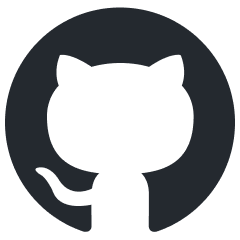
PrivateDocBot
📚 Local PDF-Integrated Chat Bot: Secure Conversations and Document Assistance with LLM-Powered Privacy
Stars: 81
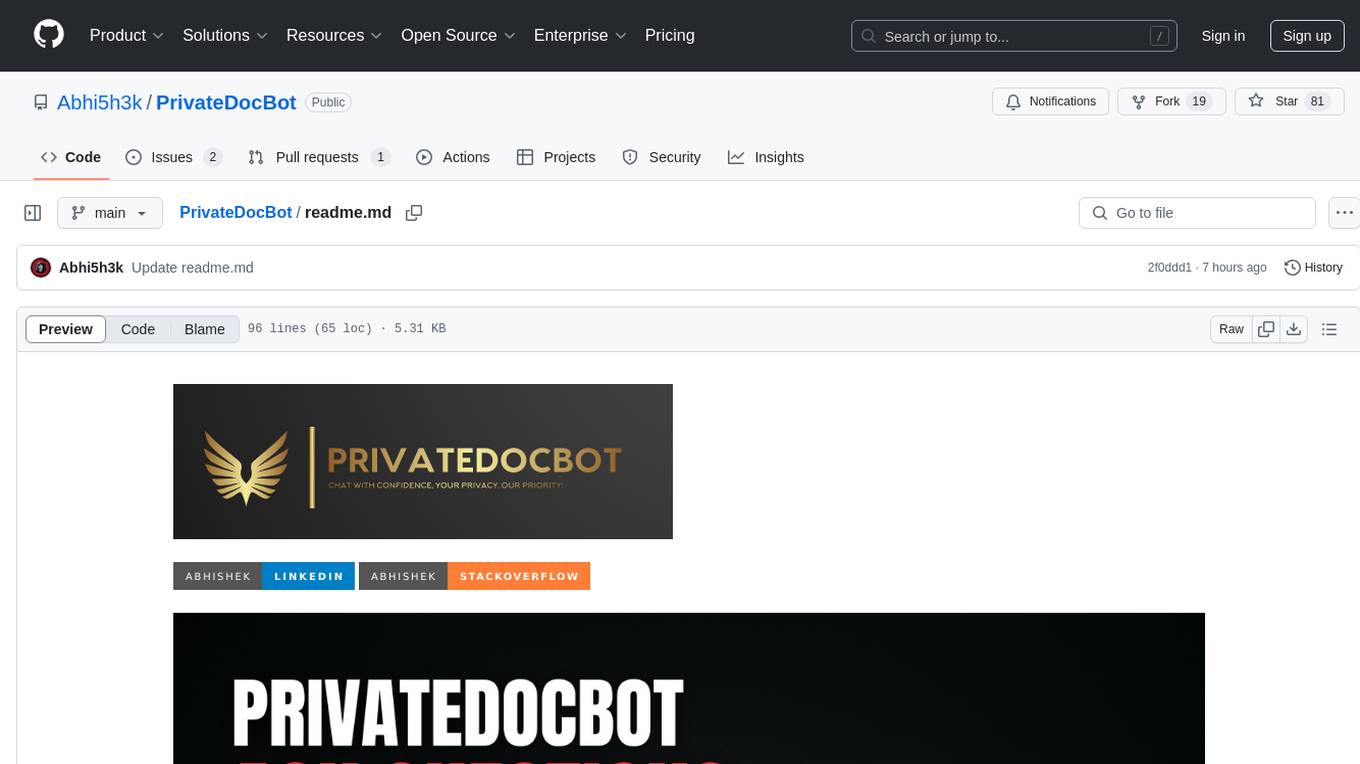
PrivateDocBot is a local LLM-powered chatbot designed for secure document interactions. It seamlessly merges Chainlit user-friendly interface with localized language models, tailored for sensitive data. The project streamlines data access by deciphering intricate user guides and extracting vital insights from complex PDF reports. Equipped with advanced technology, it offers an engaging conversational experience, redefining data interaction and empowering users with control.
README:
Discover the innovation of this chatbot project, seamlessly merging Chainlit user-friendly interface with localized language models 🌐. Tailored for sensitive data, it's a vital asset for both organizations and individuals. From deciphering intricate user guides to extracting vital insights from complex PDF reports, this project streamlines data access.
Equipped with advanced technology, it offers an engaging conversational experience. It redefines data interaction, empowering you with control.
- Effortless Research: Swiftly extract insights from intricate reports for informed decisions.
- Enhanced Productivity: Businesses streamline workflows by navigating user manuals seamlessly.
- Precise Insights: Extract vital data from complex financial reports efficiently.
- Data Confidentiality: Process sensitive data locally for utmost security.
- Personalized Access: Instantly retrieve essential information from a variety of PDF documents.
- Engaging Learning: Educational institutions offer interactive study material access.
Make sure you have the following installed:
- Python 3.11.0
- pip 23.2.1
- To utilize the online database, you can simply comment out the line
database = "local"
in theconfig.toml
file. This will ensure that the necessary dependencies are installed. - Please note that for the local mode, a functional Node.js installation is essential as it relies on the Prisma CLI for its operation. You can find more details about setting up local persistence in the Chainlit documentation.
-
Clone the project repository using Git.
-
Download the necessary model from HuggingFace by visiting the following link: Download Llama Model. Once downloaded, move the model file to the "models" directory.
- also tested with
llama-2-7b-chat.ggmlv3.q2_K.bin
andmistral-7b-openorca.Q4_K_M.gguf
- ctransformers Now Support GGUF format support for Llama and Falcon models.
- Models
- also tested with
-
Install the required Python packages by running the following command:
pip install -r requirements.txt
-
Place your PDF document in the "data" directory. You can choose the appropriate document loader from the available options to match your requirements. Refer to Document Loaders for more information. Note that the current implementation is designed for PDF documents.
-
Launch the application using the following command:
chainlit run main.py -w
-
For the initial setup, it's essential to build the vector database. Click on the "Rebuild vector" to initiate this process.
-
With the setup complete, you can now ask pertinent questions related to your PDF document. Input your queries and receive informative responses.
Follow these instructions meticulously to ensure seamless functionality and accurate outcomes. Experience the convenience of easily querying your PDF office data for insightful insights!
To modify the welcome screen, edit the chainlit.md
file at the root of your project. If you do not want a welcome screen, just leave this file empty.
pip install langchain[all]
pip install --upgrade langchain
pip install --upgrade python-box
pip install pypdf
pip install -U sentence-transformers
python -m pip install --upgrade pip
pip install ctransformers
pip install chainlit
Inference speed will depend upon CPU cores and avaialble RAM. It's recomended to have multi core CPU (Laptop / PC) with atleast 16GB RAM. You can deploy it on Server for better performance.
Tested on :
- Windows 10
- i7-10510U CPU @ 1.80GHz
- cores : 4
- RAM : 16GB
- Try adding memory
- Increase performance by using https://www.ray.io/ or something similar
- Upgrade to low latency Vector DB
- Add caching
Check Another LLM Project : 🔐 LLQuery : Your Conversational SQL Bridge
For Tasks:
Click tags to check more tools for each tasksFor Jobs:
Alternative AI tools for PrivateDocBot
Similar Open Source Tools
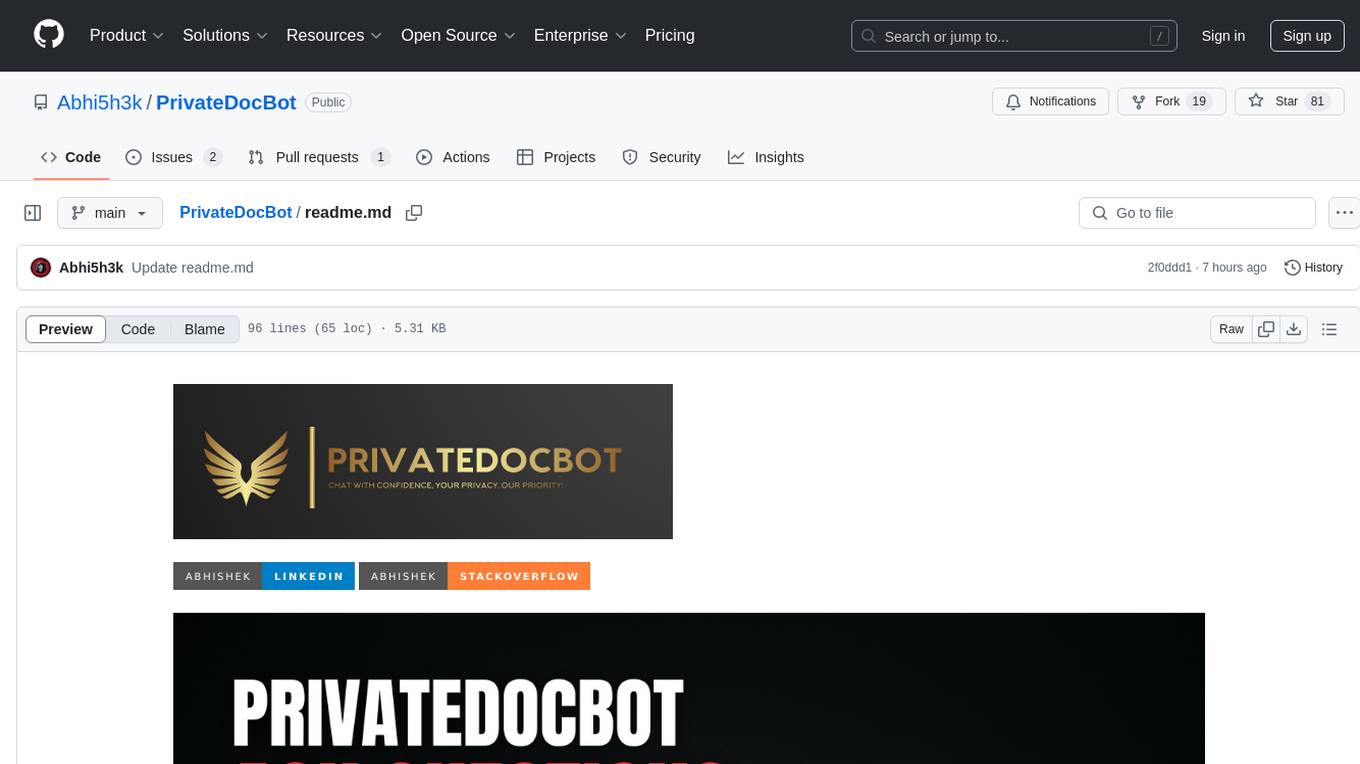
PrivateDocBot
PrivateDocBot is a local LLM-powered chatbot designed for secure document interactions. It seamlessly merges Chainlit user-friendly interface with localized language models, tailored for sensitive data. The project streamlines data access by deciphering intricate user guides and extracting vital insights from complex PDF reports. Equipped with advanced technology, it offers an engaging conversational experience, redefining data interaction and empowering users with control.
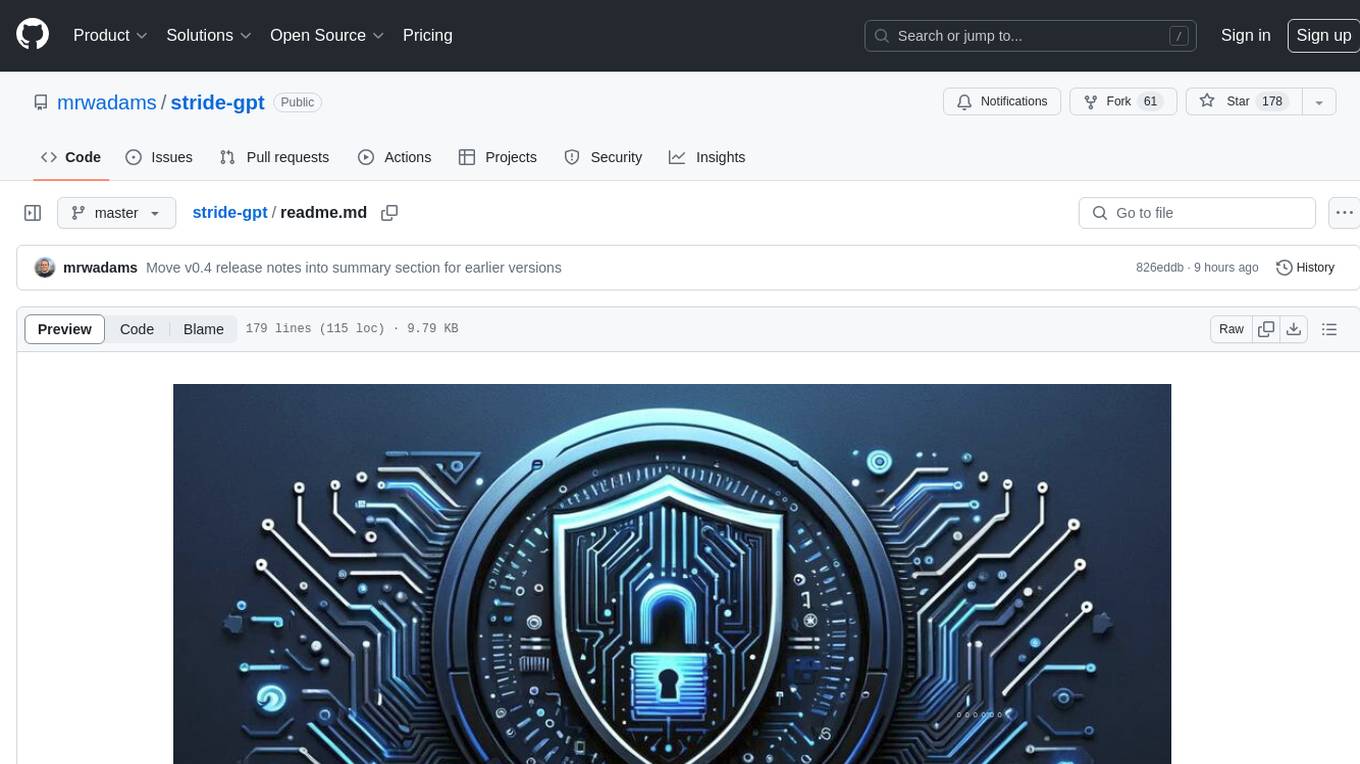
stride-gpt
STRIDE GPT is an AI-powered threat modelling tool that leverages Large Language Models (LLMs) to generate threat models and attack trees for a given application based on the STRIDE methodology. Users provide application details, such as the application type, authentication methods, and whether the application is internet-facing or processes sensitive data. The model then generates its output based on the provided information. It features a simple and user-friendly interface, supports multi-modal threat modelling, generates attack trees, suggests possible mitigations for identified threats, and does not store application details. STRIDE GPT can be accessed via OpenAI API, Azure OpenAI Service, Google AI API, or Mistral API. It is available as a Docker container image for easy deployment.
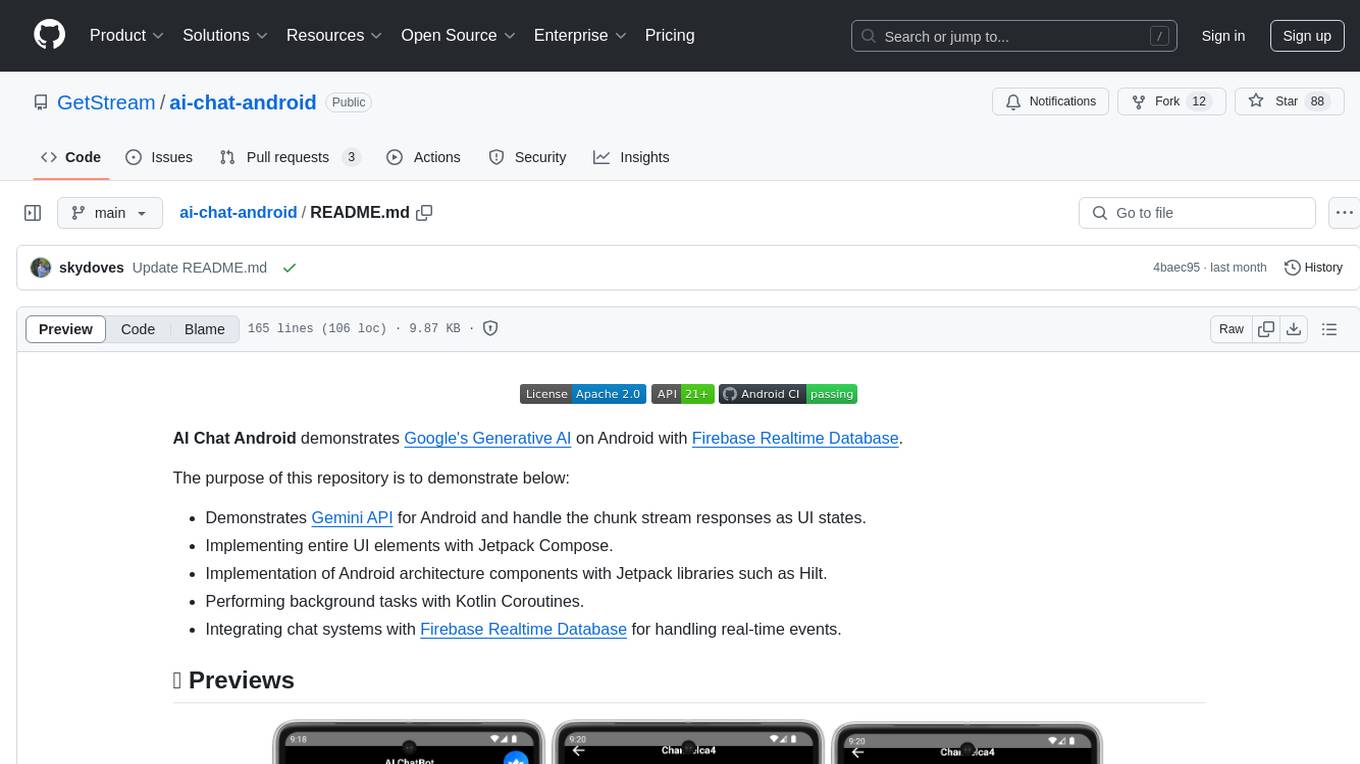
ai-chat-android
AI Chat Android demonstrates Google's Generative AI on Android with Firebase Realtime Database. It showcases Gemini API integration, Jetpack Compose UI elements, Android architecture components with Hilt, Kotlin Coroutines for background tasks, and Firebase Realtime Database integration for real-time events. The project follows Google's official architecture guidance with a modularized structure for reusability, parallel building, and decentralized focusing.
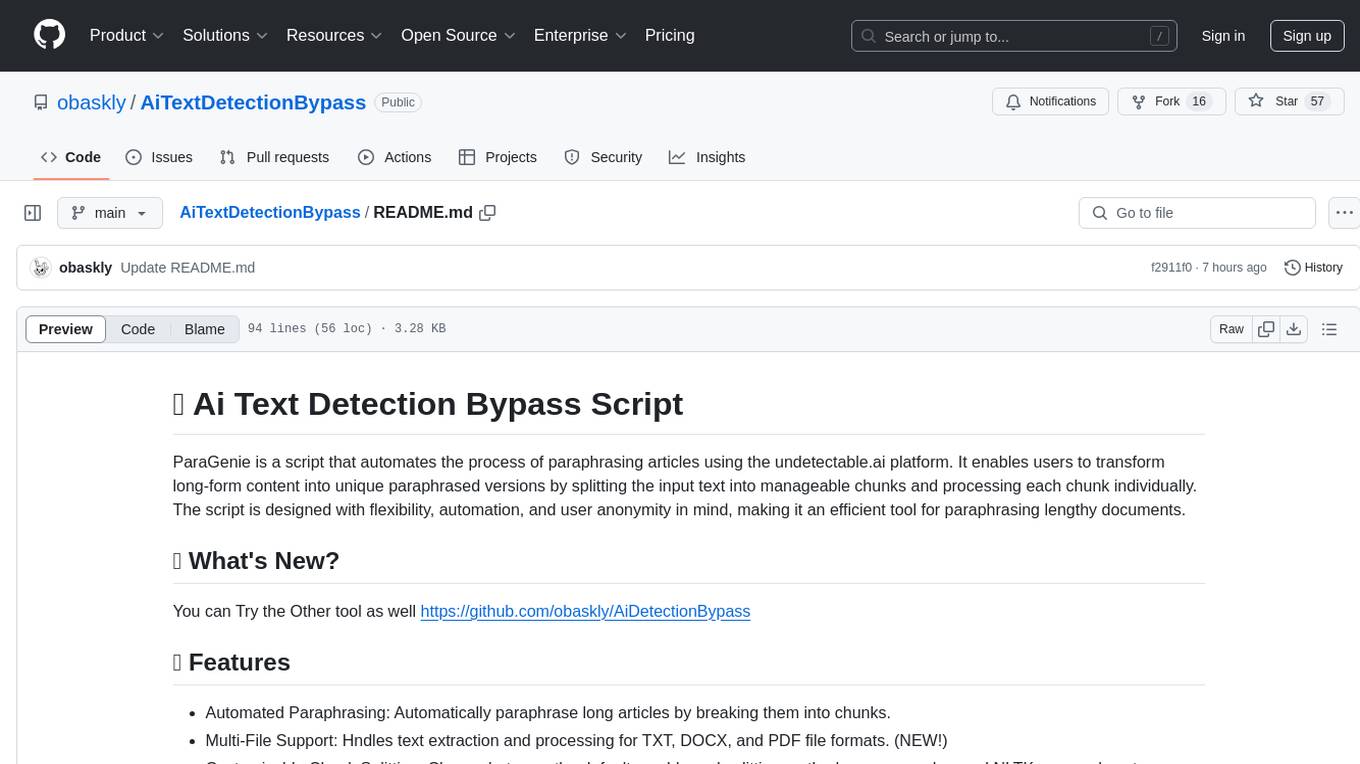
AiTextDetectionBypass
ParaGenie is a script designed to automate the process of paraphrasing articles using the undetectable.ai platform. It allows users to convert lengthy content into unique paraphrased versions by splitting the input text into manageable chunks and processing each chunk individually. The script offers features such as automated paraphrasing, multi-file support for TXT, DOCX, and PDF formats, customizable chunk splitting methods, Gmail-based registration for seamless paraphrasing, purpose-specific writing support, readability level customization, anonymity features for user privacy, error handling and recovery, and output management for easy access and organization of paraphrased content.
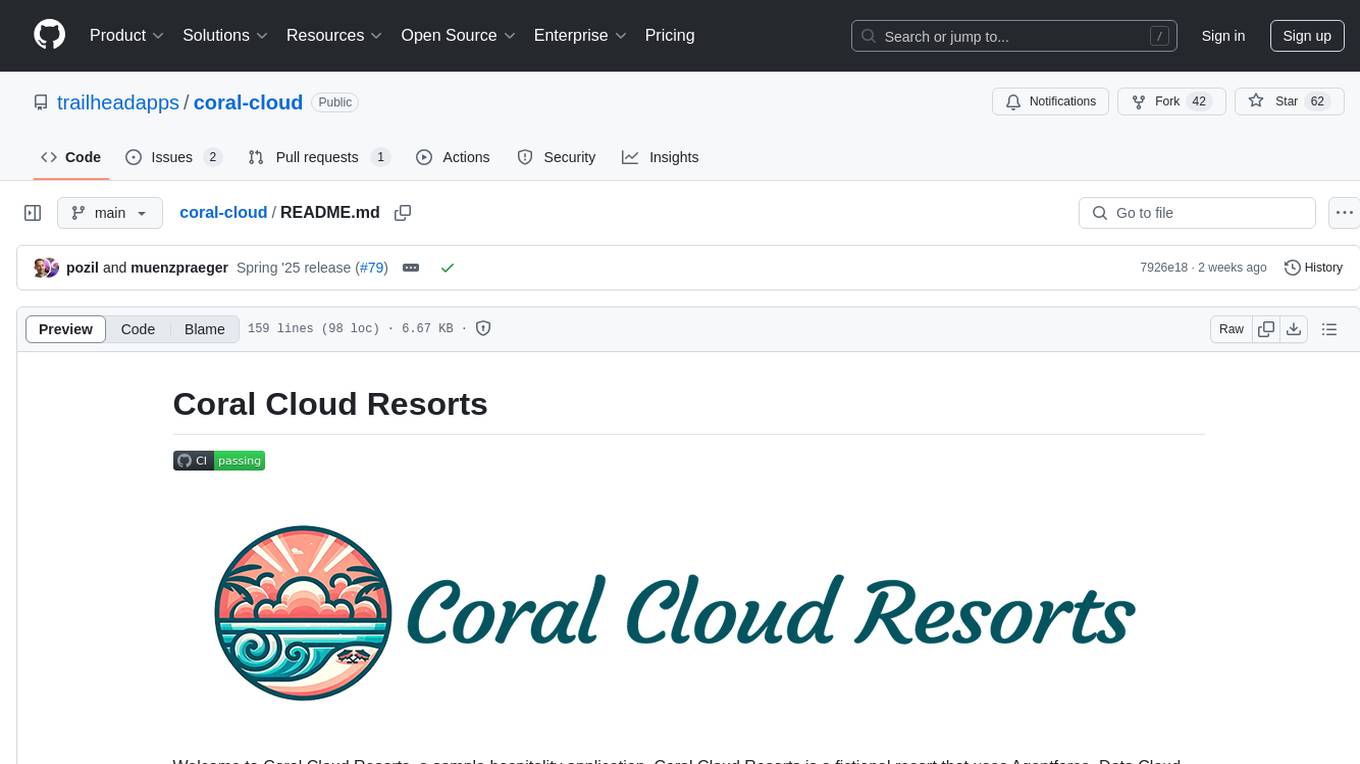
coral-cloud
Coral Cloud Resorts is a sample hospitality application that showcases Data Cloud, Agents, and Prompts. It provides highly personalized guest experiences through smart automation, content generation, and summarization. The app requires licenses for Data Cloud, Agents, Prompt Builder, and Einstein for Sales. Users can activate features, deploy metadata, assign permission sets, import sample data, and troubleshoot common issues. Additionally, the repository offers integration with modern web development tools like Prettier, ESLint, and pre-commit hooks for code formatting and linting.
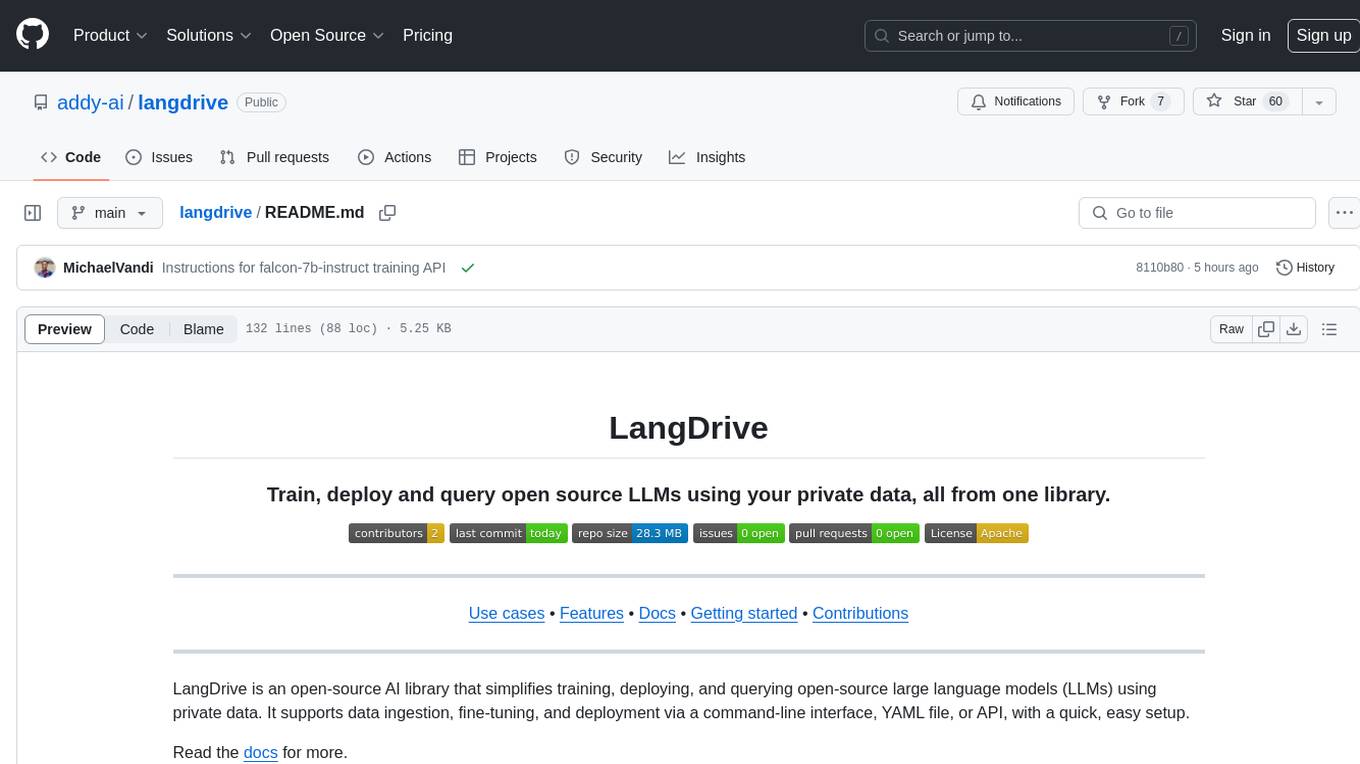
langdrive
LangDrive is an open-source AI library that simplifies training, deploying, and querying open-source large language models (LLMs) using private data. It supports data ingestion, fine-tuning, and deployment via a command-line interface, YAML file, or API, with a quick, easy setup. Users can build AI applications such as question/answering systems, chatbots, AI agents, and content generators. The library provides features like data connectors for ingestion, fine-tuning of LLMs, deployment to Hugging Face hub, inference querying, data utilities for CRUD operations, and APIs for model access. LangDrive is designed to streamline the process of working with LLMs and making AI development more accessible.
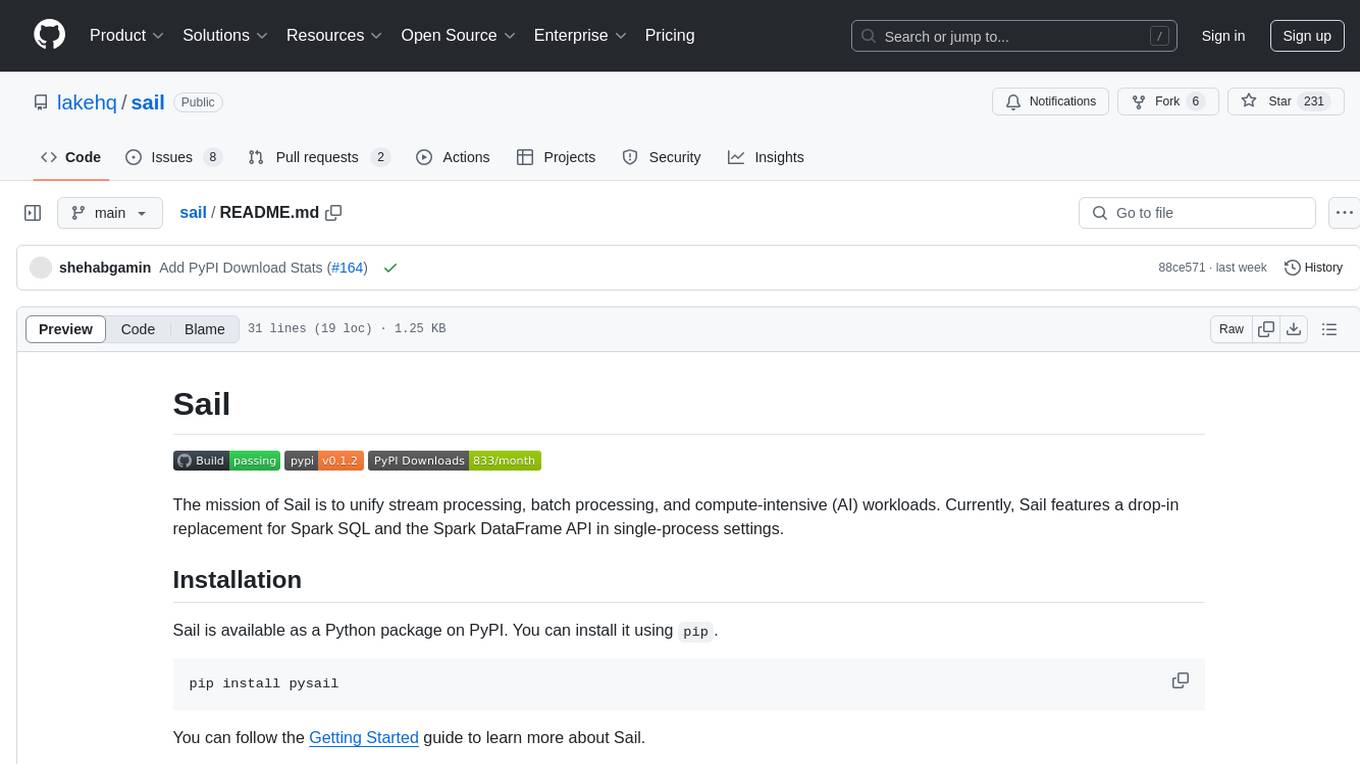
sail
Sail is a tool designed to unify stream processing, batch processing, and compute-intensive workloads, serving as a drop-in replacement for Spark SQL and the Spark DataFrame API in single-process settings. It aims to streamline data processing tasks and facilitate AI workloads.
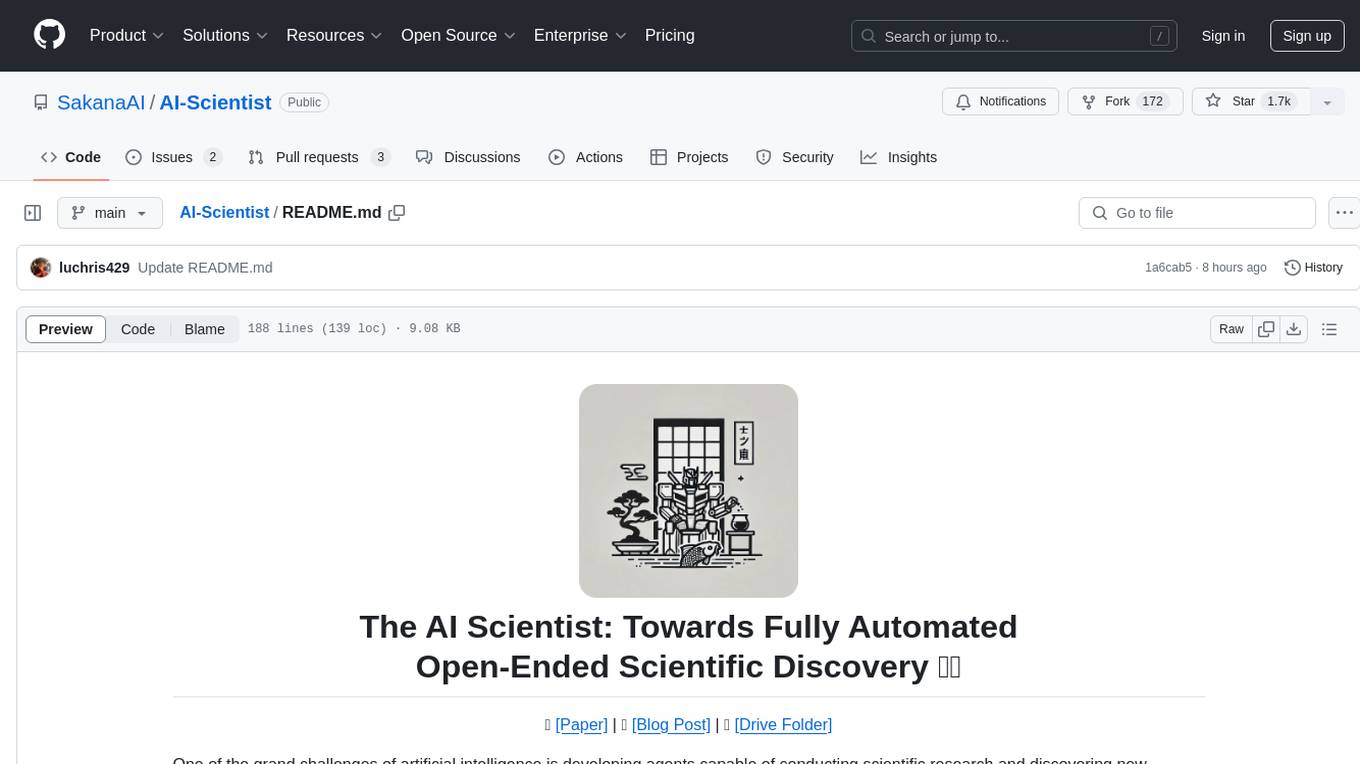
AI-Scientist
The AI Scientist is a comprehensive system for fully automatic scientific discovery, enabling Foundation Models to perform research independently. It aims to tackle the grand challenge of developing agents capable of conducting scientific research and discovering new knowledge. The tool generates papers on various topics using Large Language Models (LLMs) and provides a platform for exploring new research ideas. Users can create their own templates for specific areas of study and run experiments to generate papers. However, caution is advised as the codebase executes LLM-written code, which may pose risks such as the use of potentially dangerous packages and web access.
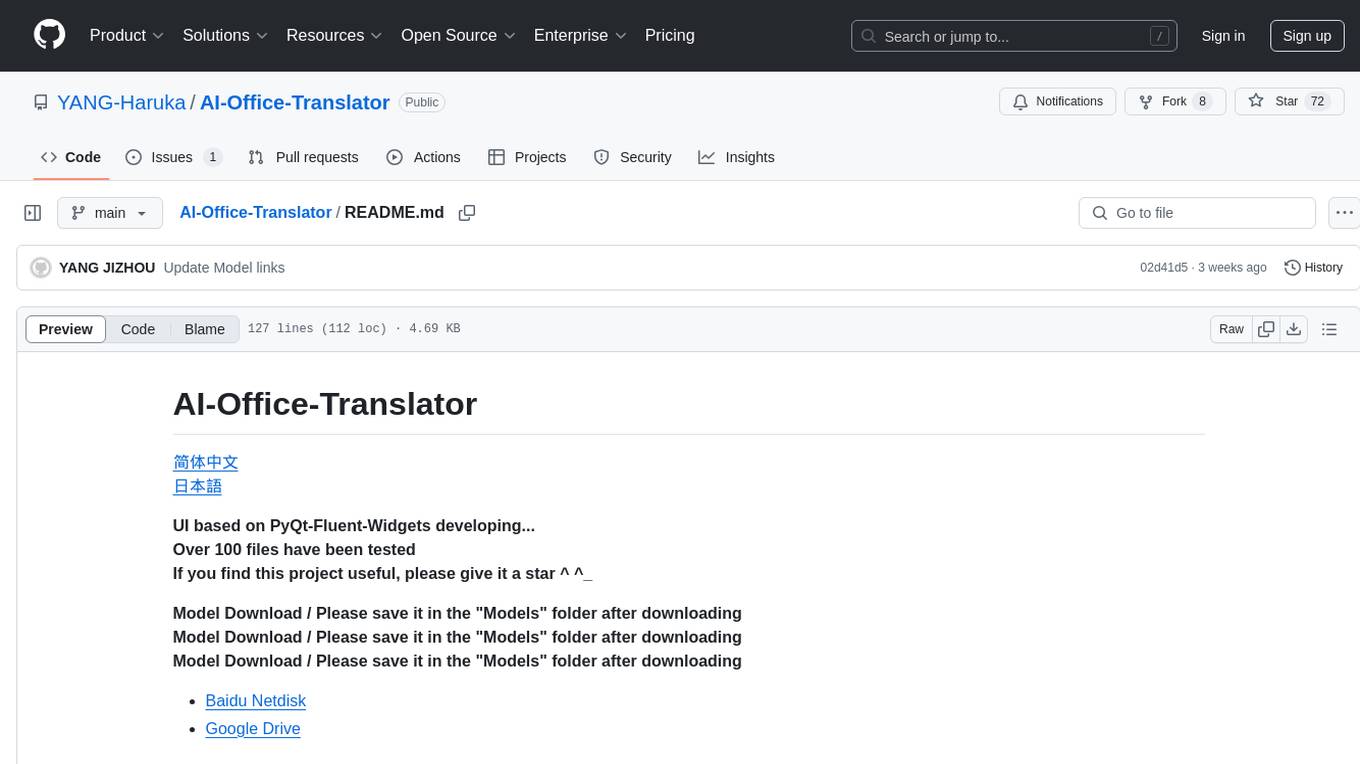
AI-Office-Translator
AI-Office-Translator is a free, fully localized, user-friendly translation tool that helps you translate Office files (Word, PowerPoint, and Excel) between different languages. It supports .docx, .pptx, and .xlsx files and allows translation between English, Chinese, and Japanese. Users can run the tool after installing CUDA, downloading Ollama dependencies and models, setting up a virtual environment (optional), and installing requirements. The tool provides a UI where users can select languages, models, upload files for translation, start translation, and download translated files. It also supports an online mode with API key integration. The software is open-source under GPL-3.0 license and only provides AI translation services, with users expected to engage in legal translation activities.
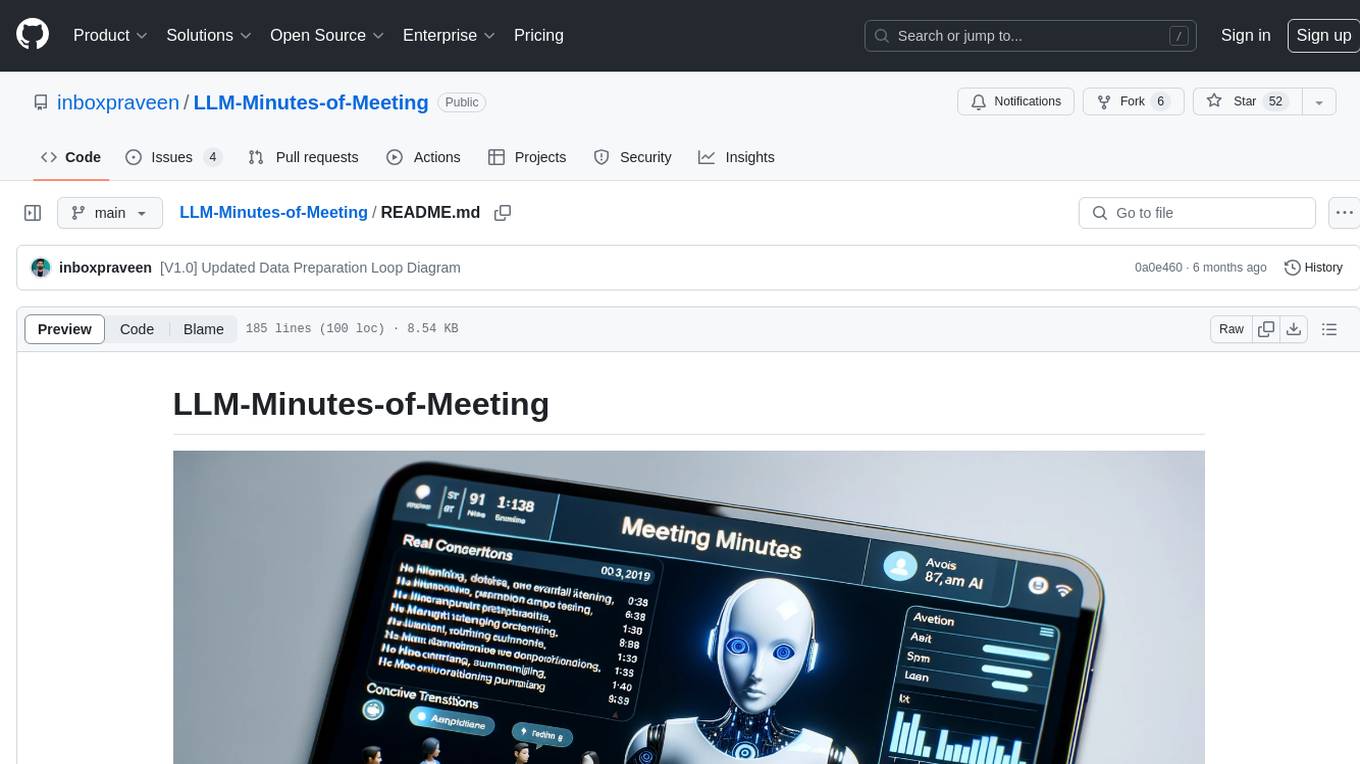
LLM-Minutes-of-Meeting
LLM-Minutes-of-Meeting is a project showcasing NLP & LLM's capability to summarize long meetings and automate the task of delegating Minutes of Meeting(MoM) emails. It converts audio/video files to text, generates editable MoM, and aims to develop a real-time python web-application for meeting automation. The tool features keyword highlighting, topic tagging, export in various formats, user-friendly interface, and uses Celery for asynchronous processing. It is designed for corporate meetings, educational institutions, legal and medical fields, accessibility, and event coverage.
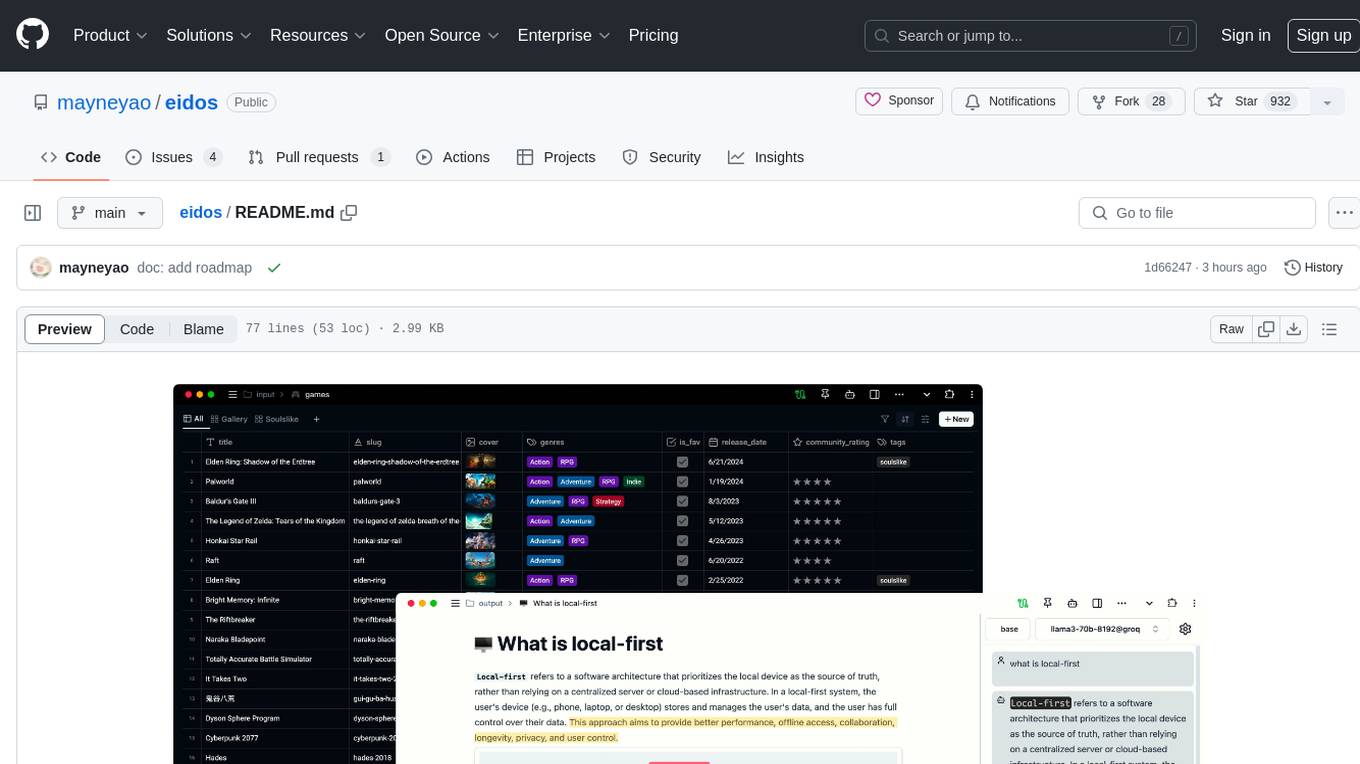
eidos
Eidos is an extensible framework for managing personal data in one place. It runs inside the browser as a PWA with offline support. It integrates AI features for translation, summarization, and data interaction. Users can customize Eidos with Prompt extension, JavaScript for Formula functions, TypeScript/JavaScript for data processing logic, and build apps using any framework. Eidos is developer-friendly with API & SDK, and uses SQLite standardization for data tables.
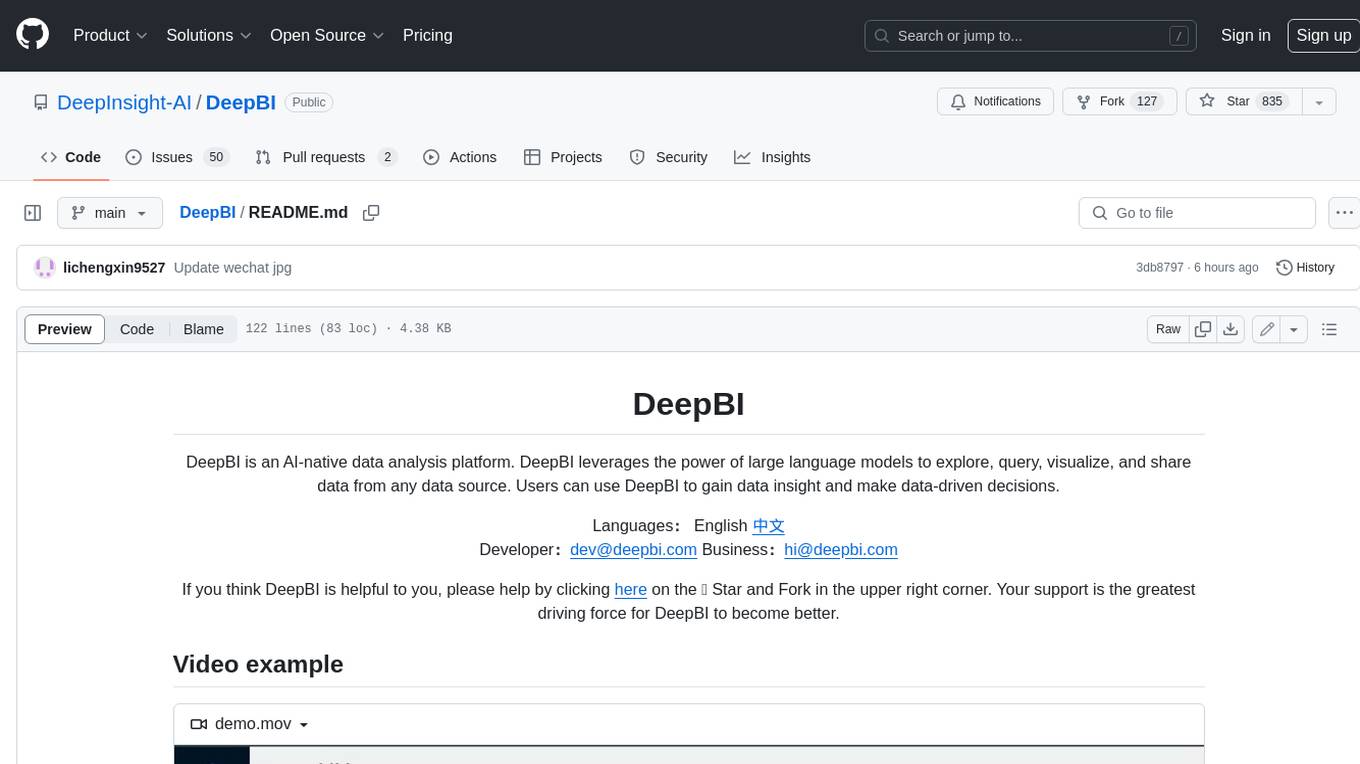
DeepBI
DeepBI is an AI-native data analysis platform that leverages the power of large language models to explore, query, visualize, and share data from any data source. Users can use DeepBI to gain data insight and make data-driven decisions.
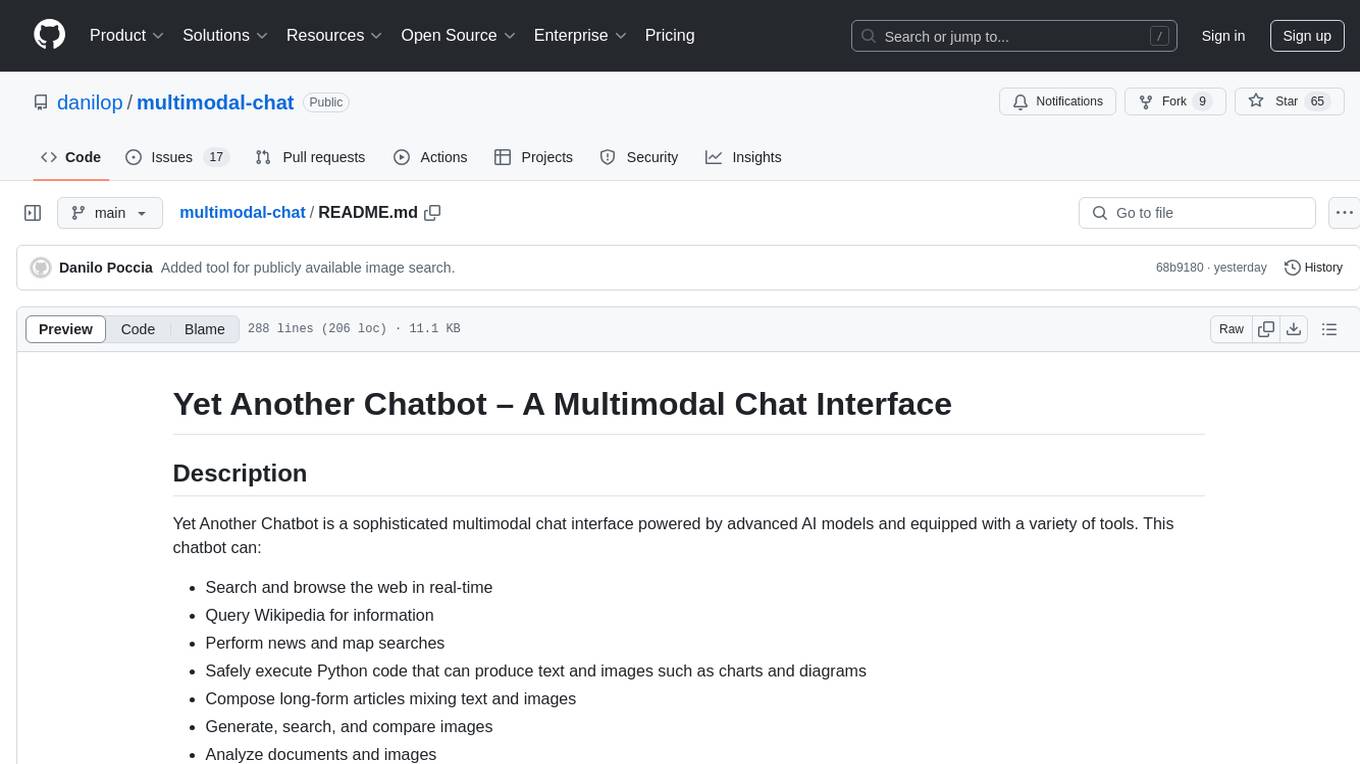
multimodal-chat
Yet Another Chatbot is a sophisticated multimodal chat interface powered by advanced AI models and equipped with a variety of tools. This chatbot can search and browse the web in real-time, query Wikipedia for information, perform news and map searches, execute Python code, compose long-form articles mixing text and images, generate, search, and compare images, analyze documents and images, search and download arXiv papers, save conversations as text and audio files, manage checklists, and track personal improvements. It offers tools for web interaction, Wikipedia search, Python scripting, content management, image handling, arXiv integration, conversation generation, file management, personal improvement, and checklist management.
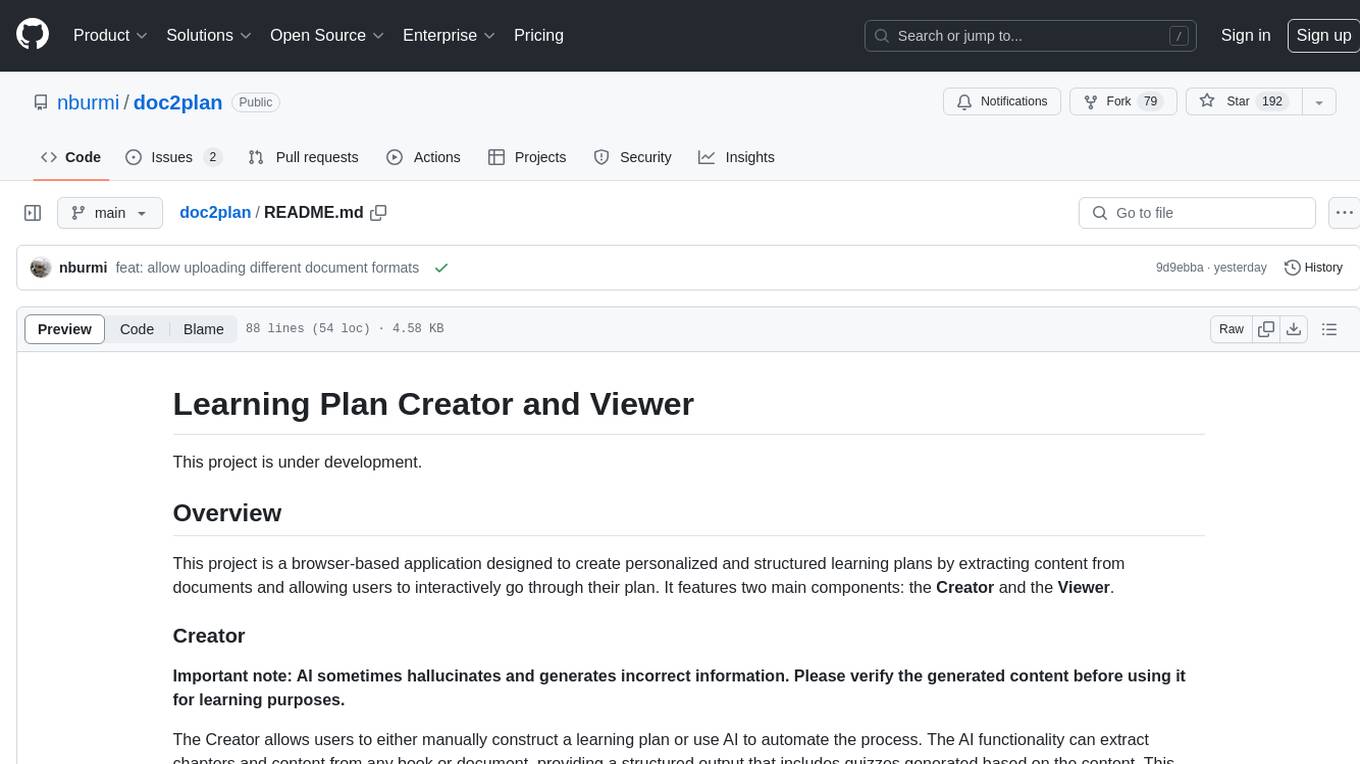
doc2plan
doc2plan is a browser-based application that helps users create personalized learning plans by extracting content from documents. It features a Creator for manual or AI-assisted plan construction and a Viewer for interactive plan navigation. Users can extract chapters, key topics, generate quizzes, and track progress. The application includes AI-driven content extraction, quiz generation, progress tracking, plan import/export, assistant management, customizable settings, viewer chat with text-to-speech and speech-to-text support, and integration with various Retrieval-Augmented Generation (RAG) models. It aims to simplify the creation of comprehensive learning modules tailored to individual needs.
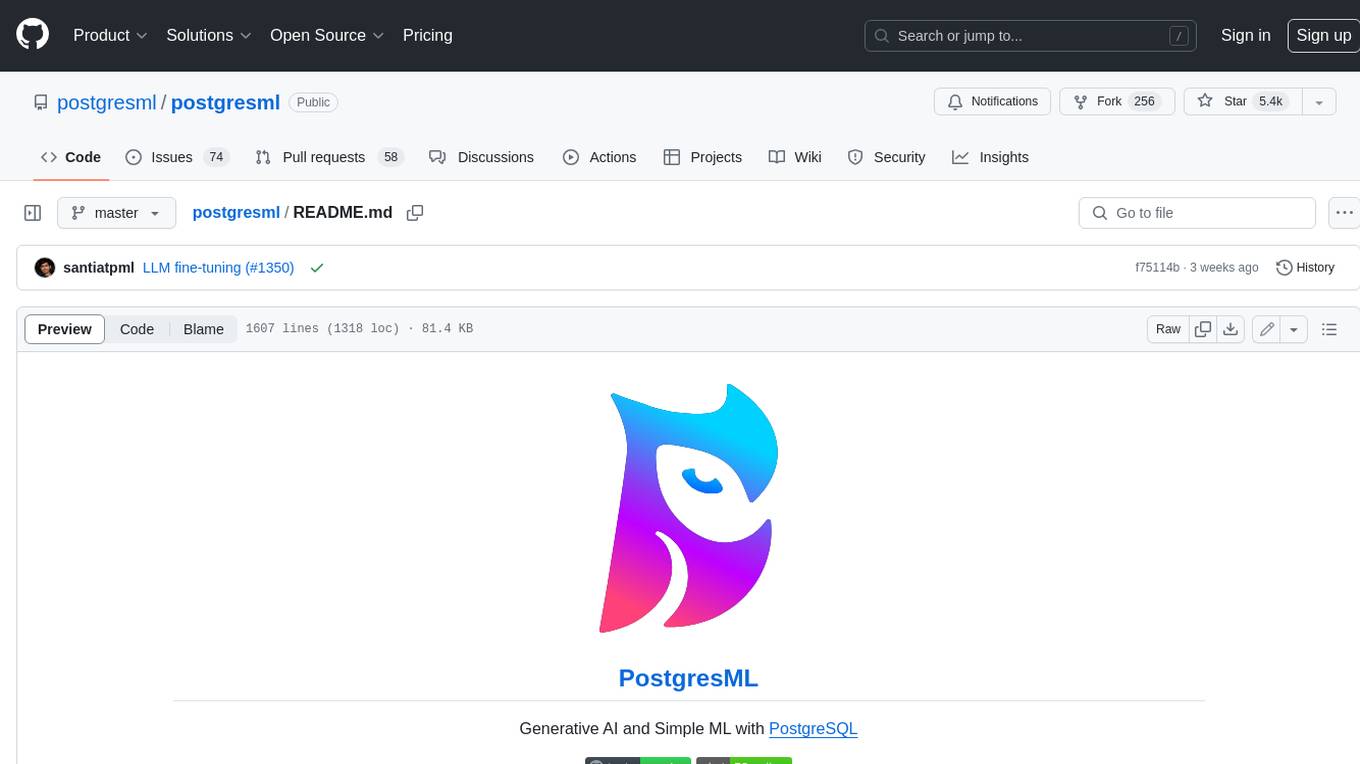
postgresml
PostgresML is a powerful Postgres extension that seamlessly combines data storage and machine learning inference within your database. It enables running machine learning and AI operations directly within PostgreSQL, leveraging GPU acceleration for faster computations, integrating state-of-the-art large language models, providing built-in functions for text processing, enabling efficient similarity search, offering diverse ML algorithms, ensuring high performance, scalability, and security, supporting a wide range of NLP tasks, and seamlessly integrating with existing PostgreSQL tools and client libraries.
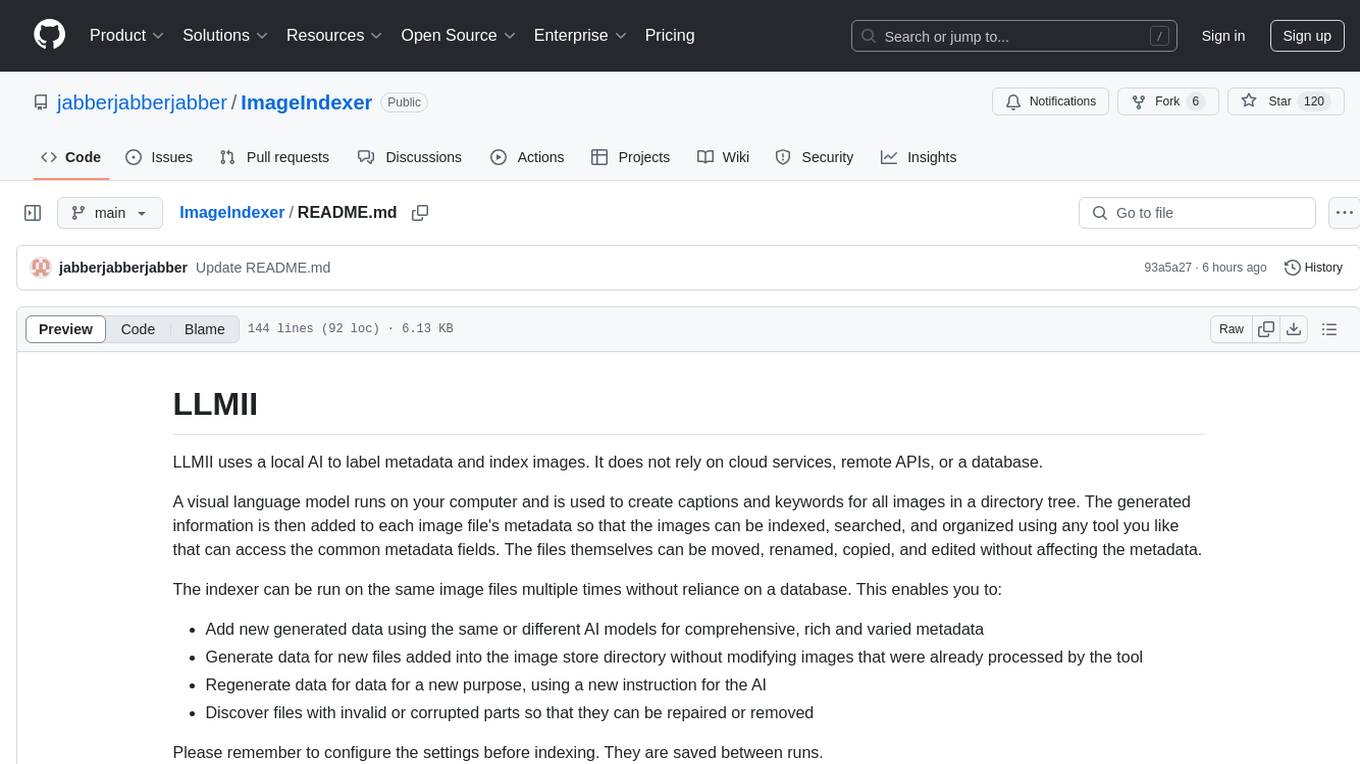
ImageIndexer
LLMII is a tool that uses a local AI model to label metadata and index images without relying on cloud services or remote APIs. It runs a visual language model on your computer to generate captions and keywords for images, enhancing their metadata for indexing, searching, and organization. The tool can be run multiple times on the same image files, allowing for adding new data, regenerating data, and discovering files with issues. It supports various image formats, offers a user-friendly GUI, and can utilize GPU acceleration for faster processing. LLMII requires Python 3.8 or higher and operates directly on image file metadata fields like MWG:Keyword and XMP:Identifier.
For similar tasks
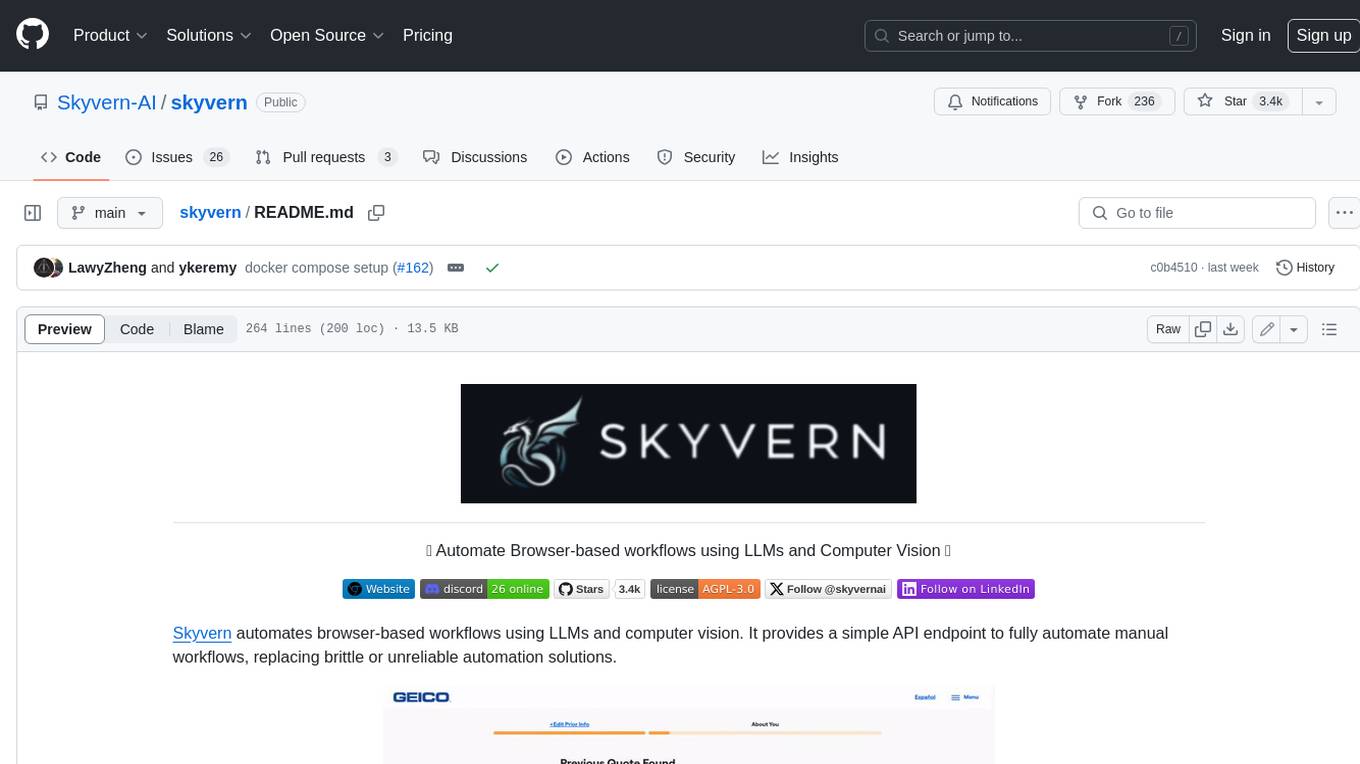
skyvern
Skyvern automates browser-based workflows using LLMs and computer vision. It provides a simple API endpoint to fully automate manual workflows, replacing brittle or unreliable automation solutions. Traditional approaches to browser automations required writing custom scripts for websites, often relying on DOM parsing and XPath-based interactions which would break whenever the website layouts changed. Instead of only relying on code-defined XPath interactions, Skyvern adds computer vision and LLMs to the mix to parse items in the viewport in real-time, create a plan for interaction and interact with them. This approach gives us a few advantages: 1. Skyvern can operate on websites it’s never seen before, as it’s able to map visual elements to actions necessary to complete a workflow, without any customized code 2. Skyvern is resistant to website layout changes, as there are no pre-determined XPaths or other selectors our system is looking for while trying to navigate 3. Skyvern leverages LLMs to reason through interactions to ensure we can cover complex situations. Examples include: 1. If you wanted to get an auto insurance quote from Geico, the answer to a common question “Were you eligible to drive at 18?” could be inferred from the driver receiving their license at age 16 2. If you were doing competitor analysis, it’s understanding that an Arnold Palmer 22 oz can at 7/11 is almost definitely the same product as a 23 oz can at Gopuff (even though the sizes are slightly different, which could be a rounding error!) Want to see examples of Skyvern in action? Jump to #real-world-examples-of- skyvern
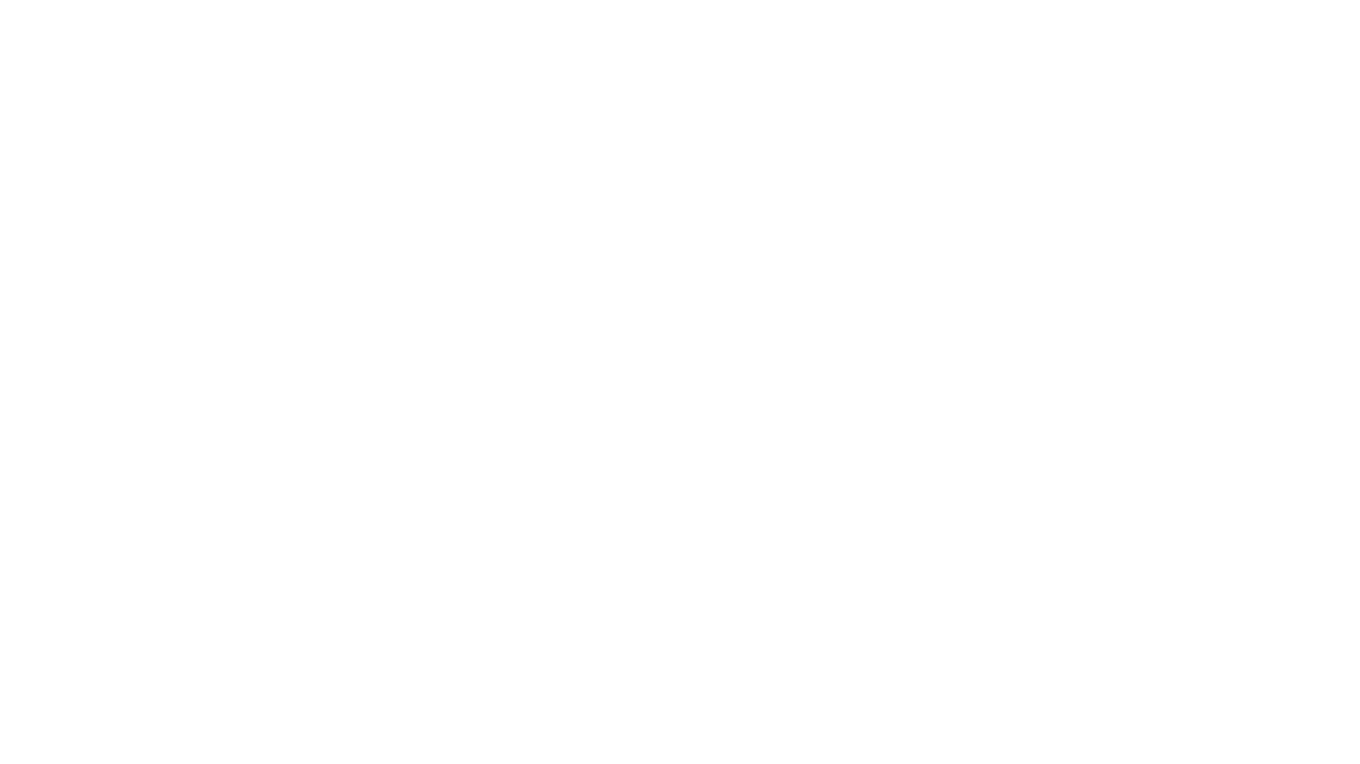
airbyte-connectors
This repository contains Airbyte connectors used in Faros and Faros Community Edition platforms as well as Airbyte Connector Development Kit (CDK) for JavaScript/TypeScript.
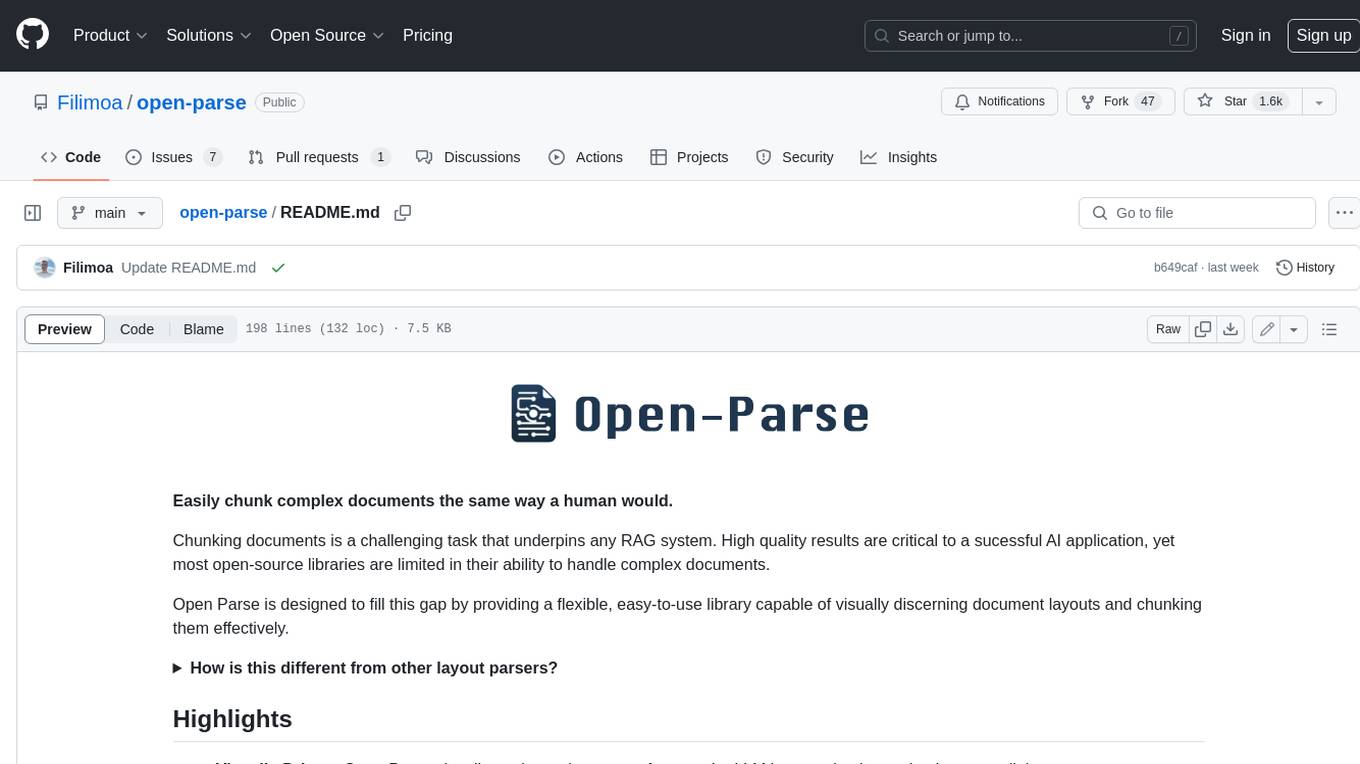
open-parse
Open Parse is a Python library for visually discerning document layouts and chunking them effectively. It is designed to fill the gap in open-source libraries for handling complex documents. Unlike text splitting, which converts a file to raw text and slices it up, Open Parse visually analyzes documents for superior LLM input. It also supports basic markdown for parsing headings, bold, and italics, and has high-precision table support, extracting tables into clean Markdown formats with accuracy that surpasses traditional tools. Open Parse is extensible, allowing users to easily implement their own post-processing steps. It is also intuitive, with great editor support and completion everywhere, making it easy to use and learn.
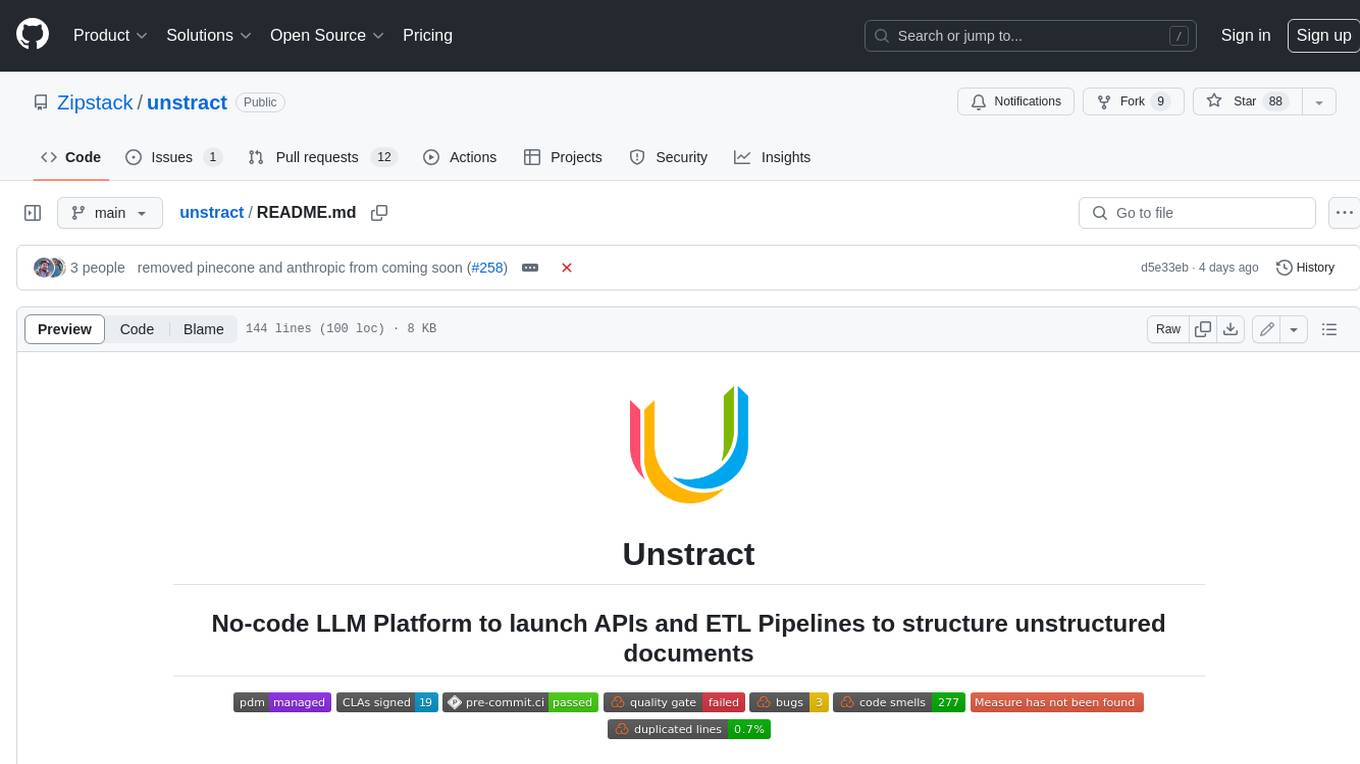
unstract
Unstract is a no-code platform that enables users to launch APIs and ETL pipelines to structure unstructured documents. With Unstract, users can go beyond co-pilots by enabling machine-to-machine automation. Unstract's Prompt Studio provides a simple, no-code approach to creating prompts for LLMs, vector databases, embedding models, and text extractors. Users can then configure Prompt Studio projects as API deployments or ETL pipelines to automate critical business processes that involve complex documents. Unstract supports a wide range of LLM providers, vector databases, embeddings, text extractors, ETL sources, and ETL destinations, providing users with the flexibility to choose the best tools for their needs.
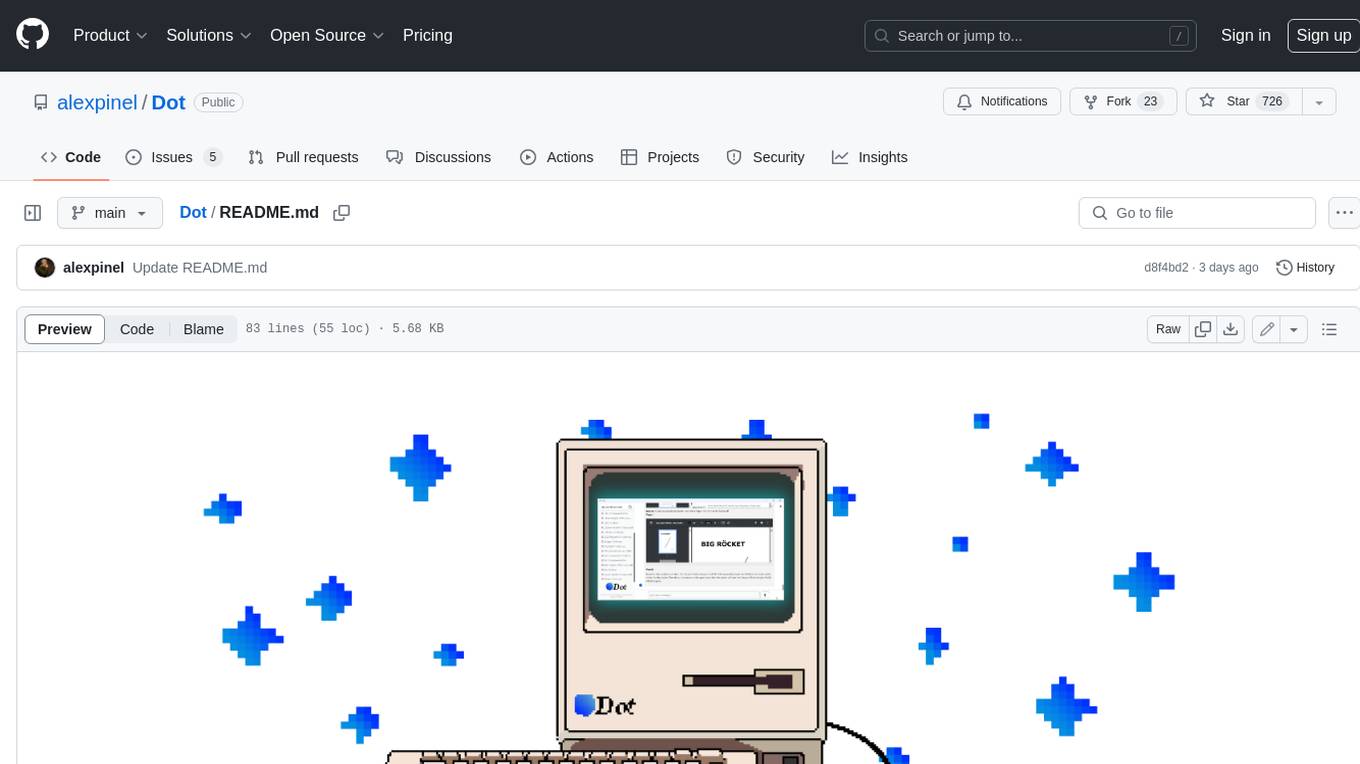
Dot
Dot is a standalone, open-source application designed for seamless interaction with documents and files using local LLMs and Retrieval Augmented Generation (RAG). It is inspired by solutions like Nvidia's Chat with RTX, providing a user-friendly interface for those without a programming background. Pre-packaged with Mistral 7B, Dot ensures accessibility and simplicity right out of the box. Dot allows you to load multiple documents into an LLM and interact with them in a fully local environment. Supported document types include PDF, DOCX, PPTX, XLSX, and Markdown. Users can also engage with Big Dot for inquiries not directly related to their documents, similar to interacting with ChatGPT. Built with Electron JS, Dot encapsulates a comprehensive Python environment that includes all necessary libraries. The application leverages libraries such as FAISS for creating local vector stores, Langchain, llama.cpp & Huggingface for setting up conversation chains, and additional tools for document management and interaction.
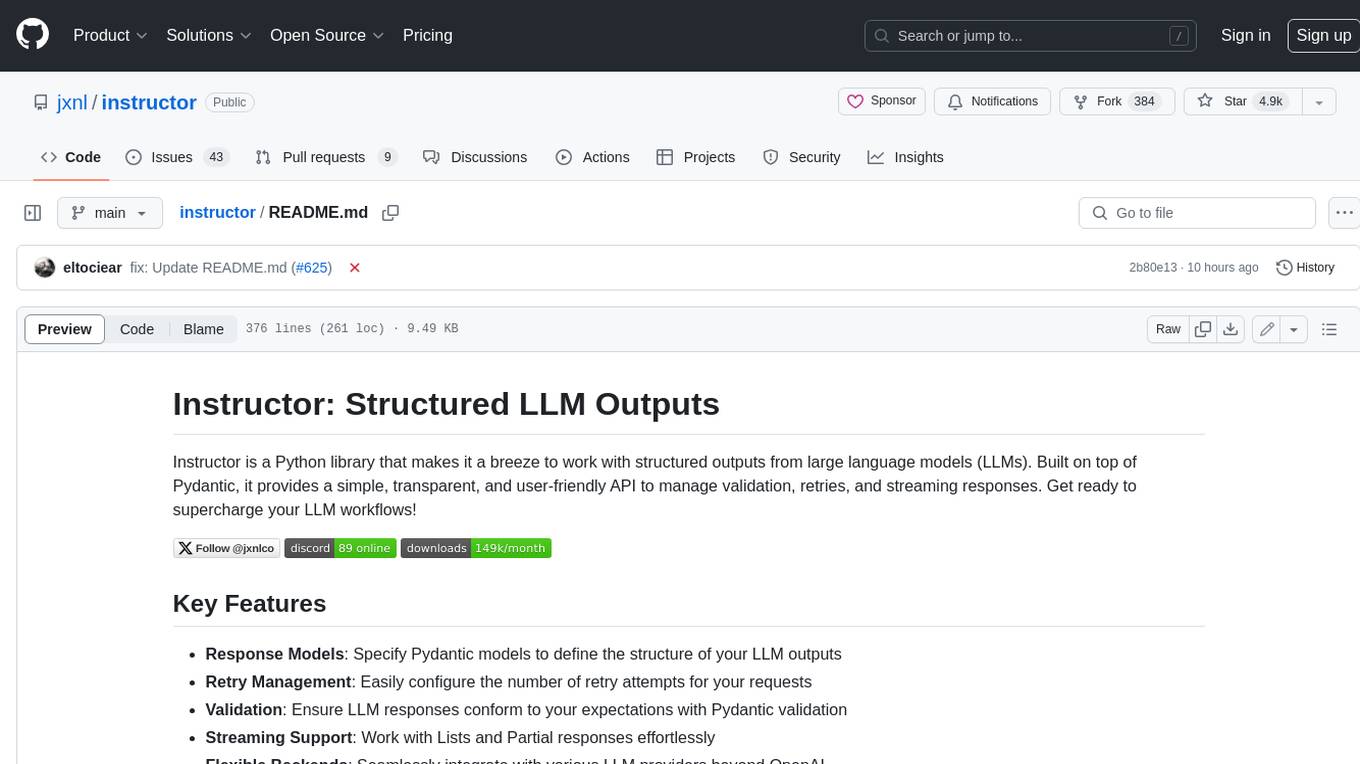
instructor
Instructor is a Python library that makes it a breeze to work with structured outputs from large language models (LLMs). Built on top of Pydantic, it provides a simple, transparent, and user-friendly API to manage validation, retries, and streaming responses. Get ready to supercharge your LLM workflows!
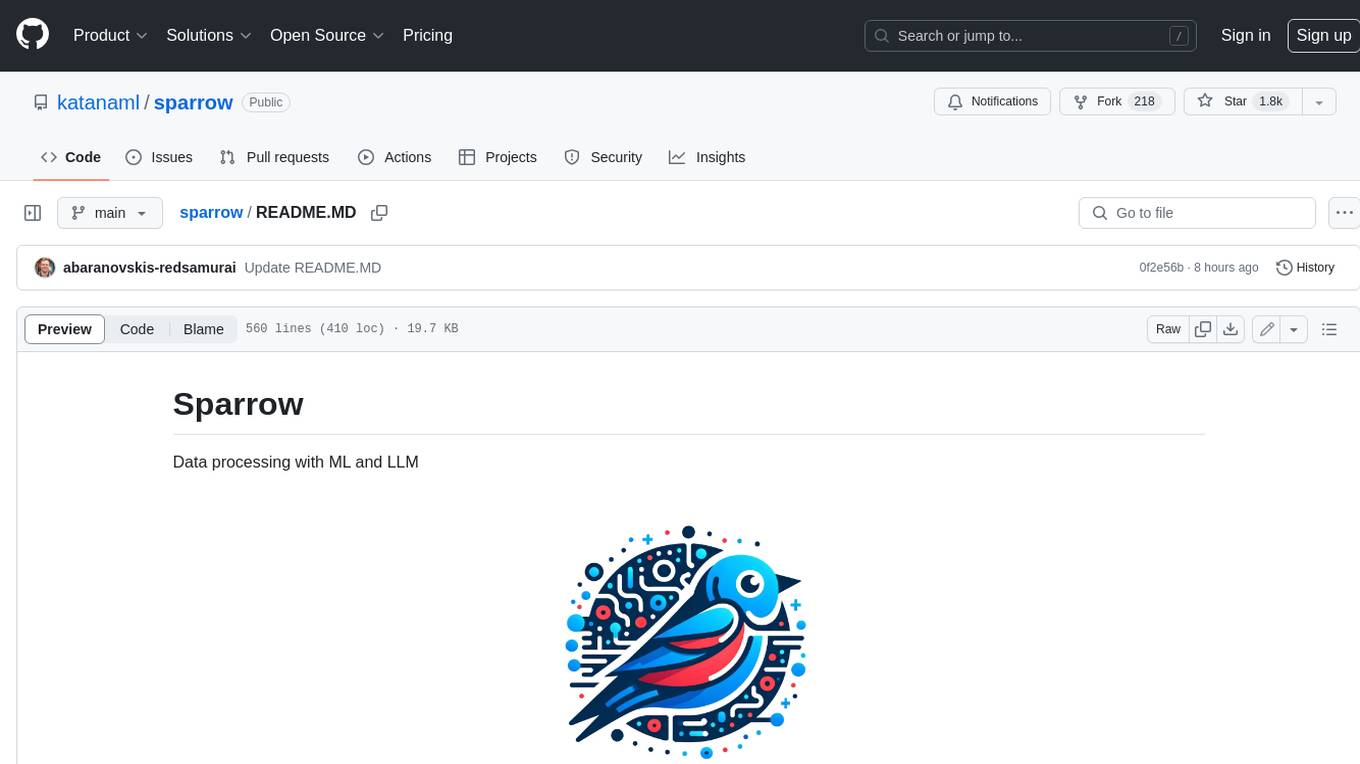
sparrow
Sparrow is an innovative open-source solution for efficient data extraction and processing from various documents and images. It seamlessly handles forms, invoices, receipts, and other unstructured data sources. Sparrow stands out with its modular architecture, offering independent services and pipelines all optimized for robust performance. One of the critical functionalities of Sparrow - pluggable architecture. You can easily integrate and run data extraction pipelines using tools and frameworks like LlamaIndex, Haystack, or Unstructured. Sparrow enables local LLM data extraction pipelines through Ollama or Apple MLX. With Sparrow solution you get API, which helps to process and transform your data into structured output, ready to be integrated with custom workflows. Sparrow Agents - with Sparrow you can build independent LLM agents, and use API to invoke them from your system. **List of available agents:** * **llamaindex** - RAG pipeline with LlamaIndex for PDF processing * **vllamaindex** - RAG pipeline with LLamaIndex multimodal for image processing * **vprocessor** - RAG pipeline with OCR and LlamaIndex for image processing * **haystack** - RAG pipeline with Haystack for PDF processing * **fcall** - Function call pipeline * **unstructured-light** - RAG pipeline with Unstructured and LangChain, supports PDF and image processing * **unstructured** - RAG pipeline with Weaviate vector DB query, Unstructured and LangChain, supports PDF and image processing * **instructor** - RAG pipeline with Unstructured and Instructor libraries, supports PDF and image processing. Works great for JSON response generation
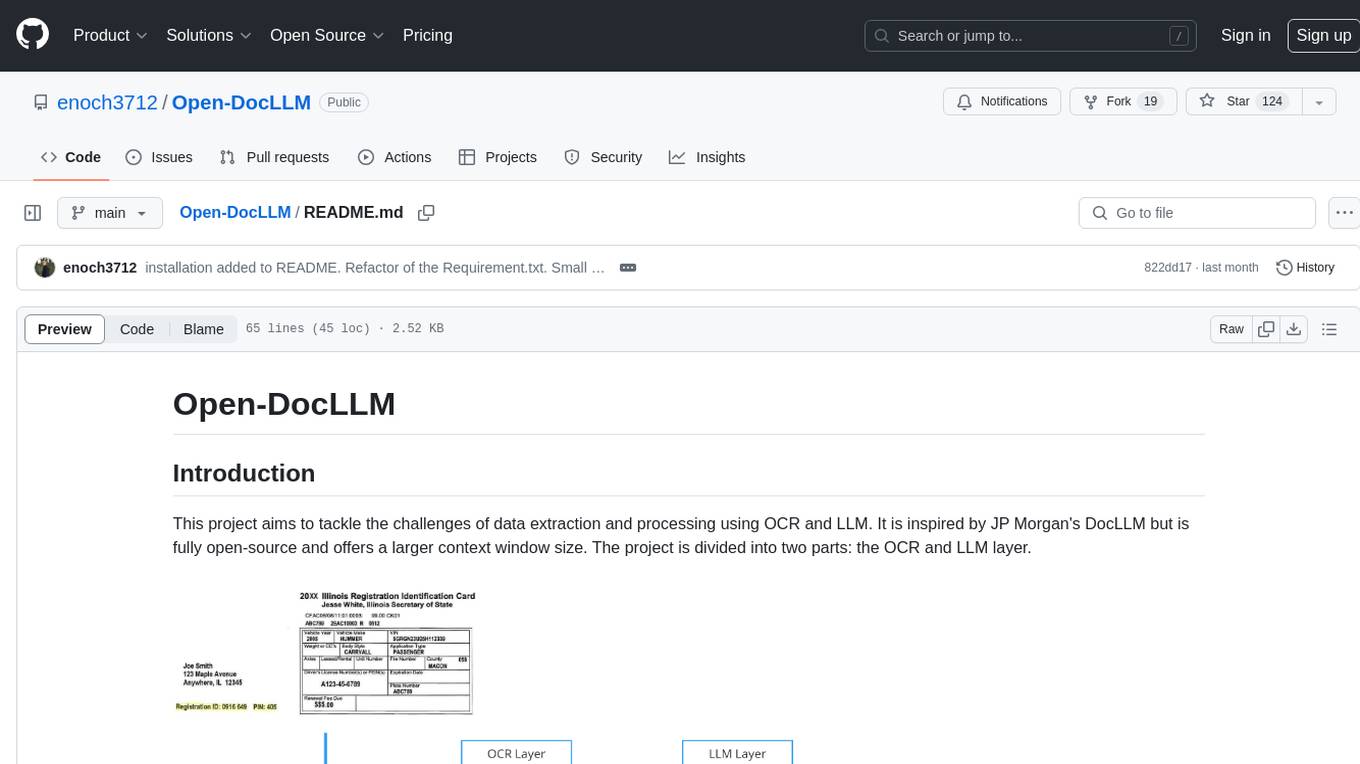
Open-DocLLM
Open-DocLLM is an open-source project that addresses data extraction and processing challenges using OCR and LLM technologies. It consists of two main layers: OCR for reading document content and LLM for extracting specific content in a structured manner. The project offers a larger context window size compared to JP Morgan's DocLLM and integrates tools like Tesseract OCR and Mistral for efficient data analysis. Users can run the models on-premises using LLM studio or Ollama, and the project includes a FastAPI app for testing purposes.
For similar jobs
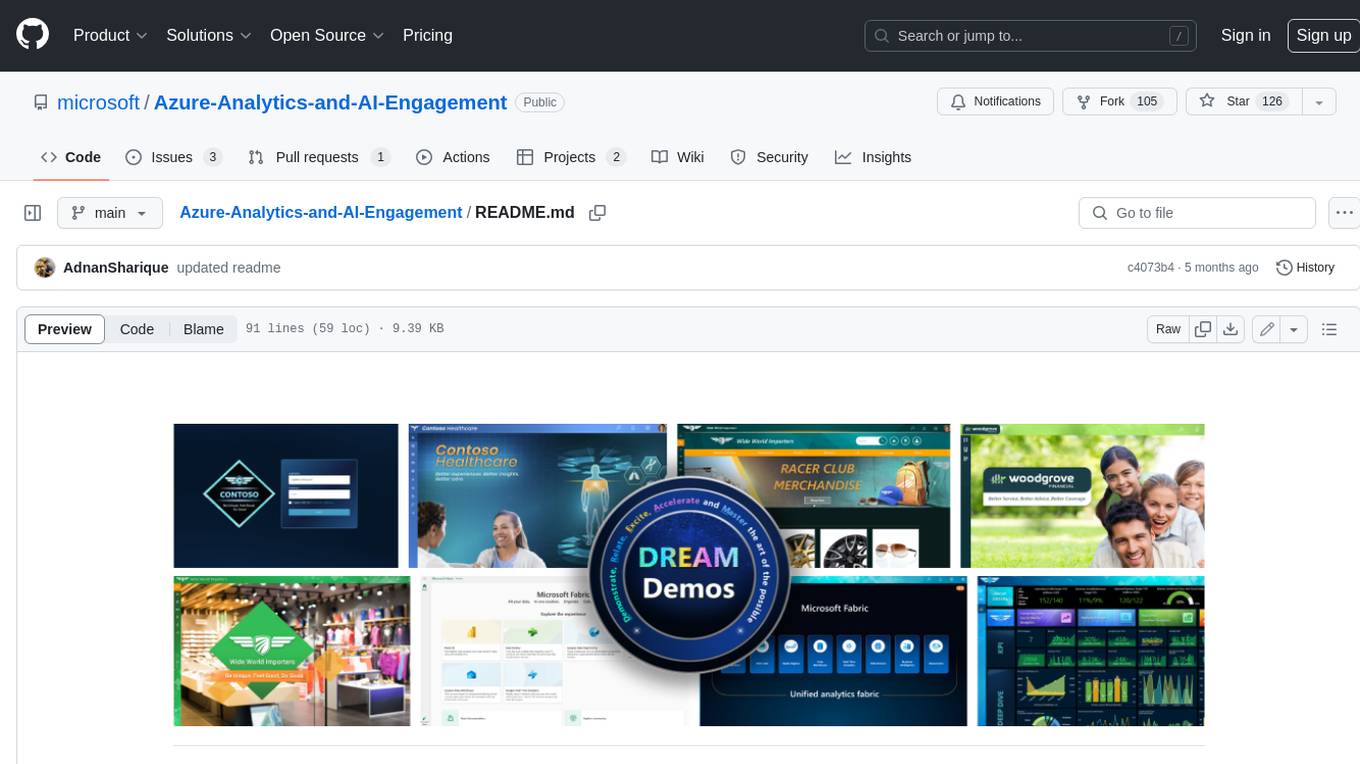
Azure-Analytics-and-AI-Engagement
The Azure-Analytics-and-AI-Engagement repository provides packaged Industry Scenario DREAM Demos with ARM templates (Containing a demo web application, Power BI reports, Synapse resources, AML Notebooks etc.) that can be deployed in a customer’s subscription using the CAPE tool within a matter of few hours. Partners can also deploy DREAM Demos in their own subscriptions using DPoC.
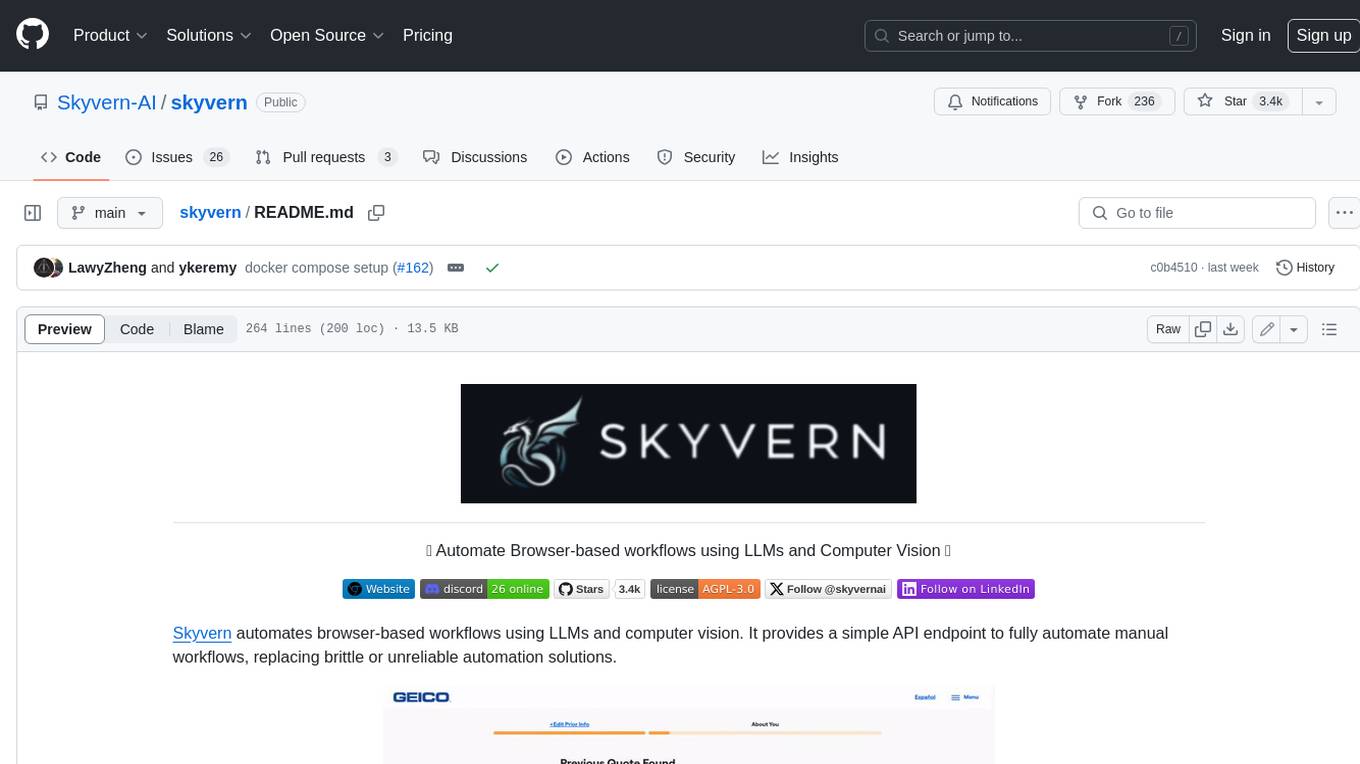
skyvern
Skyvern automates browser-based workflows using LLMs and computer vision. It provides a simple API endpoint to fully automate manual workflows, replacing brittle or unreliable automation solutions. Traditional approaches to browser automations required writing custom scripts for websites, often relying on DOM parsing and XPath-based interactions which would break whenever the website layouts changed. Instead of only relying on code-defined XPath interactions, Skyvern adds computer vision and LLMs to the mix to parse items in the viewport in real-time, create a plan for interaction and interact with them. This approach gives us a few advantages: 1. Skyvern can operate on websites it’s never seen before, as it’s able to map visual elements to actions necessary to complete a workflow, without any customized code 2. Skyvern is resistant to website layout changes, as there are no pre-determined XPaths or other selectors our system is looking for while trying to navigate 3. Skyvern leverages LLMs to reason through interactions to ensure we can cover complex situations. Examples include: 1. If you wanted to get an auto insurance quote from Geico, the answer to a common question “Were you eligible to drive at 18?” could be inferred from the driver receiving their license at age 16 2. If you were doing competitor analysis, it’s understanding that an Arnold Palmer 22 oz can at 7/11 is almost definitely the same product as a 23 oz can at Gopuff (even though the sizes are slightly different, which could be a rounding error!) Want to see examples of Skyvern in action? Jump to #real-world-examples-of- skyvern
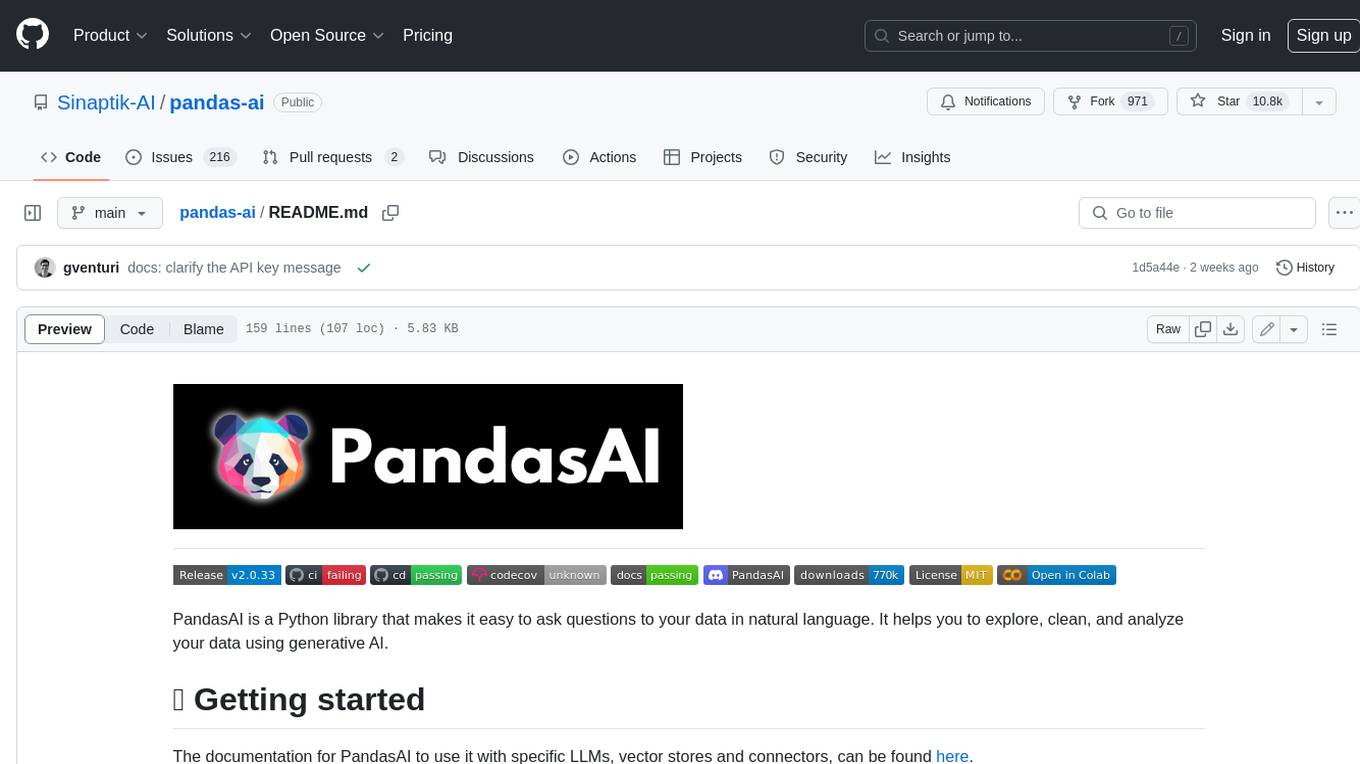
pandas-ai
PandasAI is a Python library that makes it easy to ask questions to your data in natural language. It helps you to explore, clean, and analyze your data using generative AI.
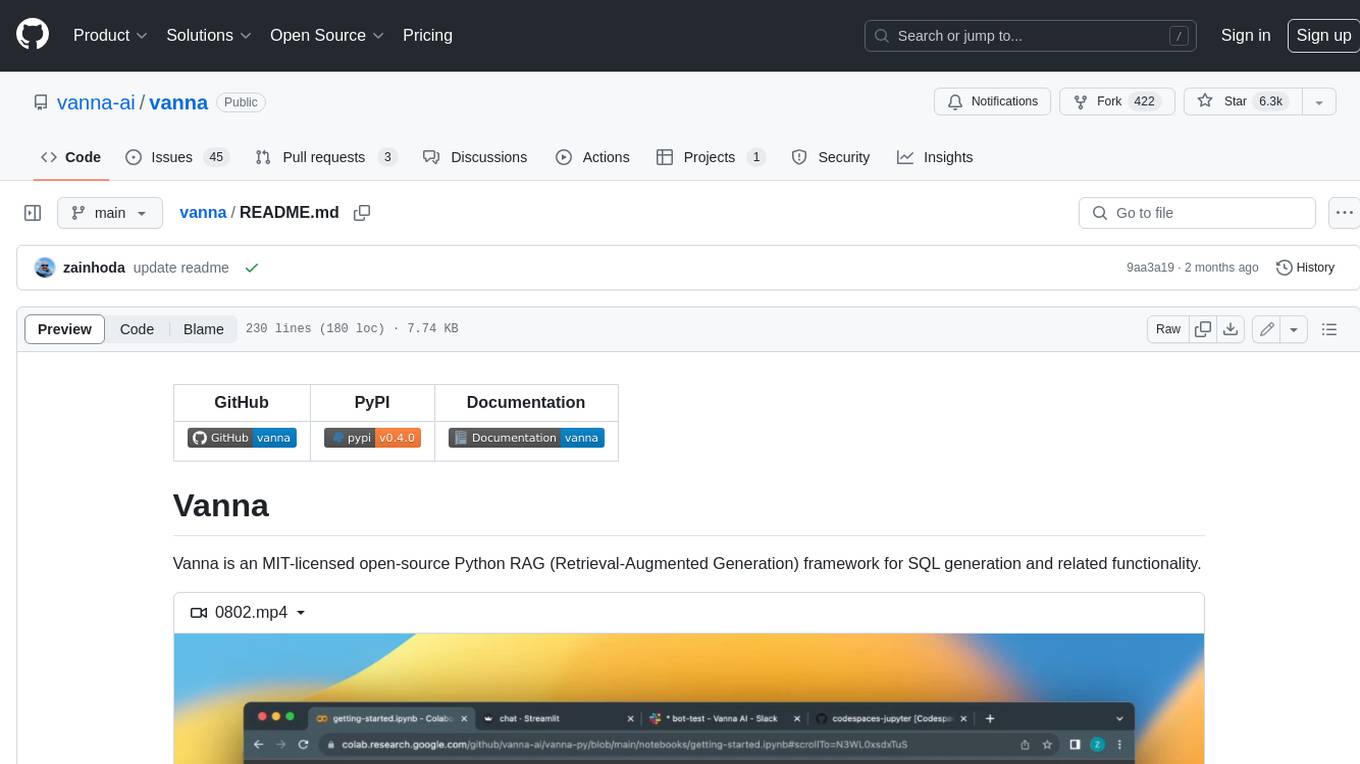
vanna
Vanna is an open-source Python framework for SQL generation and related functionality. It uses Retrieval-Augmented Generation (RAG) to train a model on your data, which can then be used to ask questions and get back SQL queries. Vanna is designed to be portable across different LLMs and vector databases, and it supports any SQL database. It is also secure and private, as your database contents are never sent to the LLM or the vector database.
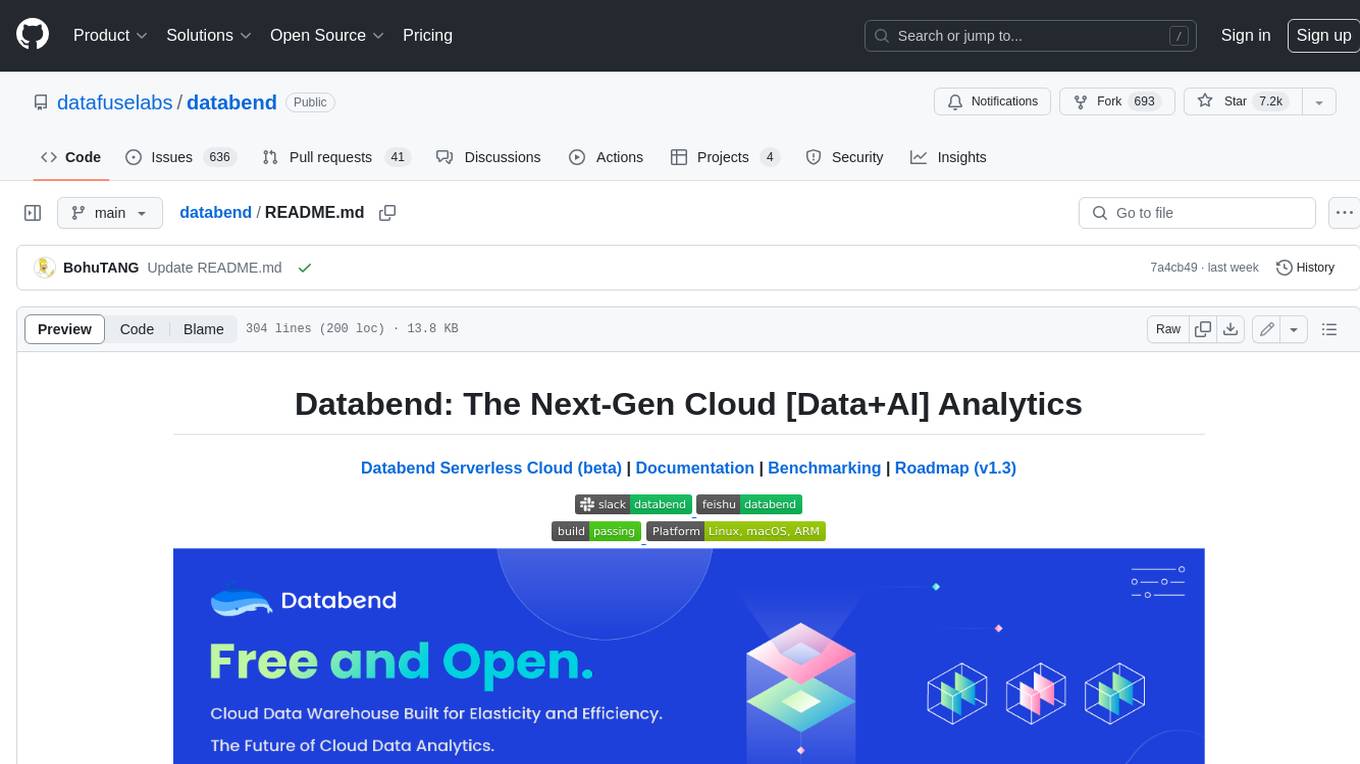
databend
Databend is an open-source cloud data warehouse that serves as a cost-effective alternative to Snowflake. With its focus on fast query execution and data ingestion, it's designed for complex analysis of the world's largest datasets.
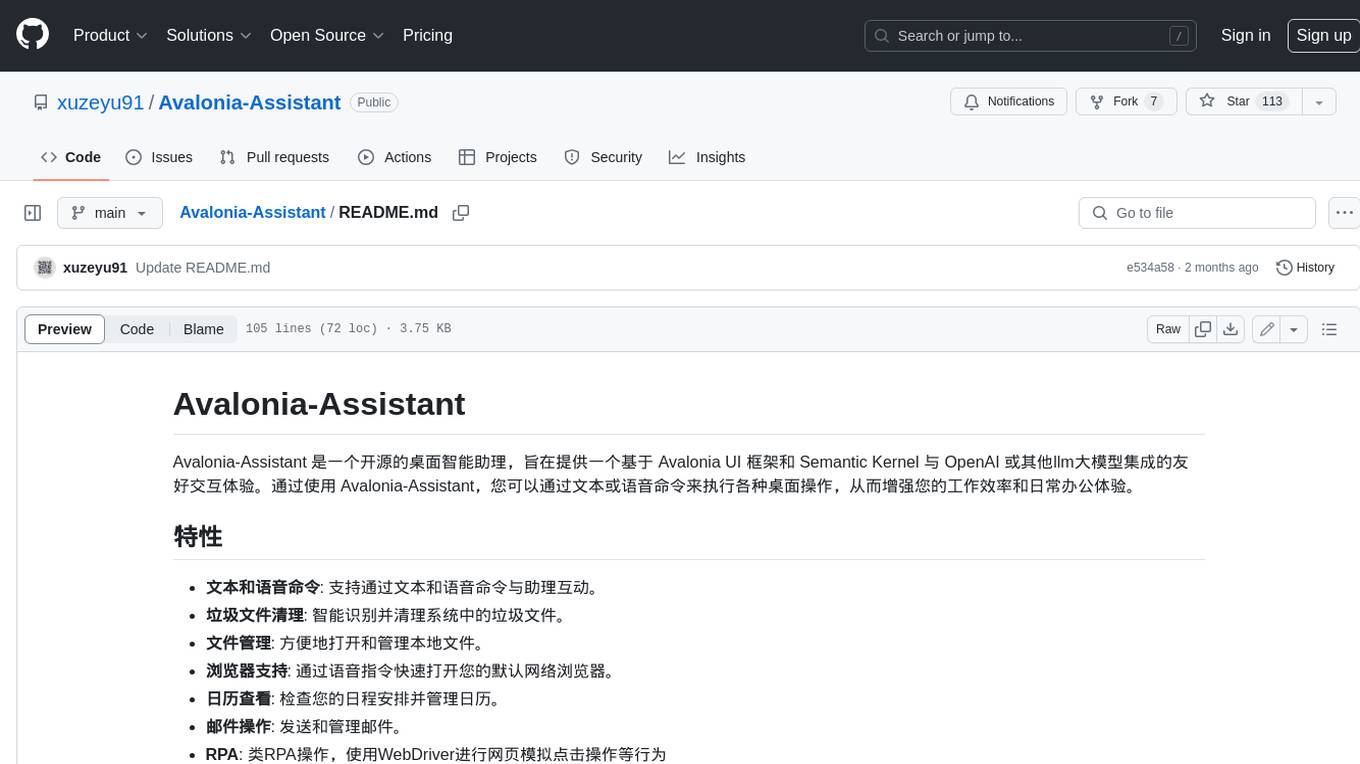
Avalonia-Assistant
Avalonia-Assistant is an open-source desktop intelligent assistant that aims to provide a user-friendly interactive experience based on the Avalonia UI framework and the integration of Semantic Kernel with OpenAI or other large LLM models. By utilizing Avalonia-Assistant, you can perform various desktop operations through text or voice commands, enhancing your productivity and daily office experience.
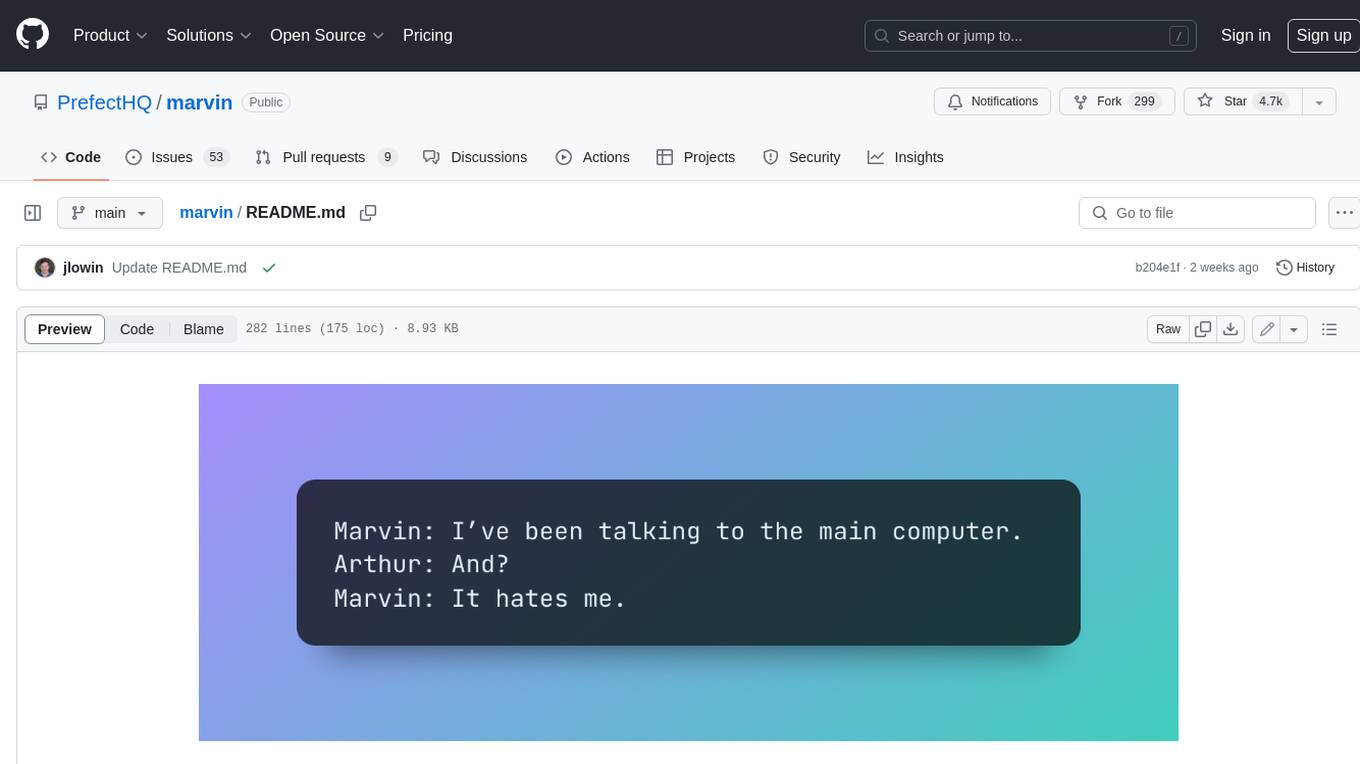
marvin
Marvin is a lightweight AI toolkit for building natural language interfaces that are reliable, scalable, and easy to trust. Each of Marvin's tools is simple and self-documenting, using AI to solve common but complex challenges like entity extraction, classification, and generating synthetic data. Each tool is independent and incrementally adoptable, so you can use them on their own or in combination with any other library. Marvin is also multi-modal, supporting both image and audio generation as well using images as inputs for extraction and classification. Marvin is for developers who care more about _using_ AI than _building_ AI, and we are focused on creating an exceptional developer experience. Marvin users should feel empowered to bring tightly-scoped "AI magic" into any traditional software project with just a few extra lines of code. Marvin aims to merge the best practices for building dependable, observable software with the best practices for building with generative AI into a single, easy-to-use library. It's a serious tool, but we hope you have fun with it. Marvin is open-source, free to use, and made with 💙 by the team at Prefect.
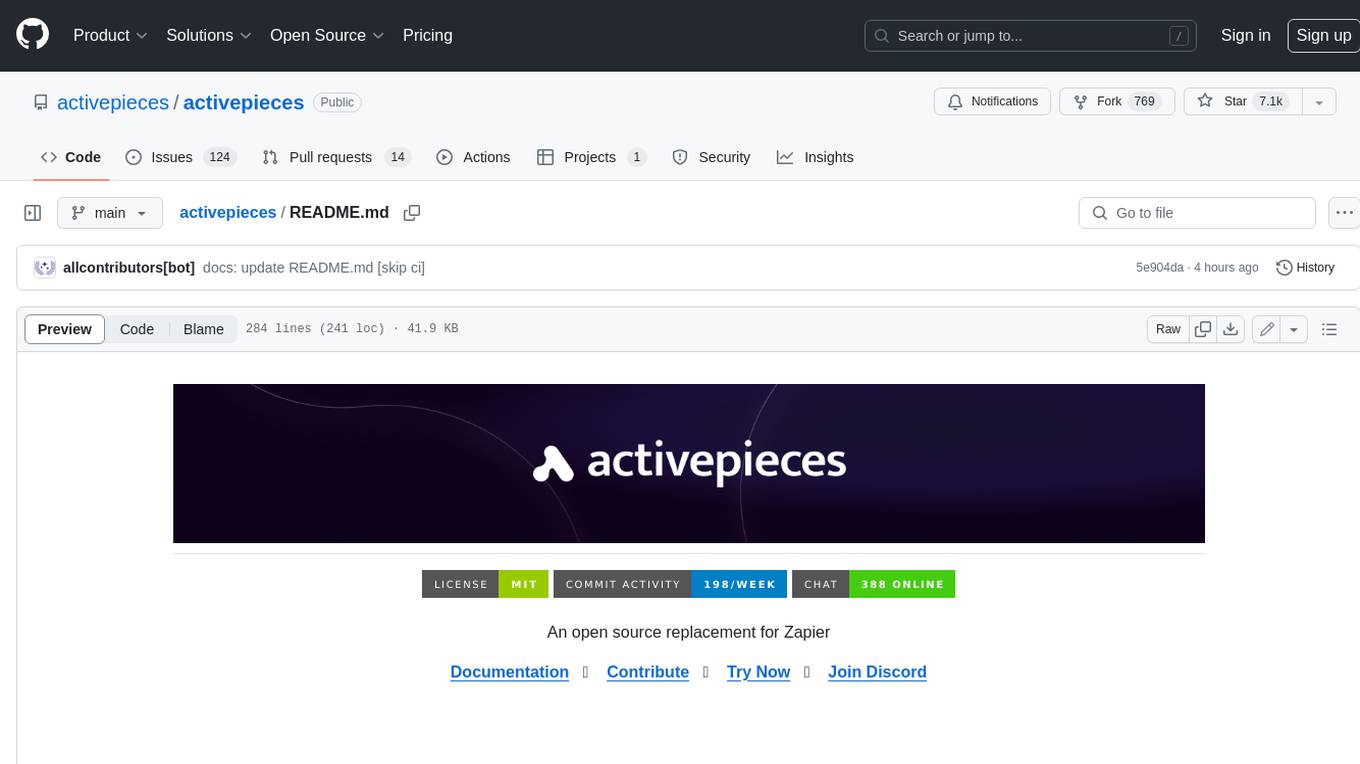
activepieces
Activepieces is an open source replacement for Zapier, designed to be extensible through a type-safe pieces framework written in Typescript. It features a user-friendly Workflow Builder with support for Branches, Loops, and Drag and Drop. Activepieces integrates with Google Sheets, OpenAI, Discord, and RSS, along with 80+ other integrations. The list of supported integrations continues to grow rapidly, thanks to valuable contributions from the community. Activepieces is an open ecosystem; all piece source code is available in the repository, and they are versioned and published directly to npmjs.com upon contributions. If you cannot find a specific piece on the pieces roadmap, please submit a request by visiting the following link: Request Piece Alternatively, if you are a developer, you can quickly build your own piece using our TypeScript framework. For guidance, please refer to the following guide: Contributor's Guide