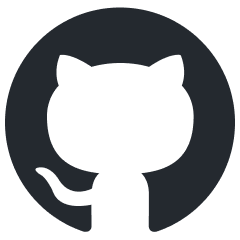
postgresml
Postgres with GPUs for ML/AI apps.
Stars: 6101
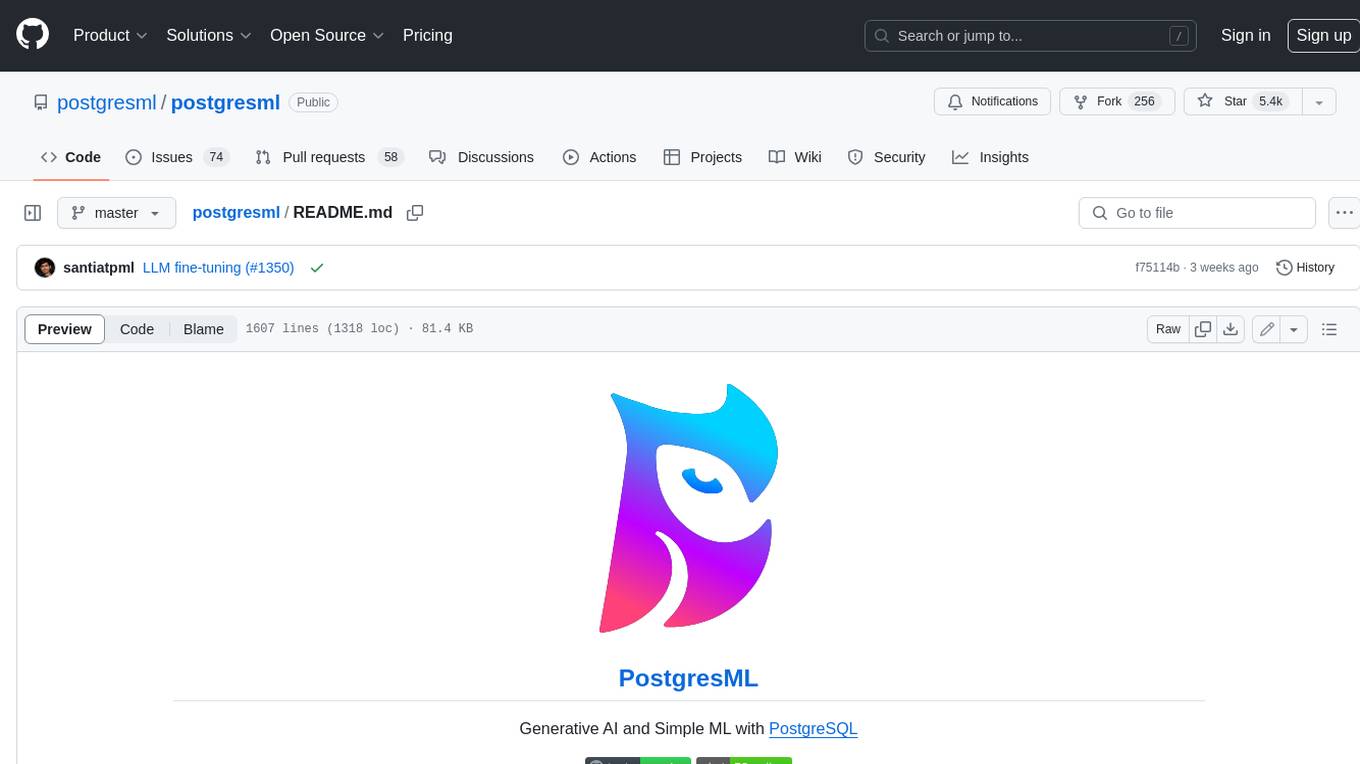
PostgresML is a powerful Postgres extension that seamlessly combines data storage and machine learning inference within your database. It enables running machine learning and AI operations directly within PostgreSQL, leveraging GPU acceleration for faster computations, integrating state-of-the-art large language models, providing built-in functions for text processing, enabling efficient similarity search, offering diverse ML algorithms, ensuring high performance, scalability, and security, supporting a wide range of NLP tasks, and seamlessly integrating with existing PostgreSQL tools and client libraries.
README:
Postgres + GPUs for ML/AI applications.
| Documentation | Blog | Discord |
Why do ML/AI in Postgres?
Data for ML & AI systems is inherently larger and more dynamic than the models. It's more efficient, manageable and reliable to move models to the database, rather than constantly moving data to the models.
- In-Database ML/AI: Run machine learning and AI operations directly within PostgreSQL
- GPU Acceleration: Leverage GPU power for faster computations and model inference
- Large Language Models: Integrate and use state-of-the-art LLMs from Hugging Face
- RAG Pipeline: Built-in functions for chunking, embedding, ranking, and transforming text
- Vector Search: Efficient similarity search using pgvector integration
- Diverse ML Algorithms: 47+ classification and regression algorithms available
- High Performance: 8-40X faster inference compared to HTTP-based model serving
- Scalability: Support for millions of transactions per second and horizontal scaling
- NLP Tasks: Wide range of natural language processing capabilities
- Security: Enhanced data privacy by keeping models and data together
- Seamless Integration: Works with existing PostgreSQL tools and client libraries
The only prerequisites for using PostgresML is a Postgres database with our open-source pgml
extension installed.
Our serverless cloud is the easiest and recommend way to get started.
Sign up for a free PostgresML account. You'll get a free database in seconds, with access to GPUs and state of the art LLMs.
If you don't want to use our cloud you can self host it.
docker run \
-it \
-v postgresml_data:/var/lib/postgresql \
-p 5433:5432 \
-p 8000:8000 \
ghcr.io/postgresml/postgresml:2.7.12 \
sudo -u postgresml psql -d postgresml
For more details, take a look at our Quick Start with Docker documentation.
We have a number of other tools and libraries that are specifically designed to work with PostgreML. Remeber PostgresML is a postgres extension running inside of Postgres so you can connect with psql
and use any of your favorite tooling and client libraries like psycopg to connect and run queries.
PostgresML Specific Client Libraries:
- Korvus - Korvus is a Python, JavaScript, Rust and C search SDK that unifies the entire RAG pipeline in a single database query.
- postgresml-django - postgresml-django is a Python module that integrates PostgresML with Django ORM.
Recommended Postgres Poolers:
- pgcat - pgcat is a PostgreSQL pooler with sharding, load balancing and failover support.
PostgresML brings models directly to your data, eliminating the need for costly and time-consuming data transfers. This approach significantly enhances performance, security, and scalability for AI-driven applications.
By running models within the database, PostgresML enables:
- Reduced latency and improved query performance
- Enhanced data privacy and security
- Simplified infrastructure management
- Seamless integration with existing database operations
PostgresML supports a wide range of state-of-the-art deep learning architectures available on the Hugging Face model hub. This integration allows you to:
- Access thousands of pre-trained models
- Utilize cutting-edge NLP, computer vision, and other AI models
- Easily experiment with different architectures
While cloud-based LLM providers offer powerful capabilities, making API calls from within the database can introduce latency, security risks, and potential compliance issues. Currently, PostgresML does not directly support integration with remote LLM providers like OpenAI.
PostgresML transforms your PostgreSQL database into a powerful vector database for Retrieval-Augmented Generation (RAG) applications. It leverages pgvector for efficient storage and retrieval of embeddings.
Our RAG implementation is built on four key SQL functions:
- Chunk: Splits text into manageable segments
- Embed: Generates vector embeddings from text using pre-trained models
- Rank: Performs similarity search on embeddings
- Transform: Applies language models for text generation or transformation
For more information on using RAG with PostgresML see our guide on Unified RAG.
The pgml.chunk
function chunks documents using the specified splitter. This is typically done before embedding.
pgml.chunk(
splitter TEXT, -- splitter name
text TEXT, -- text to embed
kwargs JSON -- optional arguments (see below)
)
See pgml.chunk docs for more information.
The pgml.embed
function generates embeddings from text using in-database models.
pgml.embed(
transformer TEXT,
"text" TEXT,
kwargs JSONB
)
See pgml.embed docs for more information.
The pgml.rank
function uses Cross-Encoders to score sentence pairs.
This is typically used as a re-ranking step when performing search.
pgml.rank(
transformer TEXT,
query TEXT,
documents TEXT[],
kwargs JSONB
)
Docs coming soon.
The pgml.transform
function can be used to generate text.
SELECT pgml.transform(
task => TEXT OR JSONB, -- Pipeline initializer arguments
inputs => TEXT[] OR BYTEA[], -- inputs for inference
args => JSONB -- (optional) arguments to the pipeline.
)
See pgml.transform docs for more information.
See our Text Generation guide for a guide on generating text.
Some highlights:
- 47+ classification and regression algorithms
- 8 - 40X faster inference than HTTP based model serving
- Millions of transactions per second
- Horizontal scalability
Training a classification model
Training
SELECT * FROM pgml.train(
'Handwritten Digit Image Classifier',
algorithm => 'xgboost',
'classification',
'pgml.digits',
'target'
);
Inference
SELECT pgml.predict(
'My Classification Project',
ARRAY[0.1, 2.0, 5.0]
) AS prediction;
The pgml.transform
function exposes a number of available NLP tasks.
Available tasks are:
For Tasks:
Click tags to check more tools for each tasksFor Jobs:
Alternative AI tools for postgresml
Similar Open Source Tools
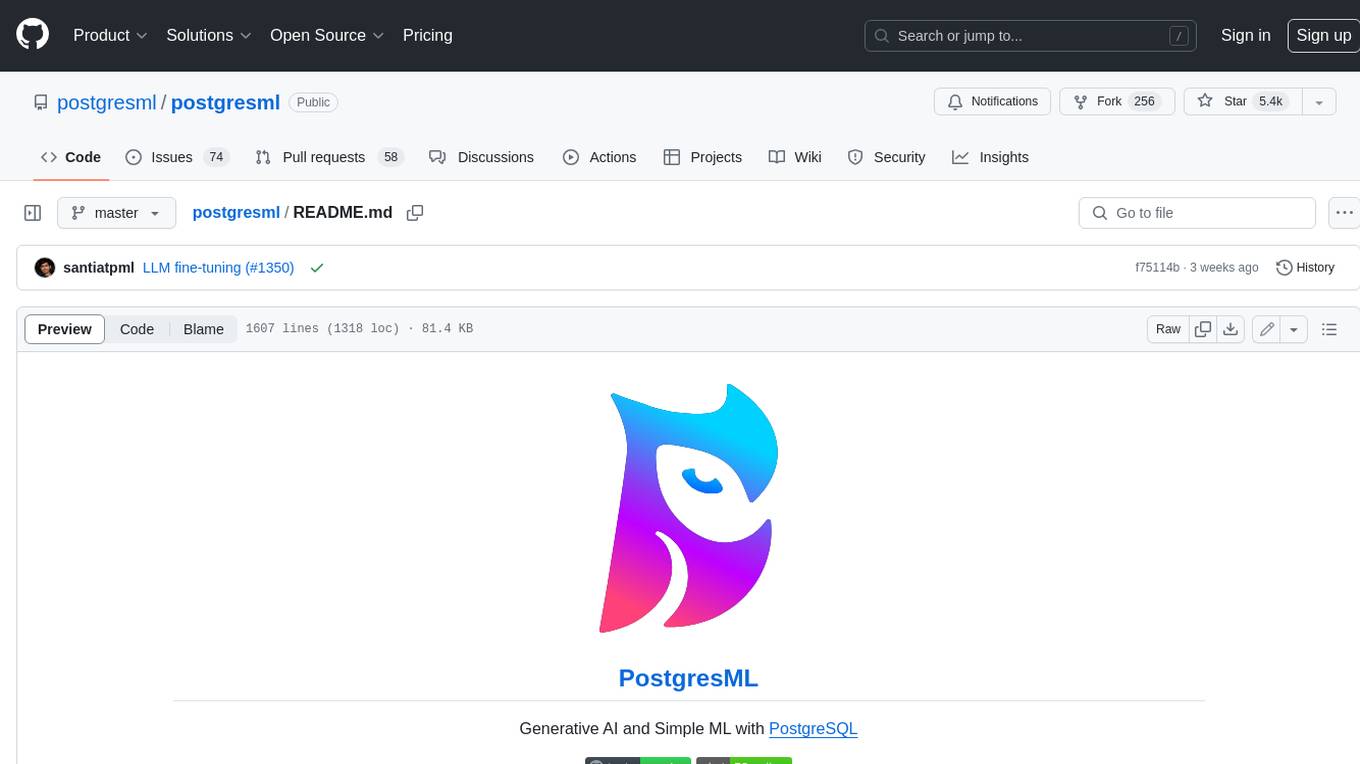
postgresml
PostgresML is a powerful Postgres extension that seamlessly combines data storage and machine learning inference within your database. It enables running machine learning and AI operations directly within PostgreSQL, leveraging GPU acceleration for faster computations, integrating state-of-the-art large language models, providing built-in functions for text processing, enabling efficient similarity search, offering diverse ML algorithms, ensuring high performance, scalability, and security, supporting a wide range of NLP tasks, and seamlessly integrating with existing PostgreSQL tools and client libraries.
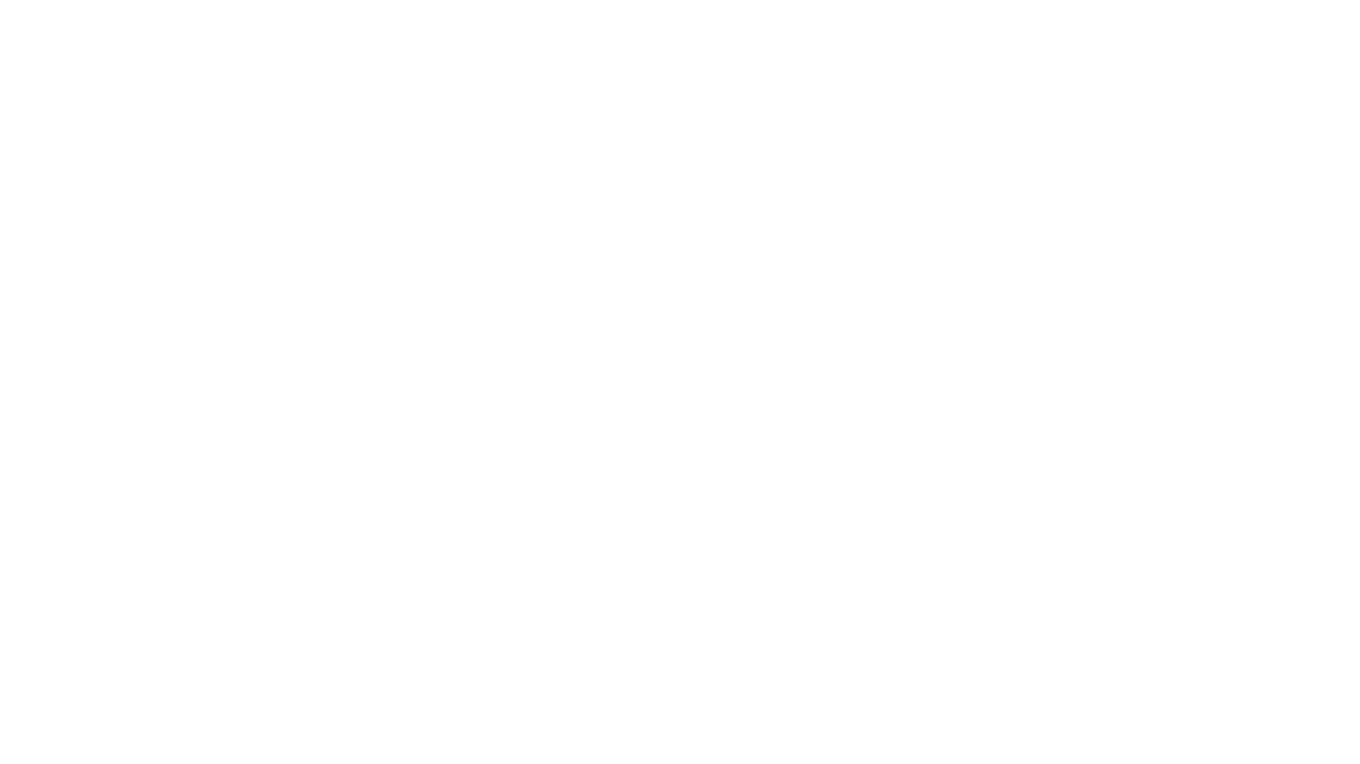
motia
Motia is an AI agent framework designed for software engineers to create, test, and deploy production-ready AI agents quickly. It provides a code-first approach, allowing developers to write agent logic in familiar languages and visualize execution in real-time. With Motia, developers can focus on business logic rather than infrastructure, offering zero infrastructure headaches, multi-language support, composable steps, built-in observability, instant APIs, and full control over AI logic. Ideal for building sophisticated agents and intelligent automations, Motia's event-driven architecture and modular steps enable the creation of GenAI-powered workflows, decision-making systems, and data processing pipelines.
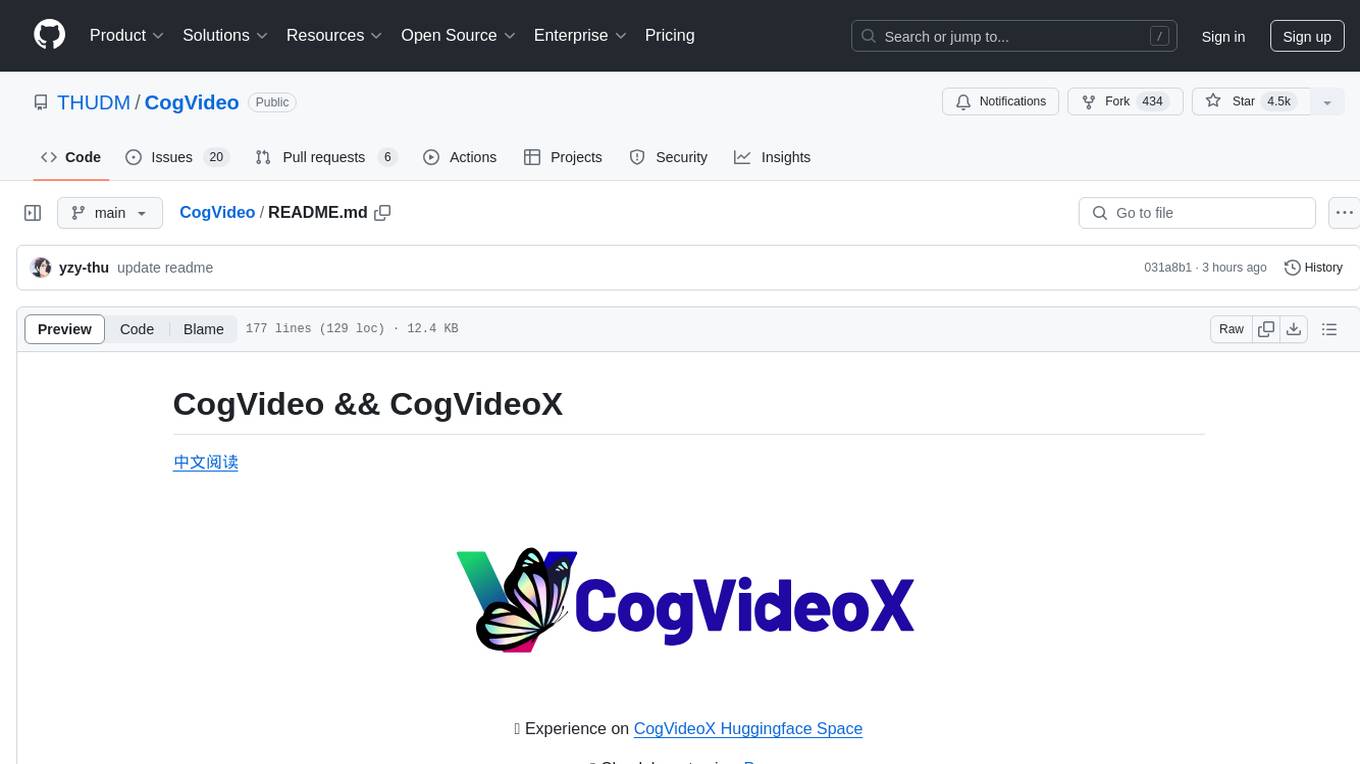
CogVideo
CogVideo is an open-source repository that provides pretrained text-to-video models for generating videos based on input text. It includes models like CogVideoX-2B and CogVideo, offering powerful video generation capabilities. The repository offers tools for inference, fine-tuning, and model conversion, along with demos showcasing the model's capabilities through CLI, web UI, and online experiences. CogVideo aims to facilitate the creation of high-quality videos from textual descriptions, catering to a wide range of applications.
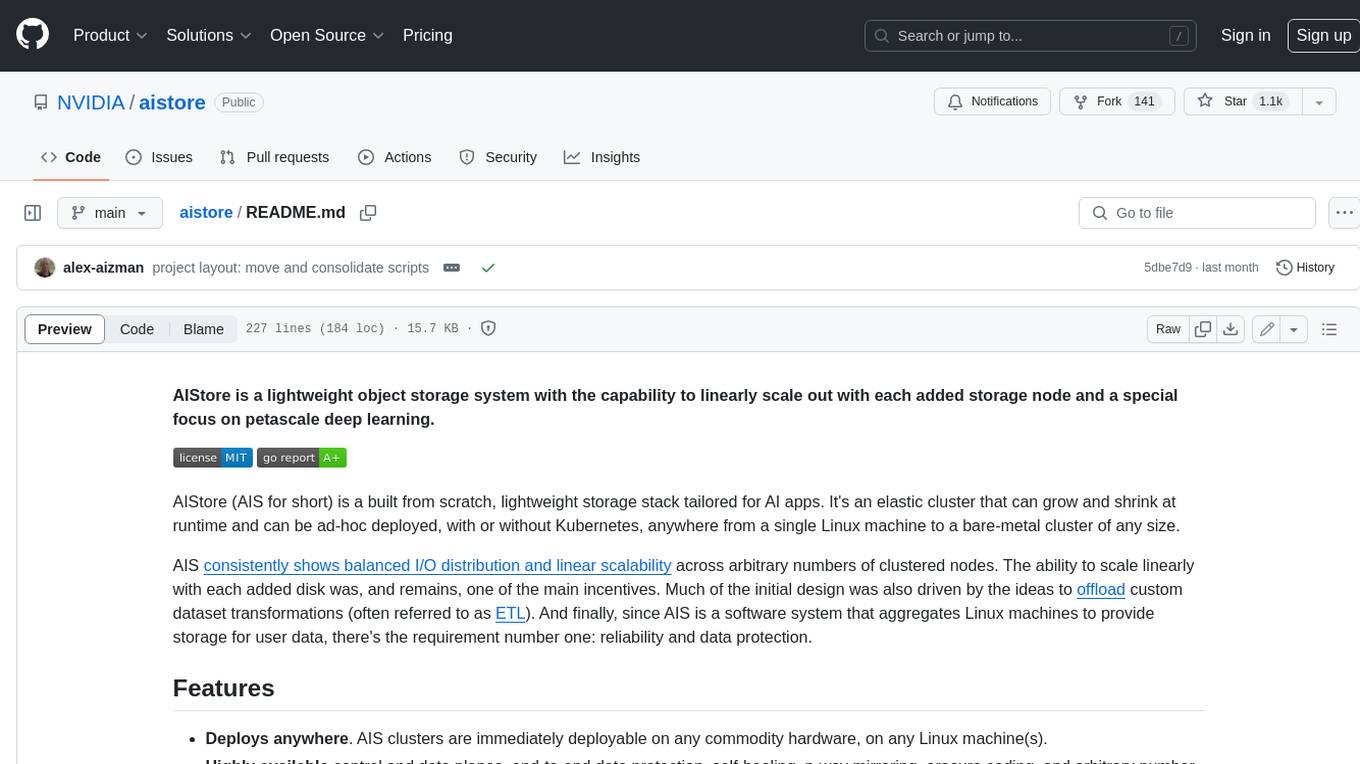
aistore
AIStore is a lightweight object storage system designed for AI applications. It is highly scalable, reliable, and easy to use. AIStore can be deployed on any commodity hardware, and it can be used to store and manage large datasets for deep learning and other AI applications.
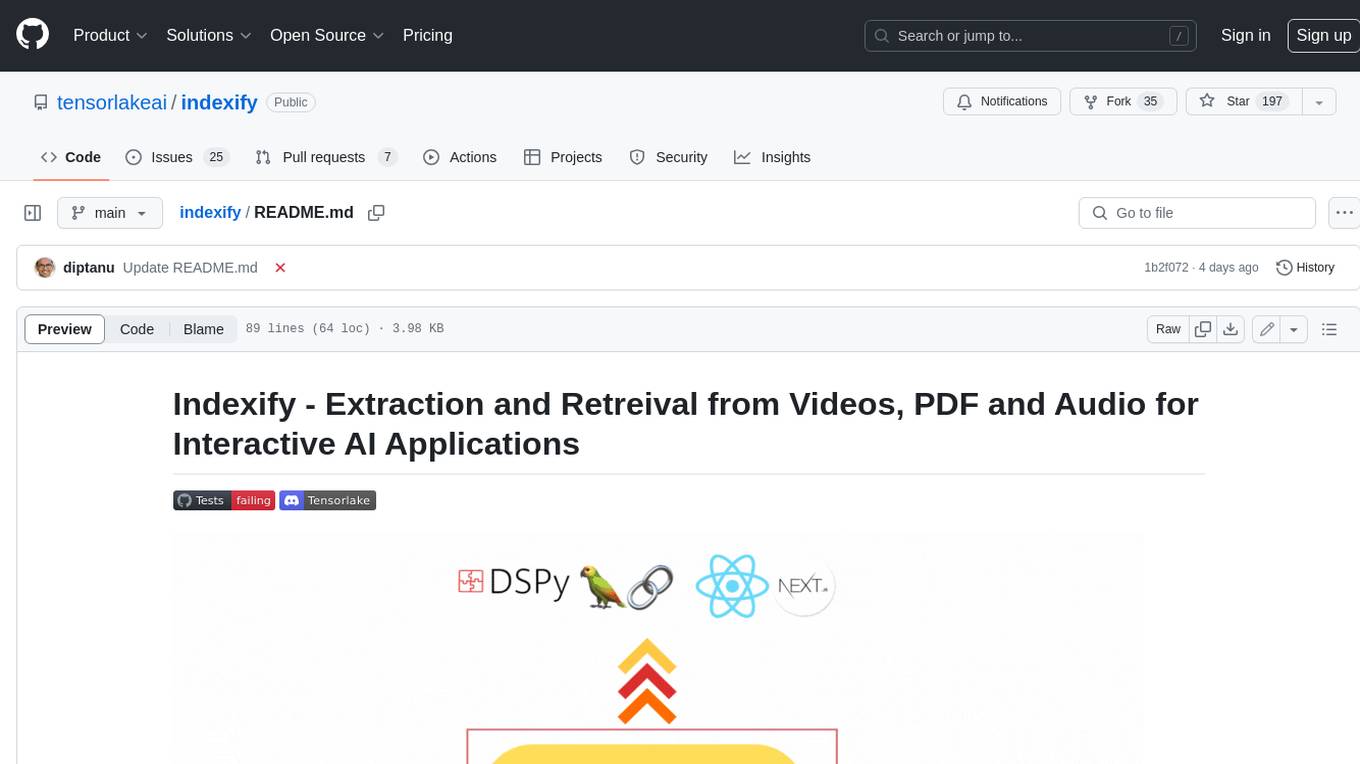
indexify
Indexify is an open-source engine for building fast data pipelines for unstructured data (video, audio, images, and documents) using reusable extractors for embedding, transformation, and feature extraction. LLM Applications can query transformed content friendly to LLMs by semantic search and SQL queries. Indexify keeps vector databases and structured databases (PostgreSQL) updated by automatically invoking the pipelines as new data is ingested into the system from external data sources. **Why use Indexify** * Makes Unstructured Data **Queryable** with **SQL** and **Semantic Search** * **Real-Time** Extraction Engine to keep indexes **automatically** updated as new data is ingested. * Create **Extraction Graph** to describe **data transformation** and extraction of **embedding** and **structured extraction**. * **Incremental Extraction** and **Selective Deletion** when content is deleted or updated. * **Extractor SDK** allows adding new extraction capabilities, and many readily available extractors for **PDF**, **Image**, and **Video** indexing and extraction. * Works with **any LLM Framework** including **Langchain**, **DSPy**, etc. * Runs on your laptop during **prototyping** and also scales to **1000s of machines** on the cloud. * Works with many **Blob Stores**, **Vector Stores**, and **Structured Databases** * We have even **Open Sourced Automation** to deploy to Kubernetes in production.
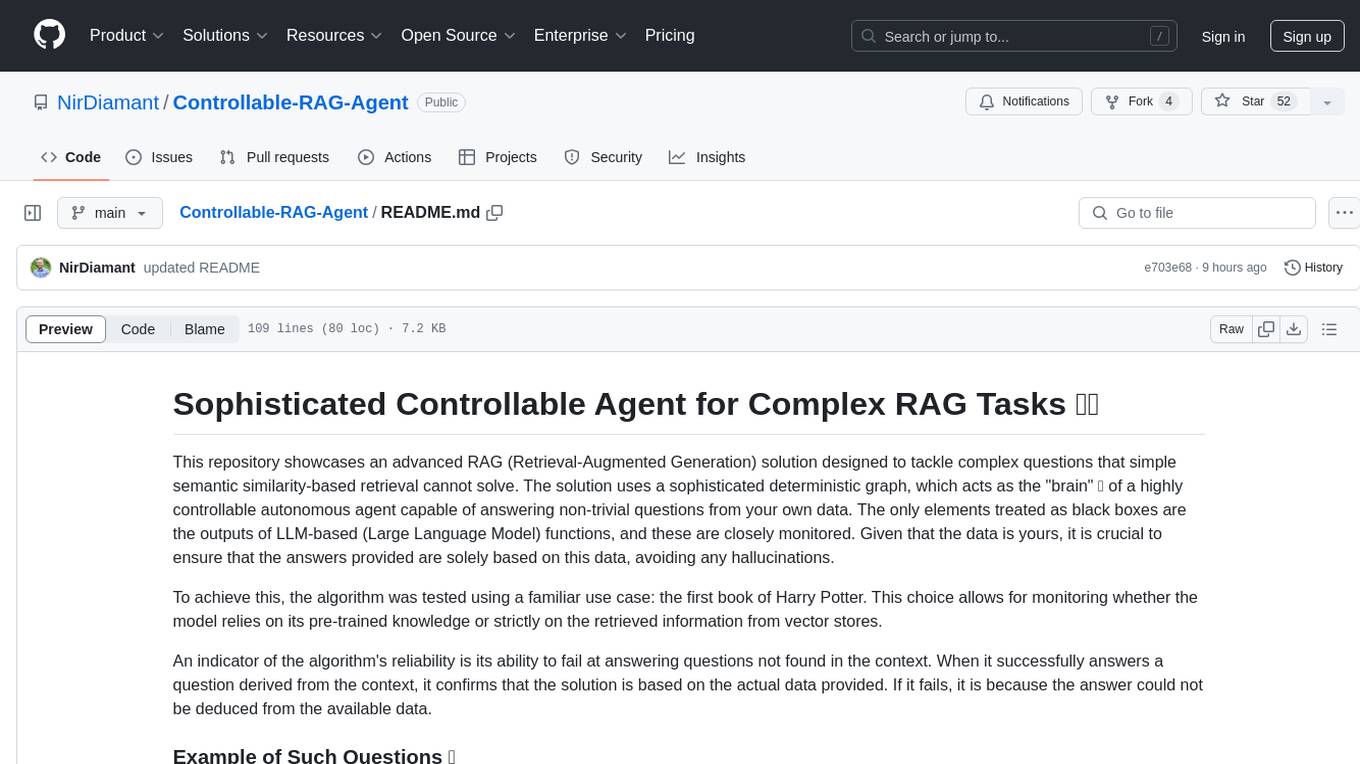
Controllable-RAG-Agent
This repository contains a sophisticated deterministic graph-based solution for answering complex questions using a controllable autonomous agent. The solution is designed to ensure that answers are solely based on the provided data, avoiding hallucinations. It involves various steps such as PDF loading, text preprocessing, summarization, database creation, encoding, and utilizing large language models. The algorithm follows a detailed workflow involving planning, retrieval, answering, replanning, content distillation, and performance evaluation. Heuristics and techniques implemented focus on content encoding, anonymizing questions, task breakdown, content distillation, chain of thought answering, verification, and model performance evaluation.
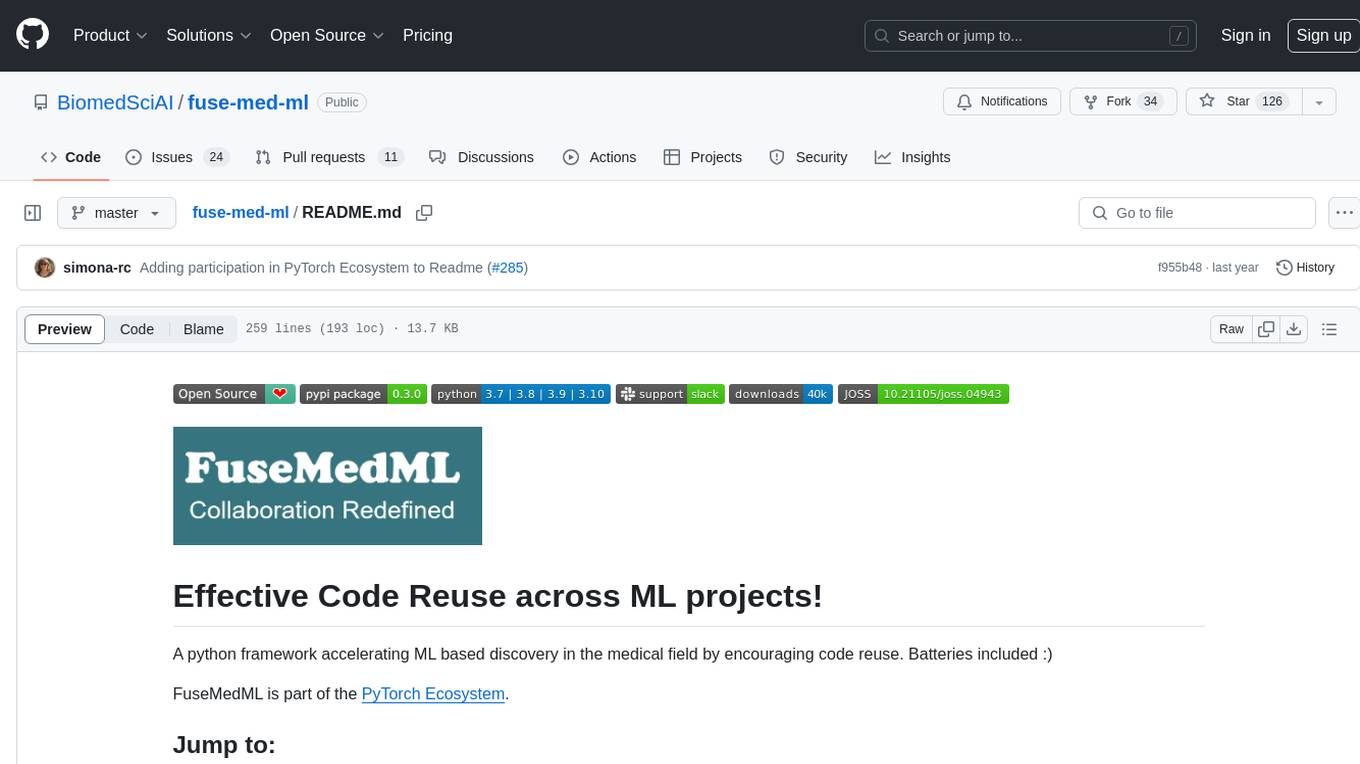
fuse-med-ml
FuseMedML is a Python framework designed to accelerate machine learning-based discovery in the medical field by promoting code reuse. It provides a flexible design concept where data is stored in a nested dictionary, allowing easy handling of multi-modality information. The framework includes components for creating custom models, loss functions, metrics, and data processing operators. Additionally, FuseMedML offers 'batteries included' key components such as fuse.data for data processing, fuse.eval for model evaluation, and fuse.dl for reusable deep learning components. It supports PyTorch and PyTorch Lightning libraries and encourages the creation of domain extensions for specific medical domains.
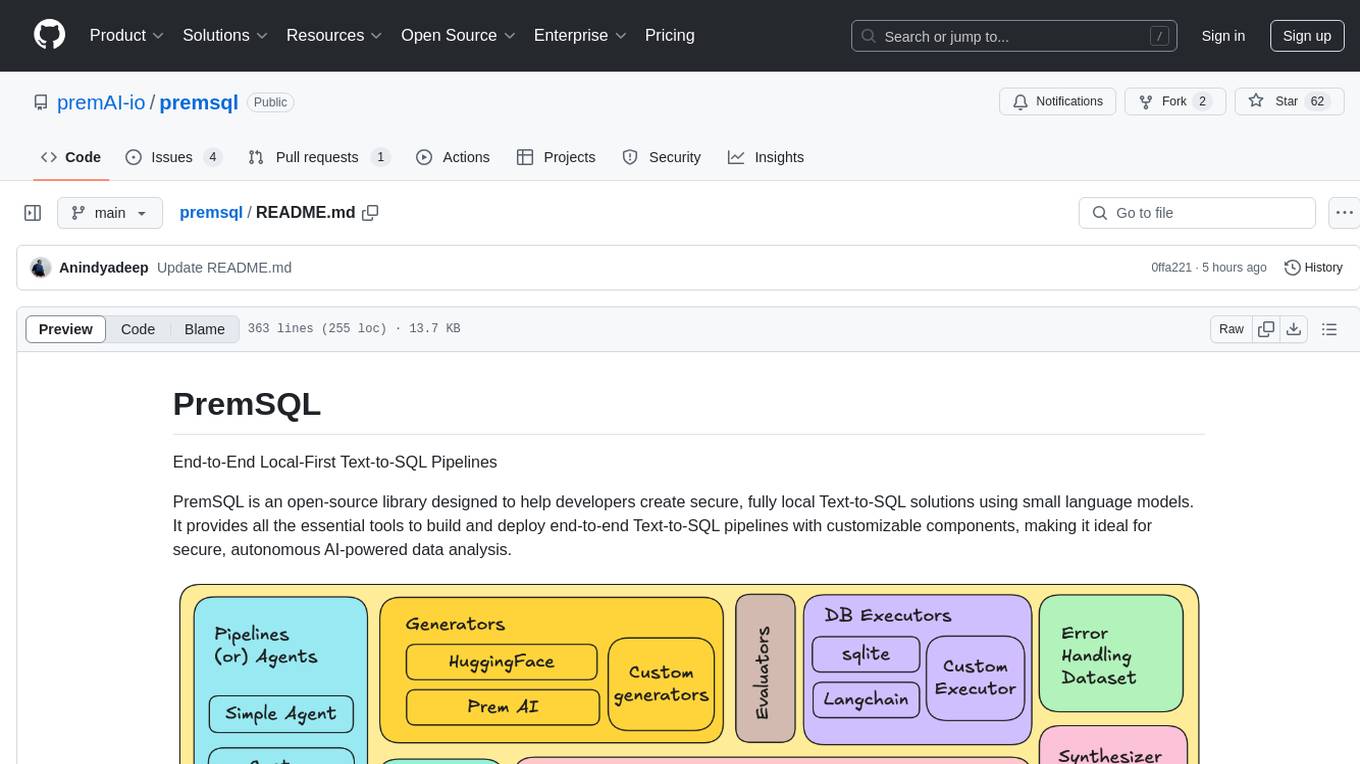
premsql
PremSQL is an open-source library designed to help developers create secure, fully local Text-to-SQL solutions using small language models. It provides essential tools for building and deploying end-to-end Text-to-SQL pipelines with customizable components, ideal for secure, autonomous AI-powered data analysis. The library offers features like Local-First approach, Customizable Datasets, Robust Executors and Evaluators, Advanced Generators, Error Handling and Self-Correction, Fine-Tuning Support, and End-to-End Pipelines. Users can fine-tune models, generate SQL queries from natural language inputs, handle errors, and evaluate model performance against predefined metrics. PremSQL is extendible for customization and private data usage.
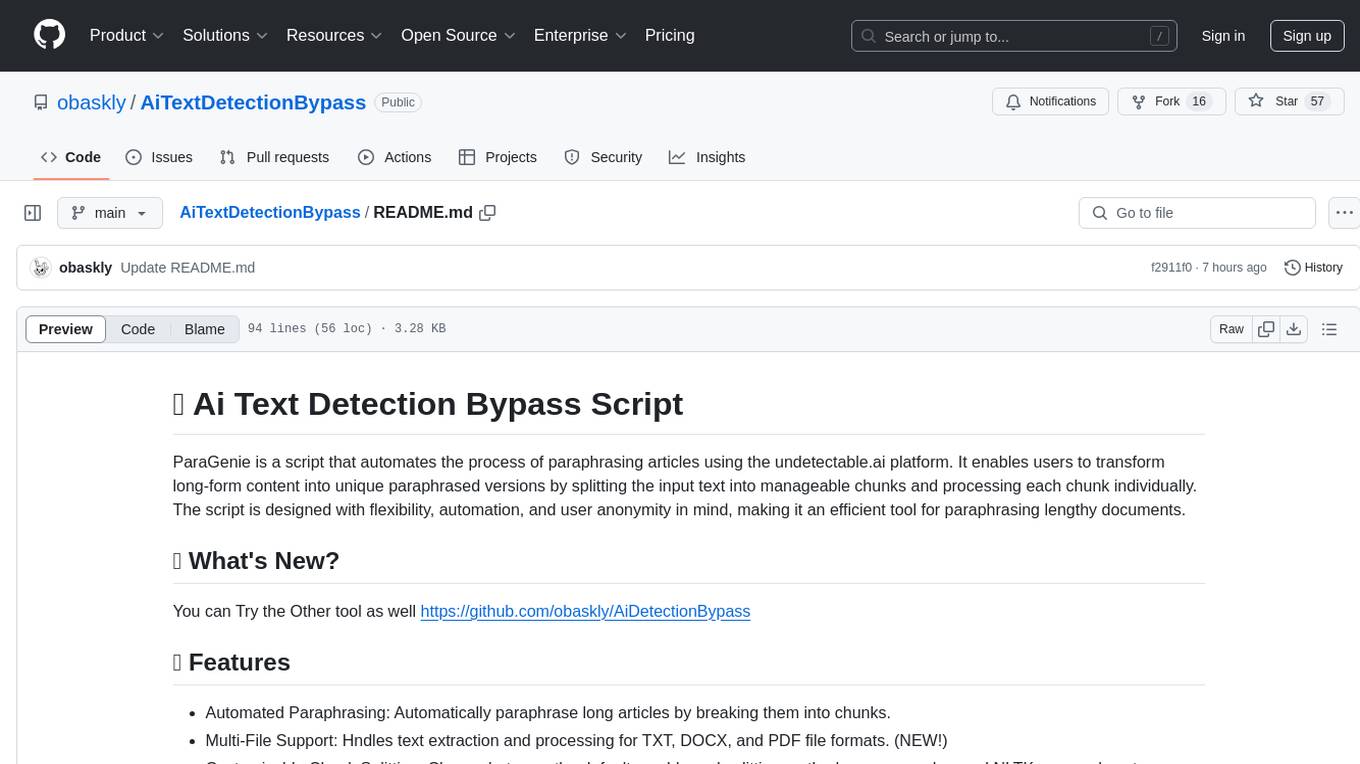
AiTextDetectionBypass
ParaGenie is a script designed to automate the process of paraphrasing articles using the undetectable.ai platform. It allows users to convert lengthy content into unique paraphrased versions by splitting the input text into manageable chunks and processing each chunk individually. The script offers features such as automated paraphrasing, multi-file support for TXT, DOCX, and PDF formats, customizable chunk splitting methods, Gmail-based registration for seamless paraphrasing, purpose-specific writing support, readability level customization, anonymity features for user privacy, error handling and recovery, and output management for easy access and organization of paraphrased content.
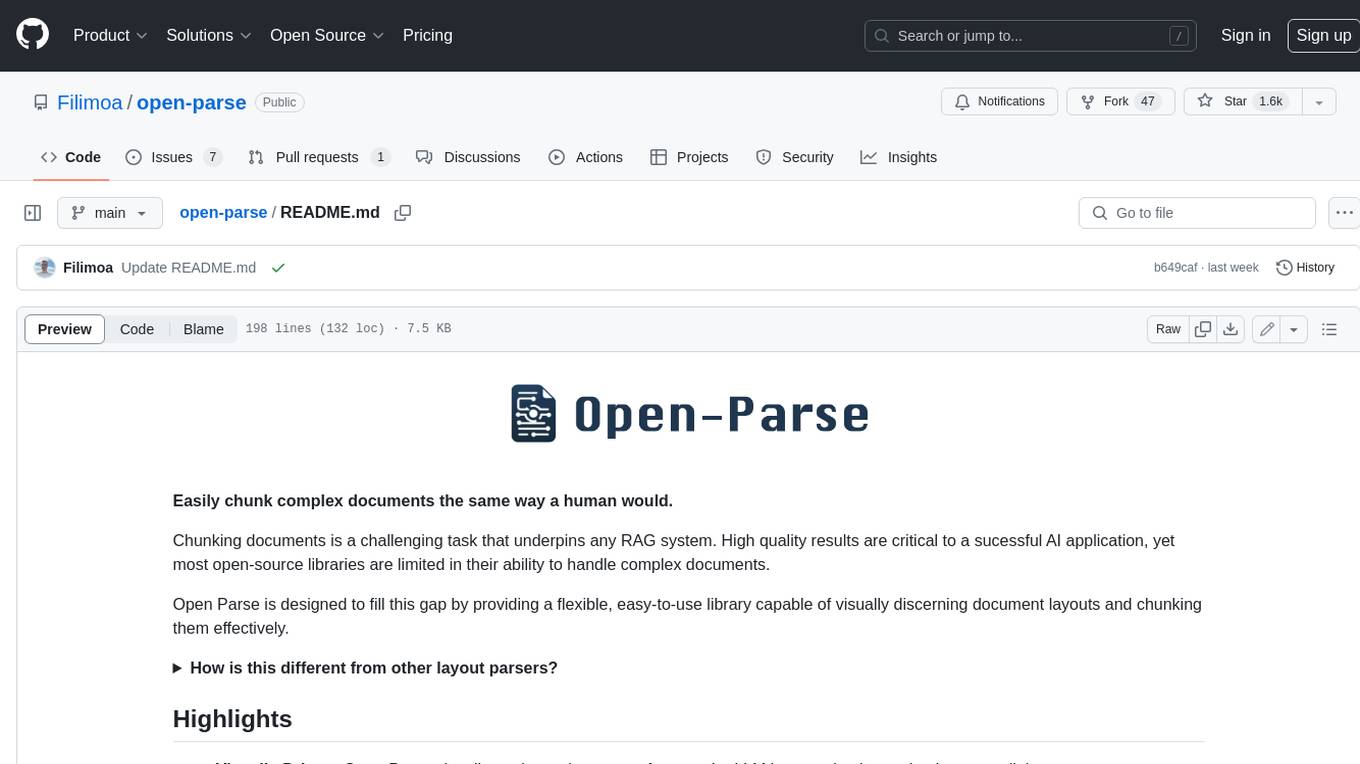
open-parse
Open Parse is a Python library for visually discerning document layouts and chunking them effectively. It is designed to fill the gap in open-source libraries for handling complex documents. Unlike text splitting, which converts a file to raw text and slices it up, Open Parse visually analyzes documents for superior LLM input. It also supports basic markdown for parsing headings, bold, and italics, and has high-precision table support, extracting tables into clean Markdown formats with accuracy that surpasses traditional tools. Open Parse is extensible, allowing users to easily implement their own post-processing steps. It is also intuitive, with great editor support and completion everywhere, making it easy to use and learn.
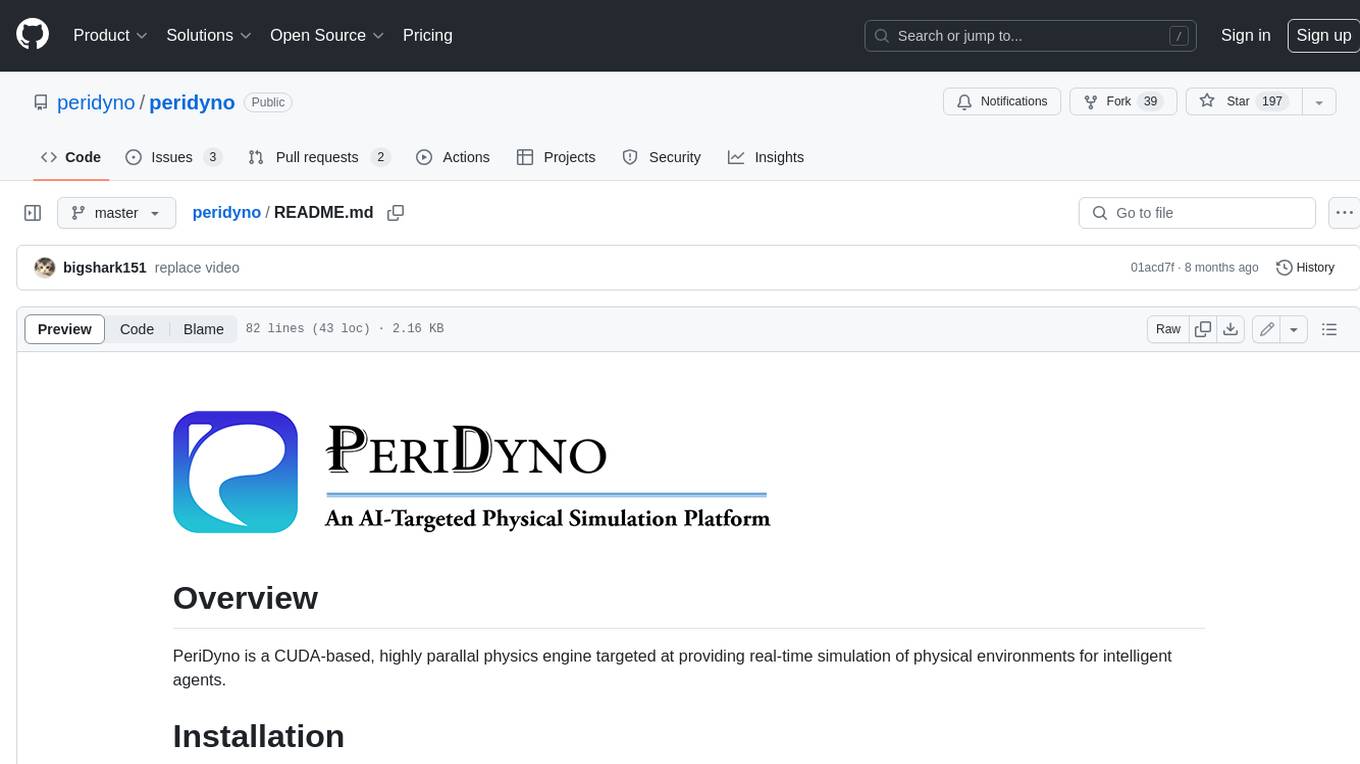
peridyno
PeriDyno is a CUDA-based, highly parallel physics engine targeted at providing real-time simulation of physical environments for intelligent agents. It is designed to be easy to use and integrate into existing projects, and it provides a wide range of features for simulating a variety of physical phenomena. PeriDyno is open source and available under the Apache 2.0 license.
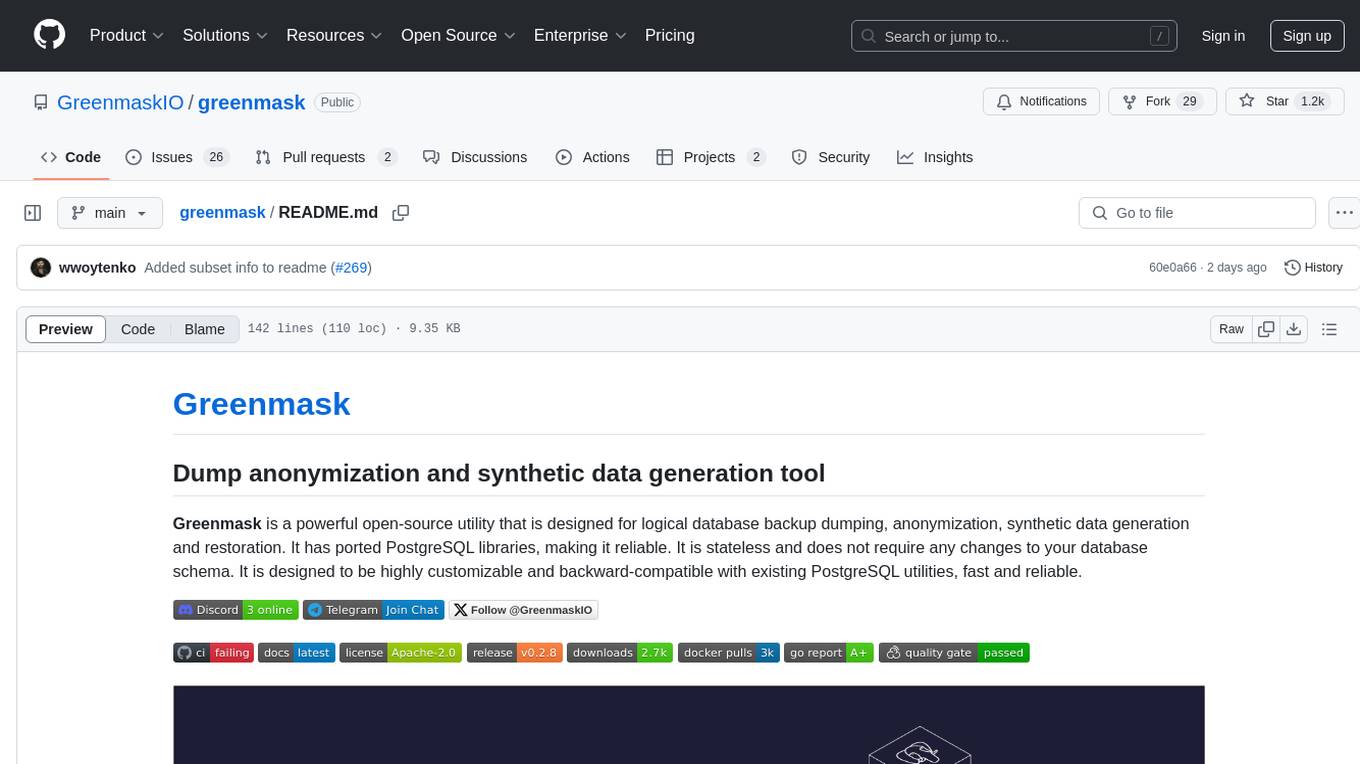
greenmask
Greenmask is a powerful open-source utility designed for logical database backup dumping, anonymization, synthetic data generation, and restoration. It is highly customizable, stateless, and backward-compatible with existing PostgreSQL utilities. Greenmask supports advanced subset systems, deterministic transformers, dynamic parameters, transformation conditions, and more. It is cross-platform, database type safe, extensible, and supports parallel execution and various storage options. Ideal for backup and restoration tasks, anonymization, transformation, and data masking.
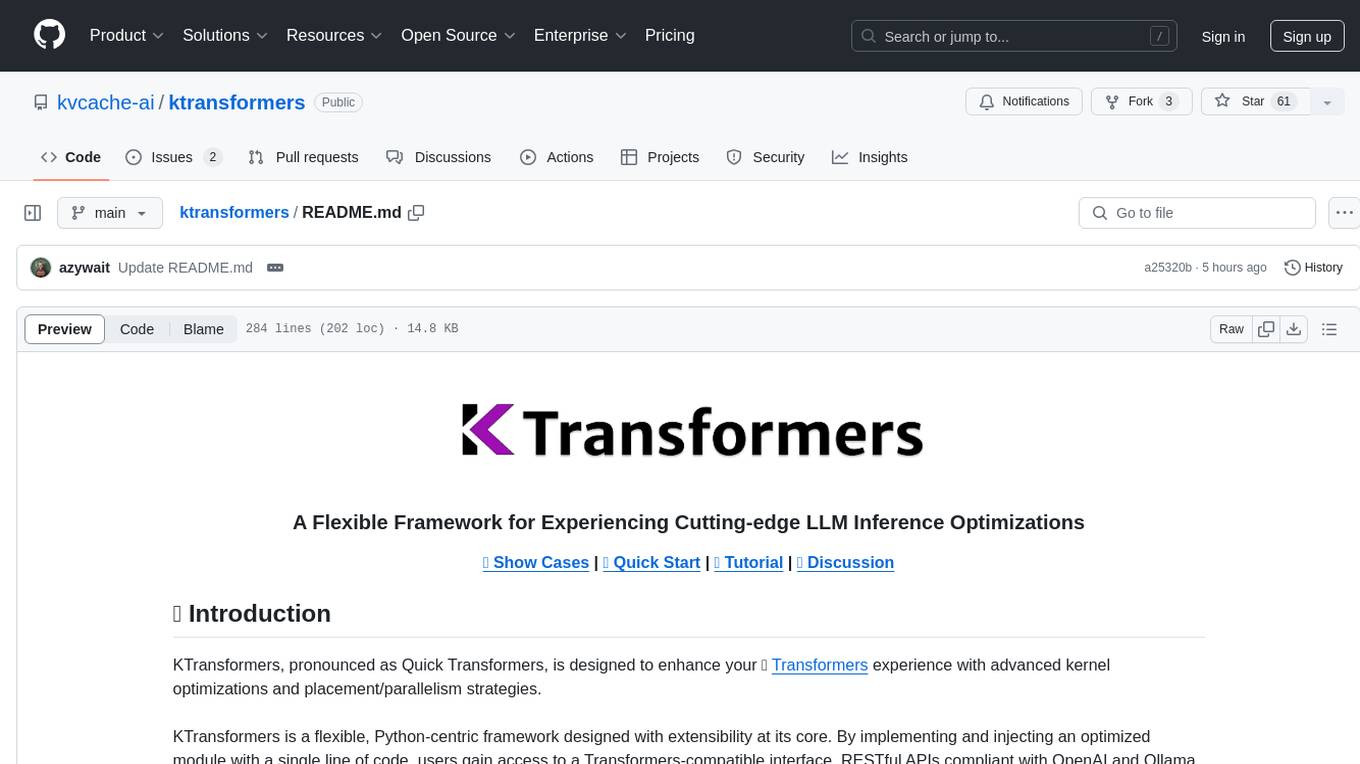
ktransformers
KTransformers is a flexible Python-centric framework designed to enhance the user's experience with advanced kernel optimizations and placement/parallelism strategies for Transformers. It provides a Transformers-compatible interface, RESTful APIs compliant with OpenAI and Ollama, and a simplified ChatGPT-like web UI. The framework aims to serve as a platform for experimenting with innovative LLM inference optimizations, focusing on local deployments constrained by limited resources and supporting heterogeneous computing opportunities like GPU/CPU offloading of quantized models.
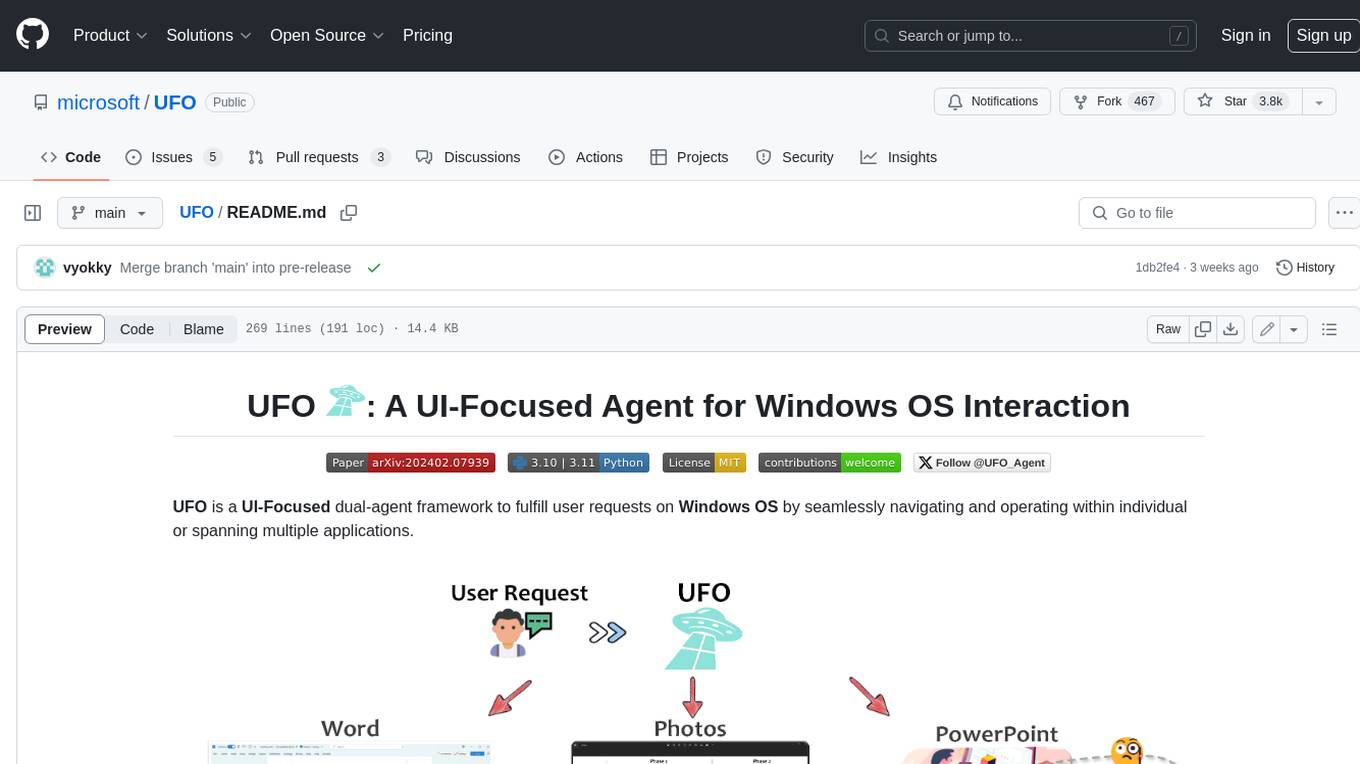
UFO
UFO is a UI-focused dual-agent framework to fulfill user requests on Windows OS by seamlessly navigating and operating within individual or spanning multiple applications.
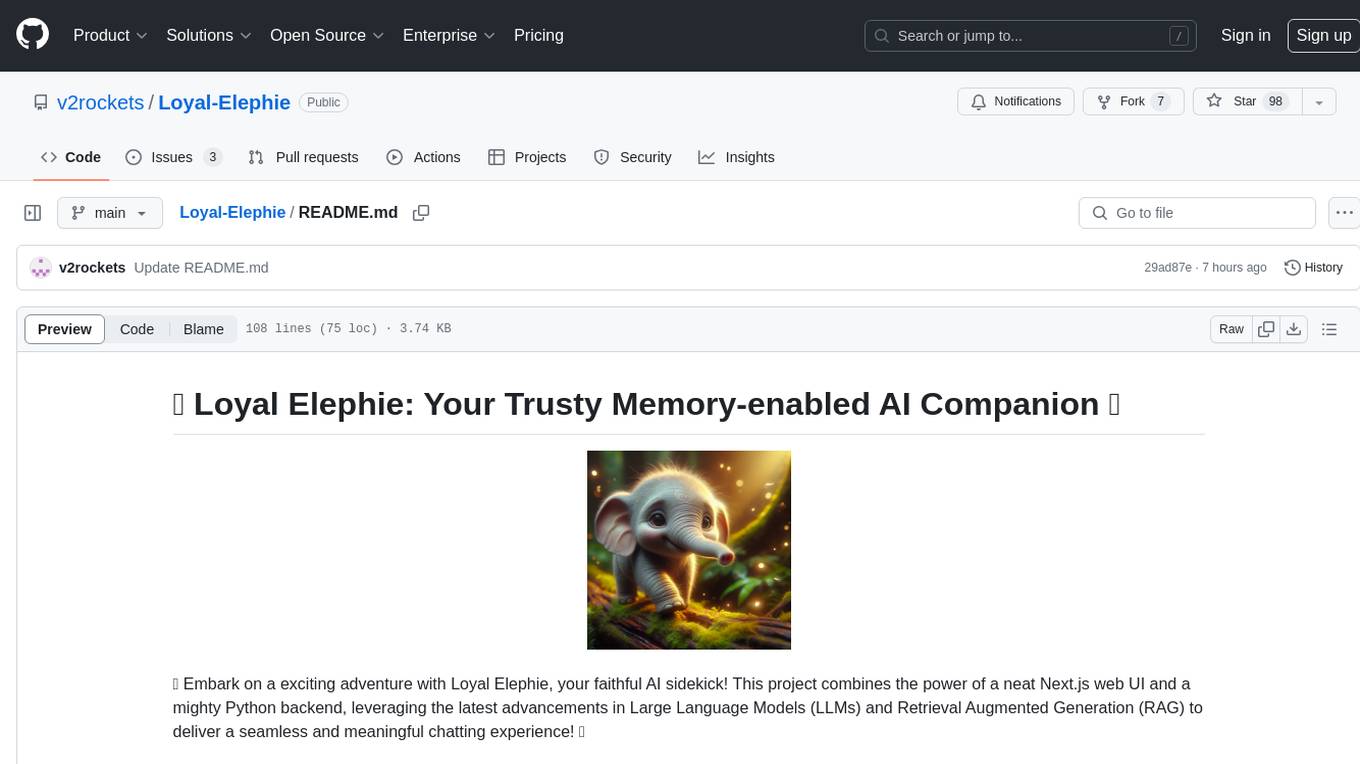
Loyal-Elephie
Embark on an exciting adventure with Loyal Elephie, your faithful AI sidekick! This project combines the power of a neat Next.js web UI and a mighty Python backend, leveraging the latest advancements in Large Language Models (LLMs) and Retrieval Augmented Generation (RAG) to deliver a seamless and meaningful chatting experience. Features include controllable memory, hybrid search, secure web access, streamlined LLM agent, and optional Markdown editor integration. Loyal Elephie supports both open and proprietary LLMs and embeddings serving as OpenAI compatible APIs.
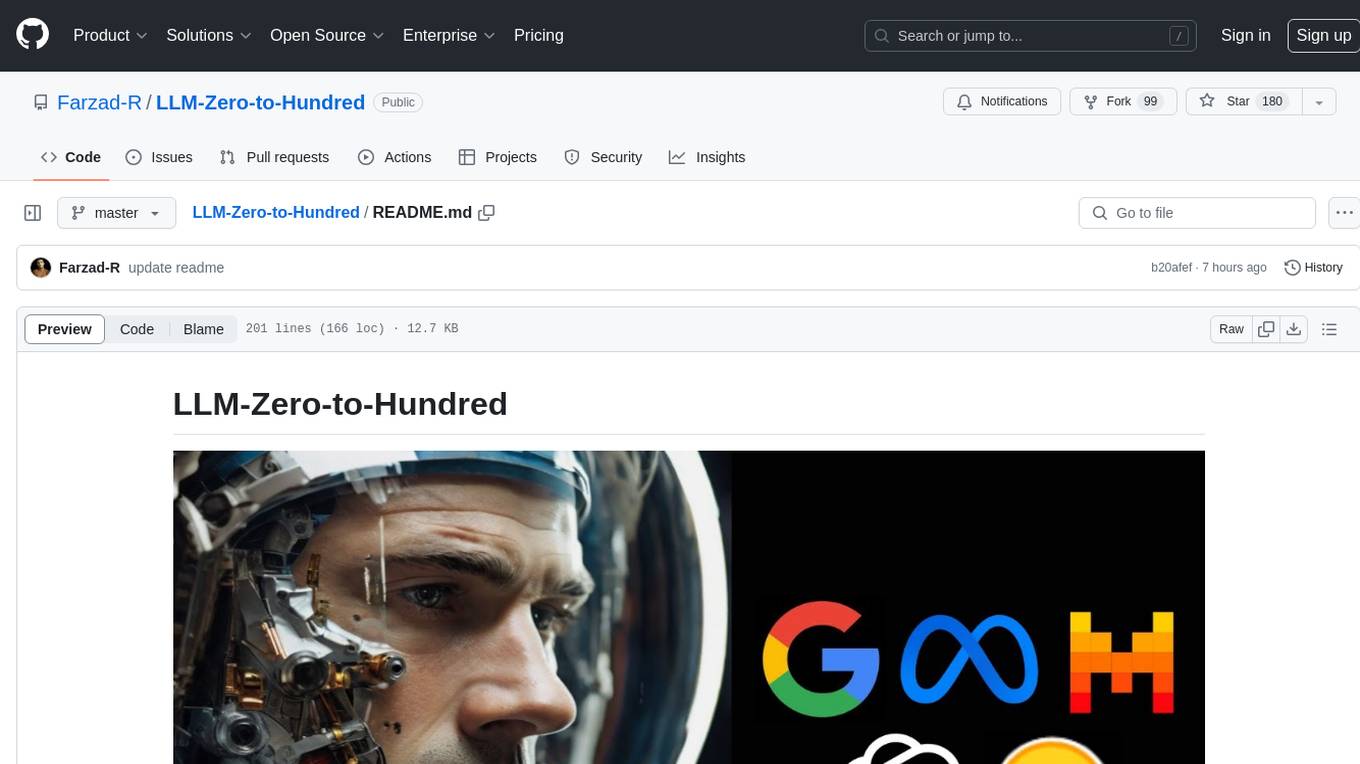
LLM-Zero-to-Hundred
LLM-Zero-to-Hundred is a repository showcasing various applications of LLM chatbots and providing insights into training and fine-tuning Language Models. It includes projects like WebGPT, RAG-GPT, WebRAGQuery, LLM Full Finetuning, RAG-Master LLamaindex vs Langchain, open-source-RAG-GEMMA, and HUMAIN: Advanced Multimodal, Multitask Chatbot. The projects cover features like ChatGPT-like interaction, RAG capabilities, image generation and understanding, DuckDuckGo integration, summarization, text and voice interaction, and memory access. Tutorials include LLM Function Calling and Visualizing Text Vectorization. The projects have a general structure with folders for README, HELPER, .env, configs, data, src, images, and utils.
For similar tasks
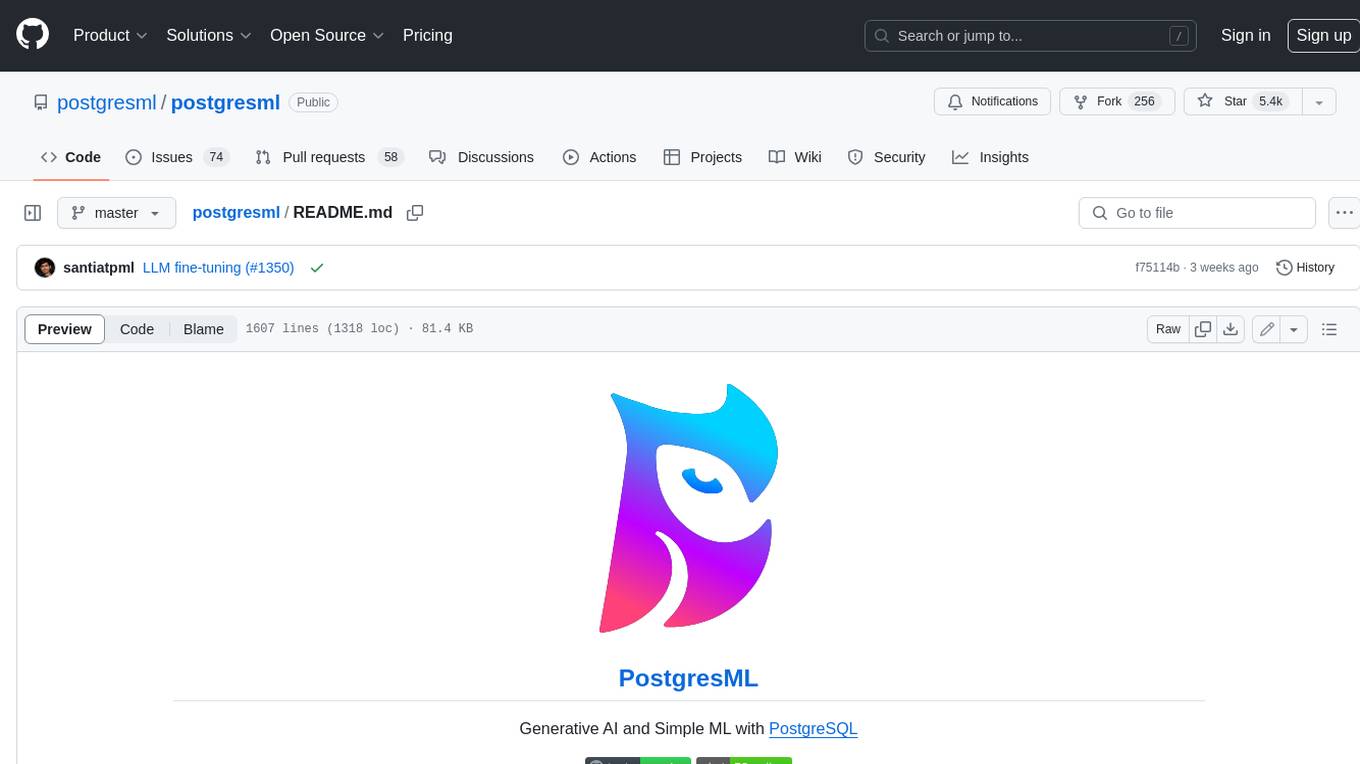
postgresml
PostgresML is a powerful Postgres extension that seamlessly combines data storage and machine learning inference within your database. It enables running machine learning and AI operations directly within PostgreSQL, leveraging GPU acceleration for faster computations, integrating state-of-the-art large language models, providing built-in functions for text processing, enabling efficient similarity search, offering diverse ML algorithms, ensuring high performance, scalability, and security, supporting a wide range of NLP tasks, and seamlessly integrating with existing PostgreSQL tools and client libraries.
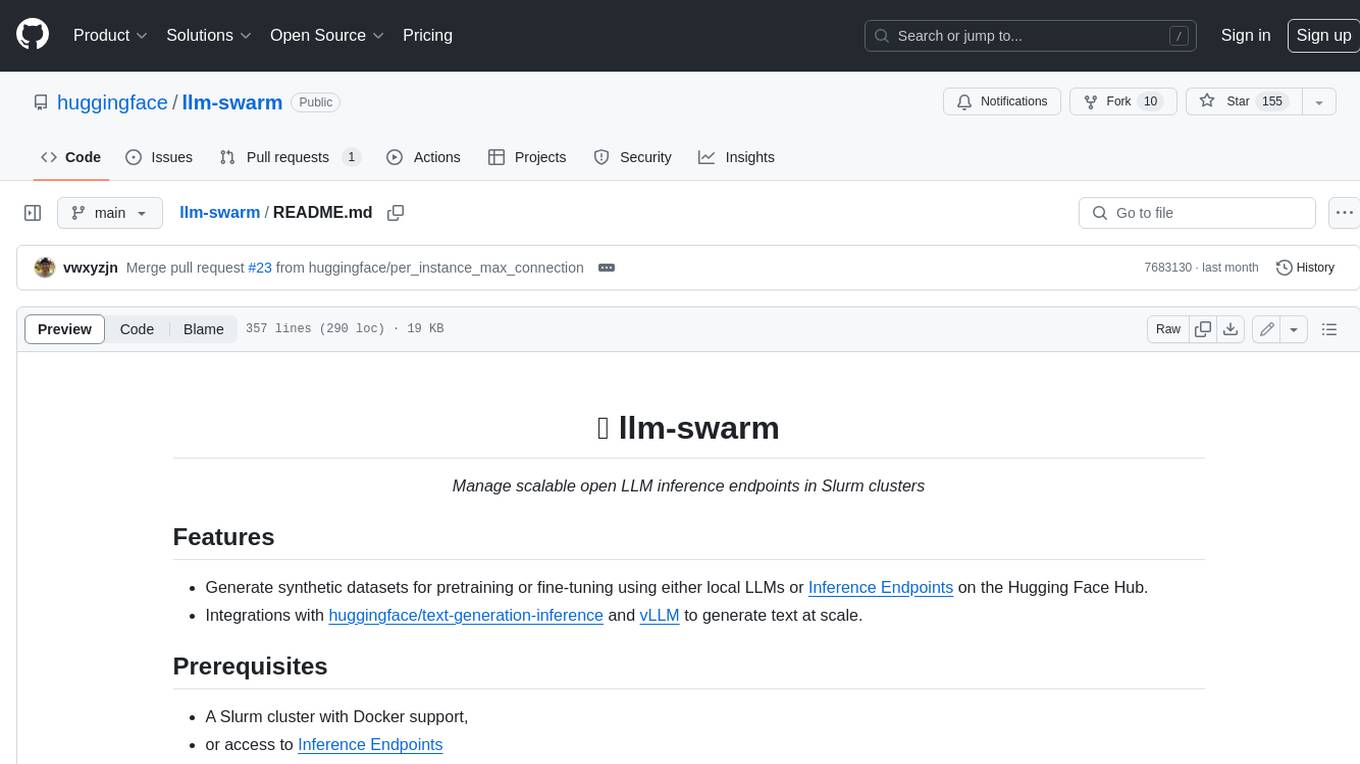
llm-swarm
llm-swarm is a tool designed to manage scalable open LLM inference endpoints in Slurm clusters. It allows users to generate synthetic datasets for pretraining or fine-tuning using local LLMs or Inference Endpoints on the Hugging Face Hub. The tool integrates with huggingface/text-generation-inference and vLLM to generate text at scale. It manages inference endpoint lifetime by automatically spinning up instances via `sbatch`, checking if they are created or connected, performing the generation job, and auto-terminating the inference endpoints to prevent idling. Additionally, it provides load balancing between multiple endpoints using a simple nginx docker for scalability. Users can create slurm files based on default configurations and inspect logs for further analysis. For users without a Slurm cluster, hosted inference endpoints are available for testing with usage limits based on registration status.
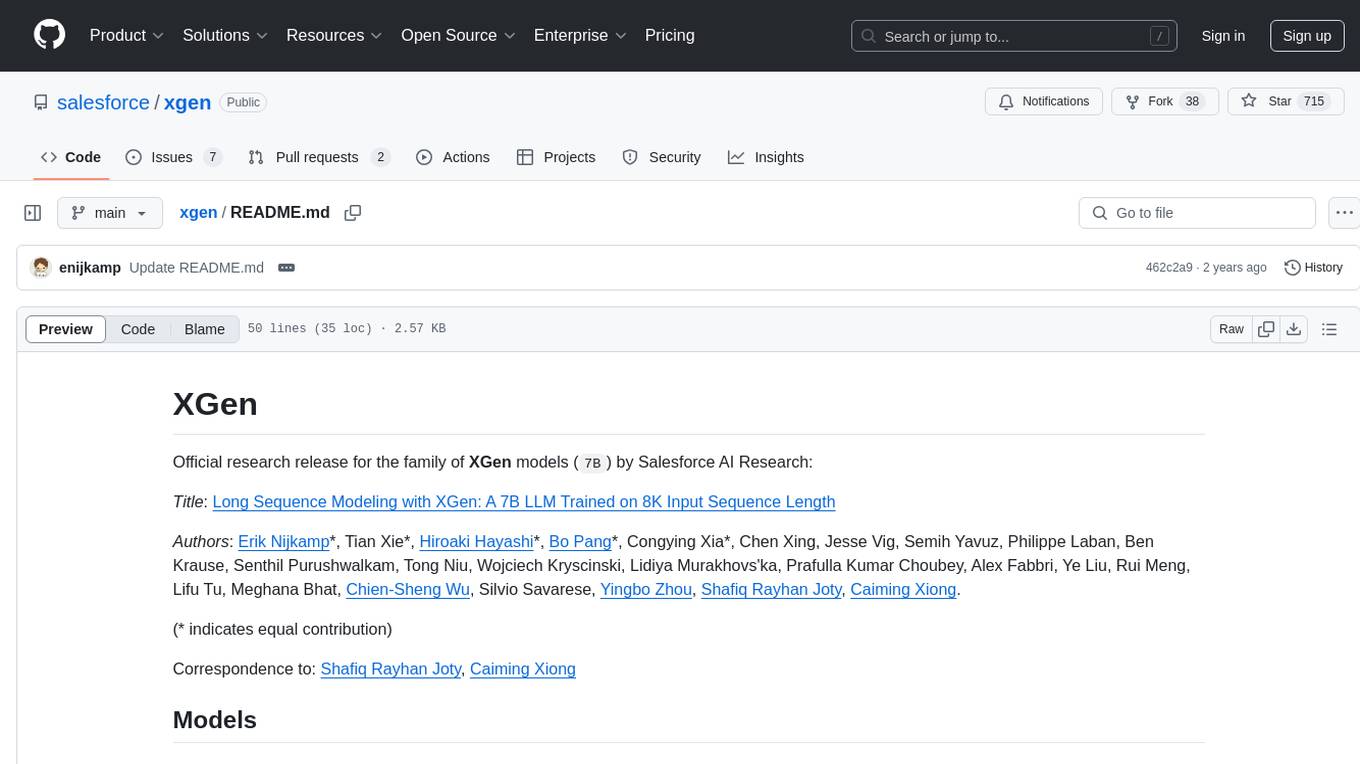
xgen
XGen is a research release for the family of XGen models (7B) by Salesforce AI Research. It includes models with support for different sequence lengths and tokenization using the OpenAI Tiktoken package. The models can be used for auto-regressive sampling in natural language generation tasks.
For similar jobs
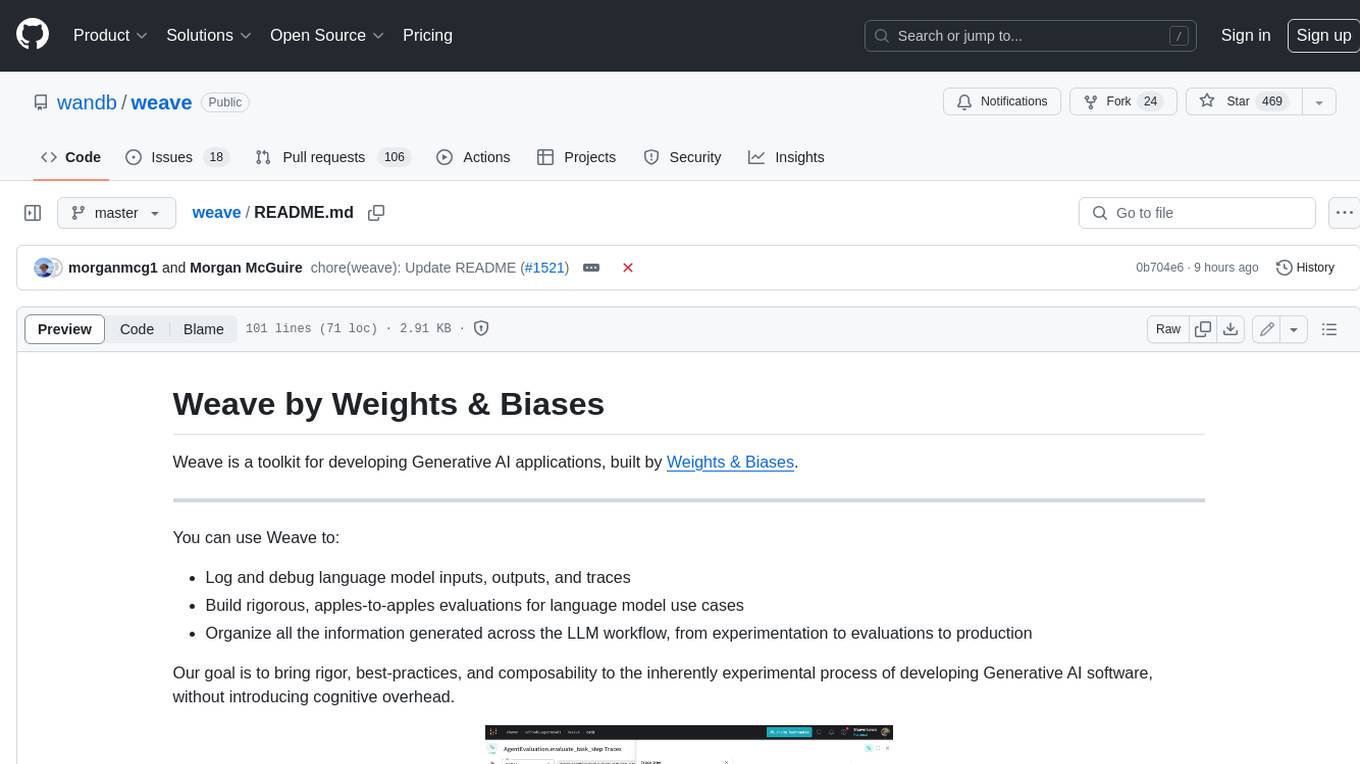
weave
Weave is a toolkit for developing Generative AI applications, built by Weights & Biases. With Weave, you can log and debug language model inputs, outputs, and traces; build rigorous, apples-to-apples evaluations for language model use cases; and organize all the information generated across the LLM workflow, from experimentation to evaluations to production. Weave aims to bring rigor, best-practices, and composability to the inherently experimental process of developing Generative AI software, without introducing cognitive overhead.
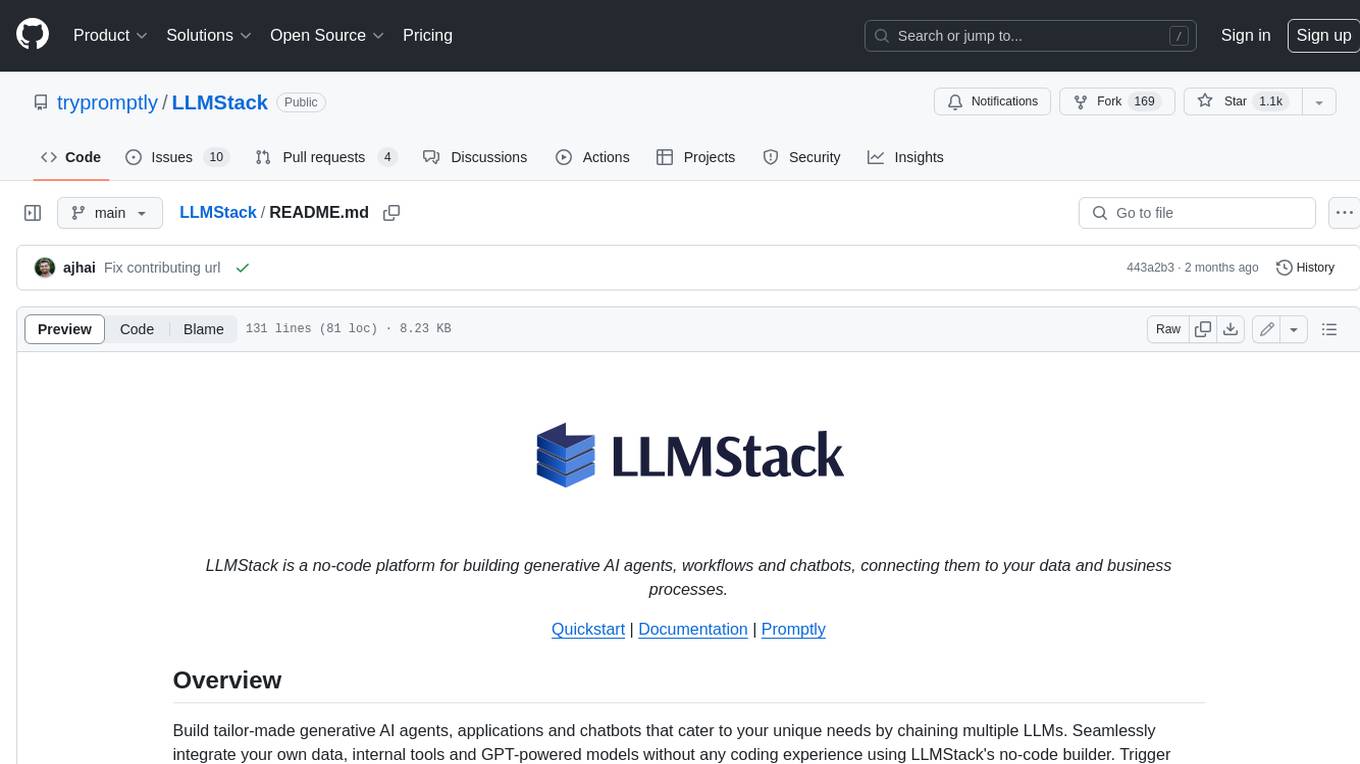
LLMStack
LLMStack is a no-code platform for building generative AI agents, workflows, and chatbots. It allows users to connect their own data, internal tools, and GPT-powered models without any coding experience. LLMStack can be deployed to the cloud or on-premise and can be accessed via HTTP API or triggered from Slack or Discord.
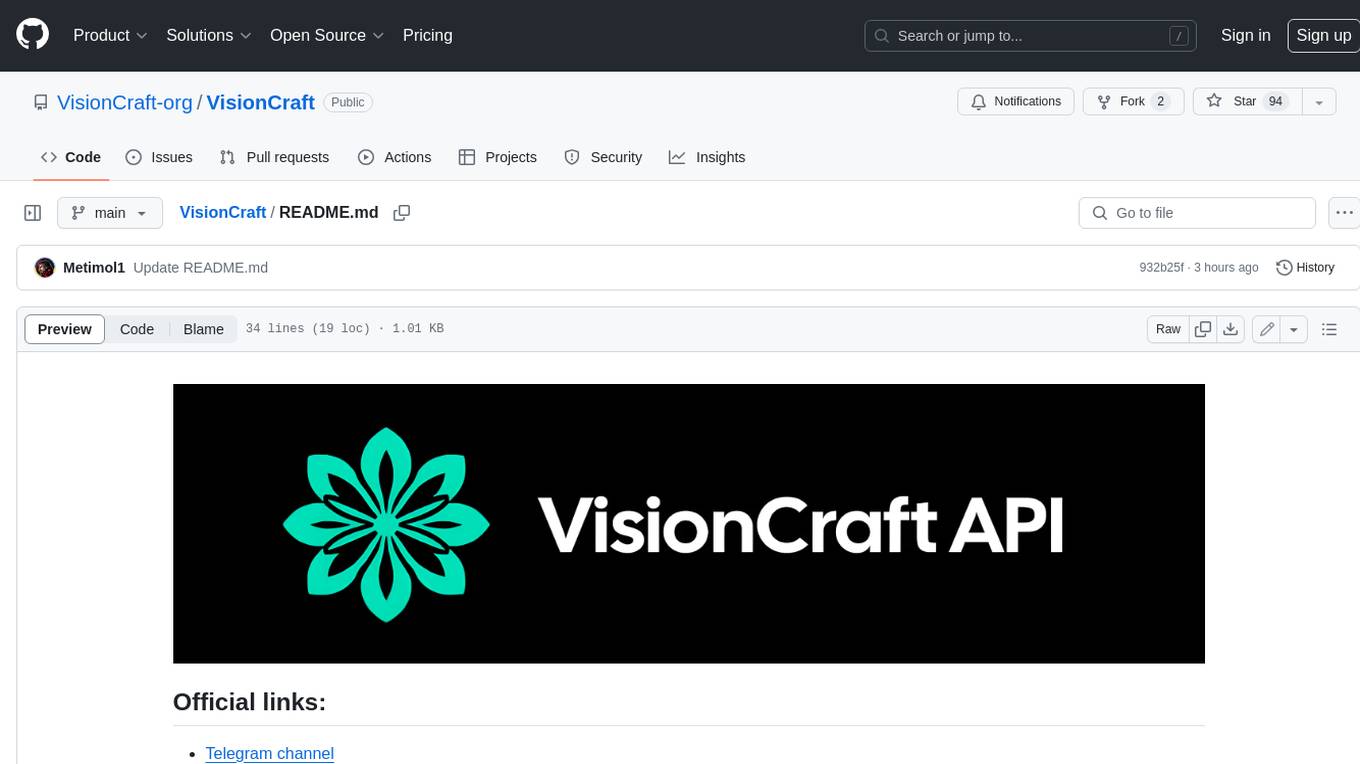
VisionCraft
The VisionCraft API is a free API for using over 100 different AI models. From images to sound.
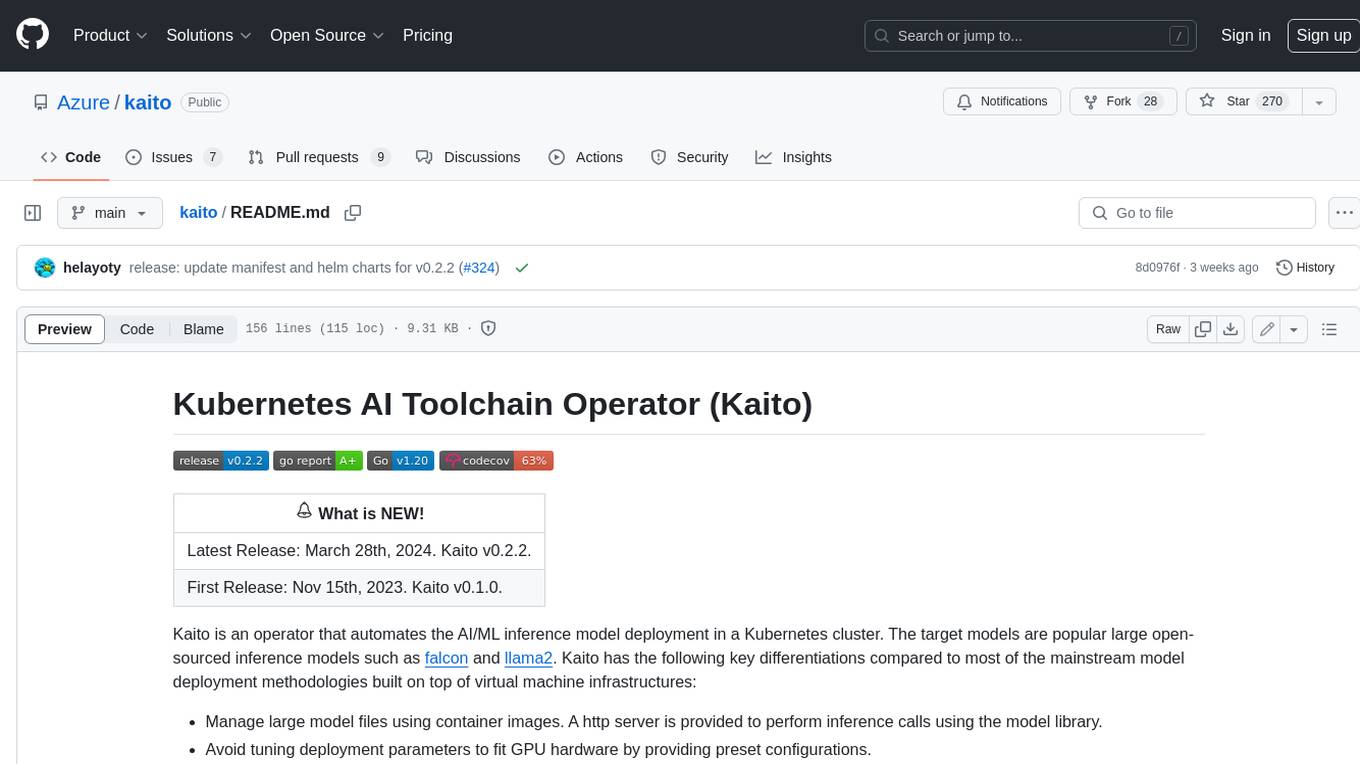
kaito
Kaito is an operator that automates the AI/ML inference model deployment in a Kubernetes cluster. It manages large model files using container images, avoids tuning deployment parameters to fit GPU hardware by providing preset configurations, auto-provisions GPU nodes based on model requirements, and hosts large model images in the public Microsoft Container Registry (MCR) if the license allows. Using Kaito, the workflow of onboarding large AI inference models in Kubernetes is largely simplified.
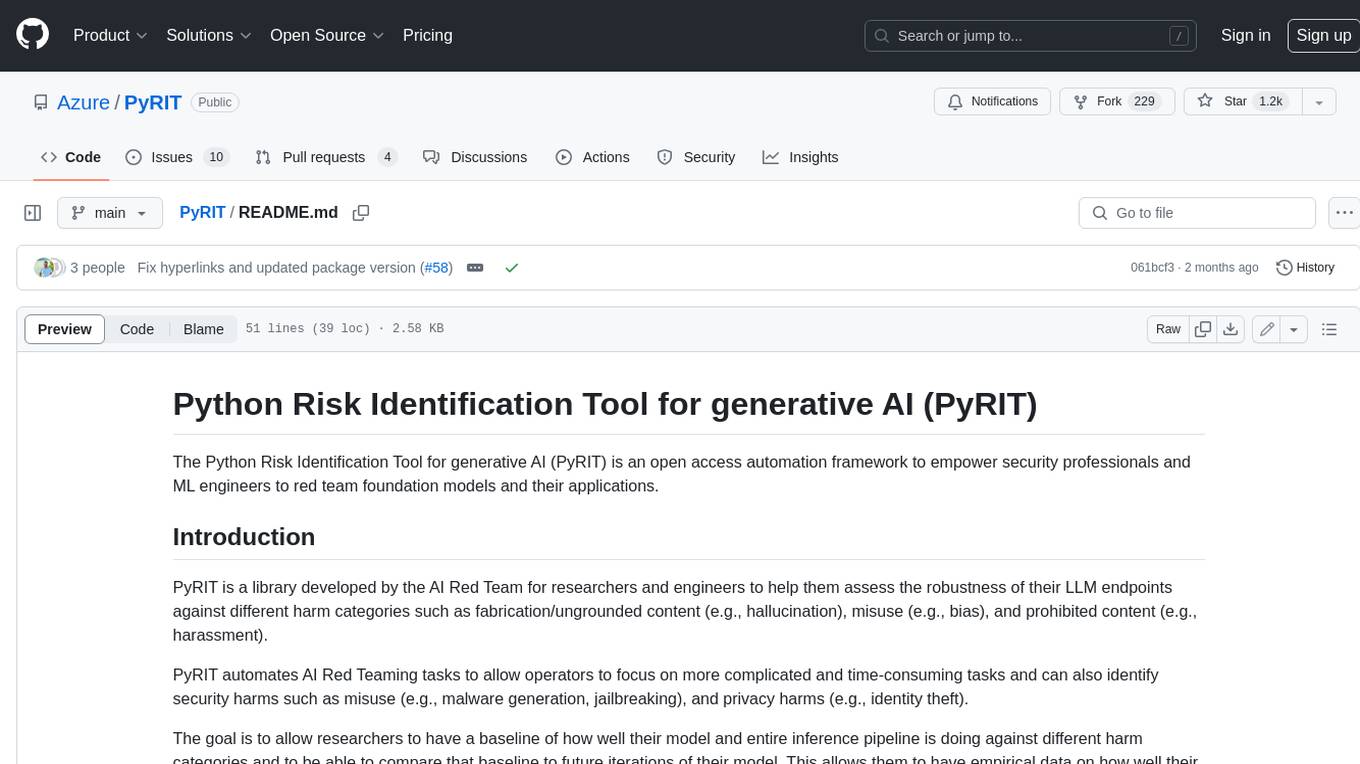
PyRIT
PyRIT is an open access automation framework designed to empower security professionals and ML engineers to red team foundation models and their applications. It automates AI Red Teaming tasks to allow operators to focus on more complicated and time-consuming tasks and can also identify security harms such as misuse (e.g., malware generation, jailbreaking), and privacy harms (e.g., identity theft). The goal is to allow researchers to have a baseline of how well their model and entire inference pipeline is doing against different harm categories and to be able to compare that baseline to future iterations of their model. This allows them to have empirical data on how well their model is doing today, and detect any degradation of performance based on future improvements.
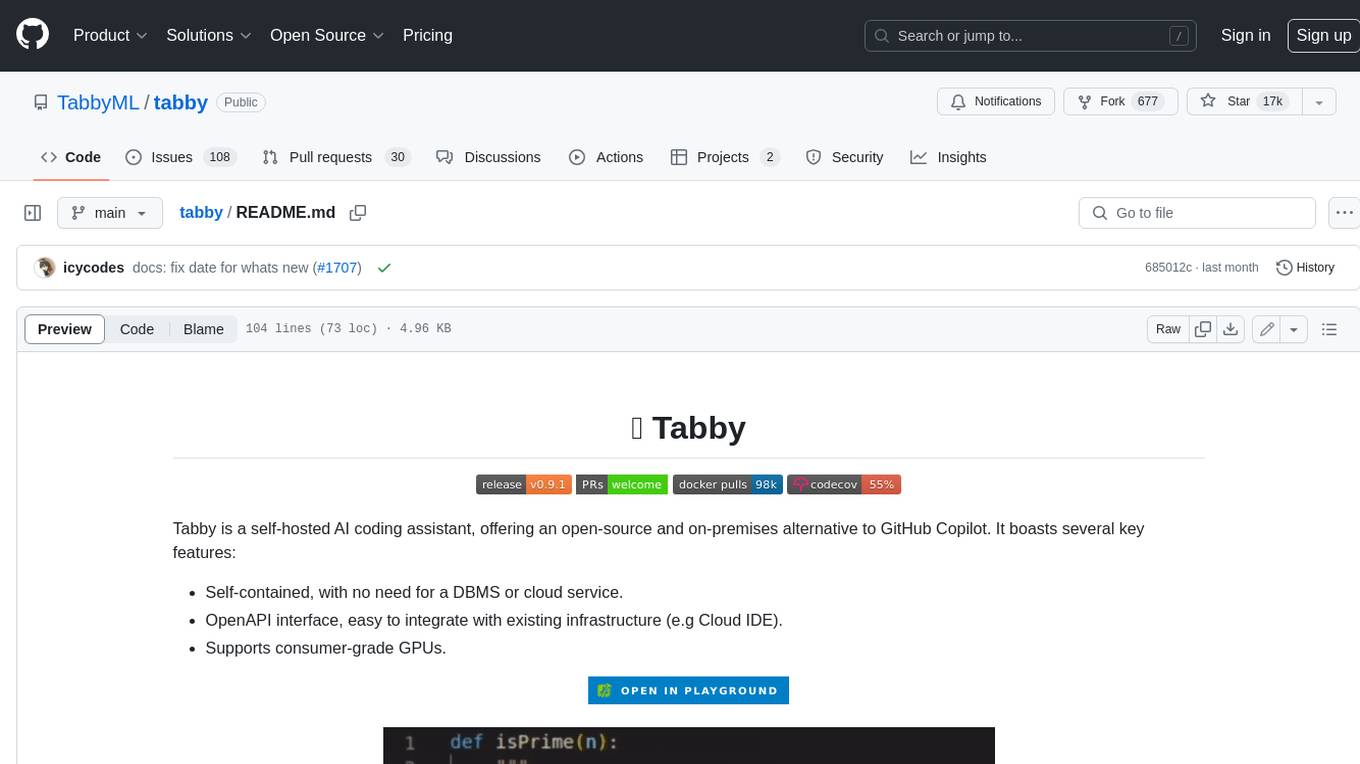
tabby
Tabby is a self-hosted AI coding assistant, offering an open-source and on-premises alternative to GitHub Copilot. It boasts several key features: * Self-contained, with no need for a DBMS or cloud service. * OpenAPI interface, easy to integrate with existing infrastructure (e.g Cloud IDE). * Supports consumer-grade GPUs.
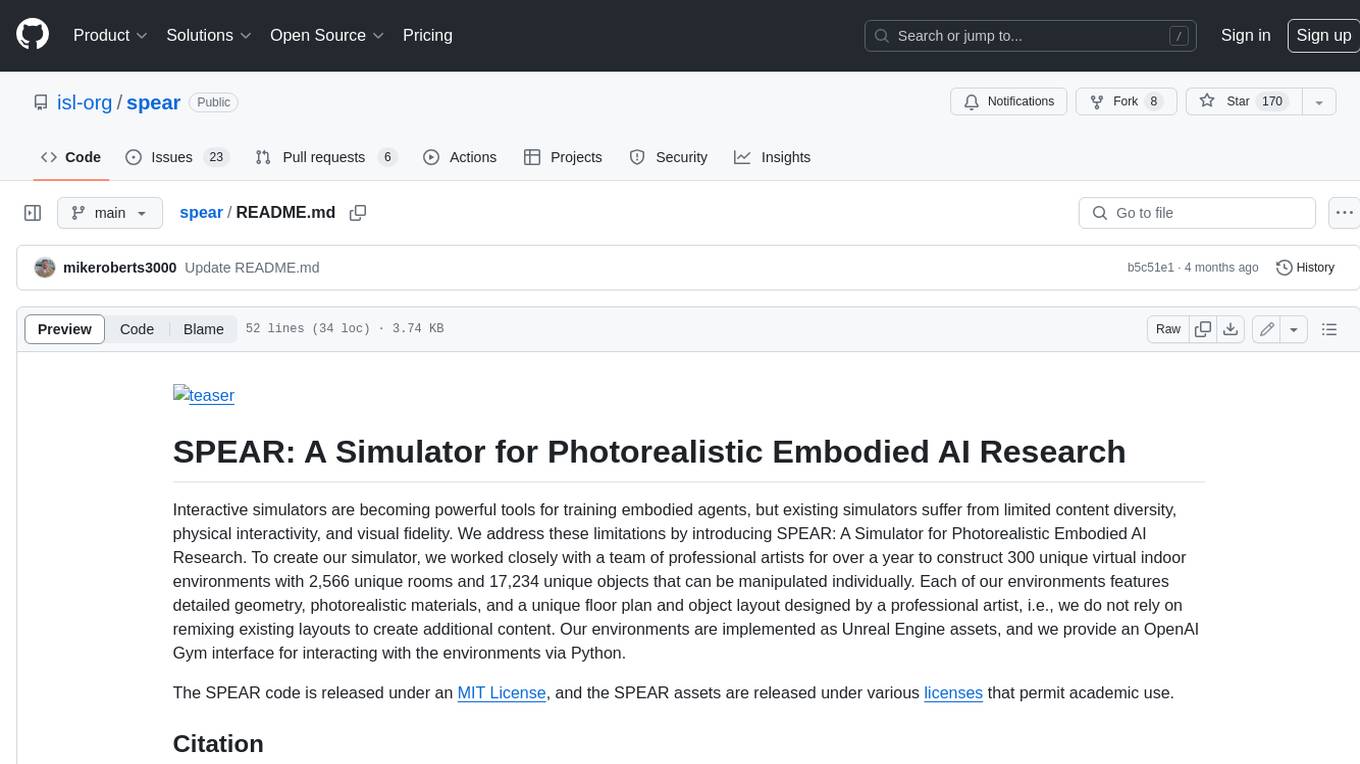
spear
SPEAR (Simulator for Photorealistic Embodied AI Research) is a powerful tool for training embodied agents. It features 300 unique virtual indoor environments with 2,566 unique rooms and 17,234 unique objects that can be manipulated individually. Each environment is designed by a professional artist and features detailed geometry, photorealistic materials, and a unique floor plan and object layout. SPEAR is implemented as Unreal Engine assets and provides an OpenAI Gym interface for interacting with the environments via Python.
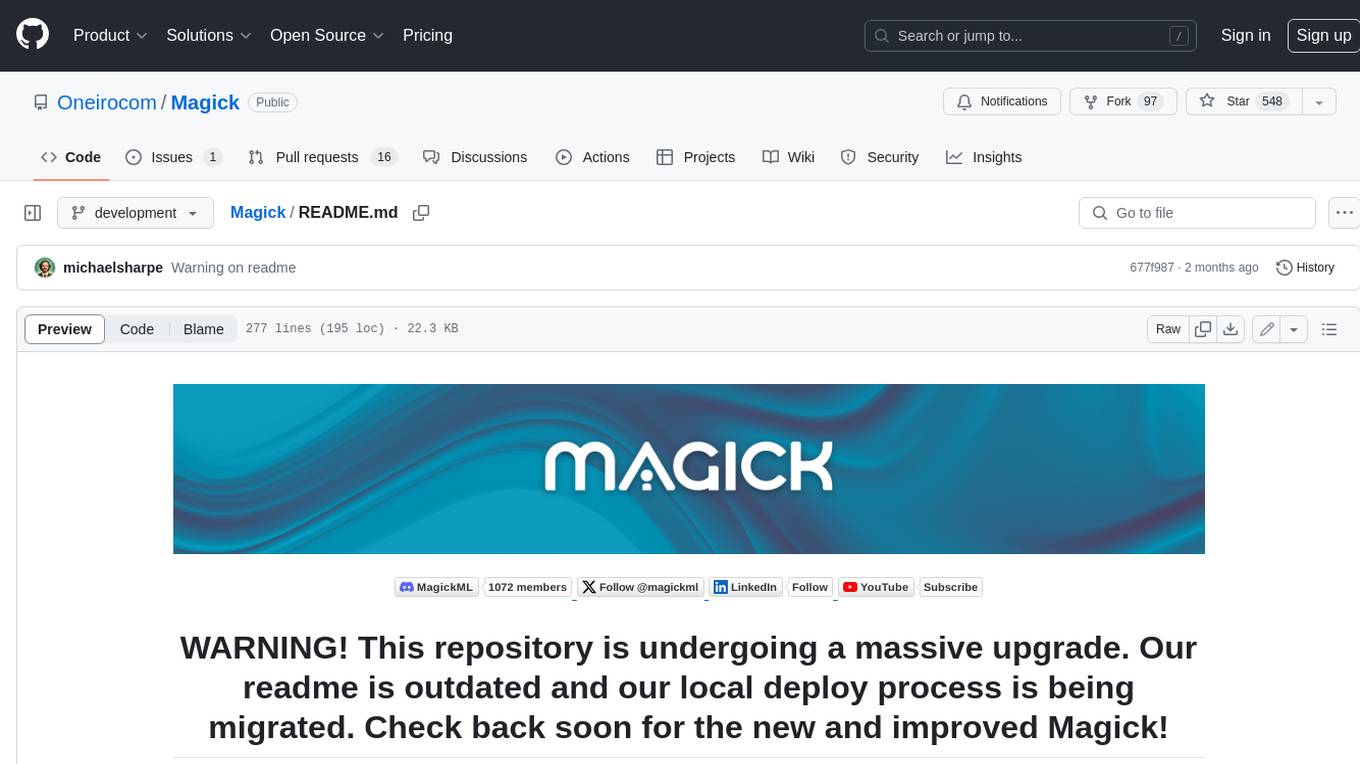
Magick
Magick is a groundbreaking visual AIDE (Artificial Intelligence Development Environment) for no-code data pipelines and multimodal agents. Magick can connect to other services and comes with nodes and templates well-suited for intelligent agents, chatbots, complex reasoning systems and realistic characters.