process is 3 |
ps-memory | amount of memory to use for the ps process is 1GB |
ps-num | number of ps containers to use for the application is 1 |
ps-cores | number of cores to use for the ps process is 2 |
queue | the queue that application submit to |
For more details, set the Submit Parameter part。
Hbox
is designed, authored, reviewed and tested by the team at the github:
@Yuance Li, @Wen OuYang, @Runying Jia, @YuHan Jia, @Lei Wang
Mail: [email protected]
QQ群:588356340
For Tasks:
Click tags to check more tools for each tasksFor Jobs:
Alternative AI tools for XLearning
Similar Open Source Tools
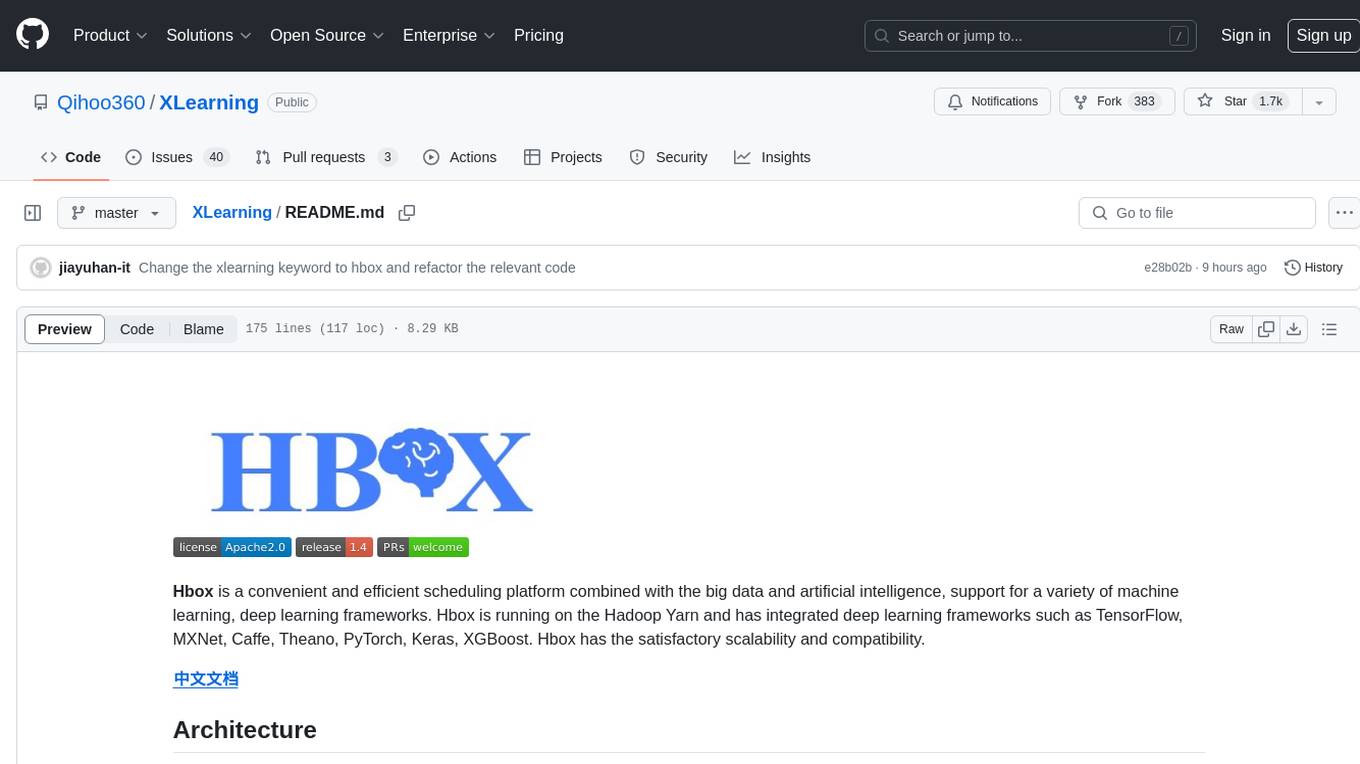
XLearning
XLearning is a scheduling platform for big data and artificial intelligence, supporting various machine learning and deep learning frameworks. It runs on Hadoop Yarn and integrates frameworks like TensorFlow, MXNet, Caffe, Theano, PyTorch, Keras, XGBoost. XLearning offers scalability, compatibility, multiple deep learning framework support, unified data management based on HDFS, visualization display, and compatibility with code at native frameworks. It provides functions for data input/output strategies, container management, TensorBoard service, and resource usage metrics display. XLearning requires JDK >= 1.7 and Maven >= 3.3 for compilation, and deployment on CentOS 7.2 with Java >= 1.7 and Hadoop 2.6, 2.7, 2.8.
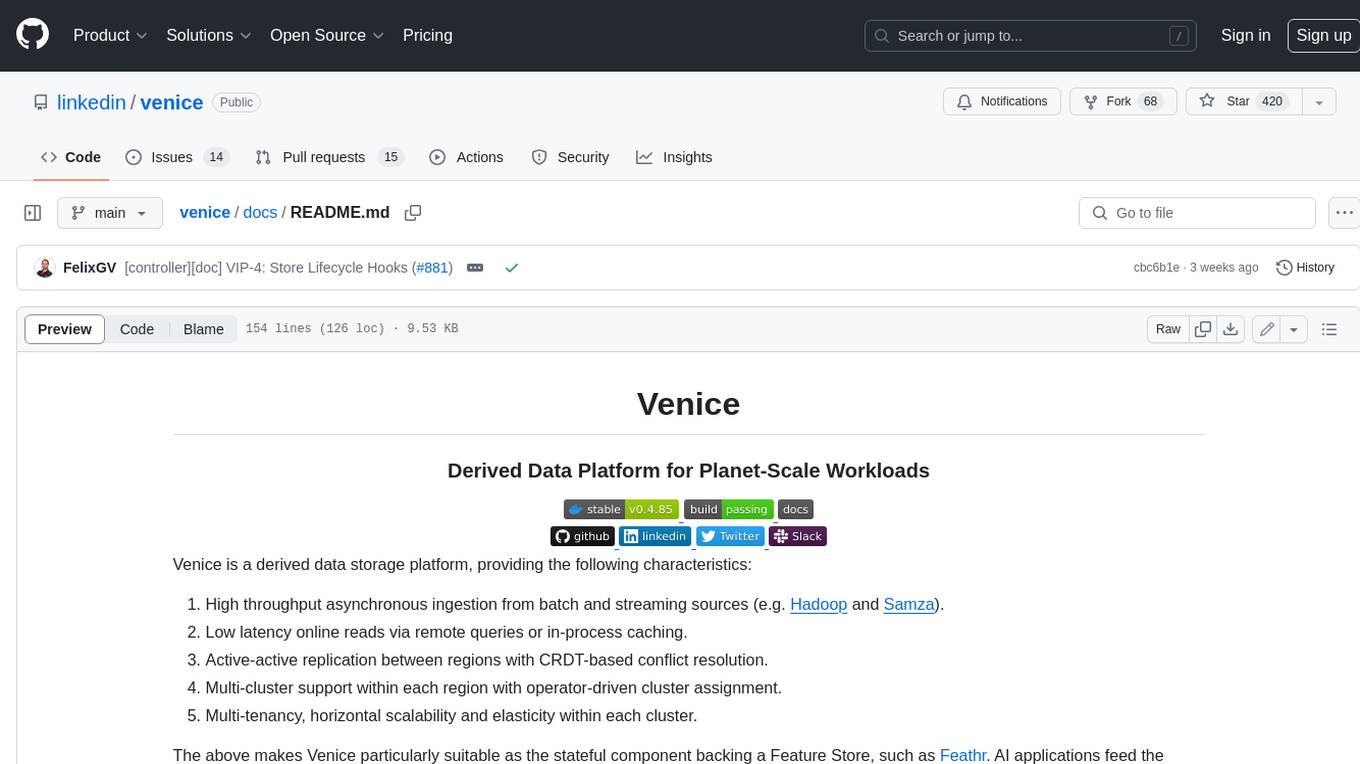
venice
Venice is a derived data storage platform, providing the following characteristics: 1. High throughput asynchronous ingestion from batch and streaming sources (e.g. Hadoop and Samza). 2. Low latency online reads via remote queries or in-process caching. 3. Active-active replication between regions with CRDT-based conflict resolution. 4. Multi-cluster support within each region with operator-driven cluster assignment. 5. Multi-tenancy, horizontal scalability and elasticity within each cluster. The above makes Venice particularly suitable as the stateful component backing a Feature Store, such as Feathr. AI applications feed the output of their ML training jobs into Venice and then query the data for use during online inference workloads.
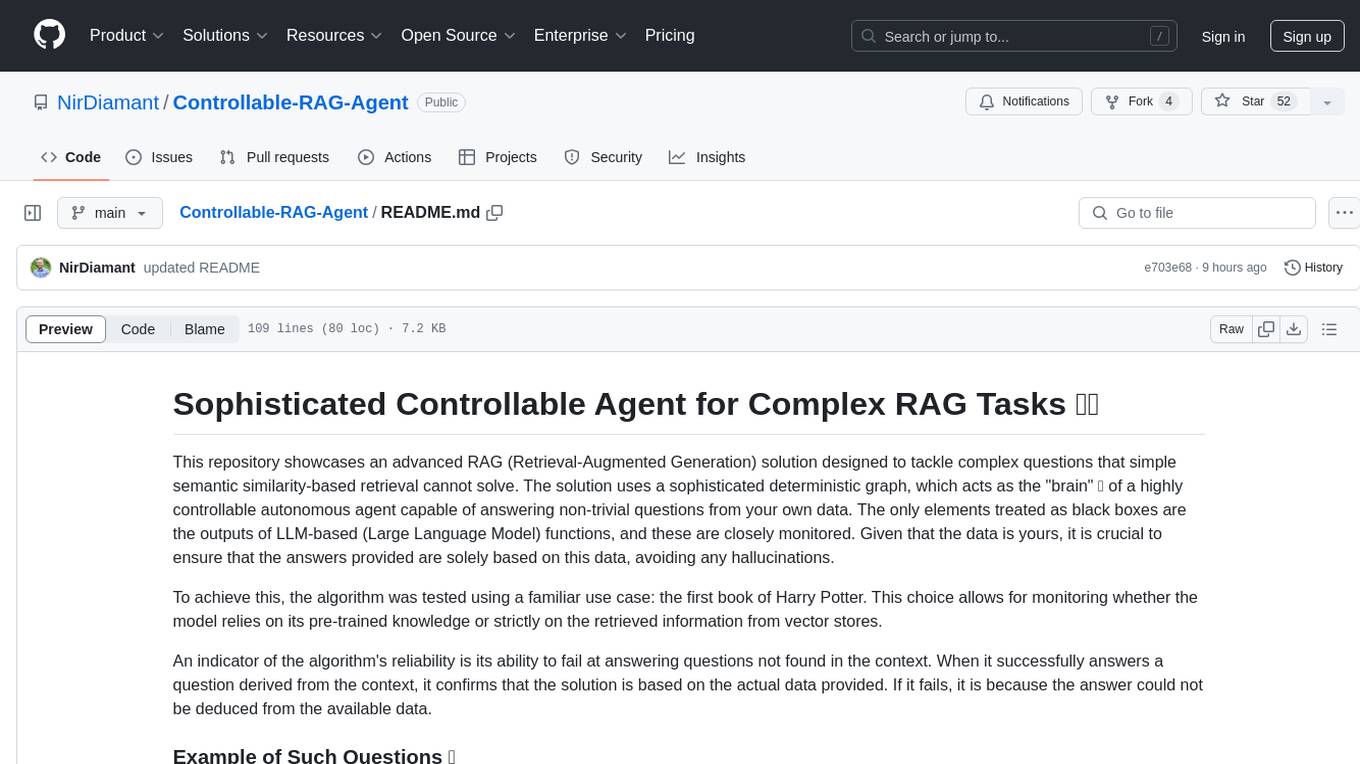
Controllable-RAG-Agent
This repository contains a sophisticated deterministic graph-based solution for answering complex questions using a controllable autonomous agent. The solution is designed to ensure that answers are solely based on the provided data, avoiding hallucinations. It involves various steps such as PDF loading, text preprocessing, summarization, database creation, encoding, and utilizing large language models. The algorithm follows a detailed workflow involving planning, retrieval, answering, replanning, content distillation, and performance evaluation. Heuristics and techniques implemented focus on content encoding, anonymizing questions, task breakdown, content distillation, chain of thought answering, verification, and model performance evaluation.
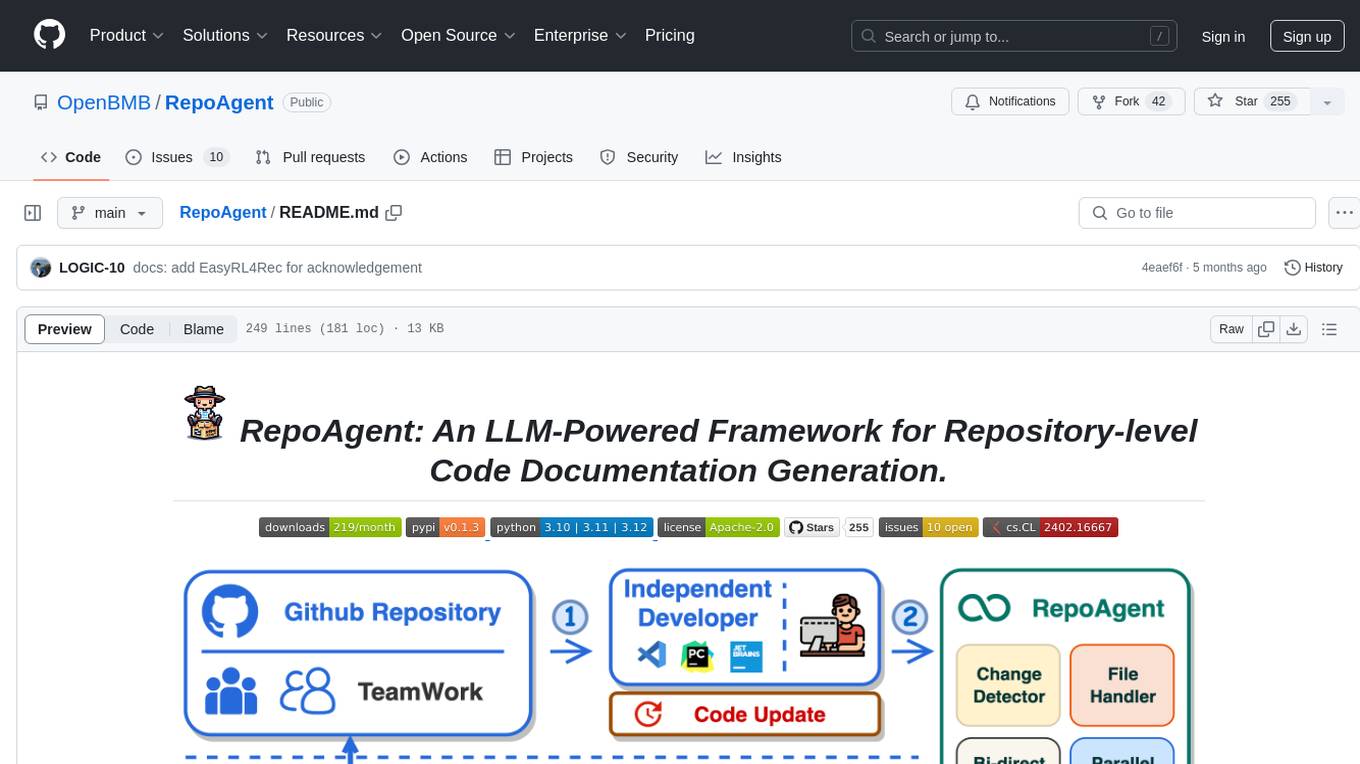
RepoAgent
RepoAgent is an LLM-powered framework designed for repository-level code documentation generation. It automates the process of detecting changes in Git repositories, analyzing code structure through AST, identifying inter-object relationships, replacing Markdown content, and executing multi-threaded operations. The tool aims to assist developers in understanding and maintaining codebases by providing comprehensive documentation, ultimately improving efficiency and saving time.
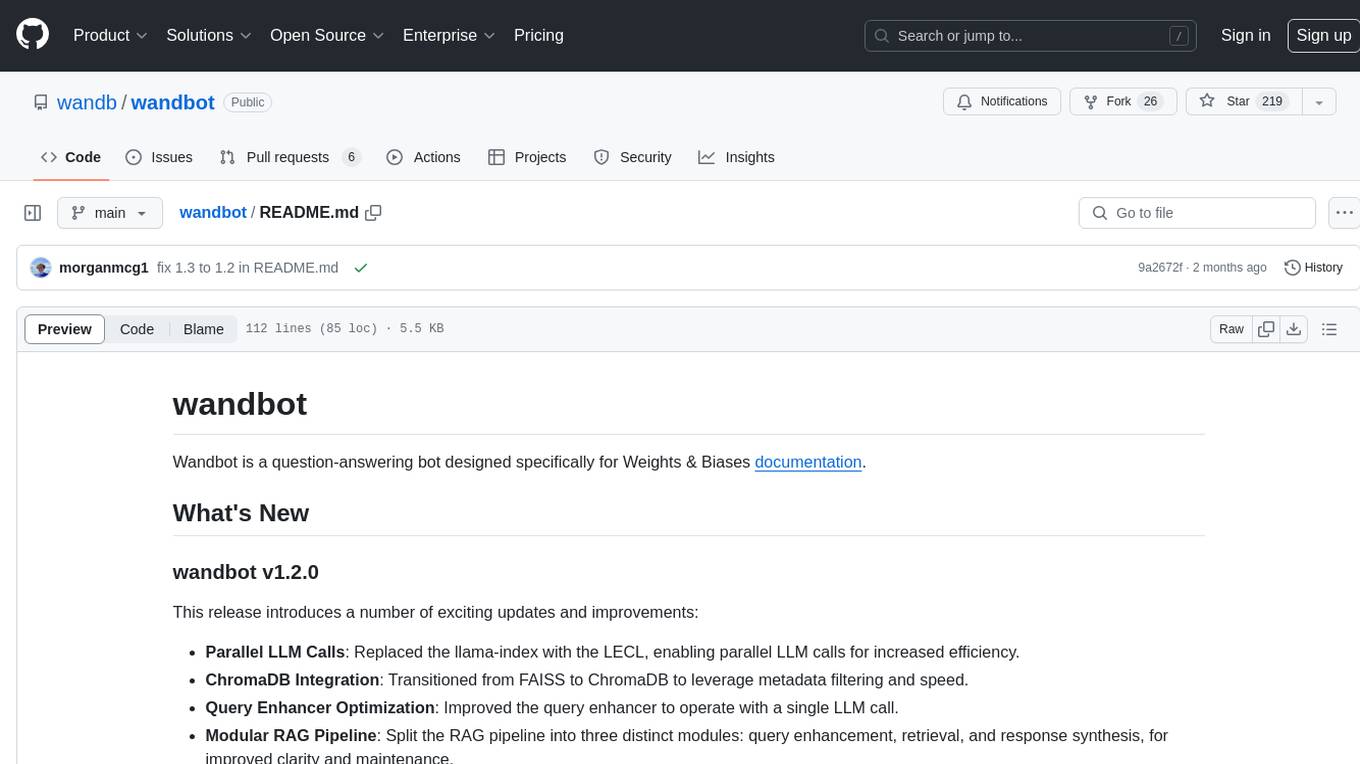
wandbot
Wandbot is a question-answering bot designed for Weights & Biases documentation. It employs Retrieval Augmented Generation with a ChromaDB backend for efficient responses. The bot features periodic data ingestion, integration with Discord and Slack, and performance monitoring through logging. It has a fallback mechanism for model selection and is evaluated based on retrieval accuracy and model-generated responses. The implementation includes creating document embeddings, constructing the Q&A RAGPipeline, model selection, deployment on FastAPI, Discord, and Slack, logging and analysis with Weights & Biases Tables, and performance evaluation.
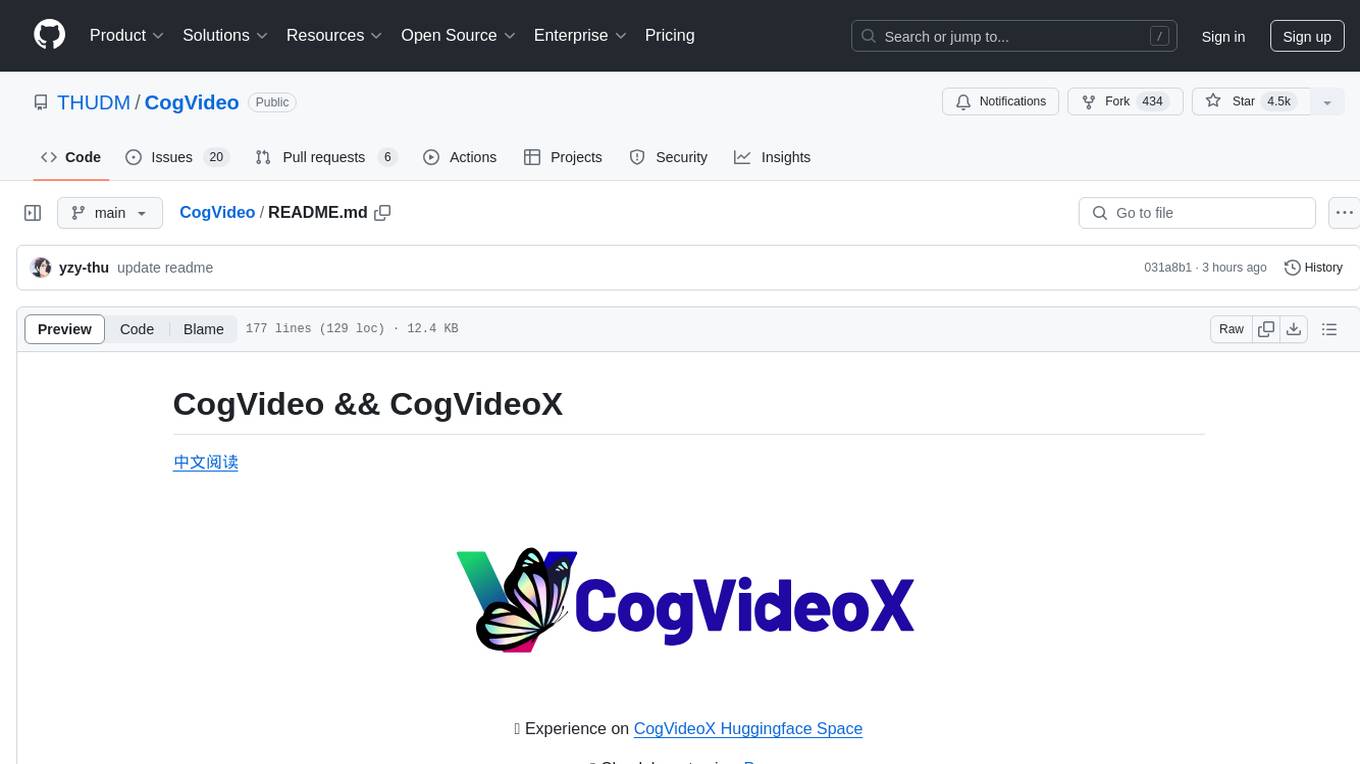
CogVideo
CogVideo is an open-source repository that provides pretrained text-to-video models for generating videos based on input text. It includes models like CogVideoX-2B and CogVideo, offering powerful video generation capabilities. The repository offers tools for inference, fine-tuning, and model conversion, along with demos showcasing the model's capabilities through CLI, web UI, and online experiences. CogVideo aims to facilitate the creation of high-quality videos from textual descriptions, catering to a wide range of applications.
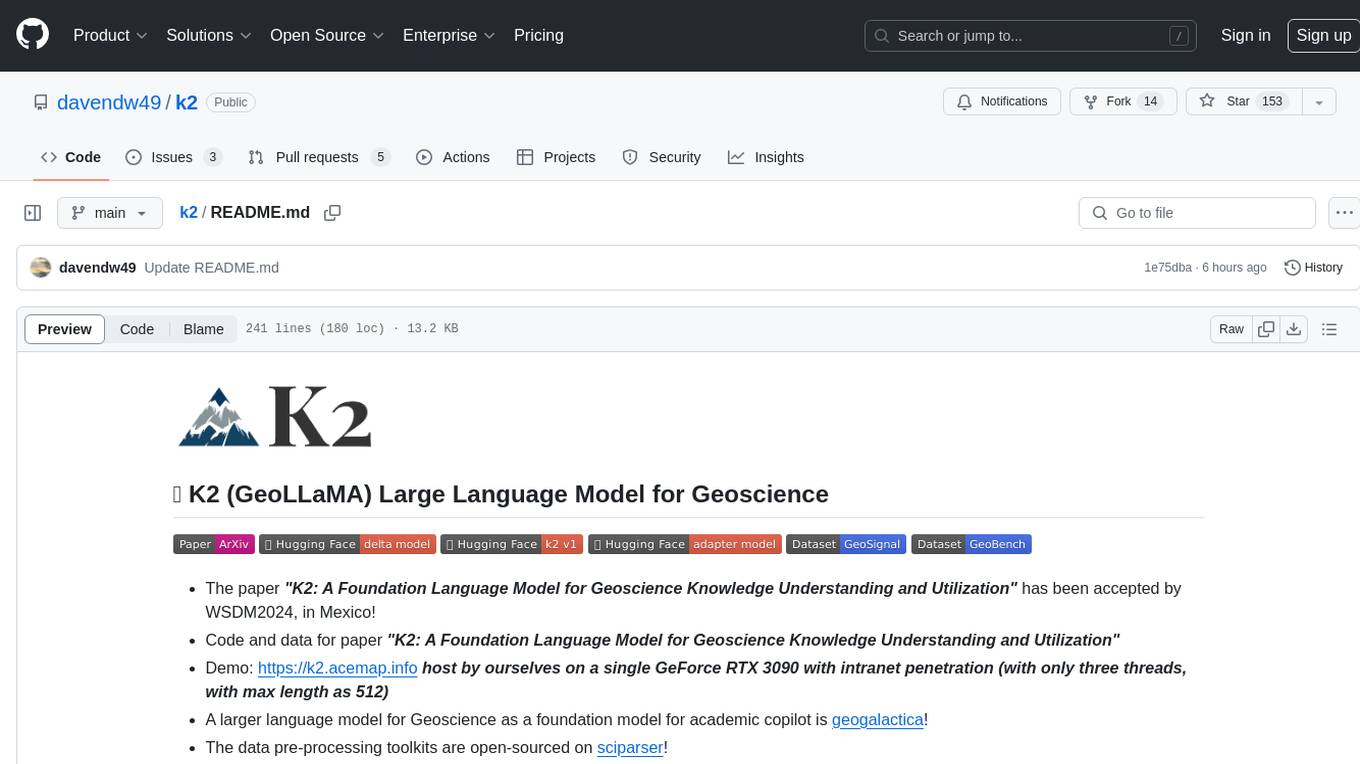
k2
K2 (GeoLLaMA) is a large language model for geoscience, trained on geoscience literature and fine-tuned with knowledge-intensive instruction data. It outperforms baseline models on objective and subjective tasks. The repository provides K2 weights, core data of GeoSignal, GeoBench benchmark, and code for further pretraining and instruction tuning. The model is available on Hugging Face for use. The project aims to create larger and more powerful geoscience language models in the future.
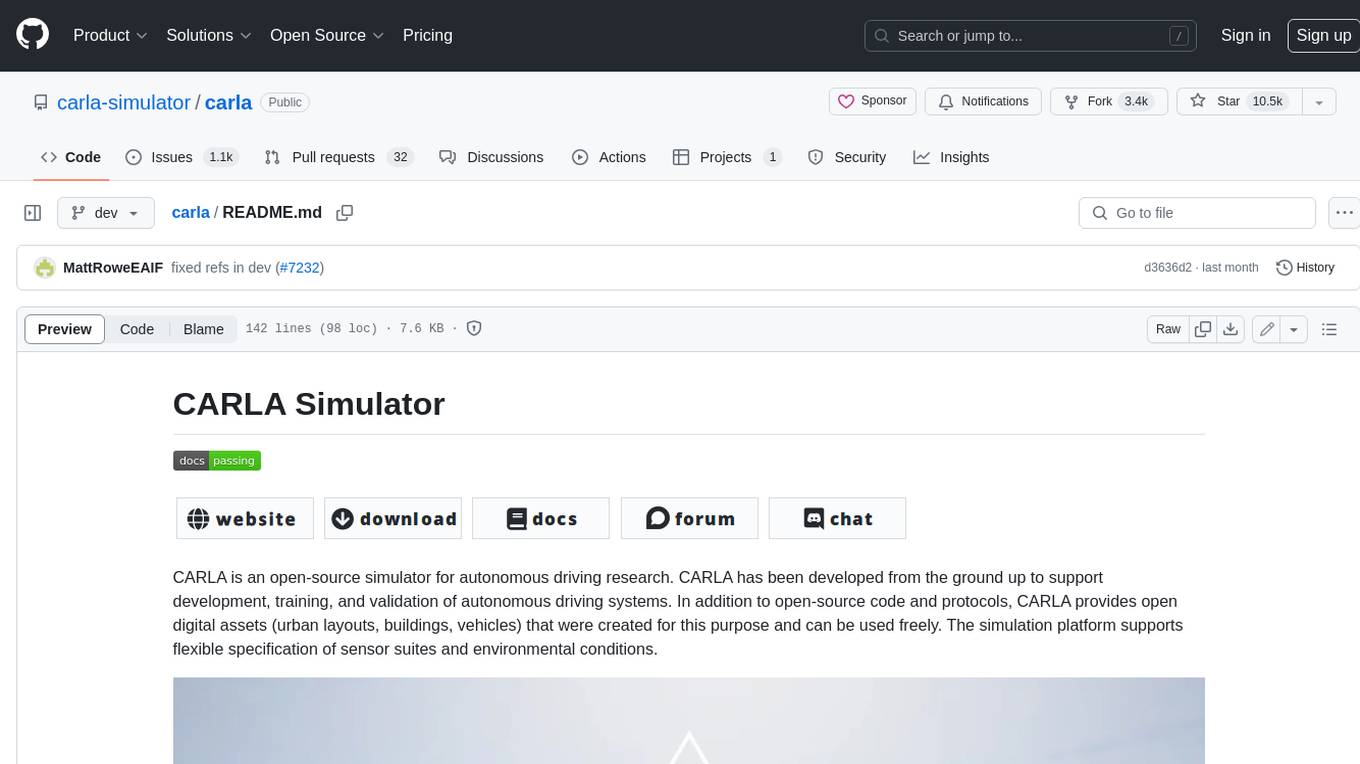
carla
CARLA is an open-source simulator for autonomous driving research. It provides open-source code, protocols, and digital assets (urban layouts, buildings, vehicles) for developing, training, and validating autonomous driving systems. CARLA supports flexible specification of sensor suites and environmental conditions.
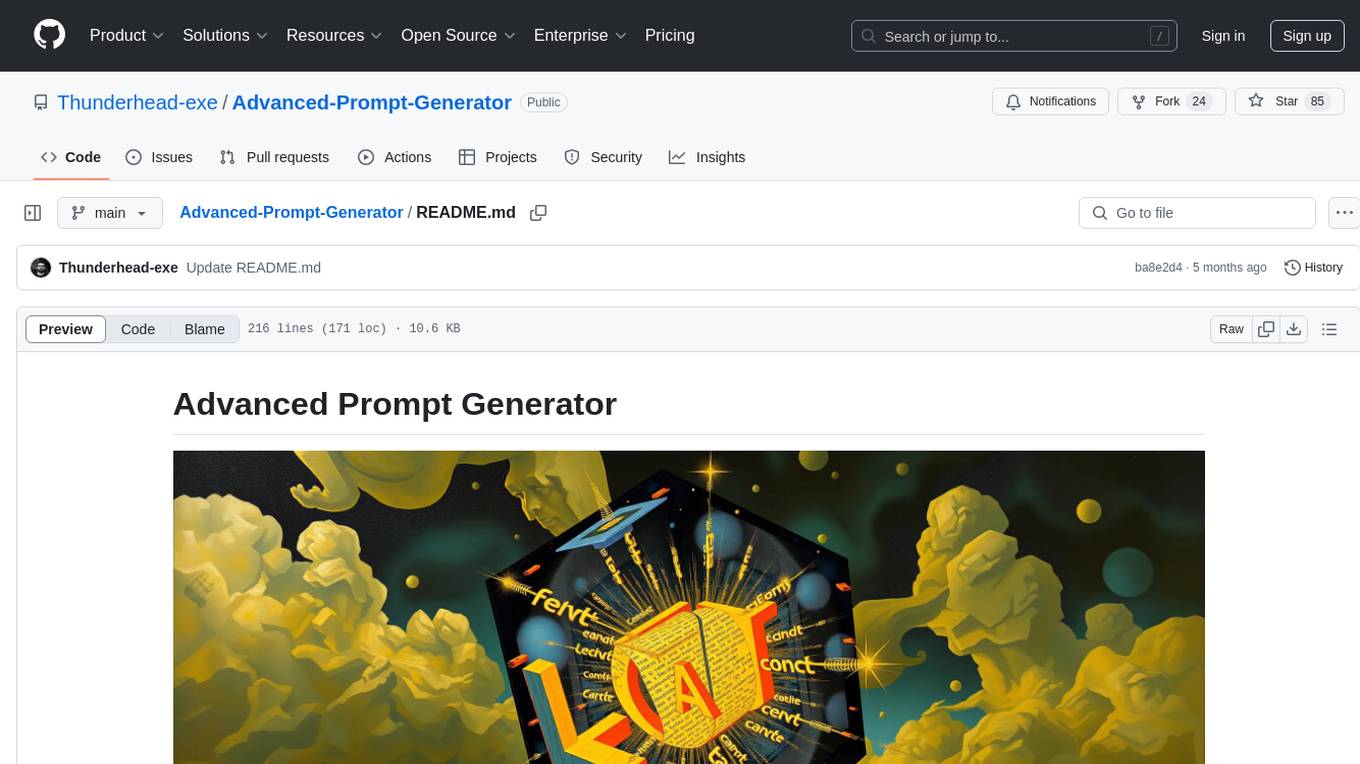
Advanced-Prompt-Generator
This project is an LLM-based Advanced Prompt Generator designed to automate the process of prompt engineering by enhancing given input prompts using large language models (LLMs). The tool can generate advanced prompts with minimal user input, leveraging LLM agents for optimized prompt generation. It supports gpt-4o or gpt-4o-mini, offers FastAPI & Docker deployment for efficiency, provides a Gradio interface for easy testing, and is hosted on Hugging Face Spaces for quick demos. Users can expand model support to offer more variety and flexibility.
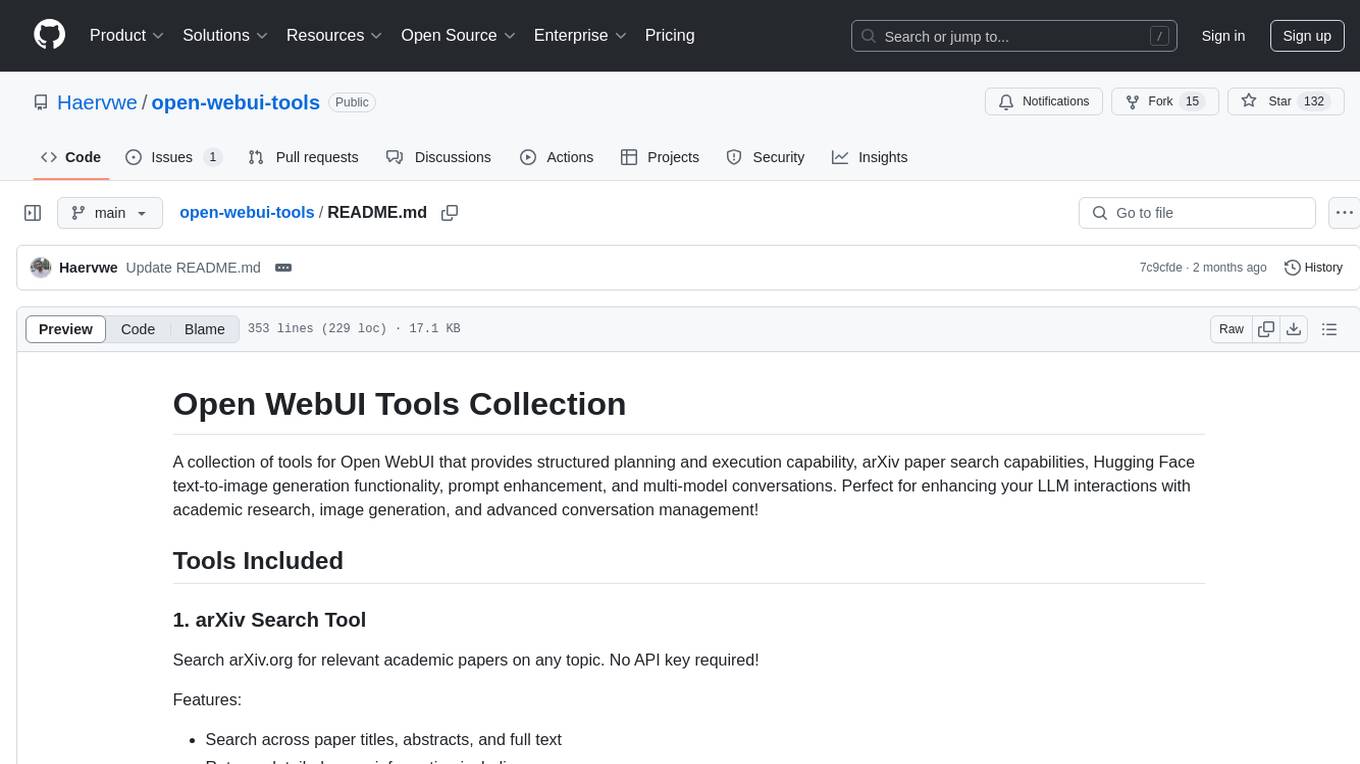
open-webui-tools
Open WebUI Tools Collection is a set of tools for structured planning, arXiv paper search, Hugging Face text-to-image generation, prompt enhancement, and multi-model conversations. It enhances LLM interactions with academic research, image generation, and conversation management. Tools include arXiv Search Tool and Hugging Face Image Generator. Function Pipes like Planner Agent offer autonomous plan generation and execution. Filters like Prompt Enhancer improve prompt quality. Installation and configuration instructions are provided for each tool and pipe.
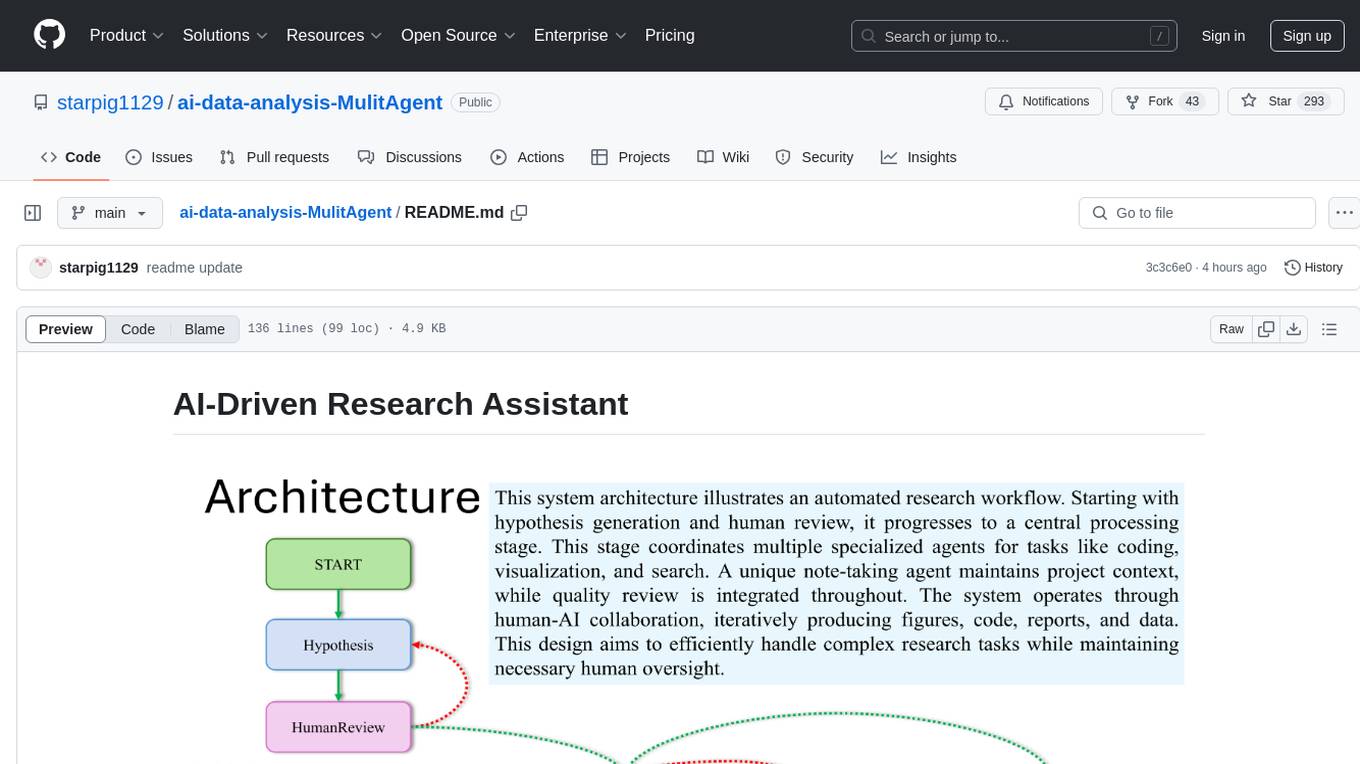
ai-data-analysis-MulitAgent
AI-Driven Research Assistant is an advanced AI-powered system utilizing specialized agents for data analysis, visualization, and report generation. It integrates LangChain, OpenAI's GPT models, and LangGraph for complex research processes. Key features include hypothesis generation, data processing, web search, code generation, and report writing. The system's unique Note Taker agent maintains project state, reducing overhead and improving context retention. System requirements include Python 3.10+ and Jupyter Notebook environment. Installation involves cloning the repository, setting up a Conda virtual environment, installing dependencies, and configuring environment variables. Usage instructions include setting data, running Jupyter Notebook, customizing research tasks, and viewing results. Main components include agents for hypothesis generation, process supervision, visualization, code writing, search, report writing, quality review, and note-taking. Workflow involves hypothesis generation, processing, quality review, and revision. Customization is possible by modifying agent creation and workflow definition. Current issues include OpenAI errors, NoteTaker efficiency, runtime optimization, and refiner improvement. Contributions via pull requests are welcome under the MIT License.
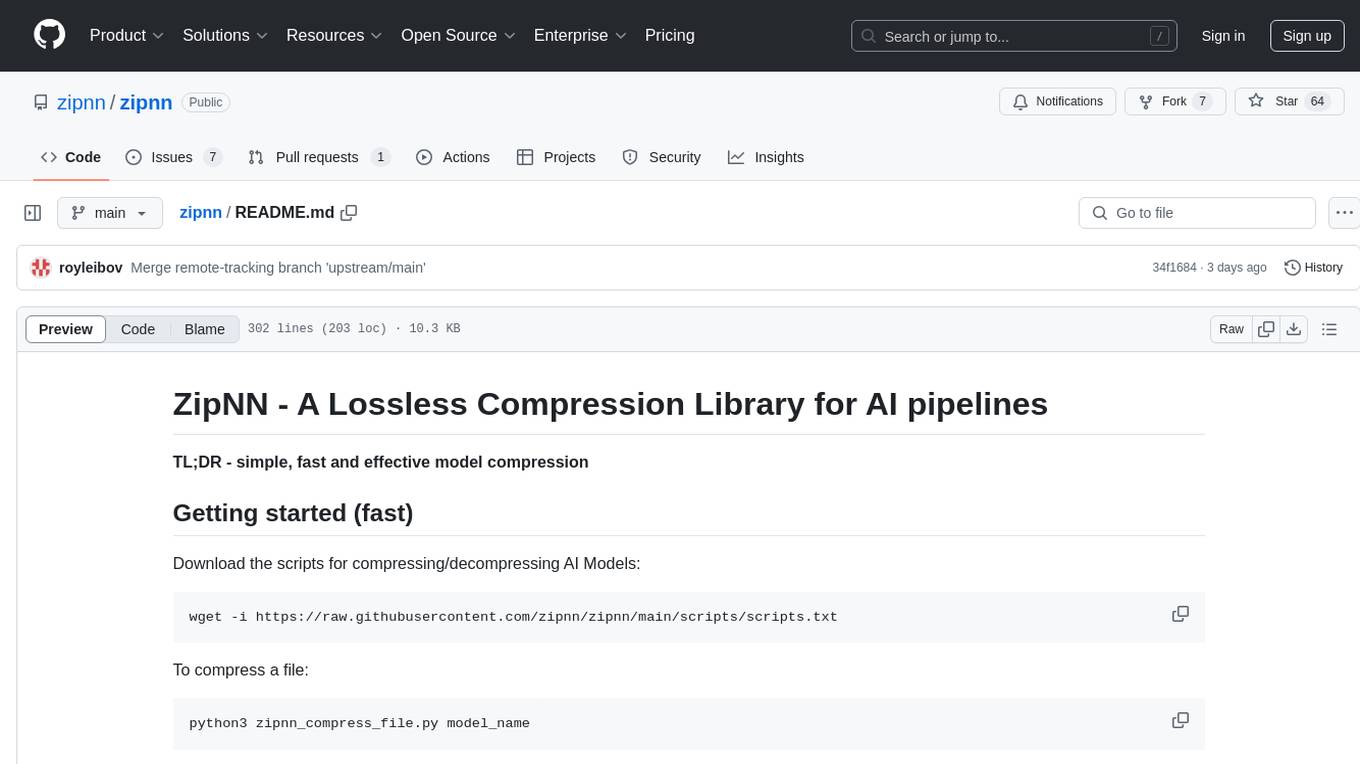
zipnn
ZipNN is a lossless and near-lossless compression library optimized for numbers/tensors in the Foundation Models environment. It automatically prepares data for compression based on its type, allowing users to focus on core tasks without worrying about compression complexities. The library delivers effective compression techniques for different data types and structures, achieving high compression ratios and rates. ZipNN supports various compression methods like ZSTD, lz4, and snappy, and provides ready-made scripts for file compression/decompression. Users can also manually import the package to compress and decompress data. The library offers advanced configuration options for customization and validation tests for different input and compression types.
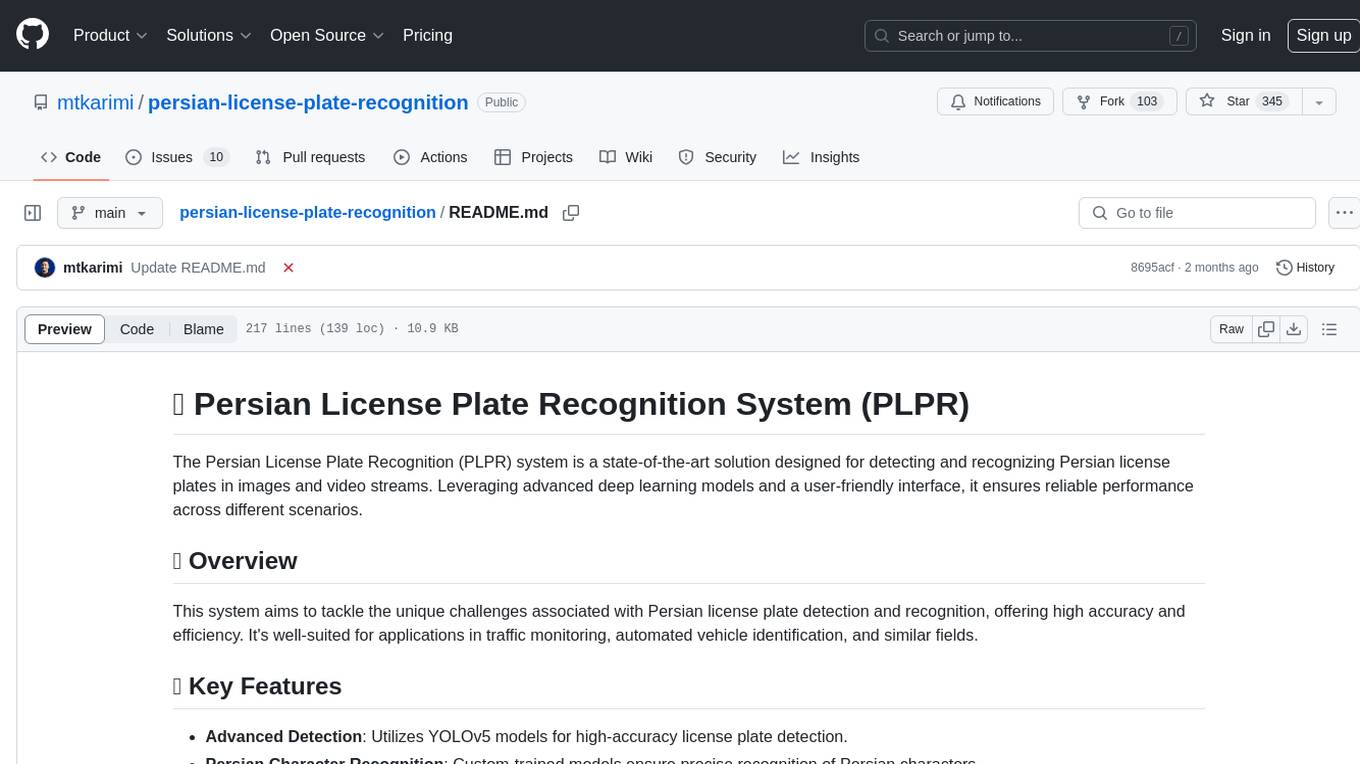
persian-license-plate-recognition
The Persian License Plate Recognition (PLPR) system is a state-of-the-art solution designed for detecting and recognizing Persian license plates in images and video streams. Leveraging advanced deep learning models and a user-friendly interface, it ensures reliable performance across different scenarios. The system offers advanced detection using YOLOv5 models, precise recognition of Persian characters, real-time processing capabilities, and a user-friendly GUI. It is well-suited for applications in traffic monitoring, automated vehicle identification, and similar fields. The system's architecture includes modules for resident management, entrance management, and a detailed flowchart explaining the process from system initialization to displaying results in the GUI. Hardware requirements include an Intel Core i5 processor, 8 GB RAM, a dedicated GPU with at least 4 GB VRAM, and an SSD with 20 GB of free space. The system can be installed by cloning the repository and installing required Python packages. Users can customize the video source for processing and run the application to upload and process images or video streams. The system's GUI allows for parameter adjustments to optimize performance, and the Wiki provides in-depth information on the system's architecture and model training.
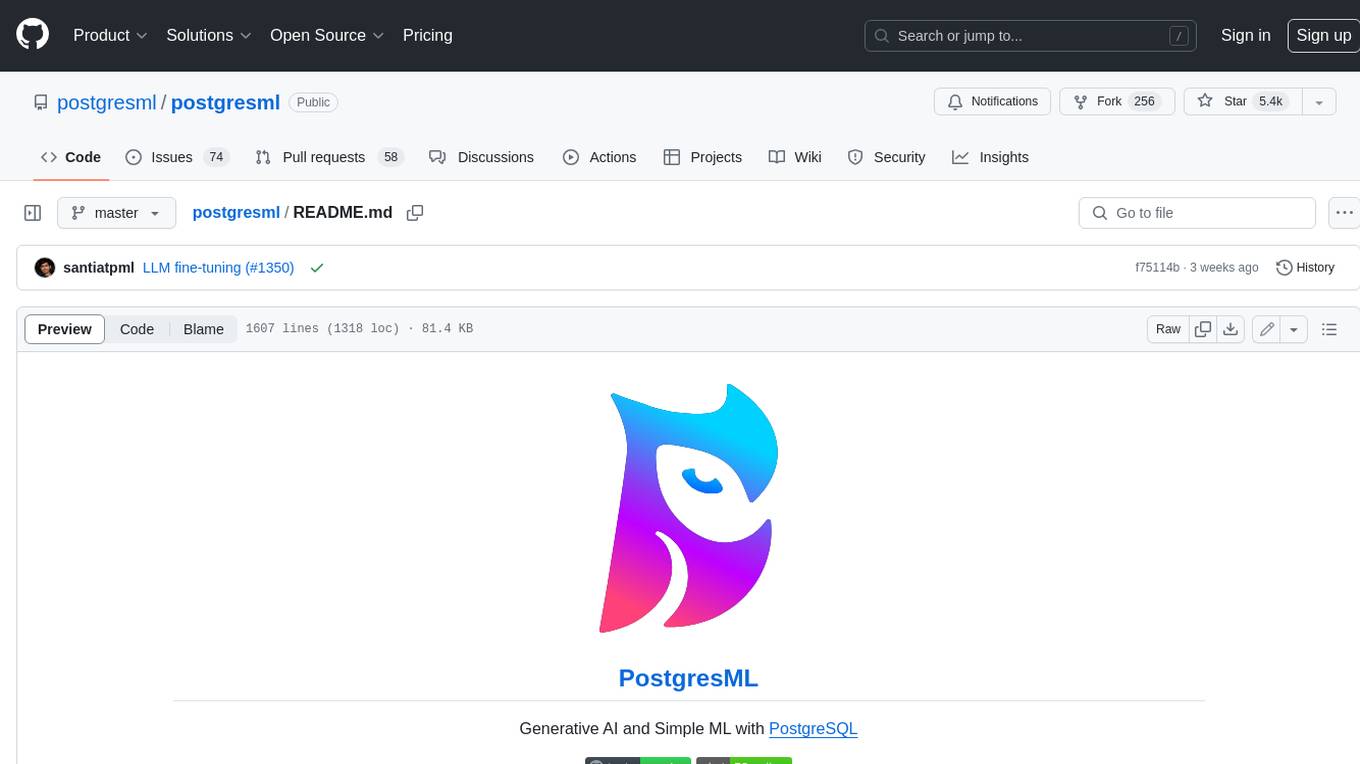
postgresml
PostgresML is a powerful Postgres extension that seamlessly combines data storage and machine learning inference within your database. It enables running machine learning and AI operations directly within PostgreSQL, leveraging GPU acceleration for faster computations, integrating state-of-the-art large language models, providing built-in functions for text processing, enabling efficient similarity search, offering diverse ML algorithms, ensuring high performance, scalability, and security, supporting a wide range of NLP tasks, and seamlessly integrating with existing PostgreSQL tools and client libraries.
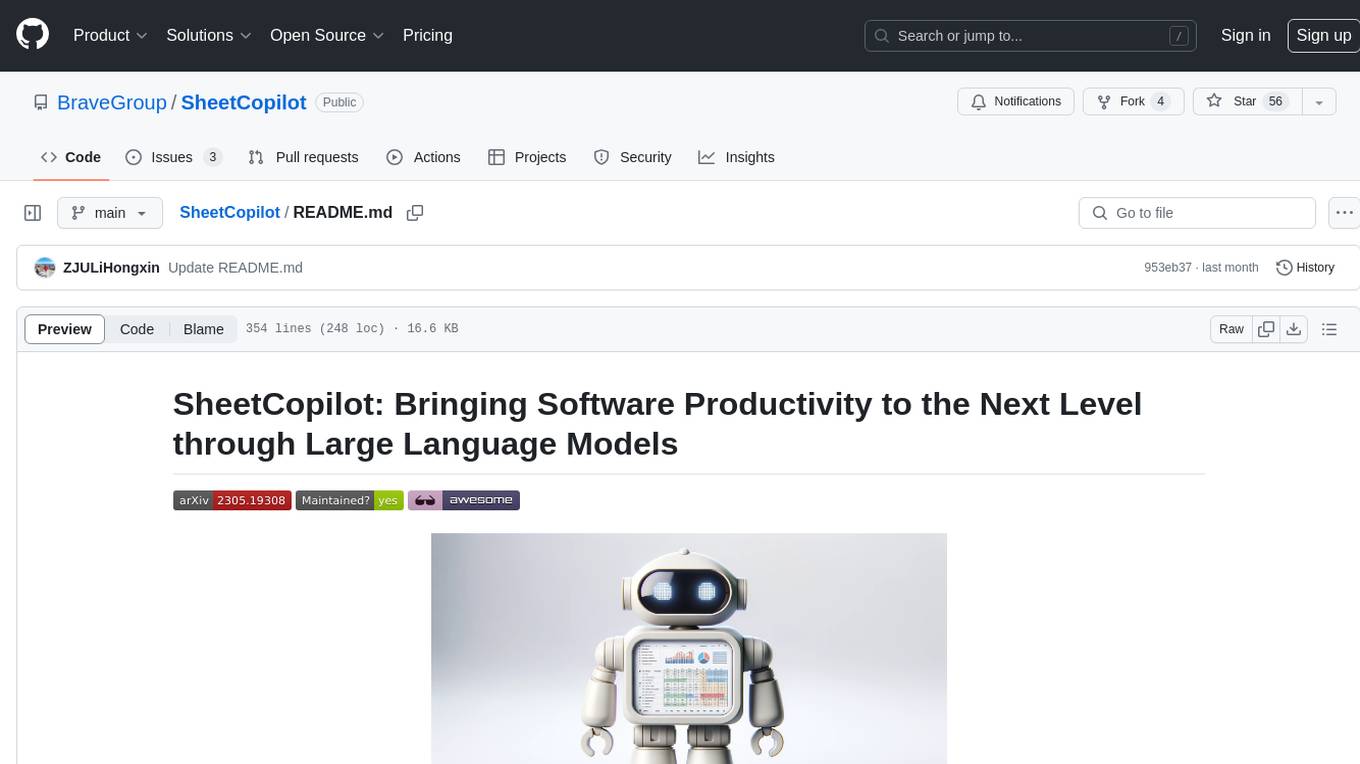
SheetCopilot
SheetCopilot is an assistant agent that manipulates spreadsheets by following user commands. It leverages Large Language Models (LLMs) to interact with spreadsheets like a human expert, enabling non-expert users to complete tasks on complex software such as Google Sheets and Excel via a language interface. The tool observes spreadsheet states, polishes generated solutions based on external action documents and error feedback, and aims to improve success rate and efficiency. SheetCopilot offers a dataset with diverse task categories and operations, supporting operations like entry & manipulation, management, formatting, charts, and pivot tables. Users can interact with SheetCopilot in Excel or Google Sheets, executing tasks like calculating revenue, creating pivot tables, and plotting charts. The tool's evaluation includes performance comparisons with leading LLMs and VBA-based methods on specific datasets, showcasing its capabilities in controlling various aspects of a spreadsheet.
For similar tasks
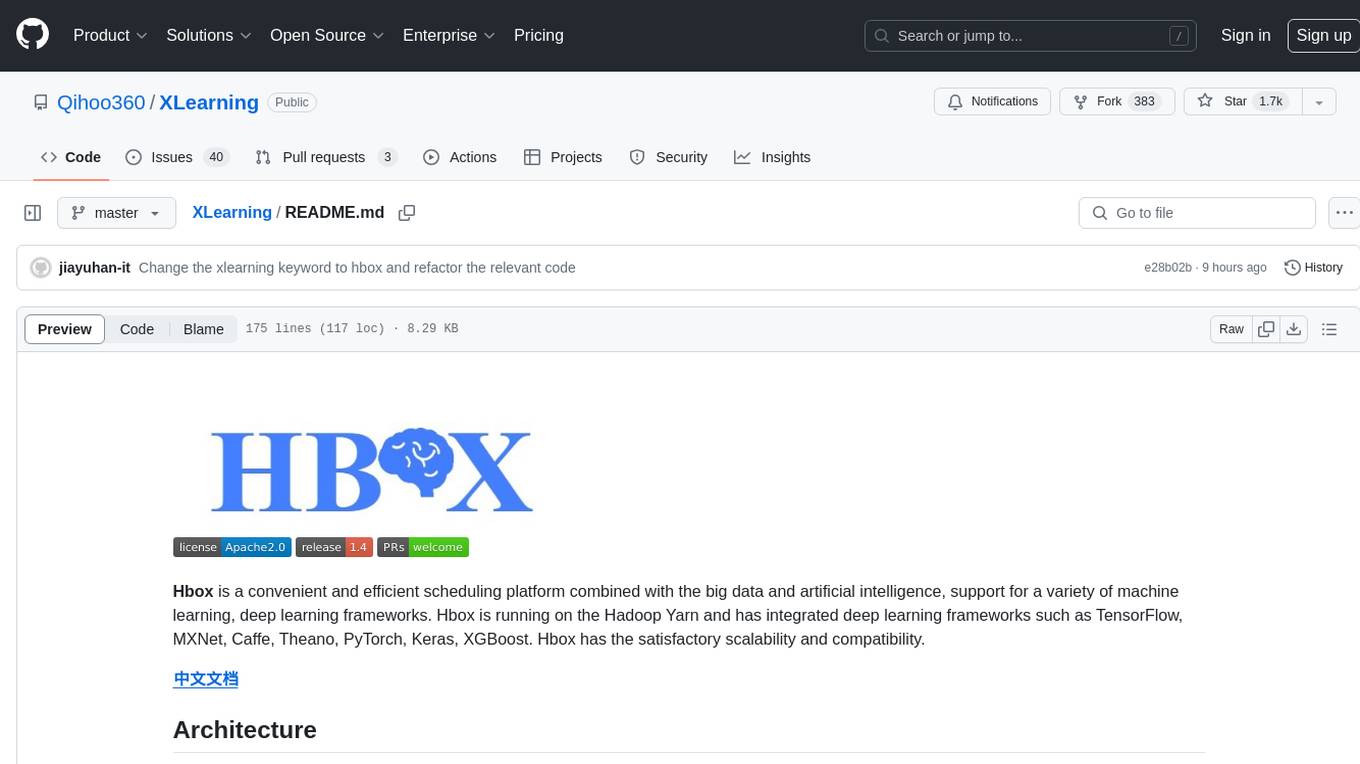
XLearning
XLearning is a scheduling platform for big data and artificial intelligence, supporting various machine learning and deep learning frameworks. It runs on Hadoop Yarn and integrates frameworks like TensorFlow, MXNet, Caffe, Theano, PyTorch, Keras, XGBoost. XLearning offers scalability, compatibility, multiple deep learning framework support, unified data management based on HDFS, visualization display, and compatibility with code at native frameworks. It provides functions for data input/output strategies, container management, TensorBoard service, and resource usage metrics display. XLearning requires JDK >= 1.7 and Maven >= 3.3 for compilation, and deployment on CentOS 7.2 with Java >= 1.7 and Hadoop 2.6, 2.7, 2.8.
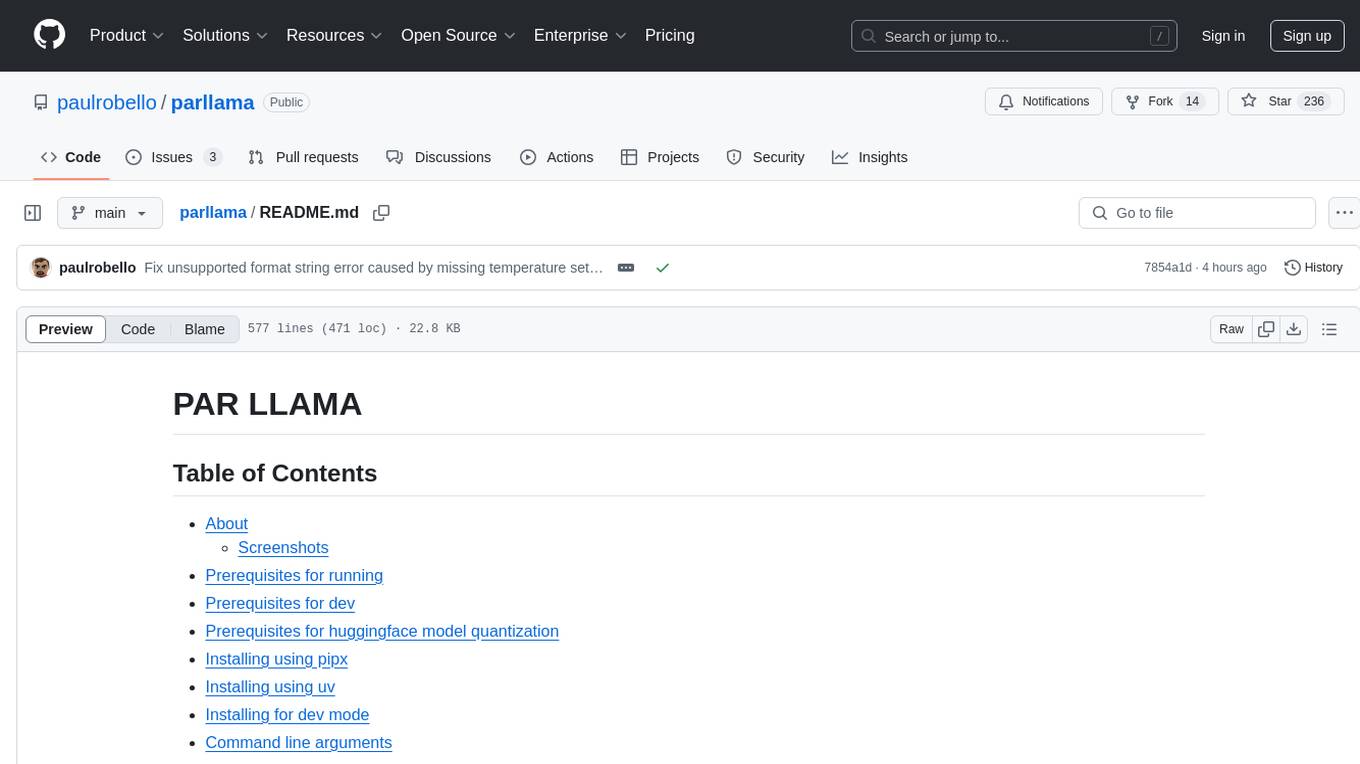
parllama
PAR LLAMA is a Text UI application for managing and using LLMs, designed with Textual and Rich and PAR AI Core. It runs on major OS's including Windows, Windows WSL, Mac, and Linux. Supports Dark and Light mode, custom themes, and various workflows like Ollama chat, image chat, and OpenAI provider chat. Offers features like custom prompts, themes, environment variables configuration, and remote instance connection. Suitable for managing and using LLMs efficiently.
For similar jobs
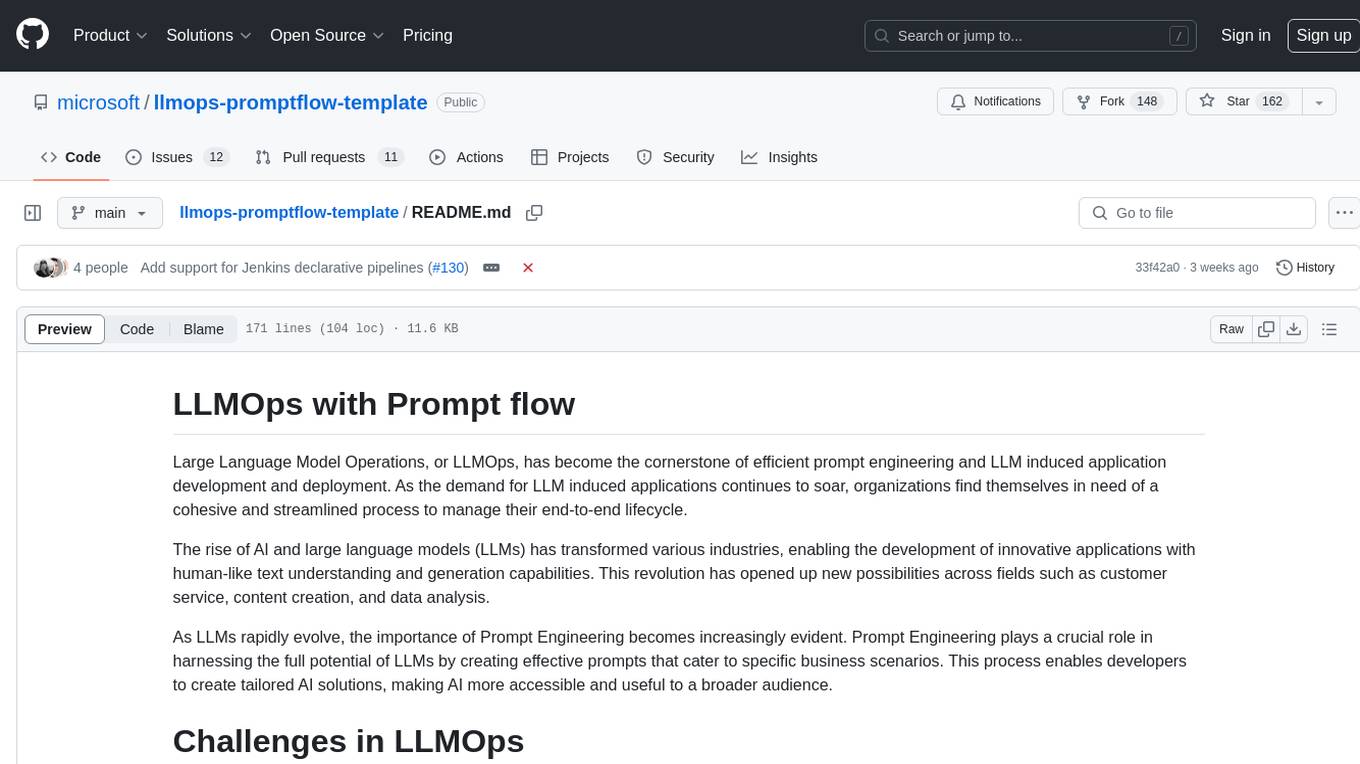
llmops-promptflow-template
LLMOps with Prompt flow is a template and guidance for building LLM-infused apps using Prompt flow. It provides centralized code hosting, lifecycle management, variant and hyperparameter experimentation, A/B deployment, many-to-many dataset/flow relationships, multiple deployment targets, comprehensive reporting, BYOF capabilities, configuration-based development, local prompt experimentation and evaluation, endpoint testing, and optional Human-in-loop validation. The tool is customizable to suit various application needs.
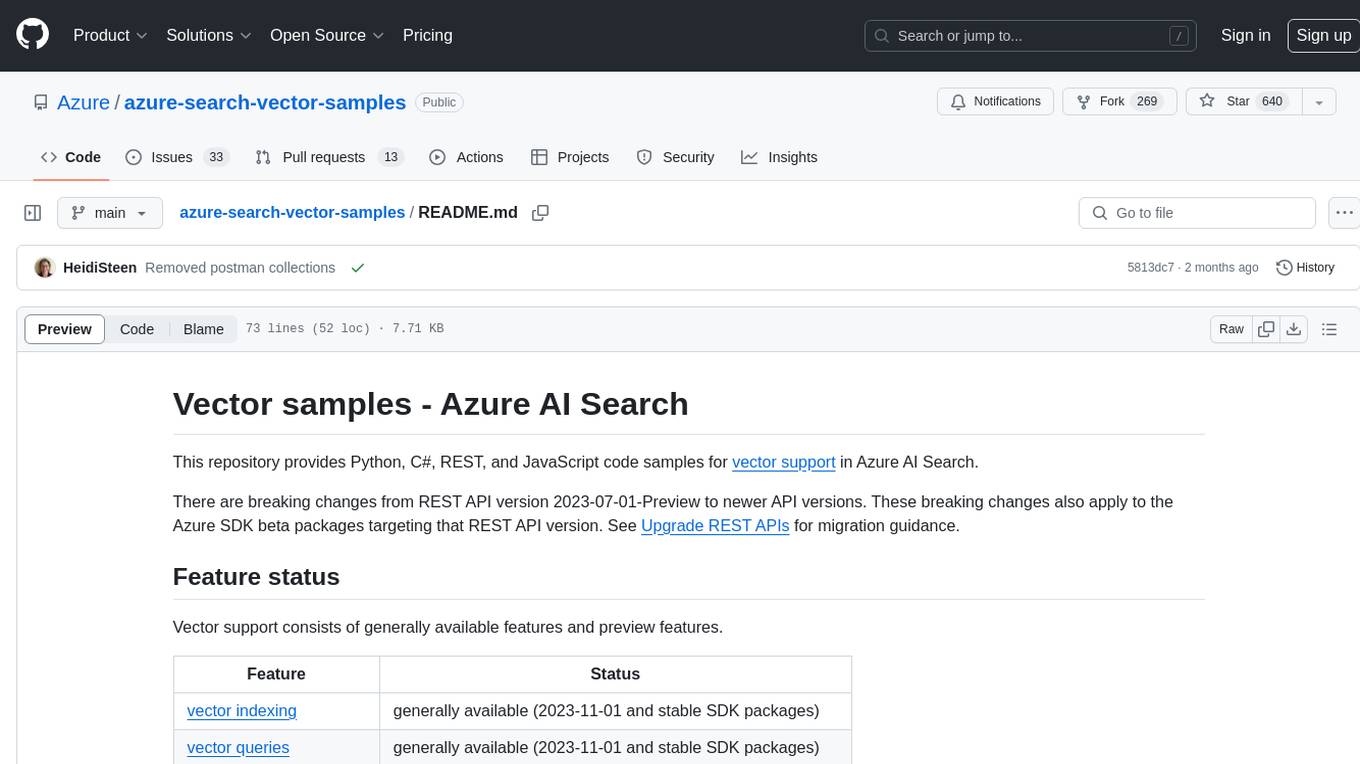
azure-search-vector-samples
This repository provides code samples in Python, C#, REST, and JavaScript for vector support in Azure AI Search. It includes demos for various languages showcasing vectorization of data, creating indexes, and querying vector data. Additionally, it offers tools like Azure AI Search Lab for experimenting with AI-enabled search scenarios in Azure and templates for deploying custom chat-with-your-data solutions. The repository also features documentation on vector search, hybrid search, creating and querying vector indexes, and REST API references for Azure AI Search and Azure OpenAI Service.
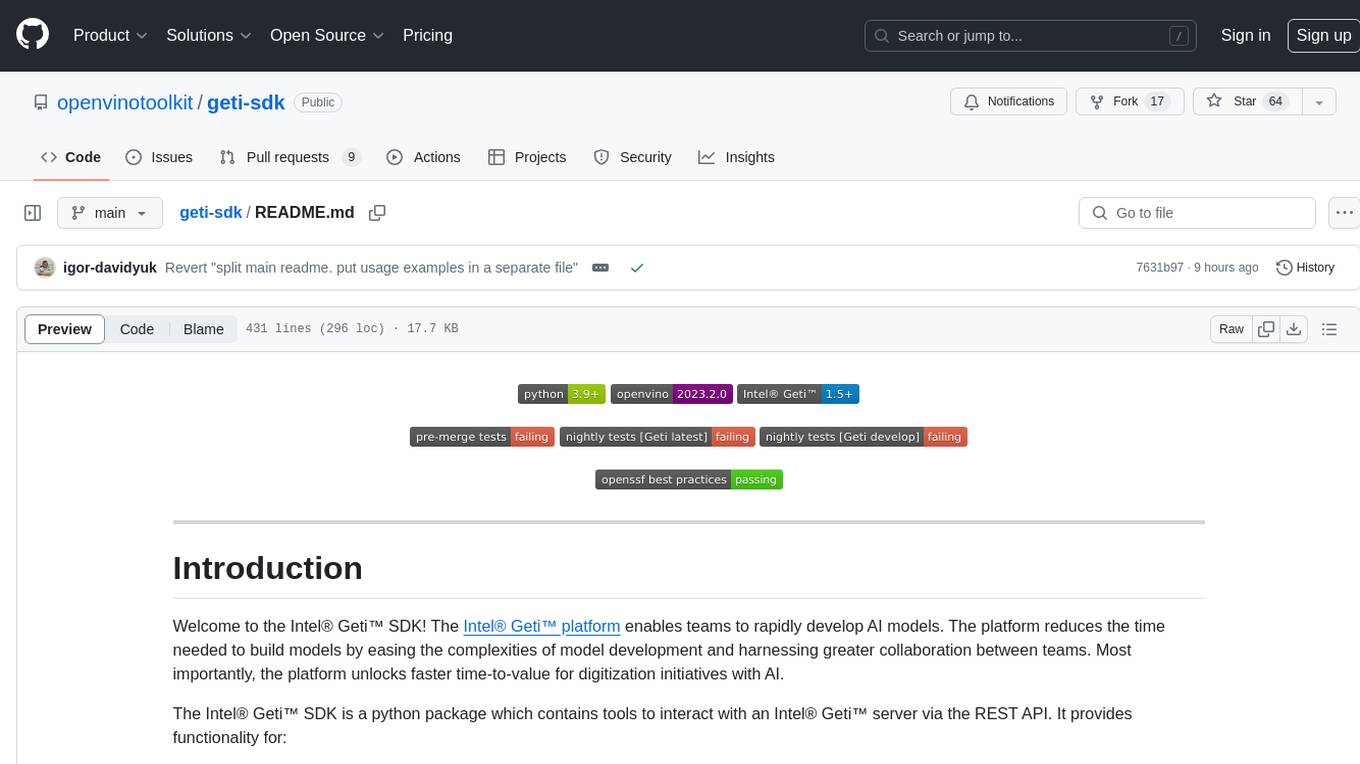
geti-sdk
The Intel® Geti™ SDK is a python package that enables teams to rapidly develop AI models by easing the complexities of model development and enhancing collaboration between teams. It provides tools to interact with an Intel® Geti™ server via the REST API, allowing for project creation, downloading, uploading, deploying for local inference with OpenVINO, setting project and model configuration, launching and monitoring training jobs, and media upload and prediction. The SDK also includes tutorial-style Jupyter notebooks demonstrating its usage.
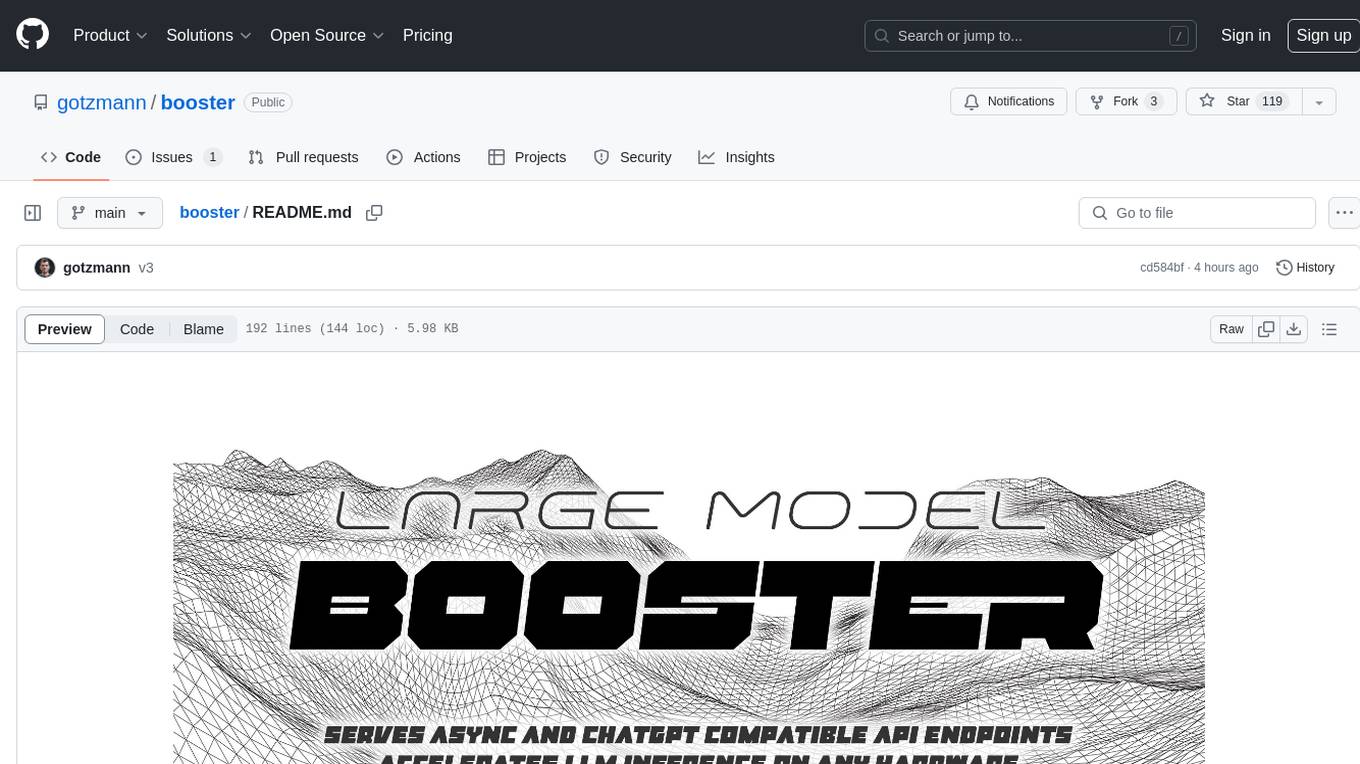
booster
Booster is a powerful inference accelerator designed for scaling large language models within production environments or for experimental purposes. It is built with performance and scaling in mind, supporting various CPUs and GPUs, including Nvidia CUDA, Apple Metal, and OpenCL cards. The tool can split large models across multiple GPUs, offering fast inference on machines with beefy GPUs. It supports both regular FP16/FP32 models and quantised versions, along with popular LLM architectures. Additionally, Booster features proprietary Janus Sampling for code generation and non-English languages.
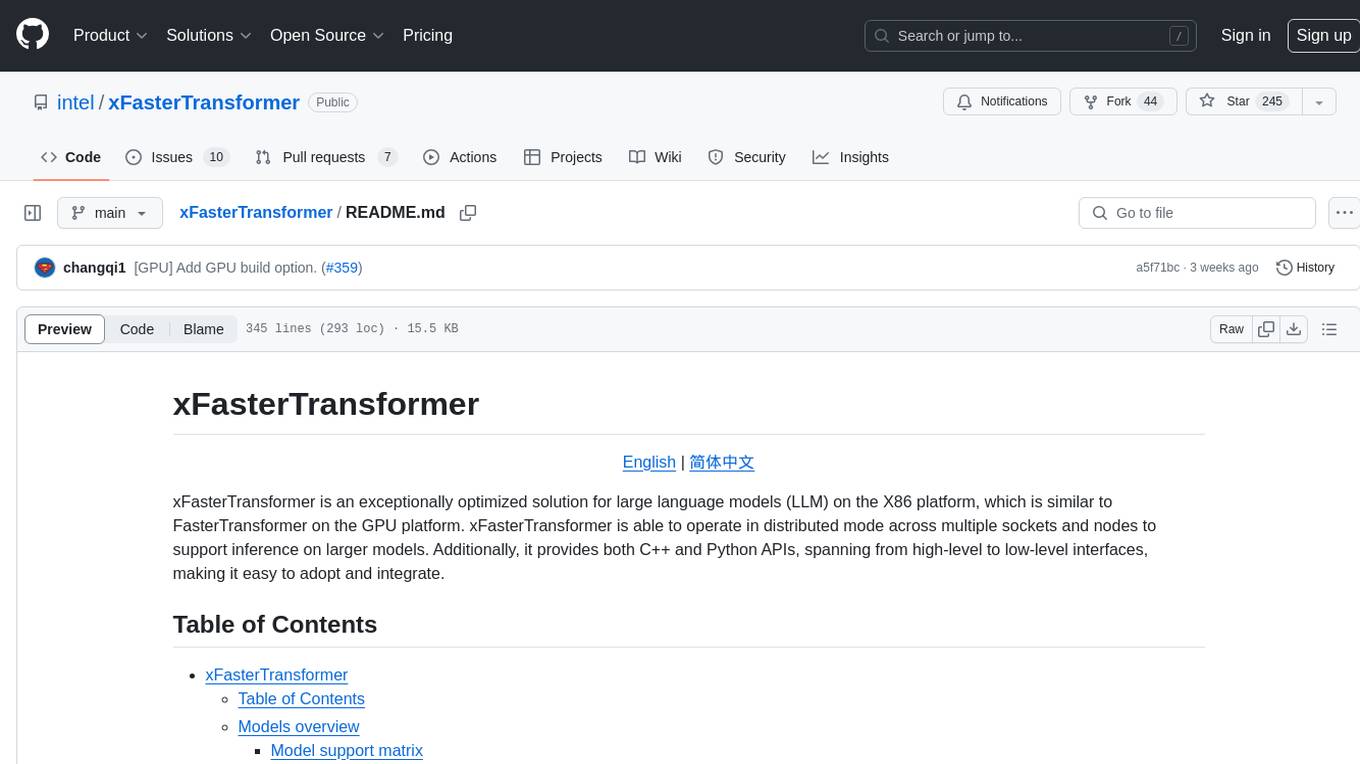
xFasterTransformer
xFasterTransformer is an optimized solution for Large Language Models (LLMs) on the X86 platform, providing high performance and scalability for inference on mainstream LLM models. It offers C++ and Python APIs for easy integration, along with example codes and benchmark scripts. Users can prepare models in a different format, convert them, and use the APIs for tasks like encoding input prompts, generating token ids, and serving inference requests. The tool supports various data types and models, and can run in single or multi-rank modes using MPI. A web demo based on Gradio is available for popular LLM models like ChatGLM and Llama2. Benchmark scripts help evaluate model inference performance quickly, and MLServer enables serving with REST and gRPC interfaces.
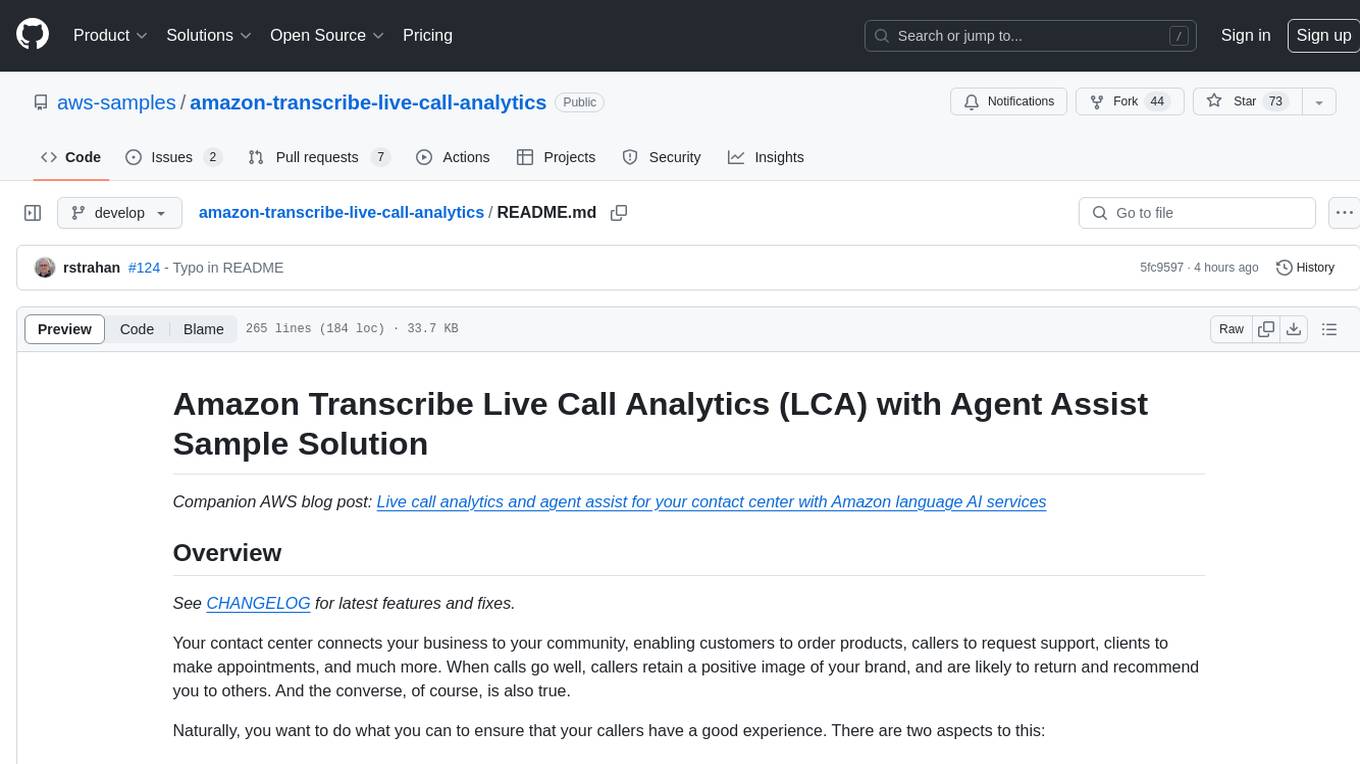
amazon-transcribe-live-call-analytics
The Amazon Transcribe Live Call Analytics (LCA) with Agent Assist Sample Solution is designed to help contact centers assess and optimize caller experiences in real time. It leverages Amazon machine learning services like Amazon Transcribe, Amazon Comprehend, and Amazon SageMaker to transcribe and extract insights from contact center audio. The solution provides real-time supervisor and agent assist features, integrates with existing contact centers, and offers a scalable, cost-effective approach to improve customer interactions. The end-to-end architecture includes features like live call transcription, call summarization, AI-powered agent assistance, and real-time analytics. The solution is event-driven, ensuring low latency and seamless processing flow from ingested speech to live webpage updates.
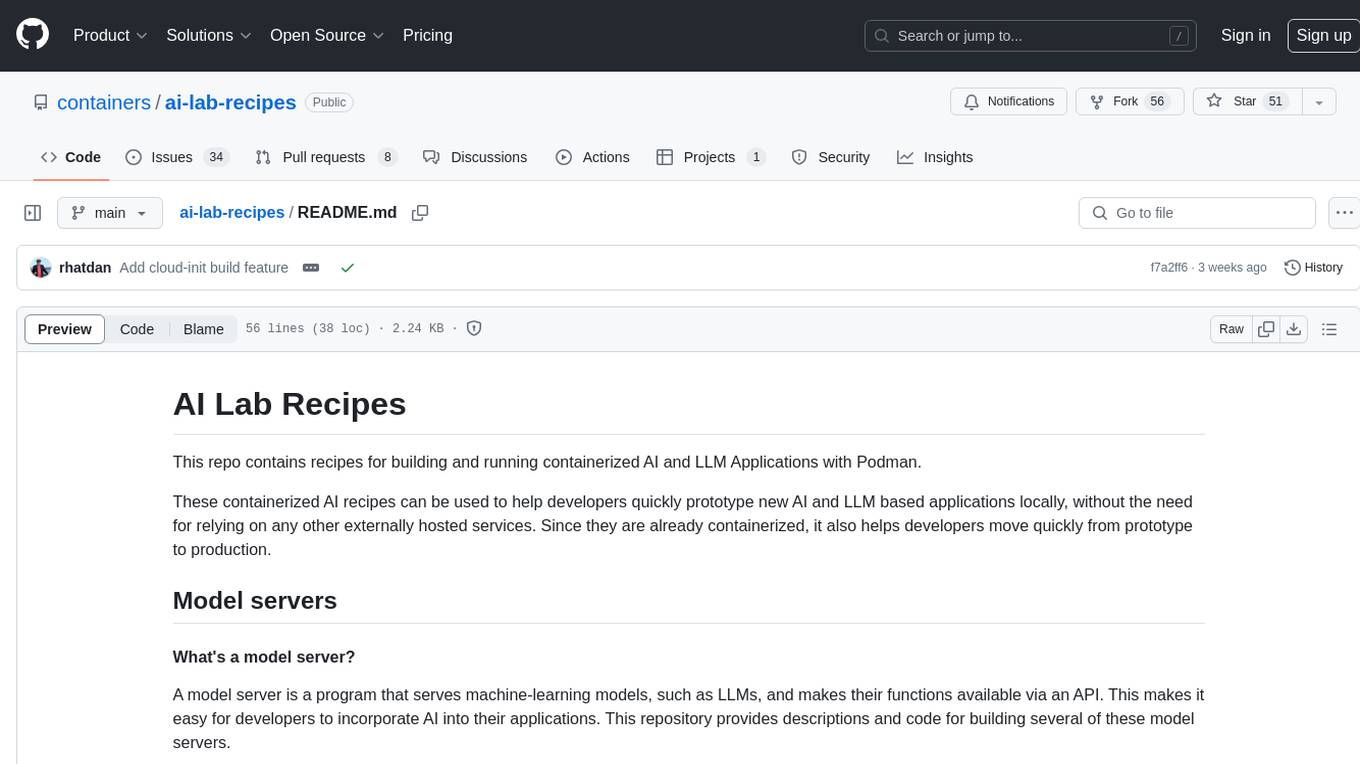
ai-lab-recipes
This repository contains recipes for building and running containerized AI and LLM applications with Podman. It provides model servers that serve machine-learning models via an API, allowing developers to quickly prototype new AI applications locally. The recipes include components like model servers and AI applications for tasks such as chat, summarization, object detection, etc. Images for sample applications and models are available in `quay.io`, and bootable containers for AI training on Linux OS are enabled.
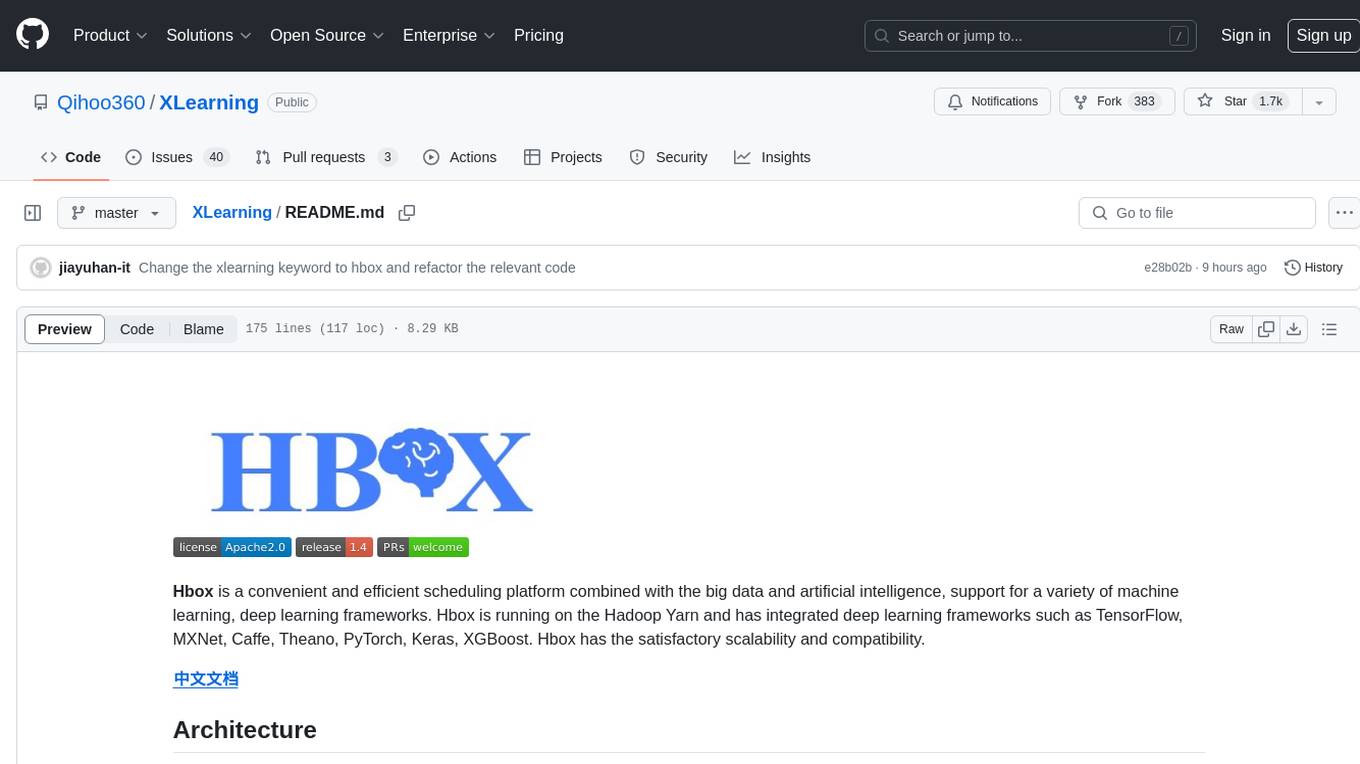
XLearning
XLearning is a scheduling platform for big data and artificial intelligence, supporting various machine learning and deep learning frameworks. It runs on Hadoop Yarn and integrates frameworks like TensorFlow, MXNet, Caffe, Theano, PyTorch, Keras, XGBoost. XLearning offers scalability, compatibility, multiple deep learning framework support, unified data management based on HDFS, visualization display, and compatibility with code at native frameworks. It provides functions for data input/output strategies, container management, TensorBoard service, and resource usage metrics display. XLearning requires JDK >= 1.7 and Maven >= 3.3 for compilation, and deployment on CentOS 7.2 with Java >= 1.7 and Hadoop 2.6, 2.7, 2.8.